NCHRP 08 71 Methodology for Estimating Life Expectancies
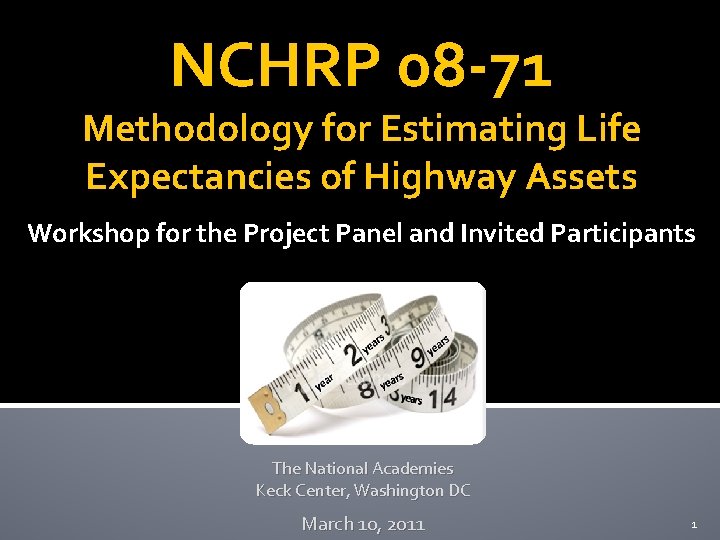
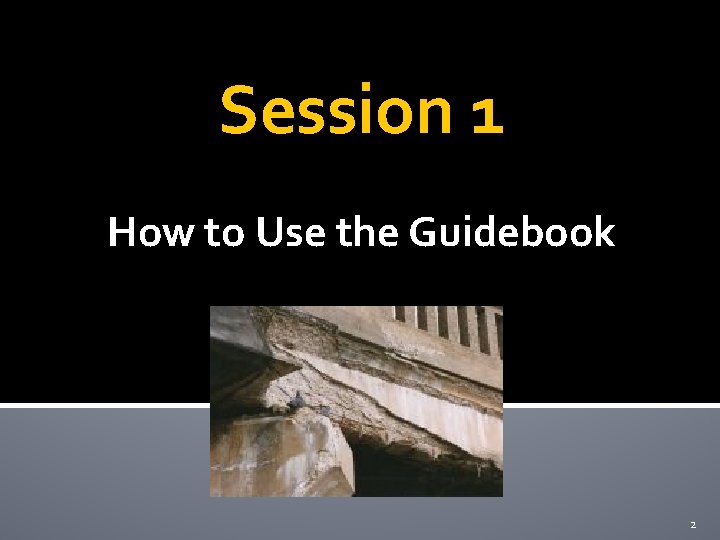
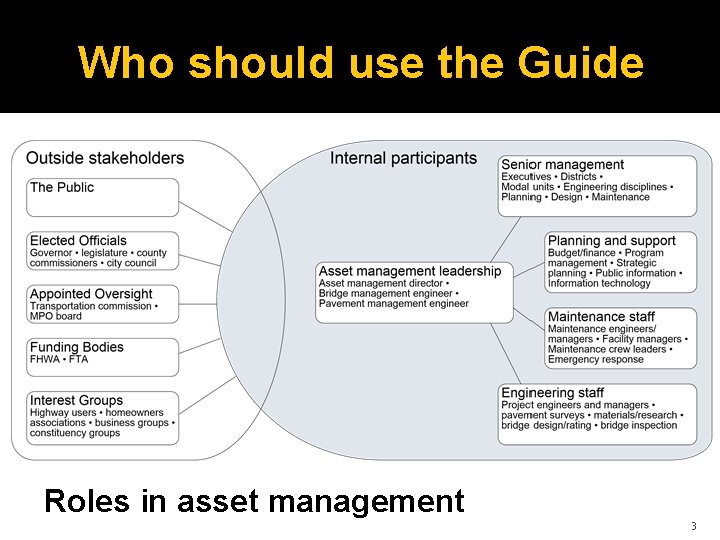
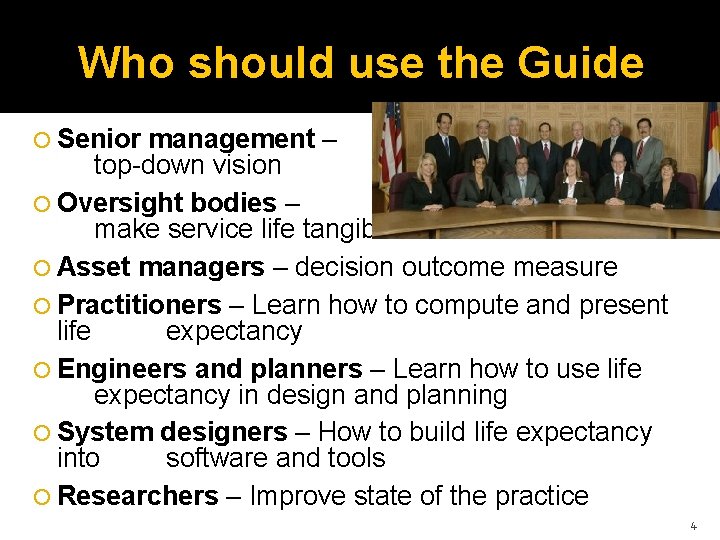
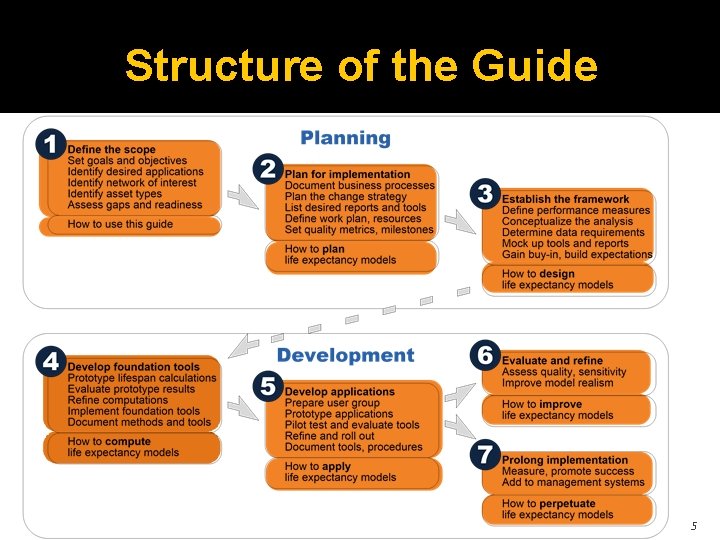
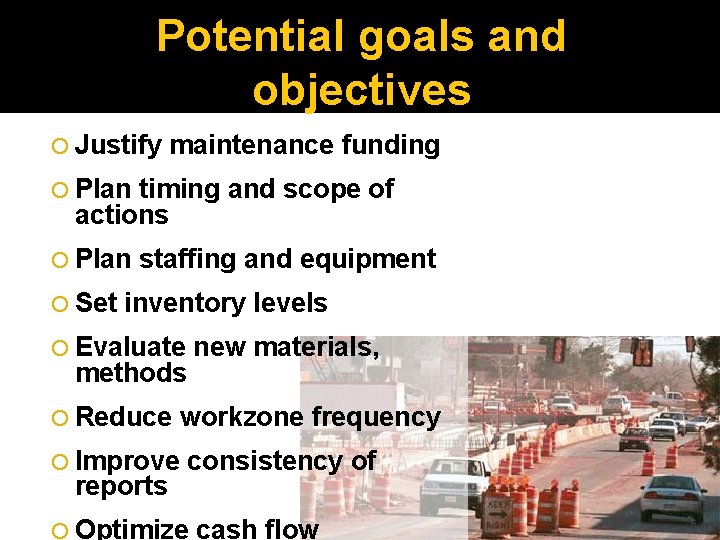
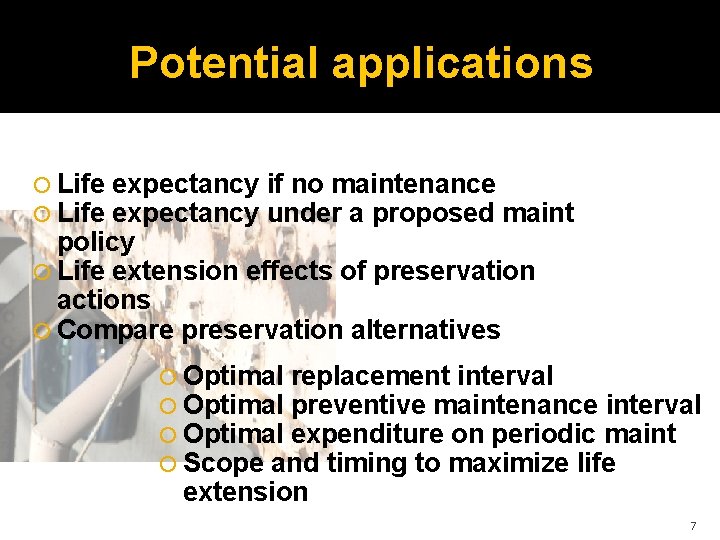
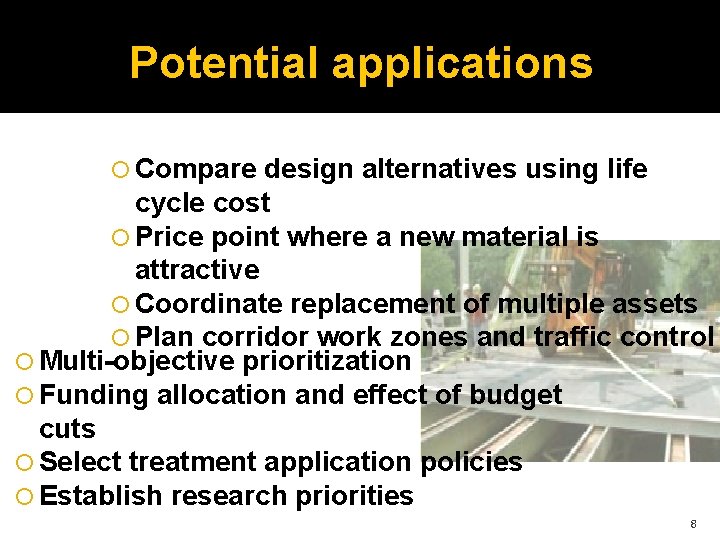
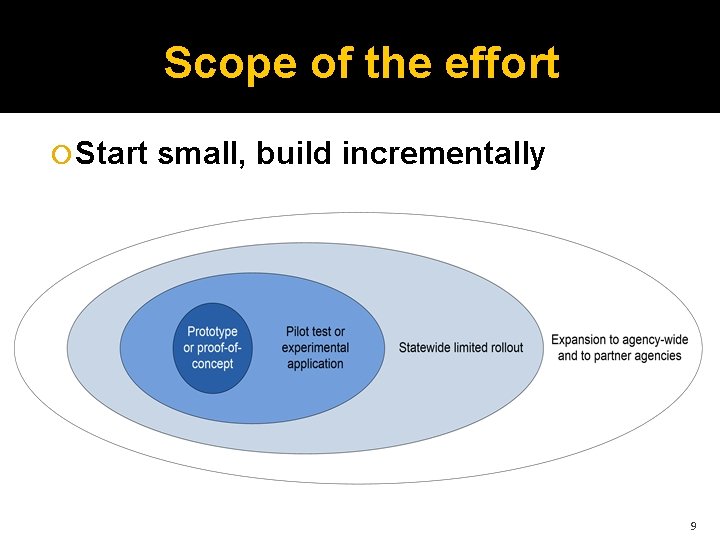
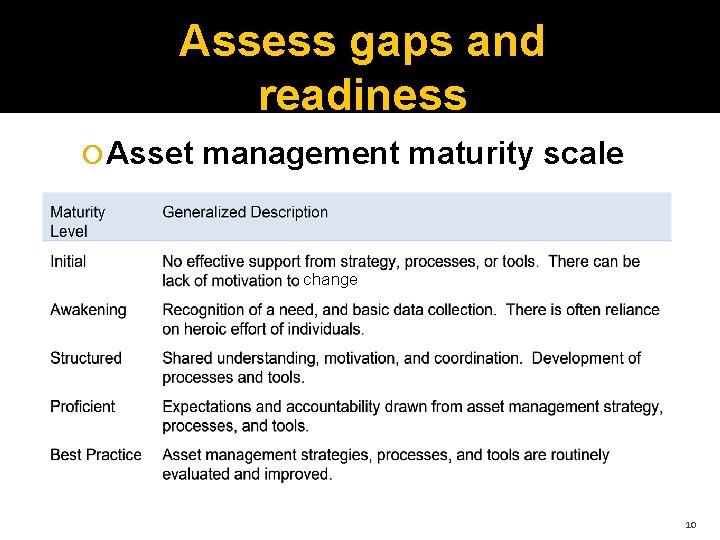
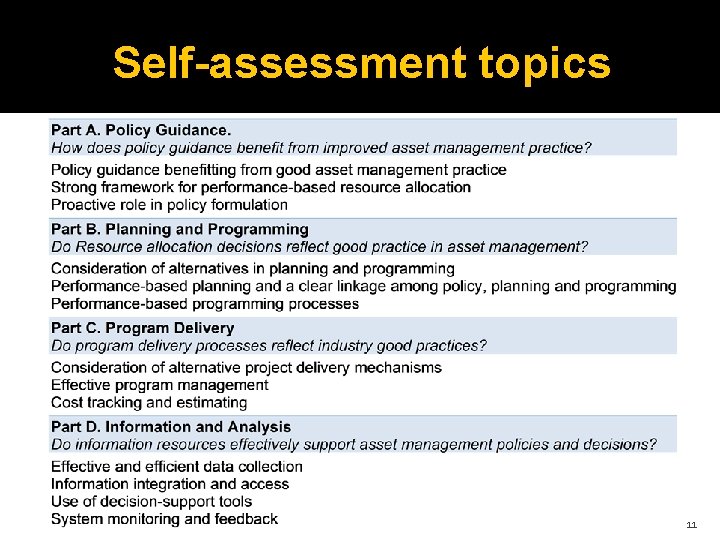
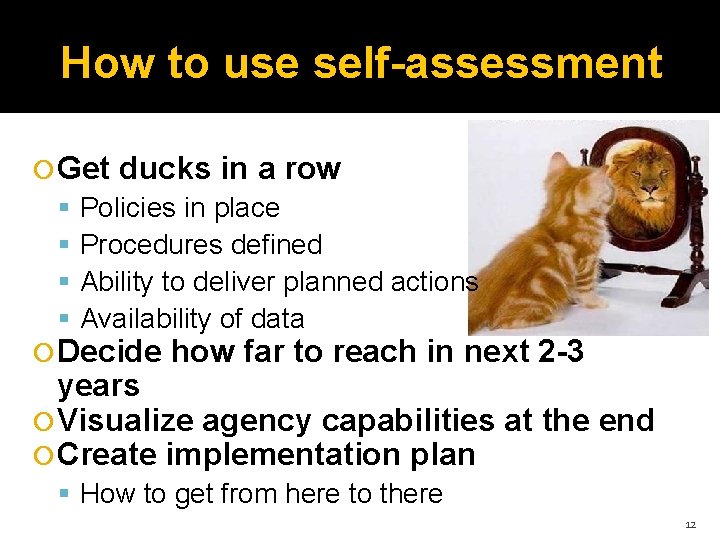
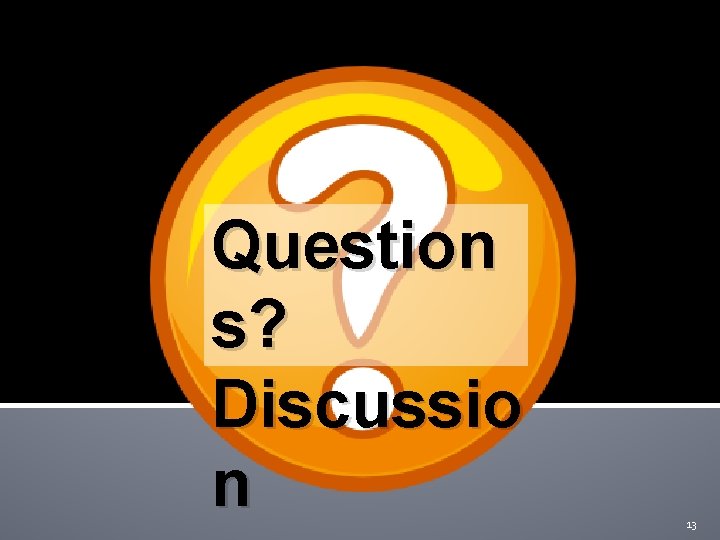
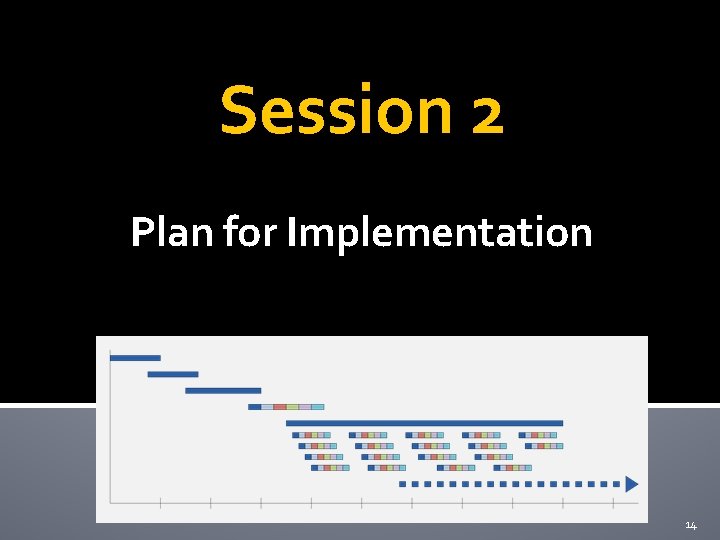
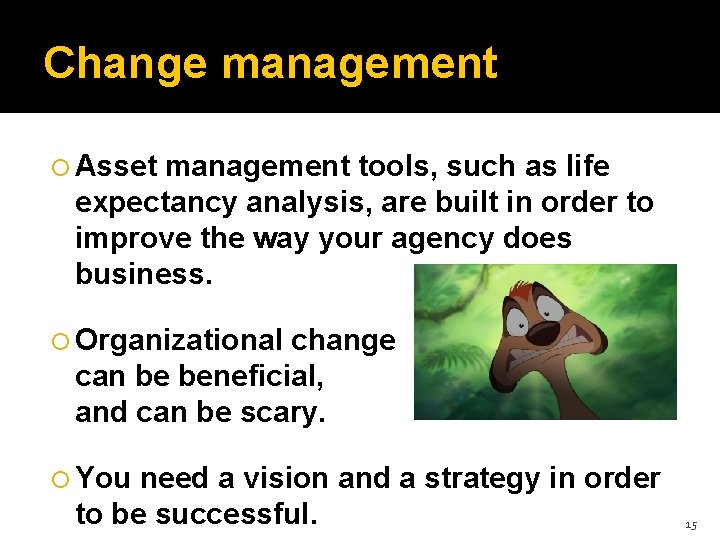
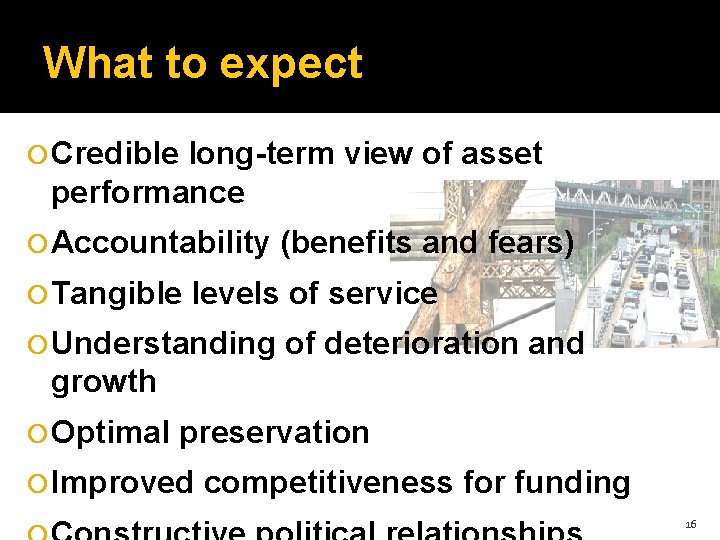
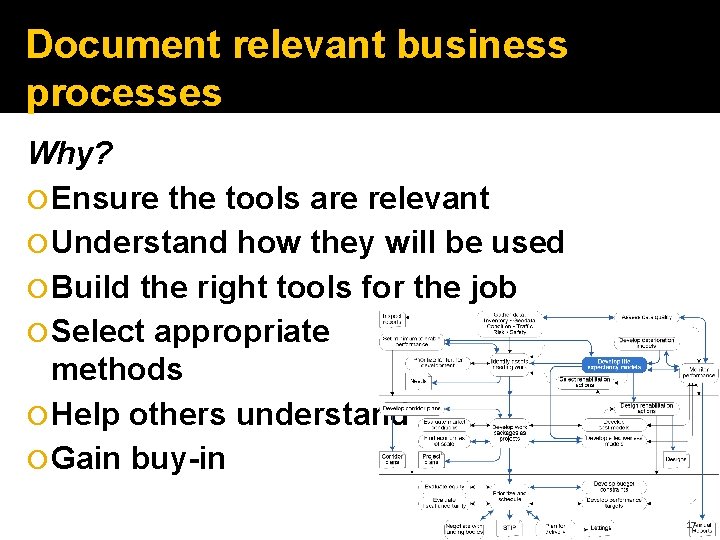
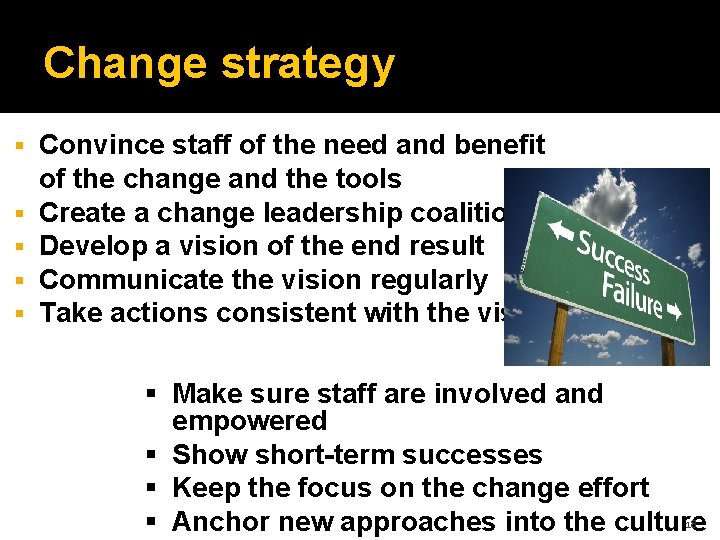
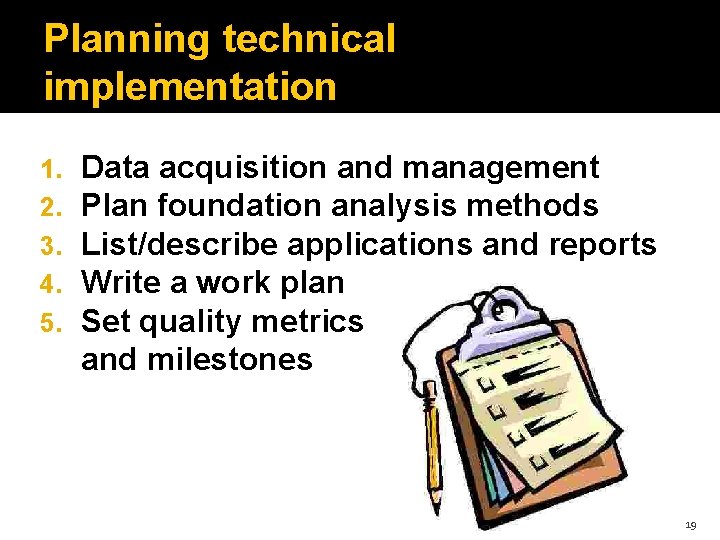
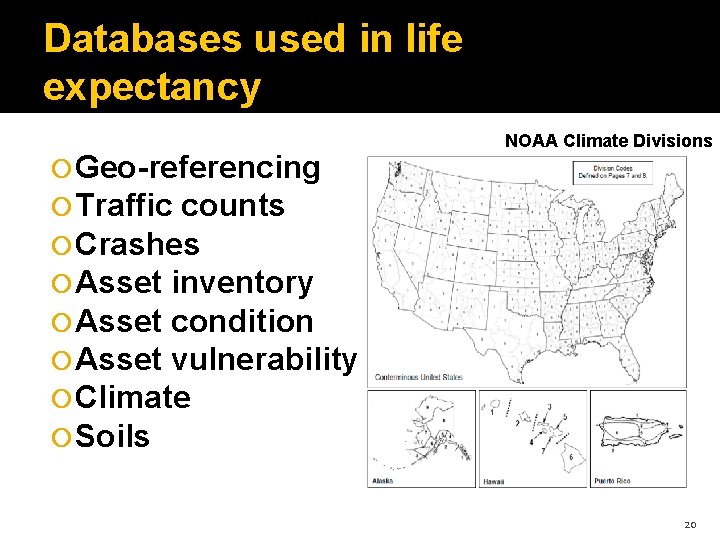
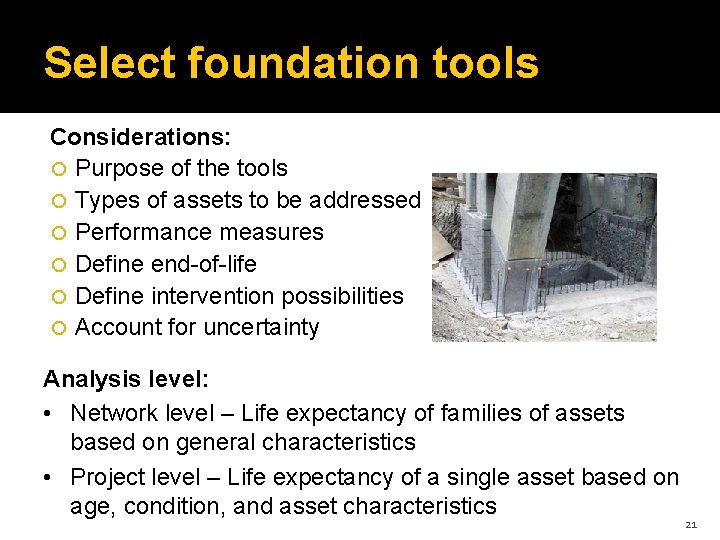
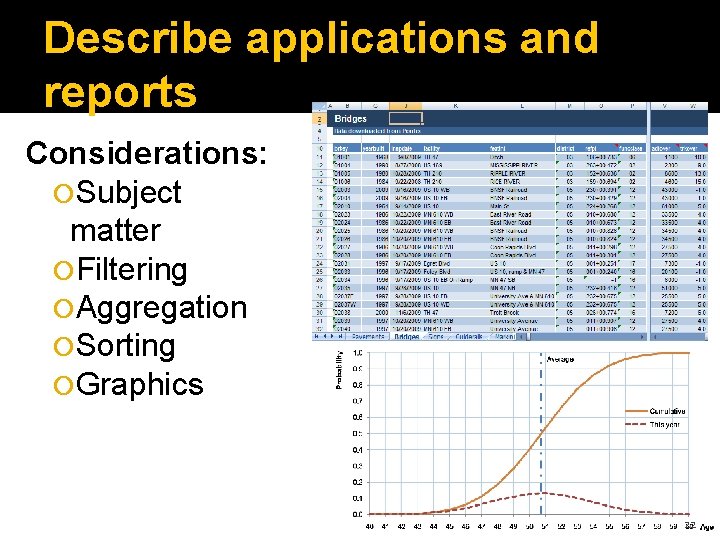
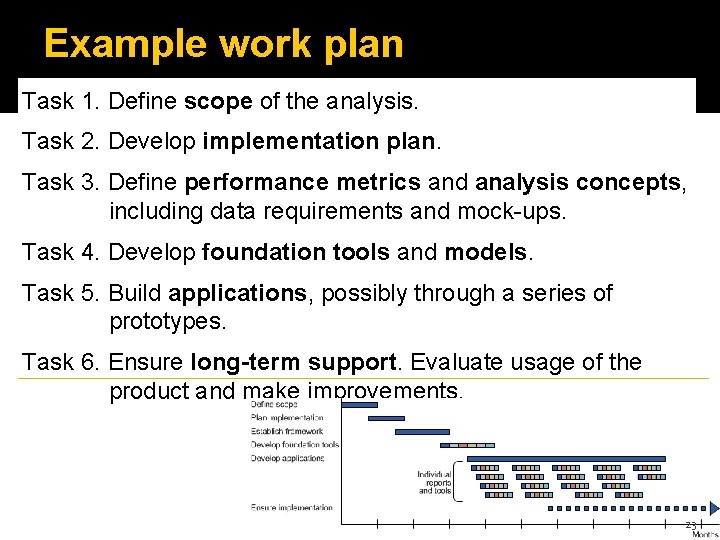
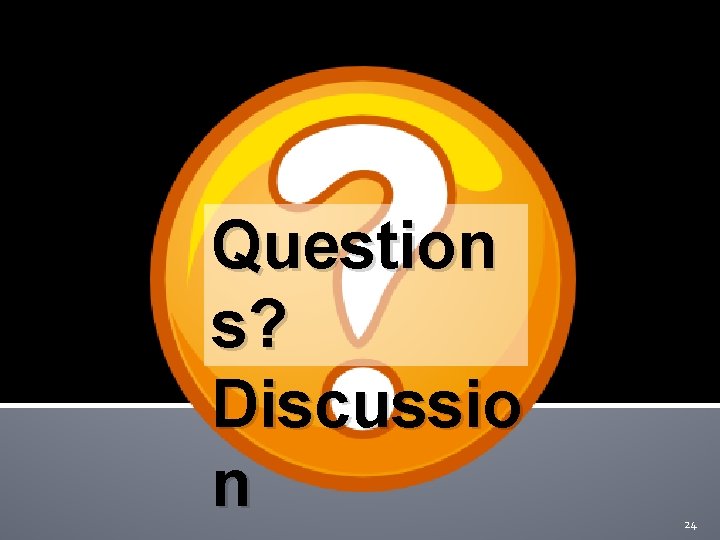
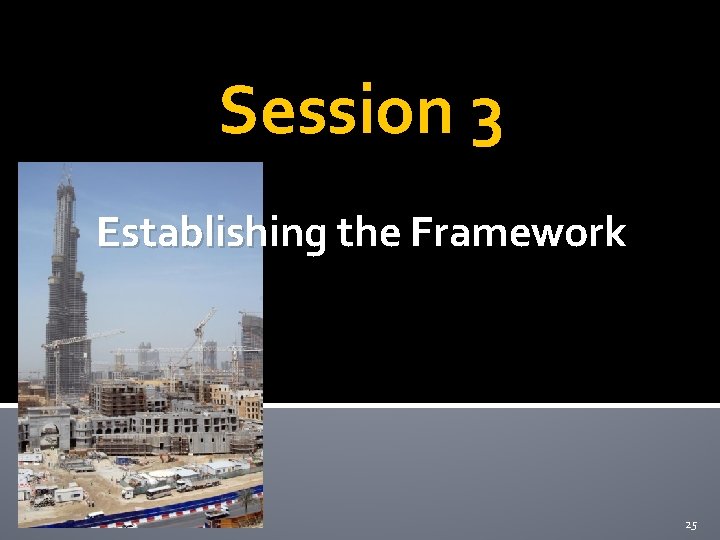
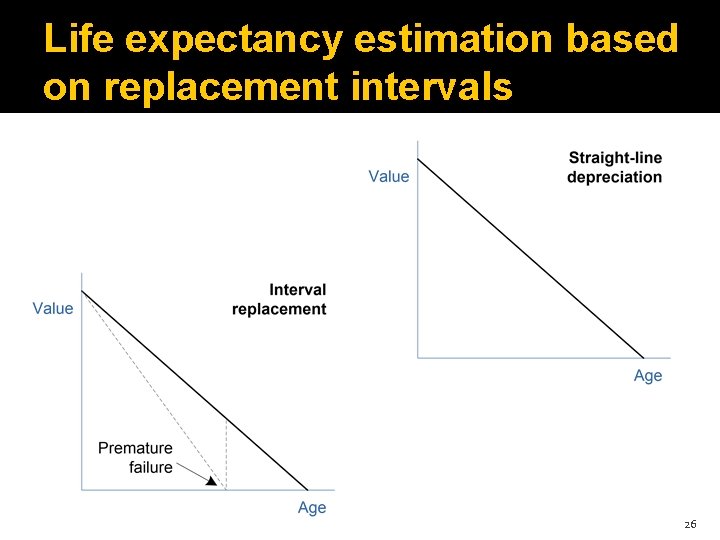
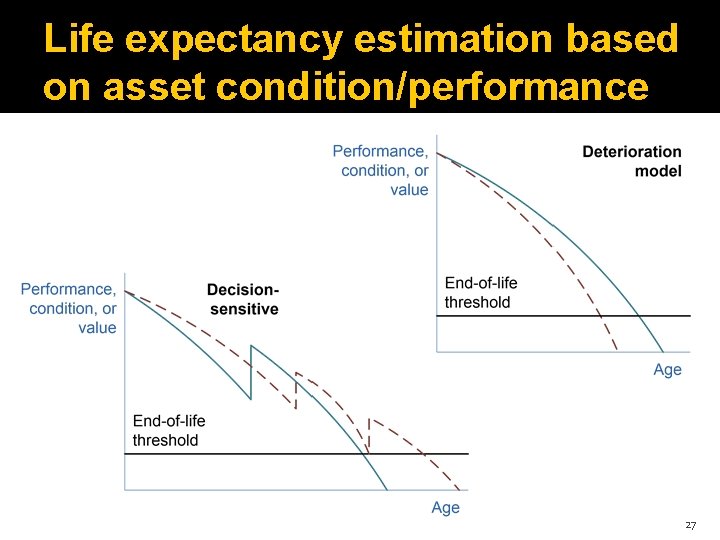
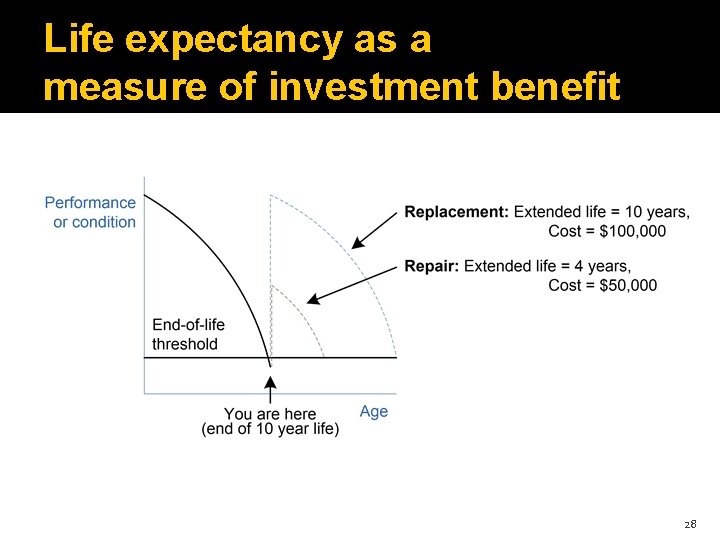
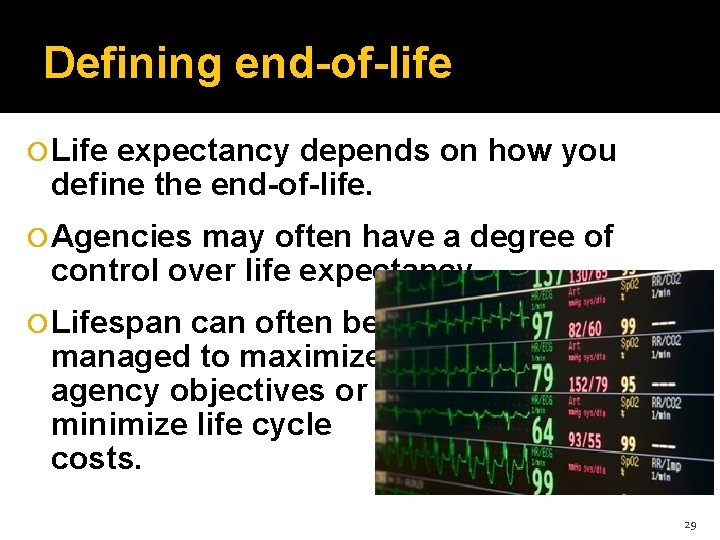
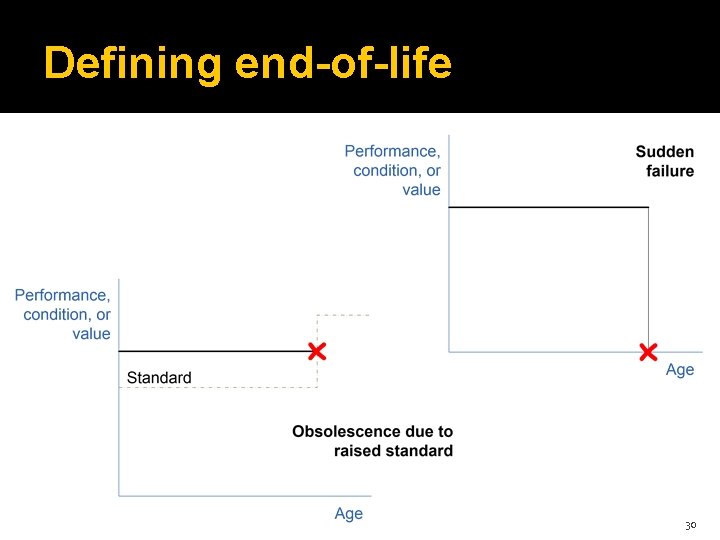
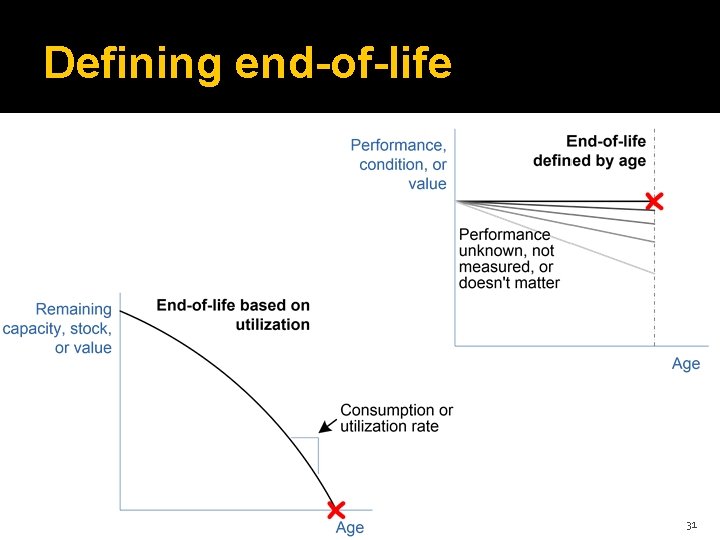
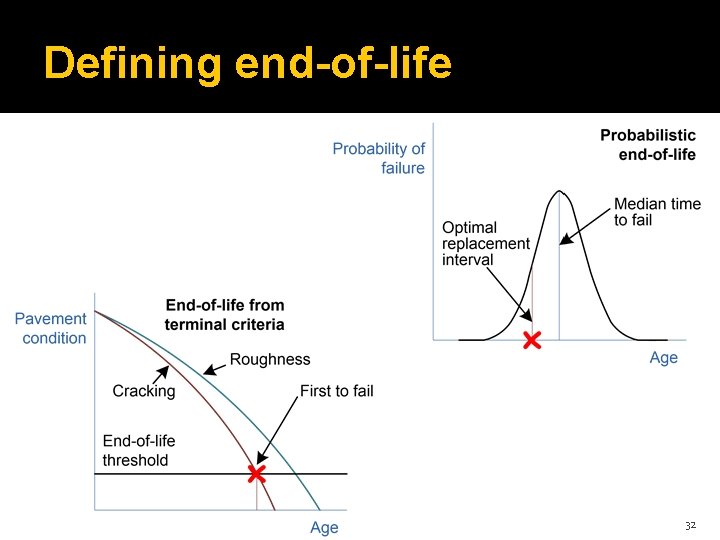
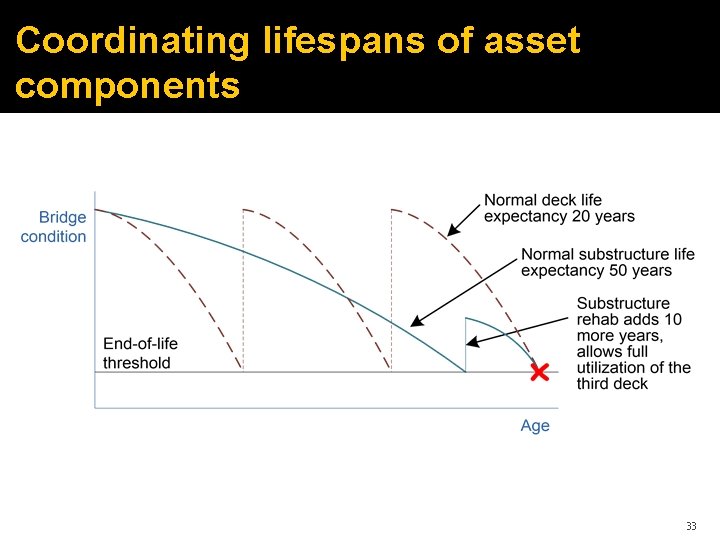
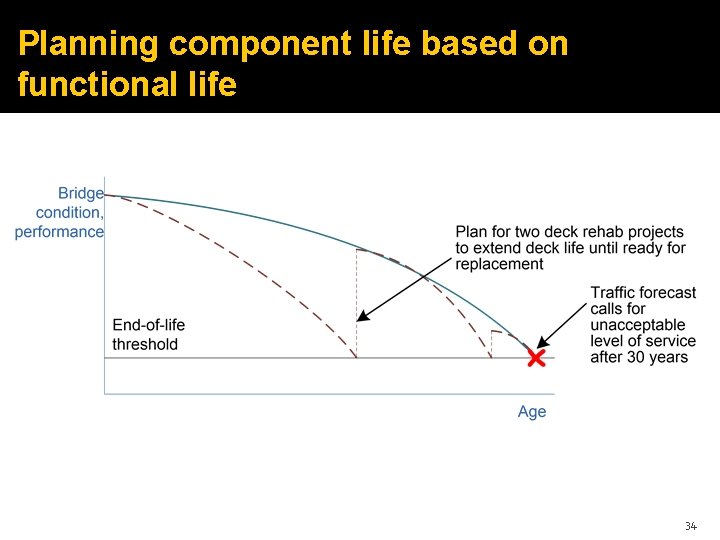
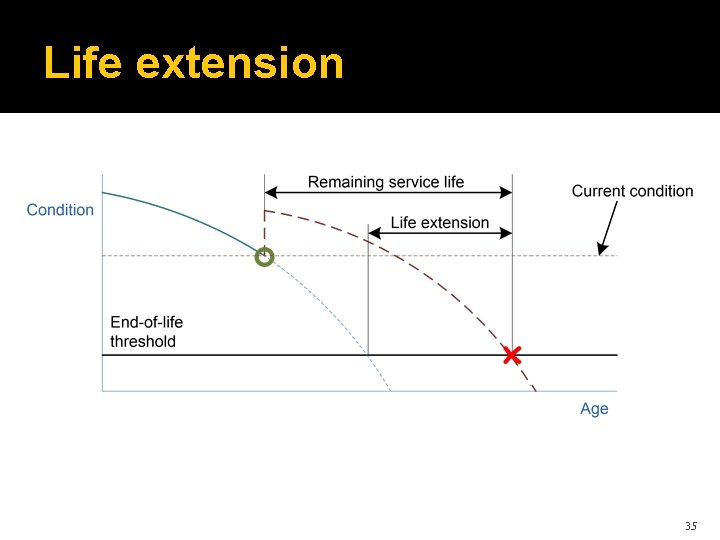
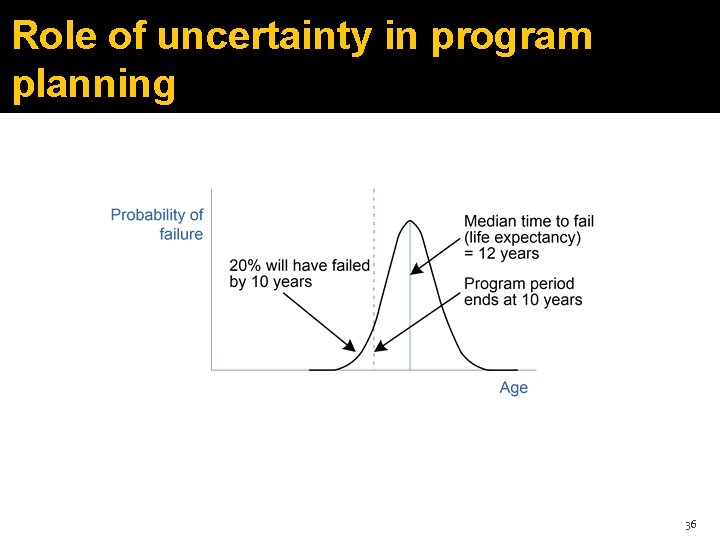
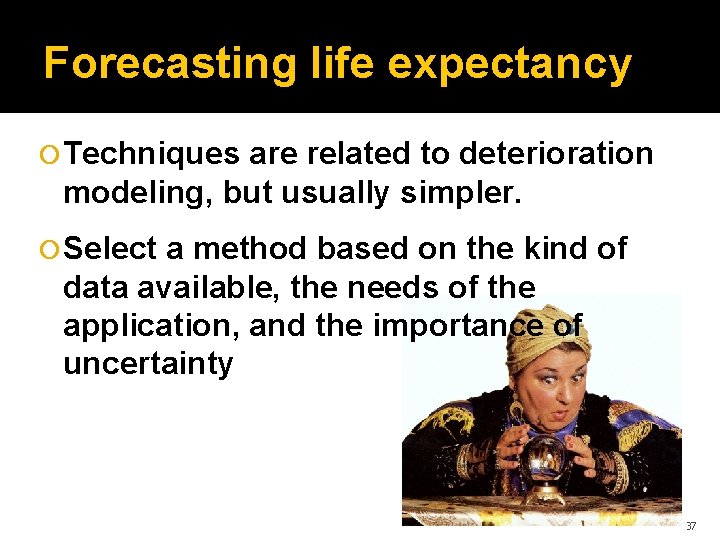
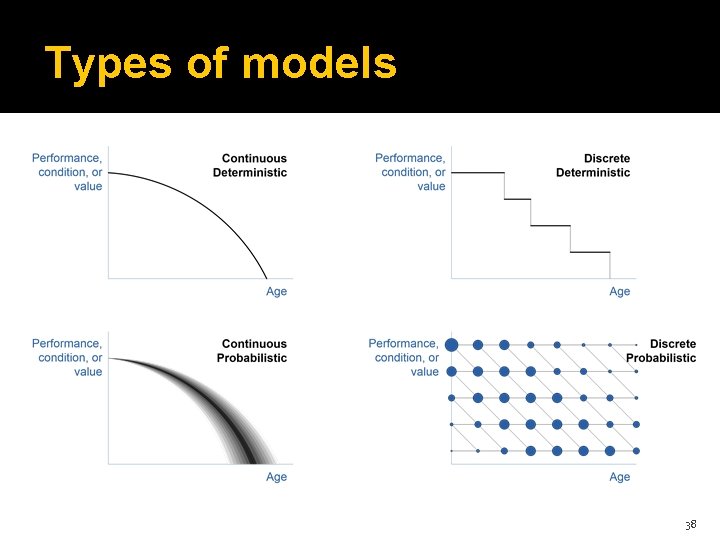
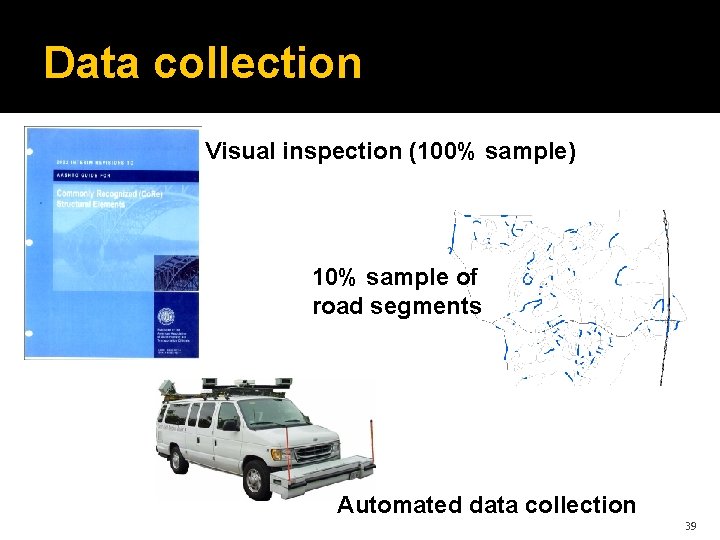
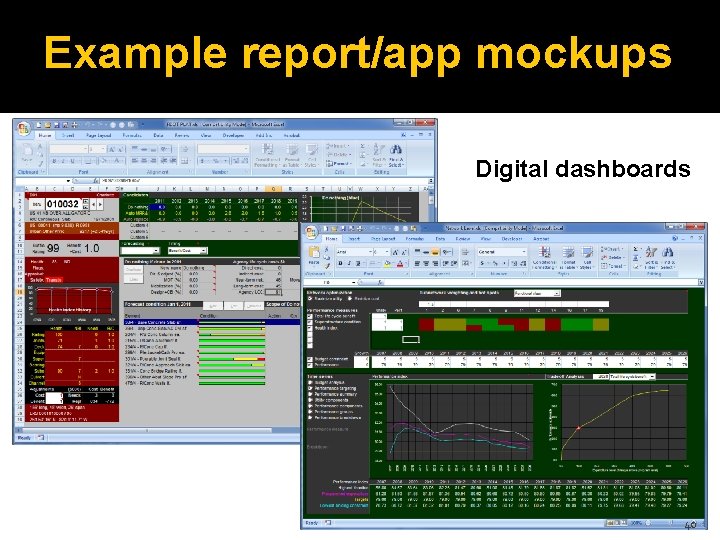
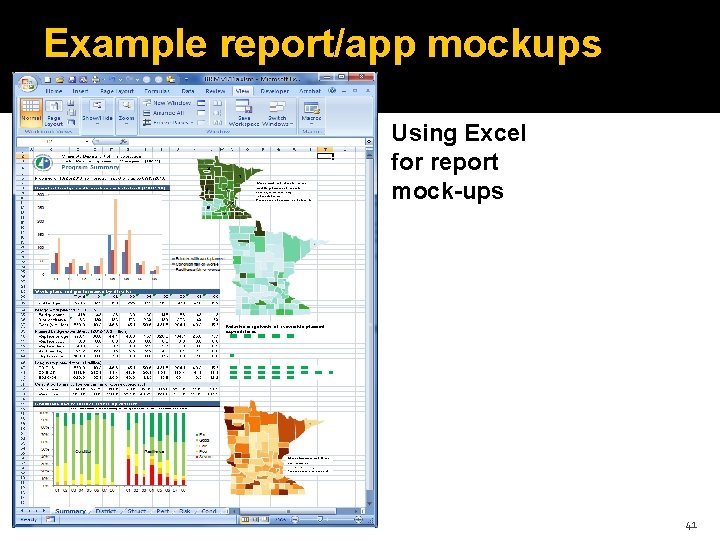
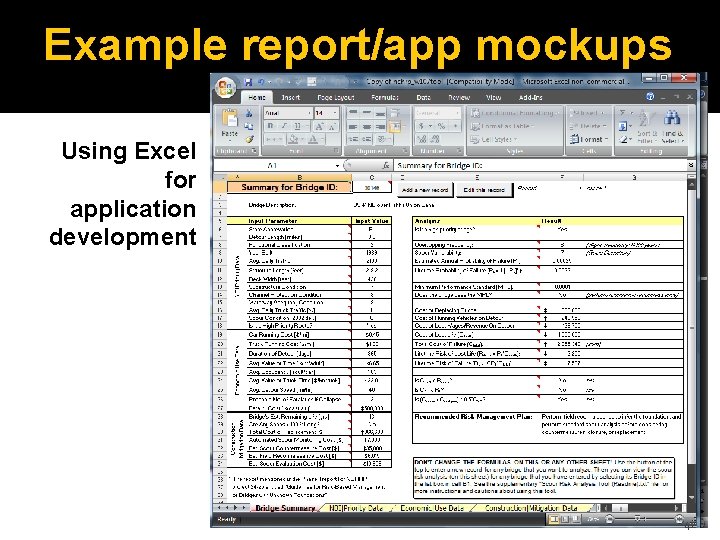
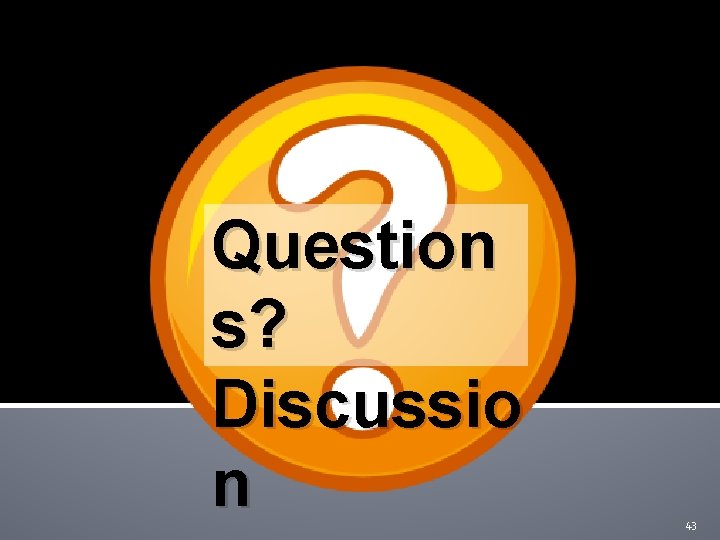
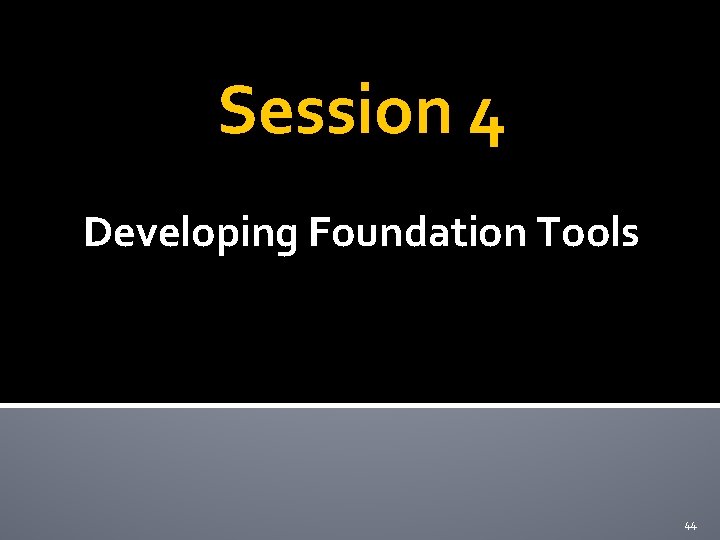
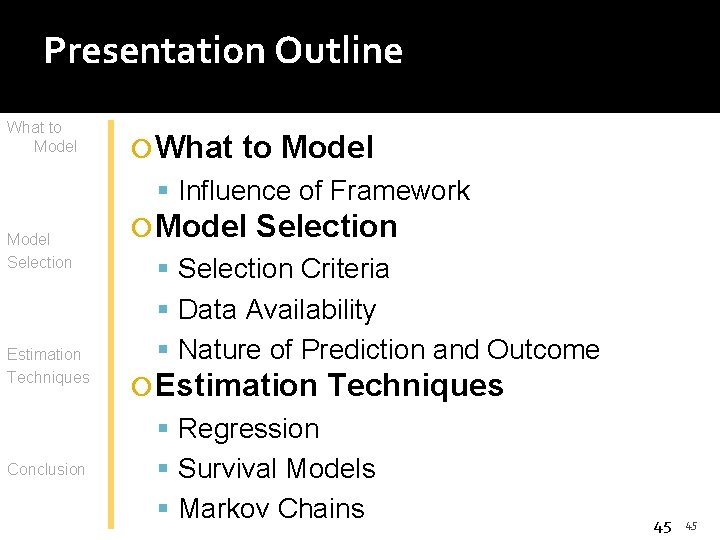
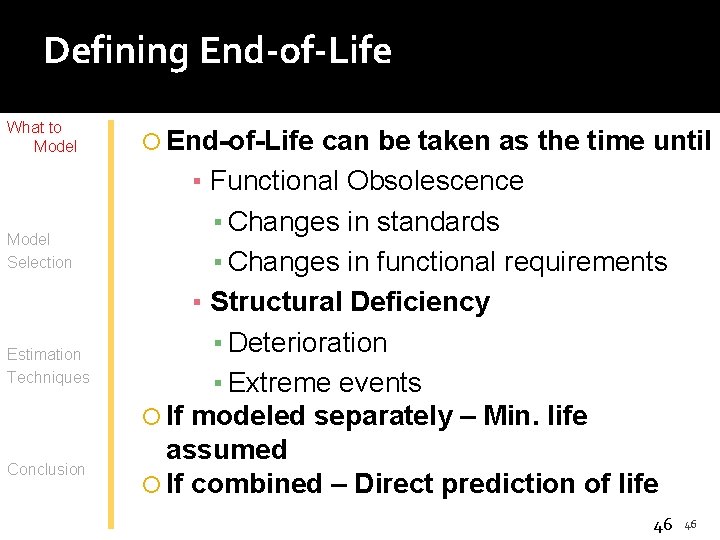
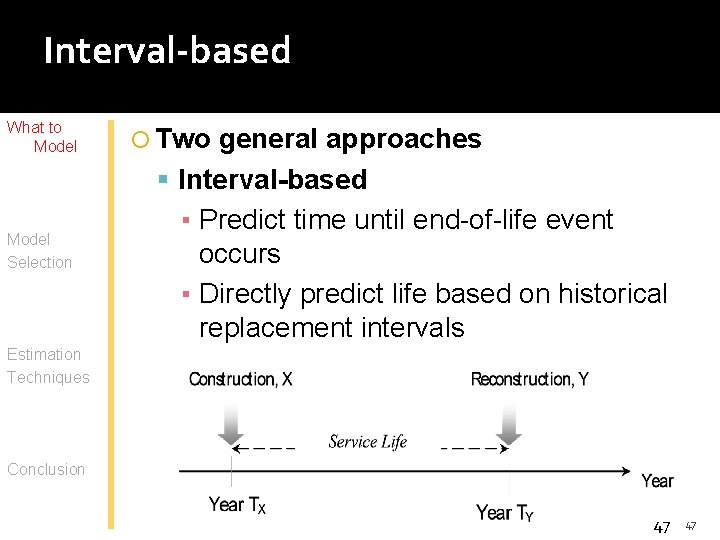
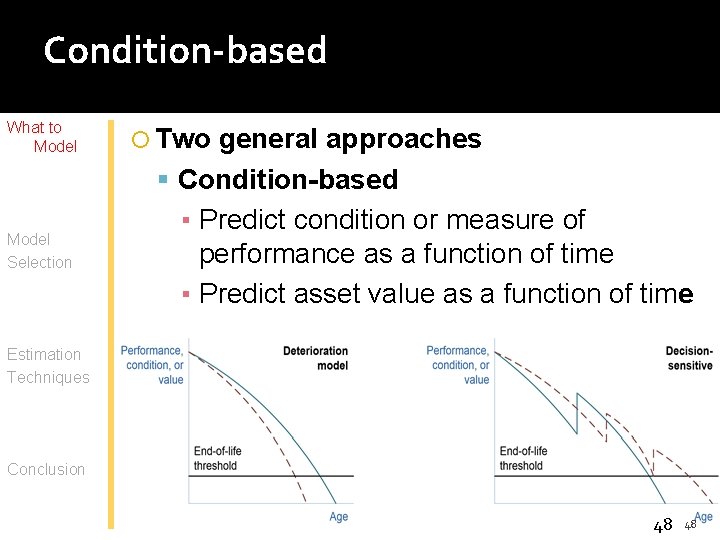
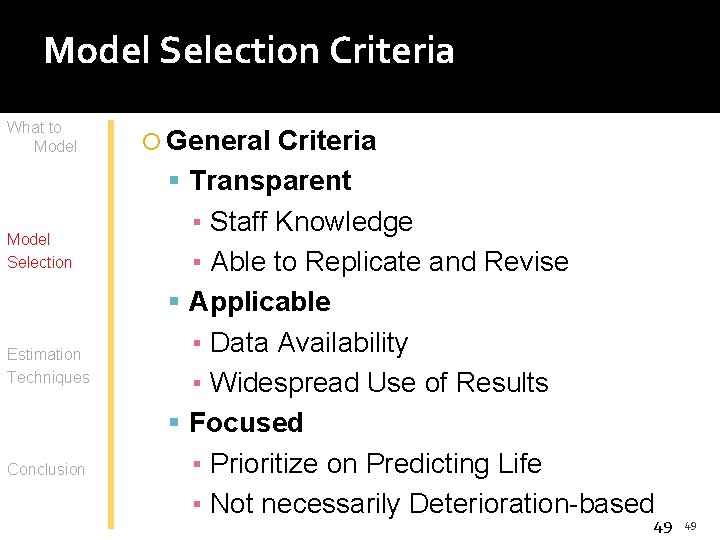
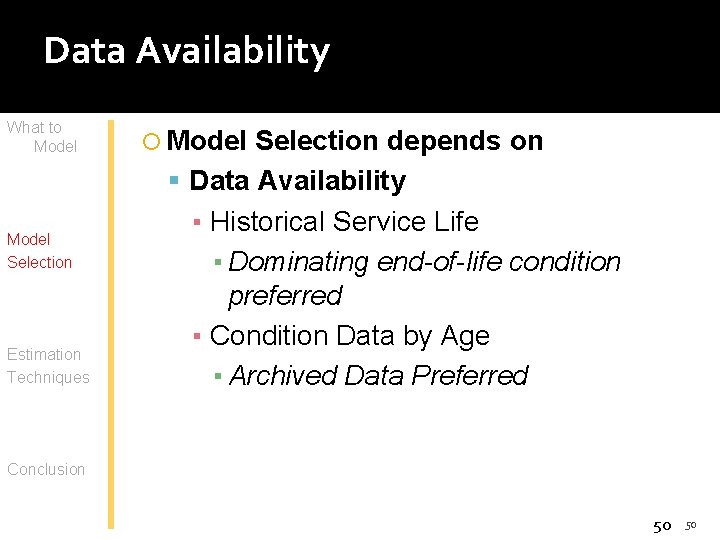
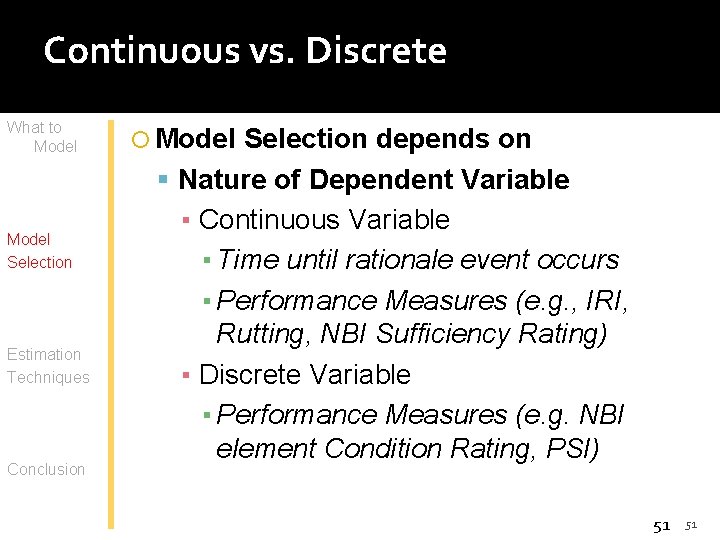
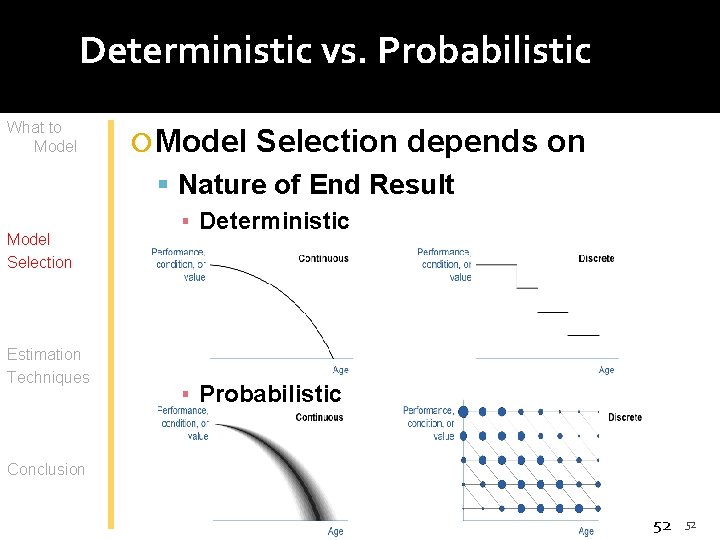
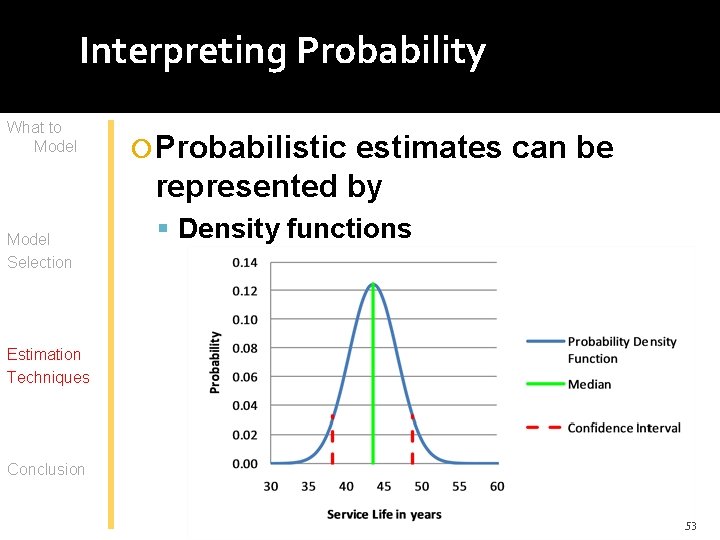
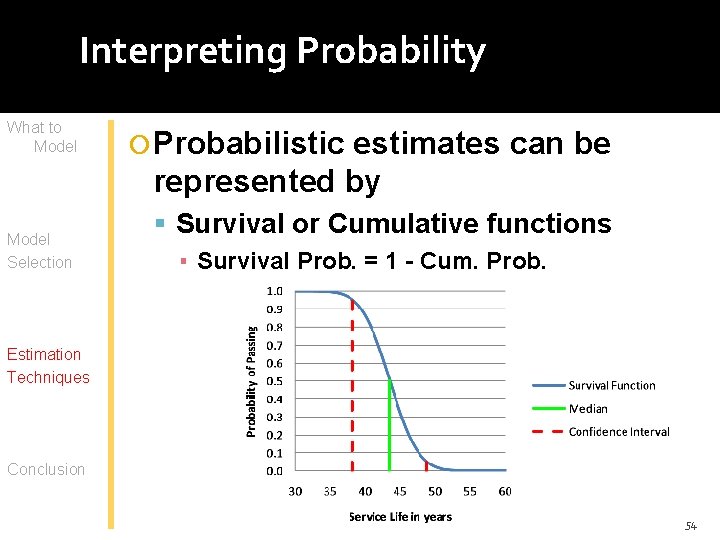
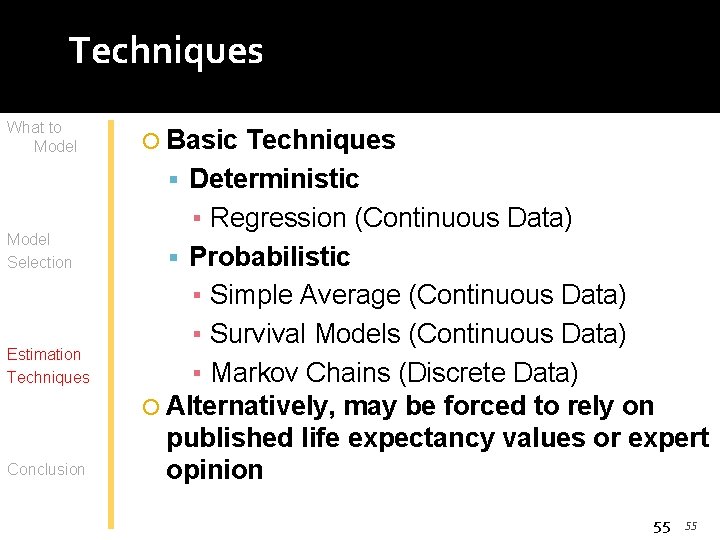
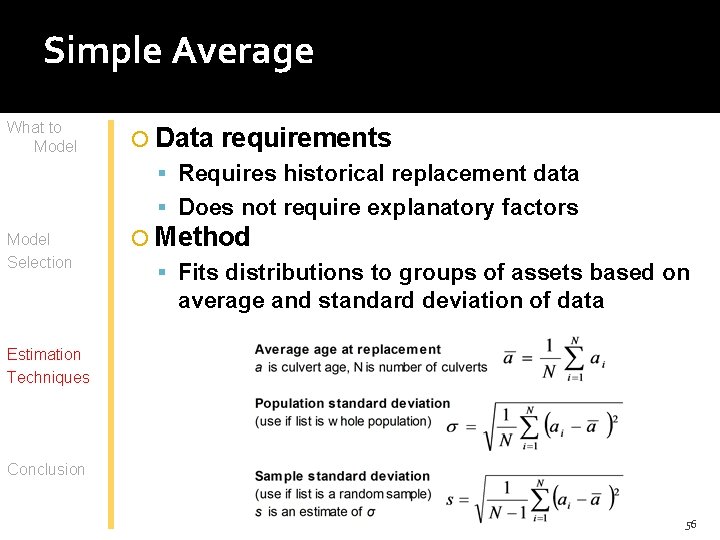
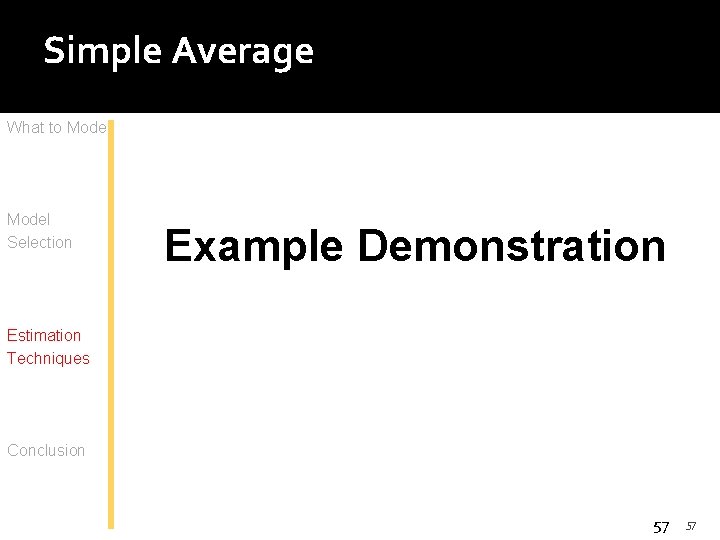
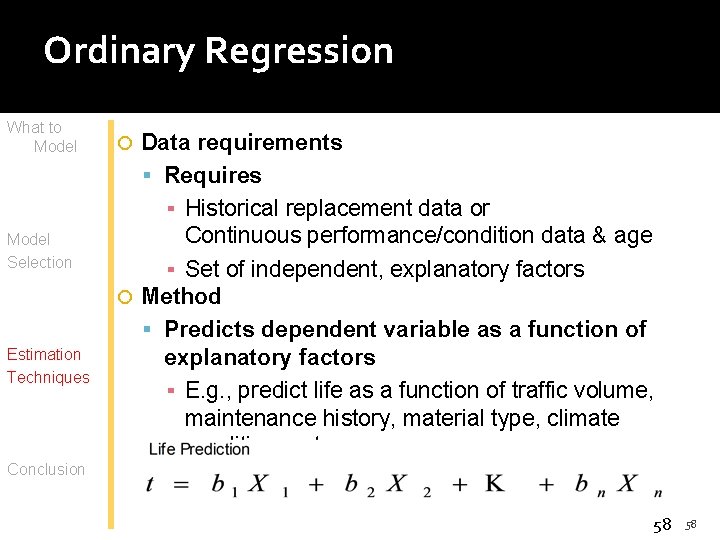
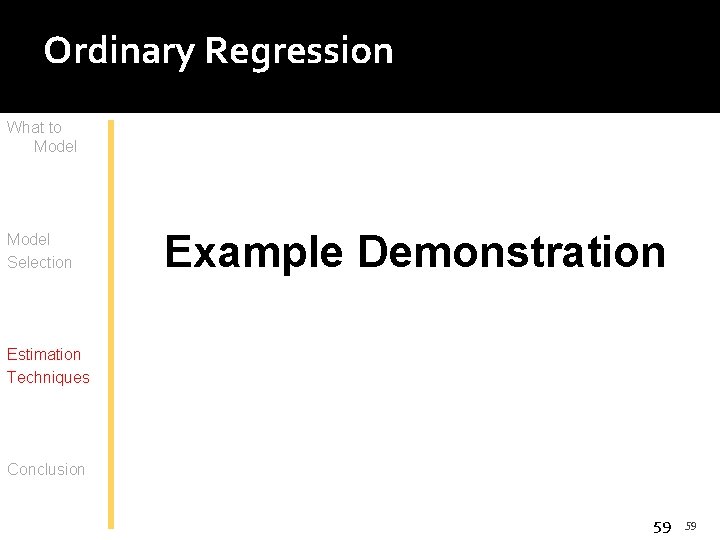
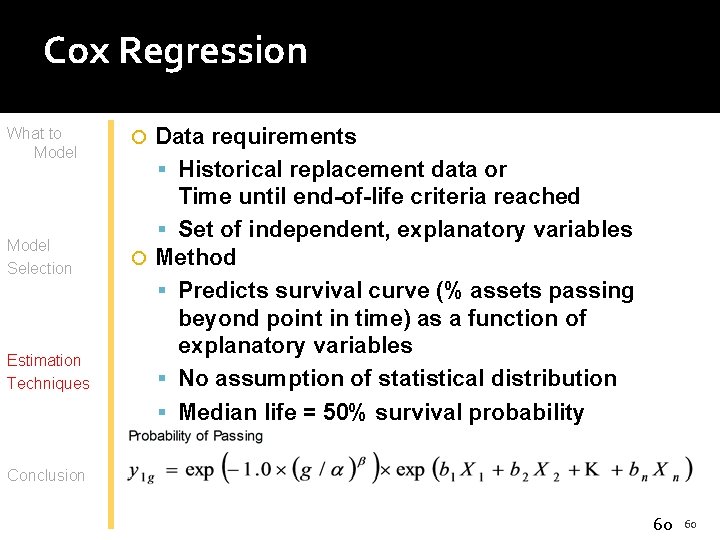
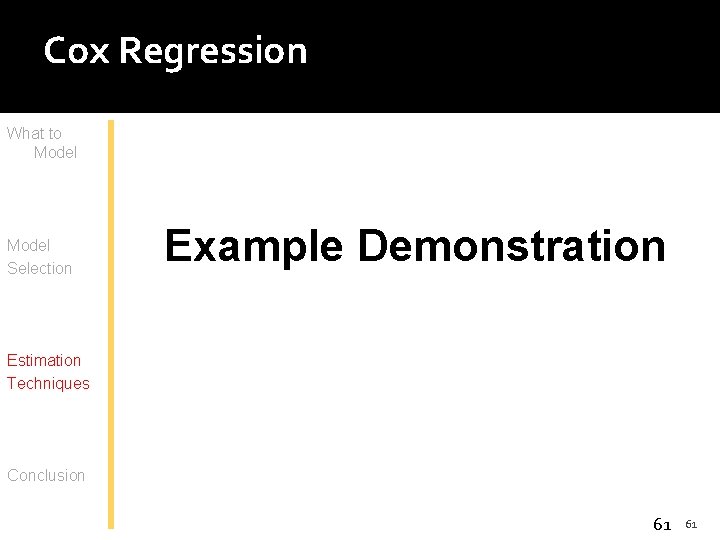
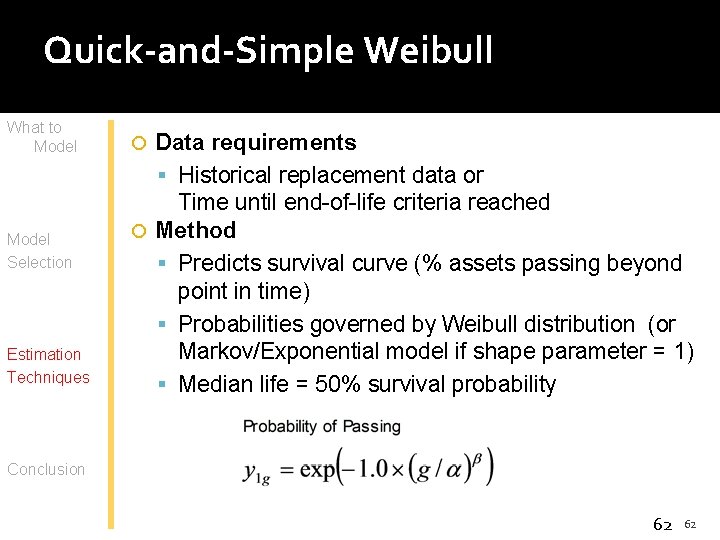
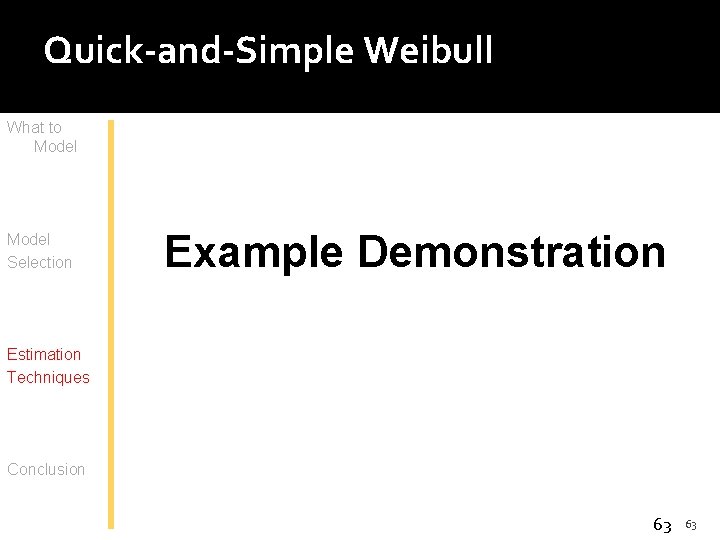
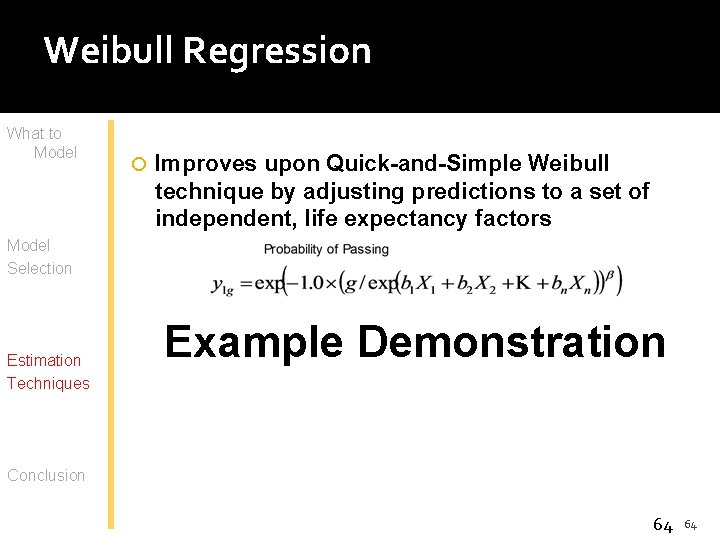
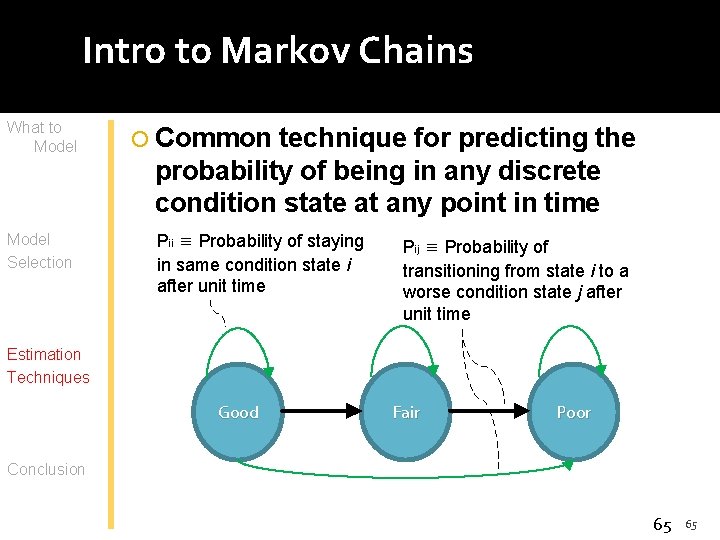
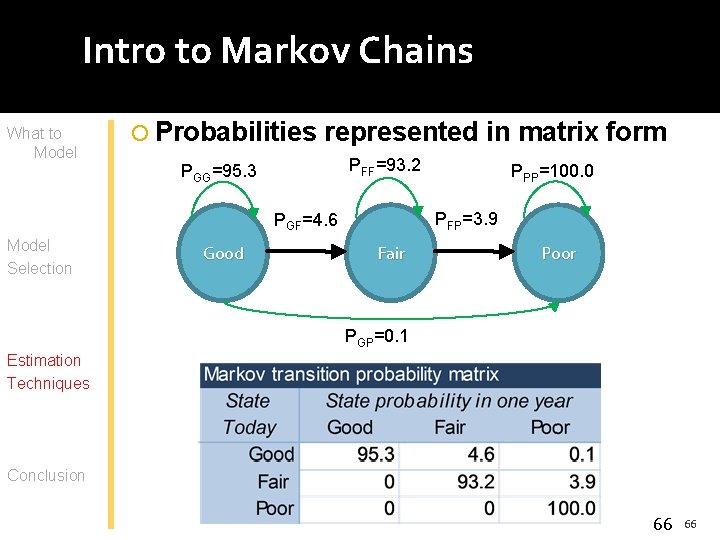
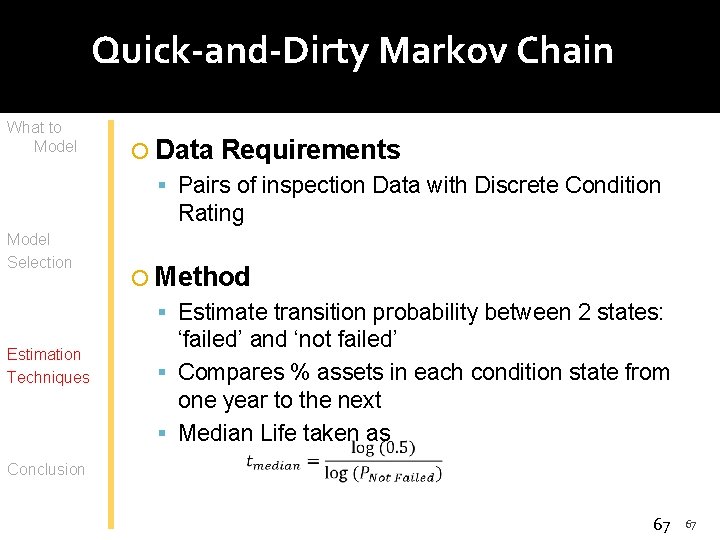
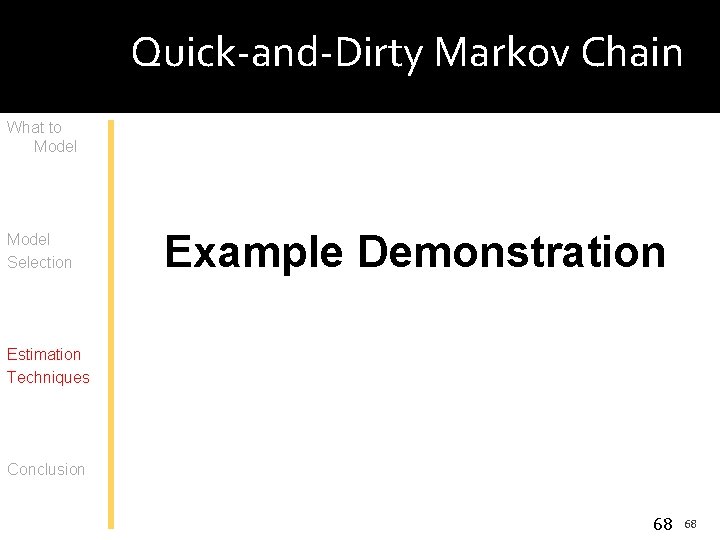
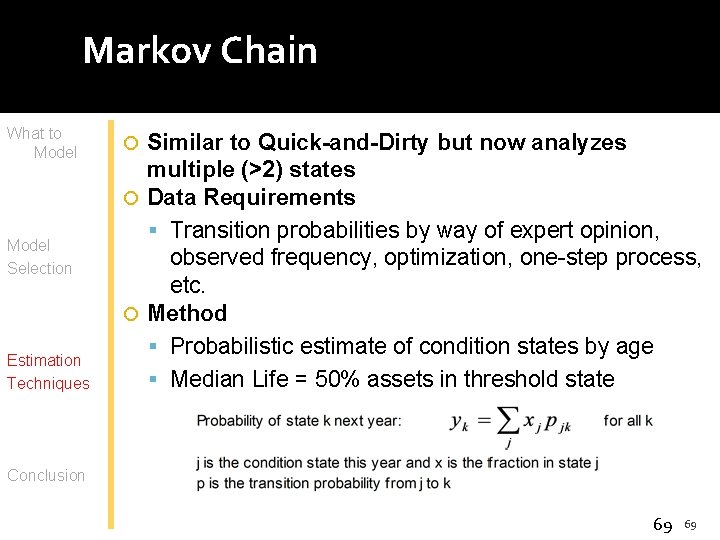
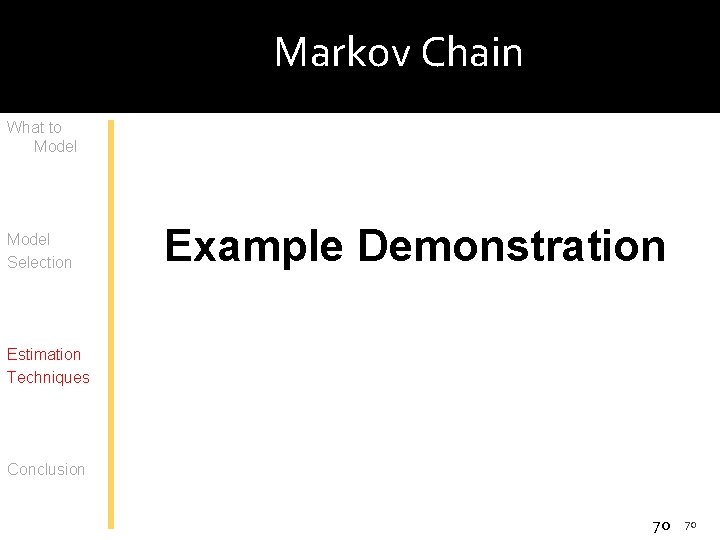
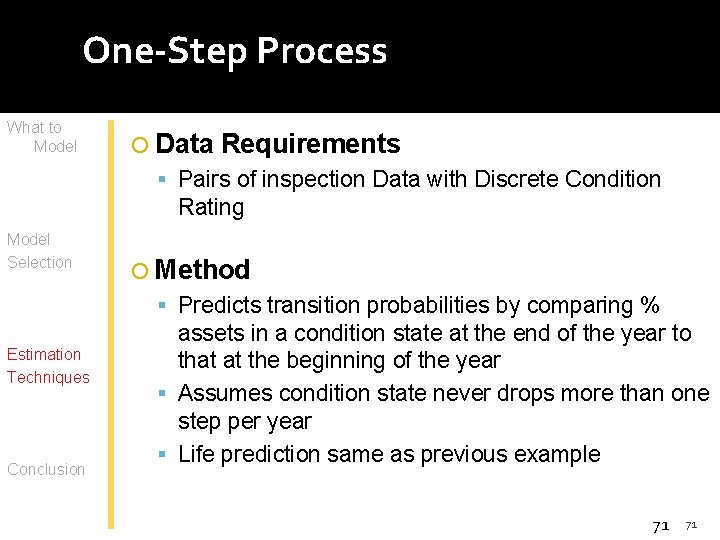
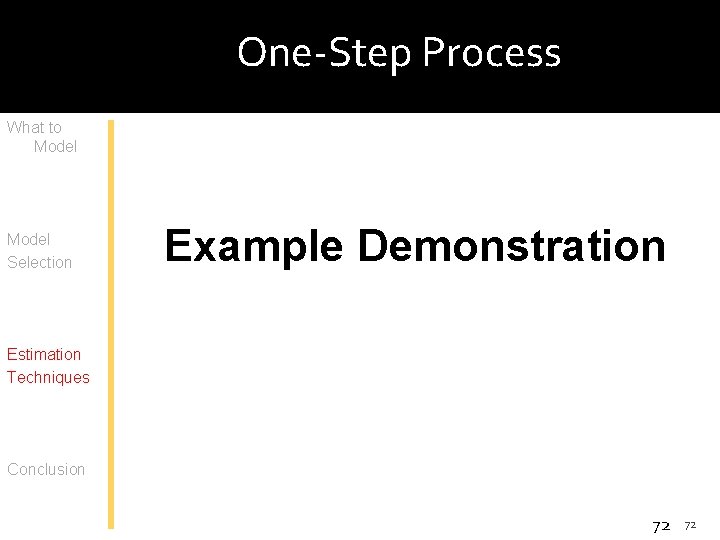
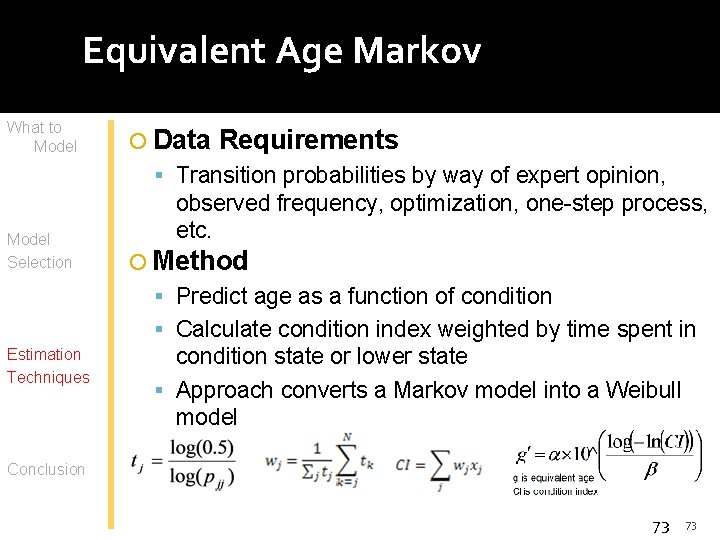
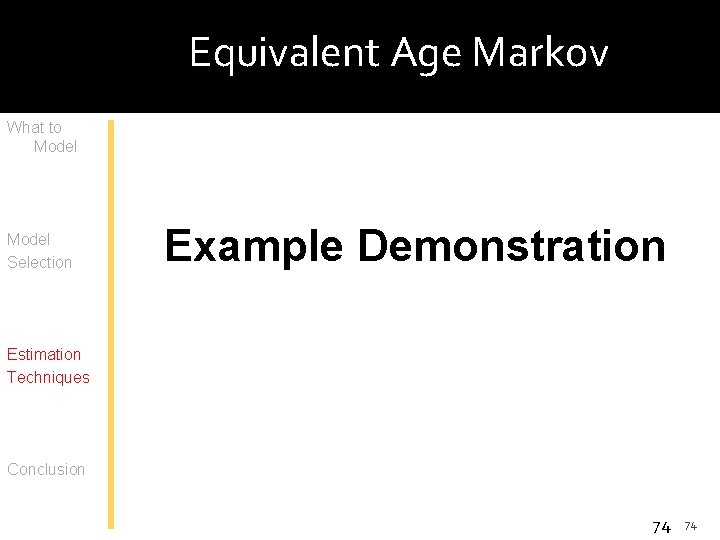
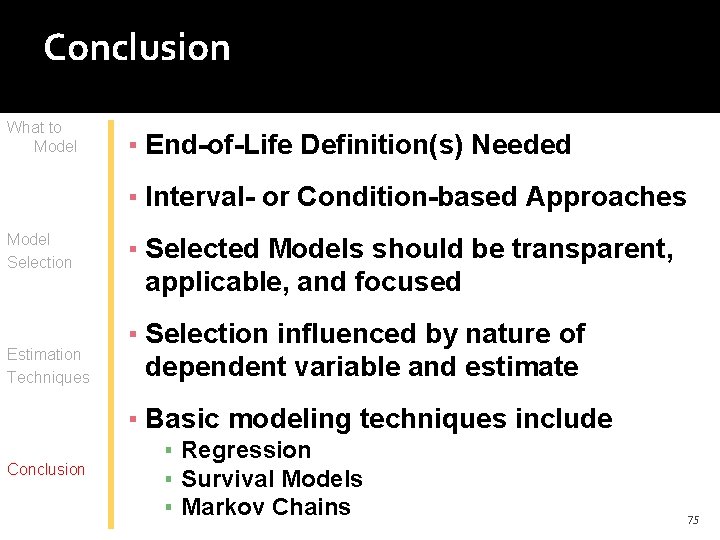
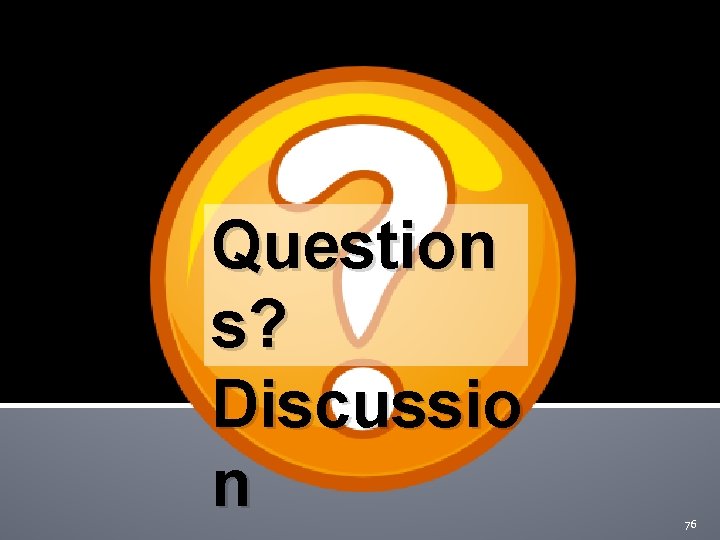
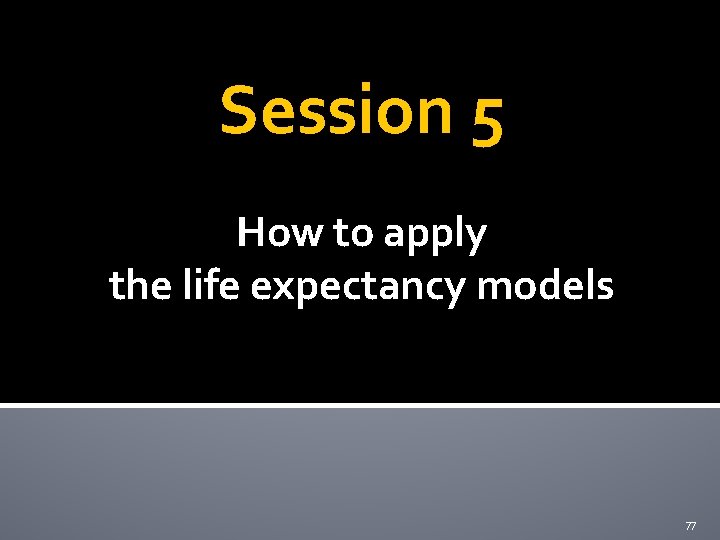
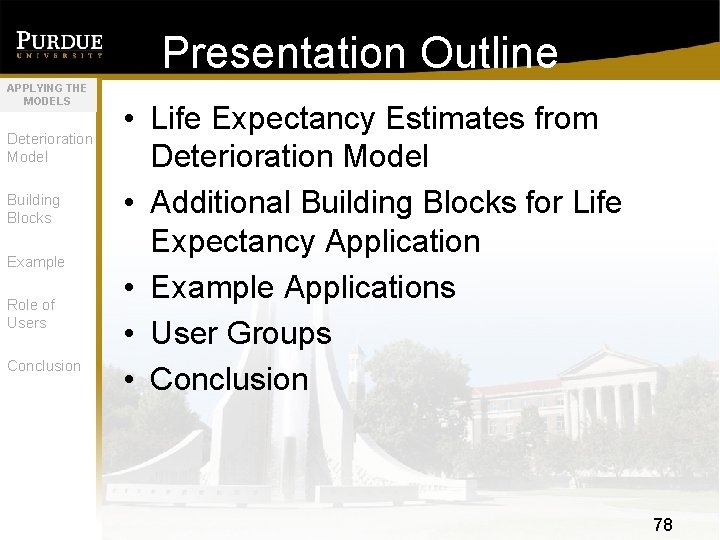
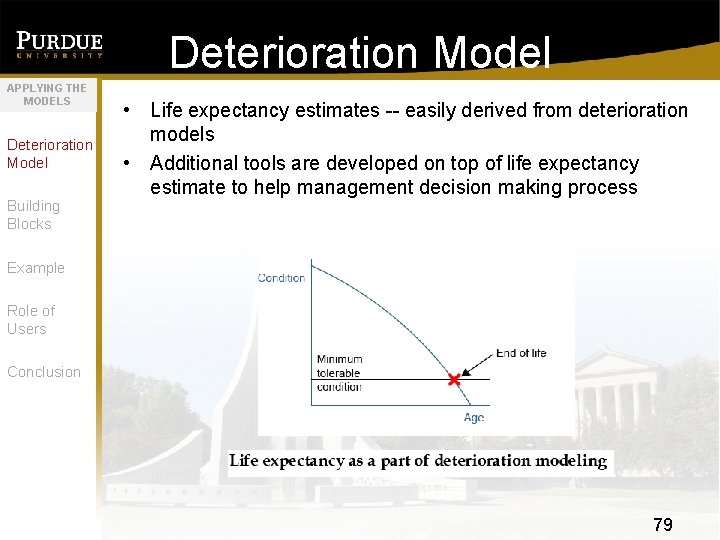
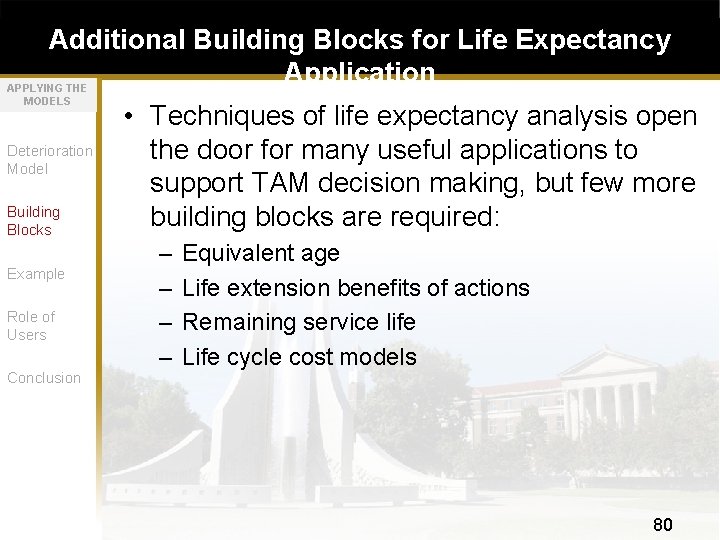
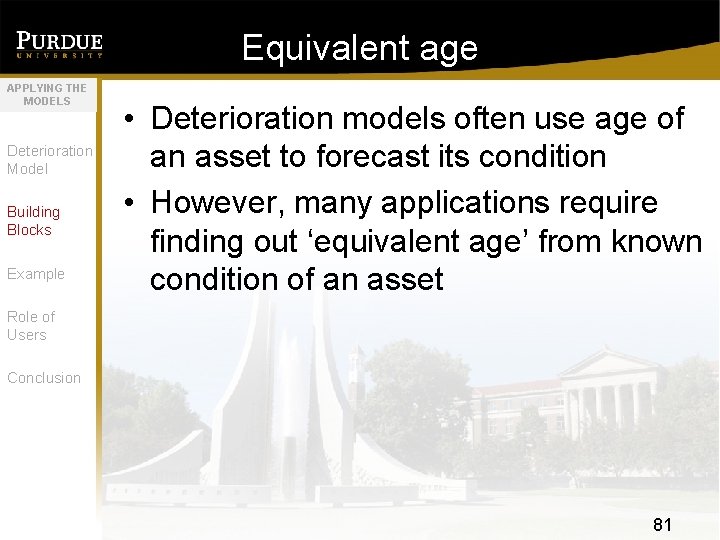
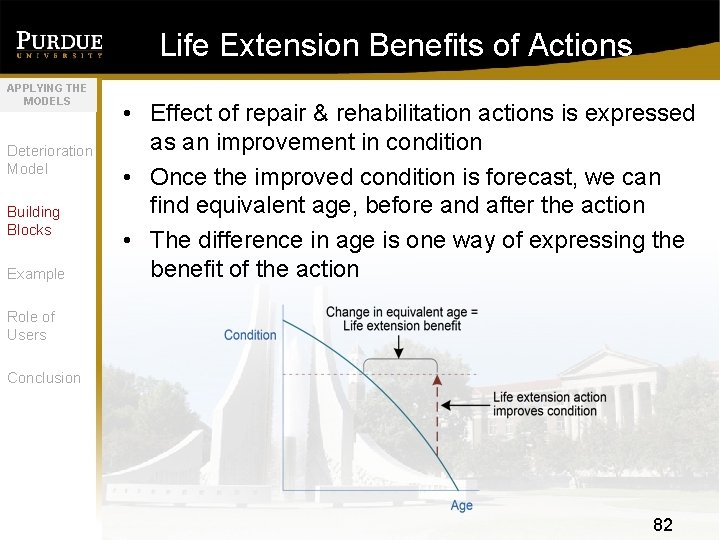
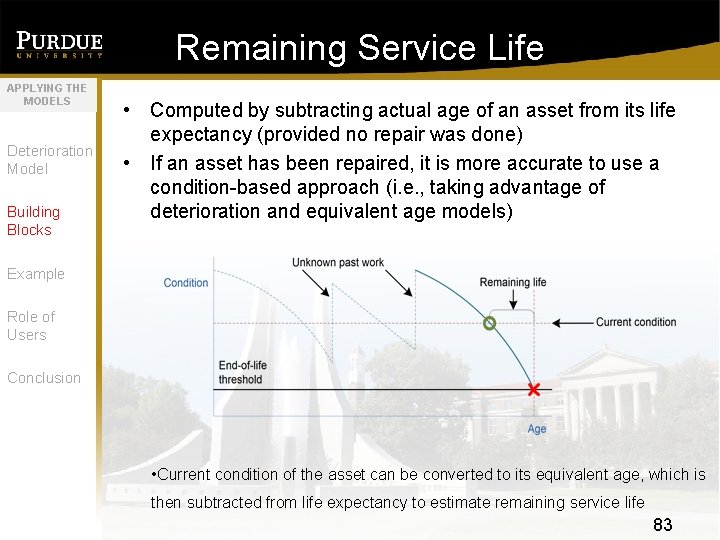
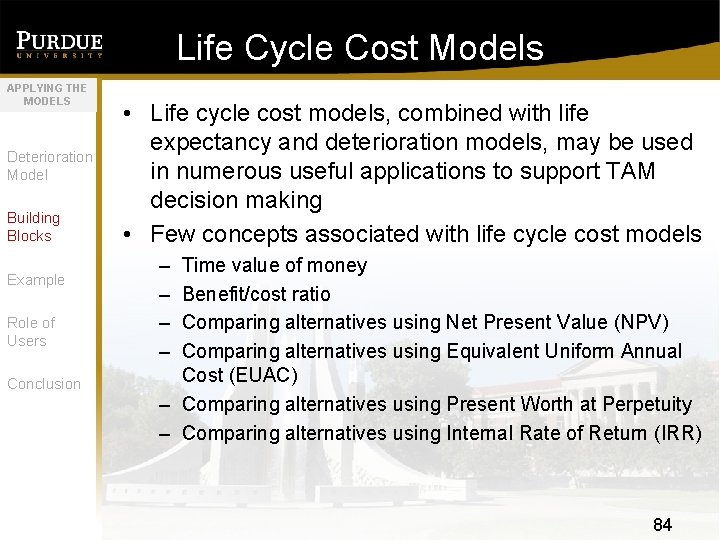
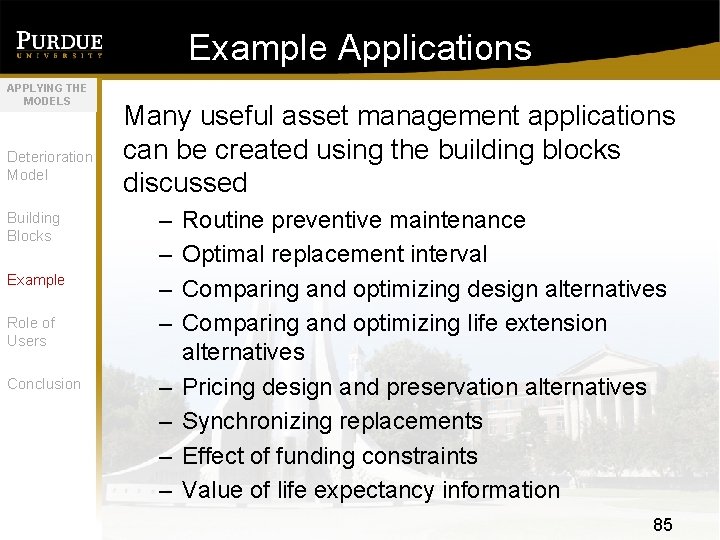
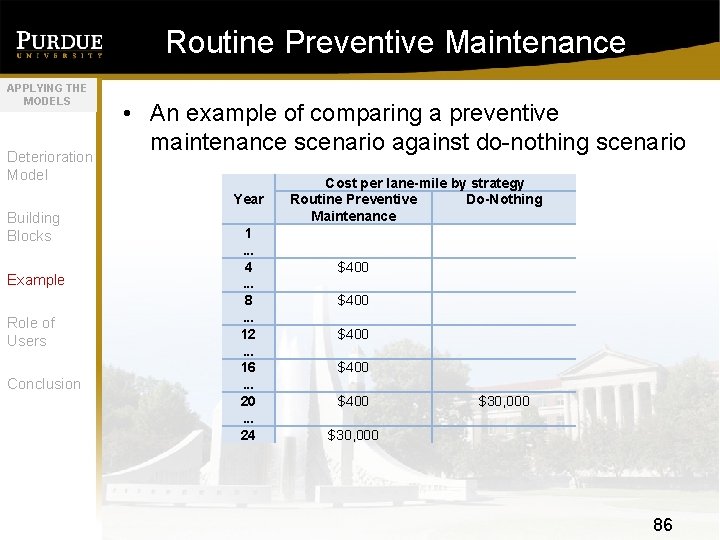
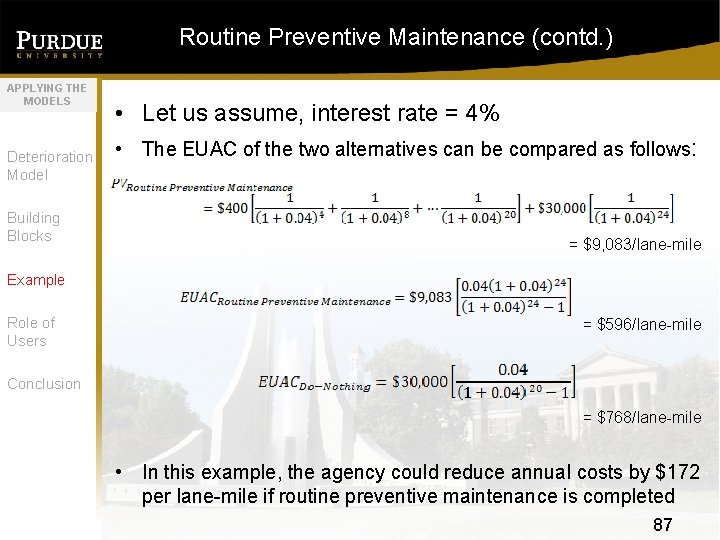
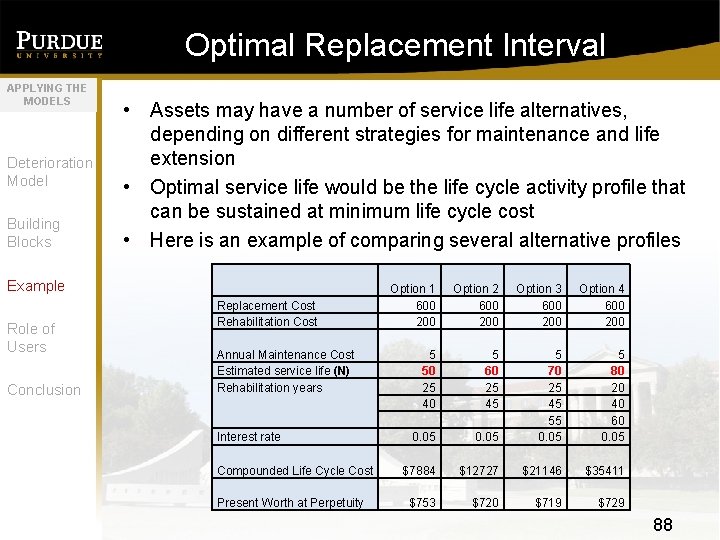
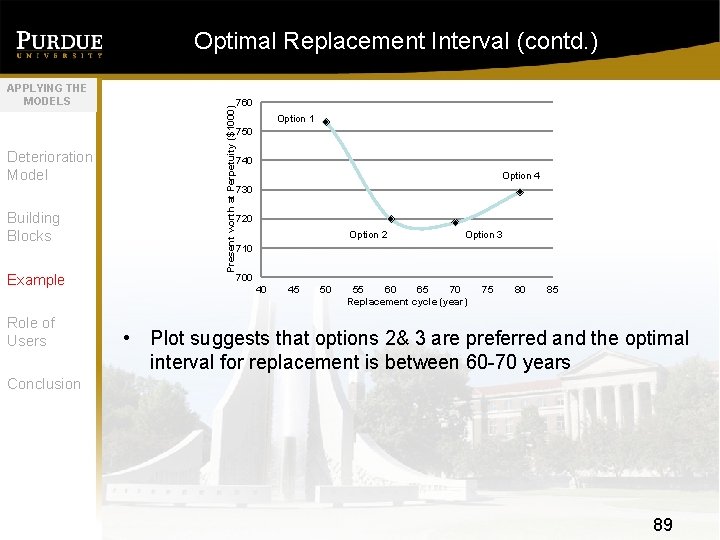
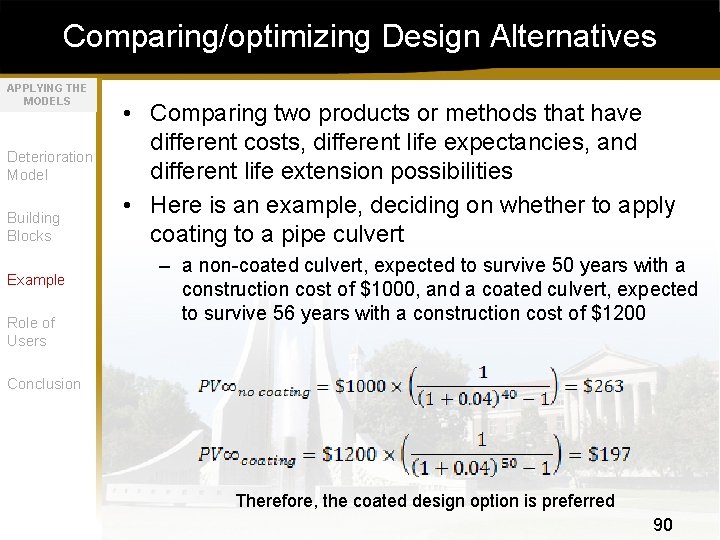
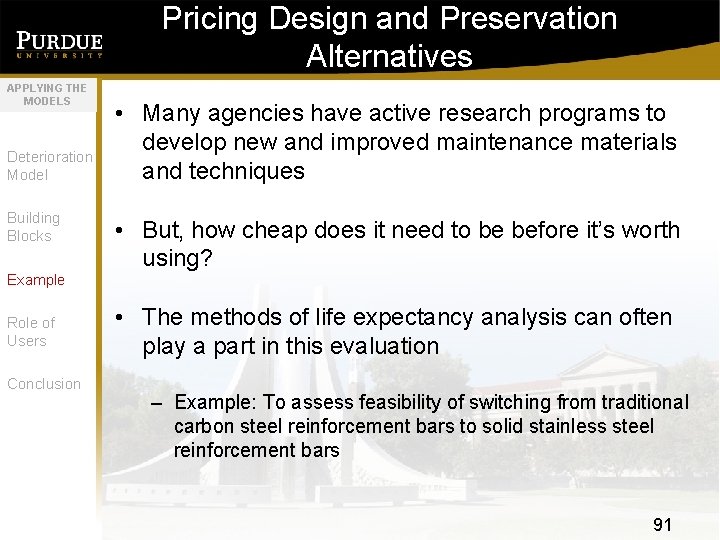
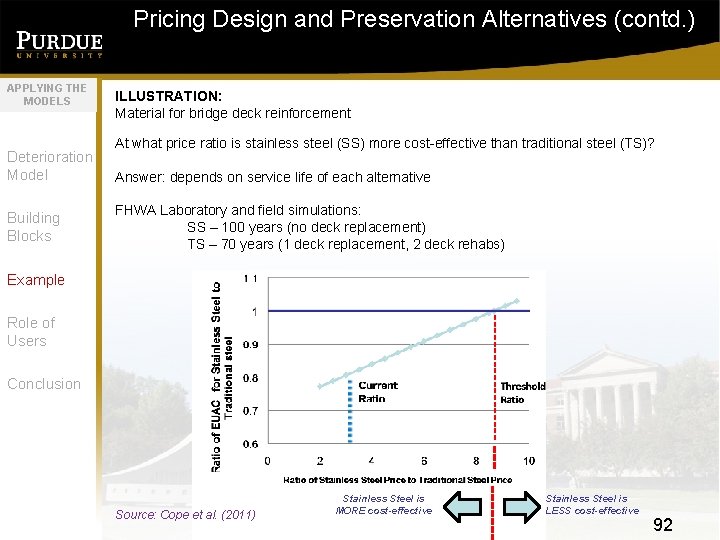
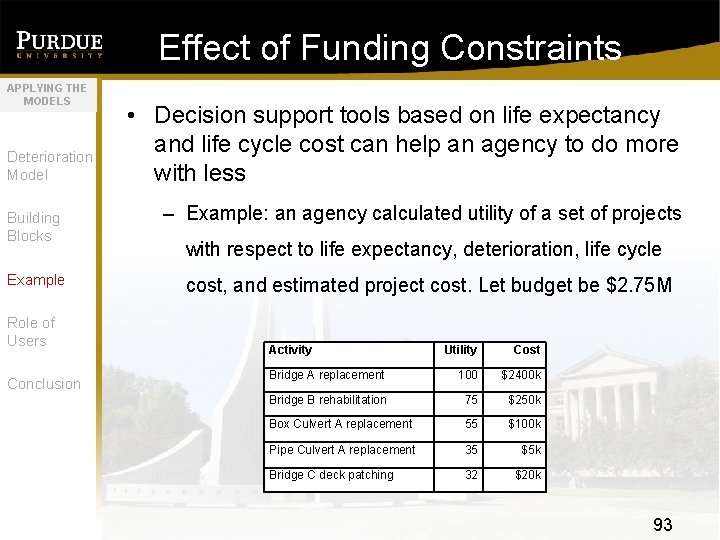
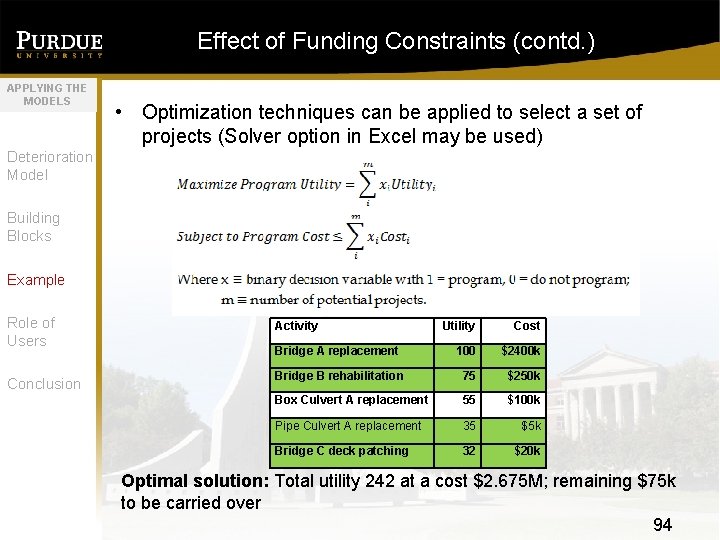
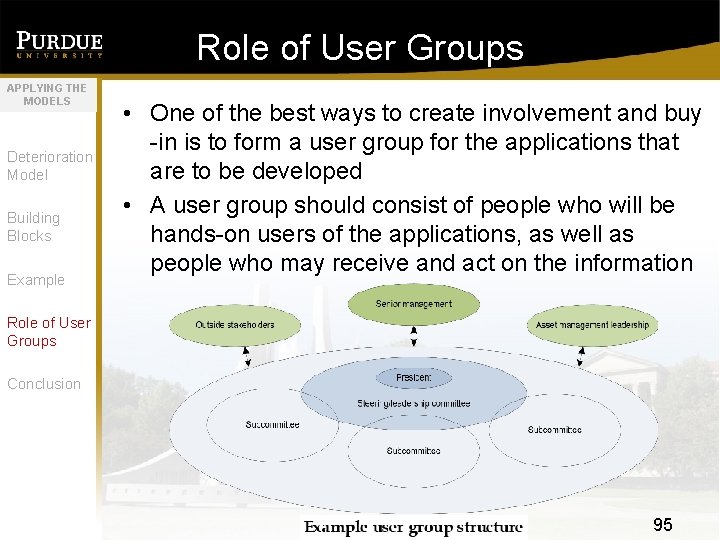
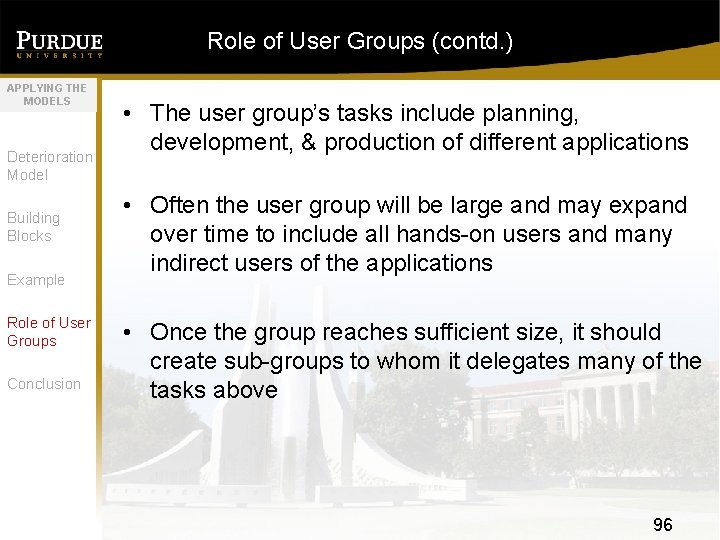
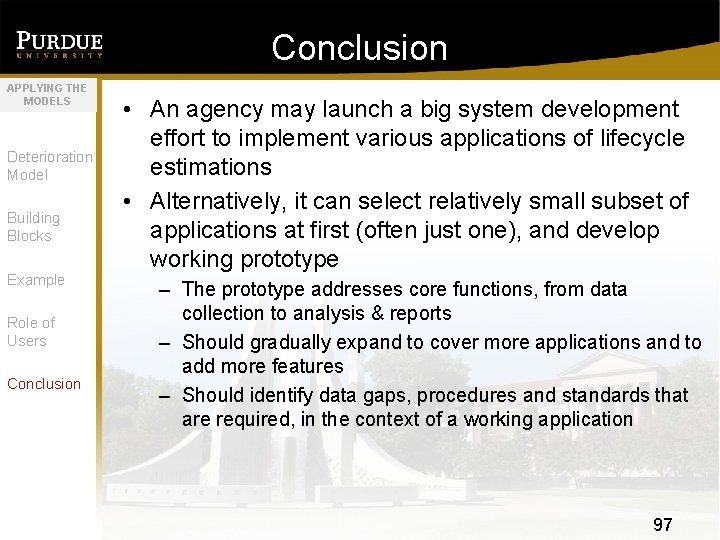
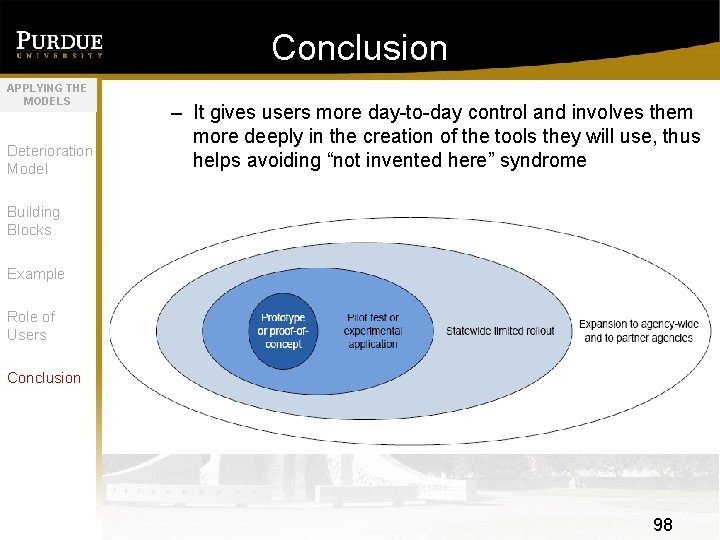
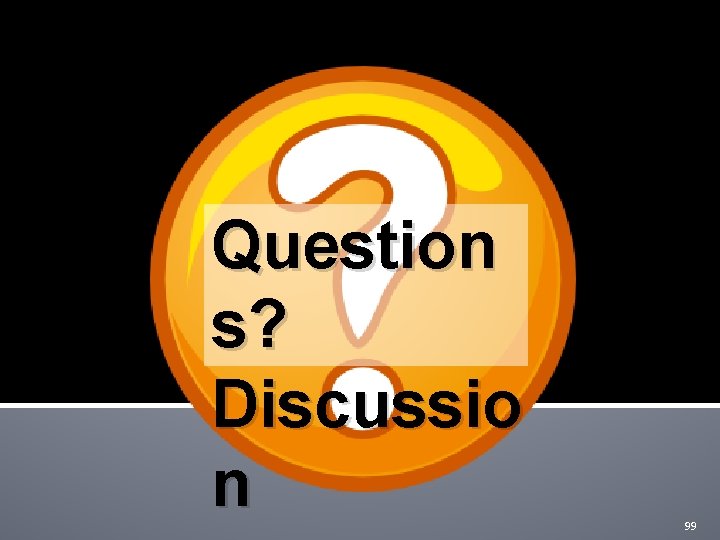
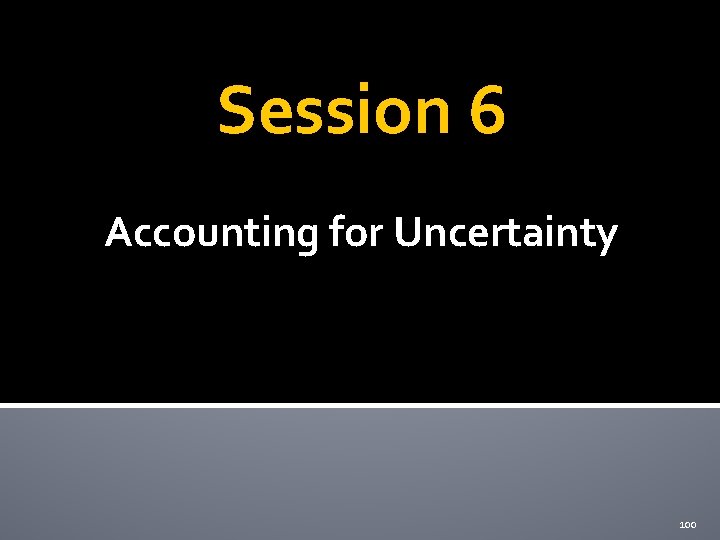
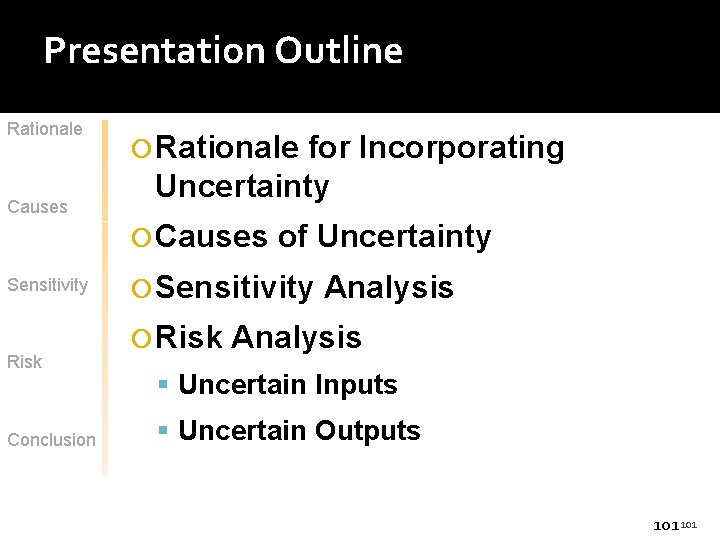
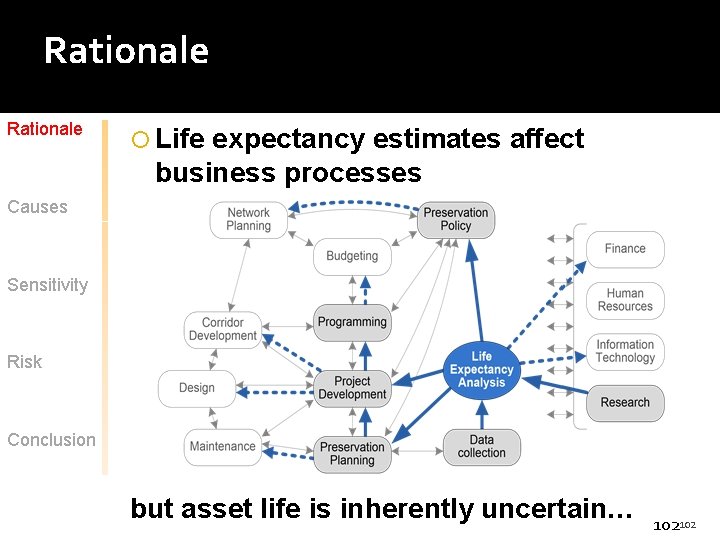
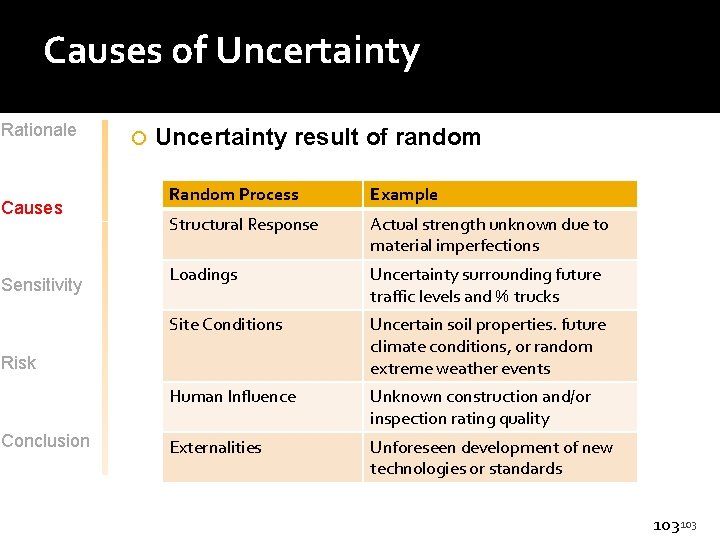
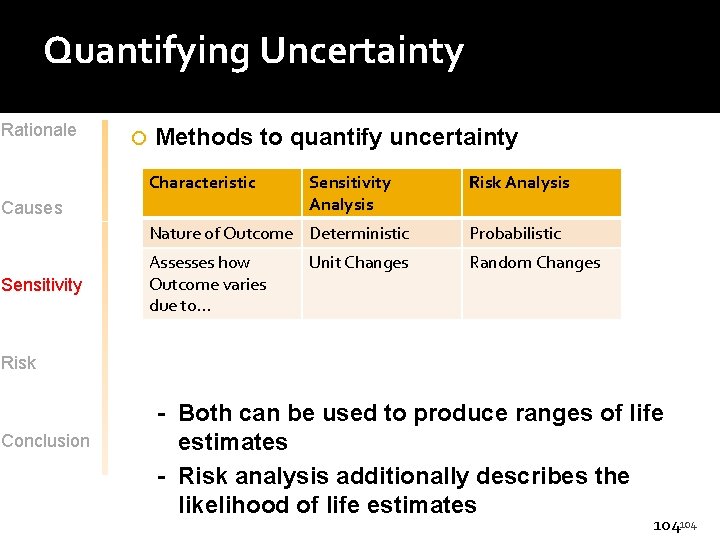
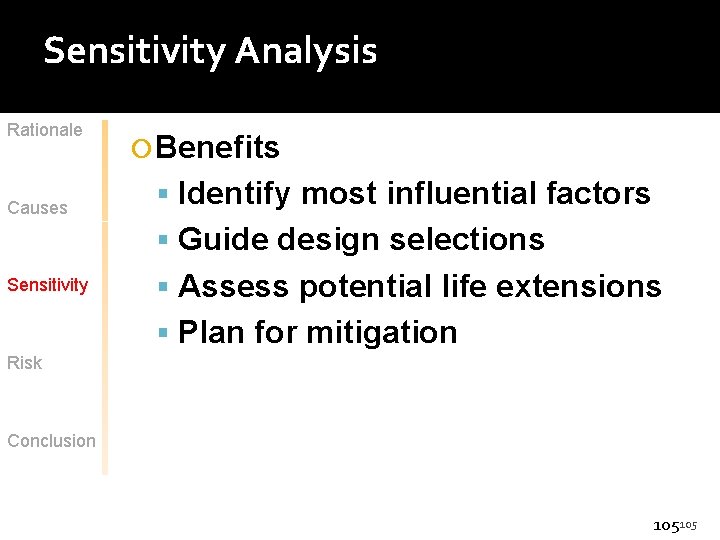
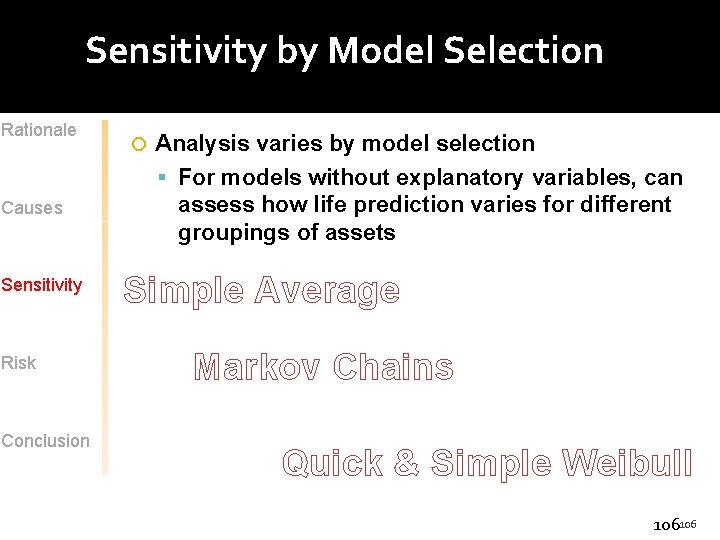
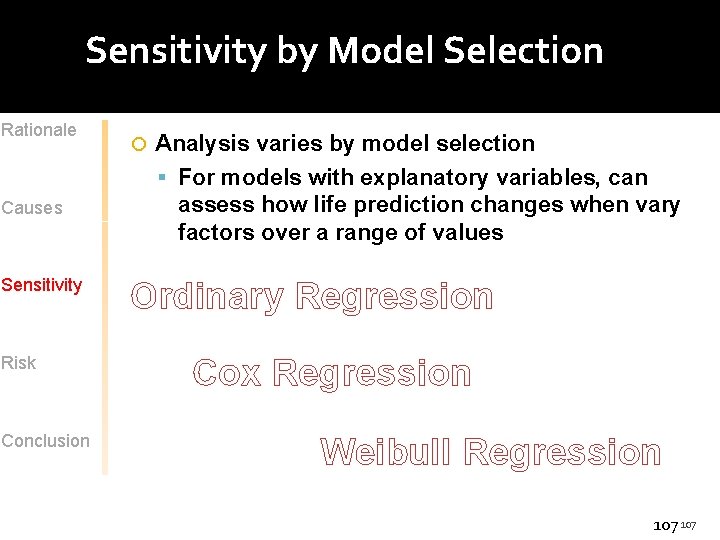
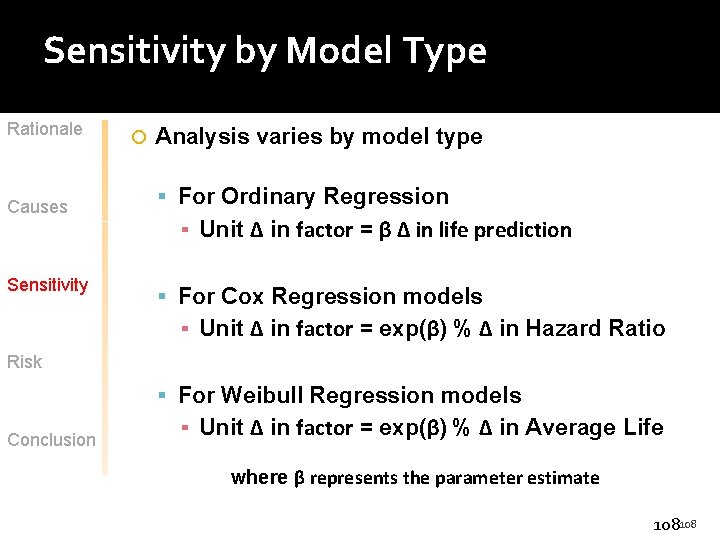
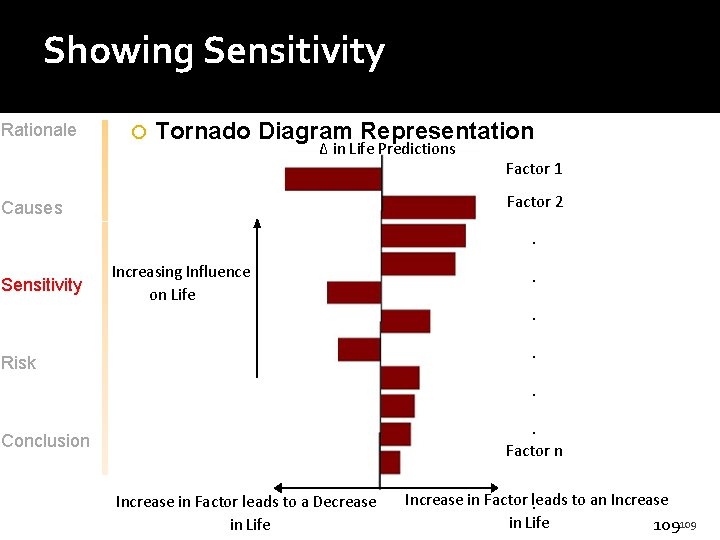
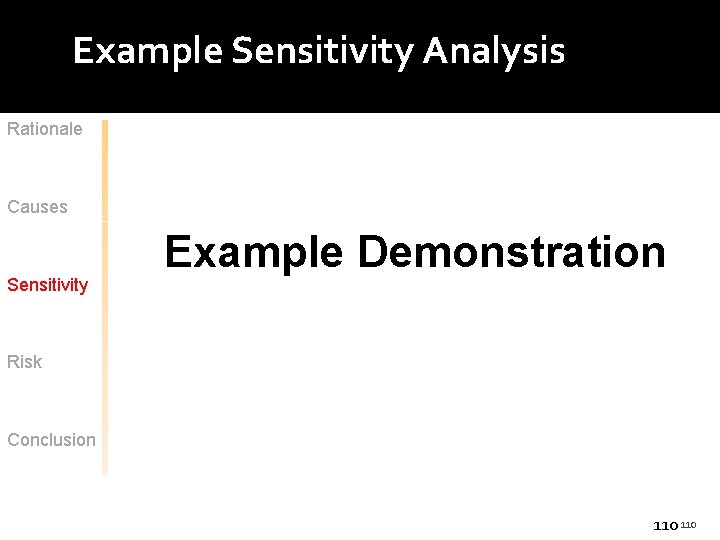
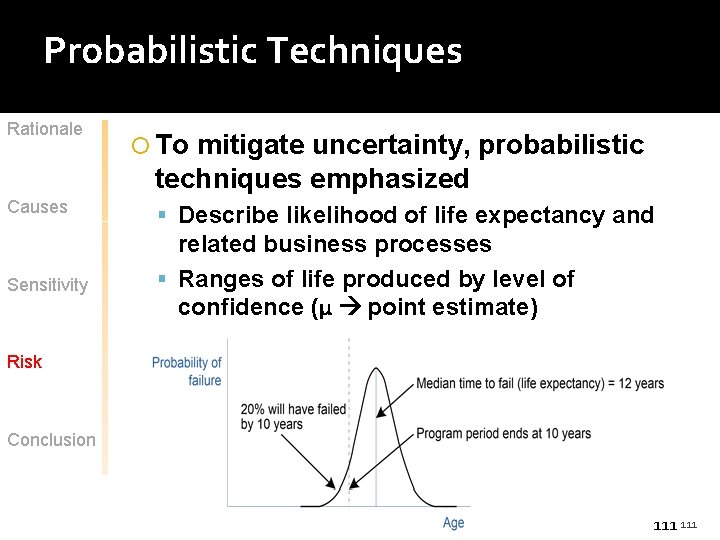
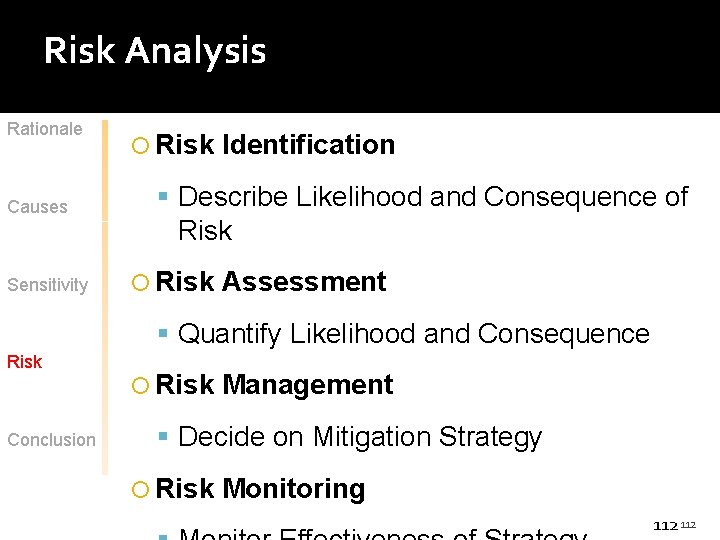
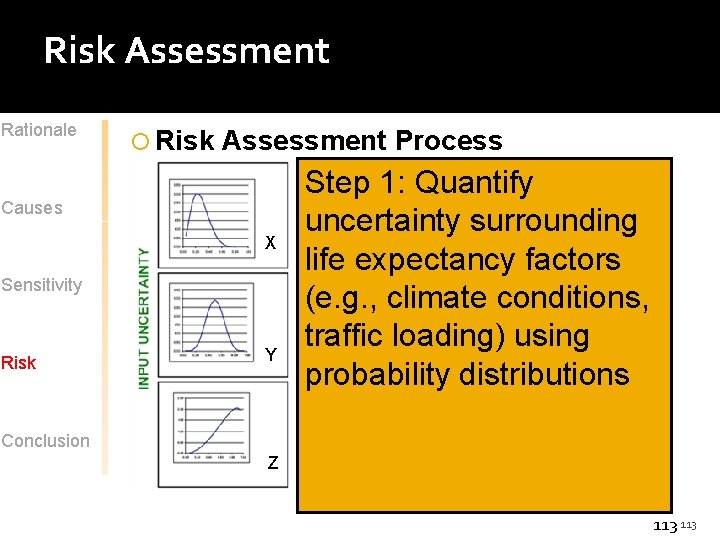
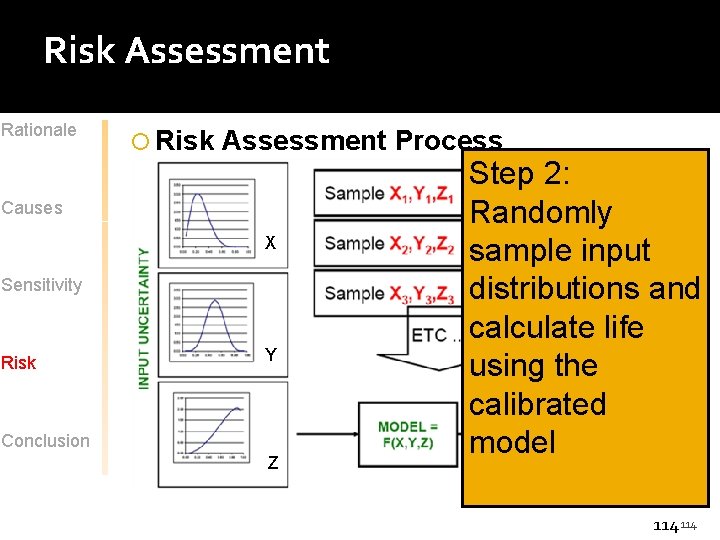
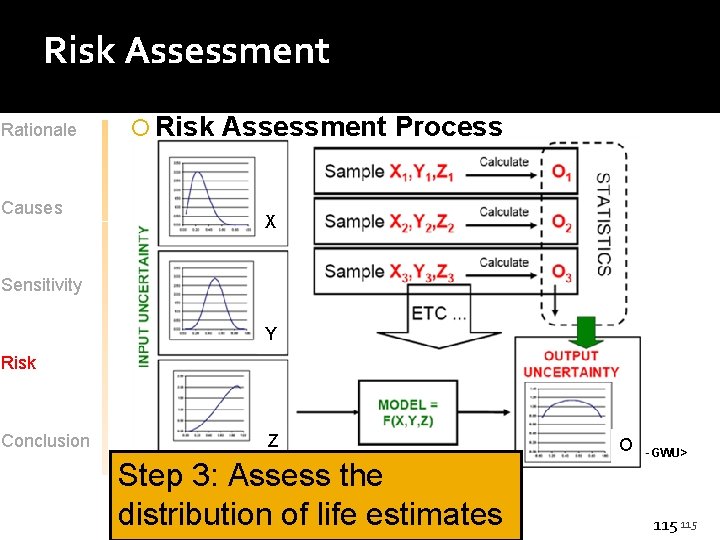
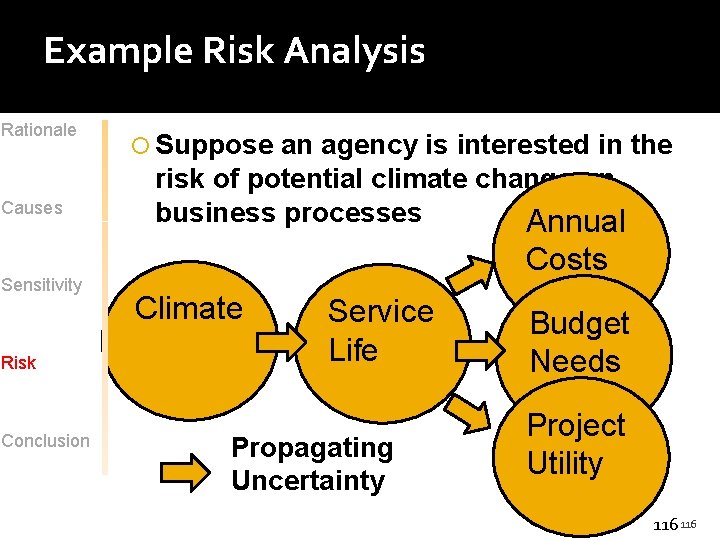
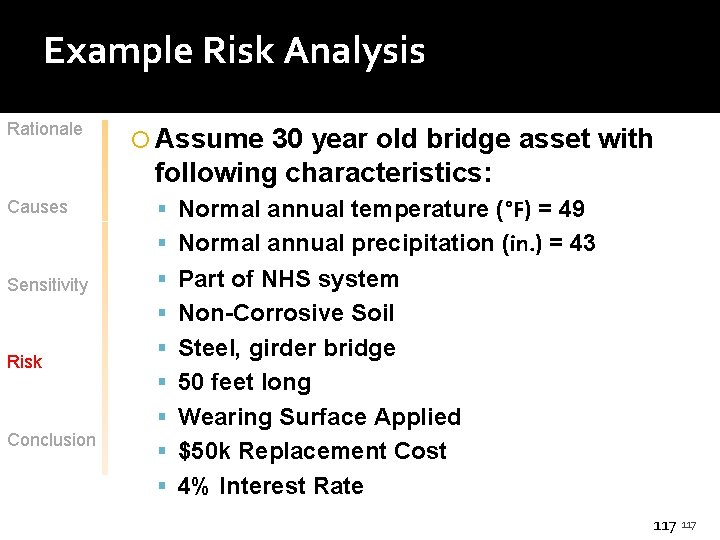
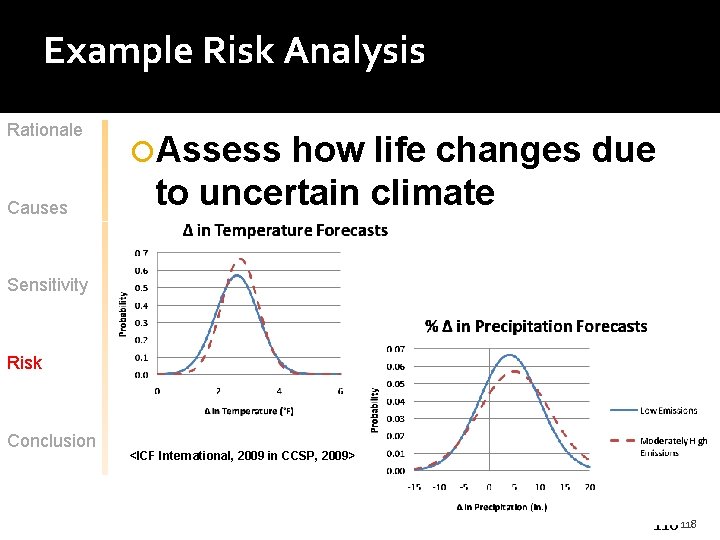
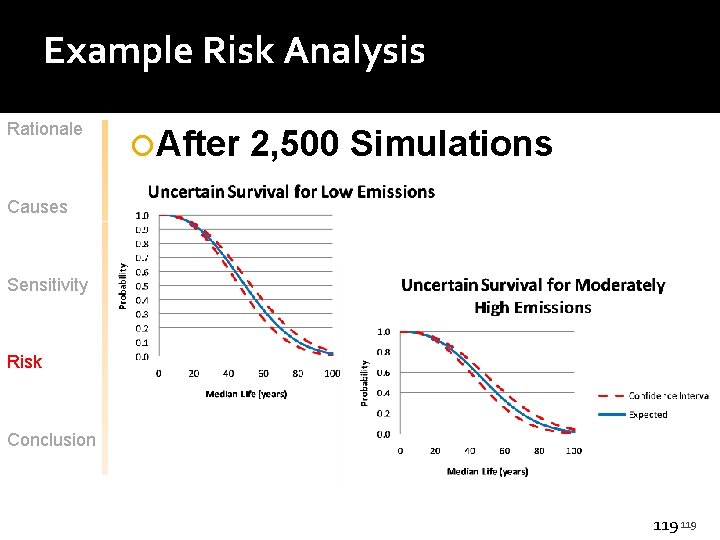
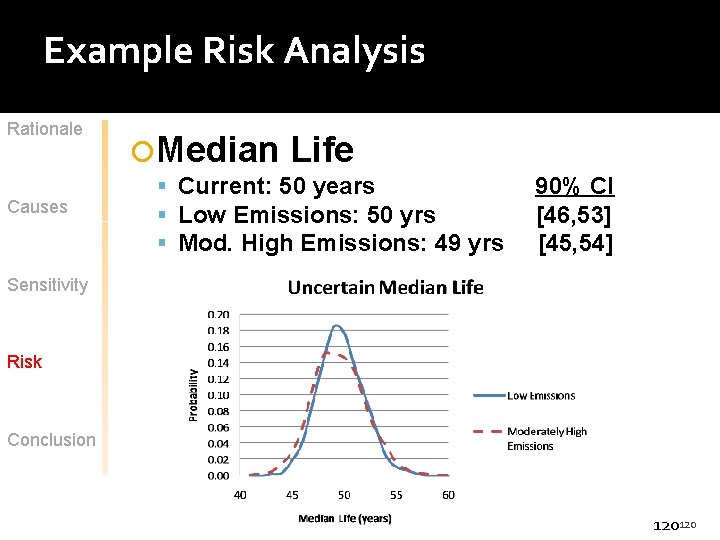
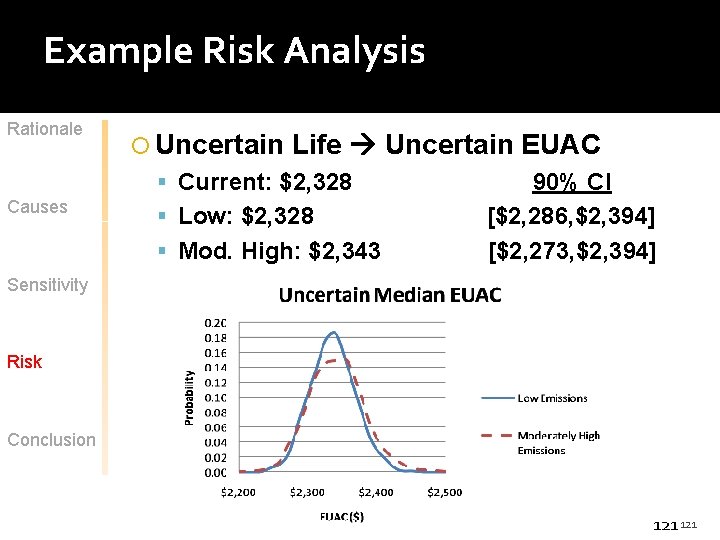
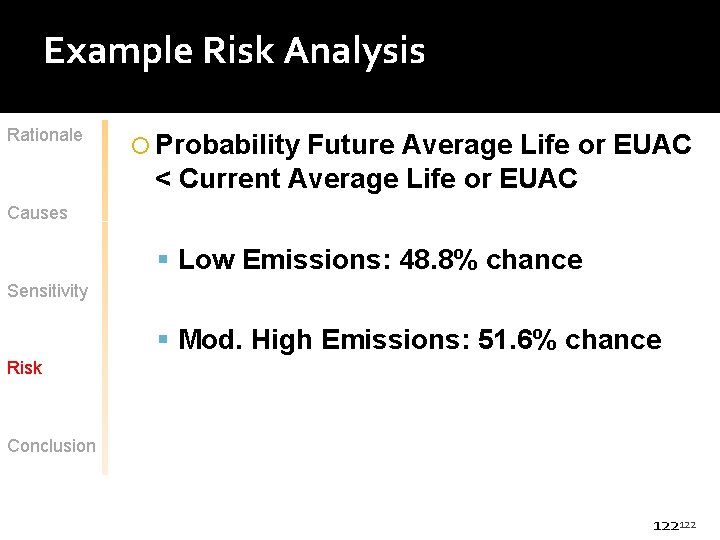
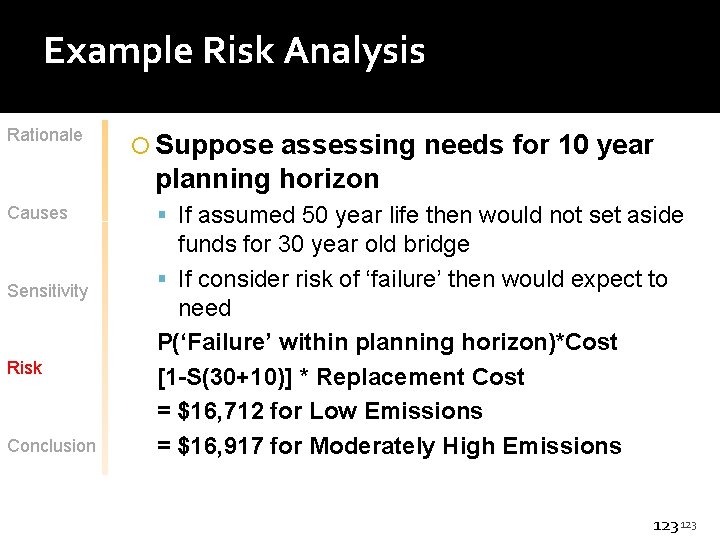
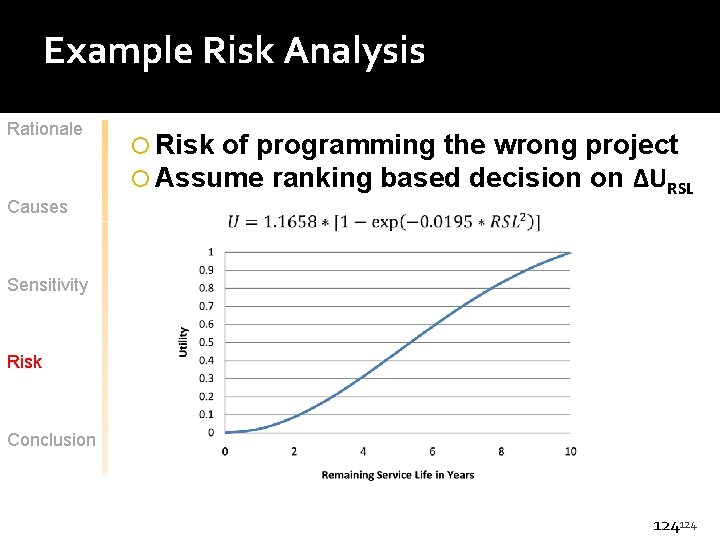
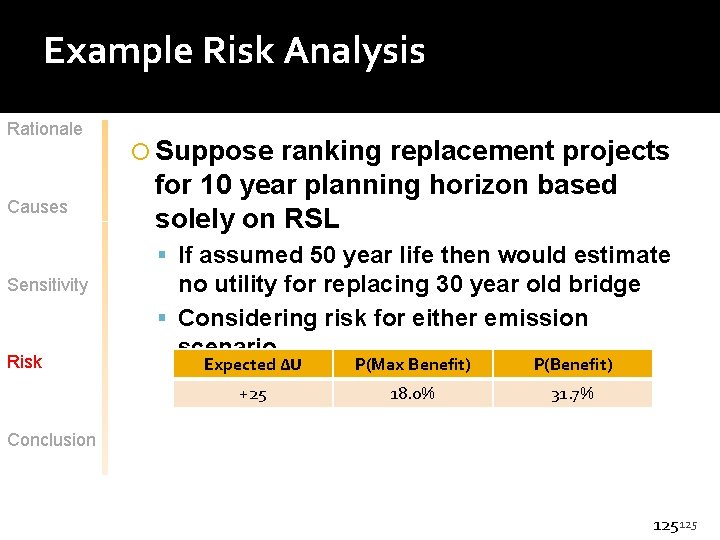
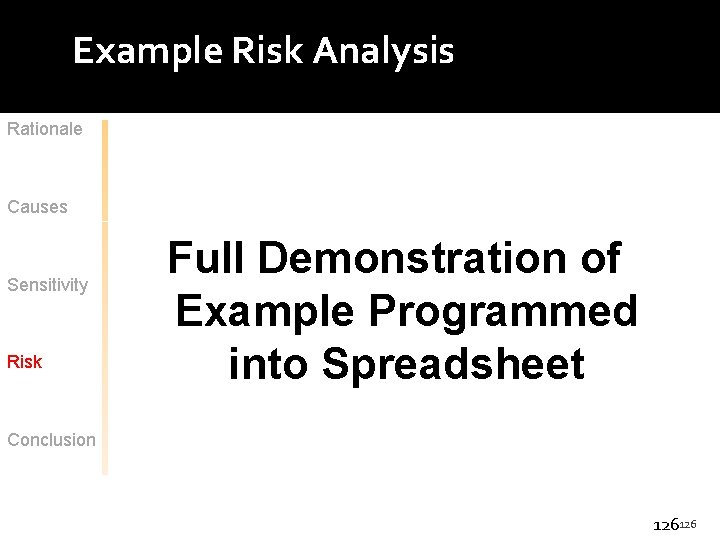
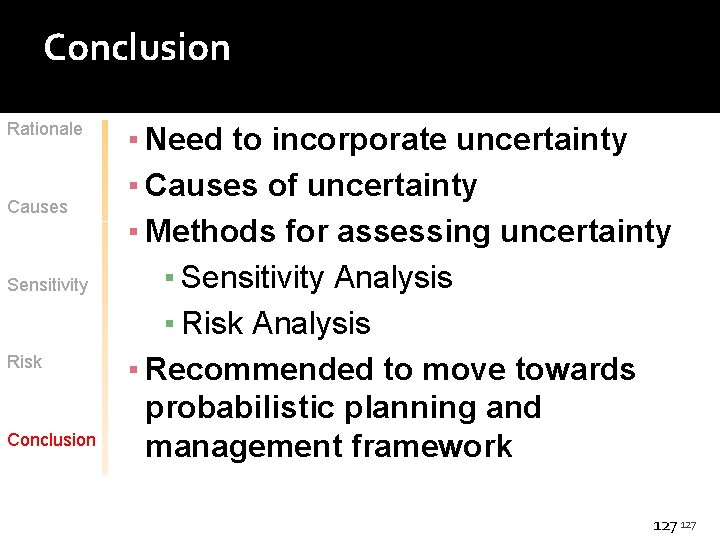
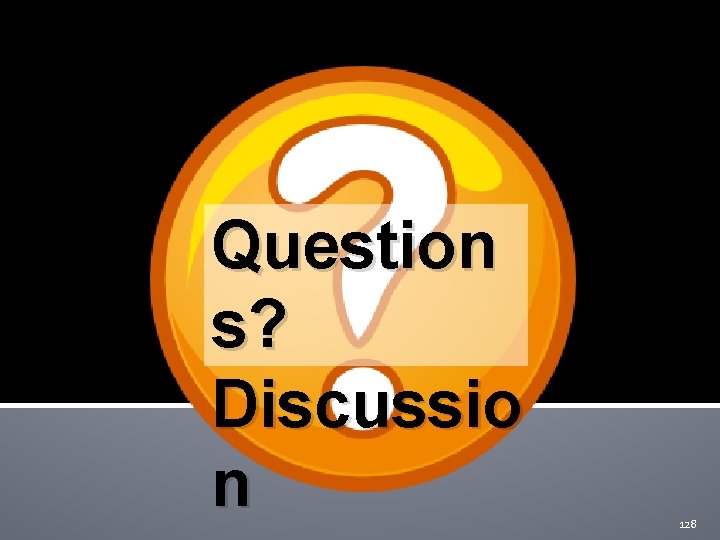
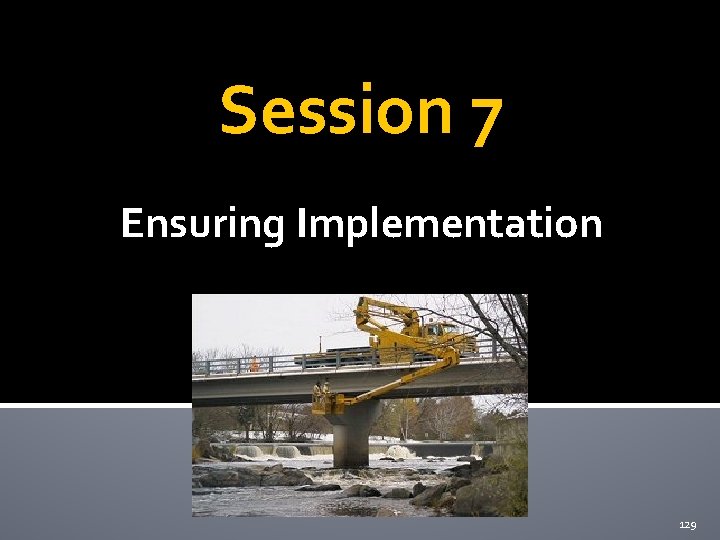
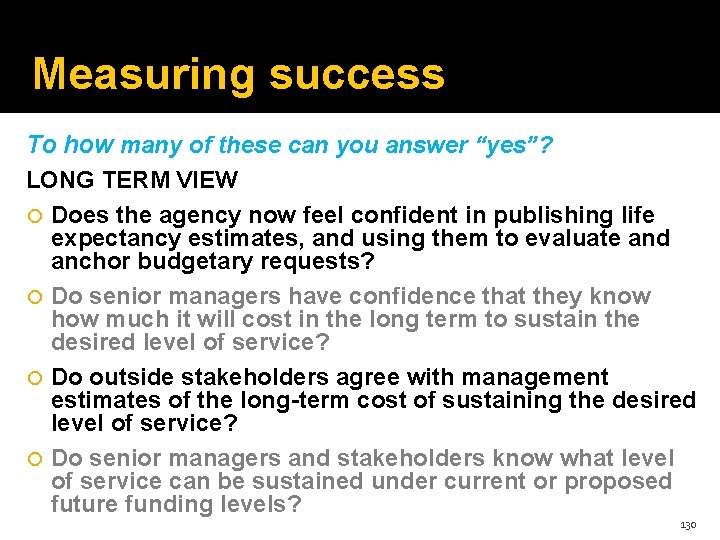
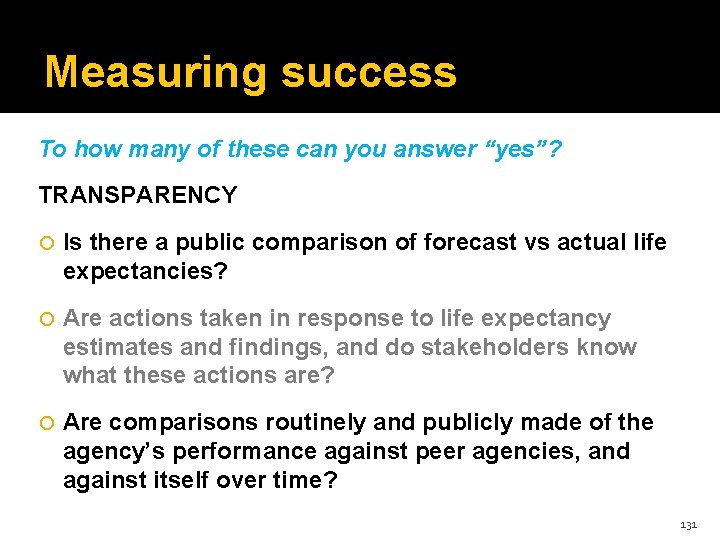
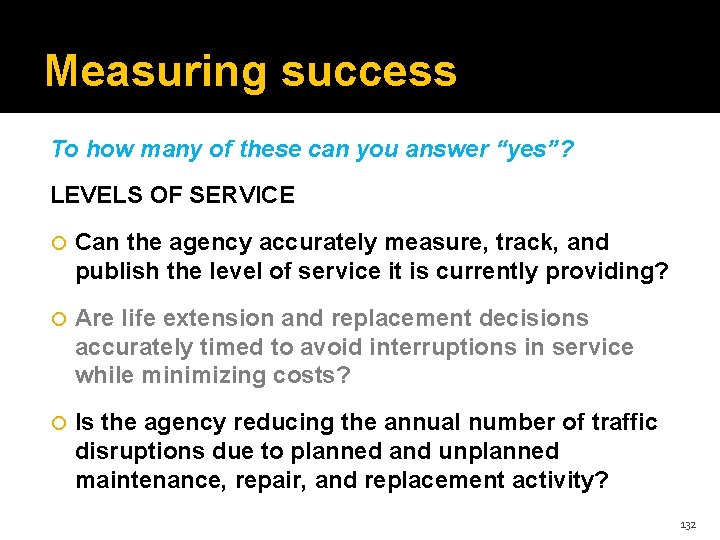
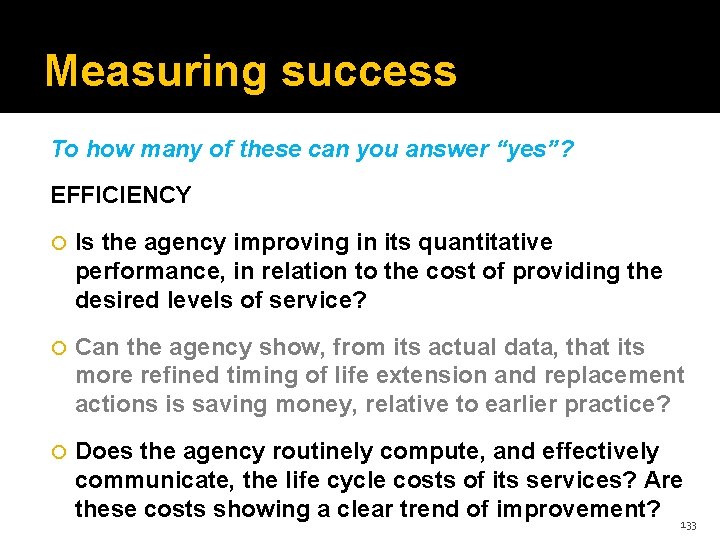
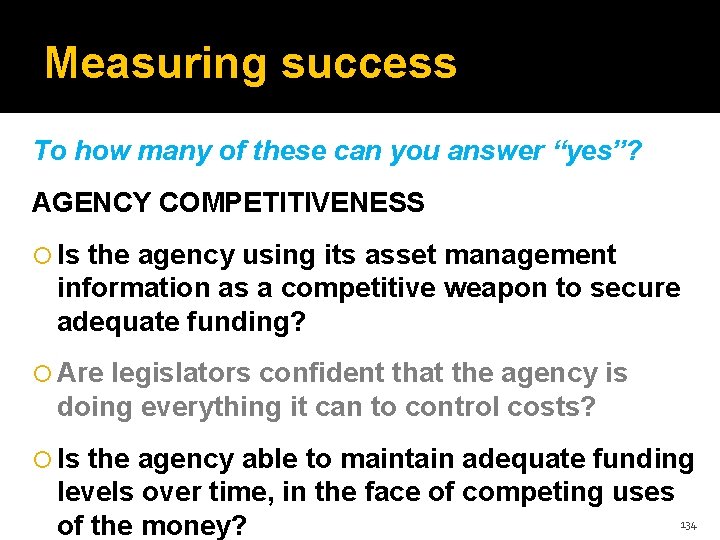
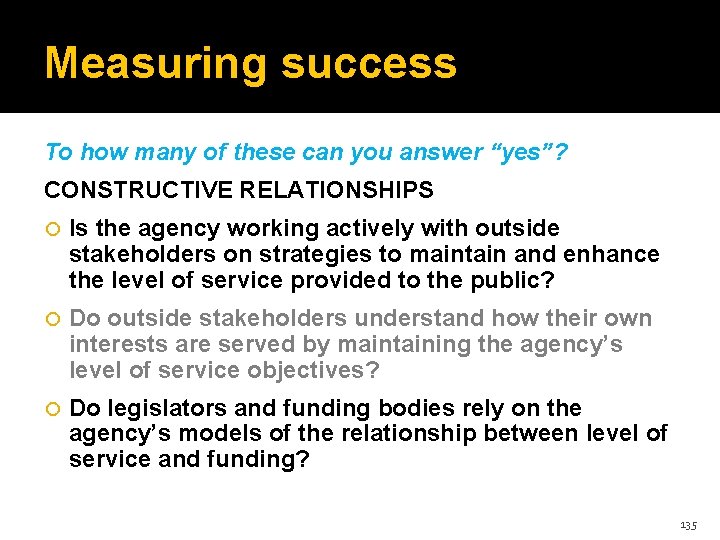
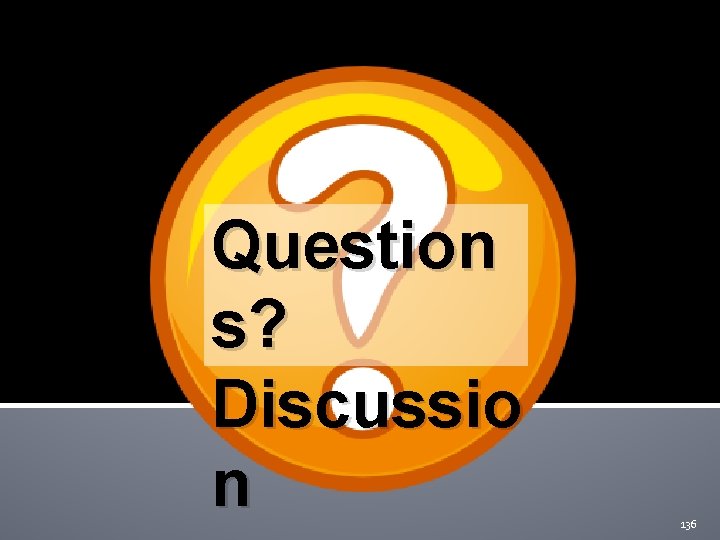
- Slides: 136
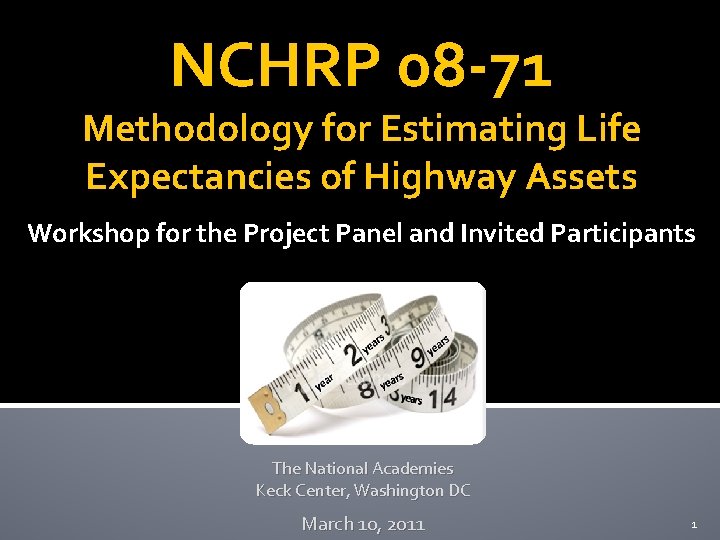
NCHRP 08 -71 Methodology for Estimating Life Expectancies of Highway Assets Workshop for the Project Panel and Invited Participants The National Academies Keck Center, Washington DC March 10, 2011 1
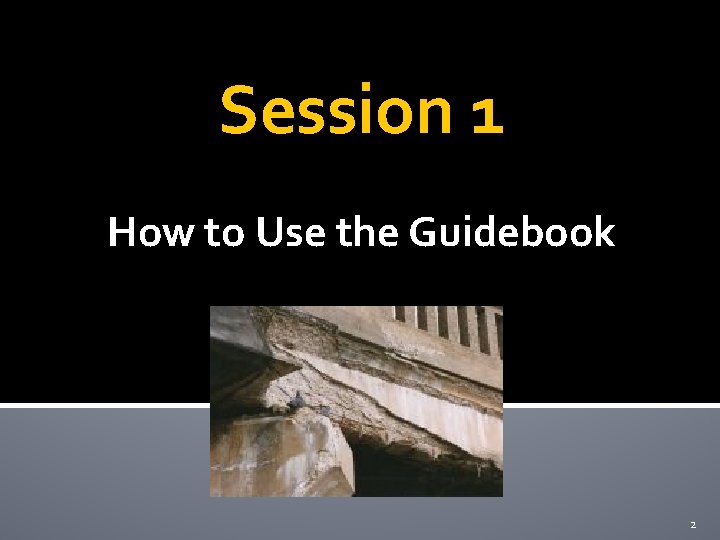
Session 1 How to Use the Guidebook 2
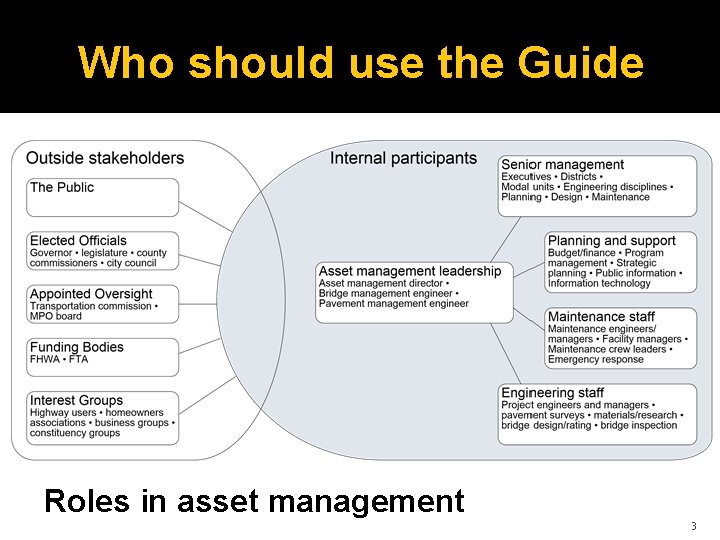
Who should use the Guide Roles in asset management 3
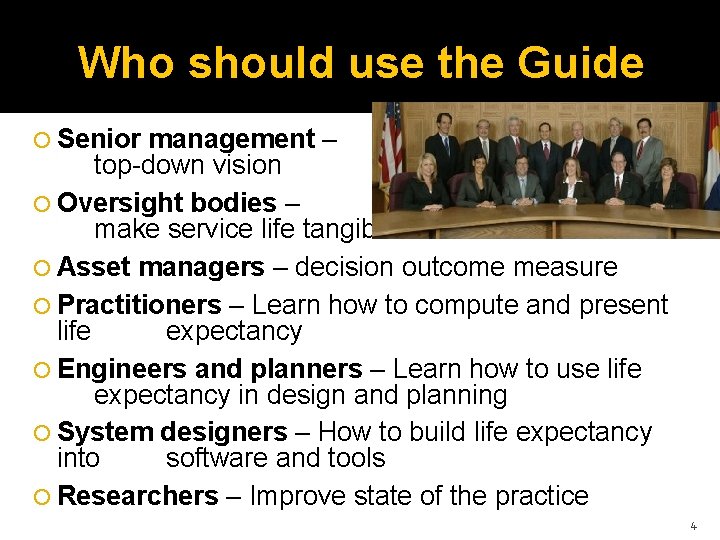
Who should use the Guide Senior management – top-down vision Oversight bodies – make service life tangible Asset managers – decision outcome measure Practitioners – Learn how to compute and present life expectancy Engineers and planners – Learn how to use life expectancy in design and planning System designers – How to build life expectancy into software and tools Researchers – Improve state of the practice 4
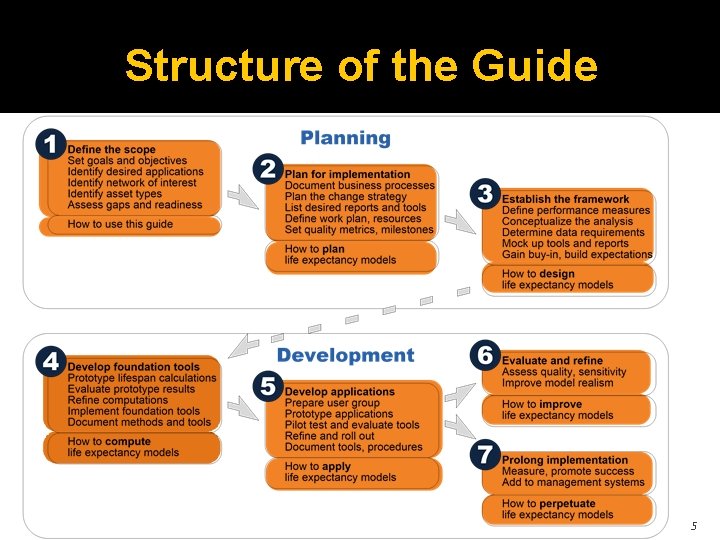
Structure of the Guide 5
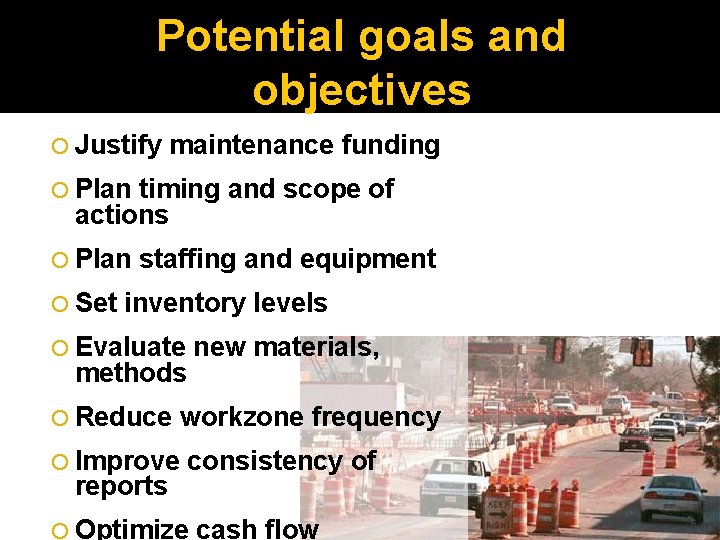
Potential goals and objectives Justify maintenance funding Plan timing and scope of actions Plan Set staffing and equipment inventory levels Evaluate new materials, methods Reduce Improve workzone frequency reports consistency of Optimize cash flow 6
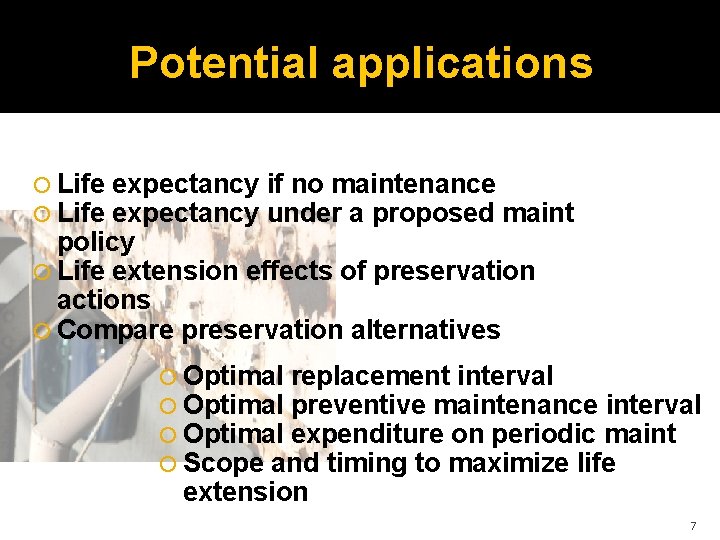
Potential applications Life expectancy if no maintenance expectancy under a proposed maint policy Life extension effects of preservation actions Compare preservation alternatives Optimal replacement interval Optimal preventive maintenance interval Optimal expenditure on periodic maint Scope and timing to maximize life extension 7
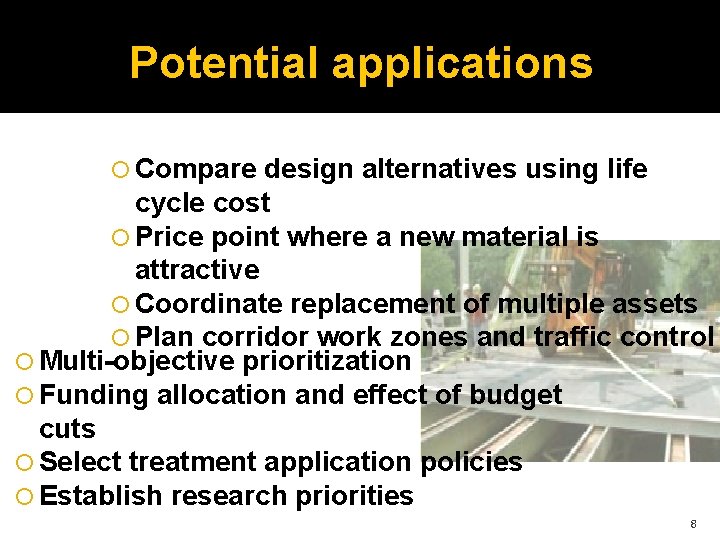
Potential applications Compare design alternatives using life cycle cost Price point where a new material is attractive Coordinate replacement of multiple assets Plan corridor work zones and traffic control Multi-objective prioritization Funding allocation and effect of budget cuts Select treatment application policies Establish research priorities 8
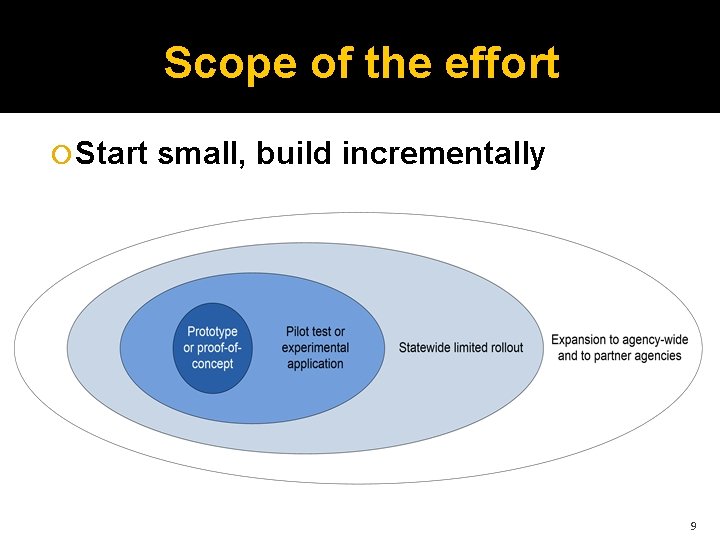
Scope of the effort Start small, build incrementally 9
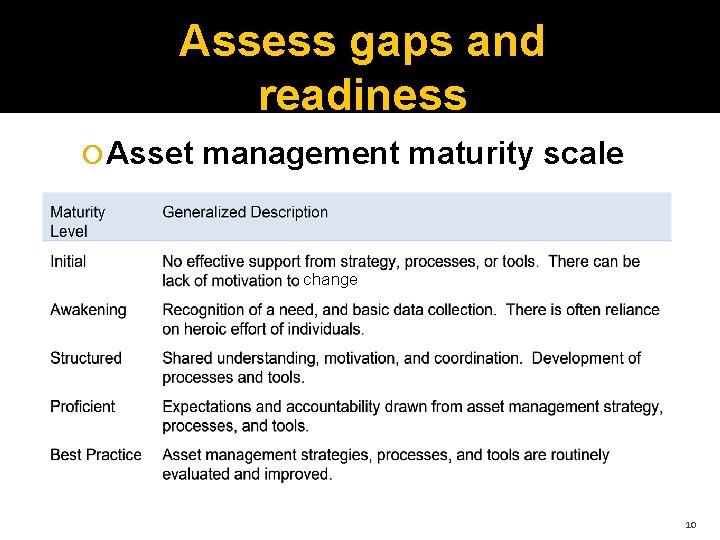
Assess gaps and readiness Asset management maturity scale change 10
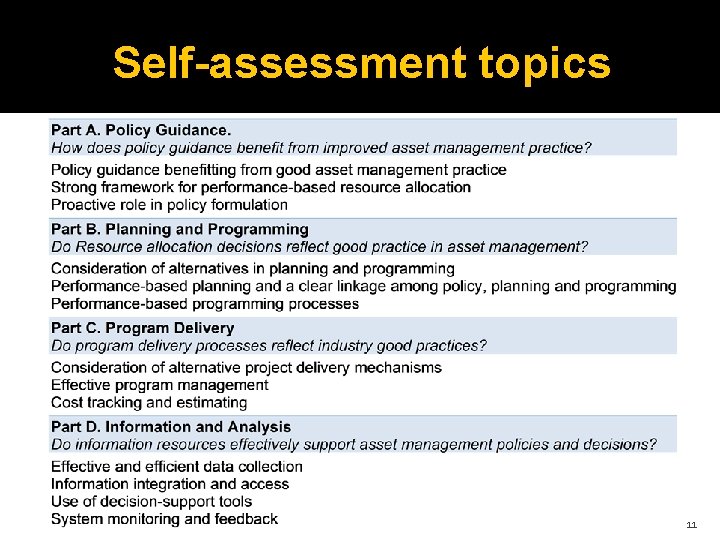
Self-assessment topics 11
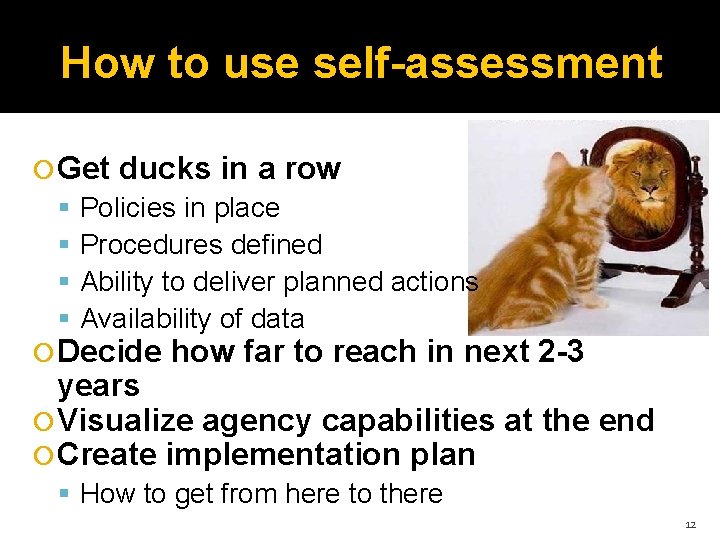
How to use self-assessment Get ducks in a row Policies in place Procedures defined Ability to deliver planned actions Availability of data Decide how far to reach in next 2 -3 years Visualize agency capabilities at the end Create implementation plan How to get from here to there 12
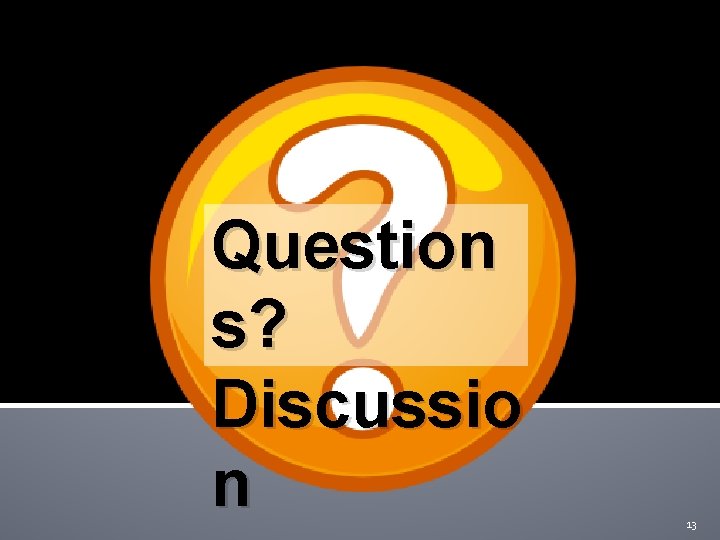
Question s? Discussio n 13
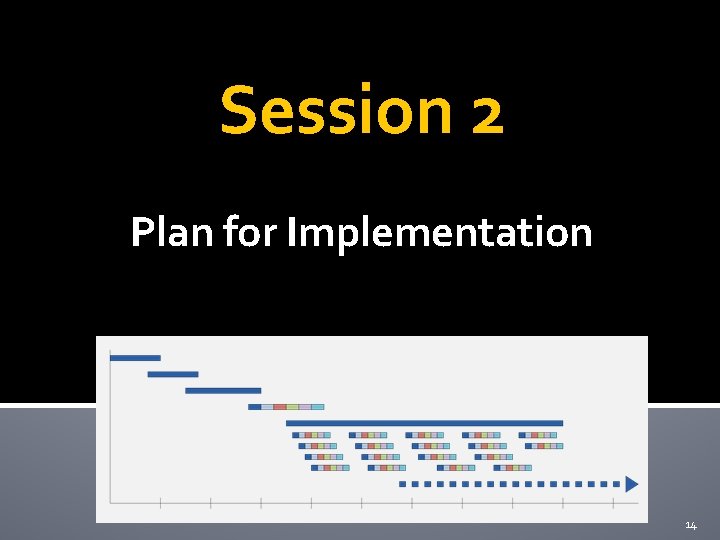
Session 2 Plan for Implementation 14
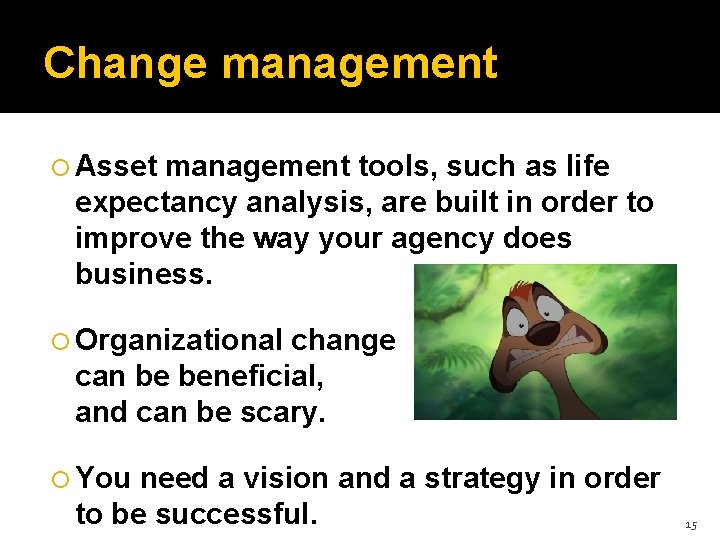
Change management Asset management tools, such as life expectancy analysis, are built in order to improve the way your agency does business. Organizational change can be beneficial, and can be scary. You need a vision and a strategy in order to be successful. 15
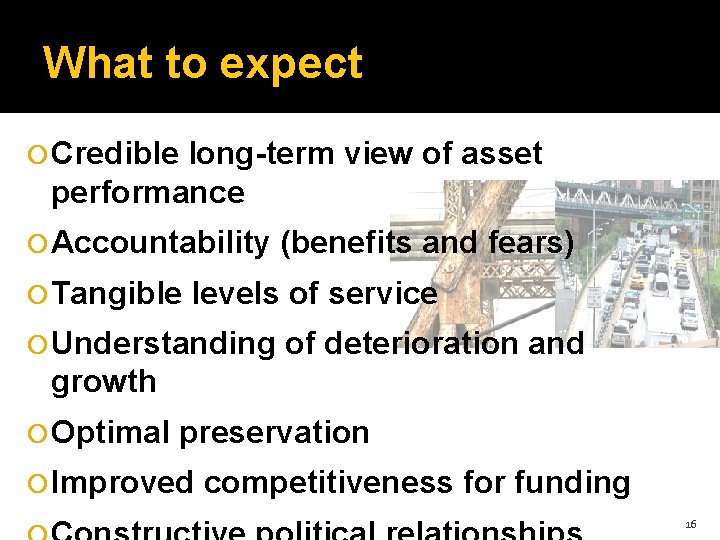
What to expect Credible long-term view of asset performance Accountability Tangible (benefits and fears) levels of service Understanding of deterioration and growth Optimal preservation Improved competitiveness for funding 16
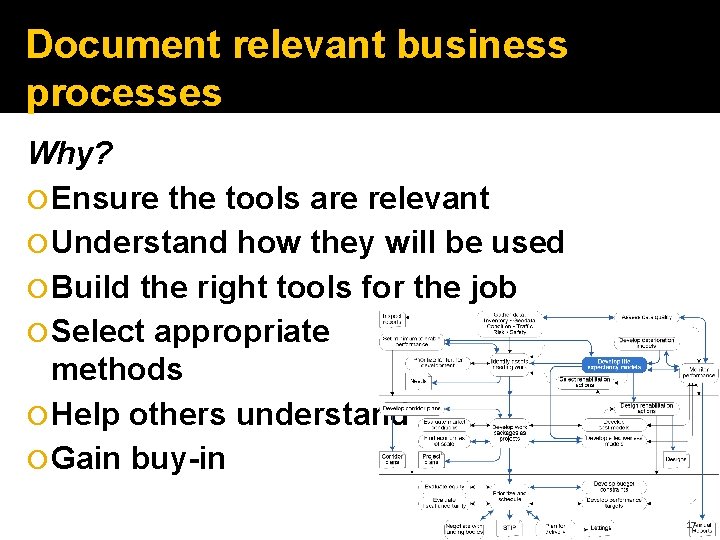
Document relevant business processes Why? Ensure the tools are relevant Understand how they will be used Build the right tools for the job Select appropriate methods Help others understand Gain buy-in 17
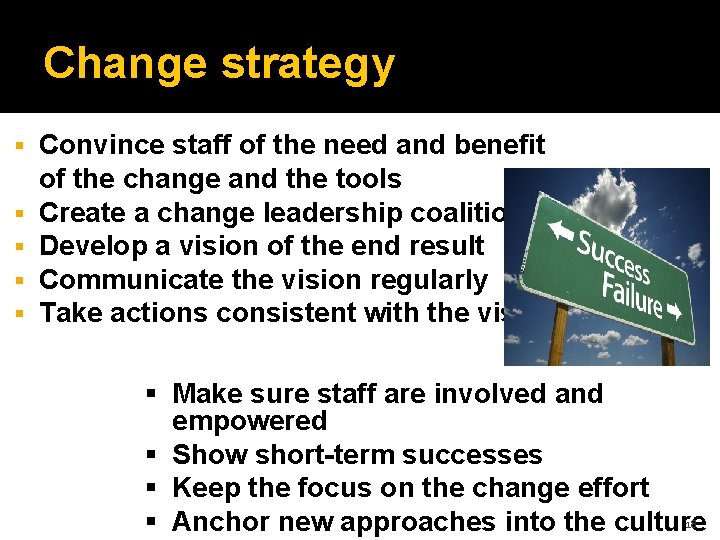
Change strategy Convince staff of the need and benefit of the change and the tools Create a change leadership coalition Develop a vision of the end result Communicate the vision regularly Take actions consistent with the vision Make sure staff are involved and empowered Show short-term successes Keep the focus on the change effort 18 Anchor new approaches into the culture
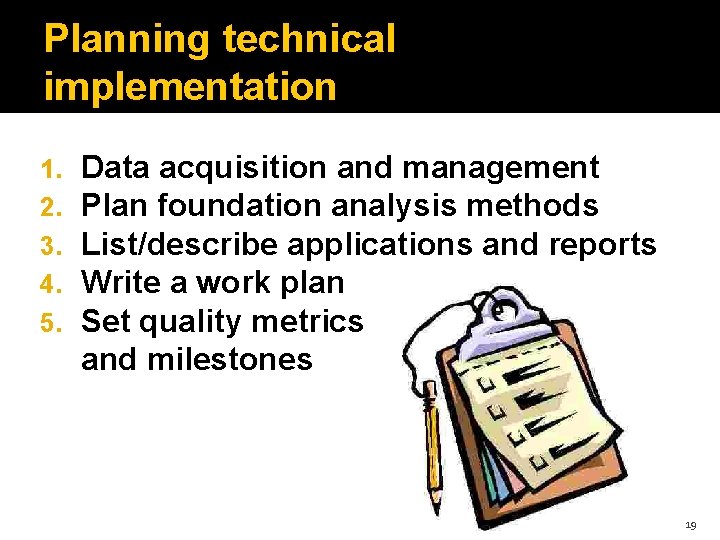
Planning technical implementation 1. 2. 3. 4. 5. Data acquisition and management Plan foundation analysis methods List/describe applications and reports Write a work plan Set quality metrics and milestones 19
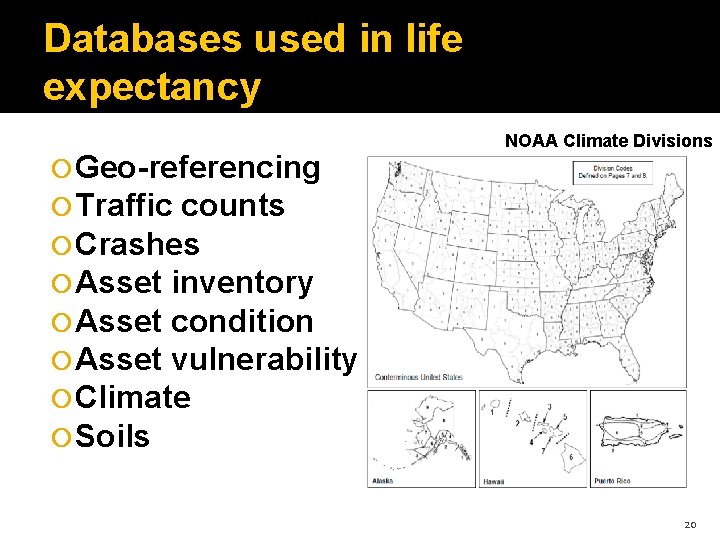
Databases used in life expectancy Geo-referencing Traffic counts Crashes Asset inventory Asset condition Asset vulnerability Climate Soils NOAA Climate Divisions 20
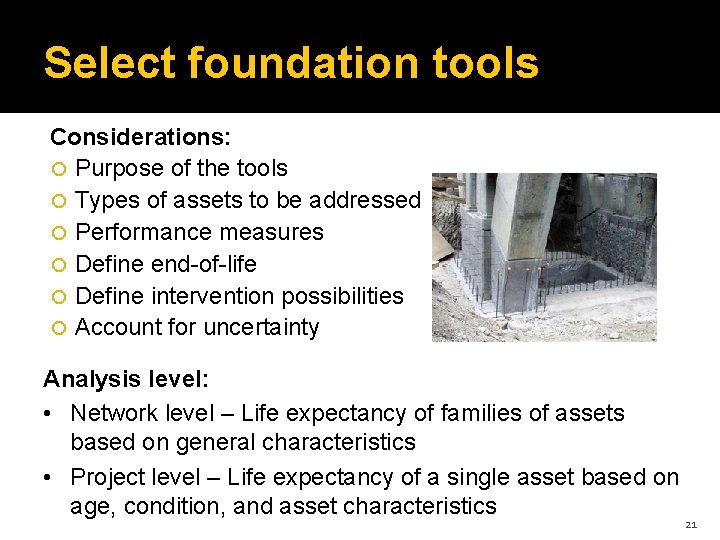
Select foundation tools Considerations: Purpose of the tools Types of assets to be addressed Performance measures Define end-of-life Define intervention possibilities Account for uncertainty Analysis level: • Network level – Life expectancy of families of assets based on general characteristics • Project level – Life expectancy of a single asset based on age, condition, and asset characteristics 21
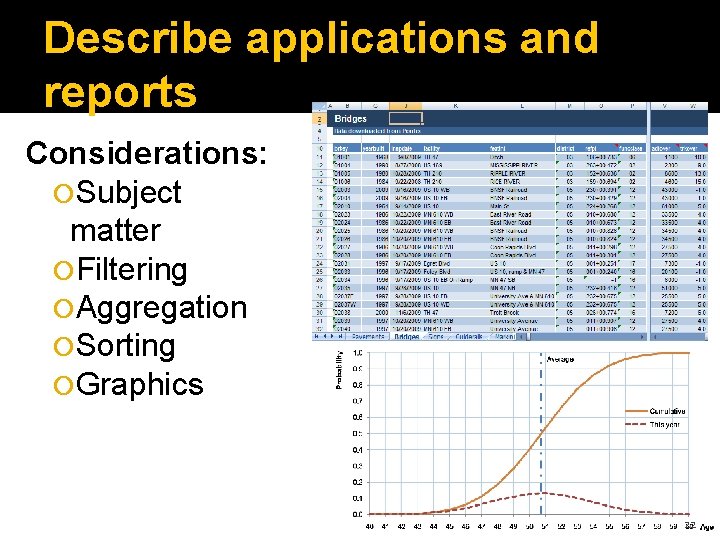
Describe applications and reports Considerations: Subject matter Filtering Aggregation Sorting Graphics 22
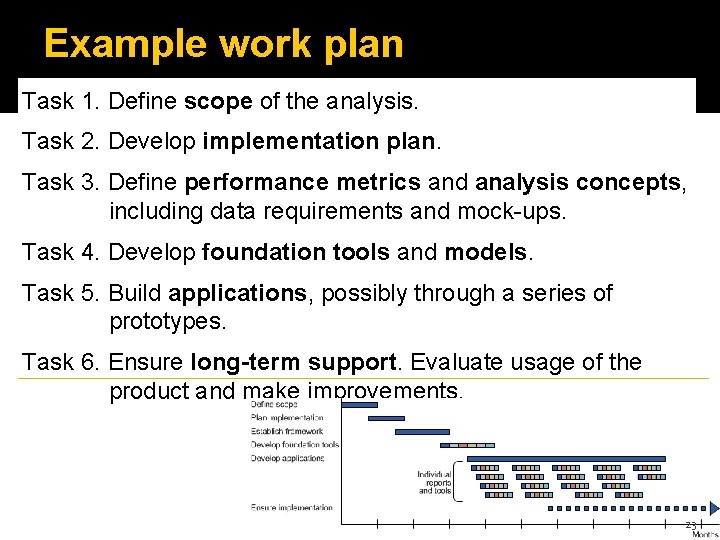
Example work plan Task 1. Define scope of the analysis. Task 2. Develop implementation plan. Task 3. Define performance metrics and analysis concepts, including data requirements and mock-ups. Task 4. Develop foundation tools and models. Task 5. Build applications, possibly through a series of prototypes. Task 6. Ensure long-term support. Evaluate usage of the product and make improvements. 23
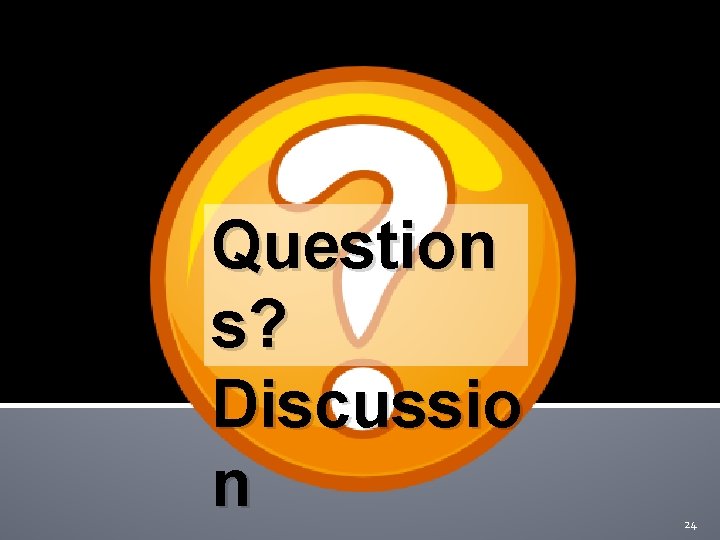
Question s? Discussio n 24
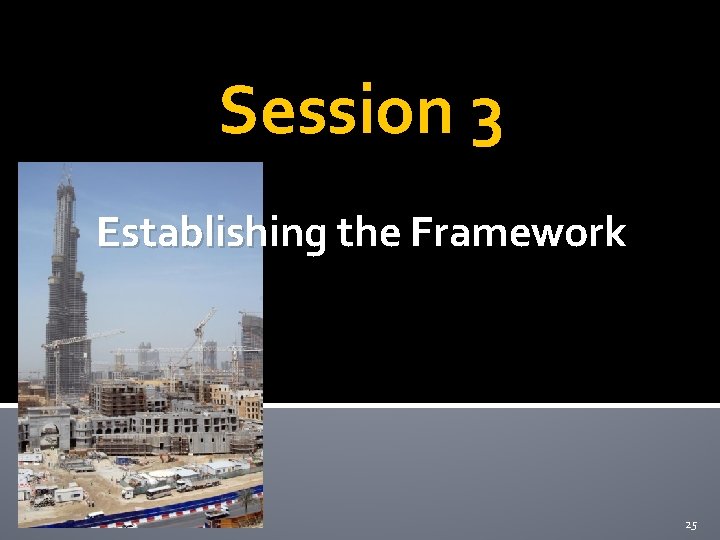
Session 3 Establishing the Framework 25
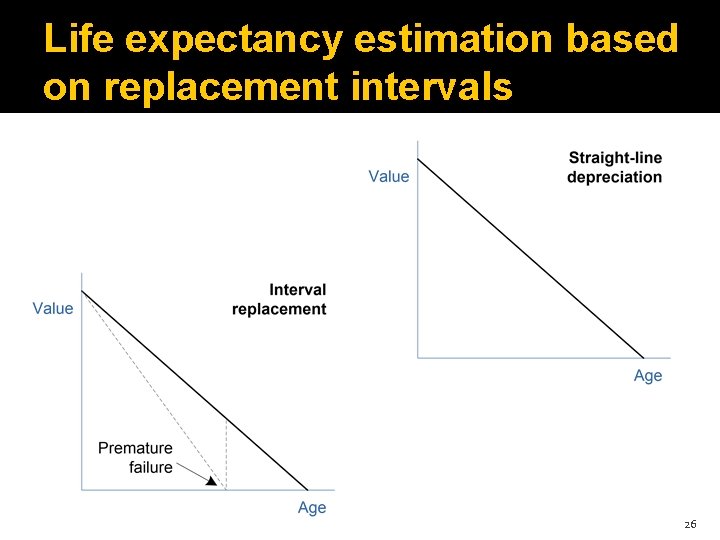
Life expectancy estimation based on replacement intervals 26
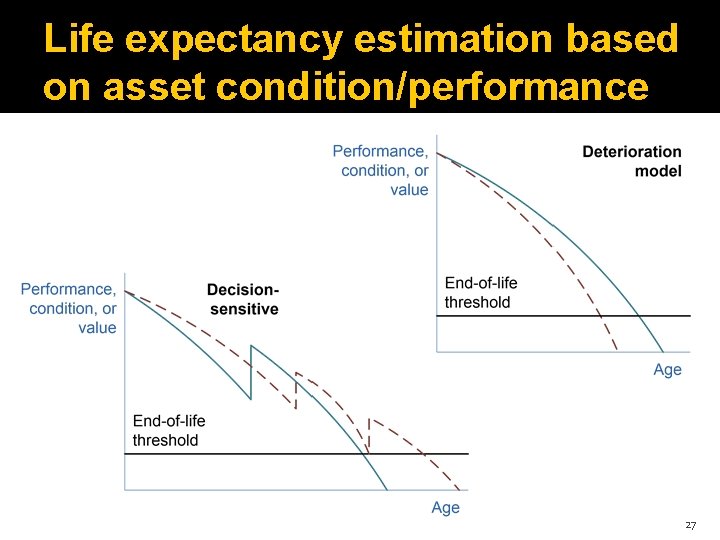
Life expectancy estimation based on asset condition/performance 27
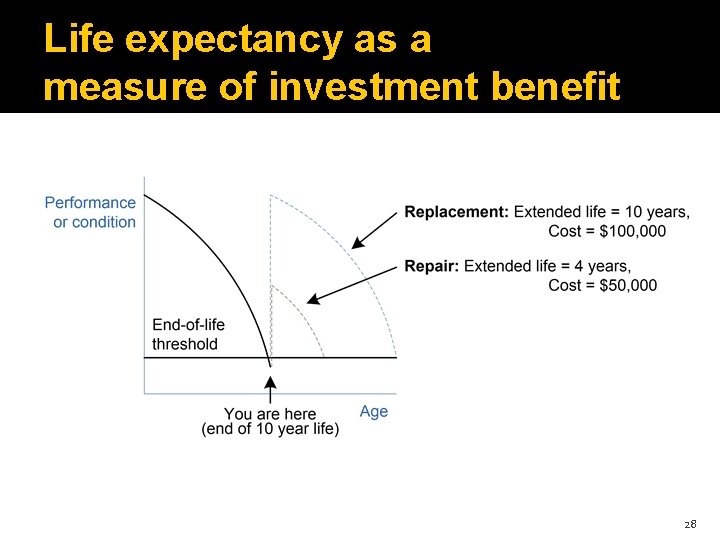
Life expectancy as a measure of investment benefit 28
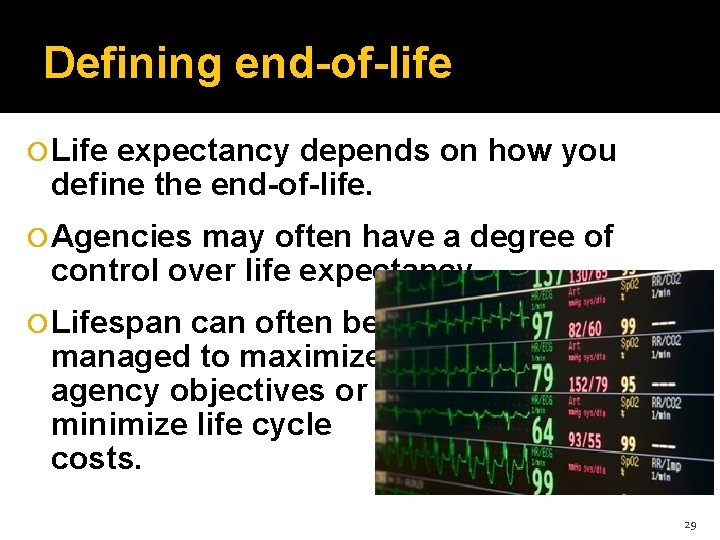
Defining end-of-life Life expectancy depends on how you define the end-of-life. Agencies may often have a degree of control over life expectancy. Lifespan can often be managed to maximize agency objectives or minimize life cycle costs. 29
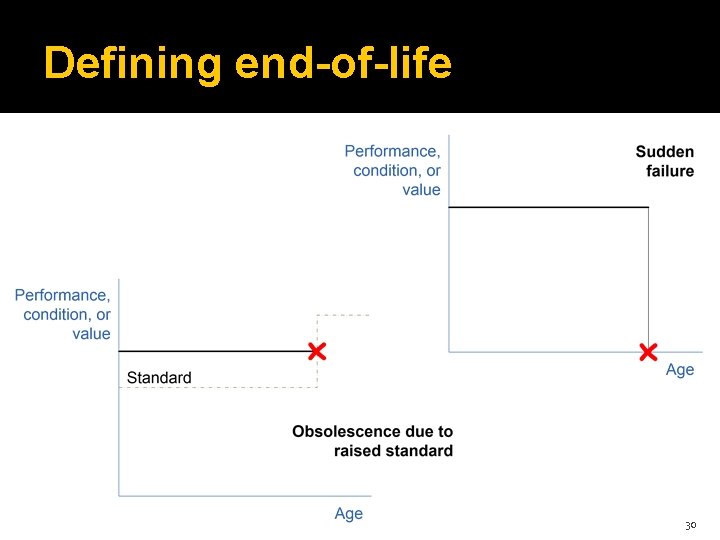
Defining end-of-life 30
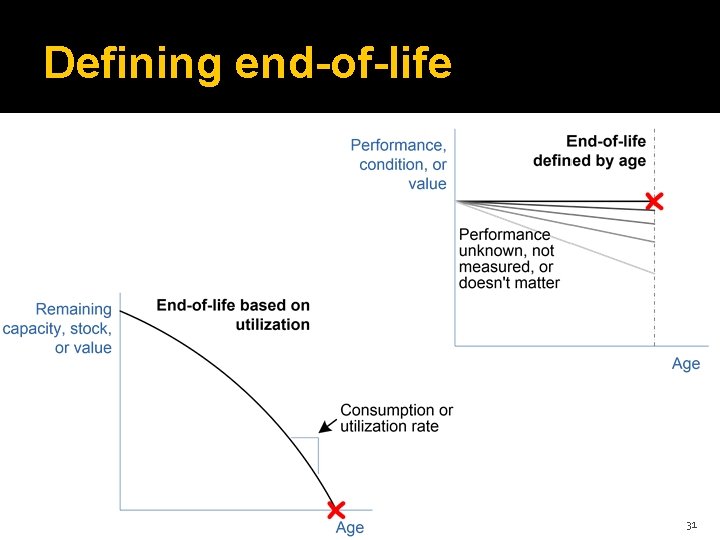
Defining end-of-life 31
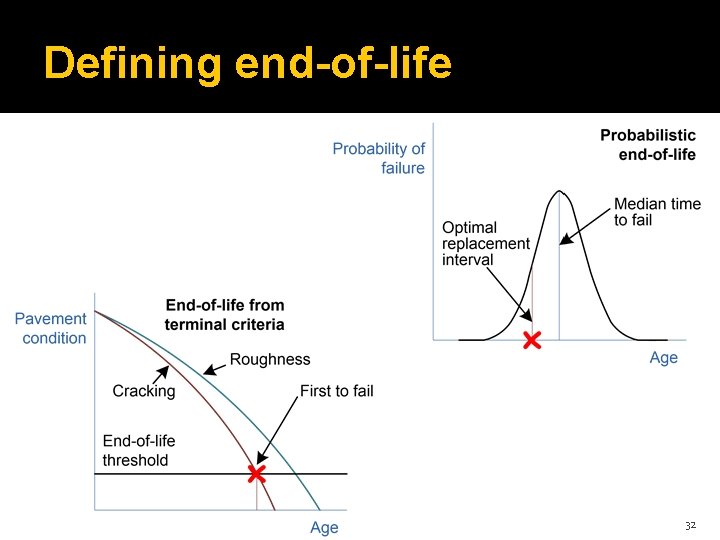
Defining end-of-life 32
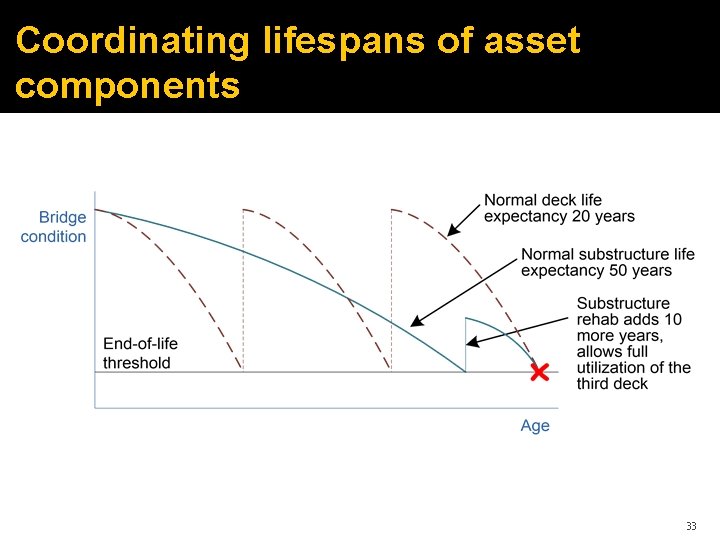
Coordinating lifespans of asset components 33
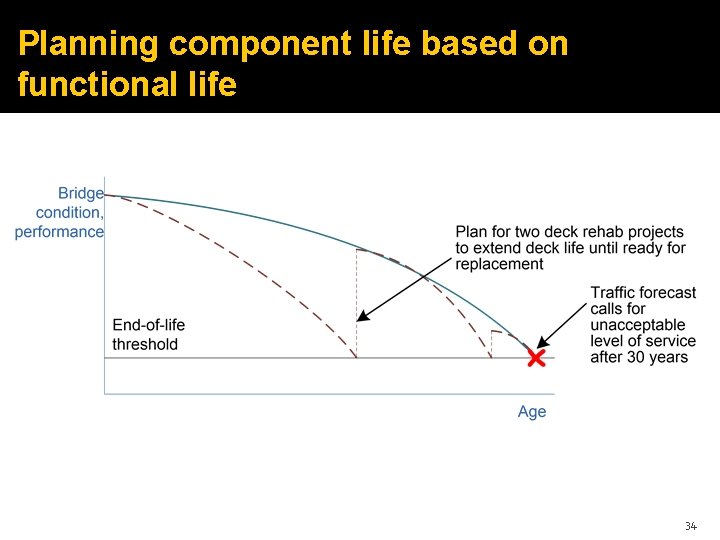
Planning component life based on functional life 34
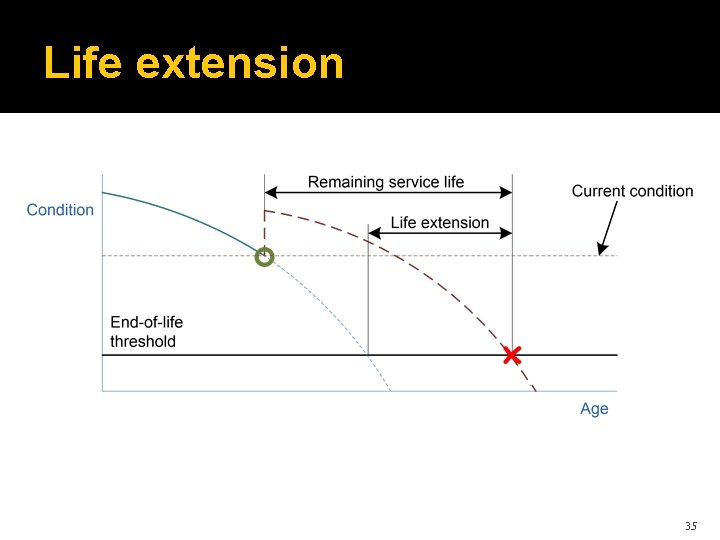
Life extension 35
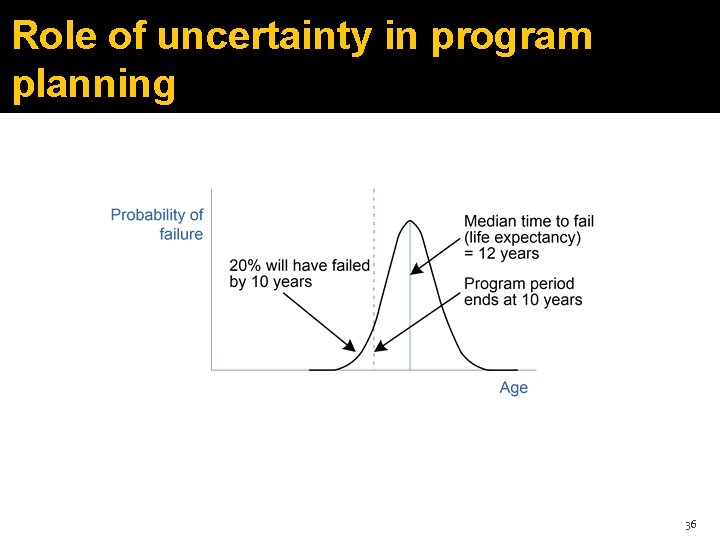
Role of uncertainty in program planning 36
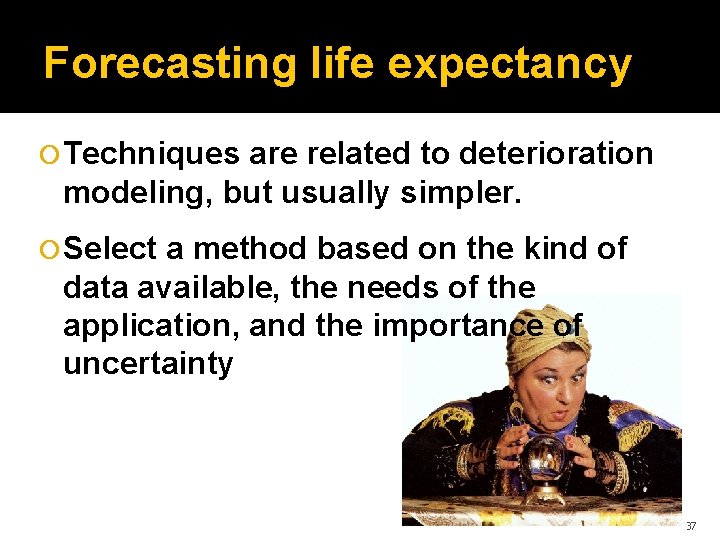
Forecasting life expectancy Techniques are related to deterioration modeling, but usually simpler. Select a method based on the kind of data available, the needs of the application, and the importance of uncertainty 37
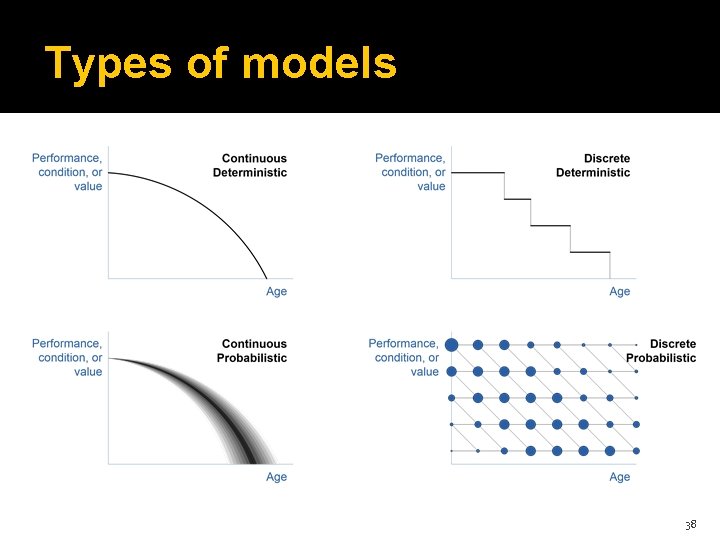
Types of models 38
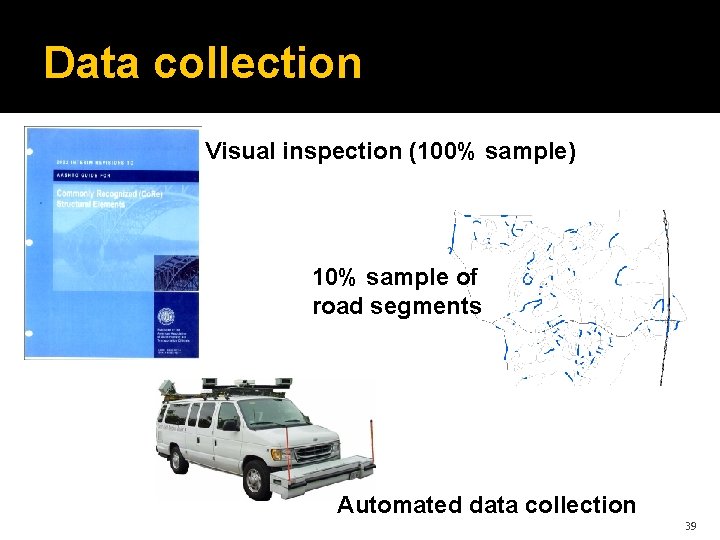
Data collection Visual inspection (100% sample) 10% sample of road segments Automated data collection 39
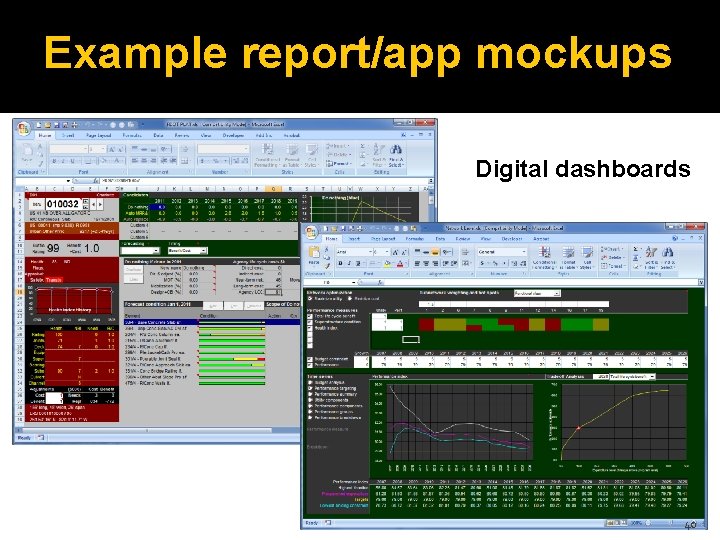
Example report/app mockups Digital dashboards 40
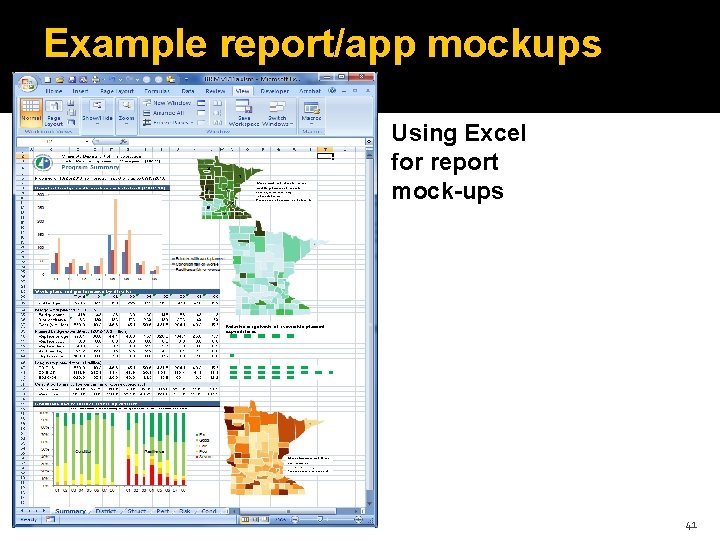
Example report/app mockups Using Excel for report mock-ups 41
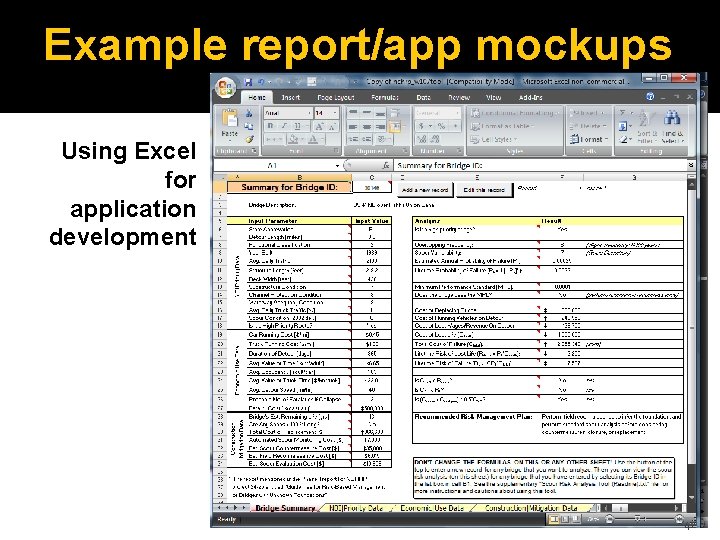
Example report/app mockups Using Excel for application development 42
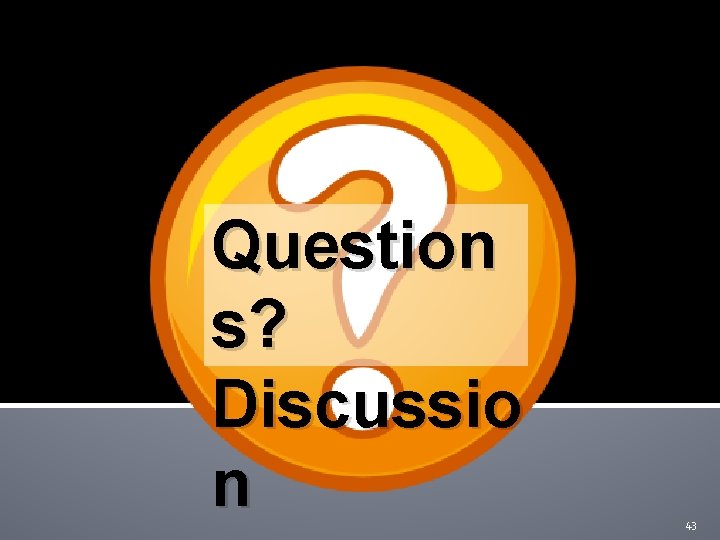
Question s? Discussio n 43
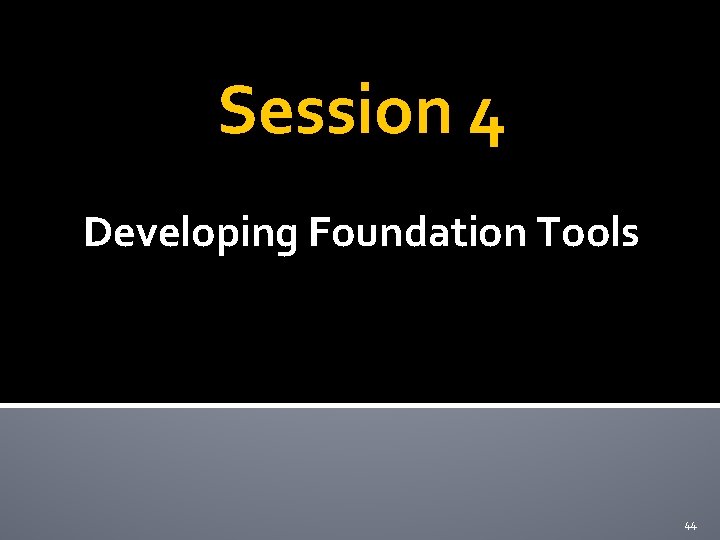
Session 4 Developing Foundation Tools 44
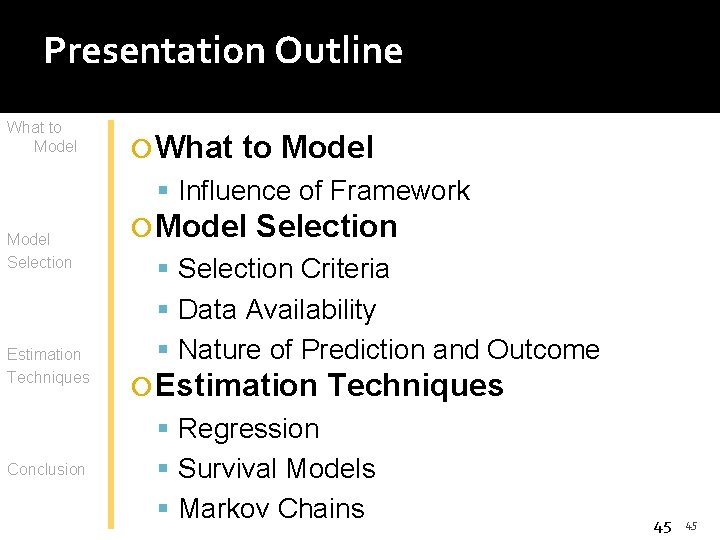
Presentation Outline What to Model Influence of Framework Model Selection Criteria Data Availability Estimation Techniques Nature of Prediction and Outcome Estimation Techniques Regression Conclusion Survival Models Markov Chains 45 45
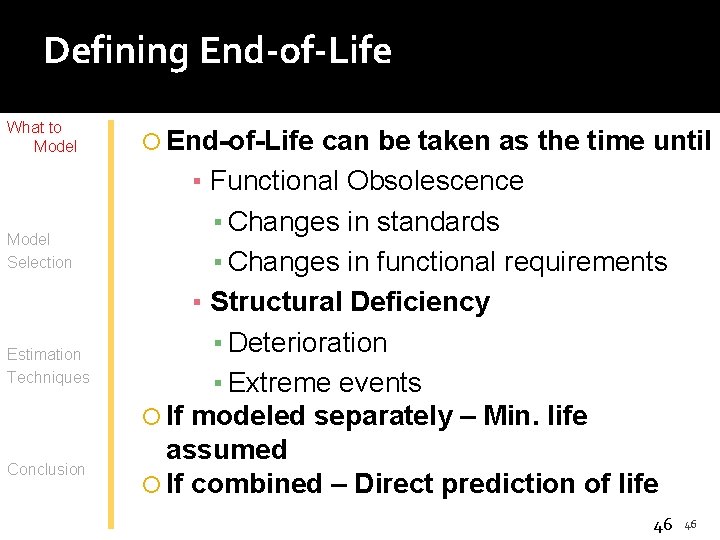
Defining End-of-Life What to Model Selection Estimation Techniques Conclusion End-of-Life can be taken as the time until ▪ Functional Obsolescence ▪ Changes in standards ▪ Changes in functional requirements ▪ Structural Deficiency ▪ Deterioration ▪ Extreme events If modeled separately – Min. life assumed If combined – Direct prediction of life 46 46
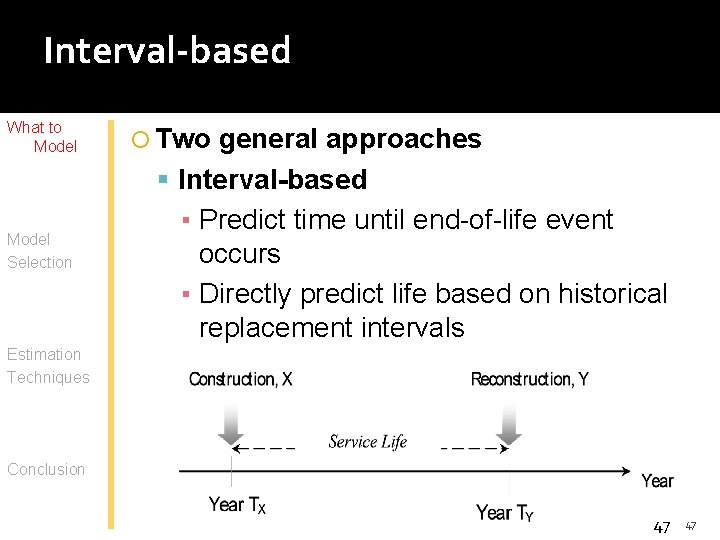
Interval-based What to Model Selection Two general approaches Interval-based ▪ Predict time until end-of-life event occurs ▪ Directly predict life based on historical replacement intervals Estimation Techniques Conclusion 47 47
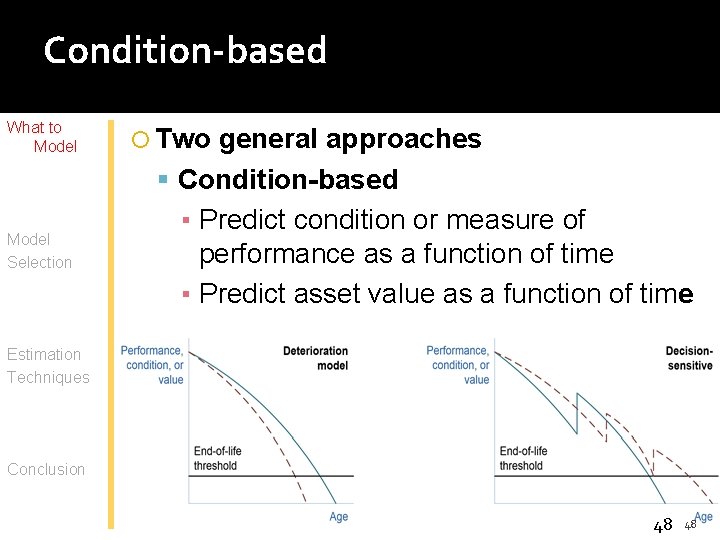
Condition-based What to Model Selection Two general approaches Condition-based ▪ Predict condition or measure of performance as a function of time ▪ Predict asset value as a function of time Estimation Techniques Conclusion 48 48
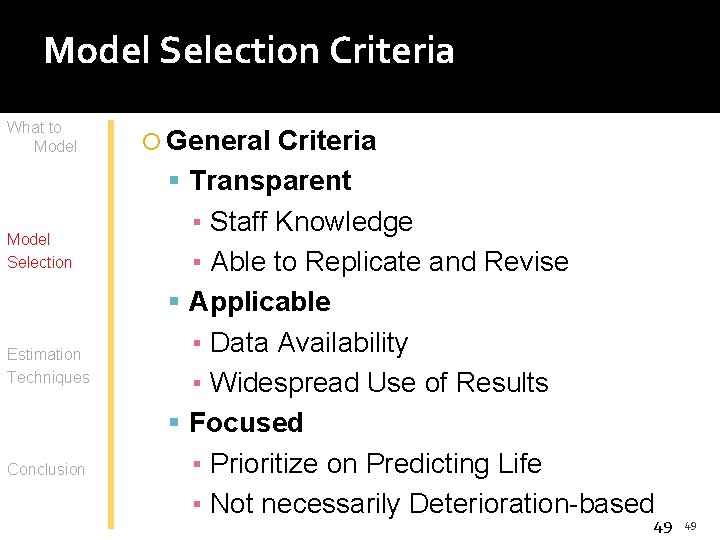
Model Selection Criteria What to Model Selection Estimation Techniques Conclusion General Criteria Transparent ▪ Staff Knowledge ▪ Able to Replicate and Revise Applicable ▪ Data Availability ▪ Widespread Use of Results Focused ▪ Prioritize on Predicting Life ▪ Not necessarily Deterioration-based 49 49
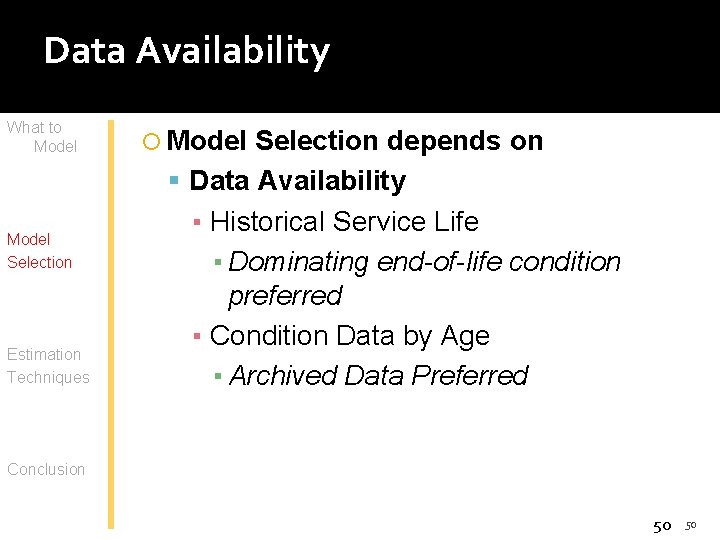
Data Availability What to Model Selection Estimation Techniques Model Selection depends on Data Availability ▪ Historical Service Life ▪ Dominating end-of-life condition preferred ▪ Condition Data by Age ▪ Archived Data Preferred Conclusion 50 50
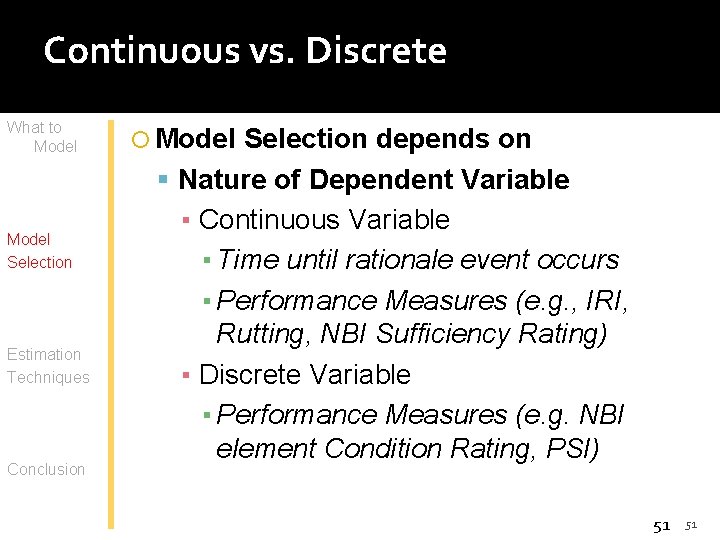
Continuous vs. Discrete What to Model Selection Estimation Techniques Conclusion Model Selection depends on Nature of Dependent Variable ▪ Continuous Variable ▪ Time until rationale event occurs ▪ Performance Measures (e. g. , IRI, Rutting, NBI Sufficiency Rating) ▪ Discrete Variable ▪ Performance Measures (e. g. NBI element Condition Rating, PSI) 51 51
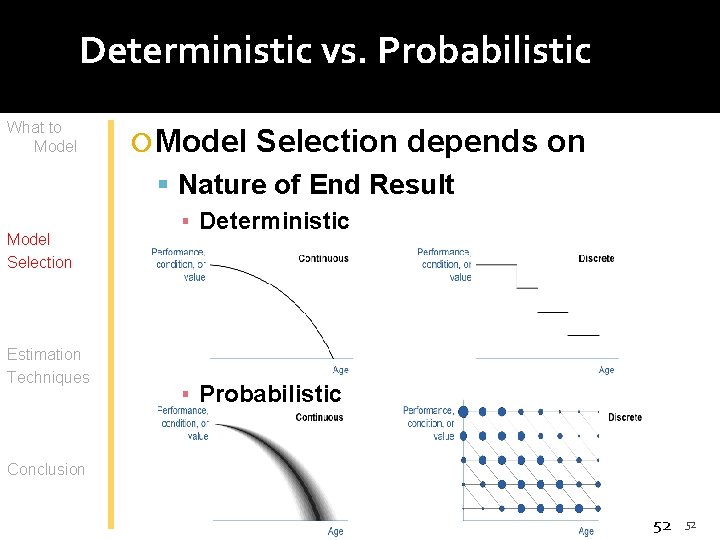
Deterministic vs. Probabilistic What to Model Selection Estimation Techniques Model Selection depends on Nature of End Result ▪ Deterministic ▪ Probabilistic Conclusion 52 52
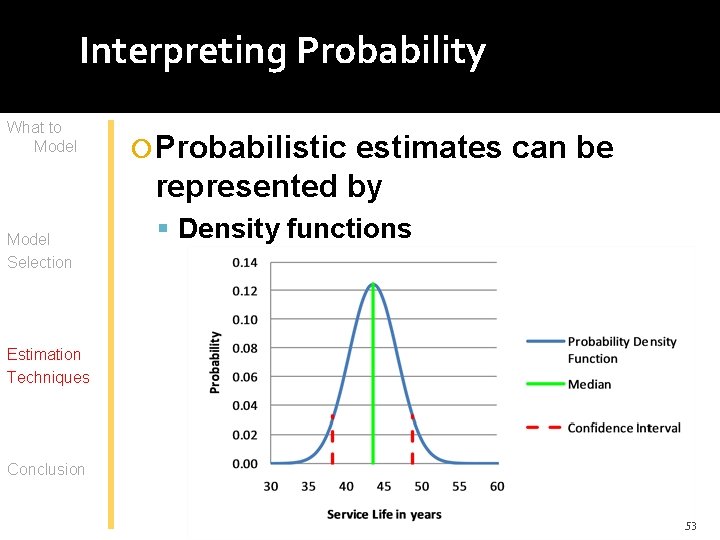
Interpreting Probability What to Model Selection Probabilistic estimates can be represented by Density functions Estimation Techniques Conclusion 53 53
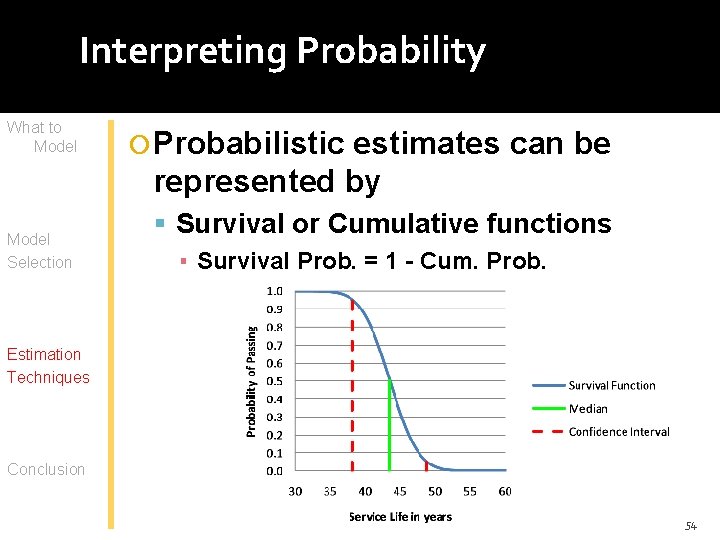
Interpreting Probability What to Model Selection Probabilistic estimates can be represented by Survival or Cumulative functions ▪ Survival Prob. = 1 - Cum. Prob. Estimation Techniques Conclusion 54 54
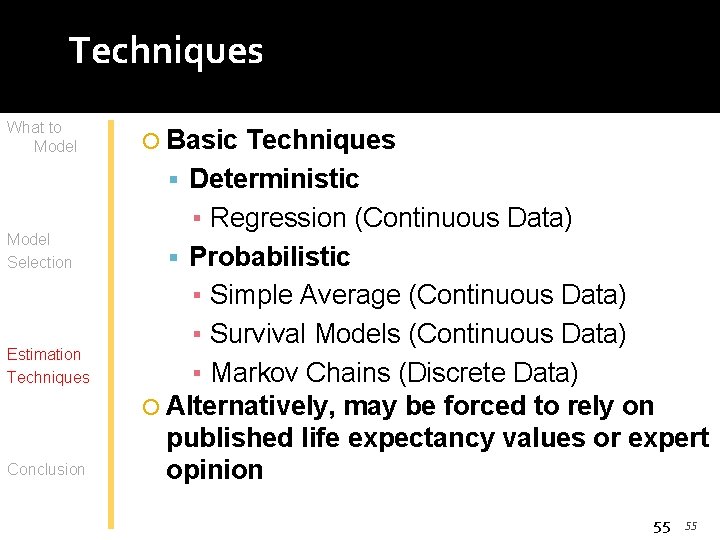
Techniques What to Model Selection Estimation Techniques Conclusion Basic Techniques Deterministic ▪ Regression (Continuous Data) Probabilistic ▪ Simple Average (Continuous Data) ▪ Survival Models (Continuous Data) ▪ Markov Chains (Discrete Data) Alternatively, may be forced to rely on published life expectancy values or expert opinion 55 55
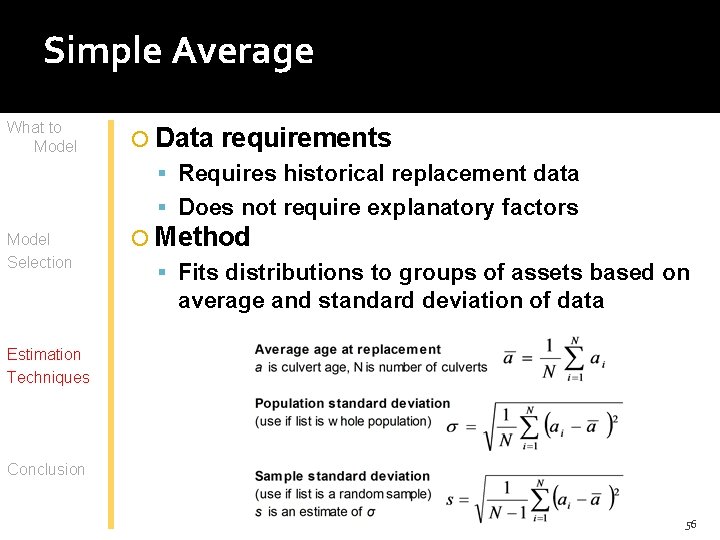
Simple Average What to Model Data requirements Requires historical replacement data Does not require explanatory factors Model Selection Method Fits distributions to groups of assets based on average and standard deviation of data Estimation Techniques Conclusion 56 56
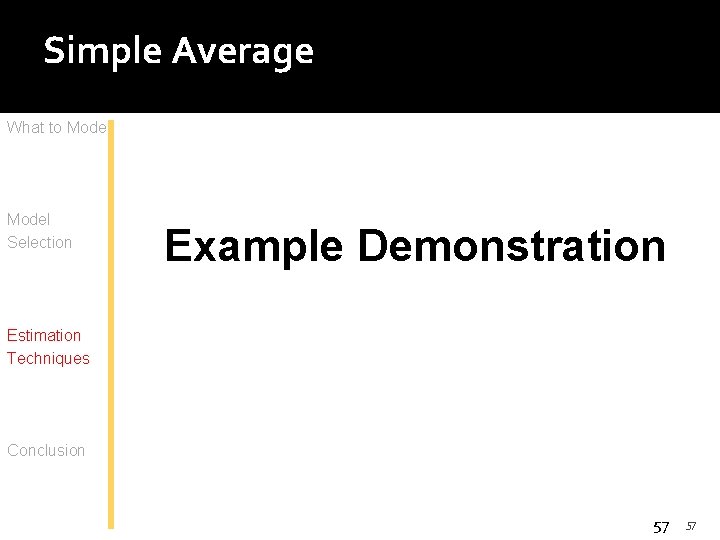
Simple Average What to Model Selection Example Demonstration Estimation Techniques Conclusion 57 57
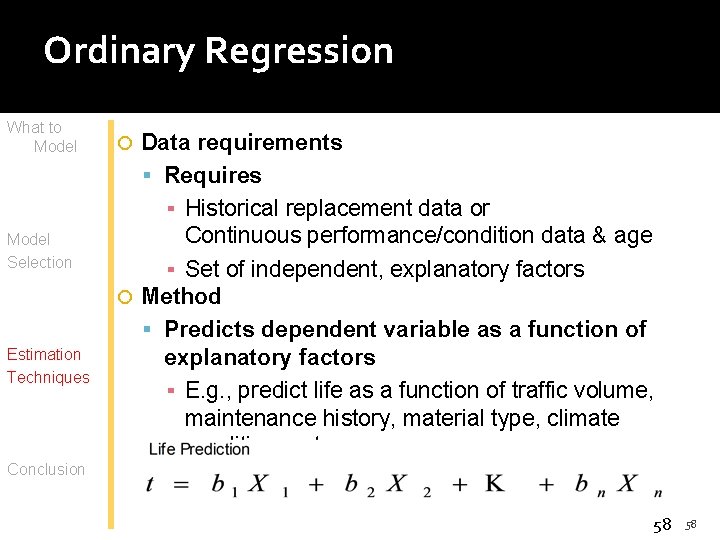
Ordinary Regression What to Model Selection Estimation Techniques Data requirements Requires ▪ Historical replacement data or Continuous performance/condition data & age ▪ Set of independent, explanatory factors Method Predicts dependent variable as a function of explanatory factors ▪ E. g. , predict life as a function of traffic volume, maintenance history, material type, climate conditions, etc. Conclusion 58 58
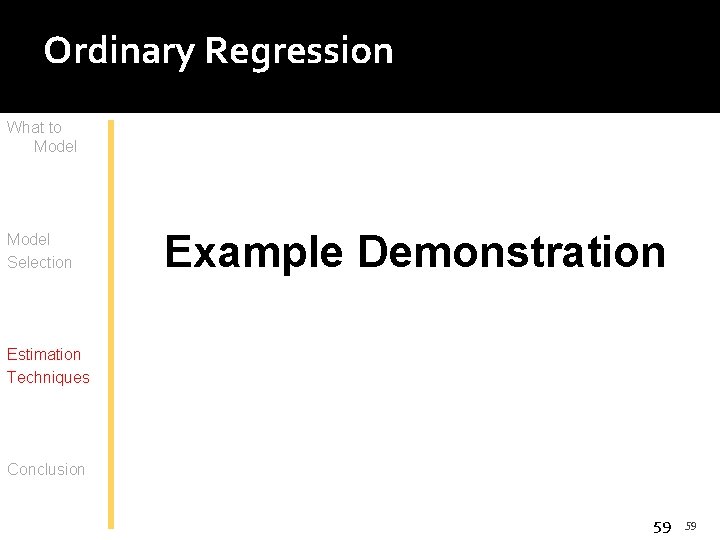
Ordinary Regression What to Model Selection Example Demonstration Estimation Techniques Conclusion 59 59
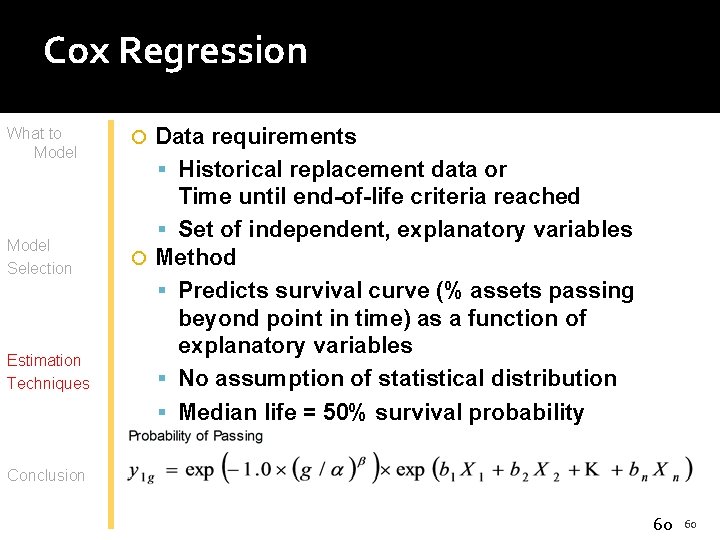
Cox Regression What to Model Selection Estimation Techniques Data requirements Historical replacement data or Time until end-of-life criteria reached Set of independent, explanatory variables Method Predicts survival curve (% assets passing beyond point in time) as a function of explanatory variables No assumption of statistical distribution Median life = 50% survival probability Conclusion 60 60
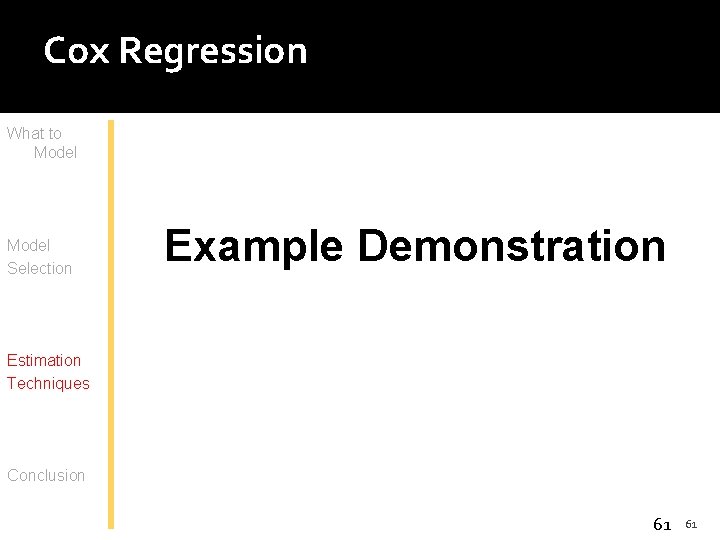
Cox Regression What to Model Selection Example Demonstration Estimation Techniques Conclusion 61 61
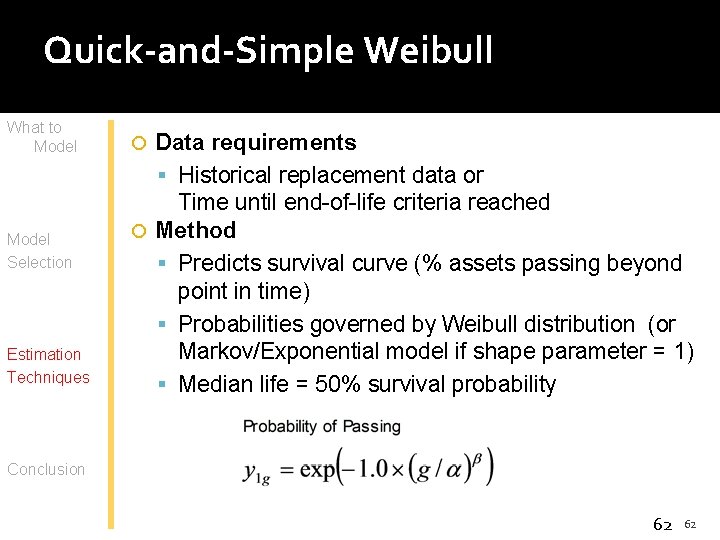
Quick-and-Simple Weibull What to Model Selection Estimation Techniques Data requirements Historical replacement data or Time until end-of-life criteria reached Method Predicts survival curve (% assets passing beyond point in time) Probabilities governed by Weibull distribution (or Markov/Exponential model if shape parameter = 1) Median life = 50% survival probability Conclusion 62 62
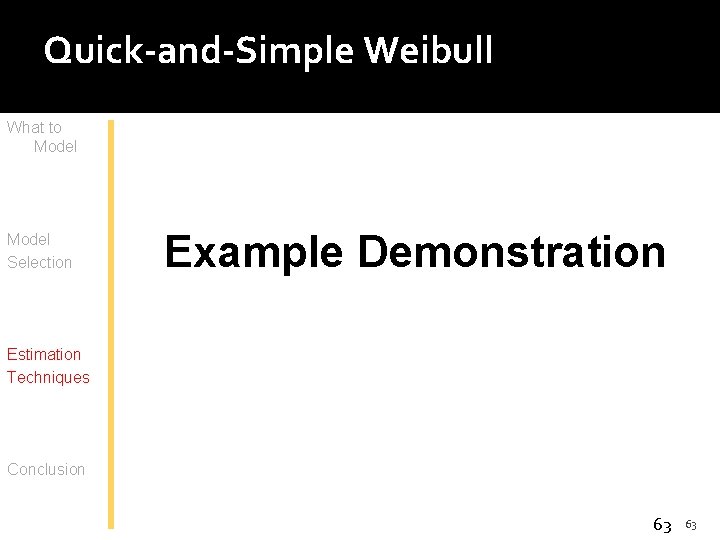
Quick-and-Simple Weibull What to Model Selection Example Demonstration Estimation Techniques Conclusion 63 63
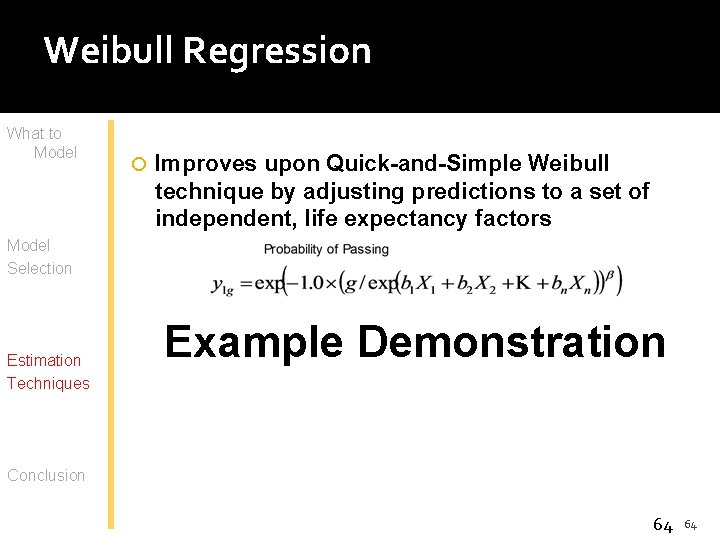
Weibull Regression What to Model Improves upon Quick-and-Simple Weibull technique by adjusting predictions to a set of independent, life expectancy factors Model Selection Estimation Techniques Example Demonstration Conclusion 64 64
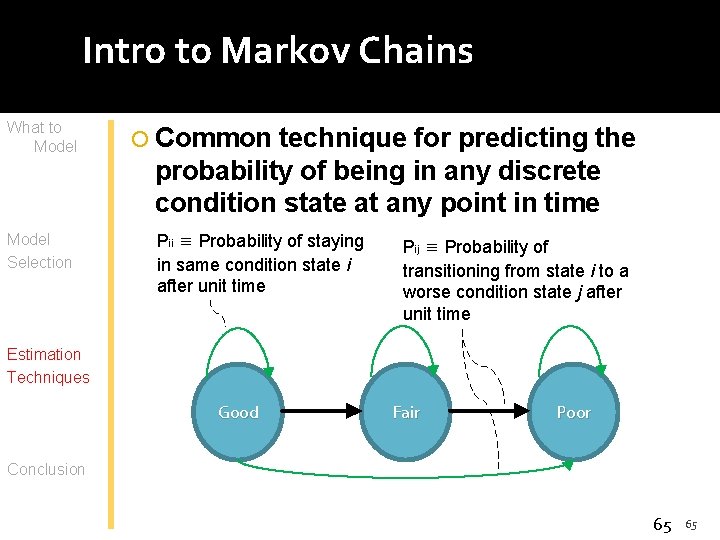
Intro to Markov Chains What to Model Selection Common technique for predicting the probability of being in any discrete condition state at any point in time Pii ≡ Probability of staying in same condition state i after unit time Pij ≡ Probability of transitioning from state i to a worse condition state j after unit time Estimation Techniques Good Fair Poor Conclusion 65 65
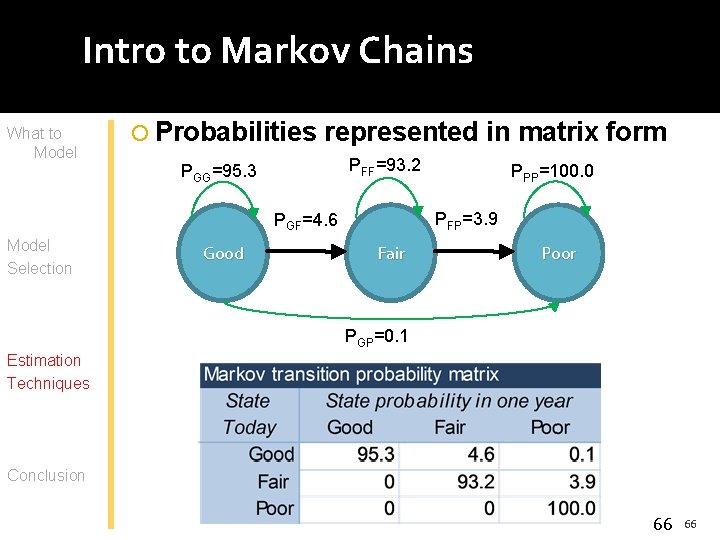
Intro to Markov Chains What to Model Probabilities represented in matrix form PFF=93. 2 PGG=95. 3 PFP=3. 9 PGF=4. 6 Model Selection Good PPP=100. 0 Fair Poor PGP=0. 1 Estimation Techniques Conclusion 66 66
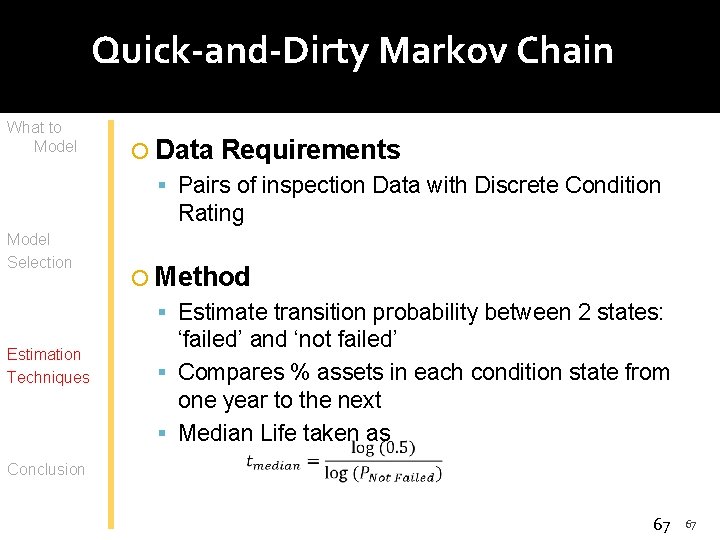
Quick-and-Dirty Markov Chain What to Model Data Requirements Pairs of inspection Data with Discrete Condition Rating Model Selection Method Estimate transition probability between 2 states: Estimation Techniques ‘failed’ and ‘not failed’ Compares % assets in each condition state from one year to the next Median Life taken as Conclusion 67 67
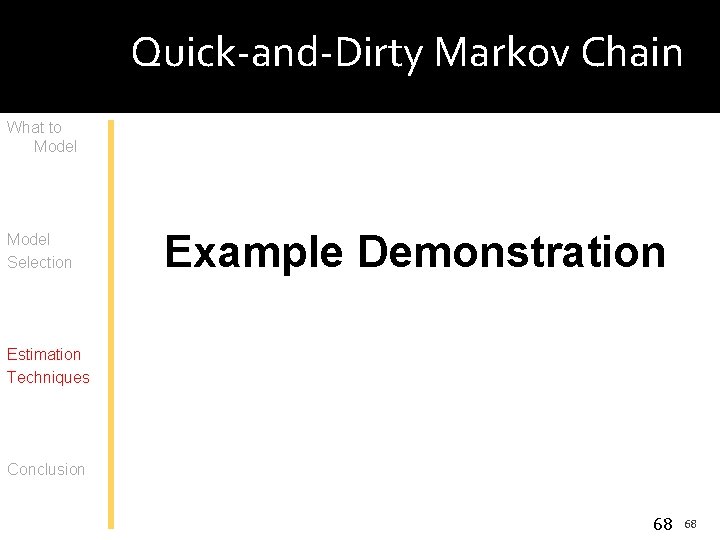
Quick-and-Dirty Markov Chain What to Model Selection Example Demonstration Estimation Techniques Conclusion 68 68
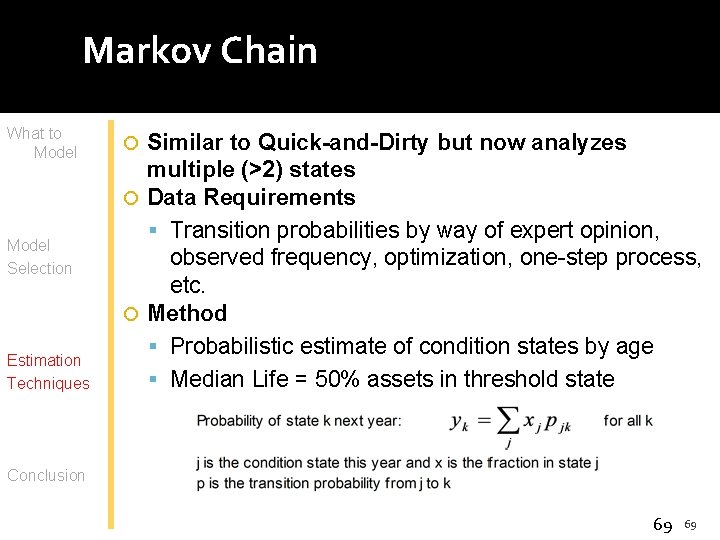
Markov Chain What to Model Selection Estimation Techniques Similar to Quick-and-Dirty but now analyzes multiple (>2) states Data Requirements Transition probabilities by way of expert opinion, observed frequency, optimization, one-step process, etc. Method Probabilistic estimate of condition states by age Median Life = 50% assets in threshold state Conclusion 69 69
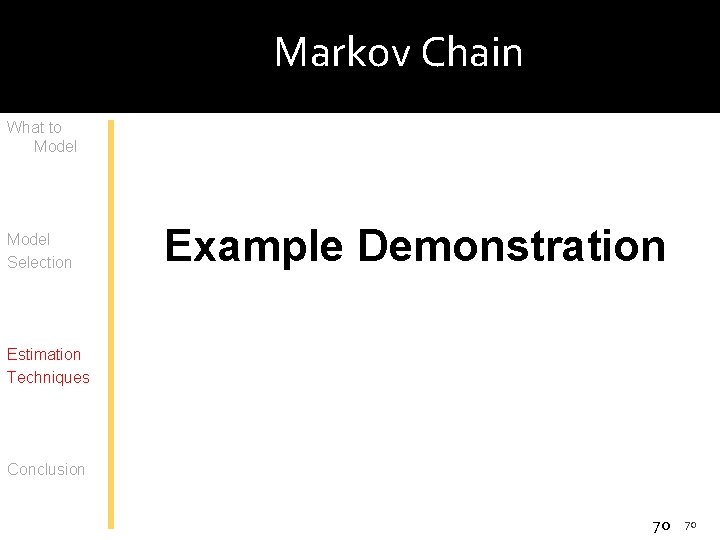
Markov Chain What to Model Selection Example Demonstration Estimation Techniques Conclusion 70 70
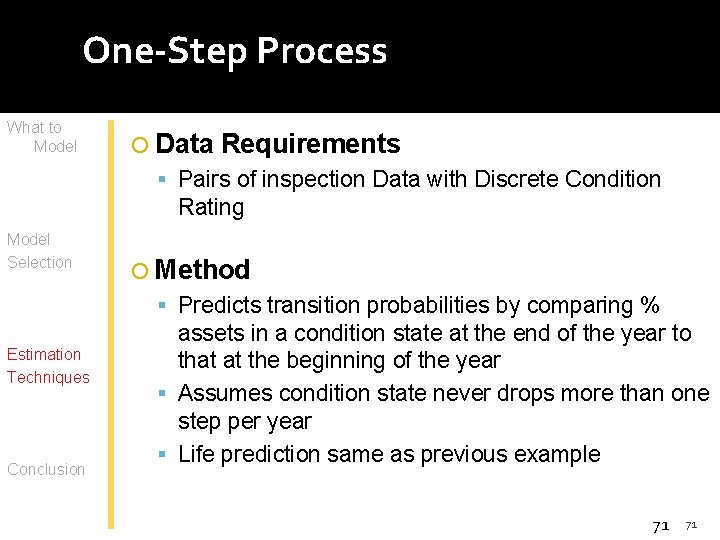
One-Step Process What to Model Data Requirements Pairs of inspection Data with Discrete Condition Rating Model Selection Method Predicts transition probabilities by comparing % Estimation Techniques Conclusion assets in a condition state at the end of the year to that at the beginning of the year Assumes condition state never drops more than one step per year Life prediction same as previous example 71 71
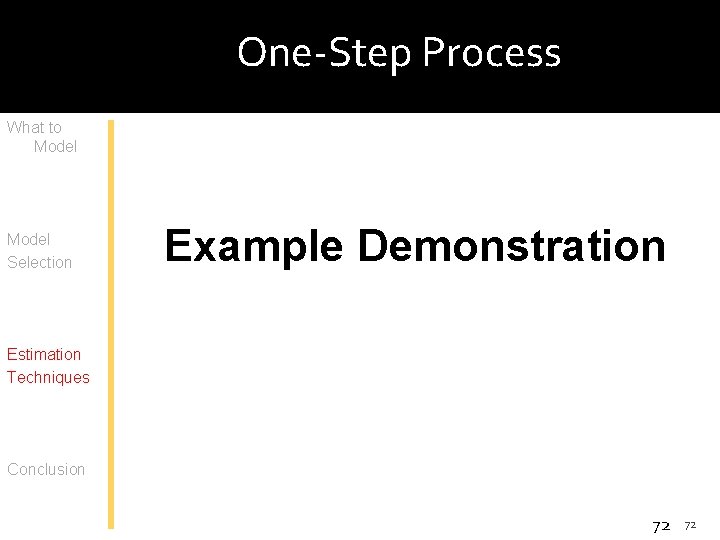
One-Step Process What to Model Selection Example Demonstration Estimation Techniques Conclusion 72 72
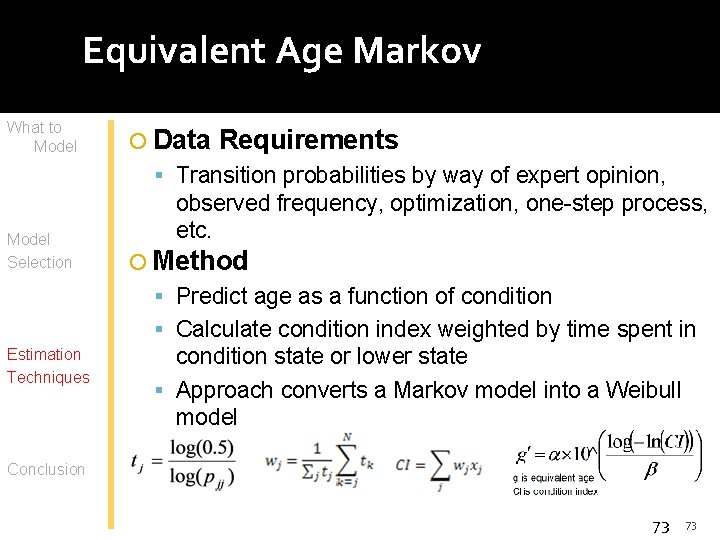
Equivalent Age Markov What to Model Data Requirements Transition probabilities by way of expert opinion, Model Selection observed frequency, optimization, one-step process, etc. Method Predict age as a function of condition Calculate condition index weighted by time spent in Estimation Techniques condition state or lower state Approach converts a Markov model into a Weibull model Conclusion 73 73
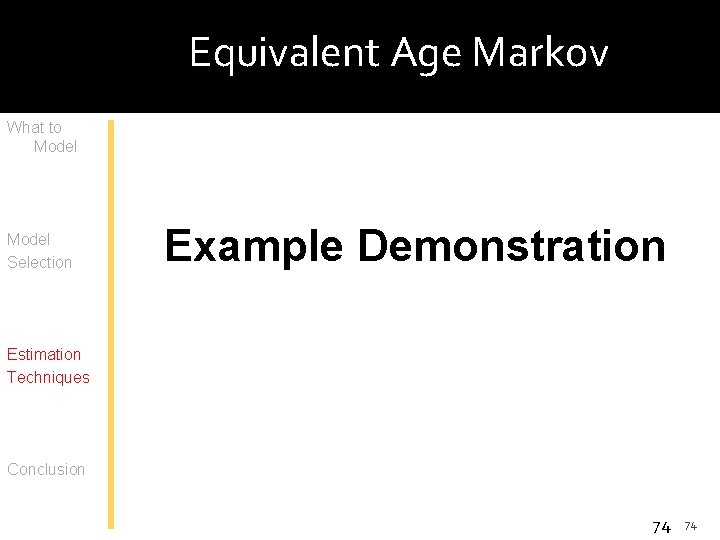
Equivalent Age Markov What to Model Selection Example Demonstration Estimation Techniques Conclusion 74 74
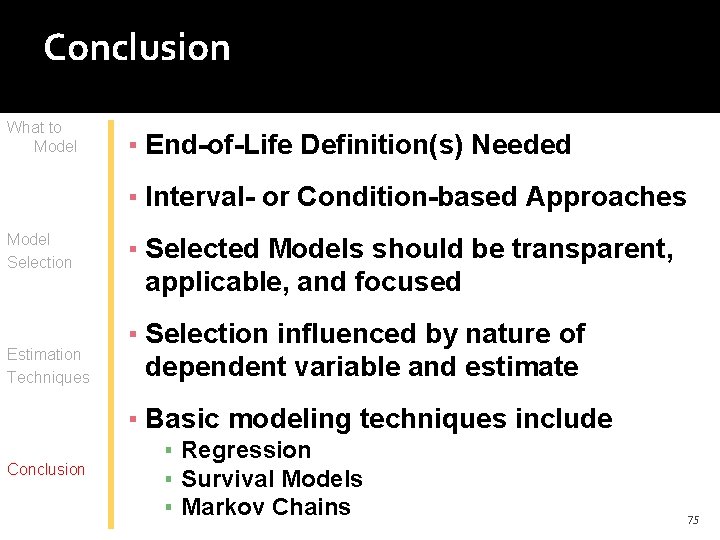
Conclusion What to Model ▪ End-of-Life Definition(s) Needed ▪ Interval- or Condition-based Approaches Model Selection Estimation Techniques ▪ Selected Models should be transparent, applicable, and focused ▪ Selection influenced by nature of dependent variable and estimate ▪ Basic modeling techniques include Conclusion ▪ Regression ▪ Survival Models ▪ Markov Chains 75
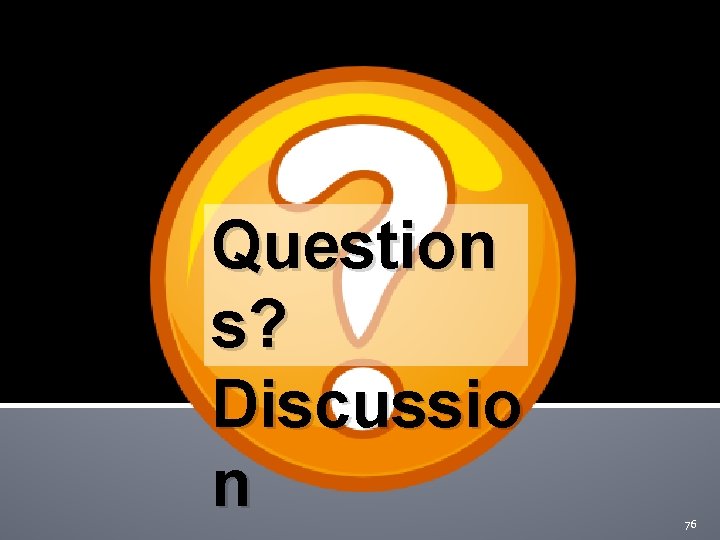
Question s? Discussio n 76
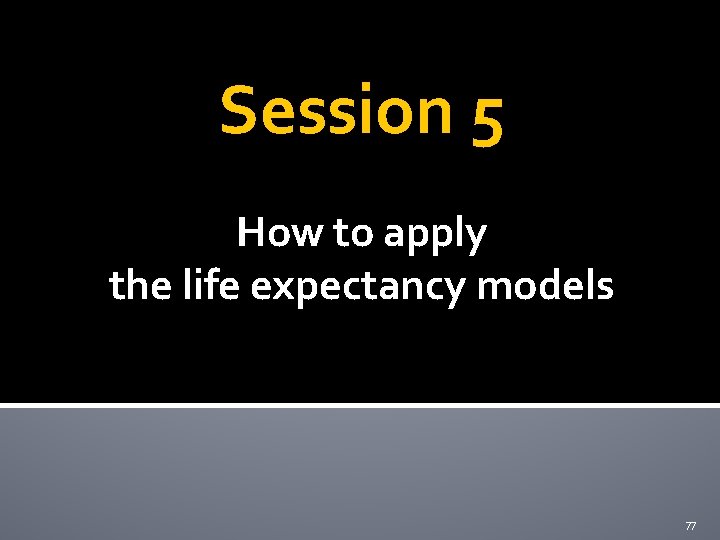
Session 5 How to apply the life expectancy models 77
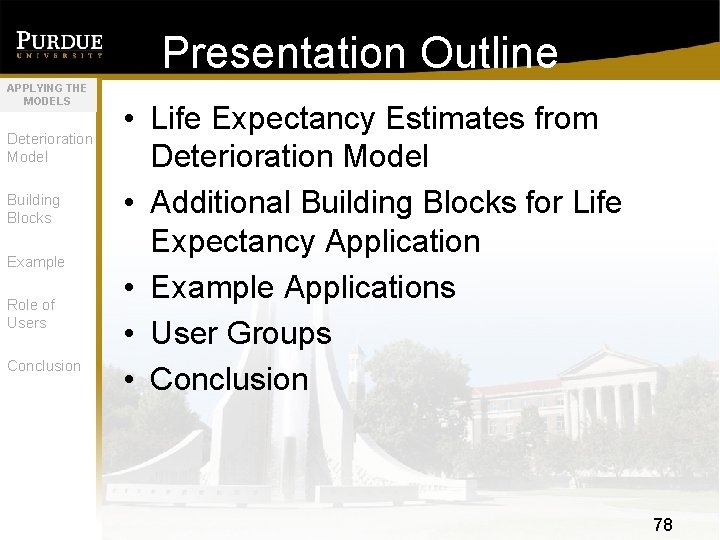
Presentation Outline APPLYING THE MODELS Deterioration Model Building Blocks Example Role of Users Conclusion • Life Expectancy Estimates from Deterioration Model • Additional Building Blocks for Life Expectancy Application • Example Applications • User Groups • Conclusion 78
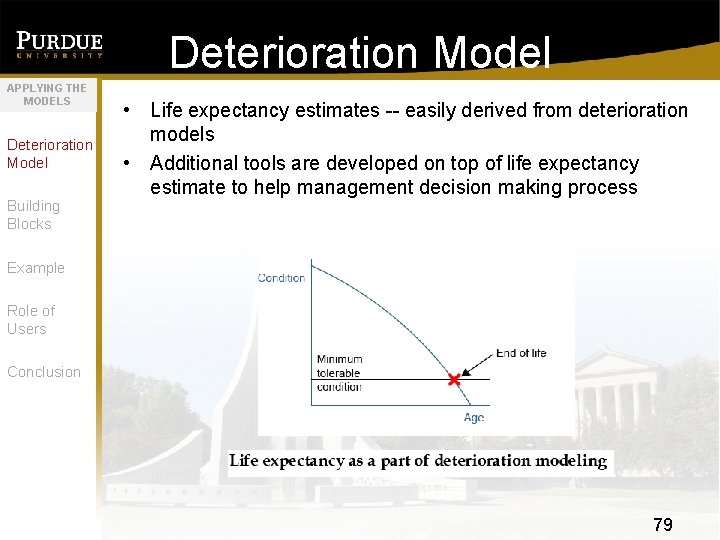
Deterioration Model APPLYING THE MODELS Deterioration Model Building Blocks • Life expectancy estimates -- easily derived from deterioration models • Additional tools are developed on top of life expectancy estimate to help management decision making process Example Role of Users Conclusion 79
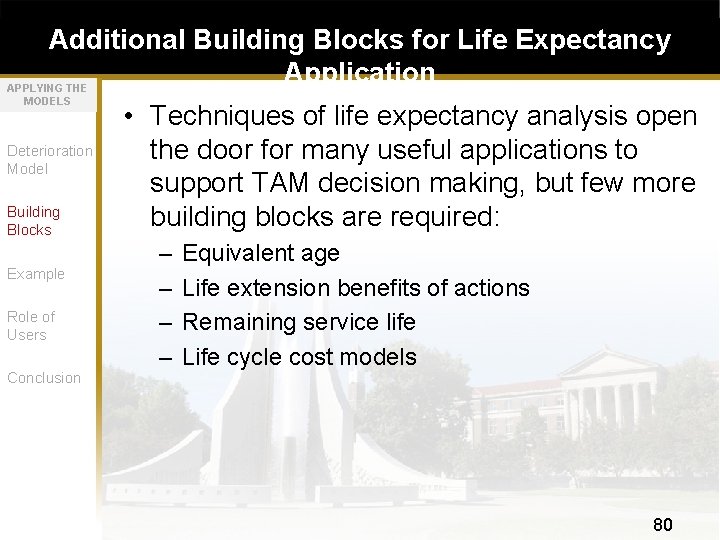
Additional Building Blocks for Life Expectancy Application APPLYING THE MODELS Deterioration Model Building Blocks Example Role of Users Conclusion • Techniques of life expectancy analysis open the door for many useful applications to support TAM decision making, but few more building blocks are required: – – Equivalent age Life extension benefits of actions Remaining service life Life cycle cost models 80
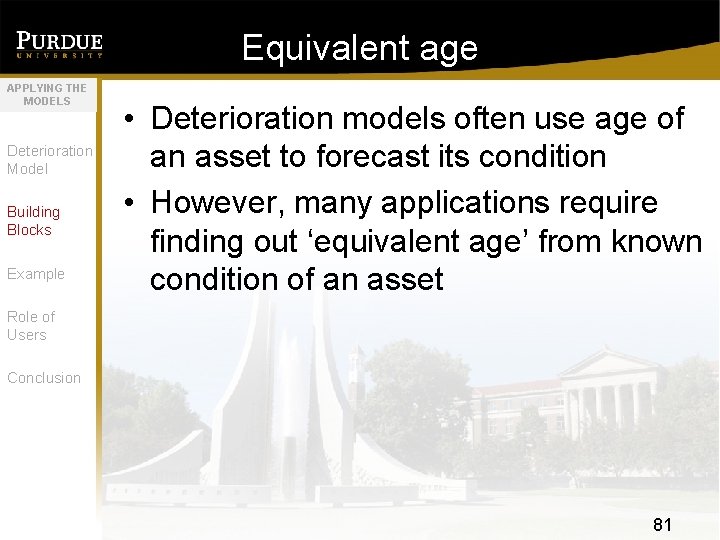
Equivalent age APPLYING THE MODELS Deterioration Model Building Blocks Example • Deterioration models often use age of an asset to forecast its condition • However, many applications require finding out ‘equivalent age’ from known condition of an asset Role of Users Conclusion 81
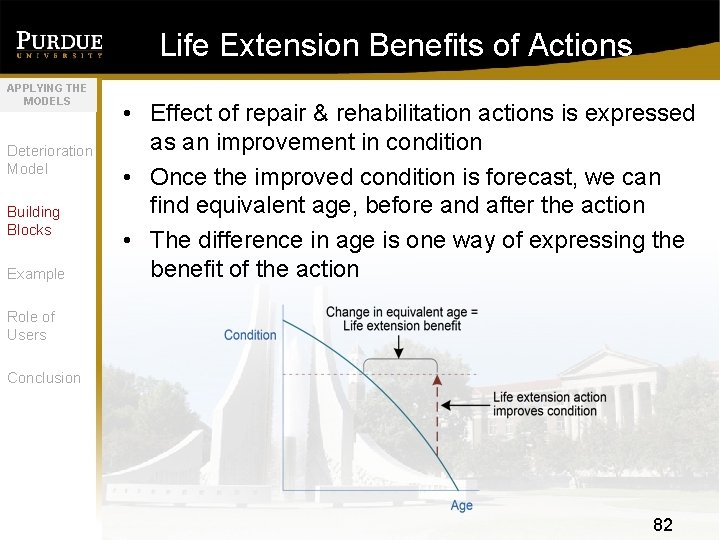
Life Extension Benefits of Actions APPLYING THE MODELS Deterioration Model Building Blocks Example • Effect of repair & rehabilitation actions is expressed as an improvement in condition • Once the improved condition is forecast, we can find equivalent age, before and after the action • The difference in age is one way of expressing the benefit of the action Role of Users Conclusion 82
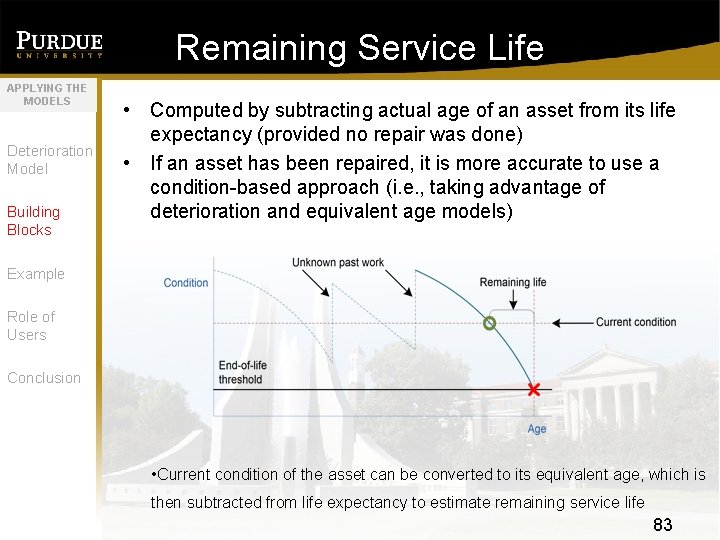
Remaining Service Life APPLYING THE MODELS Deterioration Model Building Blocks • Computed by subtracting actual age of an asset from its life expectancy (provided no repair was done) • If an asset has been repaired, it is more accurate to use a condition-based approach (i. e. , taking advantage of deterioration and equivalent age models) Example Role of Users Conclusion • Current condition of the asset can be converted to its equivalent age, which is then subtracted from life expectancy to estimate remaining service life 83
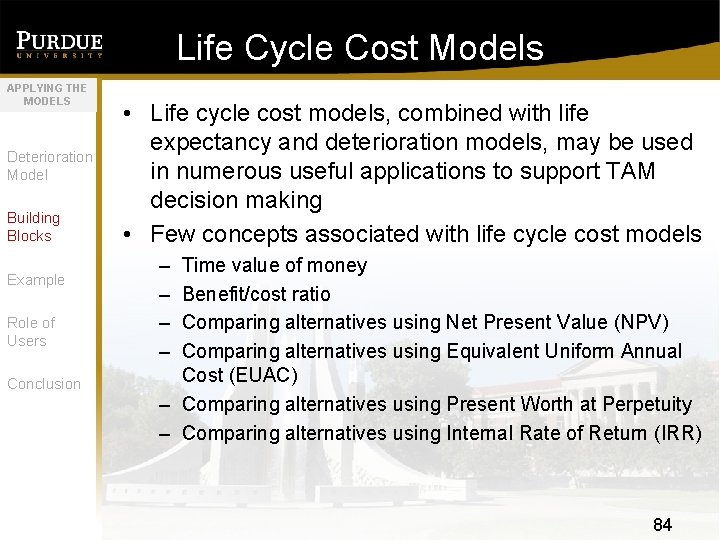
Life Cycle Cost Models APPLYING THE MODELS Deterioration Model Building Blocks Example Role of Users Conclusion • Life cycle cost models, combined with life expectancy and deterioration models, may be used in numerous useful applications to support TAM decision making • Few concepts associated with life cycle cost models – – Time value of money Benefit/cost ratio Comparing alternatives using Net Present Value (NPV) Comparing alternatives using Equivalent Uniform Annual Cost (EUAC) – Comparing alternatives using Present Worth at Perpetuity – Comparing alternatives using Internal Rate of Return (IRR) 84
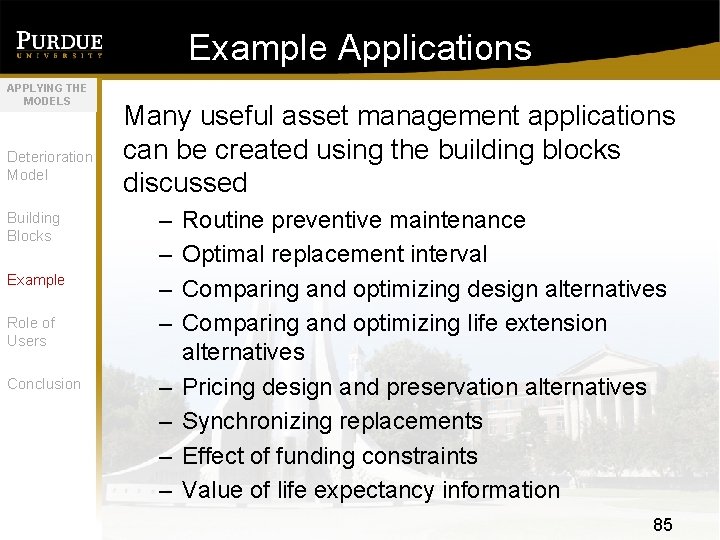
Example Applications APPLYING THE MODELS Deterioration Model Building Blocks Example Role of Users Conclusion Many useful asset management applications can be created using the building blocks discussed – – – – Routine preventive maintenance Optimal replacement interval Comparing and optimizing design alternatives Comparing and optimizing life extension alternatives Pricing design and preservation alternatives Synchronizing replacements Effect of funding constraints Value of life expectancy information 85
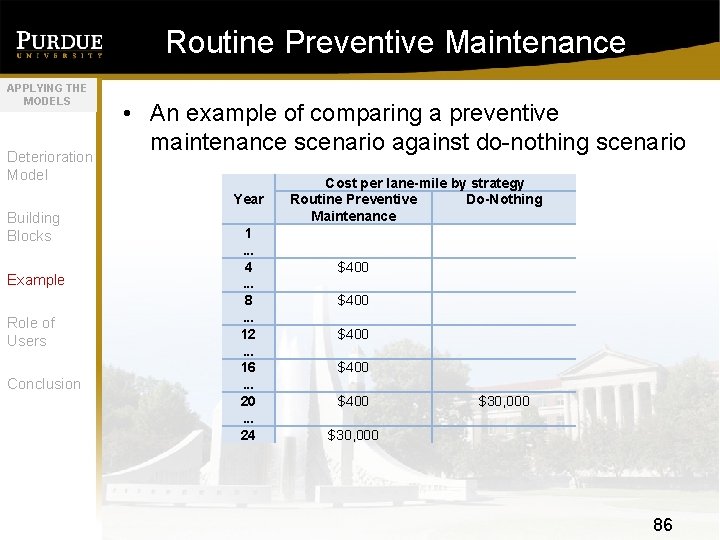
Routine Preventive Maintenance APPLYING THE MODELS Deterioration Model • An example of comparing a preventive maintenance scenario against do-nothing scenario Year Building Blocks Example Role of Users Conclusion 1. . . 4. . . 8. . . 12. . . 16. . . 20. . . 24 Cost per lane-mile by strategy Routine Preventive Do-Nothing Maintenance $400 $400 $30, 000 86
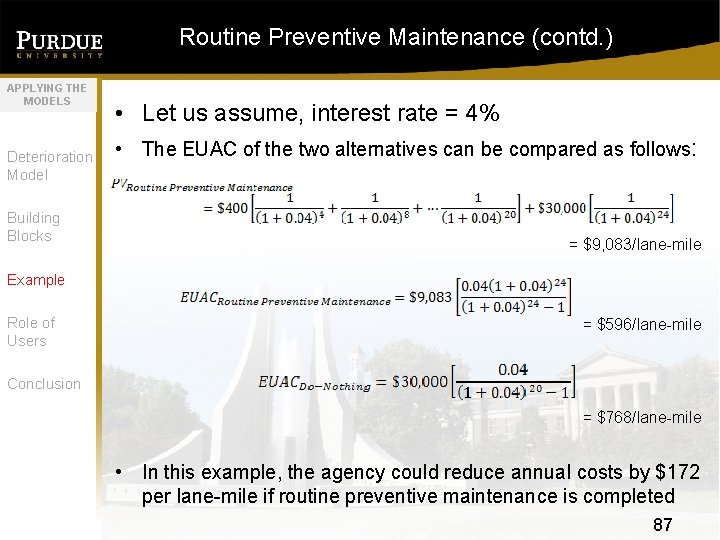
Routine Preventive Maintenance (contd. ) APPLYING THE MODELS Deterioration Model Building Blocks • Let us assume, interest rate = 4% • The EUAC of the two alternatives can be compared as follows: = $9, 083/lane-mile Example Role of Users = $596/lane-mile Conclusion = $768/lane-mile • In this example, the agency could reduce annual costs by $172 per lane-mile if routine preventive maintenance is completed 87
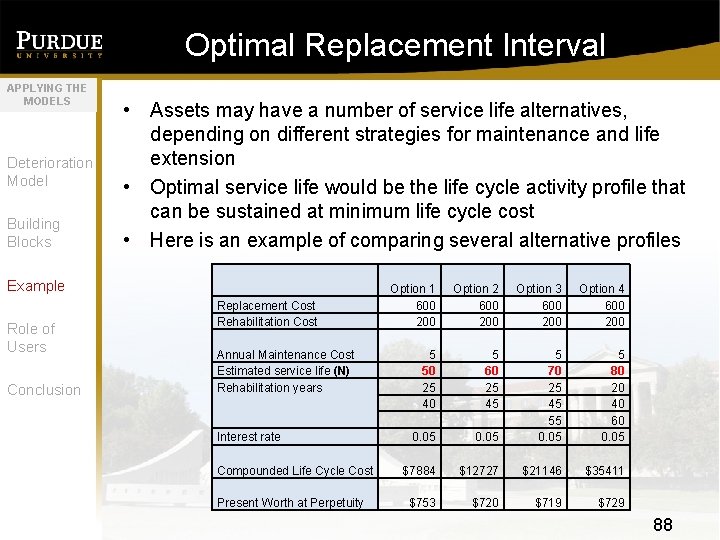
Optimal Replacement Interval APPLYING THE MODELS Deterioration Model Building Blocks • Assets may have a number of service life alternatives, depending on different strategies for maintenance and life extension • Optimal service life would be the life cycle activity profile that can be sustained at minimum life cycle cost • Here is an example of comparing several alternative profiles Example Role of Users Conclusion Replacement Cost Rehabilitation Cost Annual Maintenance Cost Estimated service life (N) Rehabilitation years Interest rate Compounded Life Cycle Cost Present Worth at Perpetuity Option 1 600 200 Option 2 600 200 Option 3 600 200 Option 4 600 200 5 50 25 40 5 60 25 45 0. 05 5 70 25 45 55 0. 05 5 80 20 40 60 0. 05 $7884 $12727 $21146 $35411 $753 $720 $719 $729 88
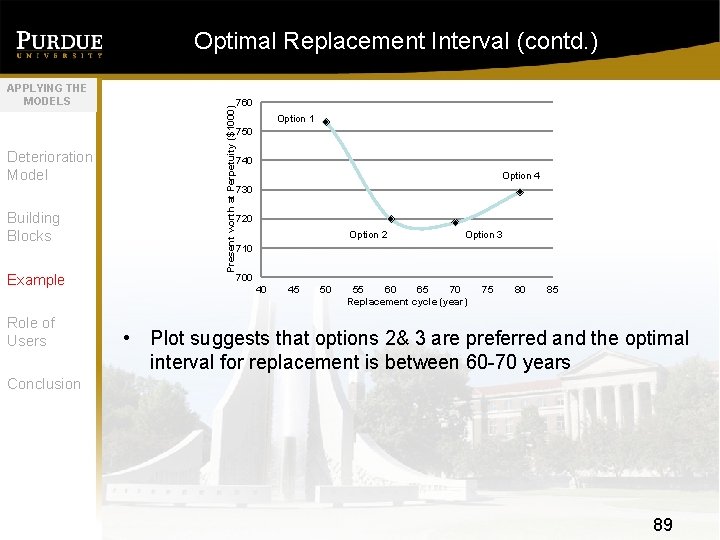
APPLYING THE MODELS Deterioration Model Building Blocks Example Role of Users Present worth at Perpetuity ($1000) Optimal Replacement Interval (contd. ) 760 Option 1 750 740 Option 4 730 720 Option 2 Option 3 710 700 40 45 50 55 60 65 70 Replacement cycle (year) 75 80 85 • Plot suggests that options 2& 3 are preferred and the optimal interval for replacement is between 60 -70 years Conclusion 89
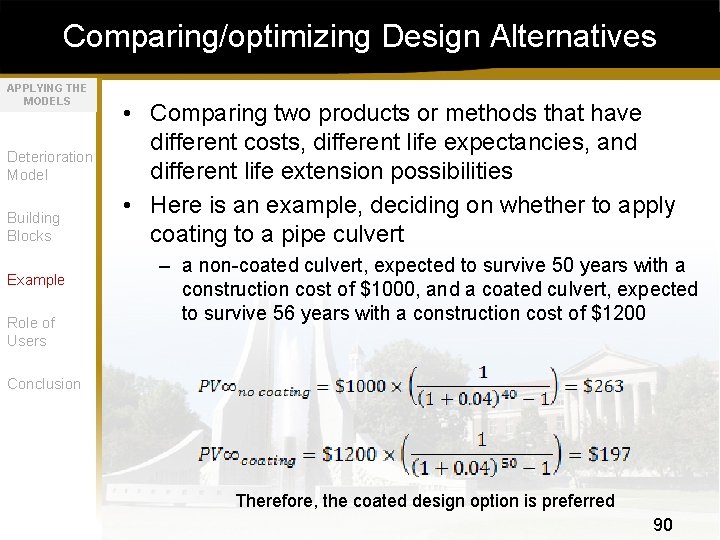
Comparing/optimizing Design Alternatives APPLYING THE MODELS Deterioration Model Building Blocks Example Role of Users • Comparing two products or methods that have different costs, different life expectancies, and different life extension possibilities • Here is an example, deciding on whether to apply coating to a pipe culvert – a non-coated culvert, expected to survive 50 years with a construction cost of $1000, and a coated culvert, expected to survive 56 years with a construction cost of $1200 Conclusion Therefore, the coated design option is preferred 90
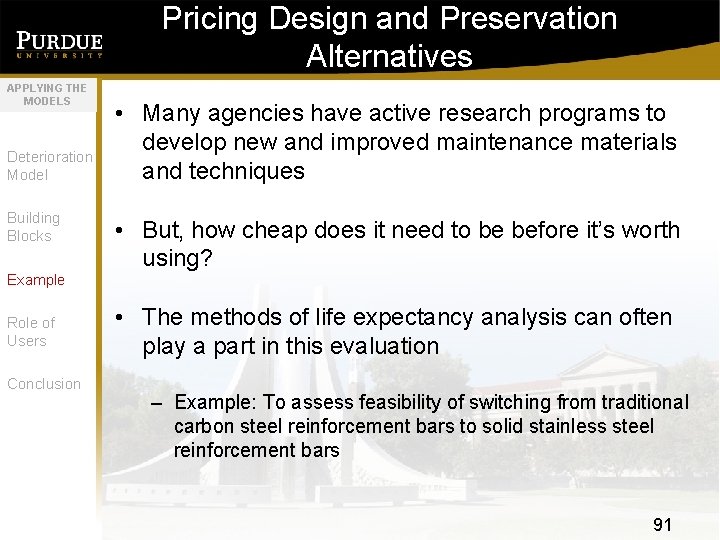
Pricing Design and Preservation Alternatives APPLYING THE MODELS Deterioration Model Building Blocks Example Role of Users Conclusion • Many agencies have active research programs to develop new and improved maintenance materials and techniques • But, how cheap does it need to be before it’s worth using? • The methods of life expectancy analysis can often play a part in this evaluation – Example: To assess feasibility of switching from traditional carbon steel reinforcement bars to solid stainless steel reinforcement bars 91
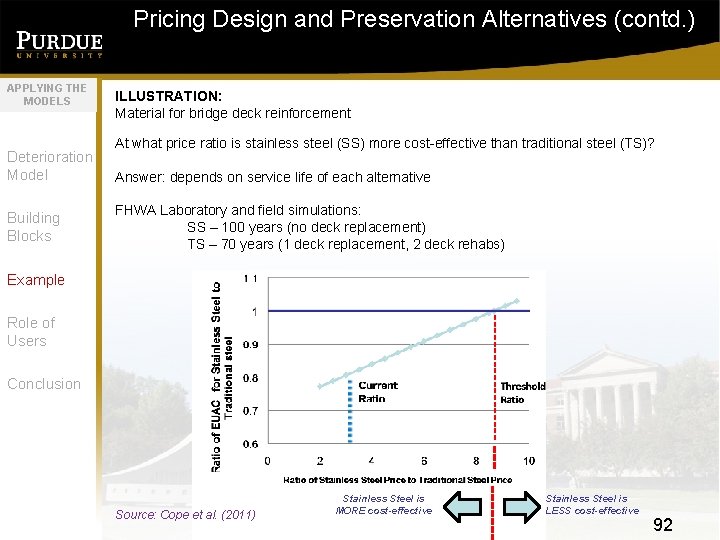
Pricing Design and Preservation Alternatives (contd. ) APPLYING THE MODELS Deterioration Model Building Blocks ILLUSTRATION: Material for bridge deck reinforcement At what price ratio is stainless steel (SS) more cost-effective than traditional steel (TS)? Answer: depends on service life of each alternative FHWA Laboratory and field simulations: SS – 100 years (no deck replacement) TS – 70 years (1 deck replacement, 2 deck rehabs) Example Role of Users Conclusion Source: Cope et al. (2011) Stainless Steel is MORE cost-effective Stainless Steel is LESS cost-effective 92
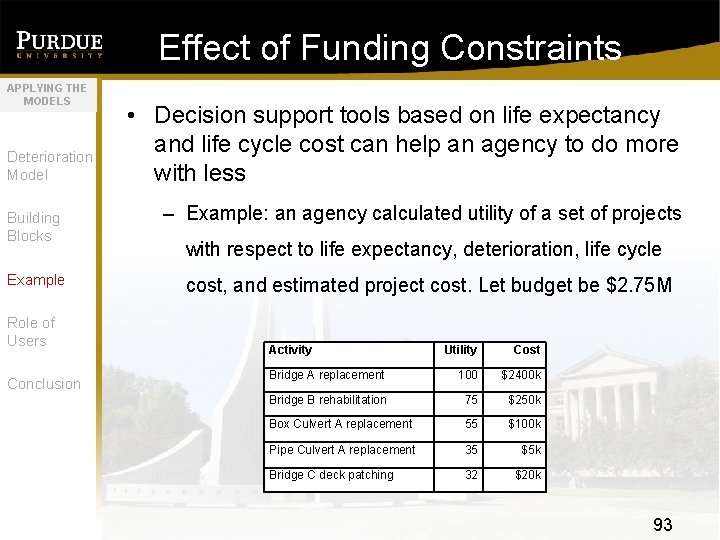
Effect of Funding Constraints APPLYING THE MODELS Deterioration Model Building Blocks Example Role of Users Conclusion • Decision support tools based on life expectancy and life cycle cost can help an agency to do more with less – Example: an agency calculated utility of a set of projects with respect to life expectancy, deterioration, life cycle cost, and estimated project cost. Let budget be $2. 75 M Activity Utility Cost Bridge A replacement 100 $2400 k Bridge B rehabilitation 75 $250 k Box Culvert A replacement 55 $100 k Pipe Culvert A replacement 35 $5 k Bridge C deck patching 32 $20 k 93
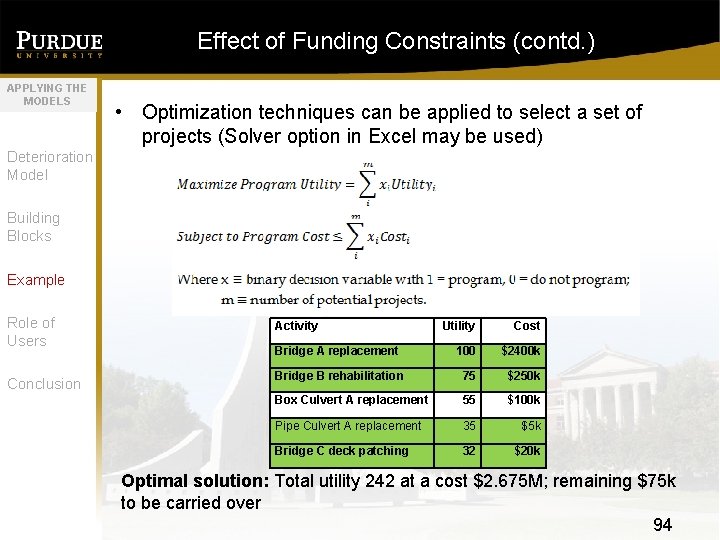
Effect of Funding Constraints (contd. ) APPLYING THE MODELS • Optimization techniques can be applied to select a set of projects (Solver option in Excel may be used) Deterioration Model Building Blocks Example Role of Users Conclusion Activity Utility Cost Bridge A replacement 100 $2400 k Bridge B rehabilitation 75 $250 k Box Culvert A replacement 55 $100 k Pipe Culvert A replacement 35 $5 k Bridge C deck patching 32 $20 k Optimal solution: Total utility 242 at a cost $2. 675 M; remaining $75 k to be carried over 94
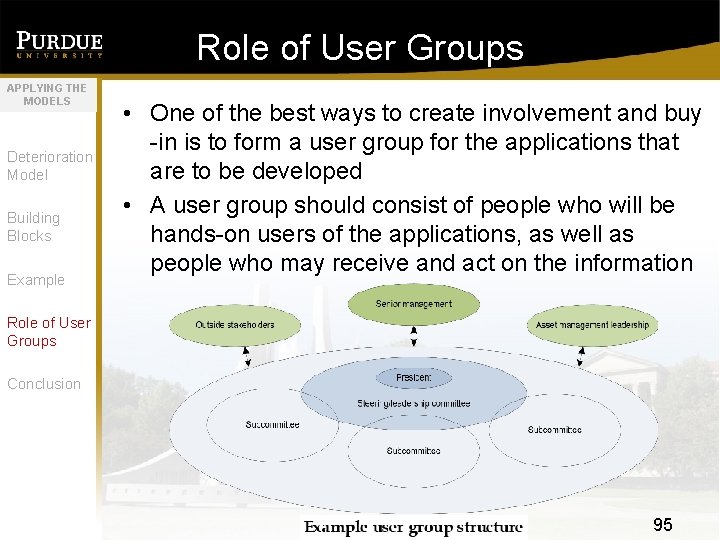
Role of User Groups APPLYING THE MODELS Deterioration Model Building Blocks Example • One of the best ways to create involvement and buy -in is to form a user group for the applications that are to be developed • A user group should consist of people who will be hands-on users of the applications, as well as people who may receive and act on the information Role of User Groups Conclusion 95
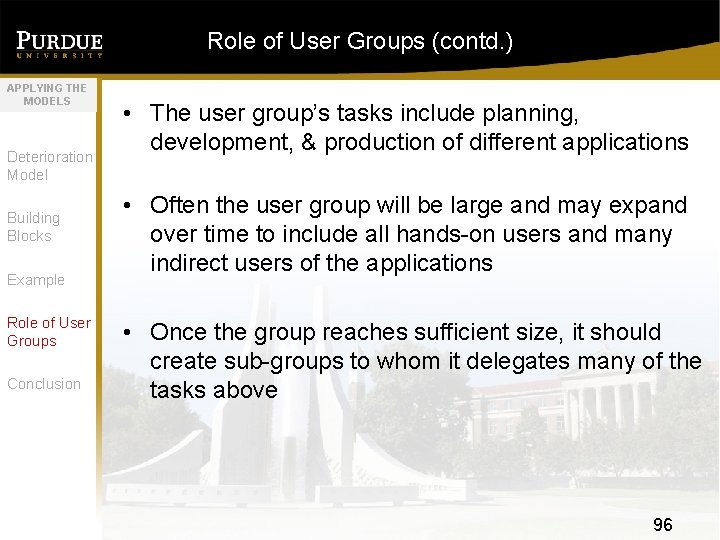
Role of User Groups (contd. ) APPLYING THE MODELS Deterioration Model Building Blocks Example Role of User Groups Conclusion • The user group’s tasks include planning, development, & production of different applications • Often the user group will be large and may expand over time to include all hands-on users and many indirect users of the applications • Once the group reaches sufficient size, it should create sub-groups to whom it delegates many of the tasks above 96
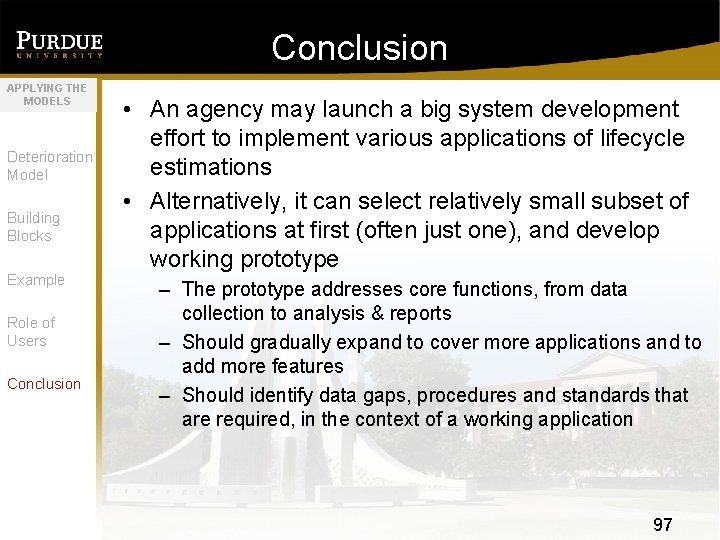
Conclusion APPLYING THE MODELS Deterioration Model Building Blocks Example Role of Users Conclusion • An agency may launch a big system development effort to implement various applications of lifecycle estimations • Alternatively, it can select relatively small subset of applications at first (often just one), and develop working prototype – The prototype addresses core functions, from data collection to analysis & reports – Should gradually expand to cover more applications and to add more features – Should identify data gaps, procedures and standards that are required, in the context of a working application 97
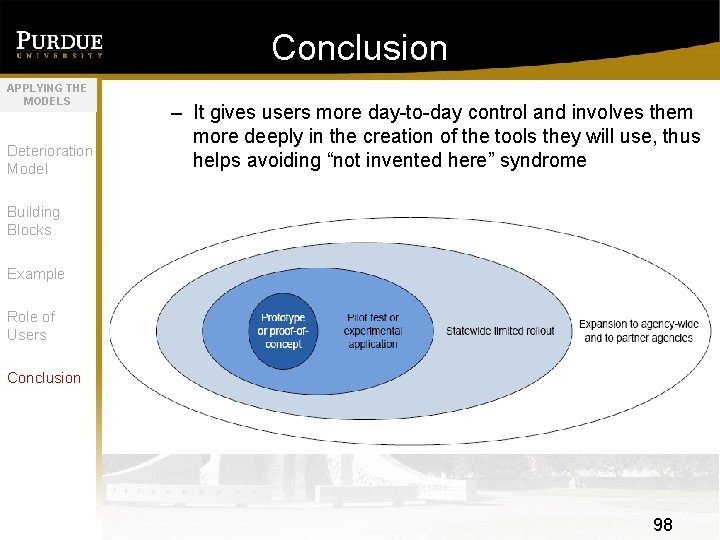
Conclusion APPLYING THE MODELS Deterioration Model – It gives users more day-to-day control and involves them more deeply in the creation of the tools they will use, thus helps avoiding “not invented here” syndrome Building Blocks Example Role of Users Conclusion 98
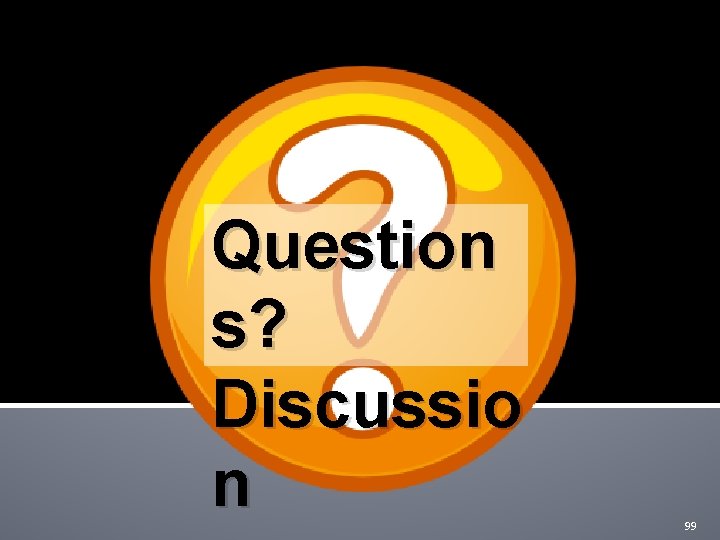
Question s? Discussio n 99
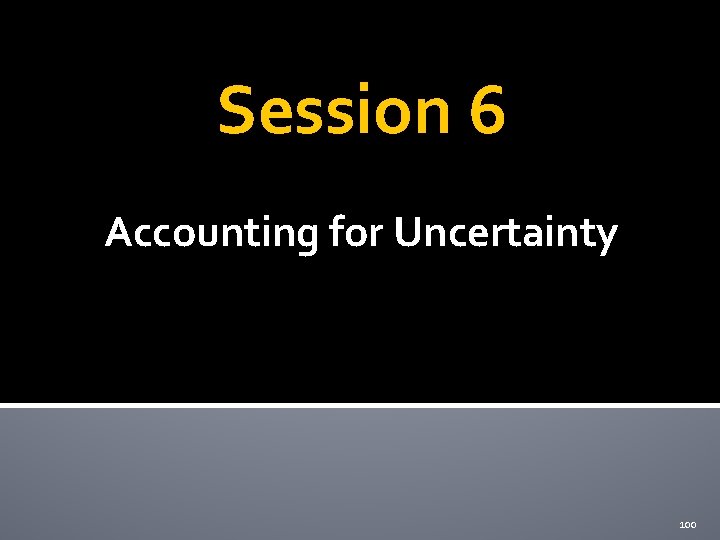
Session 6 Accounting for Uncertainty 100
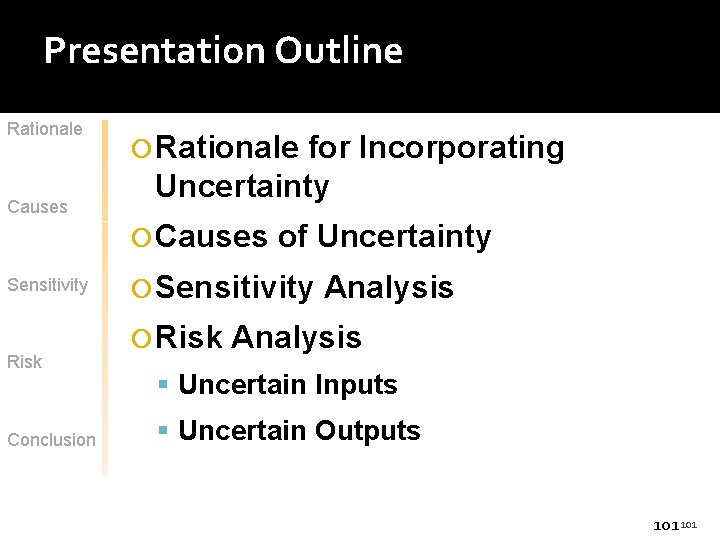
Presentation Outline Rationale Causes Rationale for Incorporating Uncertainty Causes Sensitivity Risk Conclusion of Uncertainty Sensitivity Risk Analysis Uncertain Inputs Uncertain Outputs 101
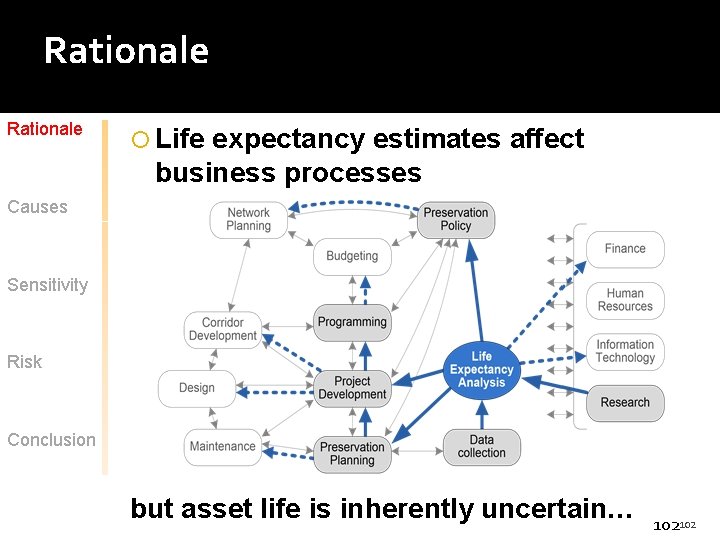
Rationale Life expectancy estimates affect business processes Causes Sensitivity Risk Conclusion but asset life is inherently uncertain… 102102
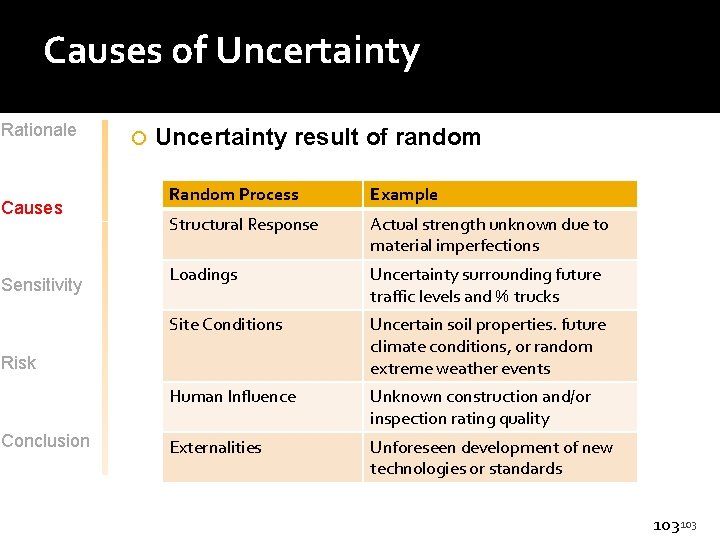
Causes of Uncertainty Rationale Causes Sensitivity Uncertainty result of random Random Process Example Structural Response Actual strength unknown due to material imperfections Loadings Uncertainty surrounding future traffic levels and % trucks Site Conditions Uncertain soil properties. future climate conditions, or random extreme weather events Human Influence Unknown construction and/or inspection rating quality Externalities Unforeseen development of new technologies or standards Risk Conclusion 103
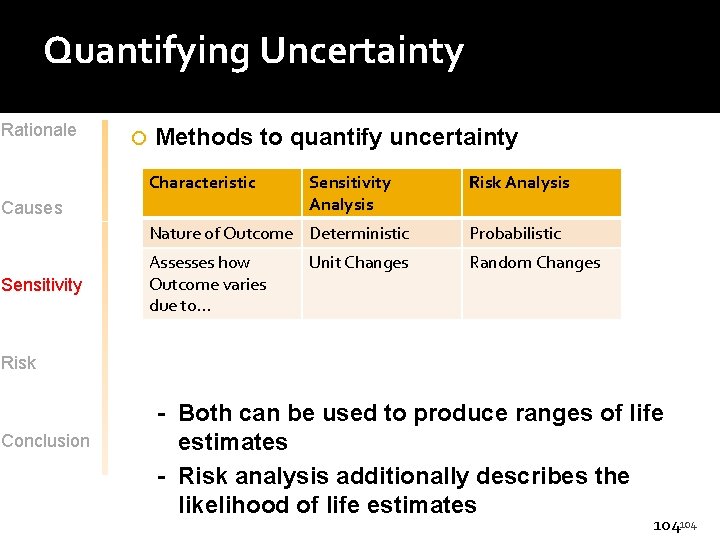
Quantifying Uncertainty Rationale Methods to quantify uncertainty Characteristic Causes Sensitivity Analysis Risk Analysis Nature of Outcome Deterministic Probabilistic Assesses how Outcome varies due to. . . Random Changes Unit Changes Risk Conclusion - Both can be used to produce ranges of life estimates - Risk analysis additionally describes the likelihood of life estimates 104104
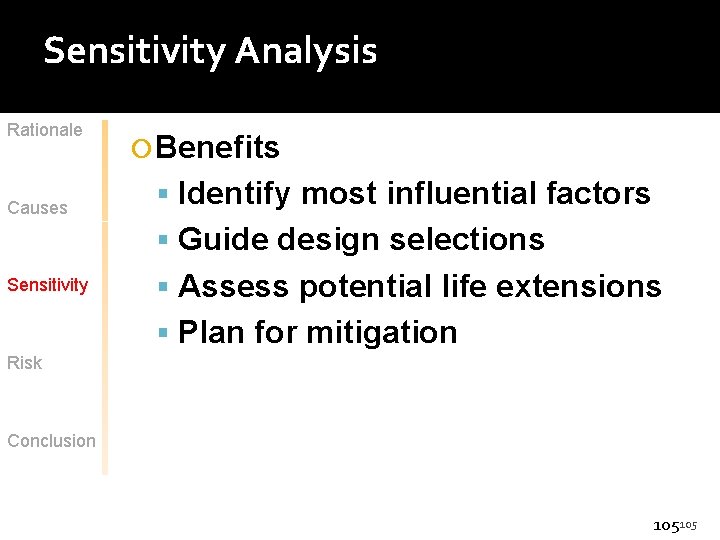
Sensitivity Analysis Rationale Causes Benefits Identify most influential factors Guide design selections Sensitivity Assess potential life extensions Plan for mitigation Risk Conclusion 105105
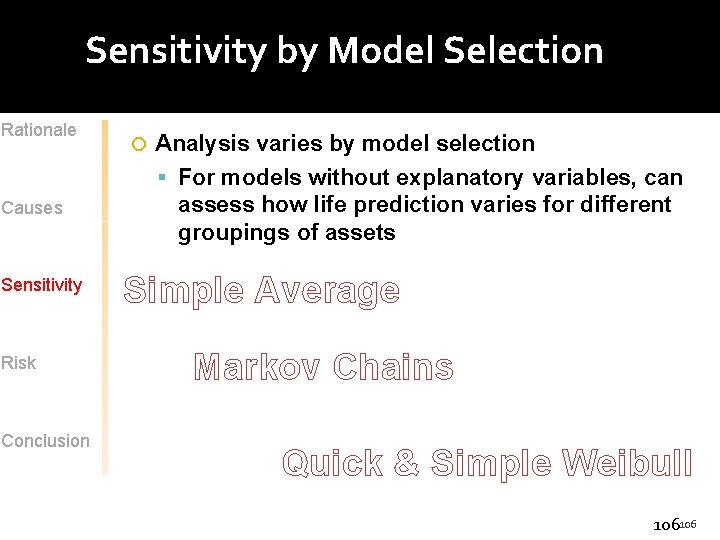
Sensitivity by Model Selection Rationale Causes Sensitivity Risk Conclusion Analysis varies by model selection For models without explanatory variables, can assess how life prediction varies for different groupings of assets Simple Average Markov Chains Quick & Simple Weibull 106106
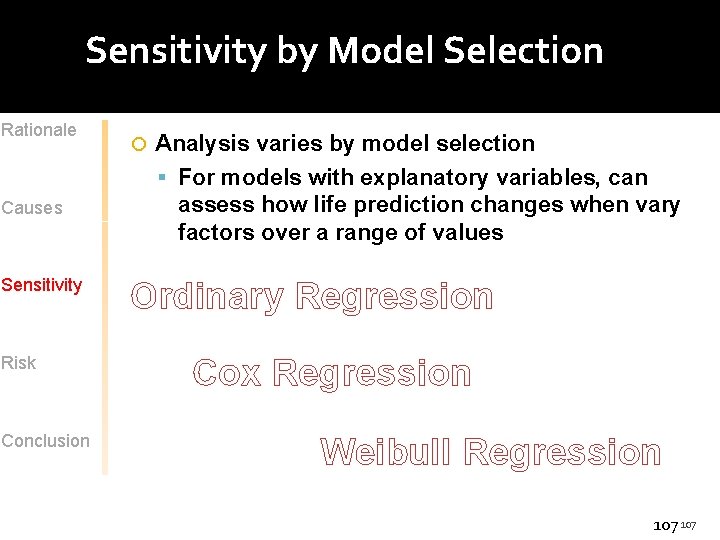
Sensitivity by Model Selection Rationale Causes Sensitivity Risk Conclusion Analysis varies by model selection For models with explanatory variables, can assess how life prediction changes when vary factors over a range of values Ordinary Regression Cox Regression Weibull Regression 107
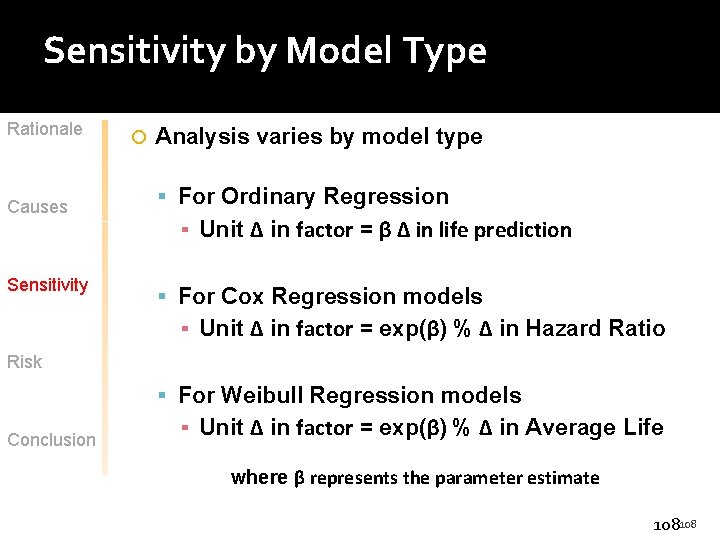
Sensitivity by Model Type Rationale Causes Sensitivity Analysis varies by model type For Ordinary Regression ▪ Unit Δ in factor = β Δ in life prediction For Cox Regression models ▪ Unit Δ in factor = exp(β) % Δ in Hazard Ratio Risk For Weibull Regression models Conclusion ▪ Unit Δ in factor = exp(β) % Δ in Average Life where β represents the parameter estimate 108108
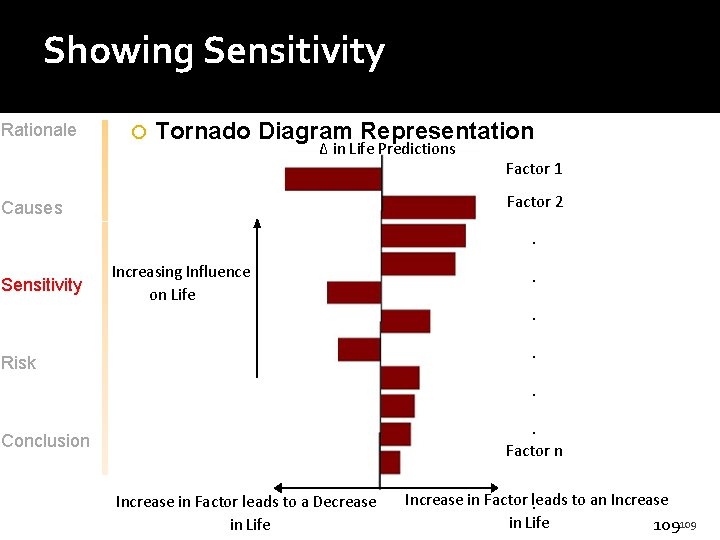
Showing Sensitivity Rationale Tornado Diagram Representation Δ in Life Predictions Factor 1 Factor 2 Causes . Sensitivity Increasing Influence on Life . . . Risk . . Factor n Conclusion Increase in Factor leads to a Decrease in Life Increase in Factor leads to an Increase. in Life 109109
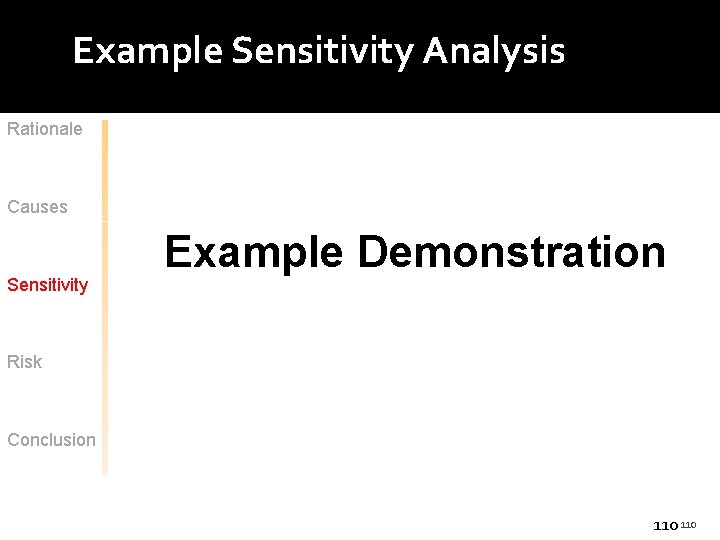
Example Sensitivity Analysis Rationale Causes Sensitivity Example Demonstration Risk Conclusion 110
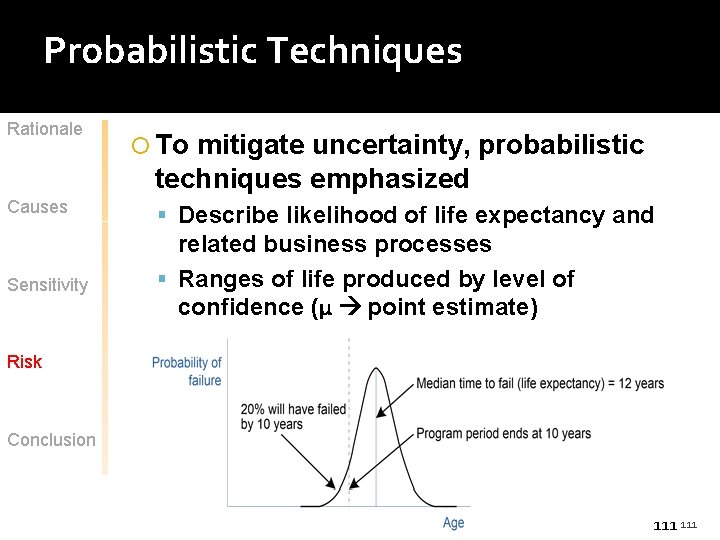
Probabilistic Techniques Rationale Causes Sensitivity To mitigate uncertainty, probabilistic techniques emphasized Describe likelihood of life expectancy and related business processes Ranges of life produced by level of confidence (μ point estimate) Risk Conclusion 111
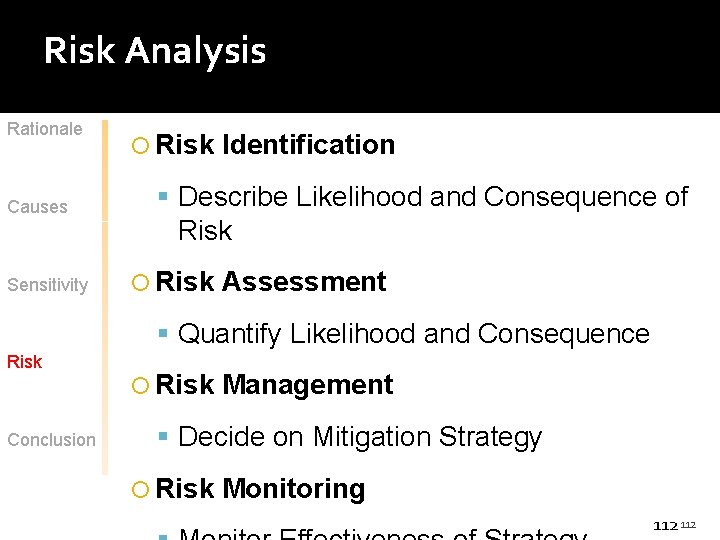
Risk Analysis Rationale Causes Sensitivity Risk Identification Describe Likelihood and Consequence of Risk Assessment Quantify Likelihood and Consequence Risk Conclusion Risk Management Decide on Mitigation Strategy Risk Monitoring 112
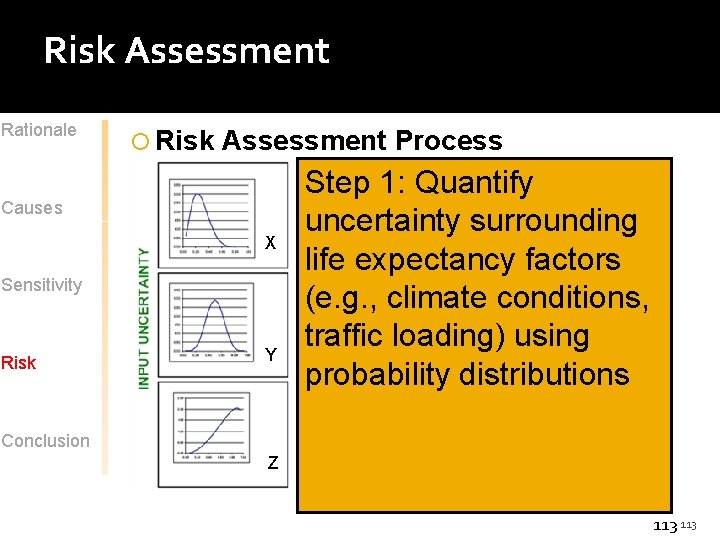
Risk Assessment Rationale Risk Assessment Process Causes X Sensitivity Risk Y Step 1: Quantify uncertainty surrounding life expectancy factors (e. g. , climate conditions, traffic loading) using probability distributions Conclusion Z <Van Dorp, 2009 – GWU> O 113
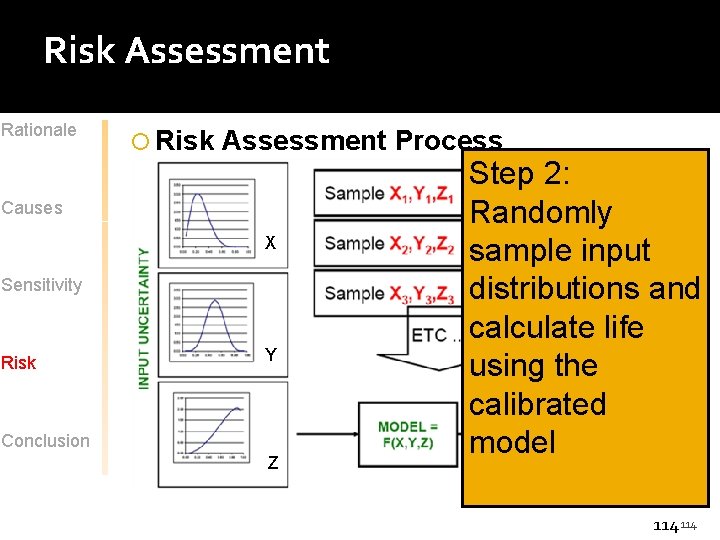
Risk Assessment Rationale Risk Assessment Process Causes X Sensitivity Risk Y Conclusion Z Step 2: Randomly sample input distributions and calculate life using the calibrated model <Van Dorp, 2009 – GWU> O 114
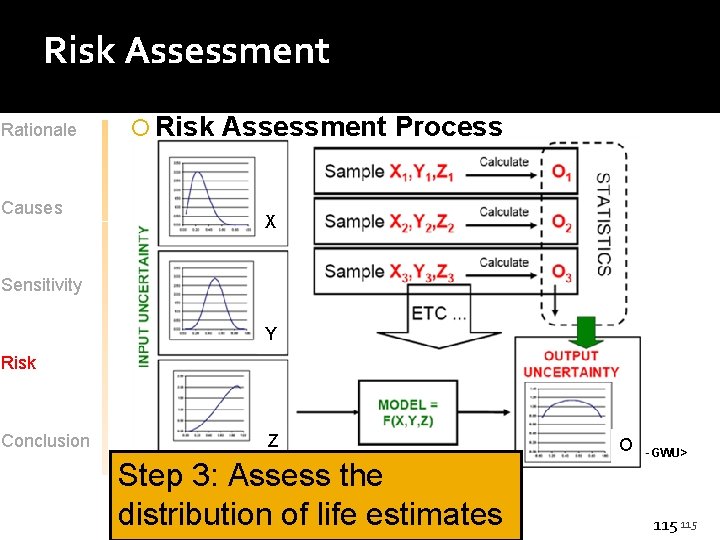
Risk Assessment Rationale Causes Risk Assessment Process X Sensitivity Y Risk Conclusion Z Step 3: Assess the distribution of life estimates O <Van Dorp, 2009 – GWU> 115
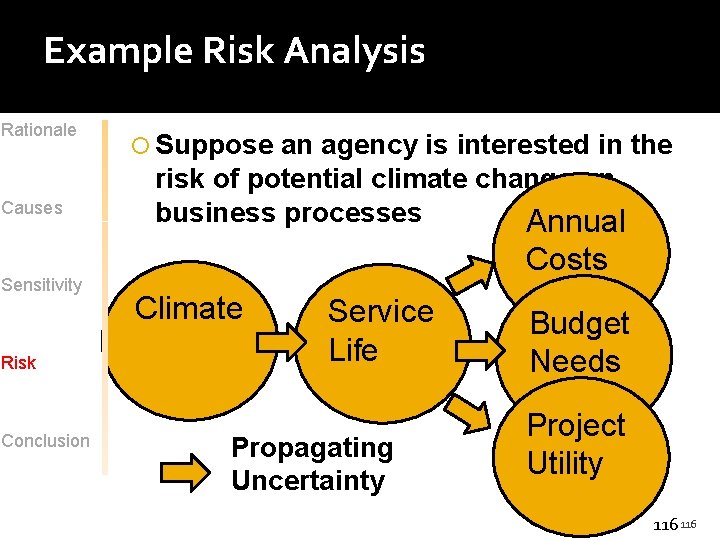
Example Risk Analysis Rationale Causes Sensitivity Risk Conclusion Suppose an agency is interested in the risk of potential climate change on business processes Annual Costs Climate Service Life Propagating Uncertainty Budget Needs Project Utility 116
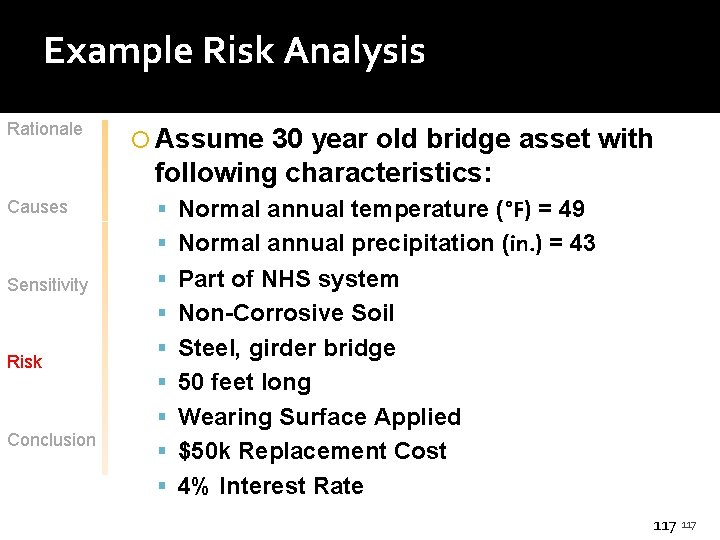
Example Risk Analysis Rationale Causes Assume 30 year old bridge asset with following characteristics: Normal annual temperature (°F) = 49 Normal annual precipitation (in. ) = 43 Sensitivity Part of NHS system Non-Corrosive Soil Risk Conclusion Steel, girder bridge 50 feet long Wearing Surface Applied $50 k Replacement Cost 4% Interest Rate 117
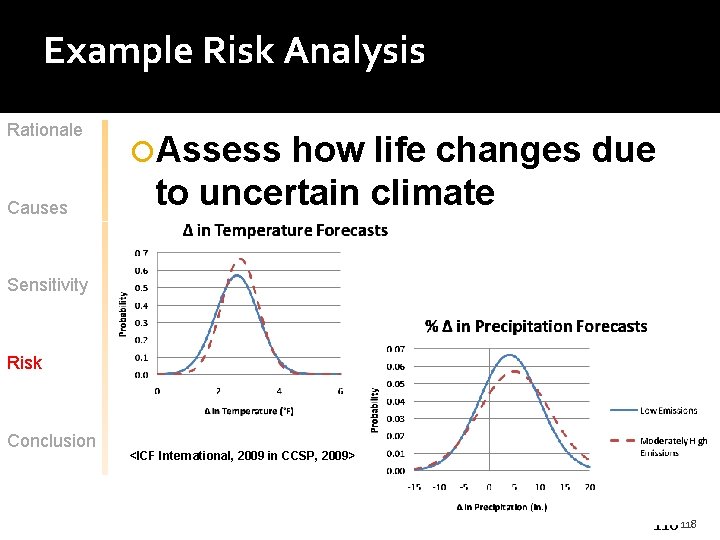
Example Risk Analysis Rationale Causes Assess how life changes due to uncertain climate Sensitivity Risk Conclusion <ICF International, 2009 in CCSP, 2009> 118
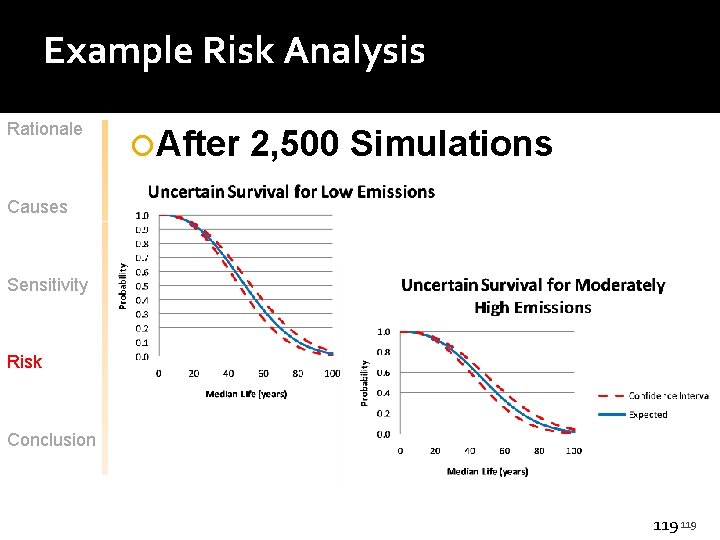
Example Risk Analysis Rationale After 2, 500 Simulations Causes Sensitivity Risk Conclusion 119
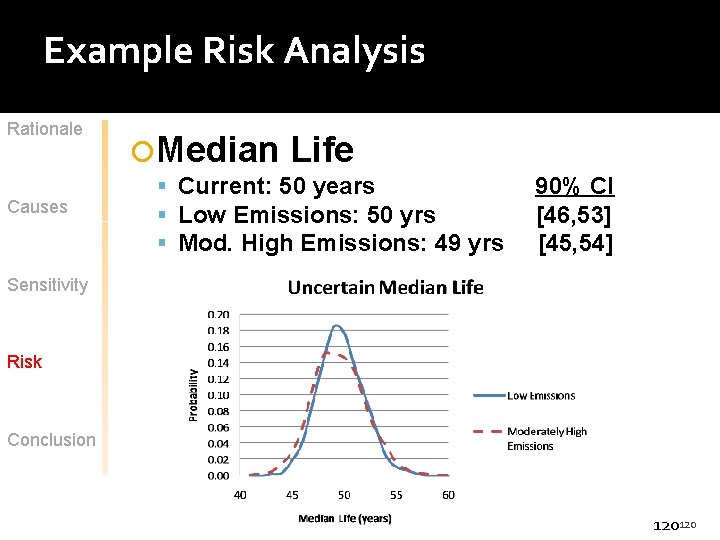
Example Risk Analysis Rationale Causes Median Life Current: 50 years Low Emissions: 50 yrs Mod. High Emissions: 49 yrs 90% CI [46, 53] [45, 54] Sensitivity Risk Conclusion 120120
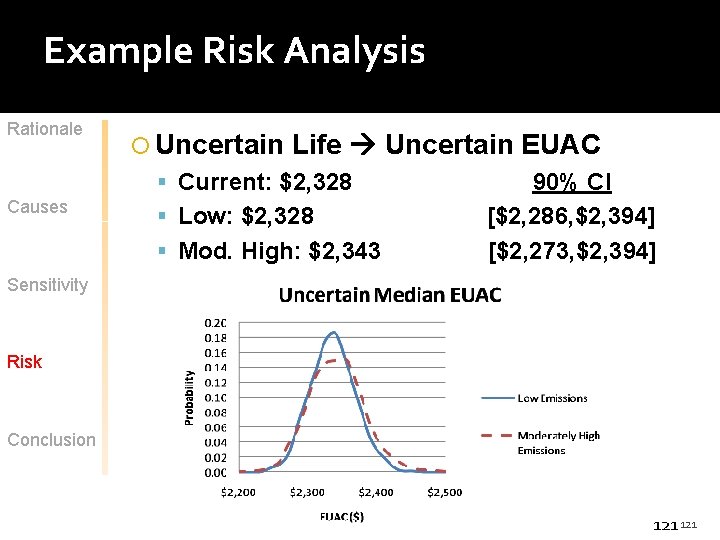
Example Risk Analysis Rationale Uncertain Life Uncertain EUAC Current: $2, 328 Causes Low: $2, 328 Mod. High: $2, 343 90% CI [$2, 286, $2, 394] [$2, 273, $2, 394] Sensitivity Risk Conclusion 121
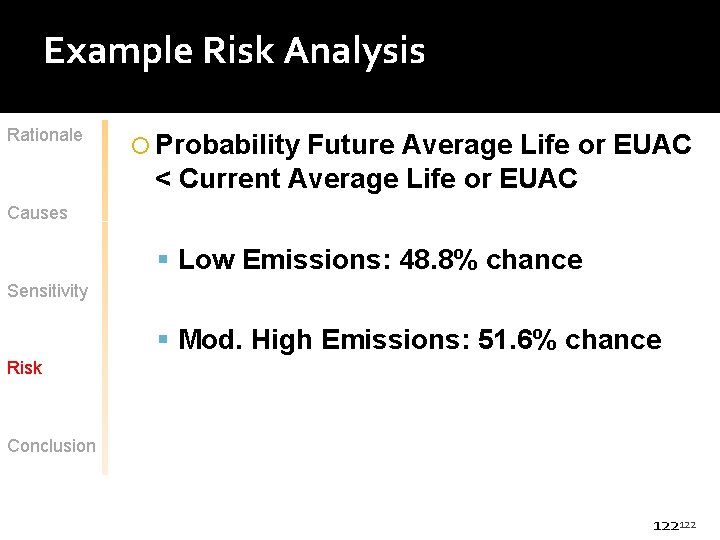
Example Risk Analysis Rationale Probability Future Average Life or EUAC < Current Average Life or EUAC Causes Low Emissions: 48. 8% chance Sensitivity Mod. High Emissions: 51. 6% chance Risk Conclusion 122122
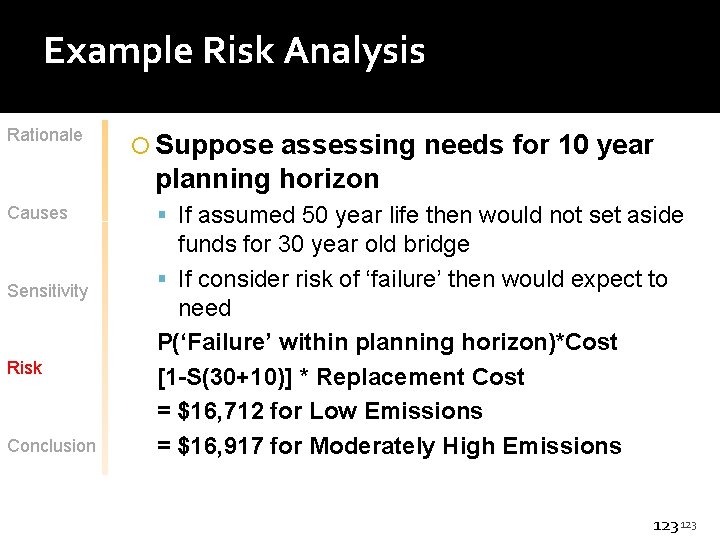
Example Risk Analysis Rationale Causes Sensitivity Risk Conclusion Suppose assessing needs for 10 year planning horizon If assumed 50 year life then would not set aside funds for 30 year old bridge If consider risk of ‘failure’ then would expect to need P(‘Failure’ within planning horizon)*Cost [1 -S(30+10)] * Replacement Cost = $16, 712 for Low Emissions = $16, 917 for Moderately High Emissions 123
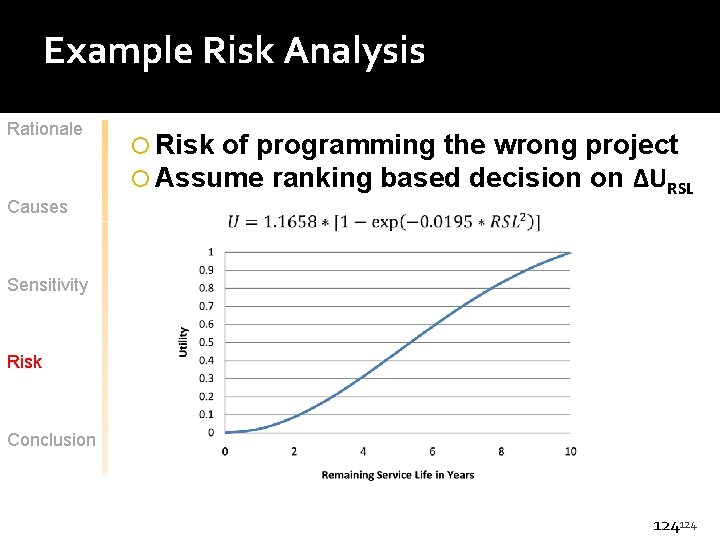
Example Risk Analysis Rationale Causes Risk of programming the wrong project Assume ranking based decision on ΔURSL Sensitivity Risk Conclusion 124124
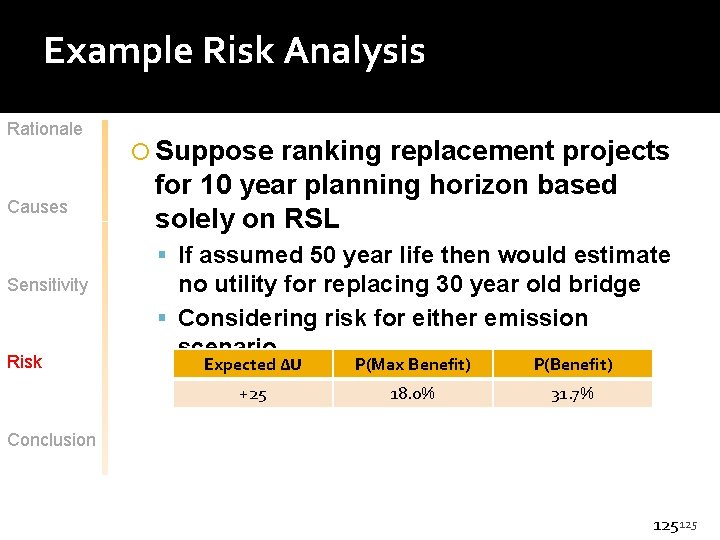
Example Risk Analysis Rationale Causes Suppose ranking replacement projects for 10 year planning horizon based solely on RSL If assumed 50 year life then would estimate Sensitivity Risk no utility for replacing 30 year old bridge Considering risk for either emission scenario… Expected ΔU P(Max Benefit) P(Benefit) +25 18. 0% 31. 7% Conclusion 125125
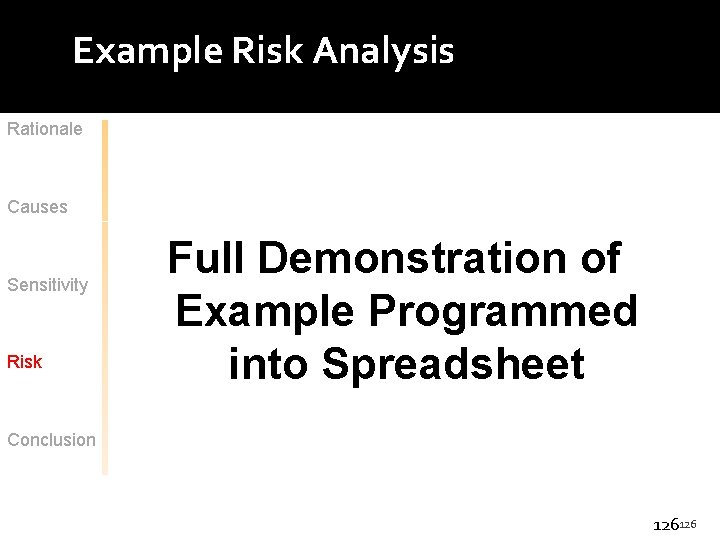
Example Risk Analysis Rationale Causes Sensitivity Risk Full Demonstration of Example Programmed into Spreadsheet Conclusion 126126
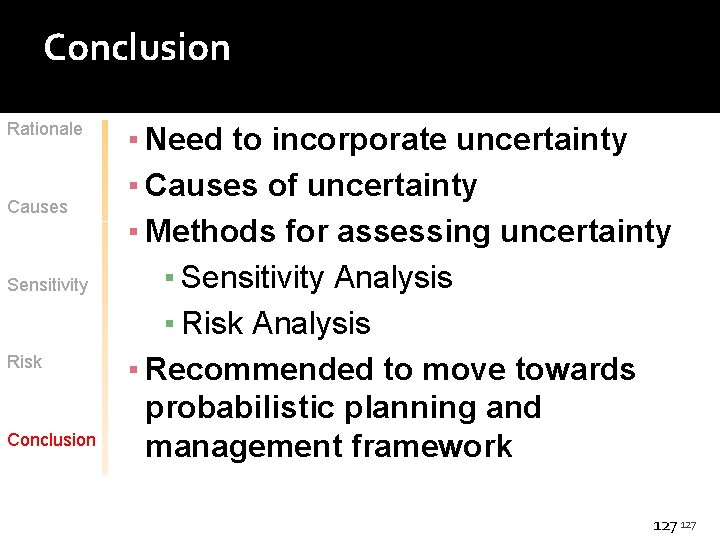
Conclusion Rationale Causes Sensitivity Risk Conclusion ▪ Need to incorporate uncertainty ▪ Causes of uncertainty ▪ Methods for assessing uncertainty ▪ Sensitivity Analysis ▪ Risk Analysis ▪ Recommended to move towards probabilistic planning and management framework 127
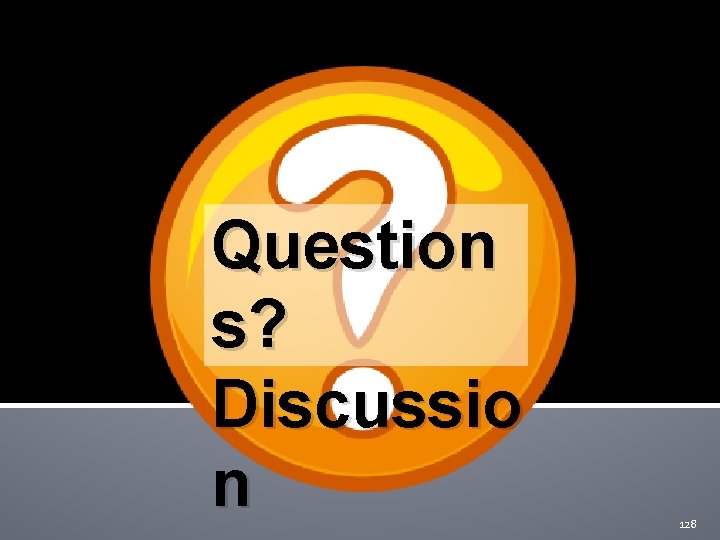
Question s? Discussio n 128
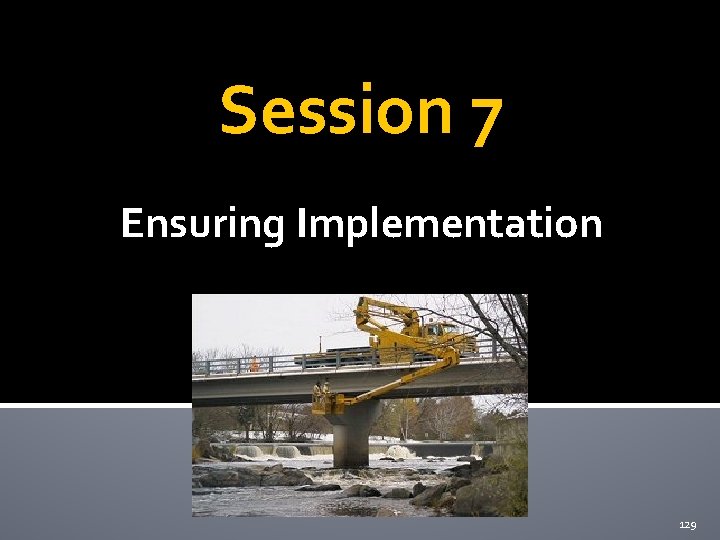
Session 7 Ensuring Implementation 129
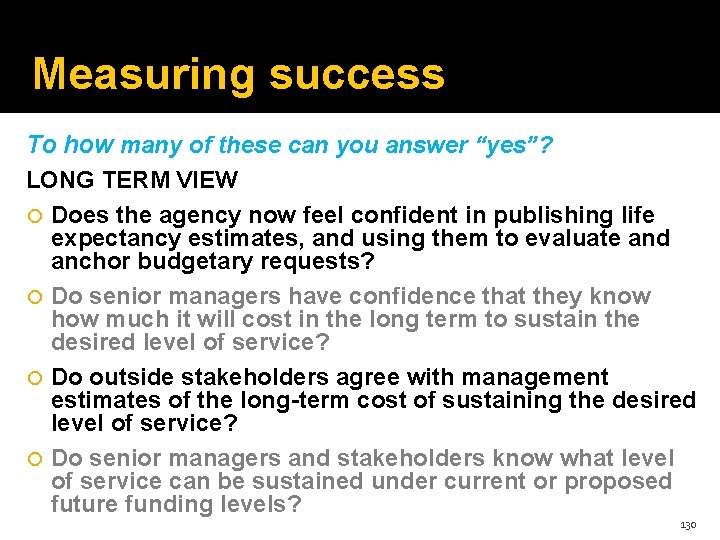
Measuring success To how many of these can you answer “yes”? LONG TERM VIEW Does the agency now feel confident in publishing life expectancy estimates, and using them to evaluate and anchor budgetary requests? Do senior managers have confidence that they know how much it will cost in the long term to sustain the desired level of service? Do outside stakeholders agree with management estimates of the long-term cost of sustaining the desired level of service? Do senior managers and stakeholders know what level of service can be sustained under current or proposed future funding levels? 130
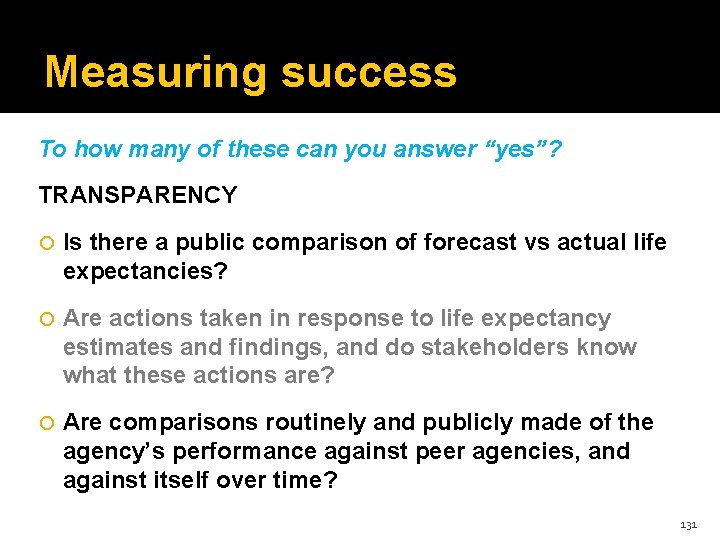
Measuring success To how many of these can you answer “yes”? TRANSPARENCY Is there a public comparison of forecast vs actual life expectancies? Are actions taken in response to life expectancy estimates and findings, and do stakeholders know what these actions are? Are comparisons routinely and publicly made of the agency’s performance against peer agencies, and against itself over time? 131
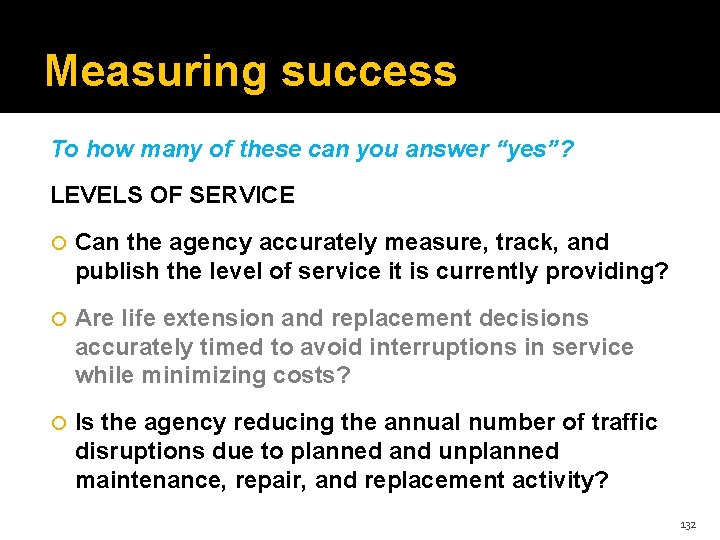
Measuring success To how many of these can you answer “yes”? LEVELS OF SERVICE Can the agency accurately measure, track, and publish the level of service it is currently providing? Are life extension and replacement decisions accurately timed to avoid interruptions in service while minimizing costs? Is the agency reducing the annual number of traffic disruptions due to planned and unplanned maintenance, repair, and replacement activity? 132
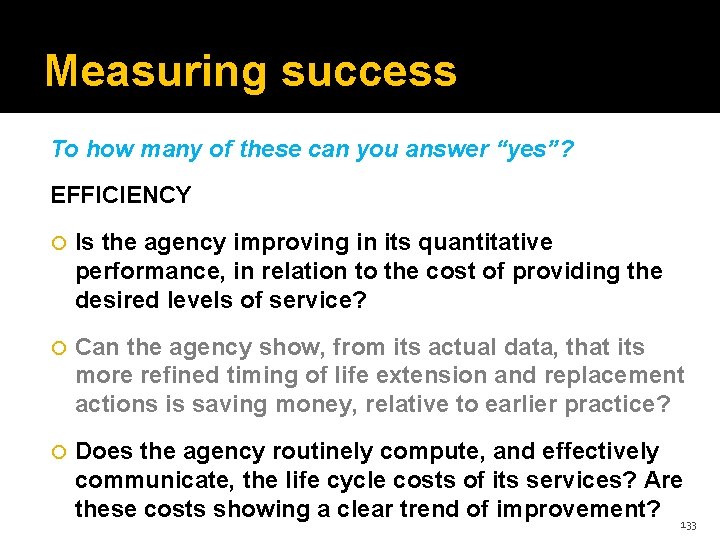
Measuring success To how many of these can you answer “yes”? EFFICIENCY Is the agency improving in its quantitative performance, in relation to the cost of providing the desired levels of service? Can the agency show, from its actual data, that its more refined timing of life extension and replacement actions is saving money, relative to earlier practice? Does the agency routinely compute, and effectively communicate, the life cycle costs of its services? Are these costs showing a clear trend of improvement? 133
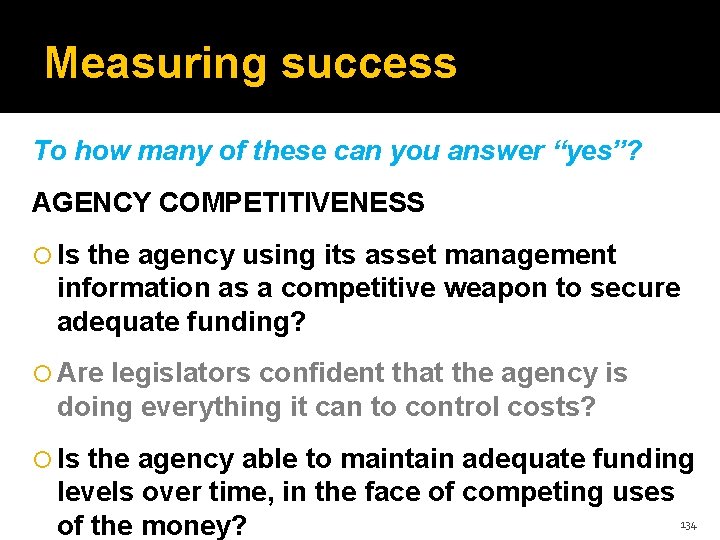
Measuring success To how many of these can you answer “yes”? AGENCY COMPETITIVENESS Is the agency using its asset management information as a competitive weapon to secure adequate funding? Are legislators confident that the agency is doing everything it can to control costs? Is the agency able to maintain adequate funding levels over time, in the face of competing uses 134 of the money?
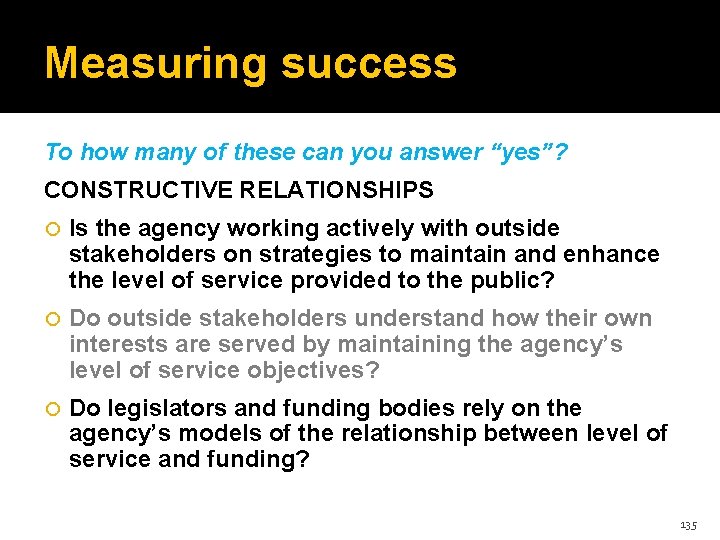
Measuring success To how many of these can you answer “yes”? CONSTRUCTIVE RELATIONSHIPS Is the agency working actively with outside stakeholders on strategies to maintain and enhance the level of service provided to the public? Do outside stakeholders understand how their own interests are served by maintaining the agency’s level of service objectives? Do legislators and funding bodies rely on the agency’s models of the relationship between level of service and funding? 135
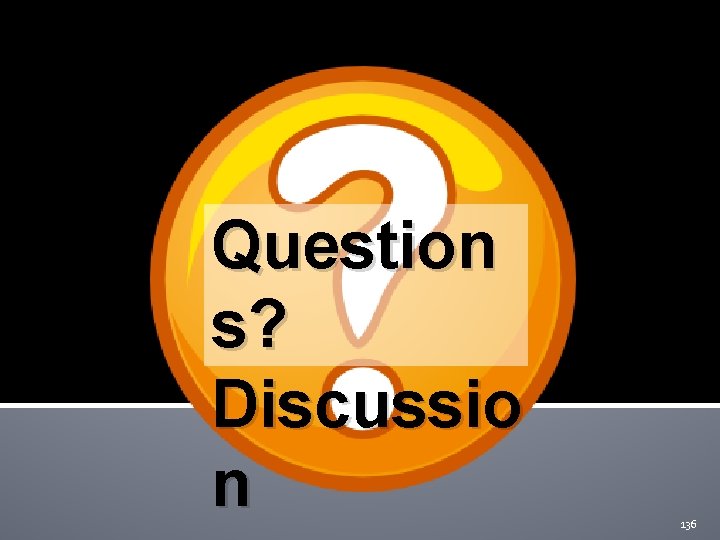
Question s? Discussio n 136
Nchrp report 659
Nchrp 825
Nchrp idea
+ p u r d u e + c o o r d i n a t i o n + d i a g r a m
Nchrp 9-60
Nchrp 17-76
Nchrp 20-44
Siklus hidup produk kfc
Iso 22301 utbildning
Novell typiska drag
Nationell inriktning för artificiell intelligens
Vad står k.r.å.k.a.n för
Varför kallas perioden 1918-1939 för mellankrigstiden?
En lathund för arbete med kontinuitetshantering
Särskild löneskatt för pensionskostnader
Personlig tidbok
Anatomi organ reproduksi
Densitet vatten
Datorkunskap för nybörjare
Boverket ka
Debattartikel struktur
Delegerande ledarstil
Nyckelkompetenser för livslångt lärande
Påbyggnader för flakfordon
Arkimedes princip formel
Svenskt ramverk för digital samverkan
Jag har gått inunder stjärnor text
Presentera för publik crossboss
Vad är ett minoritetsspråk
Kanaans land
Treserva lathund
Mjälthilus
Bästa kameran för astrofoto
Centrum för kunskap och säkerhet
Byggprocessen steg för steg
Mat för idrottare
Verktyg för automatisering av utbetalningar
Rutin för avvikelsehantering
Smärtskolan kunskap för livet
Ministerstyre för och nackdelar
Tack för att ni har lyssnat
Hur ser ett referat ut
Redogör för vad psykologi är
Stål för stötfångarsystem
Atmosfr
Borra hål för knoppar
Vilken grundregel finns det för tronföljden i sverige?
Beräkna standardavvikelse
Tack för att ni har lyssnat
Rita perspektiv
Vad är verksamhetsanalys
Tobinskatten för och nackdelar
Toppslätskivling effekt
Gibbs reflekterande cykel
Egg för emanuel
Elektronik för barn
Vad kallas den mantel som bars av kvinnor i antikens rom
Strategi för svensk viltförvaltning
Var 1721 för stormaktssverige
Humanitr
Sju för caesar
Tack för att ni lyssnade
Enheter för massa
Rim till lärare
Inköpsprocessen steg för steg
Rbk-mätning
Etik och ledarskap etisk kod för chefer
Vad är hsil
Myndigheten för delaktighet
Trög för kemist
Tillitsbaserad ledning
Läkarutlåtande för livränta
Karttecken stig
Geometriska former i förskolan
Shivaismen
Vad är vanlig celldelning
Bris för vuxna
Big brother rösta
Transaction processing system examples
Rounding and estimating
Mcaces
Estimation of degradation function
Gao cost estimating guide
Rounding and estimating decimals
Tendering and estimating
Falguni aggarwal
Dr nabil khouri
1 cubic yard of concrete weight
Estimating avogadro's number lab
Electrical estimate and costing
Cost analysis and estimating for engineering and management
What is the point estimate of μ?
12-1 estimating limits graphically
Agile estimating and planning
Calculating time of death worksheet
Estimating with percents
12-1 practice estimating limits graphically answers
Finding limits graphically and numerically
Estimate whole numbers
First principle estimating
What is front end rounding with decimals
Bluebeam estimating tools
Estimating the difference between two means
Estimating square roots to the nearest hundredth
Estimating products of fractions
Fraction sums
How to interpret confidence intervals example
Ccwfel
2352 prime factorization
Cooling load calculation software
Is 125 a perfect square
Cost analysis and estimating for engineering and management
Cost analysis and estimating for engineering and management
Voyage estimating decision support system
Estimating percents
Billick brothers is estimating its wacc
Estimating products and quotients
Estimating project time and cost
Normal vs expedited costs
Cost analysis and estimating for engineering and management
Estimating project time and cost
Voyage estimating decision support system
Activity duration estimating
Parametric estimating
Estimating soil moisture by feel and appearance
Estimating human shape and pose from a single image
Essentials of management information systems
Voyage estimating decision support system
Is 256 a perfect square
Place value and estimating
Estimating sums and differences of whole numbers
Bottom up estimating
Mining estimating software
Cost analysis and estimating for engineering and management
Chapter 8 estimating with confidence
Suppose your class is investigating the weights of snickers
Chapter 8 estimating with confidence