1 Welcome Thanks for joining this ITRC Training
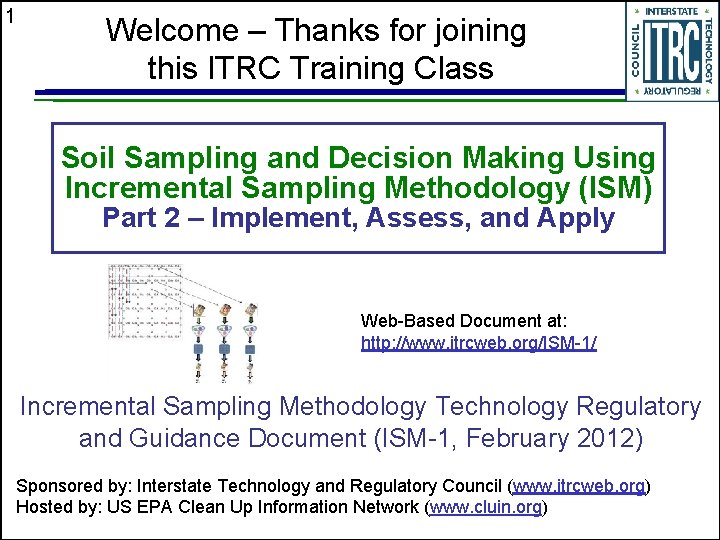
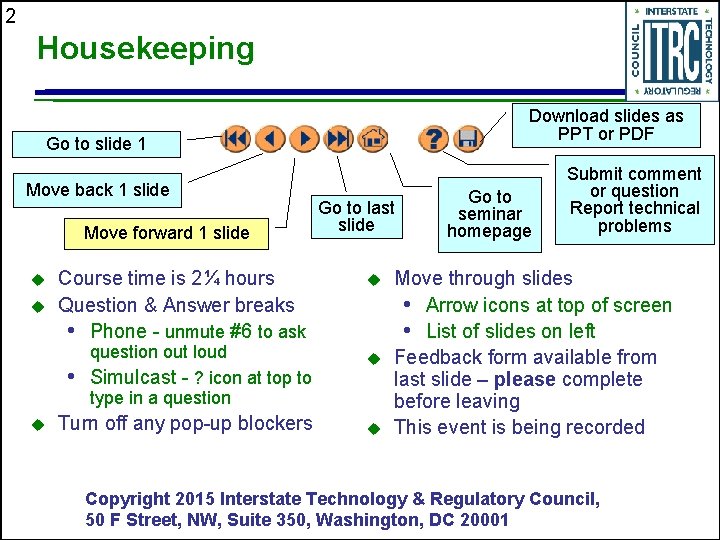
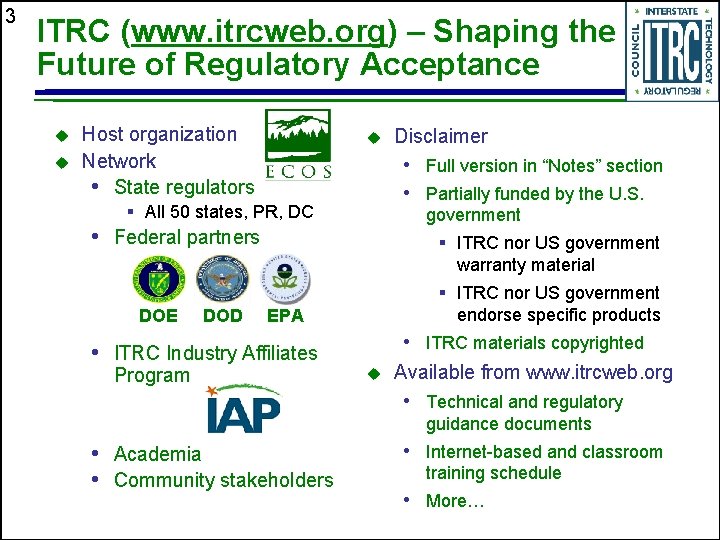
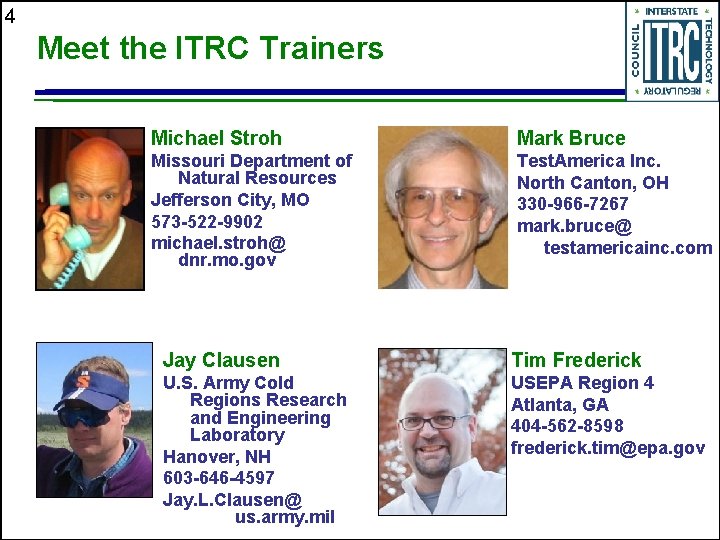
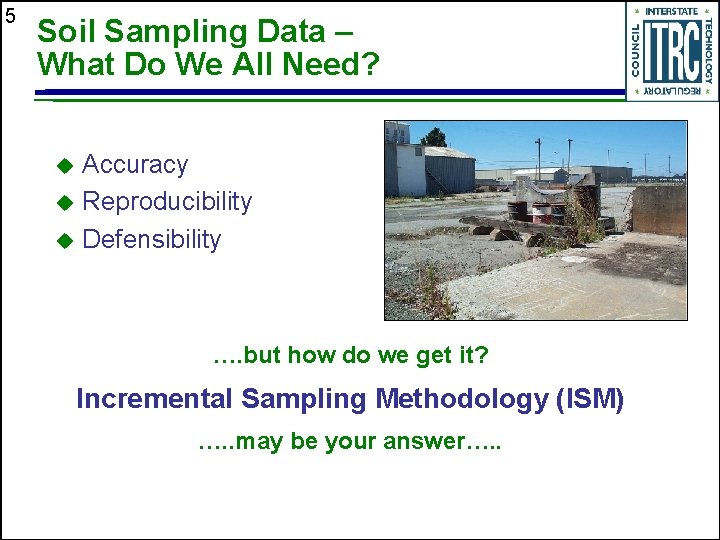
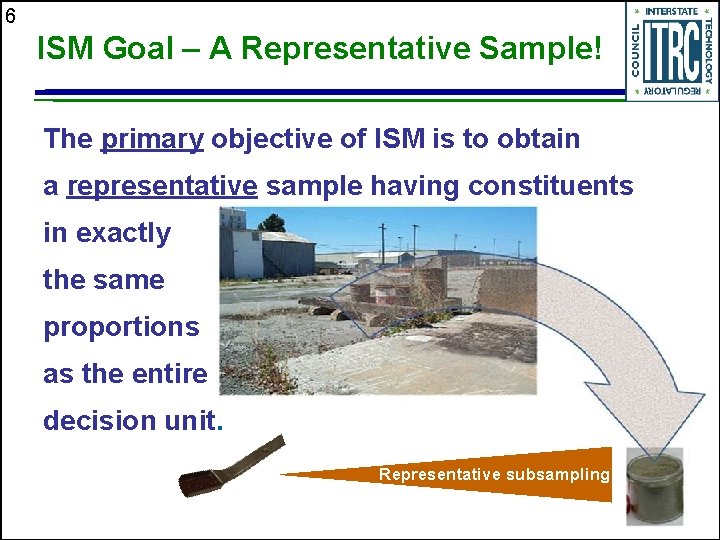
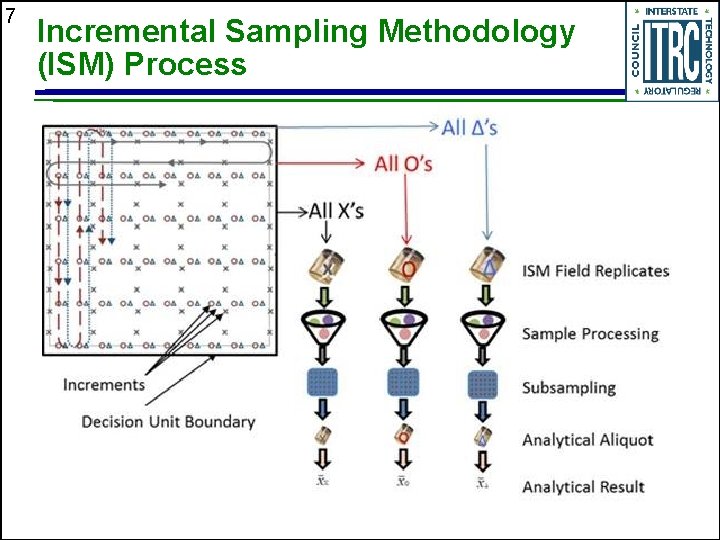
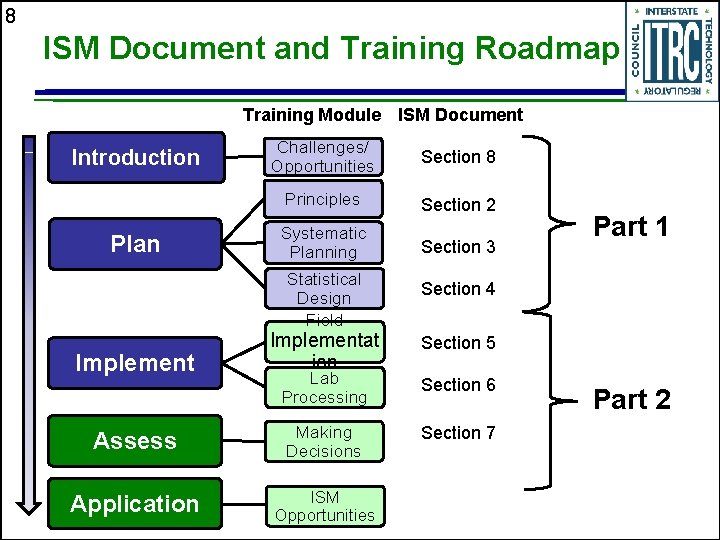
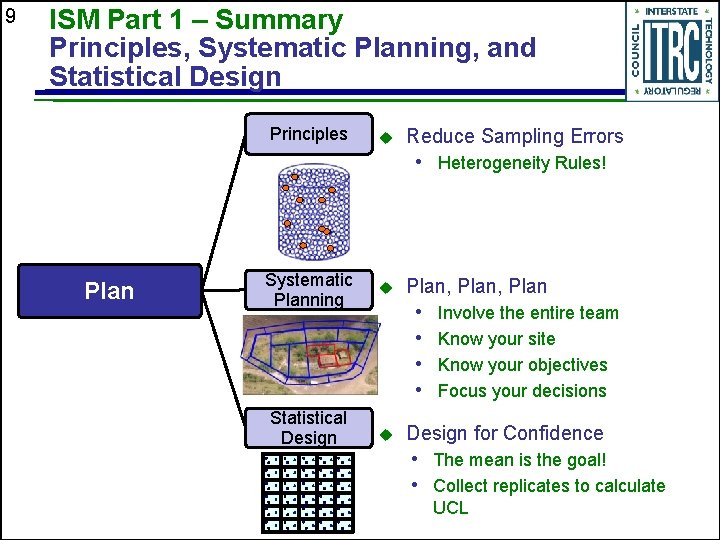
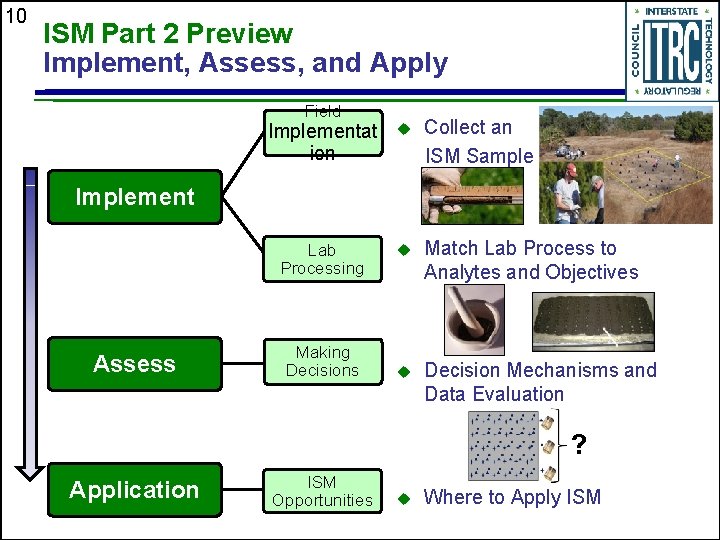
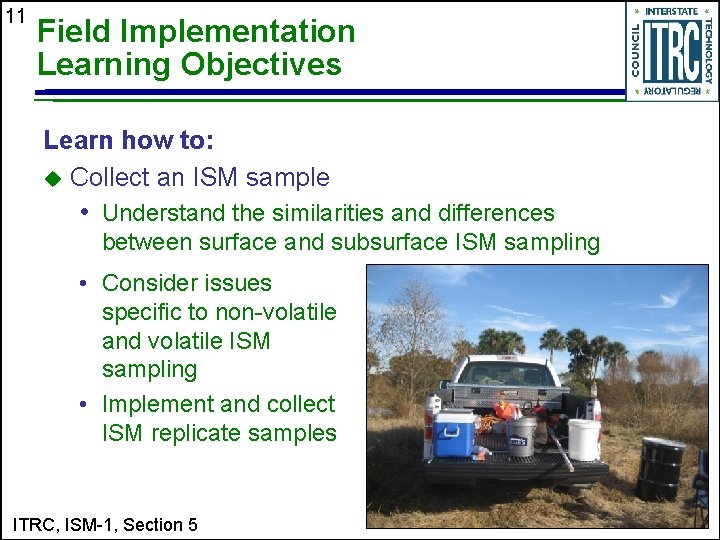
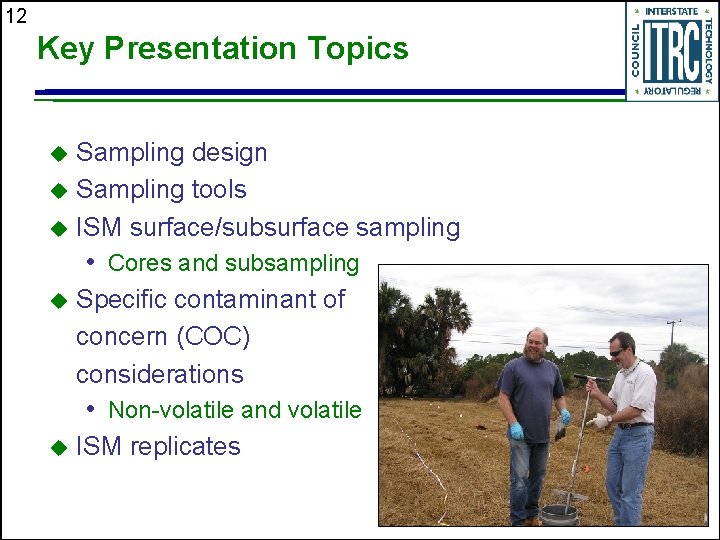
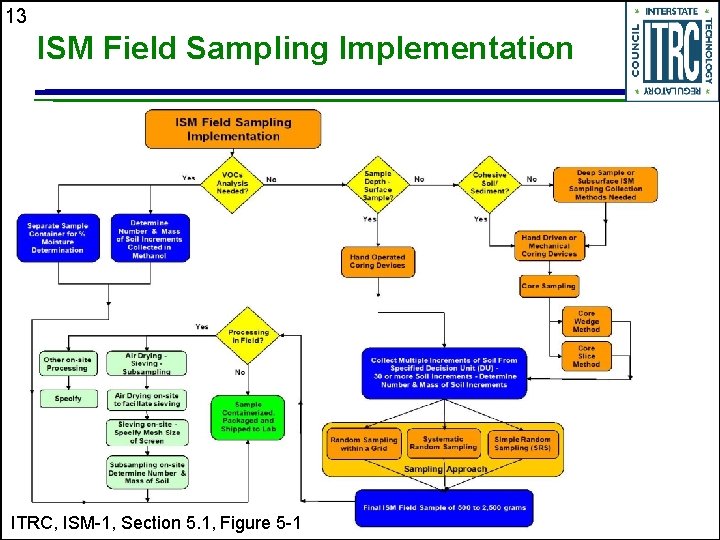
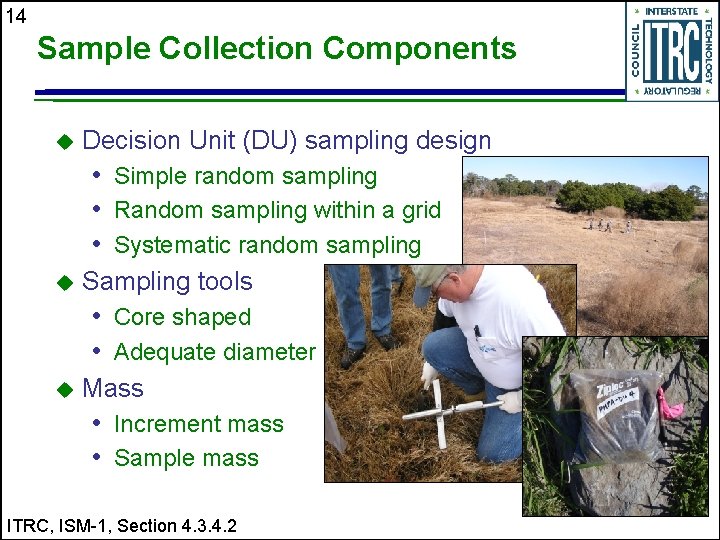
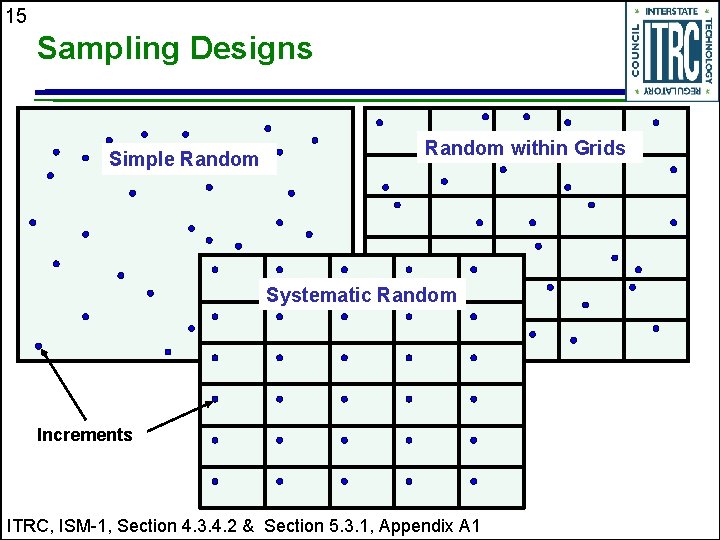
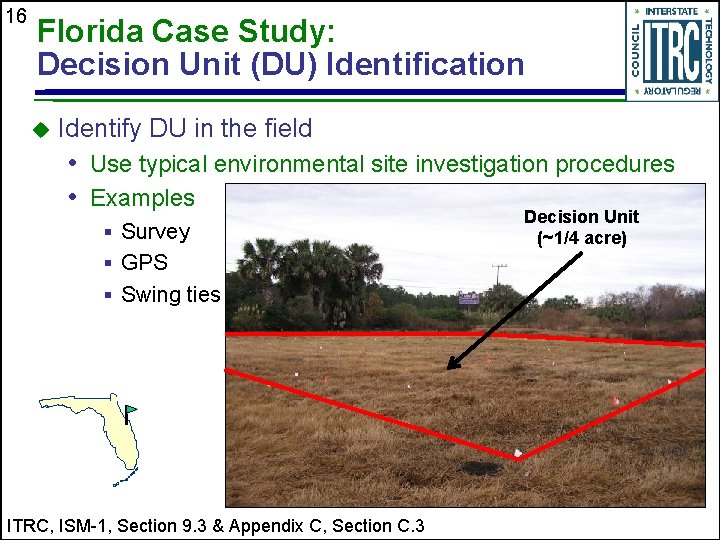
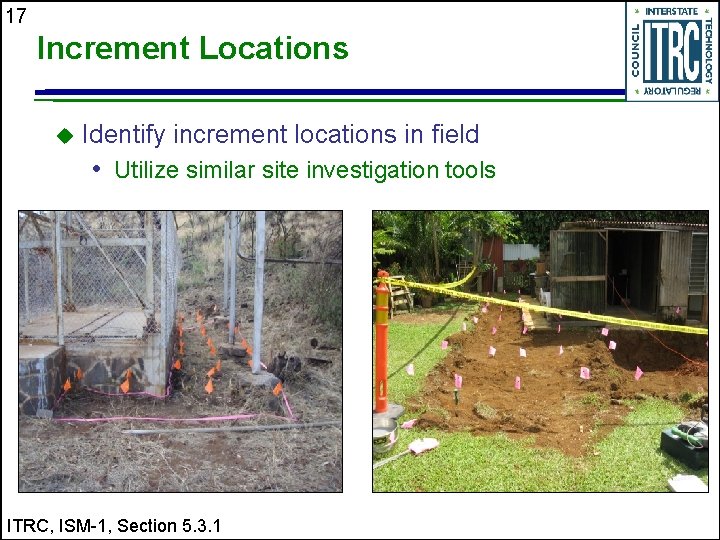
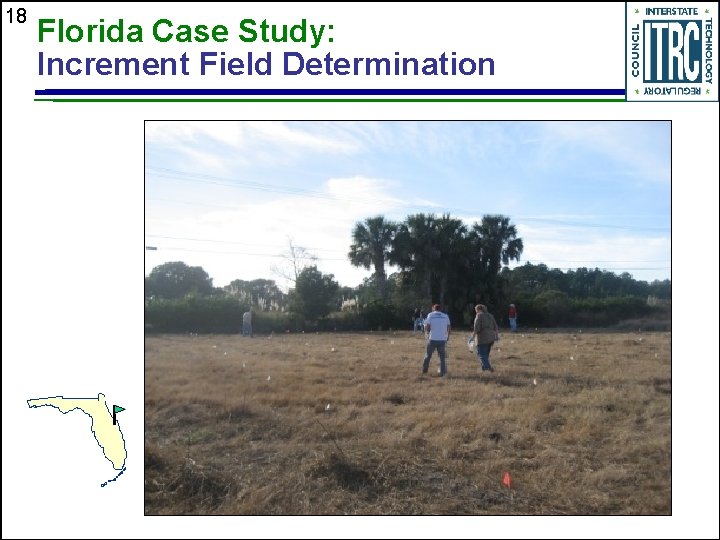
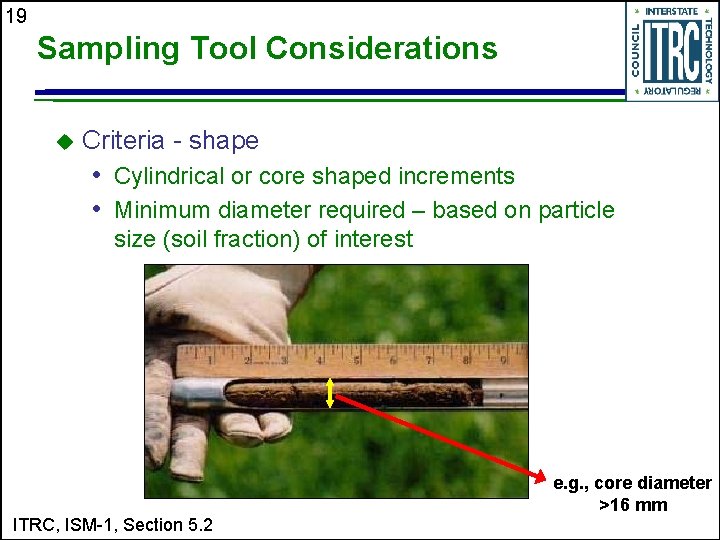
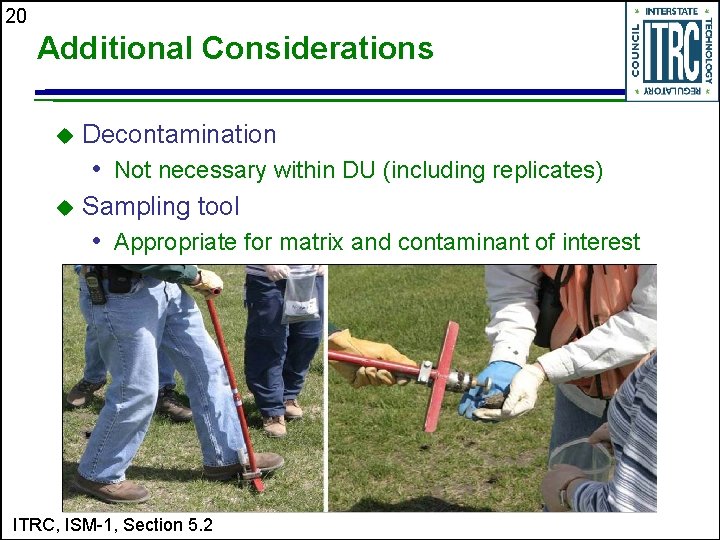
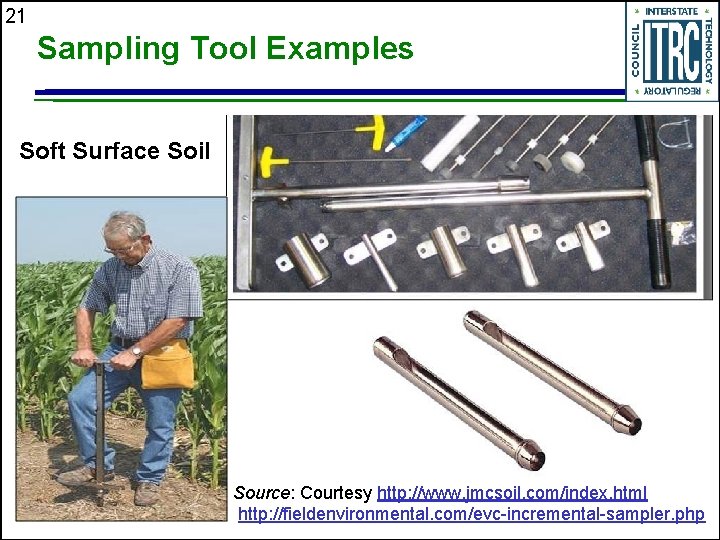
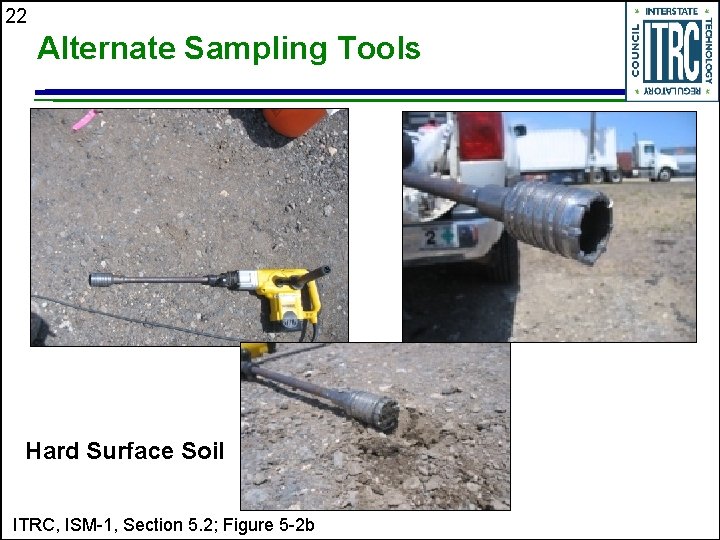
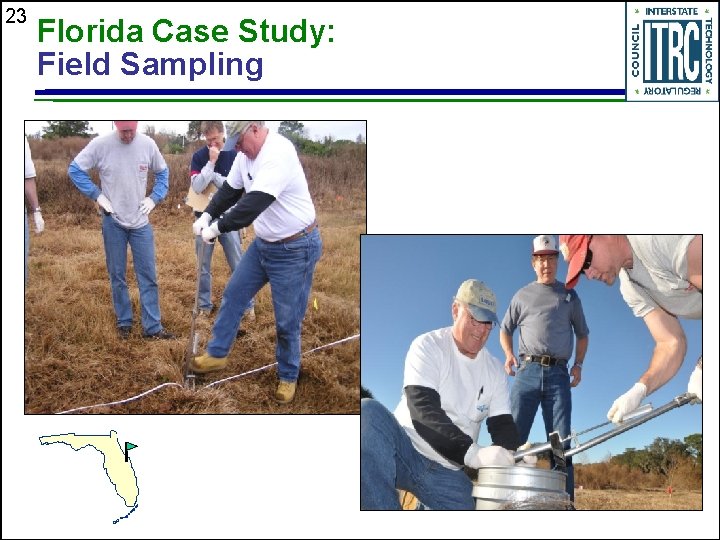
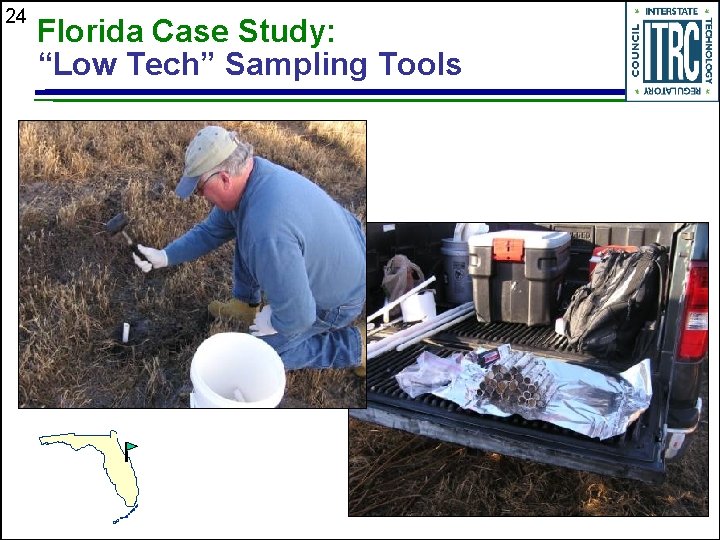
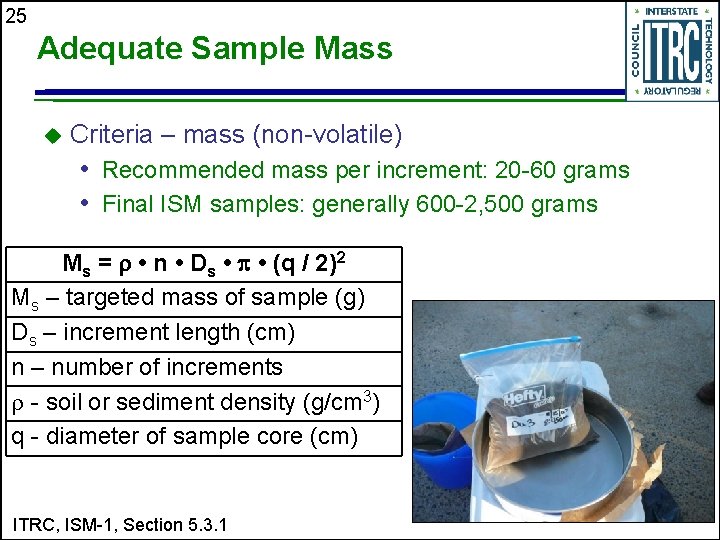
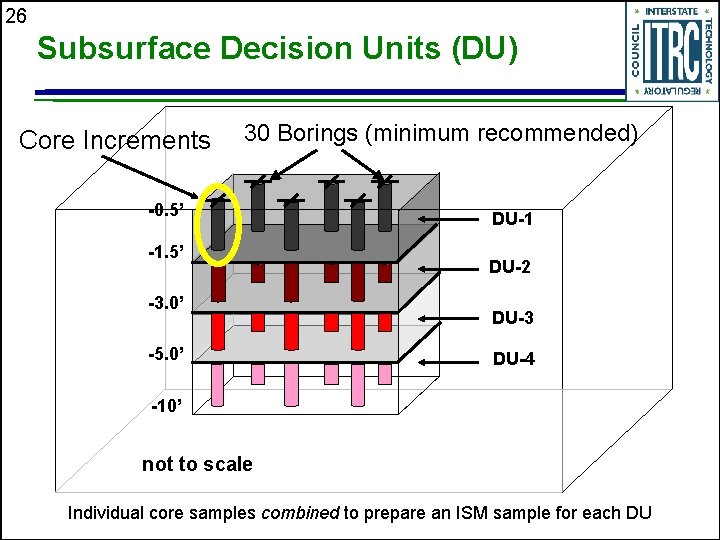
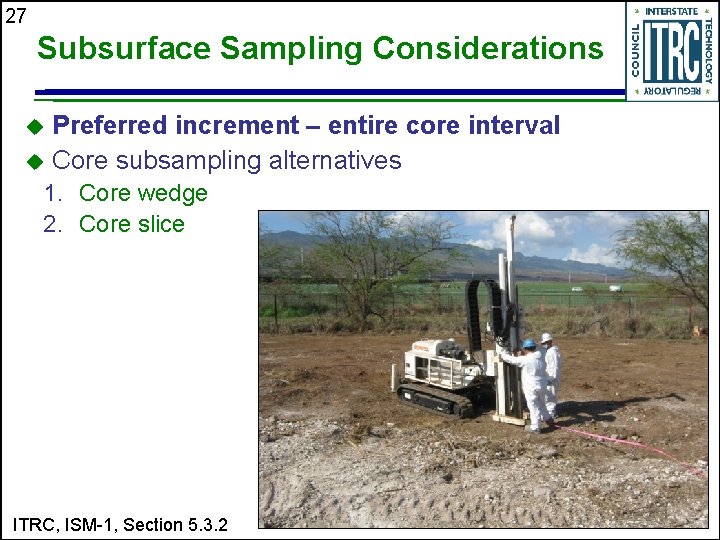
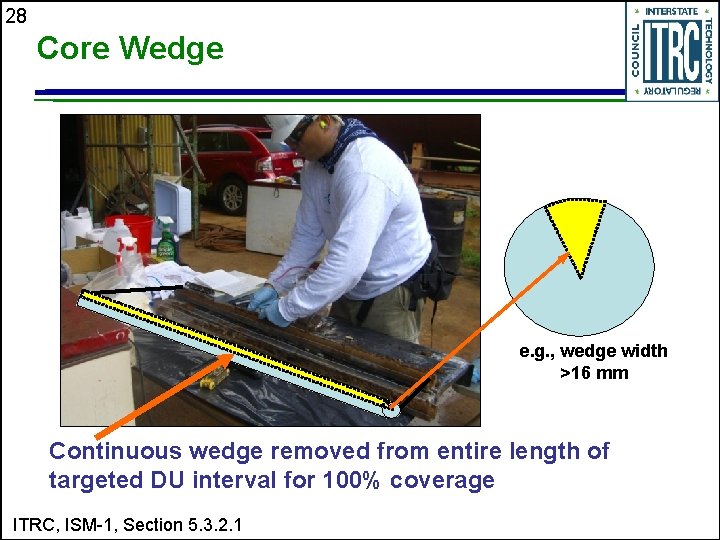
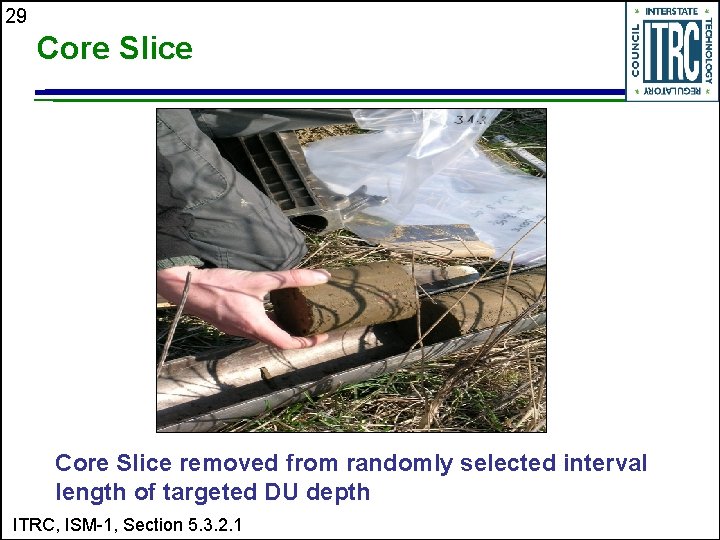
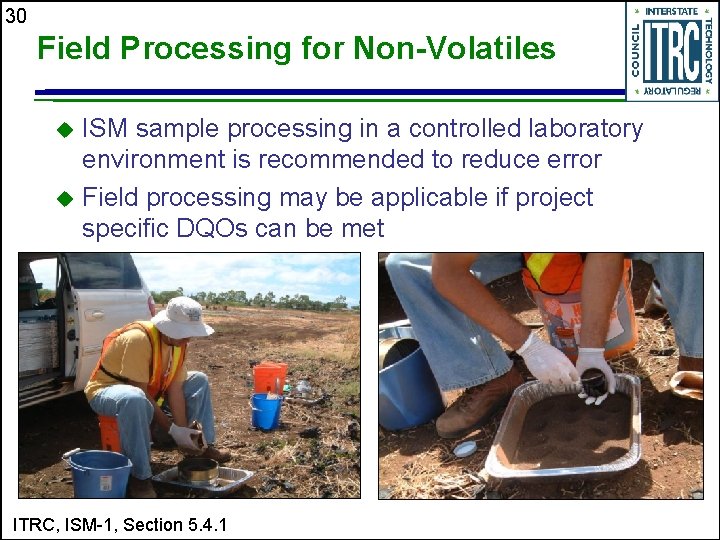
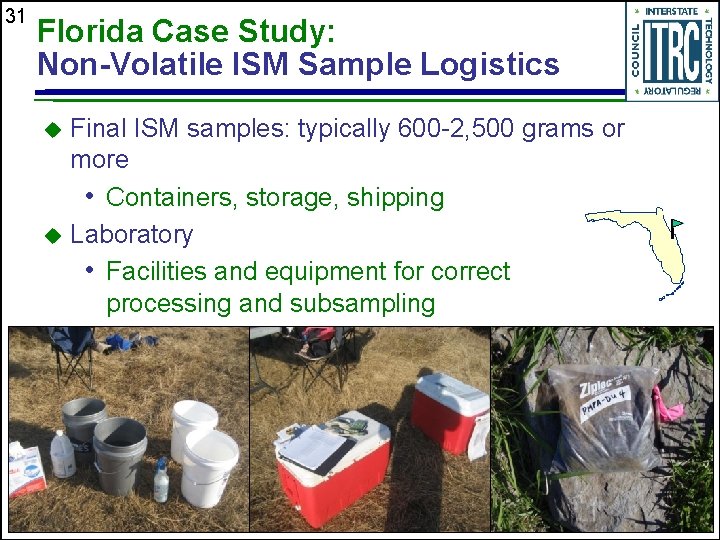
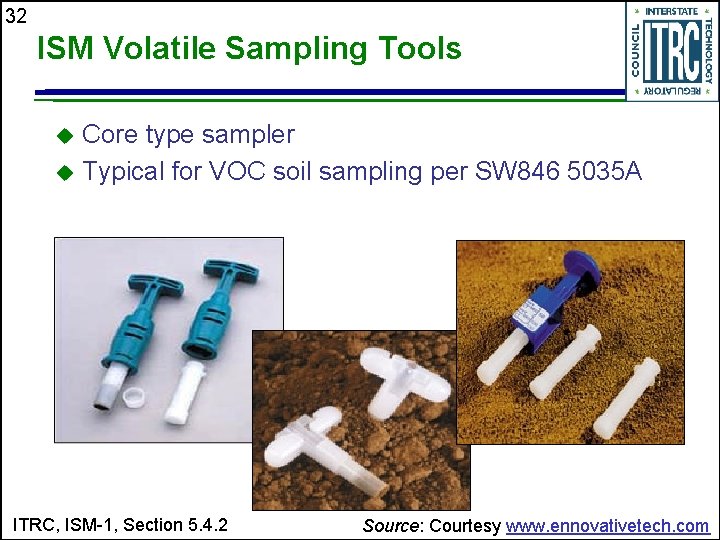
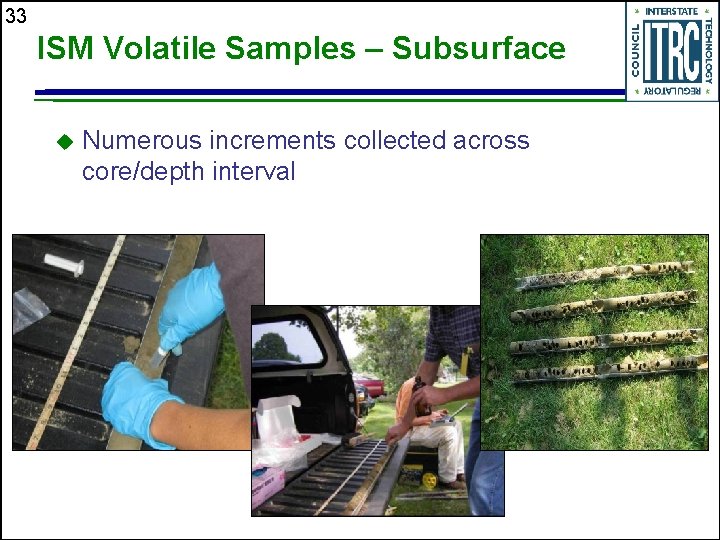
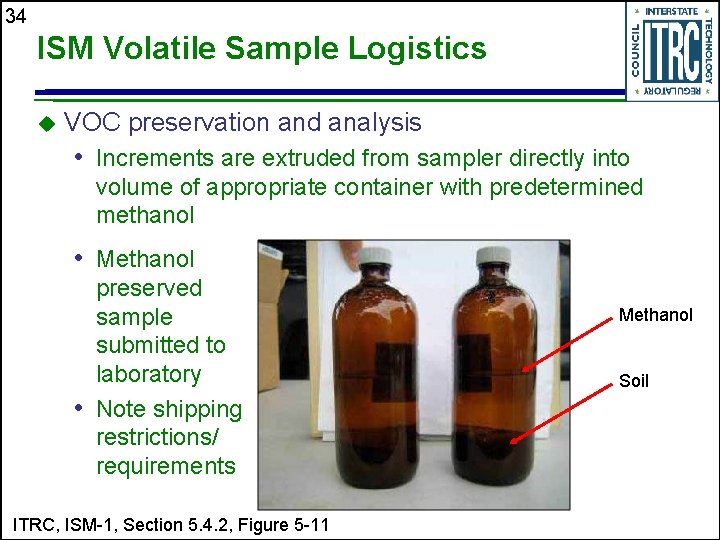
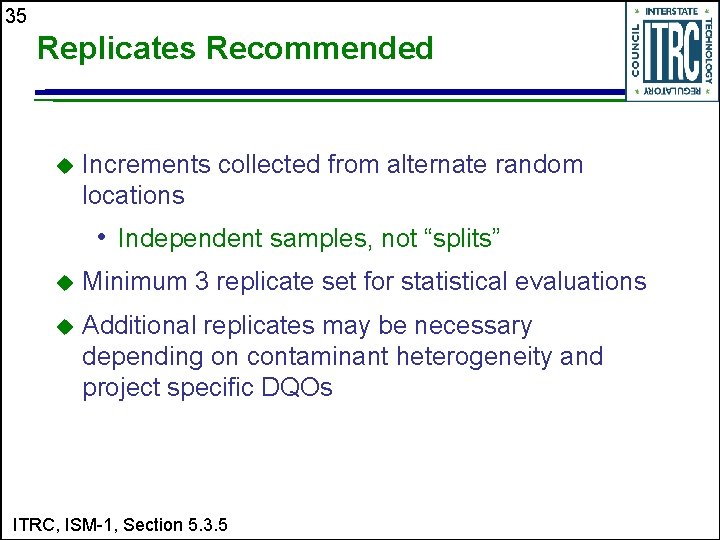
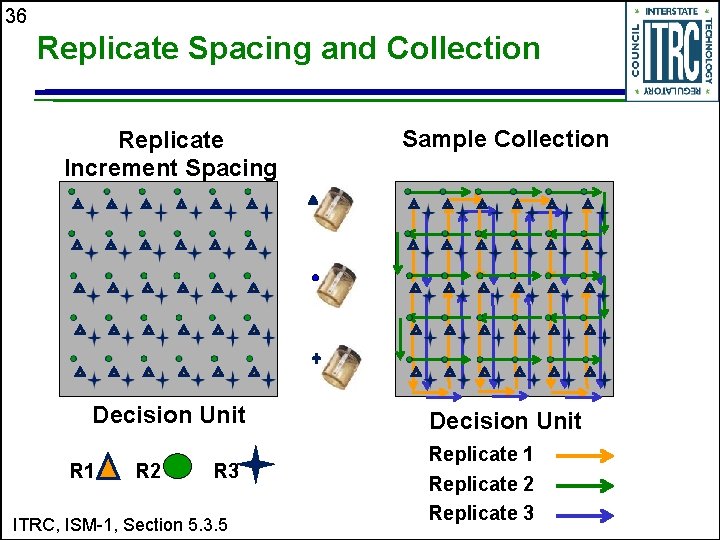
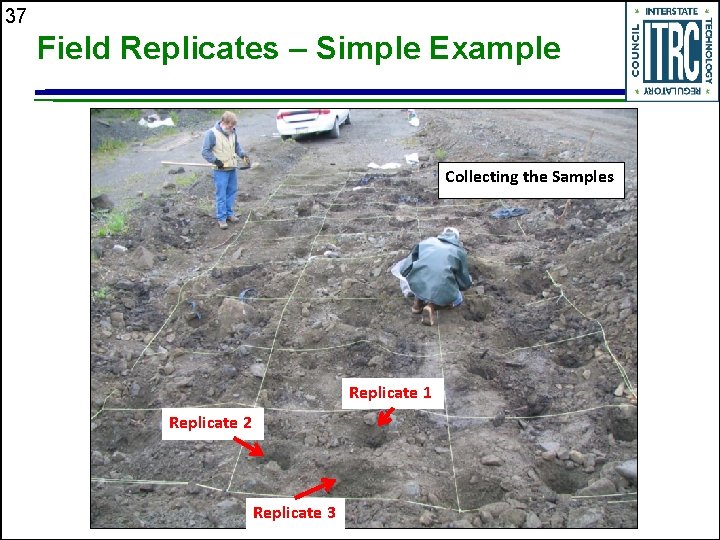
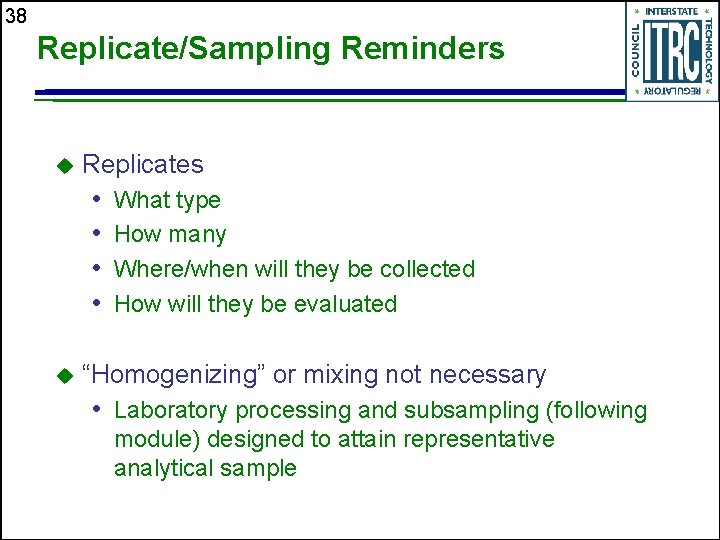
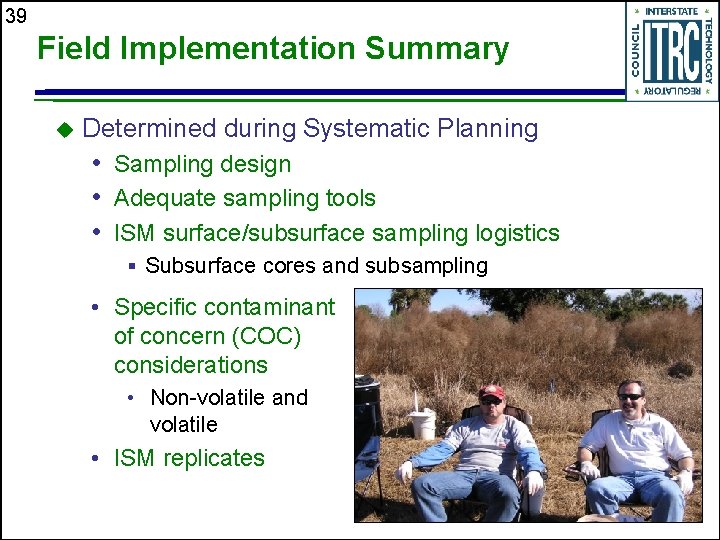
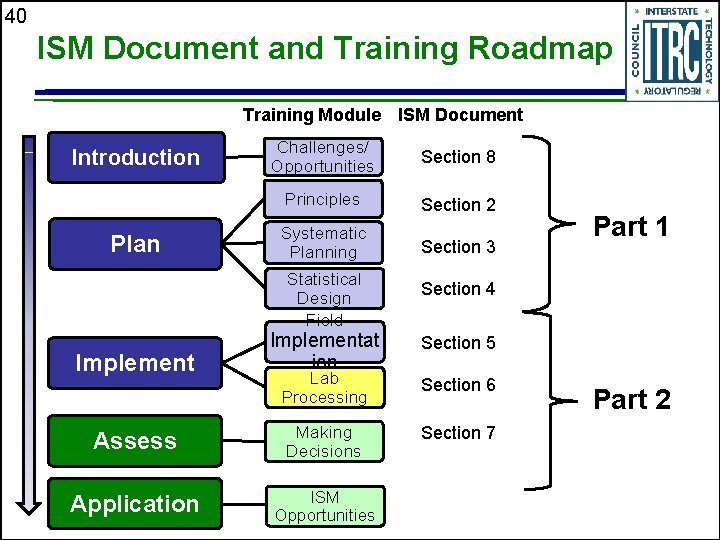
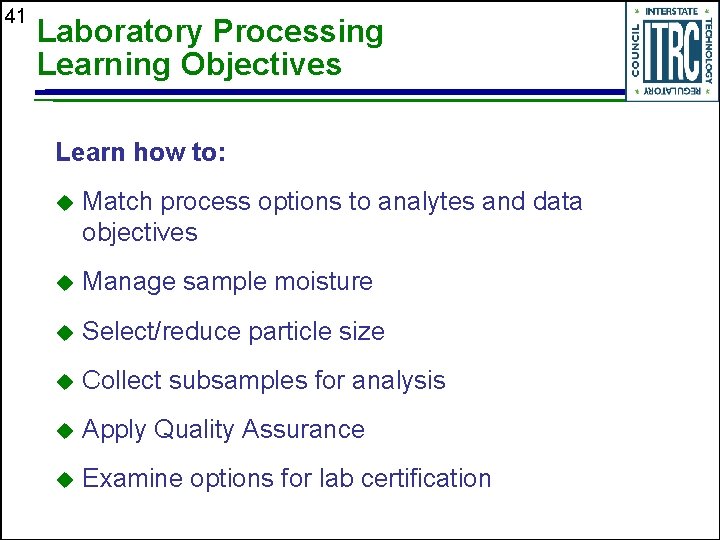
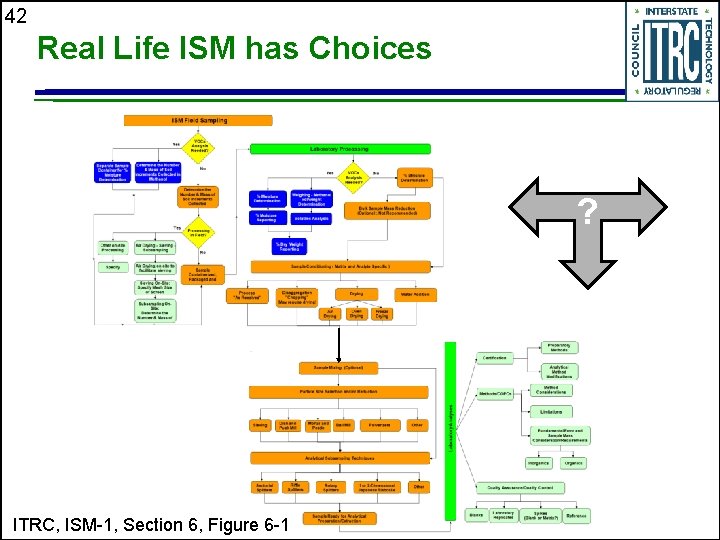
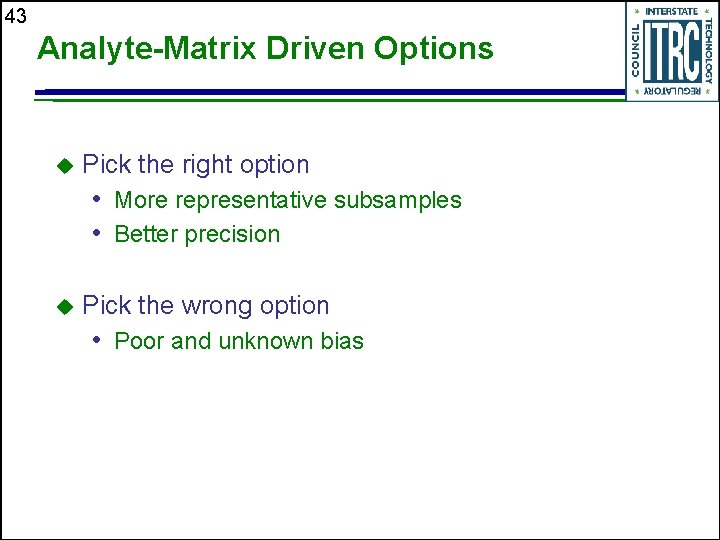
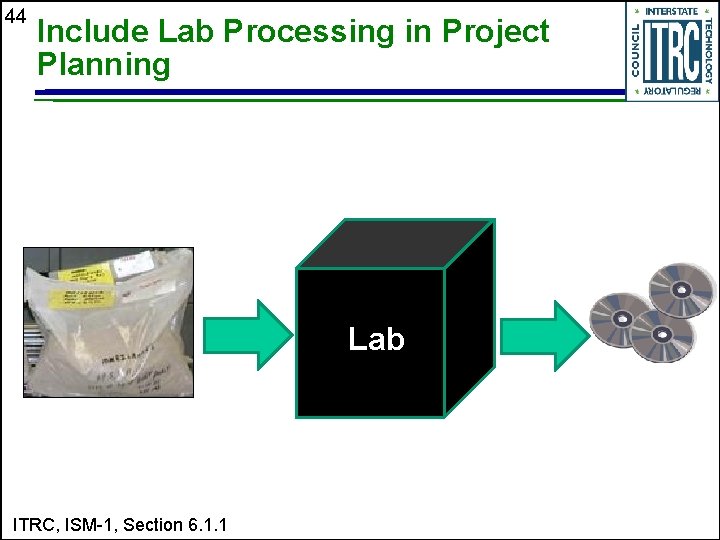
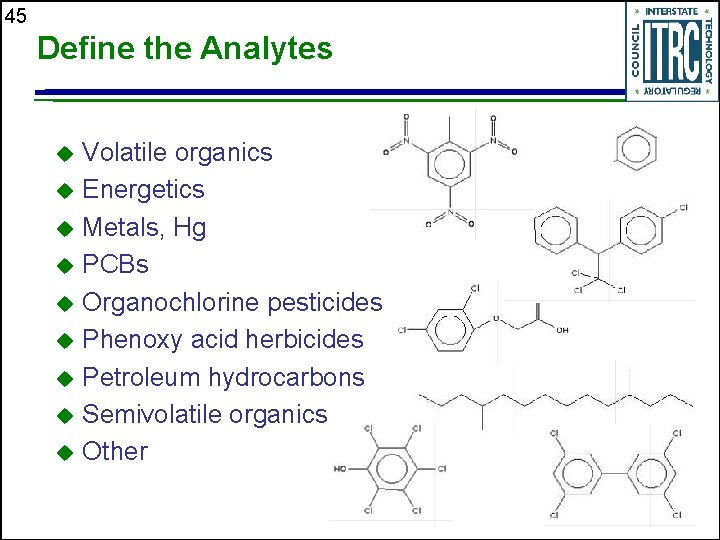
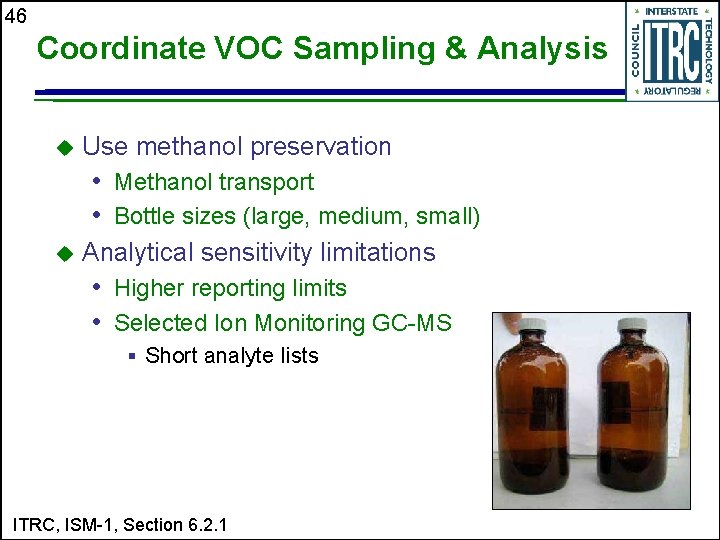
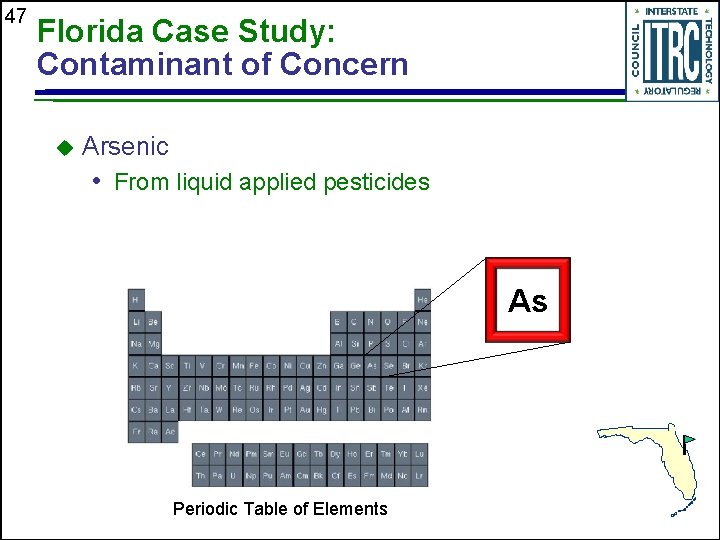
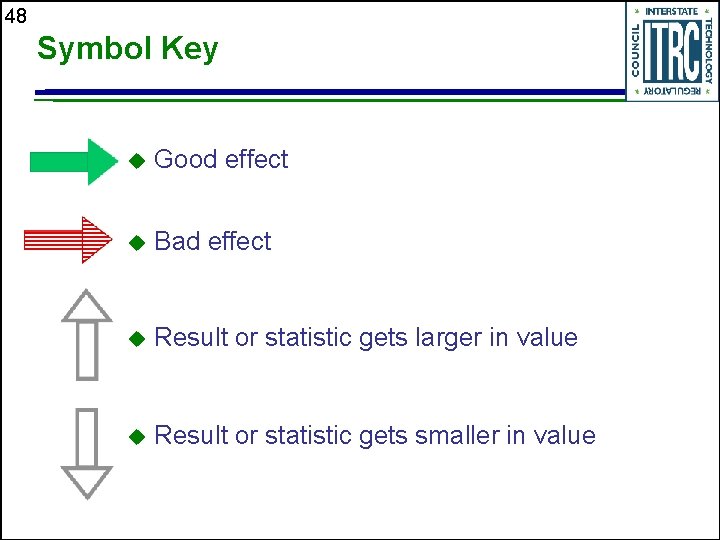
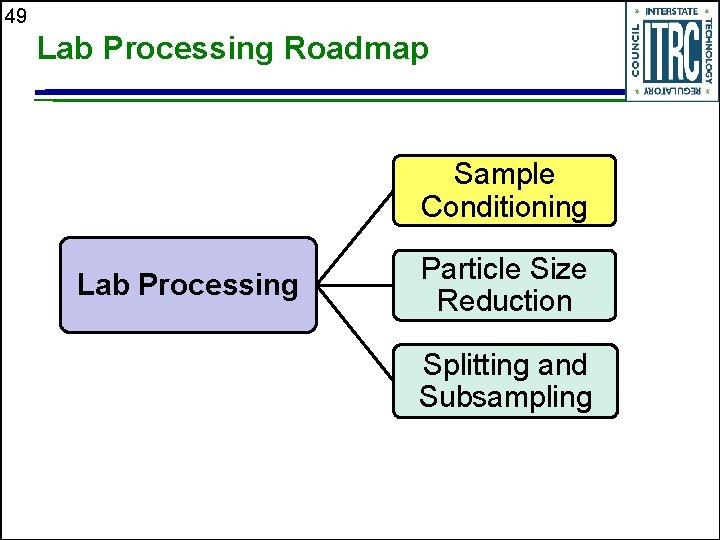
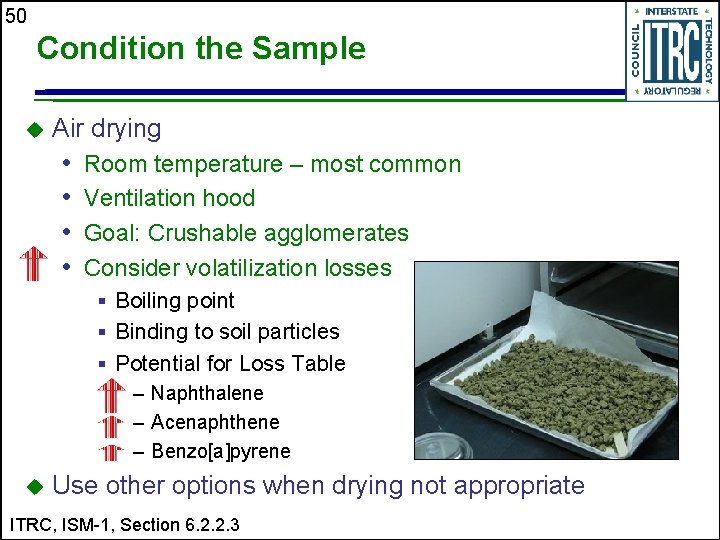
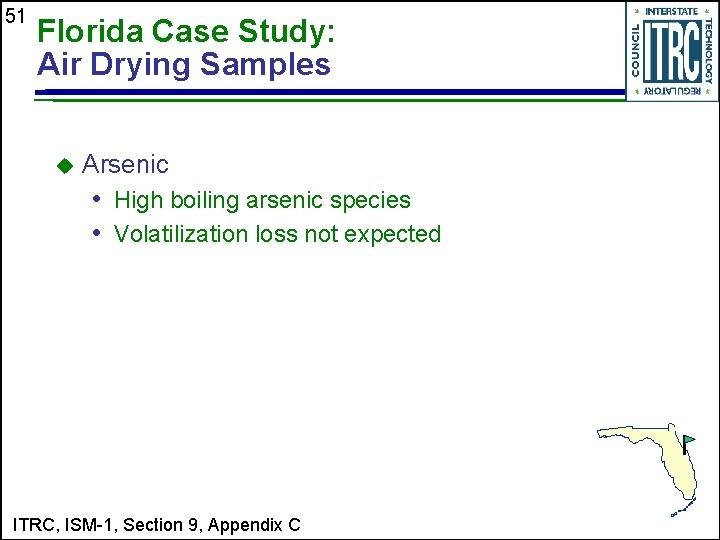
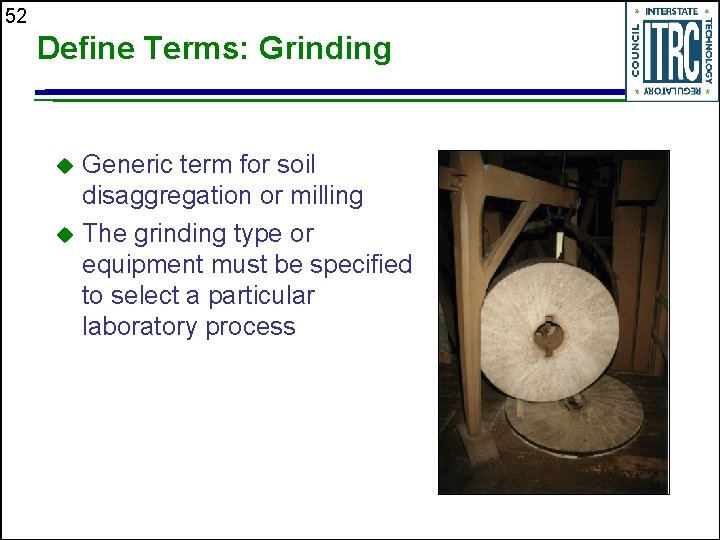
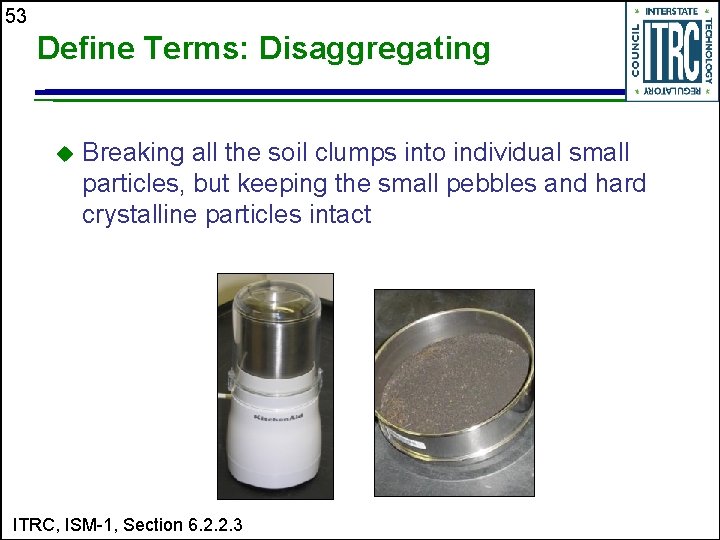
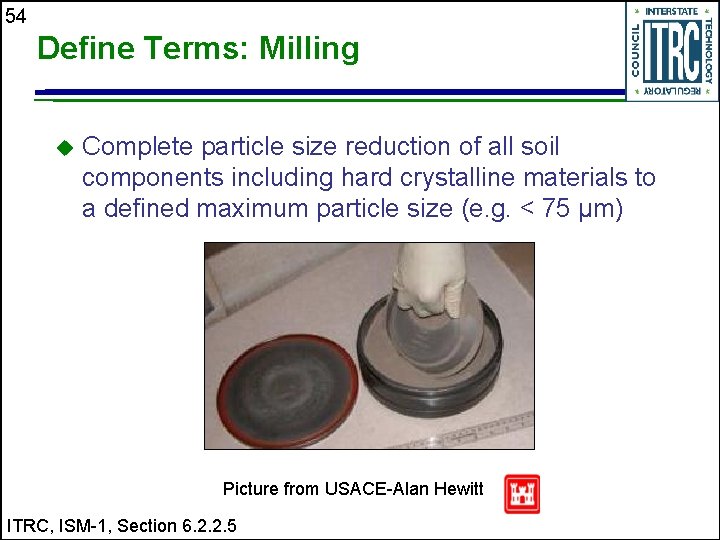
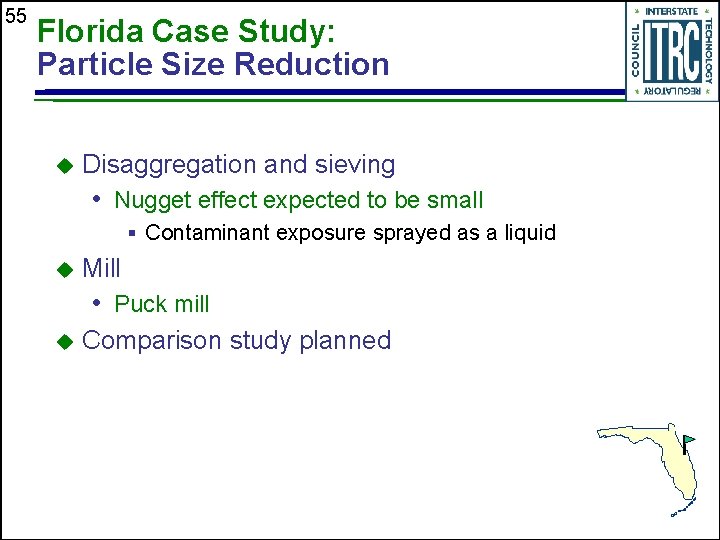
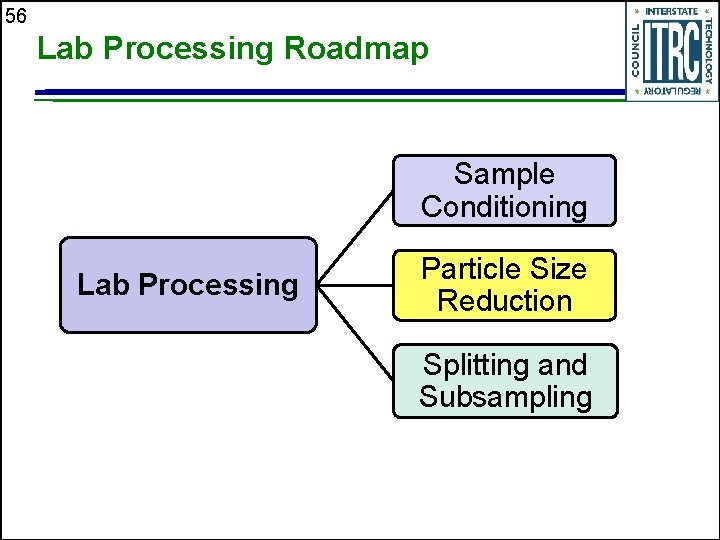
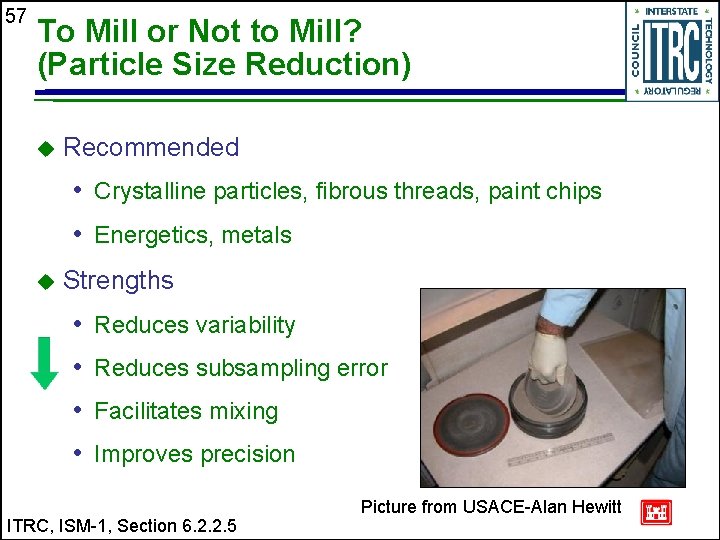
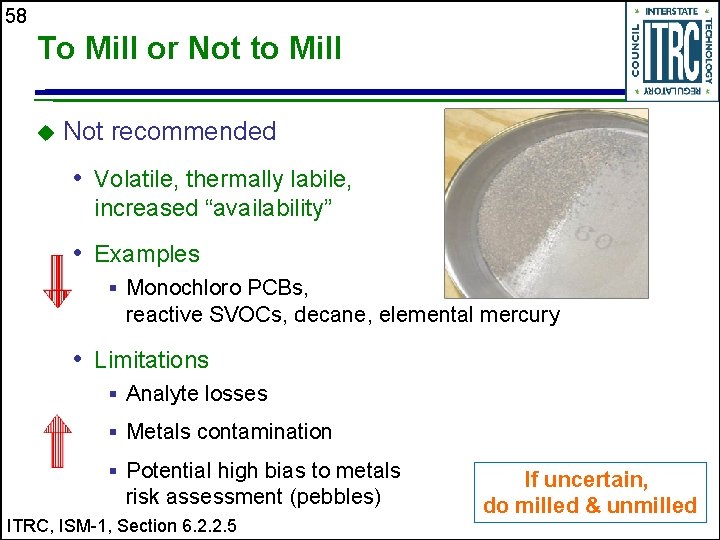
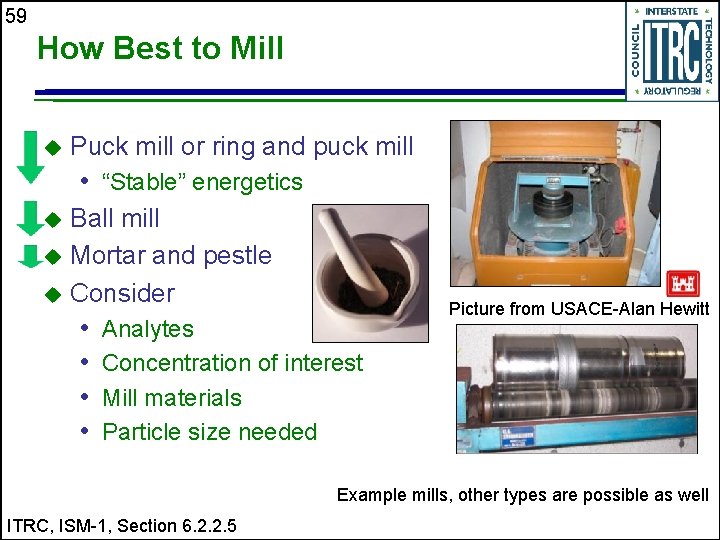
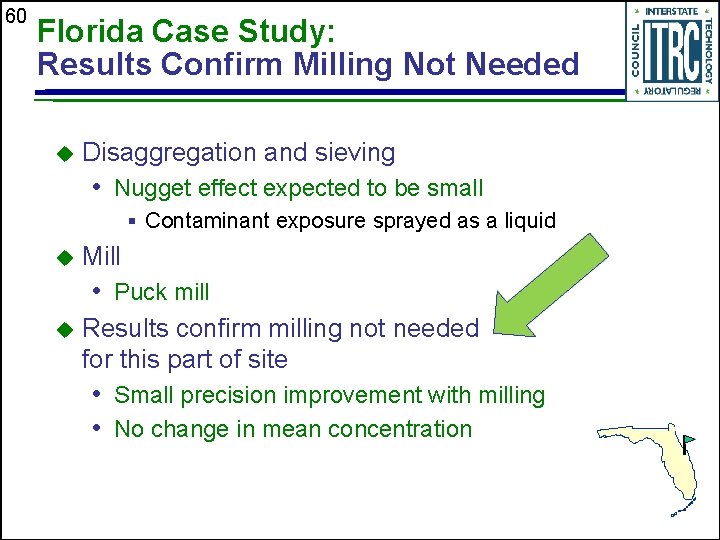
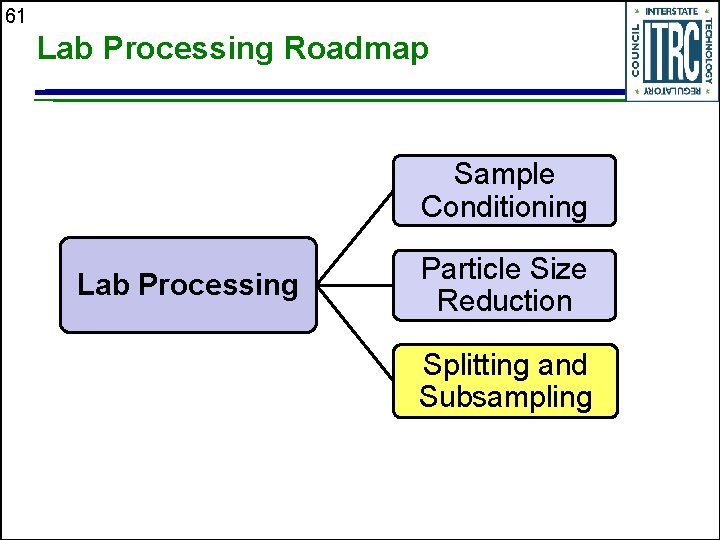
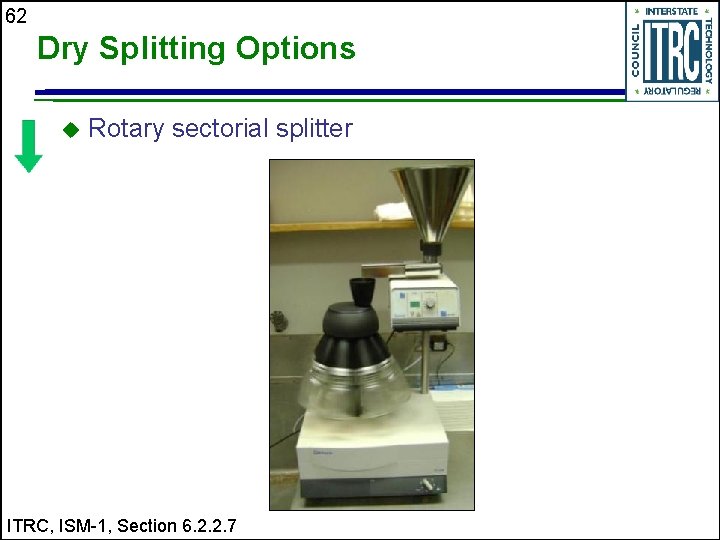
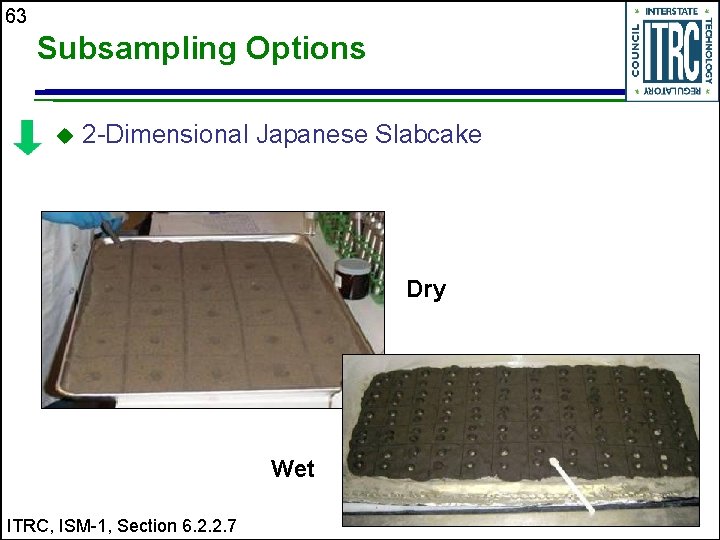
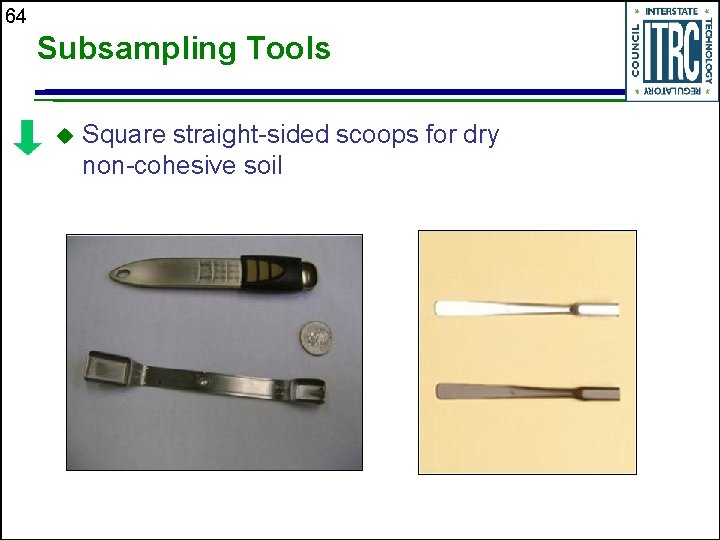
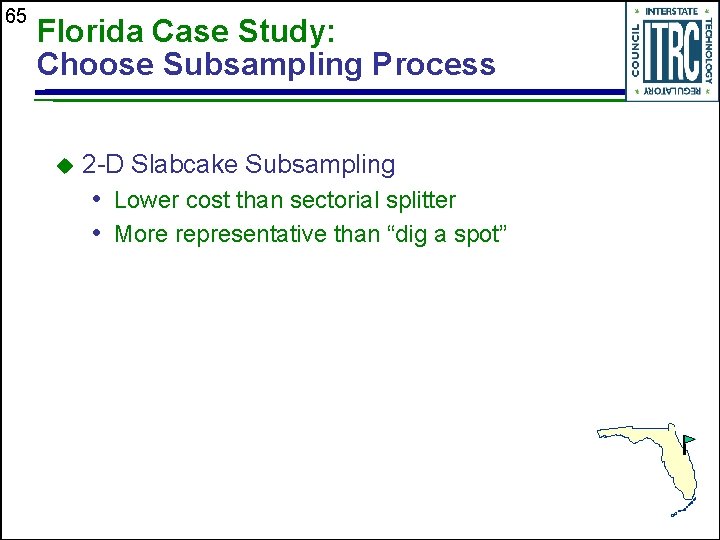
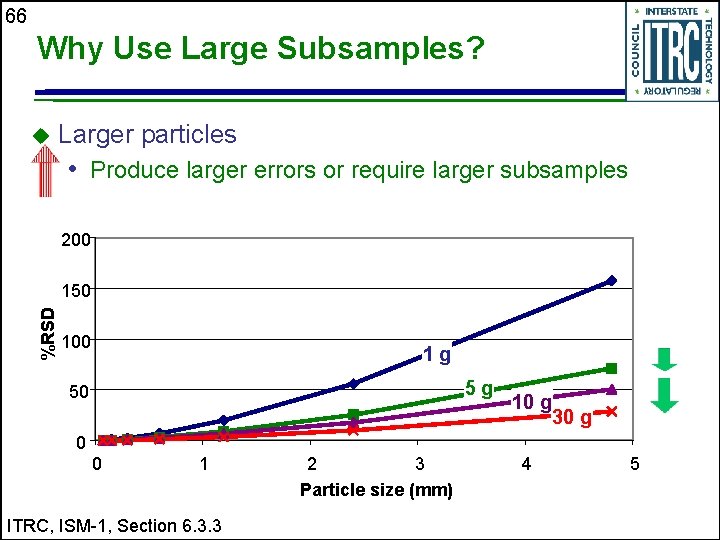
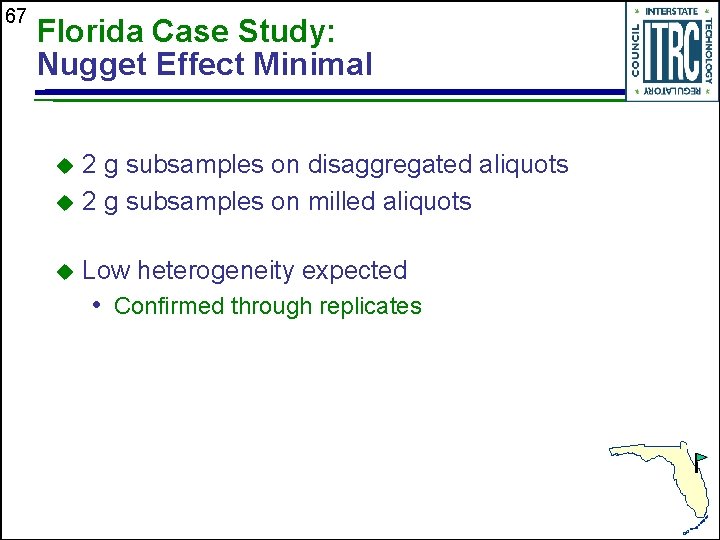
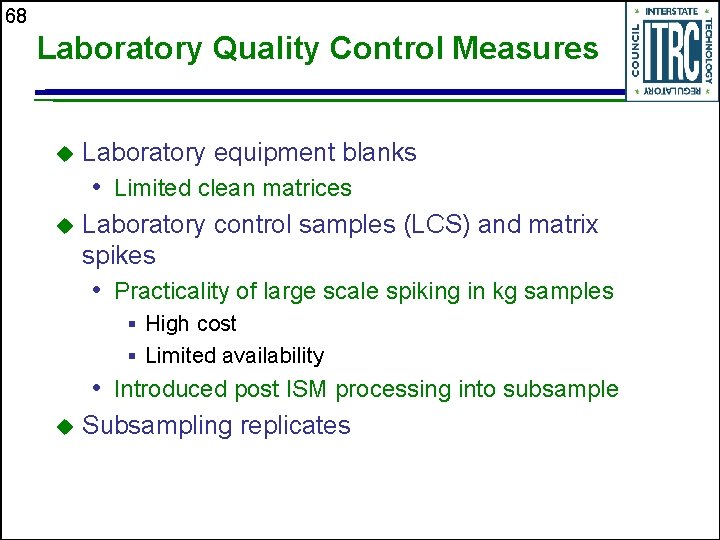
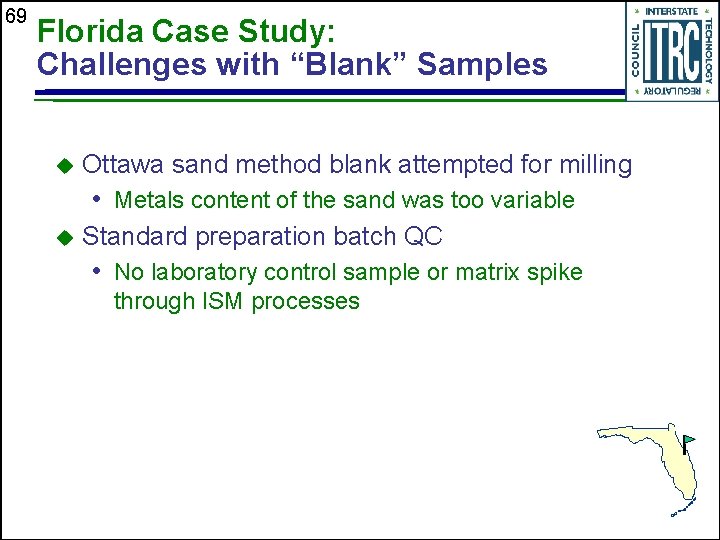
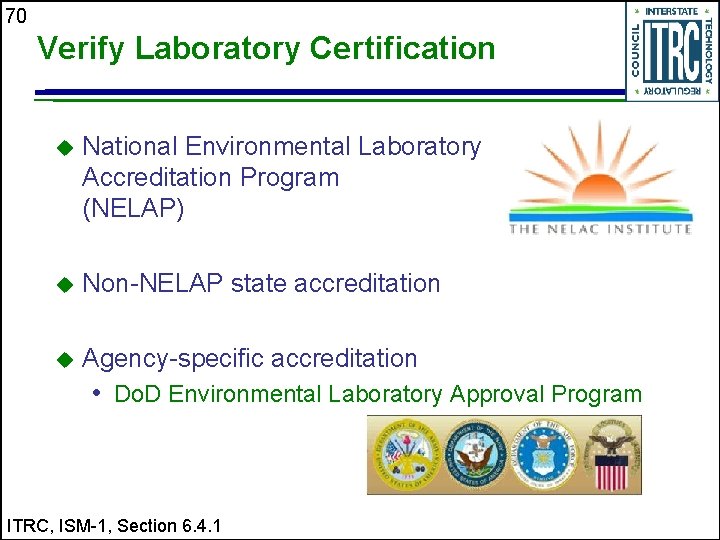
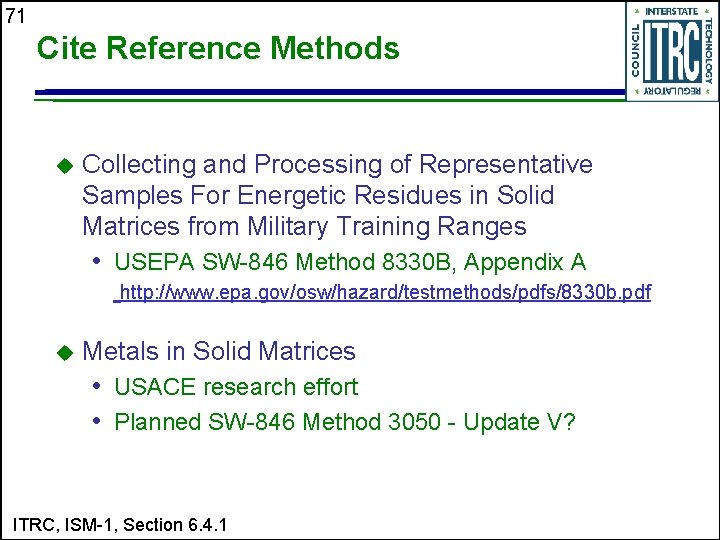
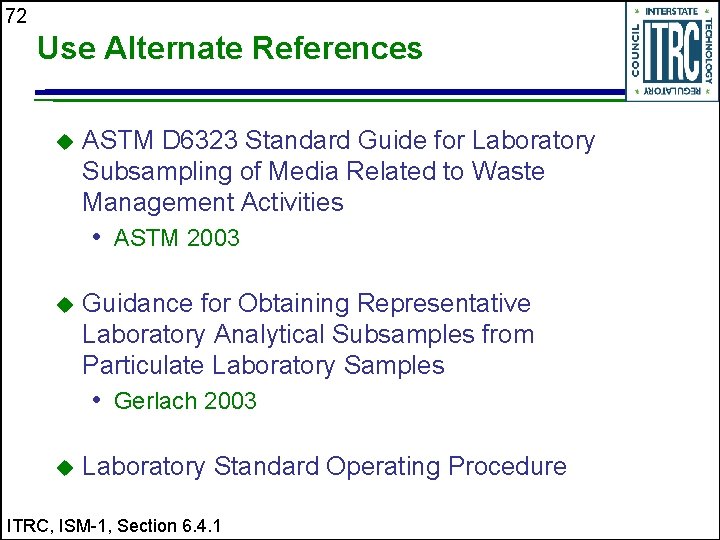
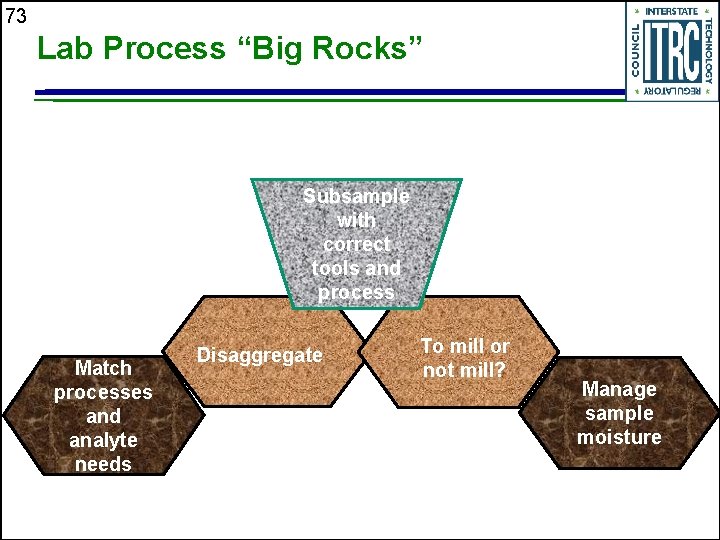
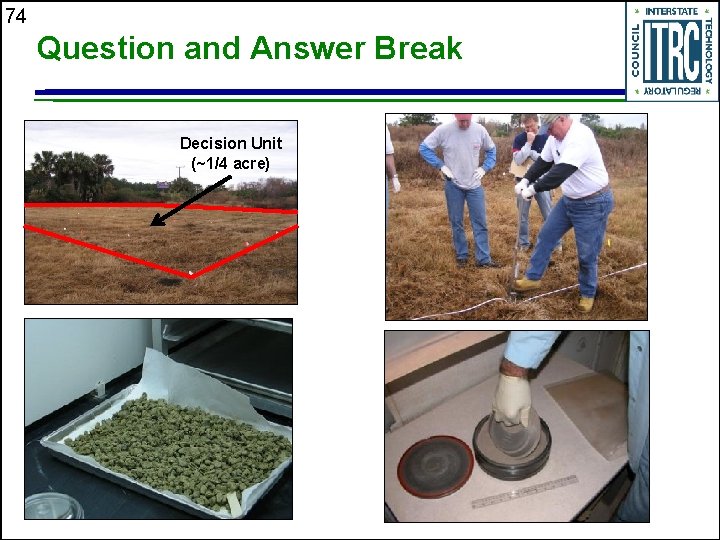
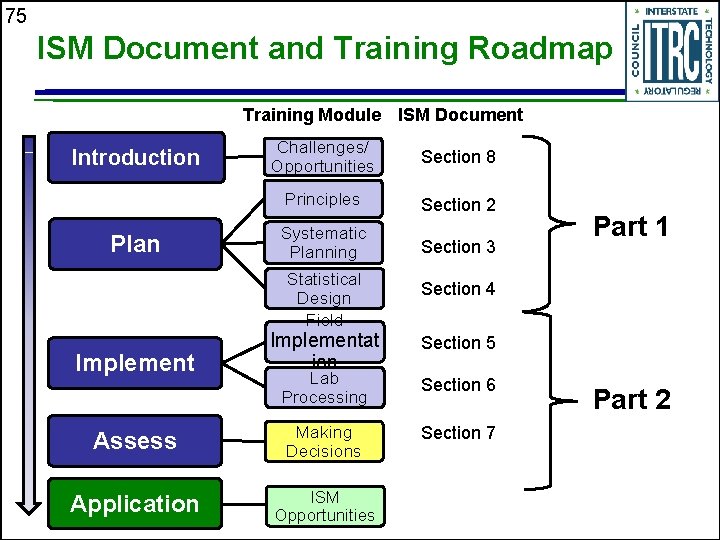
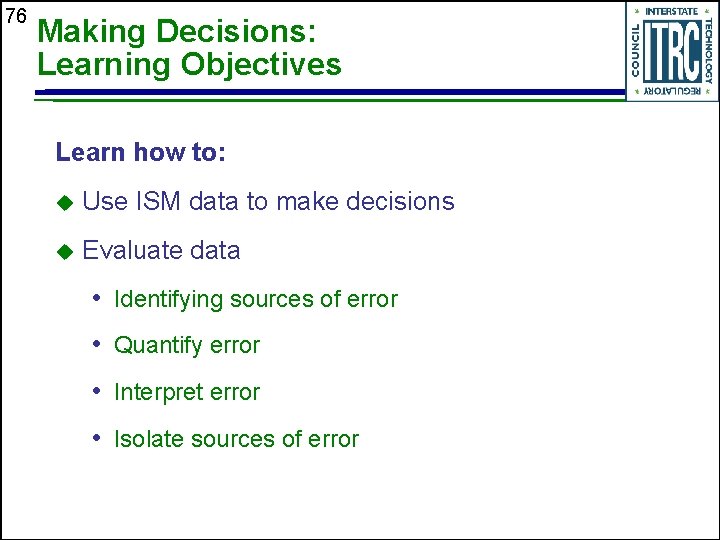
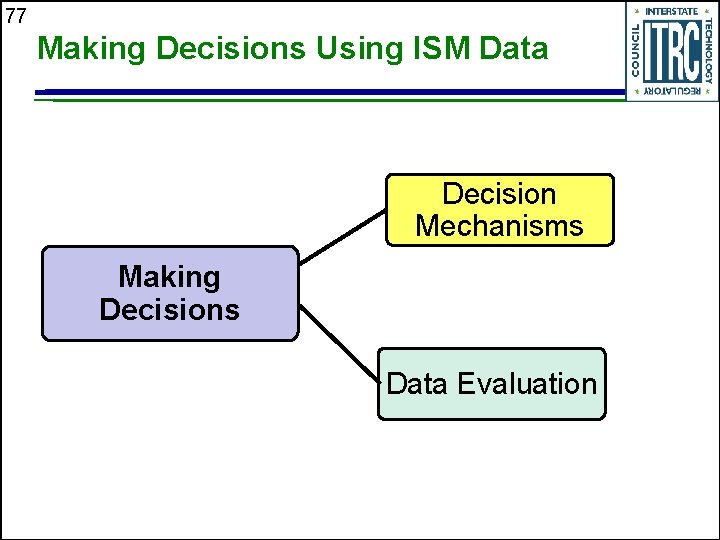
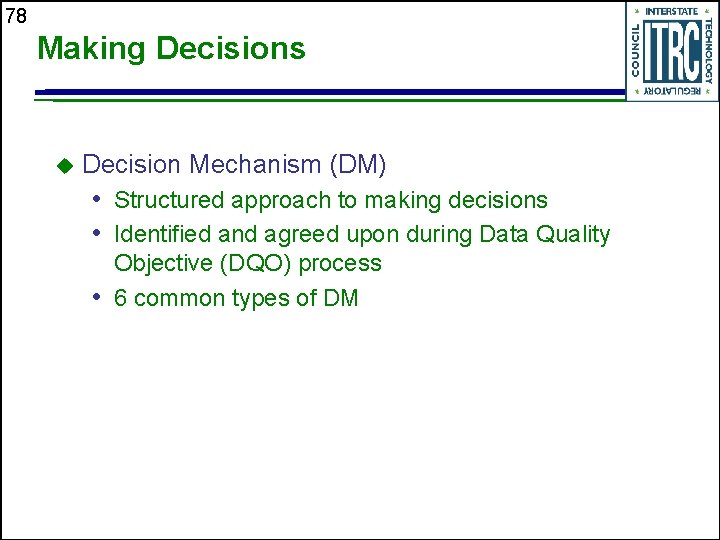
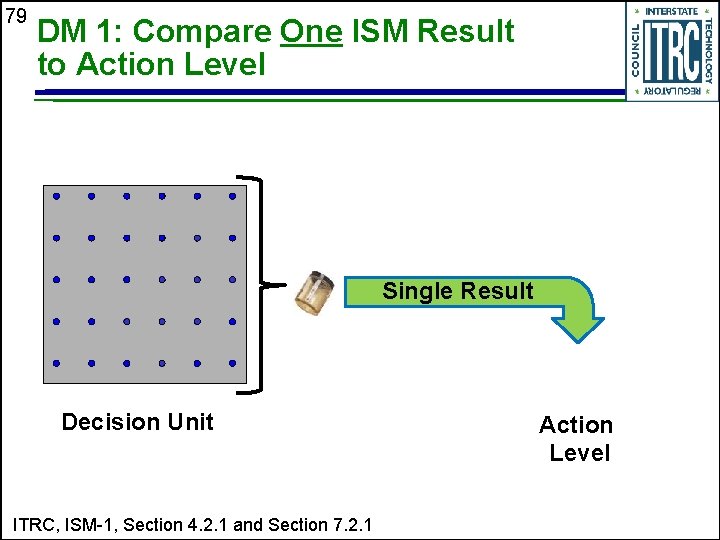
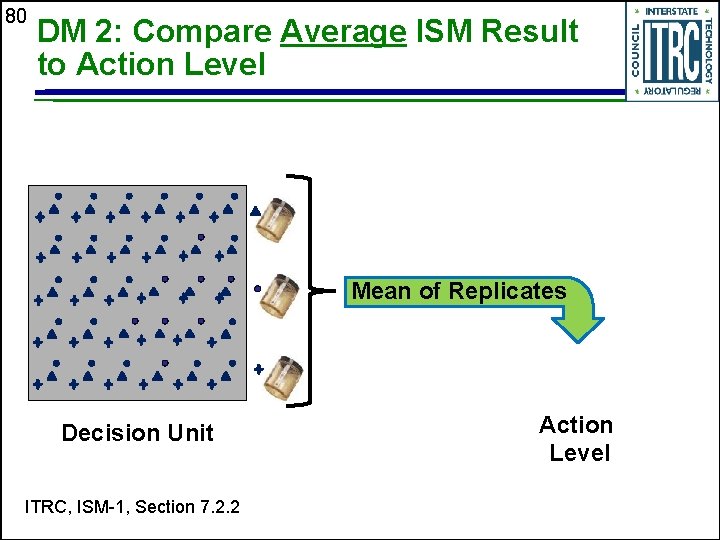
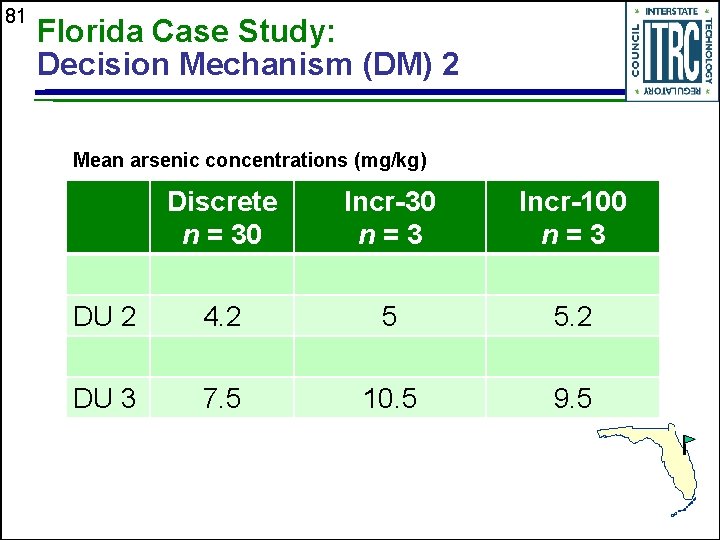
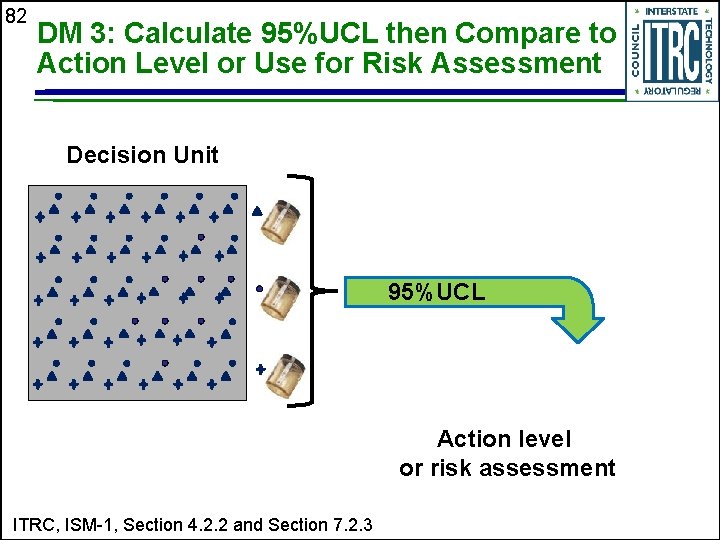
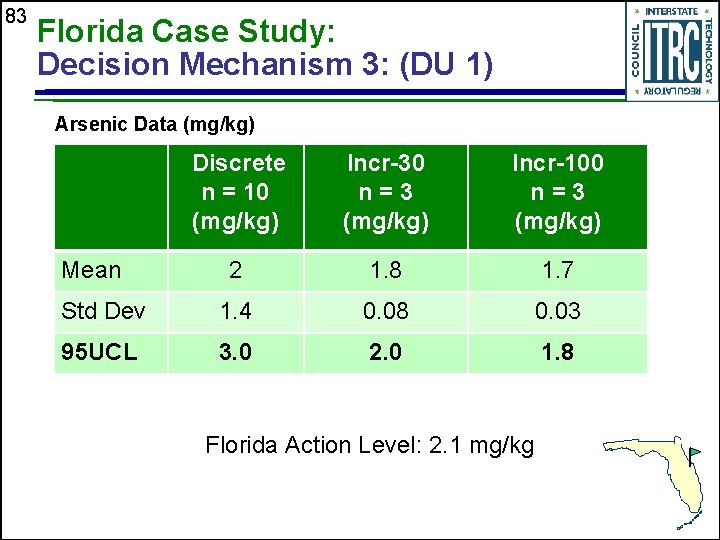
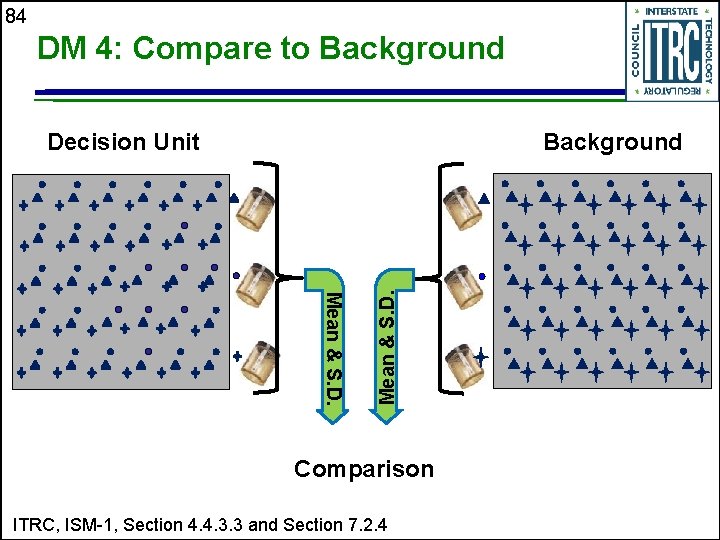
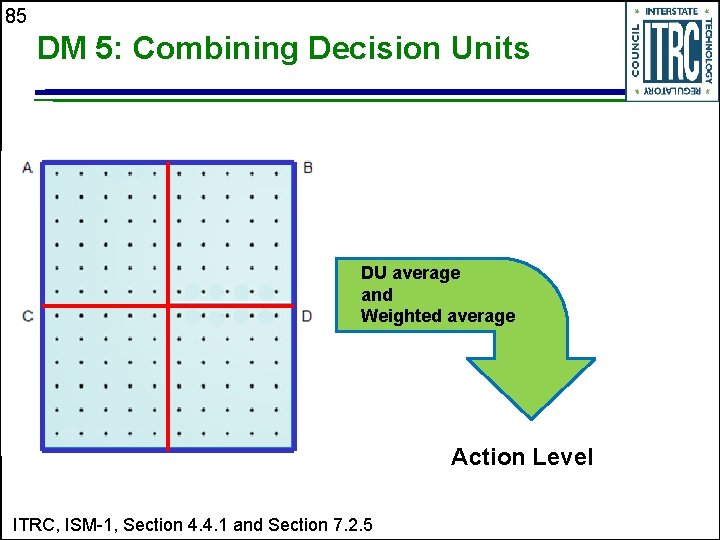
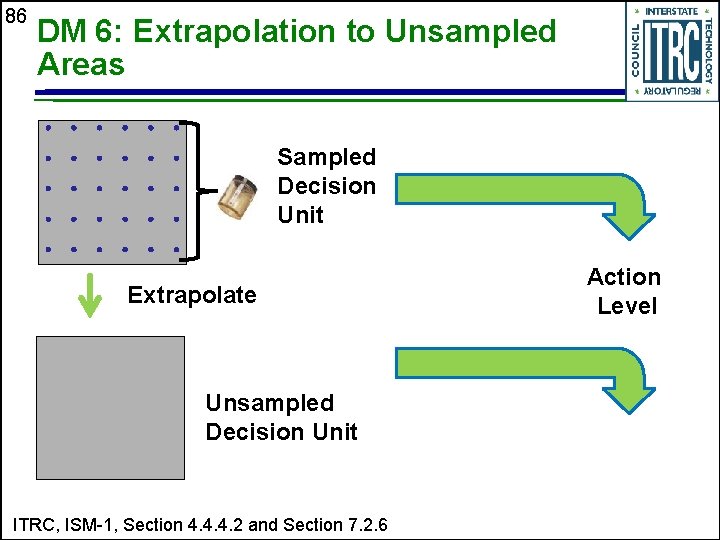
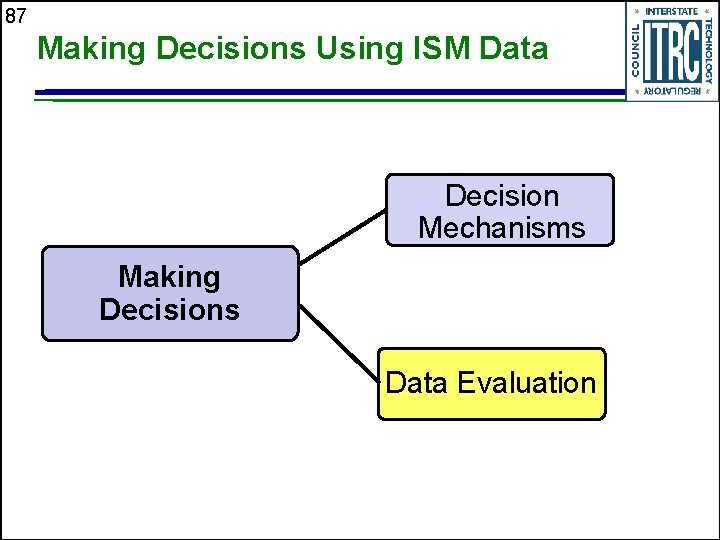
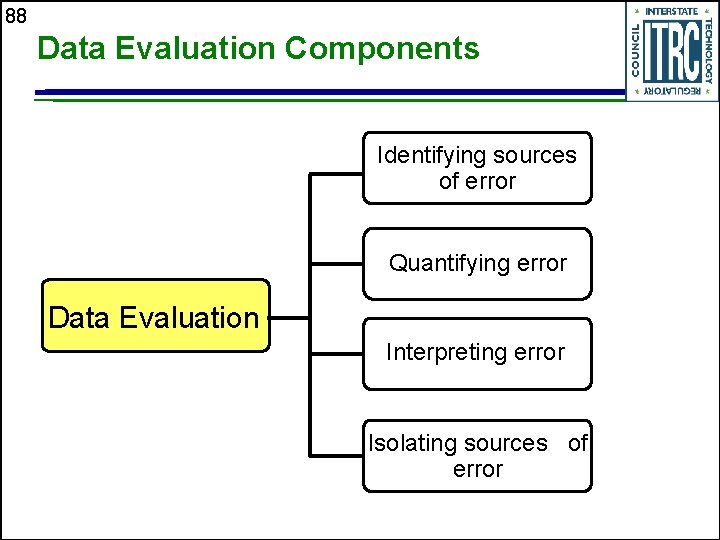
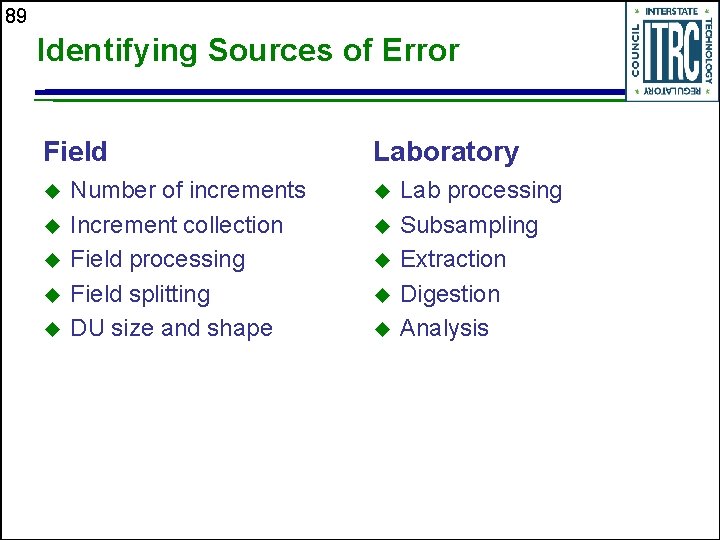
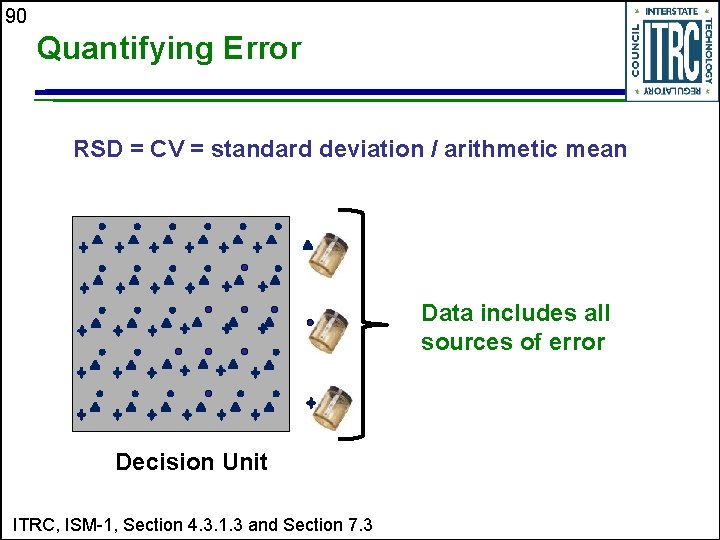
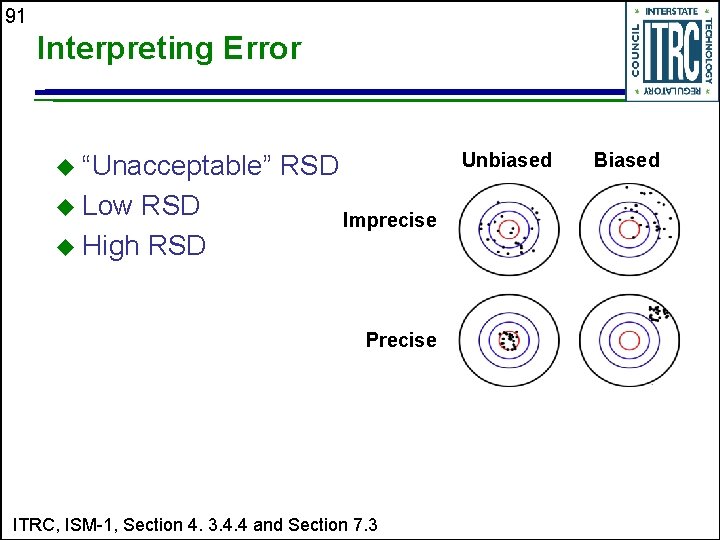
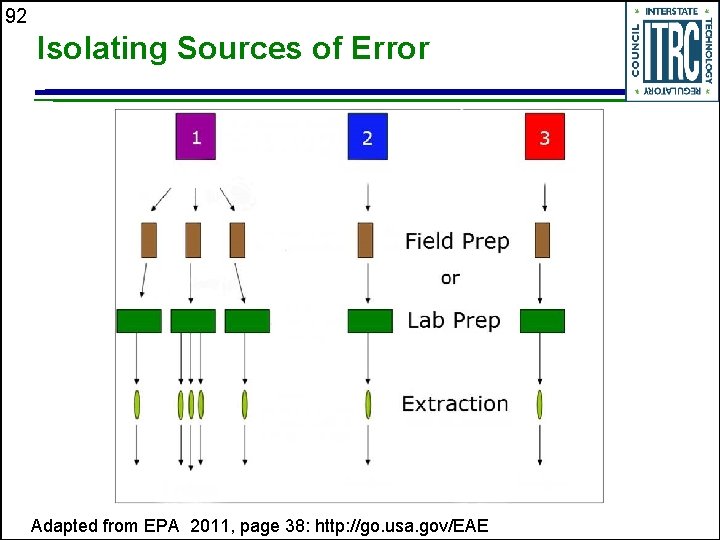
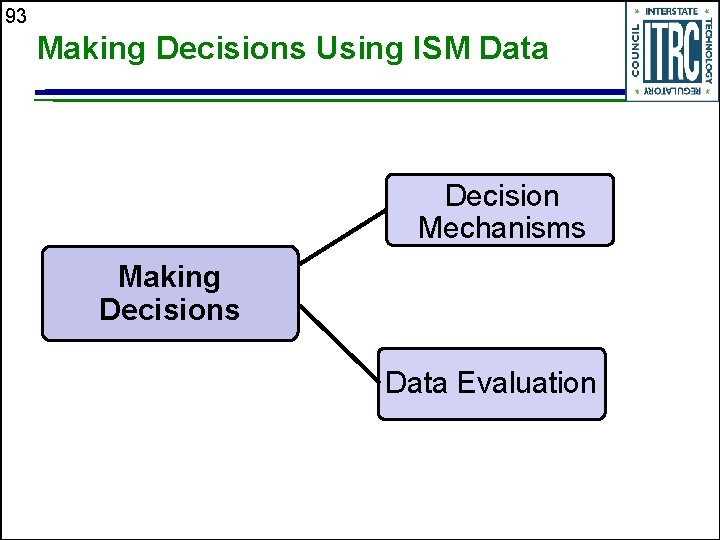
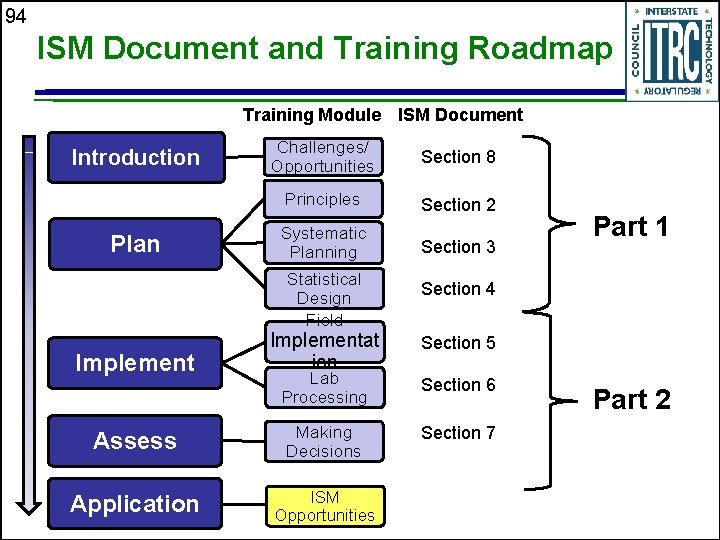
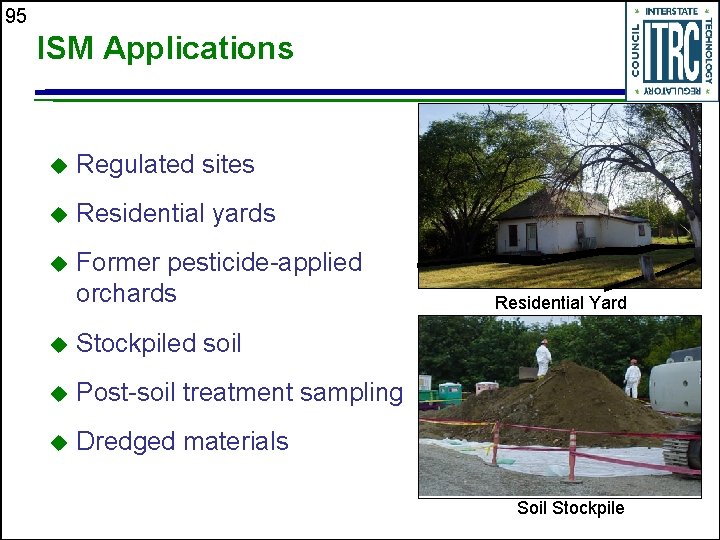
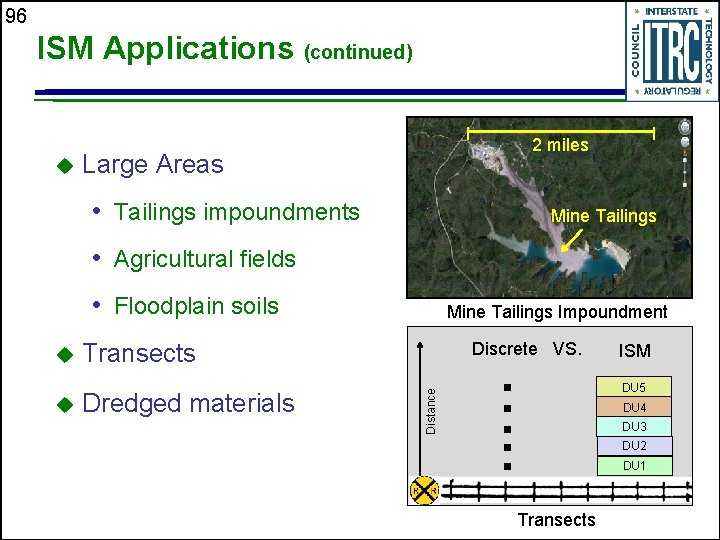
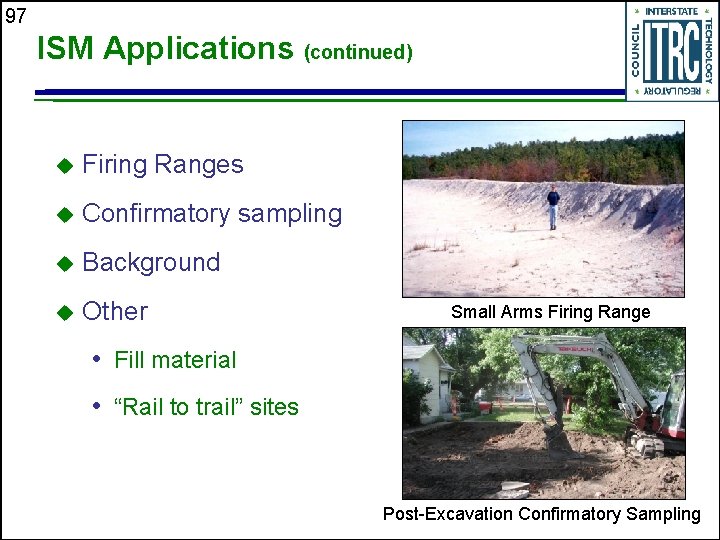
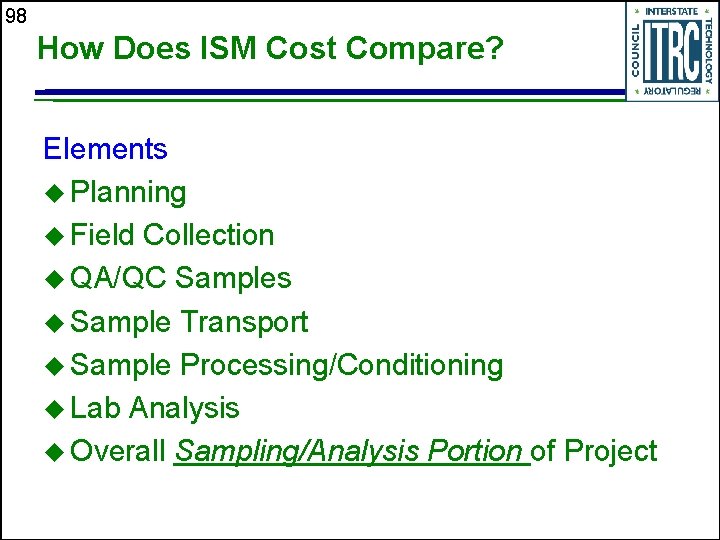
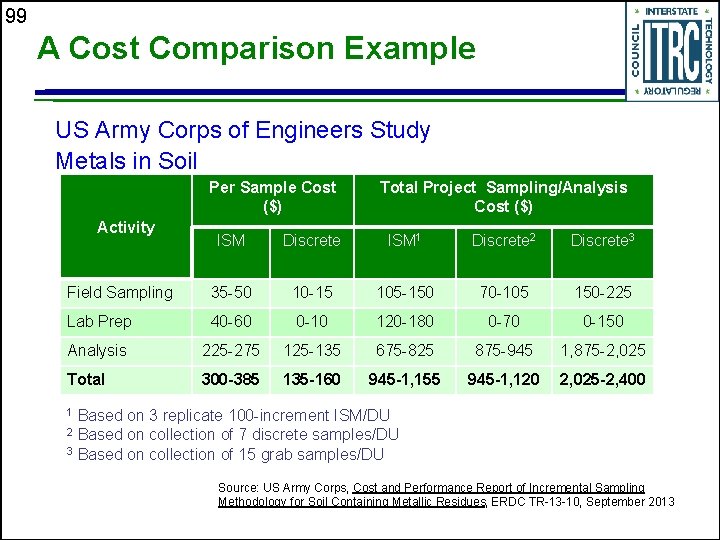
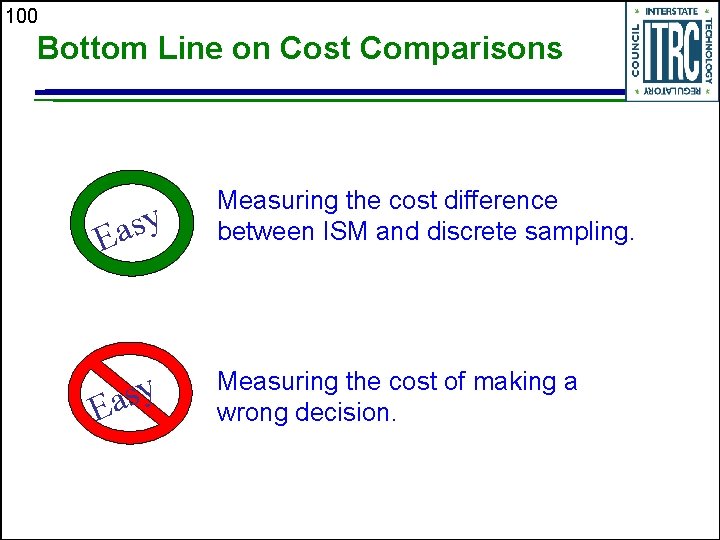
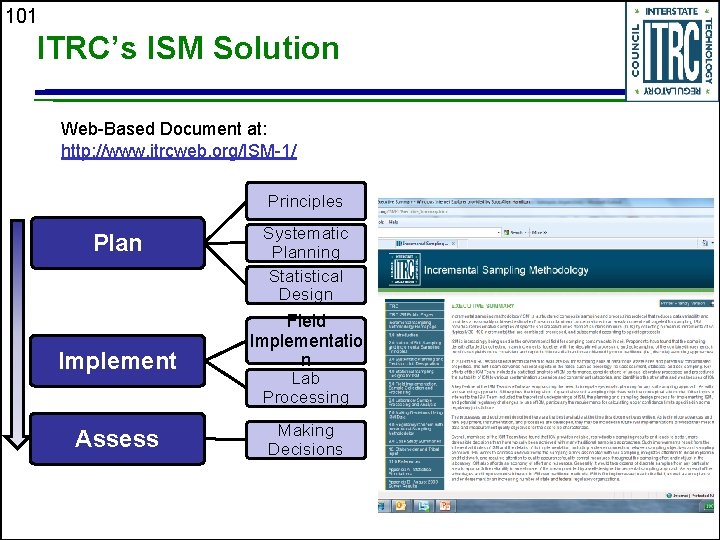
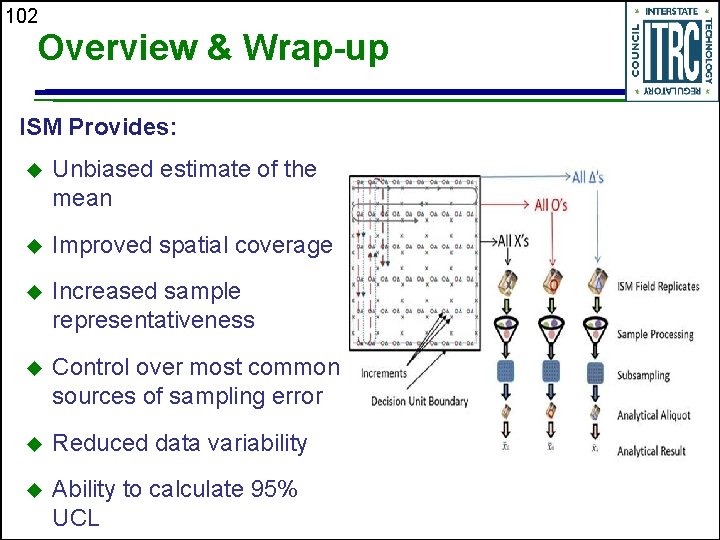
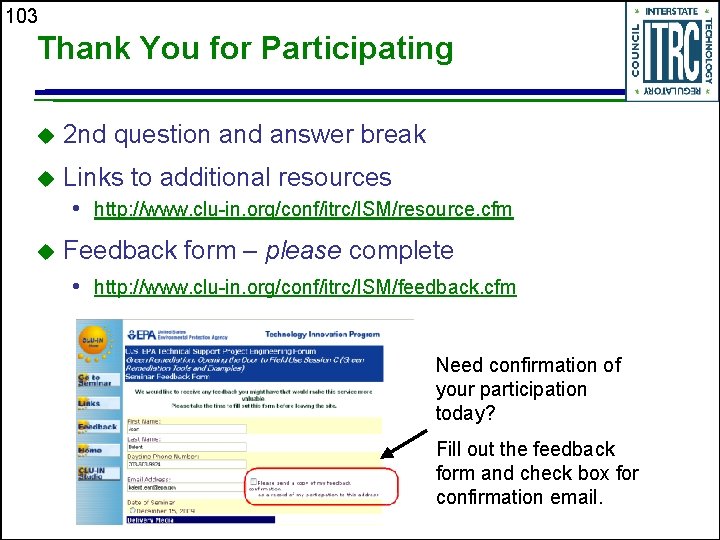
- Slides: 103
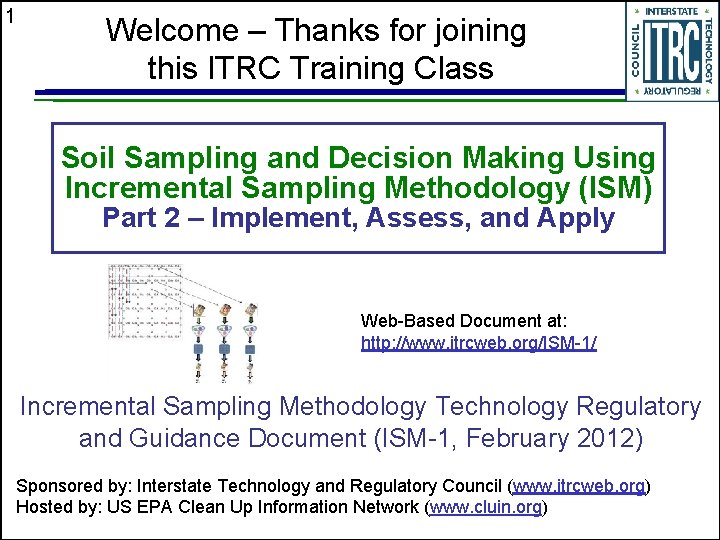
1 Welcome – Thanks for joining this ITRC Training Class Soil Sampling and Decision Making Using Incremental Sampling Methodology (ISM) Part 2 – Implement, Assess, and Apply Web-Based Document at: http: //www. itrcweb. org/ISM-1/ Incremental Sampling Methodology Technology Regulatory and Guidance Document (ISM-1, February 2012) Sponsored by: Interstate Technology and Regulatory Council (www. itrcweb. org) Hosted by: US EPA Clean Up Information Network (www. cluin. org)
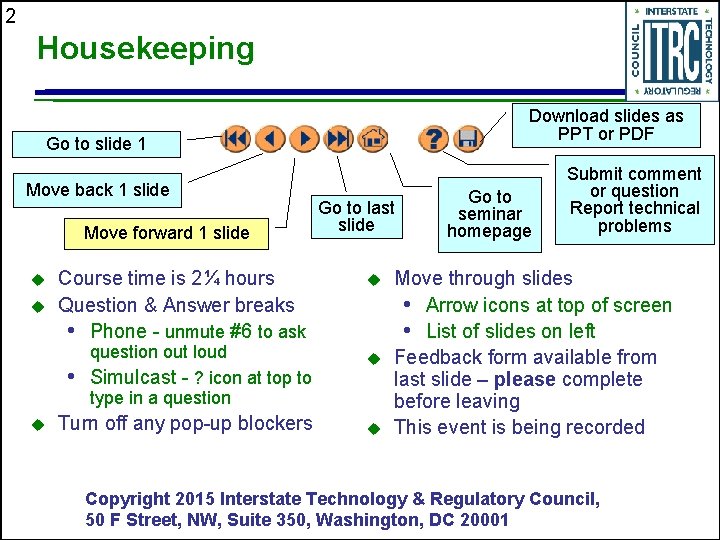
2 Housekeeping Download slides as PPT or PDF Go to slide 1 Move back 1 slide Move forward 1 slide u u Course time is 2¼ hours Question & Answer breaks • Phone - unmute #6 to ask • u question out loud Simulcast - ? icon at top to type in a question Turn off any pop-up blockers Go to last slide u u u Go to seminar homepage Submit comment or question Report technical problems Move through slides • Arrow icons at top of screen • List of slides on left Feedback form available from last slide – please complete before leaving This event is being recorded Copyright 2015 Interstate Technology & Regulatory Council, 50 F Street, NW, Suite 350, Washington, DC 20001
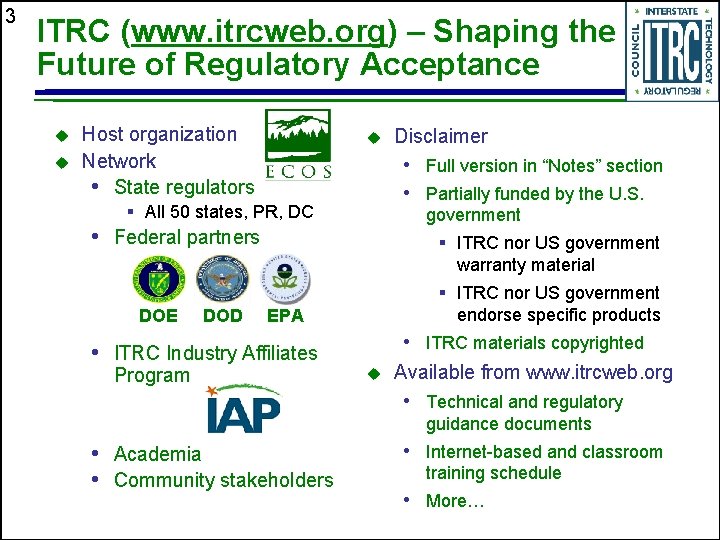
3 ITRC (www. itrcweb. org) – Shaping the Future of Regulatory Acceptance u u Host organization Network • State regulators u Disclaimer • Full version in “Notes” section • Partially funded by the U. S. § All 50 states, PR, DC government • Federal partners § ITRC nor US government warranty material § ITRC nor US government DOE DOD endorse specific products EPA • ITRC materials copyrighted • ITRC Industry Affiliates Program u Available from www. itrcweb. org • Technical and regulatory guidance documents • Academia • Community stakeholders • Internet-based and classroom training schedule • More…
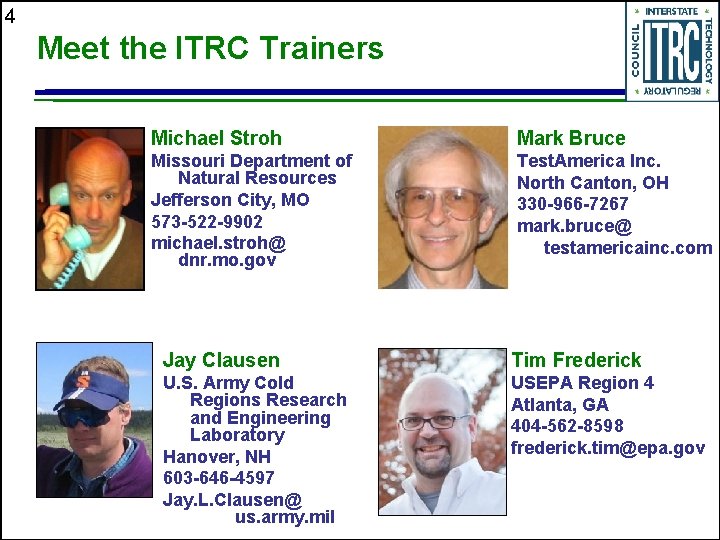
4 Meet the ITRC Trainers Michael Stroh Mark Bruce Missouri Department of Natural Resources Jefferson City, MO 573 -522 -9902 michael. stroh@ dnr. mo. gov Test. America Inc. North Canton, OH 330 -966 -7267 mark. bruce@ testamericainc. com Jay Clausen Tim Frederick U. S. Army Cold Regions Research and Engineering Laboratory Hanover, NH 603 -646 -4597 Jay. L. Clausen@ us. army. mil USEPA Region 4 Atlanta, GA 404 -562 -8598 frederick. tim@epa. gov
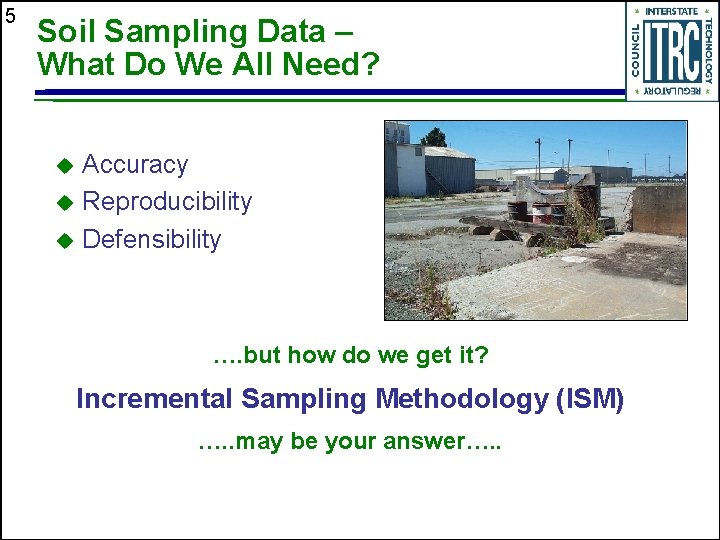
5 Soil Sampling Data – What Do We All Need? Accuracy u Reproducibility u Defensibility u …. but how do we get it? Incremental Sampling Methodology (ISM) …. . may be your answer…. .
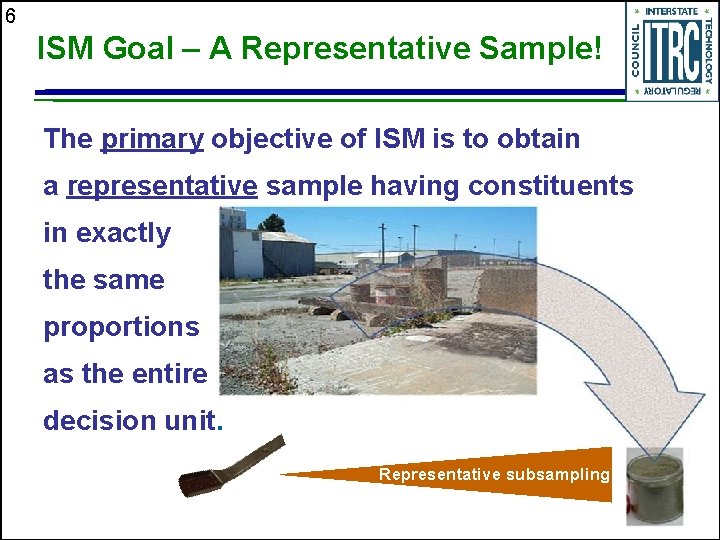
6 ISM Goal – A Representative Sample! The primary objective of ISM is to obtain a representative sample having constituents in exactly the same proportions as the entire decision unit. Representative subsampling
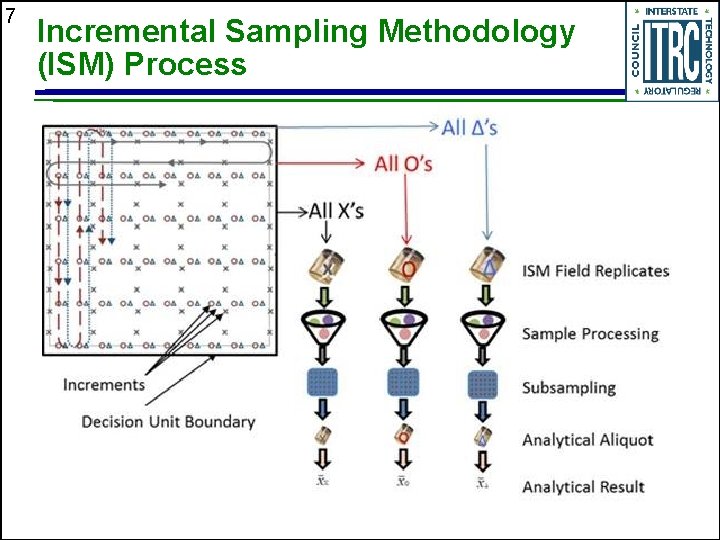
7 Incremental Sampling Methodology (ISM) Process
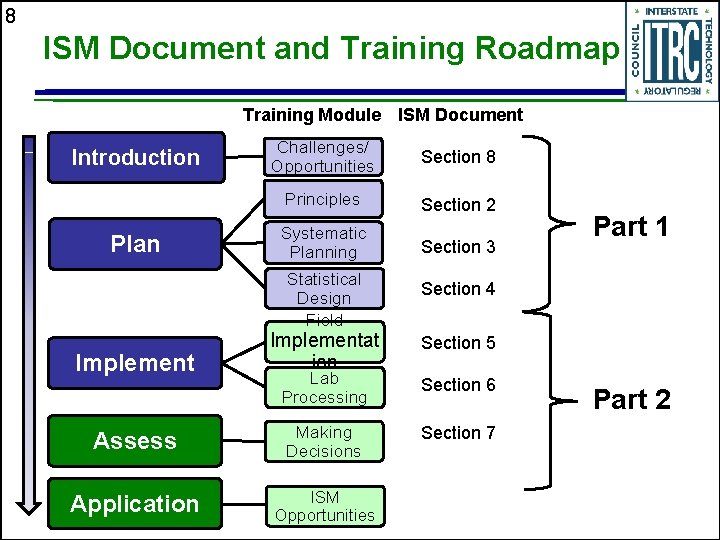
8 ISM Document and Training Roadmap Training Module ISM Document Introduction Plan Challenges/ Opportunities Section 8 Principles Section 2 Systematic Planning Statistical Design Field Implementat ion Section 3 Part 1 Section 4 Section 5 Lab Processing Section 6 Assess Making Decisions Section 7 Application ISM Opportunities Part 2
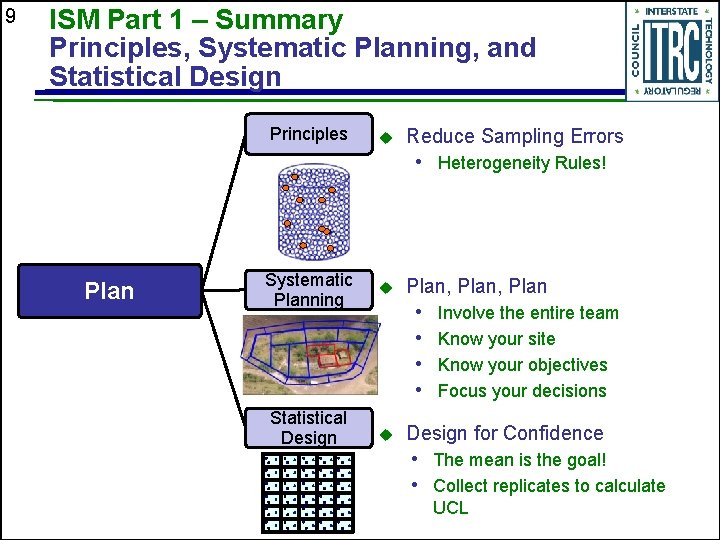
9 ISM Part 1 – Summary Principles, Systematic Planning, and Statistical Design Principles u Reduce Sampling Errors • Heterogeneity Rules! Plan Systematic Planning u Statistical Design u Plan, Plan • • Involve the entire team Know your site Know your objectives Focus your decisions Design for Confidence • The mean is the goal! • Collect replicates to calculate UCL
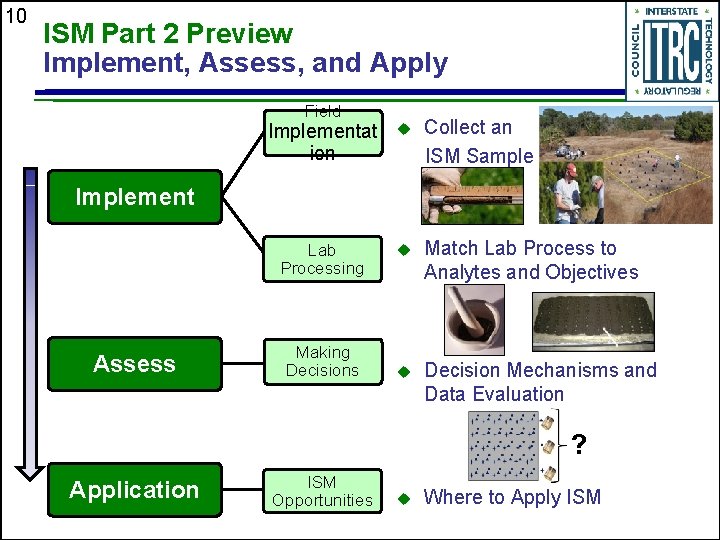
10 ISM Part 2 Preview Implement, Assess, and Apply Field Implementat u Collect an ion ISM Sample Implement Lab Processing Assess Making Decisions u Match Lab Process to Analytes and Objectives u Decision Mechanisms and Data Evaluation ? Application ISM Opportunities u Where to Apply ISM
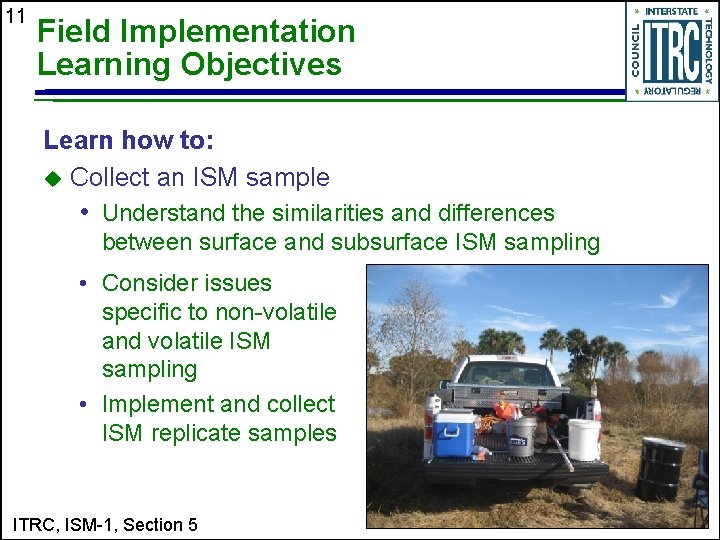
11 Field Implementation Learning Objectives Learn how to: u Collect an ISM sample • Understand the similarities and differences between surface and subsurface ISM sampling • Consider issues specific to non-volatile and volatile ISM sampling • Implement and collect ISM replicate samples ITRC, ISM-1, Section 5
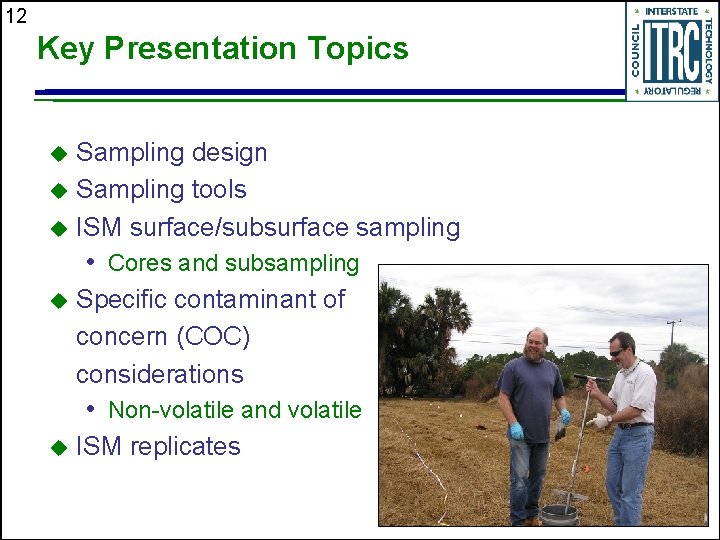
12 Key Presentation Topics Sampling design u Sampling tools u ISM surface/subsurface sampling u • Cores and subsampling u Specific contaminant of concern (COC) considerations • Non-volatile and volatile u ISM replicates
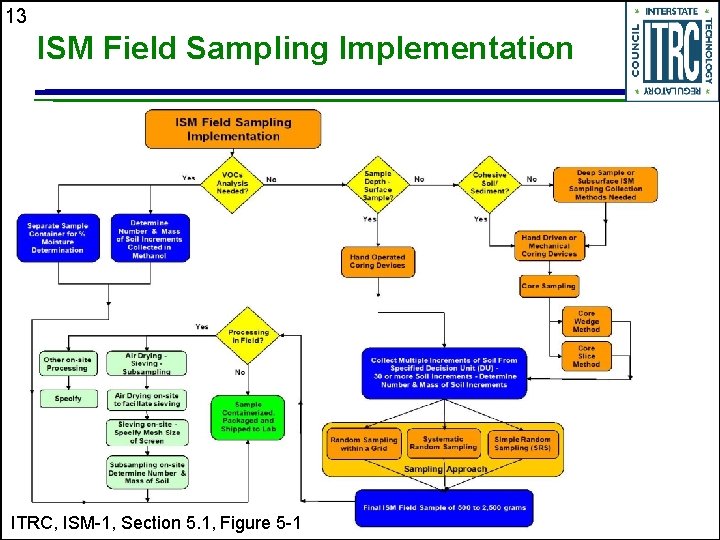
13 ISM Field Sampling Implementation ITRC, ISM-1, Section 5. 1, Figure 5 -1
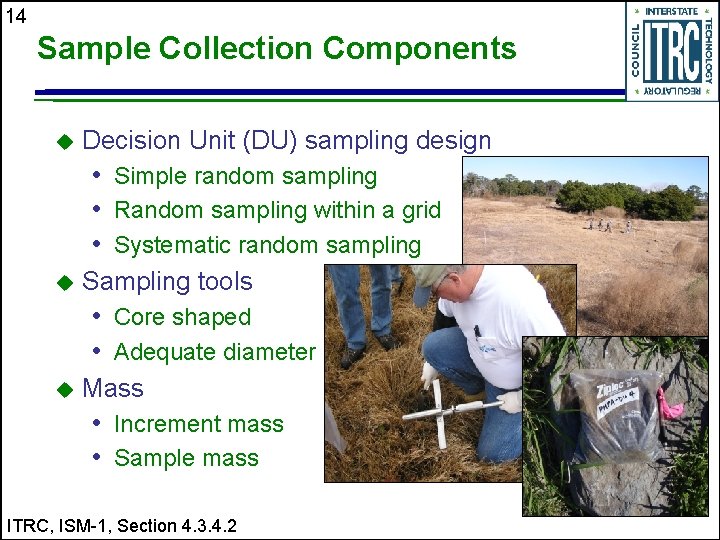
14 Sample Collection Components u Decision Unit (DU) sampling design • Simple random sampling • Random sampling within a grid • Systematic random sampling u Sampling tools • Core shaped • Adequate diameter u Mass • Increment mass • Sample mass ITRC, ISM-1, Section 4. 3. 4. 2
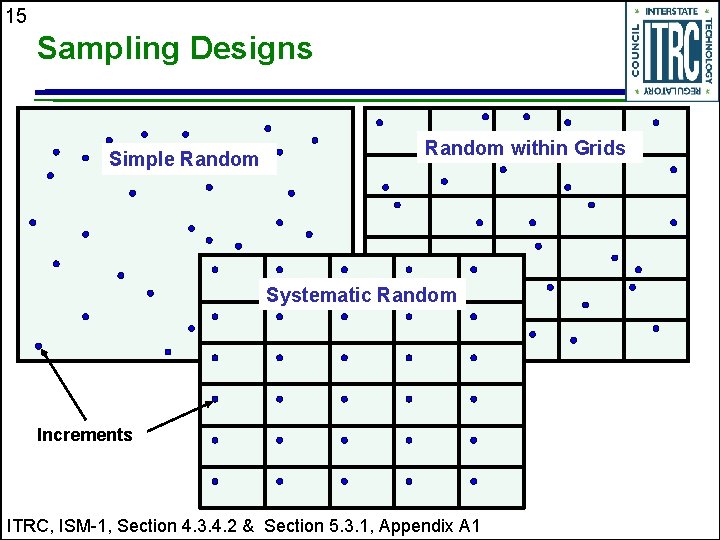
15 Sampling Designs Simple Random within Grids Systematic Random Increments ITRC, ISM-1, Section 4. 3. 4. 2 & Section 5. 3. 1, Appendix A 1
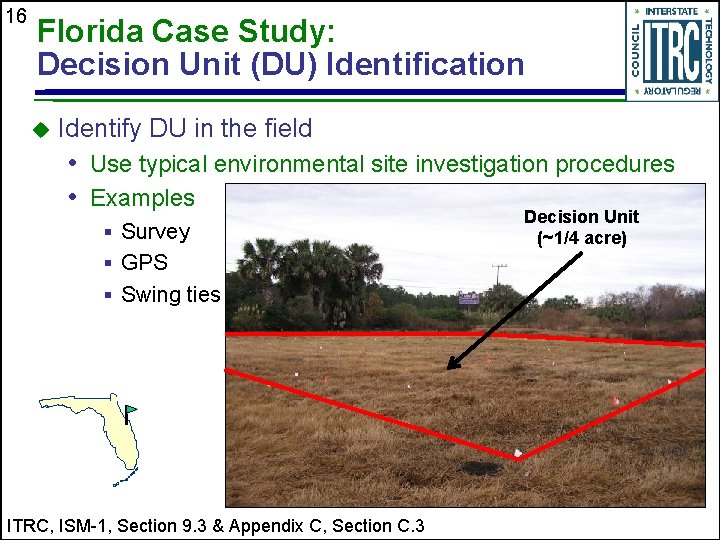
16 Florida Case Study: Decision Unit (DU) Identification u Identify DU in the field • Use typical environmental site investigation procedures • Examples § Survey § GPS § Swing ties ITRC, ISM-1, Section 9. 3 & Appendix C, Section C. 3 Decision Unit (~1/4 acre)
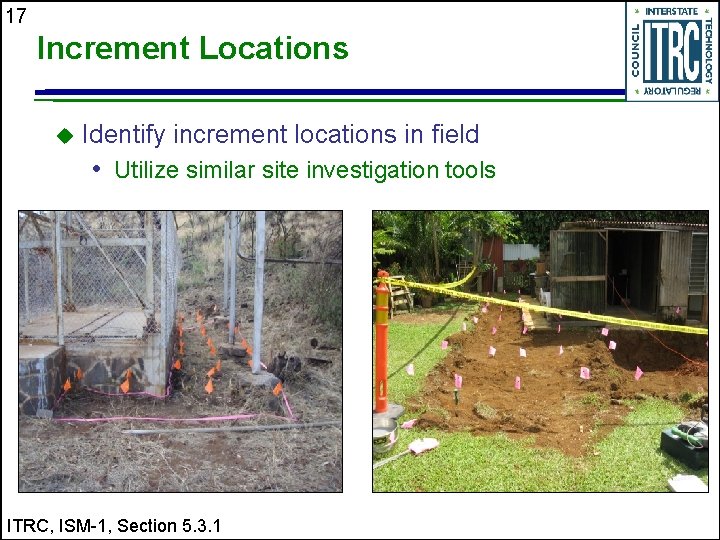
17 Increment Locations u Identify increment locations in field • Utilize similar site investigation tools ITRC, ISM-1, Section 5. 3. 1
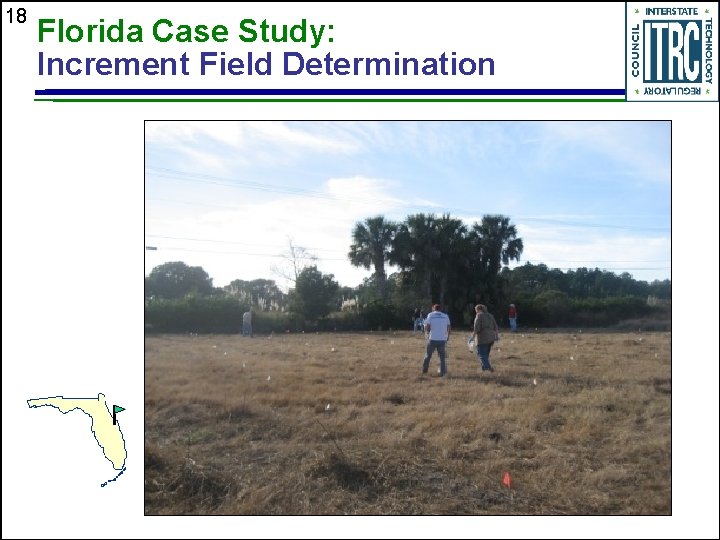
18 Florida Case Study: Increment Field Determination
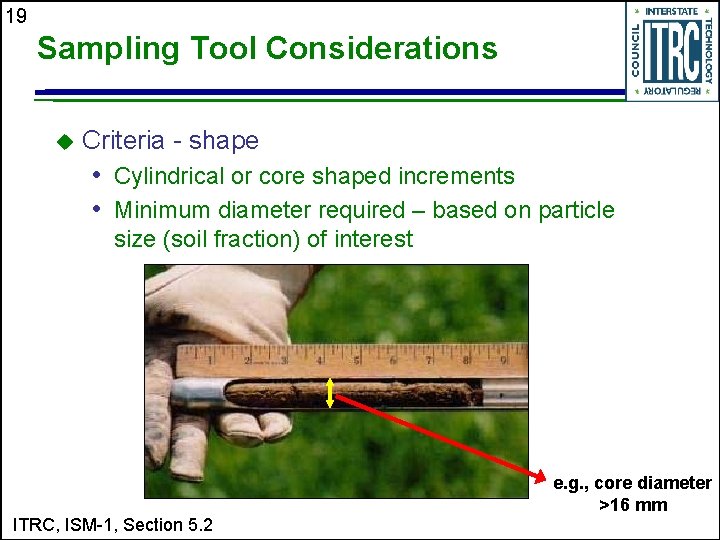
19 Sampling Tool Considerations u Criteria - shape • Cylindrical or core shaped increments • Minimum diameter required – based on particle size (soil fraction) of interest e. g. , core diameter >16 mm ITRC, ISM-1, Section 5. 2
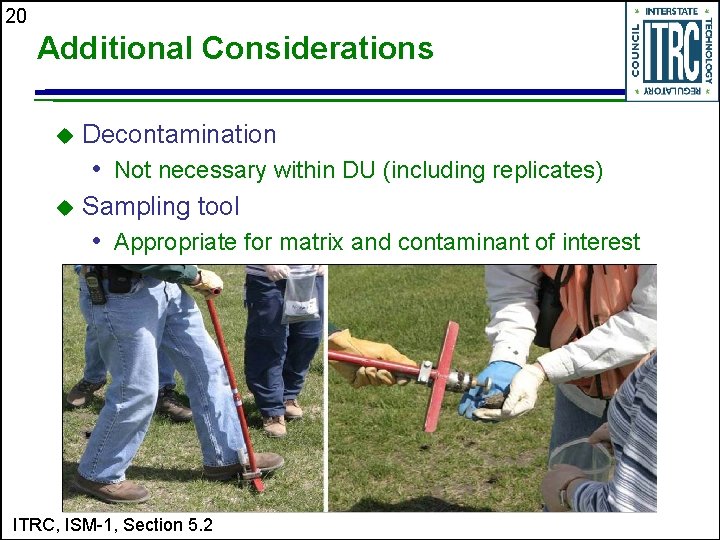
20 Additional Considerations u Decontamination • Not necessary within DU (including replicates) u Sampling tool • Appropriate for matrix and contaminant of interest ITRC, ISM-1, Section 5. 2
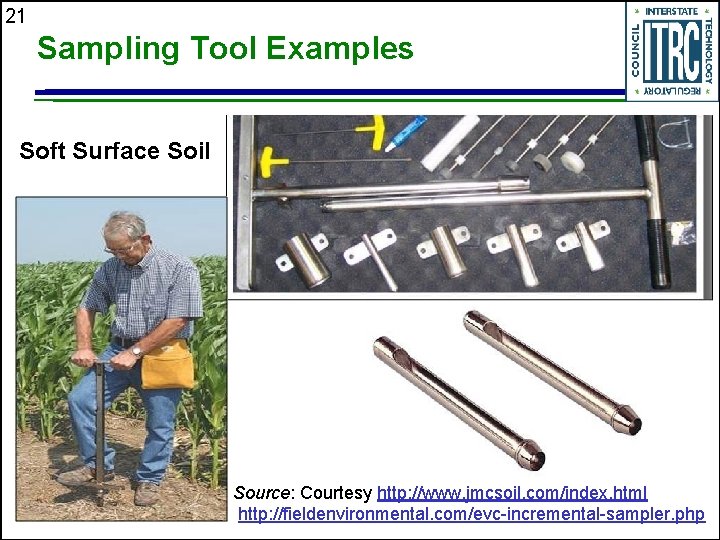
21 Sampling Tool Examples Soft Surface Soil Source: Courtesy http: //www. jmcsoil. com/index. html http: //fieldenvironmental. com/evc-incremental-sampler. php
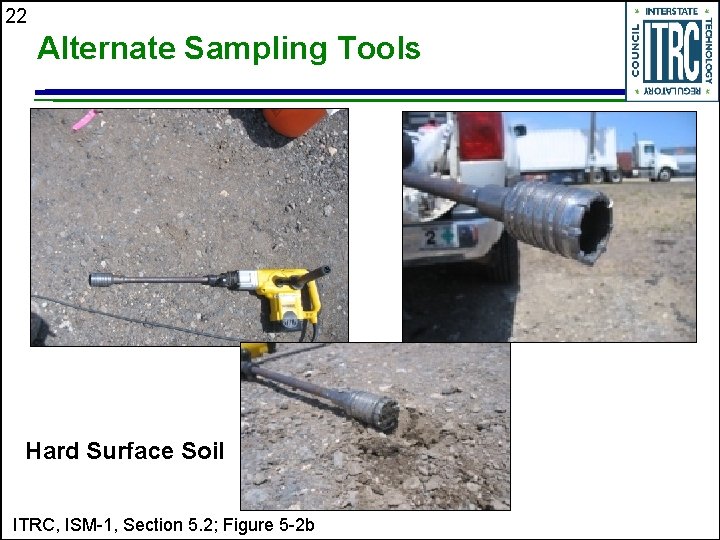
22 Alternate Sampling Tools Hard Surface Soil ITRC, ISM-1, Section 5. 2; Figure 5 -2 b
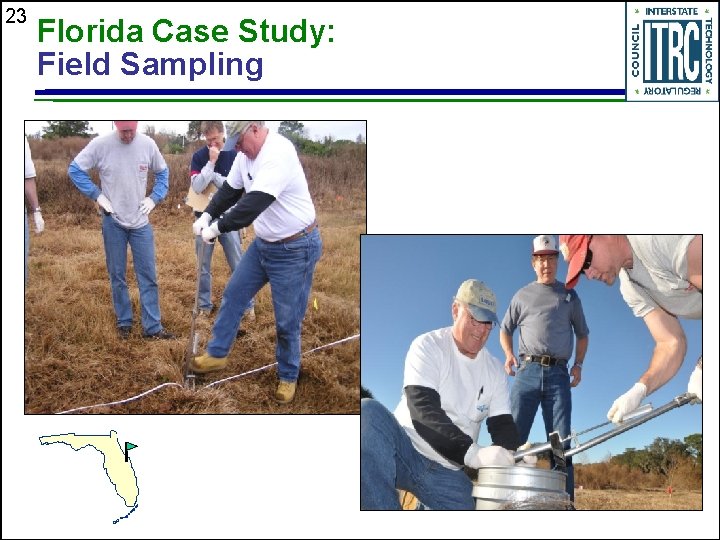
23 Florida Case Study: Field Sampling
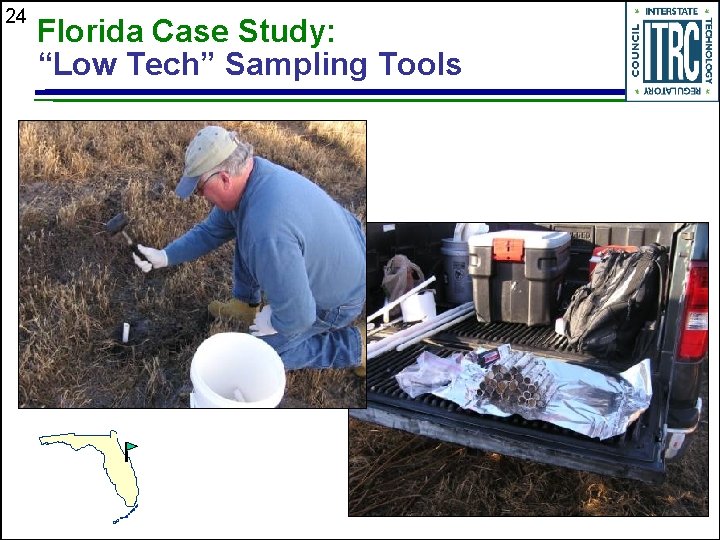
24 Florida Case Study: “Low Tech” Sampling Tools
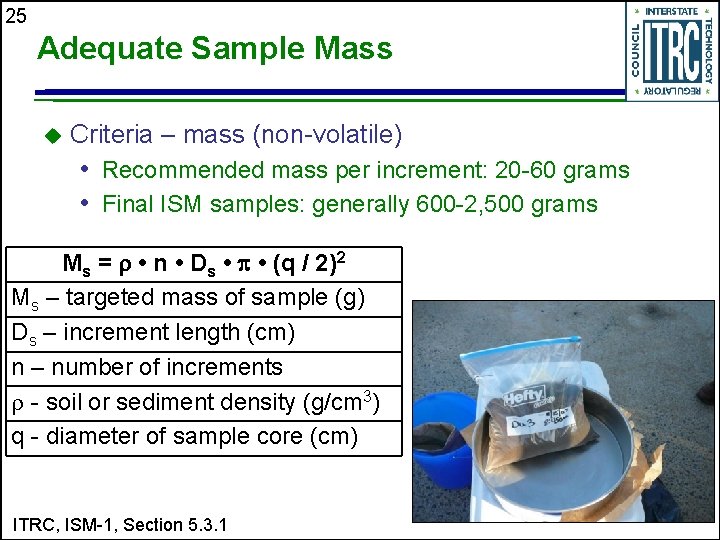
25 Adequate Sample Mass u Criteria – mass (non-volatile) • Recommended mass per increment: 20 -60 grams • Final ISM samples: generally 600 -2, 500 grams Ms = • n • Ds • • (q / 2)2 Ms – targeted mass of sample (g) Ds – increment length (cm) n – number of increments - soil or sediment density (g/cm 3) q - diameter of sample core (cm) ITRC, ISM-1, Section 5. 3. 1
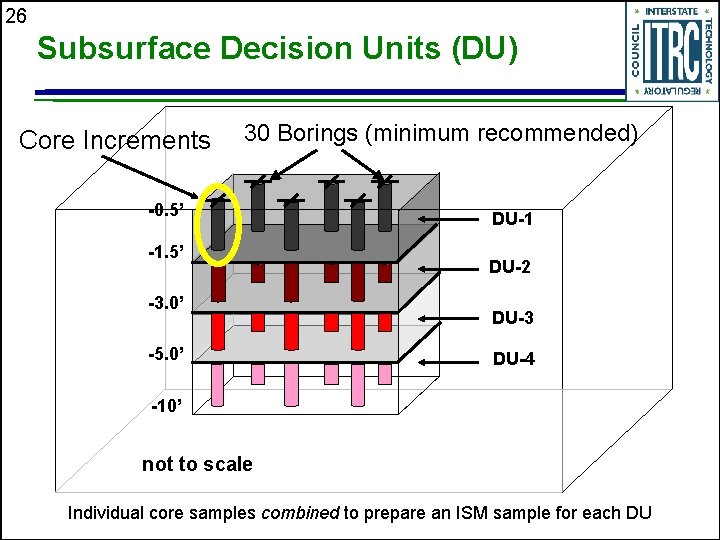
26 Subsurface Decision Units (DU) Core Increments 30 Borings (minimum recommended) -0. 5’ -1. 5’ -3. 0’ -5. 0’ DU-1 DU-2 DU-3 DU-4 -10’ not to scale Individual core samples combined to prepare an ISM sample for each DU
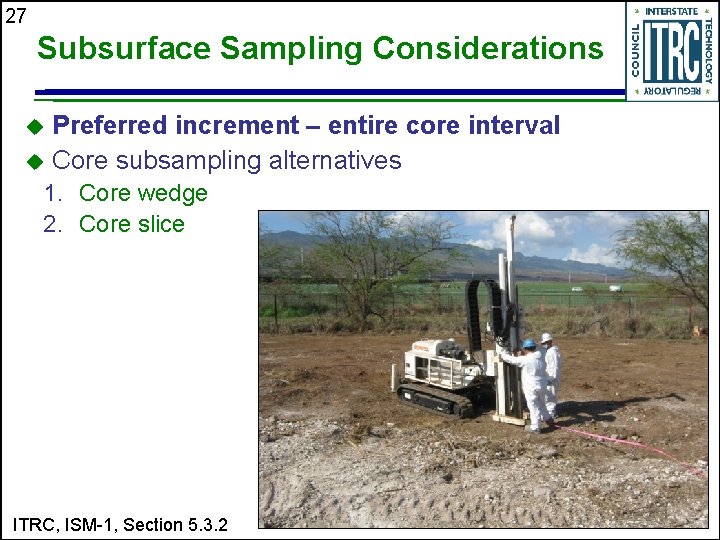
27 Subsurface Sampling Considerations Preferred increment – entire core interval u Core subsampling alternatives u 1. Core wedge 2. Core slice ITRC, ISM-1, Section 5. 3. 2
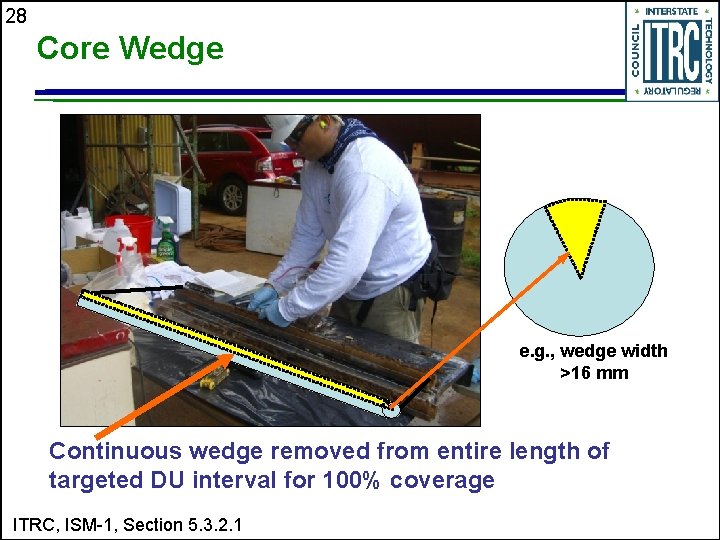
28 Core Wedge e. g. , wedge width >16 mm Continuous wedge removed from entire length of targeted DU interval for 100% coverage ITRC, ISM-1, Section 5. 3. 2. 1
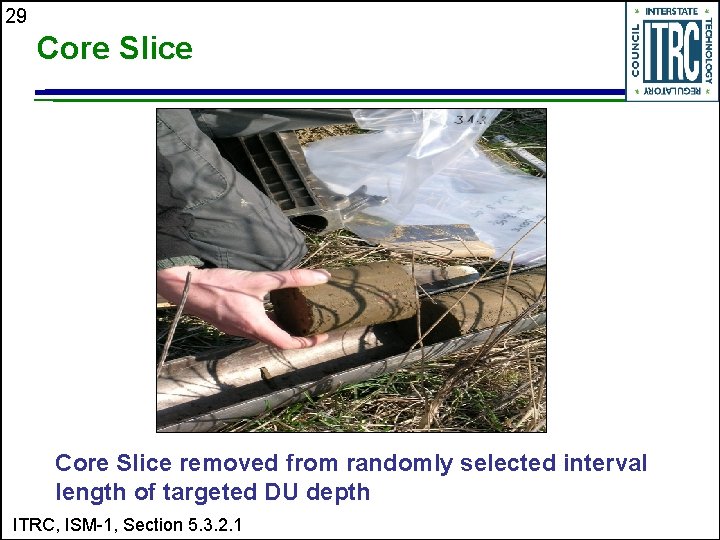
29 Core Slice removed from randomly selected interval length of targeted DU depth ITRC, ISM-1, Section 5. 3. 2. 1
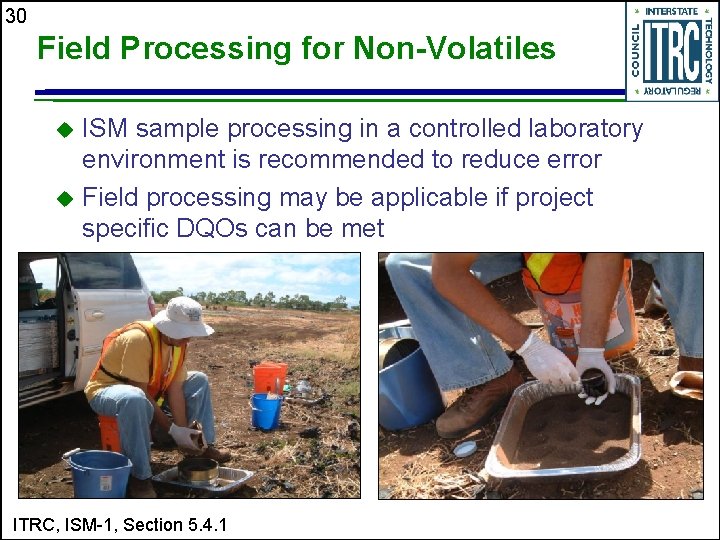
30 Field Processing for Non-Volatiles ISM sample processing in a controlled laboratory environment is recommended to reduce error u Field processing may be applicable if project specific DQOs can be met u ITRC, ISM-1, Section 5. 4. 1
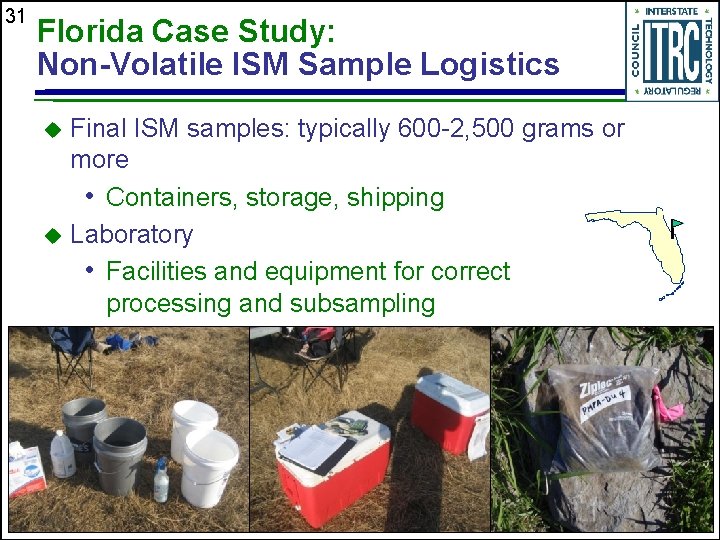
31 Florida Case Study: Non-Volatile ISM Sample Logistics Final ISM samples: typically 600 -2, 500 grams or more • Containers, storage, shipping u Laboratory • Facilities and equipment for correct processing and subsampling u
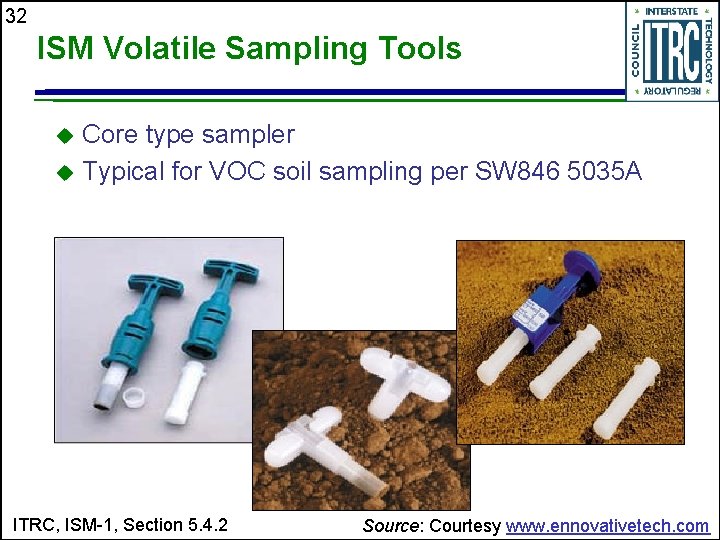
32 ISM Volatile Sampling Tools Core type sampler u Typical for VOC soil sampling per SW 846 5035 A u ITRC, ISM-1, Section 5. 4. 2 Source: Courtesy www. ennovativetech. com
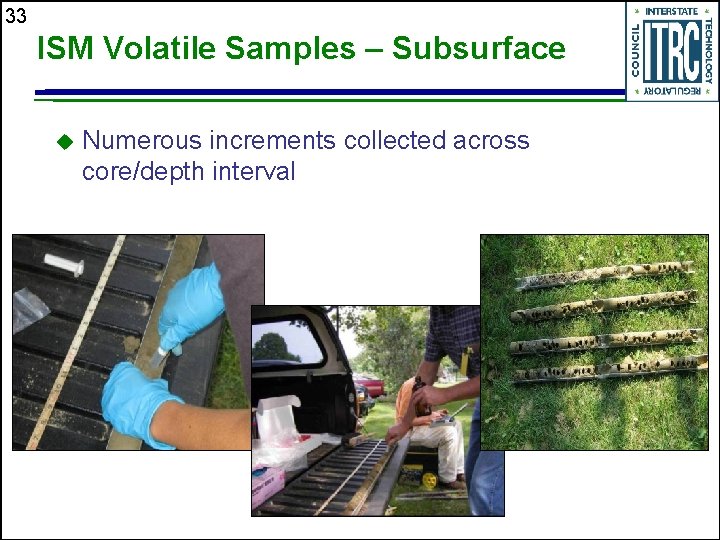
33 ISM Volatile Samples – Subsurface u Numerous increments collected across core/depth interval
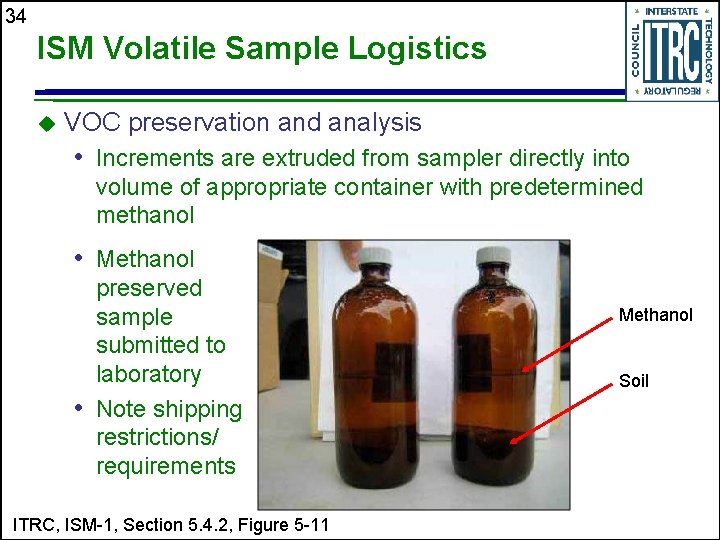
34 ISM Volatile Sample Logistics u VOC preservation and analysis • Increments are extruded from sampler directly into volume of appropriate container with predetermined methanol • Methanol preserved sample submitted to laboratory • Note shipping restrictions/ requirements ITRC, ISM-1, Section 5. 4. 2, Figure 5 -11 Methanol Soil
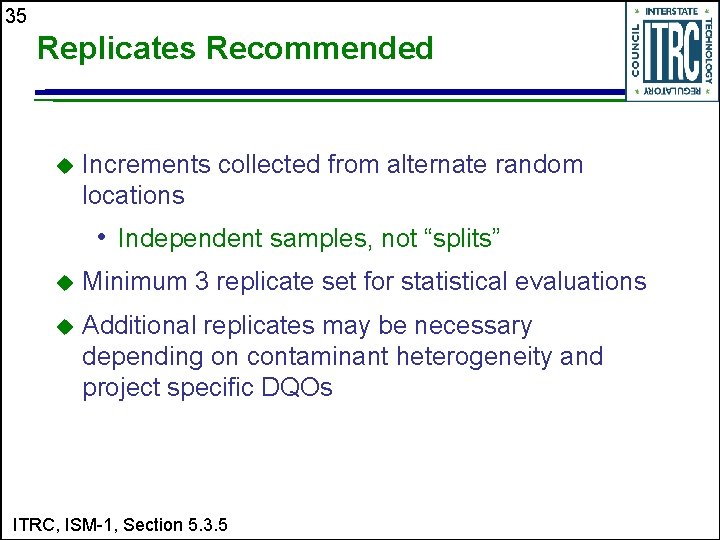
35 Replicates Recommended u Increments collected from alternate random locations • Independent samples, not “splits” u Minimum 3 replicate set for statistical evaluations u Additional replicates may be necessary depending on contaminant heterogeneity and project specific DQOs ITRC, ISM-1, Section 5. 3. 5
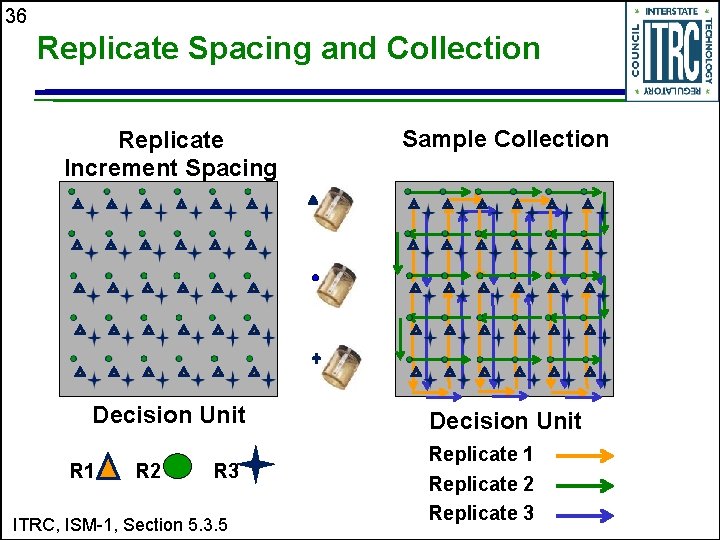
36 Replicate Spacing and Collection Replicate Increment Spacing Sample Collection Decision Unit R 1 R 2 R 3 ITRC, ISM-1, Section 5. 3. 5 Replicate 1 Replicate 2 Replicate 3
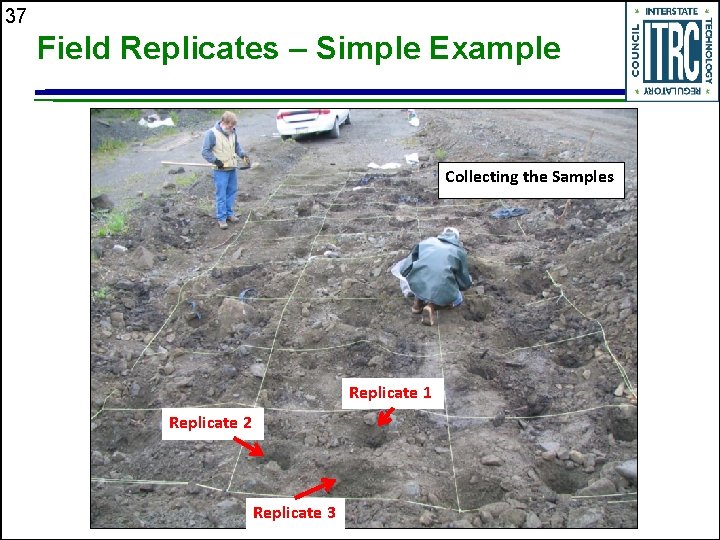
37 Field Replicates – Simple Example Collecting the Samples Replicate 1 Replicate 2 Replicate 3
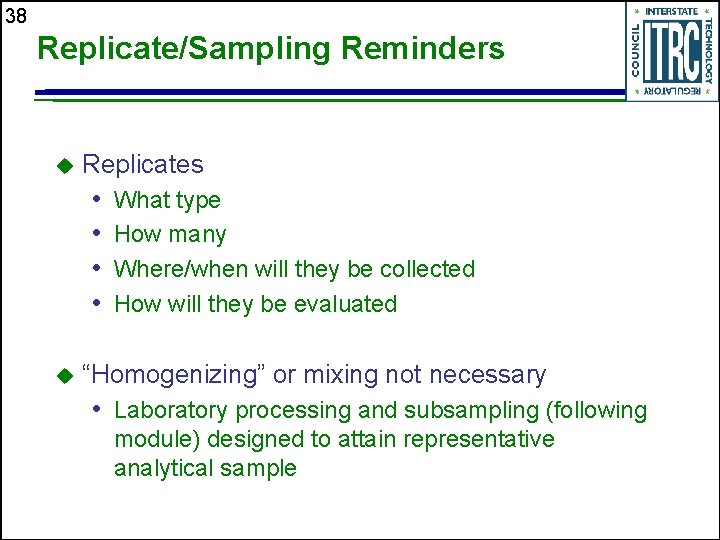
38 Replicate/Sampling Reminders u Replicates • • u What type How many Where/when will they be collected How will they be evaluated “Homogenizing” or mixing not necessary • Laboratory processing and subsampling (following module) designed to attain representative analytical sample
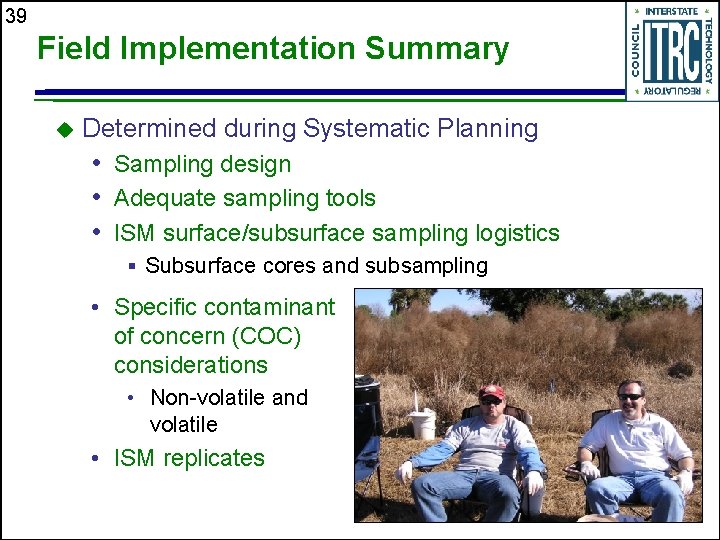
39 Field Implementation Summary u Determined during Systematic Planning • Sampling design • Adequate sampling tools • ISM surface/subsurface sampling logistics § Subsurface cores and subsampling • Specific contaminant of concern (COC) considerations • Non-volatile and volatile • ISM replicates
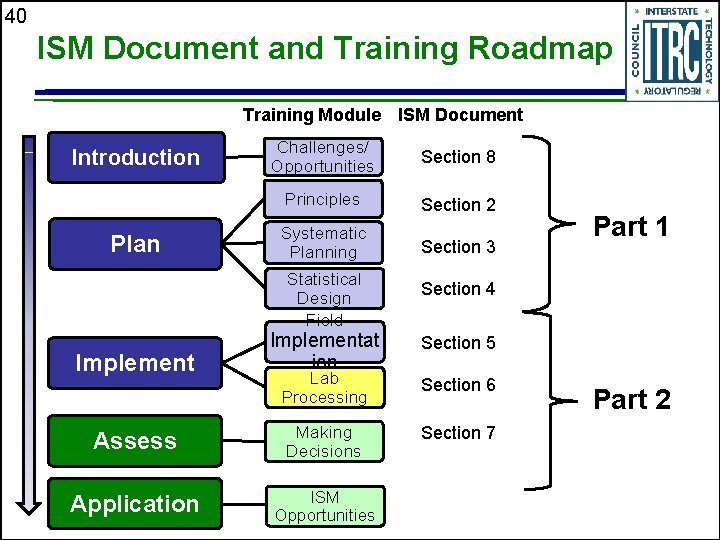
40 ISM Document and Training Roadmap Training Module ISM Document Introduction Plan Challenges/ Opportunities Section 8 Principles Section 2 Systematic Planning Statistical Design Field Implementat ion Section 3 Part 1 Section 4 Section 5 Lab Processing Section 6 Assess Making Decisions Section 7 Application ISM Opportunities Part 2
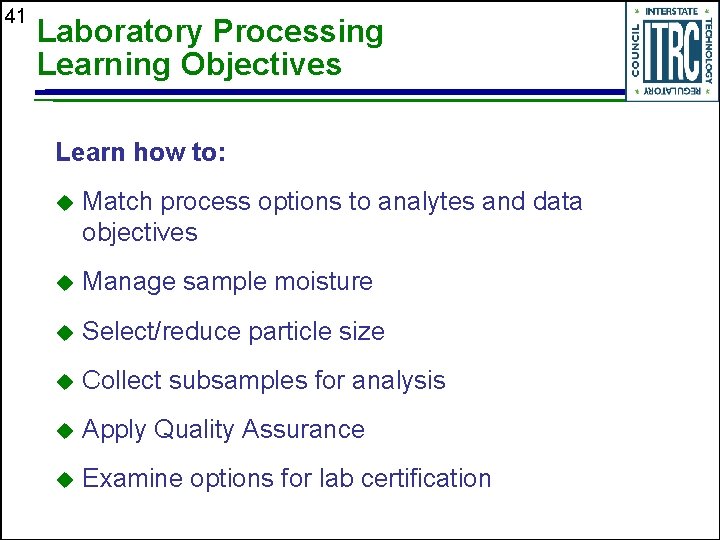
41 Laboratory Processing Learning Objectives Learn how to: u Match process options to analytes and data objectives u Manage sample moisture u Select/reduce particle size u Collect subsamples for analysis u Apply Quality Assurance u Examine options for lab certification
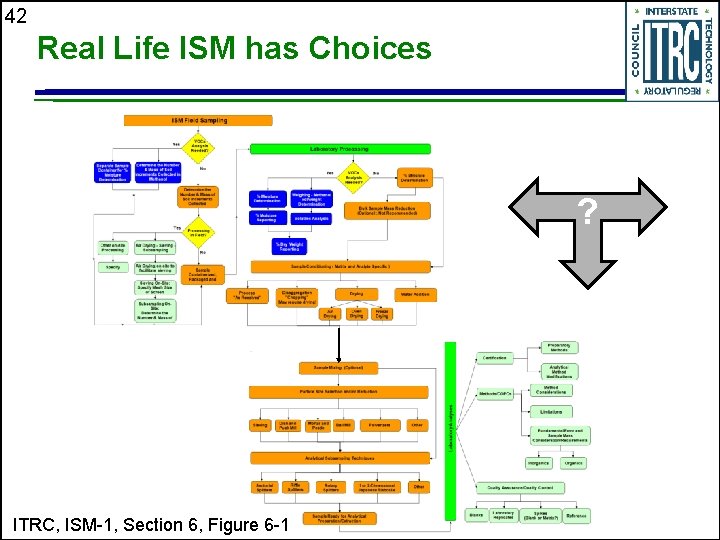
42 Real Life ISM has Choices ? ITRC, ISM-1, Section 6, Figure 6 -1
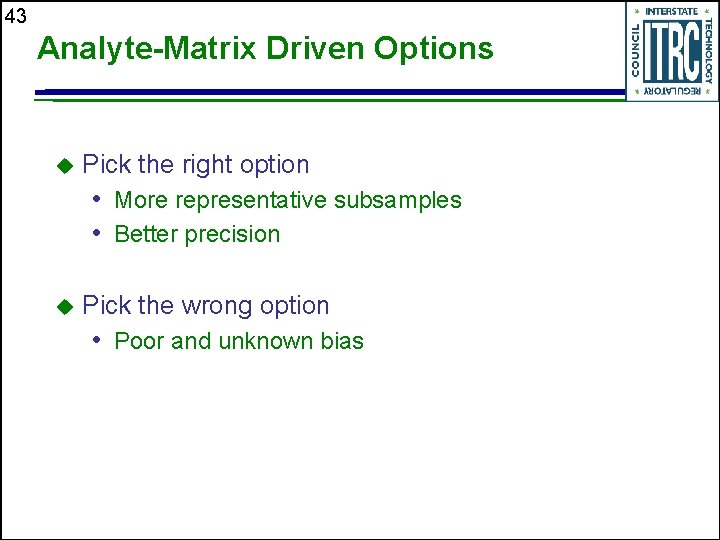
43 Analyte-Matrix Driven Options u Pick the right option • More representative subsamples • Better precision u Pick the wrong option • Poor and unknown bias
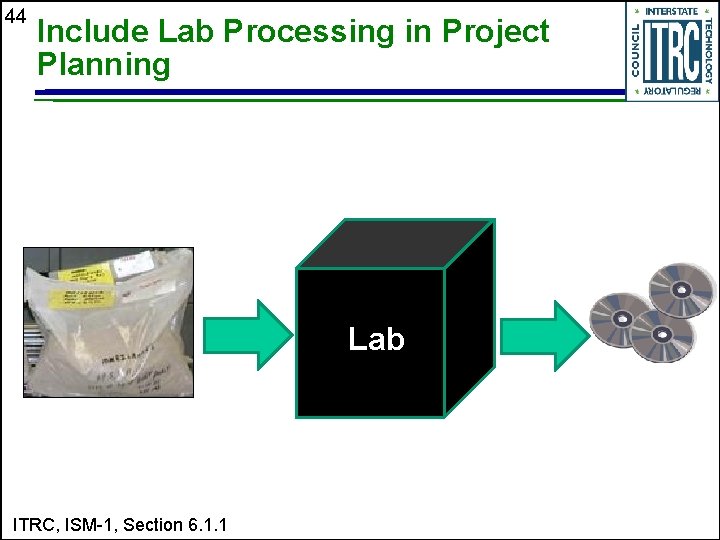
44 Include Lab Processing in Project Planning Lab ITRC, ISM-1, Section 6. 1. 1
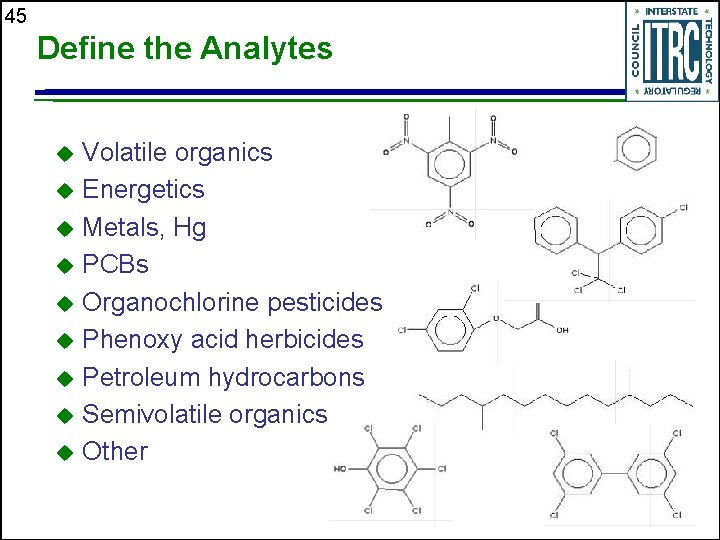
45 Define the Analytes Volatile organics u Energetics u Metals, Hg u PCBs u Organochlorine pesticides u Phenoxy acid herbicides u Petroleum hydrocarbons u Semivolatile organics u Other u
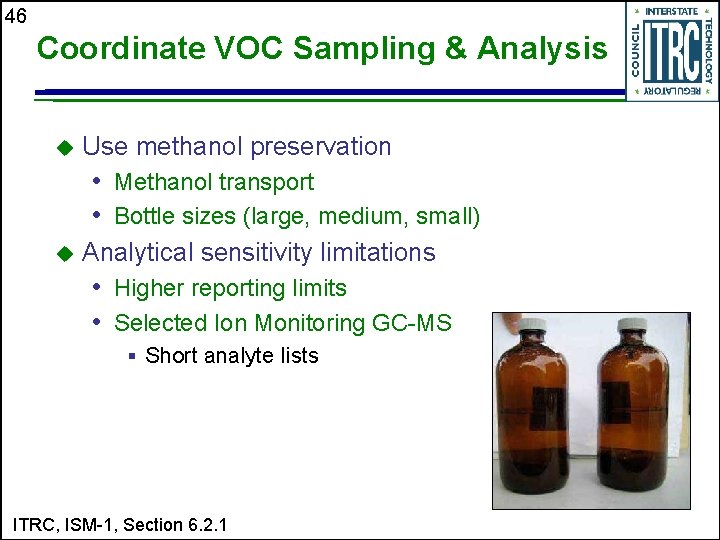
46 Coordinate VOC Sampling & Analysis u Use methanol preservation • Methanol transport • Bottle sizes (large, medium, small) u Analytical sensitivity limitations • Higher reporting limits • Selected Ion Monitoring GC-MS § Short analyte lists ITRC, ISM-1, Section 6. 2. 1
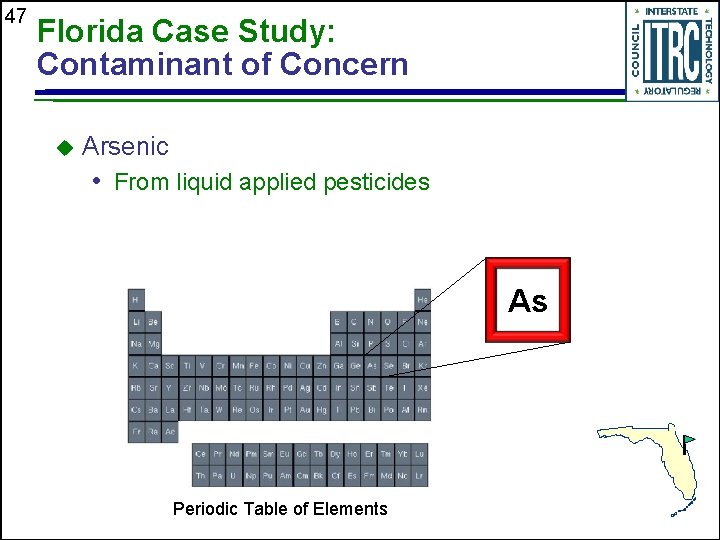
47 Florida Case Study: Contaminant of Concern u Arsenic • From liquid applied pesticides As Periodic Table of Elements
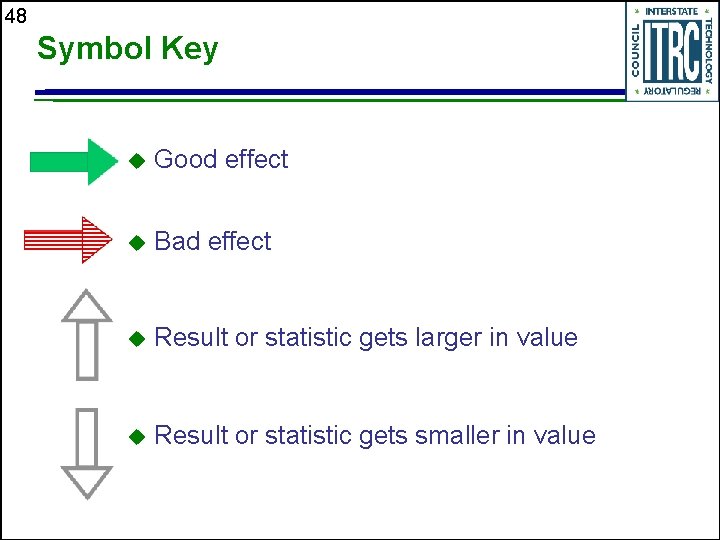
48 Symbol Key u Good effect u Bad effect u Result or statistic gets larger in value u Result or statistic gets smaller in value
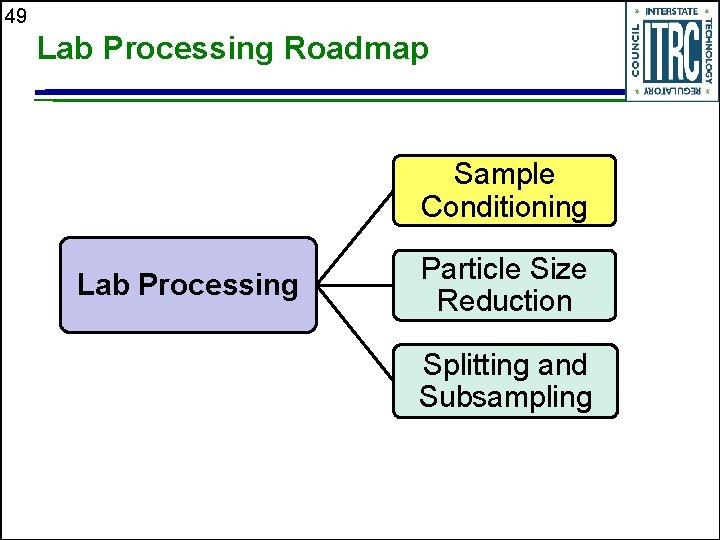
49 Lab Processing Roadmap Sample Conditioning Lab Processing Particle Size Reduction Splitting and Subsampling
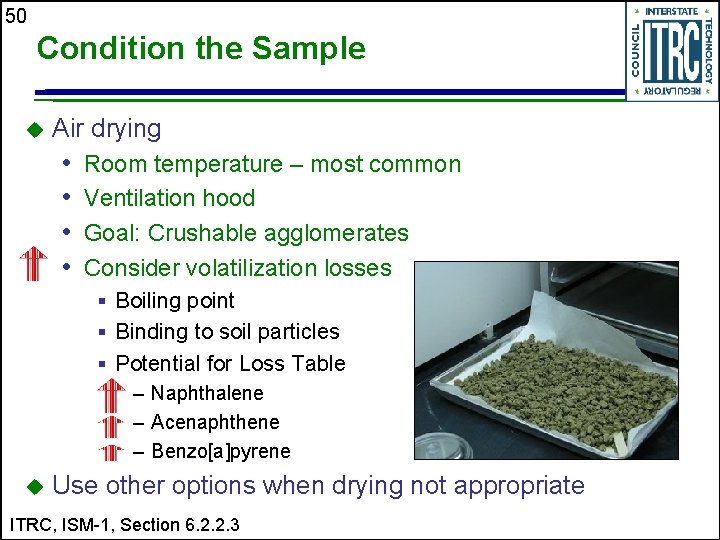
50 Condition the Sample u Air drying • • Room temperature – most common Ventilation hood Goal: Crushable agglomerates Consider volatilization losses § Boiling point § Binding to soil particles § Potential for Loss Table – Naphthalene – Acenaphthene – Benzo[a]pyrene u Use other options when drying not appropriate ITRC, ISM-1, Section 6. 2. 2. 3
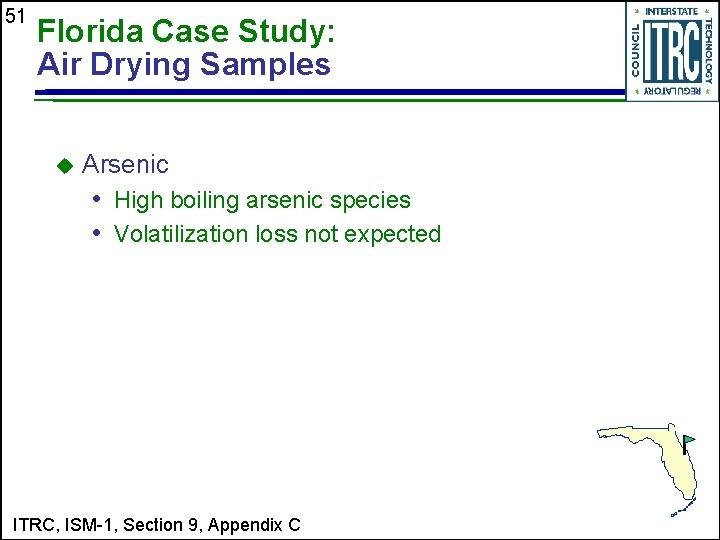
51 Florida Case Study: Air Drying Samples u Arsenic • High boiling arsenic species • Volatilization loss not expected ITRC, ISM-1, Section 9, Appendix C
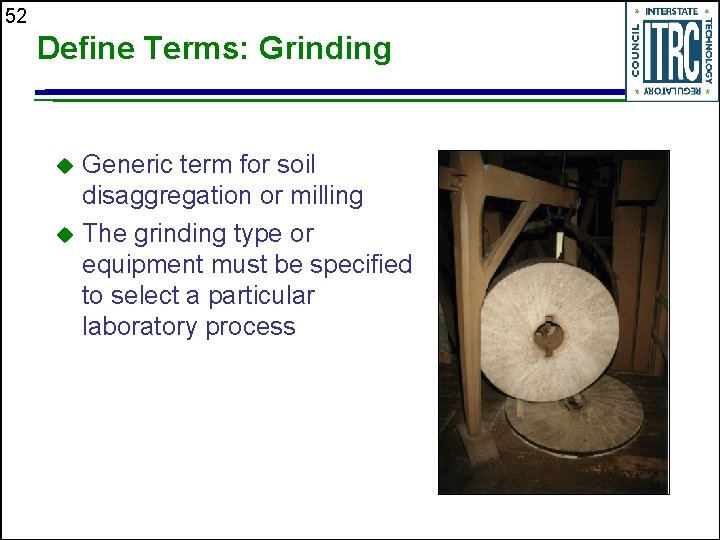
52 Define Terms: Grinding Generic term for soil disaggregation or milling u The grinding type or equipment must be specified to select a particular laboratory process u
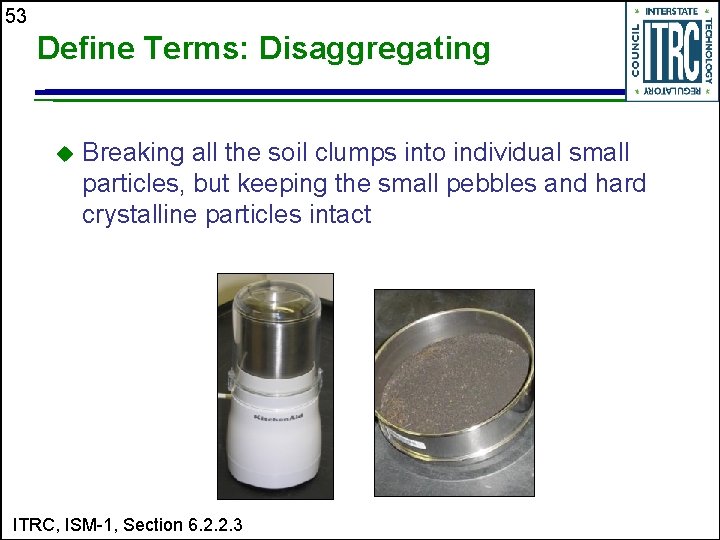
53 Define Terms: Disaggregating u Breaking all the soil clumps into individual small particles, but keeping the small pebbles and hard crystalline particles intact ITRC, ISM-1, Section 6. 2. 2. 3
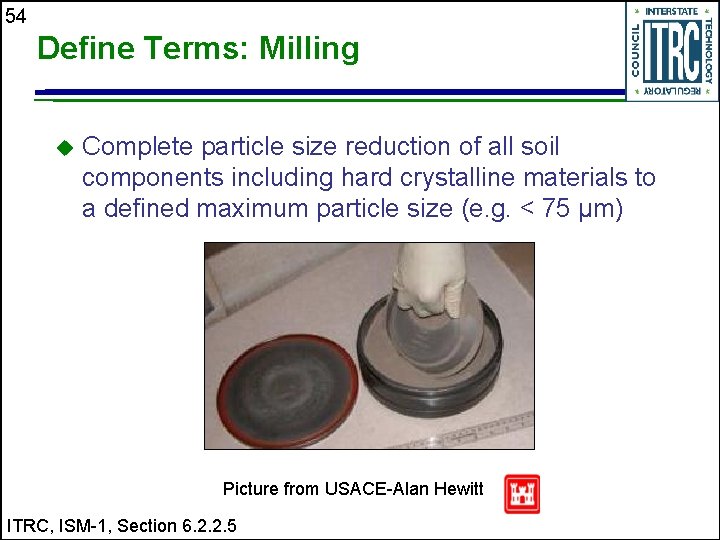
54 Define Terms: Milling u Complete particle size reduction of all soil components including hard crystalline materials to a defined maximum particle size (e. g. < 75 µm) Picture from USACE-Alan Hewitt ITRC, ISM-1, Section 6. 2. 2. 5
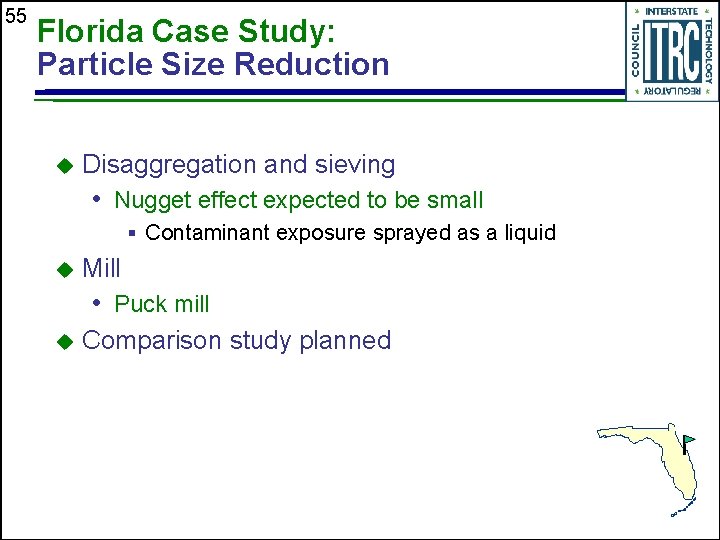
55 Florida Case Study: Particle Size Reduction u Disaggregation and sieving • Nugget effect expected to be small § Contaminant exposure sprayed as a liquid u Mill • Puck mill u Comparison study planned
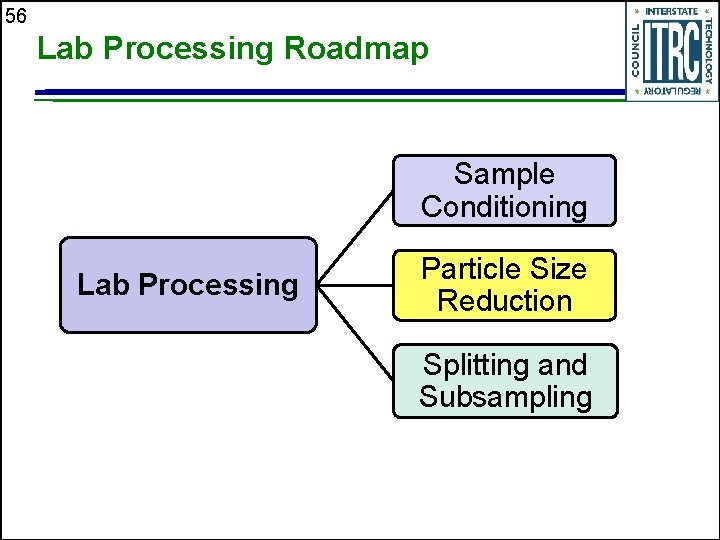
56 Lab Processing Roadmap Sample Conditioning Lab Processing Particle Size Reduction Splitting and Subsampling
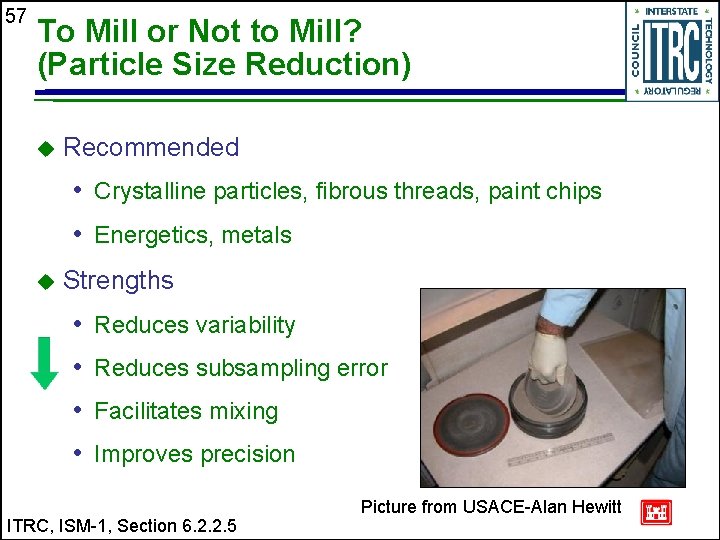
57 To Mill or Not to Mill? (Particle Size Reduction) u Recommended • Crystalline particles, fibrous threads, paint chips • Energetics, metals u Strengths • Reduces variability • Reduces subsampling error • Facilitates mixing • Improves precision ITRC, ISM-1, Section 6. 2. 2. 5 Picture from USACE-Alan Hewitt
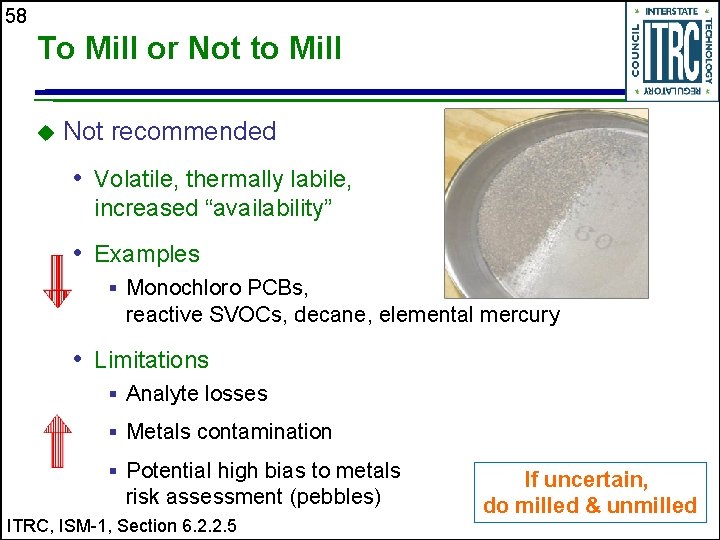
58 To Mill or Not to Mill u Not recommended • Volatile, thermally labile, increased “availability” • Examples § Monochloro PCBs, reactive SVOCs, decane, elemental mercury • Limitations § Analyte losses § Metals contamination § Potential high bias to metals risk assessment (pebbles) ITRC, ISM-1, Section 6. 2. 2. 5 If uncertain, do milled & unmilled
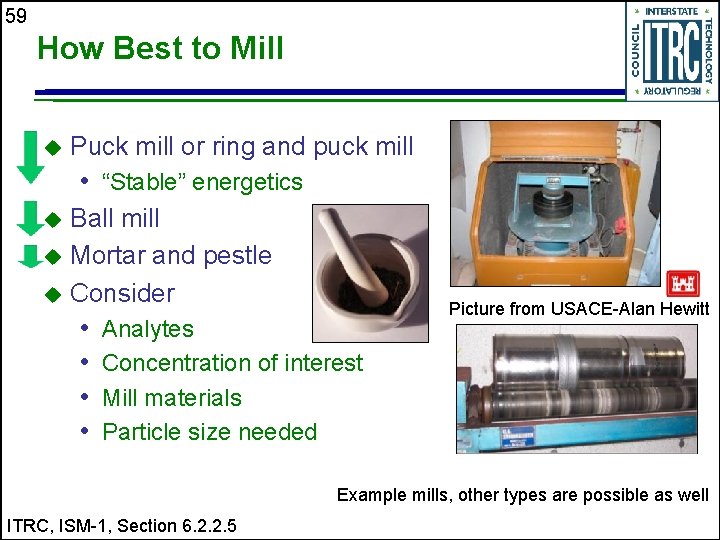
59 How Best to Mill u Puck mill or ring and puck mill • “Stable” energetics Ball mill u Mortar and pestle u Consider u • • Analytes Concentration of interest Mill materials Particle size needed Picture from USACE-Alan Hewitt Example mills, other types are possible as well ITRC, ISM-1, Section 6. 2. 2. 5
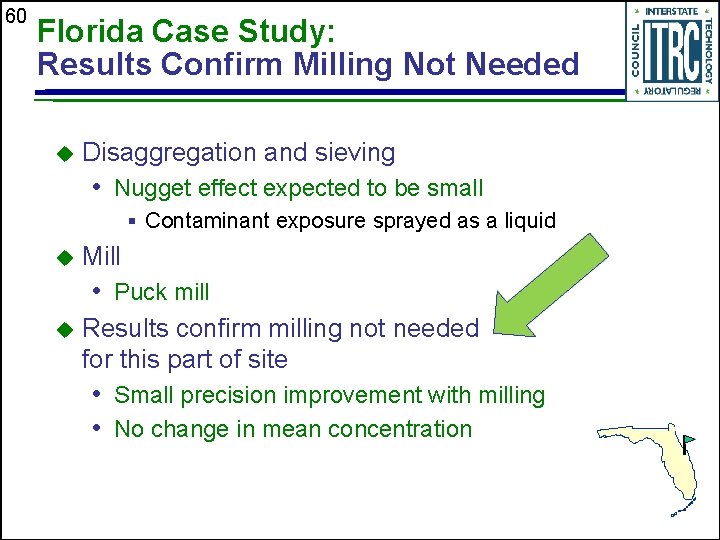
60 Florida Case Study: Results Confirm Milling Not Needed u Disaggregation and sieving • Nugget effect expected to be small § Contaminant exposure sprayed as a liquid u Mill • Puck mill u Results confirm milling not needed for this part of site • Small precision improvement with milling • No change in mean concentration
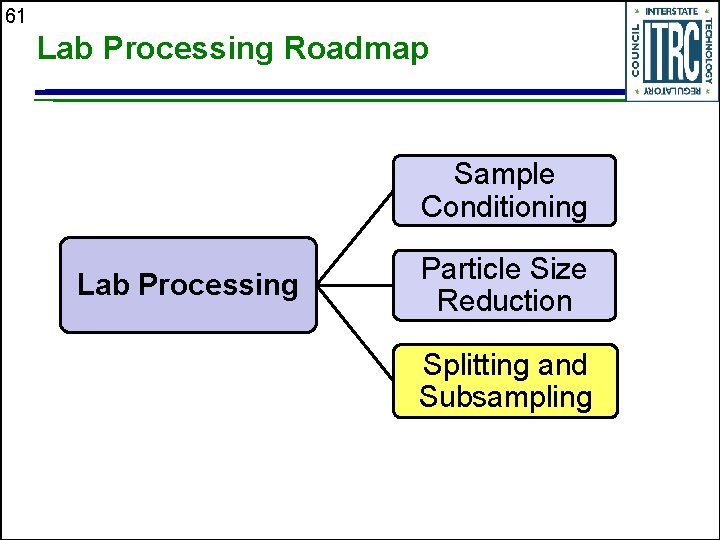
61 Lab Processing Roadmap Sample Conditioning Lab Processing Particle Size Reduction Splitting and Subsampling
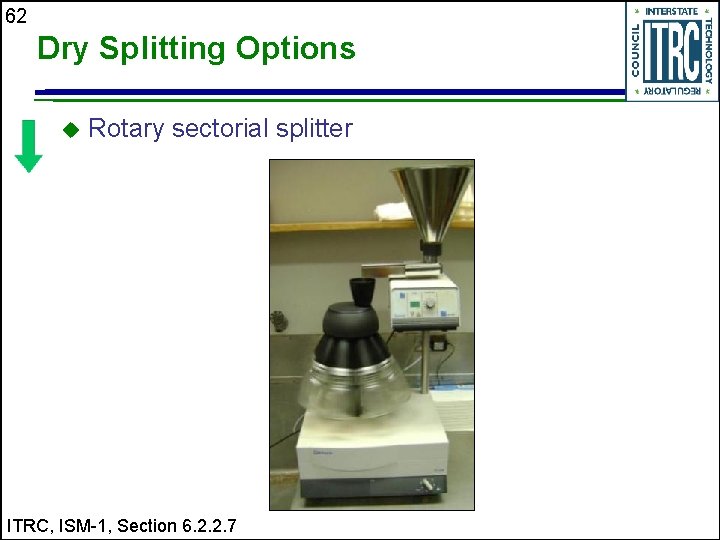
62 Dry Splitting Options u Rotary sectorial splitter ITRC, ISM-1, Section 6. 2. 2. 7
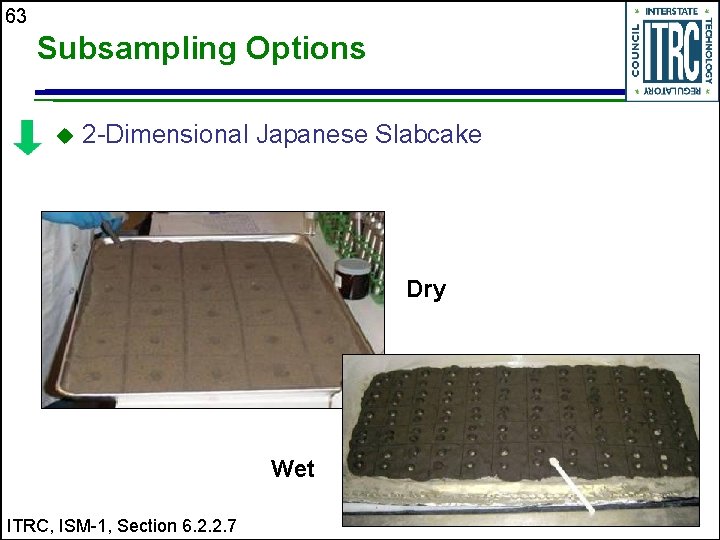
63 Subsampling Options u 2 -Dimensional Japanese Slabcake Dry Wet ITRC, ISM-1, Section 6. 2. 2. 7
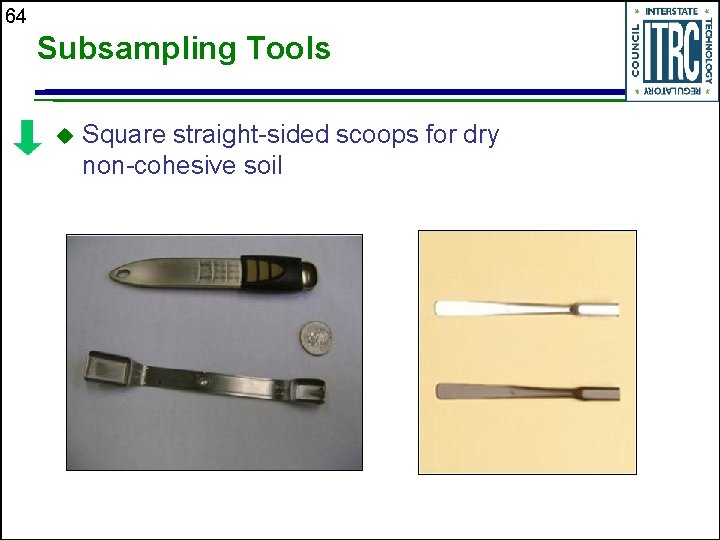
64 Subsampling Tools Square straight-sided scoops for dry non-cohesive soil u
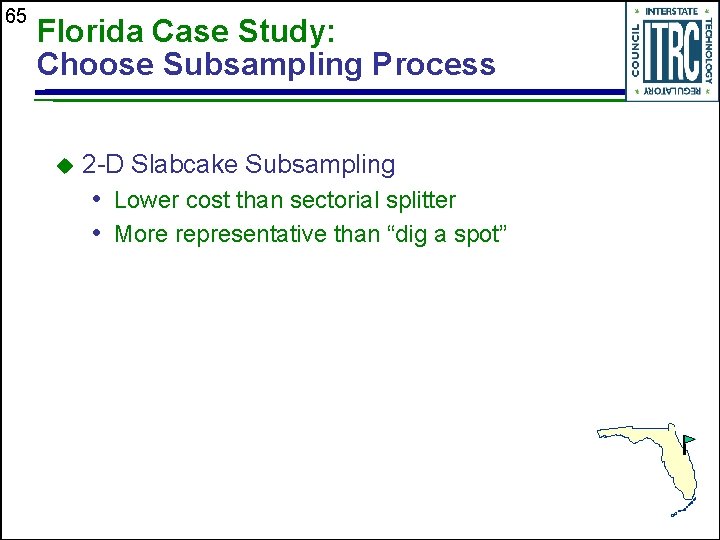
65 Florida Case Study: Choose Subsampling Process u 2 -D Slabcake Subsampling • Lower cost than sectorial splitter • More representative than “dig a spot”
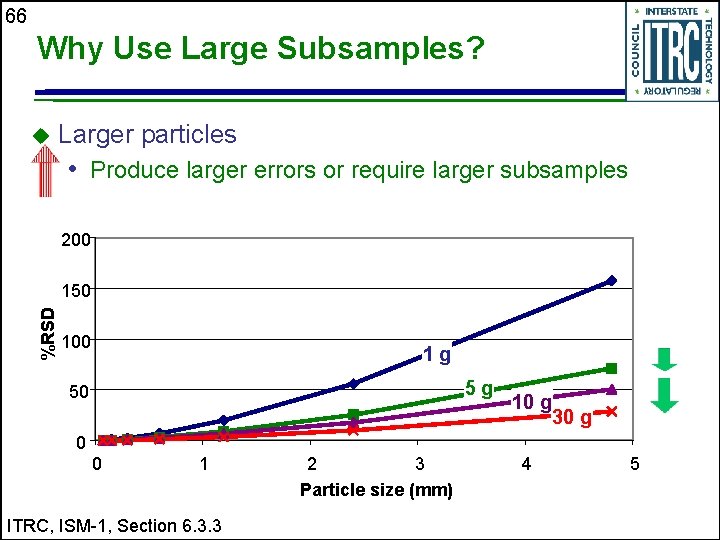
66 Why Use Large Subsamples? u Larger particles • Produce larger errors or require larger subsamples 200 %RSD 150 100 1 g 5 g 50 10 g 30 g 0 0 1 ITRC, ISM-1, Section 6. 3. 3 2 3 Particle size (mm) 4 5
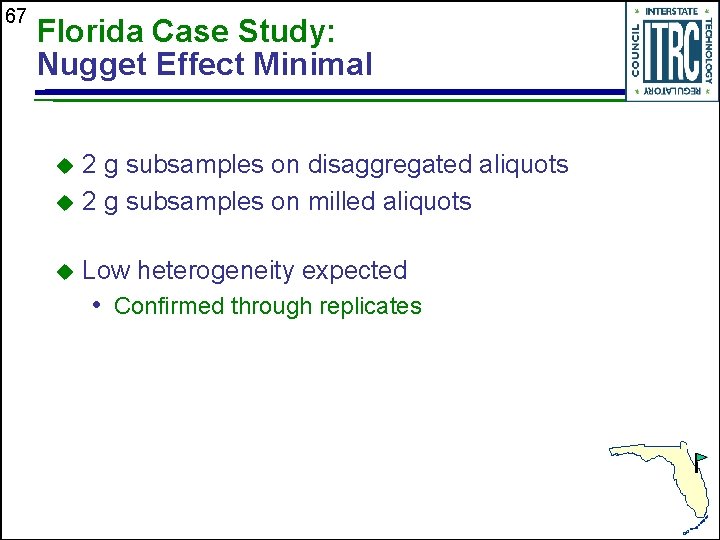
67 Florida Case Study: Nugget Effect Minimal 2 g subsamples on disaggregated aliquots u 2 g subsamples on milled aliquots u u Low heterogeneity expected • Confirmed through replicates
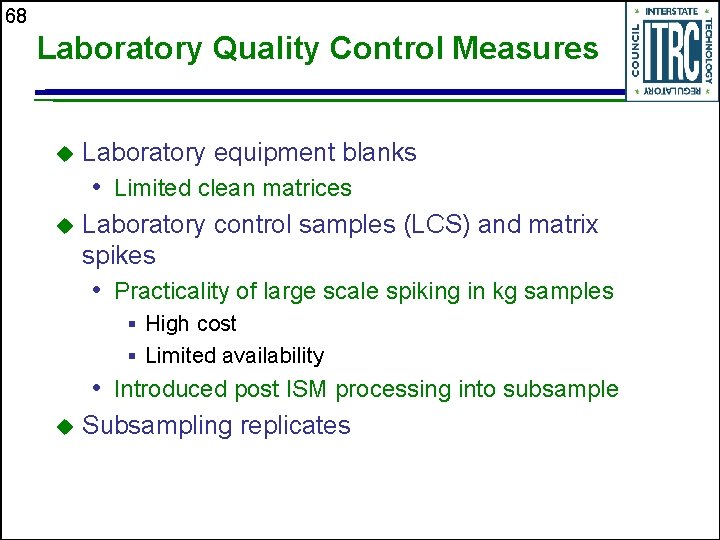
68 Laboratory Quality Control Measures u Laboratory equipment blanks • Limited clean matrices u Laboratory control samples (LCS) and matrix spikes • Practicality of large scale spiking in kg samples § High cost § Limited availability • Introduced post ISM processing into subsample u Subsampling replicates
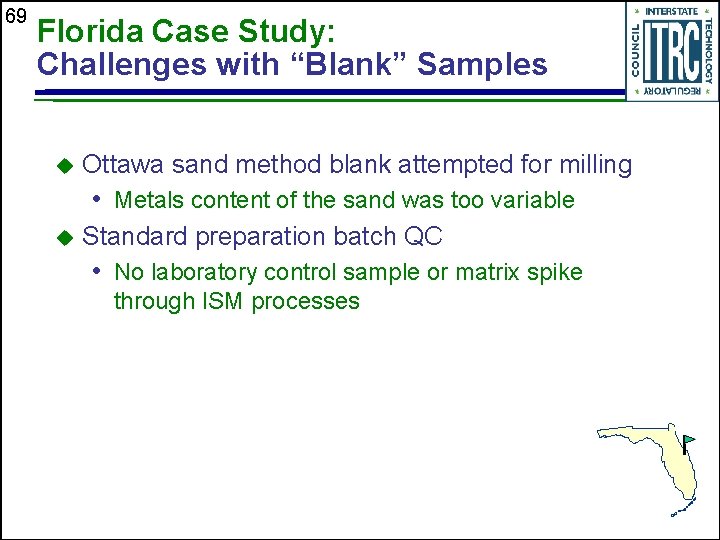
69 Florida Case Study: Challenges with “Blank” Samples u Ottawa sand method blank attempted for milling • Metals content of the sand was too variable u Standard preparation batch QC • No laboratory control sample or matrix spike through ISM processes
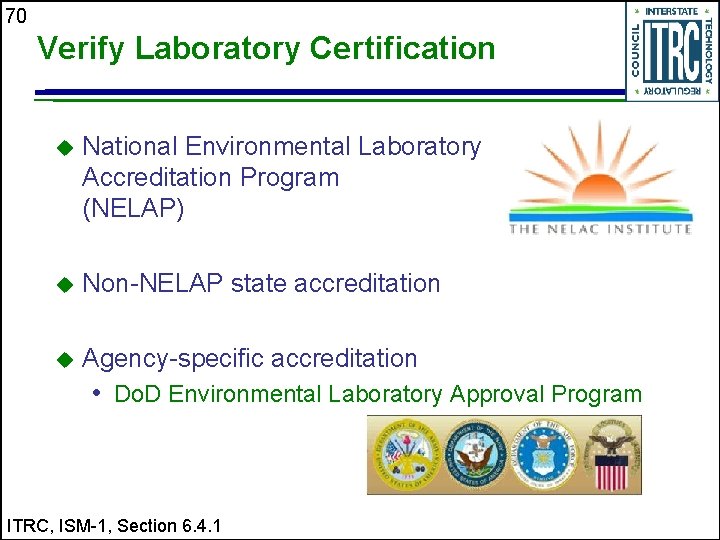
70 Verify Laboratory Certification u National Environmental Laboratory Accreditation Program (NELAP) u Non-NELAP state accreditation u Agency-specific accreditation • Do. D Environmental Laboratory Approval Program ITRC, ISM-1, Section 6. 4. 1
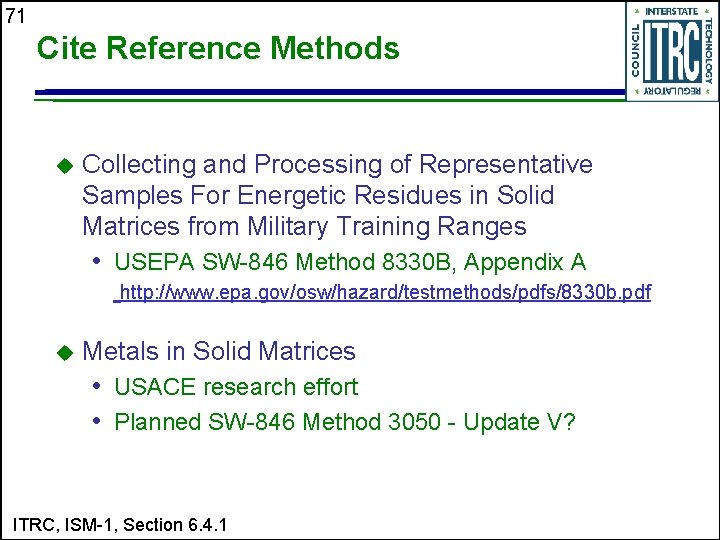
71 Cite Reference Methods u Collecting and Processing of Representative Samples For Energetic Residues in Solid Matrices from Military Training Ranges • USEPA SW-846 Method 8330 B, Appendix A http: //www. epa. gov/osw/hazard/testmethods/pdfs/8330 b. pdf u Metals in Solid Matrices • USACE research effort • Planned SW-846 Method 3050 - Update V? ITRC, ISM-1, Section 6. 4. 1
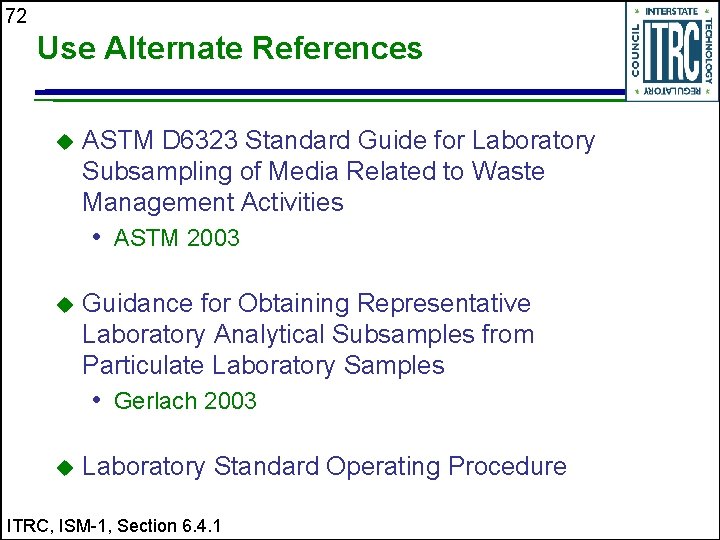
72 Use Alternate References u ASTM D 6323 Standard Guide for Laboratory Subsampling of Media Related to Waste Management Activities • ASTM 2003 u Guidance for Obtaining Representative Laboratory Analytical Subsamples from Particulate Laboratory Samples • Gerlach 2003 u Laboratory Standard Operating Procedure ITRC, ISM-1, Section 6. 4. 1
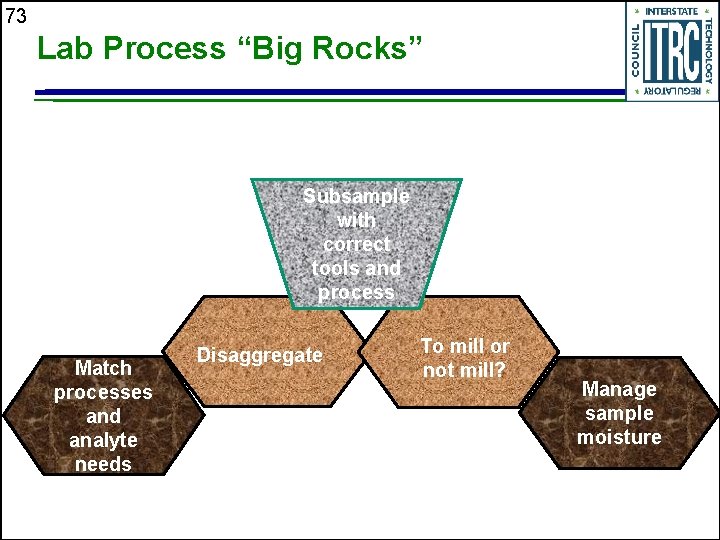
73 Lab Process “Big Rocks” Subsample with correct tools and process Match processes and analyte needs Disaggregate To mill or not mill? Manage sample moisture
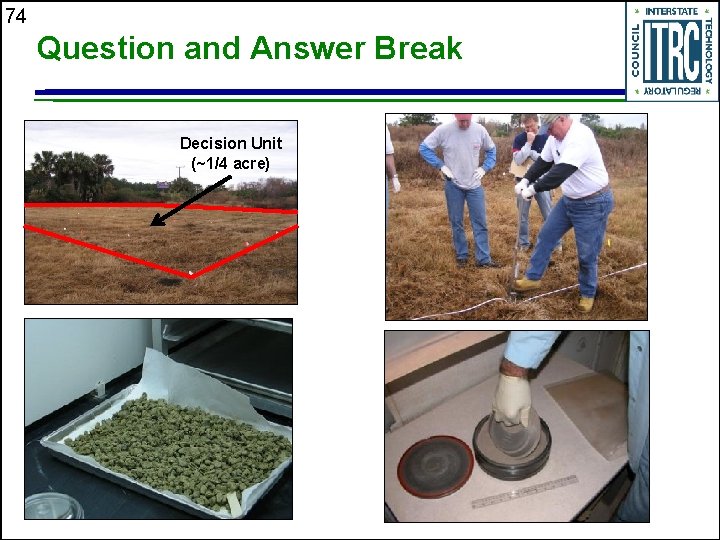
74 Question and Answer Break Decision Unit (~1/4 acre)
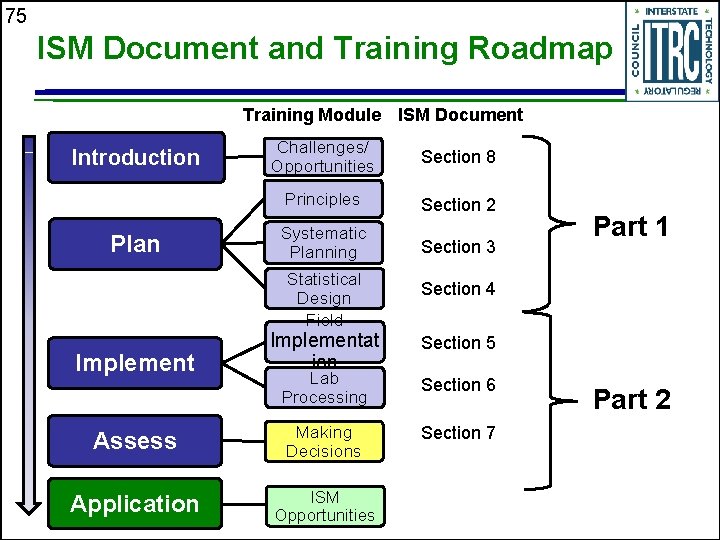
75 ISM Document and Training Roadmap Training Module ISM Document Introduction Plan Challenges/ Opportunities Section 8 Principles Section 2 Systematic Planning Statistical Design Field Implementat ion Section 3 Part 1 Section 4 Section 5 Lab Processing Section 6 Assess Making Decisions Section 7 Application ISM Opportunities Part 2
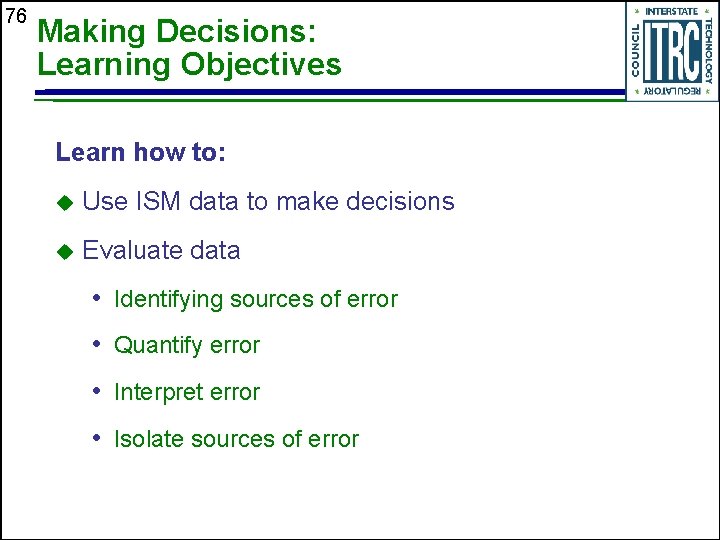
76 Making Decisions: Learning Objectives Learn how to: u Use ISM data to make decisions u Evaluate data • Identifying sources of error • Quantify error • Interpret error • Isolate sources of error
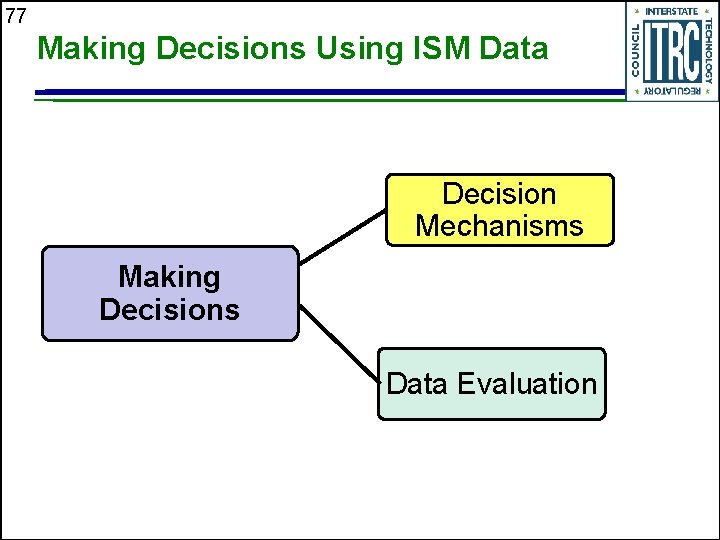
77 Making Decisions Using ISM Data Decision Mechanisms Making Decisions Data Evaluation
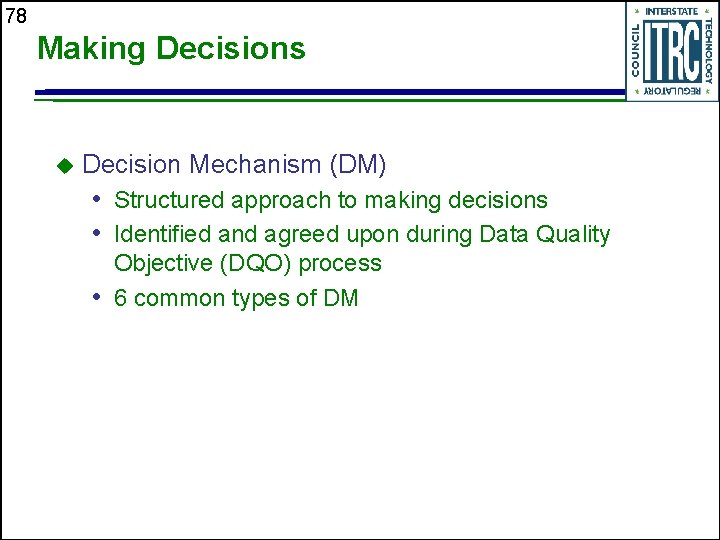
78 Making Decisions u Decision Mechanism (DM) • Structured approach to making decisions • Identified and agreed upon during Data Quality Objective (DQO) process • 6 common types of DM
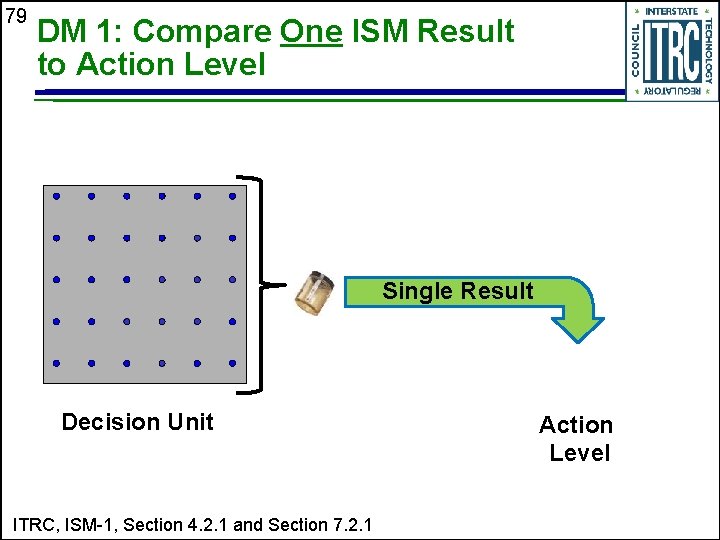
79 DM 1: Compare One ISM Result to Action Level Single Result Decision Unit ITRC, ISM-1, Section 4. 2. 1 and Section 7. 2. 1 Action Level
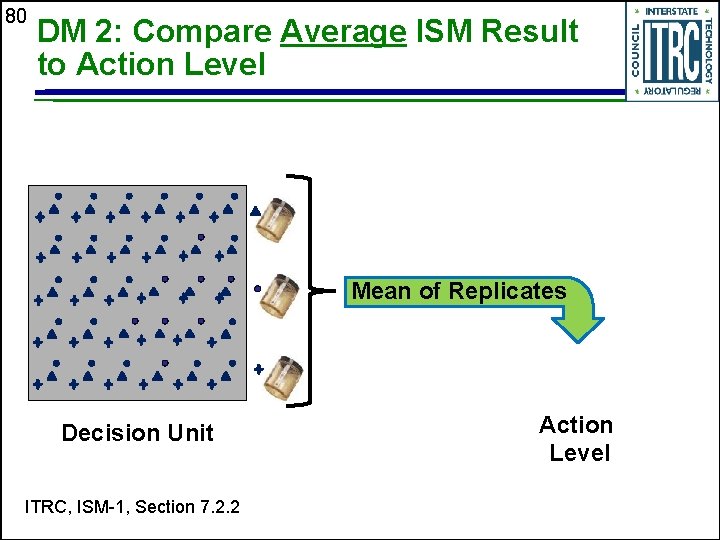
80 DM 2: Compare Average ISM Result to Action Level Mean of Replicates Decision Unit ITRC, ISM-1, Section 7. 2. 2 Action Level
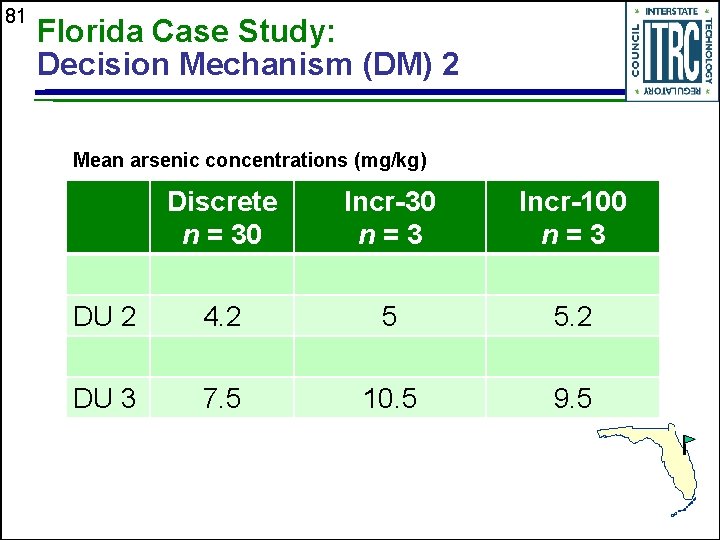
81 Florida Case Study: Decision Mechanism (DM) 2 Mean arsenic concentrations (mg/kg) Discrete n = 30 Incr-30 n=3 Incr-100 n=3 DU 2 4. 2 5 5. 2 DU 3 7. 5 10. 5 9. 5
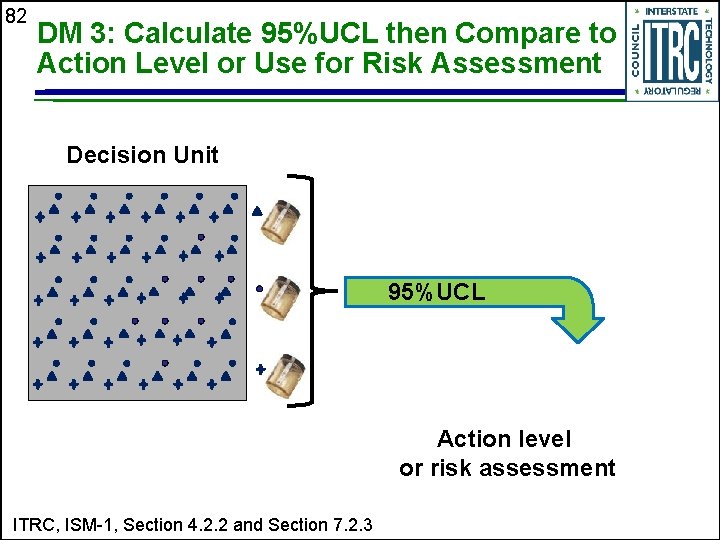
82 DM 3: Calculate 95%UCL then Compare to Action Level or Use for Risk Assessment Decision Unit 95%UCL Action level or risk assessment ITRC, ISM-1, Section 4. 2. 2 and Section 7. 2. 3
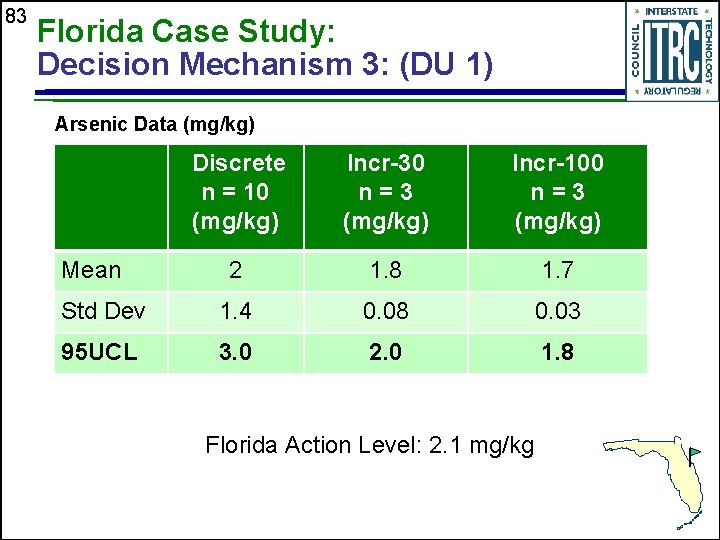
83 Florida Case Study: Decision Mechanism 3: (DU 1) Arsenic Data (mg/kg) Discrete n = 10 (mg/kg) Incr-30 n=3 (mg/kg) Incr-100 n=3 (mg/kg) 2 1. 8 1. 7 Std Dev 1. 4 0. 08 0. 03 95 UCL 3. 0 2. 0 1. 8 Mean Florida Action Level: 2. 1 mg/kg
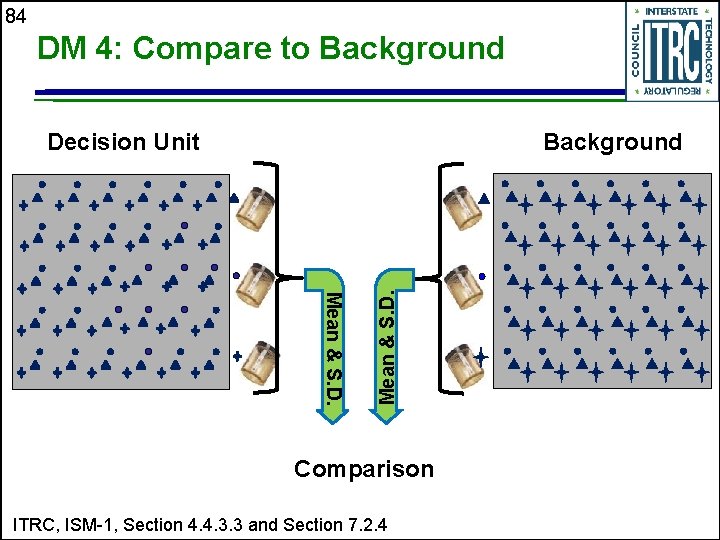
84 DM 4: Compare to Background Decision Unit Mean & S. D. Background Comparison ITRC, ISM-1, Section 4. 4. 3. 3 and Section 7. 2. 4
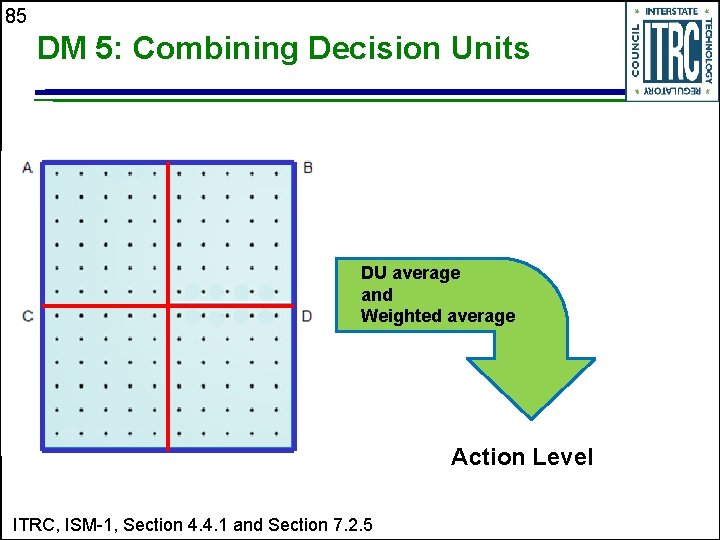
85 DM 5: Combining Decision Units DU average and Weighted average Action Level ITRC, ISM-1, Section 4. 4. 1 and Section 7. 2. 5
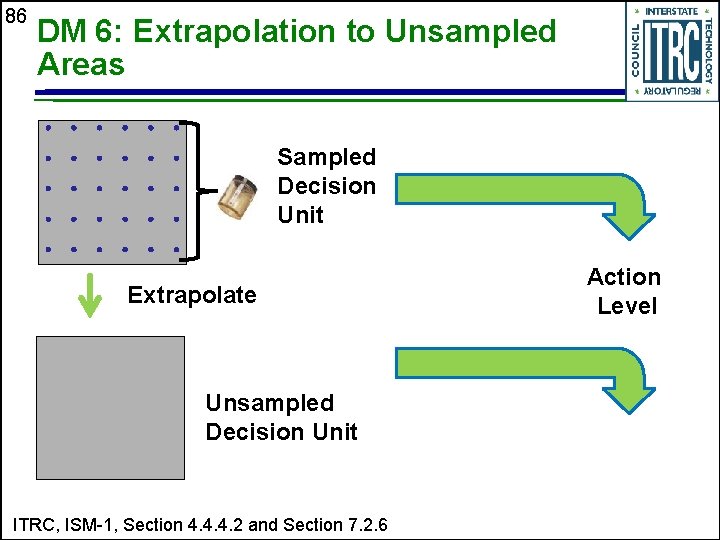
86 DM 6: Extrapolation to Unsampled Areas Sampled Decision Unit Extrapolate Unsampled Decision Unit ITRC, ISM-1, Section 4. 4. 4. 2 and Section 7. 2. 6 Action Level
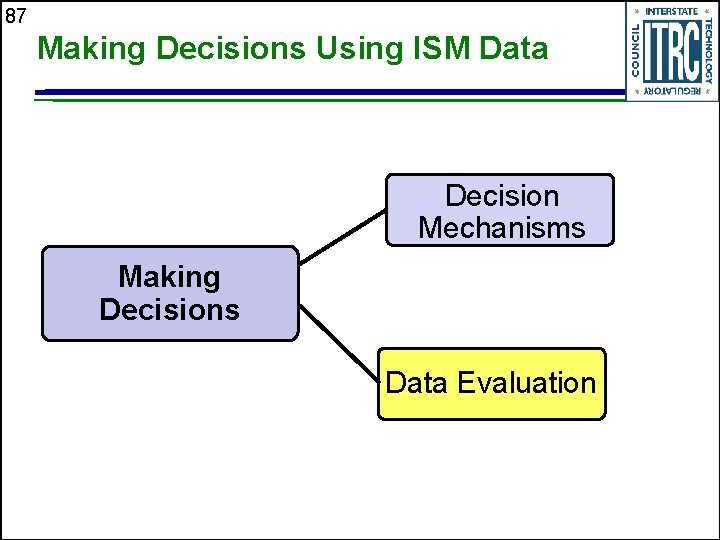
87 Making Decisions Using ISM Data Decision Mechanisms Making Decisions Data Evaluation
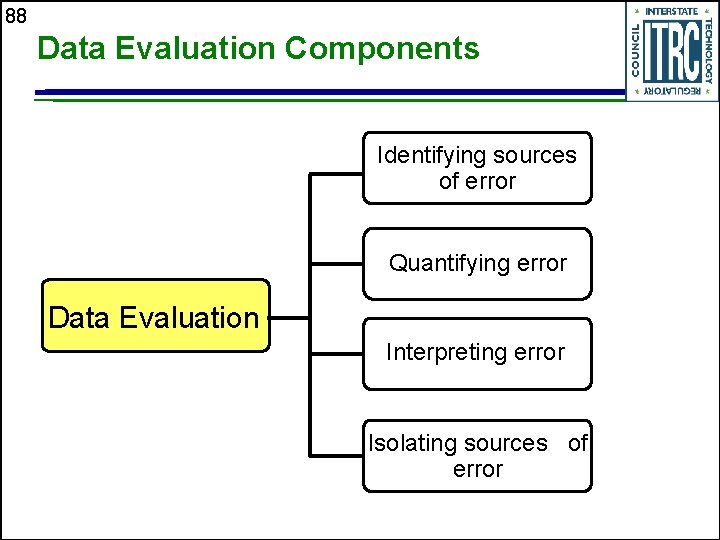
88 Data Evaluation Components Identifying sources of error Quantifying error Data Evaluation Interpreting error Isolating sources of error
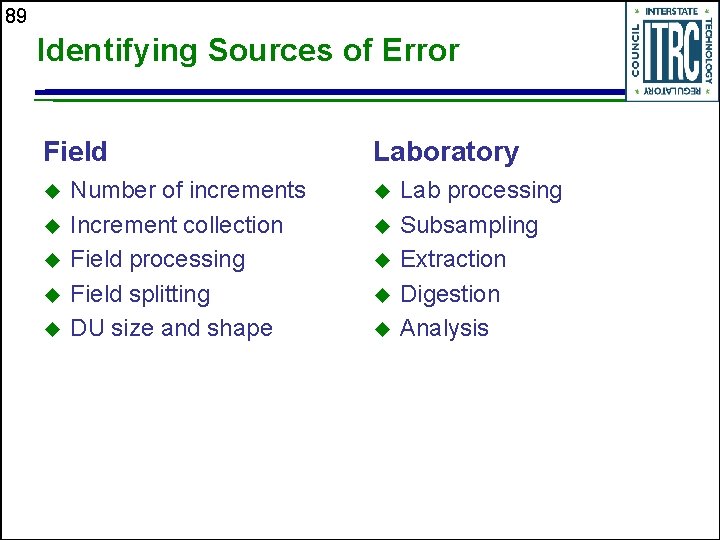
89 Identifying Sources of Error Field u u u Number of increments Increment collection Field processing Field splitting DU size and shape Laboratory u u u Lab processing Subsampling Extraction Digestion Analysis
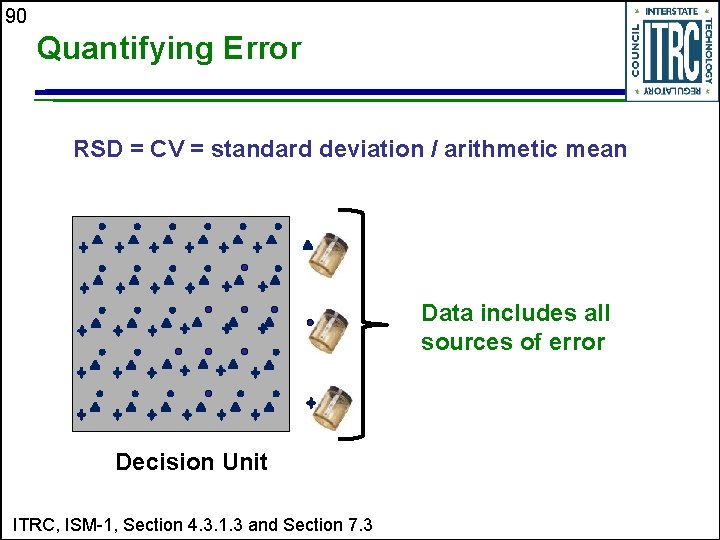
90 Quantifying Error RSD = CV = standard deviation / arithmetic mean Data includes all sources of error Decision Unit ITRC, ISM-1, Section 4. 3. 1. 3 and Section 7. 3
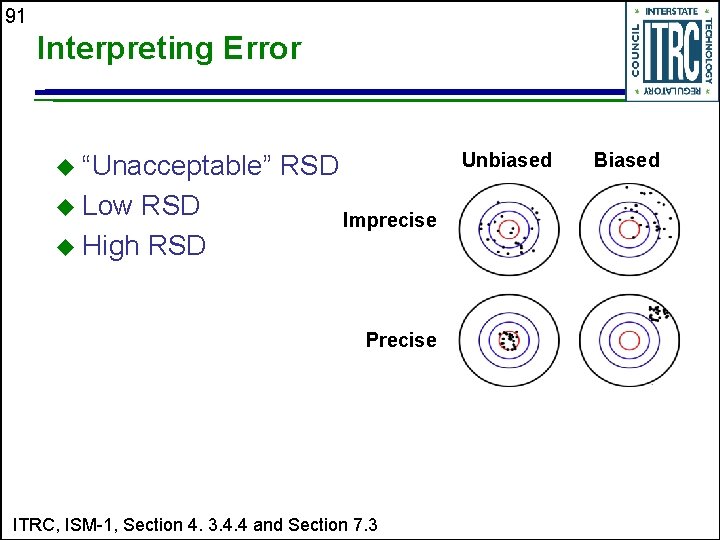
91 Interpreting Error Unbiased u “Unacceptable” RSD u Low RSD u High RSD Imprecise Precise ITRC, ISM-1, Section 4. 3. 4. 4 and Section 7. 3 Biased
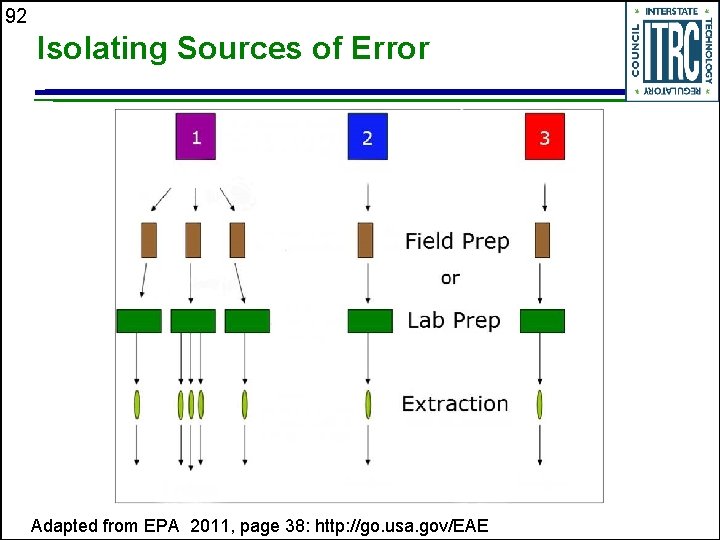
92 Isolating Sources of Error Adapted from EPA 2011, page 38: http: //go. usa. gov/EAE
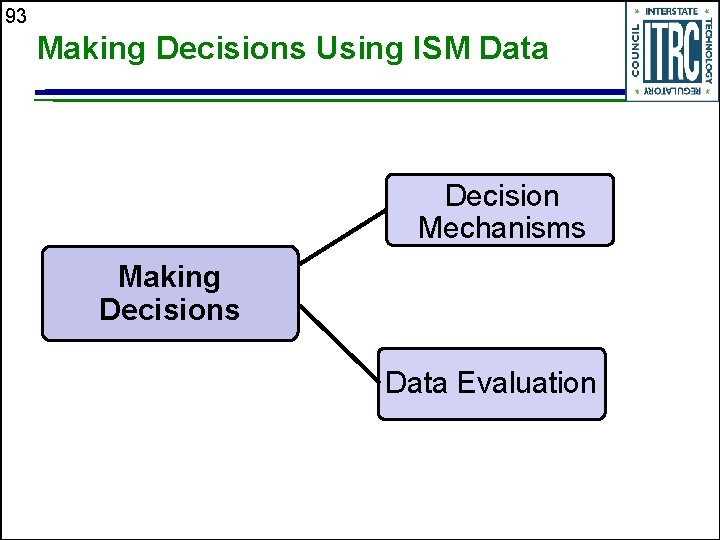
93 Making Decisions Using ISM Data Decision Mechanisms Making Decisions Data Evaluation
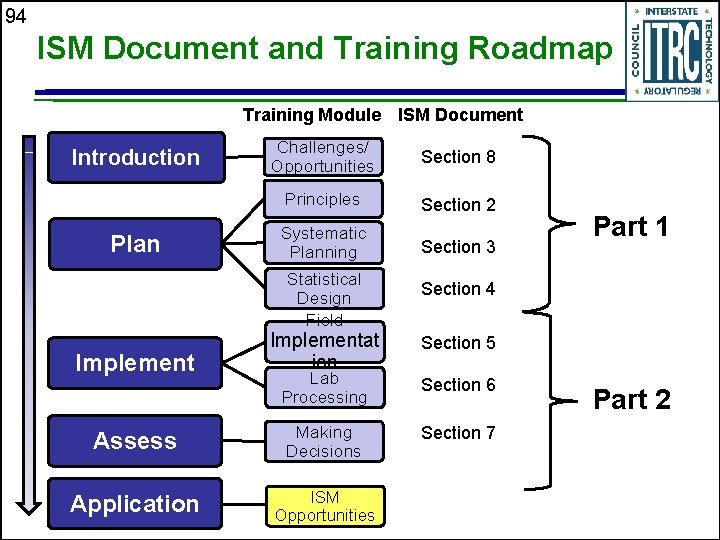
94 ISM Document and Training Roadmap Training Module ISM Document Introduction Plan Challenges/ Opportunities Section 8 Principles Section 2 Systematic Planning Statistical Design Field Implementat ion Section 3 Part 1 Section 4 Section 5 Lab Processing Section 6 Assess Making Decisions Section 7 Application ISM Opportunities Part 2
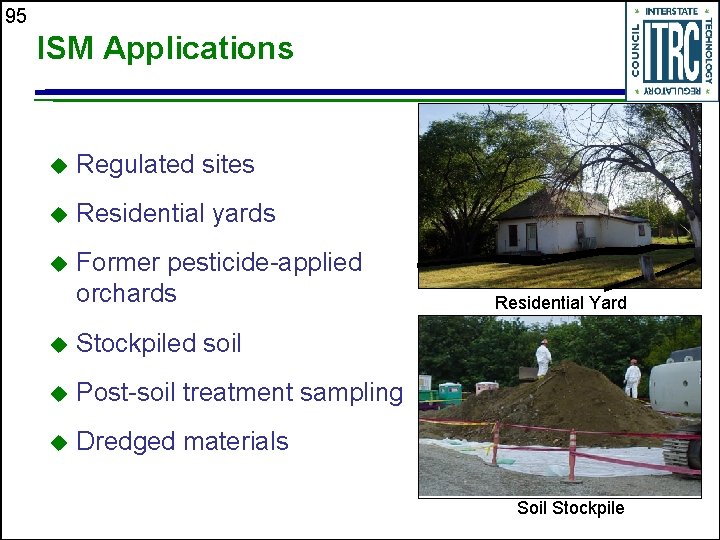
95 ISM Applications u Regulated sites u Residential yards u Former pesticide-applied orchards u Stockpiled soil u Post-soil treatment sampling u Dredged materials Residential Yard Soil Stockpile
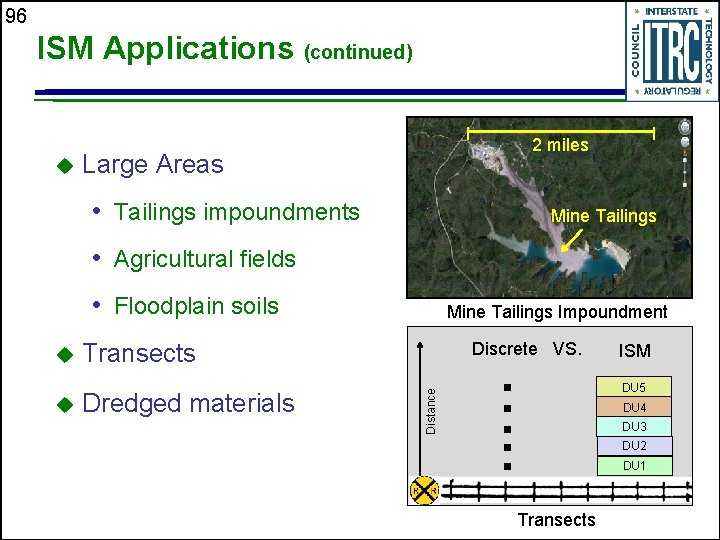
96 ISM Applications (continued) u 2 miles Large Areas • Tailings impoundments Mine Tailings • Agricultural fields • Floodplain soils u Discrete VS. Transects Dredged materials ISM DU 5 Distance u Mine Tailings Impoundment DU 4 DU 3 DU 2 DU 1 Transects
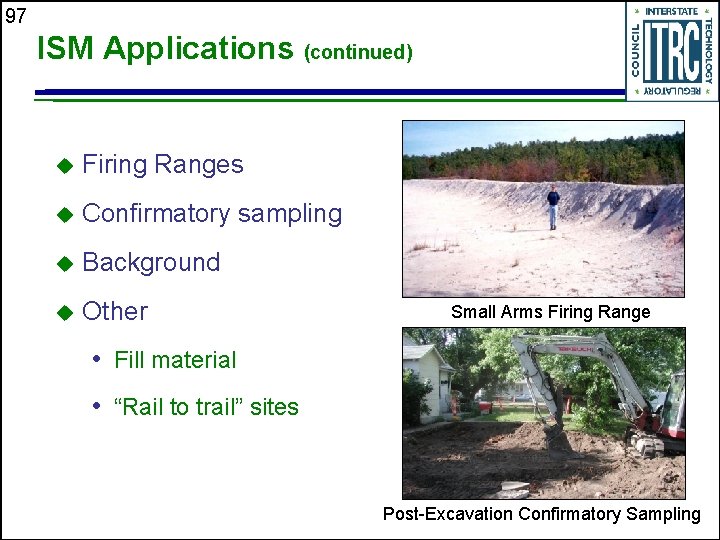
97 ISM Applications (continued) u Firing Ranges u Confirmatory sampling u Background u Other Small Arms Firing Range • Fill material • “Rail to trail” sites Post-Excavation Confirmatory Sampling
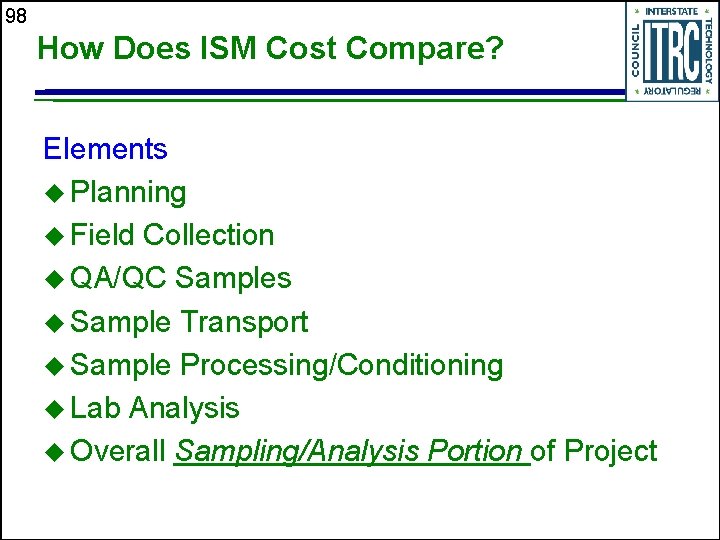
98 How Does ISM Cost Compare? Elements u Planning u Field Collection u QA/QC Samples u Sample Transport u Sample Processing/Conditioning u Lab Analysis u Overall Sampling/Analysis Portion of Project
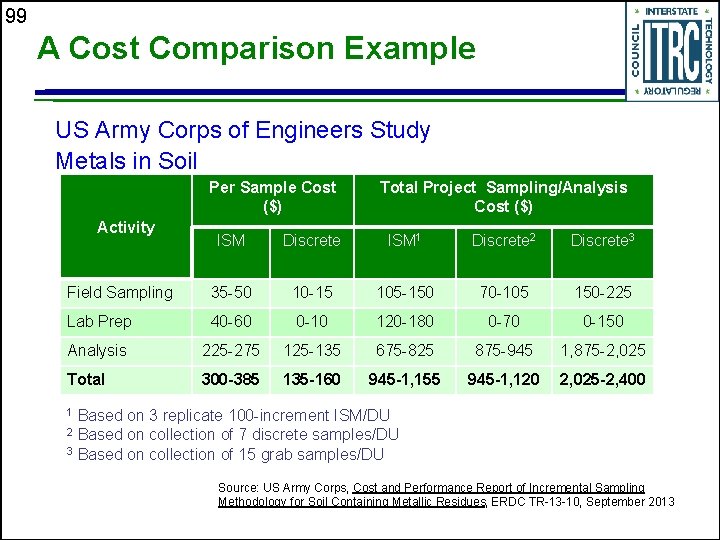
99 A Cost Comparison Example US Army Corps of Engineers Study Metals in Soil Per Sample Cost ($) Activity Total Project Sampling/Analysis Cost ($) ISM Discrete ISM 1 Discrete 2 Discrete 3 Field Sampling 35 -50 10 -15 105 -150 70 -105 150 -225 Lab Prep 40 -60 0 -10 120 -180 0 -70 0 -150 Analysis 225 -275 125 -135 675 -825 875 -945 1, 875 -2, 025 Total 300 -385 135 -160 945 -1, 155 945 -1, 120 2, 025 -2, 400 1 Based on 3 replicate 100 -increment ISM/DU 2 Based on collection of 7 discrete samples/DU 3 Based on collection of 15 grab samples/DU Source: US Army Corps, Cost and Performance Report of Incremental Sampling Methodology for Soil Containing Metallic Residues, ERDC TR-13 -10, September 2013
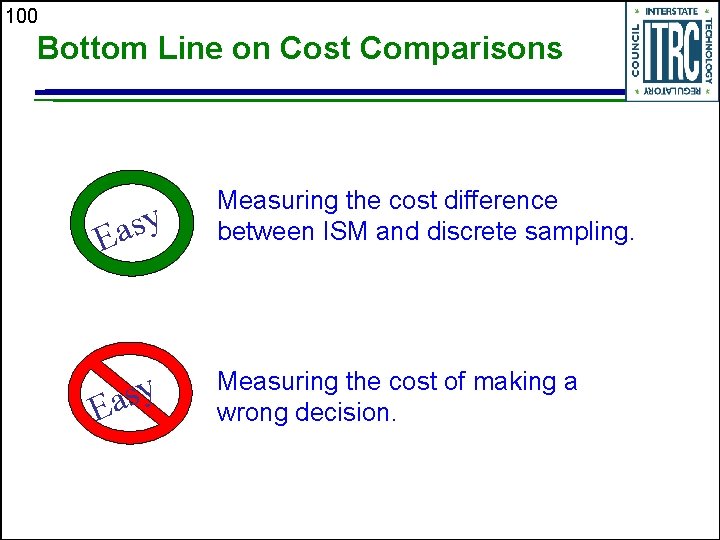
100 Bottom Line on Cost Comparisons y s a E Measuring the cost difference between ISM and discrete sampling. Measuring the cost of making a wrong decision.
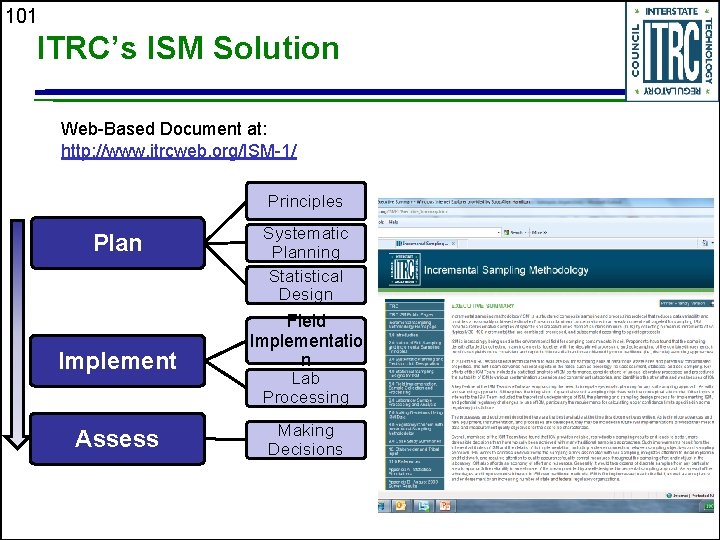
101 ITRC’s ISM Solution Web-Based Document at: http: //www. itrcweb. org/ISM-1/ Principles Plan Systematic Planning Statistical Design Implement Field Implementatio n Lab Processing Assess Making Decisions
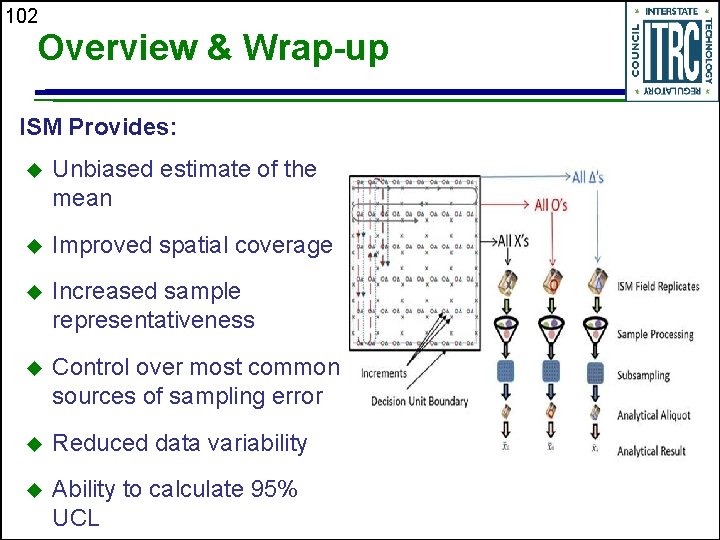
102 Overview & Wrap-up ISM Provides: u Unbiased estimate of the mean u Improved spatial coverage u Increased sample representativeness u Control over most common sources of sampling error u Reduced data variability u Ability to calculate 95% UCL
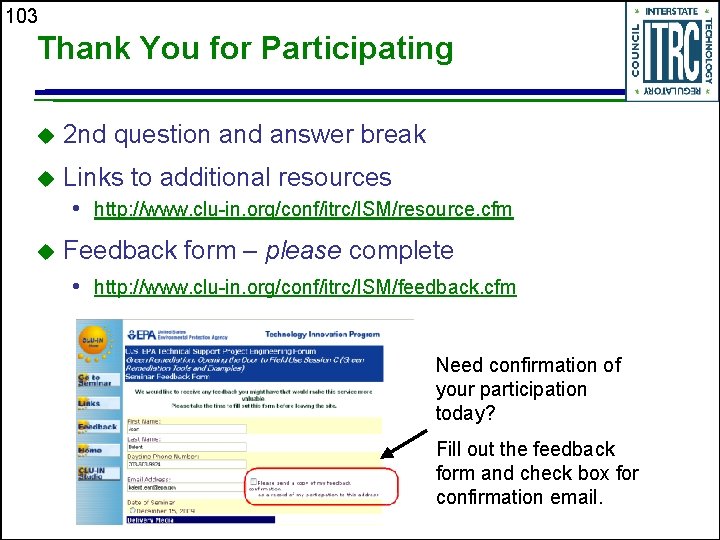
103 Thank You for Participating u 2 nd question and answer break u Links to additional resources • http: //www. clu-in. org/conf/itrc/ISM/resource. cfm u Feedback form – please complete • http: //www. clu-in. org/conf/itrc/ISM/feedback. cfm Need confirmation of your participation today? Fill out the feedback form and check box for confirmation email.
Welcome thanks for joining us
Olve
Welcome thanks for joining us
Emdtech
Welcome and thank you for joining us
Welcome and thank you for joining us
Wise men three clever are we
Iso 22301 utbildning
Novell typiska drag
Tack för att ni lyssnade bild
Ekologiskt fotavtryck
Shingelfrisyren
En lathund för arbete med kontinuitetshantering
Personalliggare bygg undantag
Personlig tidbok för yrkesförare
Sura för anatom
Vad är densitet
Datorkunskap för nybörjare
Boverket ka
Mall debattartikel
För och nackdelar med firo
Nyckelkompetenser för livslångt lärande
Påbyggnader för flakfordon
Vätsketryck formel
Publik sektor
Lyckans minut erik lindorm analys
Presentera för publik crossboss
Teckenspråk minoritetsspråk argument
Vem räknas som jude
Treserva lathund
Fimbrietratt
Bästa kameran för astrofoto
Cks
Byggprocessen steg för steg
Mat för unga idrottare
Verktyg för automatisering av utbetalningar
Rutin för avvikelsehantering
Smärtskolan kunskap för livet
Ministerstyre för och nackdelar
Tack för att ni har lyssnat
Referat mall
Redogör för vad psykologi är
Borstål, egenskaper
Atmosfr
Borra hål för knoppar
Orubbliga rättigheter
Variansen formel
Tack för att ni har lyssnat
Steg för steg rita
Verksamhetsanalys exempel
Tobinskatten för och nackdelar
Toppslätskivling effekt
Mästare lärling modell
Egg för emanuel
Elektronik för barn
Fredsgudinna pax
Strategi för svensk viltförvaltning
Kung dog 1611
Ellika andolf
Romarriket tidslinje
Tack för att ni lyssnade
Mindre än tecken
Dikt bunden form
Inköpsprocessen steg för steg
Rbk-mätning
Etik och ledarskap etisk kod för chefer
Expektans
Myndigheten för delaktighet
Trög för kemist
Tillitsbaserad ledning
Läkarutlåtande för livränta
Sten karttecken
Skapa med geometriska former
Vishnuiter
Biologiska arvet
Bris för vuxna
Big brother rösta
Welcome to the training session
Alleluia alleluia give thanks to the risen lord lyrics
American satan cda
Thank you for listening ppt
Thanks for listen
Thanks for your attention doctor
Thanks for listening
How to reply thanks
Thanks any question
Give thanks to the lord our god and king
Christian kittl
Thanks to god for my redeemer
Oh give thanks to the lord for he is good
Thanks for your attention
謝千萬聲
Thanks for your listening.
Thanks for teamwork
Kwakiutl prayer of thanks
Thanks a lot for your letter
Vote of thanks speech for webinar
Give thanks psalms
Kwakiutl prayer of thanks
Dear ben thanks for your letter
Challengers leaders niche players visionaries
Give thanks michael w smith
First of all thanks to allah
Farabi is flora's best friend passage model question