UNIVERSIDADE DE SO PAULO CENTRO DE ENERGIA NUCLEAR
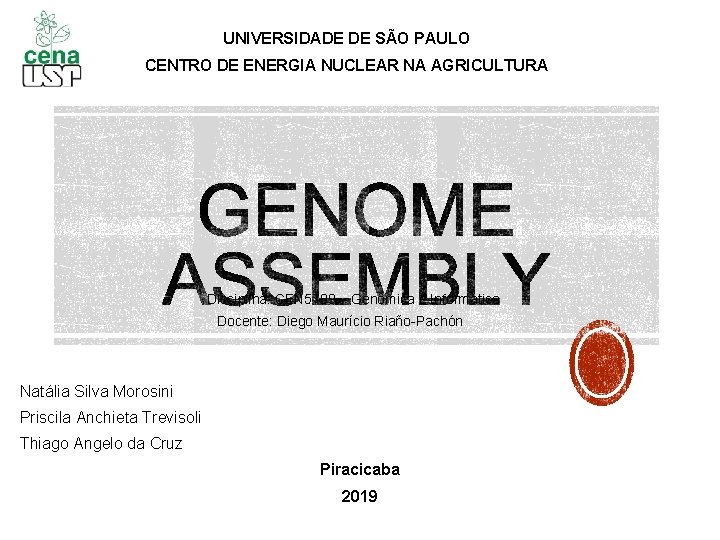
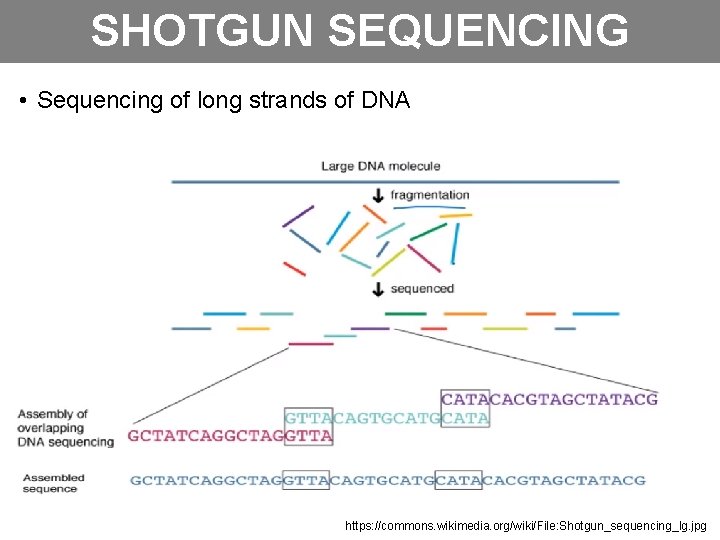
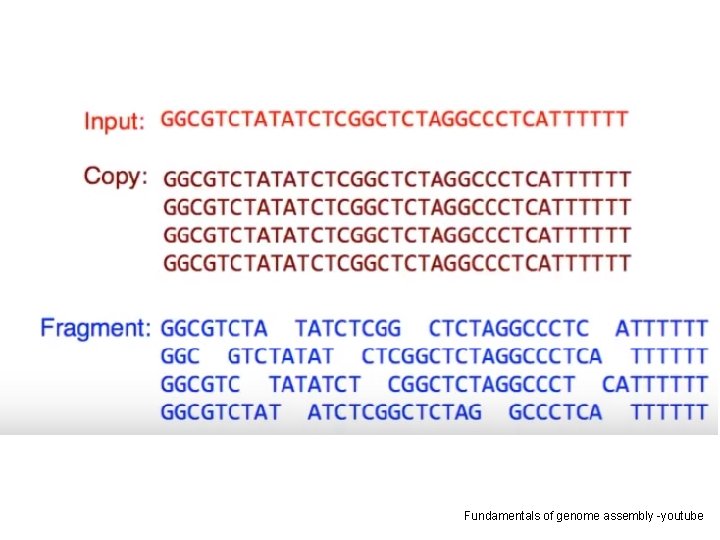
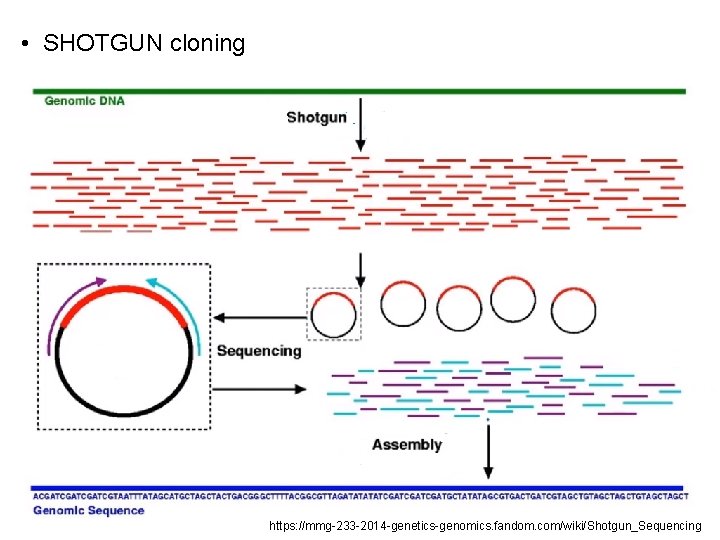
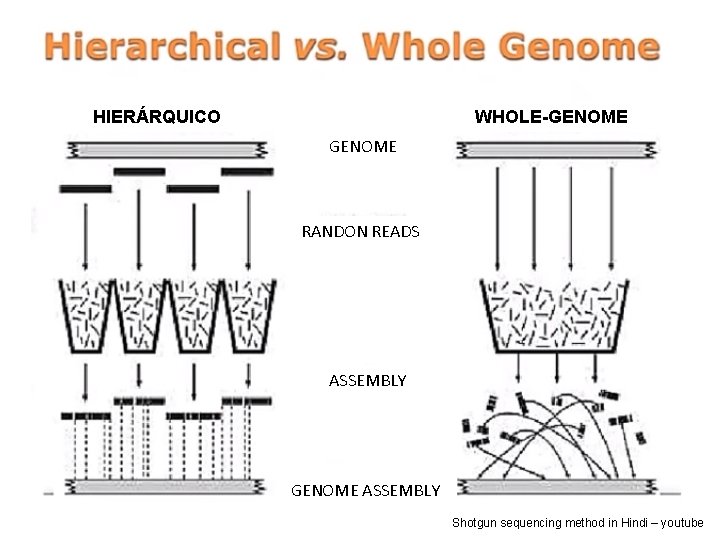
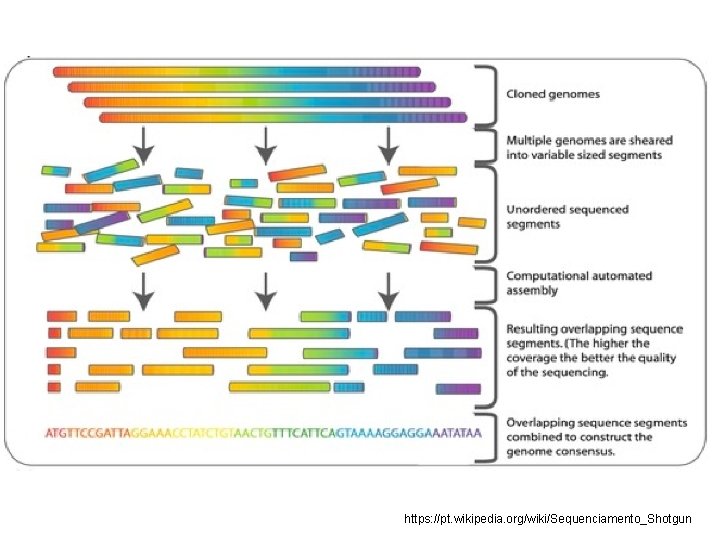
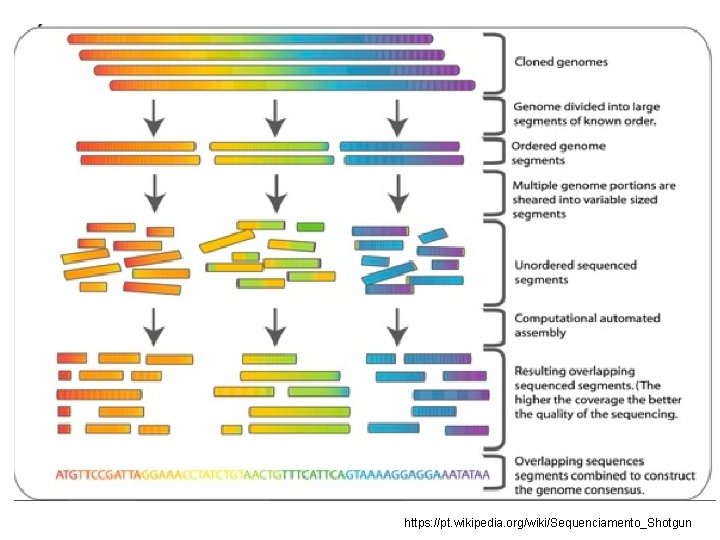
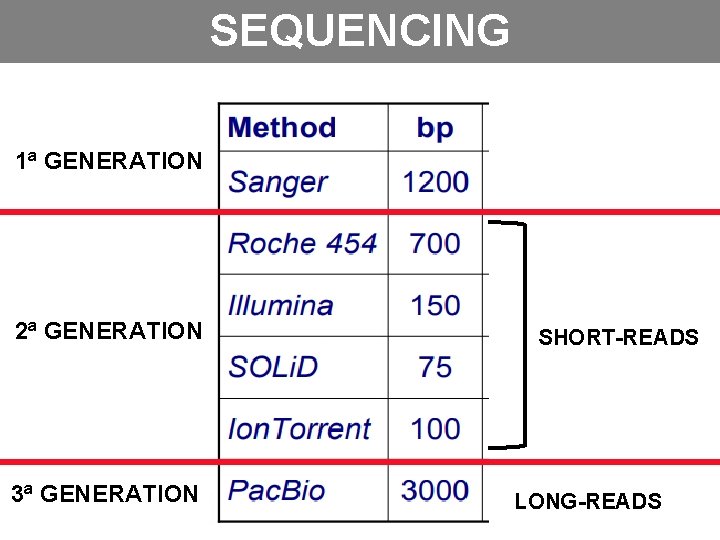
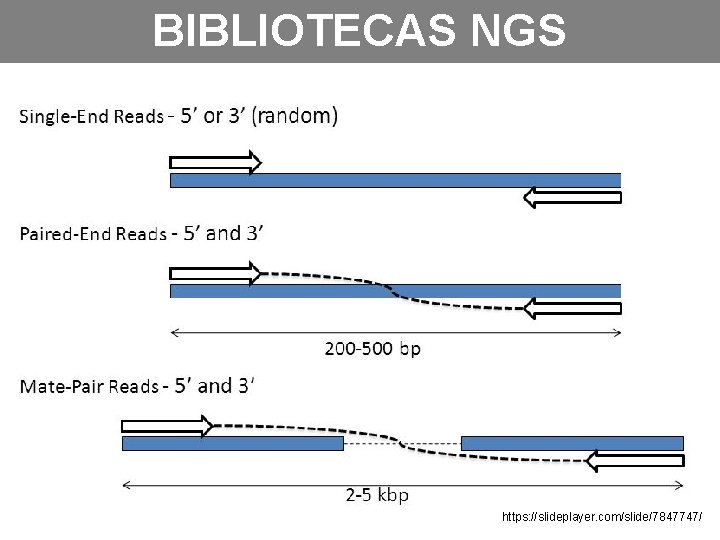
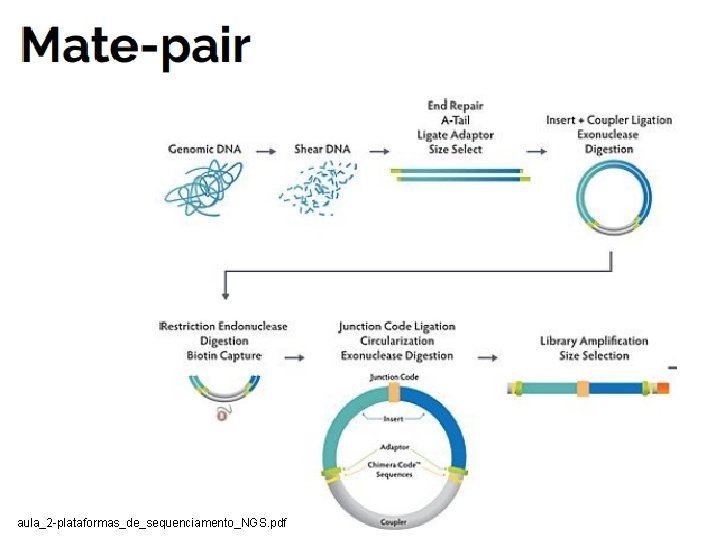
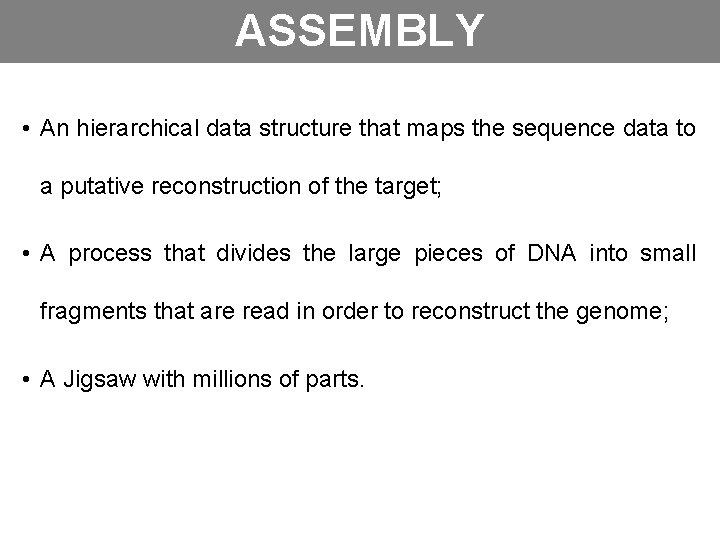
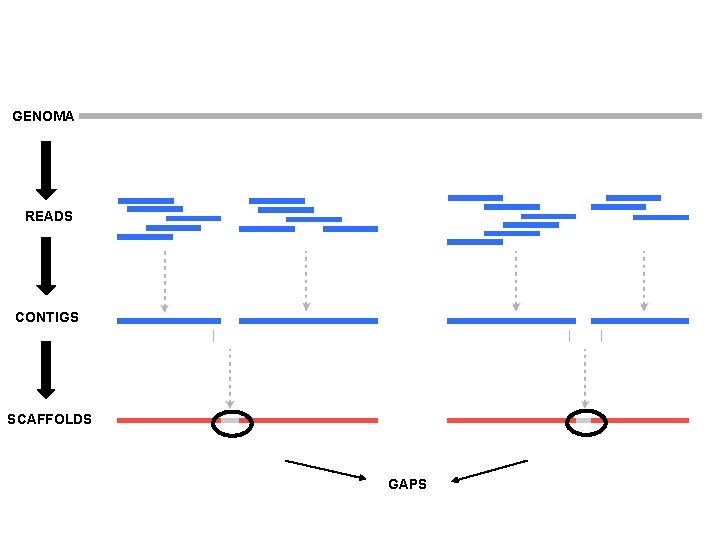
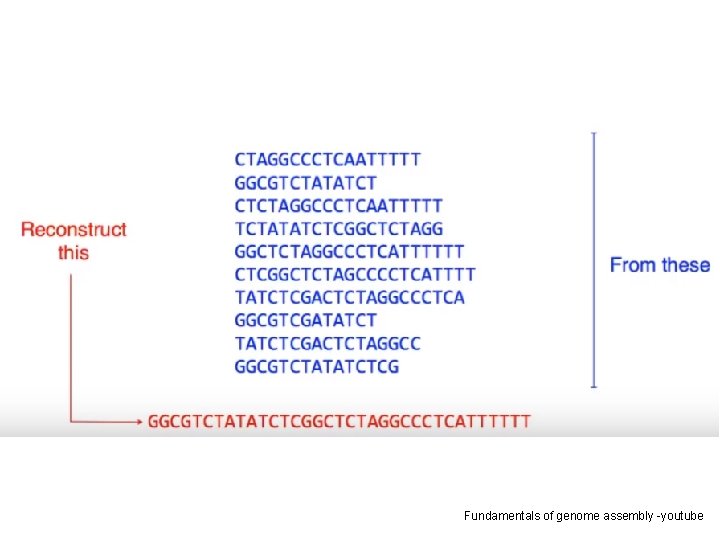
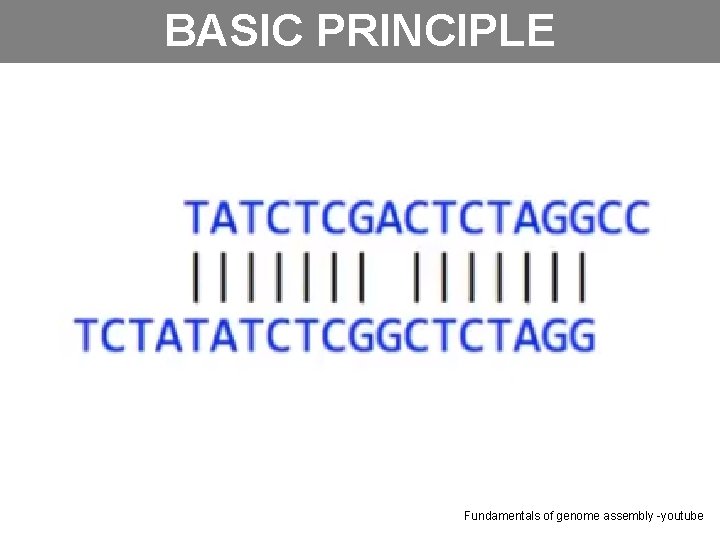
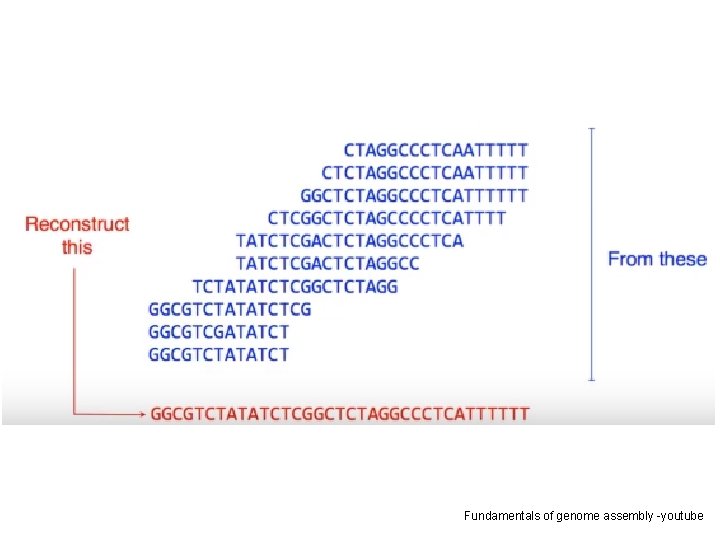
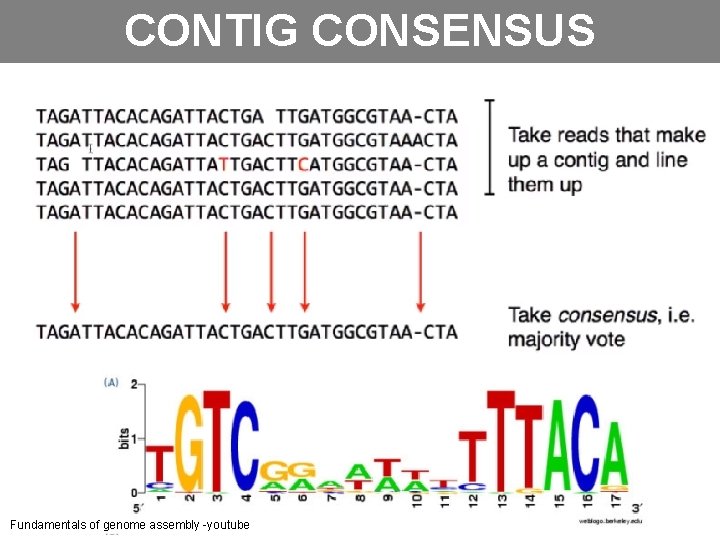
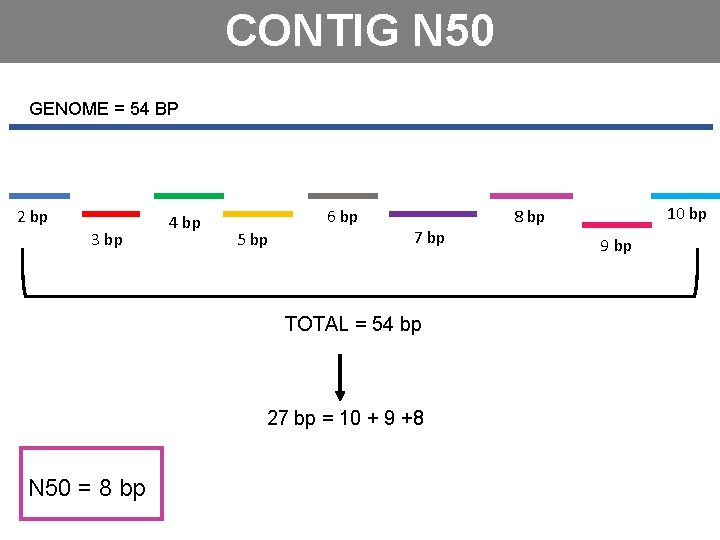
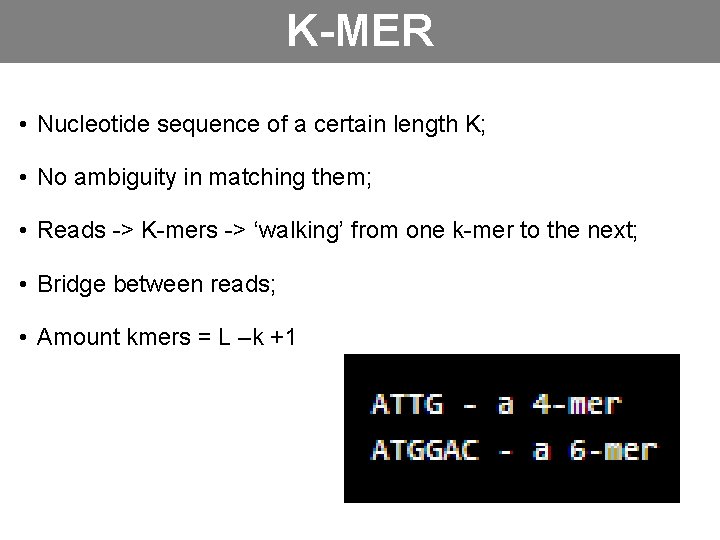
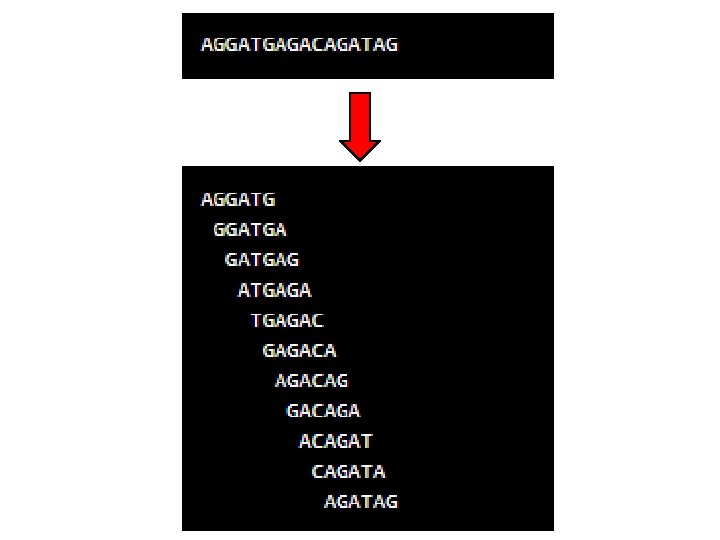
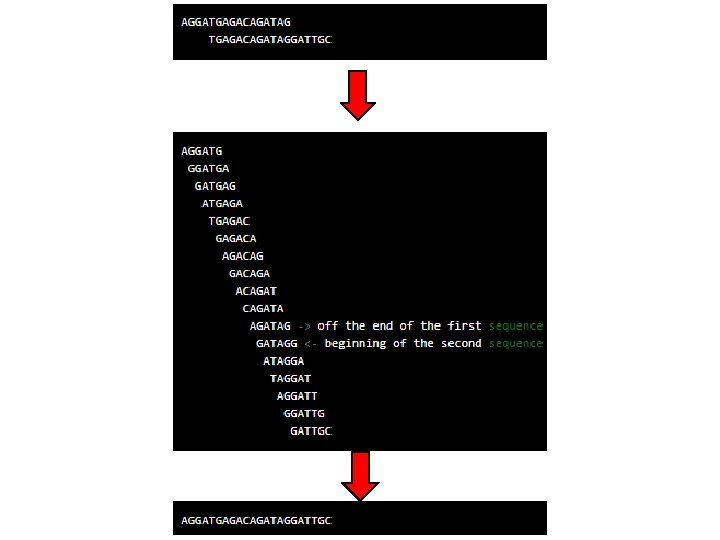
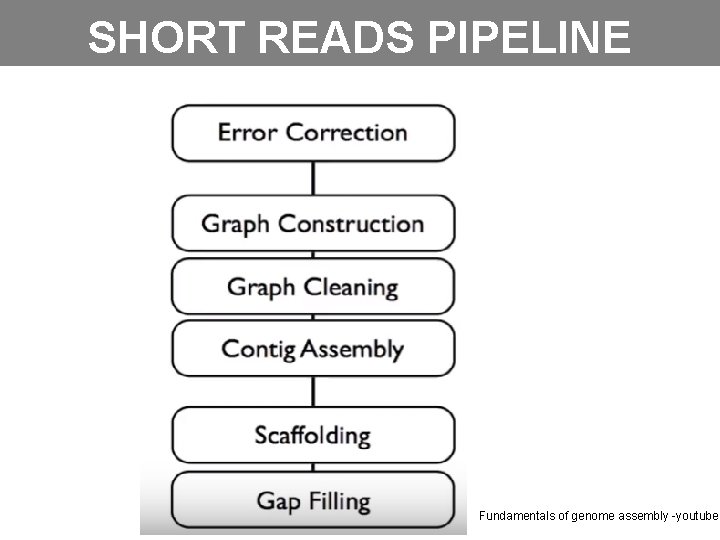
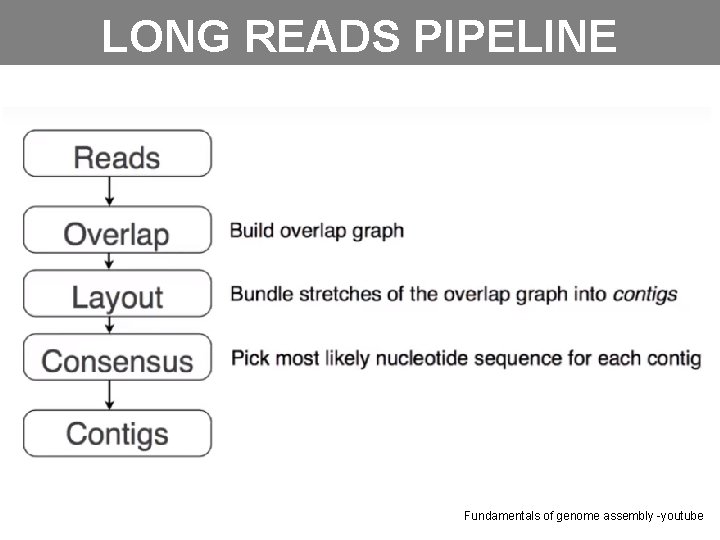
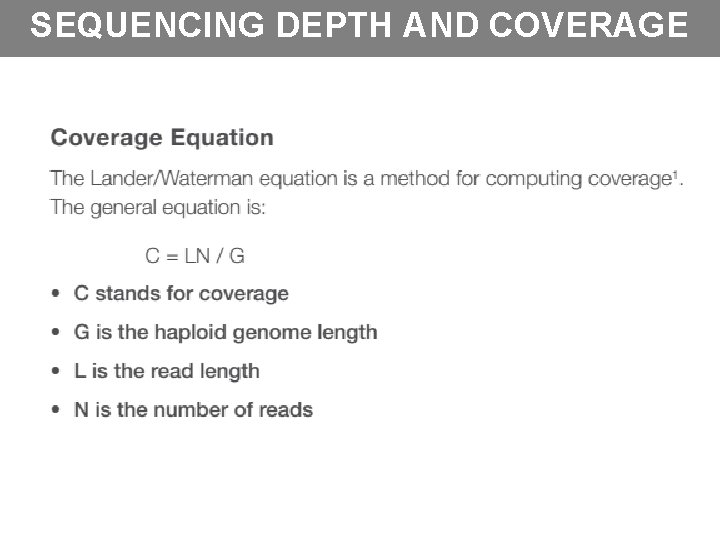
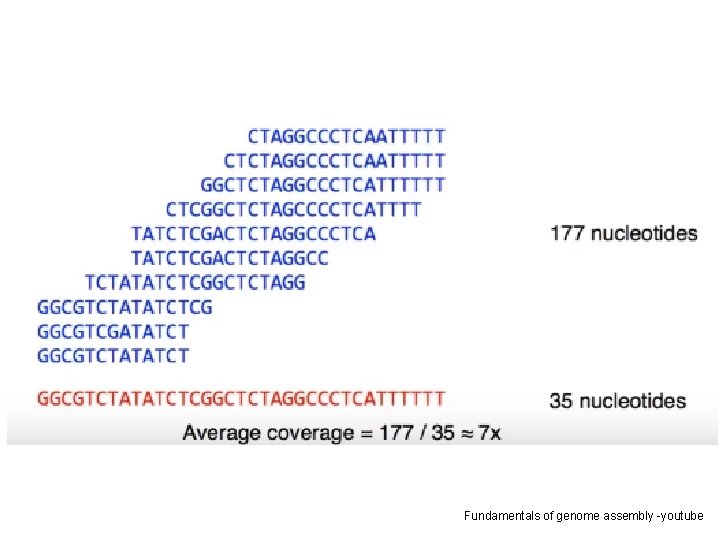
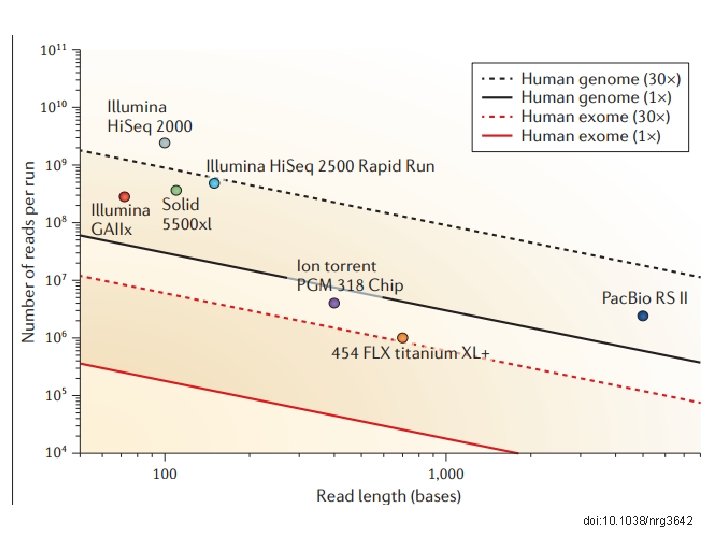
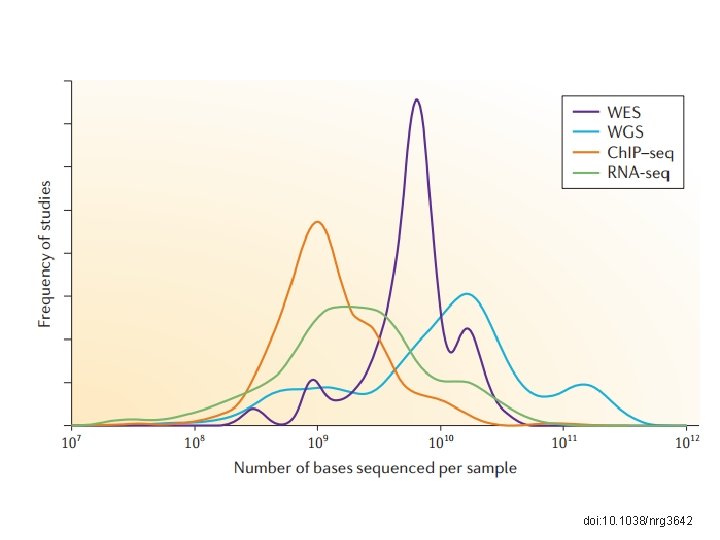
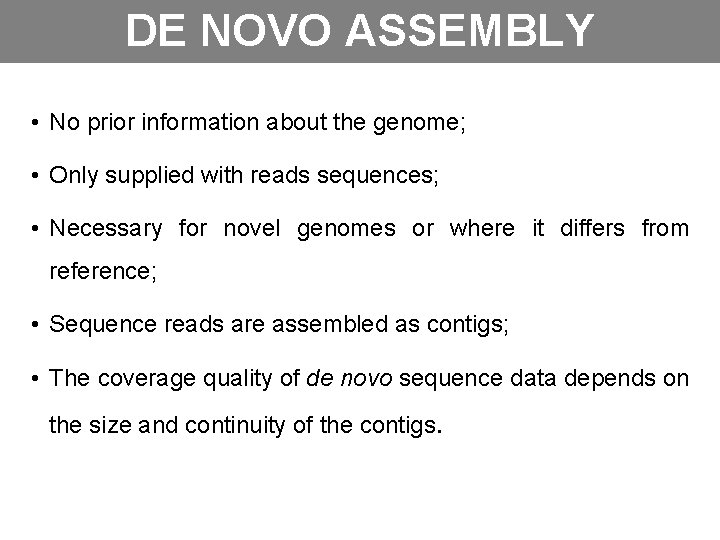
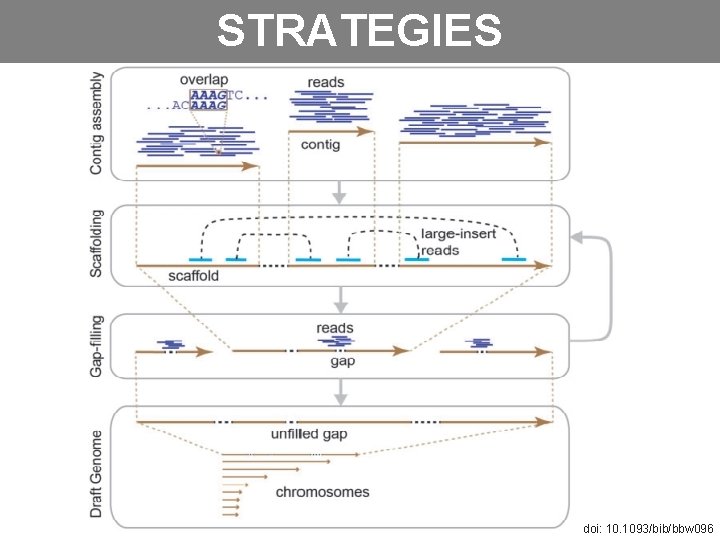
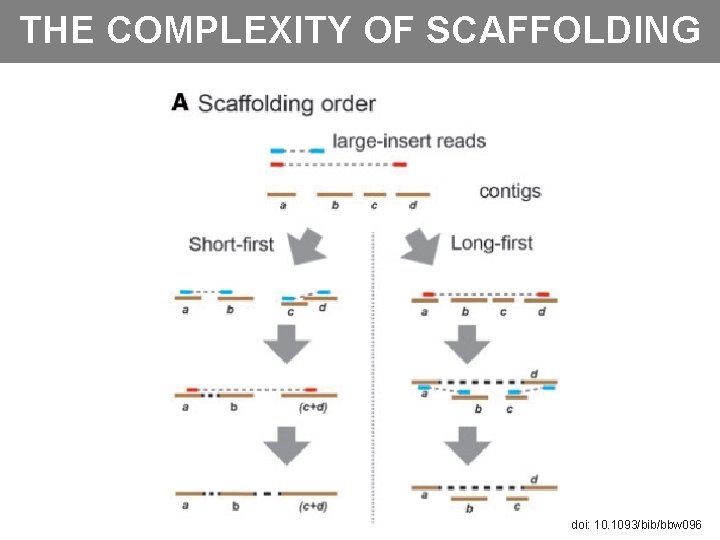
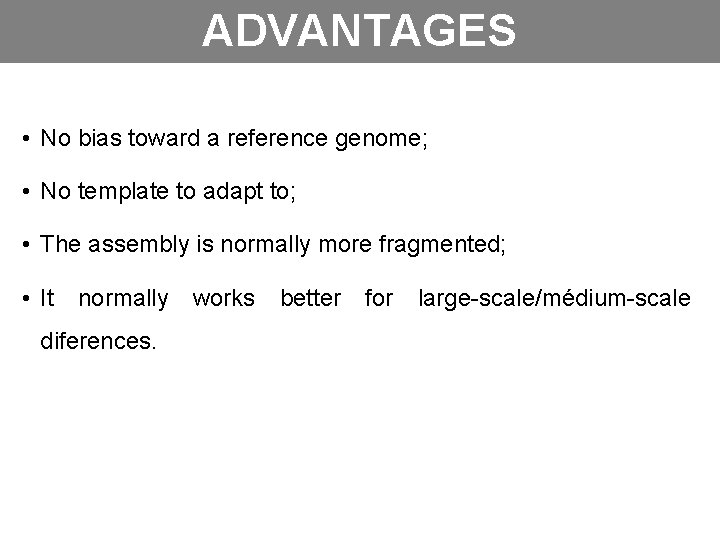
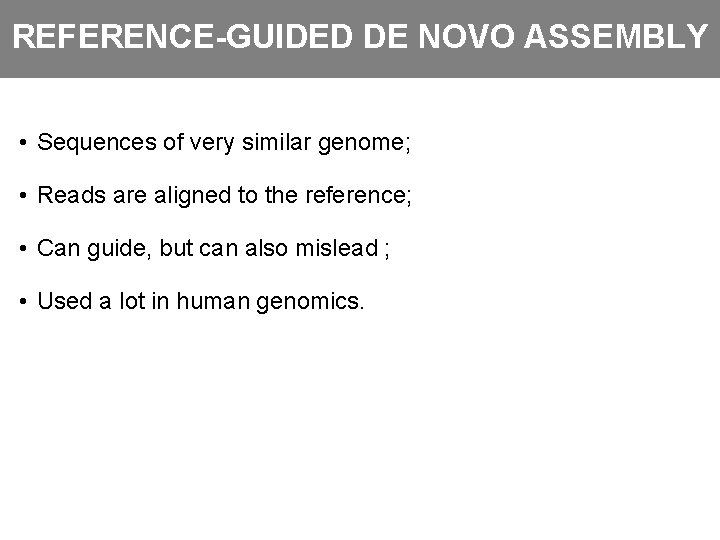
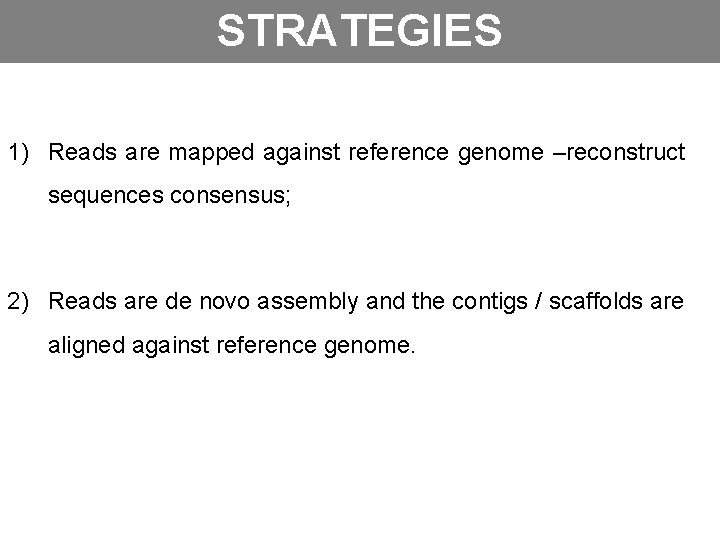
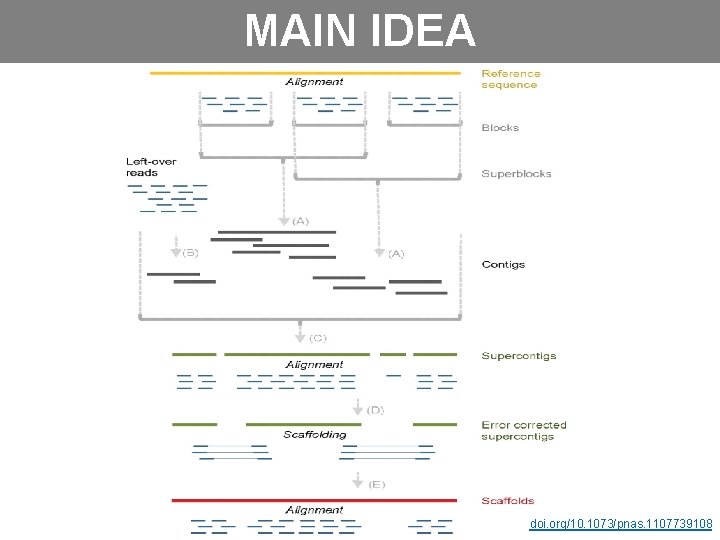
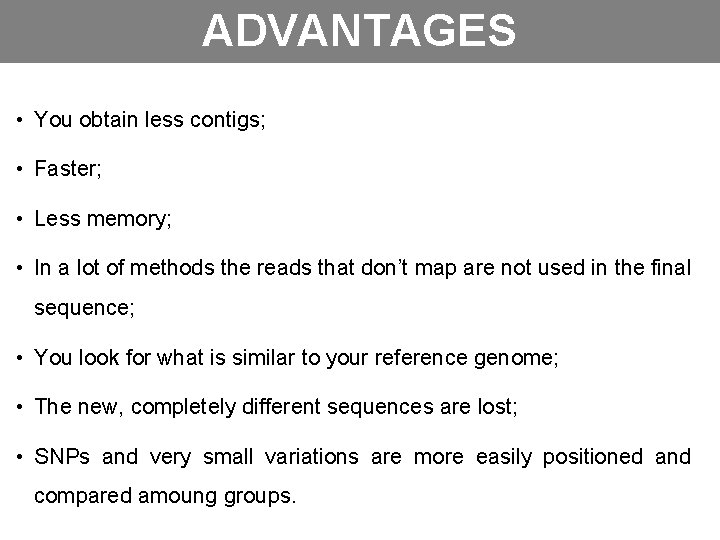
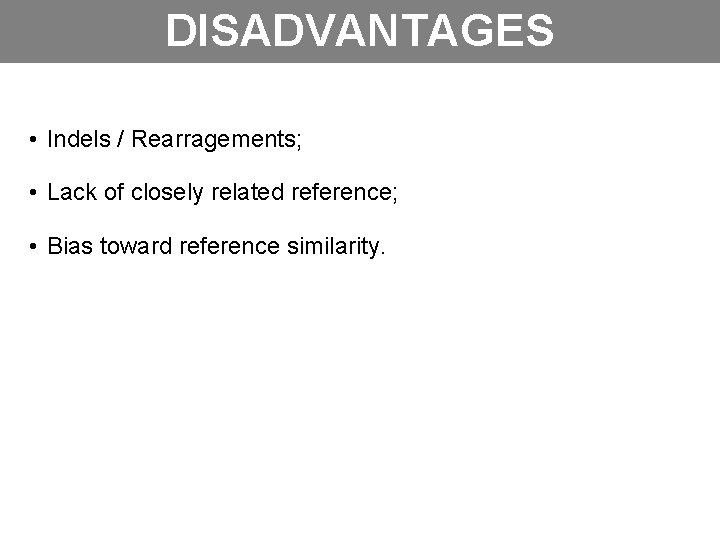
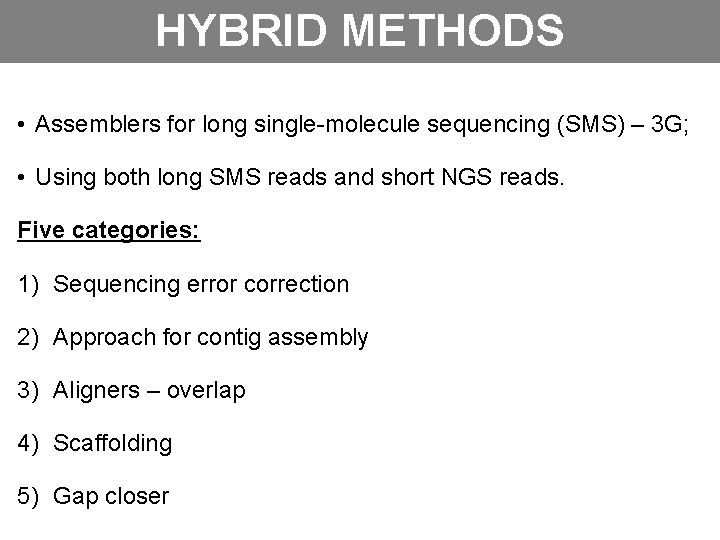
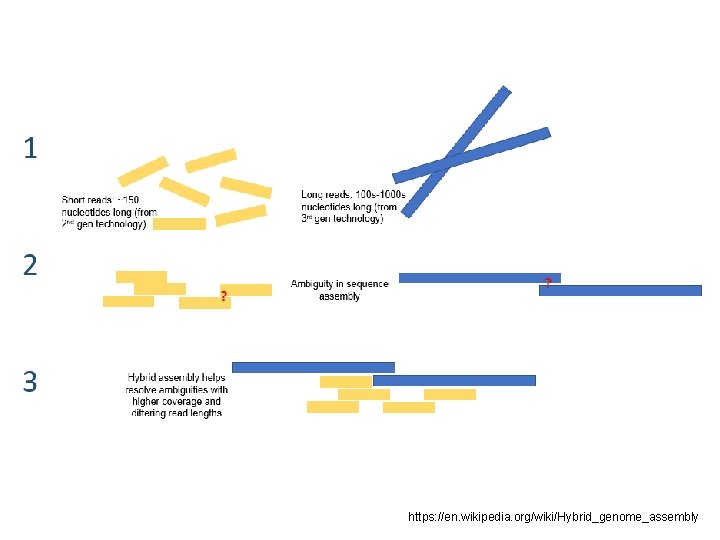
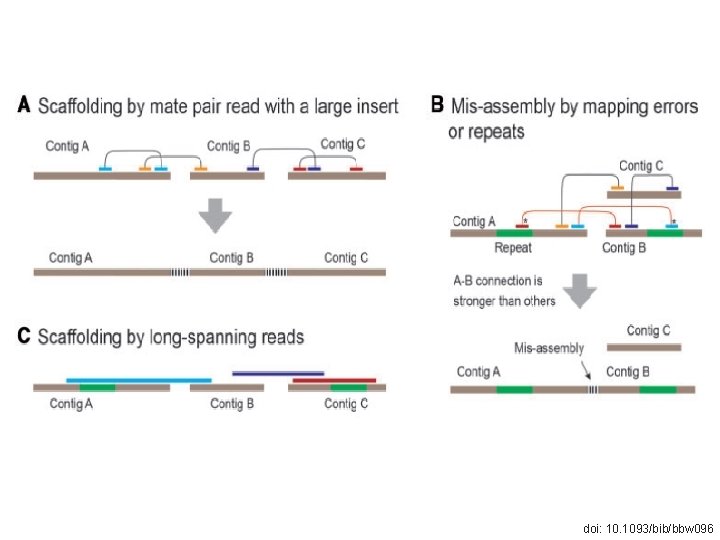
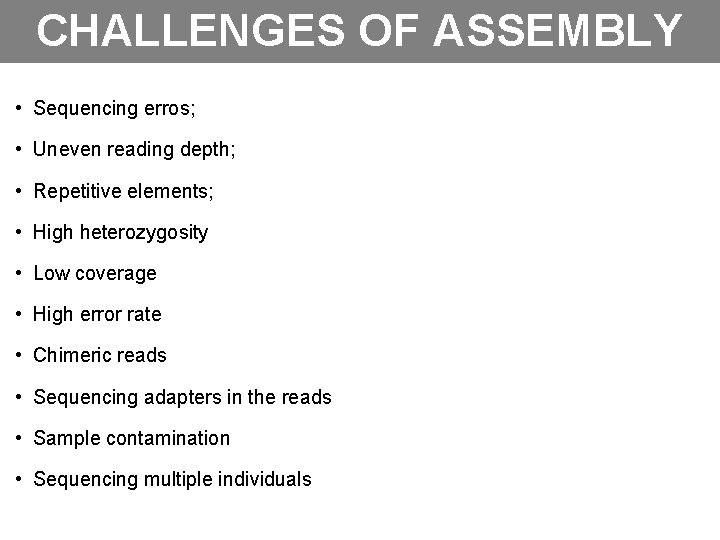
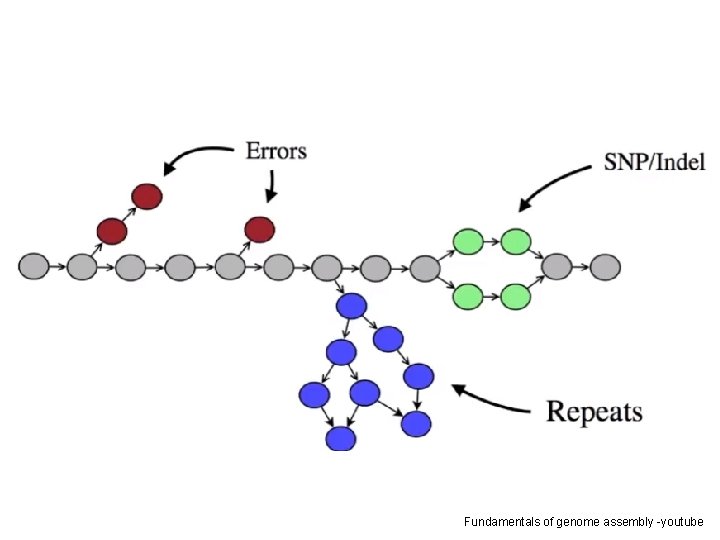
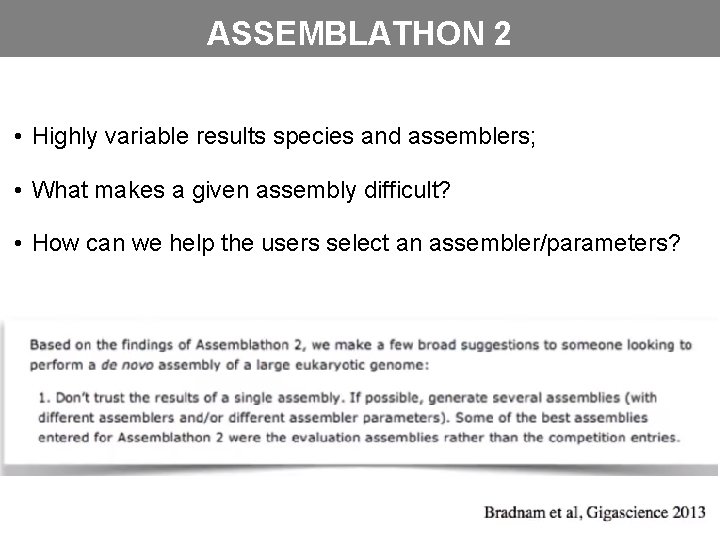
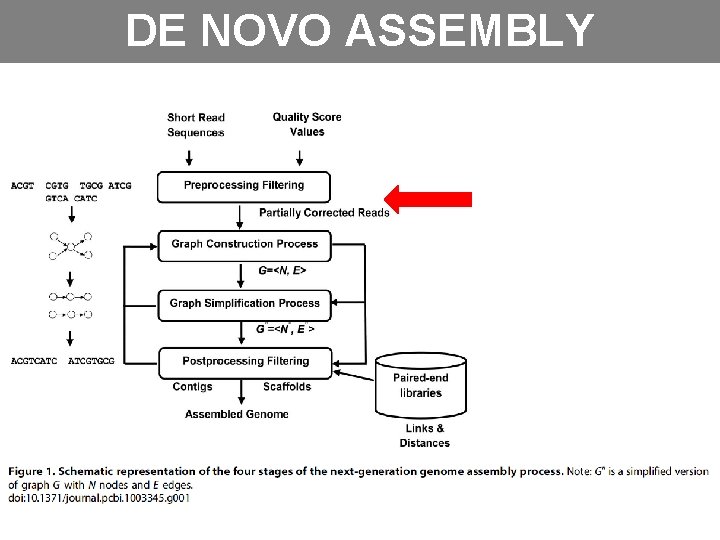
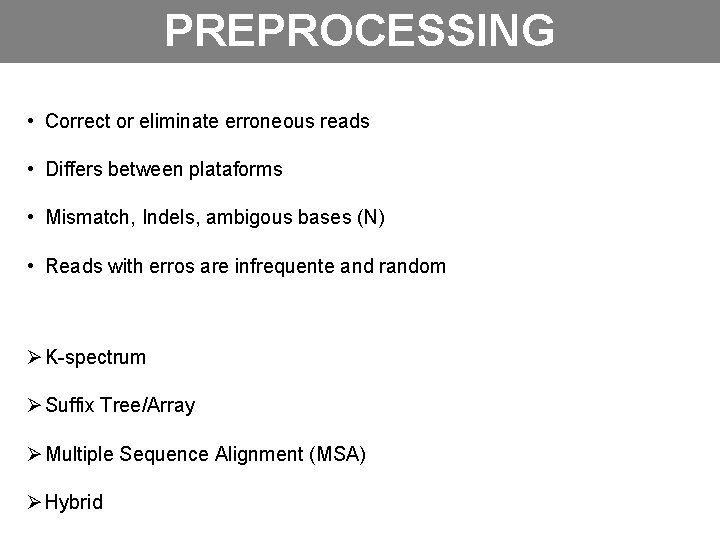
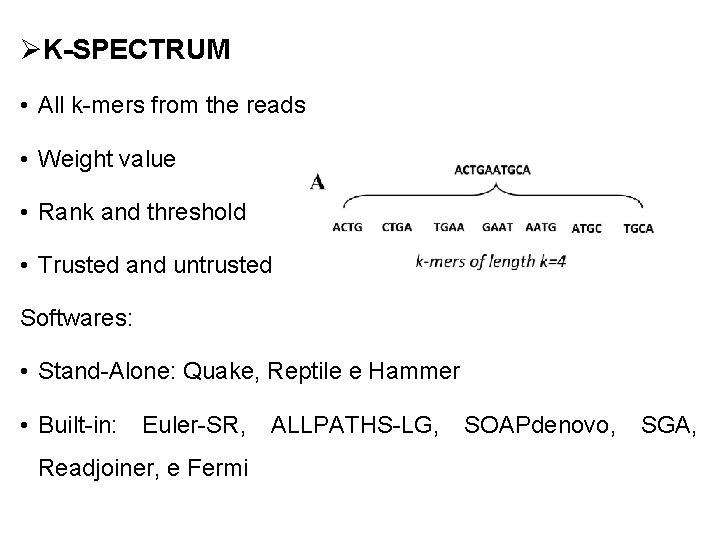
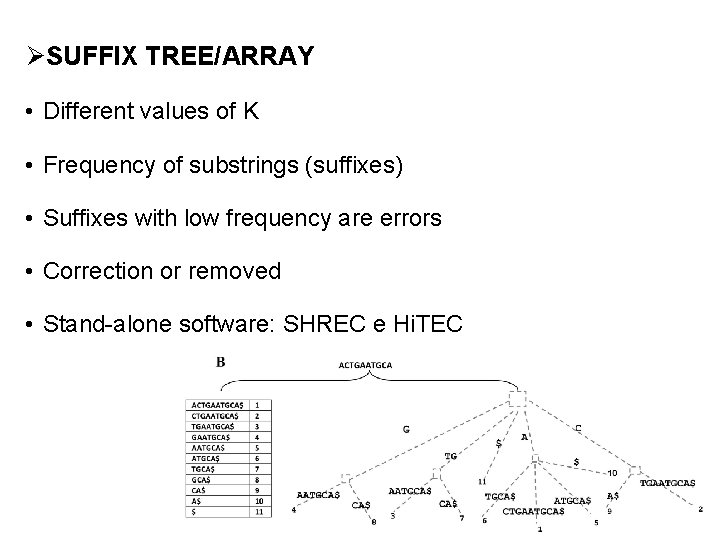
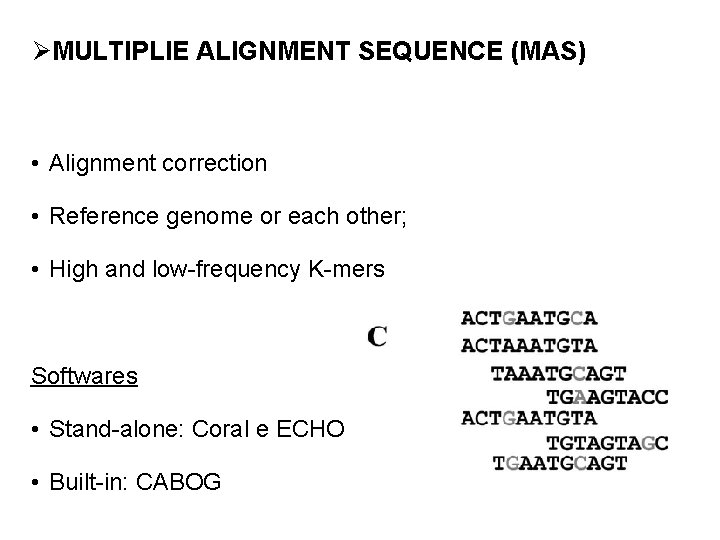
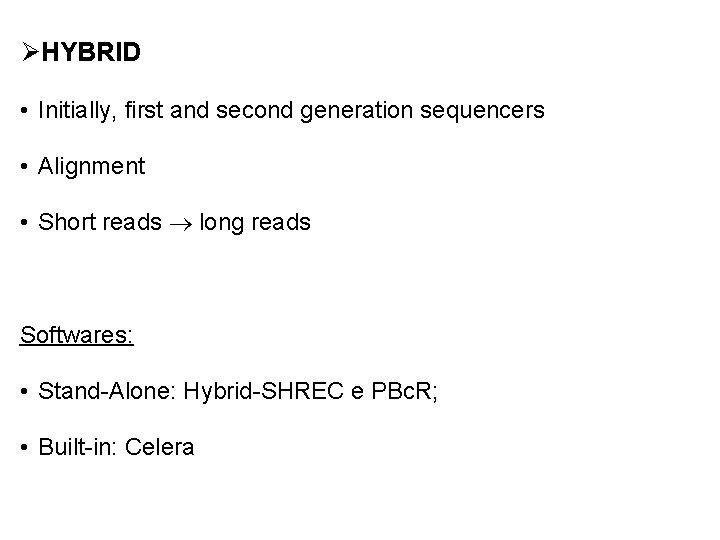
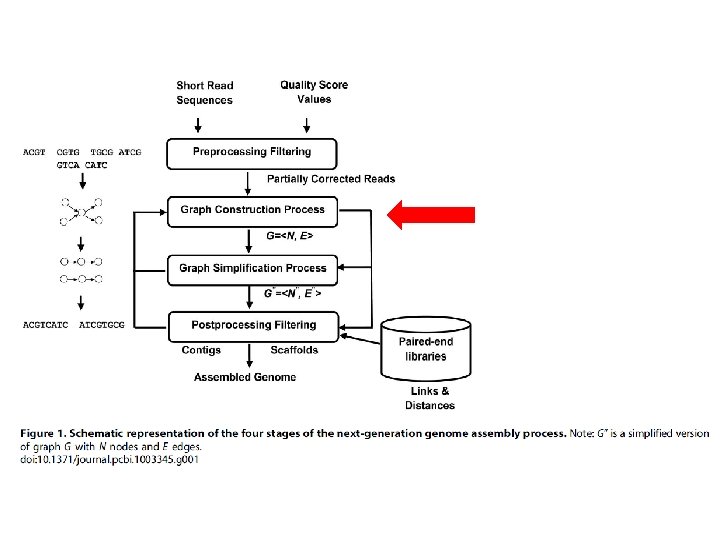
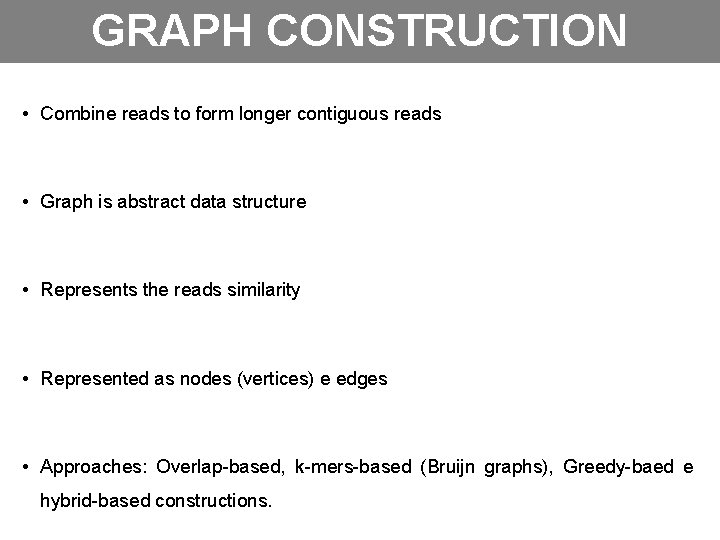
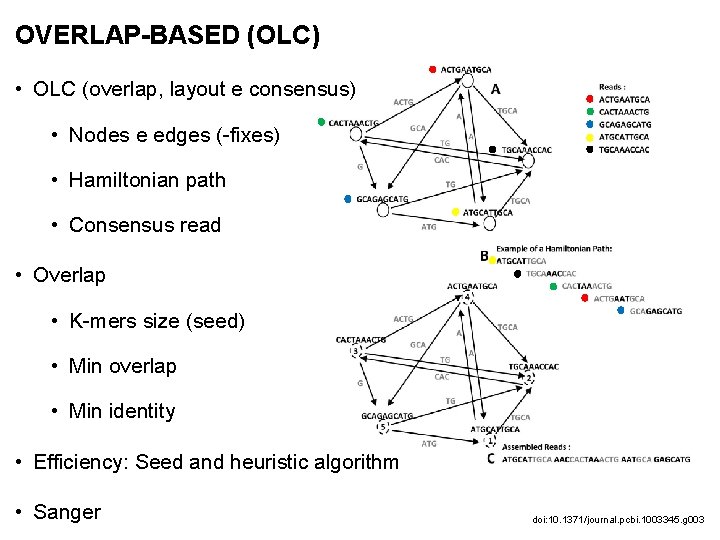
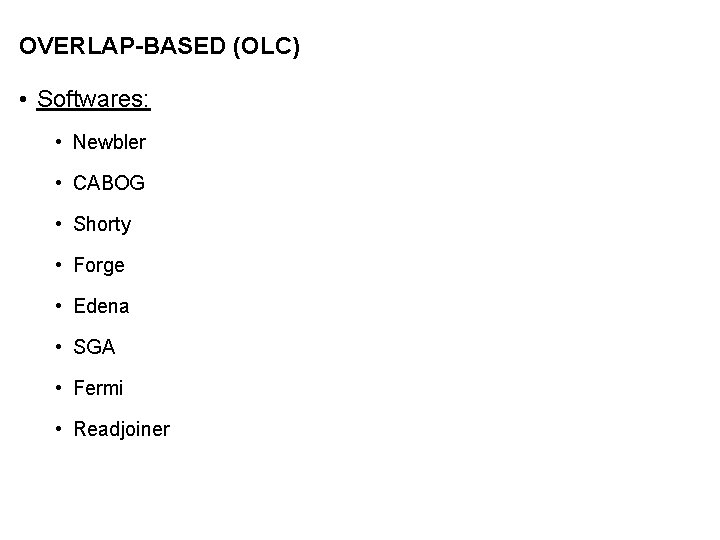
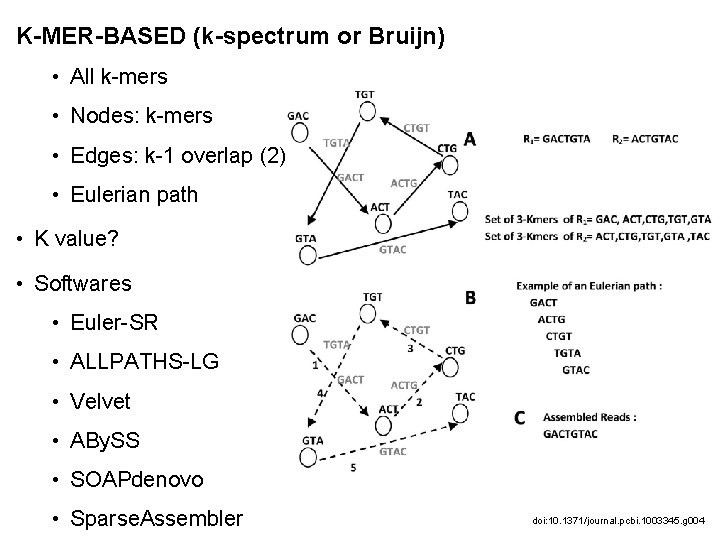
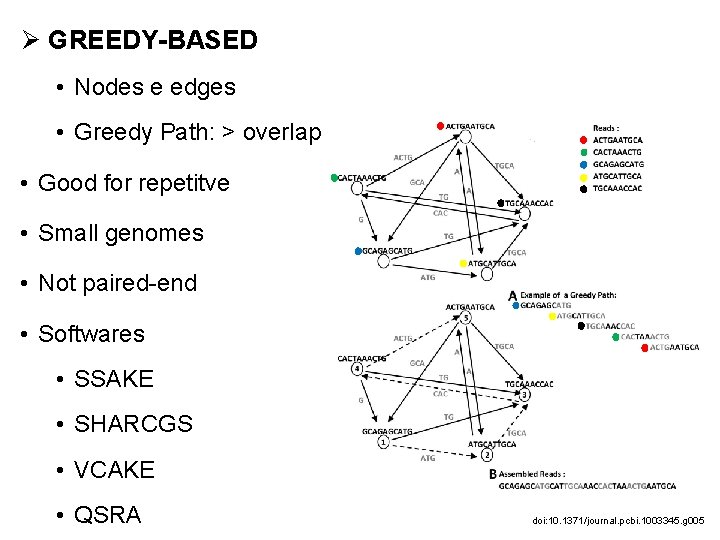
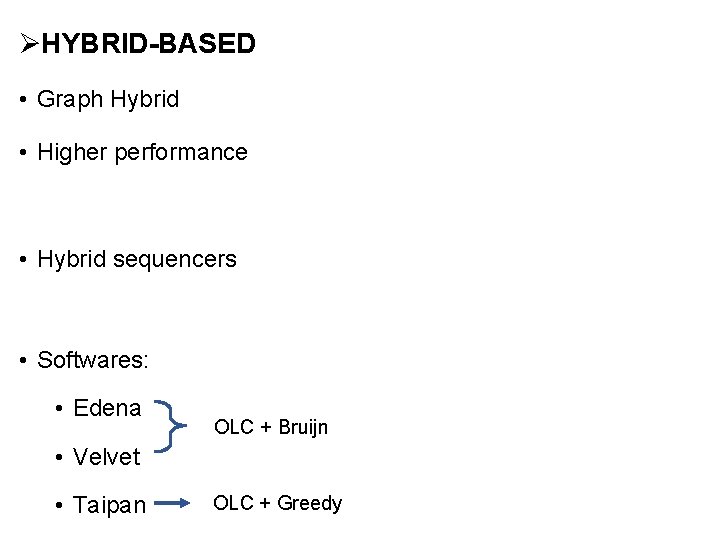
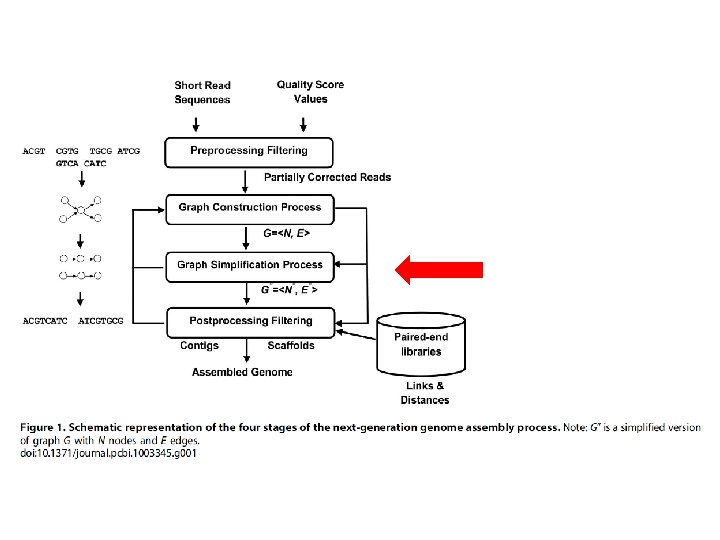
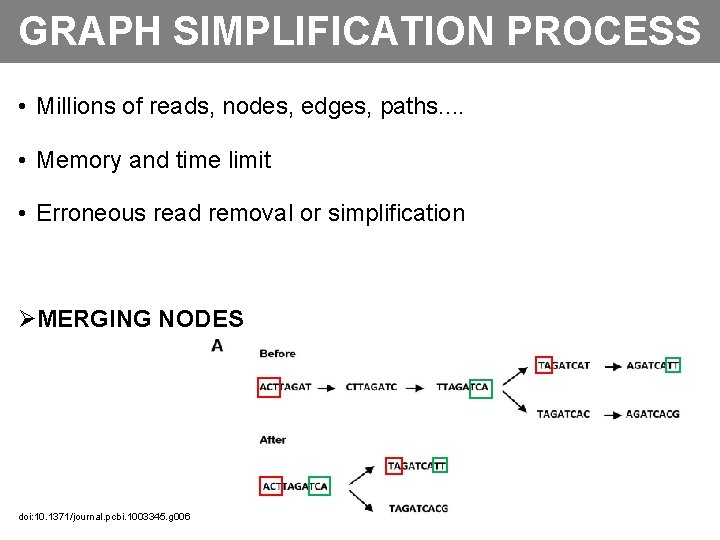
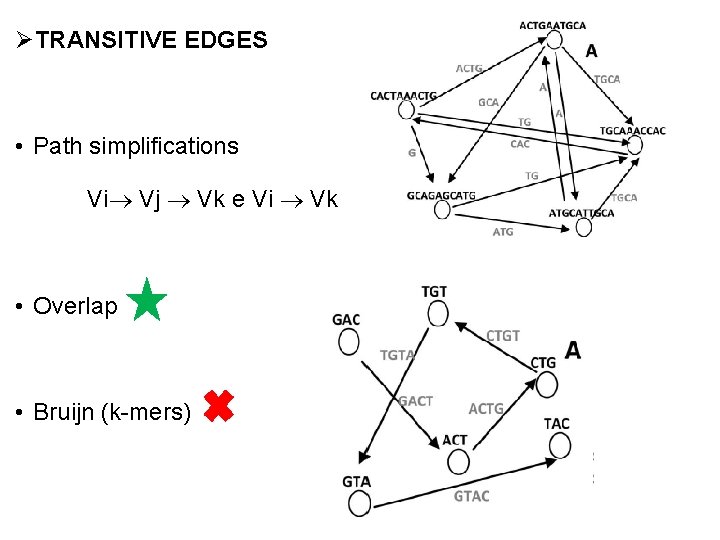
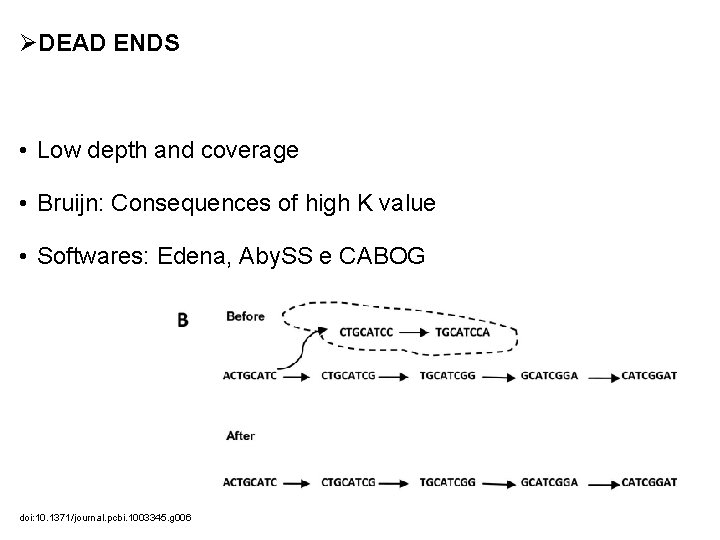
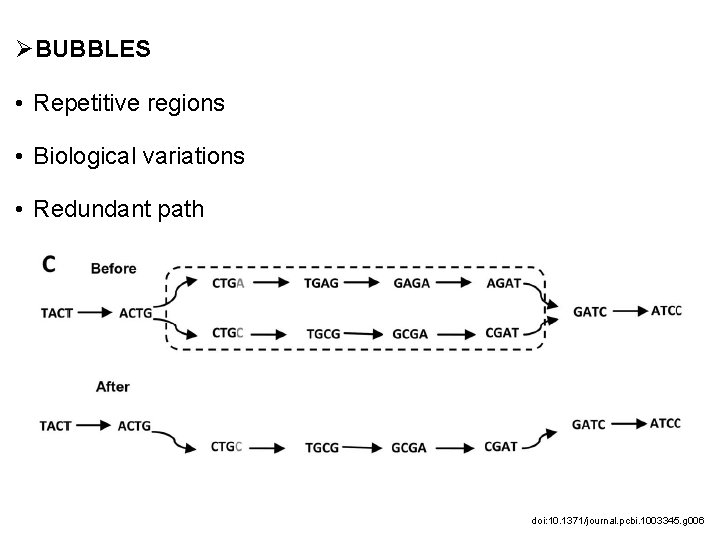
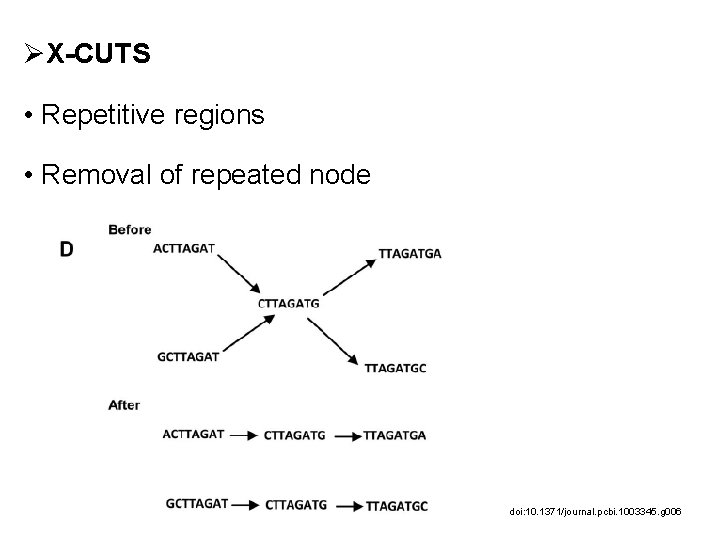
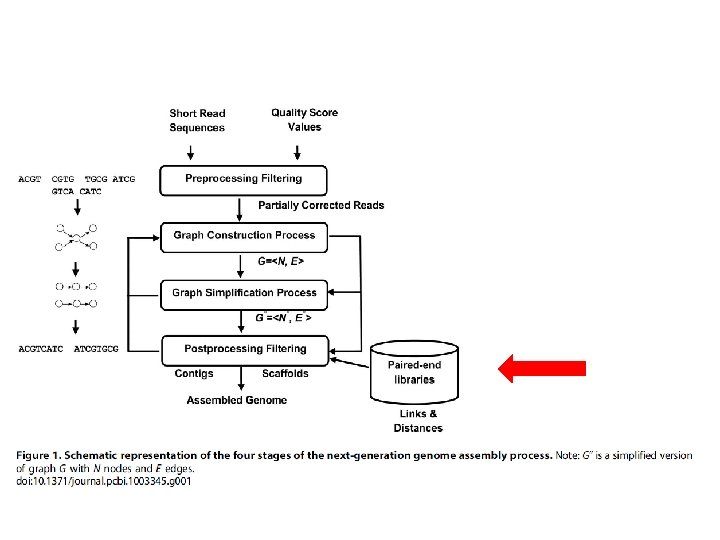
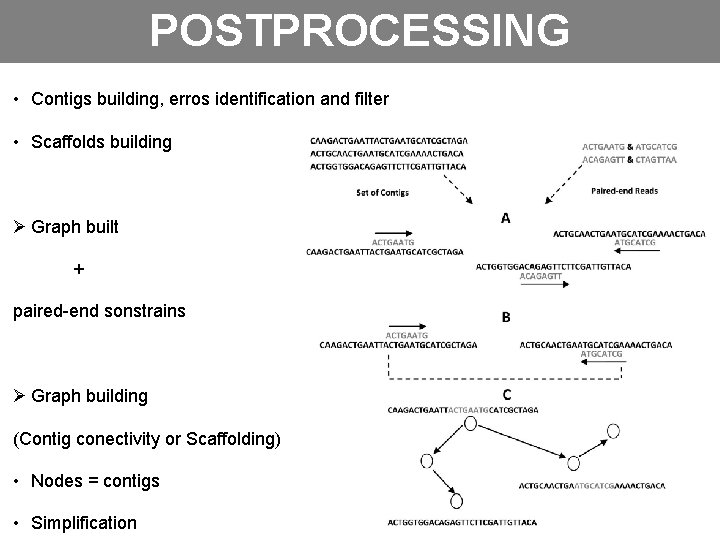
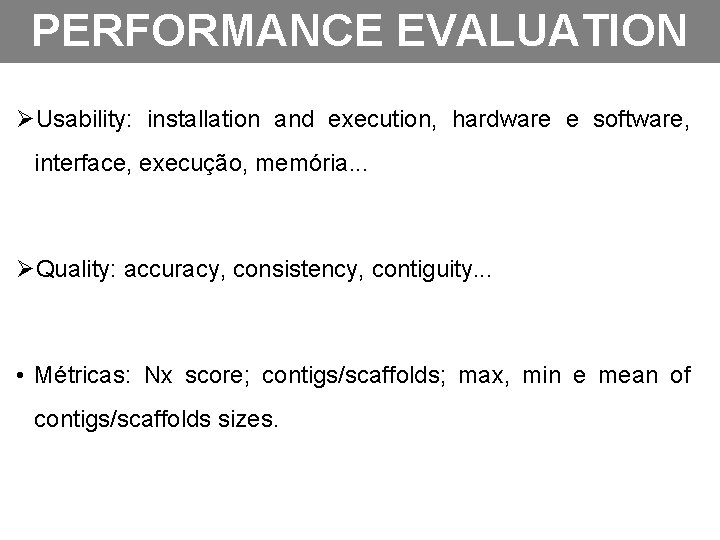
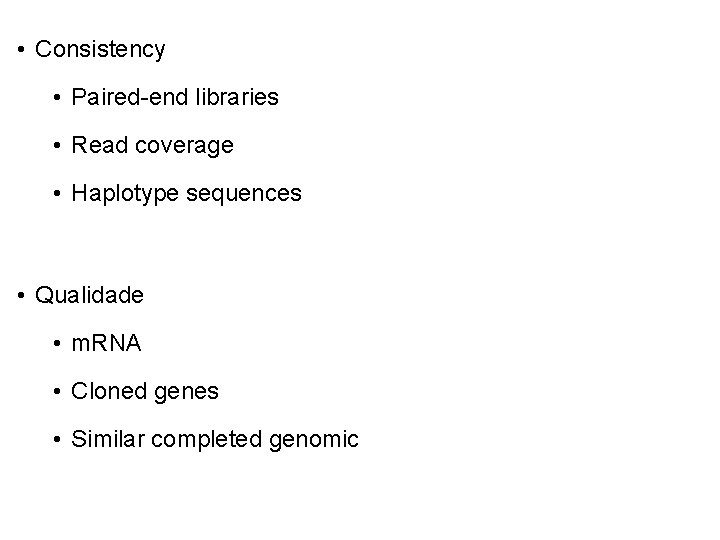
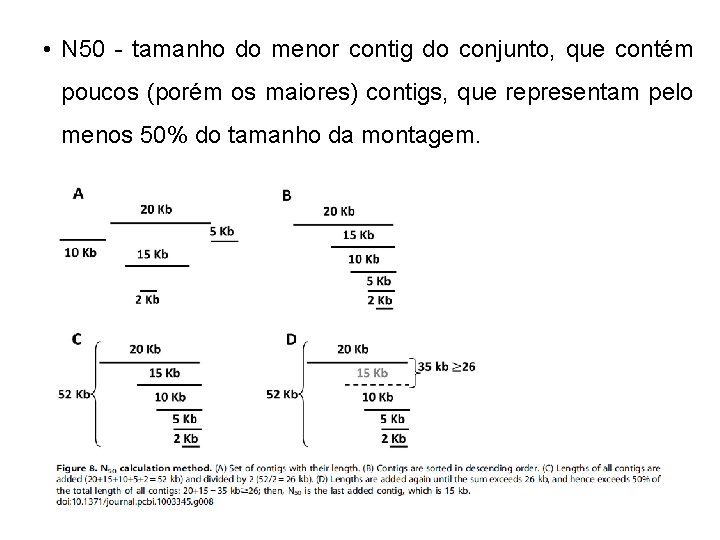
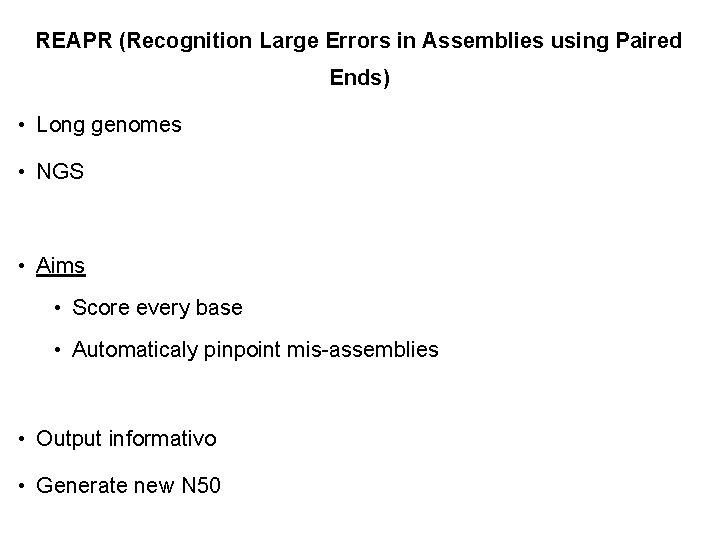
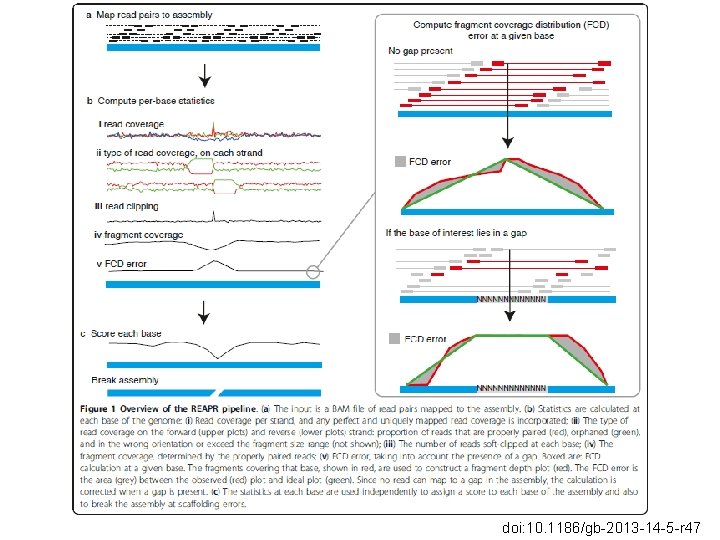
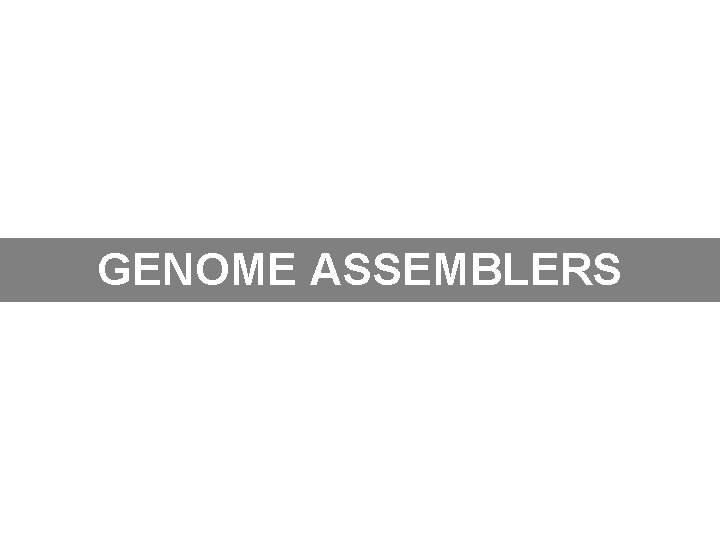
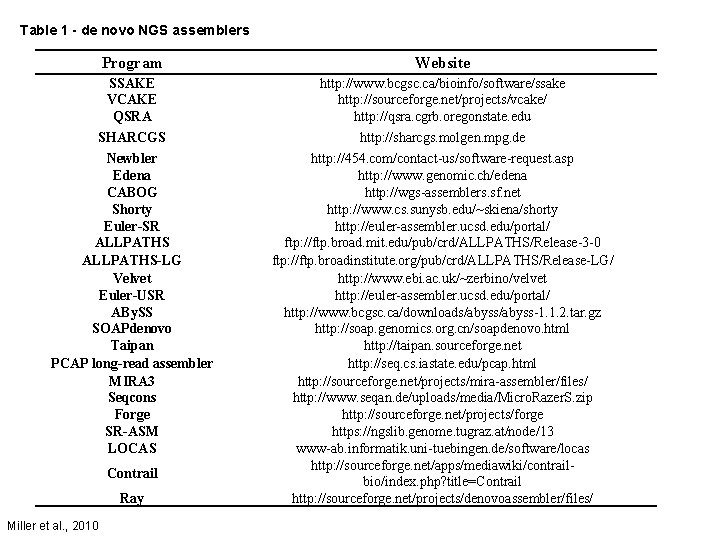
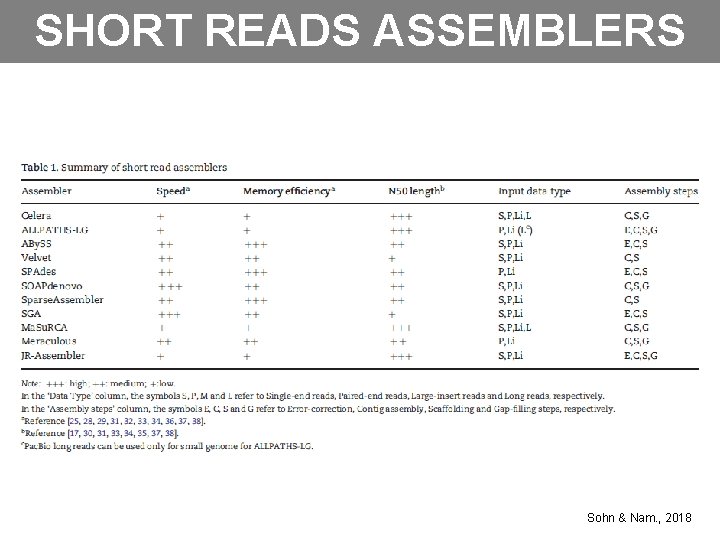
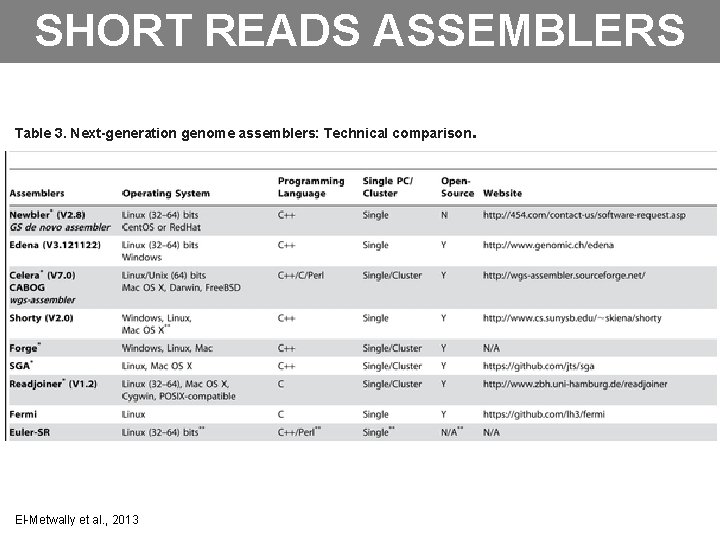
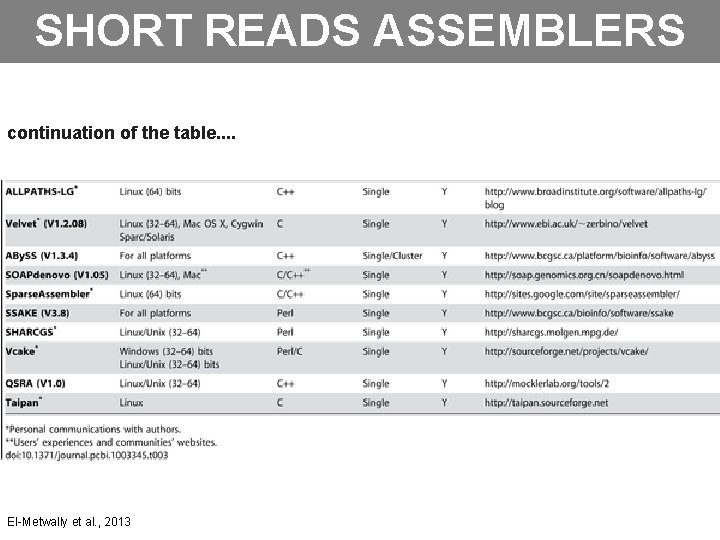
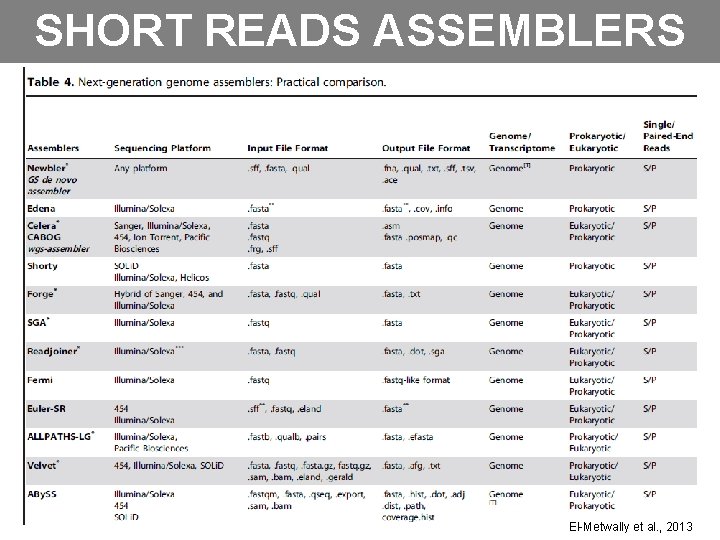
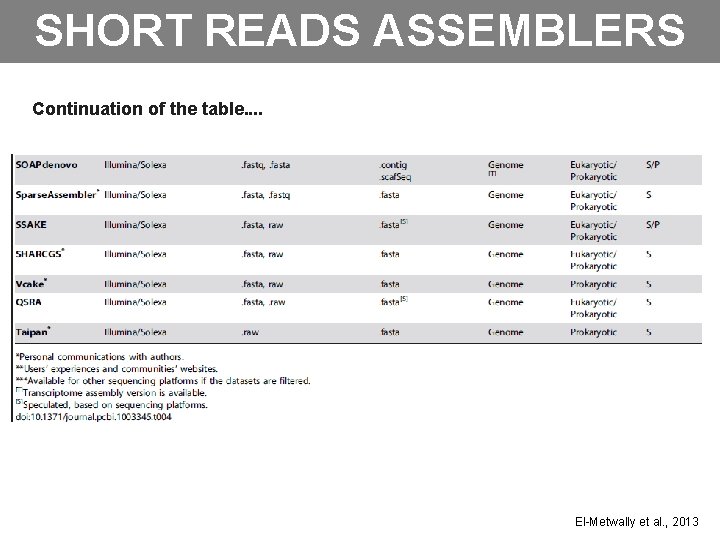
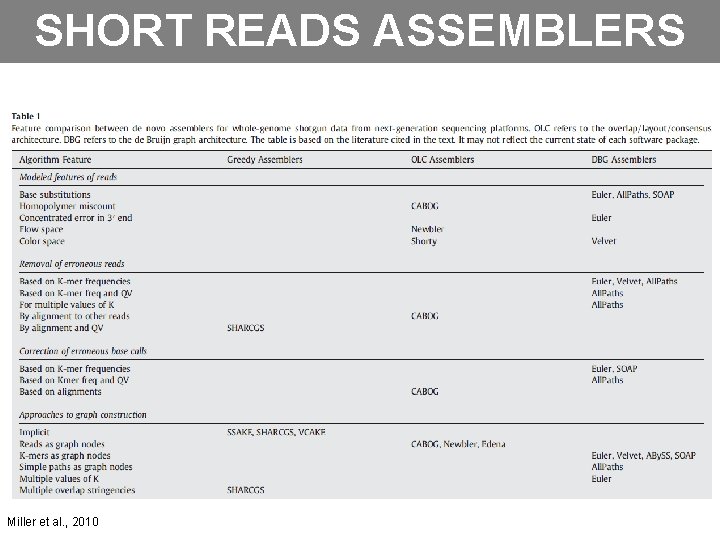
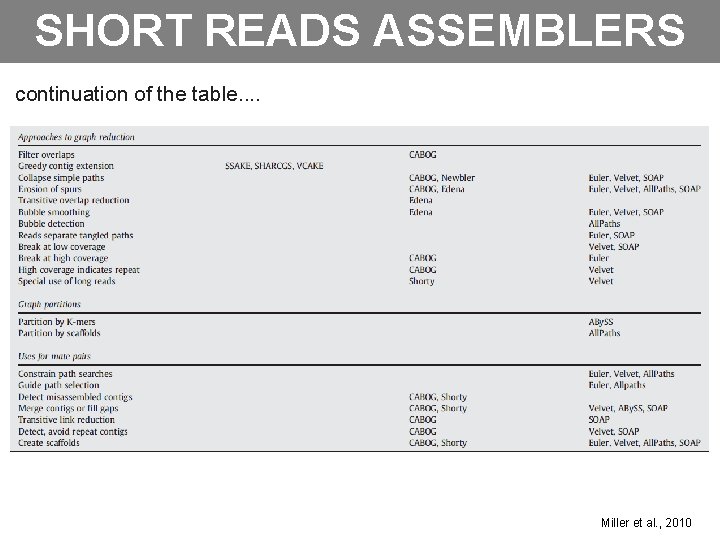
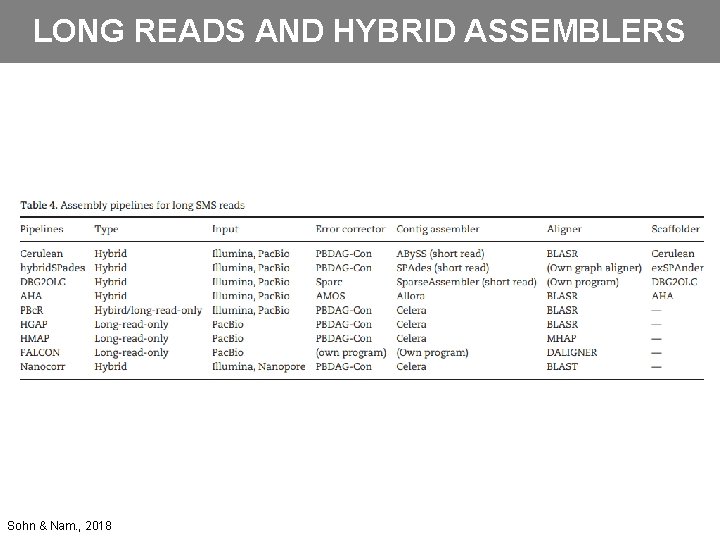
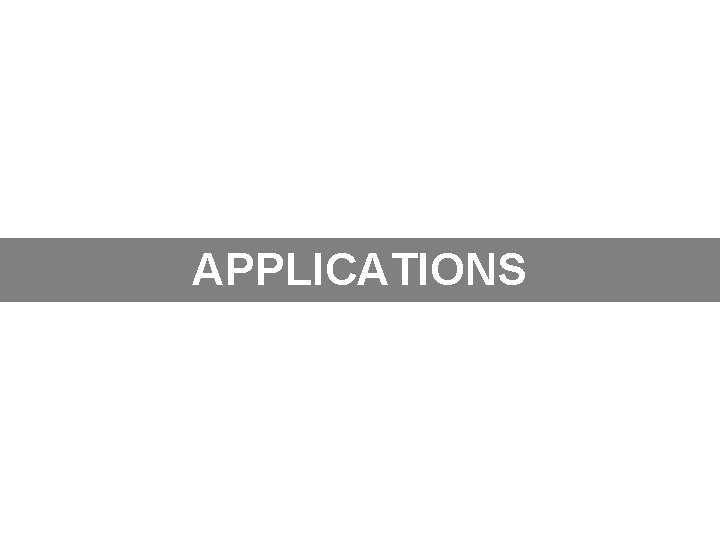
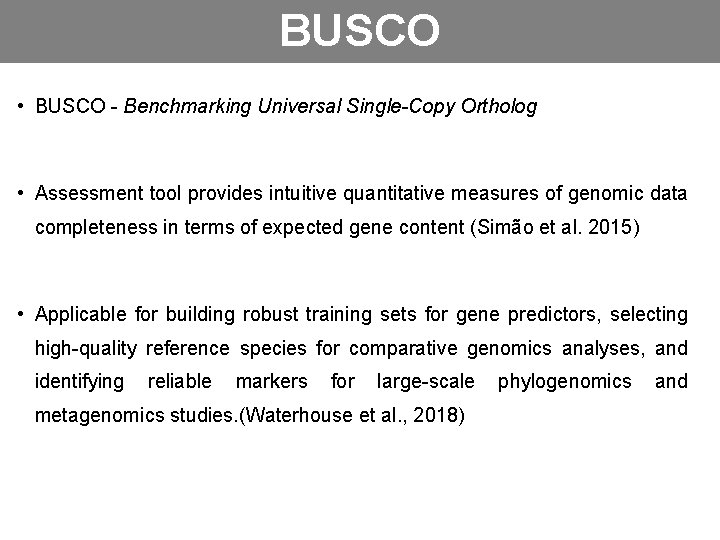
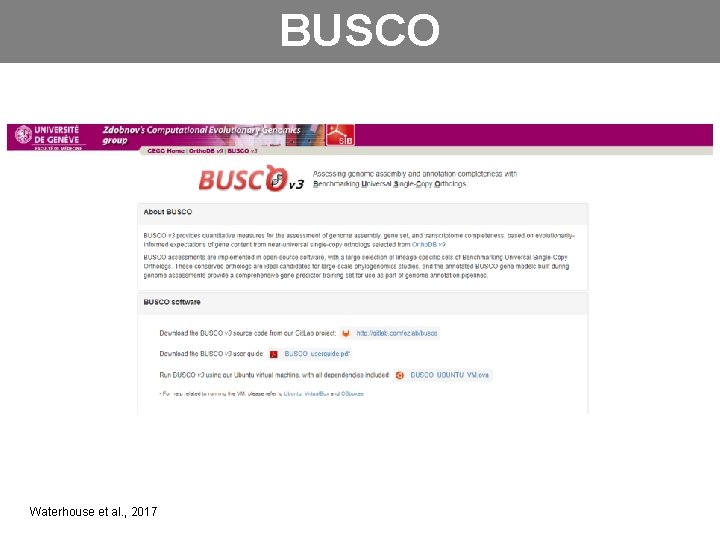
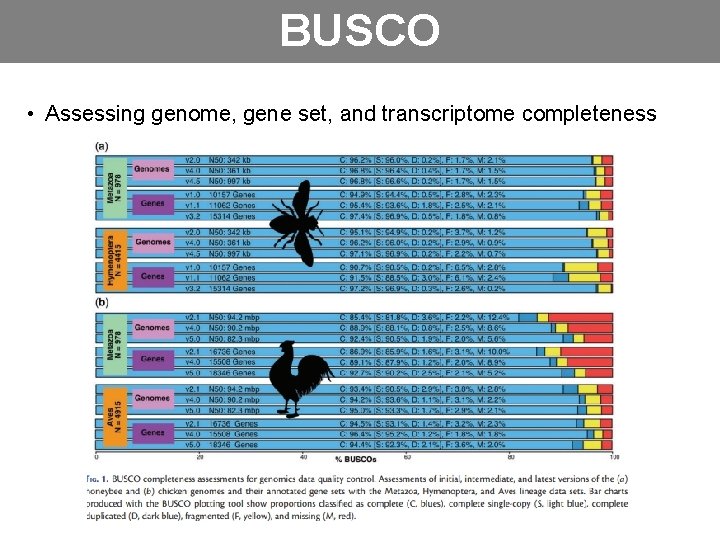
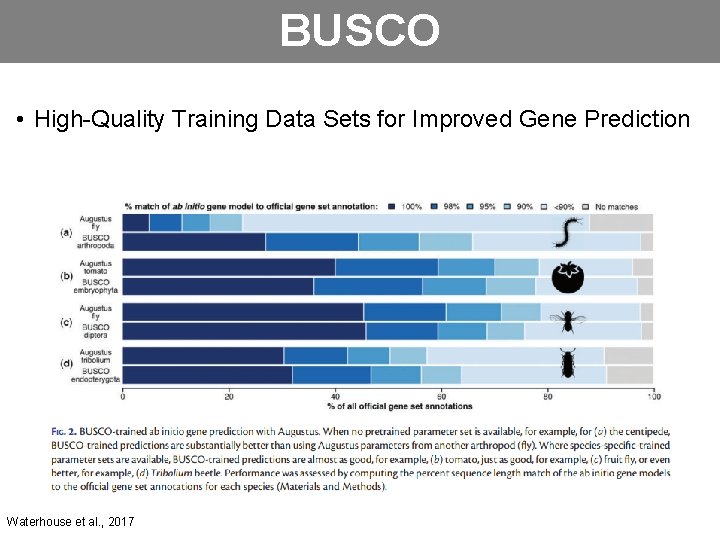
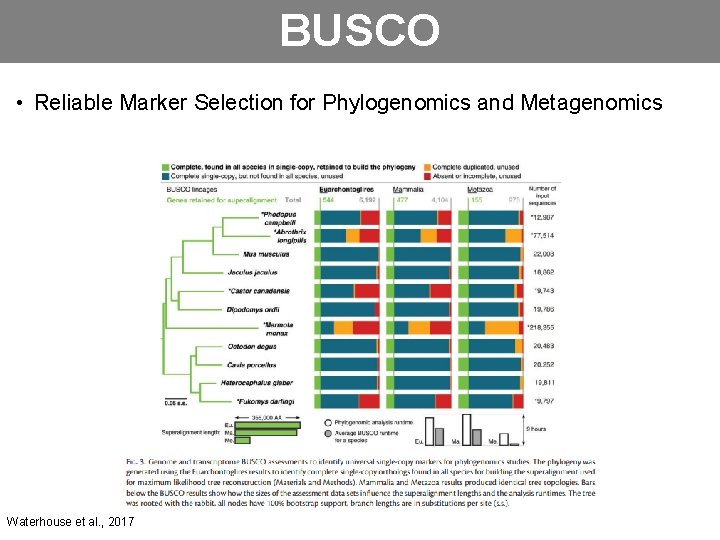
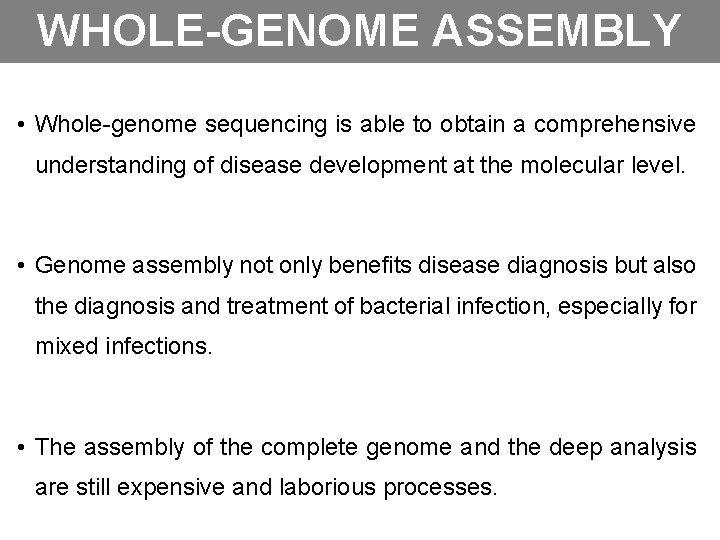
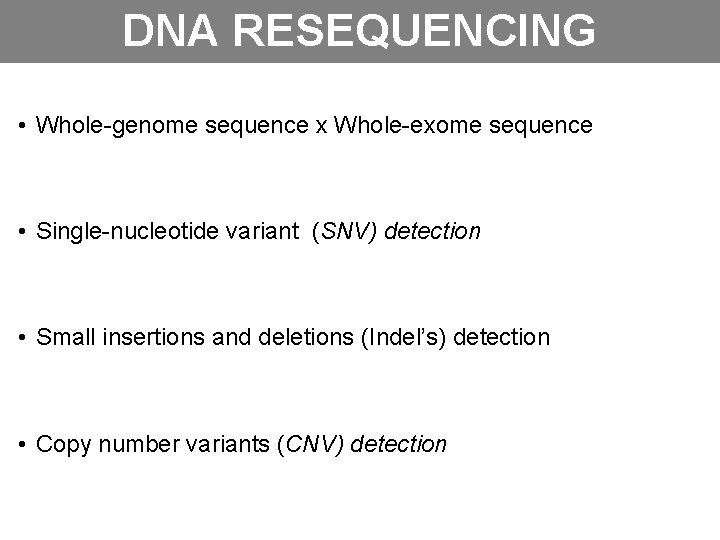
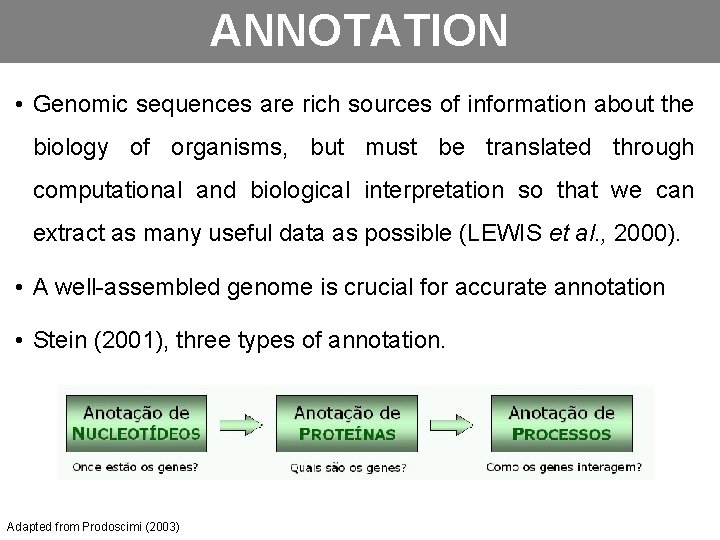
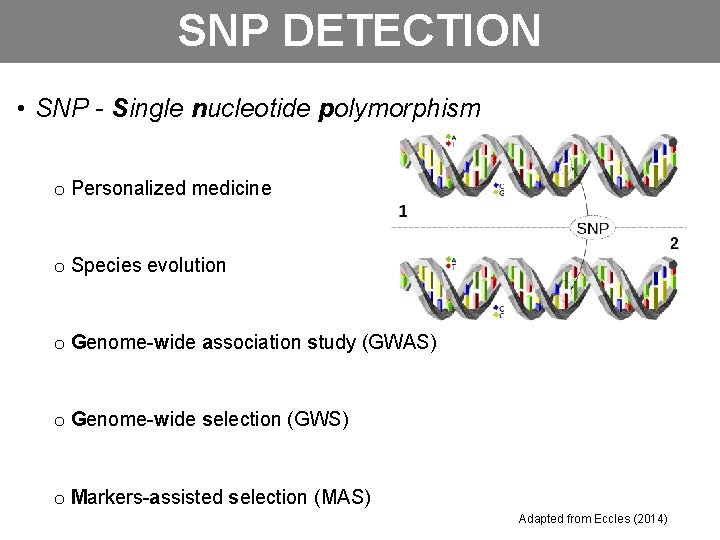
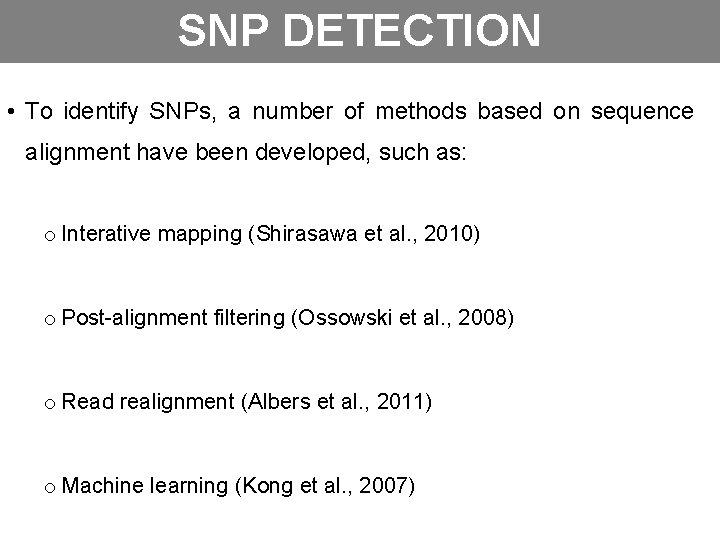
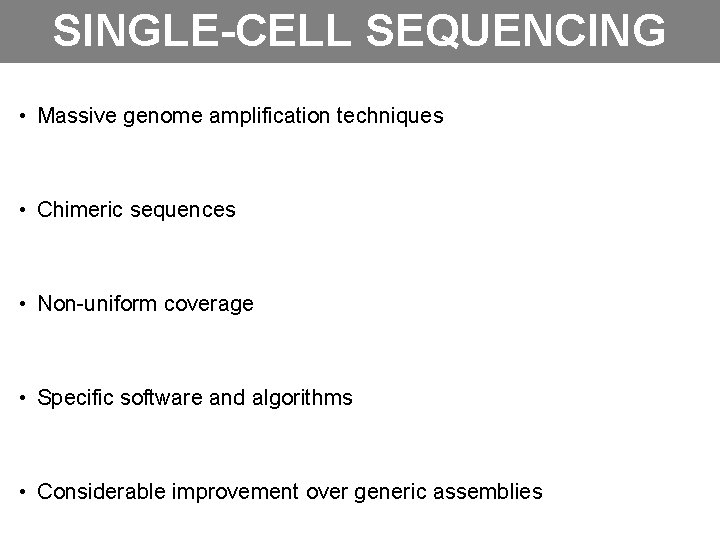
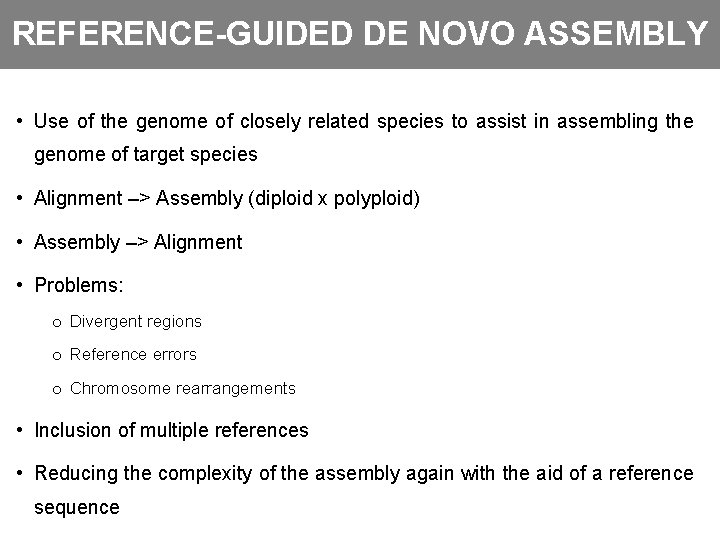
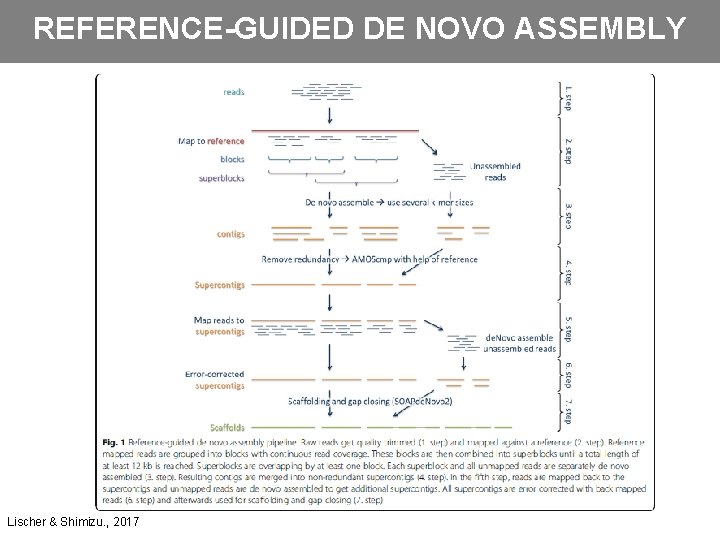
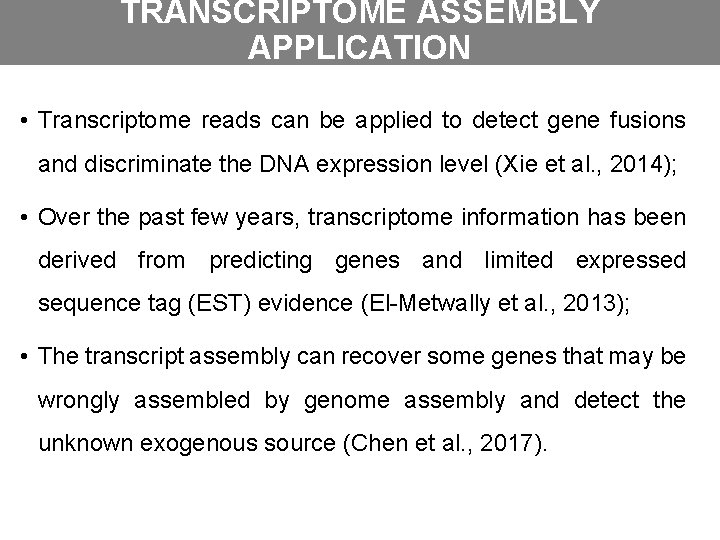
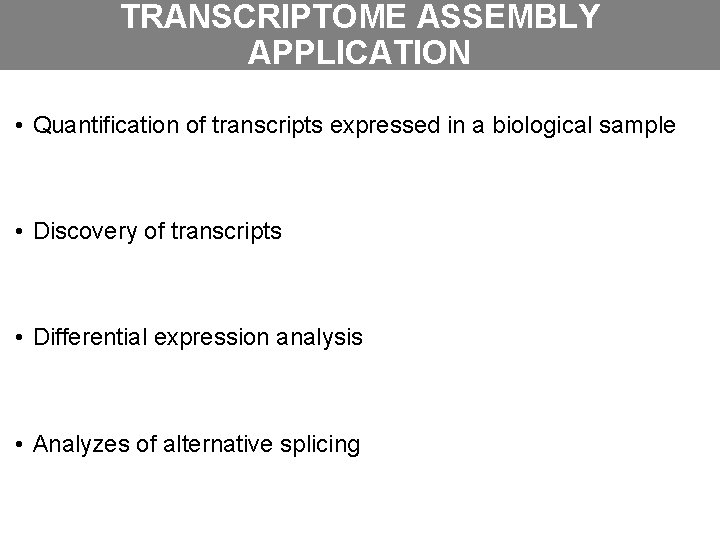
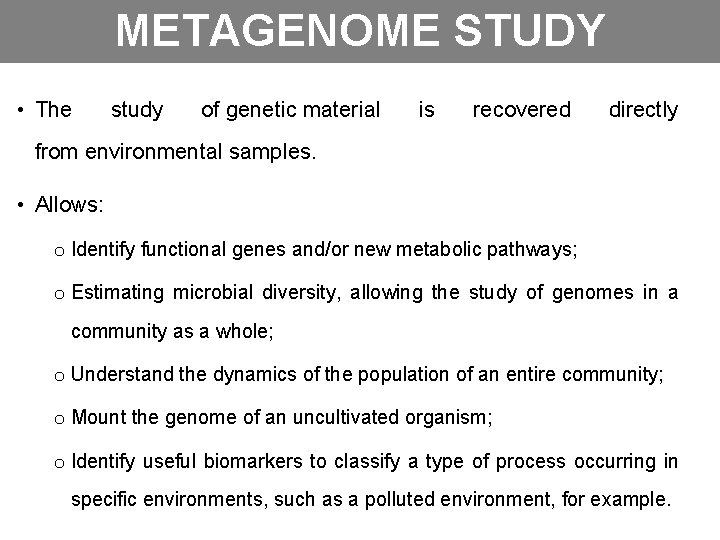
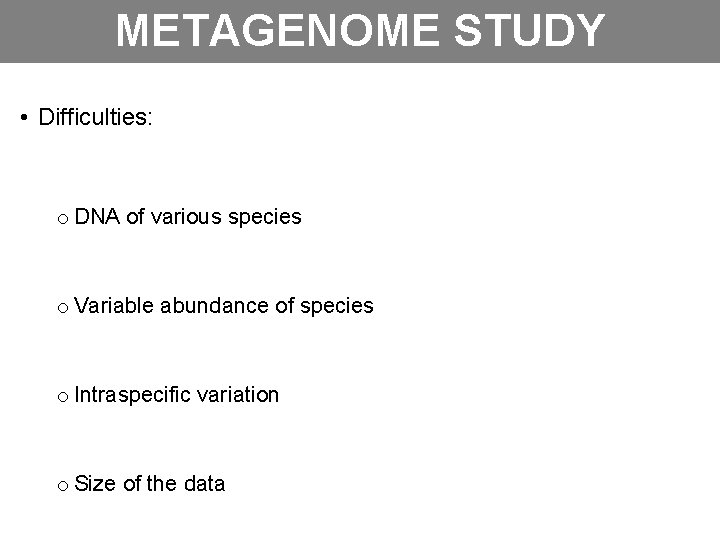
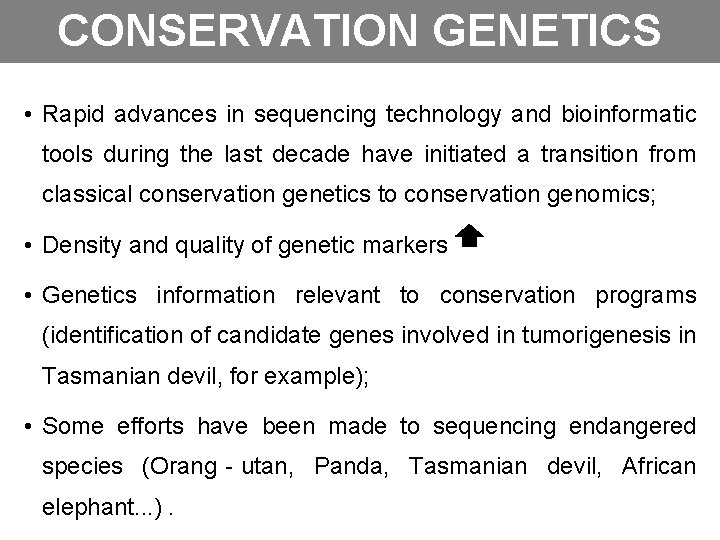
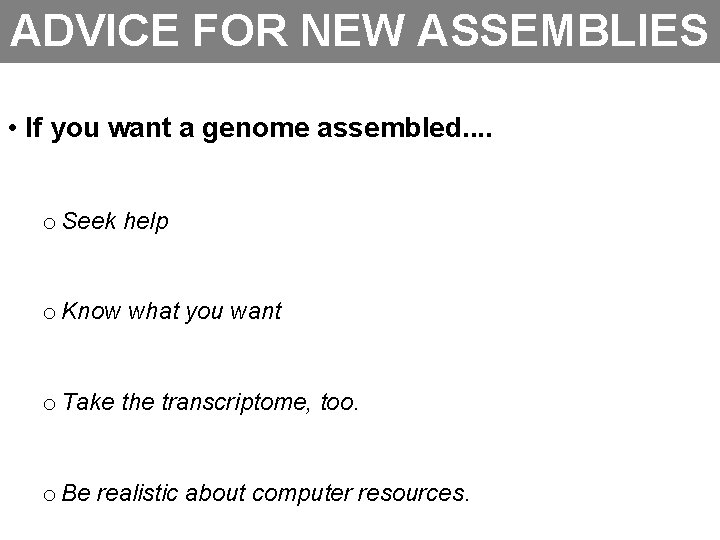
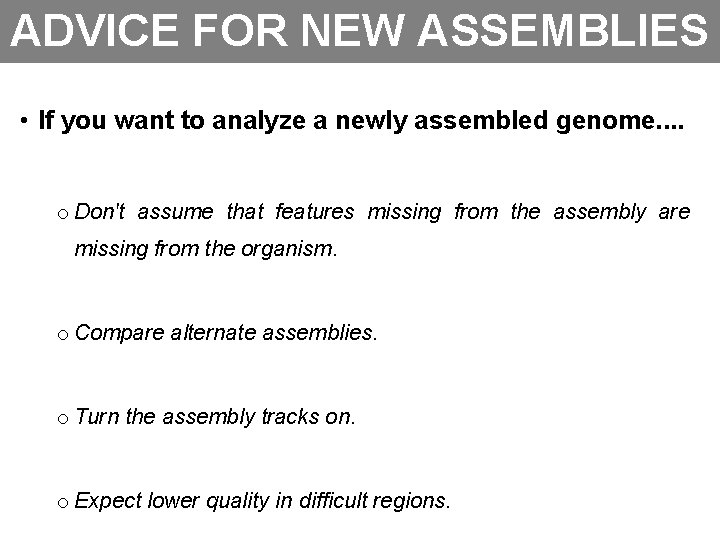
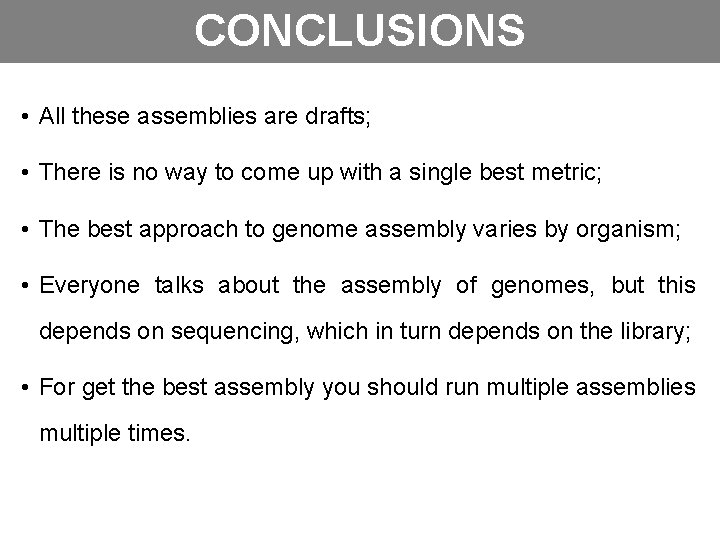
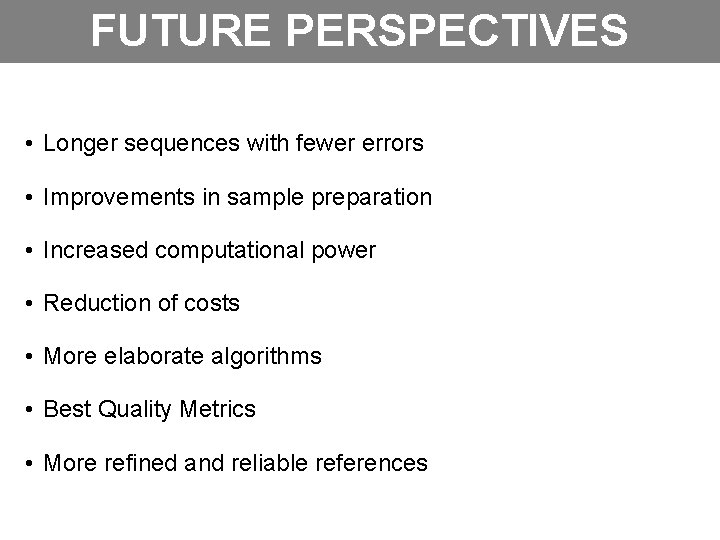
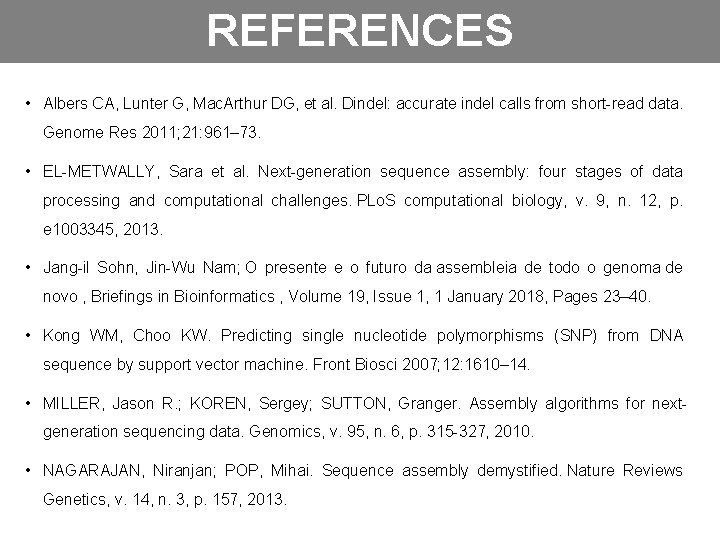
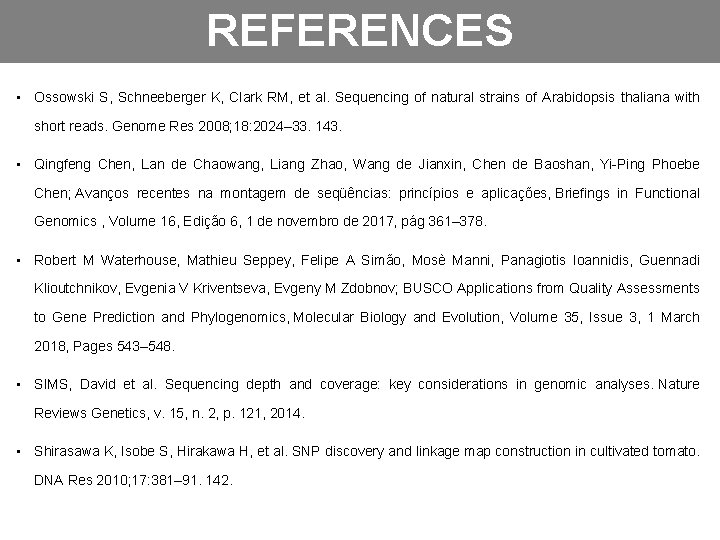
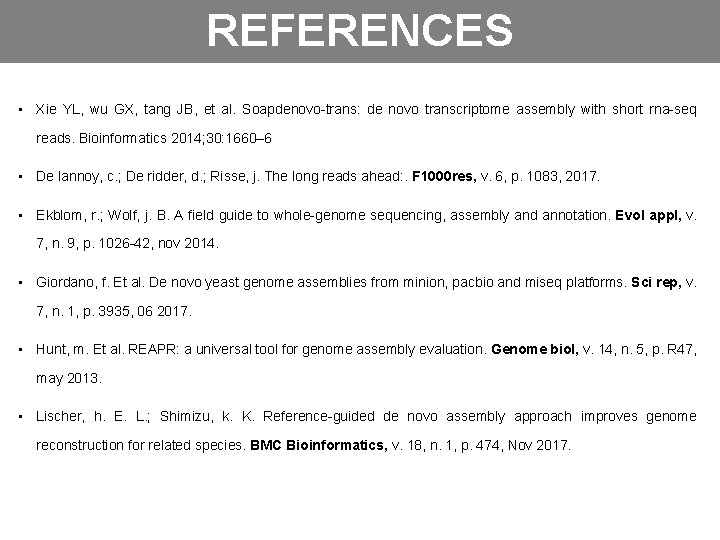
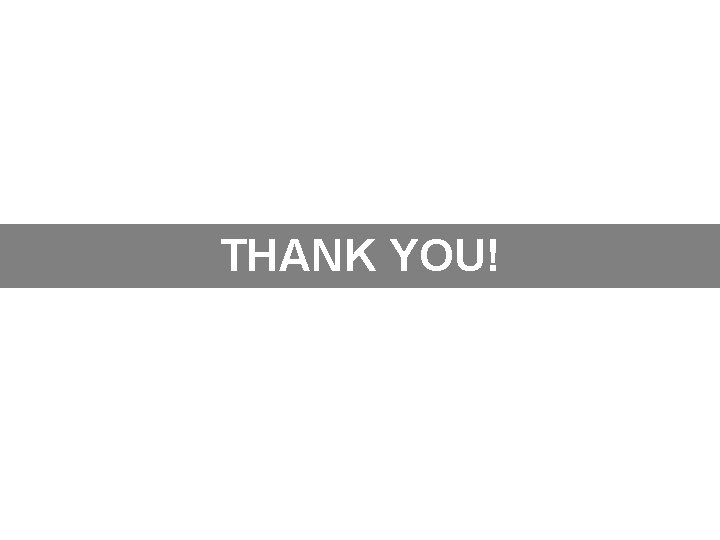
- Slides: 104
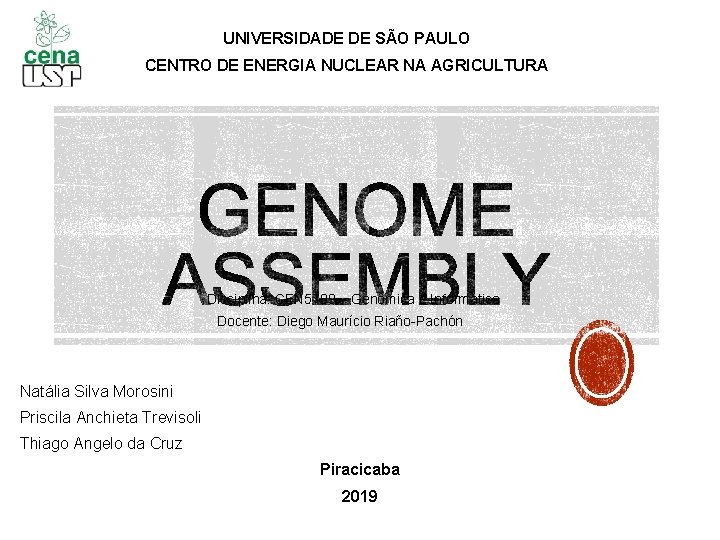
UNIVERSIDADE DE SÃO PAULO CENTRO DE ENERGIA NUCLEAR NA AGRICULTURA Disciplina: CEN 5789 – Genômica e Informática Docente: Diego Maurício Riaño-Pachón Natália Silva Morosini Priscila Anchieta Trevisoli Thiago Angelo da Cruz Piracicaba 2019
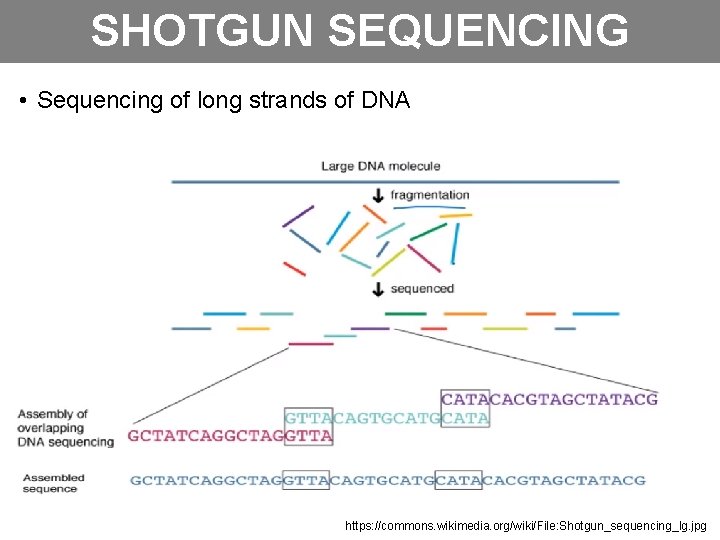
SHOTGUN SEQUENCING • Sequencing of long strands of DNA https: //commons. wikimedia. org/wiki/File: Shotgun_sequencing_lg. jpg
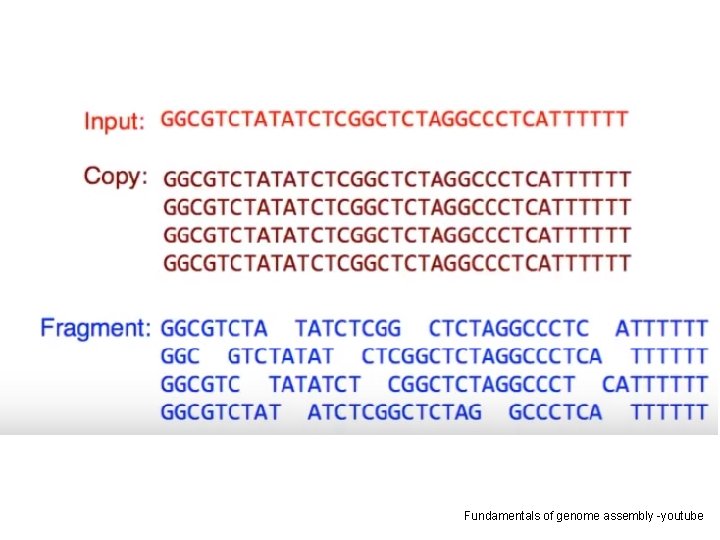
Fundamentals of genome assembly -youtube
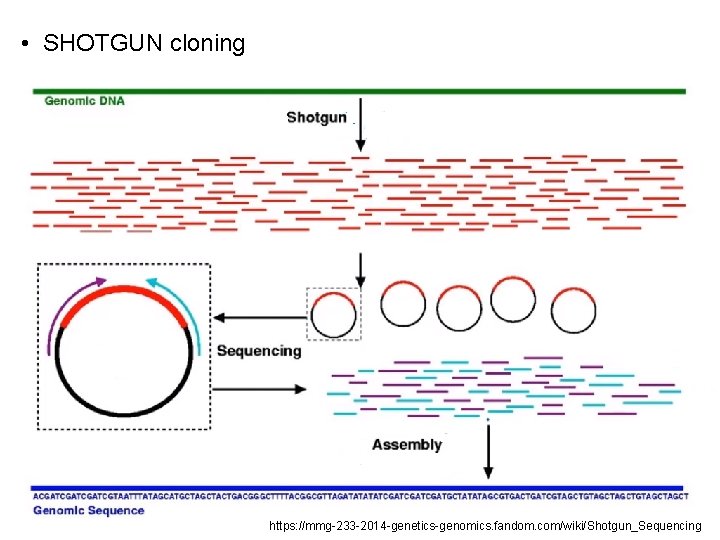
• SHOTGUN cloning https: //mmg-233 -2014 -genetics-genomics. fandom. com/wiki/Shotgun_Sequencing
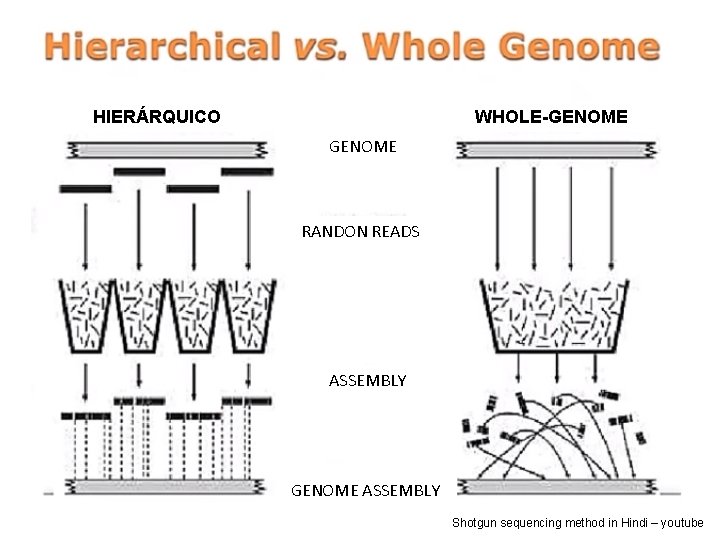
HIERÁRQUICO WHOLE-GENOME RANDON READS ASSEMBLY GENOME ASSEMBLY Shotgun sequencing method in Hindi – youtube
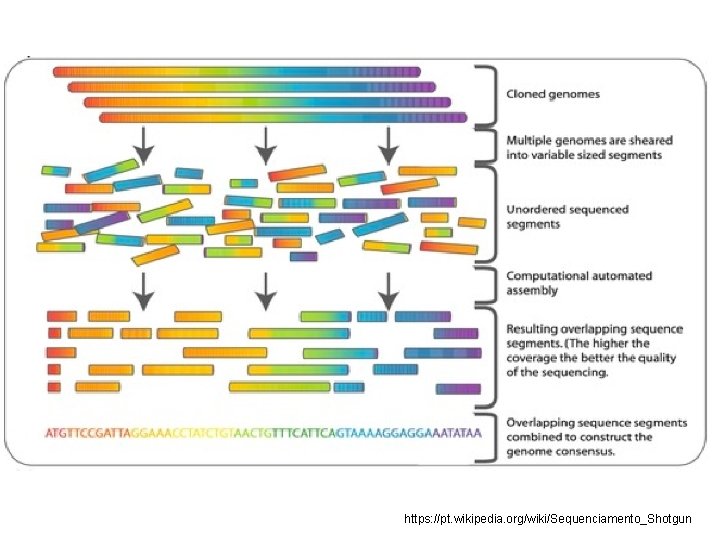
https: //pt. wikipedia. org/wiki/Sequenciamento_Shotgun
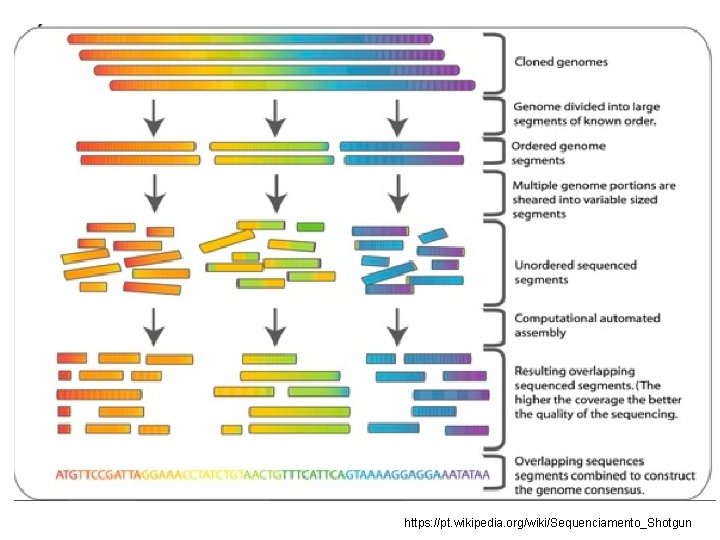
https: //pt. wikipedia. org/wiki/Sequenciamento_Shotgun
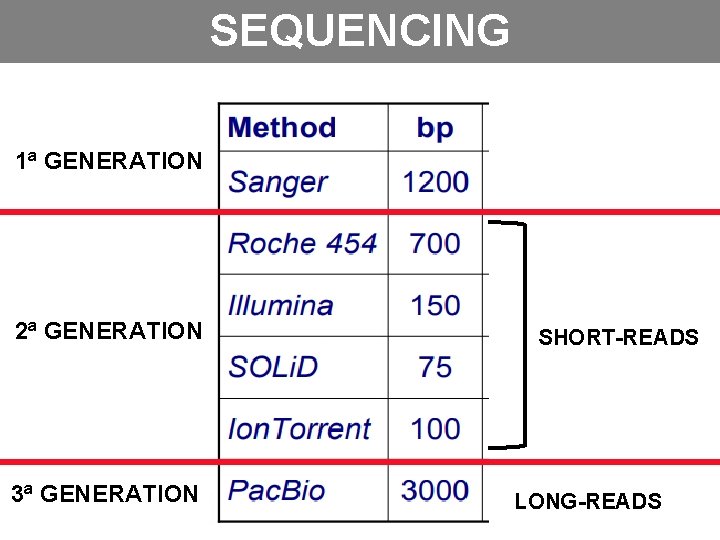
SEQUENCING 1ª GENERATION 2ª GENERATION 3ª GENERATION SHORT-READS LONG-READS
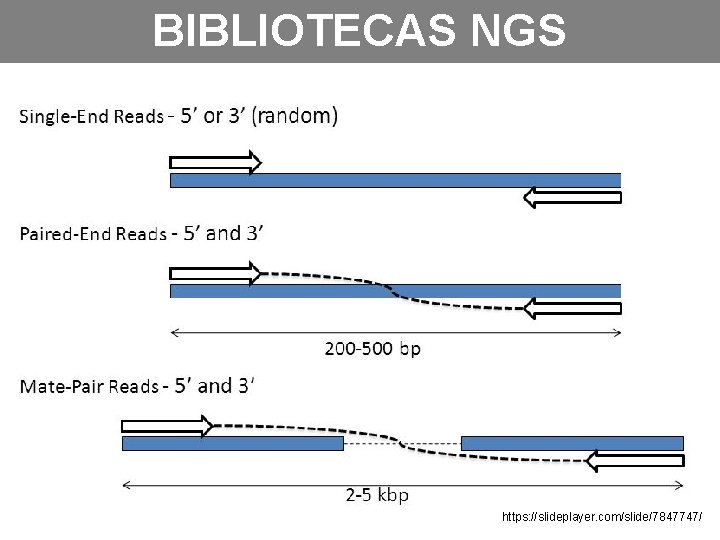
BIBLIOTECAS NGS https: //slideplayer. com/slide/7847747/
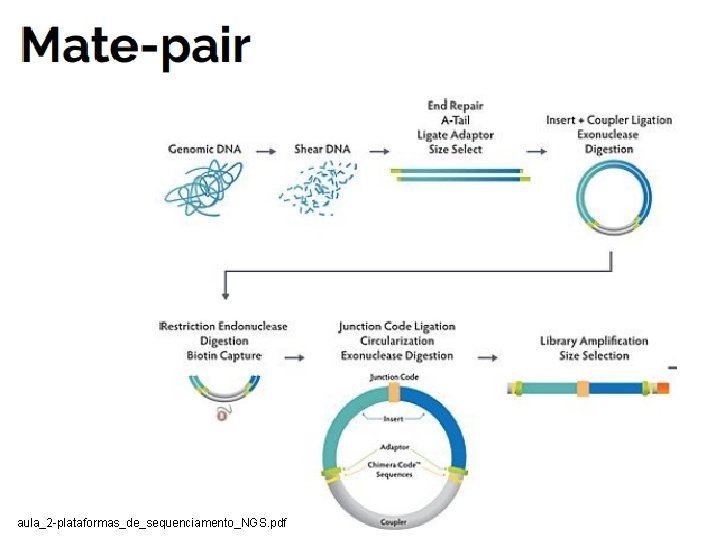
aula_2 -plataformas_de_sequenciamento_NGS. pdf
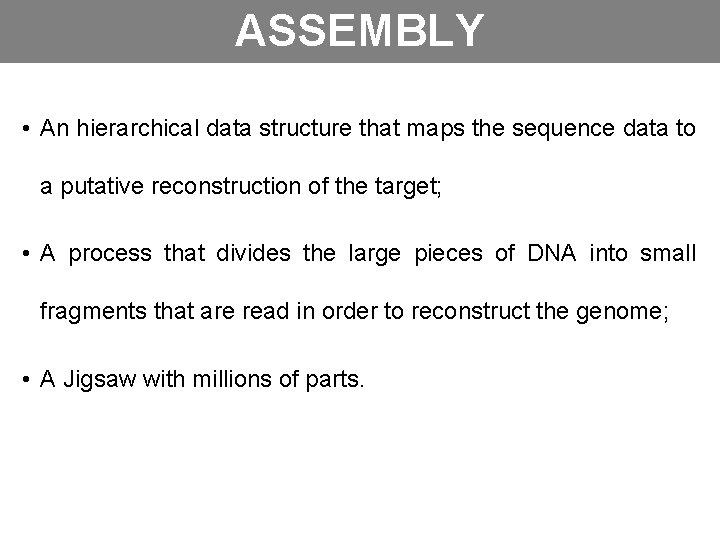
ASSEMBLY • An hierarchical data structure that maps the sequence data to a putative reconstruction of the target; • A process that divides the large pieces of DNA into small fragments that are read in order to reconstruct the genome; • A Jigsaw with millions of parts.
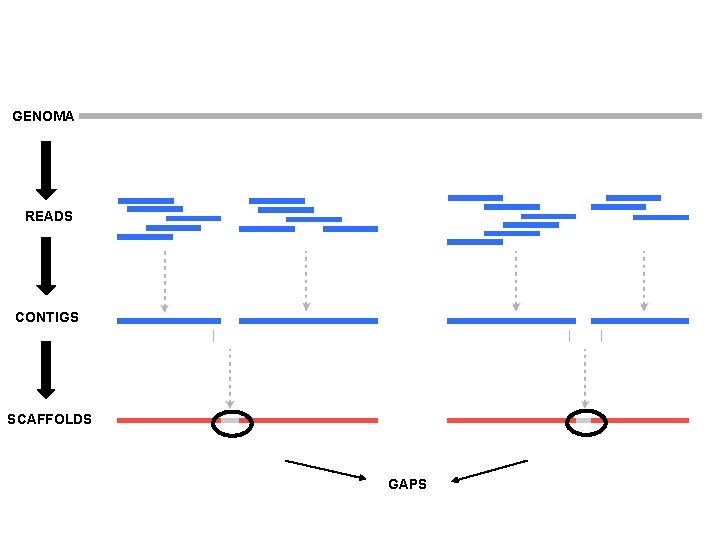
GENOMA READS CONTIGS SCAFFOLDS GAPS
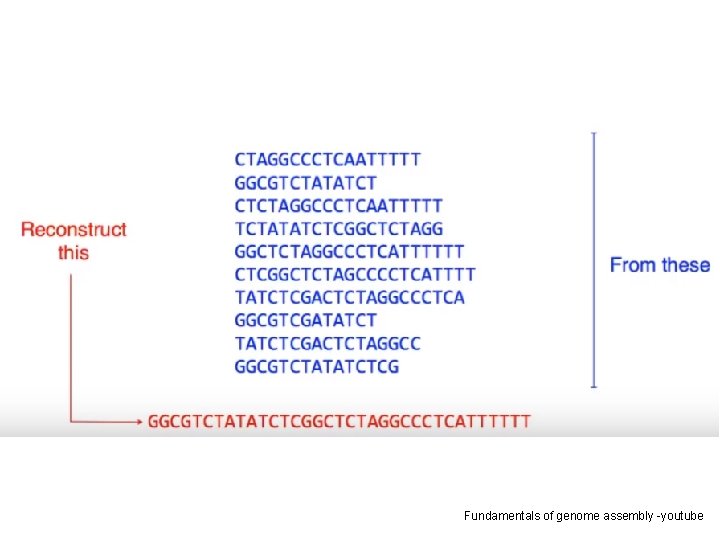
Fundamentals of genome assembly -youtube
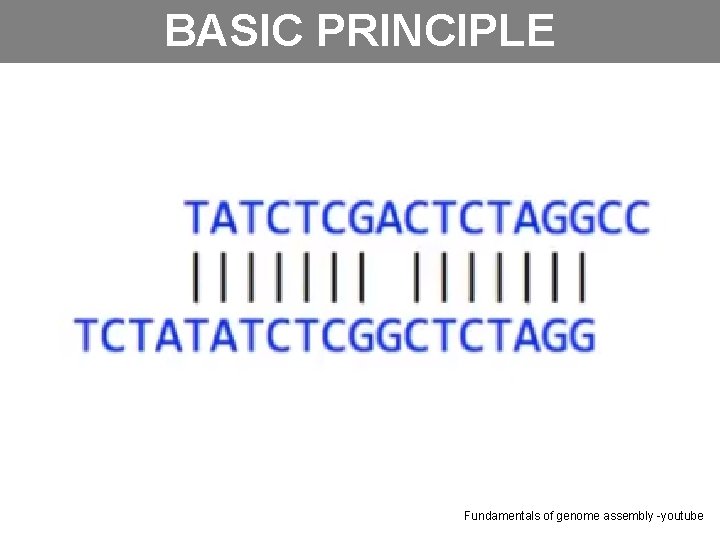
BASIC PRINCIPLE Fundamentals of genome assembly -youtube
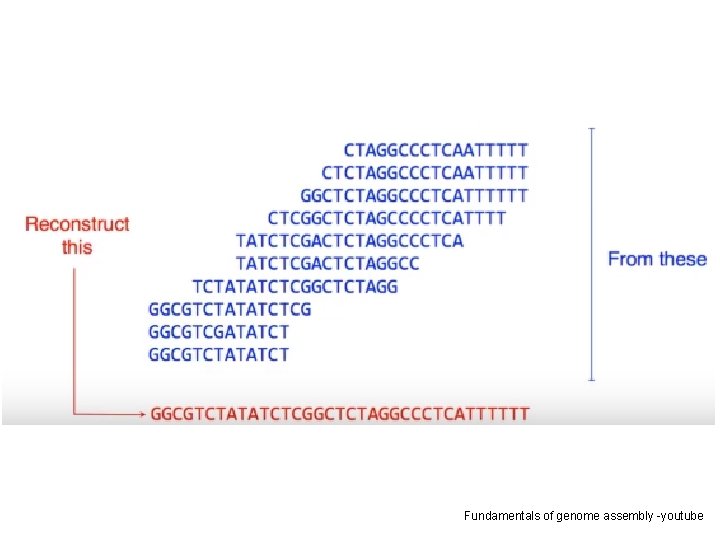
Fundamentals of genome assembly -youtube
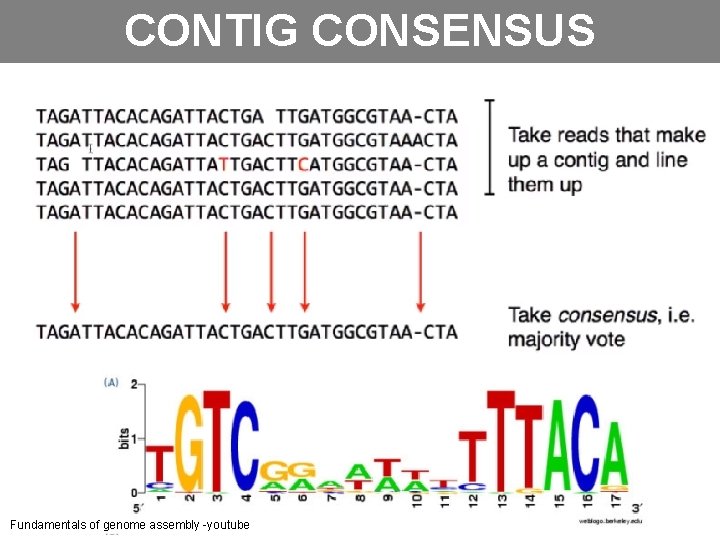
CONTIG CONSENSUS Fundamentals of genome assembly -youtube
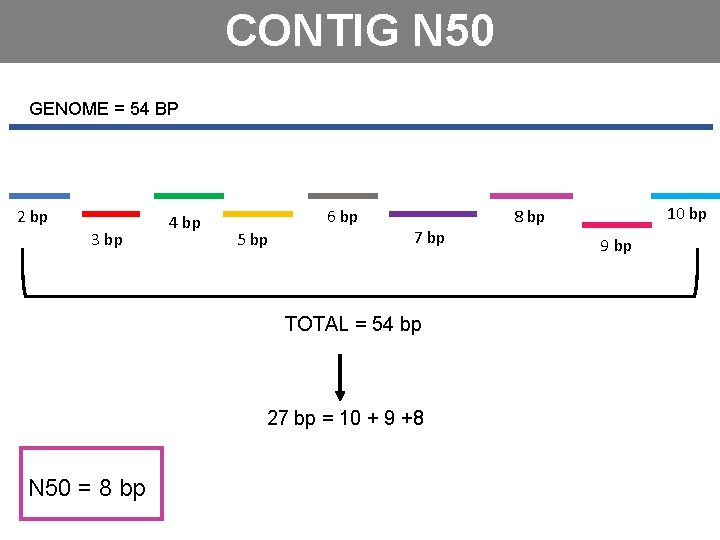
CONTIG N 50 GENOME = 54 BP 2 bp 3 bp 4 bp 6 bp 5 bp 7 bp TOTAL = 54 bp 27 bp = 10 + 9 +8 N 50 = 8 bp 10 bp 8 bp 9 bp
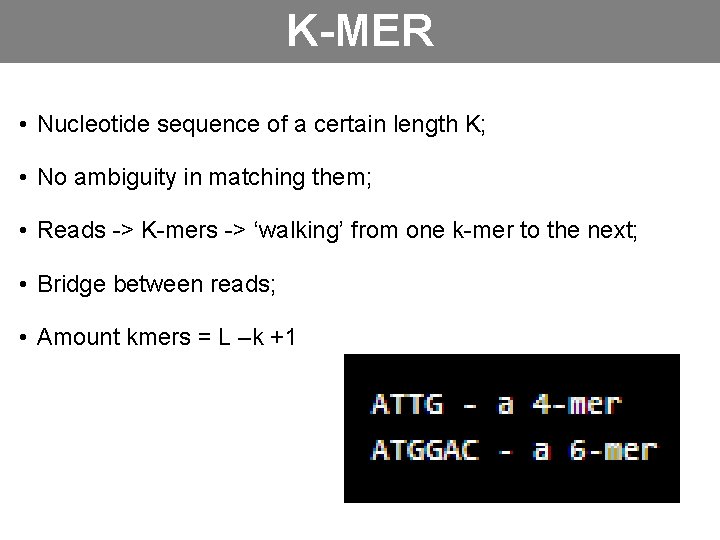
K-MER • Nucleotide sequence of a certain length K; • No ambiguity in matching them; • Reads -> K-mers -> ‘walking’ from one k-mer to the next; • Bridge between reads; • Amount kmers = L –k +1
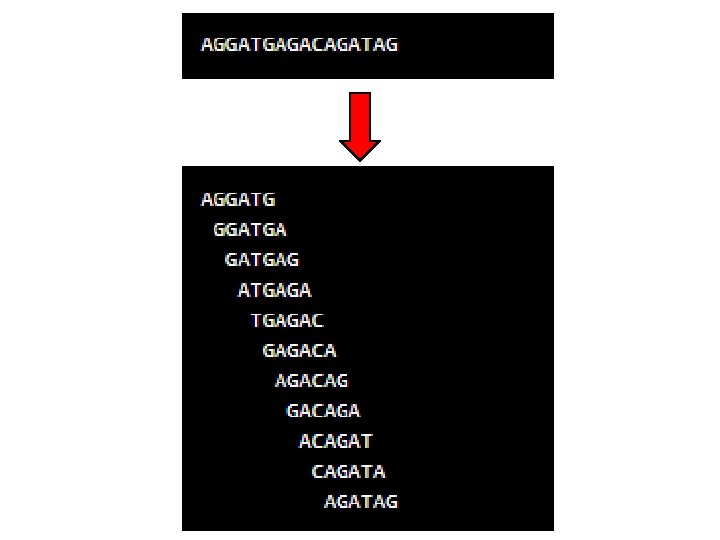
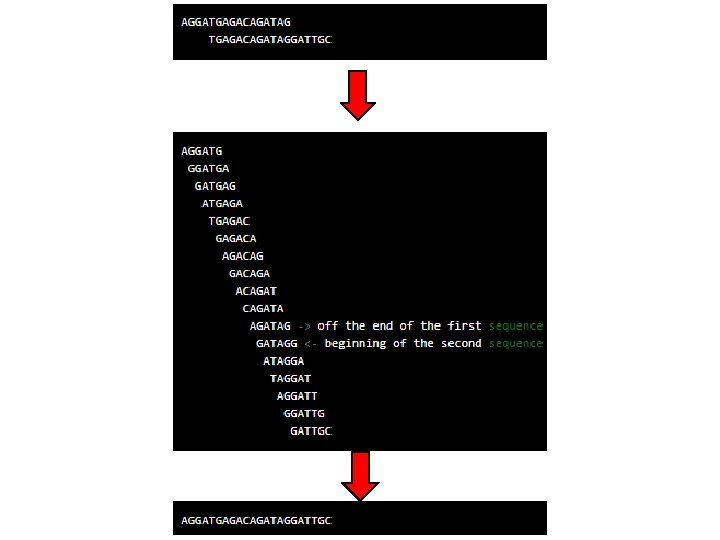
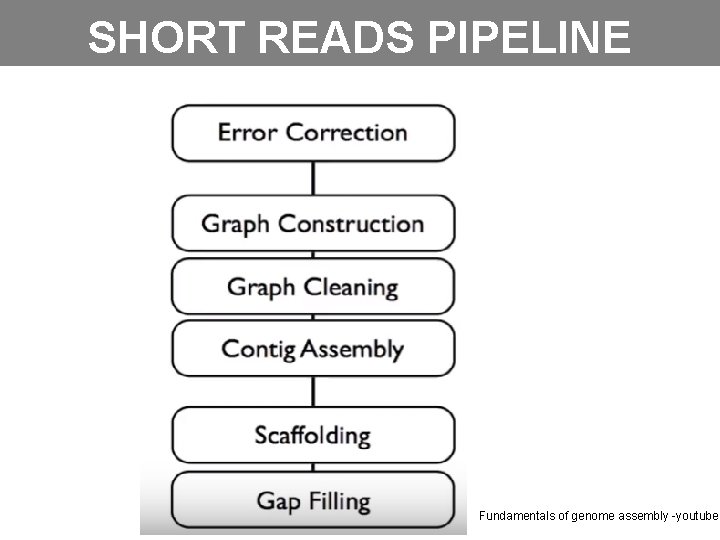
SHORT READS PIPELINE Fundamentals of genome assembly -youtube
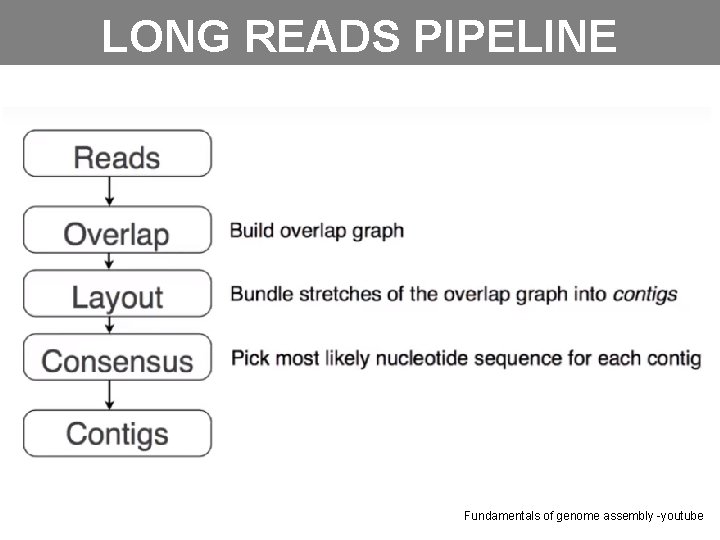
LONG READS PIPELINE Fundamentals of genome assembly -youtube
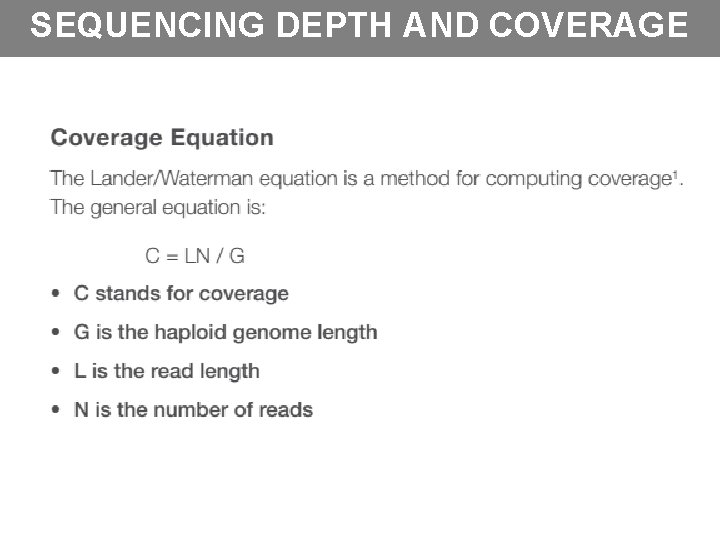
SEQUENCING DEPTH AND COVERAGE
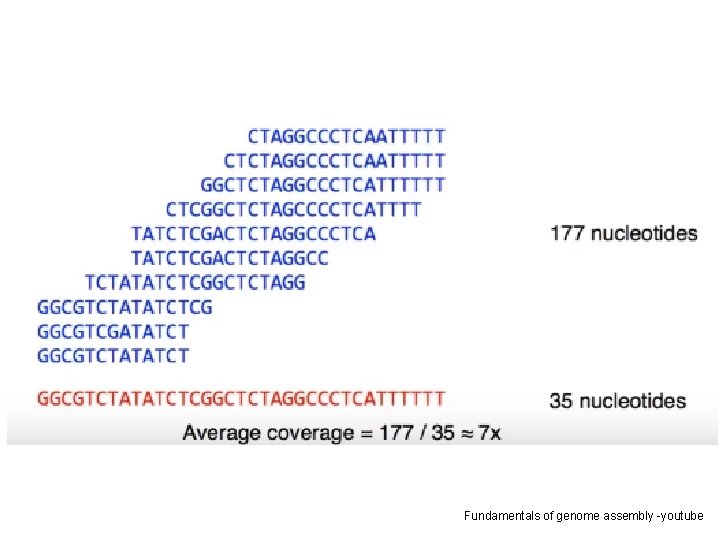
Fundamentals of genome assembly -youtube
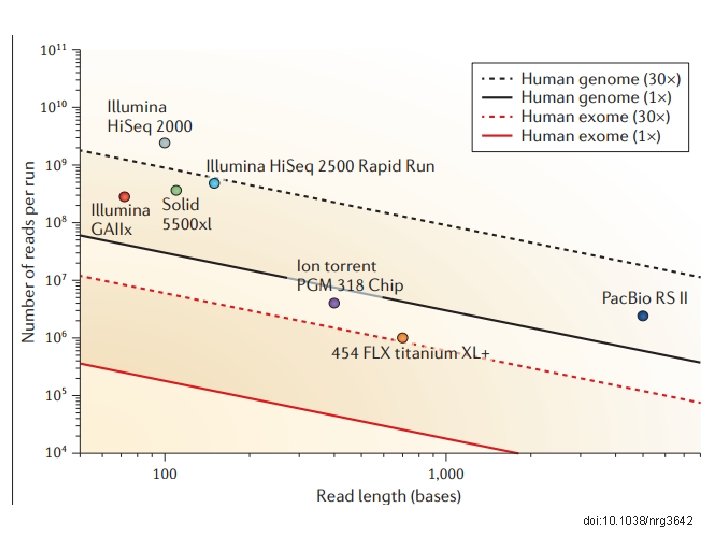
doi: 10. 1038/nrg 3642
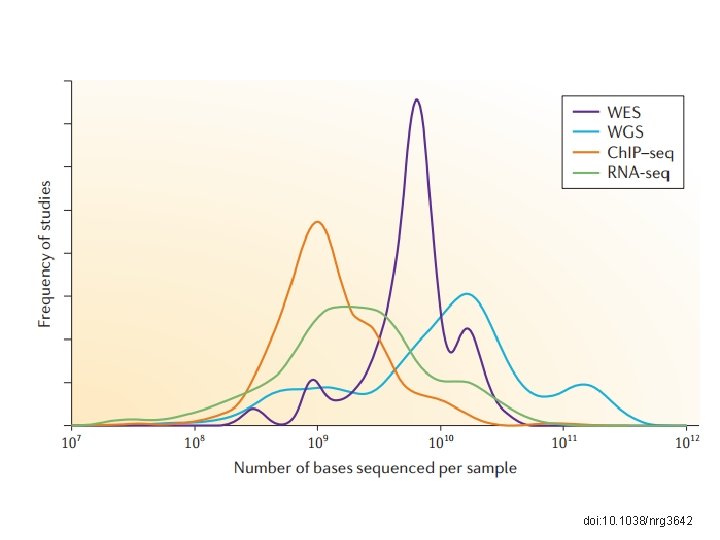
doi: 10. 1038/nrg 3642
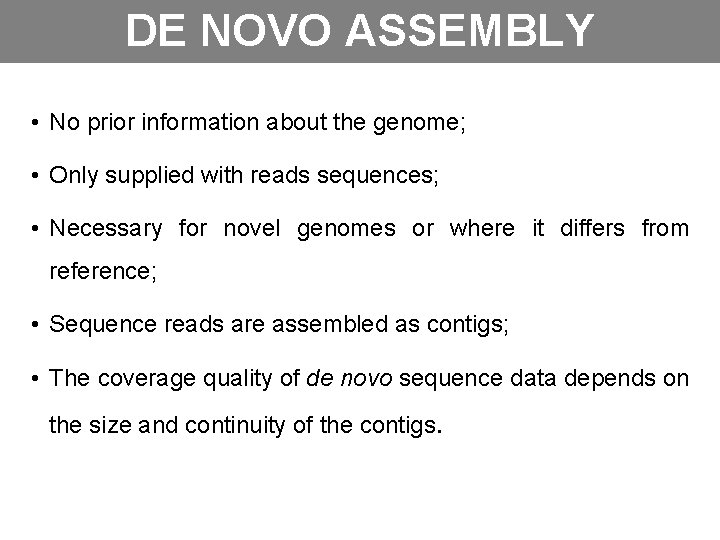
DE NOVO ASSEMBLY • No prior information about the genome; • Only supplied with reads sequences; • Necessary for novel genomes or where it differs from reference; • Sequence reads are assembled as contigs; • The coverage quality of de novo sequence data depends on the size and continuity of the contigs.
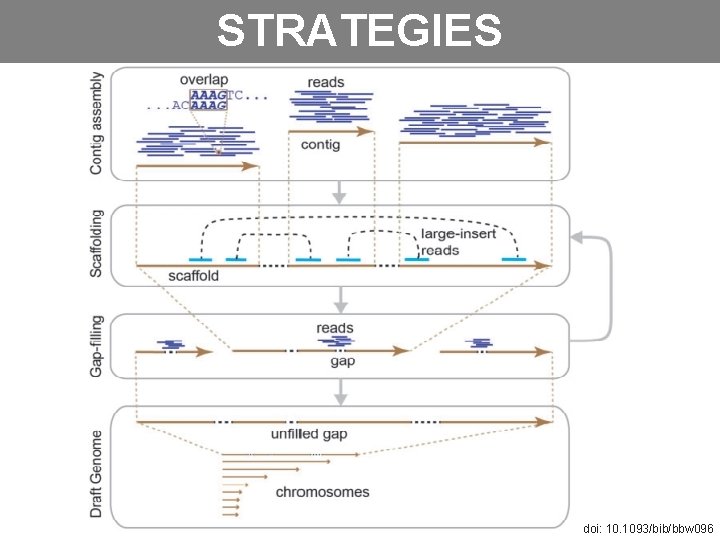
STRATEGIES doi: 10. 1093/bib/bbw 096
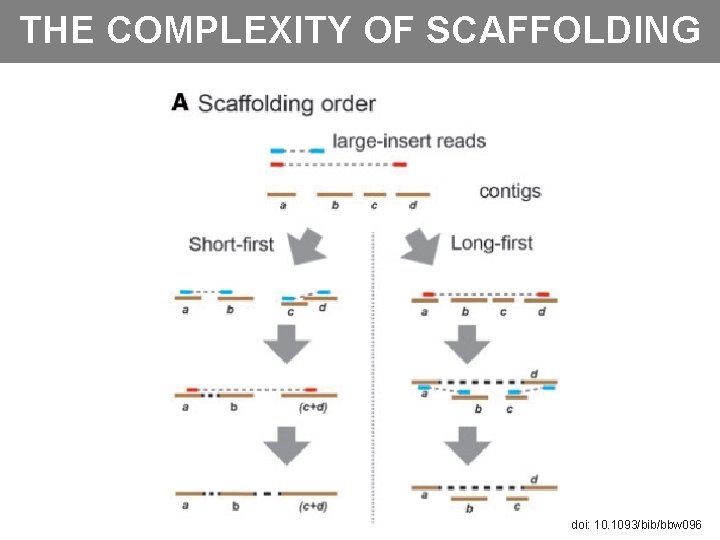
THE COMPLEXITY OF SCAFFOLDING doi: 10. 1093/bib/bbw 096
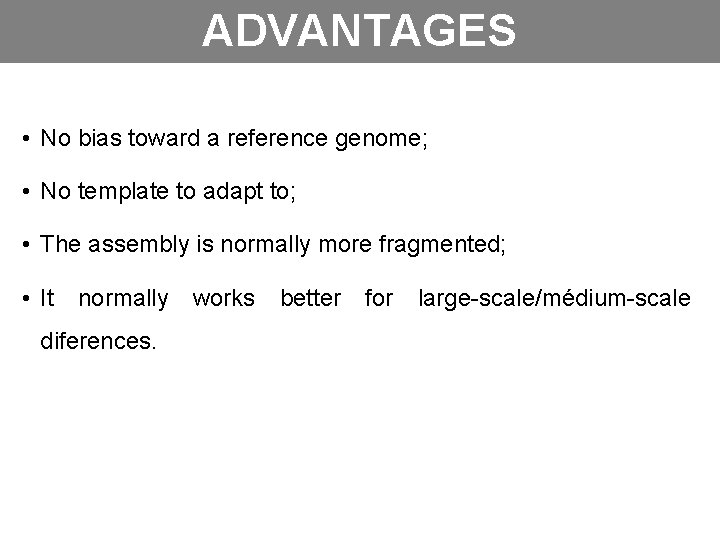
ADVANTAGES • No bias toward a reference genome; • No template to adapt to; • The assembly is normally more fragmented; • It normally works better for large-scale/médium-scale diferences.
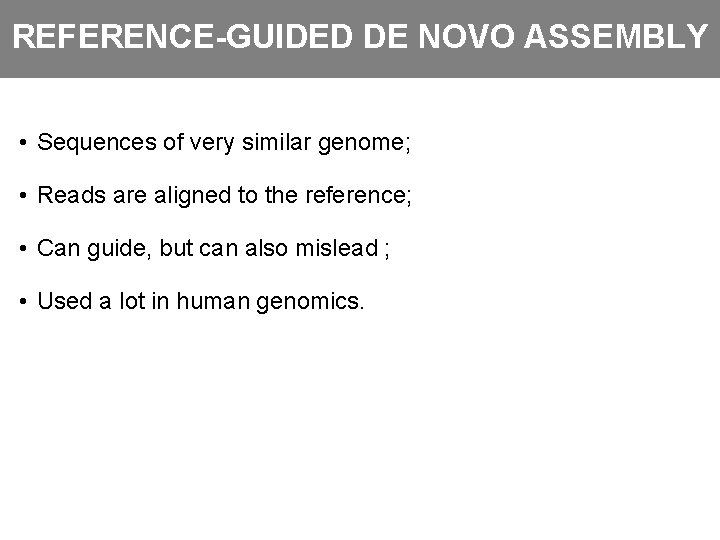
REFERENCE-GUIDED DE NOVO ASSEMBLY • Sequences of very similar genome; • Reads are aligned to the reference; • Can guide, but can also mislead ; • Used a lot in human genomics.
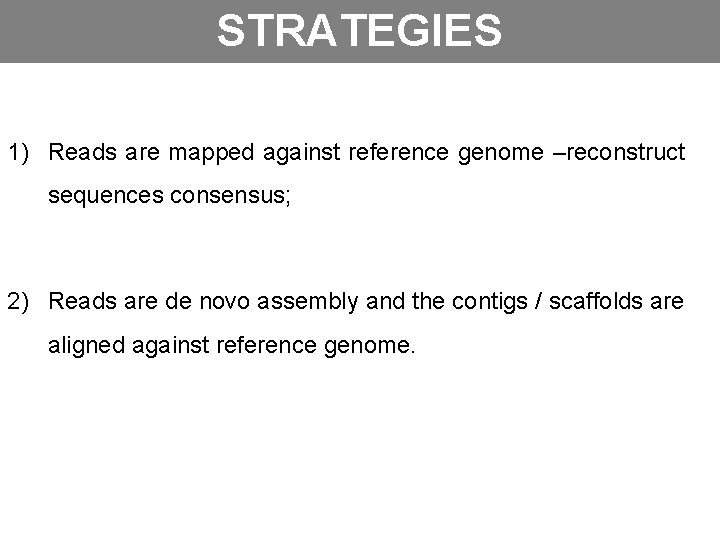
STRATEGIES 1) Reads are mapped against reference genome –reconstruct sequences consensus; 2) Reads are de novo assembly and the contigs / scaffolds are aligned against reference genome.
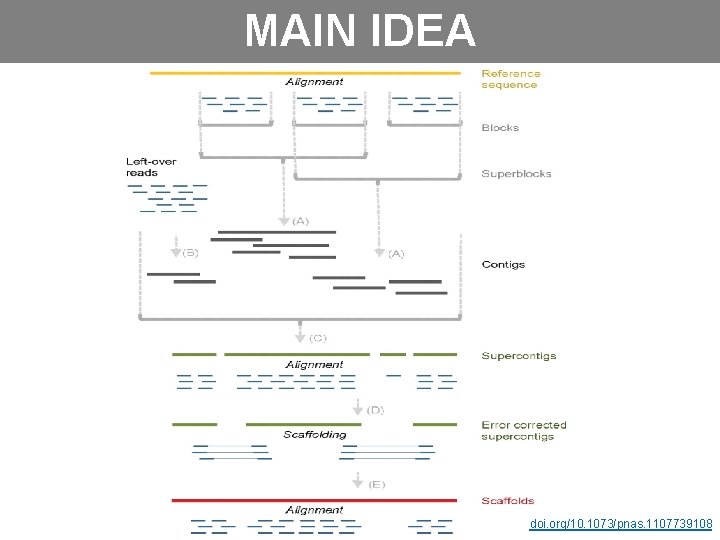
MAIN IDEA doi. org/10. 1073/pnas. 1107739108
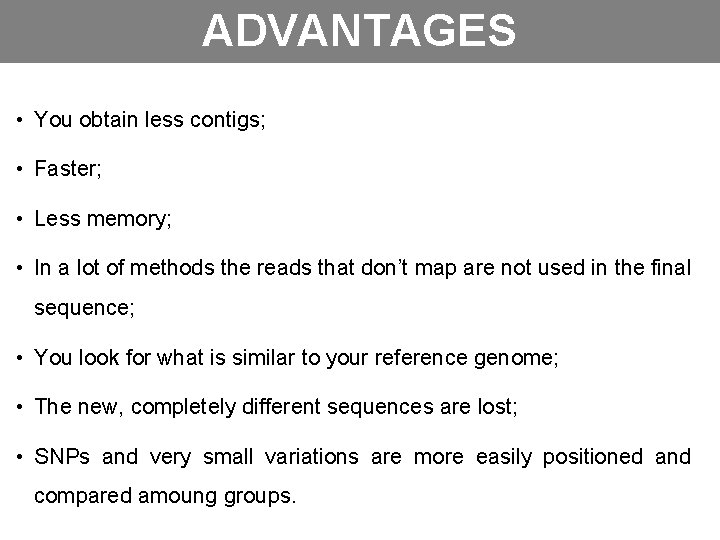
ADVANTAGES • You obtain less contigs; • Faster; • Less memory; • In a lot of methods the reads that don’t map are not used in the final sequence; • You look for what is similar to your reference genome; • The new, completely different sequences are lost; • SNPs and very small variations are more easily positioned and compared amoung groups.
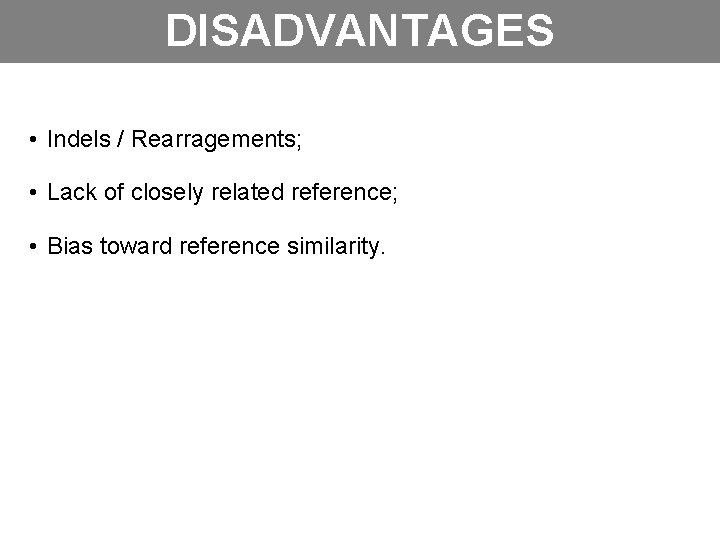
DISADVANTAGES • Indels / Rearragements; • Lack of closely related reference; • Bias toward reference similarity.
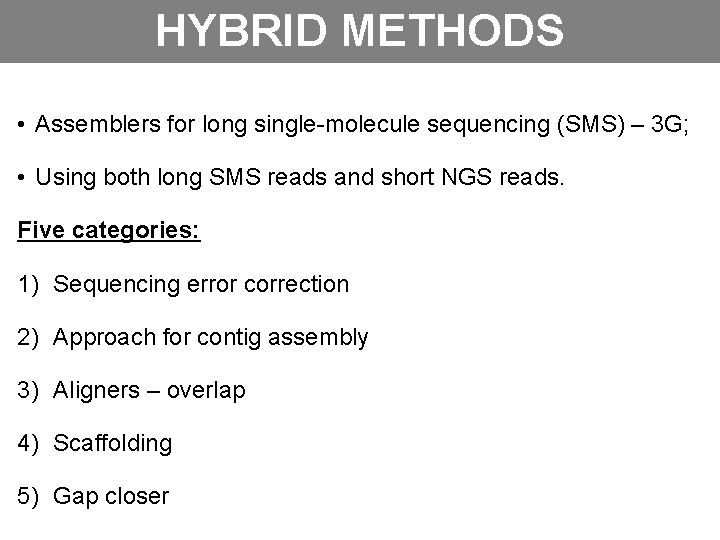
HYBRID METHODS • Assemblers for long single-molecule sequencing (SMS) – 3 G; • Using both long SMS reads and short NGS reads. Five categories: 1) Sequencing error correction 2) Approach for contig assembly 3) Aligners – overlap 4) Scaffolding 5) Gap closer
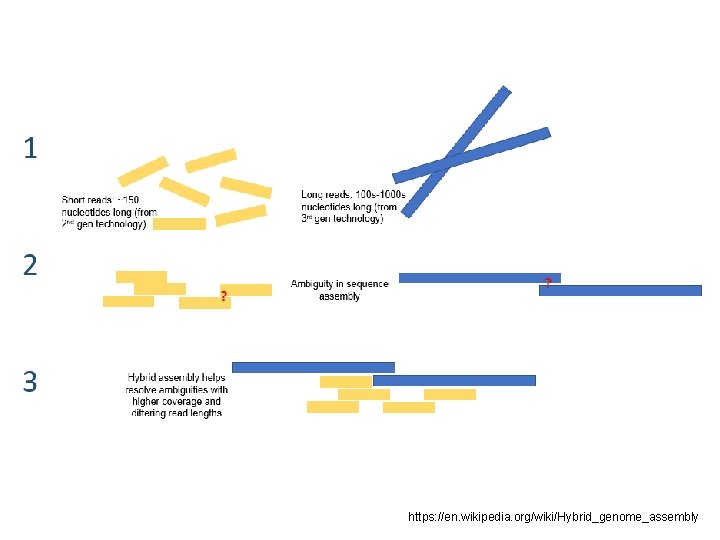
https: //en. wikipedia. org/wiki/Hybrid_genome_assembly
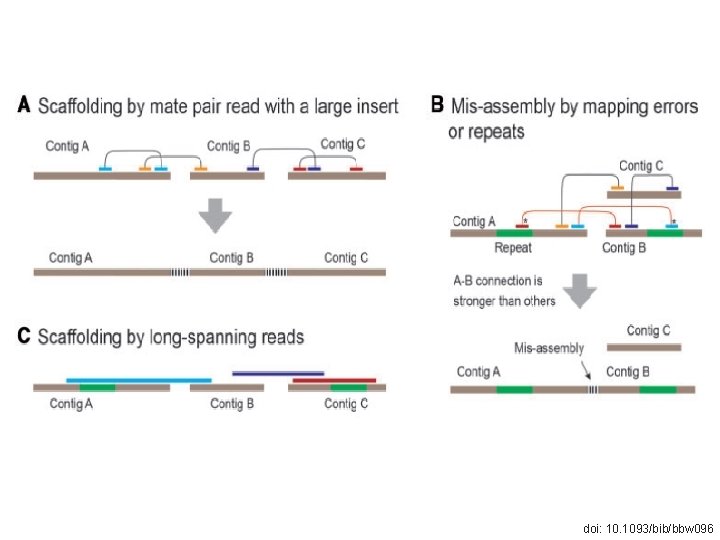
doi: 10. 1093/bib/bbw 096
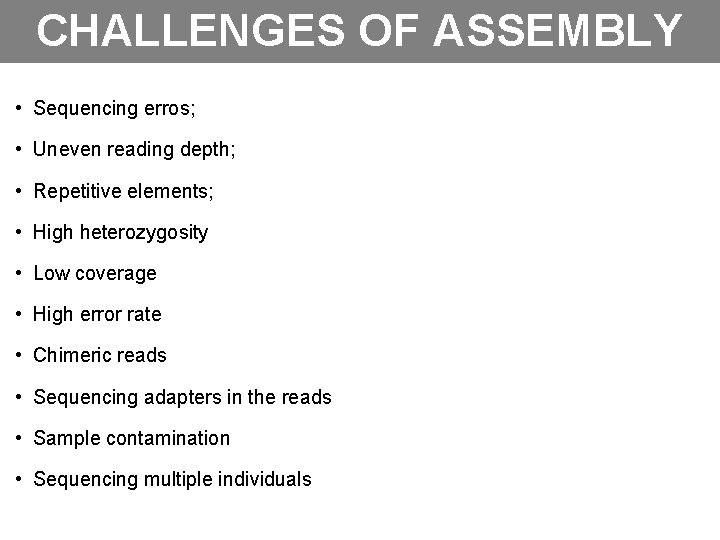
CHALLENGES OF ASSEMBLY • Sequencing erros; • Uneven reading depth; • Repetitive elements; • High heterozygosity • Low coverage • High error rate • Chimeric reads • Sequencing adapters in the reads • Sample contamination • Sequencing multiple individuals
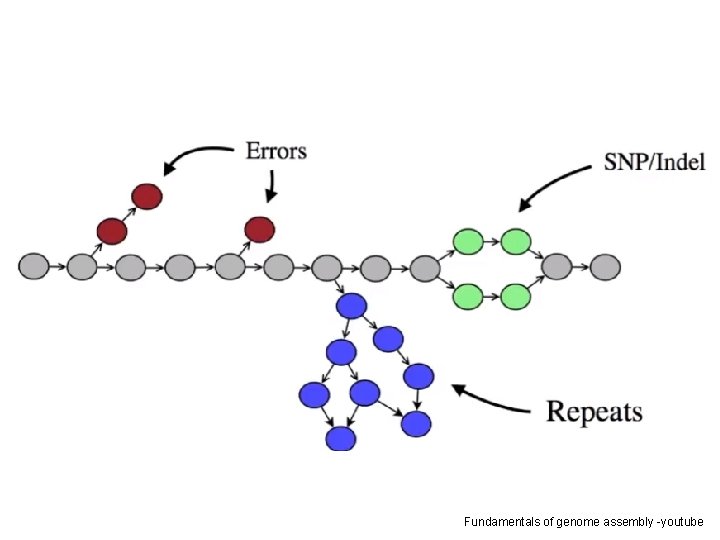
Fundamentals of genome assembly -youtube
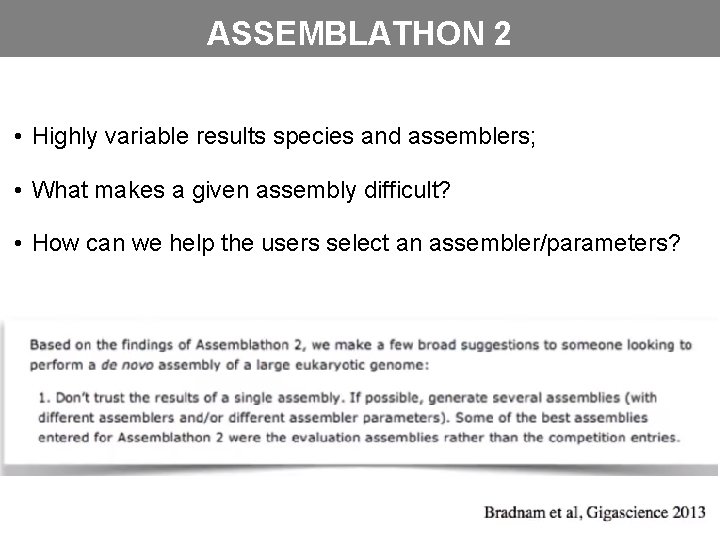
ASSEMBLATHON 2 • Highly variable results species and assemblers; • What makes a given assembly difficult? • How can we help the users select an assembler/parameters?
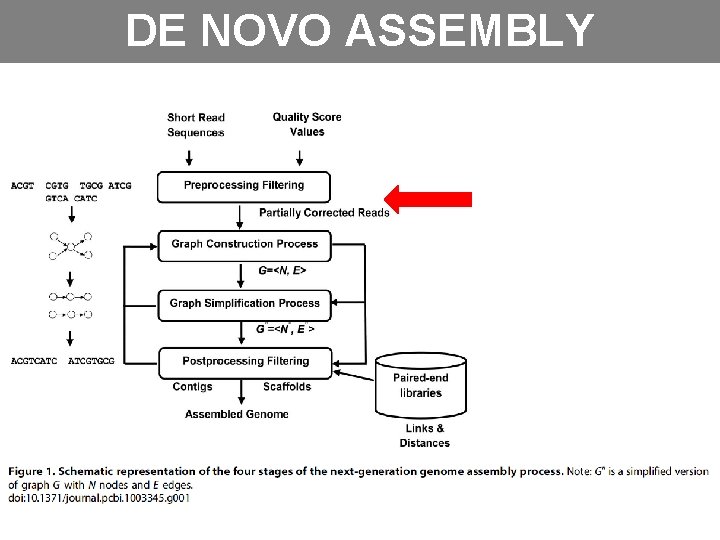
DE NOVO ASSEMBLY
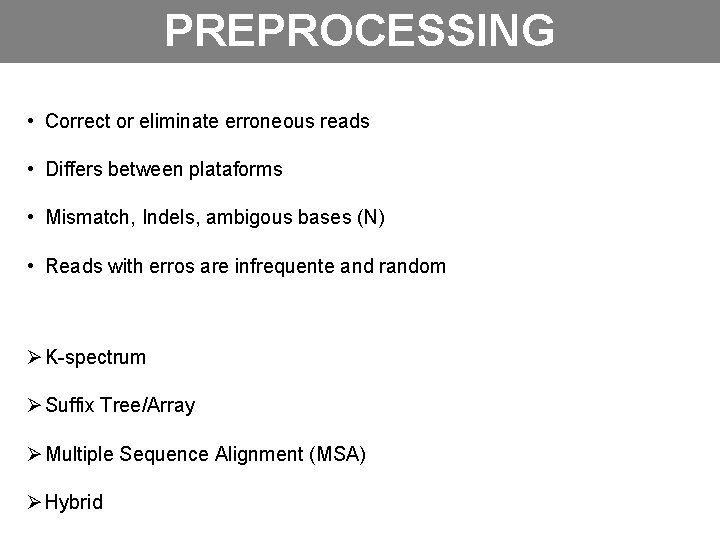
PREPROCESSING • Correct or eliminate erroneous reads • Differs between plataforms • Mismatch, Indels, ambigous bases (N) • Reads with erros are infrequente and random Ø K-spectrum Ø Suffix Tree/Array Ø Multiple Sequence Alignment (MSA) Ø Hybrid
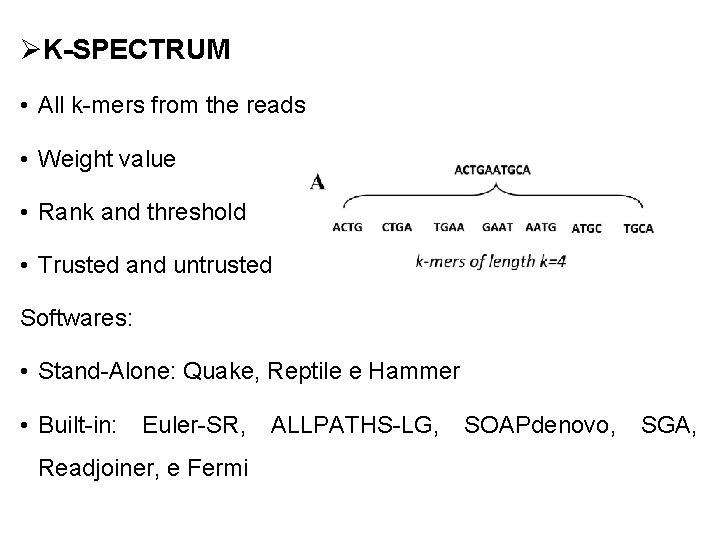
ØK-SPECTRUM • All k-mers from the reads • Weight value • Rank and threshold • Trusted and untrusted Softwares: • Stand-Alone: Quake, Reptile e Hammer • Built-in: Euler-SR, ALLPATHS-LG, SOAPdenovo, SGA, Readjoiner, e Fermi
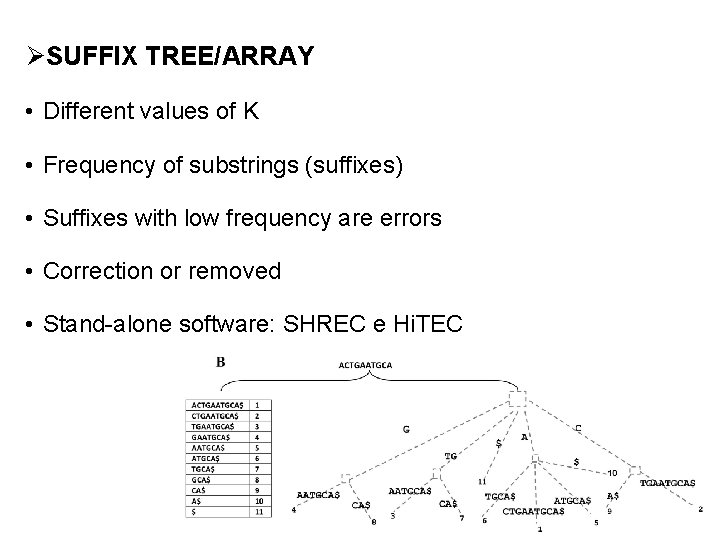
ØSUFFIX TREE/ARRAY • Different values of K • Frequency of substrings (suffixes) • Suffixes with low frequency are errors • Correction or removed • Stand-alone software: SHREC e Hi. TEC
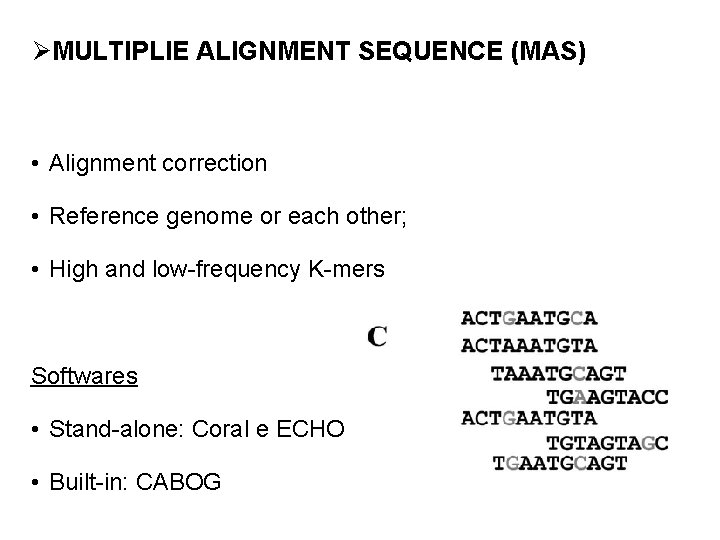
ØMULTIPLIE ALIGNMENT SEQUENCE (MAS) • Alignment correction • Reference genome or each other; • High and low-frequency K-mers Softwares • Stand-alone: Coral e ECHO • Built-in: CABOG
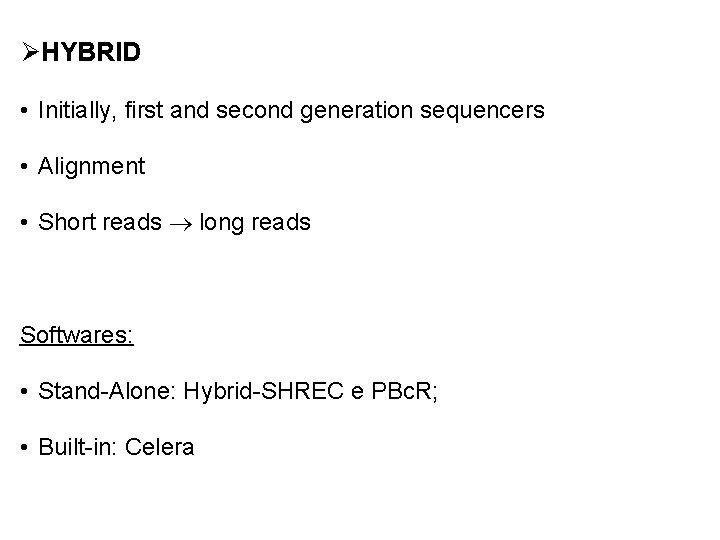
ØHYBRID • Initially, first and second generation sequencers • Alignment • Short reads long reads Softwares: • Stand-Alone: Hybrid-SHREC e PBc. R; • Built-in: Celera
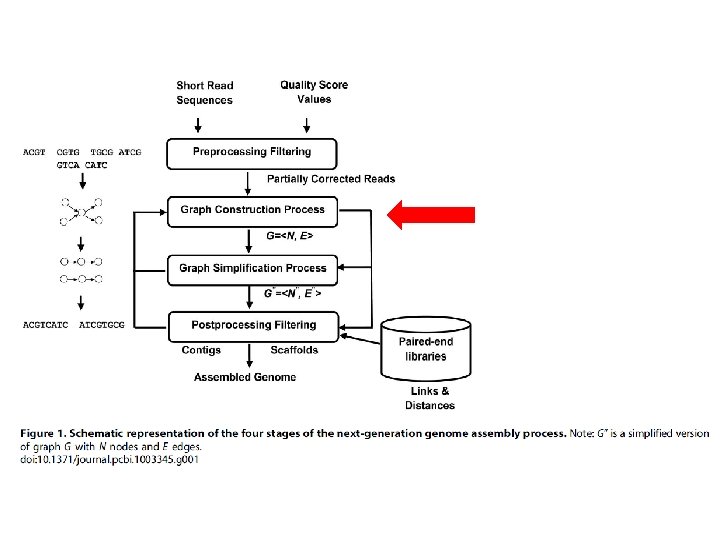
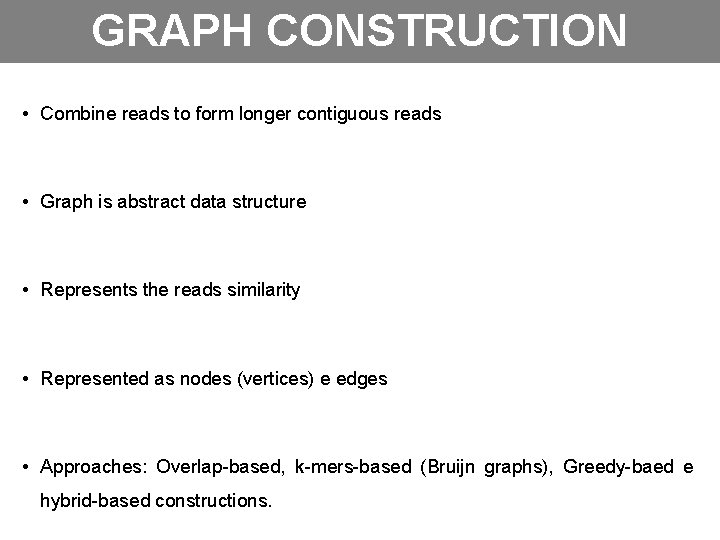
GRAPH CONSTRUCTION • Combine reads to form longer contiguous reads • Graph is abstract data structure • Represents the reads similarity • Represented as nodes (vertices) e edges • Approaches: Overlap-based, k-mers-based (Bruijn graphs), Greedy-baed e hybrid-based constructions.
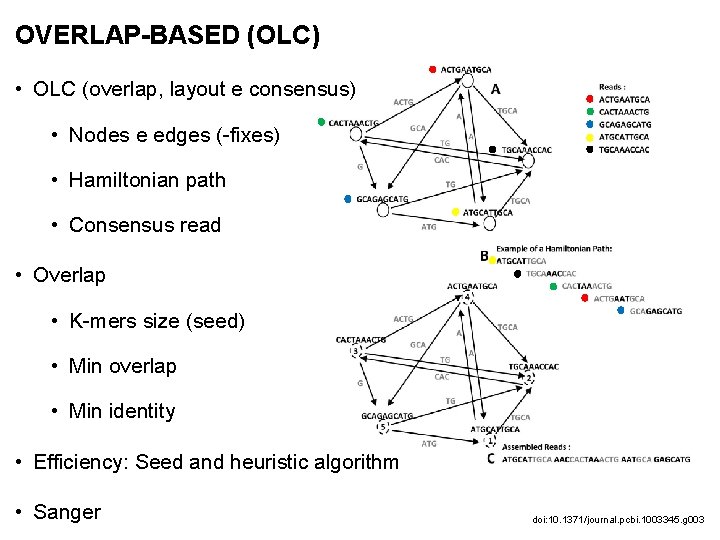
OVERLAP-BASED (OLC) • OLC (overlap, layout e consensus) • Nodes e edges (-fixes) • Hamiltonian path • Consensus read • Overlap • K-mers size (seed) • Min overlap • Min identity • Efficiency: Seed and heuristic algorithm • Sanger doi: 10. 1371/journal. pcbi. 1003345. g 003
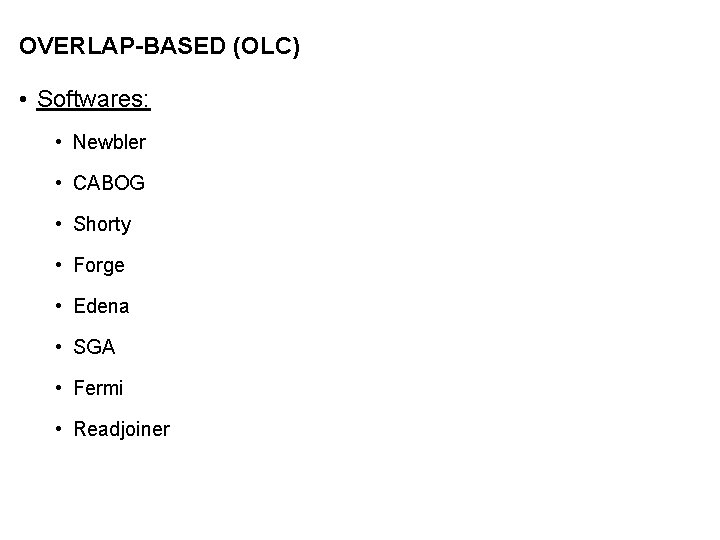
OVERLAP-BASED (OLC) • Softwares: • Newbler • CABOG • Shorty • Forge • Edena • SGA • Fermi • Readjoiner
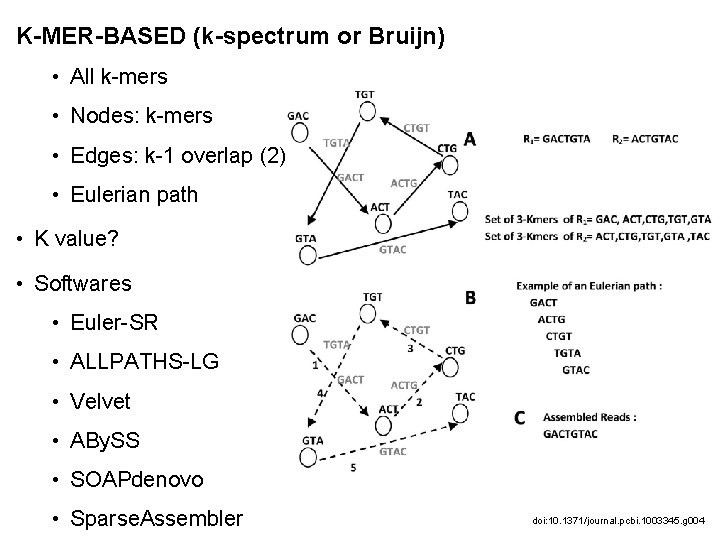
K-MER-BASED (k-spectrum or Bruijn) • All k-mers • Nodes: k-mers • Edges: k-1 overlap (2) • Eulerian path • K value? • Softwares • Euler-SR • ALLPATHS-LG • Velvet • ABy. SS • SOAPdenovo • Sparse. Assembler doi: 10. 1371/journal. pcbi. 1003345. g 004
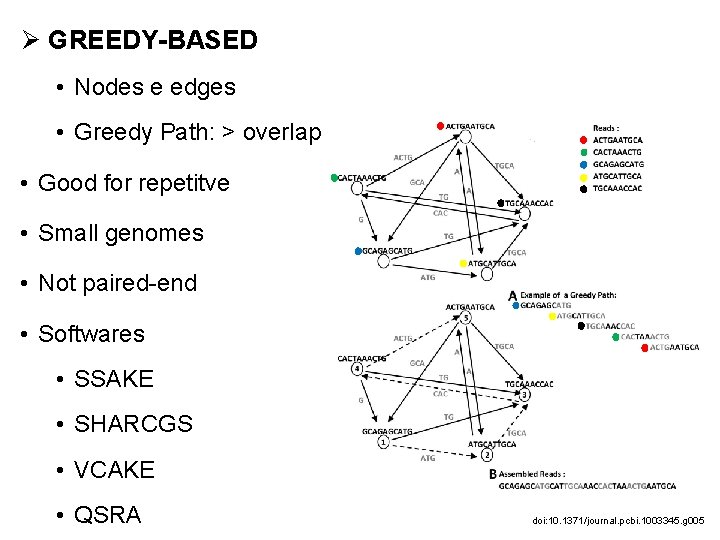
Ø GREEDY-BASED • Nodes e edges • Greedy Path: > overlap • Good for repetitve • Small genomes • Not paired-end • Softwares • SSAKE • SHARCGS • VCAKE • QSRA doi: 10. 1371/journal. pcbi. 1003345. g 005
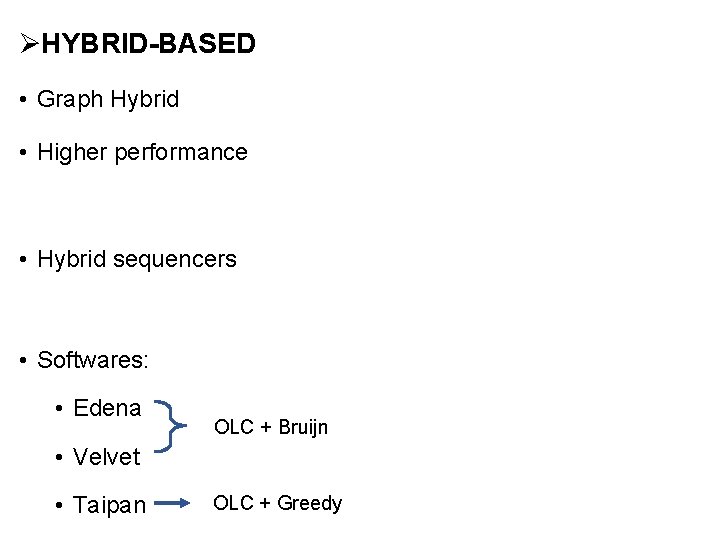
ØHYBRID-BASED • Graph Hybrid • Higher performance • Hybrid sequencers • Softwares: • Edena OLC + Bruijn • Velvet • Taipan OLC + Greedy
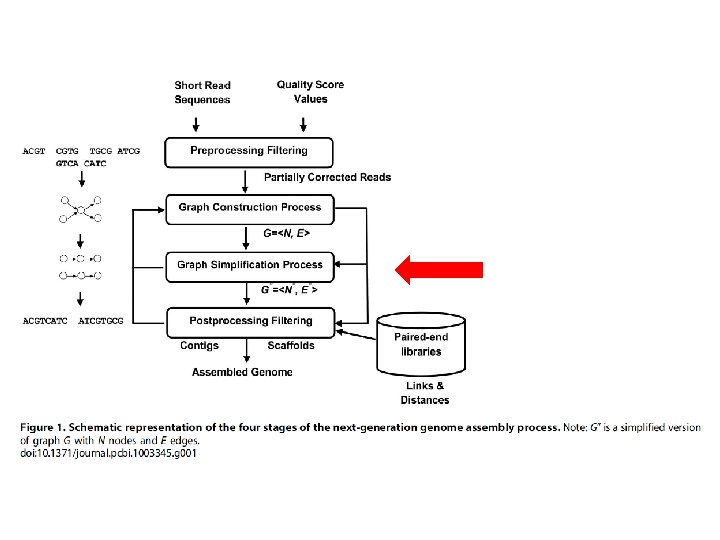
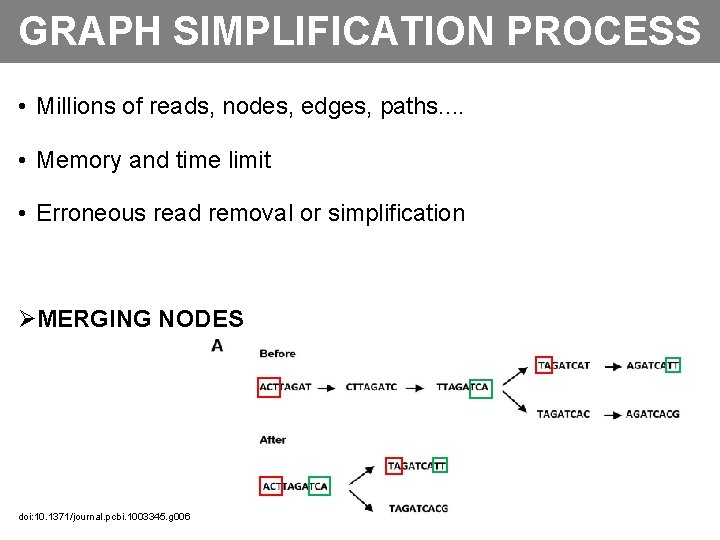
GRAPH SIMPLIFICATION PROCESS • Millions of reads, nodes, edges, paths. . • Memory and time limit • Erroneous read removal or simplification ØMERGING NODES doi: 10. 1371/journal. pcbi. 1003345. g 006
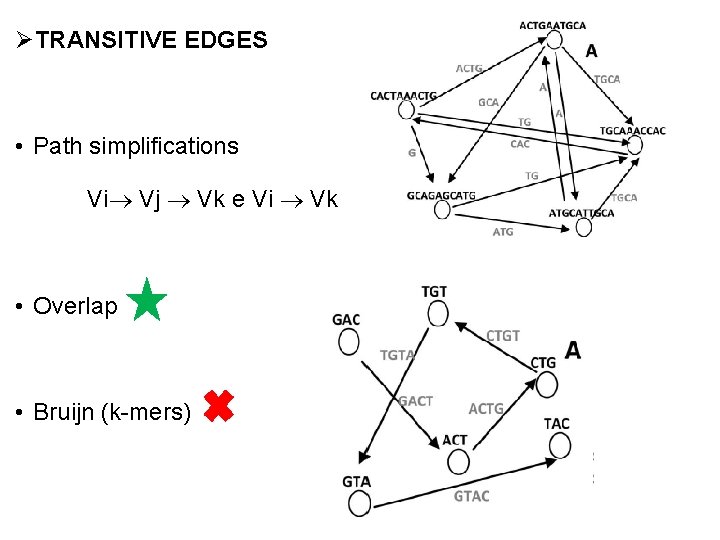
ØTRANSITIVE EDGES • Path simplifications Vi Vj Vk e Vi Vk • Overlap • Bruijn (k-mers)
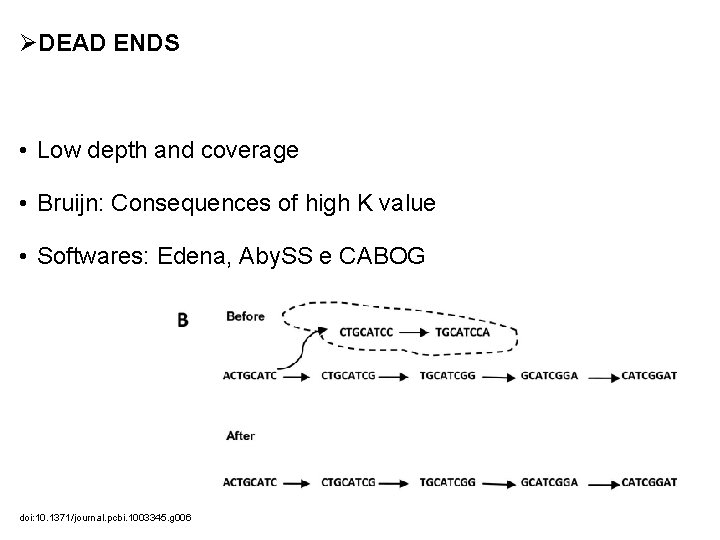
ØDEAD ENDS • Low depth and coverage • Bruijn: Consequences of high K value • Softwares: Edena, Aby. SS e CABOG doi: 10. 1371/journal. pcbi. 1003345. g 006
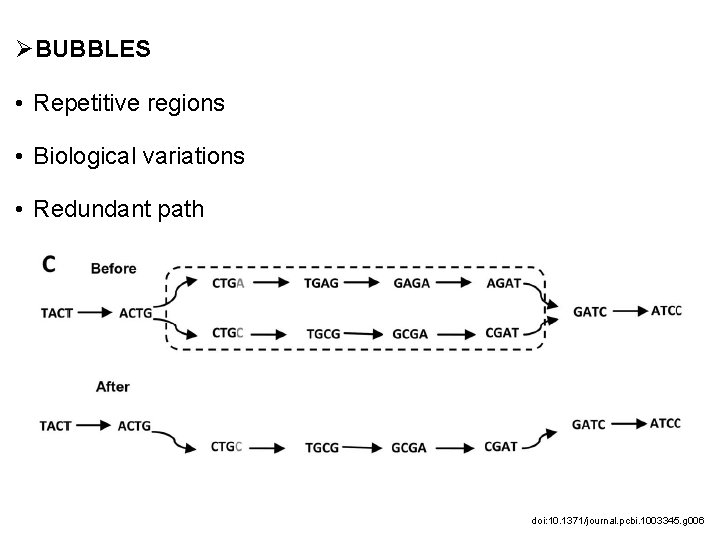
ØBUBBLES • Repetitive regions • Biological variations • Redundant path doi: 10. 1371/journal. pcbi. 1003345. g 006
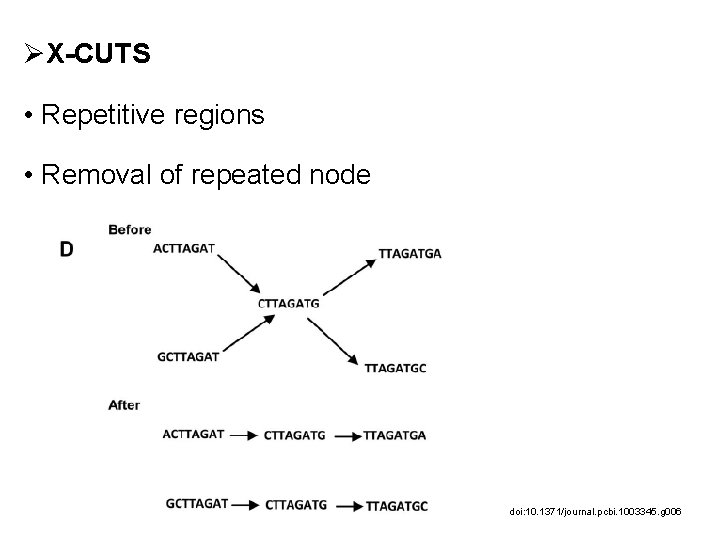
ØX-CUTS • Repetitive regions • Removal of repeated node doi: 10. 1371/journal. pcbi. 1003345. g 006
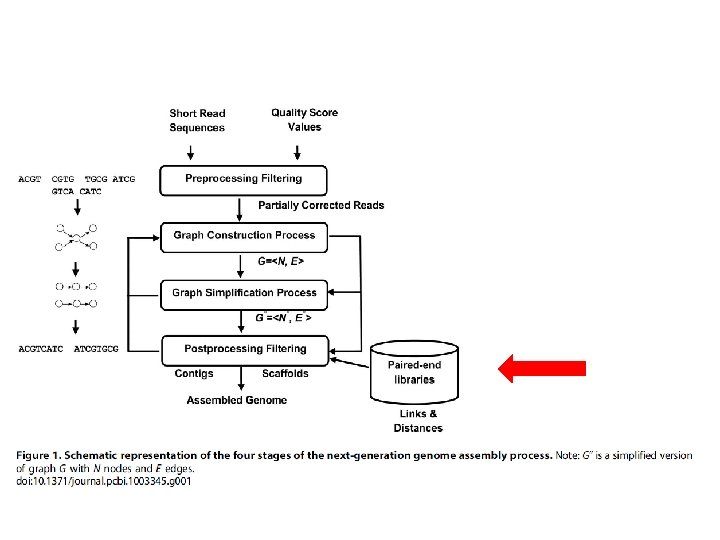
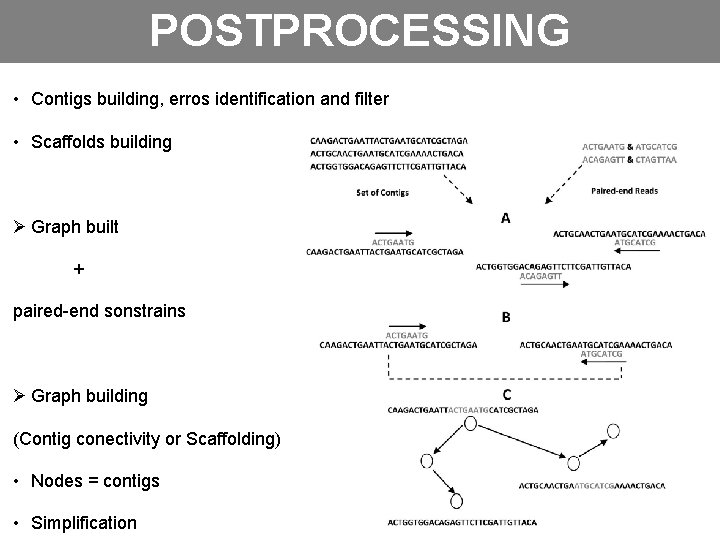
POSTPROCESSING • Contigs building, erros identification and filter • Scaffolds building Ø Graph built + paired-end sonstrains Ø Graph building (Contig conectivity or Scaffolding) • Nodes = contigs • Simplification
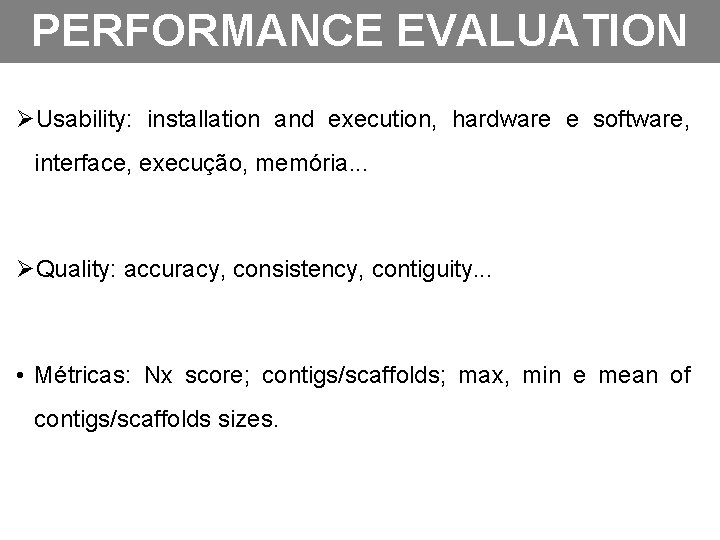
PERFORMANCE EVALUATION ØUsability: installation and execution, hardware e software, interface, execução, memória. . . ØQuality: accuracy, consistency, contiguity. . . • Métricas: Nx score; contigs/scaffolds; max, min e mean of contigs/scaffolds sizes.
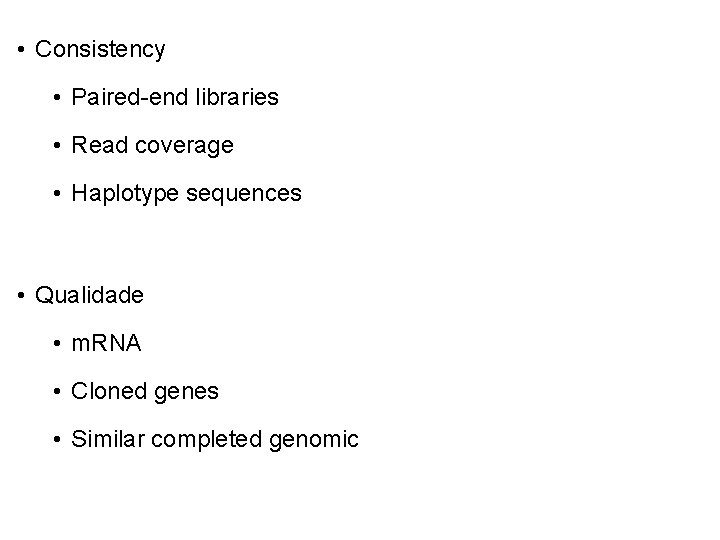
• Consistency • Paired-end libraries • Read coverage • Haplotype sequences • Qualidade • m. RNA • Cloned genes • Similar completed genomic
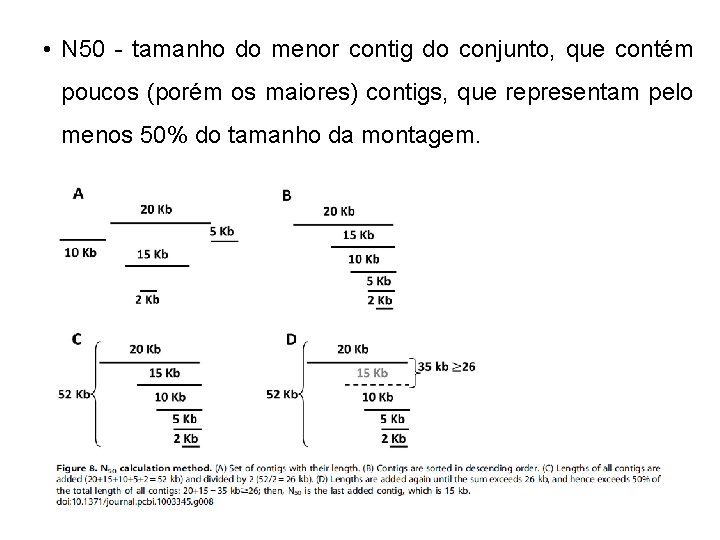
• N 50 - tamanho do menor contig do conjunto, que contém poucos (porém os maiores) contigs, que representam pelo menos 50% do tamanho da montagem.
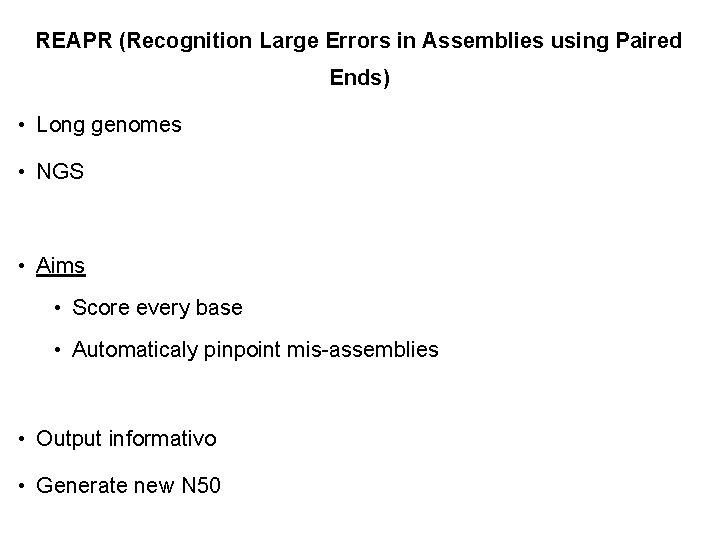
REAPR (Recognition Large Errors in Assemblies using Paired Ends) • Long genomes • NGS • Aims • Score every base • Automaticaly pinpoint mis-assemblies • Output informativo • Generate new N 50
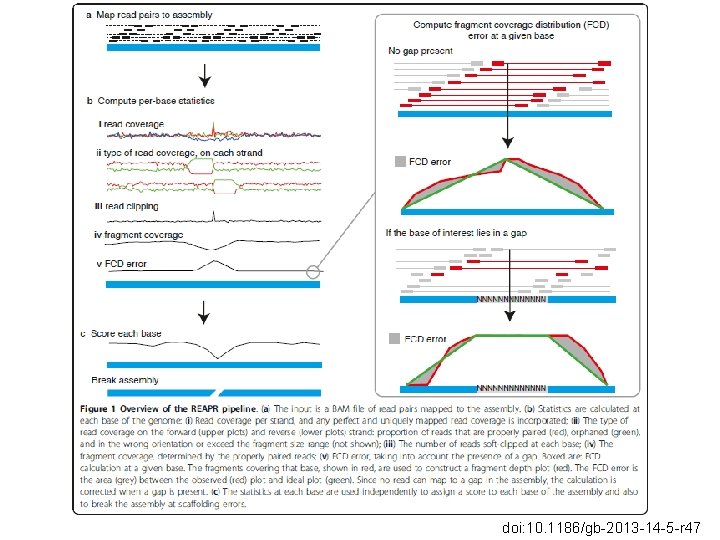
doi: 10. 1186/gb-2013 -14 -5 -r 47
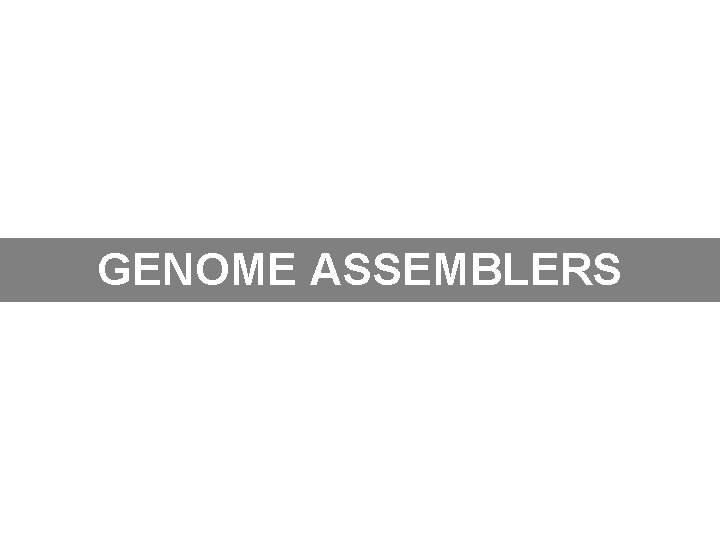
GENOME ASSEMBLERS
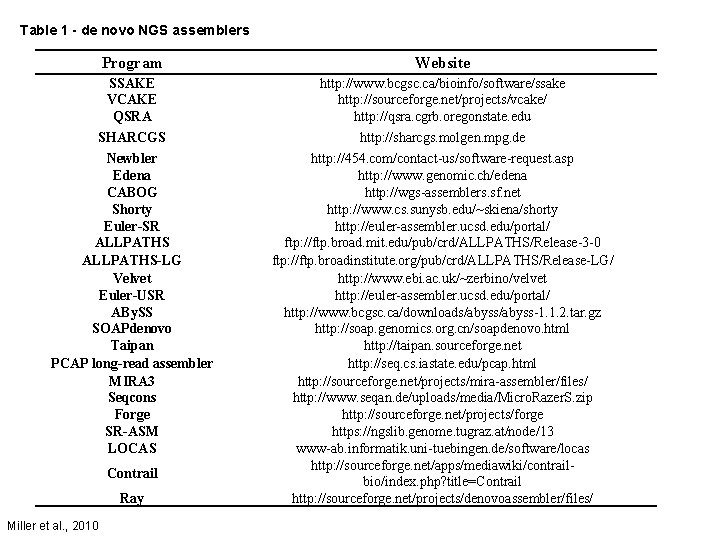
Table 1 - de novo NGS assemblers Program Website SSAKE VCAKE QSRA http: //www. bcgsc. ca/bioinfo/software/ssake http: //sourceforge. net/projects/vcake/ http: //qsra. cgrb. oregonstate. edu SHARCGS http: //sharcgs. molgen. mpg. de Newbler Edena CABOG Shorty Euler-SR ALLPATHS-LG Velvet Euler-USR ABy. SS SOAPdenovo Taipan PCAP long-read assembler MIRA 3 Seqcons Forge SR-ASM LOCAS http: //454. com/contact-us/software-request. asp http: //www. genomic. ch/edena http: //wgs-assemblers. sf. net http: //www. cs. sunysb. edu/~skiena/shorty http: //euler-assembler. ucsd. edu/portal/ ftp: //ftp. broad. mit. edu/pub/crd/ALLPATHS/Release-3 -0 ftp: //ftp. broadinstitute. org/pub/crd/ALLPATHS/Release-LG/ http: //www. ebi. ac. uk/~zerbino/velvet http: //euler-assembler. ucsd. edu/portal/ http: //www. bcgsc. ca/downloads/abyss-1. 1. 2. tar. gz http: //soap. genomics. org. cn/soapdenovo. html http: //taipan. sourceforge. net http: //seq. cs. iastate. edu/pcap. html http: //sourceforge. net/projects/mira-assembler/files/ http: //www. seqan. de/uploads/media/Micro. Razer. S. zip http: //sourceforge. net/projects/forge https: //ngslib. genome. tugraz. at/node/13 www-ab. informatik. uni-tuebingen. de/software/locas http: //sourceforge. net/apps/mediawiki/contrailbio/index. php? title=Contrail http: //sourceforge. net/projects/denovoassembler/files/ Contrail Ray Miller et al. , 2010
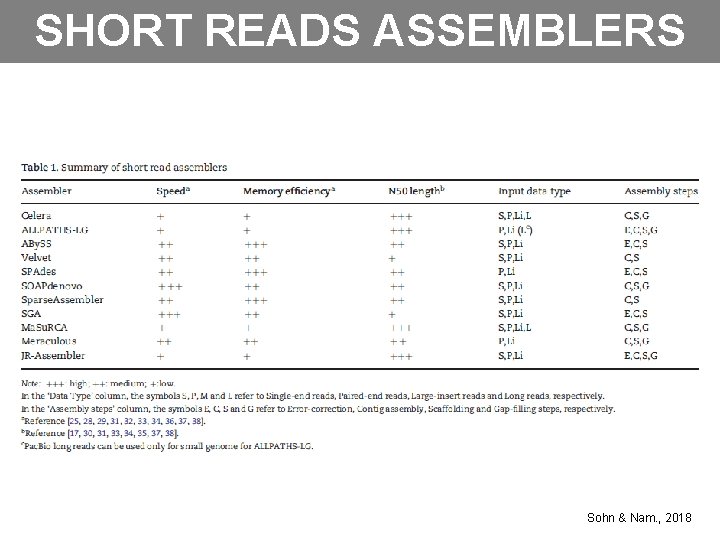
SHORT READS ASSEMBLERS Sohn & Nam. , 2018
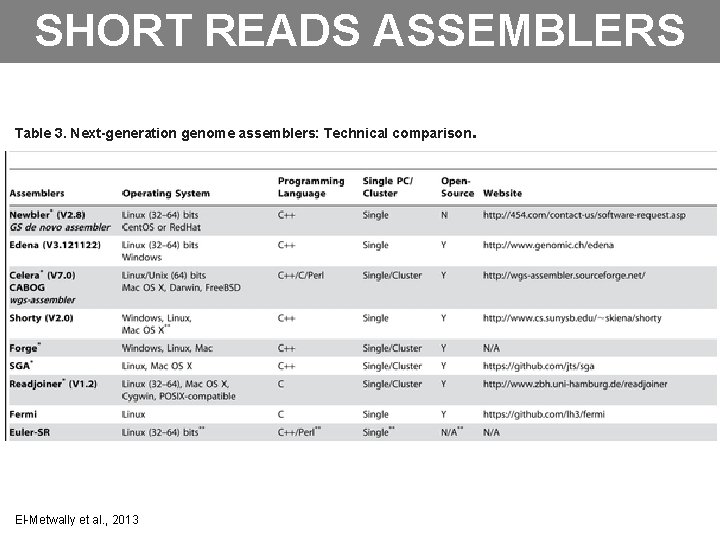
SHORT READS ASSEMBLERS Table 3. Next-generation genome assemblers: Technical comparison. El-Metwally et al. , 2013
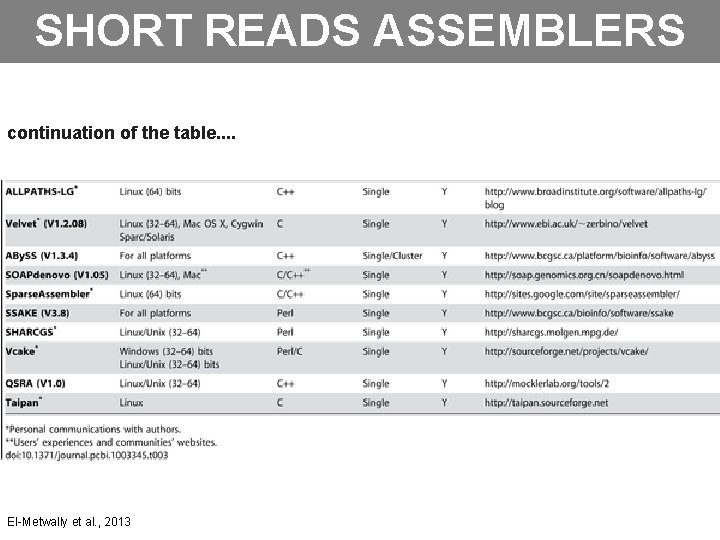
SHORT READS ASSEMBLERS continuation of the table. . El-Metwally et al. , 2013
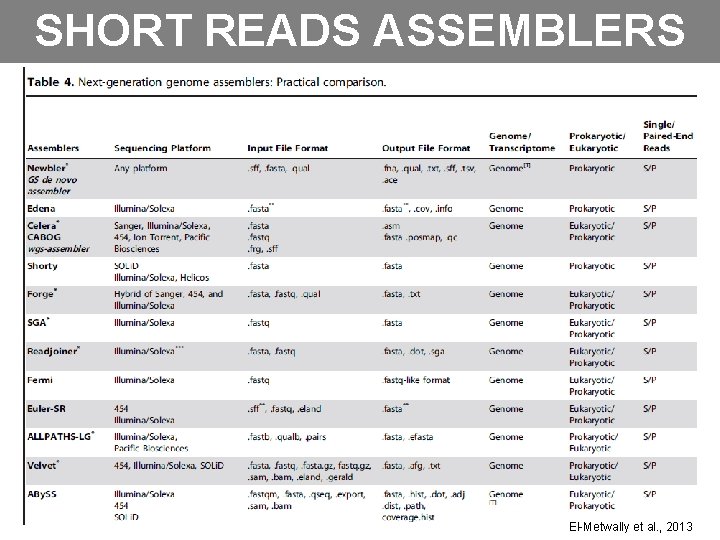
SHORT READS ASSEMBLERS El-Metwally et al. , 2013
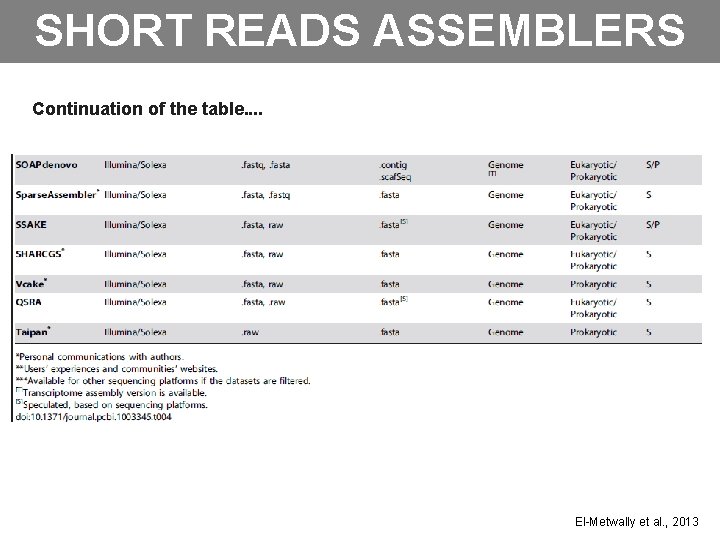
SHORT READS ASSEMBLERS Continuation of the table. . El-Metwally et al. , 2013
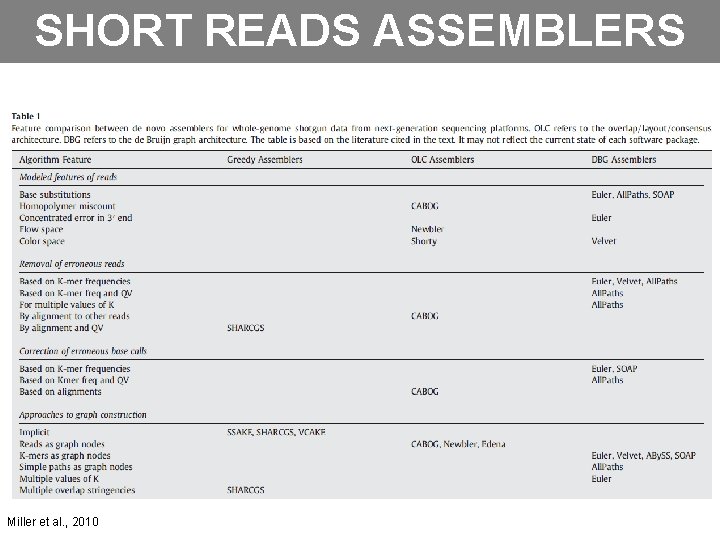
SHORT READS ASSEMBLERS Miller et al. , 2010
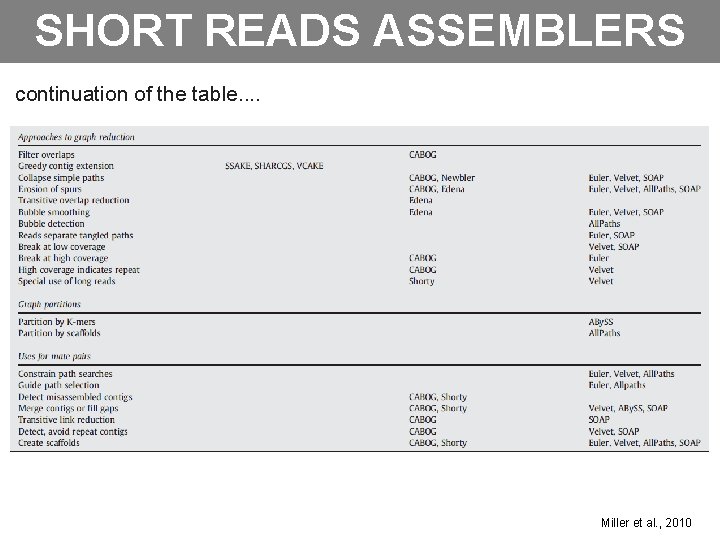
SHORT READS ASSEMBLERS continuation of the table. . Miller et al. , 2010
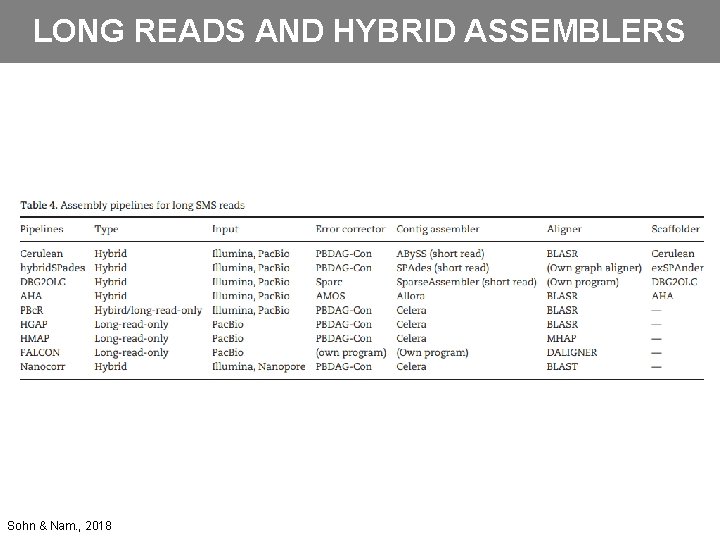
LONG READS AND HYBRID ASSEMBLERS Sohn & Nam. , 2018
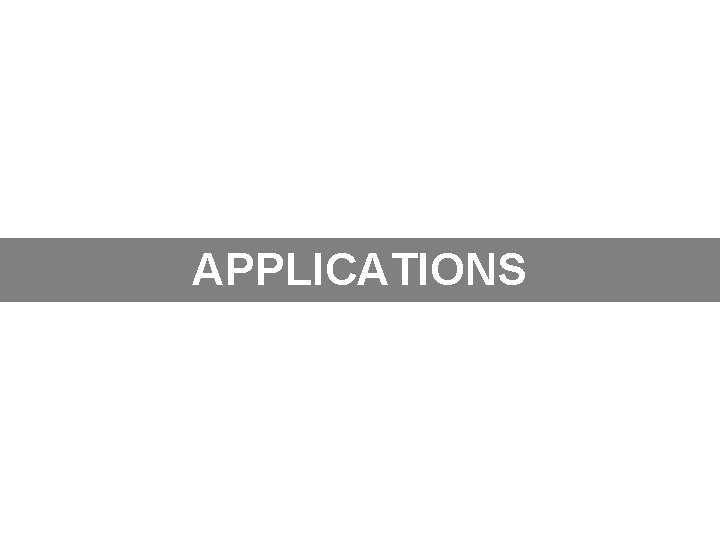
APPLICATIONS
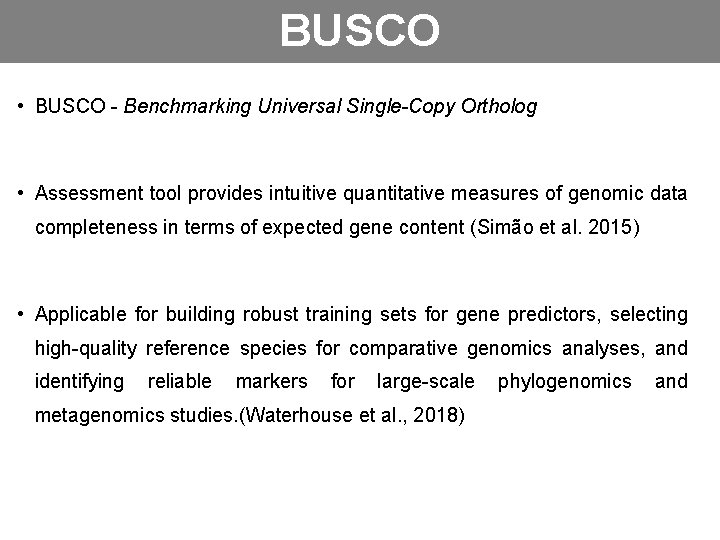
BUSCO • BUSCO - Benchmarking Universal Single-Copy Ortholog • Assessment tool provides intuitive quantitative measures of genomic data completeness in terms of expected gene content (Simão et al. 2015) • Applicable for building robust training sets for gene predictors, selecting high-quality reference species for comparative genomics analyses, and identifying reliable markers for large-scale phylogenomics and metagenomics studies. (Waterhouse et al. , 2018)
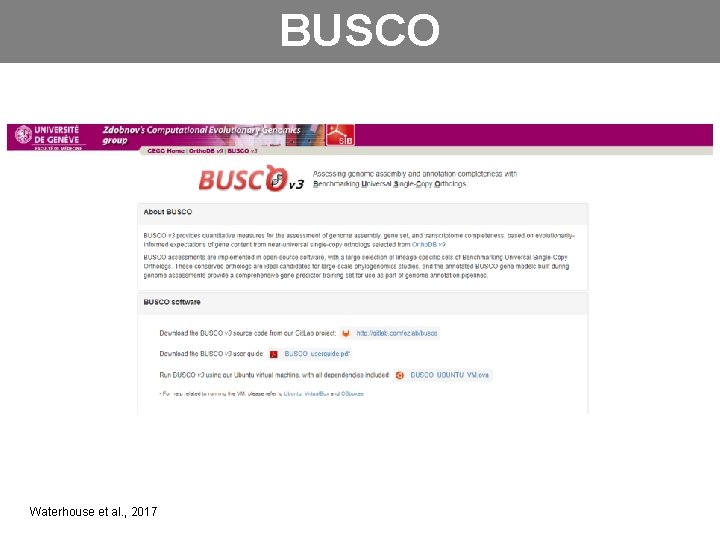
BUSCO Waterhouse et al. , 2017
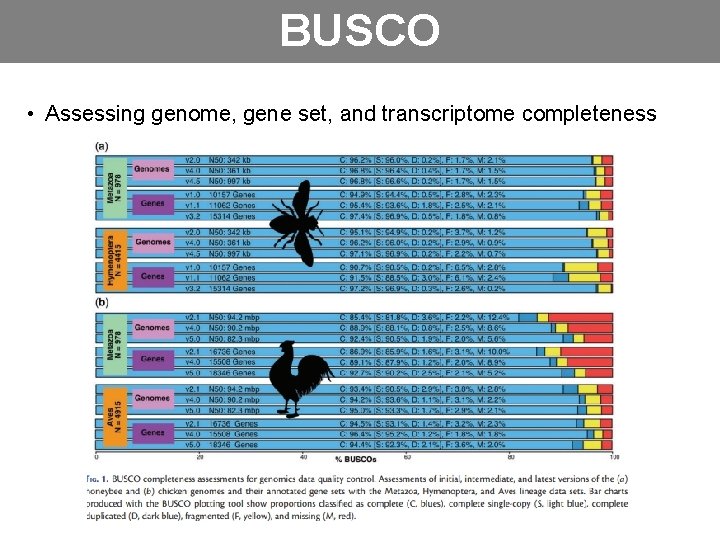
BUSCO • Assessing genome, gene set, and transcriptome completeness
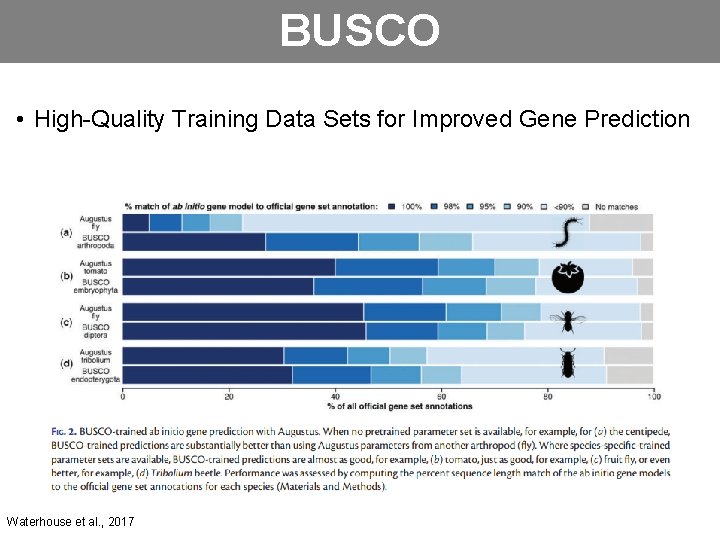
BUSCO • High-Quality Training Data Sets for Improved Gene Prediction Waterhouse et al. , 2017
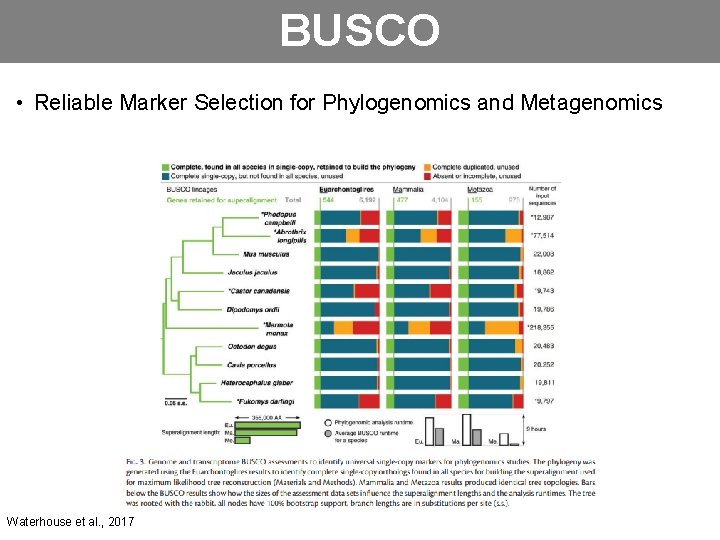
BUSCO • Reliable Marker Selection for Phylogenomics and Metagenomics Waterhouse et al. , 2017
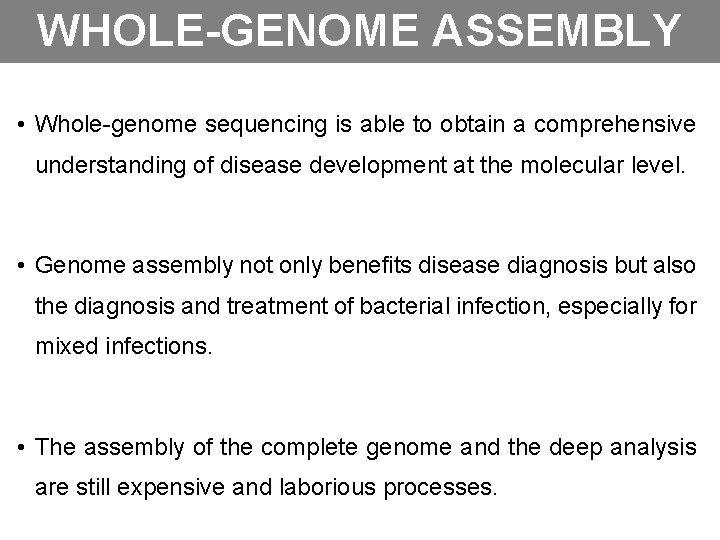
WHOLE-GENOME ASSEMBLY • Whole-genome sequencing is able to obtain a comprehensive understanding of disease development at the molecular level. • Genome assembly not only benefits disease diagnosis but also the diagnosis and treatment of bacterial infection, especially for mixed infections. • The assembly of the complete genome and the deep analysis are still expensive and laborious processes.
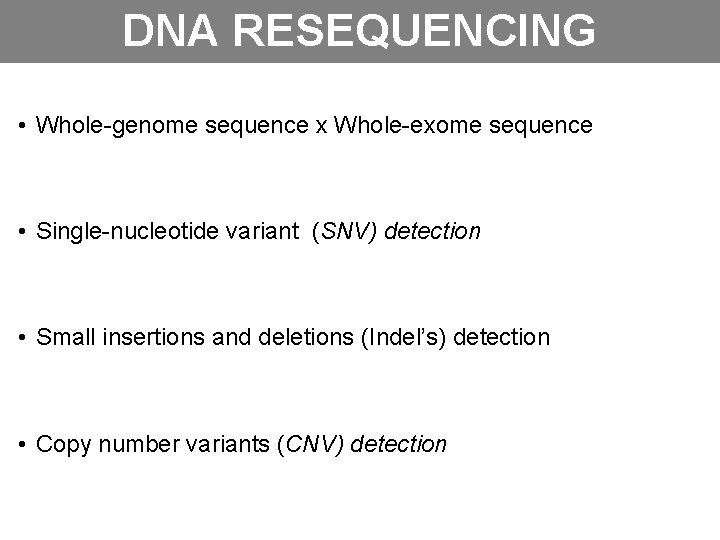
DNA RESEQUENCING • Whole-genome sequence x Whole-exome sequence • Single-nucleotide variant (SNV) detection • Small insertions and deletions (Indel’s) detection • Copy number variants (CNV) detection
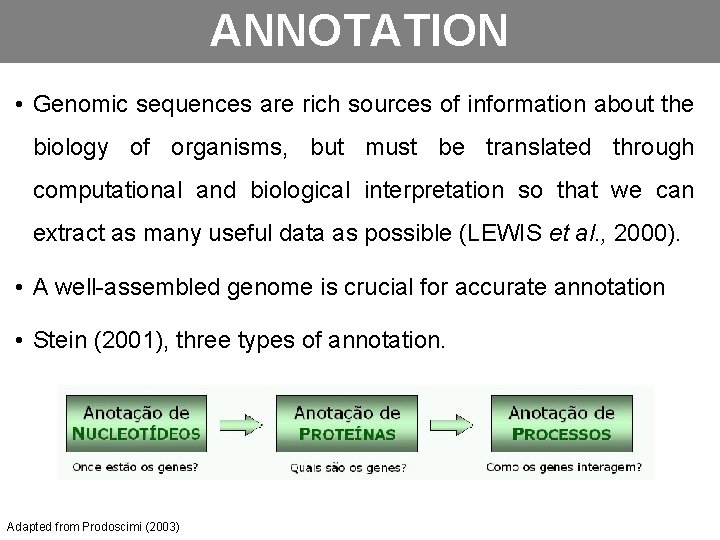
ANNOTATION • Genomic sequences are rich sources of information about the biology of organisms, but must be translated through computational and biological interpretation so that we can extract as many useful data as possible (LEWIS et al. , 2000). • A well-assembled genome is crucial for accurate annotation • Stein (2001), three types of annotation. Adapted from Prodoscimi (2003)
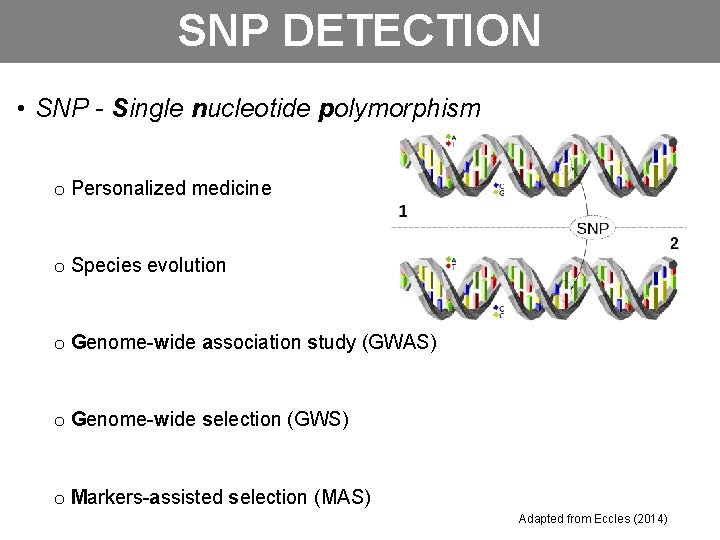
SNP DETECTION • SNP - Single nucleotide polymorphism o Personalized medicine o Species evolution o Genome-wide association study (GWAS) o Genome-wide selection (GWS) o Markers-assisted selection (MAS) Adapted from Eccles (2014)
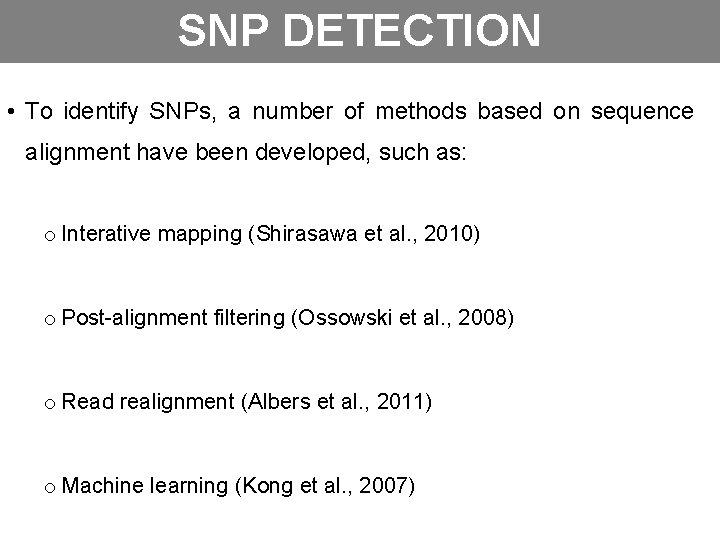
SNP DETECTION • To identify SNPs, a number of methods based on sequence alignment have been developed, such as: o Interative mapping (Shirasawa et al. , 2010) o Post-alignment filtering (Ossowski et al. , 2008) o Read realignment (Albers et al. , 2011) o Machine learning (Kong et al. , 2007)
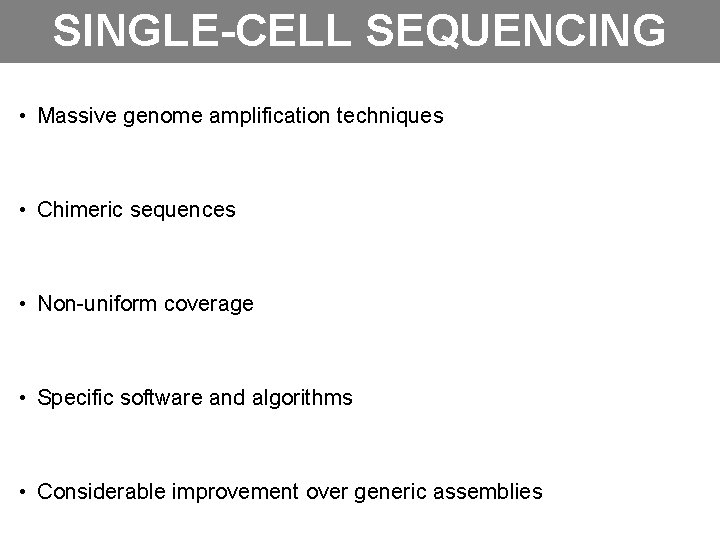
SINGLE-CELL SEQUENCING • Massive genome amplification techniques • Chimeric sequences • Non-uniform coverage • Specific software and algorithms • Considerable improvement over generic assemblies
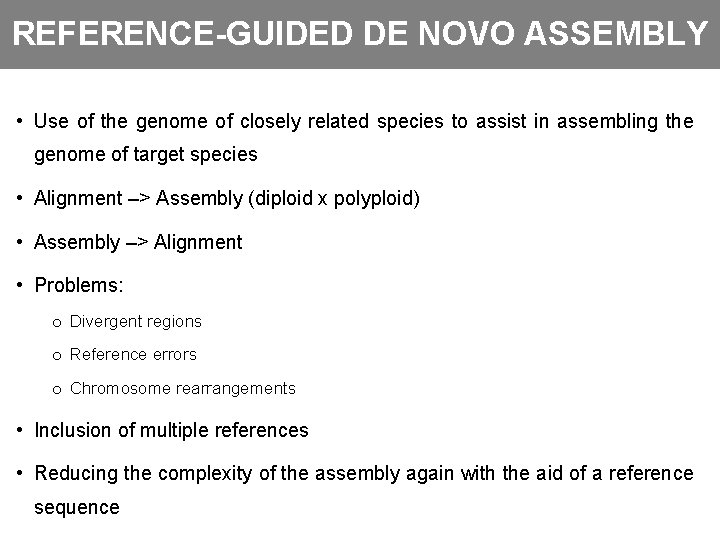
REFERENCE-GUIDED DE NOVO ASSEMBLY • Use of the genome of closely related species to assist in assembling the genome of target species • Alignment –> Assembly (diploid x polyploid) • Assembly –> Alignment • Problems: o Divergent regions o Reference errors o Chromosome rearrangements • Inclusion of multiple references • Reducing the complexity of the assembly again with the aid of a reference sequence
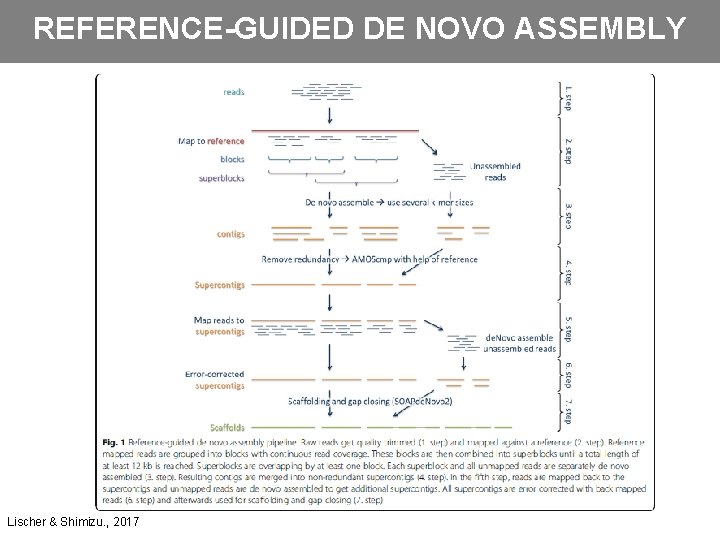
REFERENCE-GUIDED DE NOVO ASSEMBLY Lischer & Shimizu. , 2017
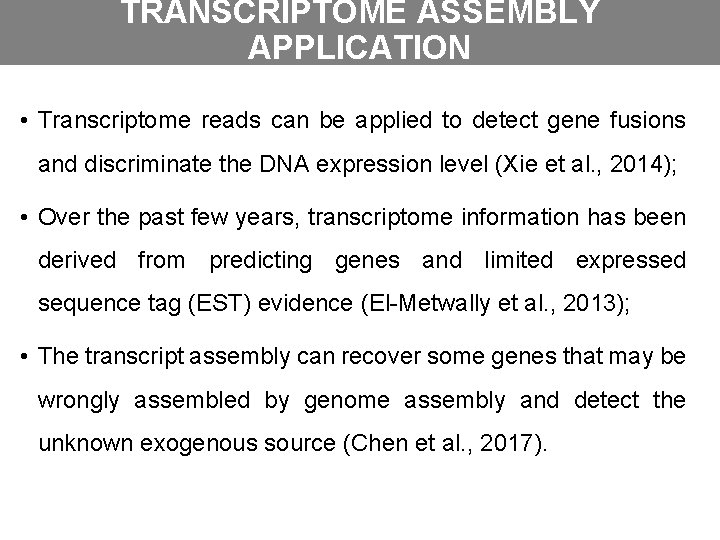
TRANSCRIPTOME ASSEMBLY APPLICATION • Transcriptome reads can be applied to detect gene fusions and discriminate the DNA expression level (Xie et al. , 2014); • Over the past few years, transcriptome information has been derived from predicting genes and limited expressed sequence tag (EST) evidence (El-Metwally et al. , 2013); • The transcript assembly can recover some genes that may be wrongly assembled by genome assembly and detect the unknown exogenous source (Chen et al. , 2017).
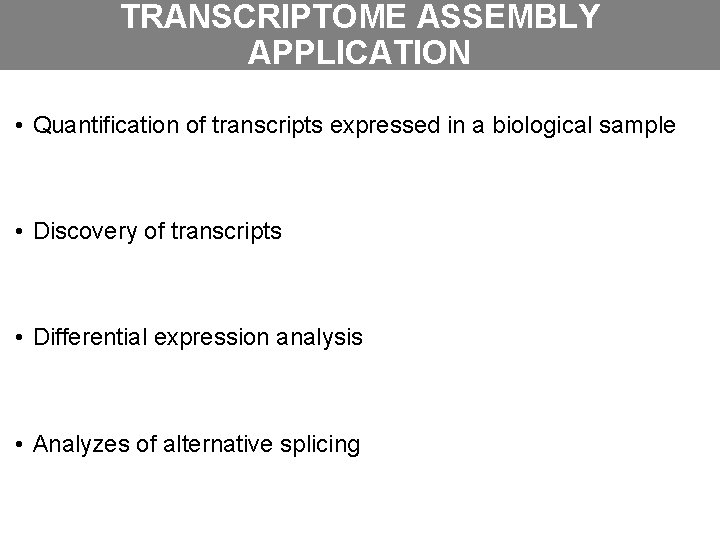
TRANSCRIPTOME ASSEMBLY APPLICATION • Quantification of transcripts expressed in a biological sample • Discovery of transcripts • Differential expression analysis • Analyzes of alternative splicing
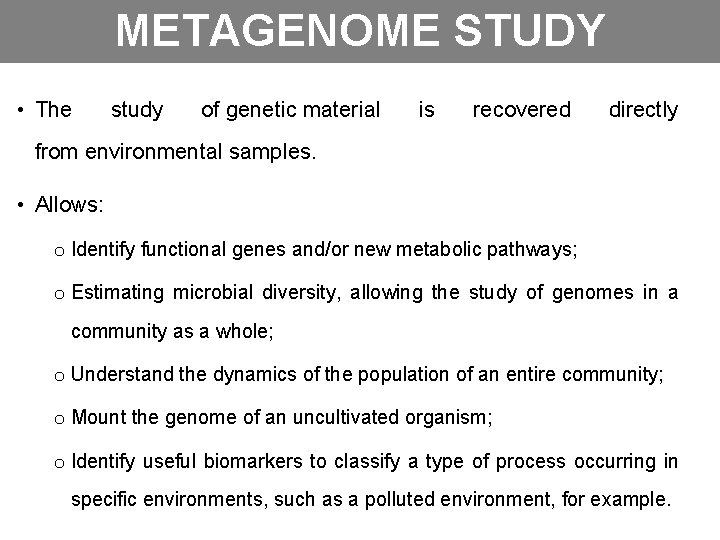
METAGENOME STUDY • The study of genetic material is recovered directly from environmental samples. • Allows: o Identify functional genes and/or new metabolic pathways; o Estimating microbial diversity, allowing the study of genomes in a community as a whole; o Understand the dynamics of the population of an entire community; o Mount the genome of an uncultivated organism; o Identify useful biomarkers to classify a type of process occurring in specific environments, such as a polluted environment, for example.
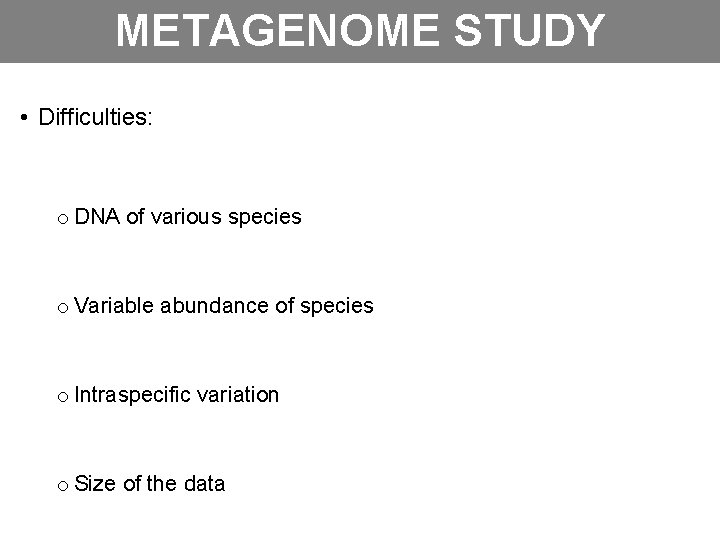
METAGENOME STUDY • Difficulties: o DNA of various species o Variable abundance of species o Intraspecific variation o Size of the data
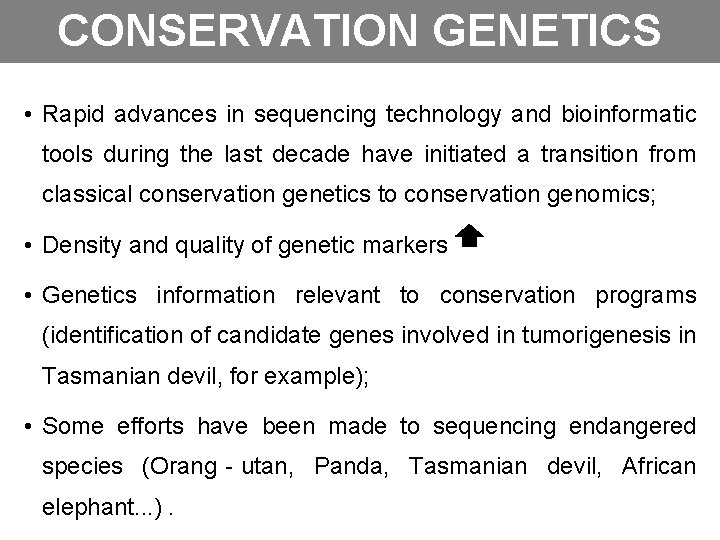
CONSERVATION GENETICS • Rapid advances in sequencing technology and bioinformatic tools during the last decade have initiated a transition from classical conservation genetics to conservation genomics; • Density and quality of genetic markers • Genetics information relevant to conservation programs (identification of candidate genes involved in tumorigenesis in Tasmanian devil, for example); • Some efforts have been made to sequencing endangered species (Orang‐utan, Panda, Tasmanian devil, African elephant. . . ).
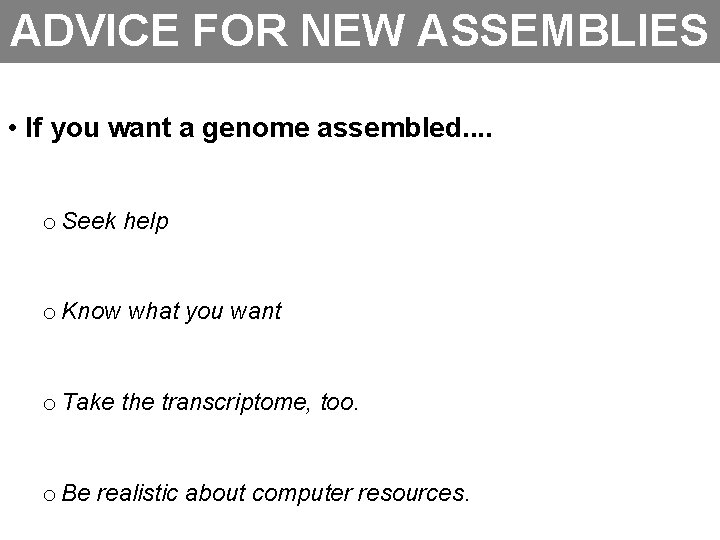
ADVICE FOR NEW ASSEMBLIES • If you want a genome assembled. . o Seek help o Know what you want o Take the transcriptome, too. o Be realistic about computer resources.
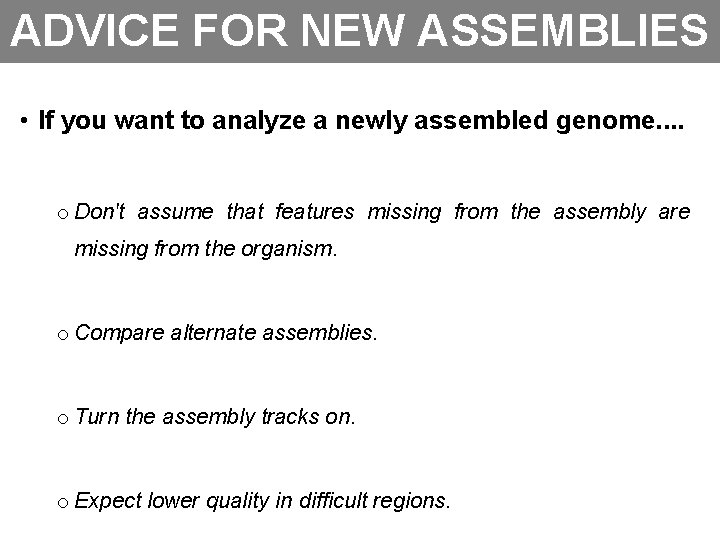
ADVICE FOR NEW ASSEMBLIES • If you want to analyze a newly assembled genome. . o Don't assume that features missing from the assembly are missing from the organism. o Compare alternate assemblies. o Turn the assembly tracks on. o Expect lower quality in difficult regions.
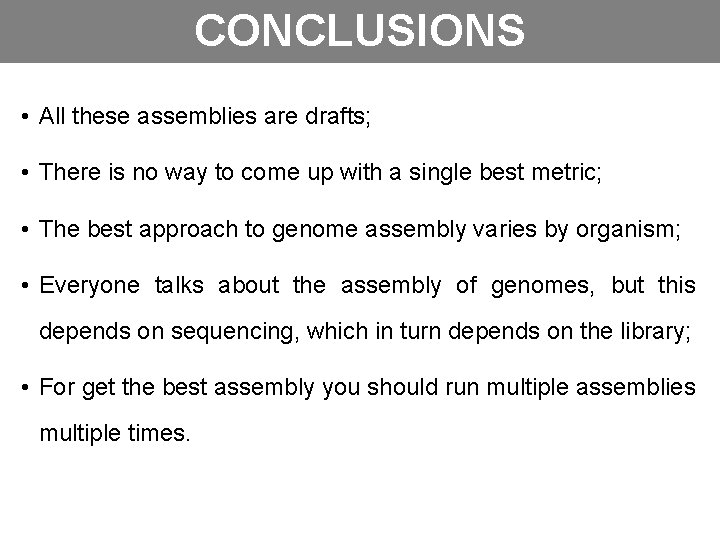
CONCLUSIONS • All these assemblies are drafts; • There is no way to come up with a single best metric; • The best approach to genome assembly varies by organism; • Everyone talks about the assembly of genomes, but this depends on sequencing, which in turn depends on the library; • For get the best assembly you should run multiple assemblies multiple times.
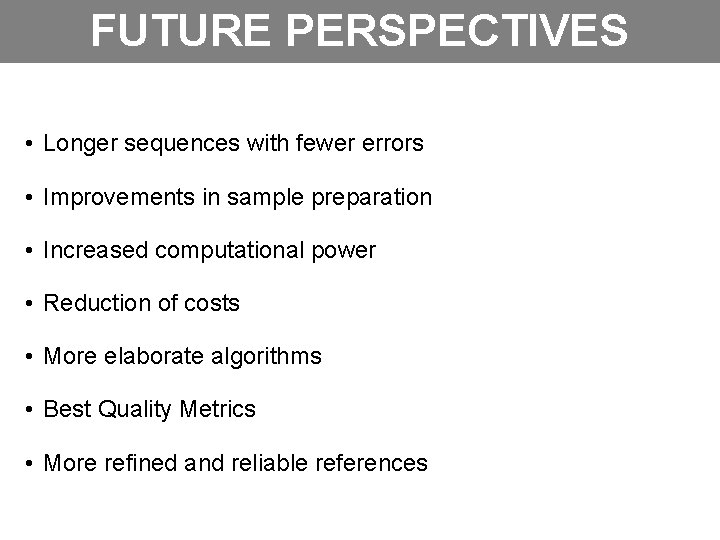
FUTURE PERSPECTIVES • Longer sequences with fewer errors • Improvements in sample preparation • Increased computational power • Reduction of costs • More elaborate algorithms • Best Quality Metrics • More refined and reliable references
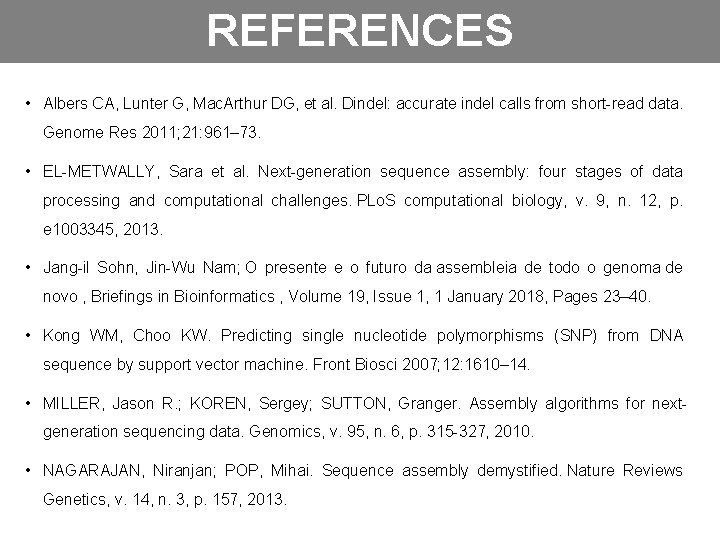
REFERENCES • Albers CA, Lunter G, Mac. Arthur DG, et al. Dindel: accurate indel calls from short-read data. Genome Res 2011; 21: 961– 73. • EL-METWALLY, Sara et al. Next-generation sequence assembly: four stages of data processing and computational challenges. PLo. S computational biology, v. 9, n. 12, p. e 1003345, 2013. • Jang-il Sohn, Jin-Wu Nam; O presente e o futuro da assembleia de todo o genoma de novo , Briefings in Bioinformatics , Volume 19, Issue 1, 1 January 2018, Pages 23– 40. • Kong WM, Choo KW. Predicting single nucleotide polymorphisms (SNP) from DNA sequence by support vector machine. Front Biosci 2007; 12: 1610– 14. • MILLER, Jason R. ; KOREN, Sergey; SUTTON, Granger. Assembly algorithms for nextgeneration sequencing data. Genomics, v. 95, n. 6, p. 315 -327, 2010. • NAGARAJAN, Niranjan; POP, Mihai. Sequence assembly demystified. Nature Reviews Genetics, v. 14, n. 3, p. 157, 2013.
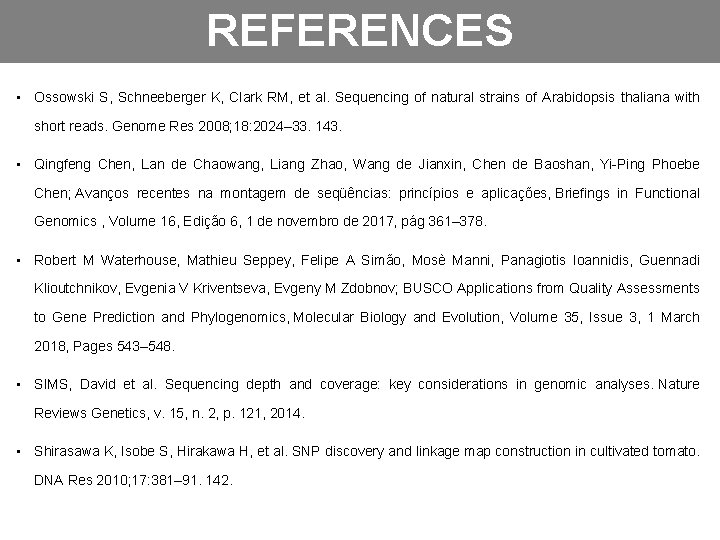
REFERENCES • Ossowski S, Schneeberger K, Clark RM, et al. Sequencing of natural strains of Arabidopsis thaliana with short reads. Genome Res 2008; 18: 2024– 33. 143. • Qingfeng Chen, Lan de Chaowang, Liang Zhao, Wang de Jianxin, Chen de Baoshan, Yi-Ping Phoebe Chen; Avanços recentes na montagem de seqüências: princípios e aplicações, Briefings in Functional Genomics , Volume 16, Edição 6, 1 de novembro de 2017, pág 361– 378. • Robert M Waterhouse, Mathieu Seppey, Felipe A Simão, Mosè Manni, Panagiotis Ioannidis, Guennadi Klioutchnikov, Evgenia V Kriventseva, Evgeny M Zdobnov; BUSCO Applications from Quality Assessments to Gene Prediction and Phylogenomics, Molecular Biology and Evolution, Volume 35, Issue 3, 1 March 2018, Pages 543– 548. • SIMS, David et al. Sequencing depth and coverage: key considerations in genomic analyses. Nature Reviews Genetics, v. 15, n. 2, p. 121, 2014. • Shirasawa K, Isobe S, Hirakawa H, et al. SNP discovery and linkage map construction in cultivated tomato. DNA Res 2010; 17: 381– 91. 142.
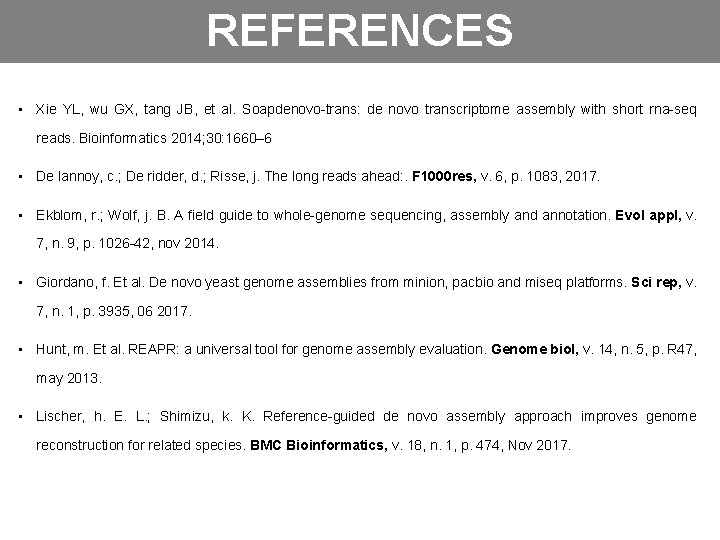
REFERENCES • Xie YL, wu GX, tang JB, et al. Soapdenovo-trans: de novo transcriptome assembly with short rna-seq reads. Bioinformatics 2014; 30: 1660– 6 • De lannoy, c. ; De ridder, d. ; Risse, j. The long reads ahead: . F 1000 res, v. 6, p. 1083, 2017. • Ekblom, r. ; Wolf, j. B. A field guide to whole-genome sequencing, assembly and annotation. Evol appl, v. 7, n. 9, p. 1026 -42, nov 2014. • Giordano, f. Et al. De novo yeast genome assemblies from minion, pacbio and miseq platforms. Sci rep, v. 7, n. 1, p. 3935, 06 2017. • Hunt, m. Et al. REAPR: a universal tool for genome assembly evaluation. Genome biol, v. 14, n. 5, p. R 47, may 2013. • Lischer, h. E. L. ; Shimizu, k. K. Reference-guided de novo assembly approach improves genome reconstruction for related species. BMC Bioinformatics, v. 18, n. 1, p. 474, Nov 2017.
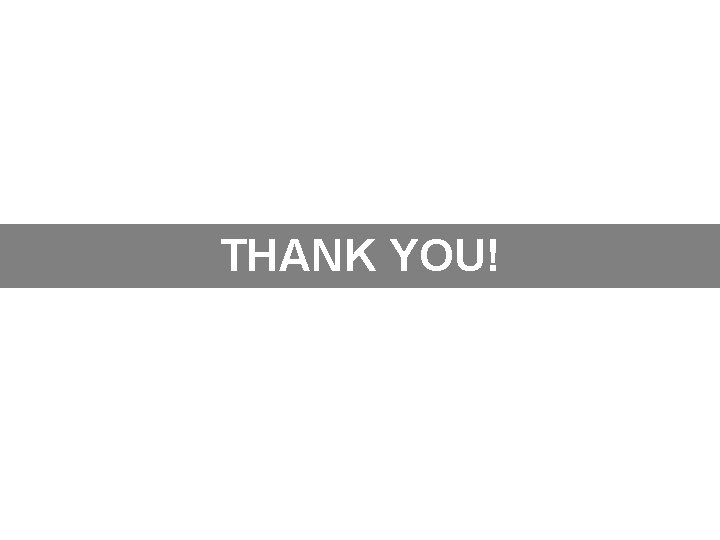
THANK YOU!
Universidade federal campus osasco
Universidade castelo branco centro
Universidade castelo branco centro
Centro regional de educación superior paulo freire
Lesson 15 nuclear quest nuclear reactions
Fisión nuclear vs fision nuclear
Energia nuclear
Como funciona la energia nuclear
Energia nuclear concepto
Cz norte centro historico
Centro de massa
Classificação de compressores
Circuito elettrico scuola primaria
Conservarea energiei
Universidade federal do rio grande do norte
Universidade federal do rio grande do norte
Universidade federal do amazonas medicina
"prof universidade paulista unip"
Unisesi
Mulheres dominantes
Universidade estadual paulista subsidiárias
Universidade cvc
Catolica lisbon moodle
Angiogênese
Universidade federal de santa catarina
"prof universidade paulista unip"
Reologia
"universidade paulista unip instituto"
Universidade federal de santa catarina brazil
Universidade federal de santa catarina
Biblivre
Unip engenharia quimica
Universidade federal do amazonas
Universidade de newcastle
Univap - universidade do vale do paraíba
Universidade federal de santa catarina
Texto-base adaptada universidade federal de alagoas ufal
"prof universidade paulista unip"
Paulo de tarso
Leitura da carta de são paulo aos filipenses 4 4-9
Paulo freire
Paulo sancho
Cerrando circulos paulo coelho libro
Paulo roberto gaefke
Paulo roberto gaefke wikipédia
Dieci cose che ho imparato dalla vita
Paulo sentelhas e angelocci geadas aula lce 306
Raquel julia rita carolina fernando paulo
Paulo freire nasceu em recife em 1921
Sonhar com onça preta
Paulo salgado idade
Personalidade de paulo
Blog jakes paulo
Alfabetización paulo freire
Pensamento pedagógico de paulo freire
Paulo cabello
Certo dia o professor paulo
Paulo sergio valente amor dos santos
Paulo guimares
Conjunto sao paulo
Paulo frayer
Encerrando ciclos
Imagen paulo freire
Sao paulo futmax
Morte dos apostolos
Pestlee
Slidetodoc.com
Hino da caridade de são paulo
"hospital são paulo"
Primeira viagem missionária de paulo e barnabé
Es imposible enseñar sin ese coraje
Paulo reglus neves freire
Paulo roberto gaefke wikipédia
Paulo sancho
Meteorologia cesar
El comedor paulo souza
Un bonito cuento de paulo coelho
Paulo coelho prayer
Paulo freire mural
Detectación
Encerrando ciclos paulo coelho
Enem para realizar a viagem dos sonhos
Casa butanta interior
Epstola
Primeira viagem missionária de paulo
Paulo coelho knygos
Lucije emilije paulo
Paulo ivo cortez de araujo
Lançam-se dois dados honestos com faces numeradas de 1 a 6
Paulo frayer
Marcus porcius cato censorius maior
Etapas de paulo coelho
Paulo leitao
Paulo ferrinho
Un bonito cuento
Terceira viagem de paulo
Carta de são paulo aos coríntios sobre o amor
Paulo frayer
Alkeemik paulo coelho
Paulo roberto gaefke
Paulo freire biography
Colegio estadual paulo leminski
Paulo cesar sentelhas
Cuento de paulo coelho
Poema josé paulo paes