The ChiSquare test Assistant prof Dr Mayasah A
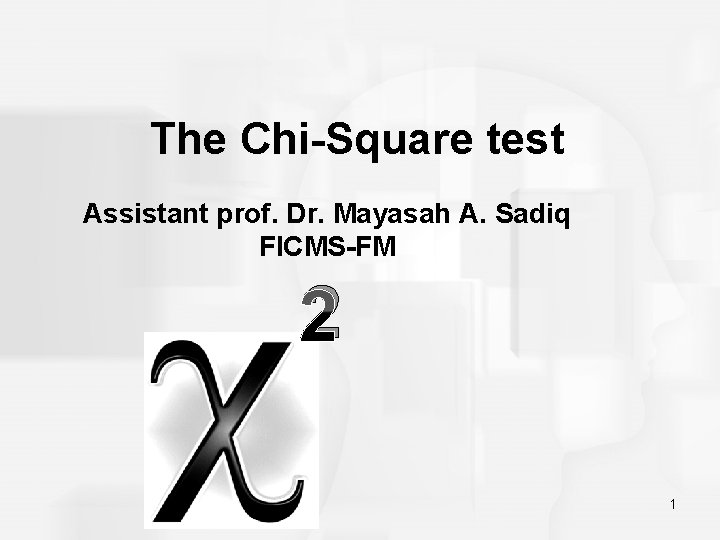
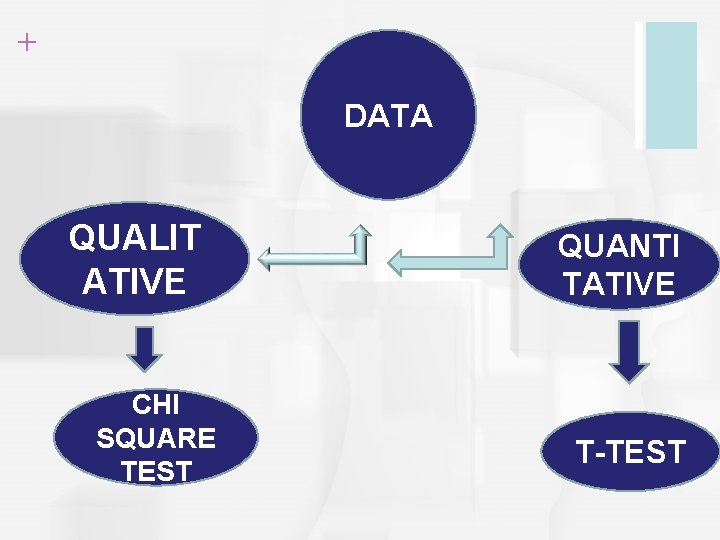
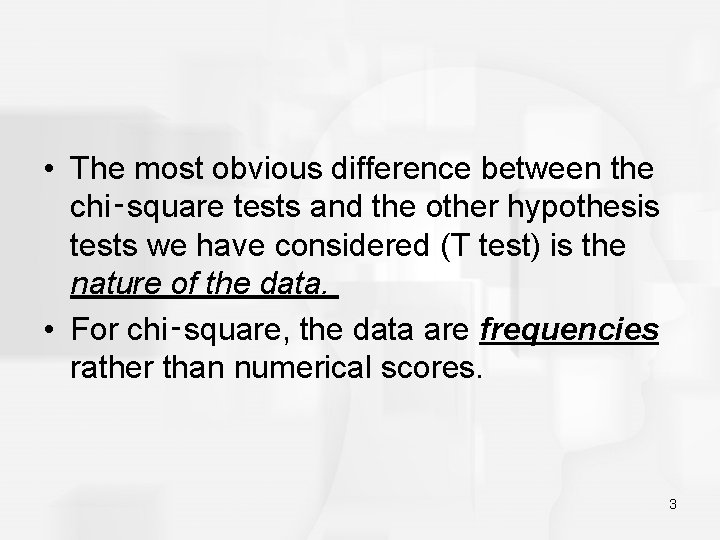
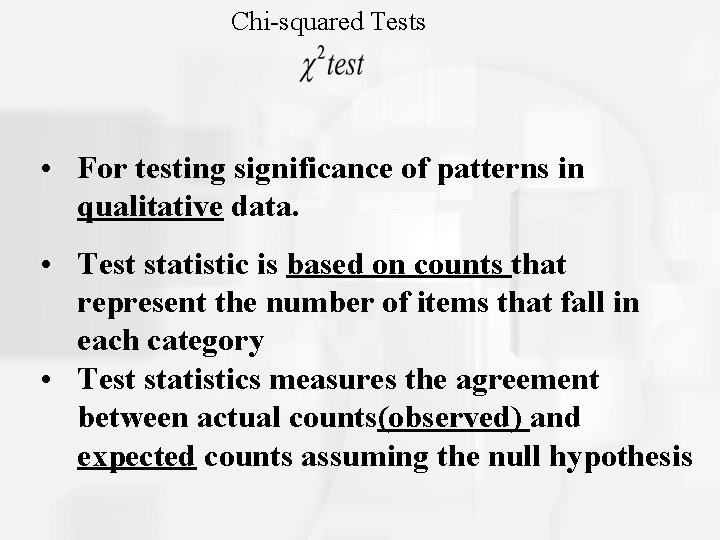
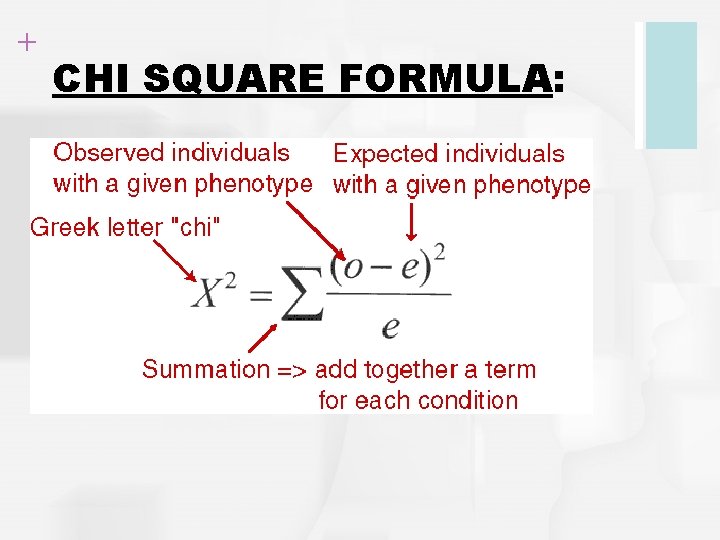
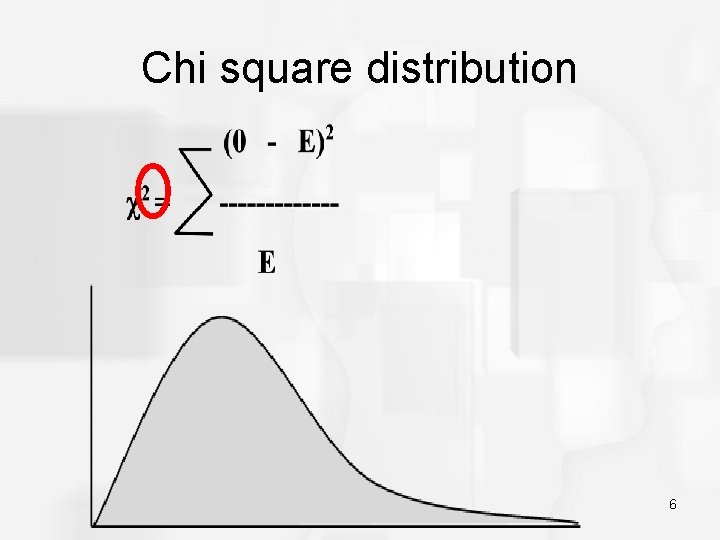
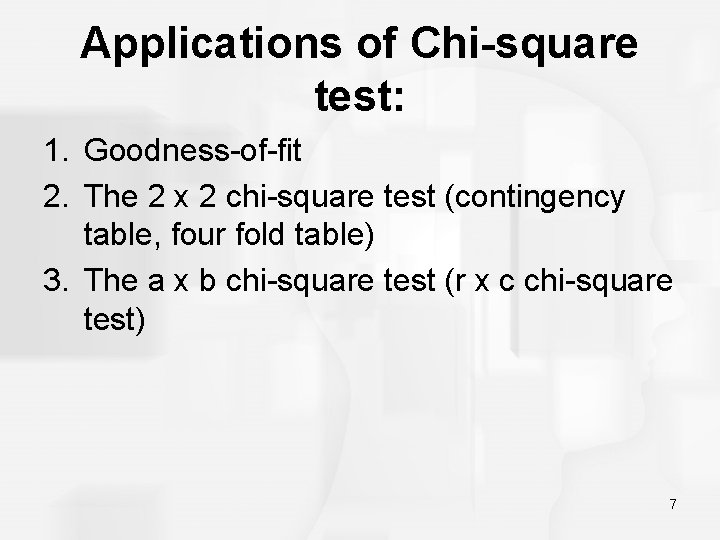
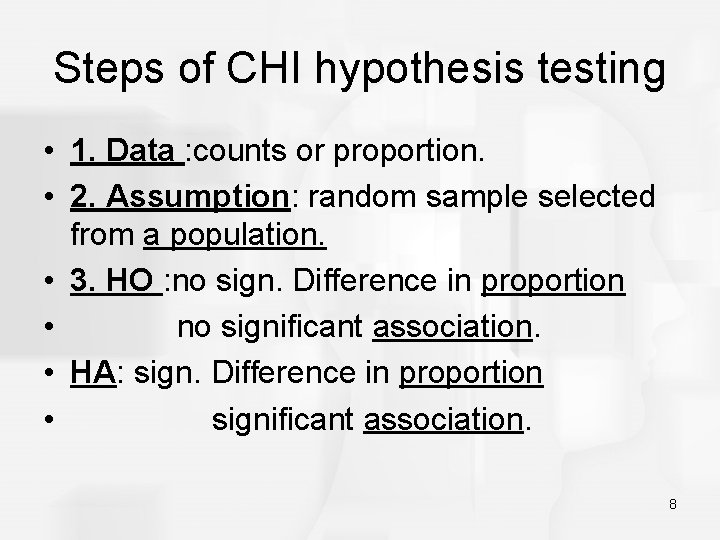
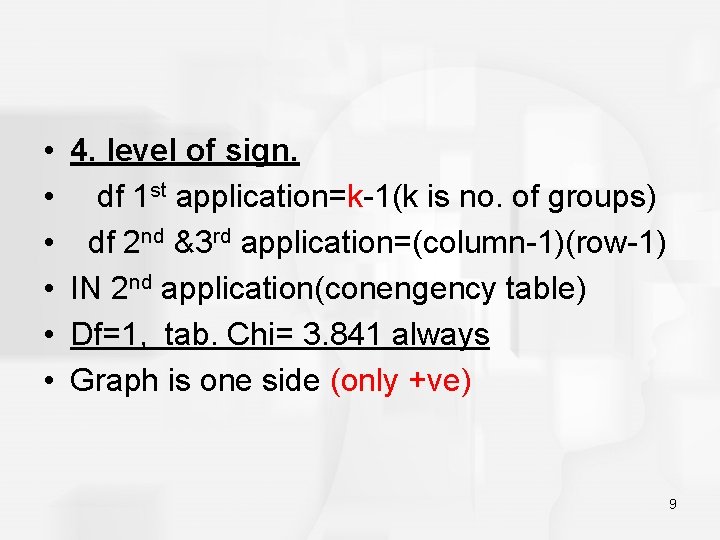
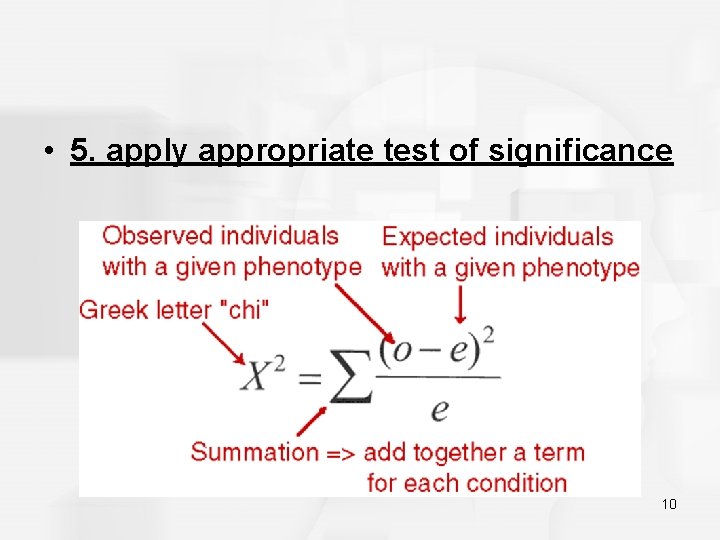
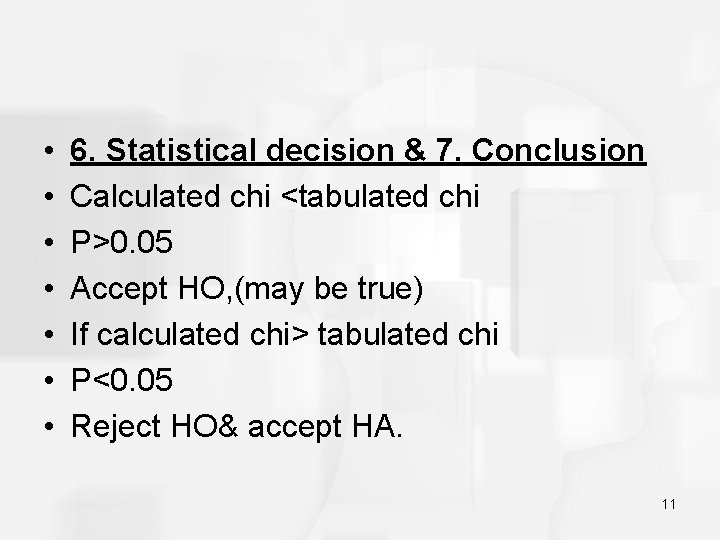
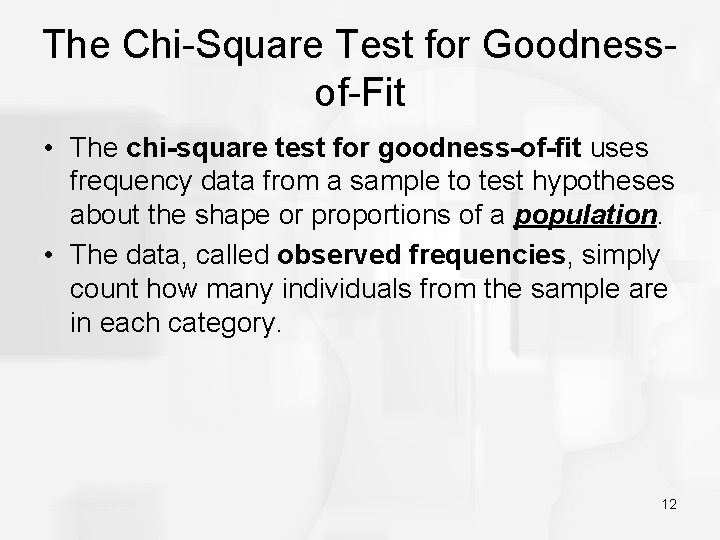
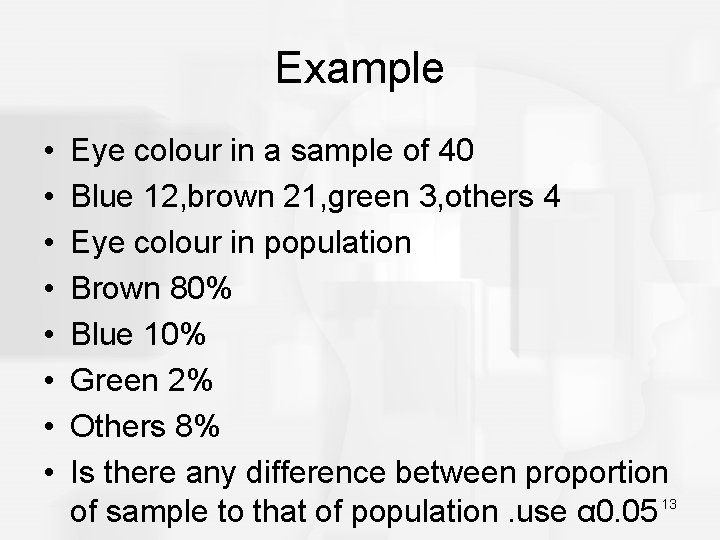
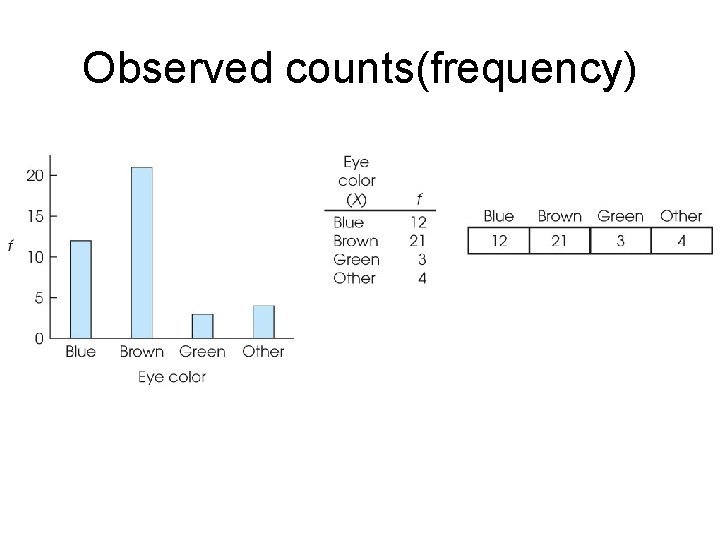
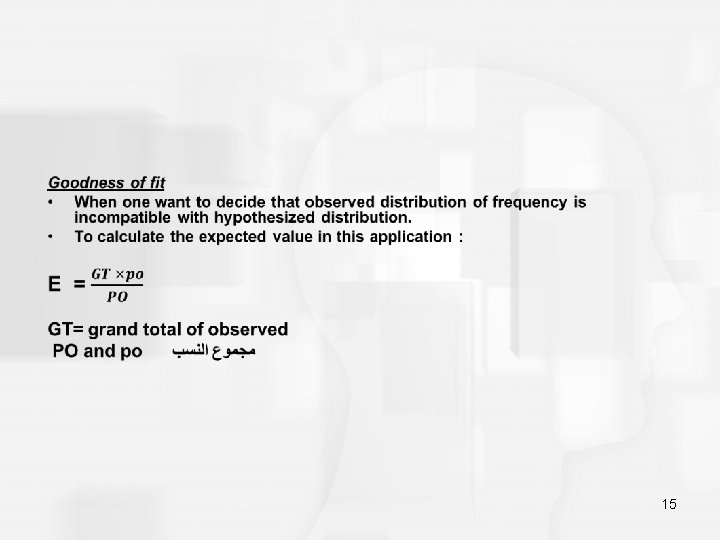
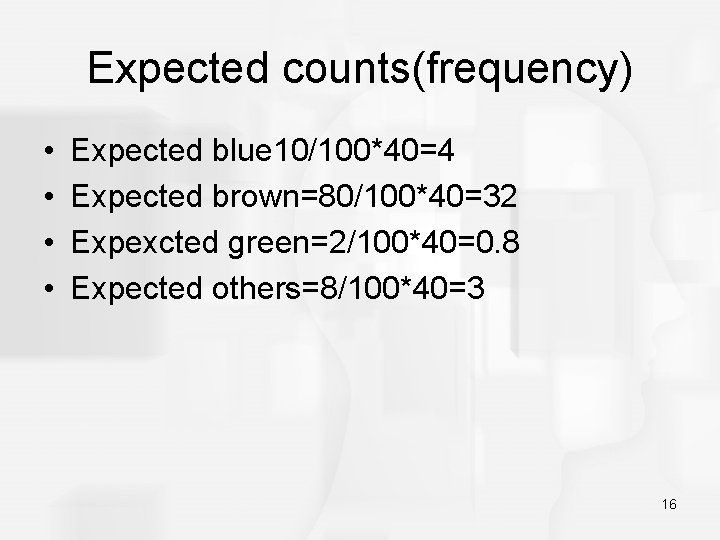
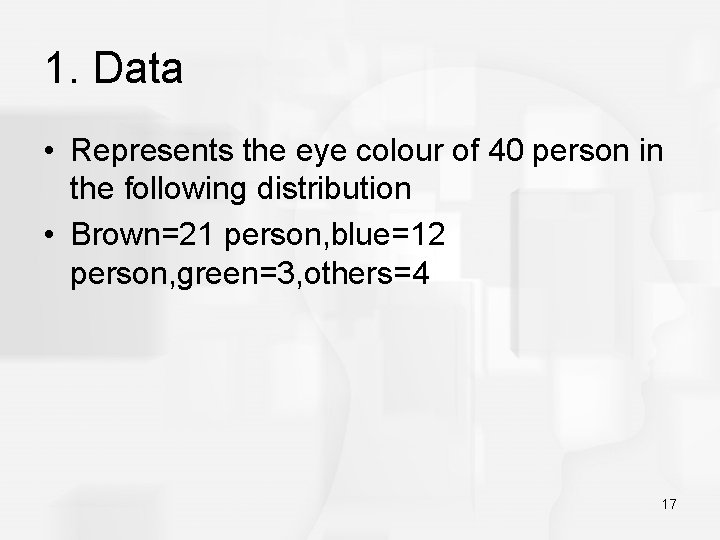
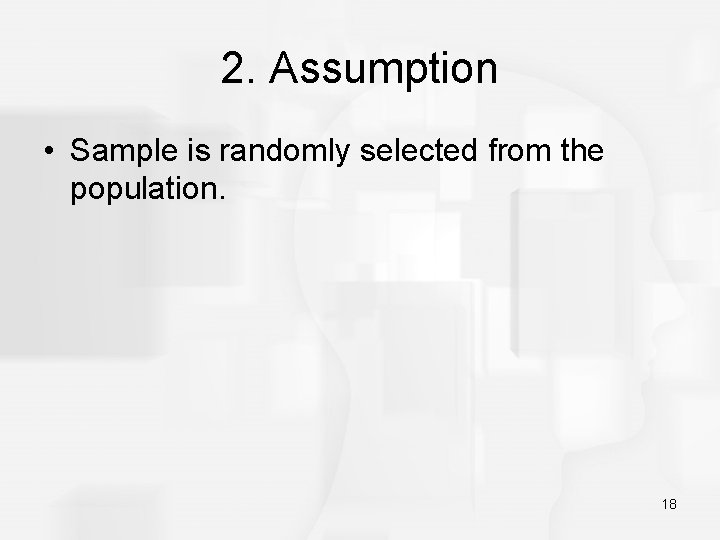
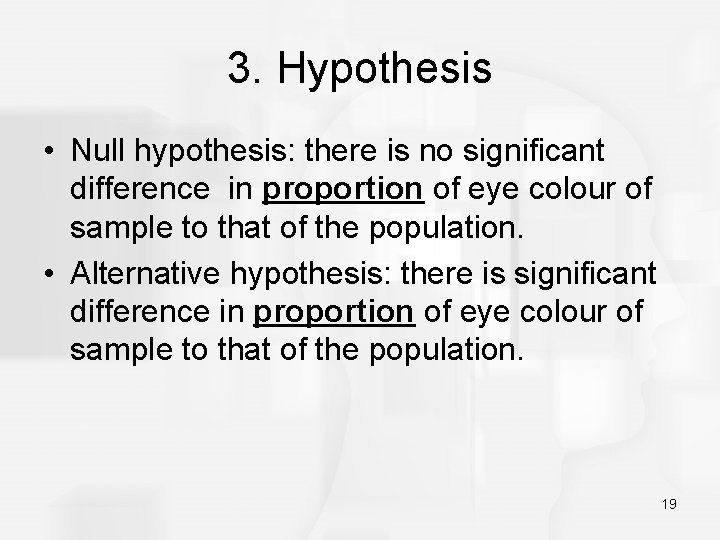
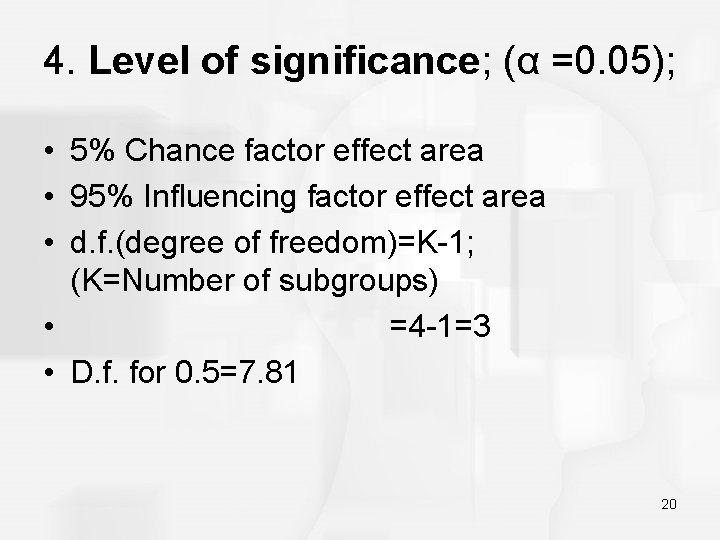
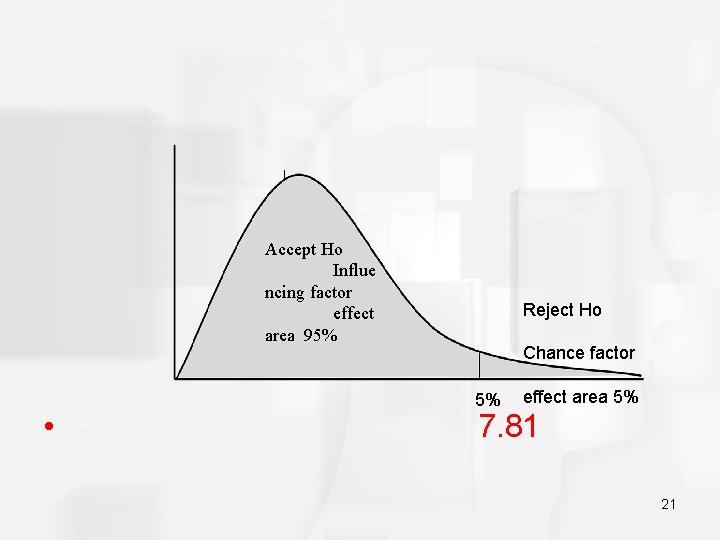
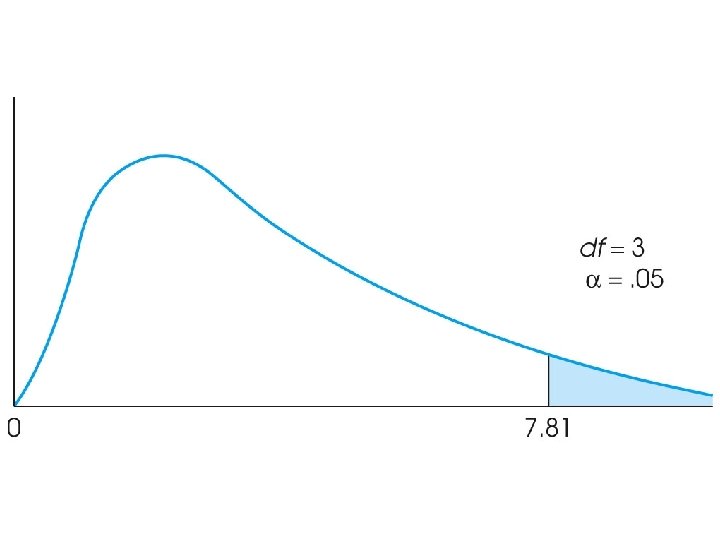
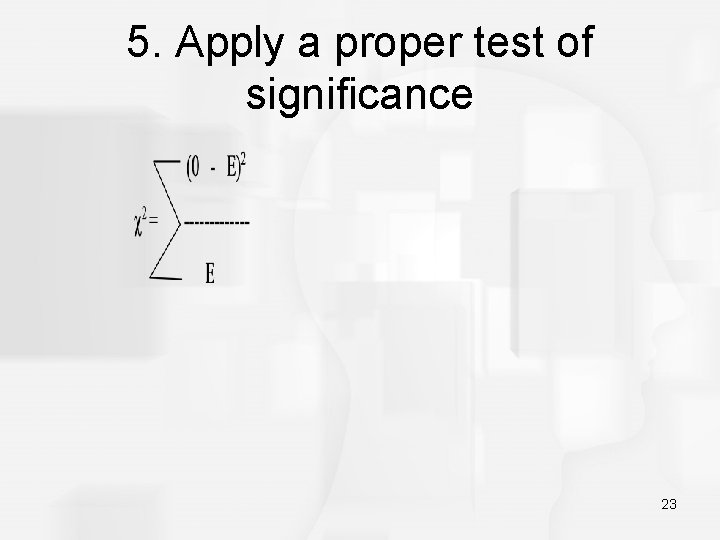
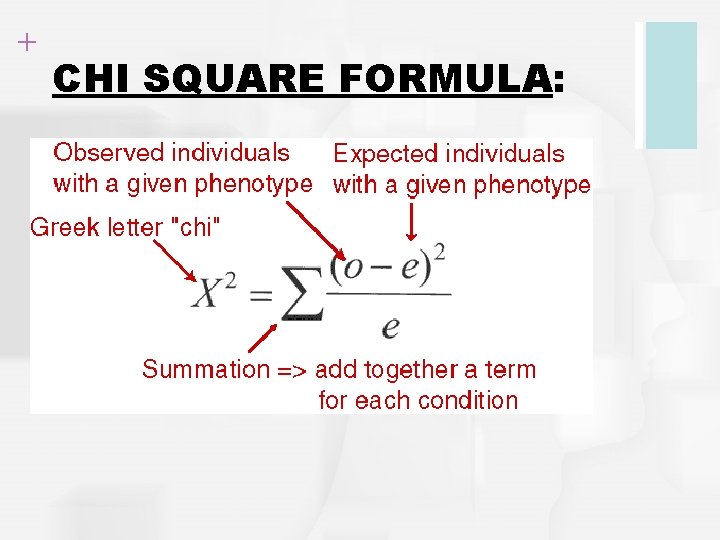
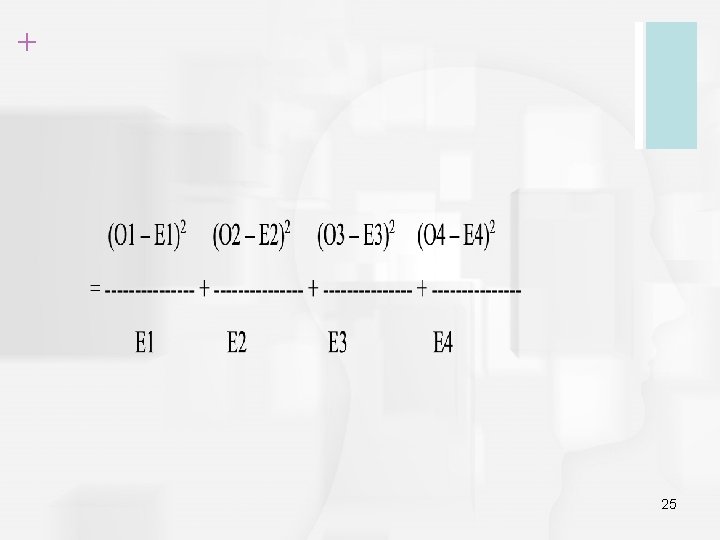
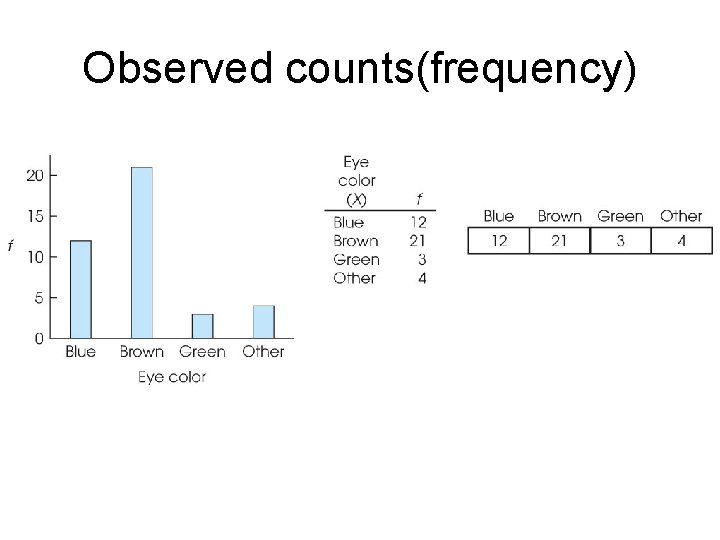
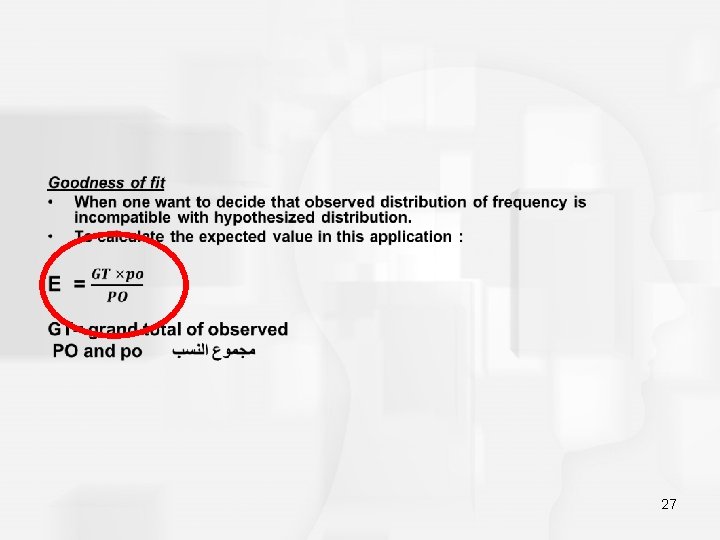
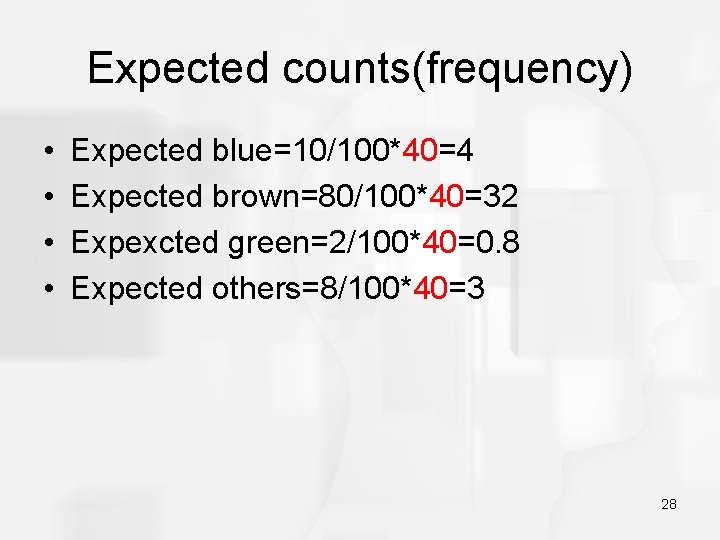
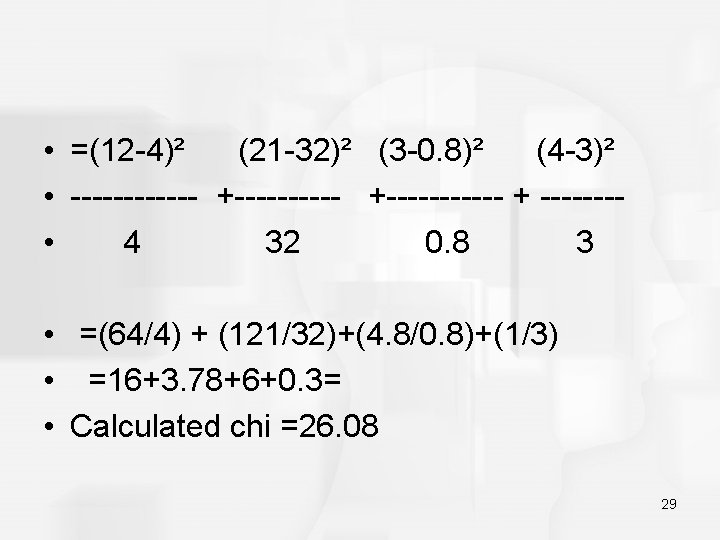
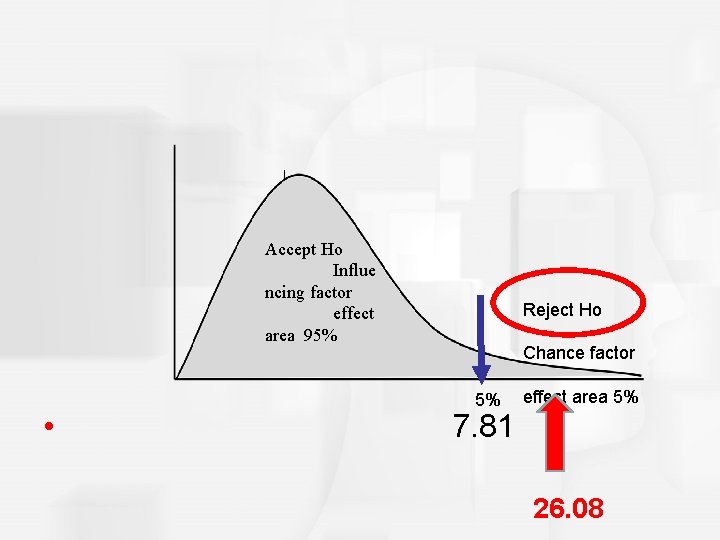
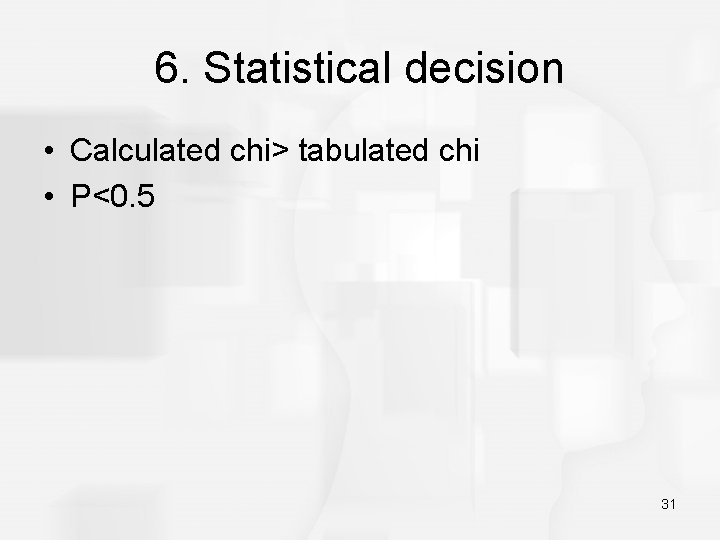
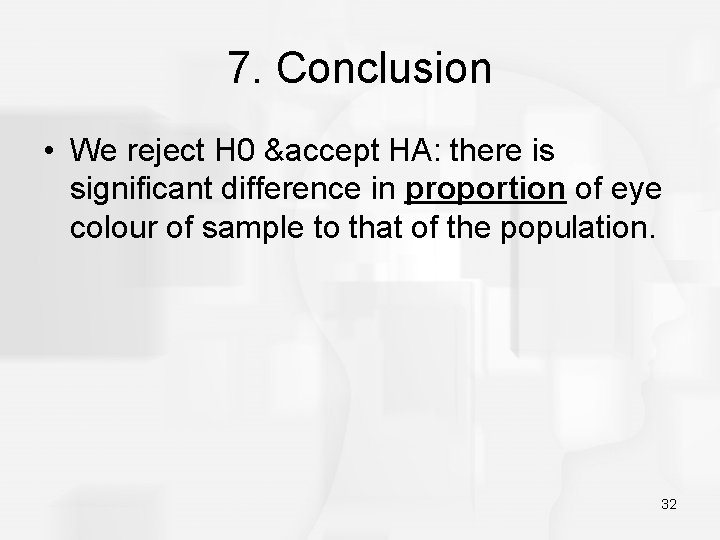
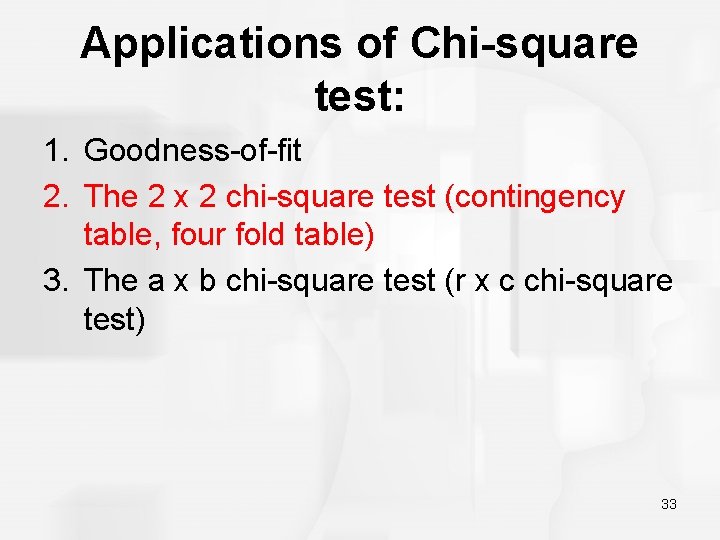
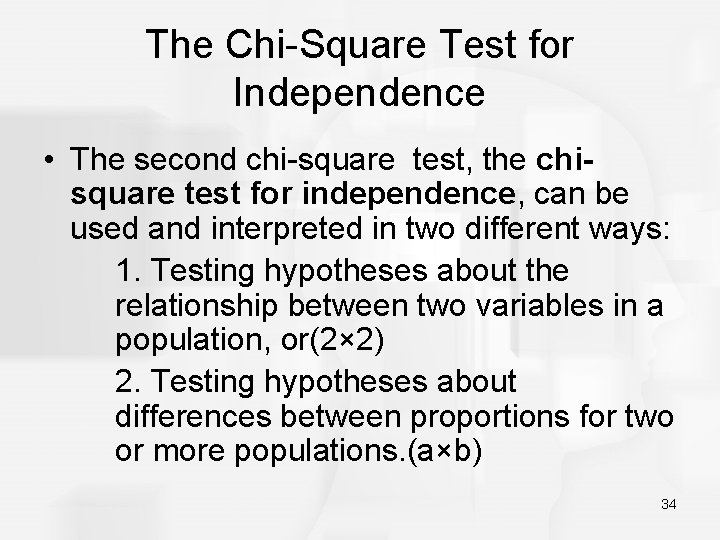
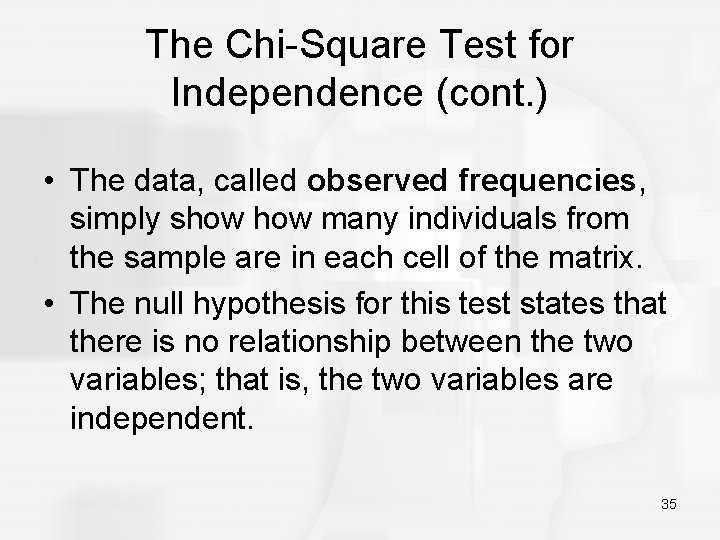
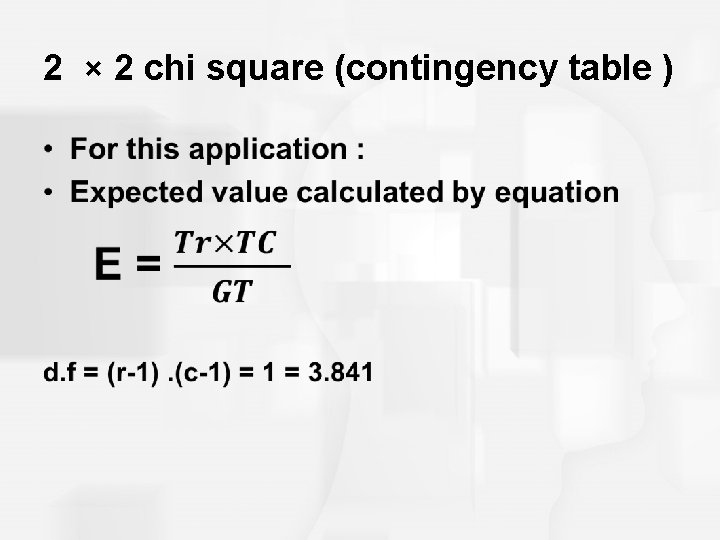
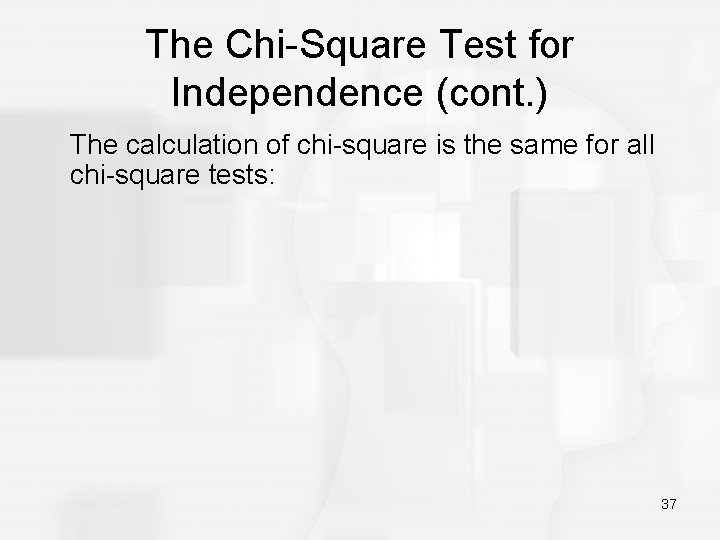
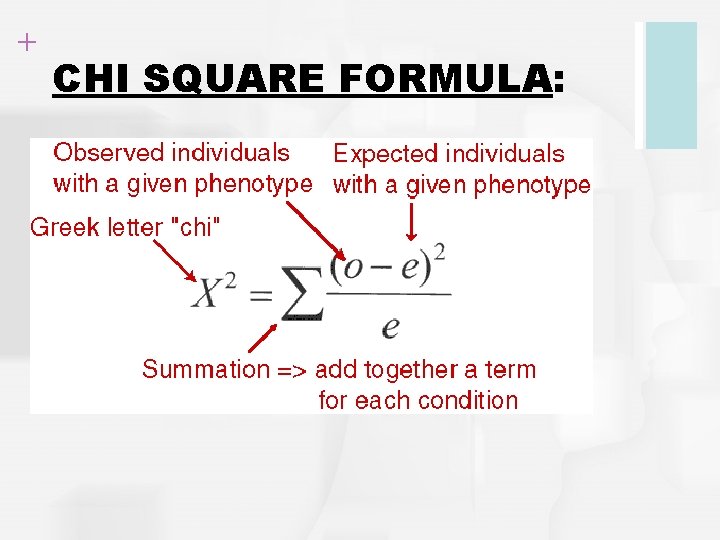
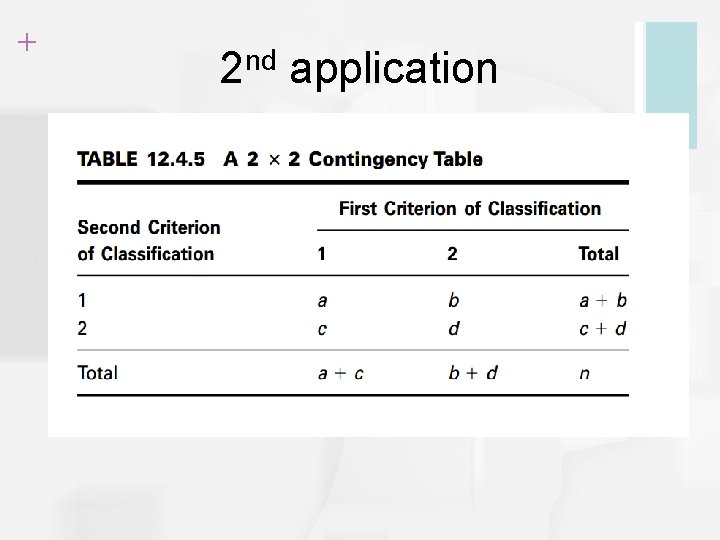
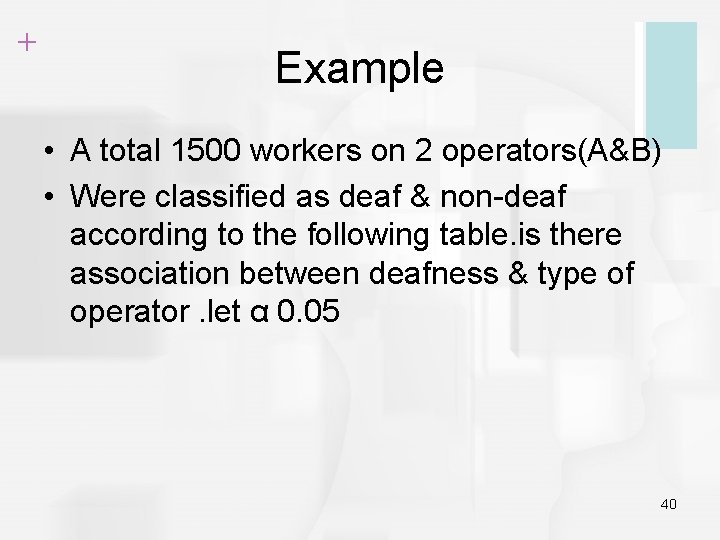
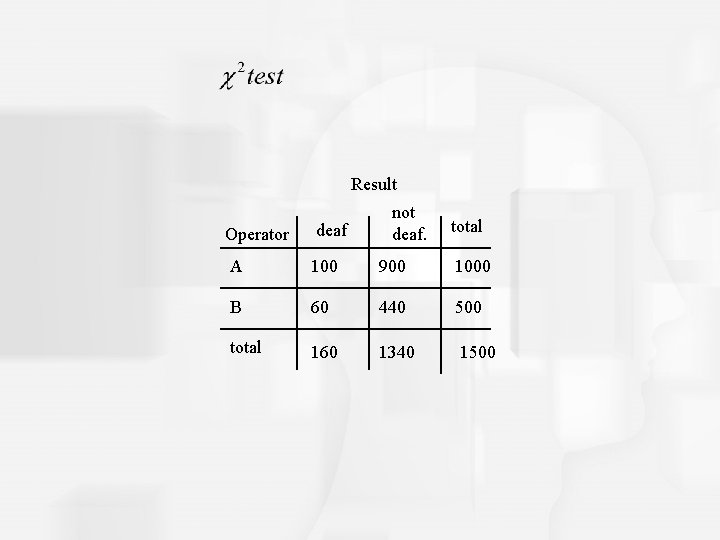
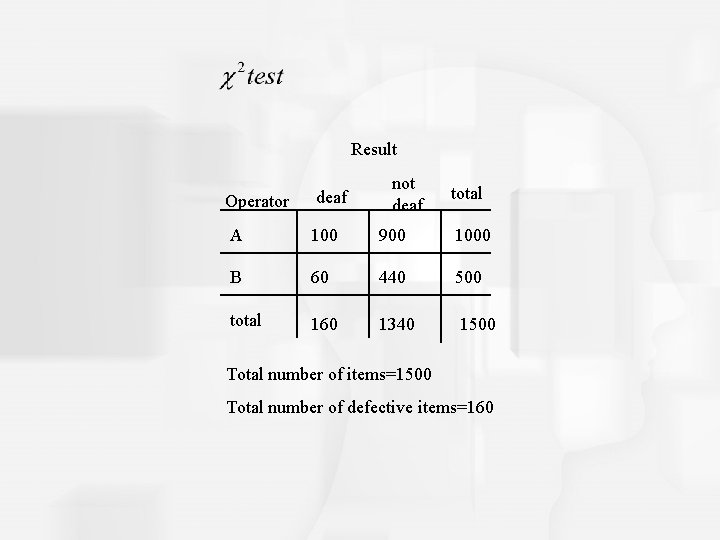
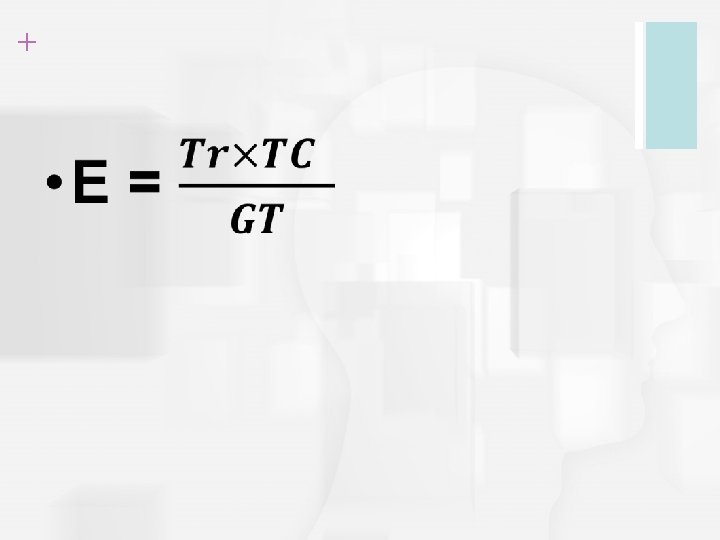
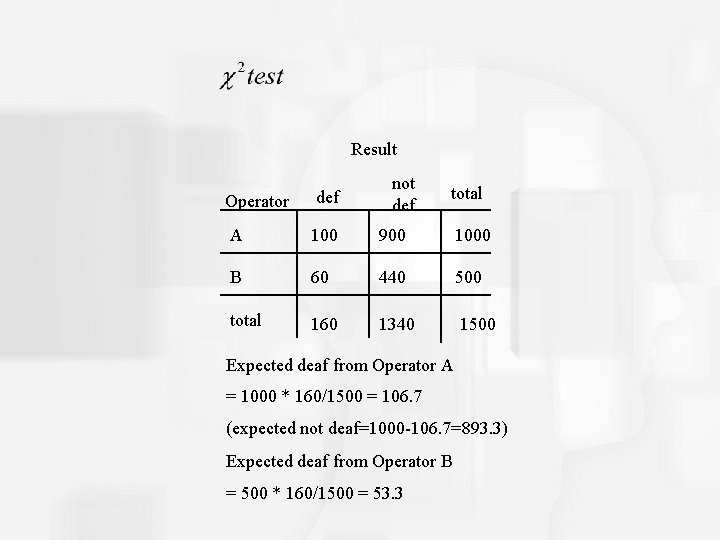
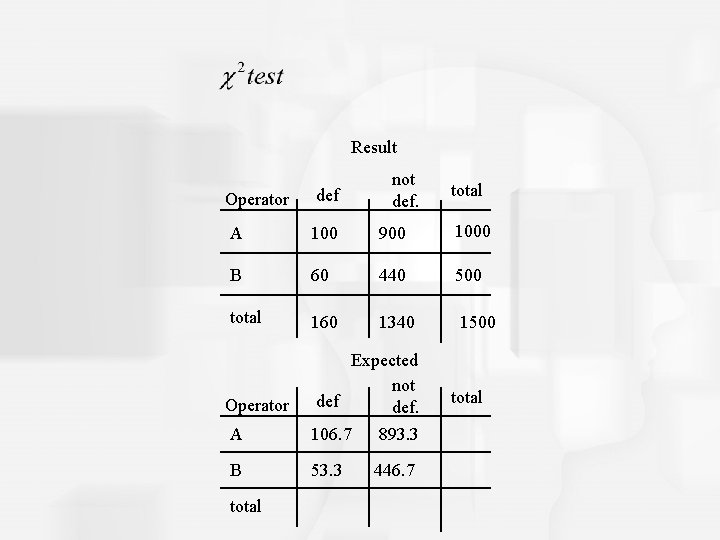
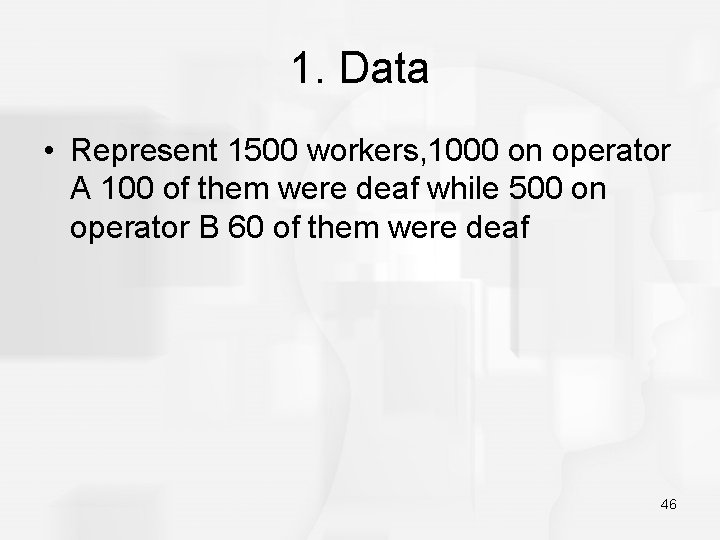
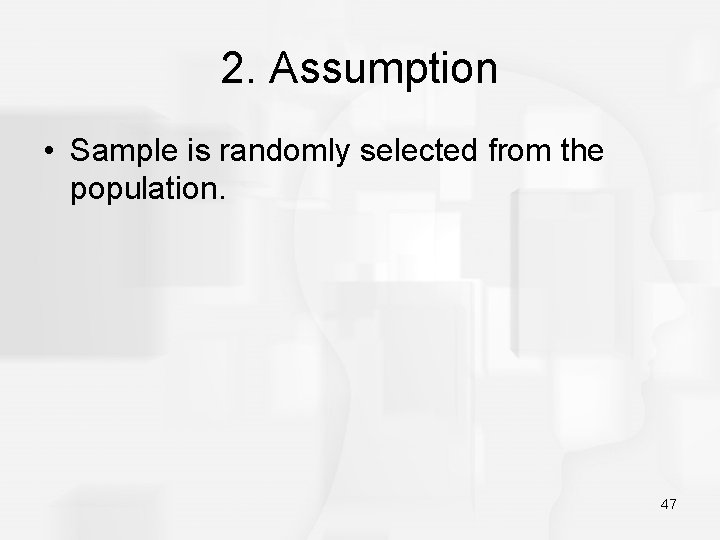
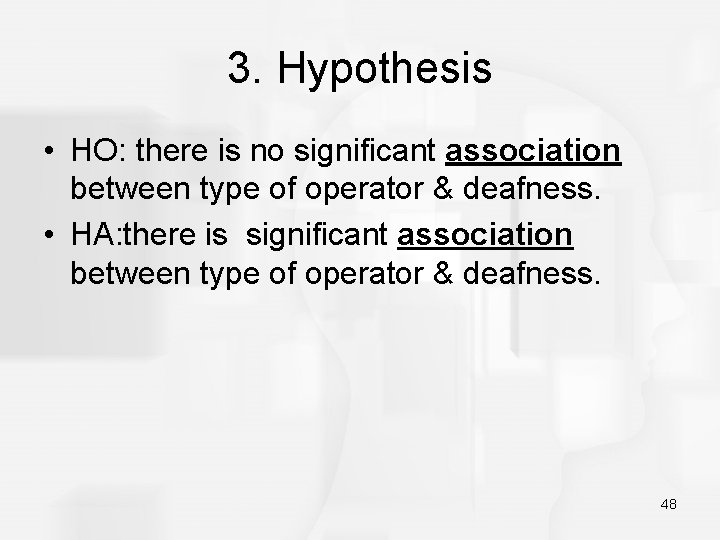
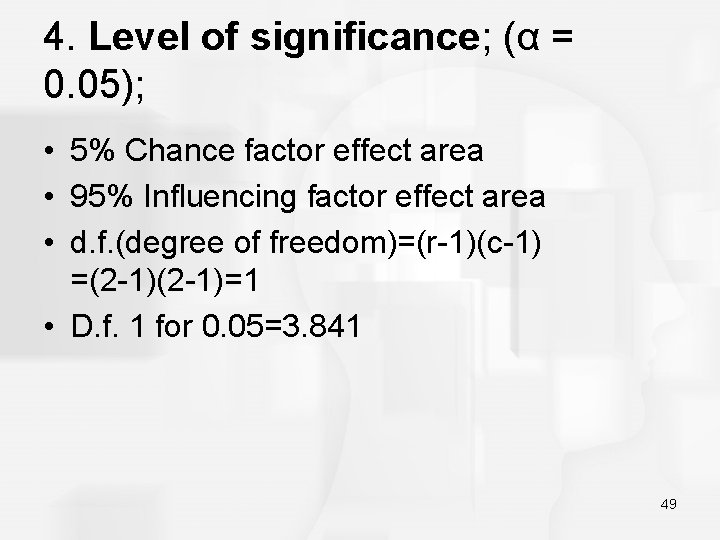
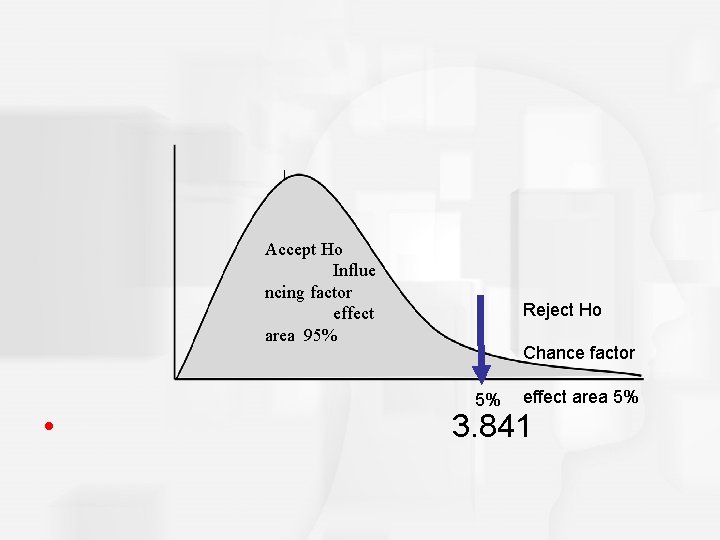
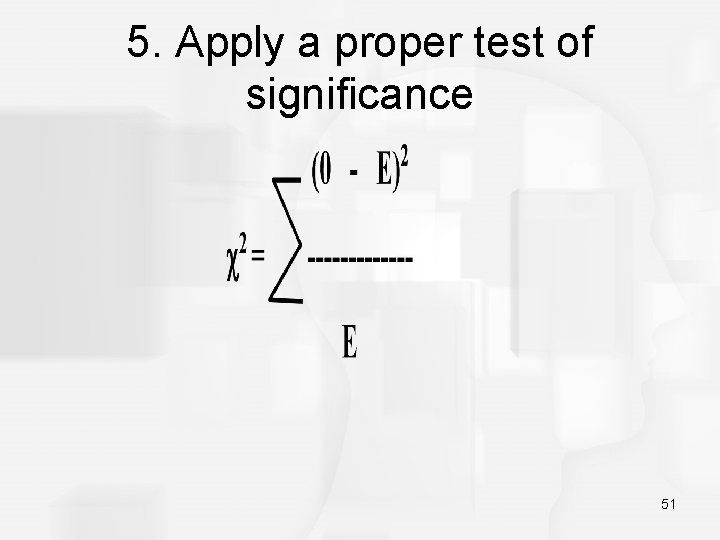
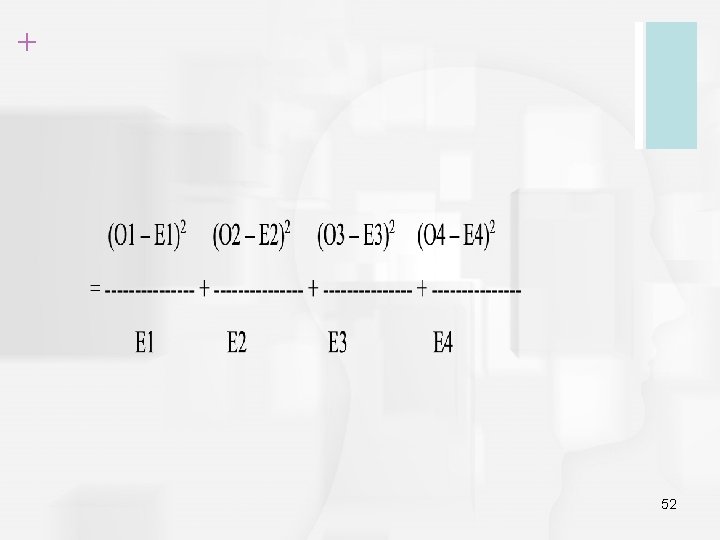
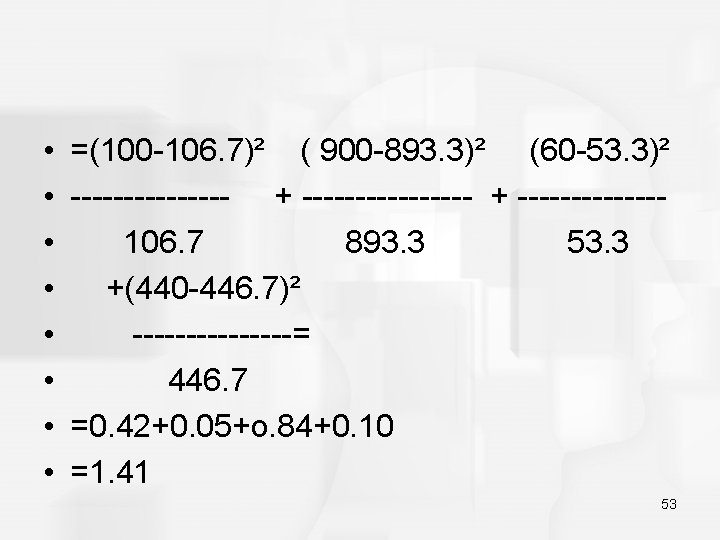
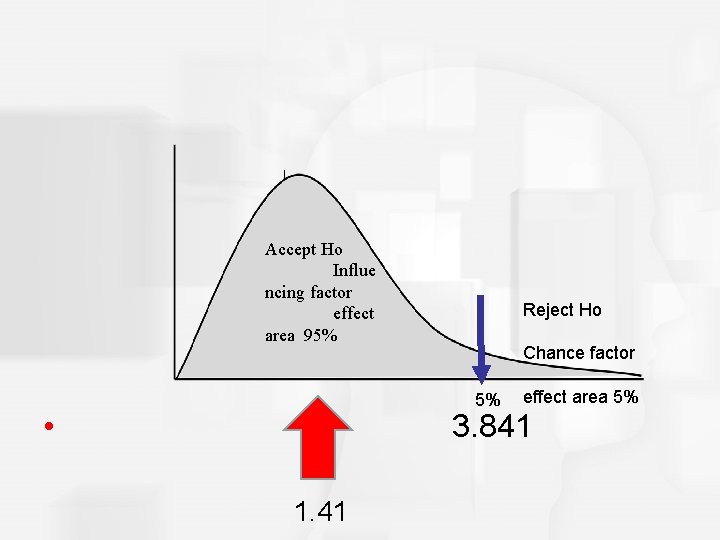
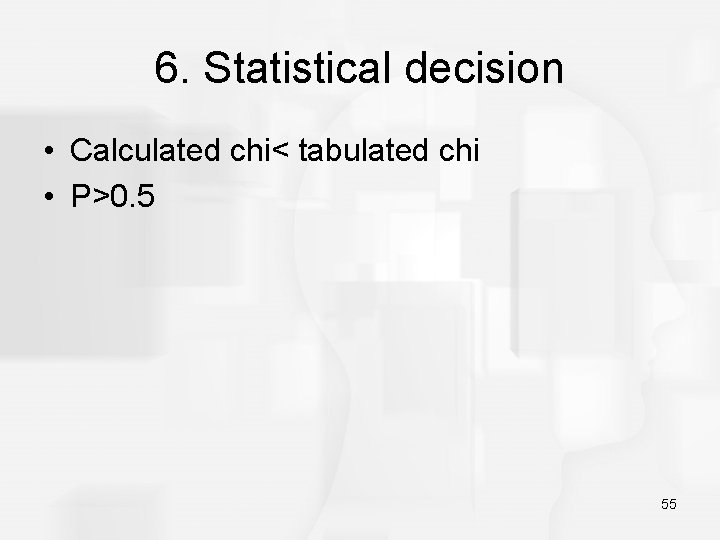
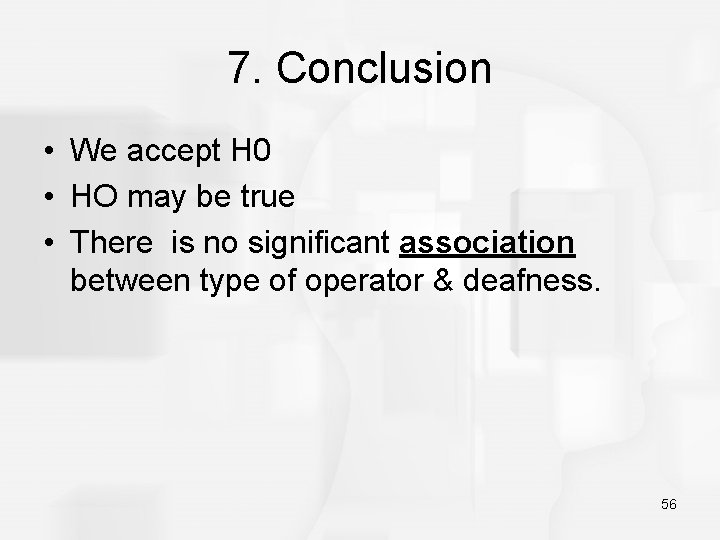
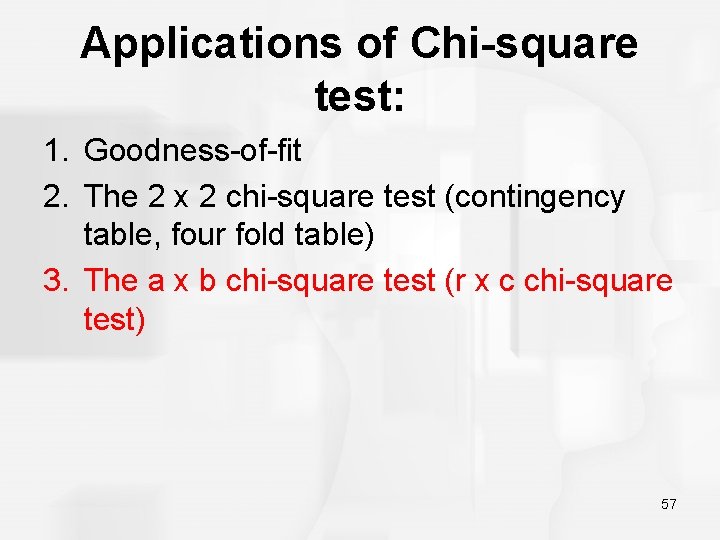
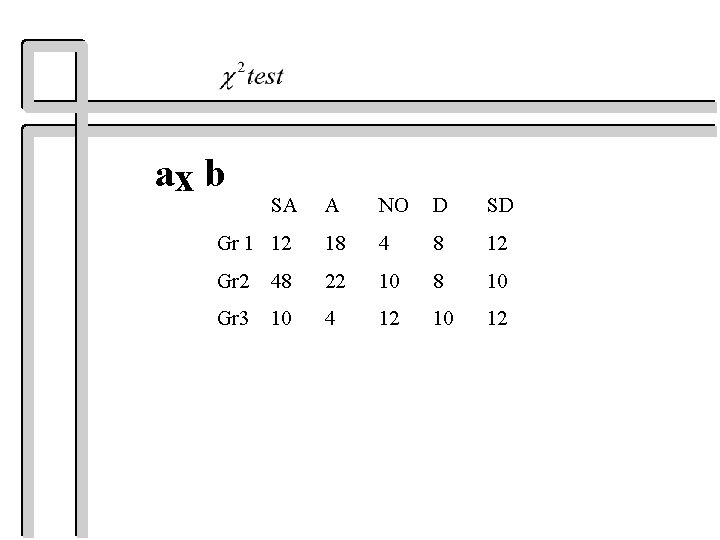
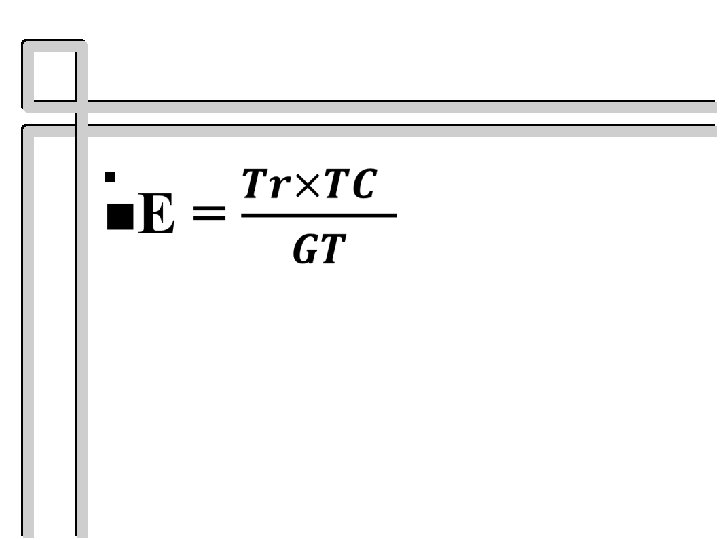
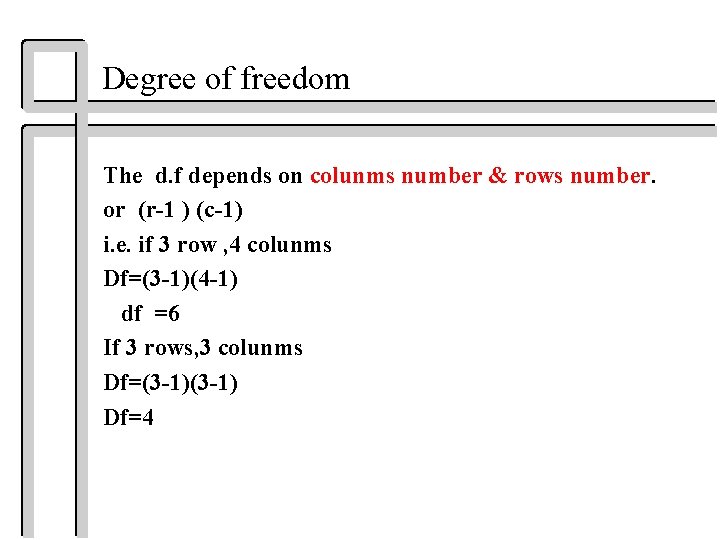
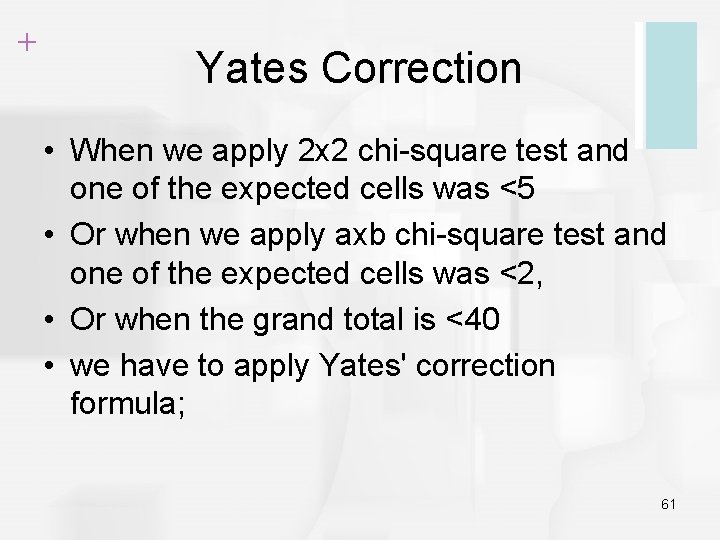
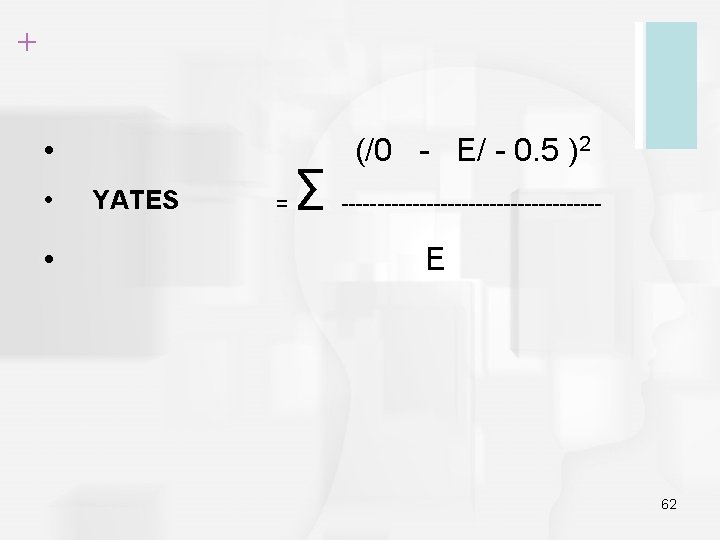
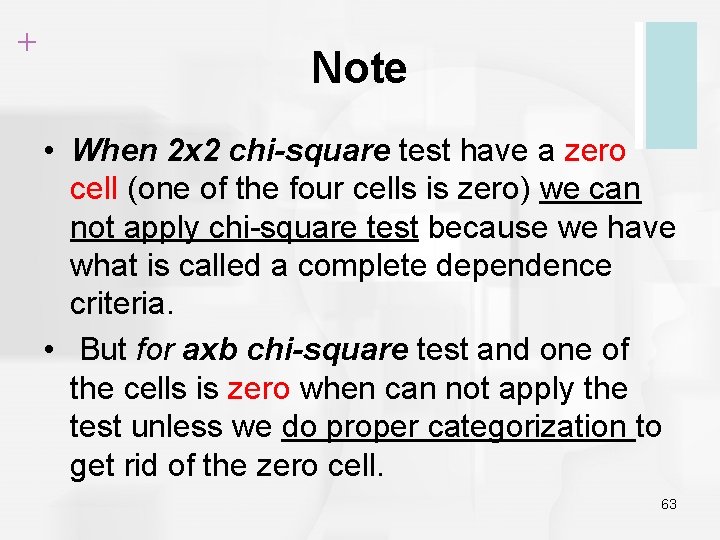
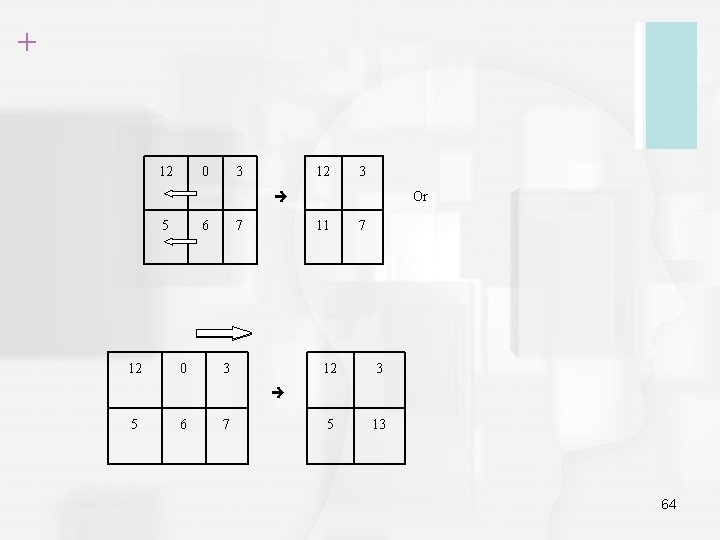
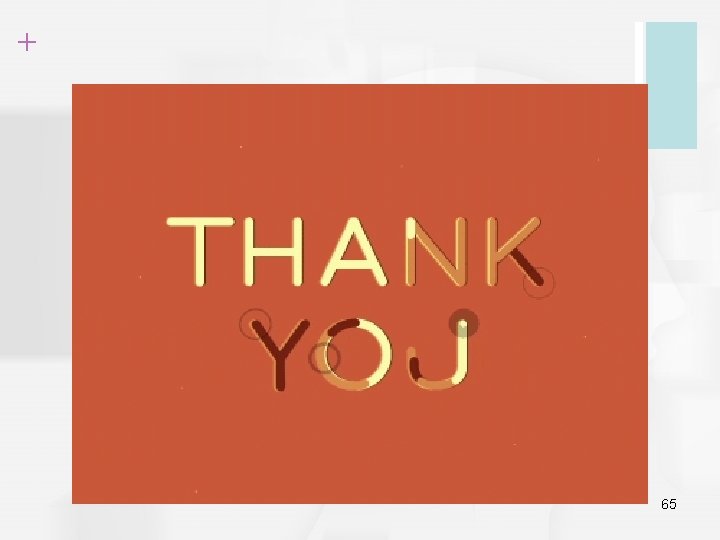
- Slides: 65
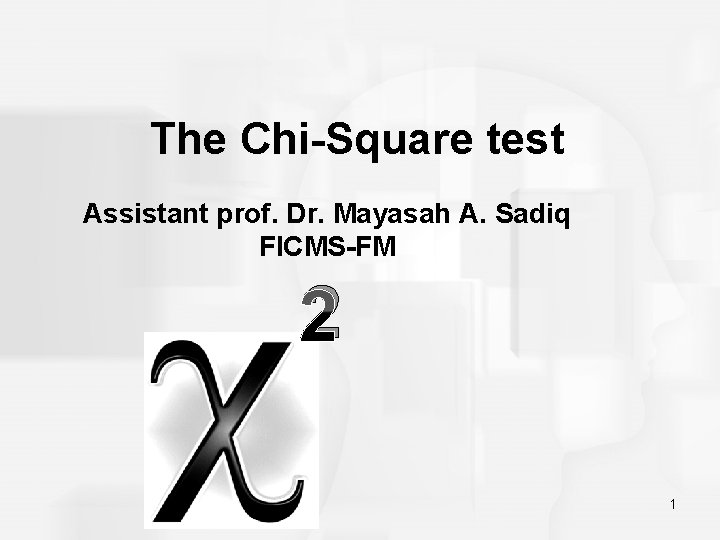
The Chi-Square test Assistant prof. Dr. Mayasah A. Sadiq FICMS-FM 2 1
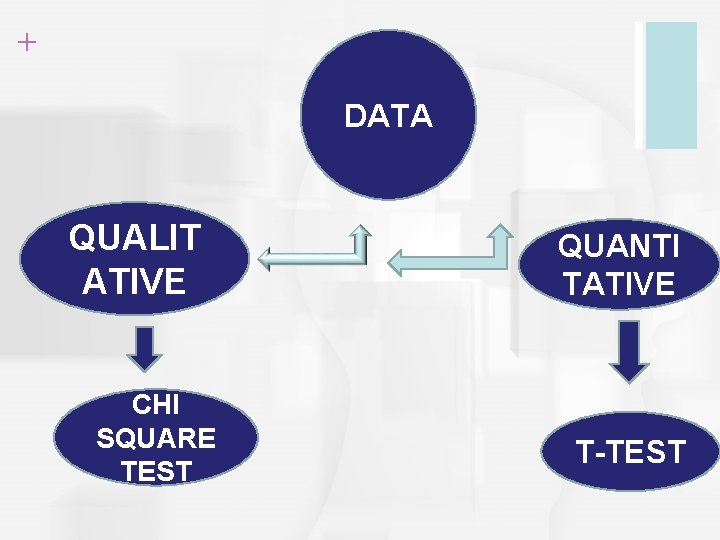
+ DATA QUALIT ATIVE CHI SQUARE TEST QUANTI TATIVE T-TEST
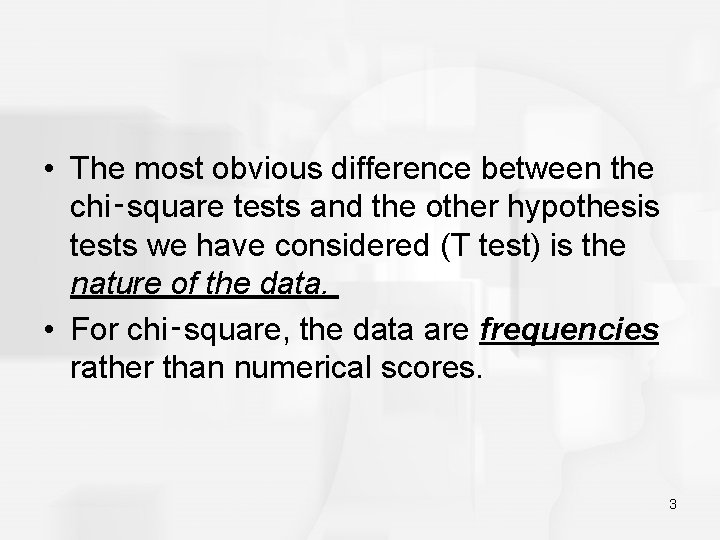
• The most obvious difference between the chi‑square tests and the other hypothesis tests we have considered (T test) is the nature of the data. • For chi‑square, the data are frequencies rather than numerical scores. 3
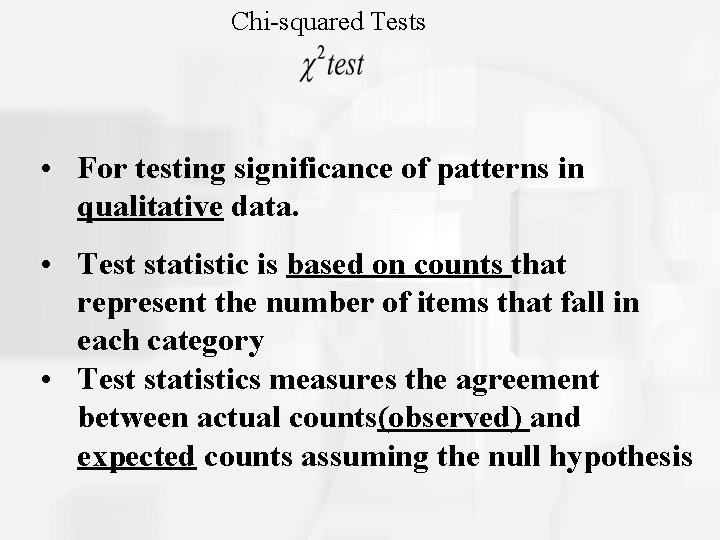
Chi-squared Tests • For testing significance of patterns in qualitative data. • Test statistic is based on counts that represent the number of items that fall in each category • Test statistics measures the agreement between actual counts(observed) and expected counts assuming the null hypothesis
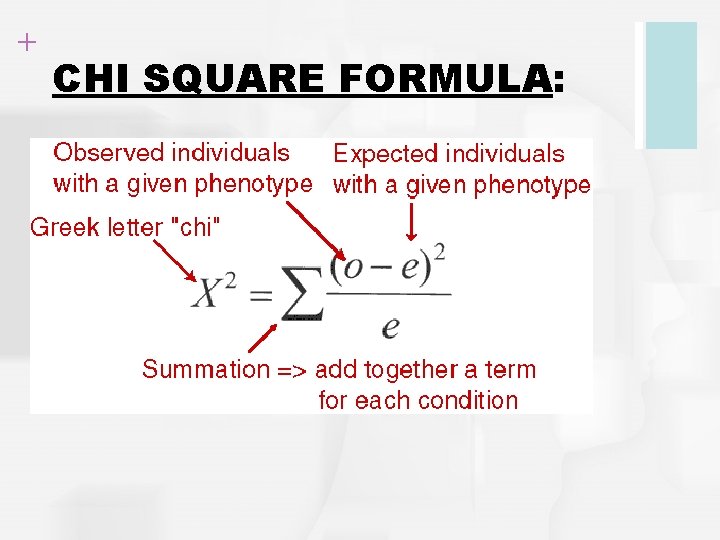
+ CHI SQUARE FORMULA:
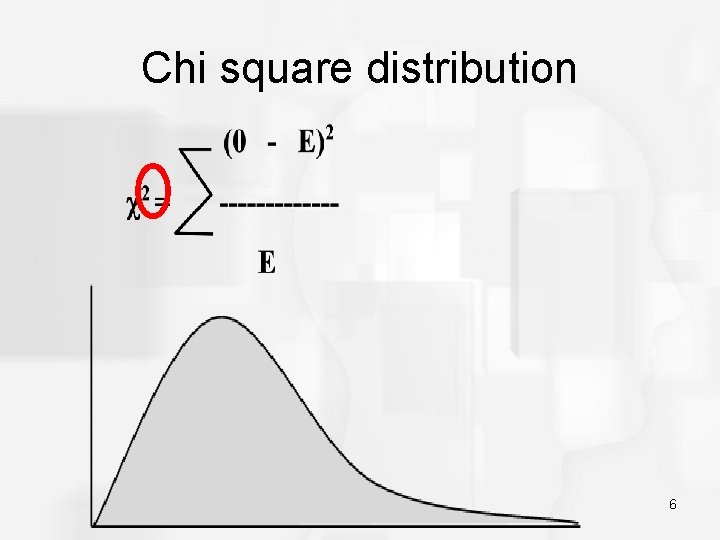
Chi square distribution 6
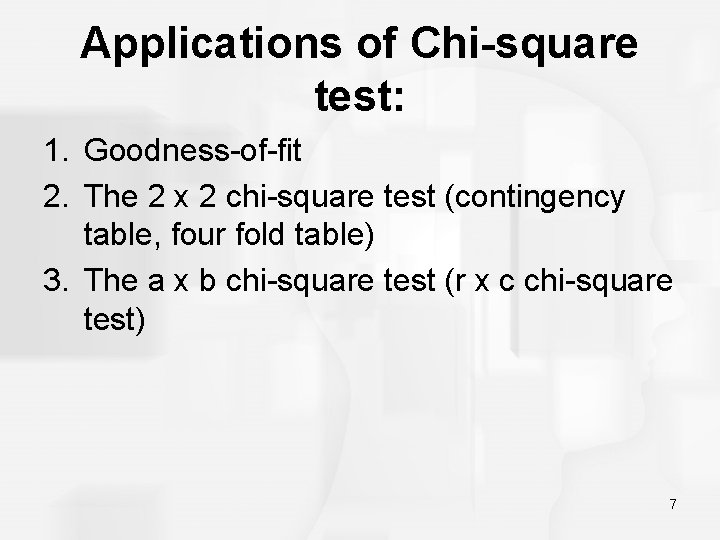
Applications of Chi-square test: 1. Goodness-of-fit 2. The 2 x 2 chi-square test (contingency table, four fold table) 3. The a x b chi-square test (r x c chi-square test) 7
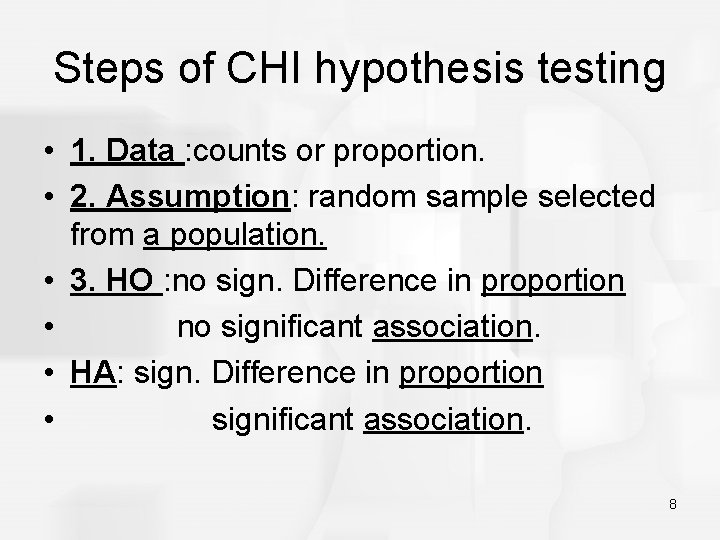
Steps of CHI hypothesis testing • 1. Data : counts or proportion. • 2. Assumption: random sample selected from a population. • 3. HO : no sign. Difference in proportion • no significant association. • HA: sign. Difference in proportion • significant association. 8
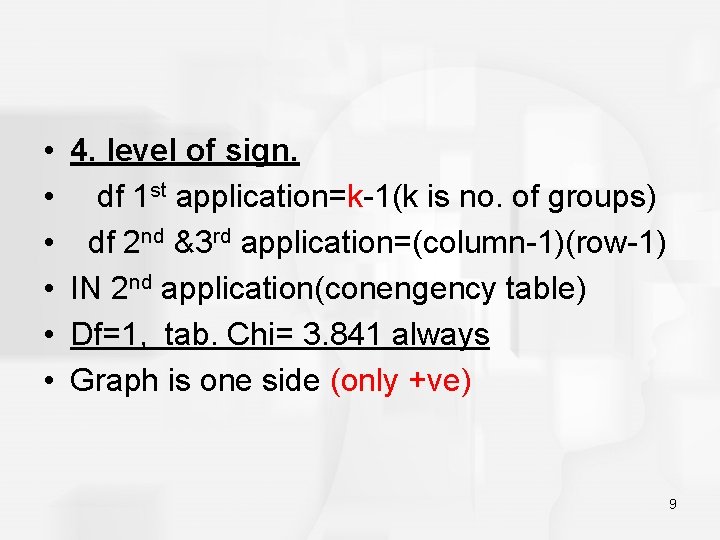
• • • 4. level of sign. df 1 st application=k-1(k is no. of groups) df 2 nd &3 rd application=(column-1)(row-1) IN 2 nd application(conengency table) Df=1, tab. Chi= 3. 841 always Graph is one side (only +ve) 9
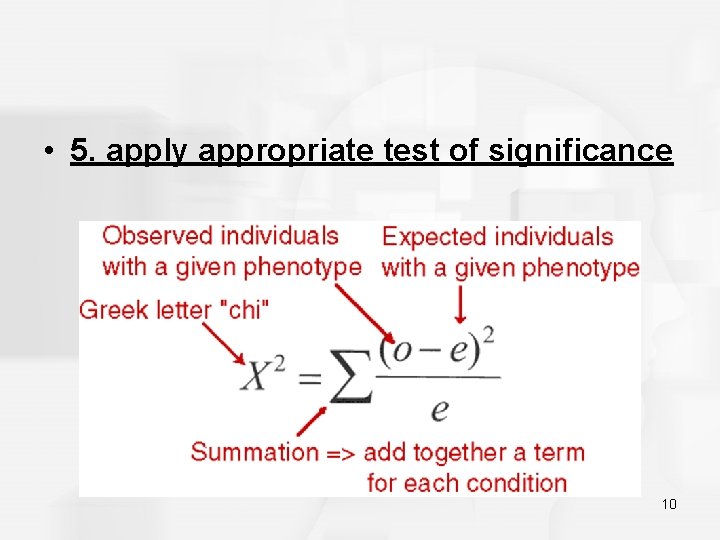
• 5. apply appropriate test of significance 10
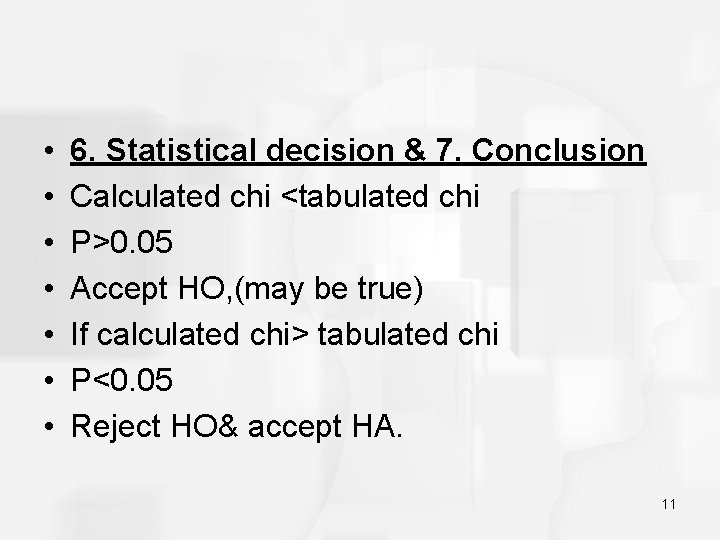
• • 6. Statistical decision & 7. Conclusion Calculated chi <tabulated chi P>0. 05 Accept HO, (may be true) If calculated chi> tabulated chi P<0. 05 Reject HO& accept HA. 11
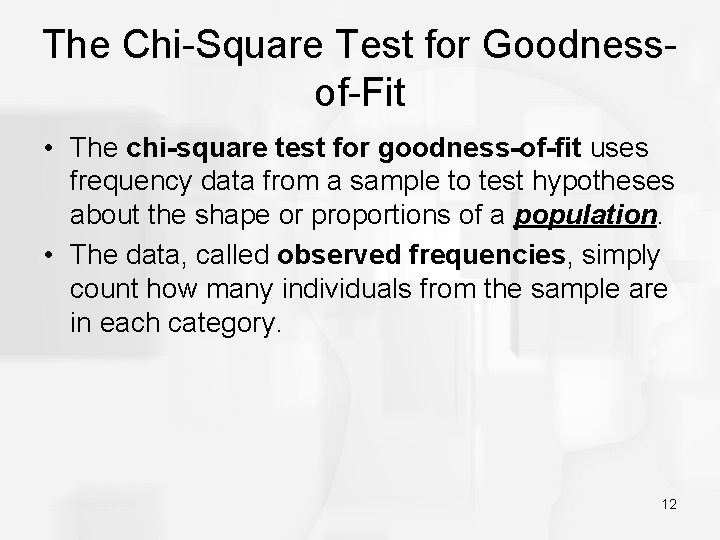
The Chi-Square Test for Goodnessof-Fit • The chi-square test for goodness-of-fit uses frequency data from a sample to test hypotheses about the shape or proportions of a population. • The data, called observed frequencies, simply count how many individuals from the sample are in each category. 12
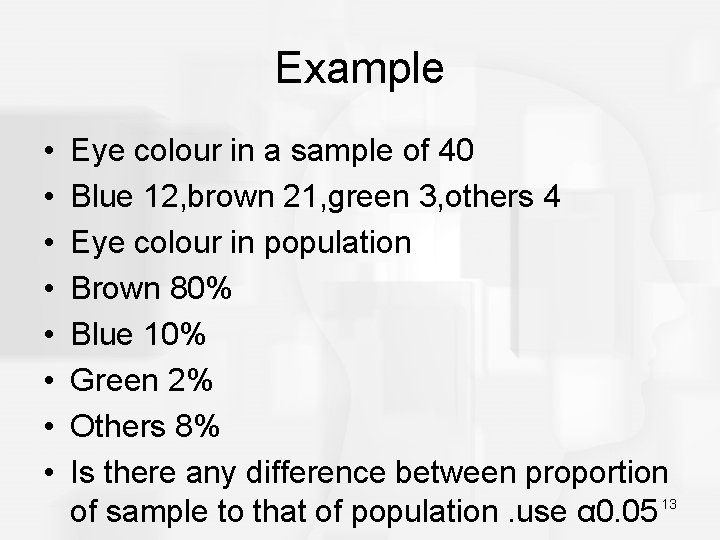
Example • • Eye colour in a sample of 40 Blue 12, brown 21, green 3, others 4 Eye colour in population Brown 80% Blue 10% Green 2% Others 8% Is there any difference between proportion of sample to that of population. use α 0. 05 13
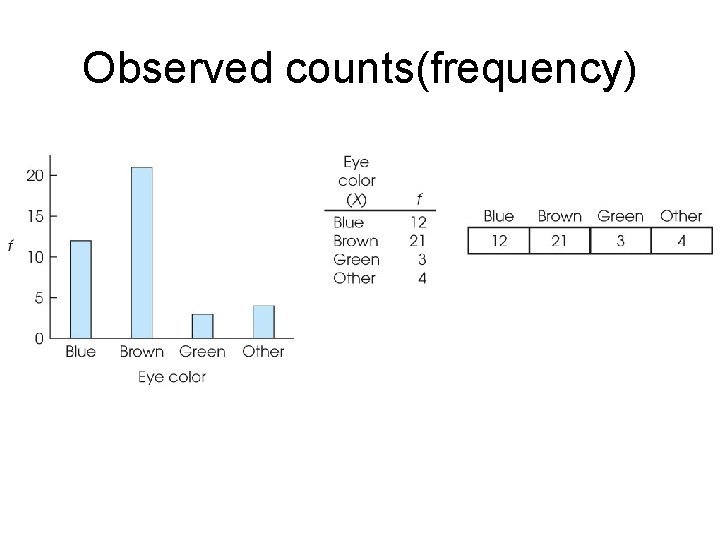
Observed counts(frequency)
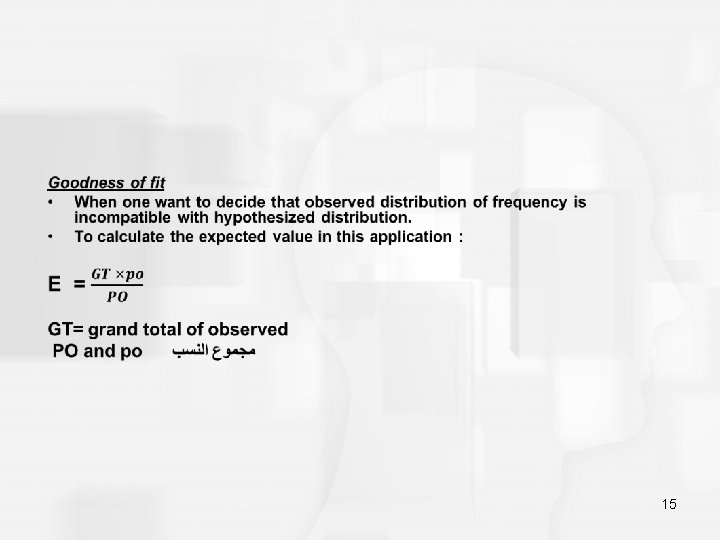
15
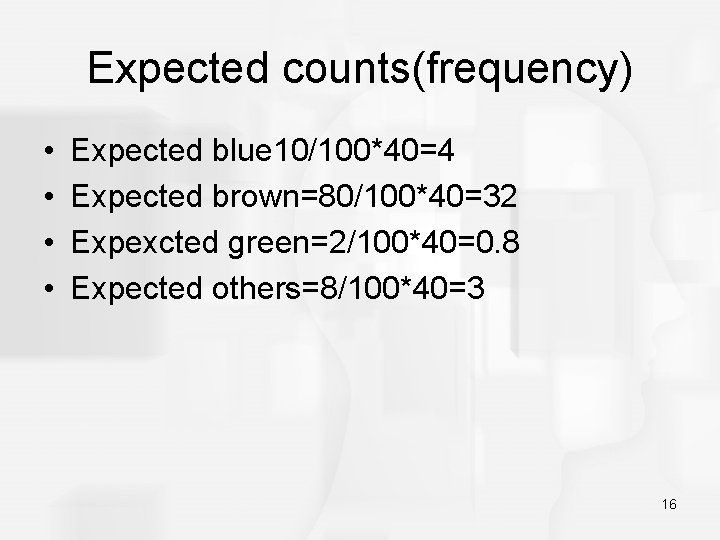
Expected counts(frequency) • • Expected blue 10/100*40=4 Expected brown=80/100*40=32 Expexcted green=2/100*40=0. 8 Expected others=8/100*40=3 16
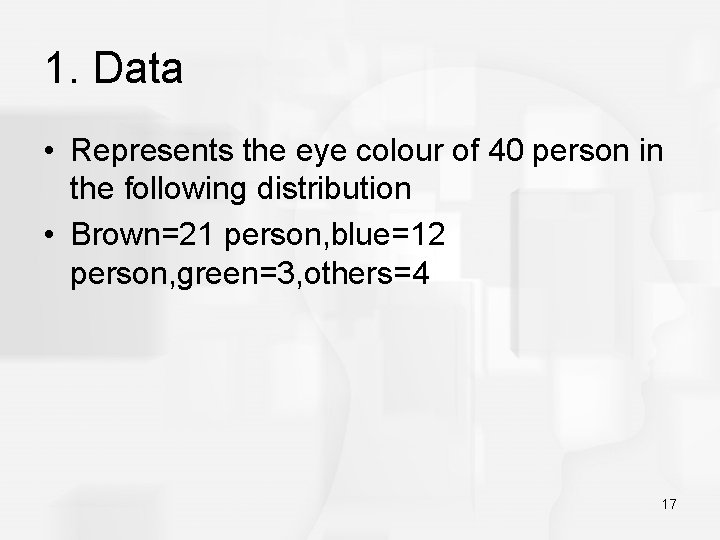
1. Data • Represents the eye colour of 40 person in the following distribution • Brown=21 person, blue=12 person, green=3, others=4 17
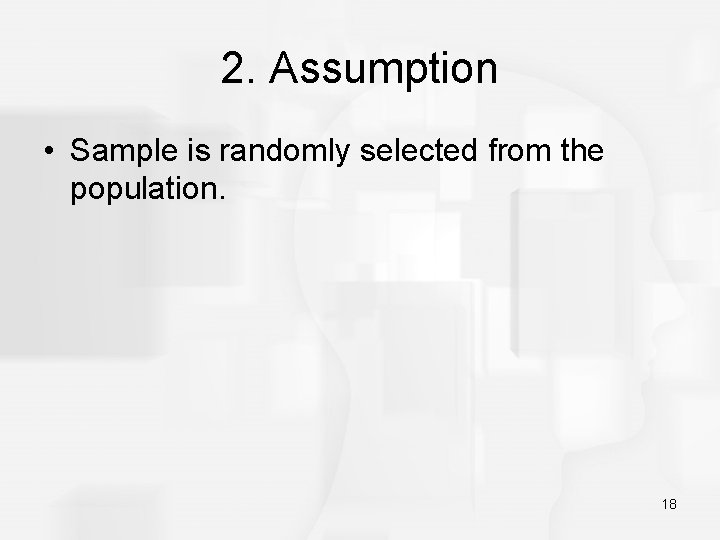
2. Assumption • Sample is randomly selected from the population. 18
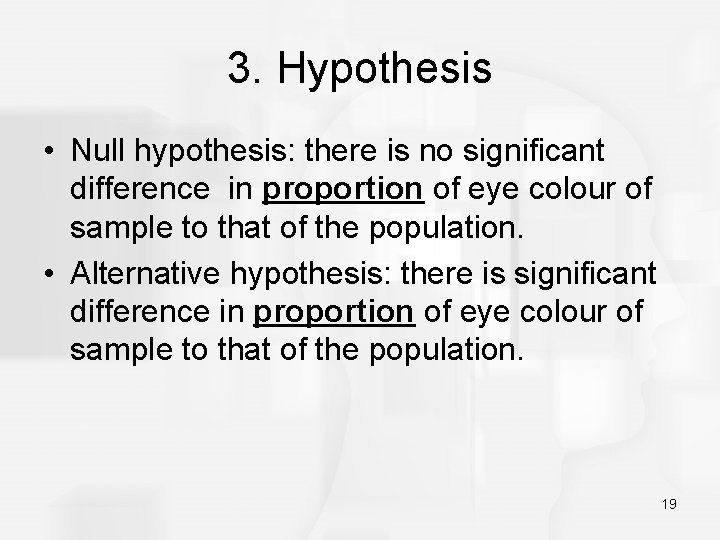
3. Hypothesis • Null hypothesis: there is no significant difference in proportion of eye colour of sample to that of the population. • Alternative hypothesis: there is significant difference in proportion of eye colour of sample to that of the population. 19
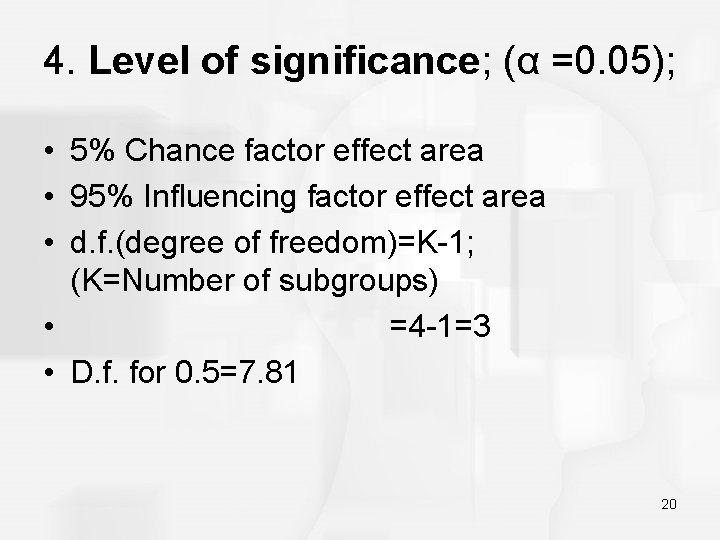
4. Level of significance; (α =0. 05); • 5% Chance factor effect area • 95% Influencing factor effect area • d. f. (degree of freedom)=K-1; (K=Number of subgroups) • =4 -1=3 • D. f. for 0. 5=7. 81 20
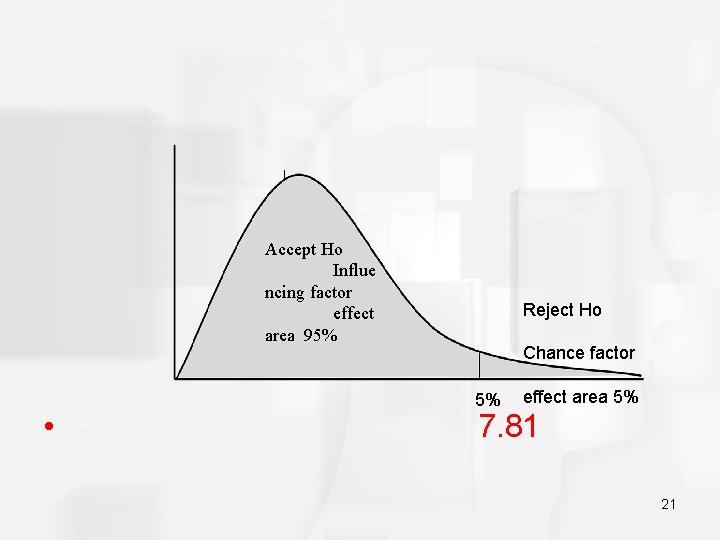
Accept Ho Influe ncing factor effect area 95% 5% Reject Ho Chance factor effect area 5% • 7. 81 21
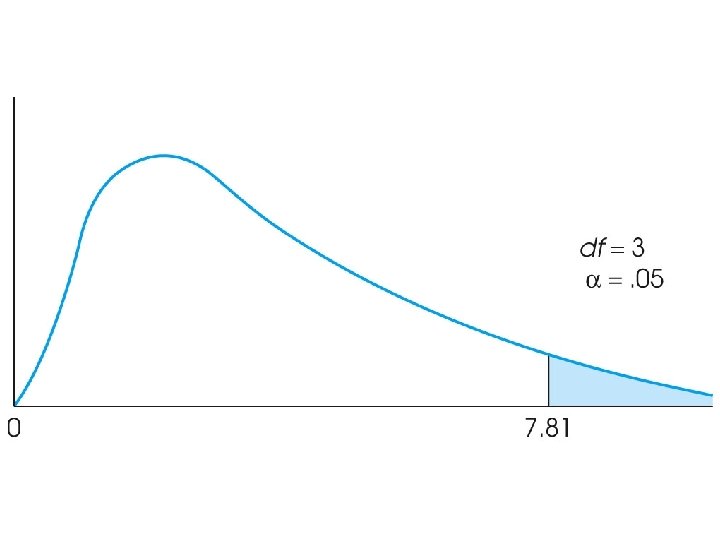
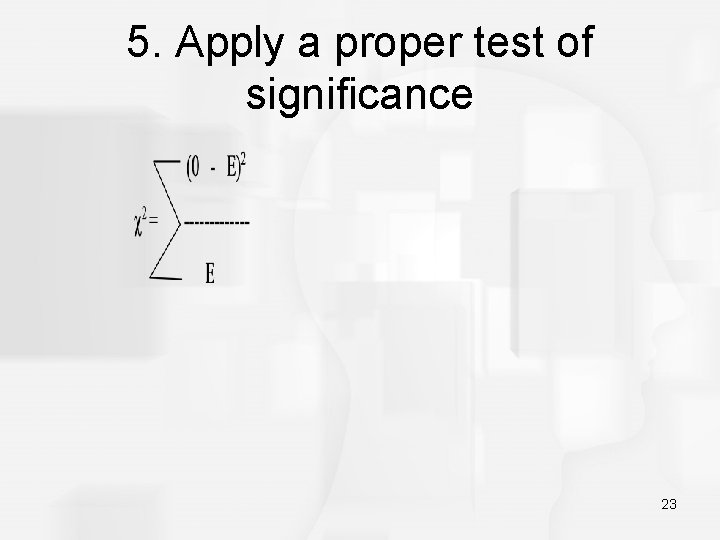
5. Apply a proper test of significance 23
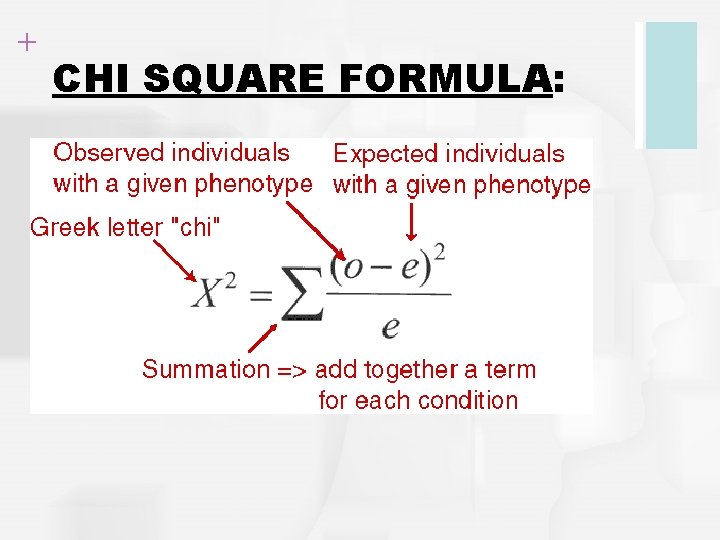
+ CHI SQUARE FORMULA:
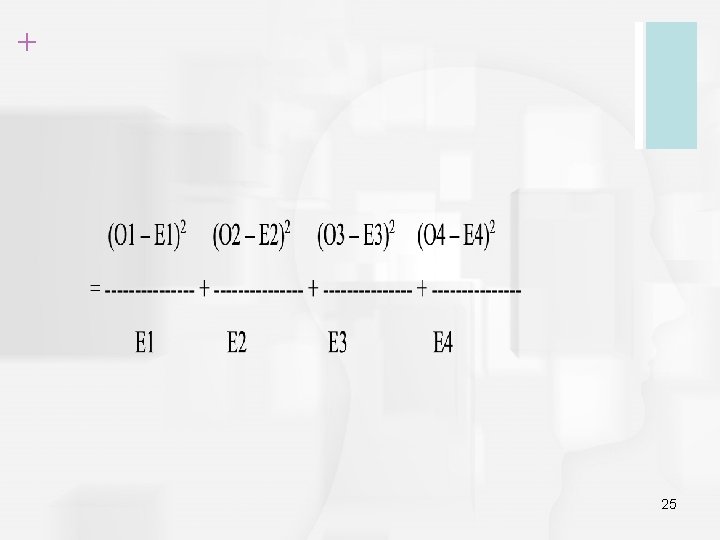
+ 25
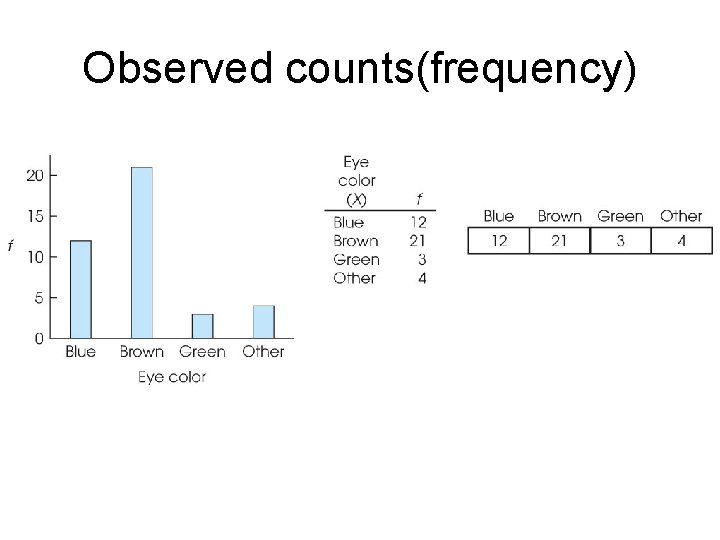
Observed counts(frequency)
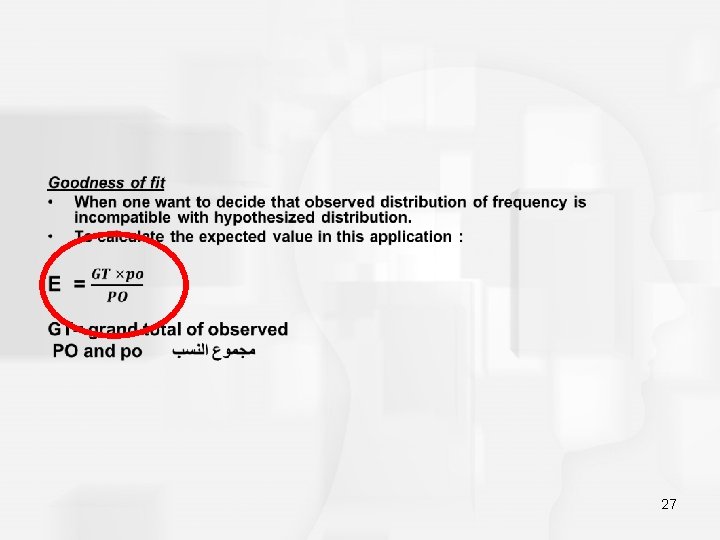
27
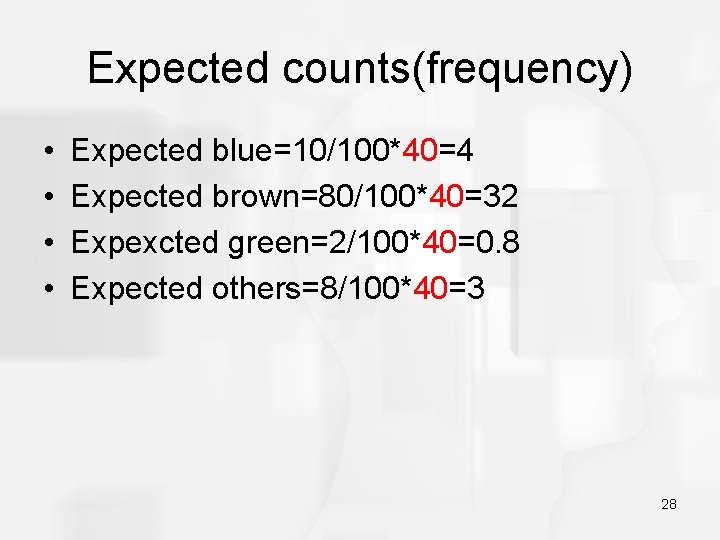
Expected counts(frequency) • • Expected blue=10/100*40=4 Expected brown=80/100*40=32 Expexcted green=2/100*40=0. 8 Expected others=8/100*40=3 28
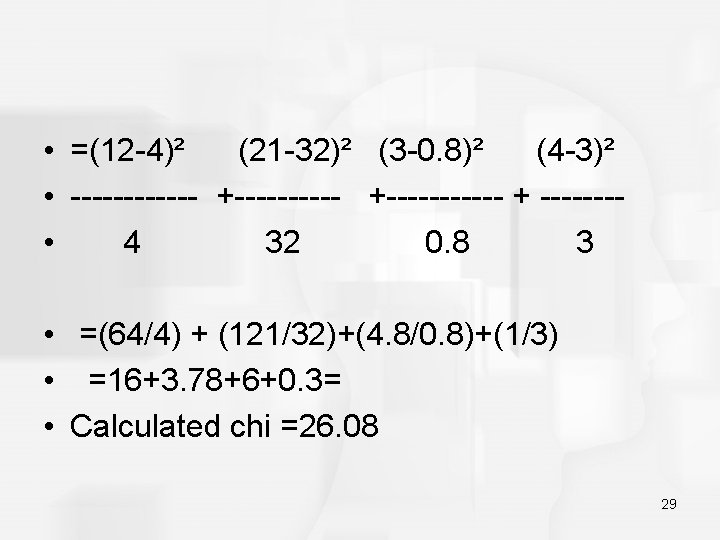
• =(12 -4)² (21 -32)² (3 -0. 8)² (4 -3)² • ------ +------ + ------- • 4 32 0. 8 3 • =(64/4) + (121/32)+(4. 8/0. 8)+(1/3) • =16+3. 78+6+0. 3= • Calculated chi =26. 08 29
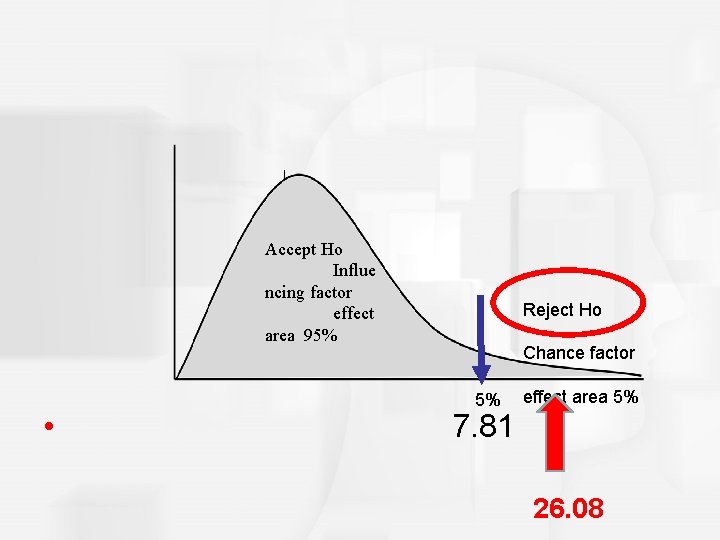
Accept Ho Influe ncing factor effect area 95% 5% Reject Ho Chance factor effect area 5% • 7. 81 26. 08
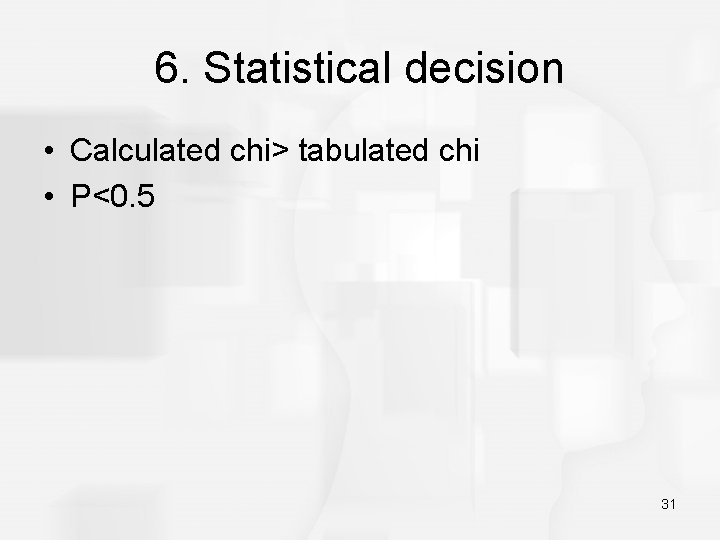
6. Statistical decision • Calculated chi> tabulated chi • P<0. 5 31
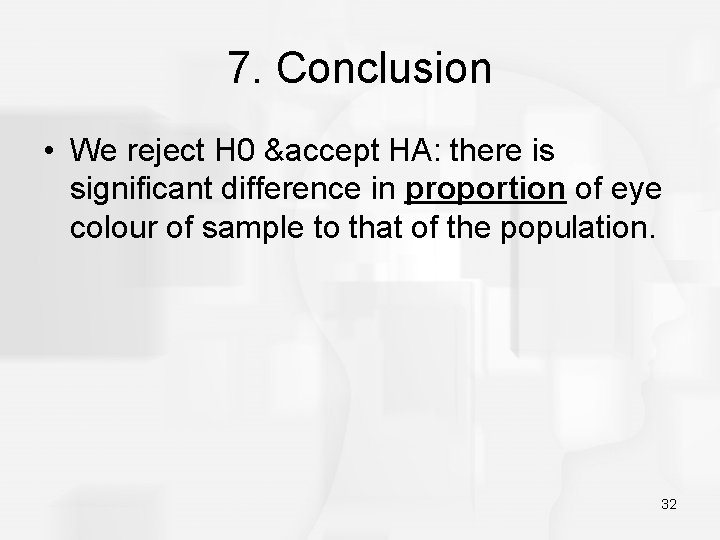
7. Conclusion • We reject H 0 &accept HA: there is significant difference in proportion of eye colour of sample to that of the population. 32
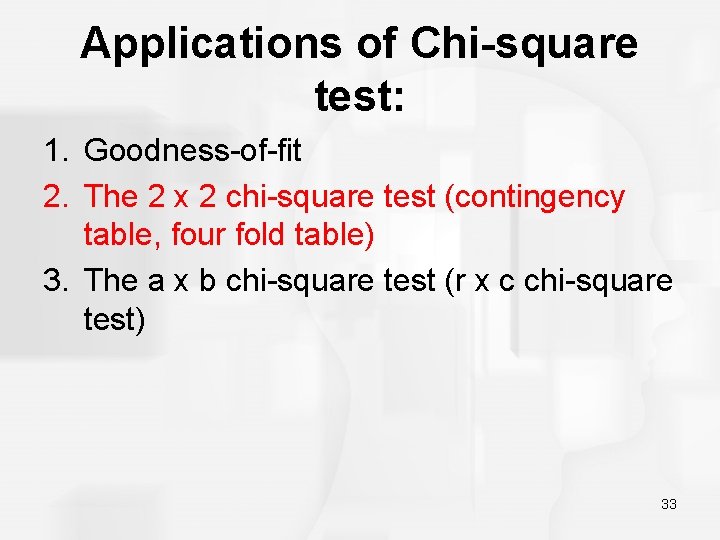
Applications of Chi-square test: 1. Goodness-of-fit 2. The 2 x 2 chi-square test (contingency table, four fold table) 3. The a x b chi-square test (r x c chi-square test) 33
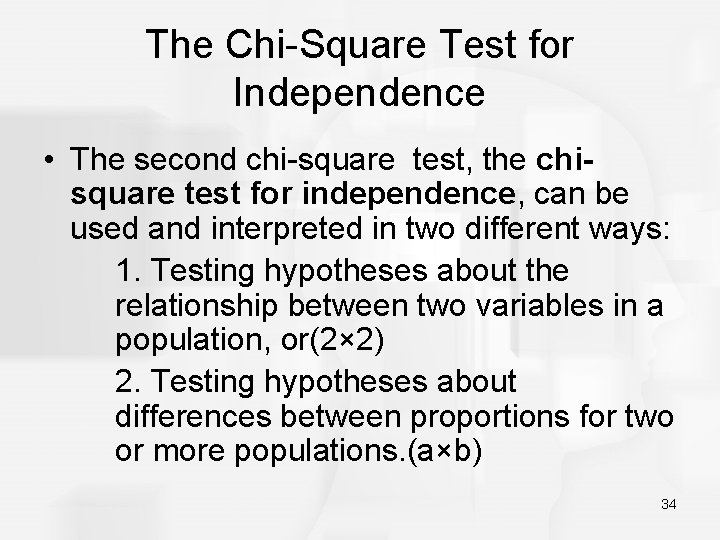
The Chi-Square Test for Independence • The second chi-square test, the chisquare test for independence, can be used and interpreted in two different ways: 1. Testing hypotheses about the relationship between two variables in a population, or(2× 2) 2. Testing hypotheses about differences between proportions for two or more populations. (a×b) 34
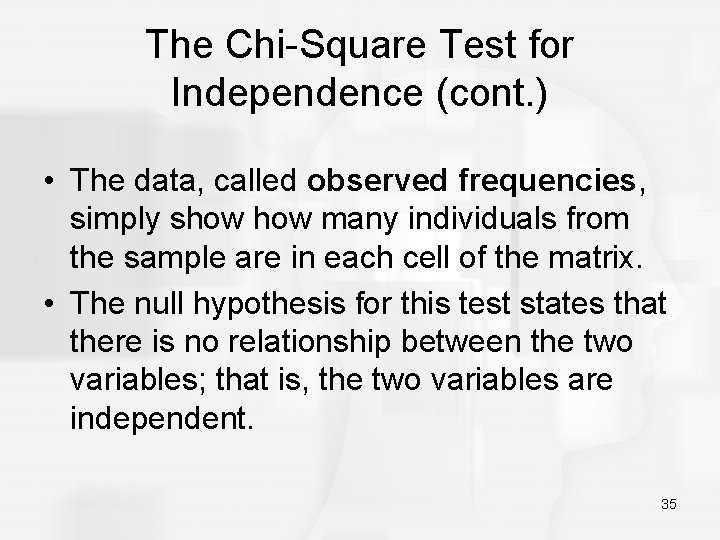
The Chi-Square Test for Independence (cont. ) • The data, called observed frequencies, simply show many individuals from the sample are in each cell of the matrix. • The null hypothesis for this test states that there is no relationship between the two variables; that is, the two variables are independent. 35
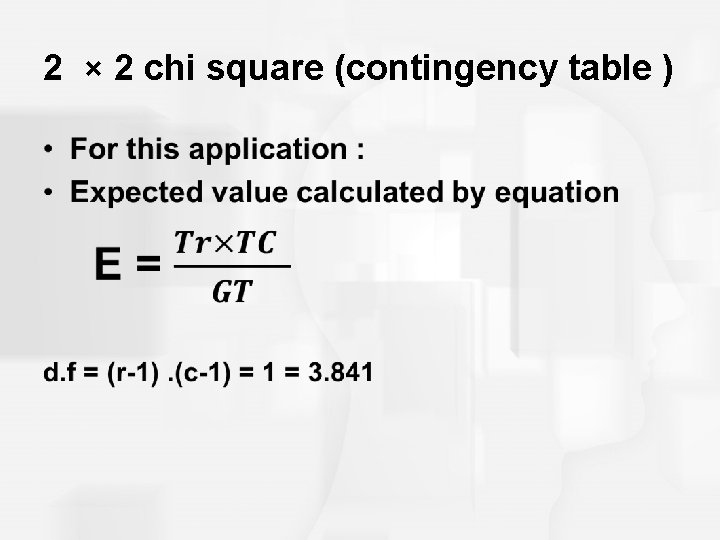
2 × 2 chi square (contingency table ) •
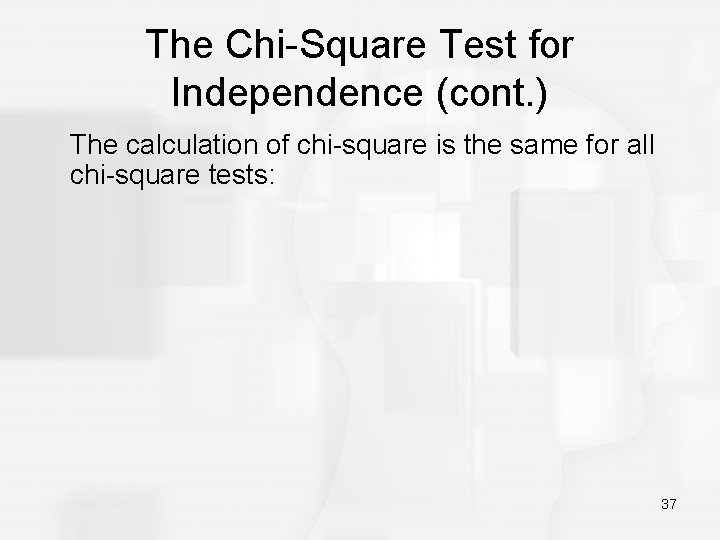
The Chi-Square Test for Independence (cont. ) The calculation of chi-square is the same for all chi-square tests: 37
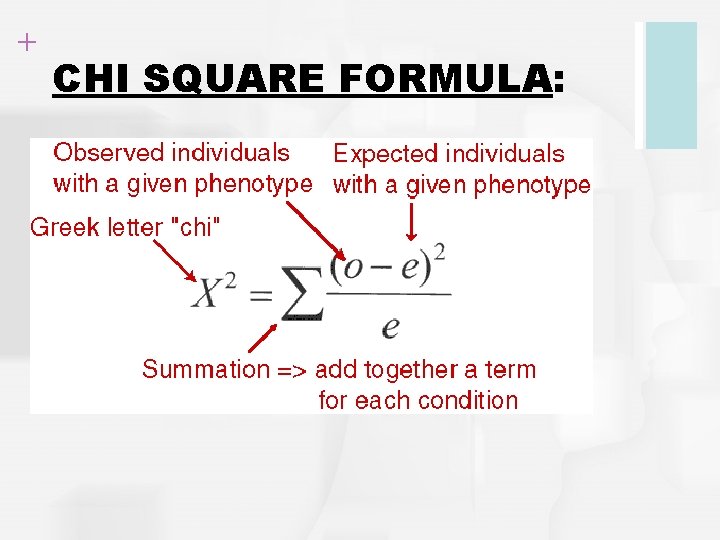
+ CHI SQUARE FORMULA:
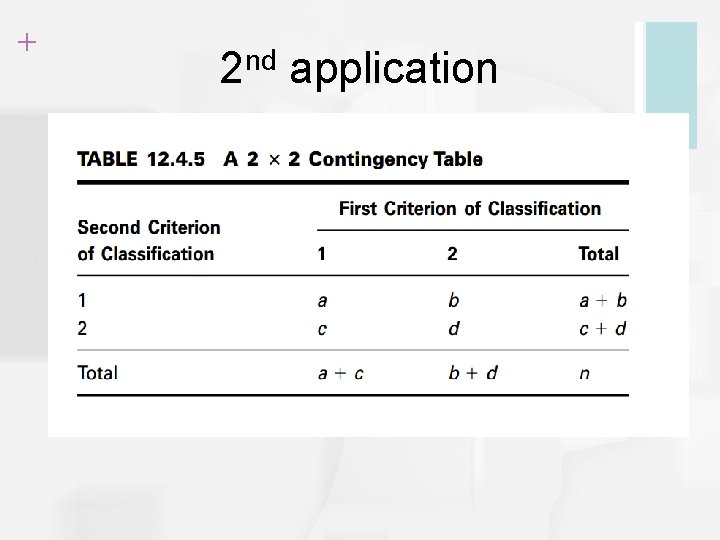
+ 2 nd application
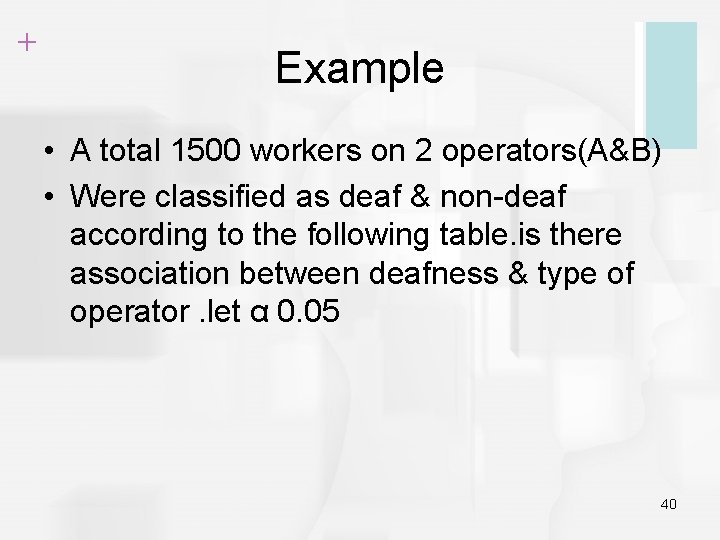
+ Example • A total 1500 workers on 2 operators(A&B) • Were classified as deaf & non-deaf according to the following table. is there association between deafness & type of operator. let α 0. 05 40
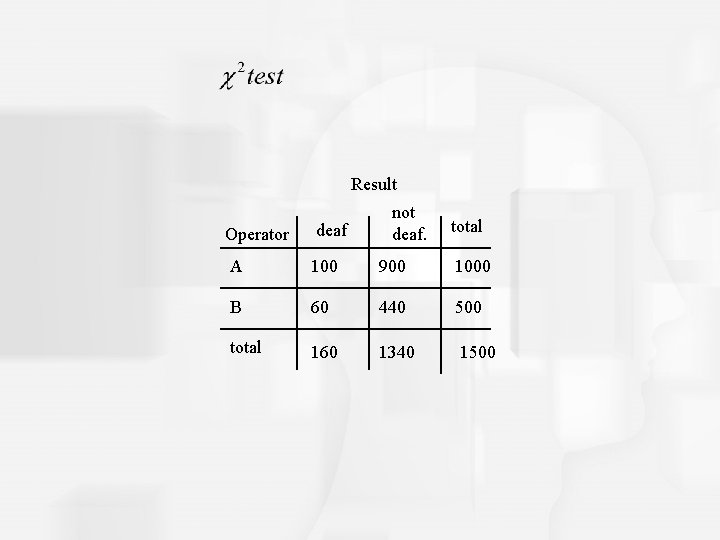
Result Operator deaf not deaf. total A 100 900 1000 B 60 440 500 total 160 1340 1500
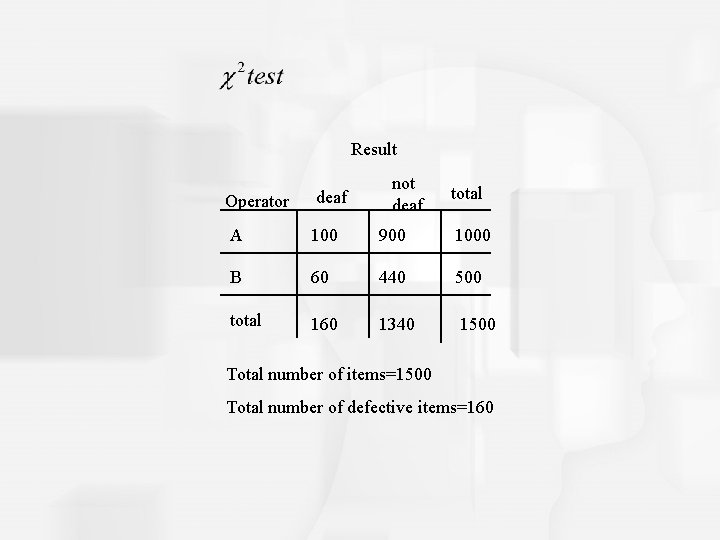
Result Operator deaf not deaf. total A 100 900 1000 B 60 440 500 total 160 1340 1500 Total number of items=1500 Total number of defective items=160
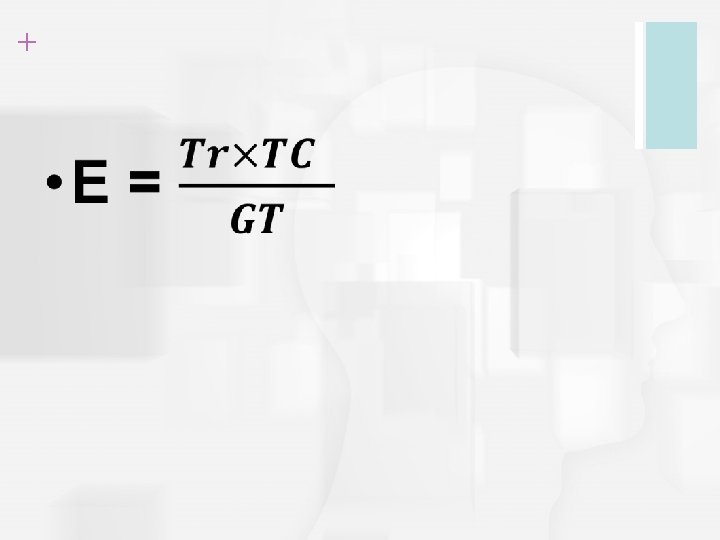
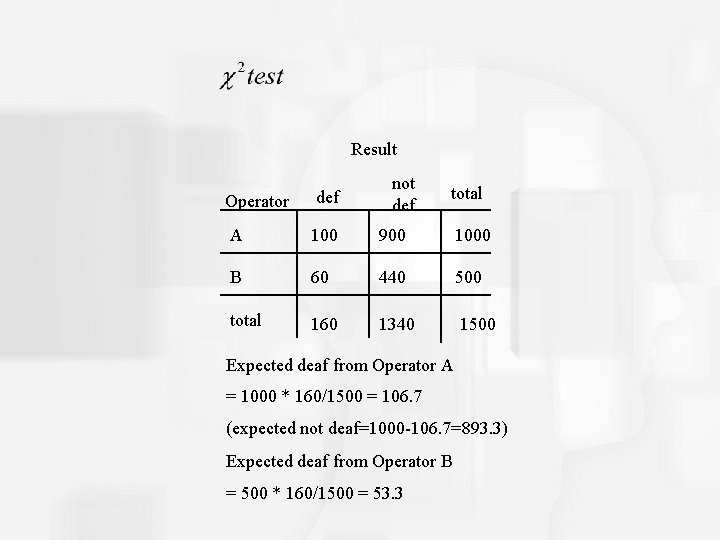
Result Operator def not def. total A 100 900 1000 B 60 440 500 total 160 1340 1500 Expected deaf from Operator A = 1000 * 160/1500 = 106. 7 (expected not deaf=1000 -106. 7=893. 3) Expected deaf from Operator B = 500 * 160/1500 = 53. 3
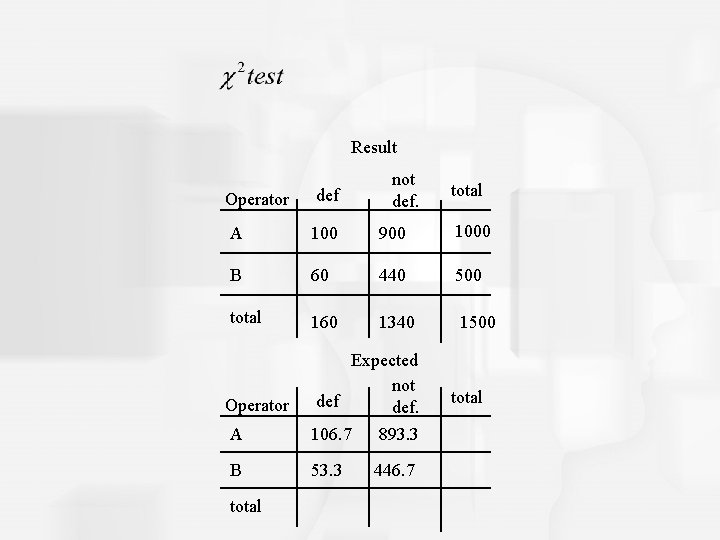
Result Operator def not def. total A 100 900 1000 B 60 440 500 total 160 1340 1500 A Expected not def. 893. 3 106. 7 B 53. 3 Operator total 446. 7 total
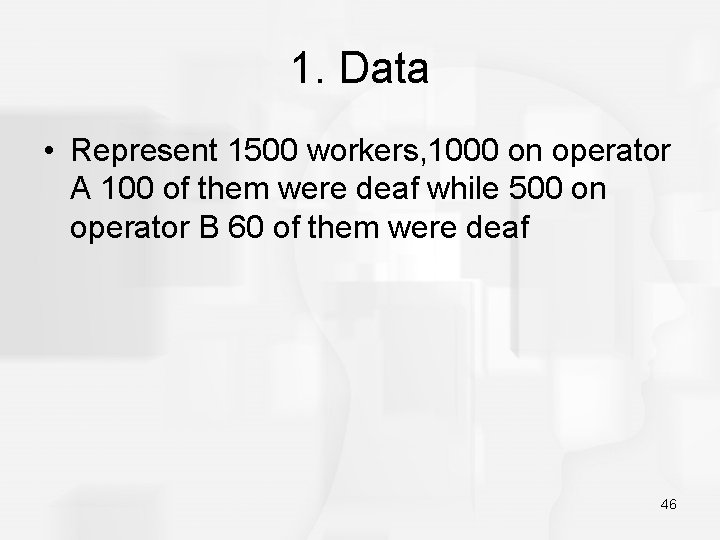
1. Data • Represent 1500 workers, 1000 on operator A 100 of them were deaf while 500 on operator B 60 of them were deaf 46
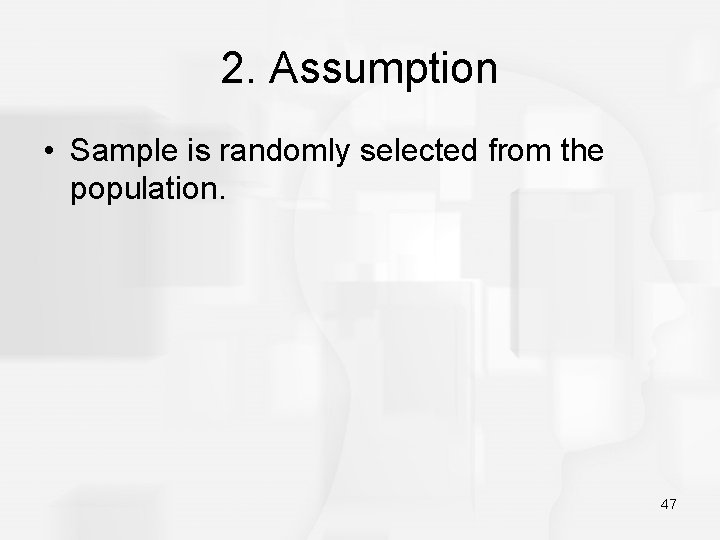
2. Assumption • Sample is randomly selected from the population. 47
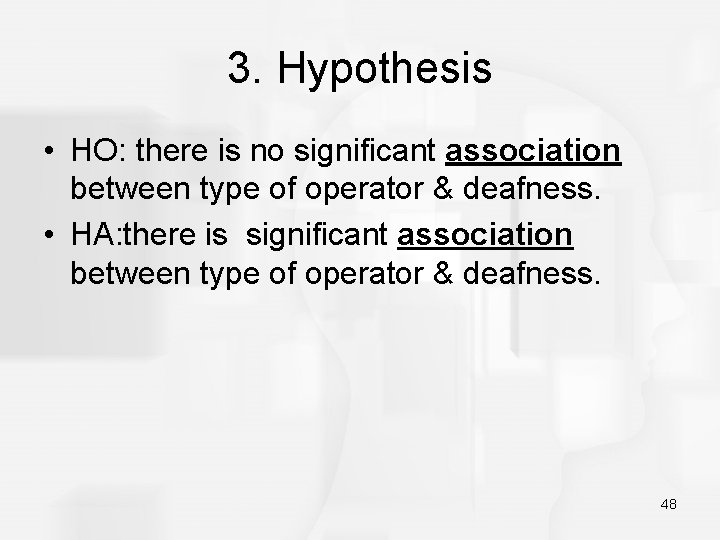
3. Hypothesis • HO: there is no significant association between type of operator & deafness. • HA: there is significant association between type of operator & deafness. 48
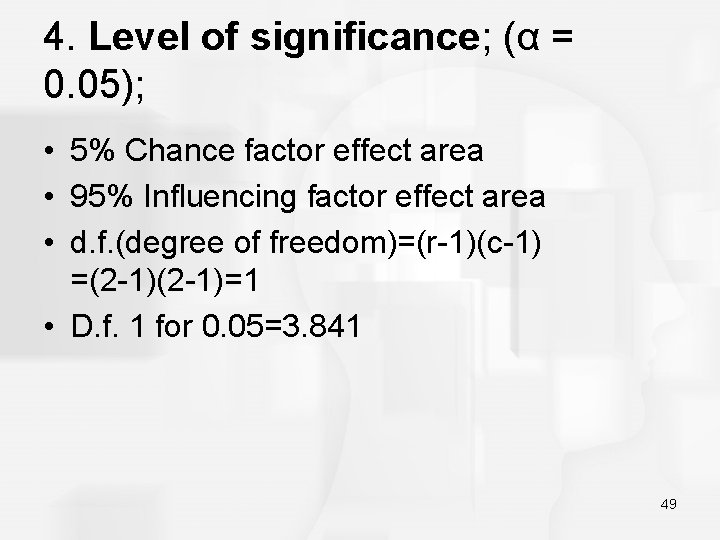
4. Level of significance; (α = 0. 05); • 5% Chance factor effect area • 95% Influencing factor effect area • d. f. (degree of freedom)=(r-1)(c-1) =(2 -1)=1 • D. f. 1 for 0. 05=3. 841 49
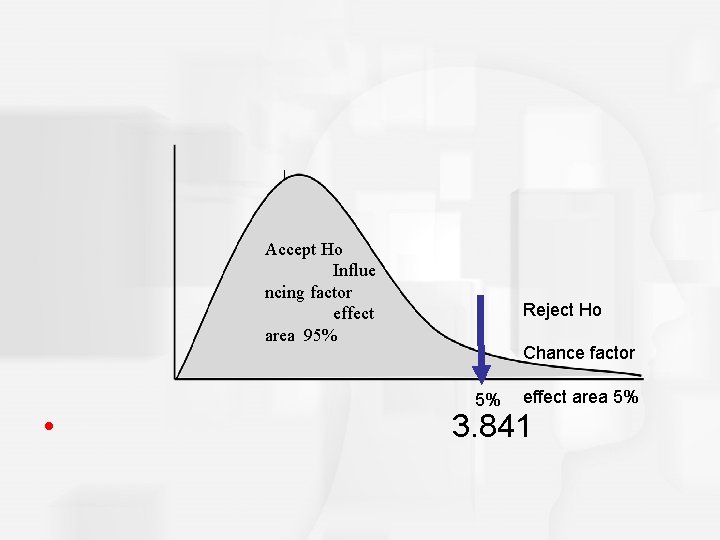
Accept Ho Influe ncing factor effect area 95% 5% Reject Ho Chance factor effect area 5% • 3. 841
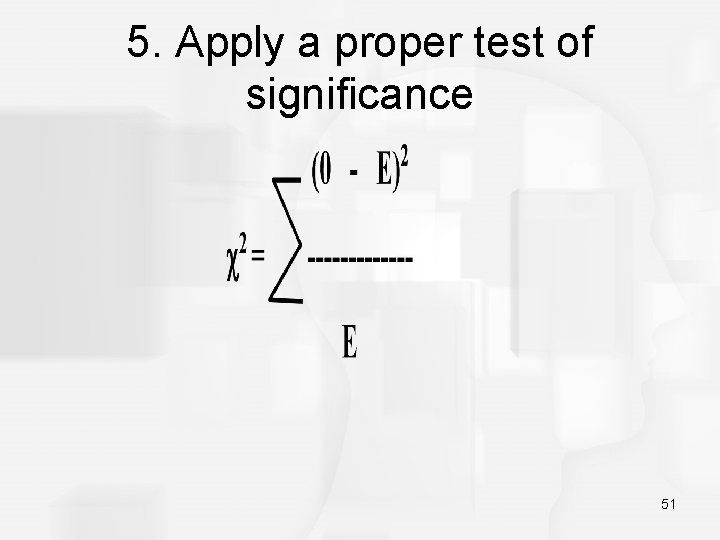
5. Apply a proper test of significance 51
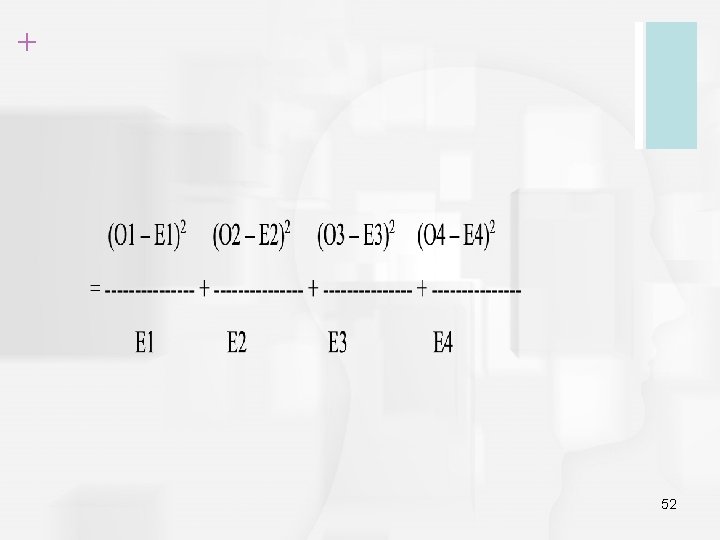
+ 52
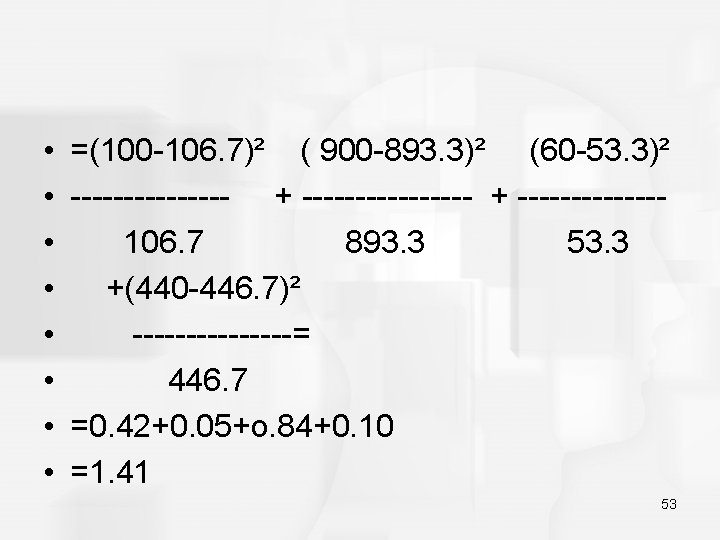
• • =(100 -106. 7)² ( 900 -893. 3)² (60 -53. 3)² --------------- + ------- 106. 7 893. 3 53. 3 +(440 -446. 7)² --------= 446. 7 =0. 42+0. 05+o. 84+0. 10 =1. 41 53
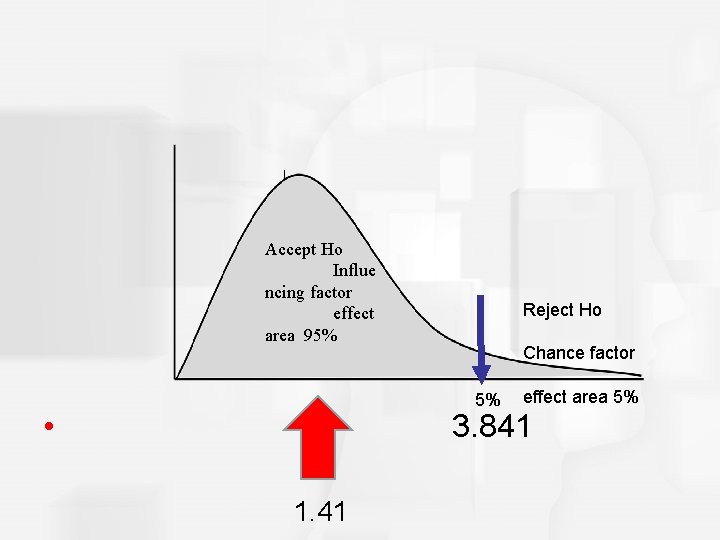
Accept Ho Influe ncing factor effect area 95% 5% Reject Ho Chance factor effect area 5% • 3. 841 1. 41
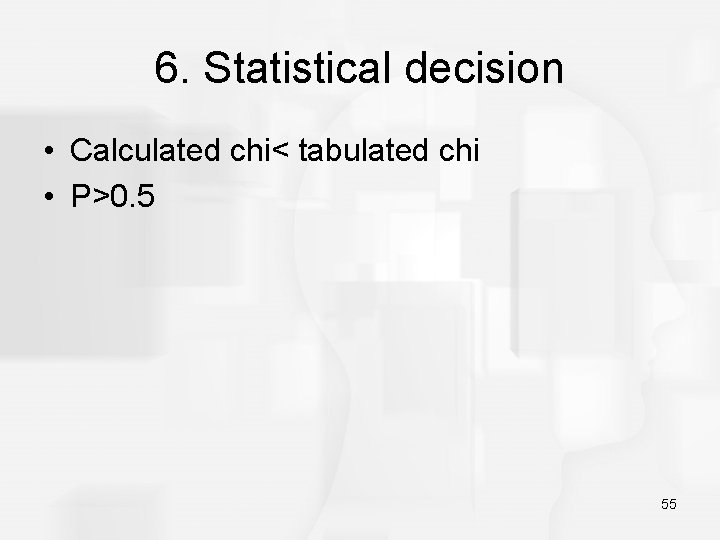
6. Statistical decision • Calculated chi< tabulated chi • P>0. 5 55
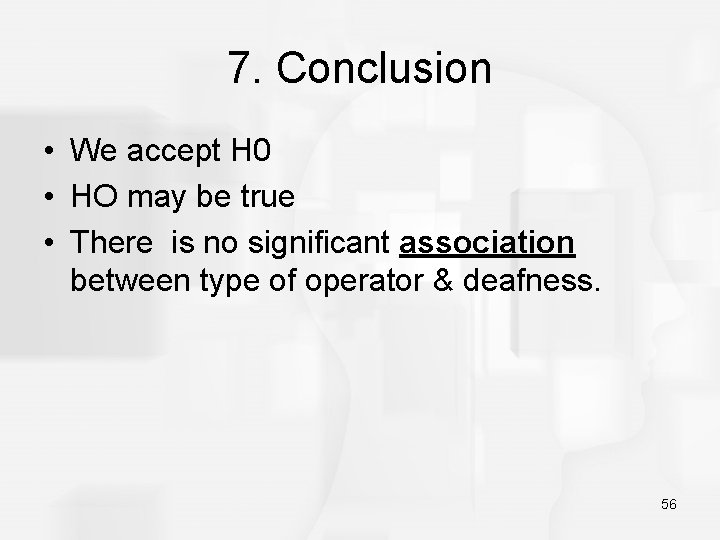
7. Conclusion • We accept H 0 • HO may be true • There is no significant association between type of operator & deafness. 56
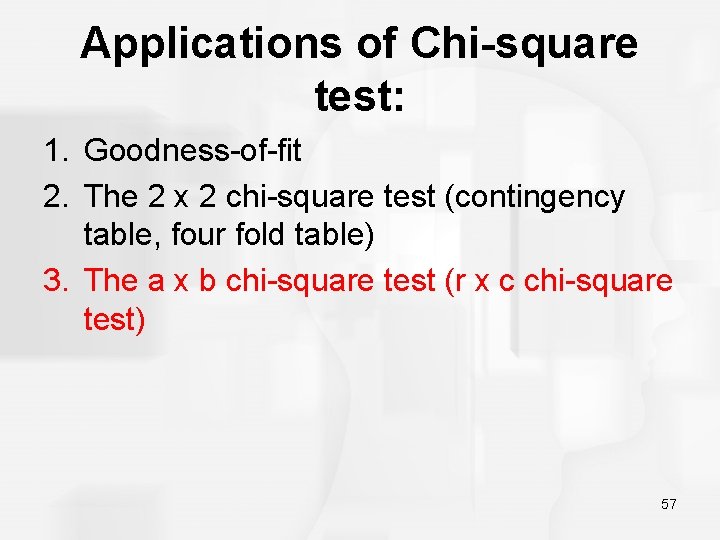
Applications of Chi-square test: 1. Goodness-of-fit 2. The 2 x 2 chi-square test (contingency table, four fold table) 3. The a x b chi-square test (r x c chi-square test) 57
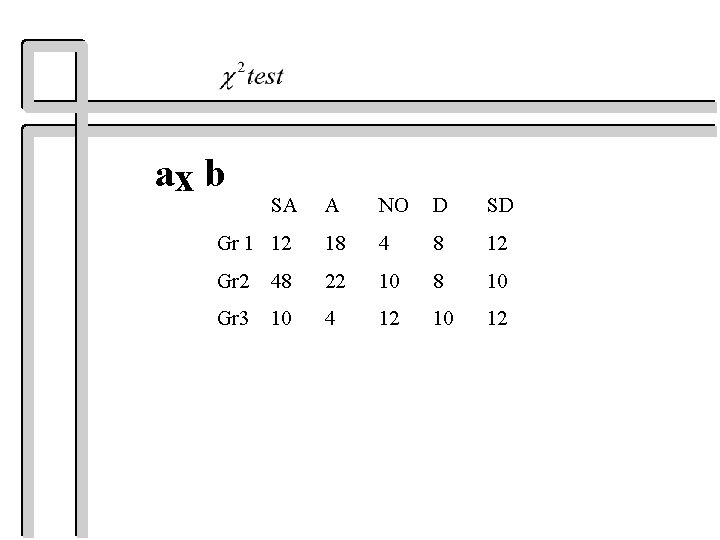
ₓ a b SA A NO D SD Gr 1 12 18 4 8 12 Gr 2 48 22 10 8 10 Gr 3 10 4 12 10 12
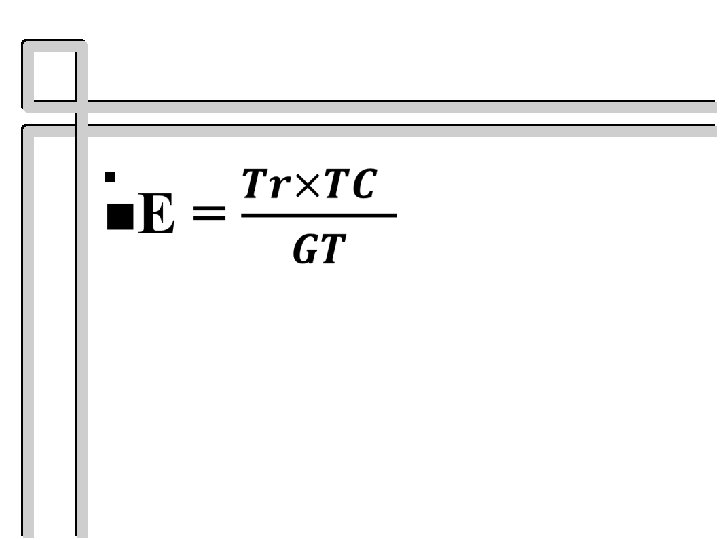
n
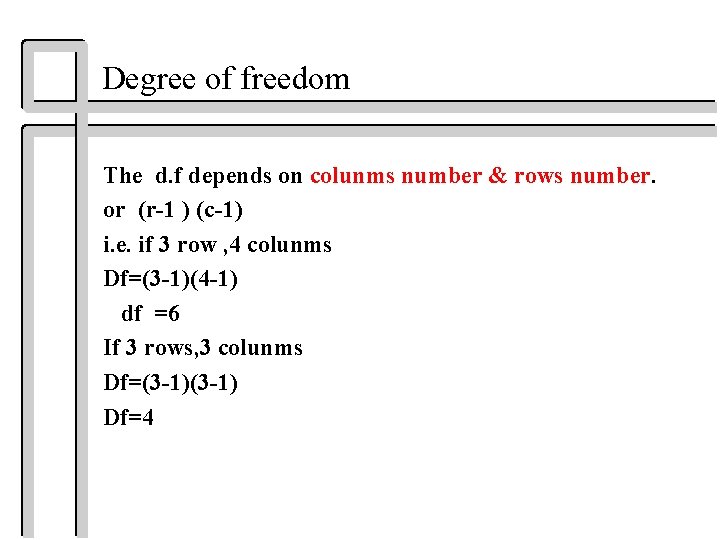
Degree of freedom The d. f depends on colunms number & rows number. or (r-1 ) (c-1) i. e. if 3 row , 4 colunms Df=(3 -1)(4 -1) df =6 If 3 rows, 3 colunms Df=(3 -1) Df=4
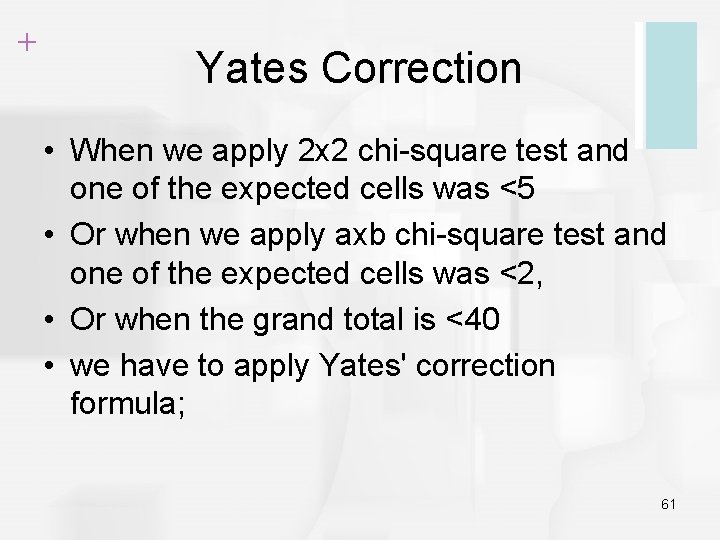
+ Yates Correction • When we apply 2 x 2 chi-square test and one of the expected cells was <5 • Or when we apply axb chi-square test and one of the expected cells was <2, • Or when the grand total is <40 • we have to apply Yates' correction formula; 61
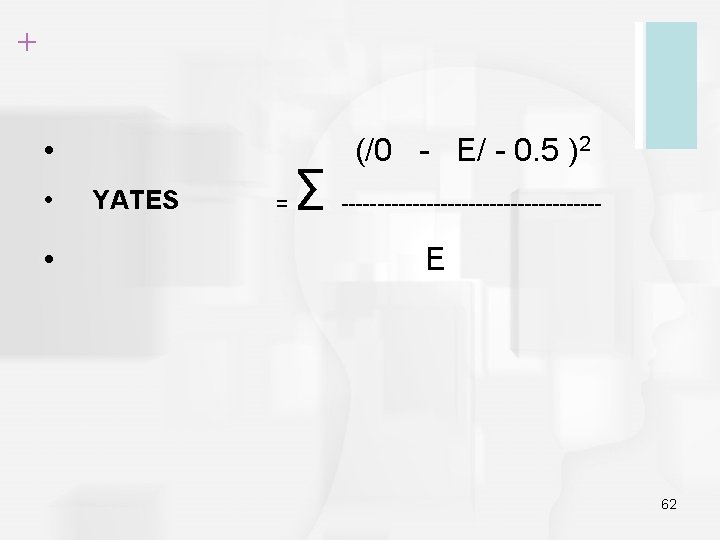
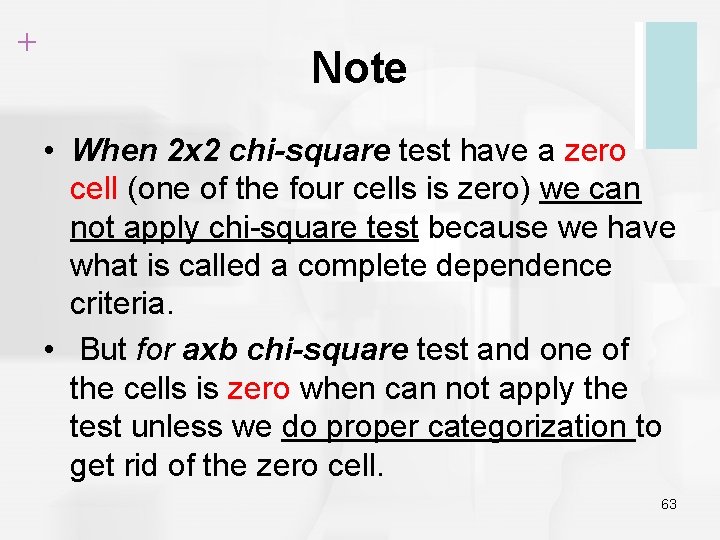
+ Note • When 2 x 2 chi-square test have a zero cell (one of the four cells is zero) we can not apply chi-square test because we have what is called a complete dependence criteria. • But for axb chi-square test and one of the cells is zero when can not apply the test unless we do proper categorization to get rid of the zero cell. 63
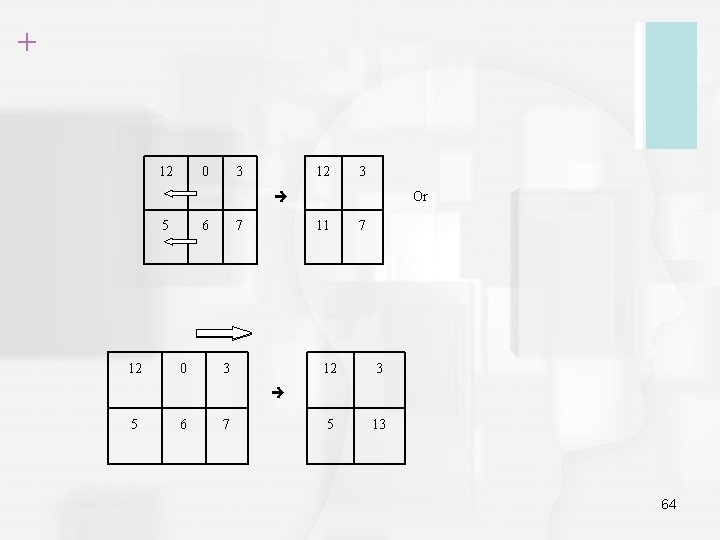
+ 12 0 3 12 6 0 7 3 11 12 7 3 6 7 5 Or 5 5 13 64
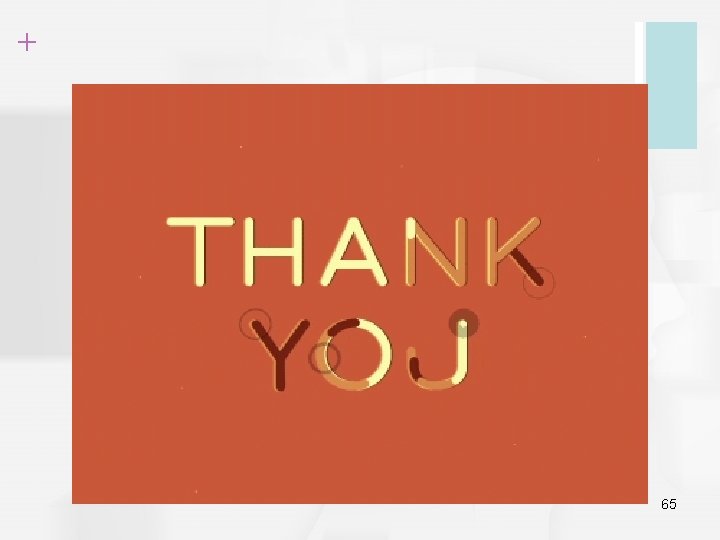
+ 65
Chi-square symbol
Hình ảnh bộ gõ cơ thể búng tay
Lp html
Bổ thể
Tỉ lệ cơ thể trẻ em
Voi kéo gỗ như thế nào
Tư thế worm breton
Chúa sống lại
Kể tên các môn thể thao
Thế nào là hệ số cao nhất
Các châu lục và đại dương trên thế giới
Công thức tính độ biến thiên đông lượng
Trời xanh đây là của chúng ta thể thơ
Mật thư anh em như thể tay chân
Phép trừ bù
Phản ứng thế ankan
Các châu lục và đại dương trên thế giới
Thơ thất ngôn tứ tuyệt đường luật
Quá trình desamine hóa có thể tạo ra
Một số thể thơ truyền thống
Cái miệng nó xinh thế chỉ nói điều hay thôi
Vẽ hình chiếu vuông góc của vật thể sau
Nguyên nhân của sự mỏi cơ sinh 8
đặc điểm cơ thể của người tối cổ
Thế nào là giọng cùng tên
Vẽ hình chiếu đứng bằng cạnh của vật thể
Fecboak
Thẻ vin
đại từ thay thế
điện thế nghỉ
Tư thế ngồi viết
Diễn thế sinh thái là
Dot
Số nguyên tố là
Tư thế ngồi viết
Lời thề hippocrates
Thiếu nhi thế giới liên hoan
ưu thế lai là gì
Khi nào hổ con có thể sống độc lập
Khi nào hổ mẹ dạy hổ con săn mồi
Sơ đồ cơ thể người
Từ ngữ thể hiện lòng nhân hậu
Thế nào là mạng điện lắp đặt kiểu nổi
What are writing conventions
Workkeys applied mathematics level 4 answers
What credential is issued by the danb
Fglair google home europe
Rop medical assisting
Promotion from associate professor to professor
Mia irwan
Cisco sbcs
Kpu health care assistant
Virtually there e ticket receipt
Cooperative interview questions
Ibm software and support
Recording assistant hikvision
Rog4d
Cuhk salary scale 2020
Scorer and assistant scorer
Assistant district commissioner scouts
Mrs rajlaxmi is working
Cashflow assistant
Anwser
Attribute assistant arcmap
Shop assistant good evening what can i do for you
Assistant head girl manifesto