COPLINK A National Model for Law Enforcement Information
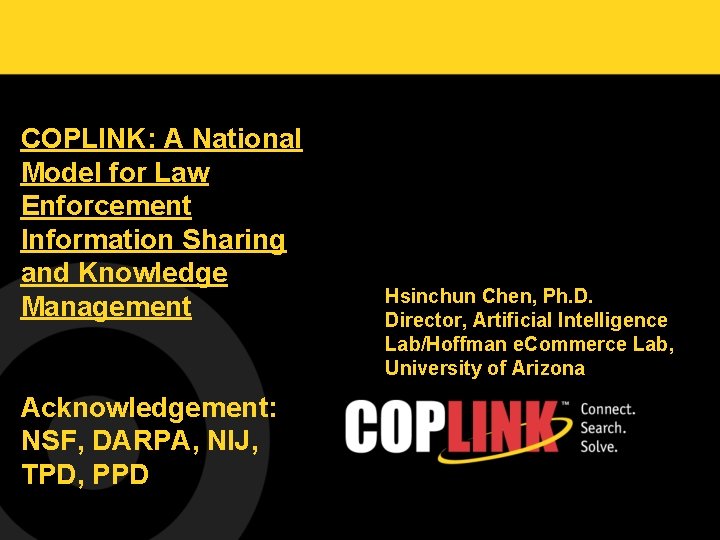
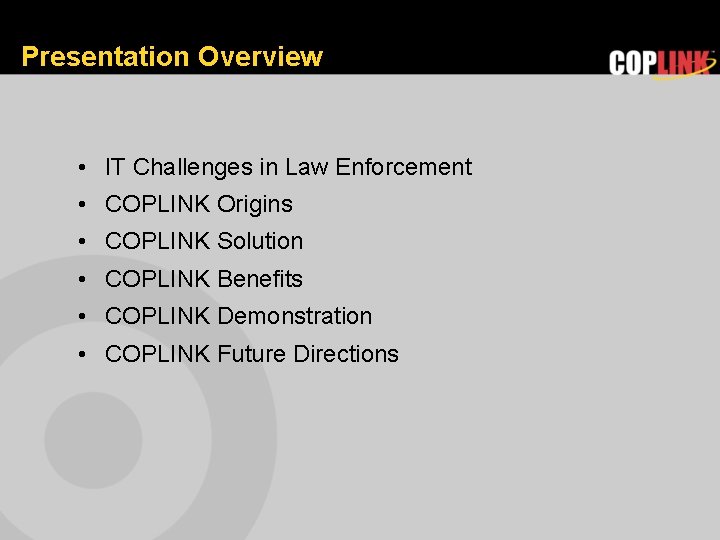
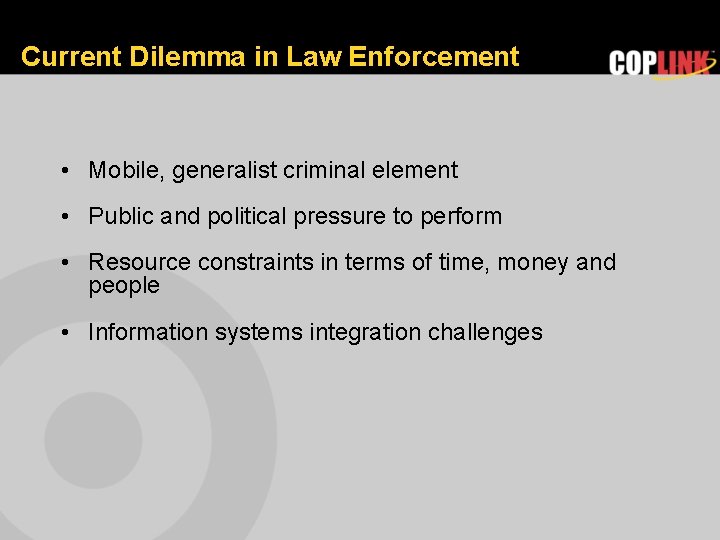
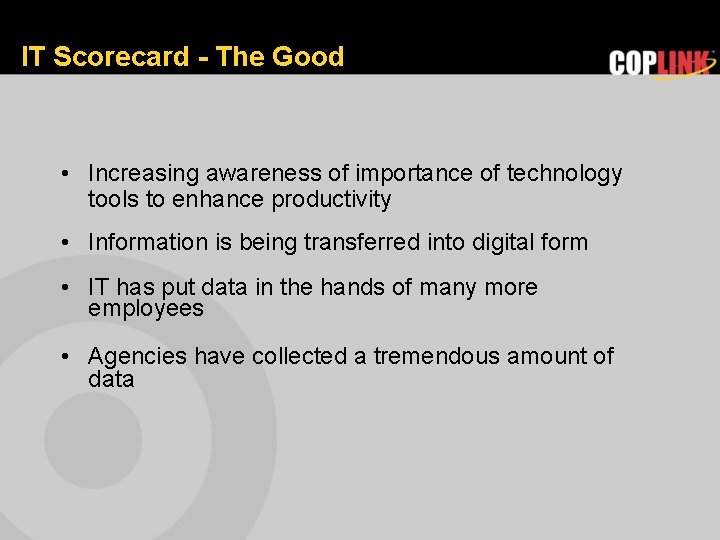
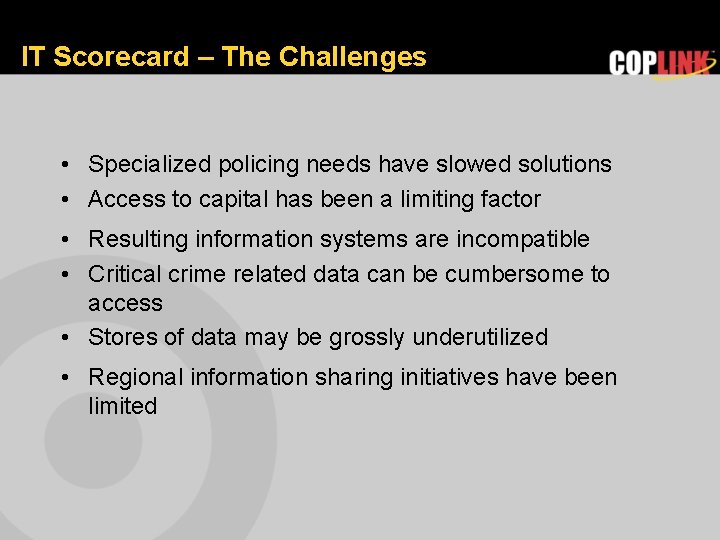
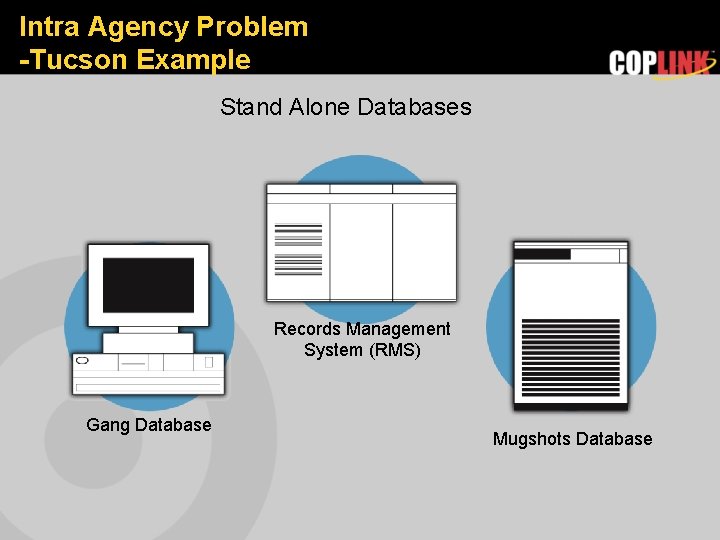
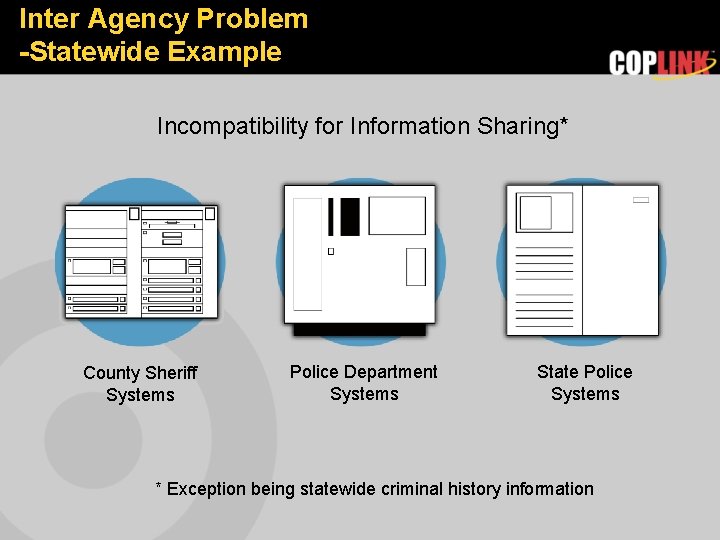
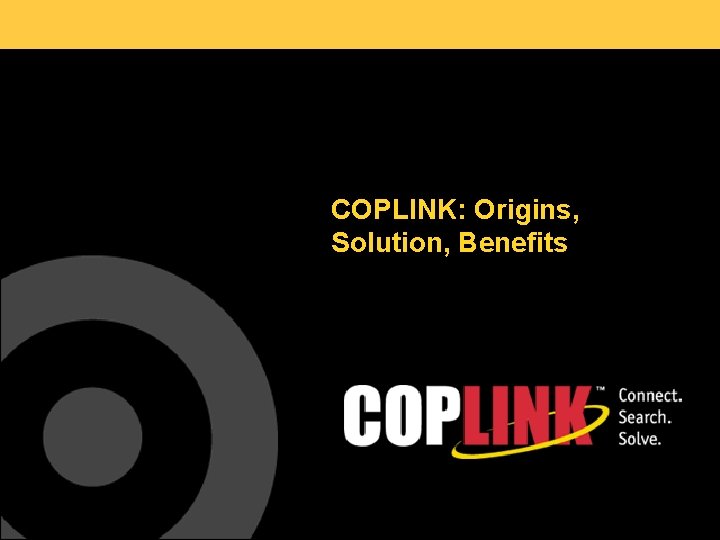
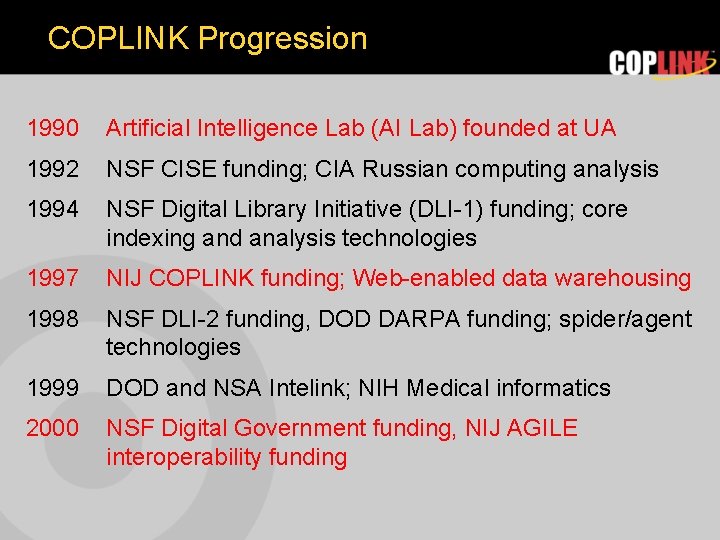
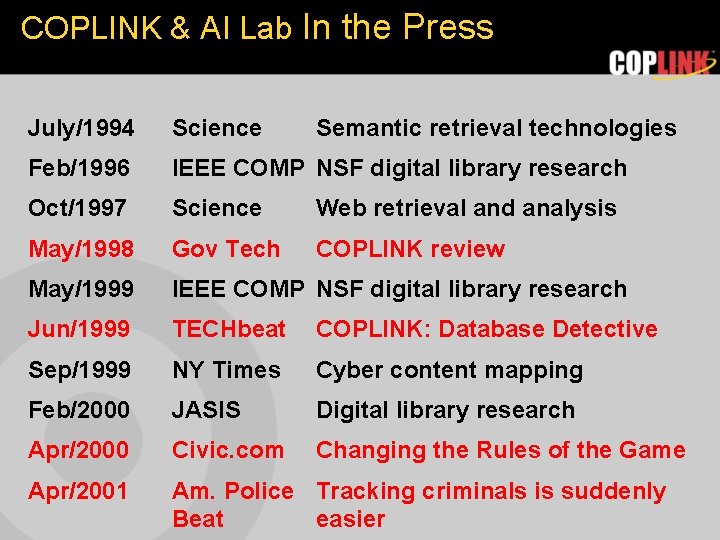
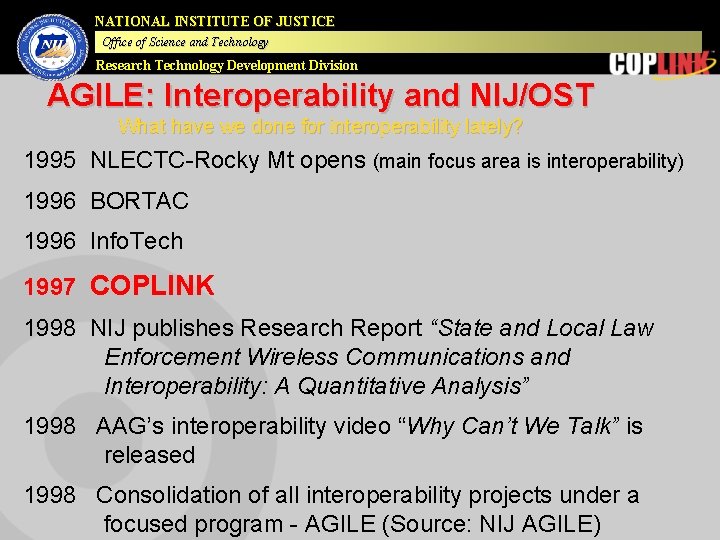
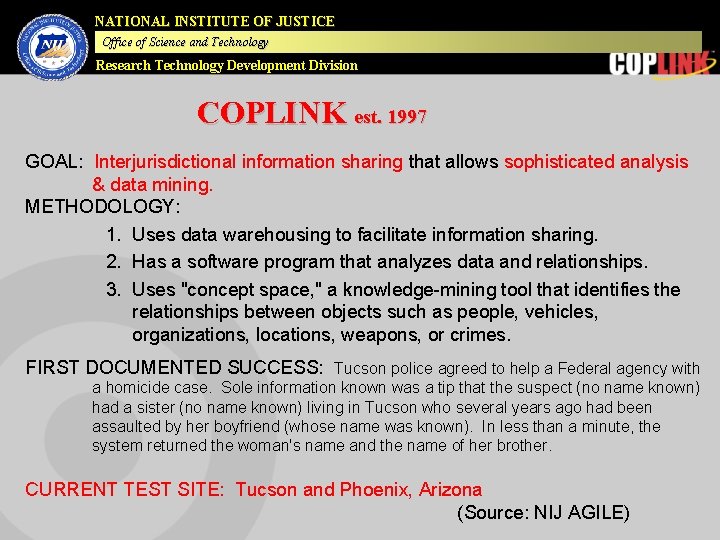
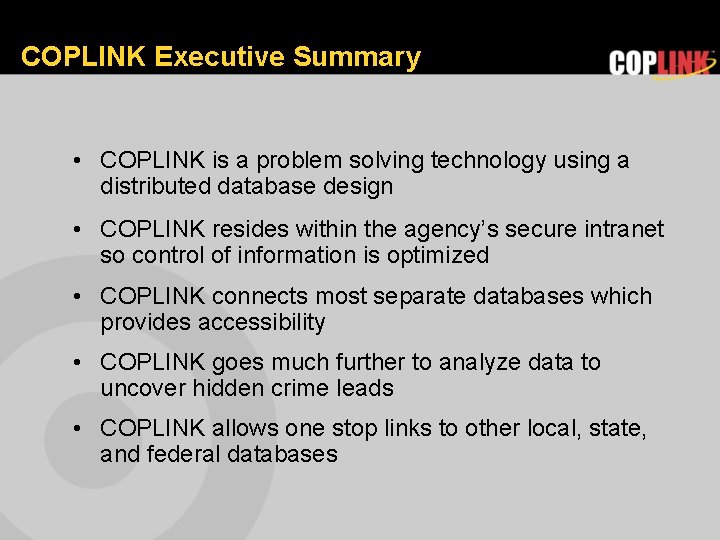
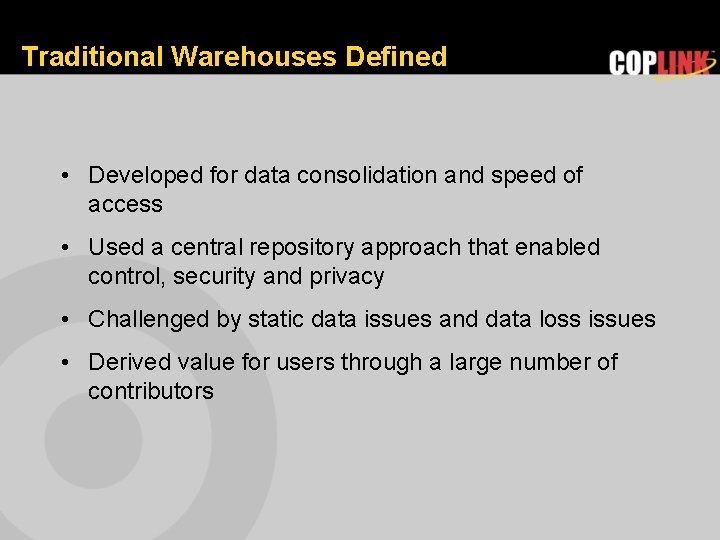
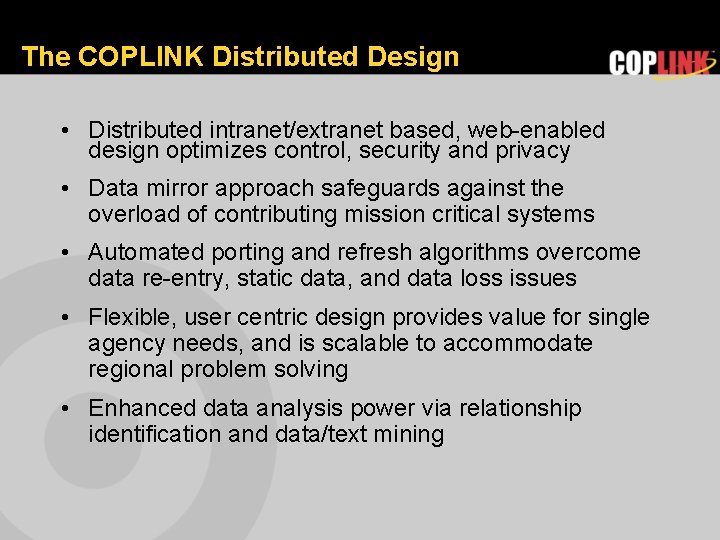
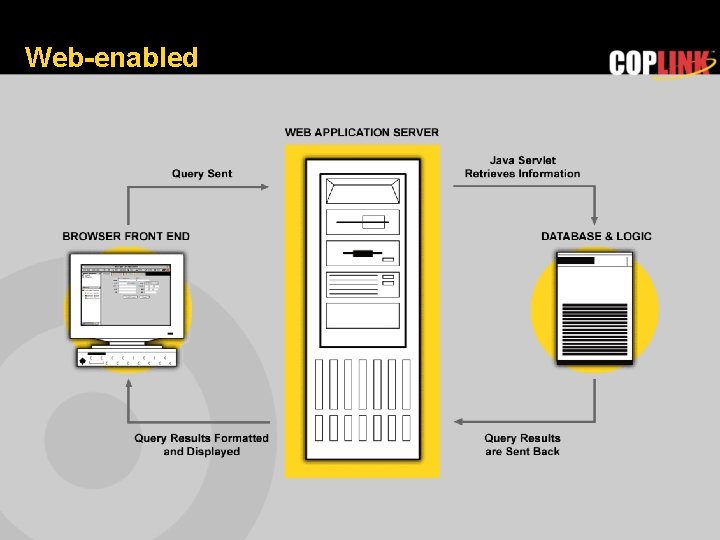
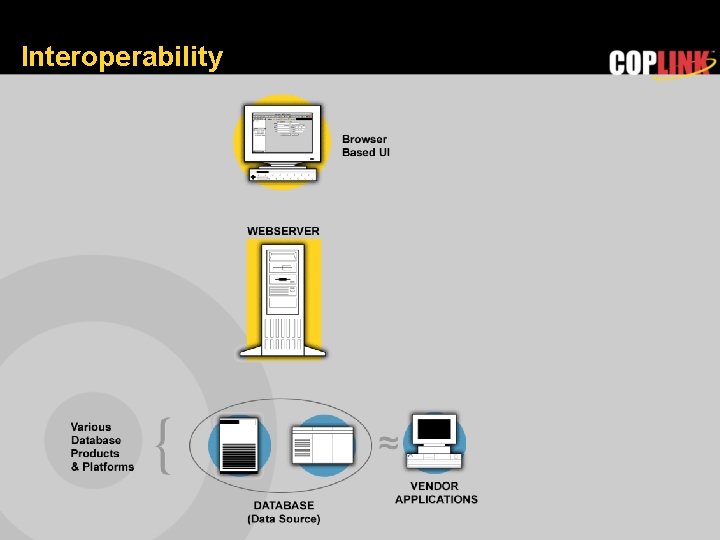
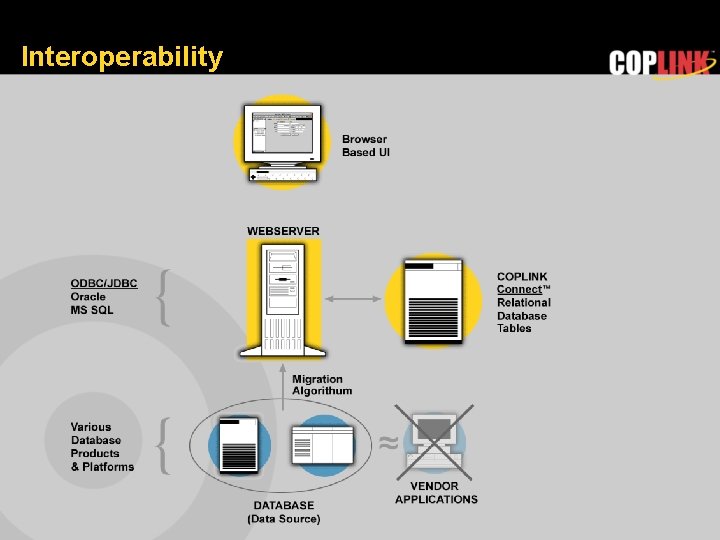
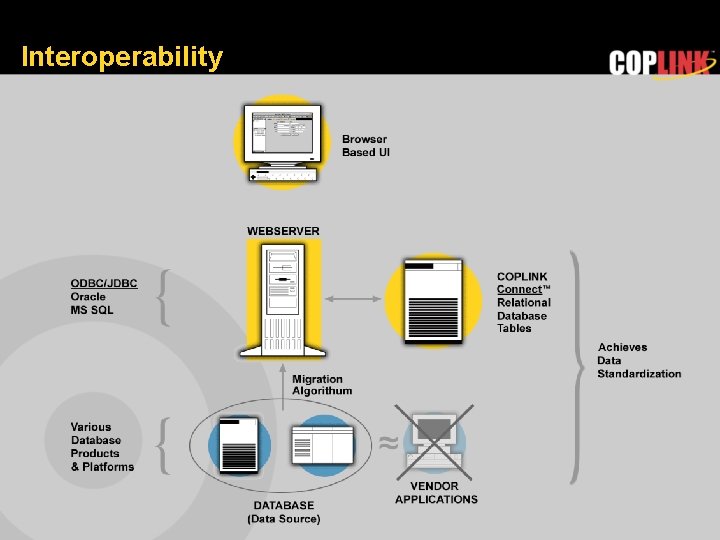
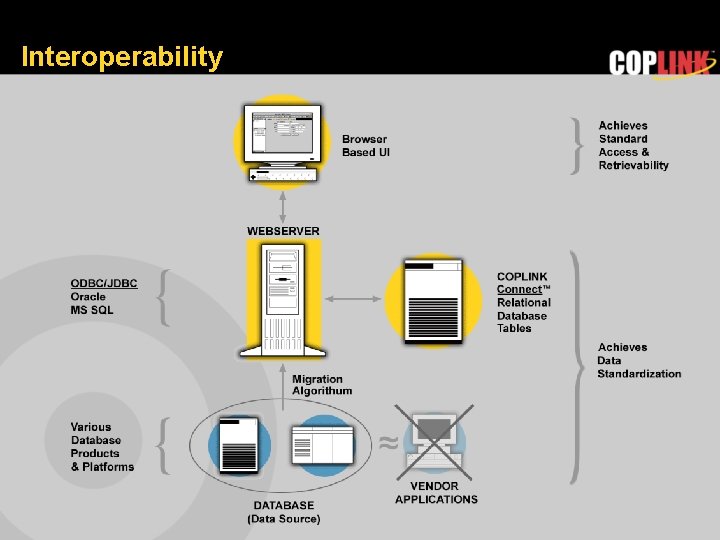
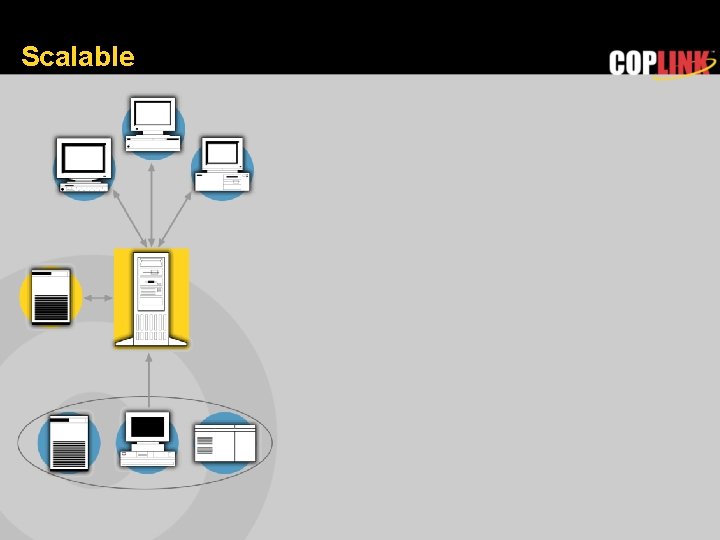
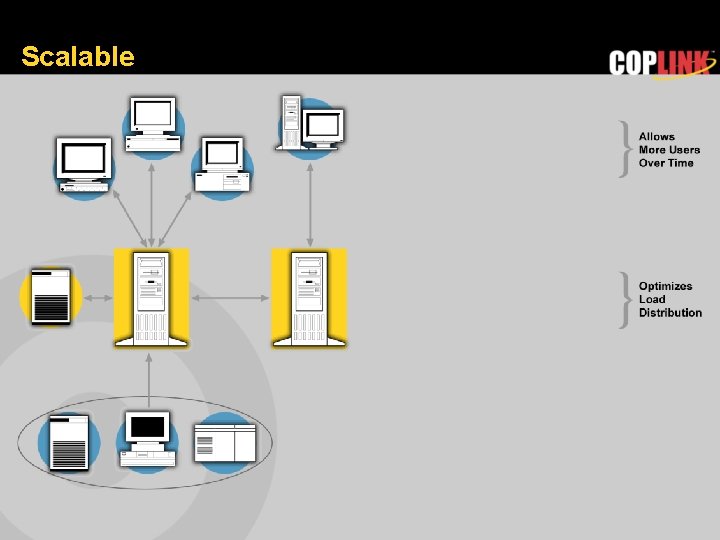
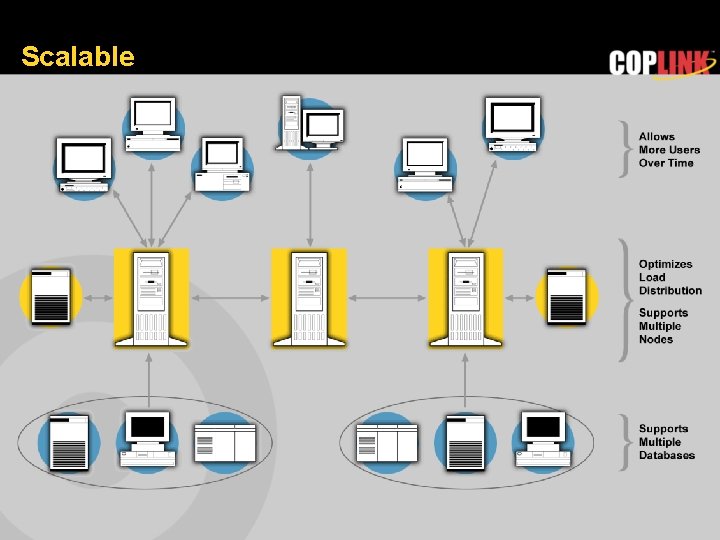
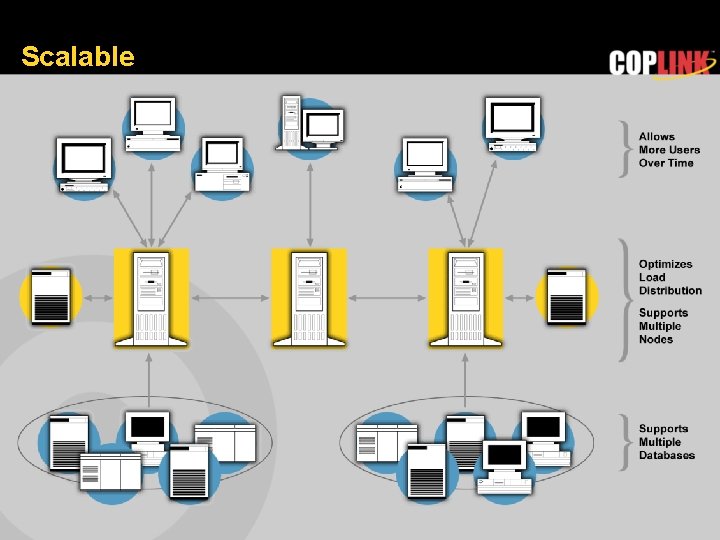
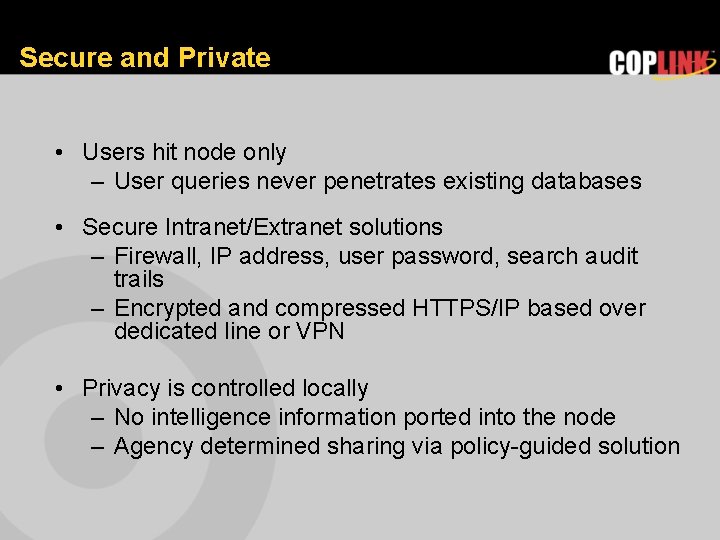
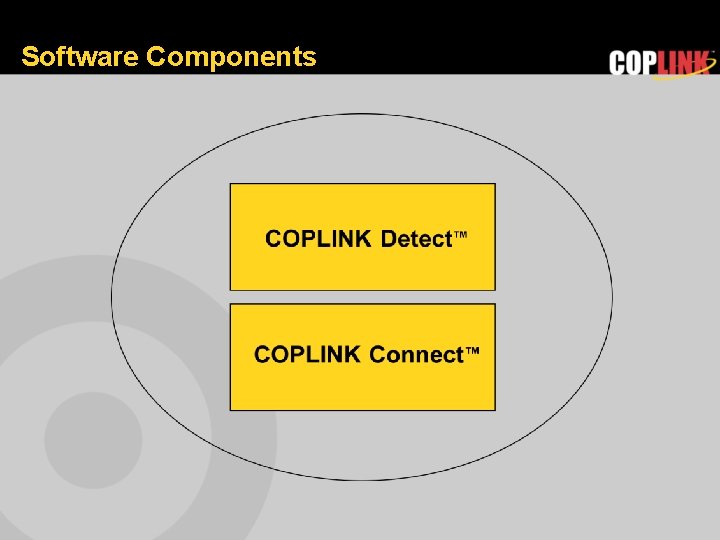
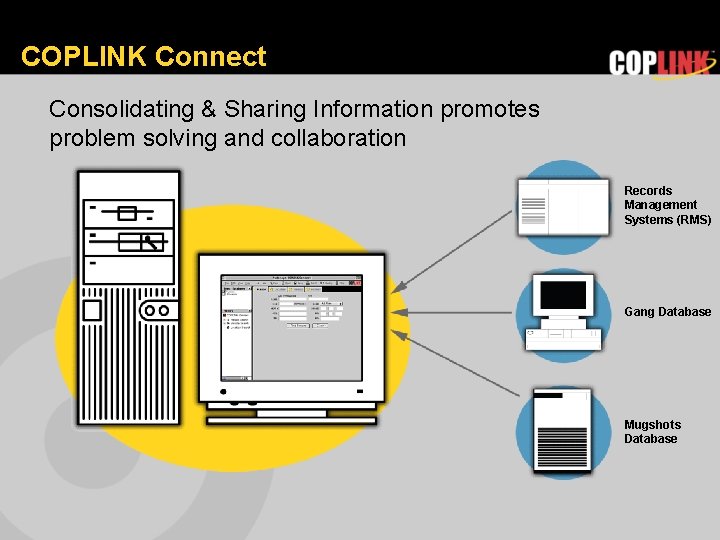
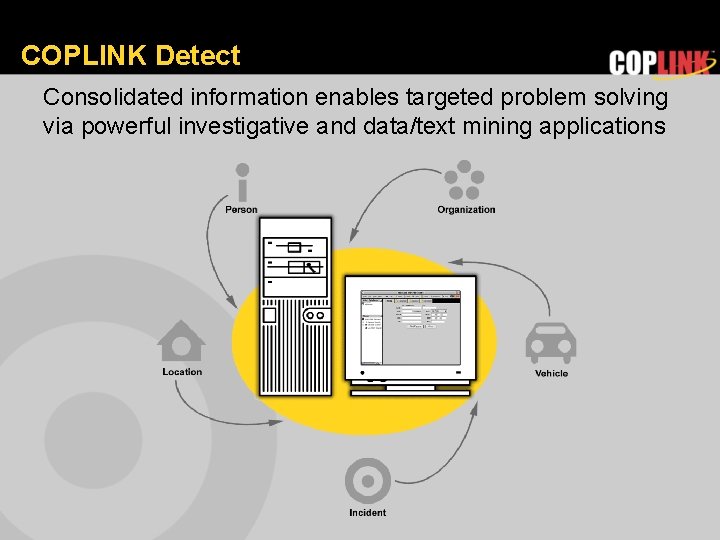
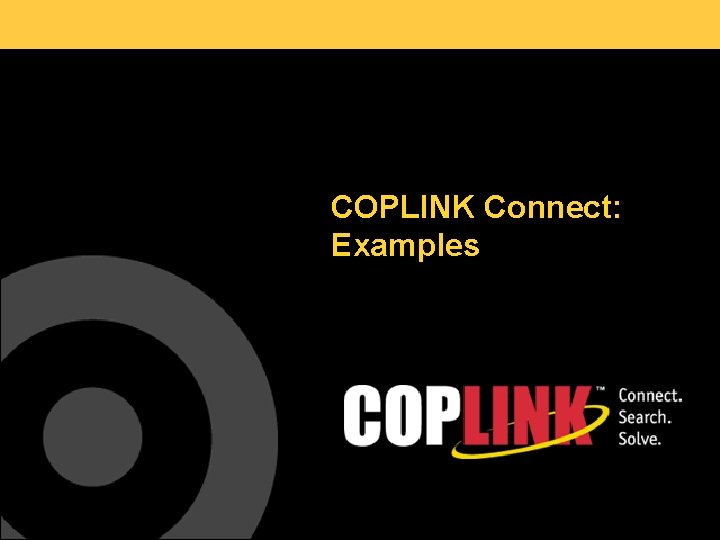
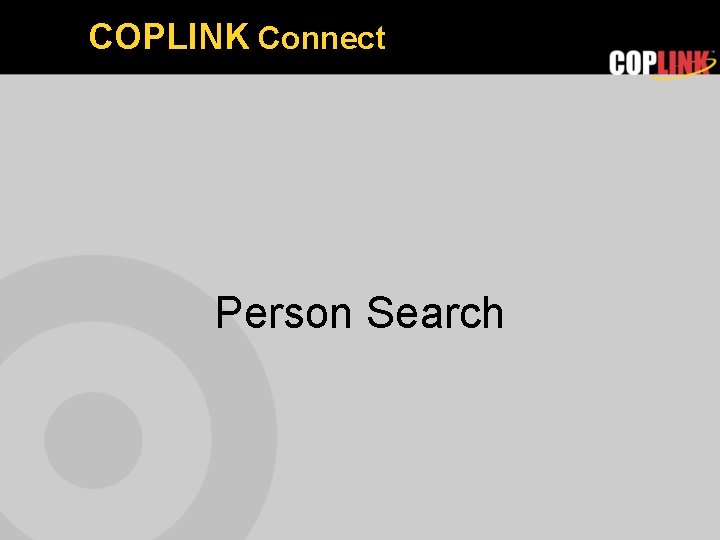
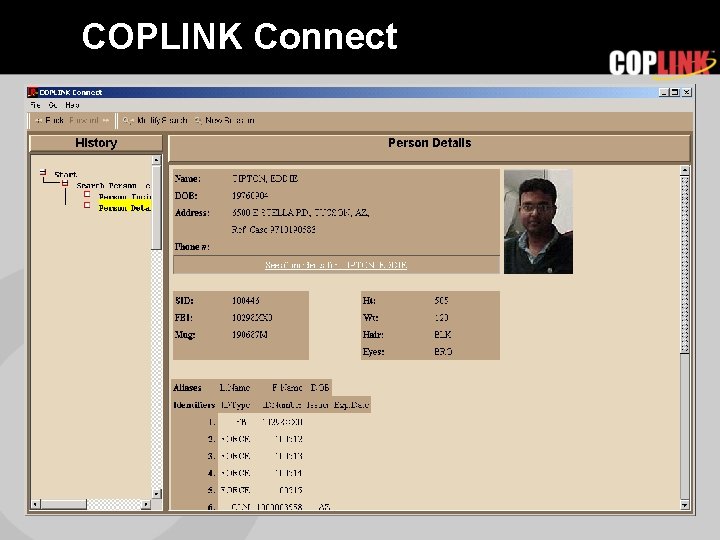
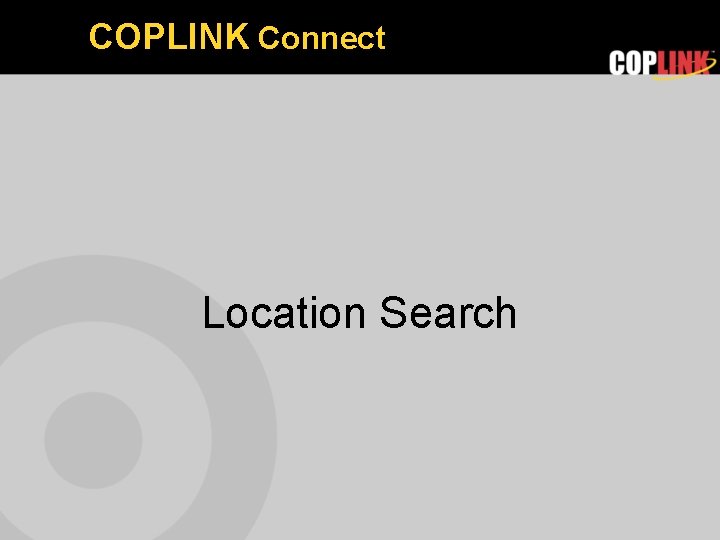
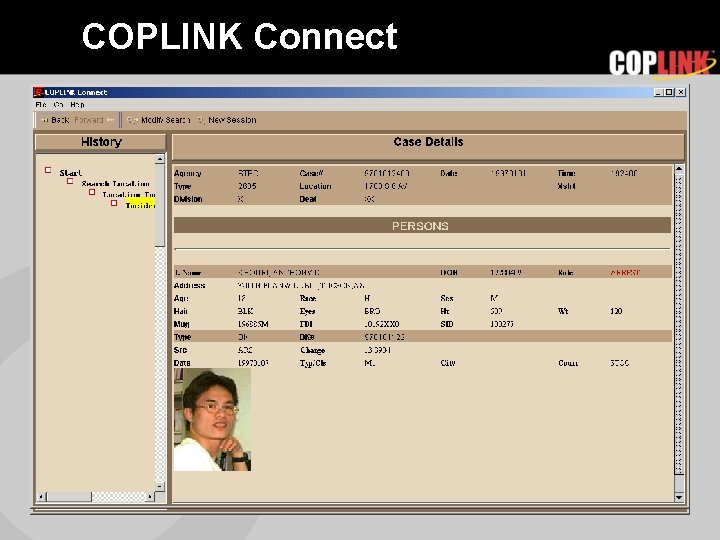
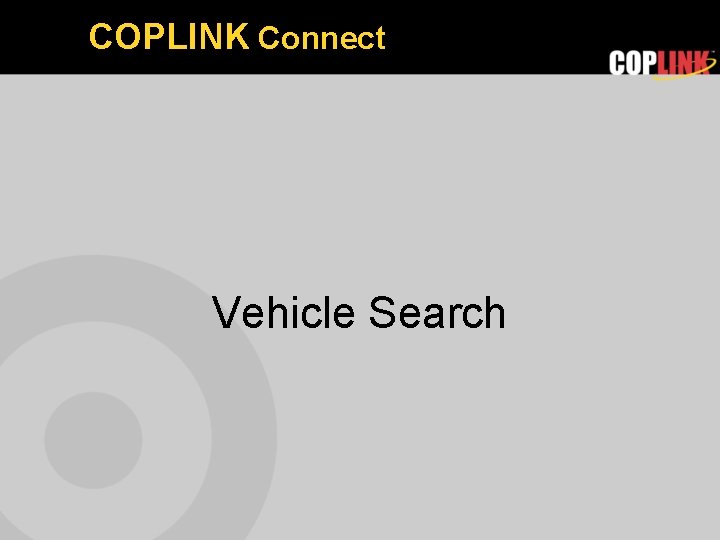
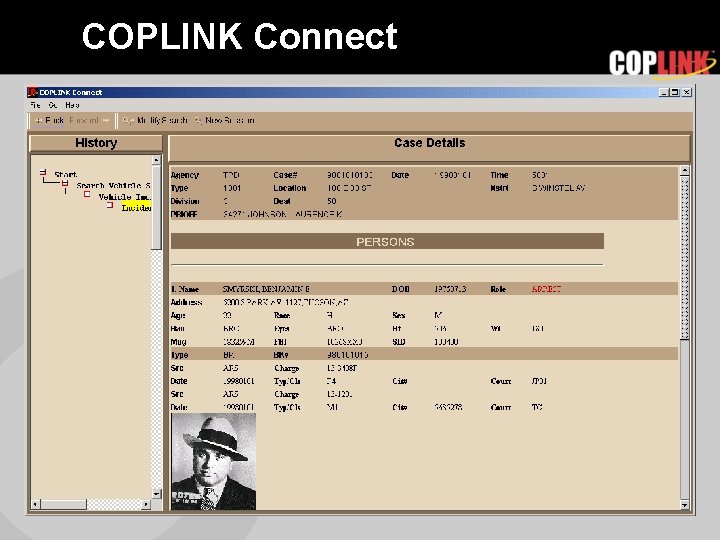
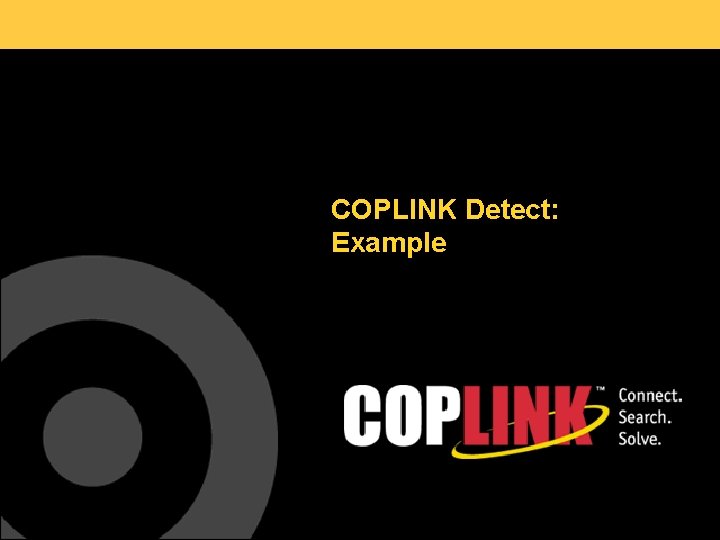
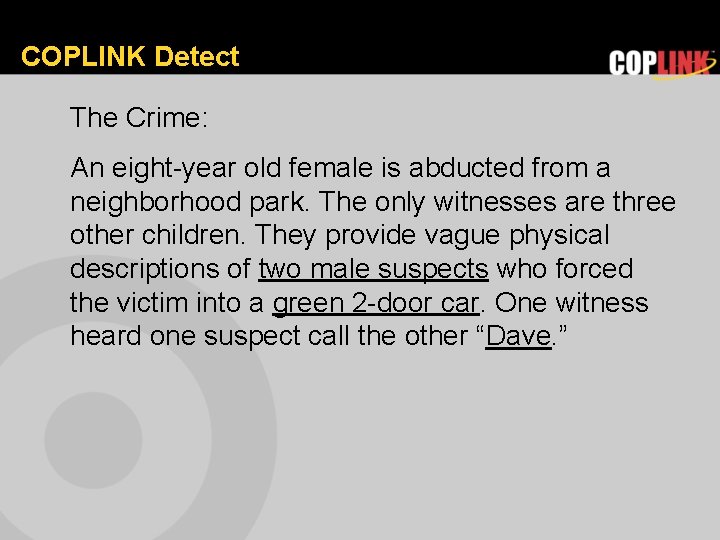
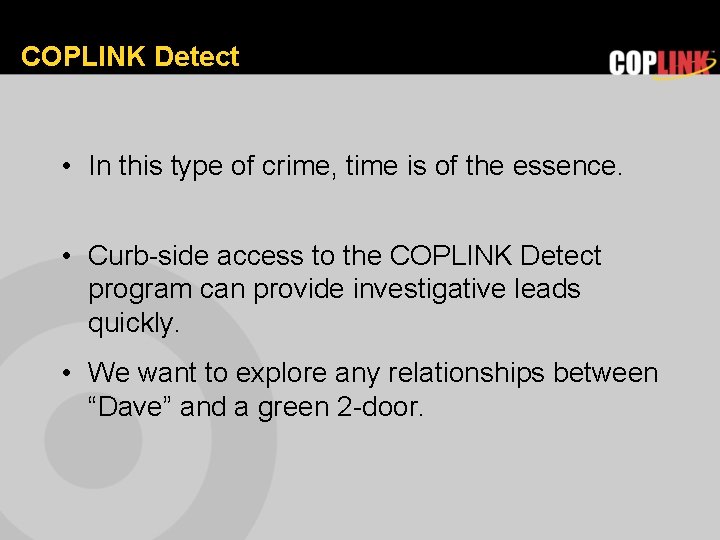
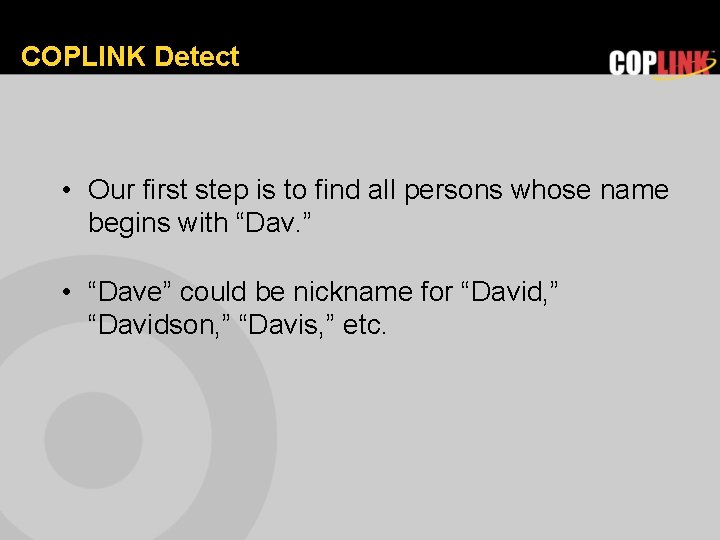
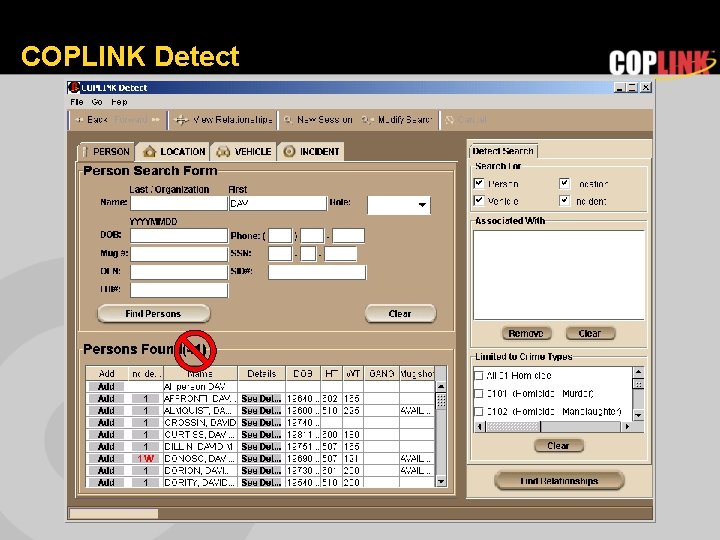
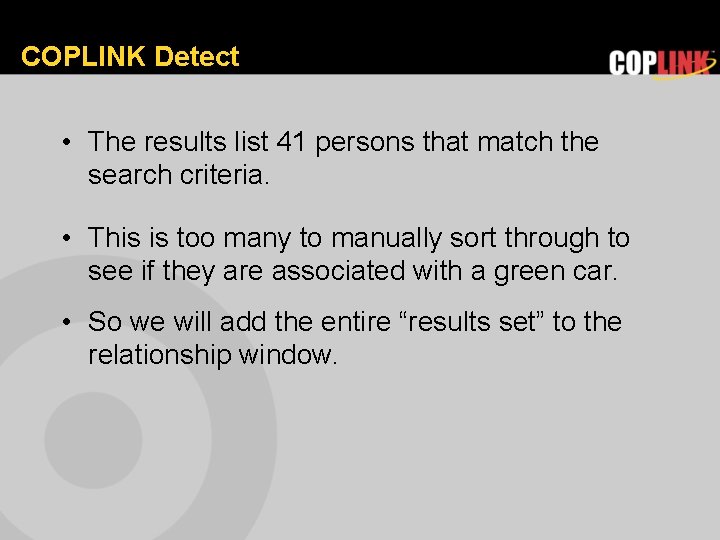
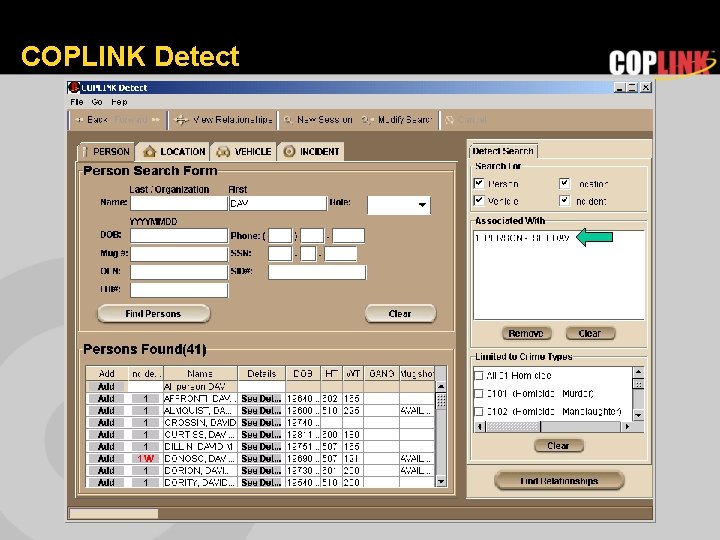
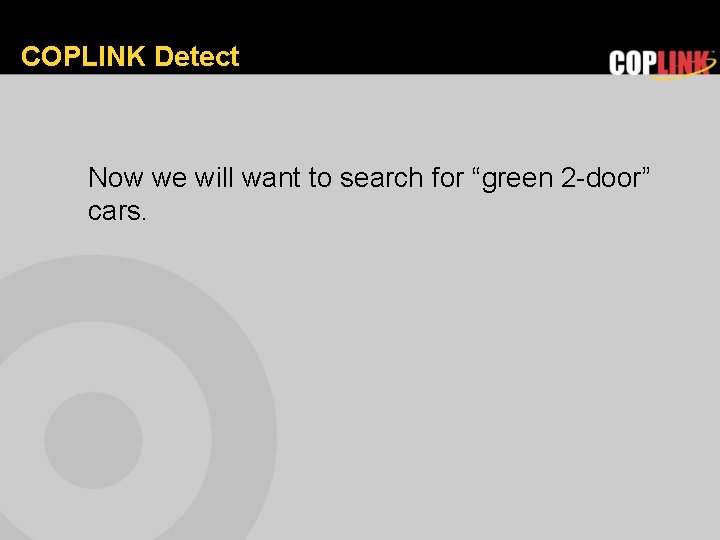
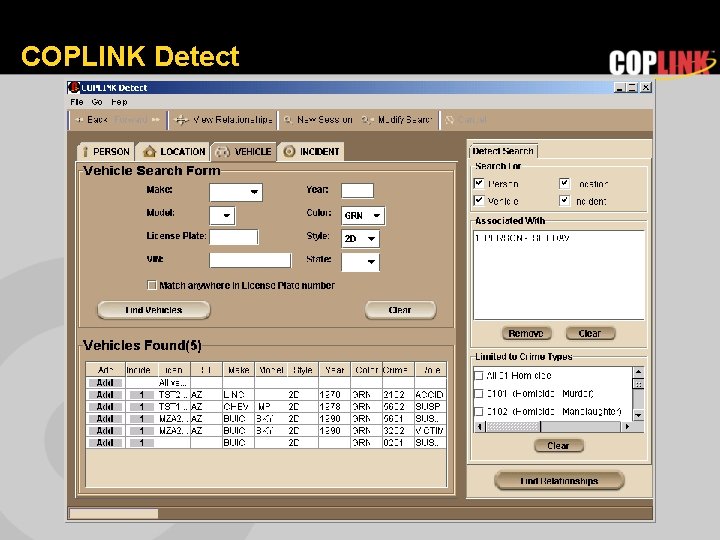
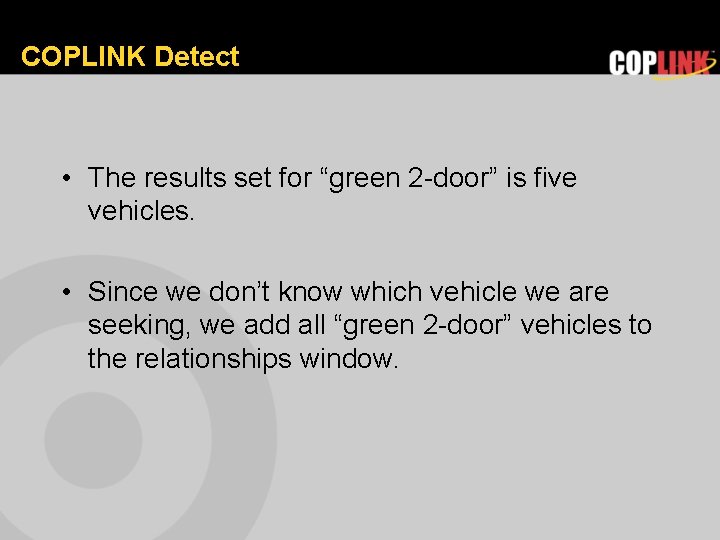
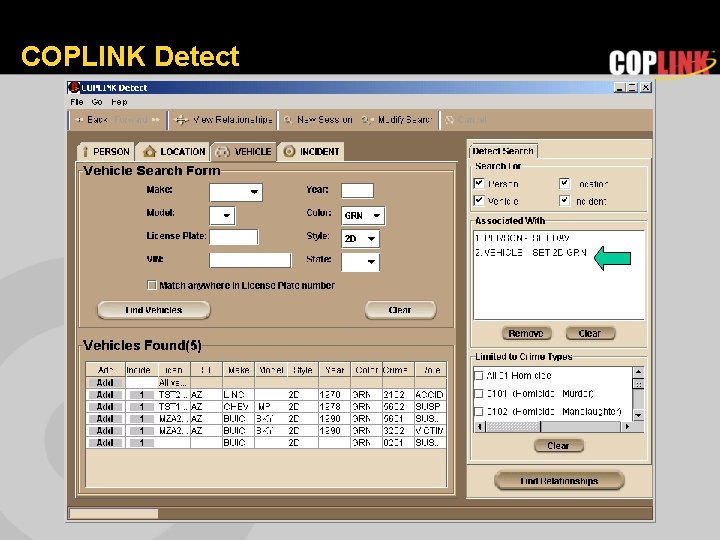
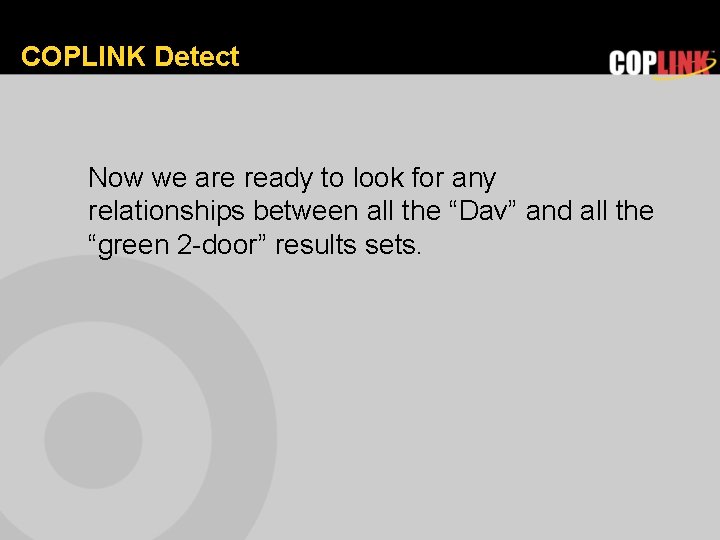
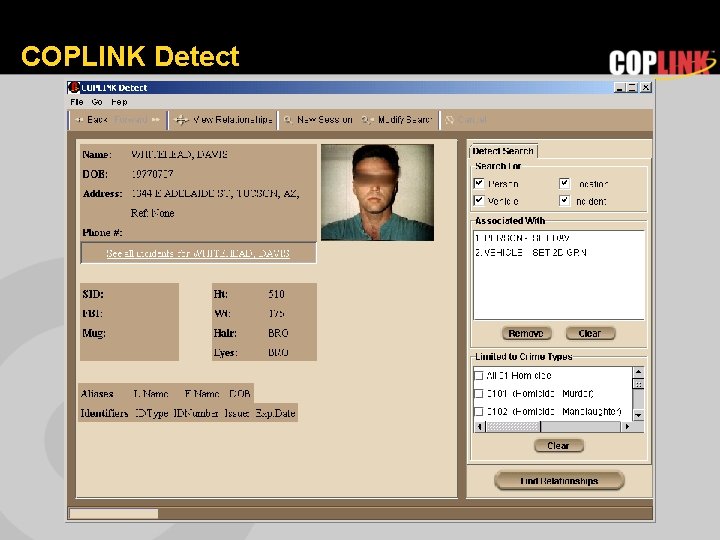
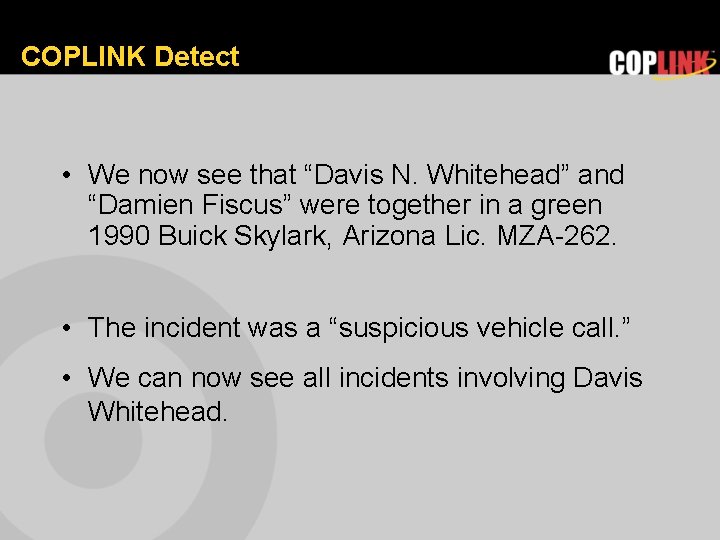
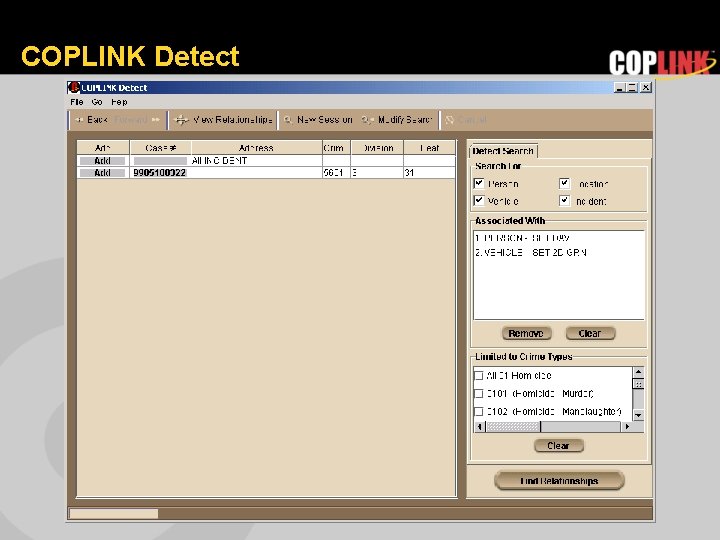
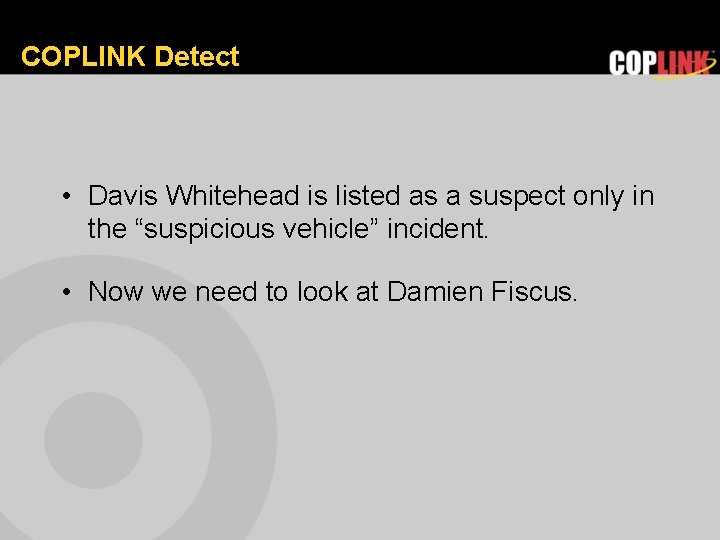
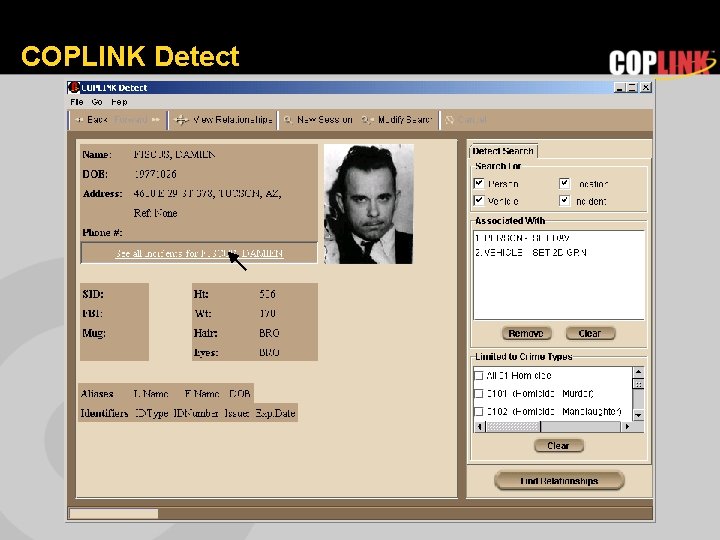
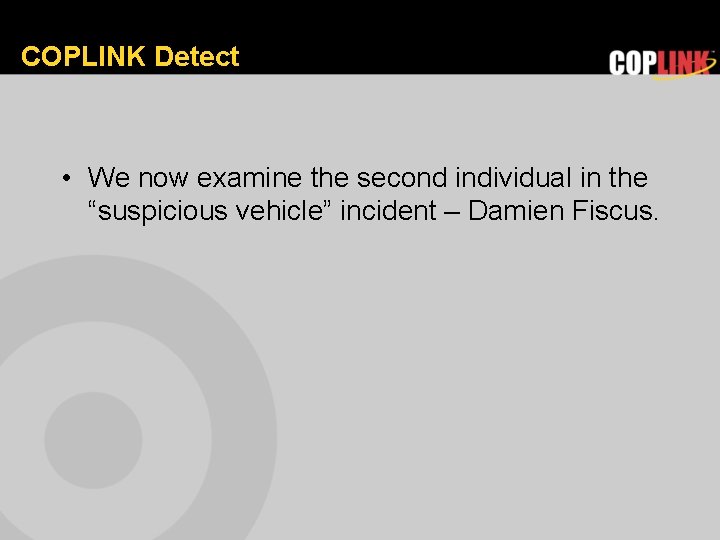
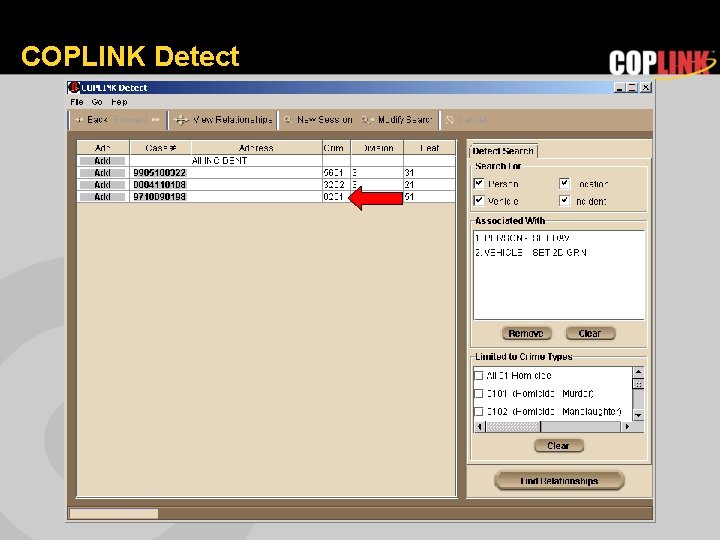
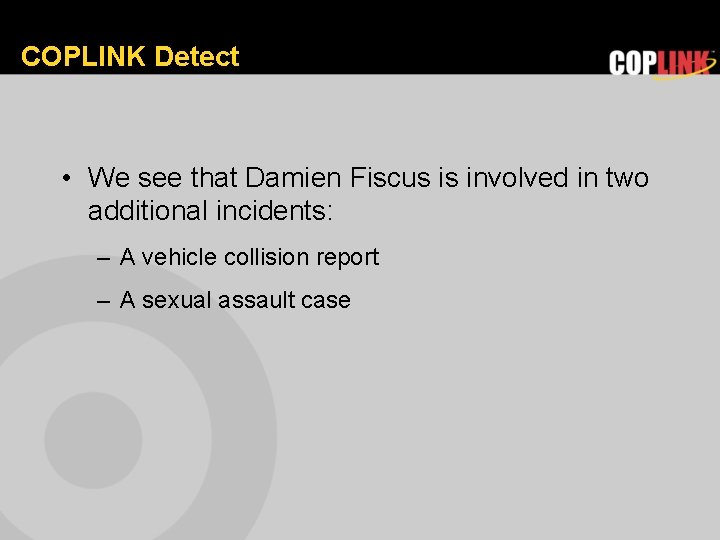
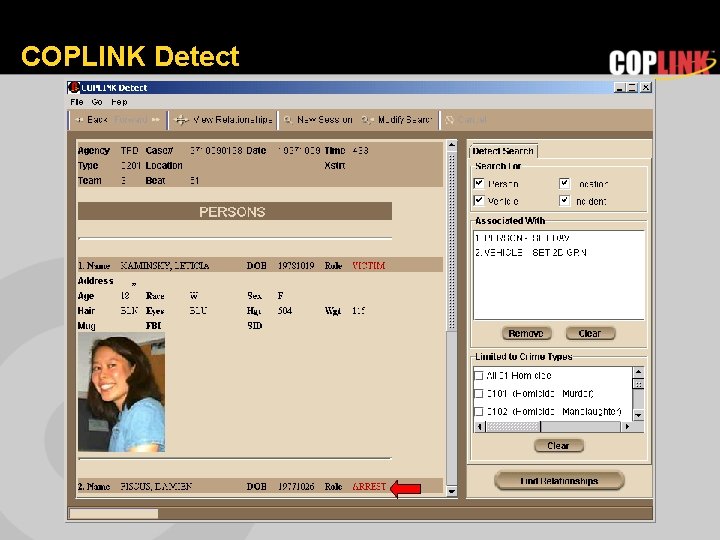
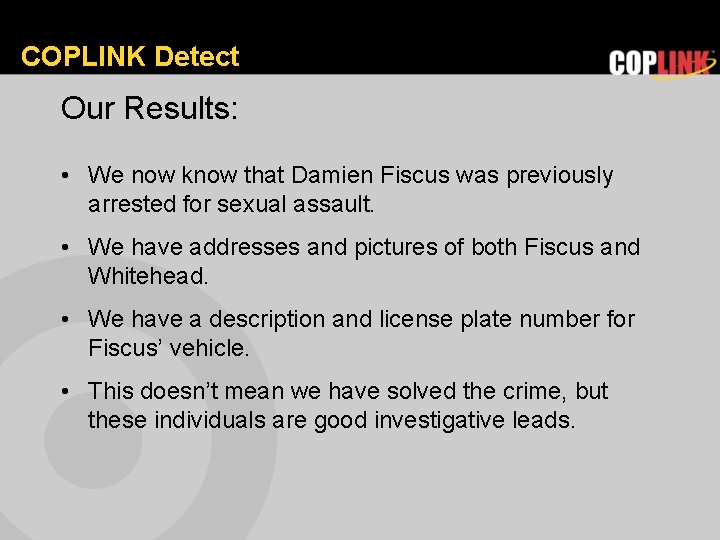
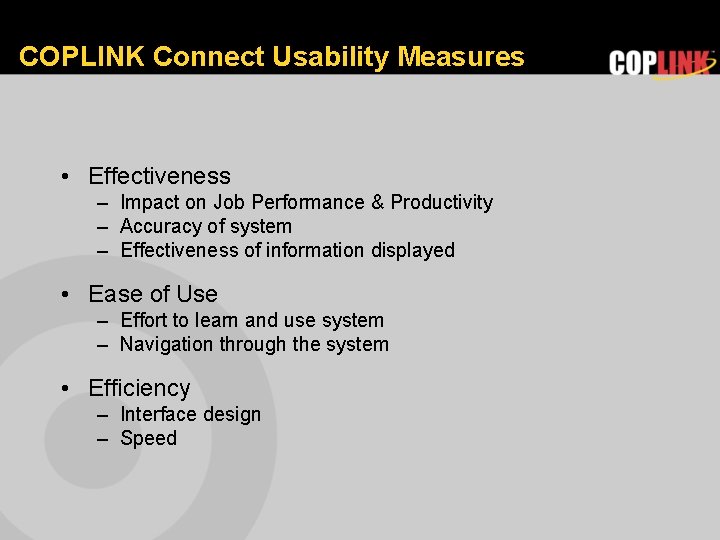
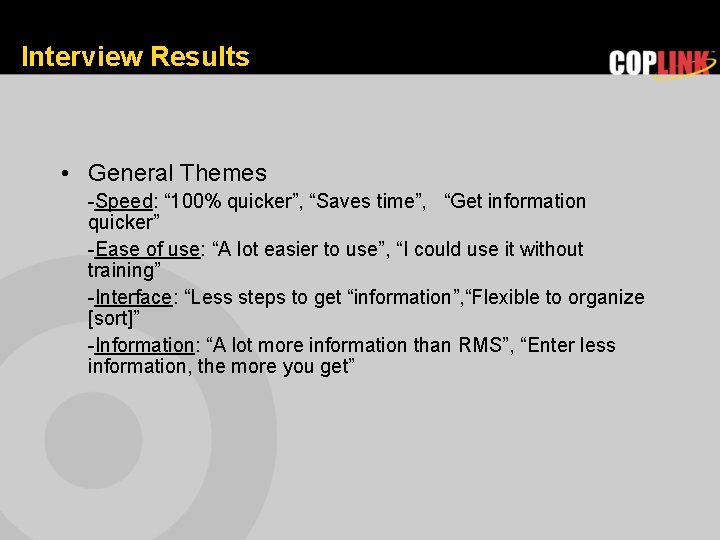
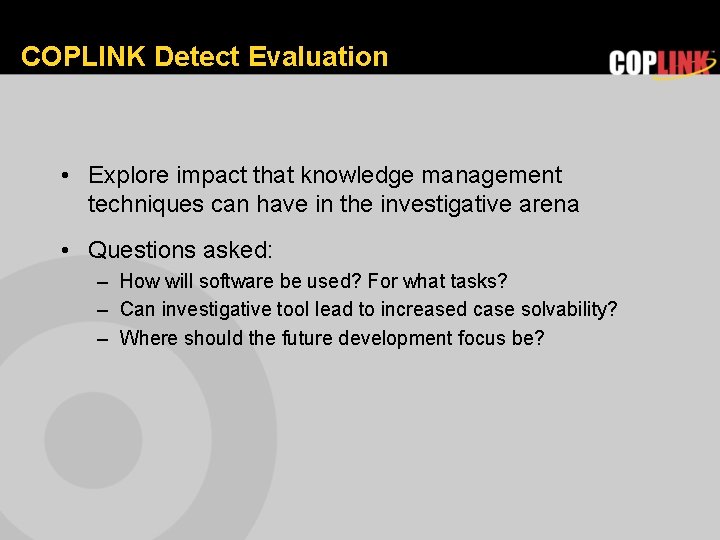
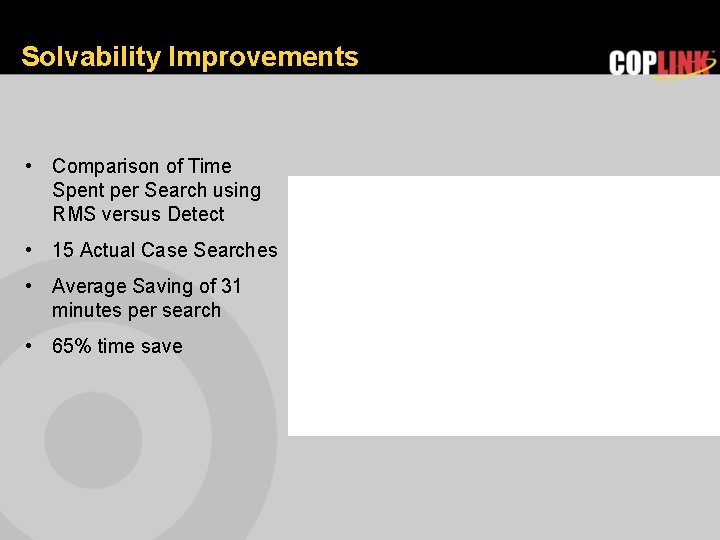
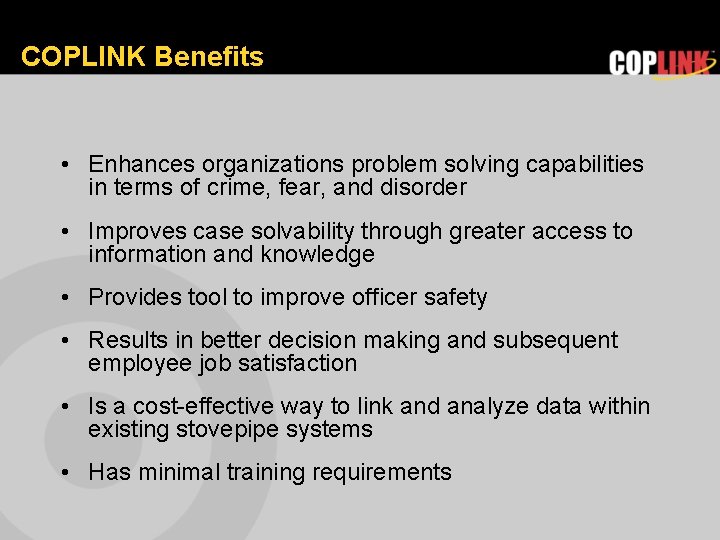
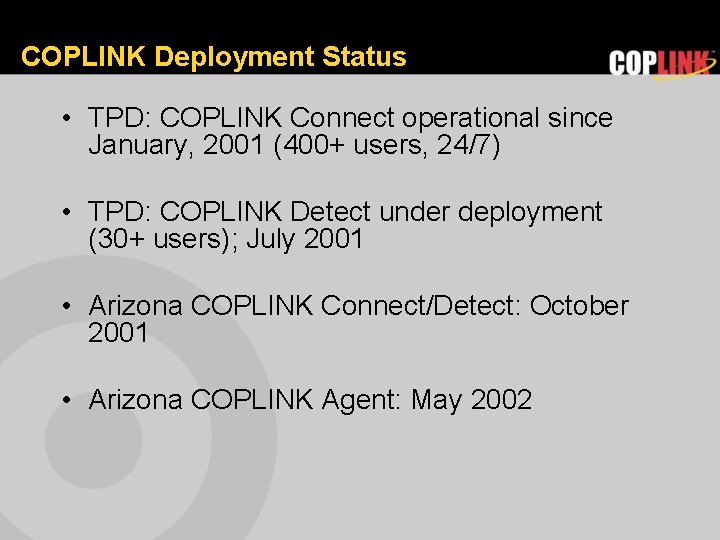
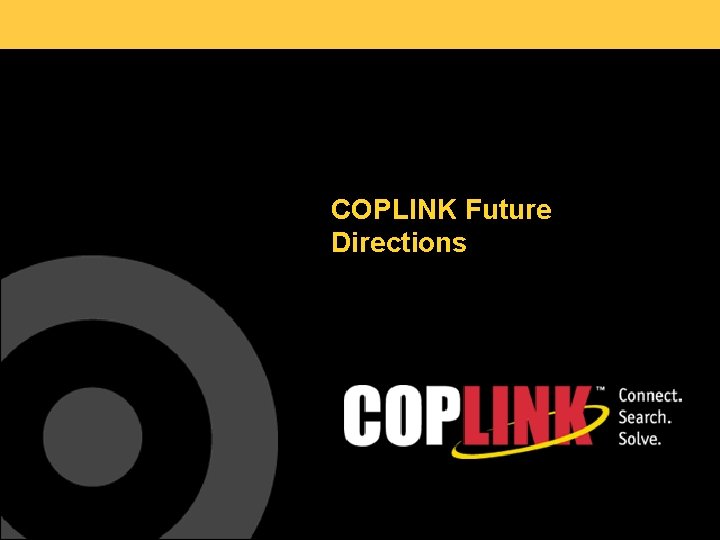
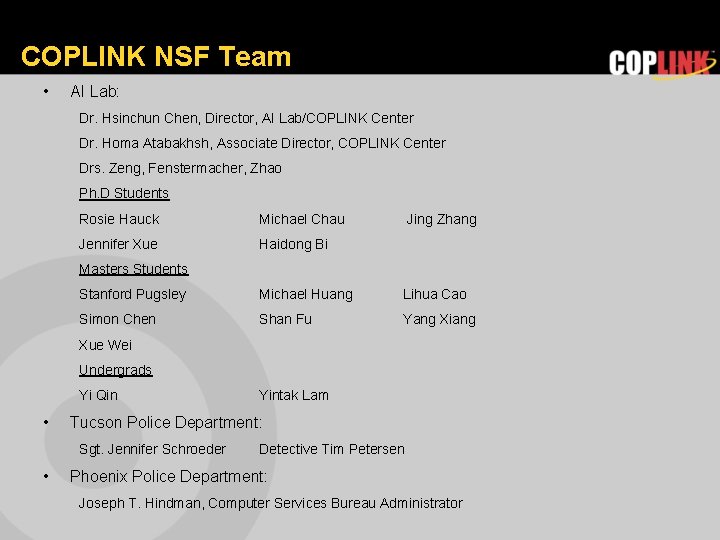
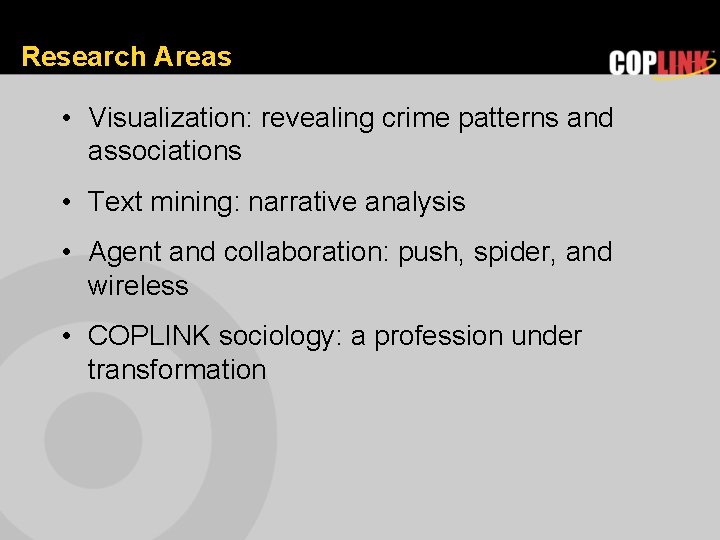
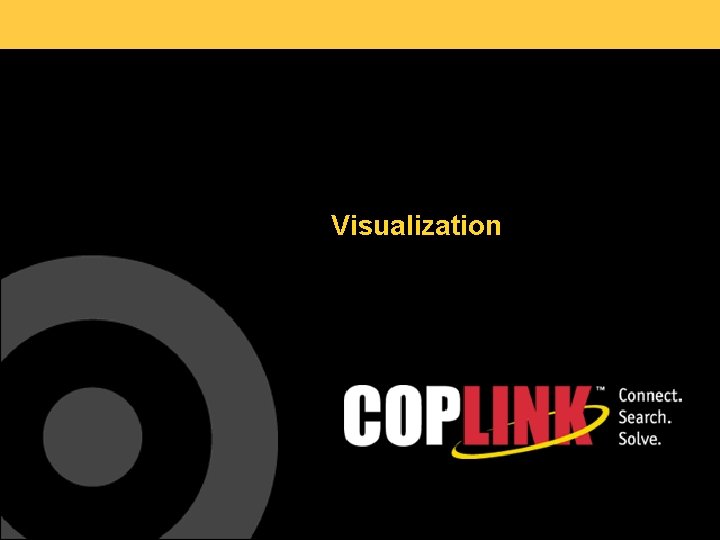
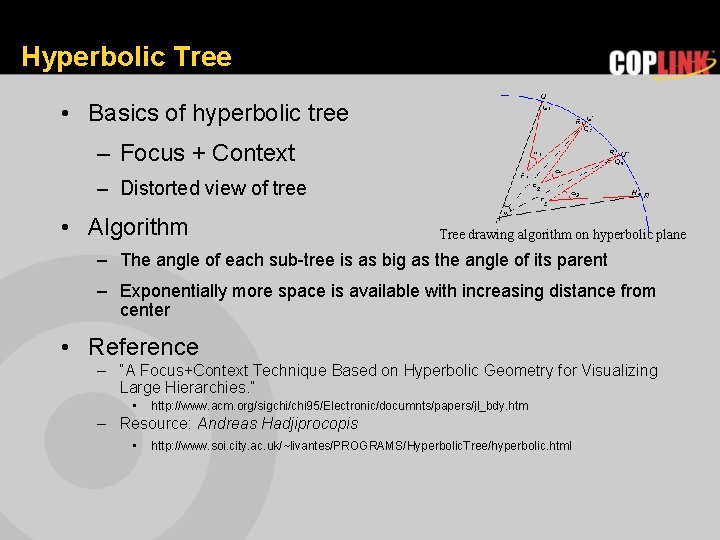
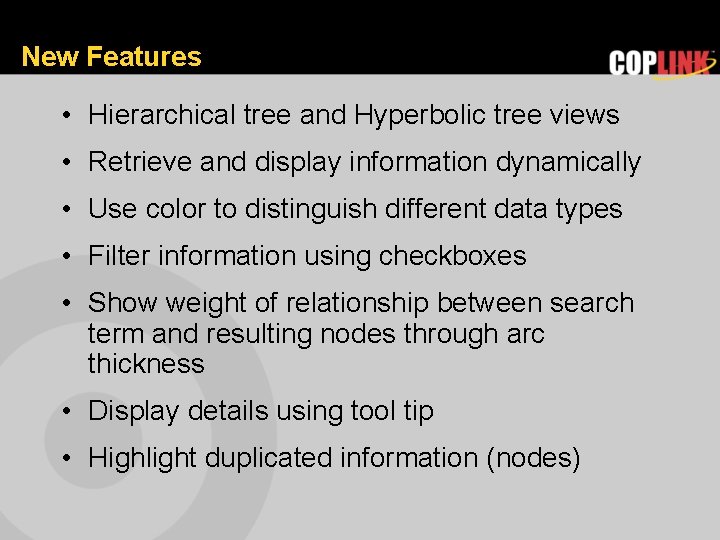
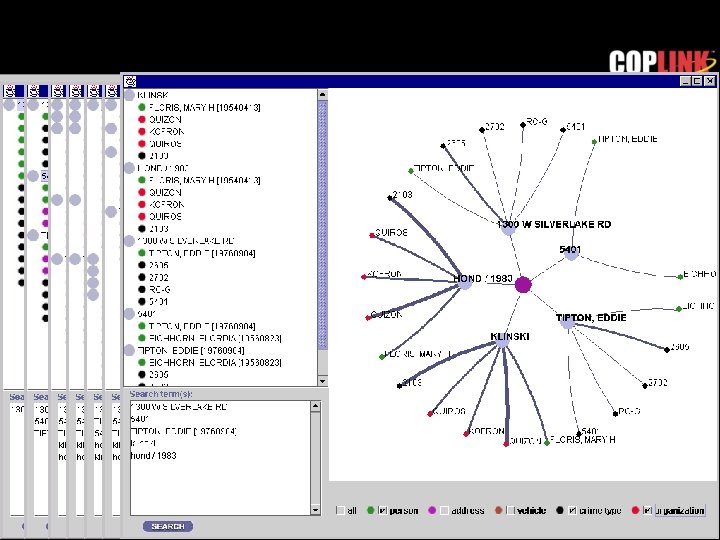
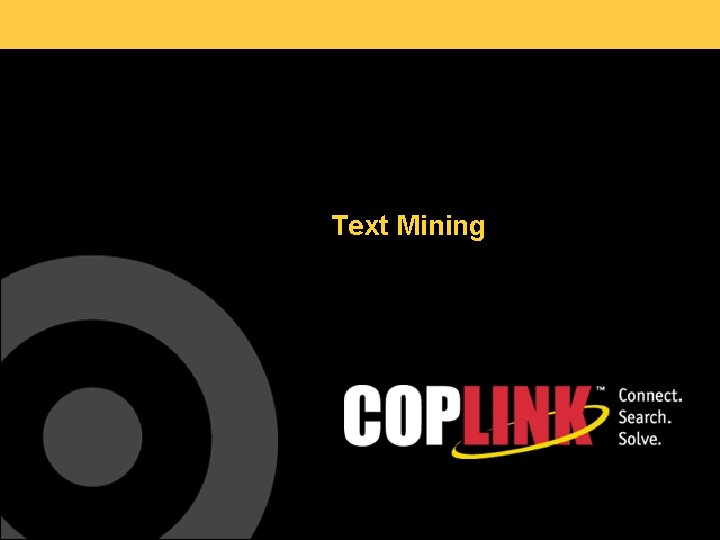
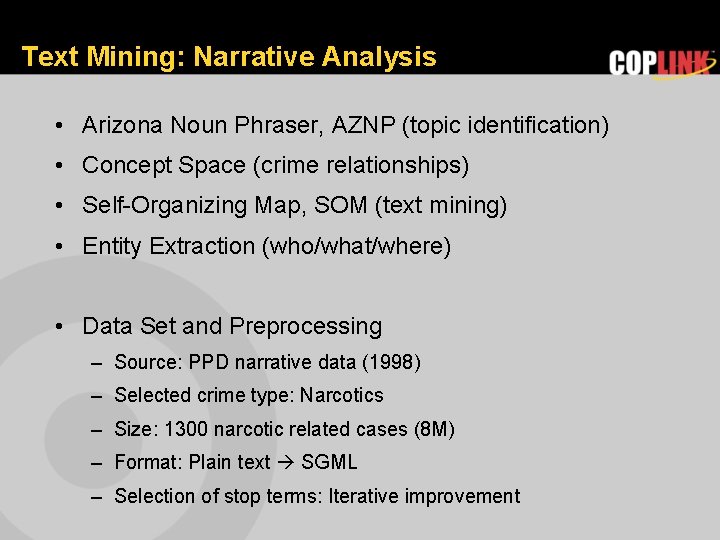
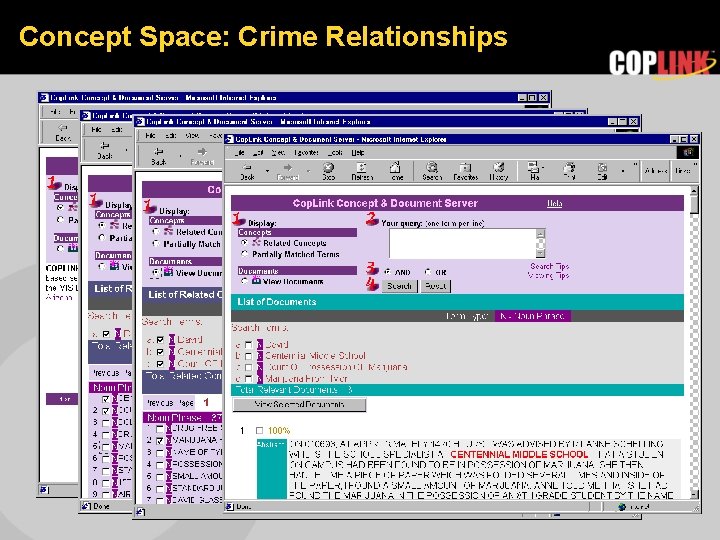
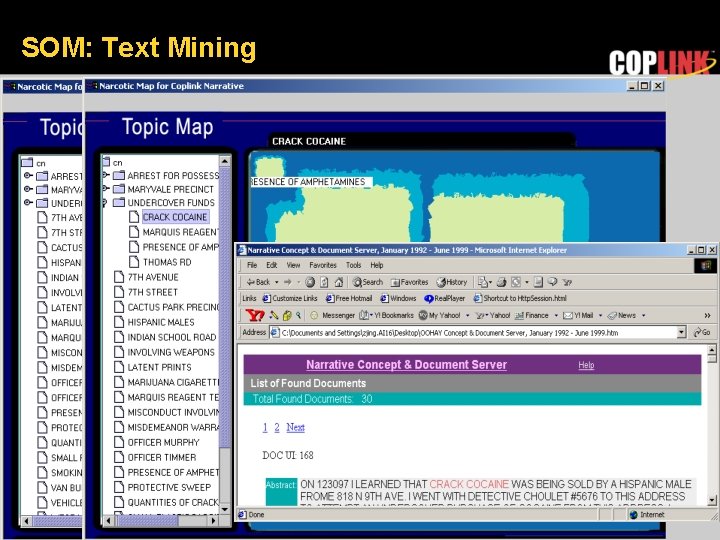
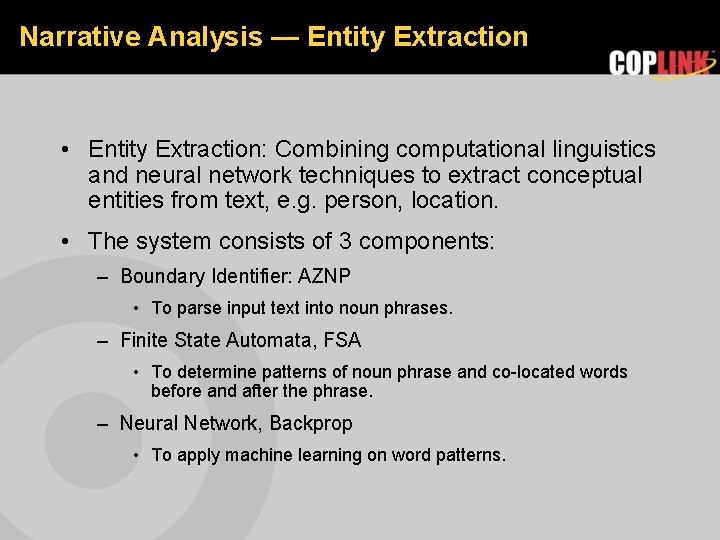
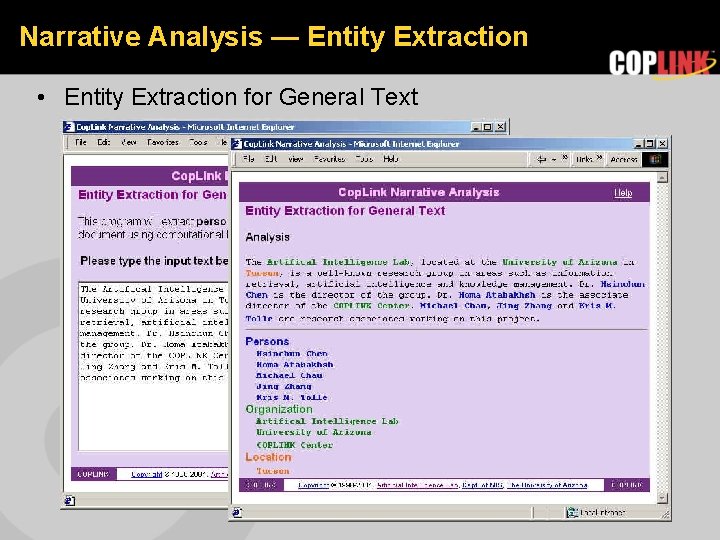
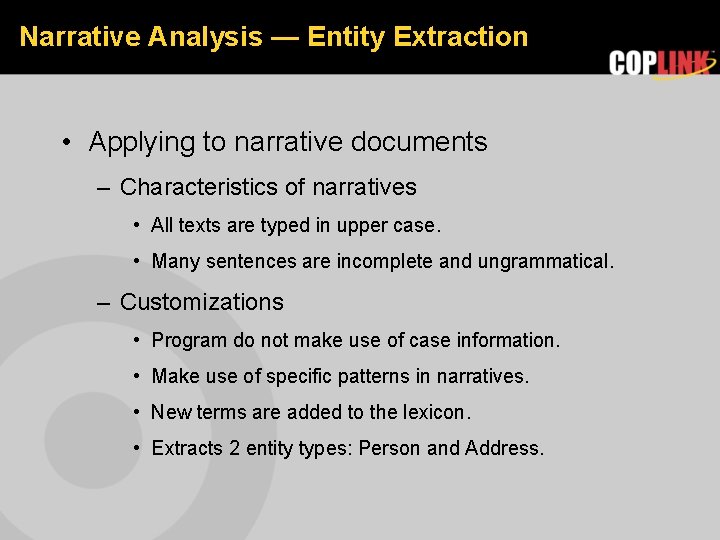
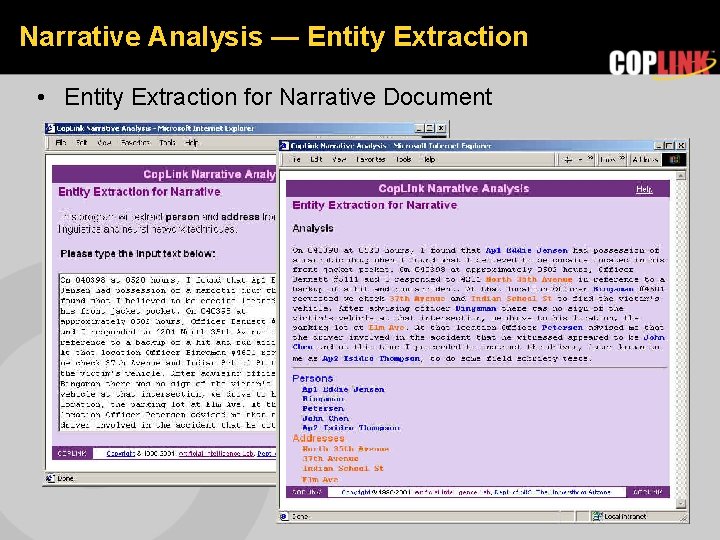
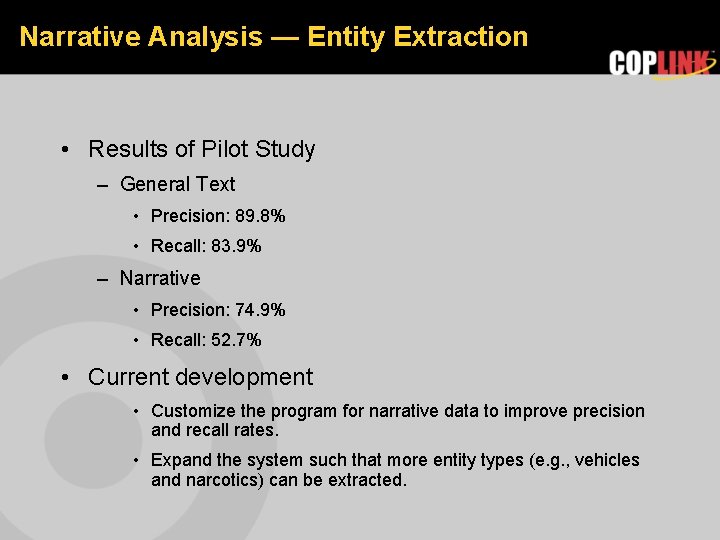
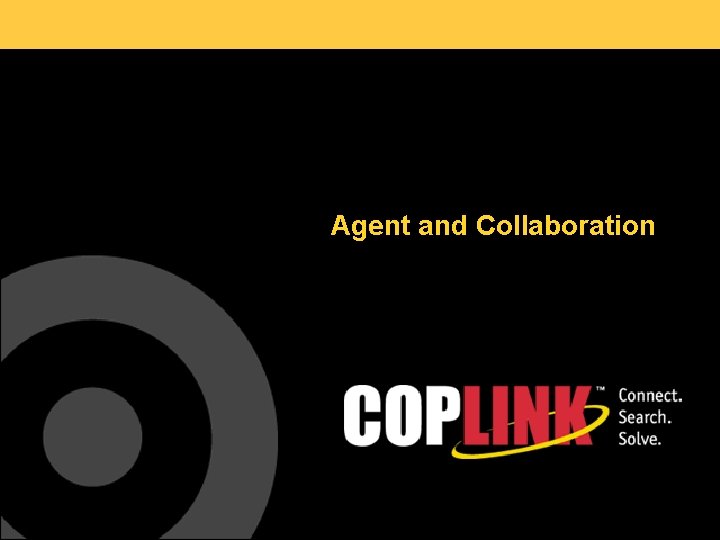
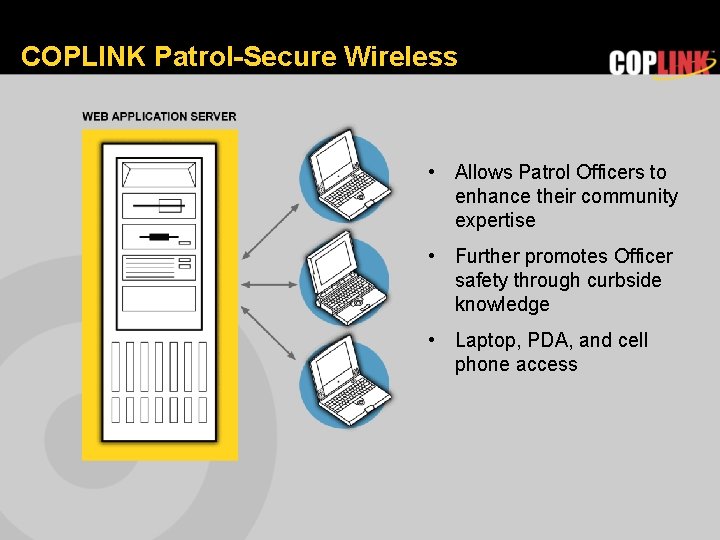
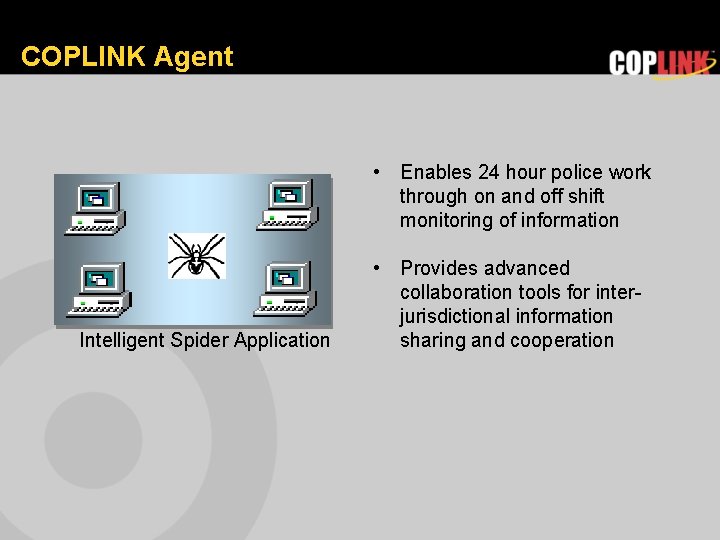
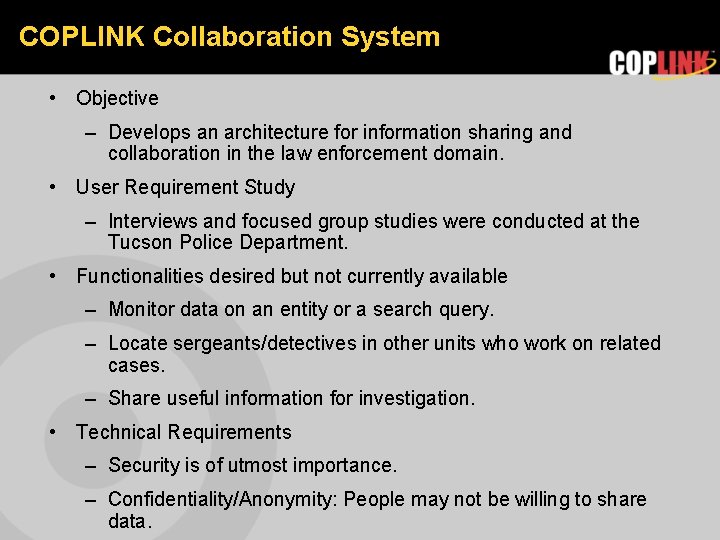
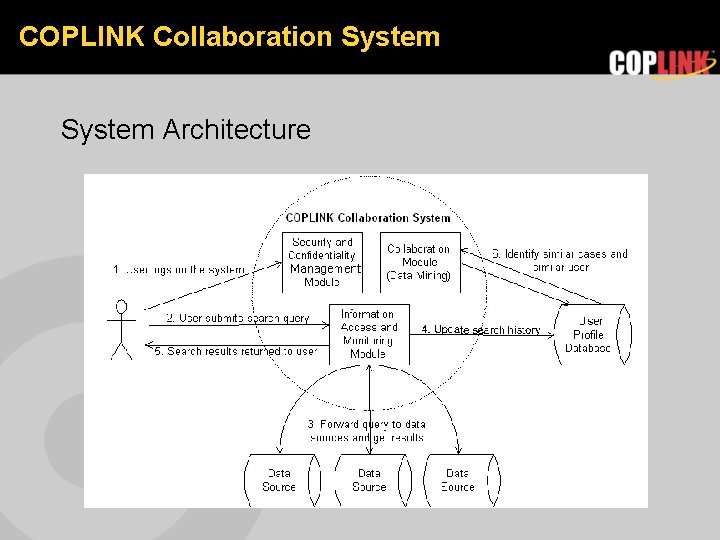
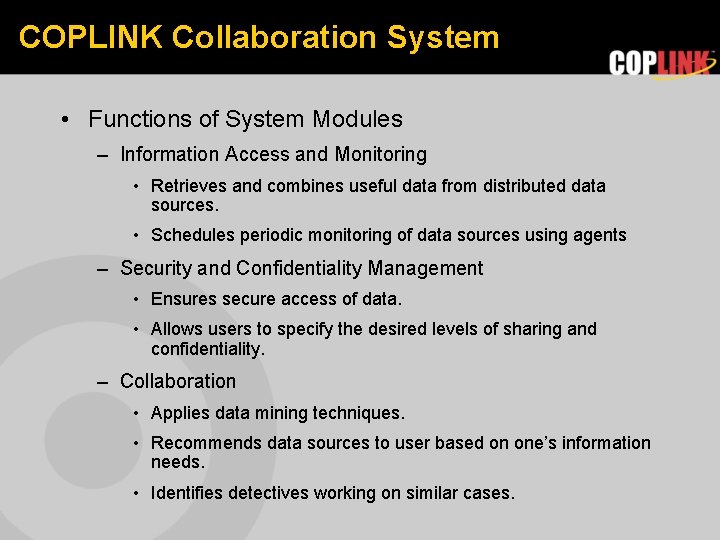
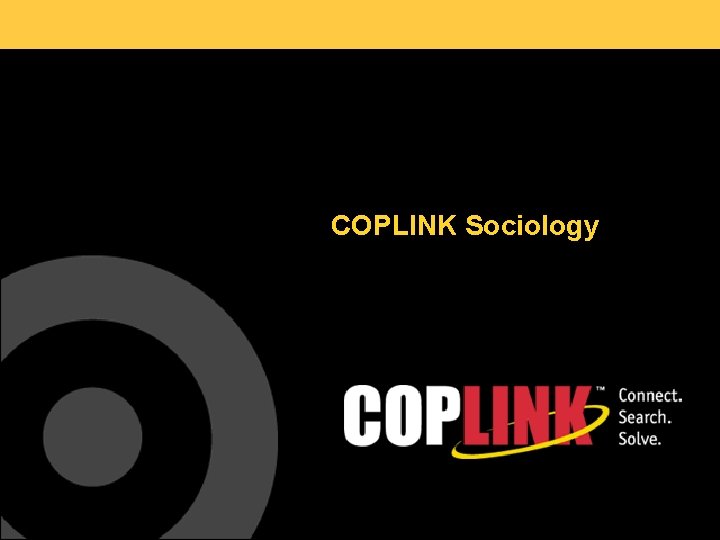
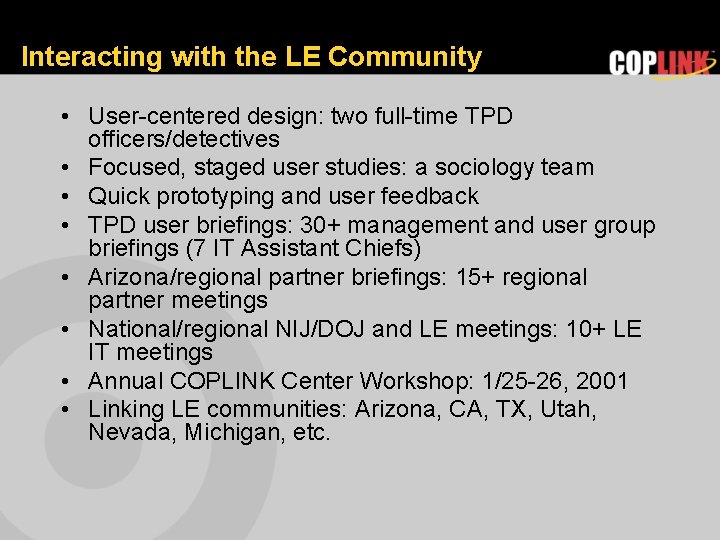
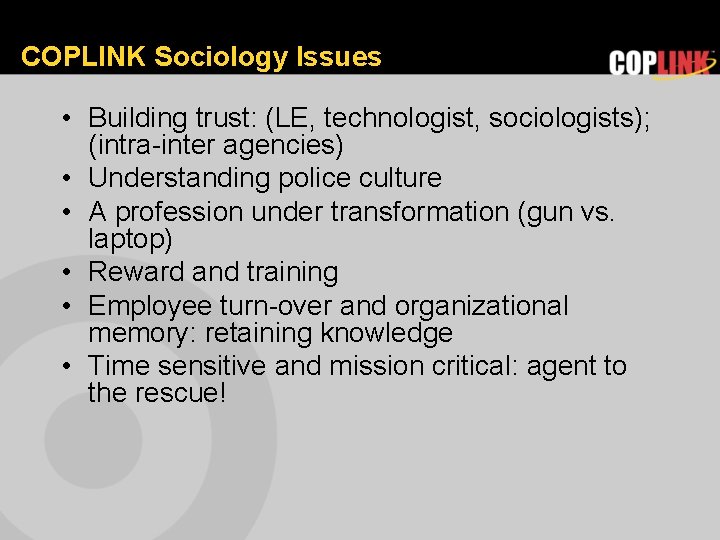
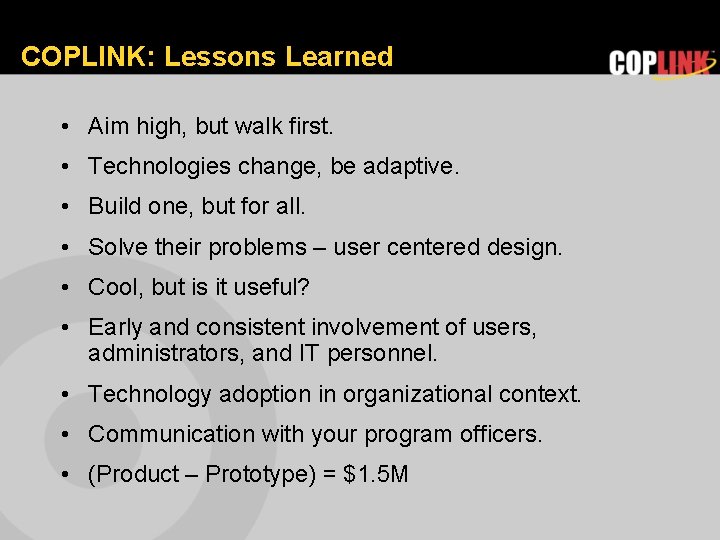
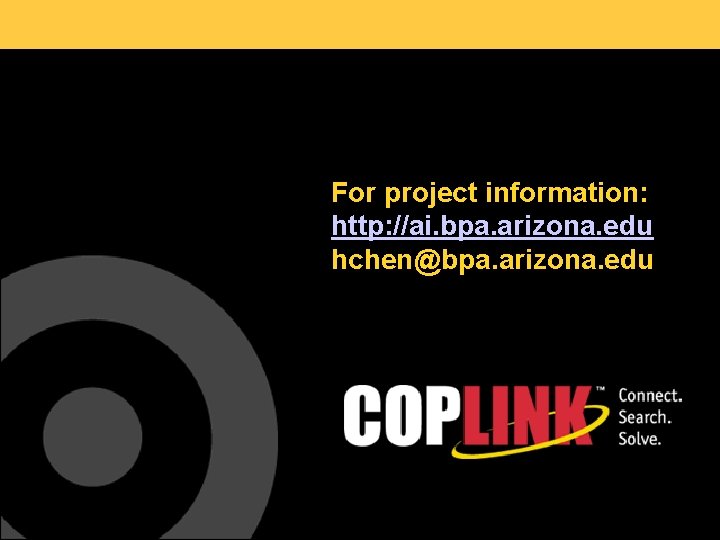
- Slides: 90
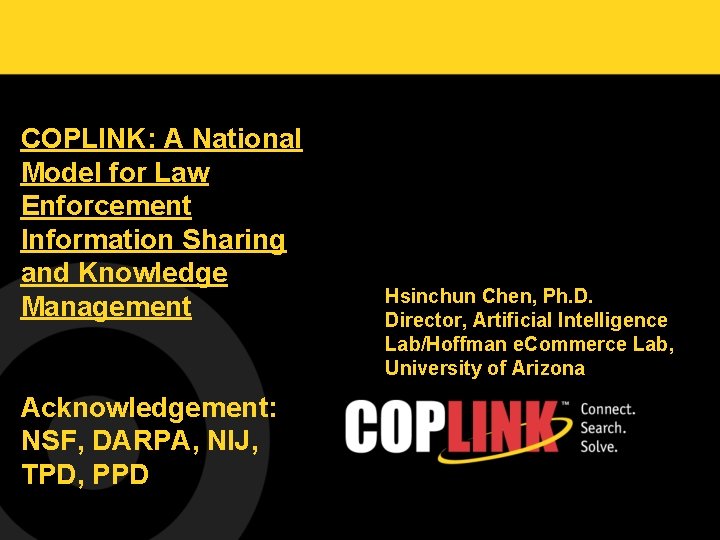
COPLINK: A National Model for Law Enforcement Information Sharing and Knowledge Management Acknowledgement: NSF, DARPA, NIJ, TPD, PPD Hsinchun Chen, Ph. D. Director, Artificial Intelligence Lab/Hoffman e. Commerce Lab, University of Arizona
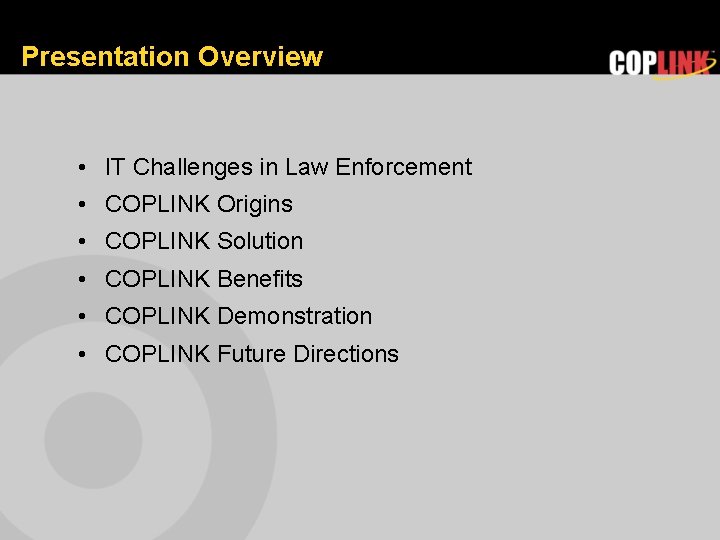
Presentation Overview • IT Challenges in Law Enforcement • COPLINK Origins • COPLINK Solution • COPLINK Benefits • COPLINK Demonstration • COPLINK Future Directions
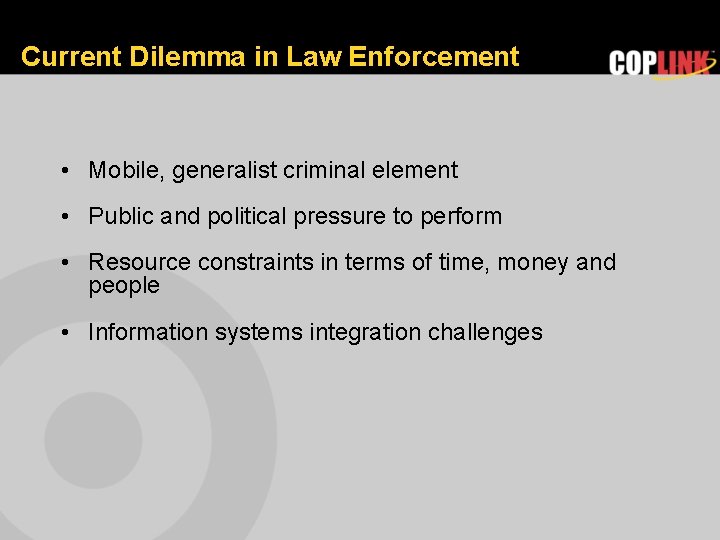
Current Dilemma in Law Enforcement • Mobile, generalist criminal element • Public and political pressure to perform • Resource constraints in terms of time, money and people • Information systems integration challenges
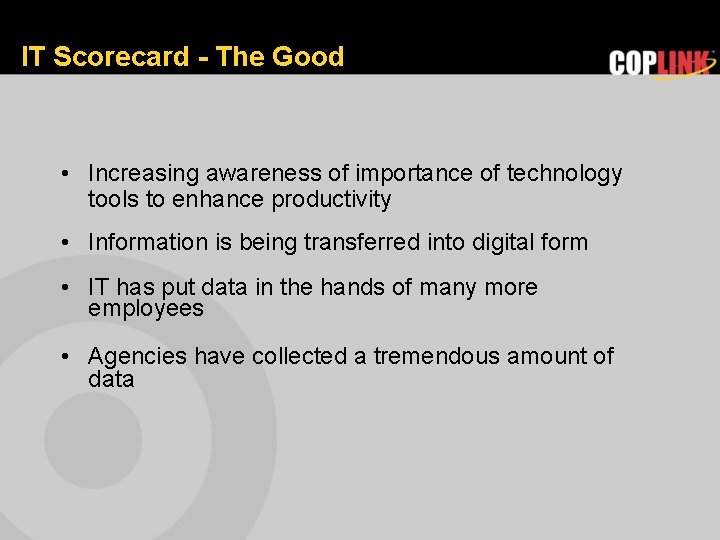
IT Scorecard - The Good • Increasing awareness of importance of technology tools to enhance productivity • Information is being transferred into digital form • IT has put data in the hands of many more employees • Agencies have collected a tremendous amount of data
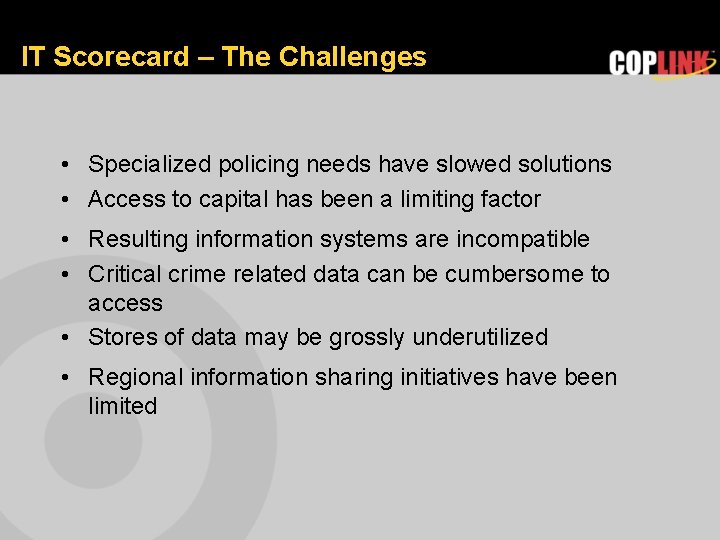
IT Scorecard – The Challenges • Specialized policing needs have slowed solutions • Access to capital has been a limiting factor • Resulting information systems are incompatible • Critical crime related data can be cumbersome to access • Stores of data may be grossly underutilized • Regional information sharing initiatives have been limited
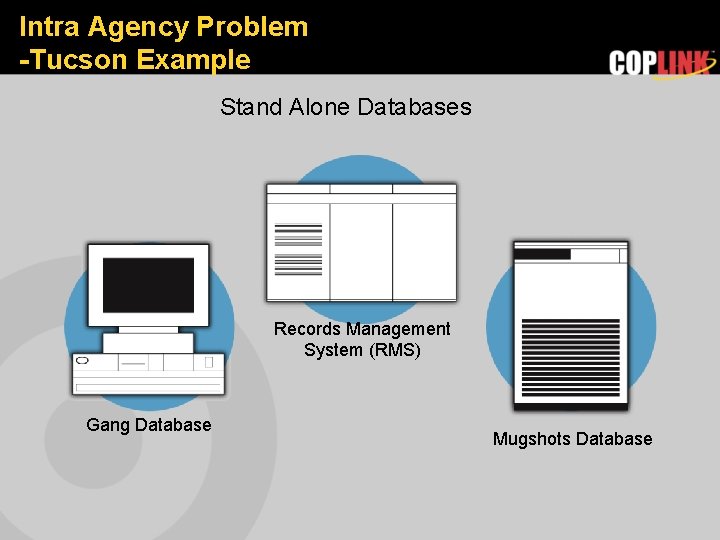
Intra Agency Problem -Tucson Example Stand Alone Databases Records Management System (RMS) Gang Database Mugshots Database
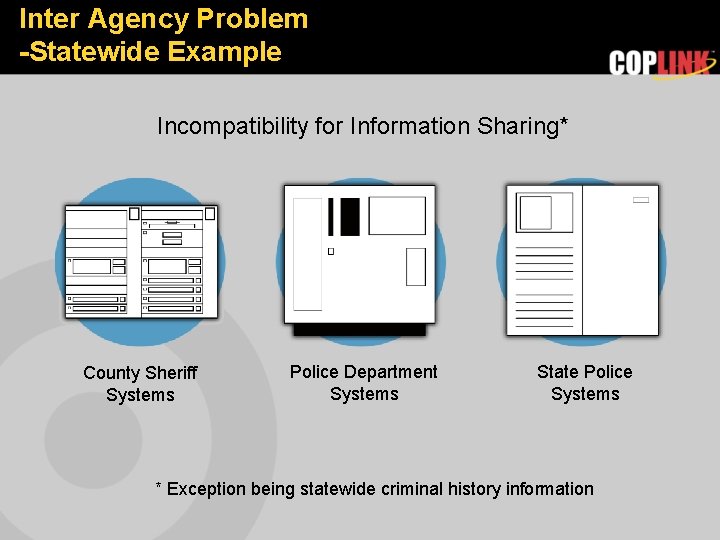
Inter Agency Problem -Statewide Example Incompatibility for Information Sharing* County Sheriff Systems Police Department Systems State Police Systems * Exception being statewide criminal history information
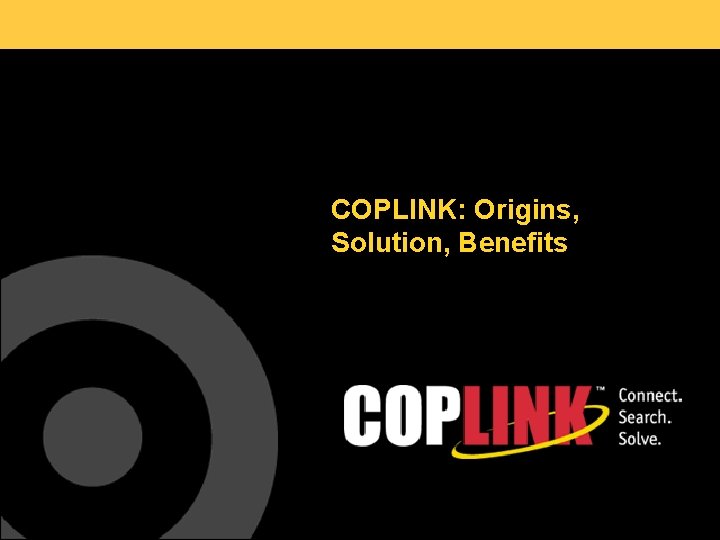
COPLINK: Origins, Solution, Benefits
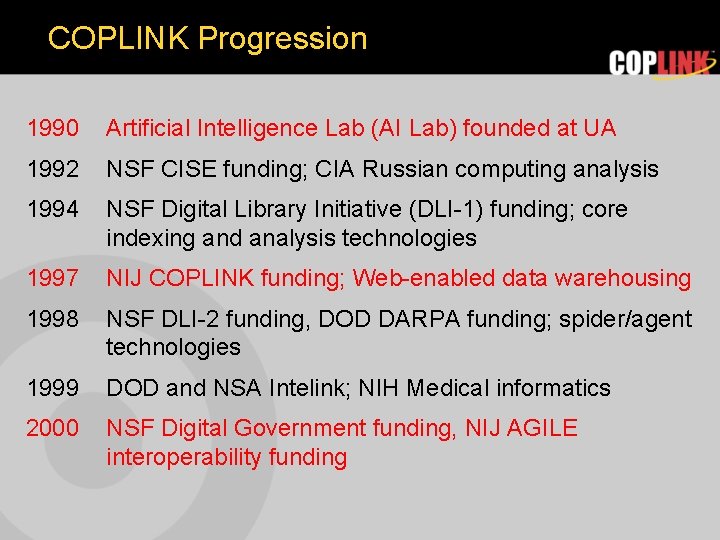
COPLINK Progression 1990 Artificial Intelligence Lab (AI Lab) founded at UA 1992 NSF CISE funding; CIA Russian computing analysis 1994 NSF Digital Library Initiative (DLI-1) funding; core indexing and analysis technologies 1997 NIJ COPLINK funding; Web-enabled data warehousing 1998 NSF DLI-2 funding, DOD DARPA funding; spider/agent technologies 1999 DOD and NSA Intelink; NIH Medical informatics 2000 NSF Digital Government funding, NIJ AGILE interoperability funding
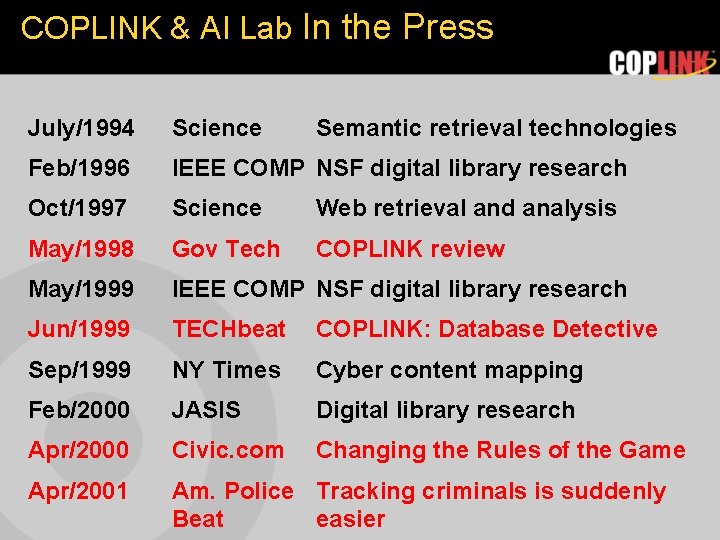
COPLINK & AI Lab In the Press July/1994 Science Semantic retrieval technologies Feb/1996 IEEE COMP NSF digital library research Oct/1997 Science Web retrieval and analysis May/1998 Gov Tech COPLINK review May/1999 IEEE COMP NSF digital library research Jun/1999 TECHbeat COPLINK: Database Detective Sep/1999 NY Times Cyber content mapping Feb/2000 JASIS Digital library research Apr/2000 Civic. com Changing the Rules of the Game Apr/2001 Am. Police Tracking criminals is suddenly Beat easier
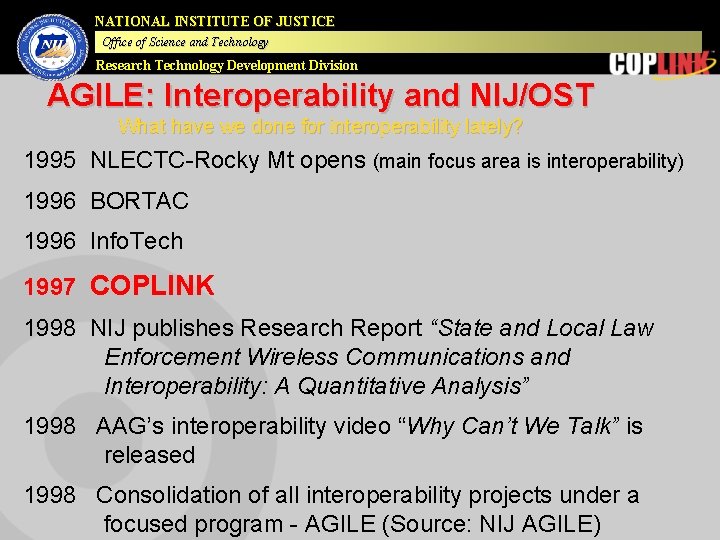
NATIONAL INSTITUTE OF JUSTICE Office of Science and Technology Research Technology Development Division AGILE: Interoperability and NIJ/OST What have we done for interoperability lately? 1995 NLECTC-Rocky Mt opens (main focus area is interoperability) 1996 BORTAC 1996 Info. Tech 1997 COPLINK 1998 NIJ publishes Research Report “State and Local Law Enforcement Wireless Communications and Interoperability: A Quantitative Analysis” 1998 AAG’s interoperability video “Why Can’t We Talk” is released 1998 Consolidation of all interoperability projects under a focused program - AGILE (Source: NIJ AGILE)
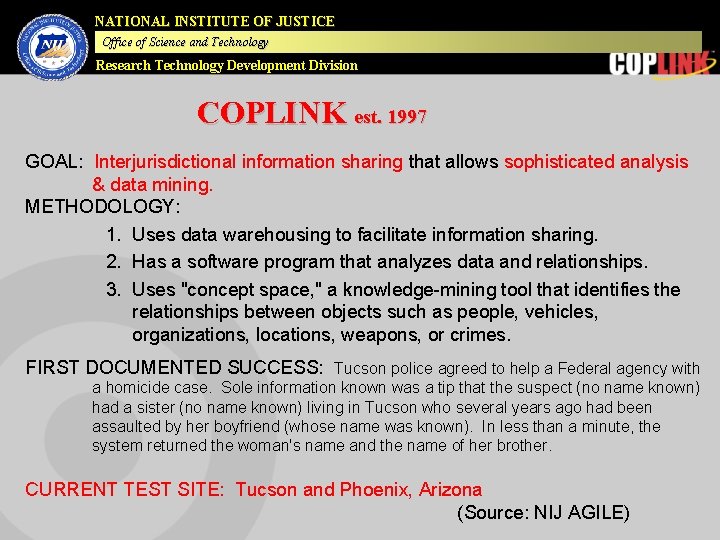
NATIONAL INSTITUTE OF JUSTICE Office of Science and Technology Research Technology Development Division COPLINK est. 1997 GOAL: Interjurisdictional information sharing that allows sophisticated analysis & data mining. METHODOLOGY: 1. Uses data warehousing to facilitate information sharing. 2. Has a software program that analyzes data and relationships. 3. Uses "concept space, " a knowledge-mining tool that identifies the relationships between objects such as people, vehicles, organizations, locations, weapons, or crimes. FIRST DOCUMENTED SUCCESS: Tucson police agreed to help a Federal agency with a homicide case. Sole information known was a tip that the suspect (no name known) had a sister (no name known) living in Tucson who several years ago had been assaulted by her boyfriend (whose name was known). In less than a minute, the system returned the woman's name and the name of her brother. CURRENT TEST SITE: Tucson and Phoenix, Arizona (Source: NIJ AGILE)
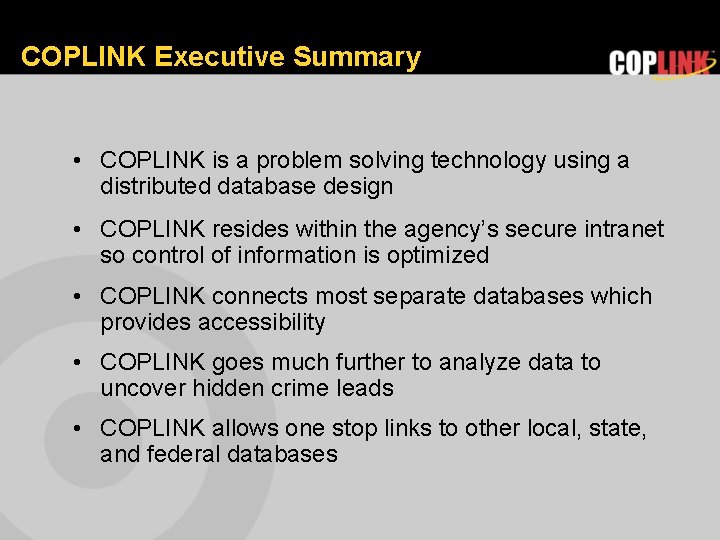
COPLINK Executive Summary • COPLINK is a problem solving technology using a distributed database design • COPLINK resides within the agency’s secure intranet so control of information is optimized • COPLINK connects most separate databases which provides accessibility • COPLINK goes much further to analyze data to uncover hidden crime leads • COPLINK allows one stop links to other local, state, and federal databases
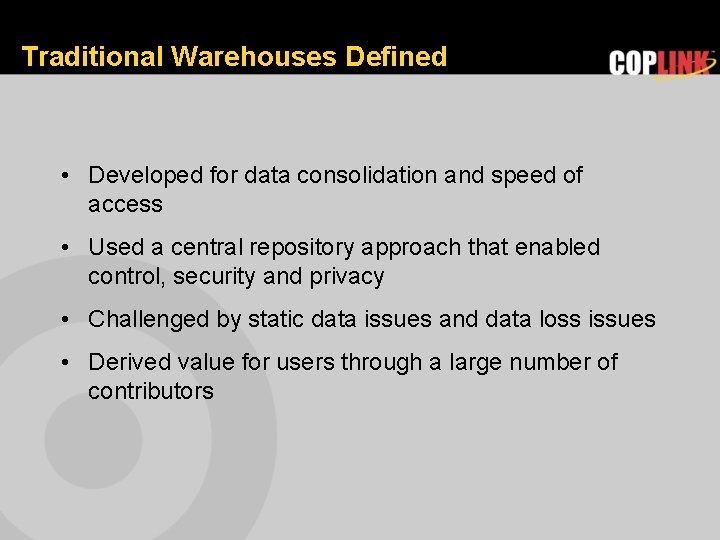
Traditional Warehouses Defined • Developed for data consolidation and speed of access • Used a central repository approach that enabled control, security and privacy • Challenged by static data issues and data loss issues • Derived value for users through a large number of contributors
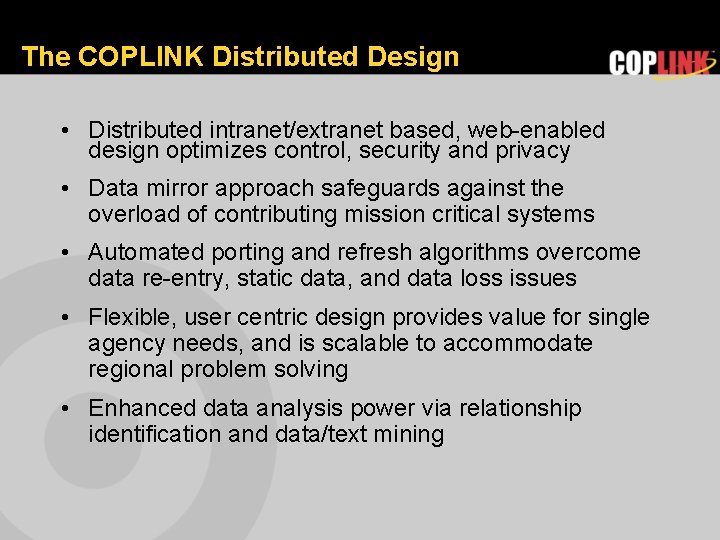
The COPLINK Distributed Design • Distributed intranet/extranet based, web-enabled design optimizes control, security and privacy • Data mirror approach safeguards against the overload of contributing mission critical systems • Automated porting and refresh algorithms overcome data re-entry, static data, and data loss issues • Flexible, user centric design provides value for single agency needs, and is scalable to accommodate regional problem solving • Enhanced data analysis power via relationship identification and data/text mining
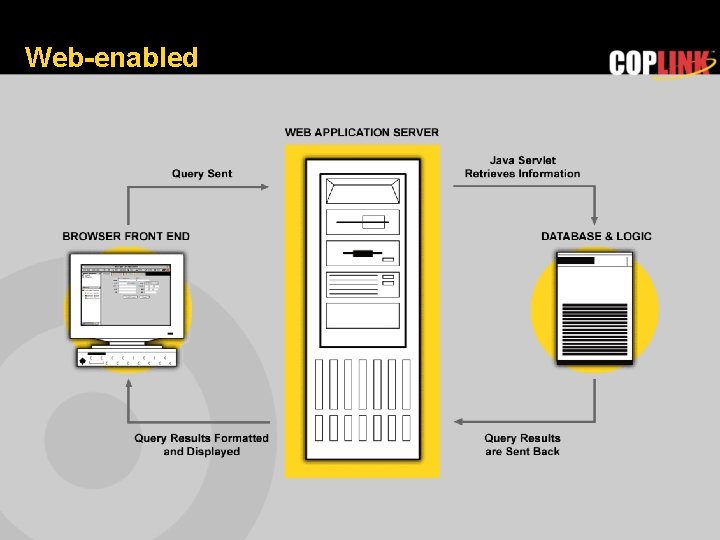
Web-enabled
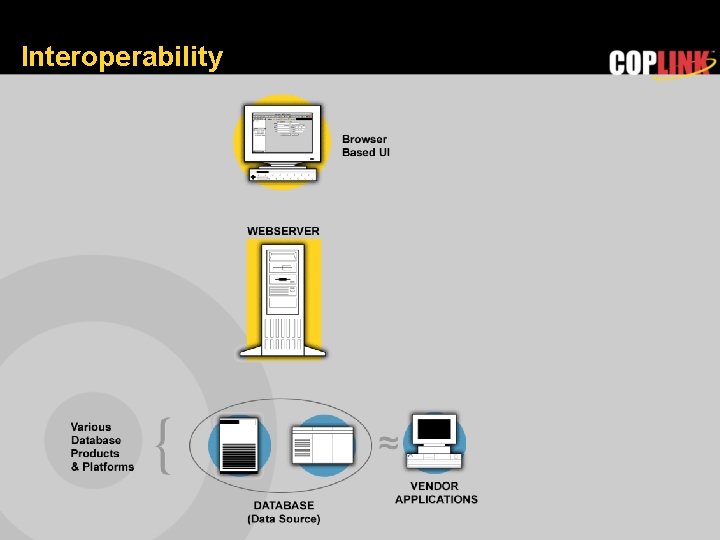
Interoperability
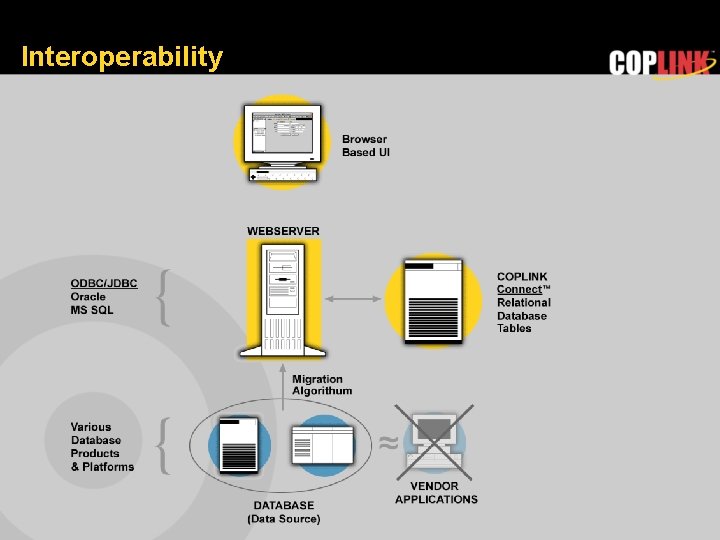
Interoperability
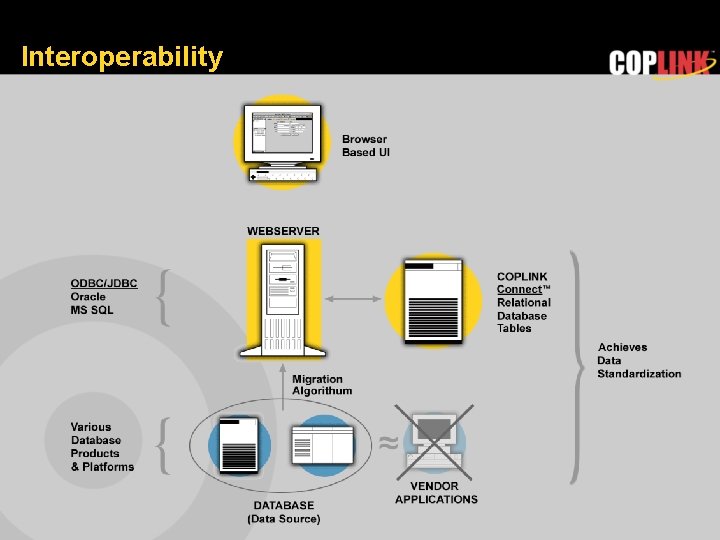
Interoperability
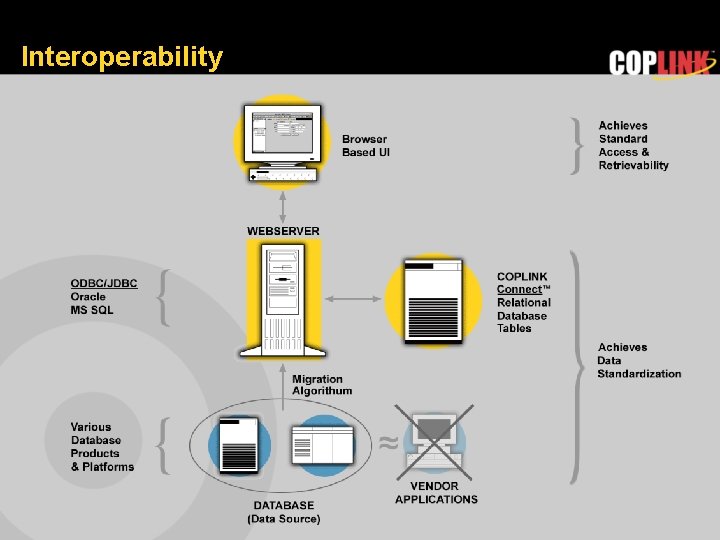
Interoperability
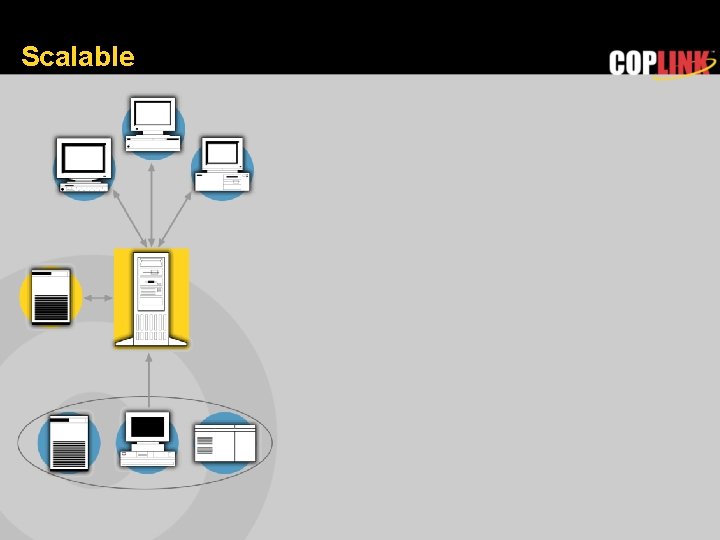
Scalable
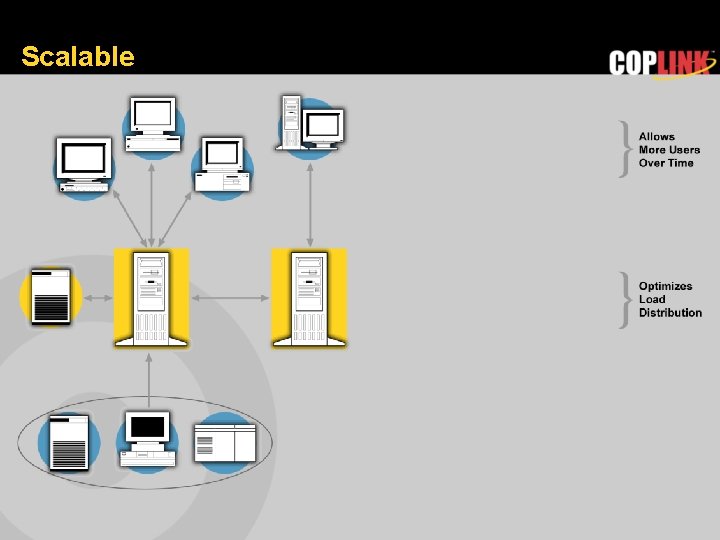
Scalable
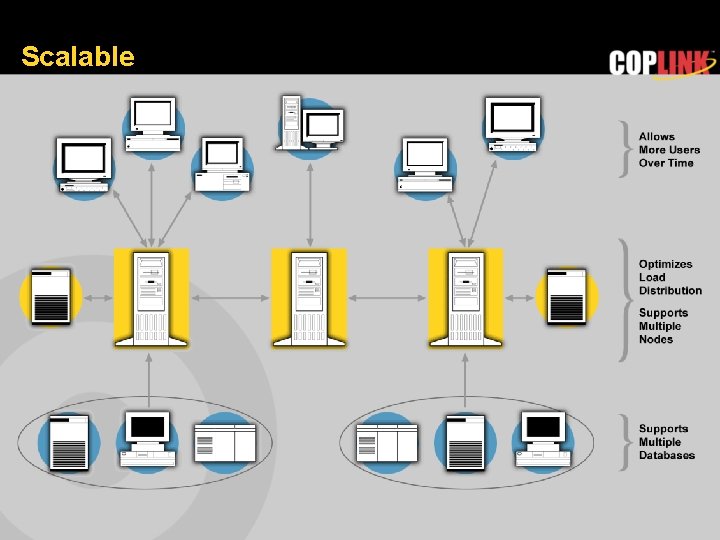
Scalable
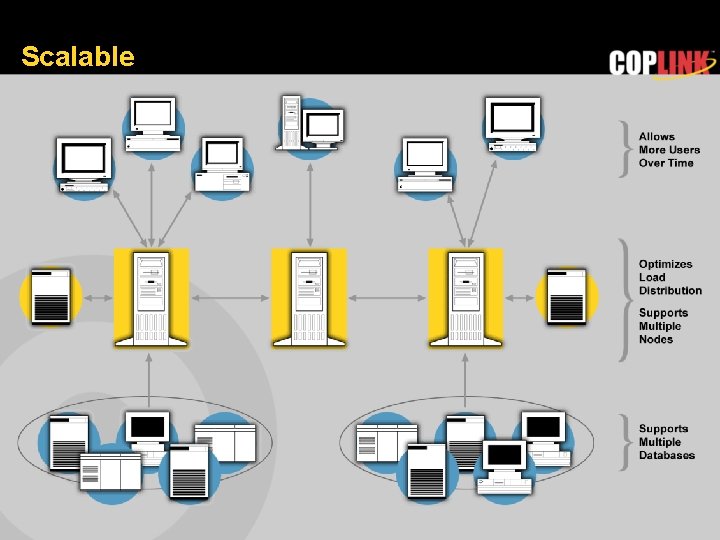
Scalable
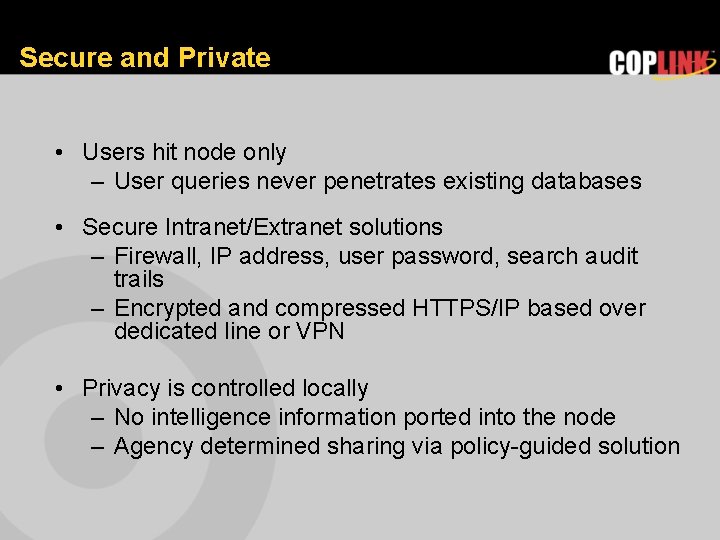
Secure and Private • Users hit node only – User queries never penetrates existing databases • Secure Intranet/Extranet solutions – Firewall, IP address, user password, search audit trails – Encrypted and compressed HTTPS/IP based over dedicated line or VPN • Privacy is controlled locally – No intelligence information ported into the node – Agency determined sharing via policy-guided solution
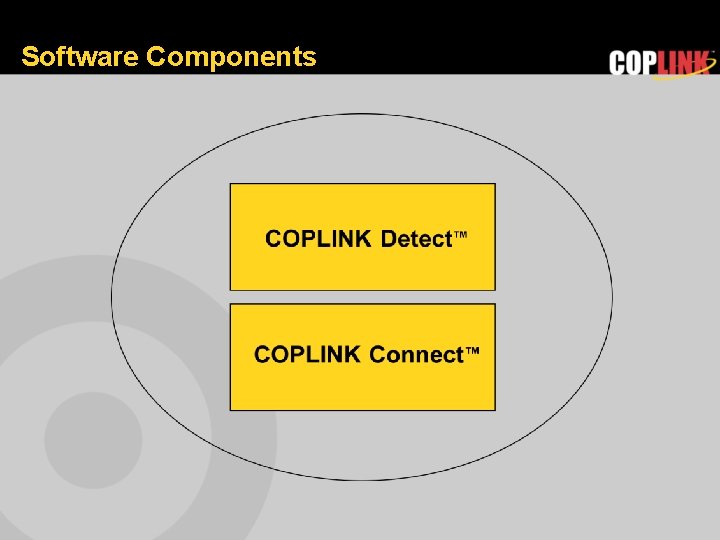
Software Components
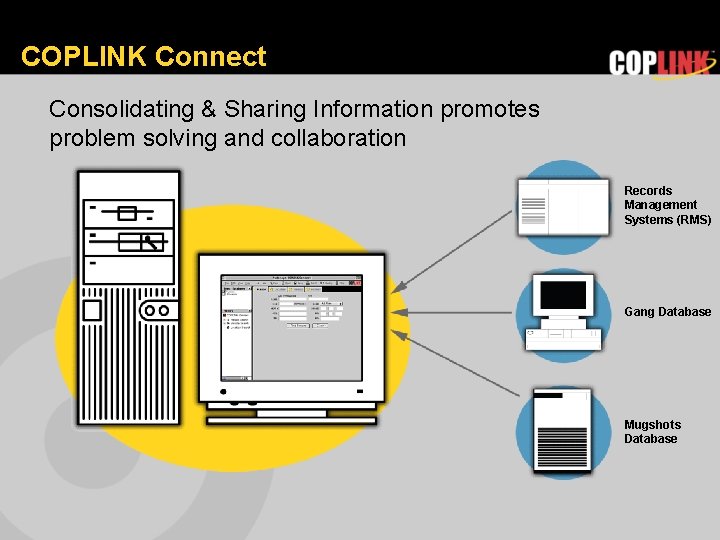
COPLINK Connect Consolidating & Sharing Information promotes problem solving and collaboration Records Management Systems (RMS) Gang Database Mugshots Database
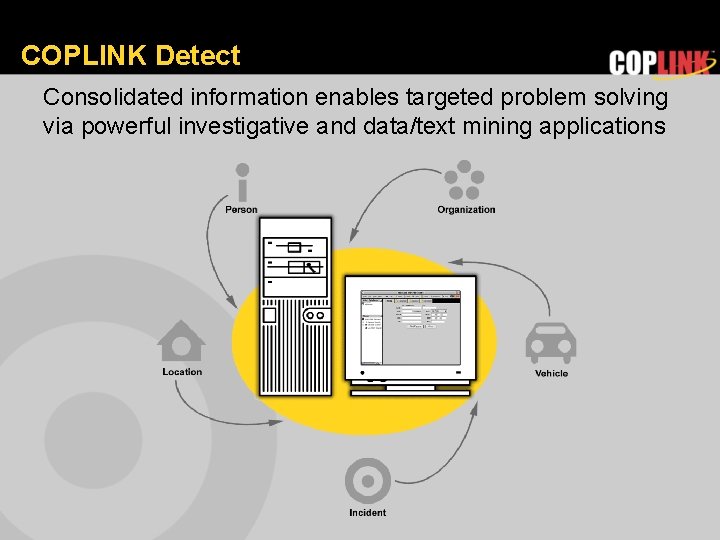
COPLINK Detect Consolidated information enables targeted problem solving via powerful investigative and data/text mining applications
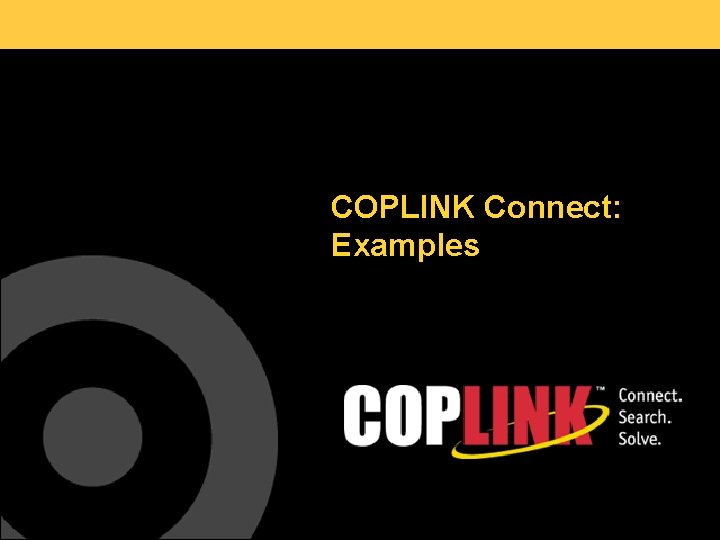
COPLINK Connect: Examples
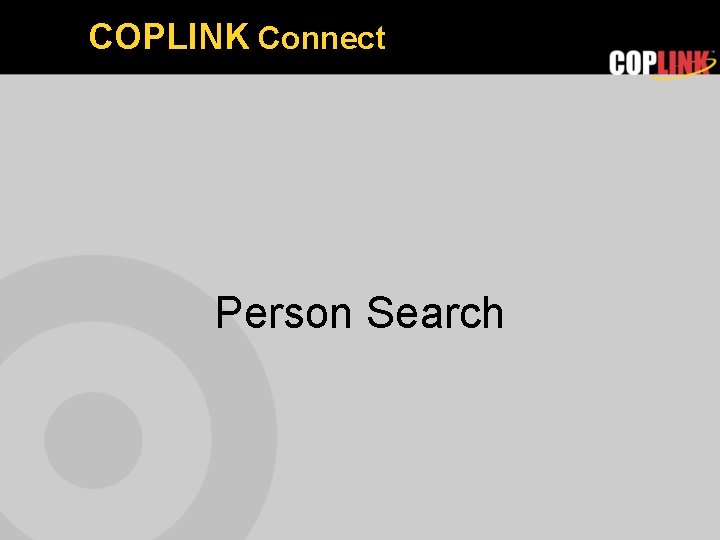
COPLINK Connect Person Search
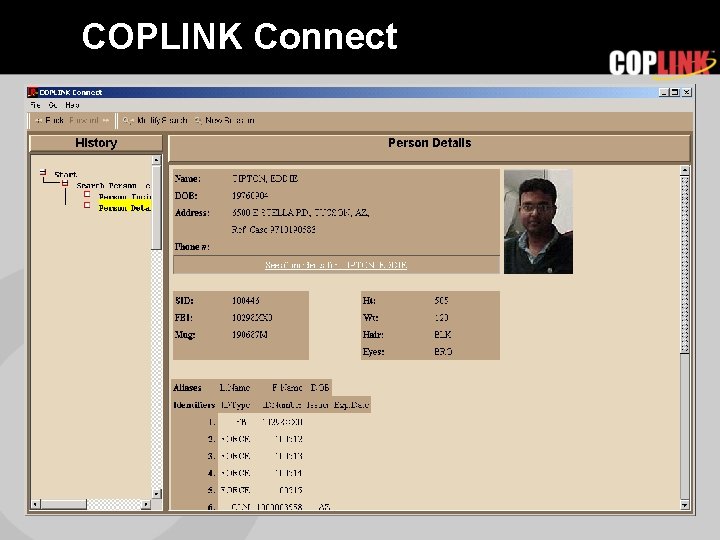
COPLINK Connect
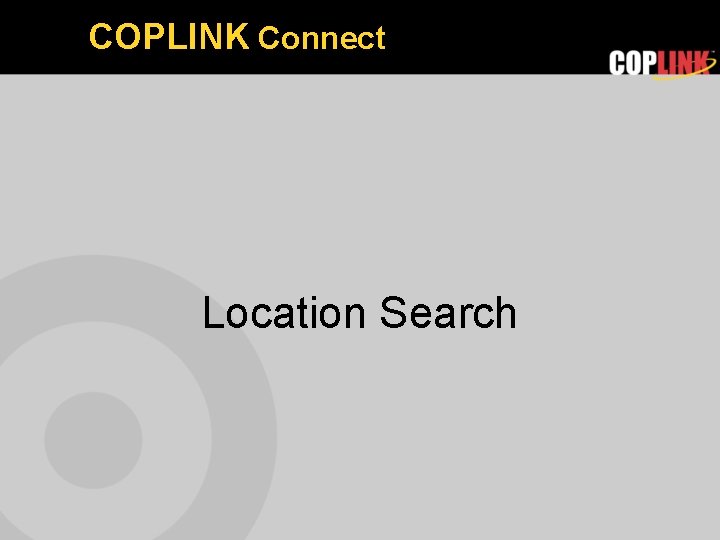
COPLINK Connect Location Search
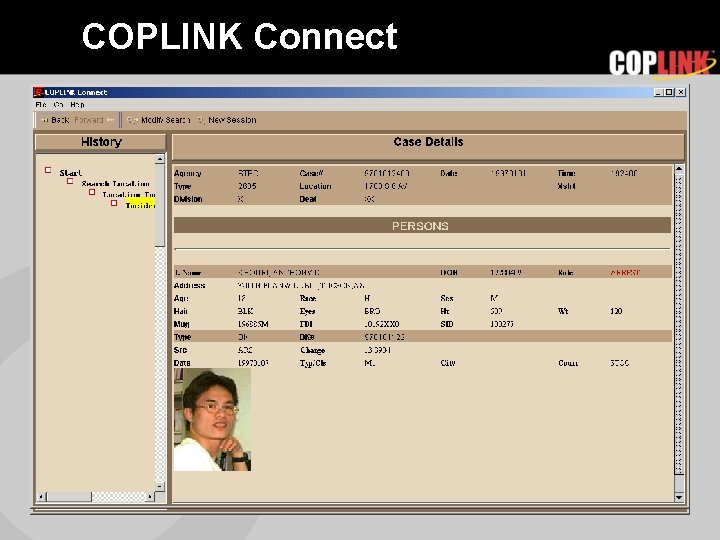
COPLINK Connect
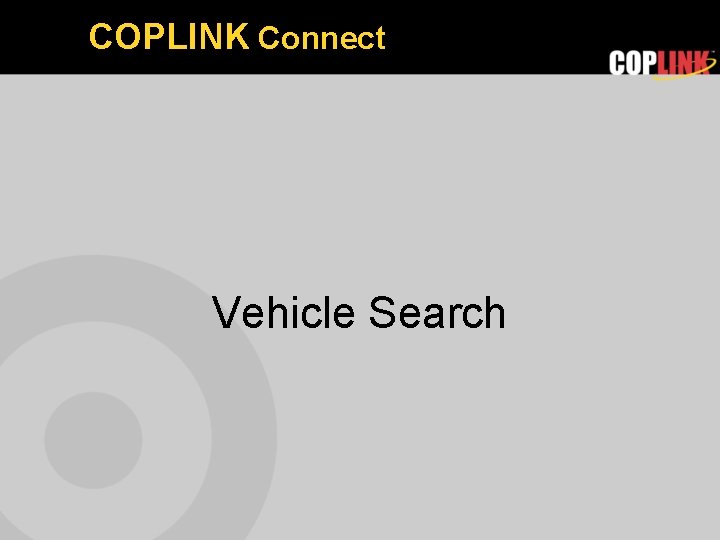
COPLINK Connect Vehicle Search
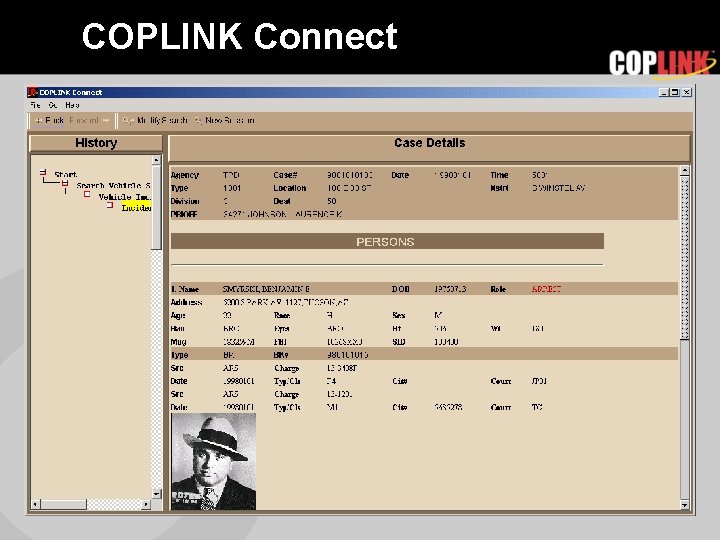
COPLINK Connect
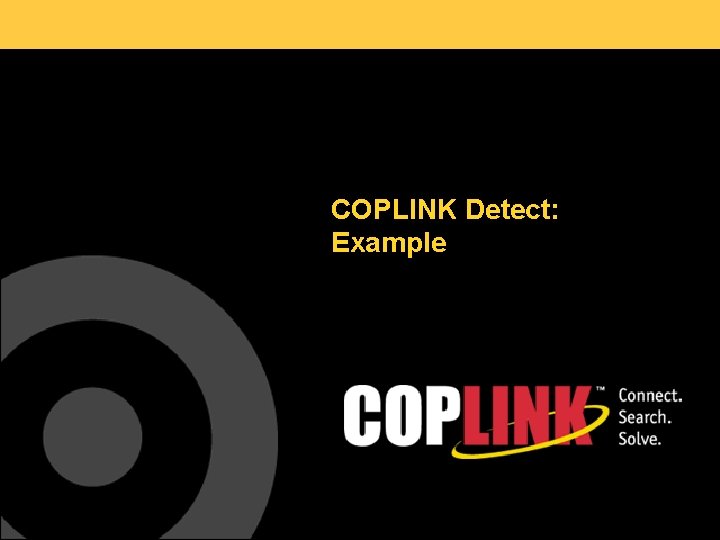
COPLINK Detect: Example
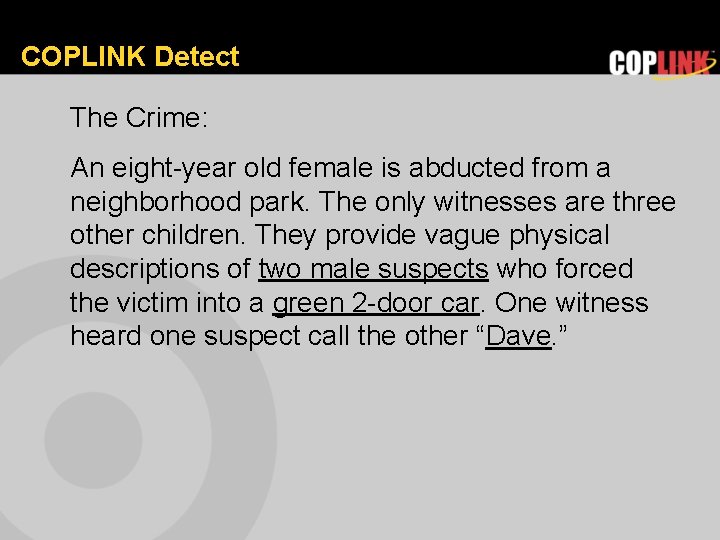
COPLINK Detect The Crime: An eight-year old female is abducted from a neighborhood park. The only witnesses are three other children. They provide vague physical descriptions of two male suspects who forced the victim into a green 2 -door car. One witness heard one suspect call the other “Dave. ”
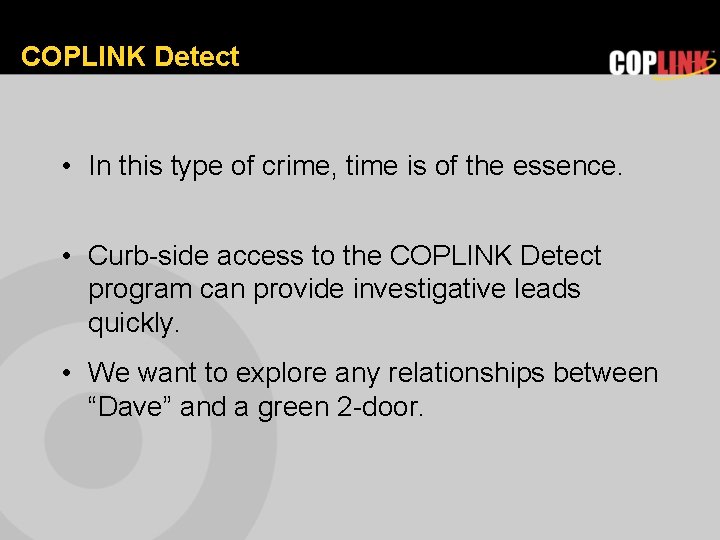
COPLINK Detect • In this type of crime, time is of the essence. • Curb-side access to the COPLINK Detect program can provide investigative leads quickly. • We want to explore any relationships between “Dave” and a green 2 -door.
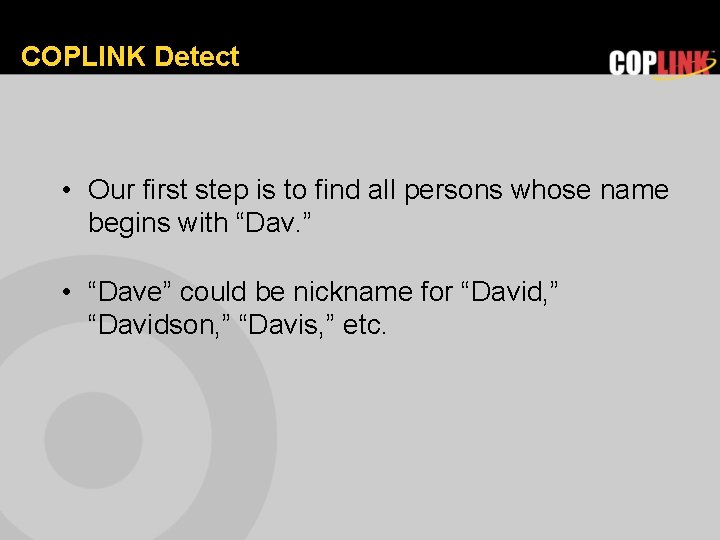
COPLINK Detect • Our first step is to find all persons whose name begins with “Dav. ” • “Dave” could be nickname for “David, ” “Davidson, ” “Davis, ” etc.
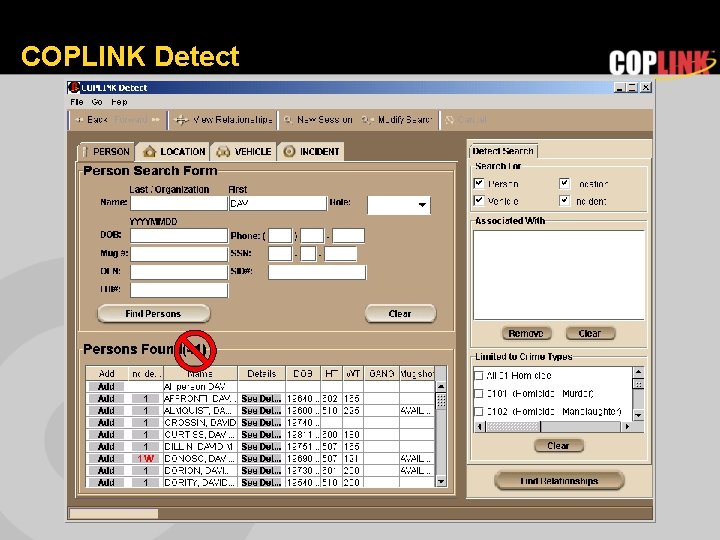
COPLINK Detect
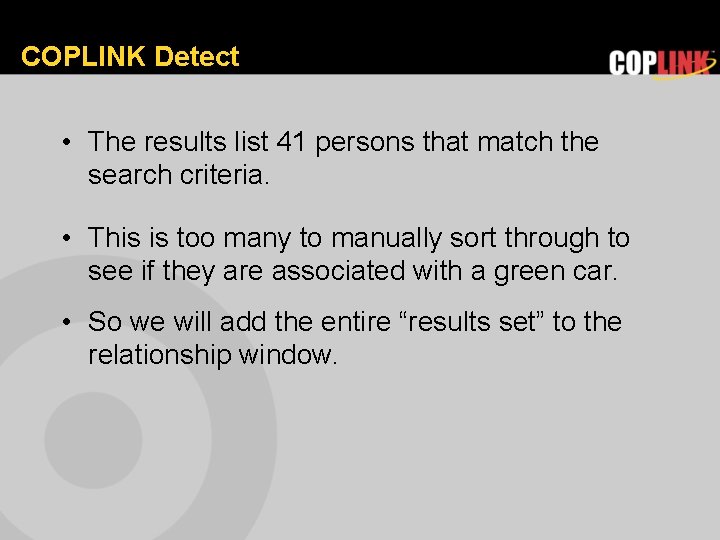
COPLINK Detect • The results list 41 persons that match the search criteria. • This is too many to manually sort through to see if they are associated with a green car. • So we will add the entire “results set” to the relationship window.
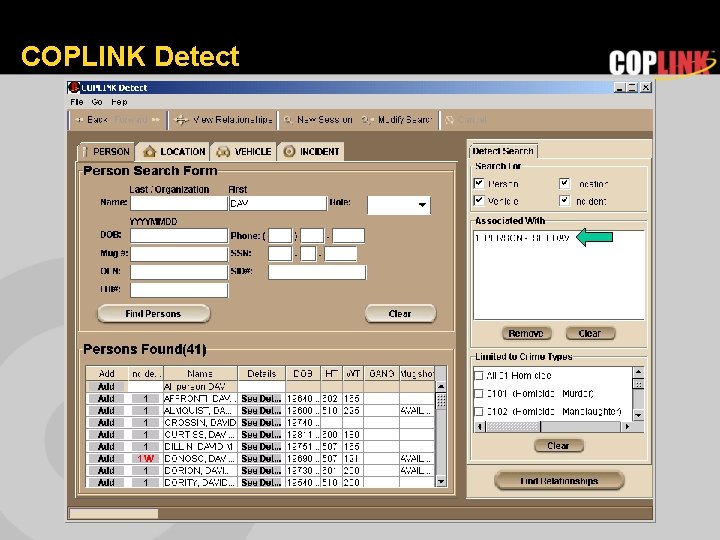
COPLINK Detect
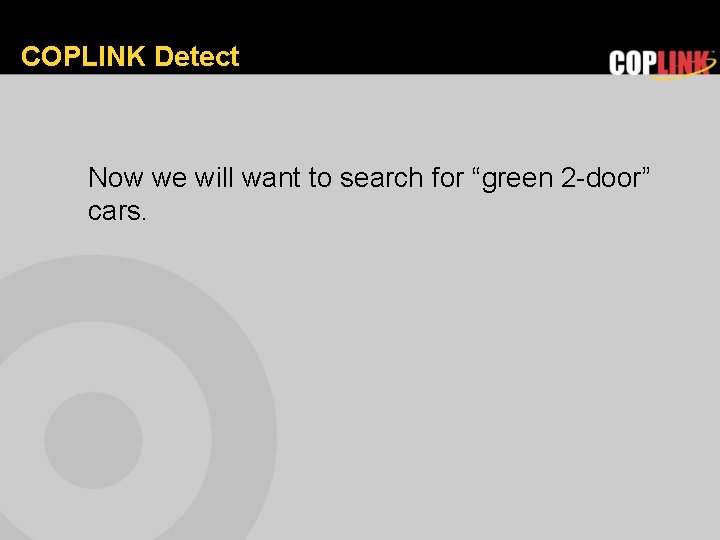
COPLINK Detect Now we will want to search for “green 2 -door” cars.
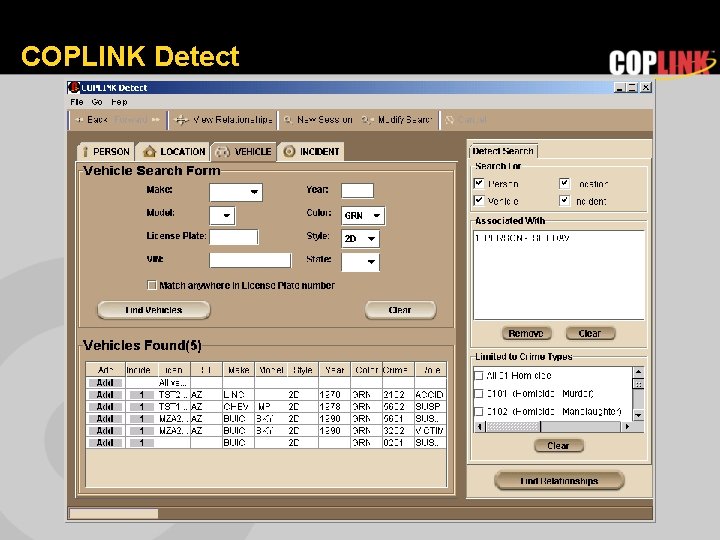
COPLINK Detect
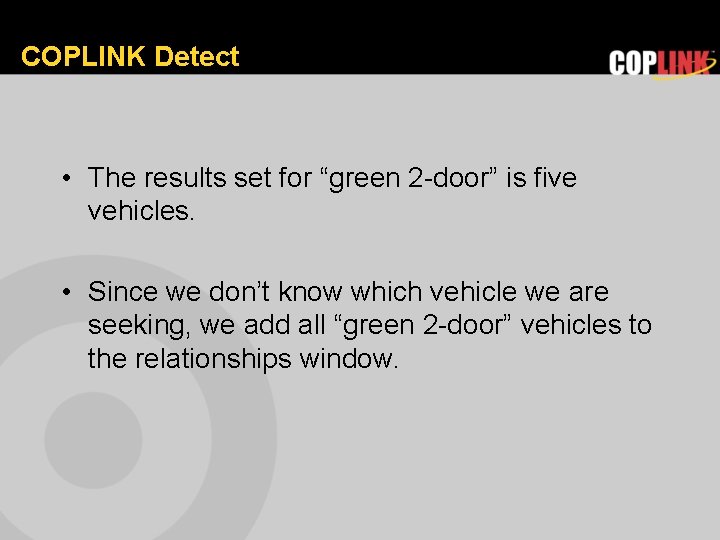
COPLINK Detect • The results set for “green 2 -door” is five vehicles. • Since we don’t know which vehicle we are seeking, we add all “green 2 -door” vehicles to the relationships window.
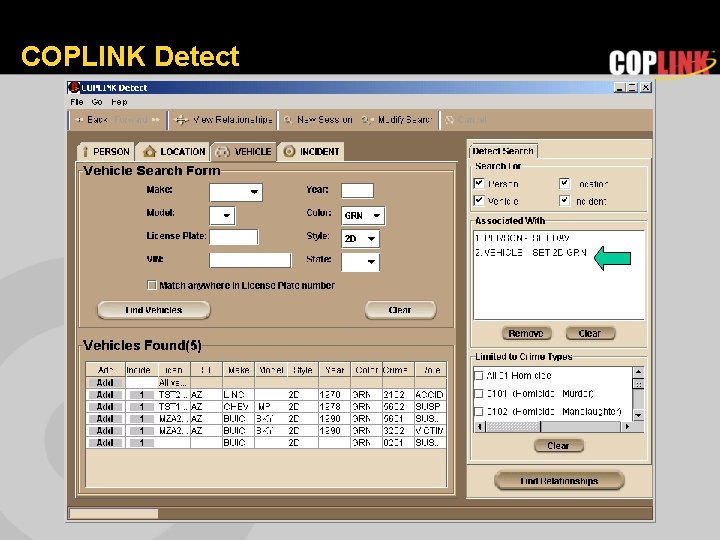
COPLINK Detect
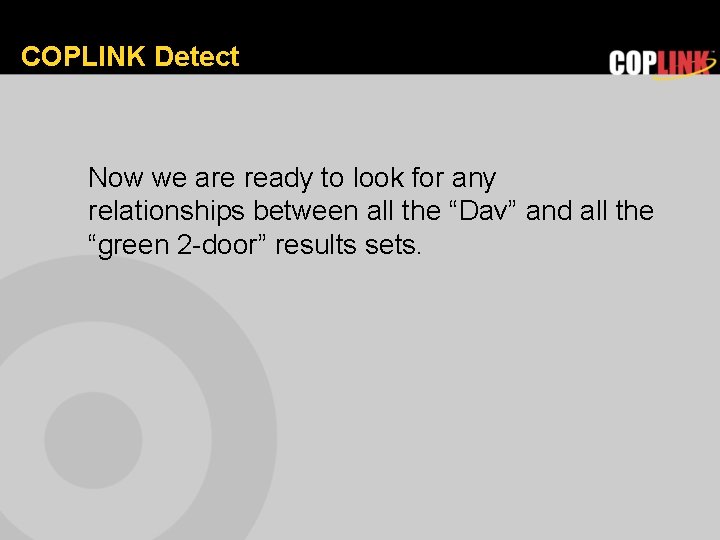
COPLINK Detect Now we are ready to look for any relationships between all the “Dav” and all the “green 2 -door” results sets.
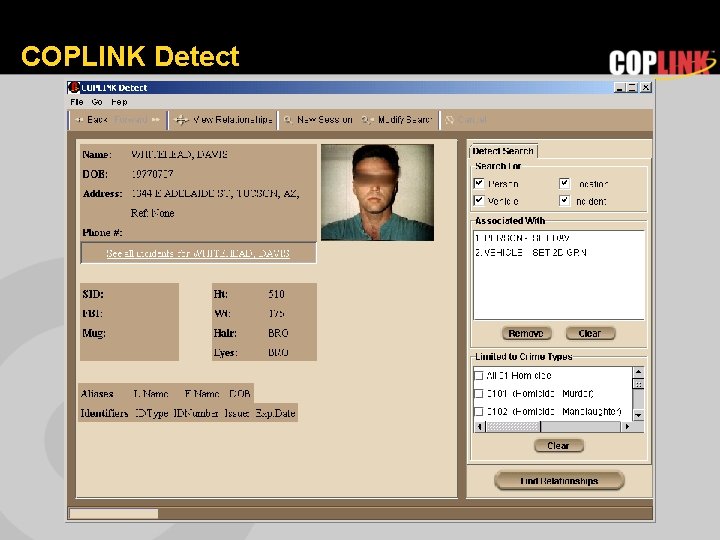
COPLINK Detect
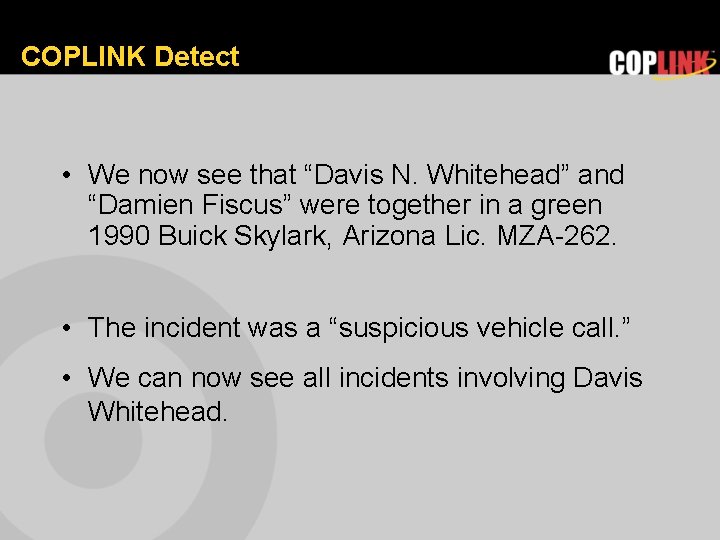
COPLINK Detect • We now see that “Davis N. Whitehead” and “Damien Fiscus” were together in a green 1990 Buick Skylark, Arizona Lic. MZA-262. • The incident was a “suspicious vehicle call. ” • We can now see all incidents involving Davis Whitehead.
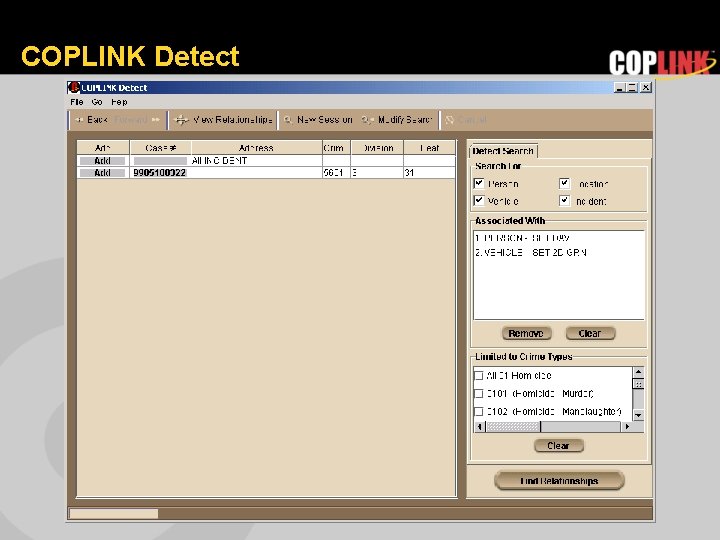
COPLINK Detect
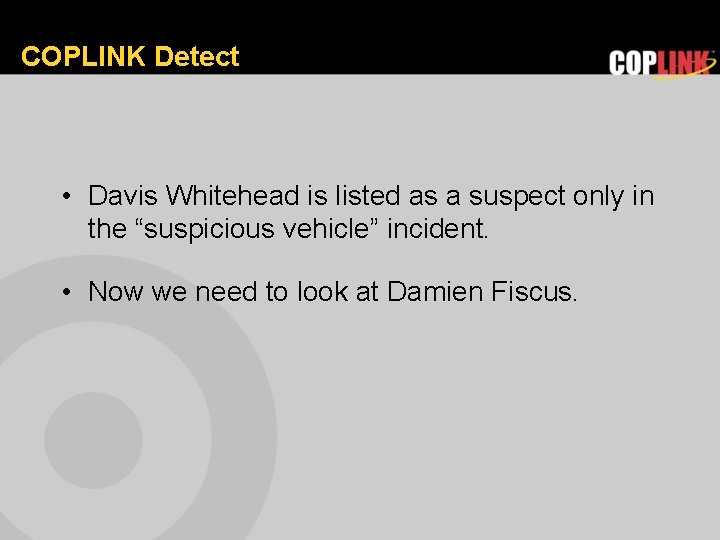
COPLINK Detect • Davis Whitehead is listed as a suspect only in the “suspicious vehicle” incident. • Now we need to look at Damien Fiscus.
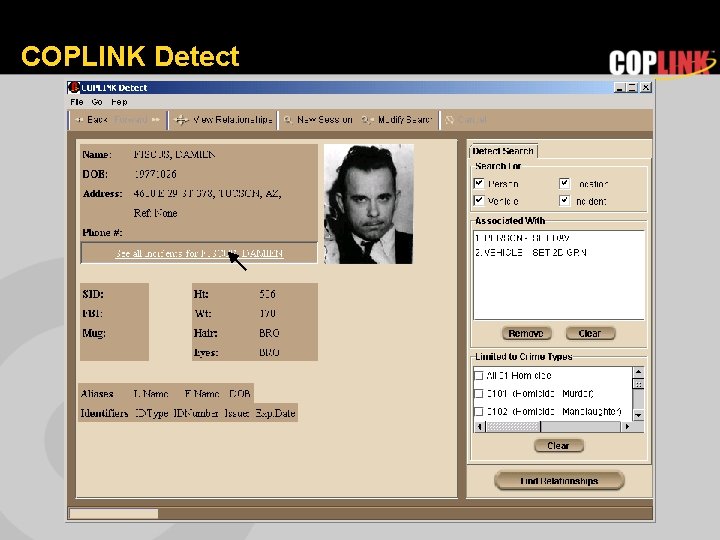
COPLINK Detect
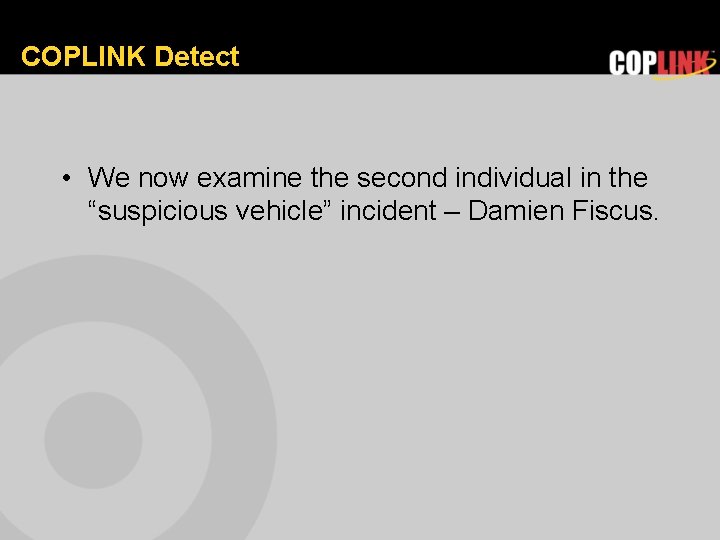
COPLINK Detect • We now examine the second individual in the “suspicious vehicle” incident – Damien Fiscus.
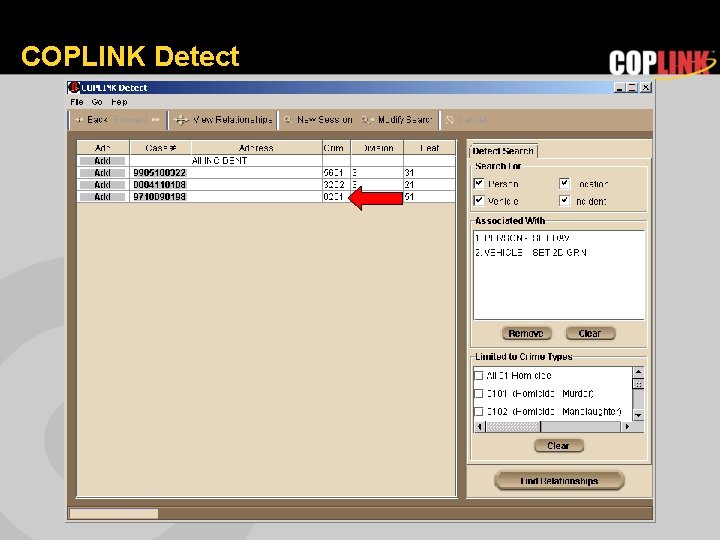
COPLINK Detect
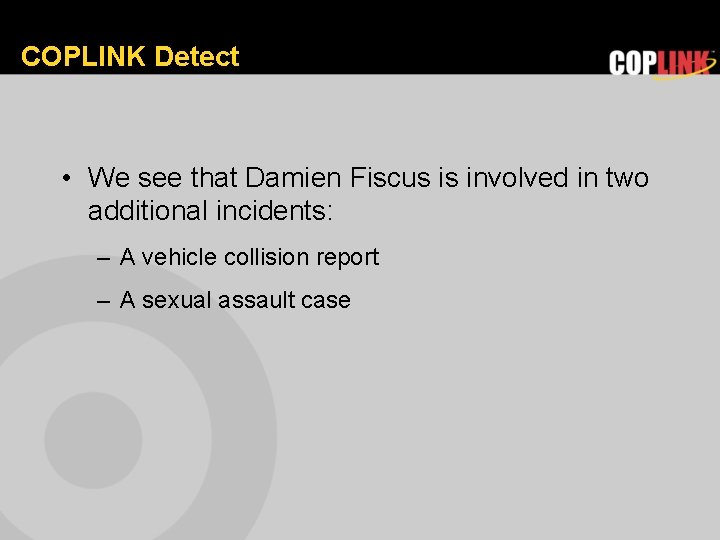
COPLINK Detect • We see that Damien Fiscus is involved in two additional incidents: – A vehicle collision report – A sexual assault case
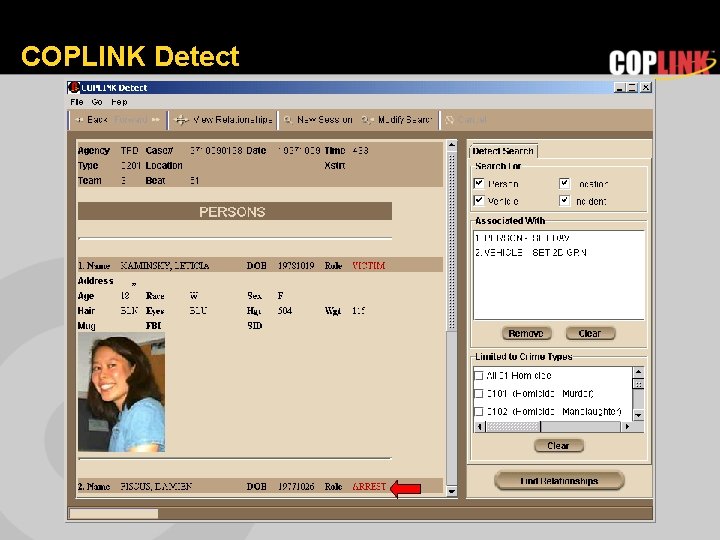
COPLINK Detect
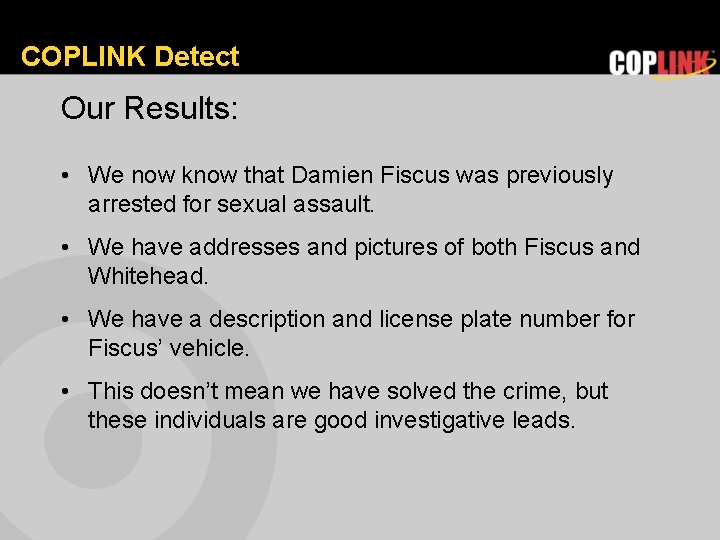
COPLINK Detect Our Results: • We now know that Damien Fiscus was previously arrested for sexual assault. • We have addresses and pictures of both Fiscus and Whitehead. • We have a description and license plate number for Fiscus’ vehicle. • This doesn’t mean we have solved the crime, but these individuals are good investigative leads.
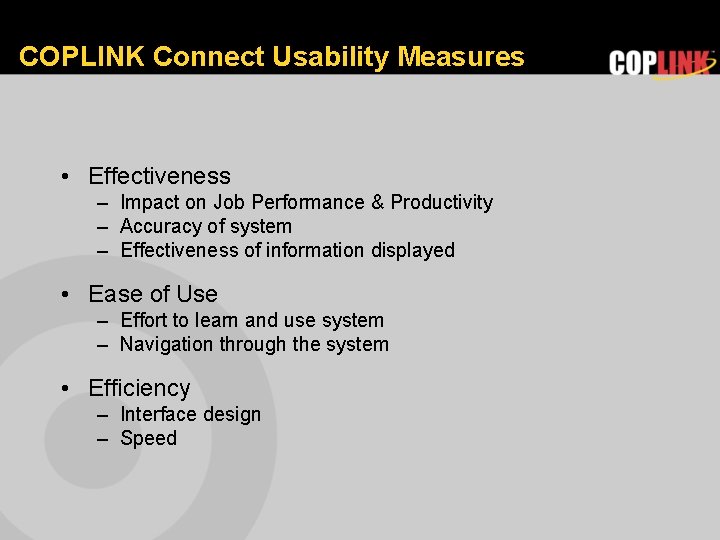
COPLINK Connect Usability Measures • Effectiveness – Impact on Job Performance & Productivity – Accuracy of system – Effectiveness of information displayed • Ease of Use – Effort to learn and use system – Navigation through the system • Efficiency – Interface design – Speed
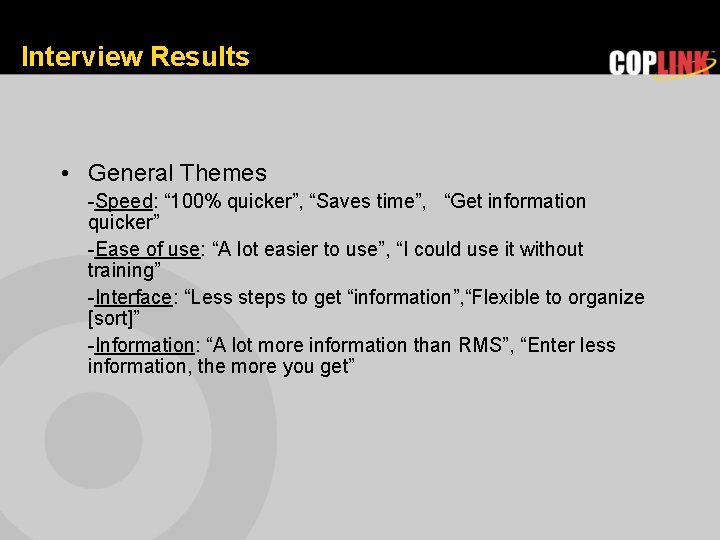
Interview Results • General Themes -Speed: “ 100% quicker”, “Saves time”, “Get information quicker” -Ease of use: “A lot easier to use”, “I could use it without training” -Interface: “Less steps to get “information”, “Flexible to organize [sort]” -Information: “A lot more information than RMS”, “Enter less information, the more you get”
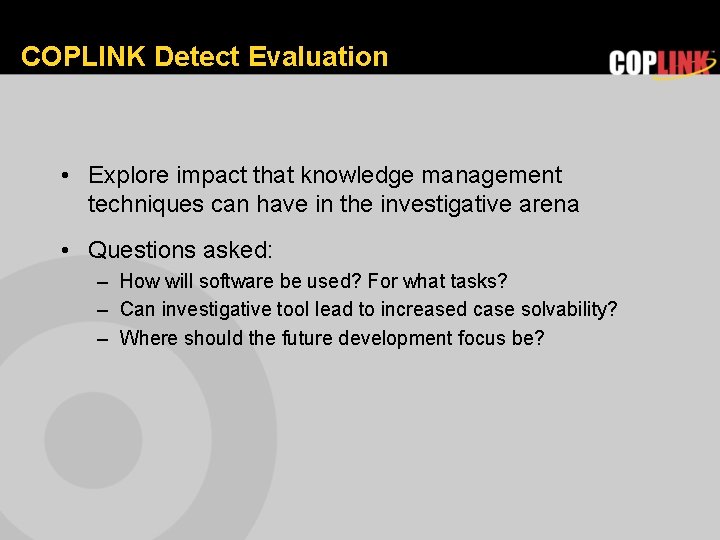
COPLINK Detect Evaluation • Explore impact that knowledge management techniques can have in the investigative arena • Questions asked: – How will software be used? For what tasks? – Can investigative tool lead to increased case solvability? – Where should the future development focus be?
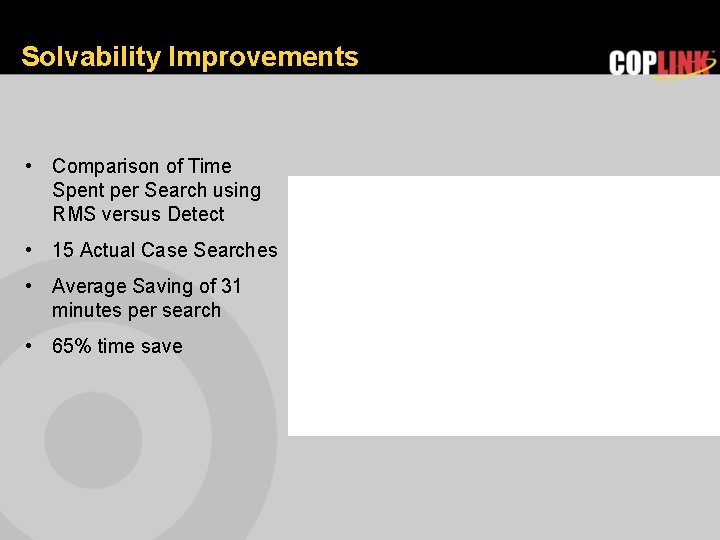
Solvability Improvements • Comparison of Time Spent per Search using RMS versus Detect • 15 Actual Case Searches • Average Saving of 31 minutes per search • 65% time save
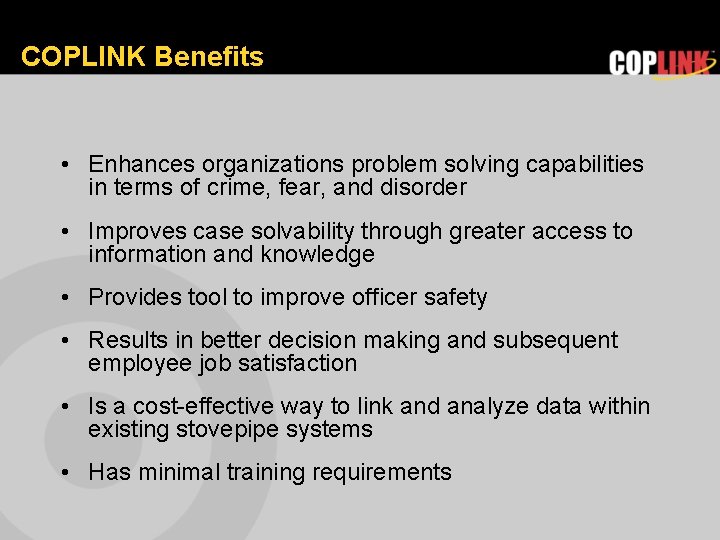
COPLINK Benefits • Enhances organizations problem solving capabilities in terms of crime, fear, and disorder • Improves case solvability through greater access to information and knowledge • Provides tool to improve officer safety • Results in better decision making and subsequent employee job satisfaction • Is a cost-effective way to link and analyze data within existing stovepipe systems • Has minimal training requirements
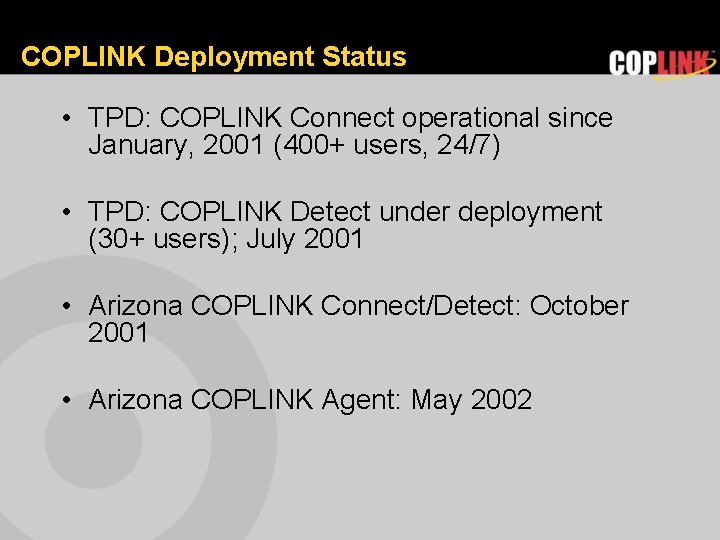
COPLINK Deployment Status • TPD: COPLINK Connect operational since January, 2001 (400+ users, 24/7) • TPD: COPLINK Detect under deployment (30+ users); July 2001 • Arizona COPLINK Connect/Detect: October 2001 • Arizona COPLINK Agent: May 2002
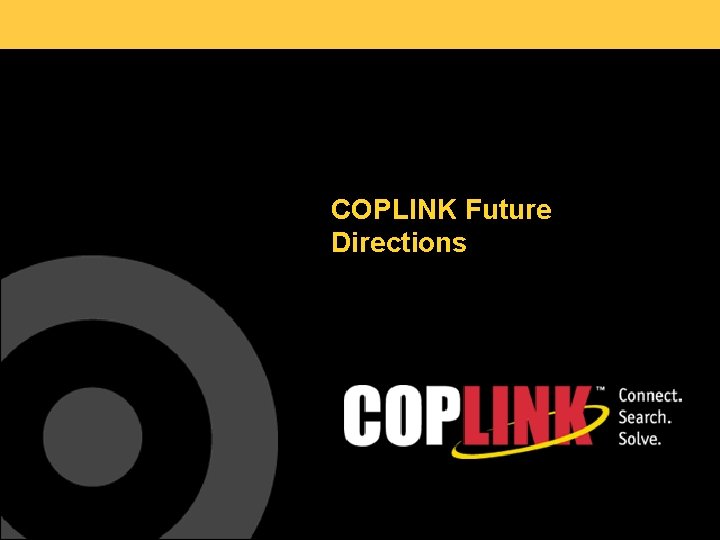
COPLINK Future Directions
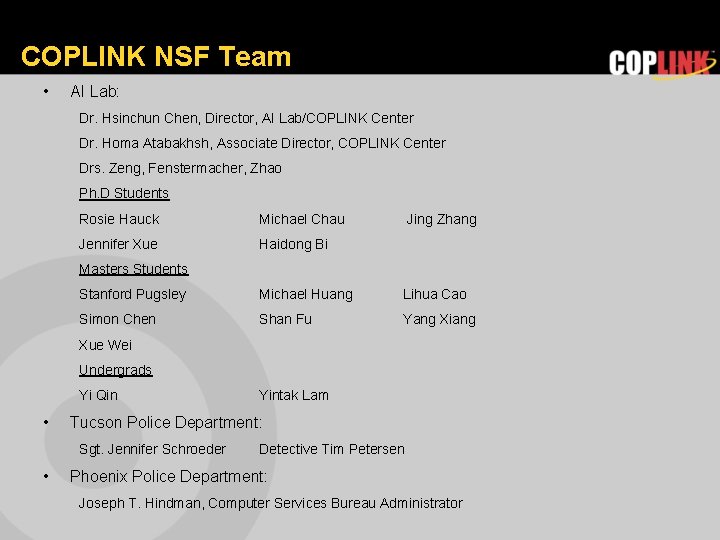
COPLINK NSF Team • AI Lab: Dr. Hsinchun Chen, Director, AI Lab/COPLINK Center Dr. Homa Atabakhsh, Associate Director, COPLINK Center Drs. Zeng, Fenstermacher, Zhao Ph. D Students Rosie Hauck Michael Chau Jennifer Xue Haidong Bi Jing Zhang Masters Students Stanford Pugsley Michael Huang Lihua Cao Simon Chen Shan Fu Yang Xiang Xue Wei Undergrads Yi Qin • Tucson Police Department: Sgt. Jennifer Schroeder • Yintak Lam Detective Tim Petersen Phoenix Police Department: Joseph T. Hindman, Computer Services Bureau Administrator
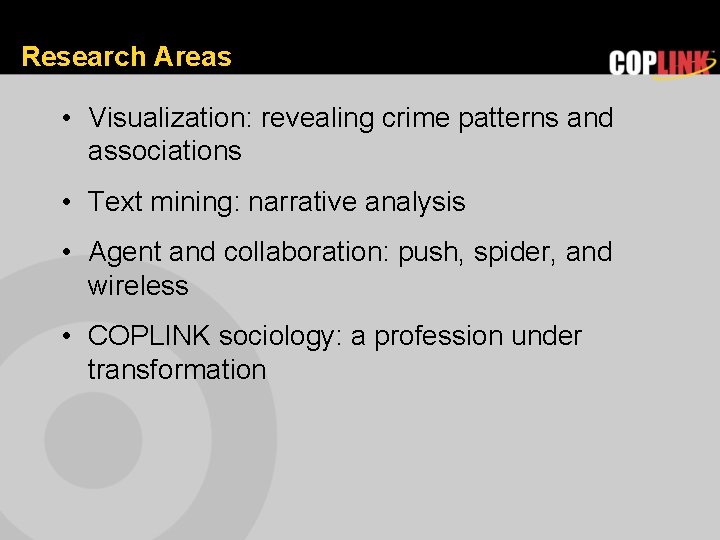
Research Areas • Visualization: revealing crime patterns and associations • Text mining: narrative analysis • Agent and collaboration: push, spider, and wireless • COPLINK sociology: a profession under transformation
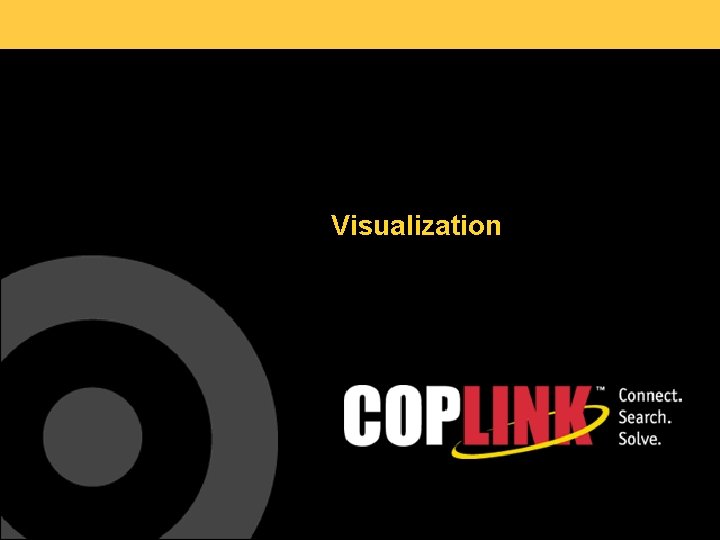
Visualization
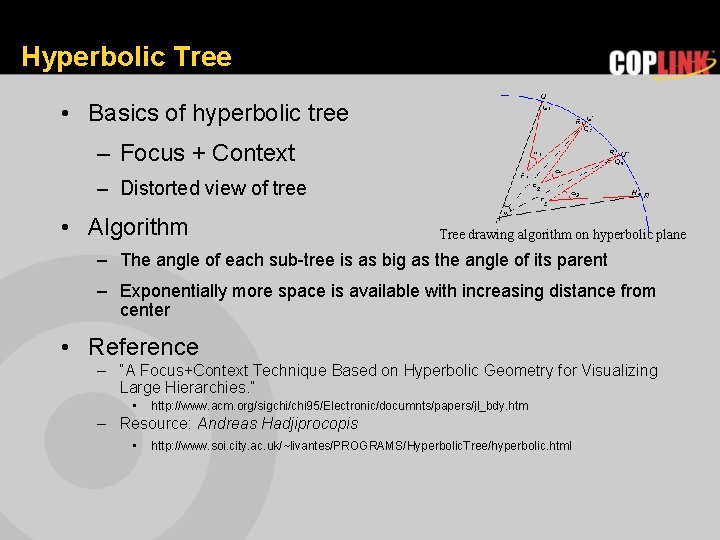
Hyperbolic Tree • Basics of hyperbolic tree – Focus + Context – Distorted view of tree • Algorithm Tree drawing algorithm on hyperbolic plane – The angle of each sub-tree is as big as the angle of its parent – Exponentially more space is available with increasing distance from center • Reference – “A Focus+Context Technique Based on Hyperbolic Geometry for Visualizing Large Hierarchies. ” • http: //www. acm. org/sigchi/chi 95/Electronic/documnts/papers/jl_bdy. htm – Resource: Andreas Hadjiprocopis • http: //www. soi. city. ac. uk/~livantes/PROGRAMS/Hyperbolic. Tree/hyperbolic. html
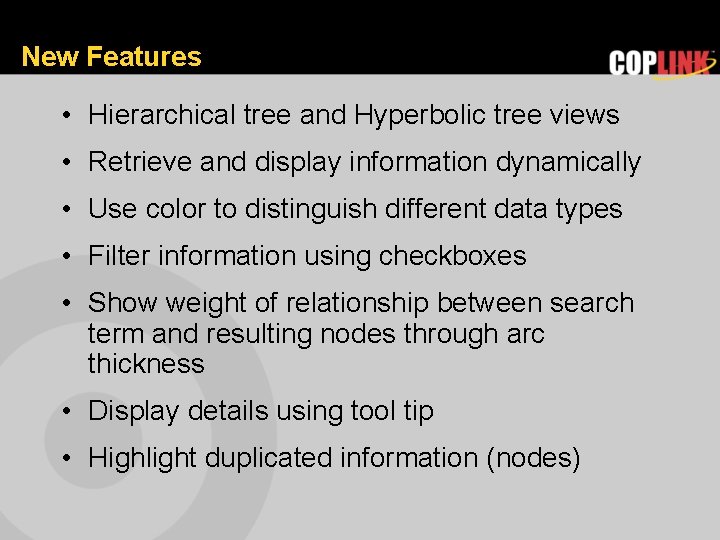
New Features • Hierarchical tree and Hyperbolic tree views • Retrieve and display information dynamically • Use color to distinguish different data types • Filter information using checkboxes • Show weight of relationship between search term and resulting nodes through arc thickness • Display details using tool tip • Highlight duplicated information (nodes)
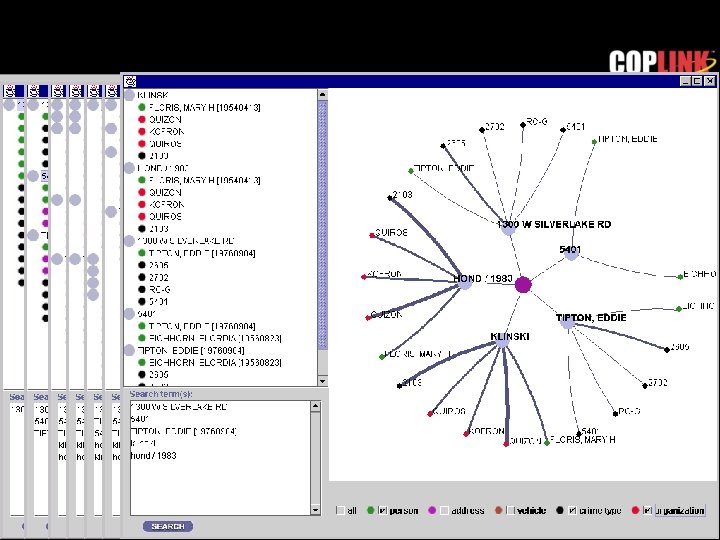
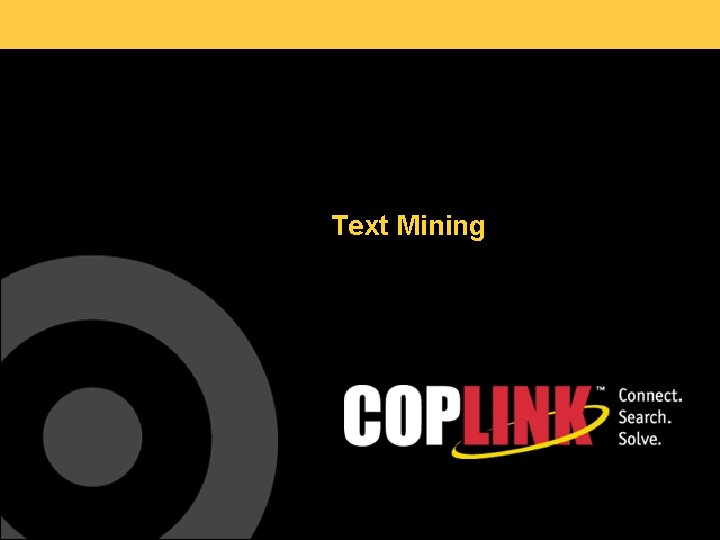
Text Mining
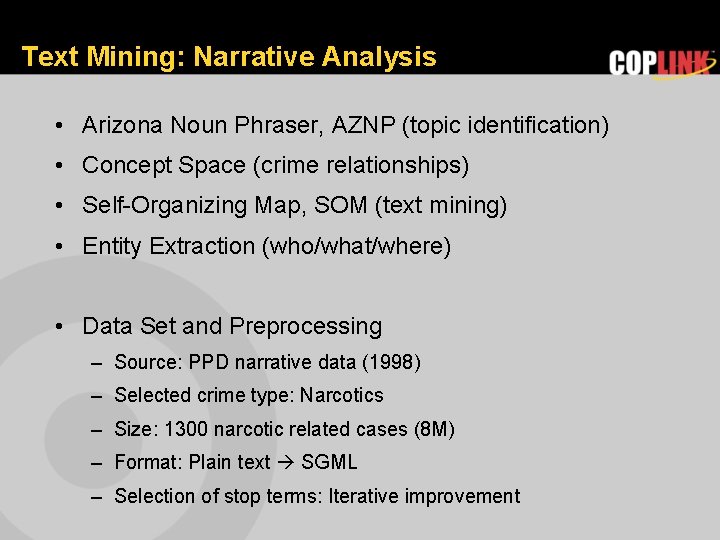
Text Mining: Narrative Analysis • Arizona Noun Phraser, AZNP (topic identification) • Concept Space (crime relationships) • Self-Organizing Map, SOM (text mining) • Entity Extraction (who/what/where) • Data Set and Preprocessing – Source: PPD narrative data (1998) – Selected crime type: Narcotics – Size: 1300 narcotic related cases (8 M) – Format: Plain text SGML – Selection of stop terms: Iterative improvement
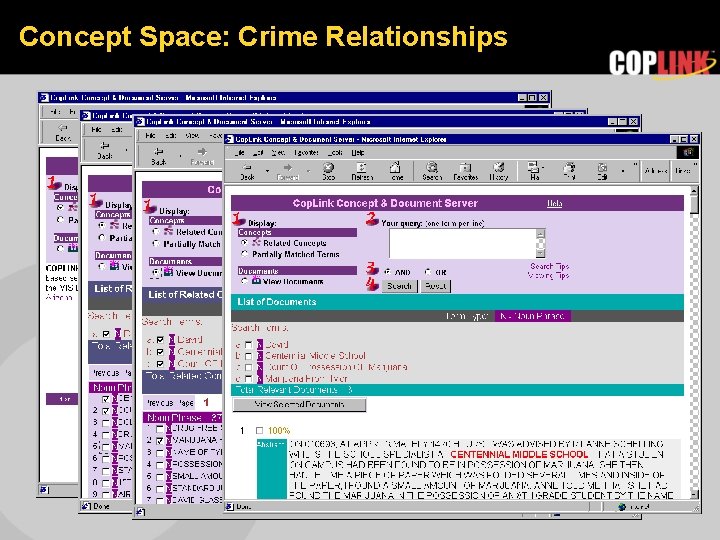
Concept Space: Crime Relationships
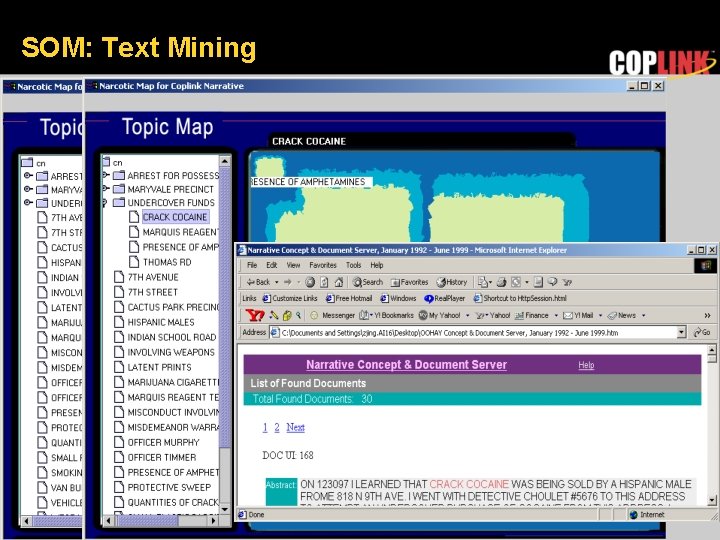
SOM: Text Mining
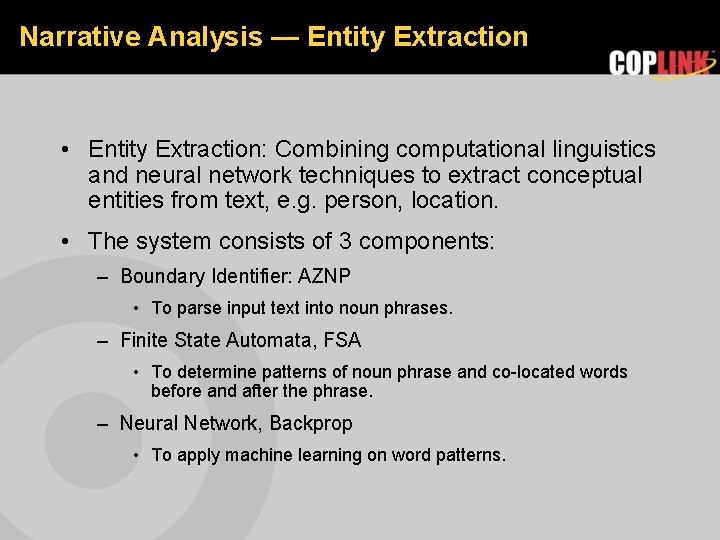
Narrative Analysis — Entity Extraction • Entity Extraction: Combining computational linguistics and neural network techniques to extract conceptual entities from text, e. g. person, location. • The system consists of 3 components: – Boundary Identifier: AZNP • To parse input text into noun phrases. – Finite State Automata, FSA • To determine patterns of noun phrase and co-located words before and after the phrase. – Neural Network, Backprop • To apply machine learning on word patterns.
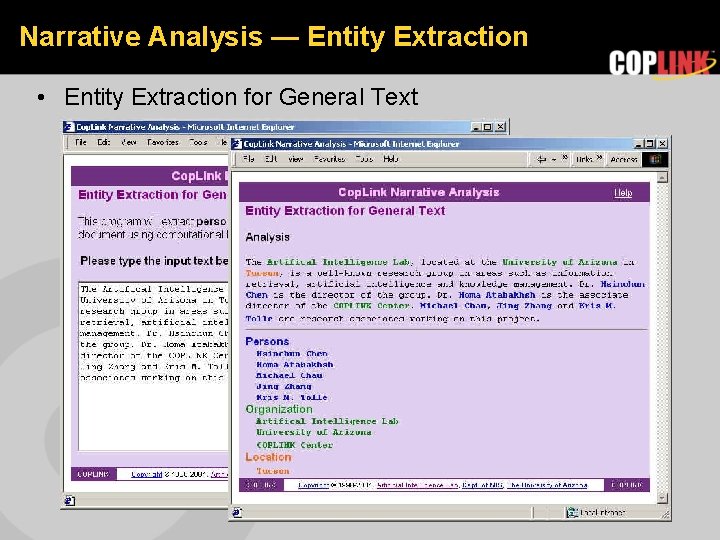
Narrative Analysis — Entity Extraction • Entity Extraction for General Text
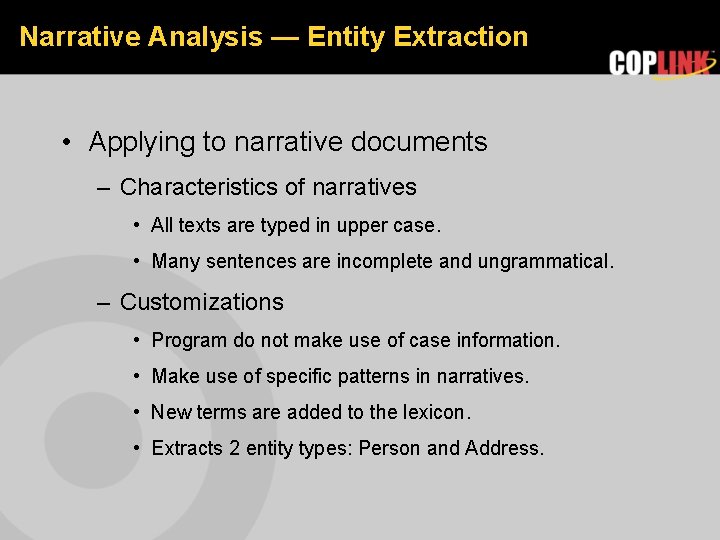
Narrative Analysis — Entity Extraction • Applying to narrative documents – Characteristics of narratives • All texts are typed in upper case. • Many sentences are incomplete and ungrammatical. – Customizations • Program do not make use of case information. • Make use of specific patterns in narratives. • New terms are added to the lexicon. • Extracts 2 entity types: Person and Address.
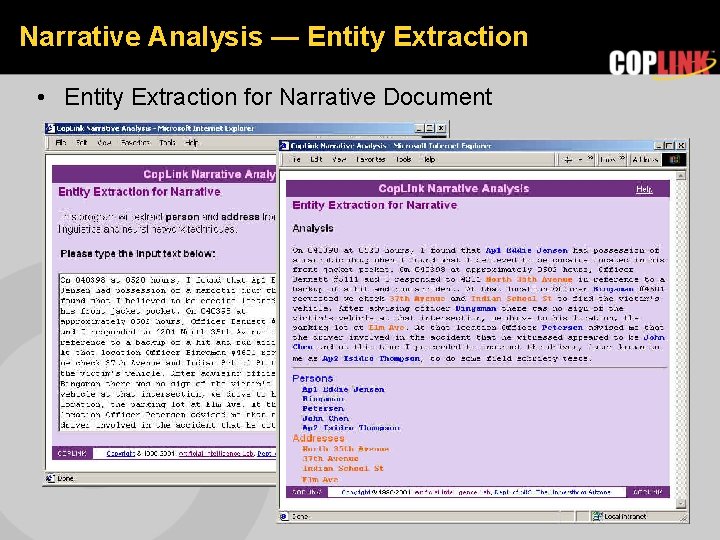
Narrative Analysis — Entity Extraction • Entity Extraction for Narrative Document
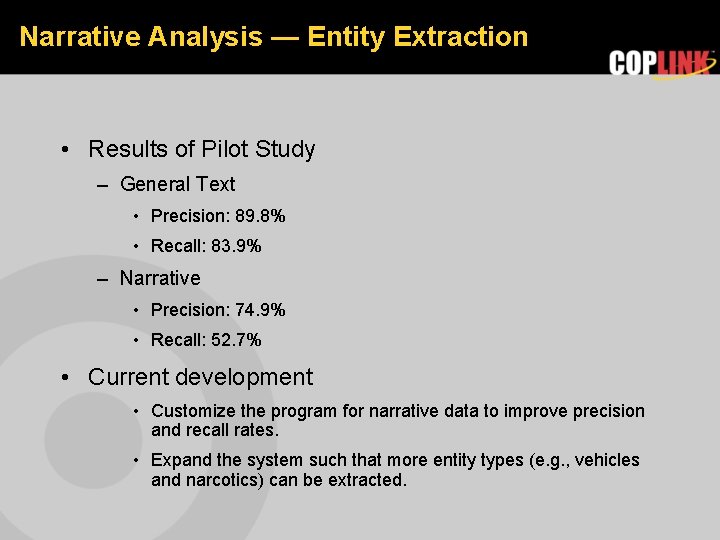
Narrative Analysis — Entity Extraction • Results of Pilot Study – General Text • Precision: 89. 8% • Recall: 83. 9% – Narrative • Precision: 74. 9% • Recall: 52. 7% • Current development • Customize the program for narrative data to improve precision and recall rates. • Expand the system such that more entity types (e. g. , vehicles and narcotics) can be extracted.
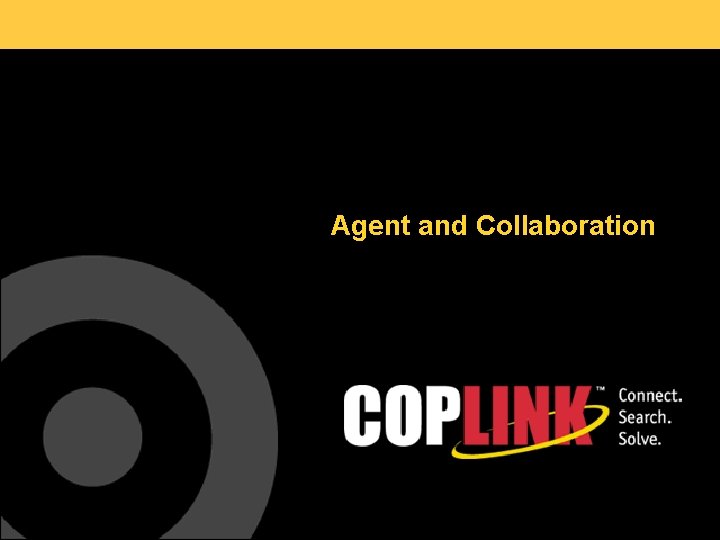
Agent and Collaboration
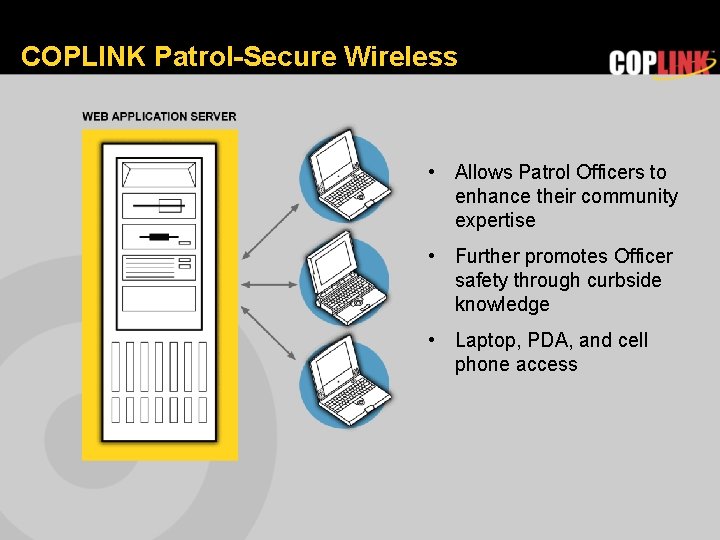
COPLINK Patrol-Secure Wireless • Allows Patrol Officers to enhance their community expertise • Further promotes Officer safety through curbside knowledge • Laptop, PDA, and cell phone access
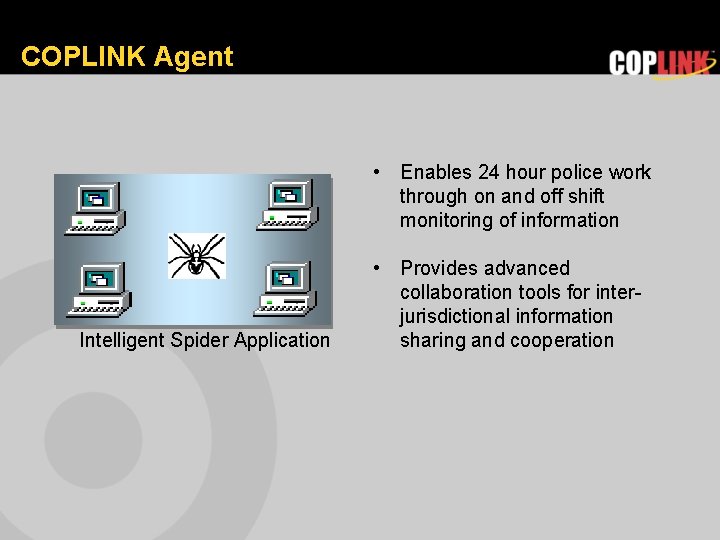
COPLINK Agent • Enables 24 hour police work through on and off shift monitoring of information Intelligent Spider Application • Provides advanced collaboration tools for interjurisdictional information sharing and cooperation
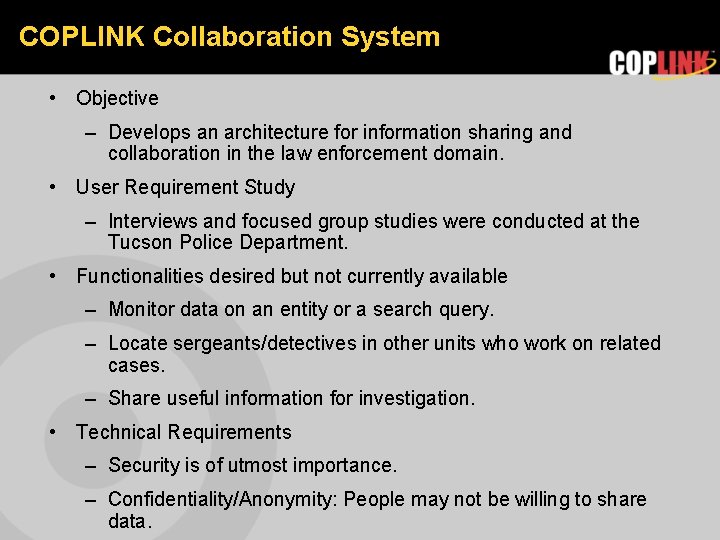
COPLINK Collaboration System • Objective – Develops an architecture for information sharing and collaboration in the law enforcement domain. • User Requirement Study – Interviews and focused group studies were conducted at the Tucson Police Department. • Functionalities desired but not currently available – Monitor data on an entity or a search query. – Locate sergeants/detectives in other units who work on related cases. – Share useful information for investigation. • Technical Requirements – Security is of utmost importance. – Confidentiality/Anonymity: People may not be willing to share data.
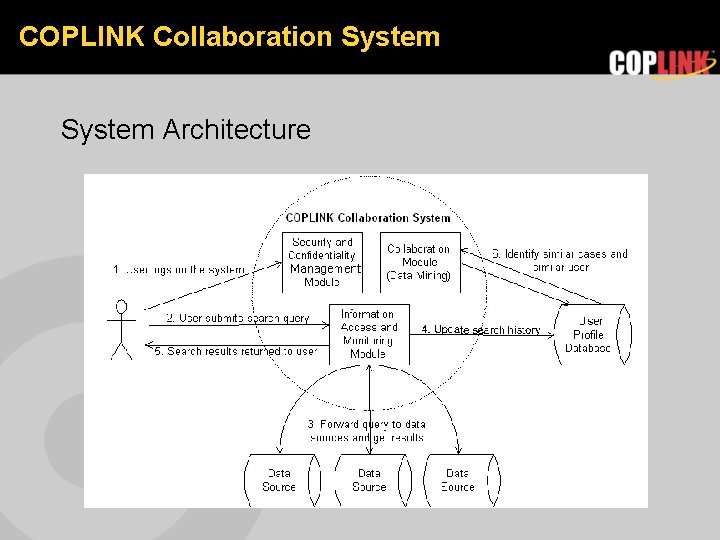
COPLINK Collaboration System Architecture
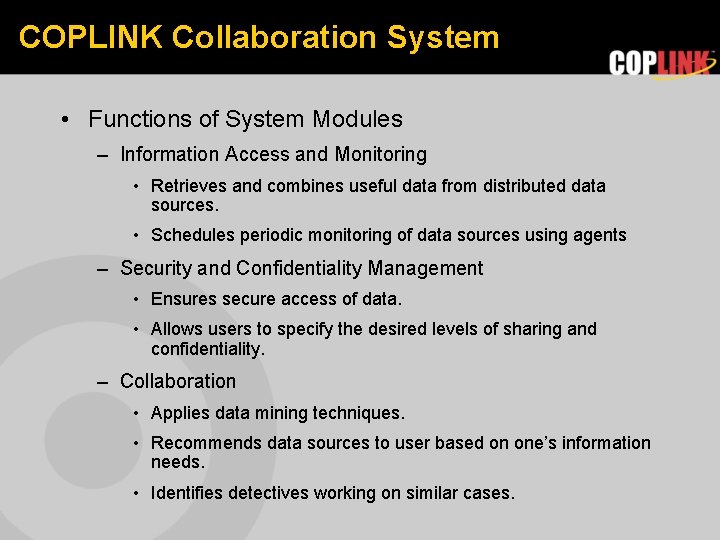
COPLINK Collaboration System • Functions of System Modules – Information Access and Monitoring • Retrieves and combines useful data from distributed data sources. • Schedules periodic monitoring of data sources using agents – Security and Confidentiality Management • Ensures secure access of data. • Allows users to specify the desired levels of sharing and confidentiality. – Collaboration • Applies data mining techniques. • Recommends data sources to user based on one’s information needs. • Identifies detectives working on similar cases.
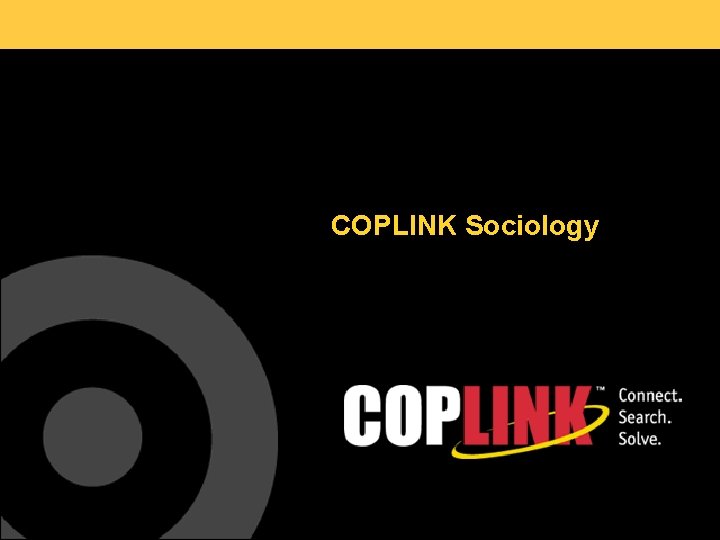
COPLINK Sociology
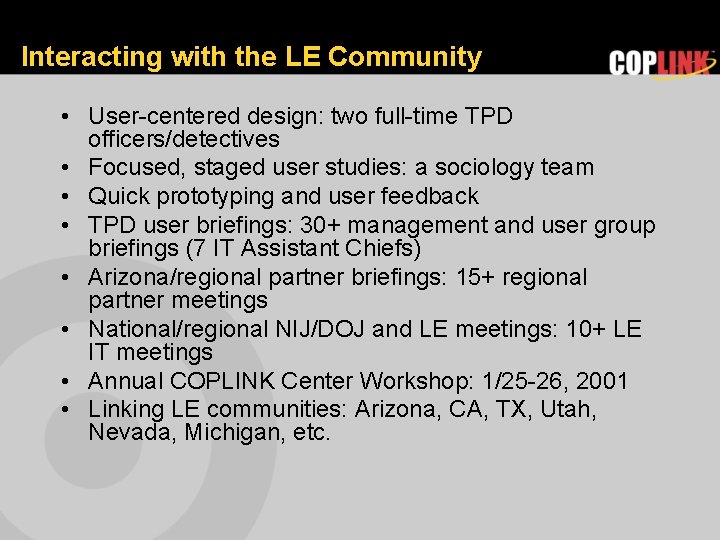
Interacting with the LE Community • User-centered design: two full-time TPD officers/detectives • Focused, staged user studies: a sociology team • Quick prototyping and user feedback • TPD user briefings: 30+ management and user group briefings (7 IT Assistant Chiefs) • Arizona/regional partner briefings: 15+ regional partner meetings • National/regional NIJ/DOJ and LE meetings: 10+ LE IT meetings • Annual COPLINK Center Workshop: 1/25 -26, 2001 • Linking LE communities: Arizona, CA, TX, Utah, Nevada, Michigan, etc.
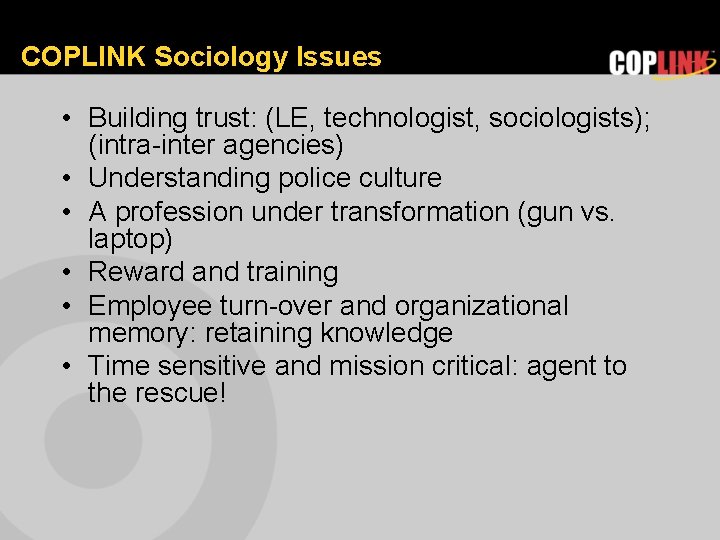
COPLINK Sociology Issues • Building trust: (LE, technologist, sociologists); (intra-inter agencies) • Understanding police culture • A profession under transformation (gun vs. laptop) • Reward and training • Employee turn-over and organizational memory: retaining knowledge • Time sensitive and mission critical: agent to the rescue!
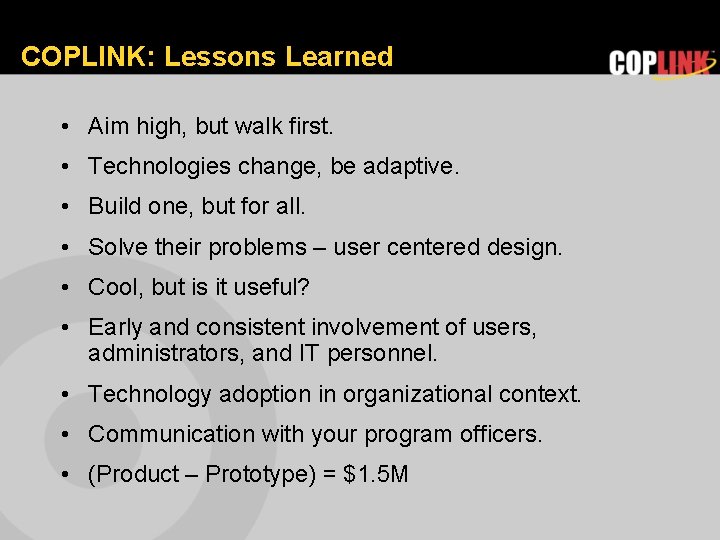
COPLINK: Lessons Learned • Aim high, but walk first. • Technologies change, be adaptive. • Build one, but for all. • Solve their problems – user centered design. • Cool, but is it useful? • Early and consistent involvement of users, administrators, and IT personnel. • Technology adoption in organizational context. • Communication with your program officers. • (Product – Prototype) = $1. 5 M
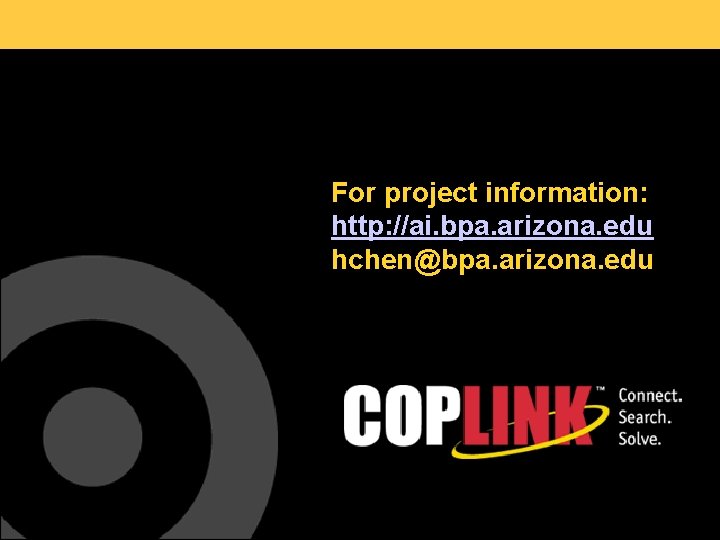
For project information: http: //ai. bpa. arizona. edu hchen@bpa. arizona. edu
Nllea
Law enforcement information exchange
Linx law enforcement information exchange
National enforcement body
Texas commission on law enforcement
Crane night vision lease program
Leads provides access to
Law enforcement mental health and wellness act
Law enforcement video association
Virginia law enforcement assistance program
Elvis vehicle inspection
Military and law enforcement
Military and law enforcement
Law enforcement agencies
Law enforcement first responder
Law enforcement agency
Calphoto dmv law enforcement
Massachusetts association for professional law enforcement
Indiana law enforcement training board
Gst law enforcement
Newton's first law and second law and third law
Newton's first law
Boyles law
Boyle's law charles law avogadro's law
Niem data model
National information exchange model
Second line enforcement adalah
Mto enforcement officer
Florida association of code enforcement
Fines enforcement number
City of columbus code enforcement
Atlanta code enforcement
Planning enforcement
Njdep ust guidance
[email protected]
Hpd enforcement desk bed bugs
Nfhs penalty enforcement
Scrimmage kick
What is dragon assistant 3
City of wenatchee code enforcement
Asean wildlife enforcement network
Nc license and theft bureau locations
Planning enforcement officers association
Volusia county building department
Tec traffic enforcement centre
Director of labour market enforcement
Kontinuitetshantering
Typiska drag för en novell
Tack för att ni lyssnade bild
Vad står k.r.å.k.a.n för
Shingelfrisyren
En lathund för arbete med kontinuitetshantering
Adressändring ideell förening
Tidböcker
A gastrica
Förklara densitet för barn
Datorkunskap för nybörjare
Tack för att ni lyssnade bild
Debatt mall
Delegerande ledarstil
Nyckelkompetenser för livslångt lärande
Påbyggnader för flakfordon
Arkimedes princip formel
Svenskt ramverk för digital samverkan
Jag har nigit för nymånens skära
Presentera för publik crossboss
Jiddisch
Kanaans land
Klassificeringsstruktur för kommunala verksamheter
Fimbrietratt
Claes martinsson
Centrum för kunskap och säkerhet
Programskede byggprocessen
Bra mat för unga idrottare
Verktyg för automatisering av utbetalningar
Rutin för avvikelsehantering
Smärtskolan kunskap för livet
Ministerstyre för och nackdelar
Tack för att ni har lyssnat
Referat mall
Redogör för vad psykologi är
Borstål, egenskaper
Tack för att ni har lyssnat
Borra hål för knoppar
Vilken grundregel finns det för tronföljden i sverige?
Fr formel
Tack för att ni har lyssnat
Steg för steg rita
Vad är verksamhetsanalys
Tobinskatten för och nackdelar
Toppslätskivling dos