1 Preprocessing of FMRI Data f MRI Graduate
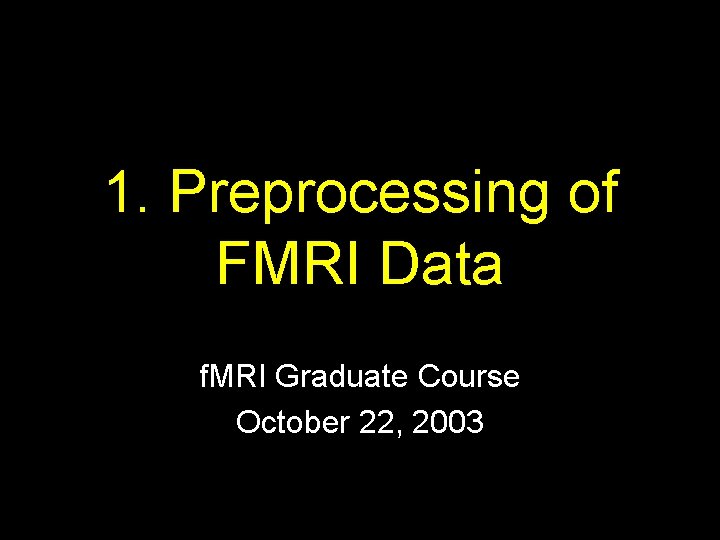
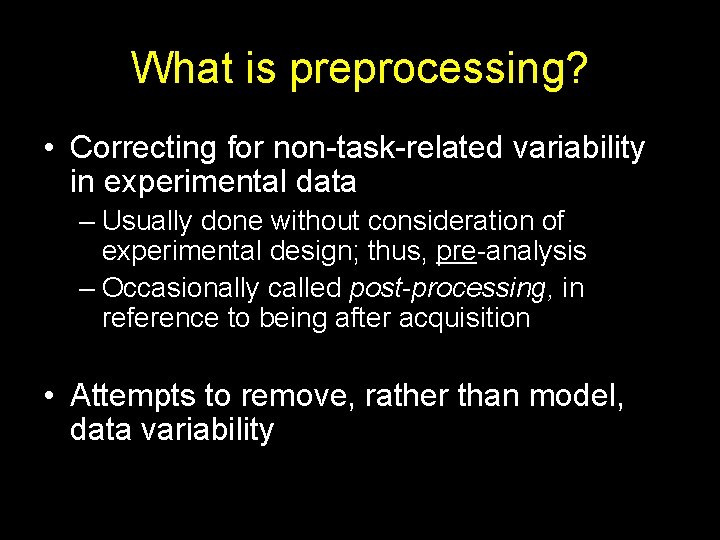
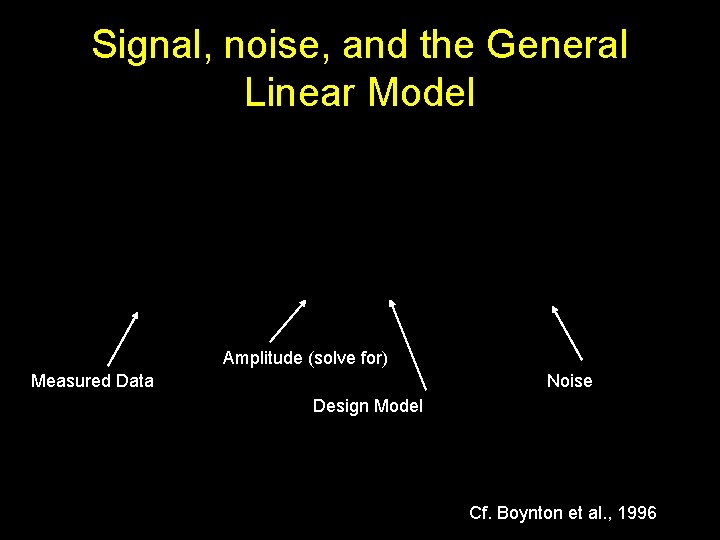
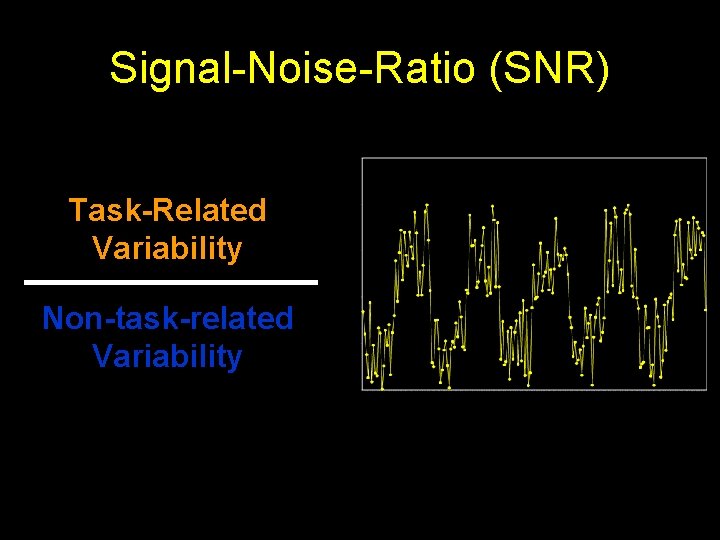
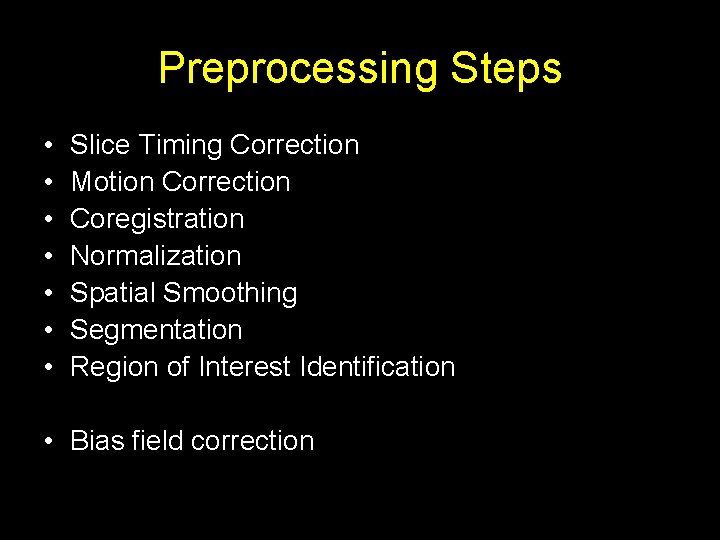
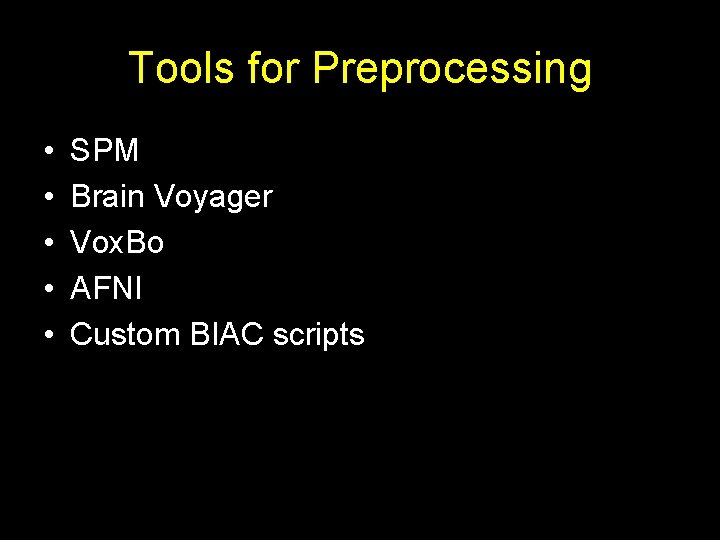
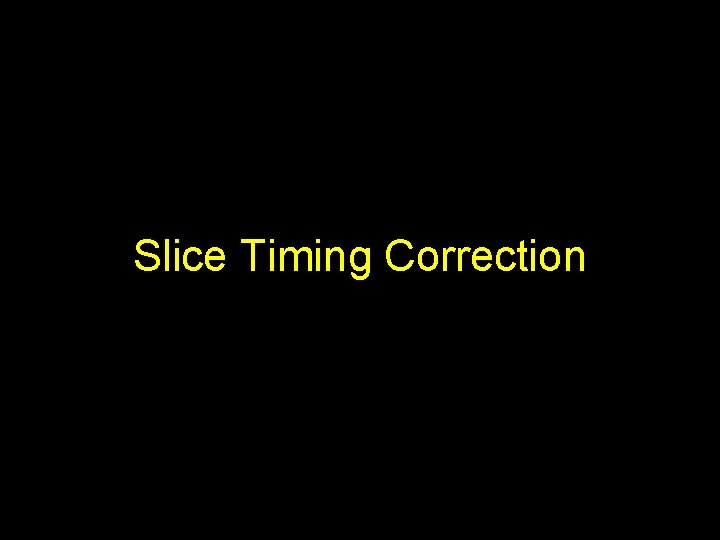
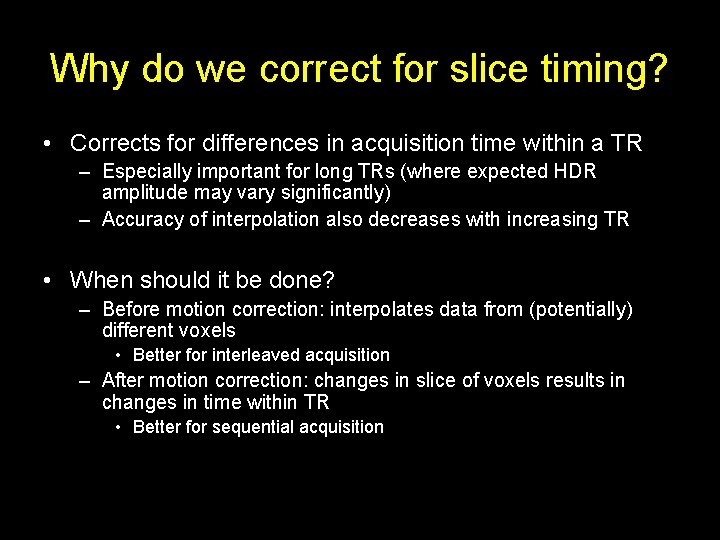
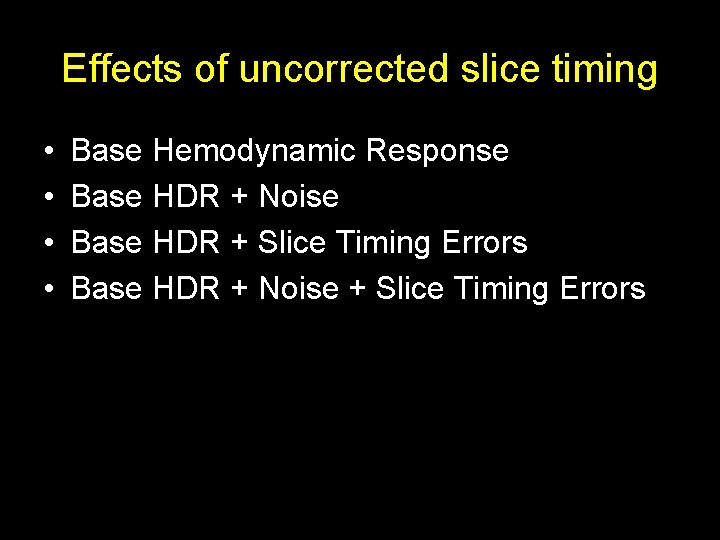
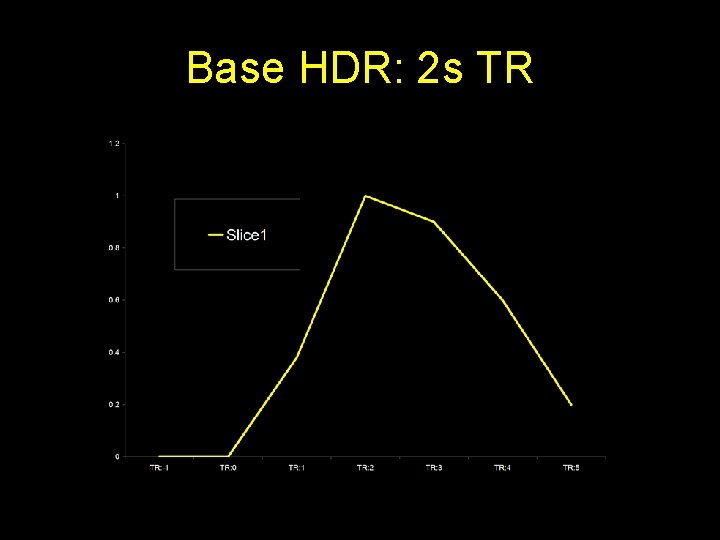
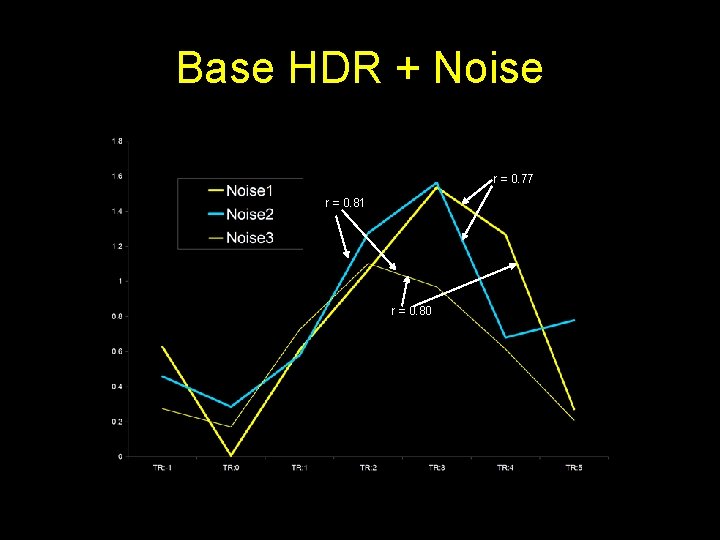
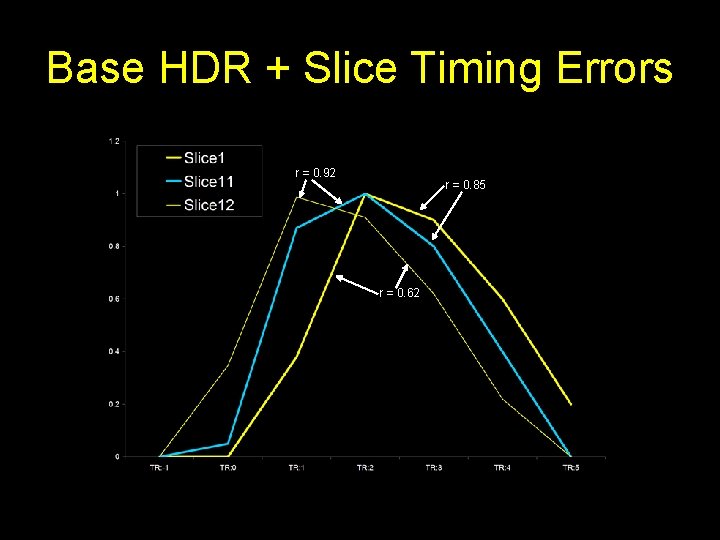
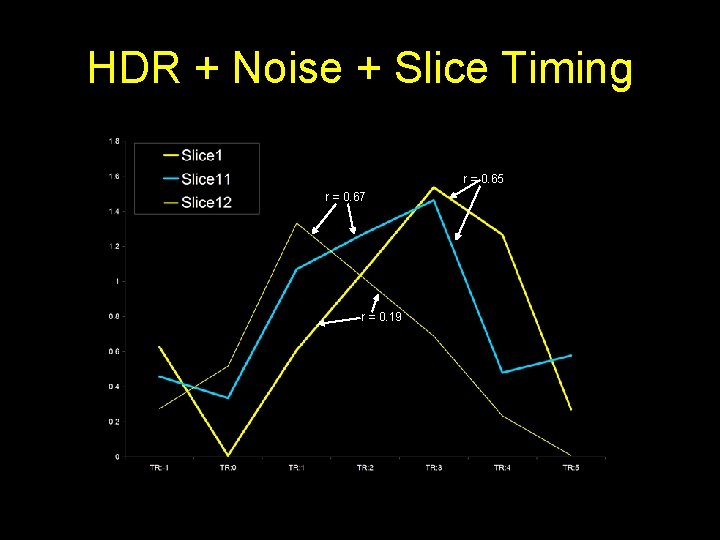
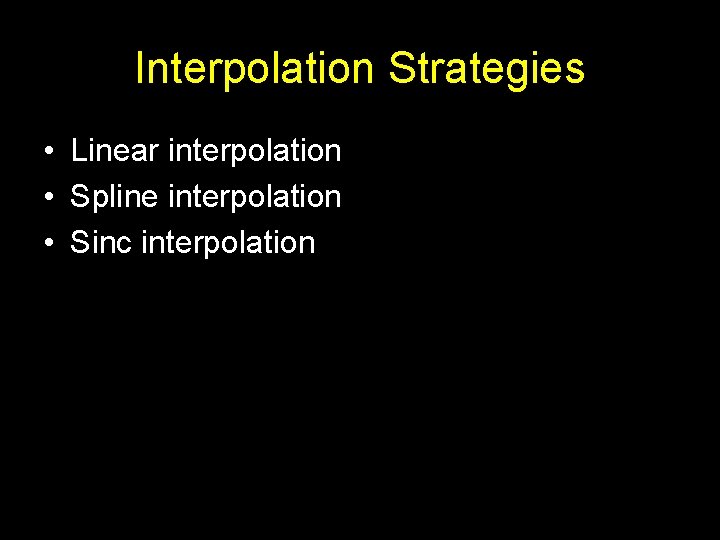
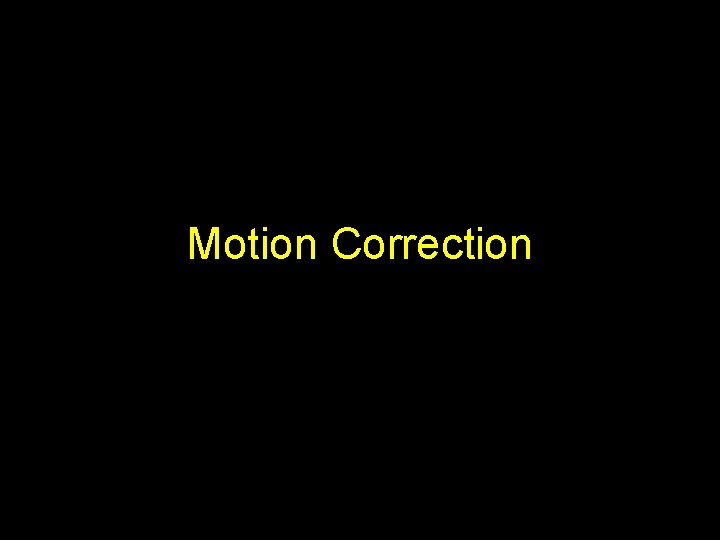
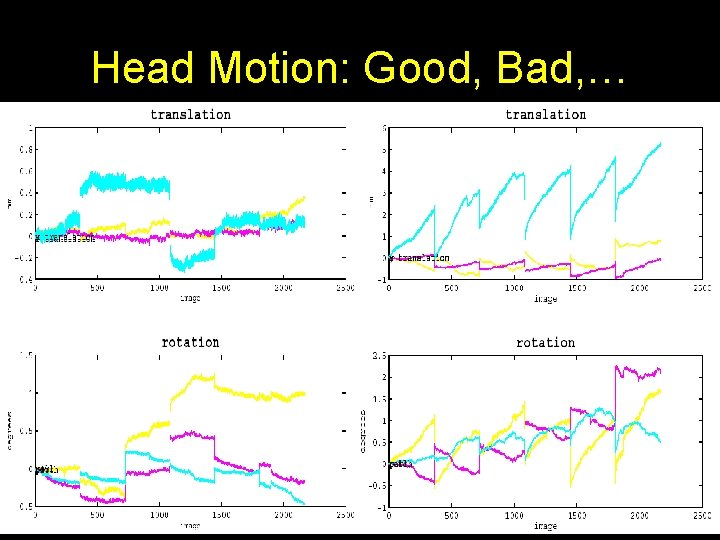
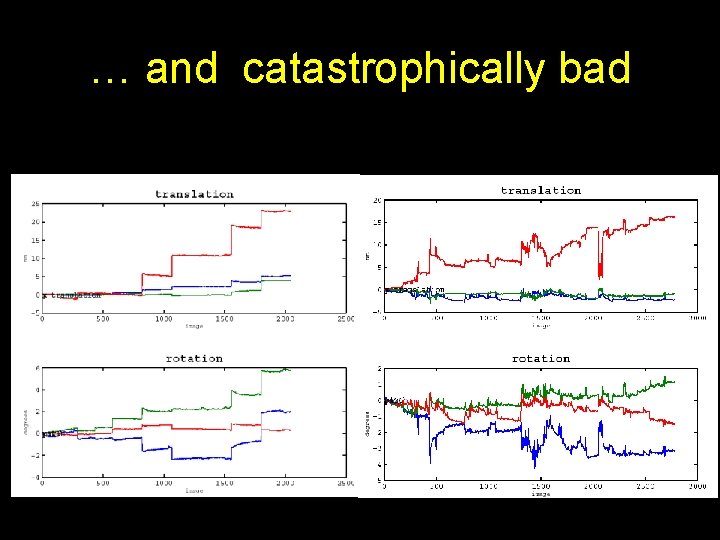
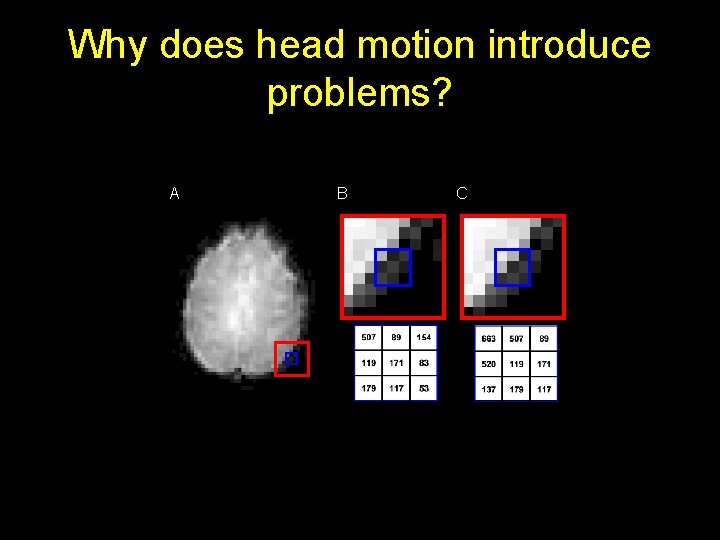
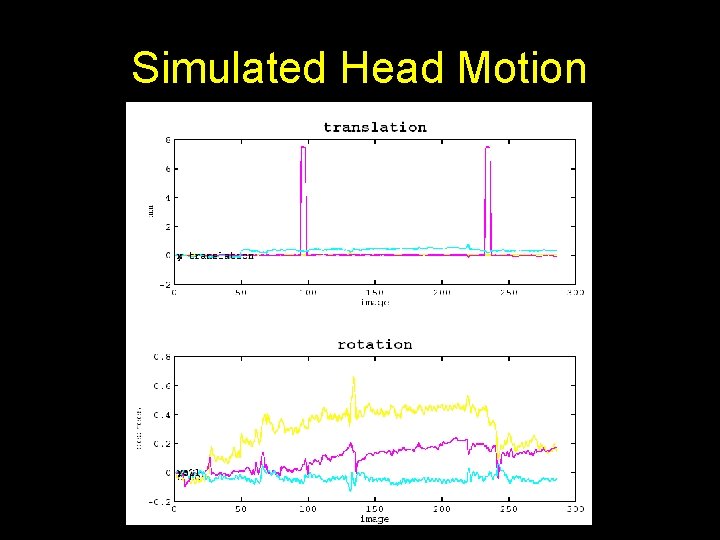
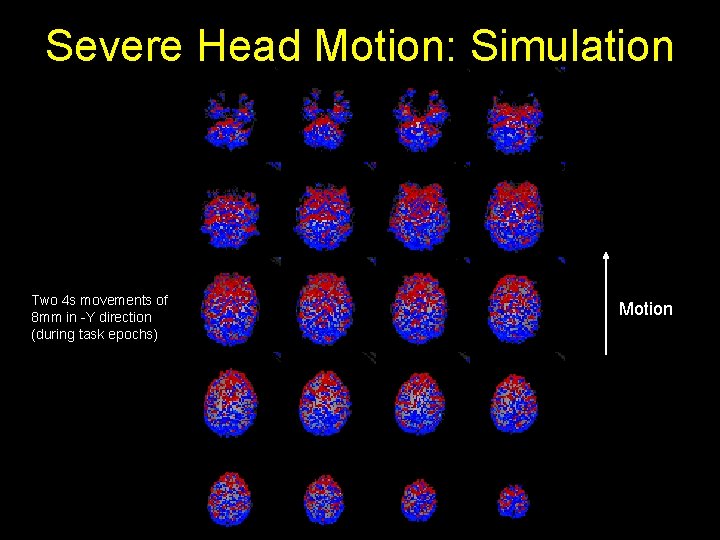
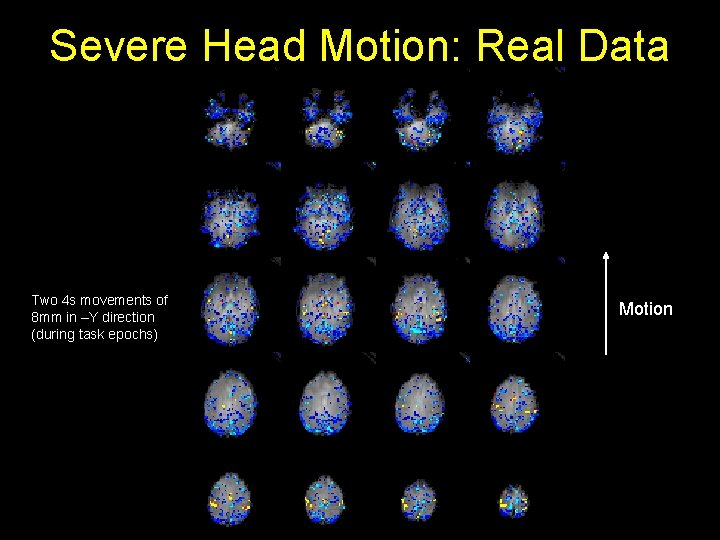
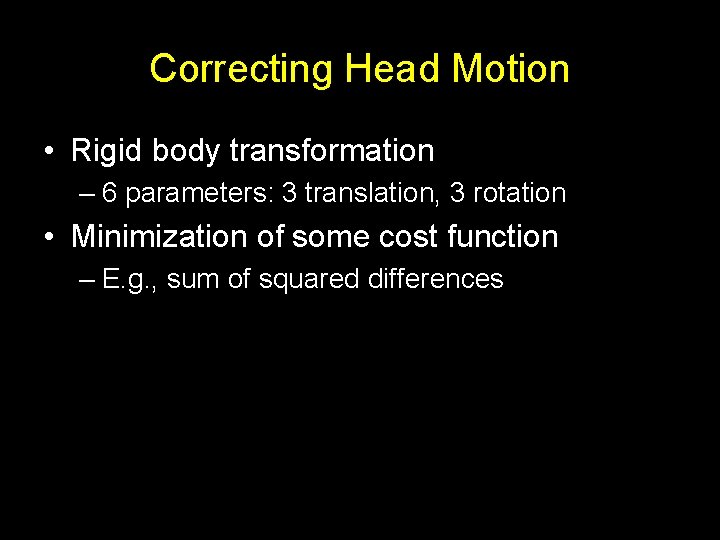
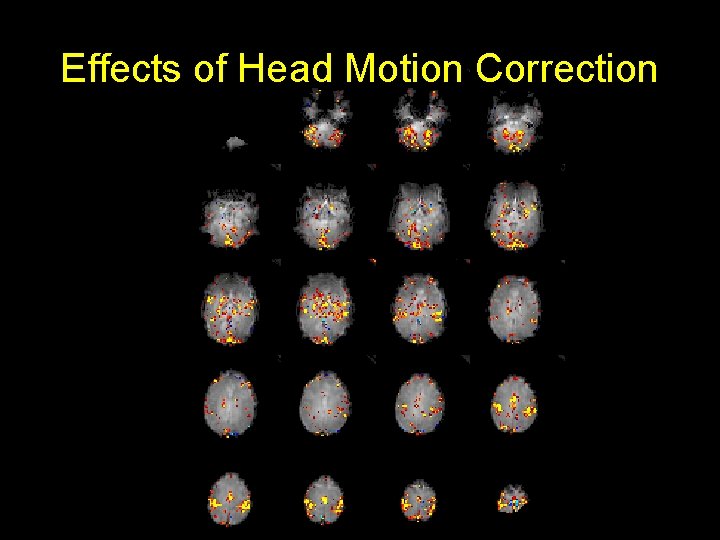
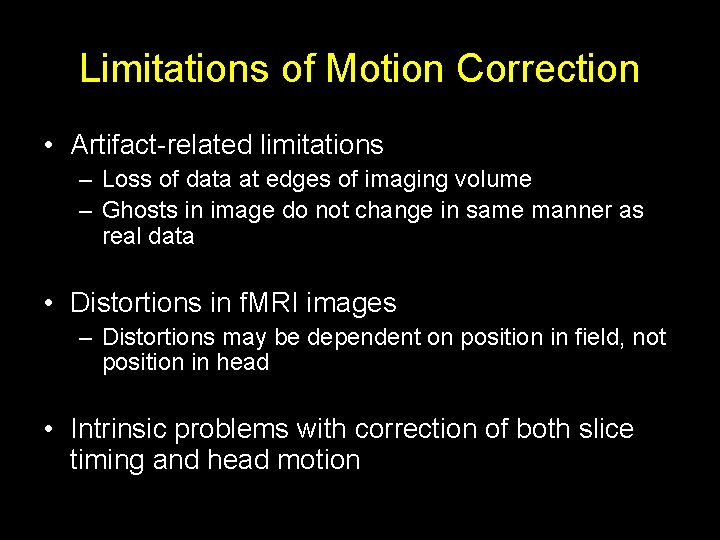
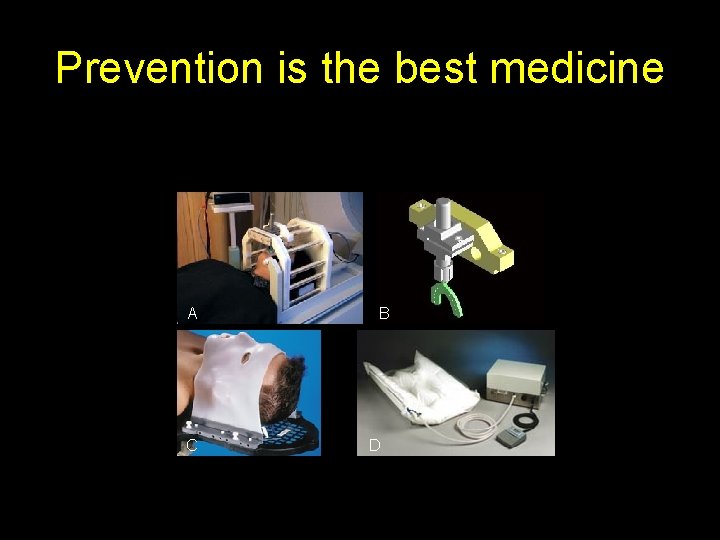
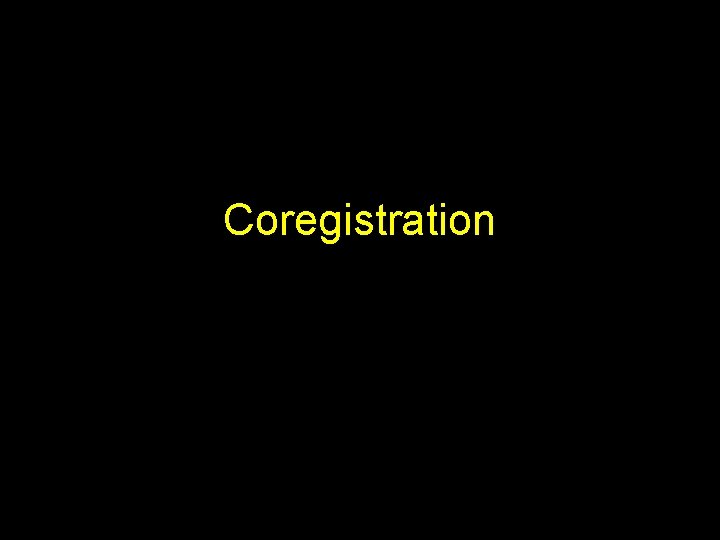
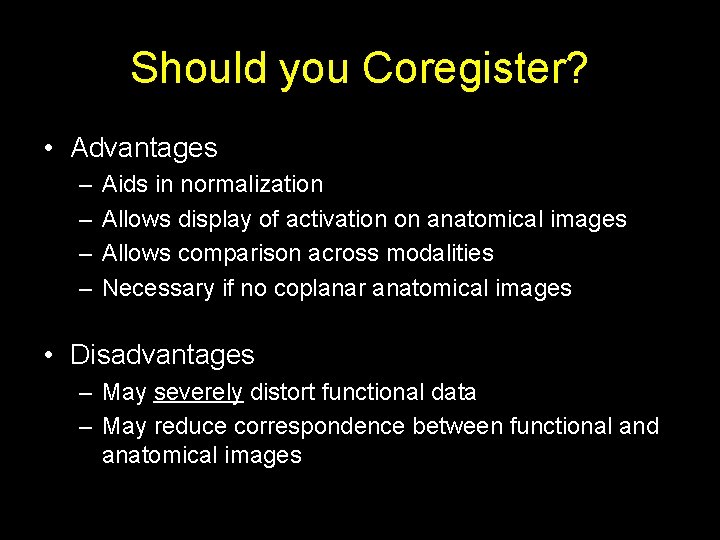
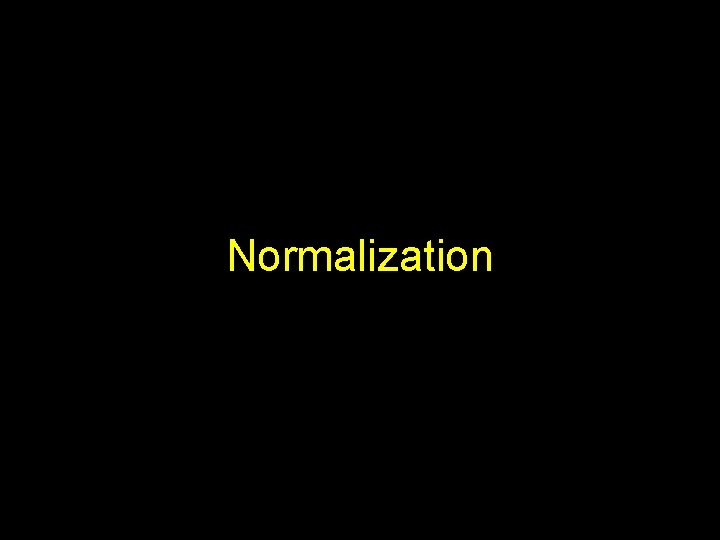
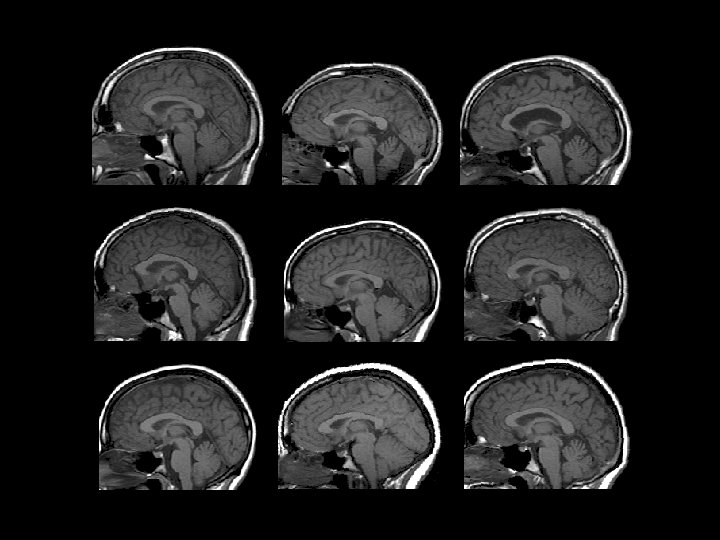
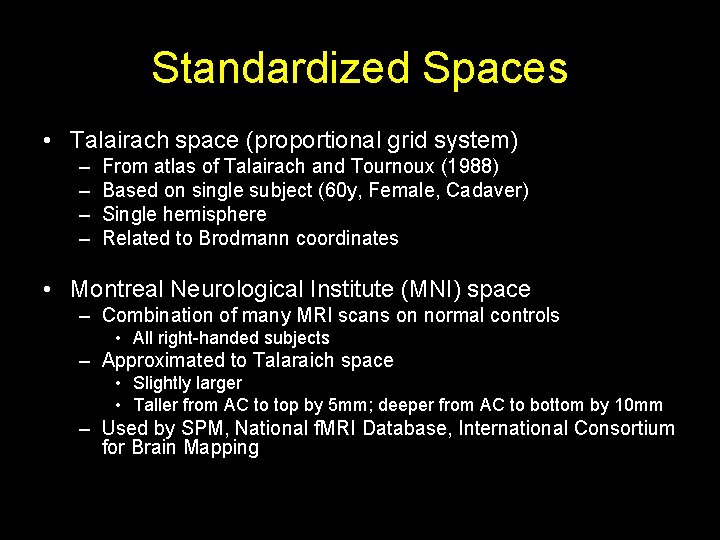
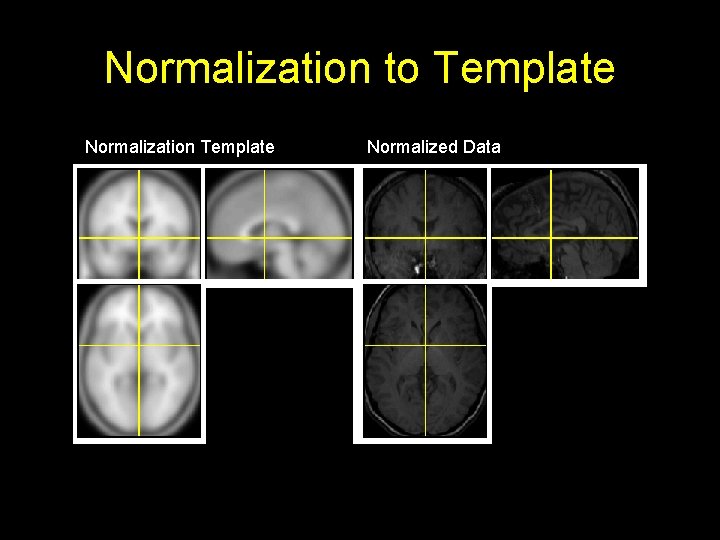
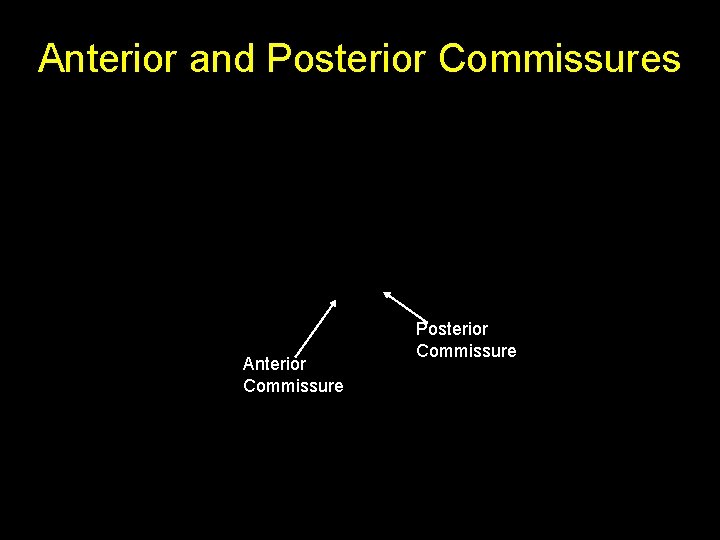
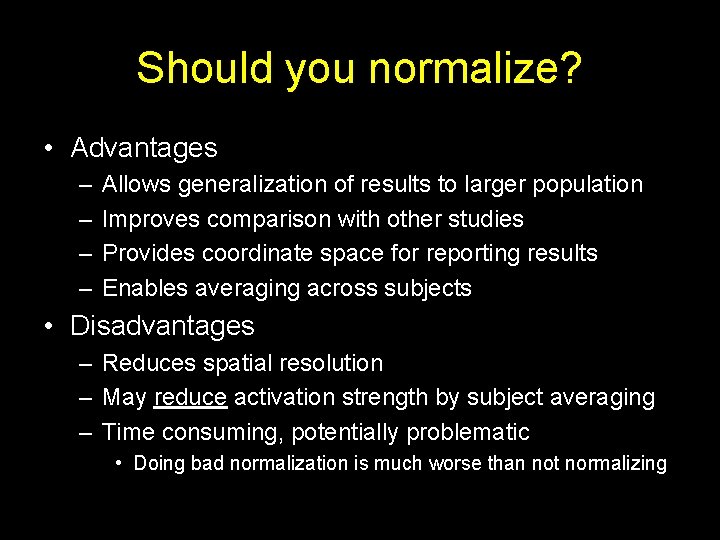
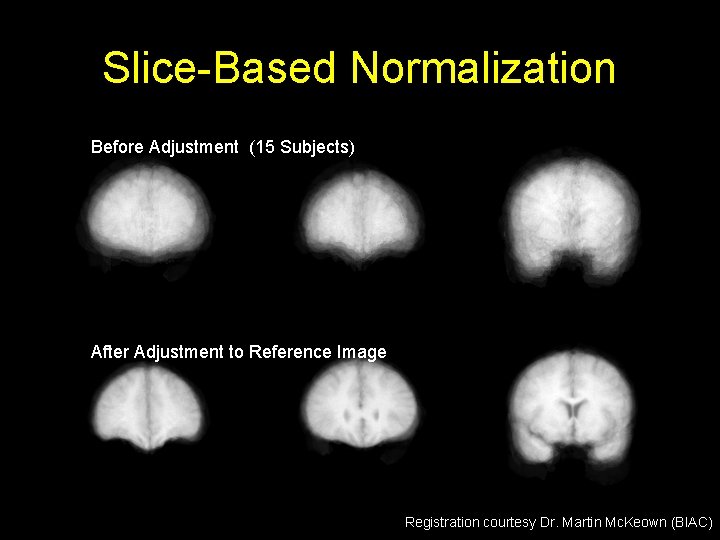
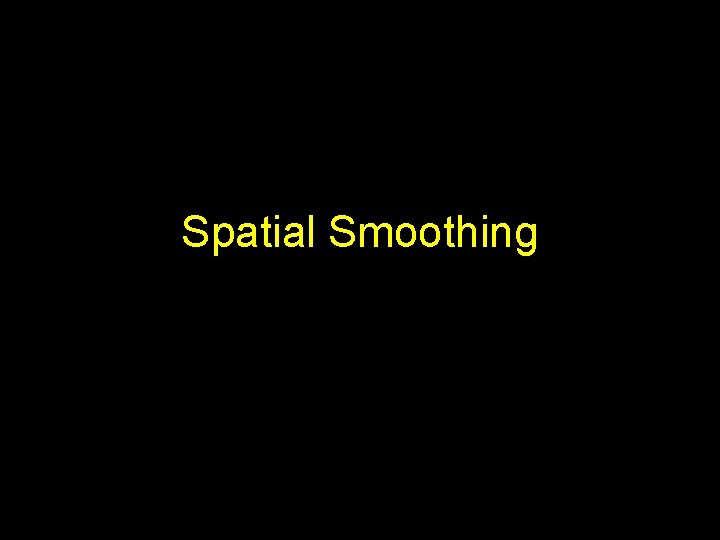
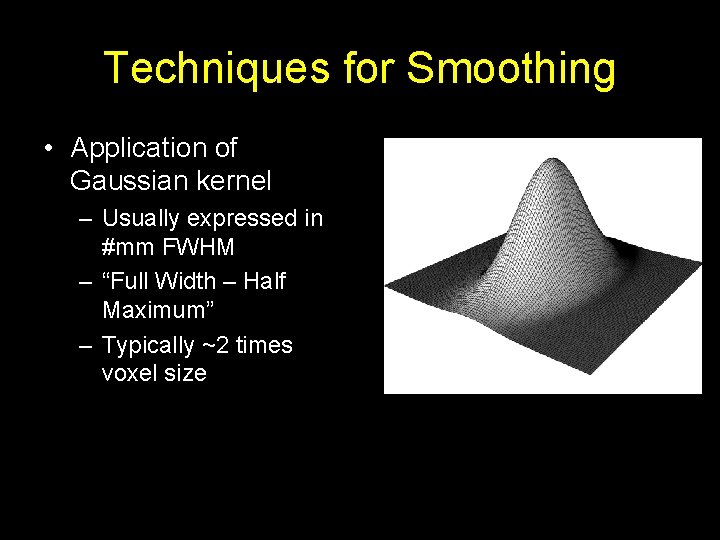
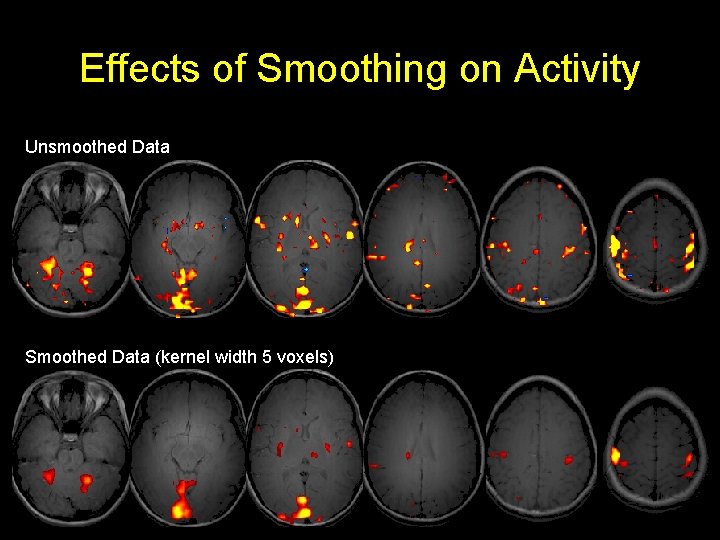
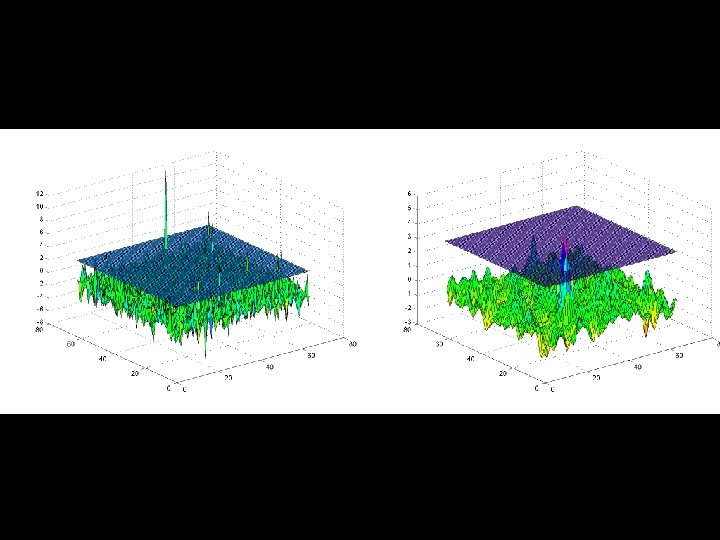
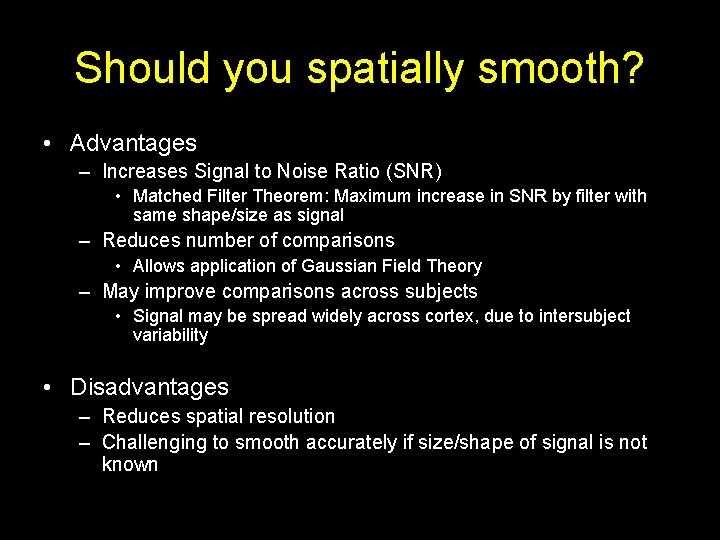
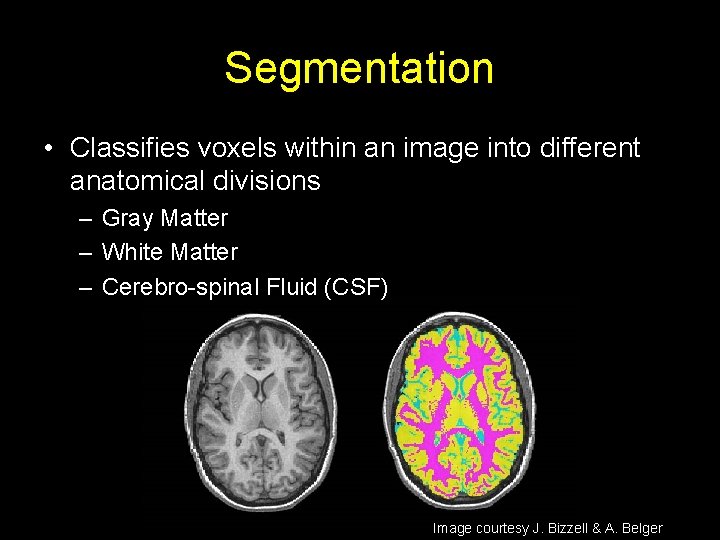
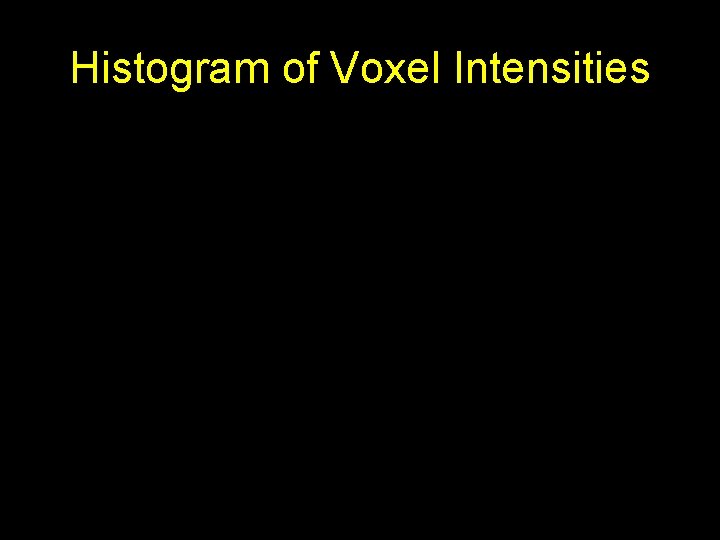
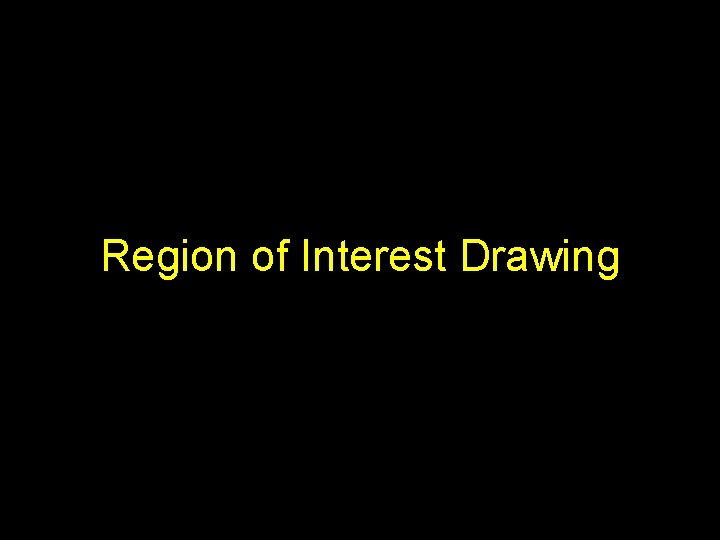
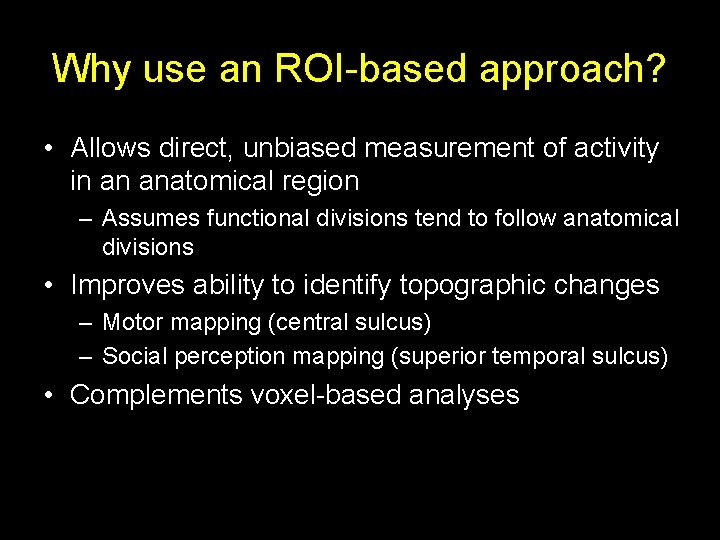
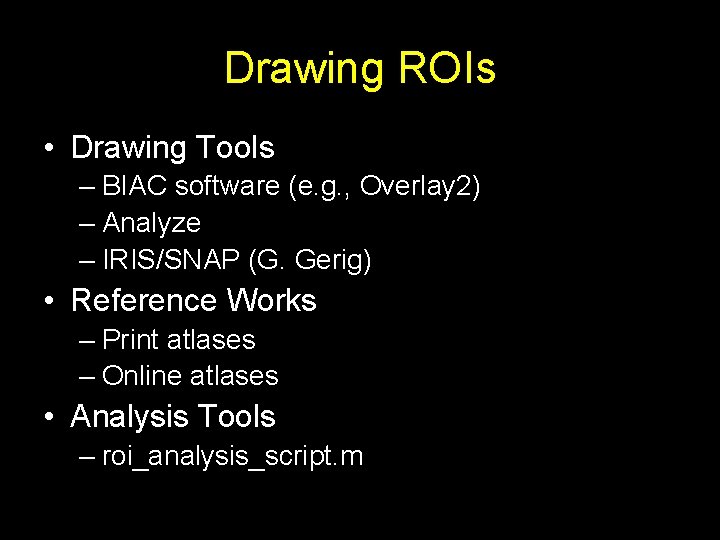
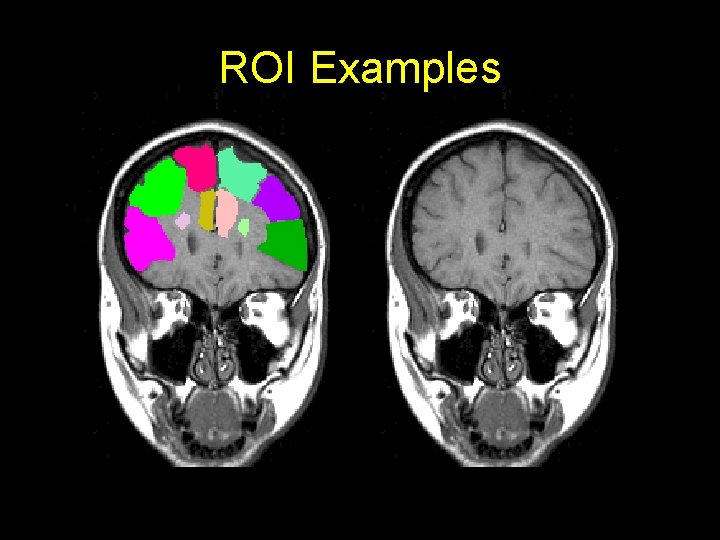
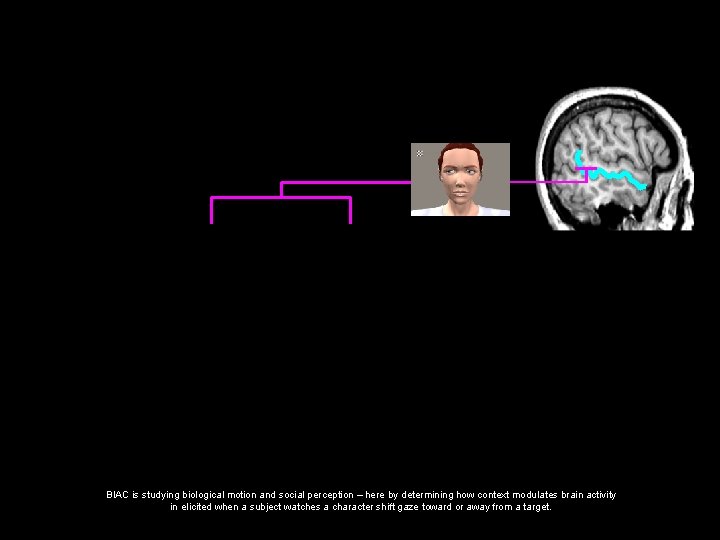
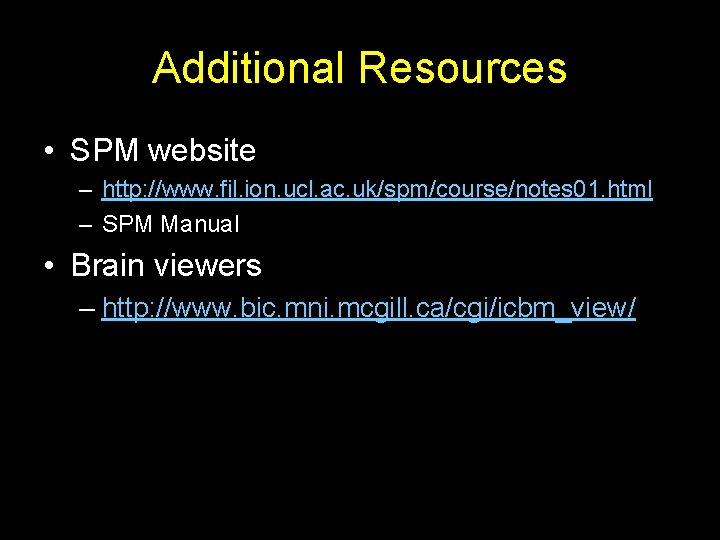
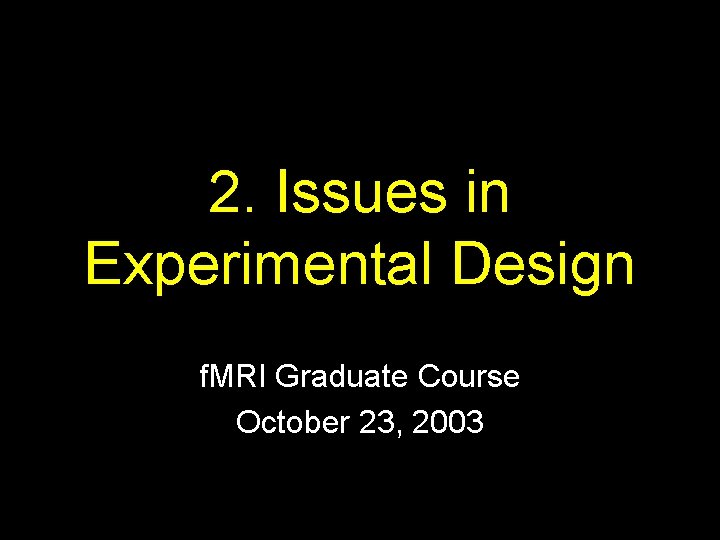
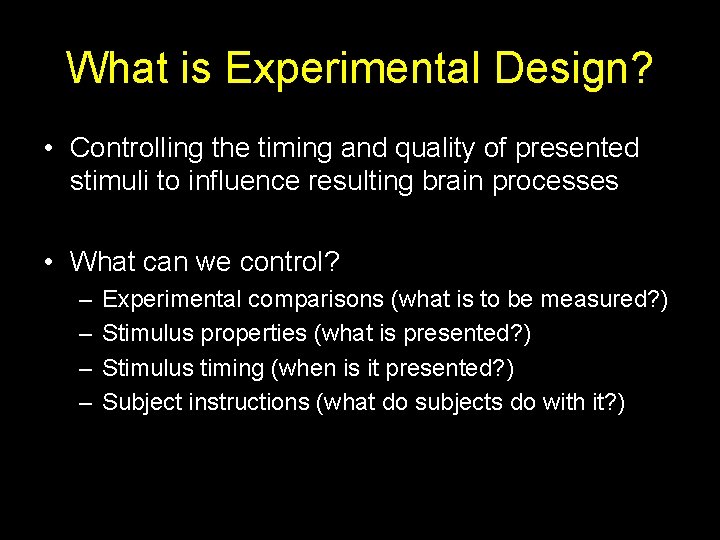
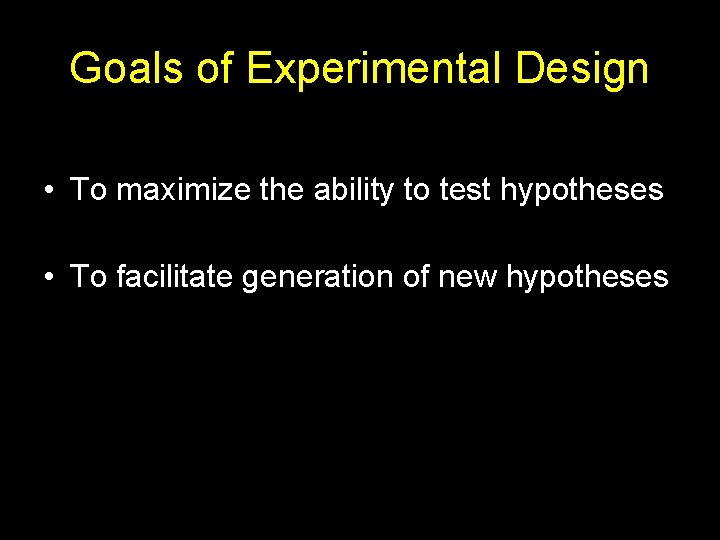
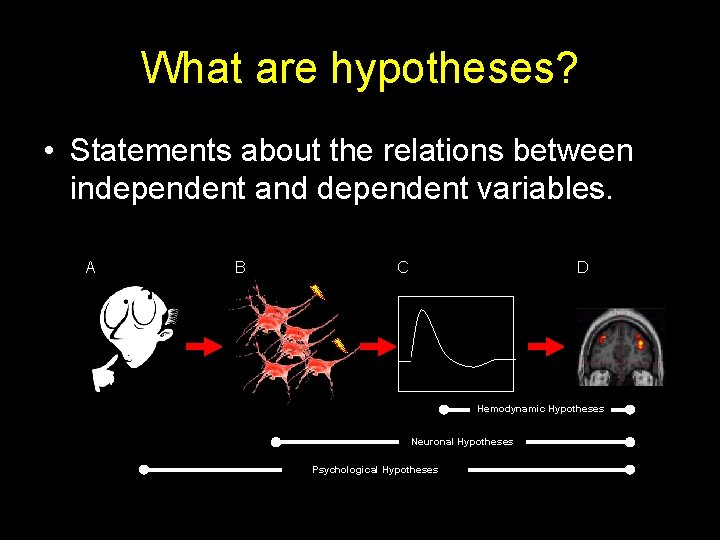
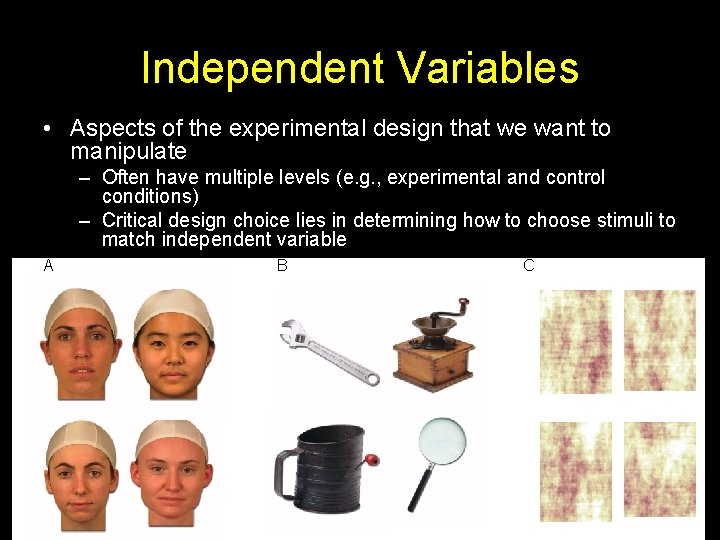
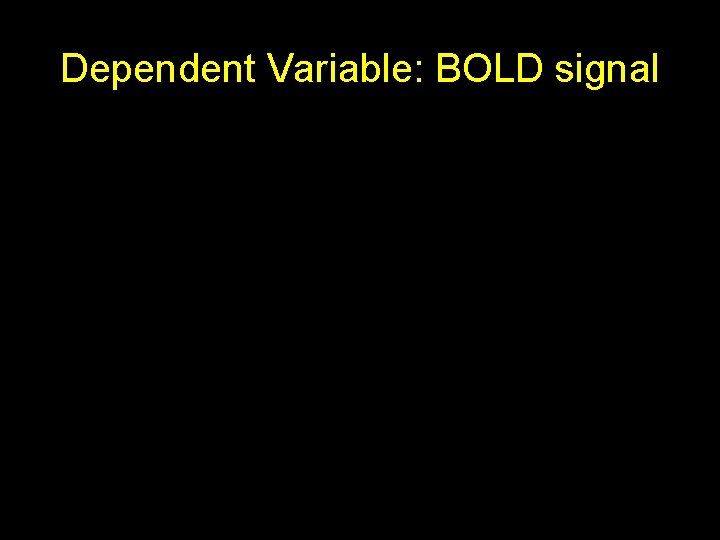
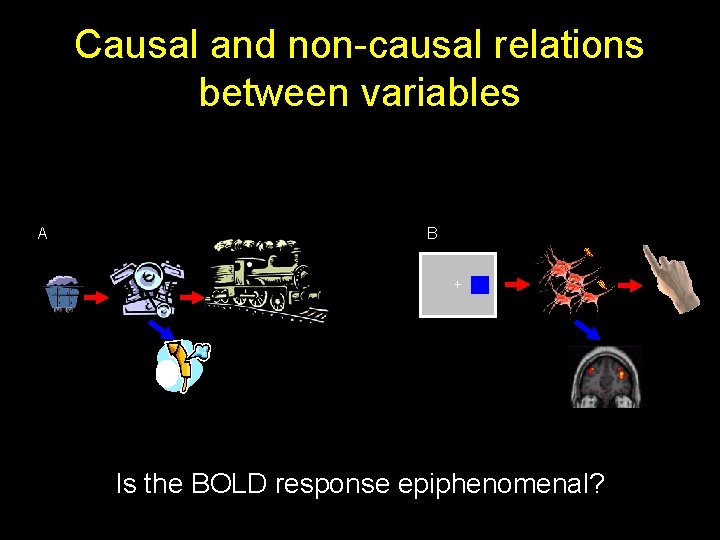
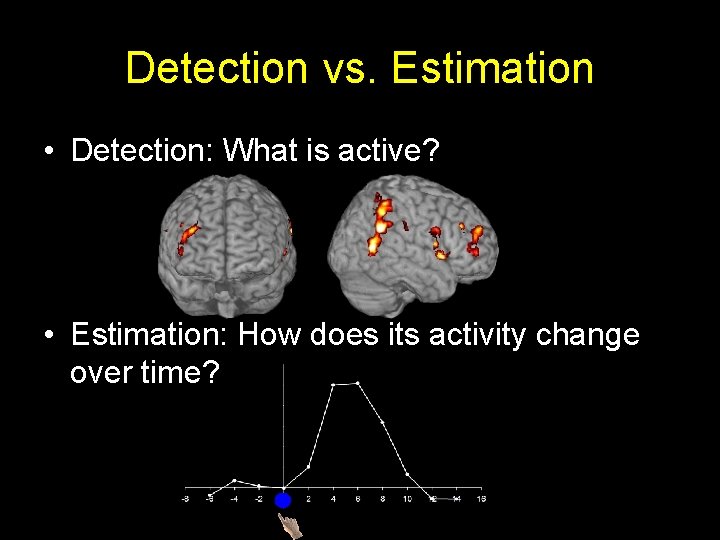
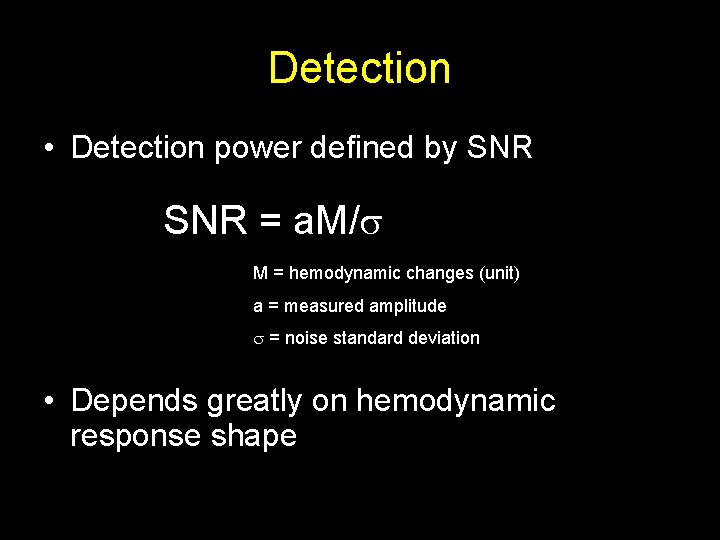
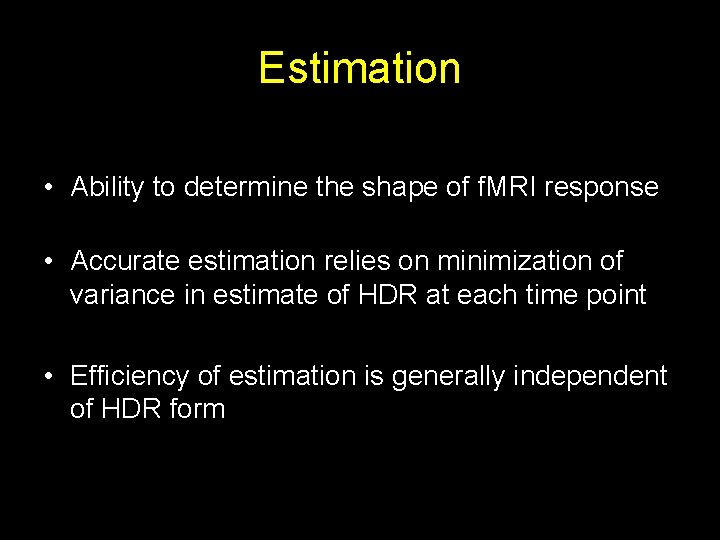
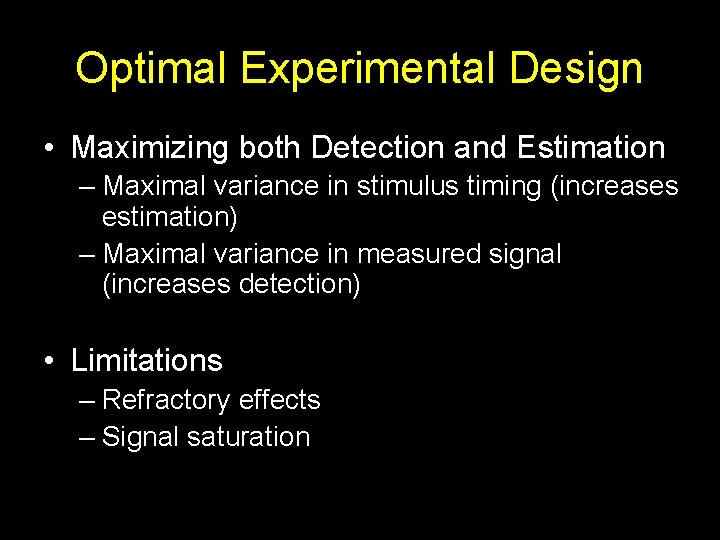
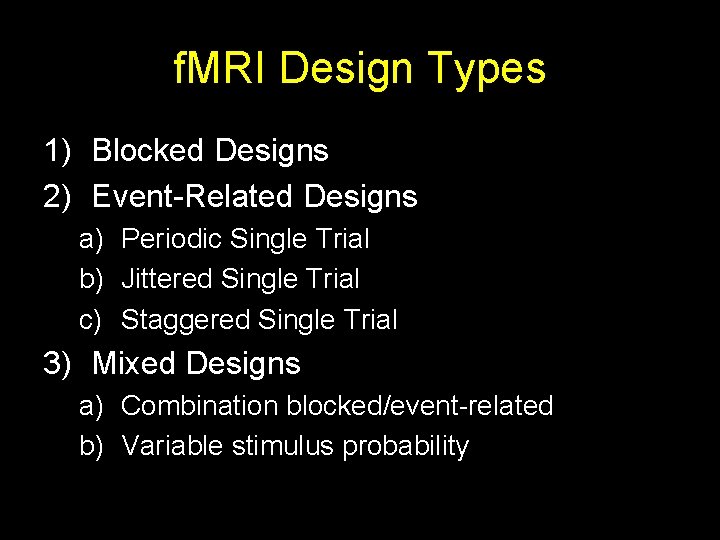
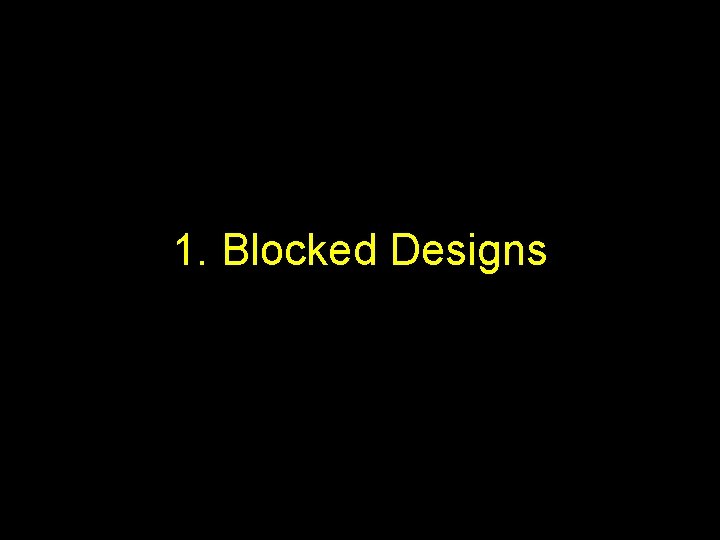
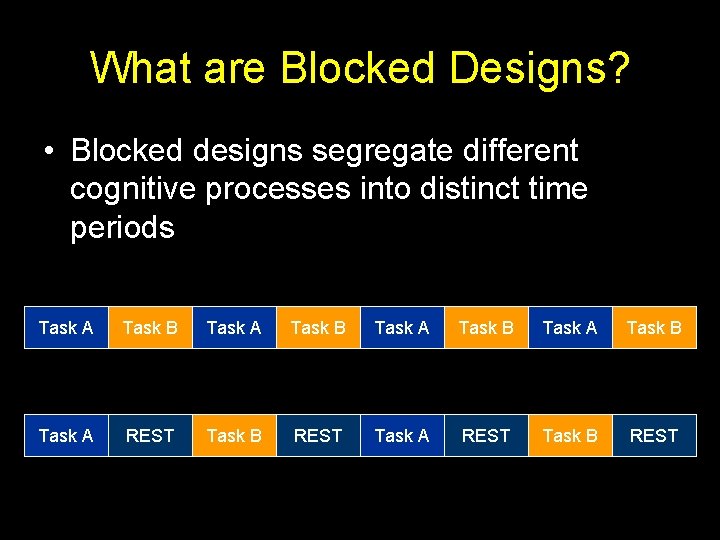
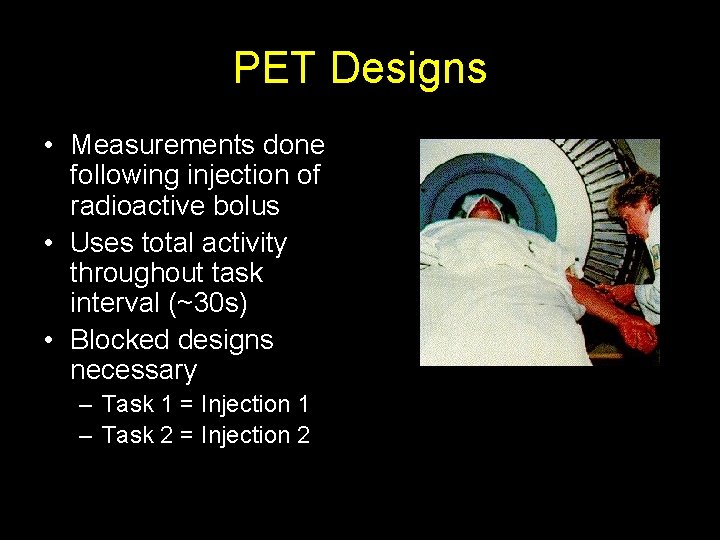
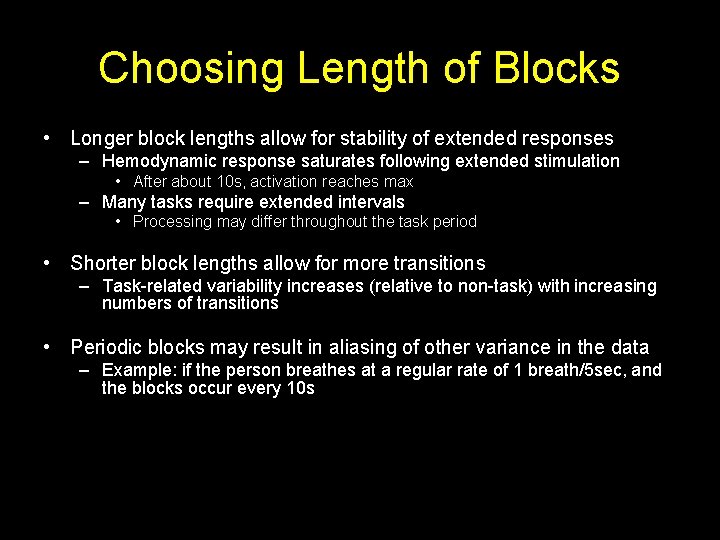
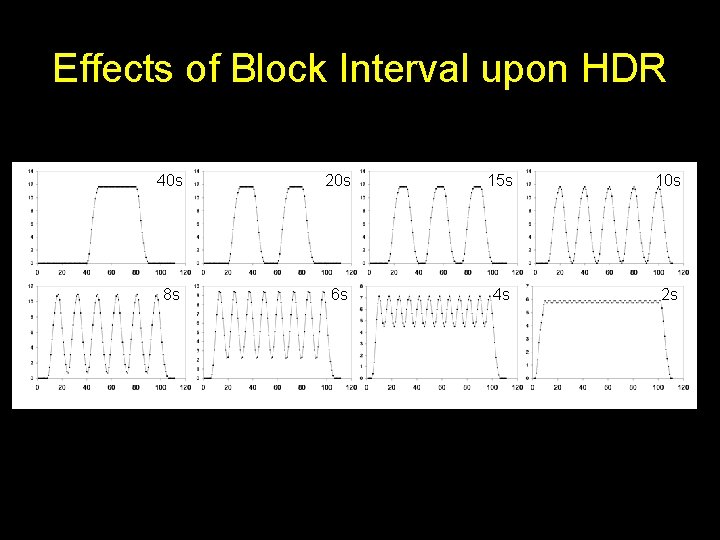
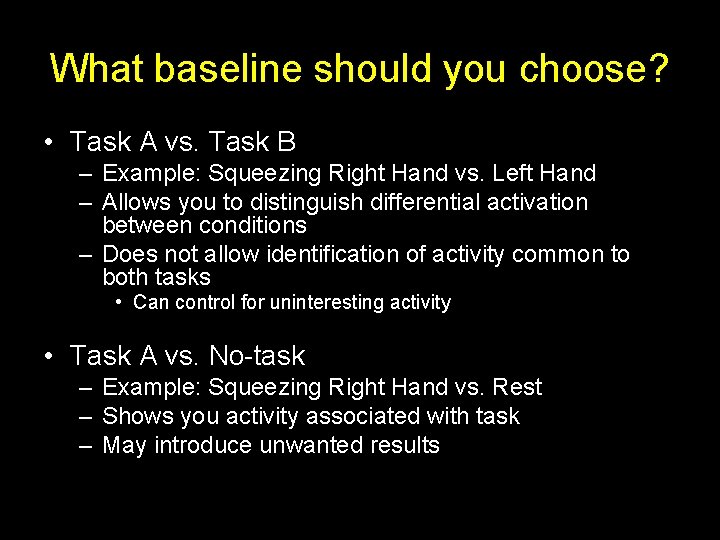
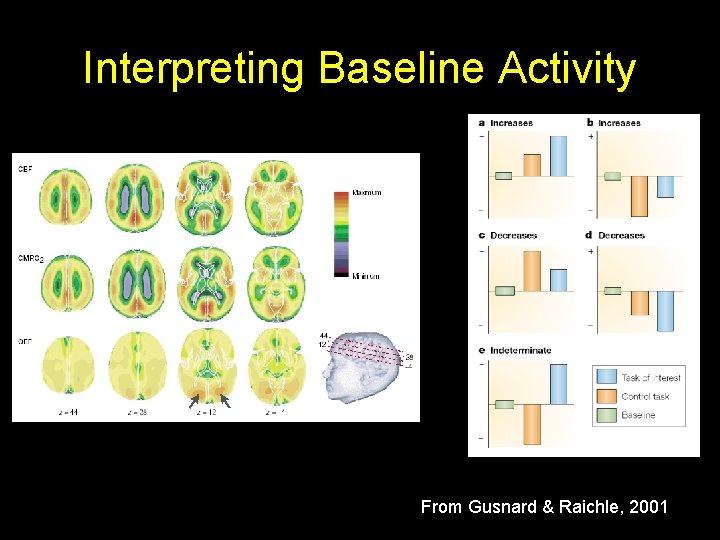
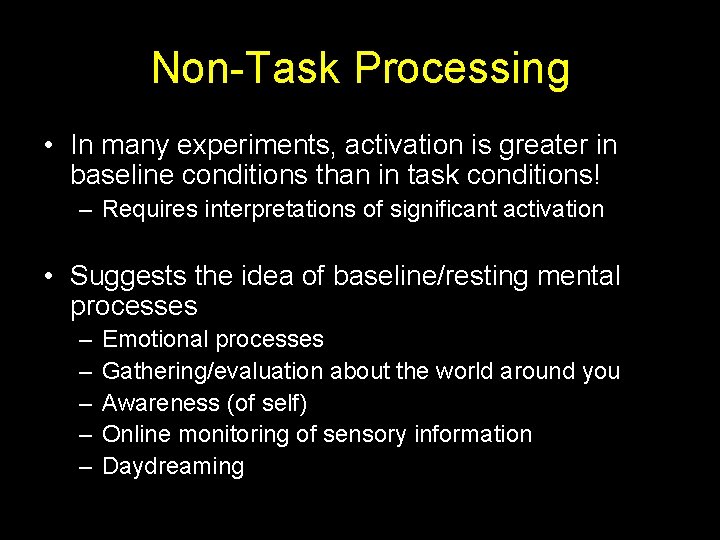
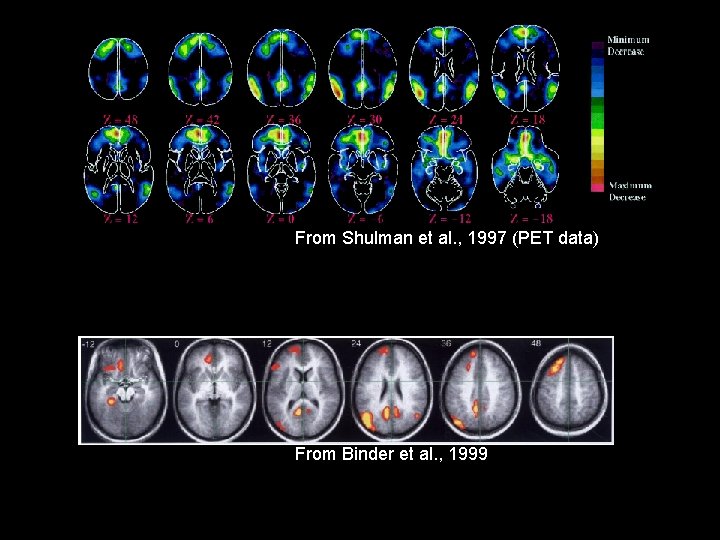
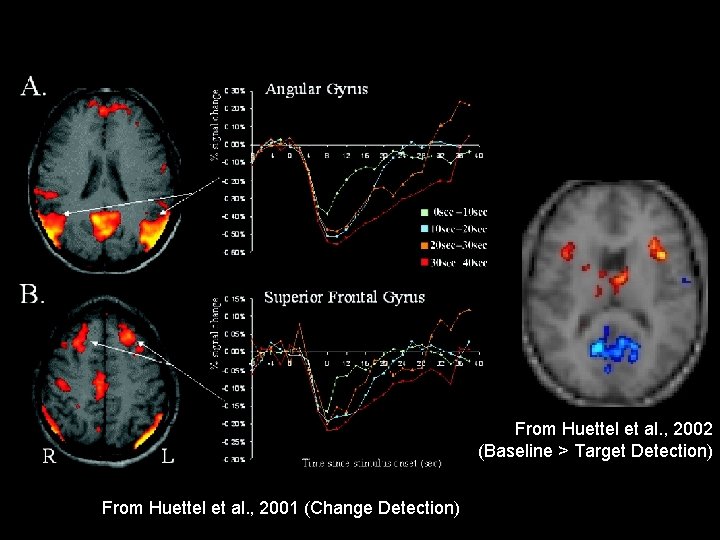
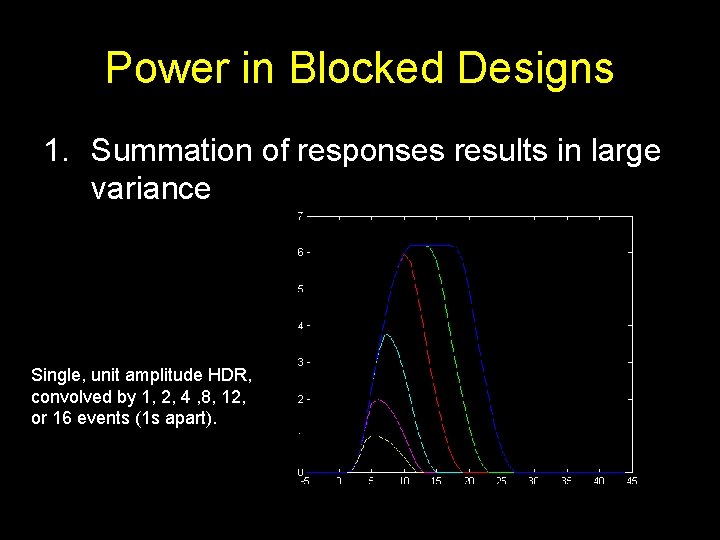
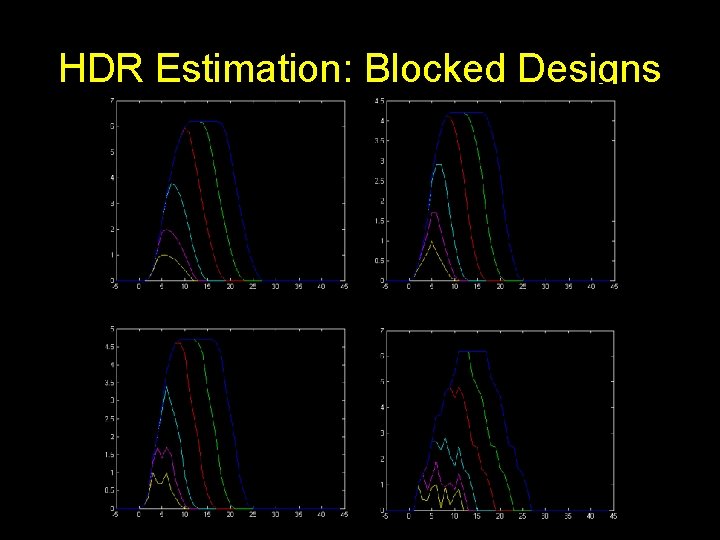
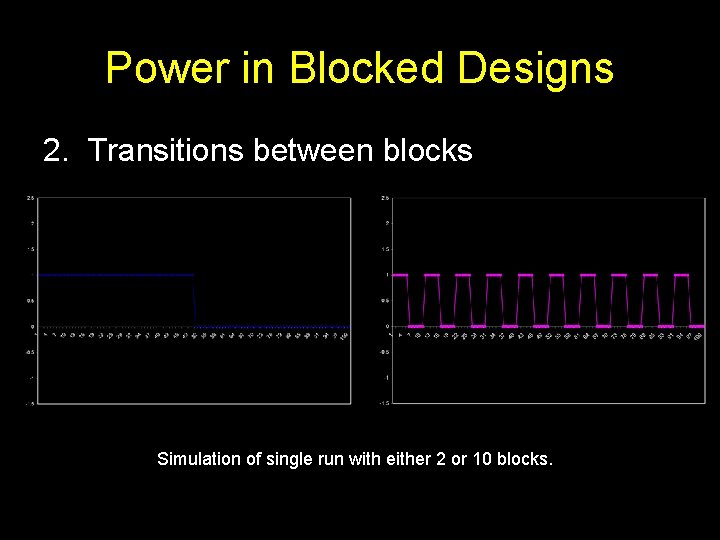
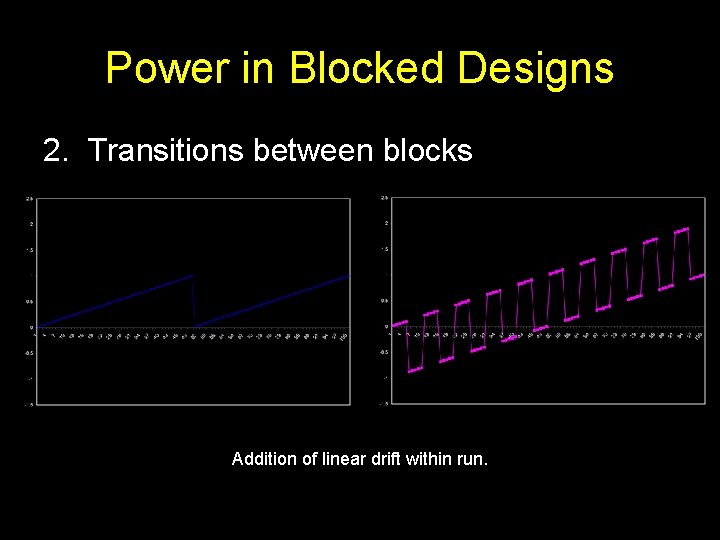
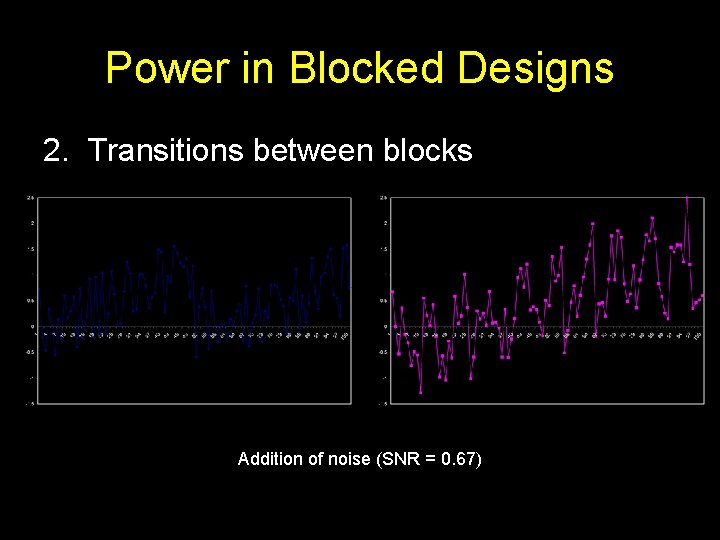
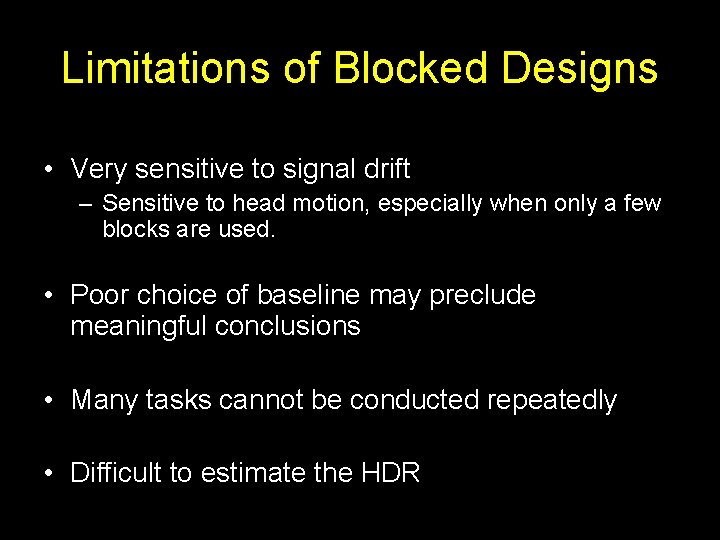
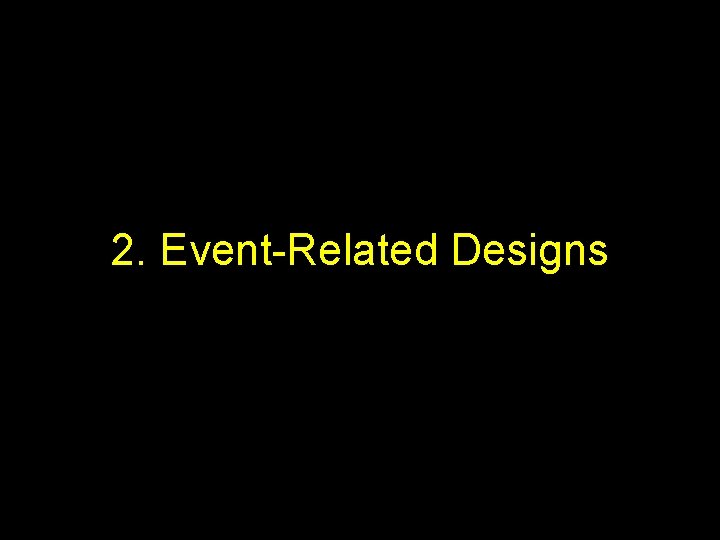
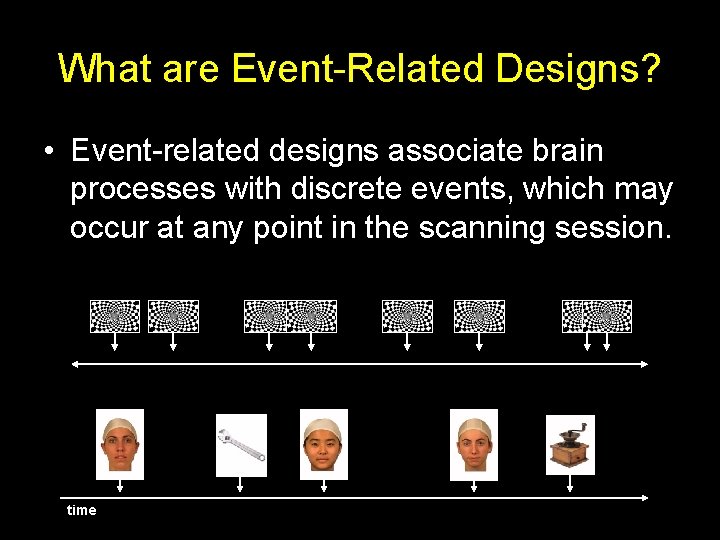
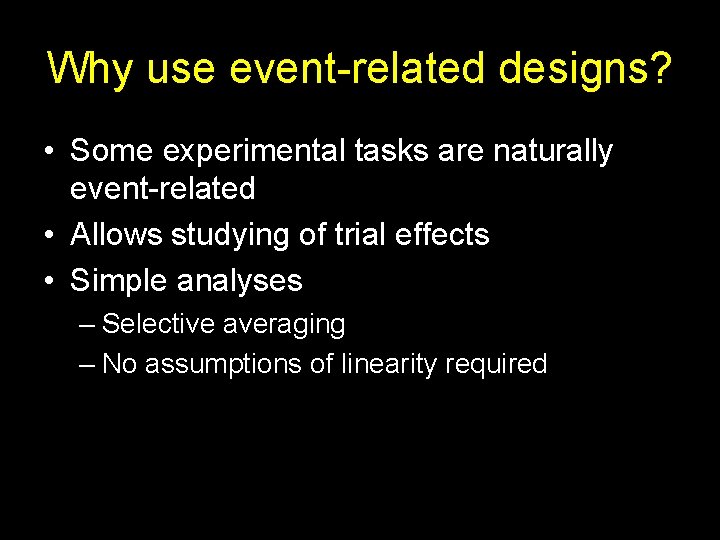
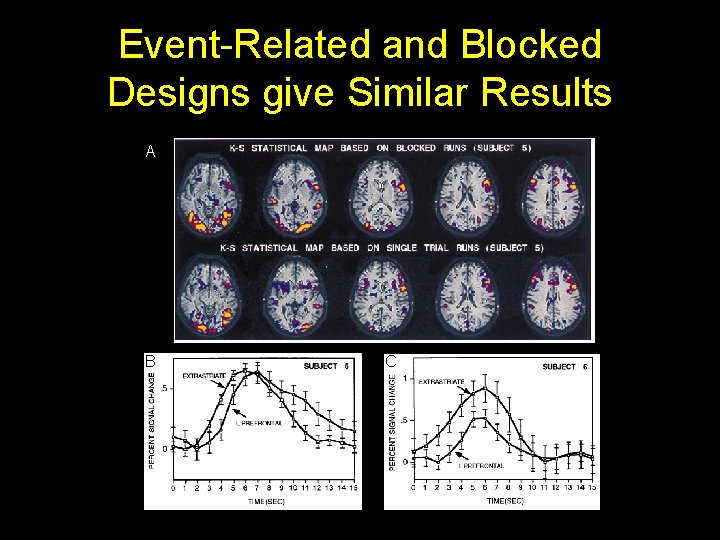
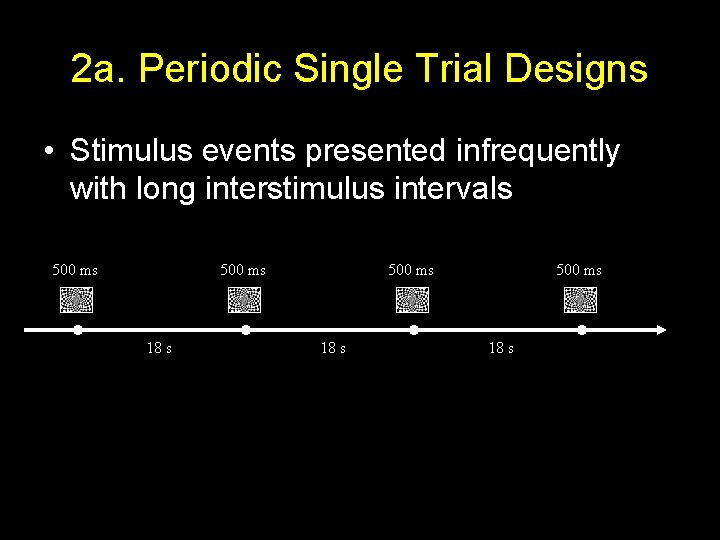
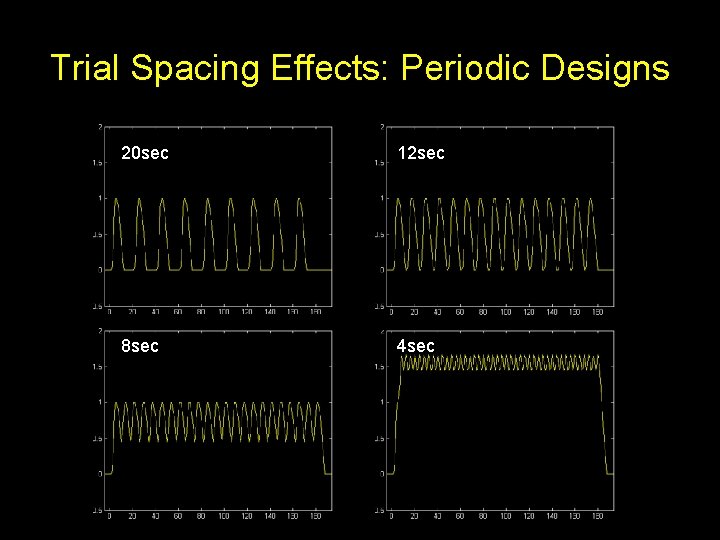
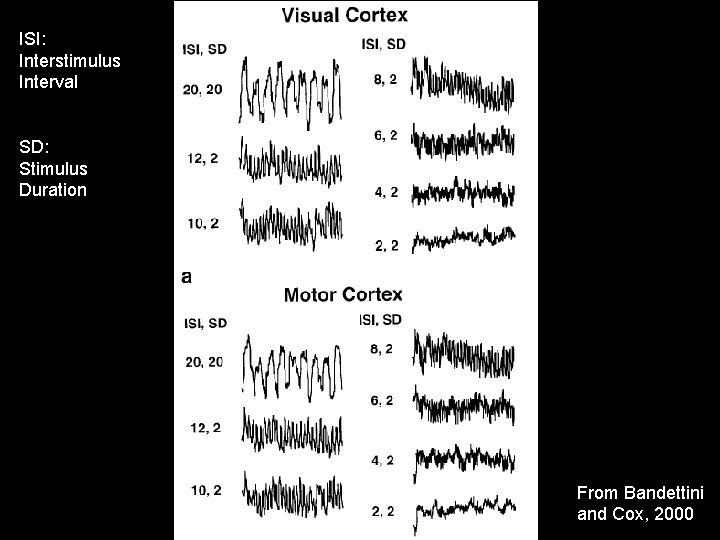
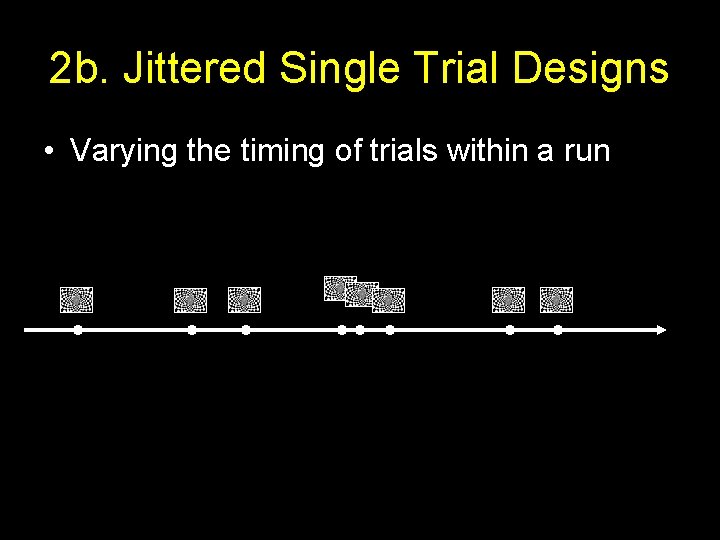
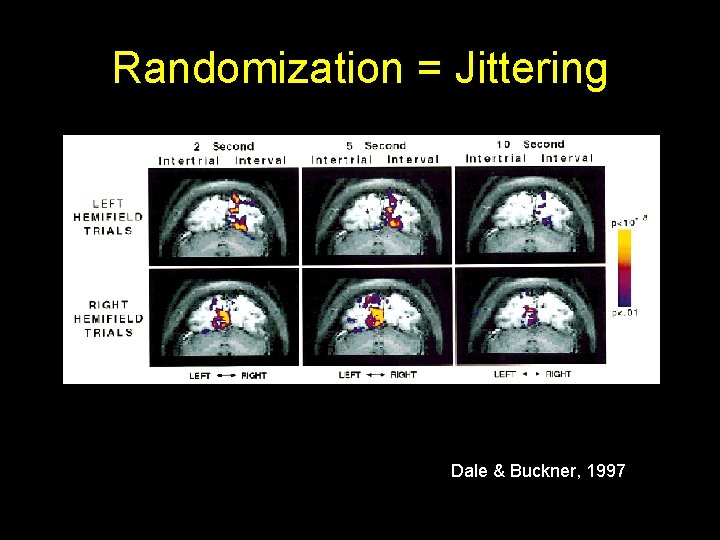
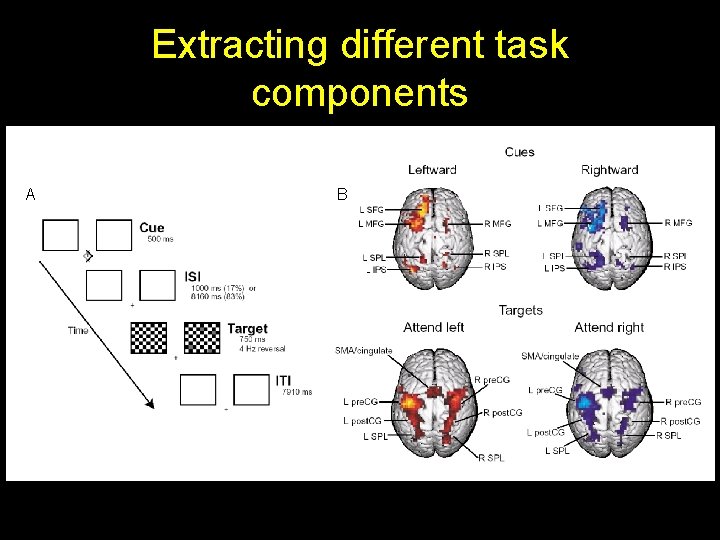
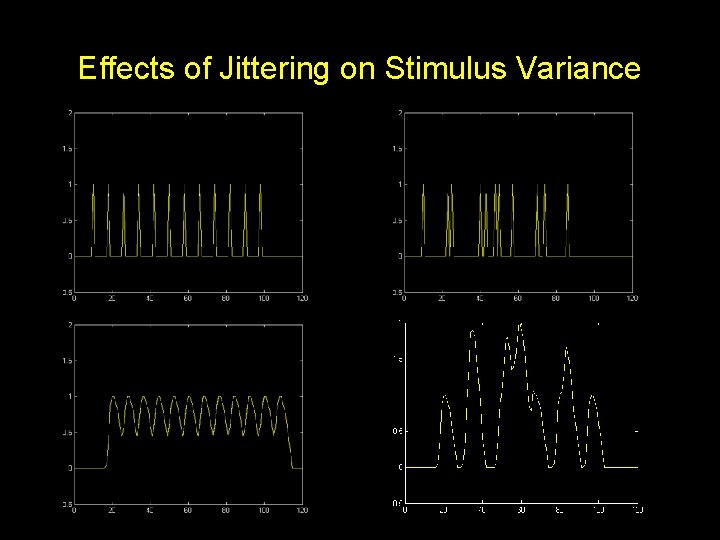
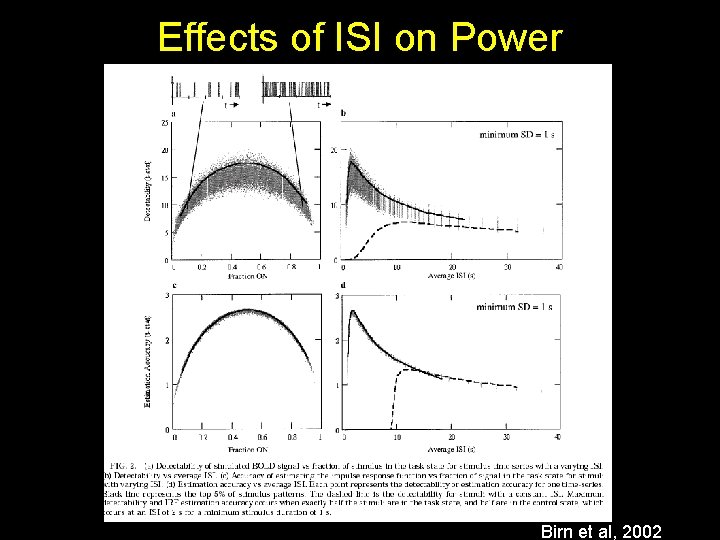
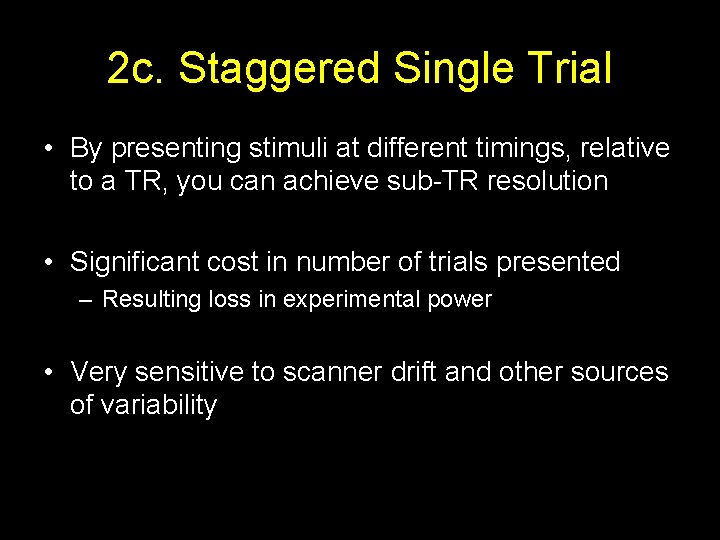
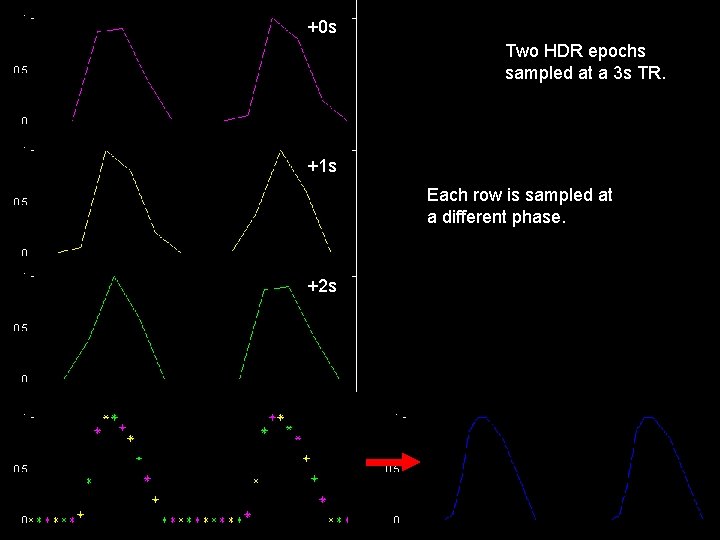
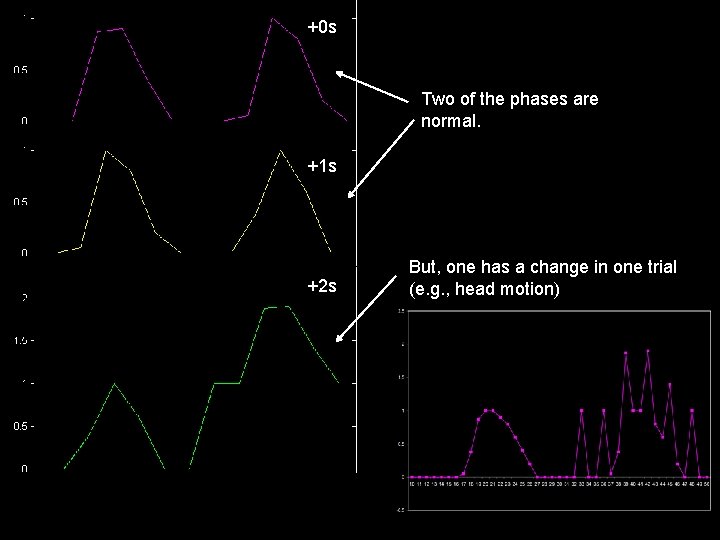
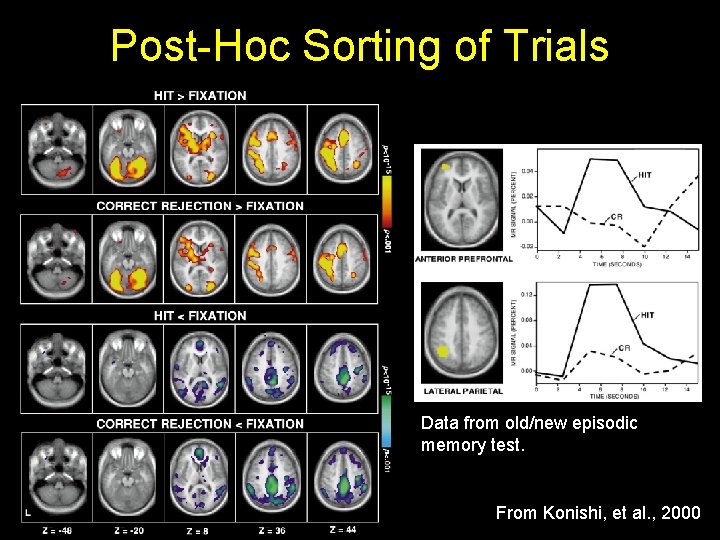
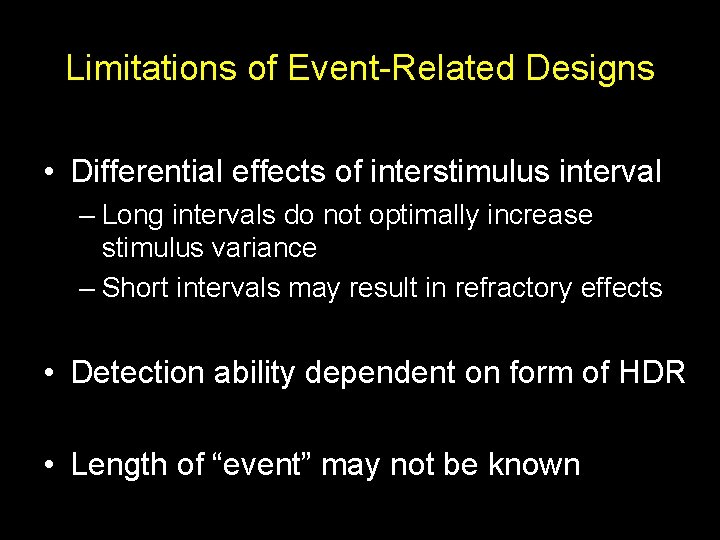
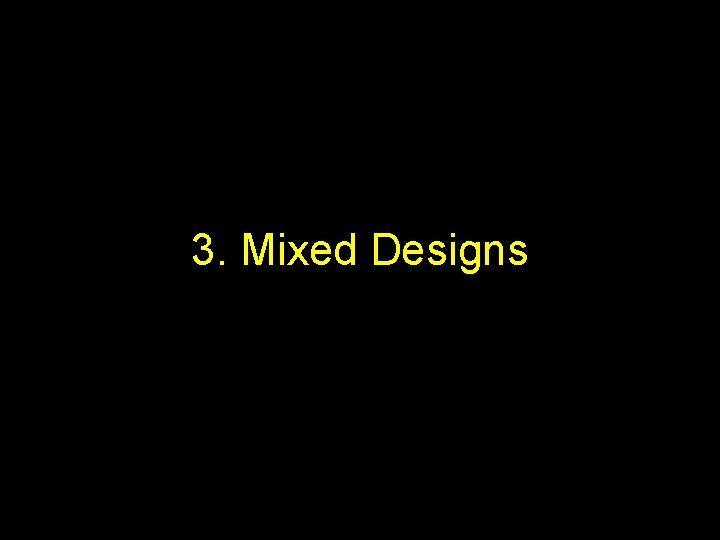
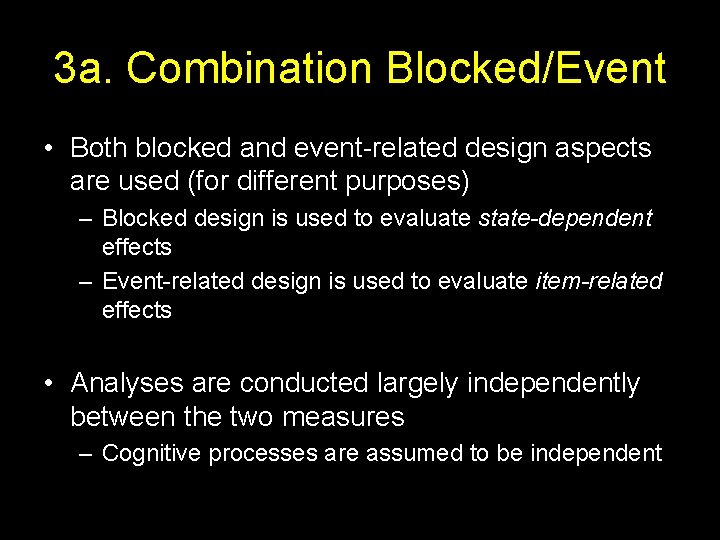
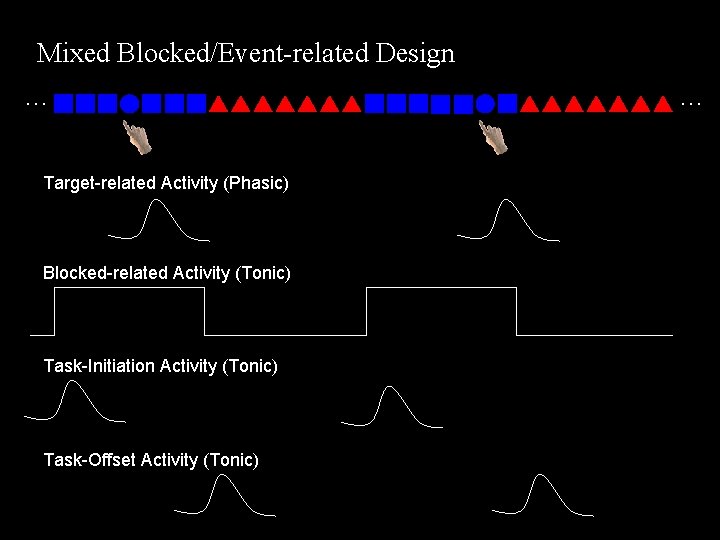
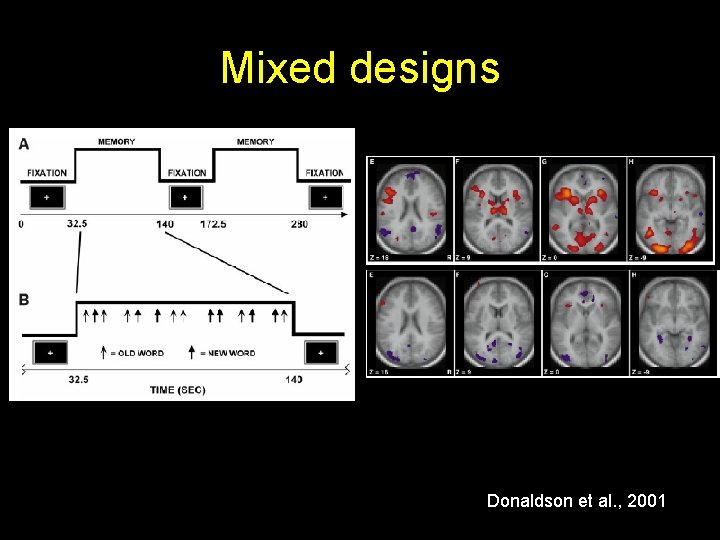
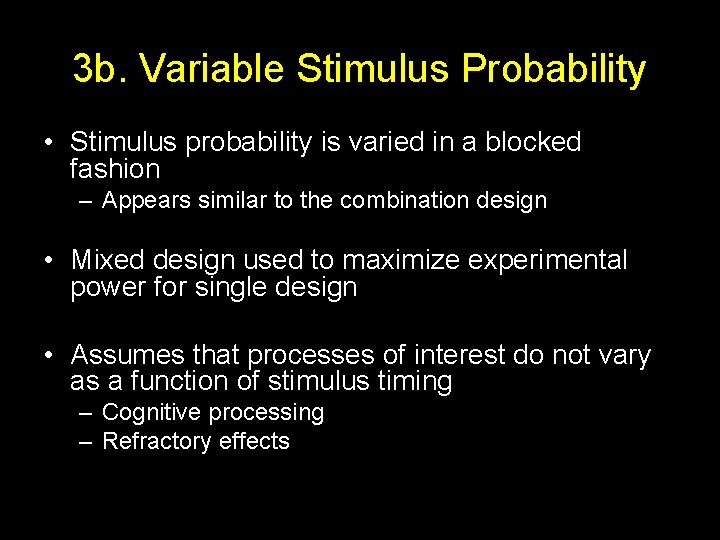
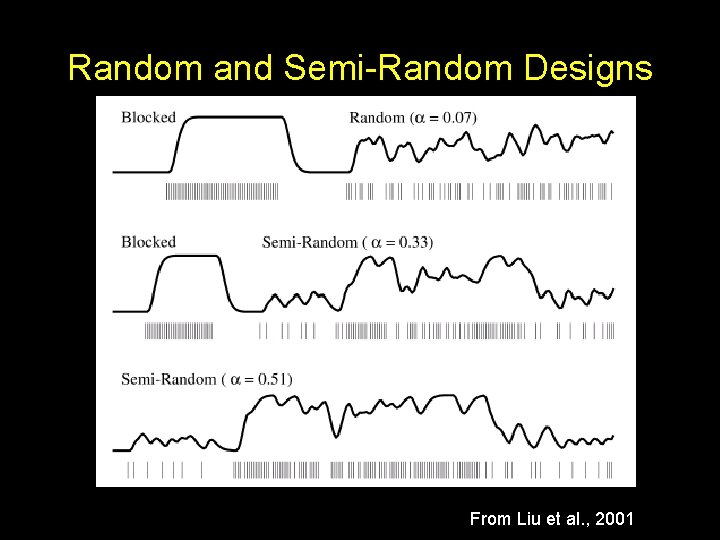
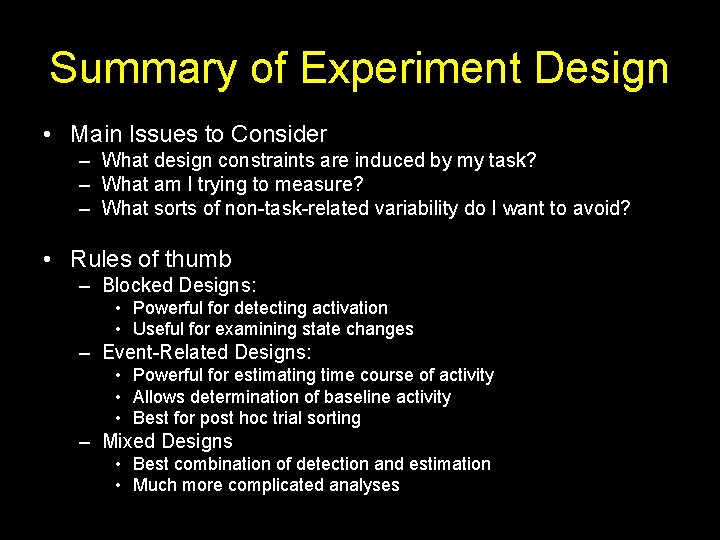
- Slides: 99
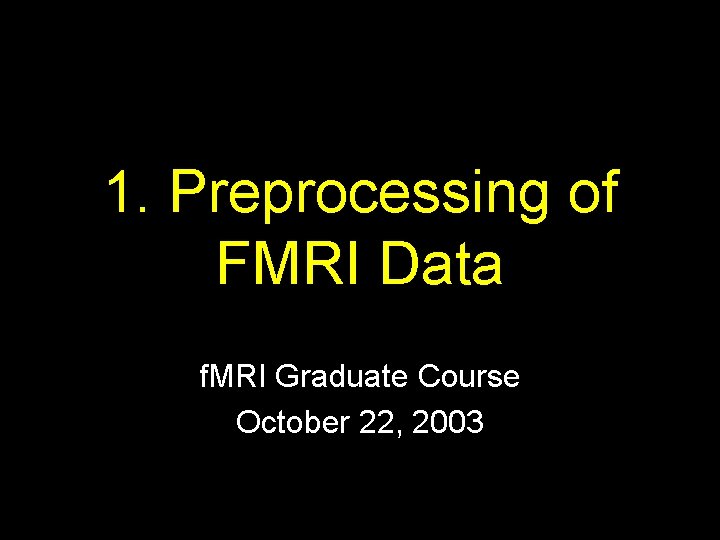
1. Preprocessing of FMRI Data f. MRI Graduate Course October 22, 2003
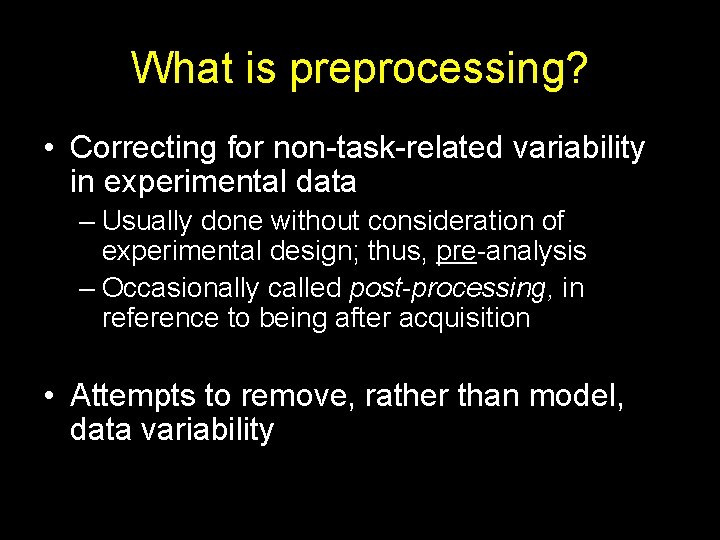
What is preprocessing? • Correcting for non-task-related variability in experimental data – Usually done without consideration of experimental design; thus, pre-analysis – Occasionally called post-processing, in reference to being after acquisition • Attempts to remove, rather than model, data variability
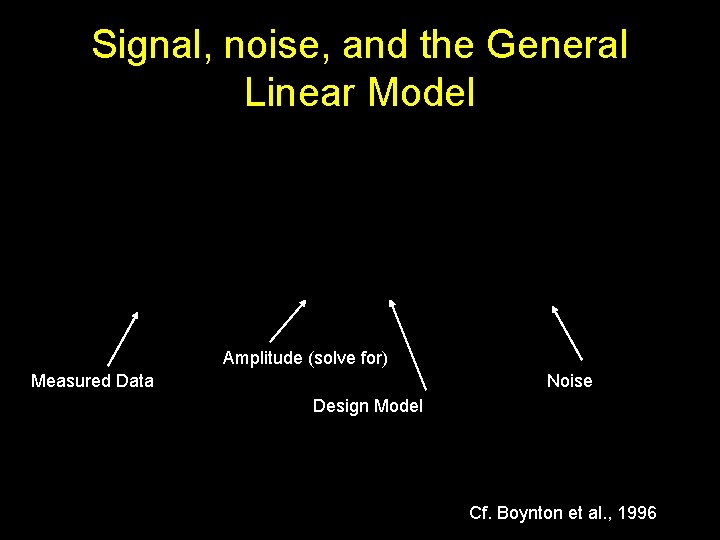
Signal, noise, and the General Linear Model Amplitude (solve for) Measured Data Noise Design Model Cf. Boynton et al. , 1996
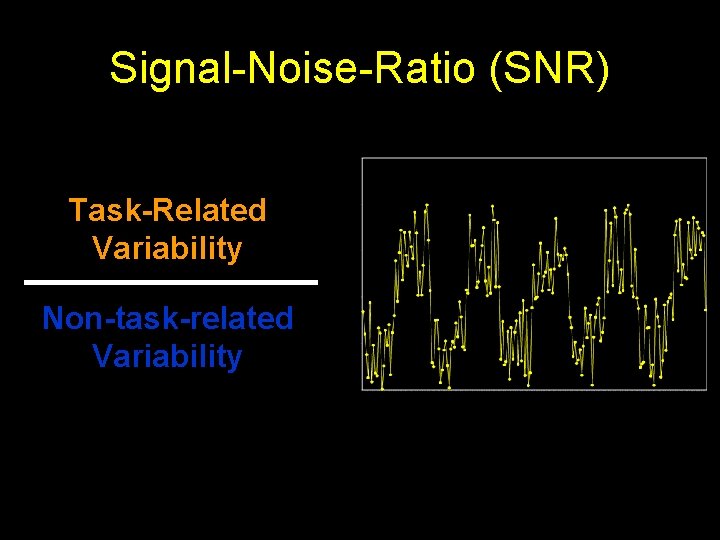
Signal-Noise-Ratio (SNR) Task-Related Variability Non-task-related Variability
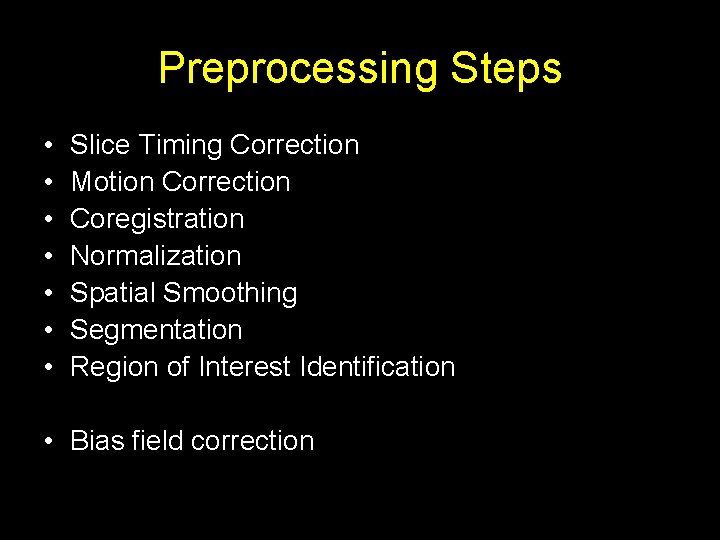
Preprocessing Steps • • Slice Timing Correction Motion Correction Coregistration Normalization Spatial Smoothing Segmentation Region of Interest Identification • Bias field correction
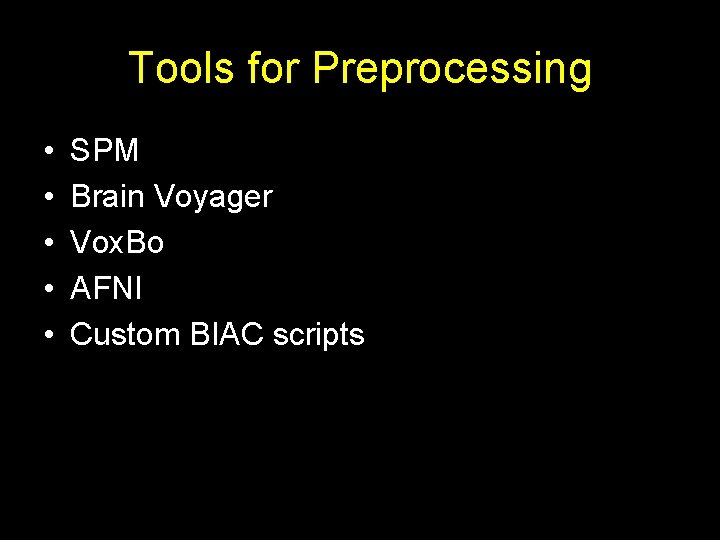
Tools for Preprocessing • • • SPM Brain Voyager Vox. Bo AFNI Custom BIAC scripts
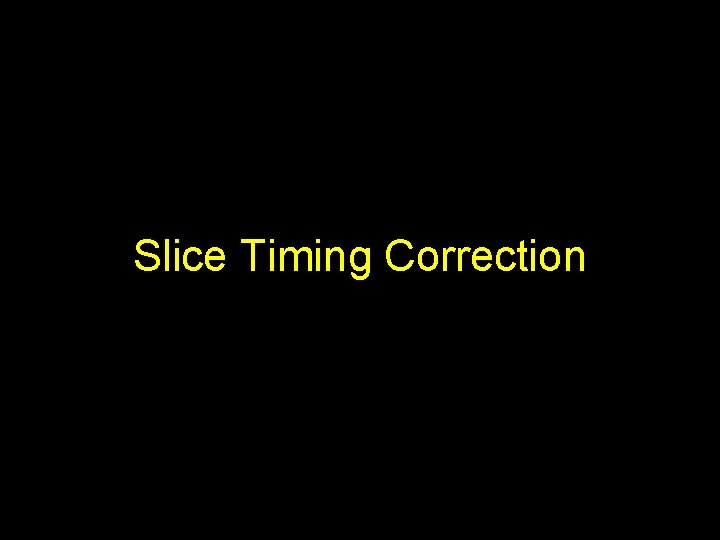
Slice Timing Correction
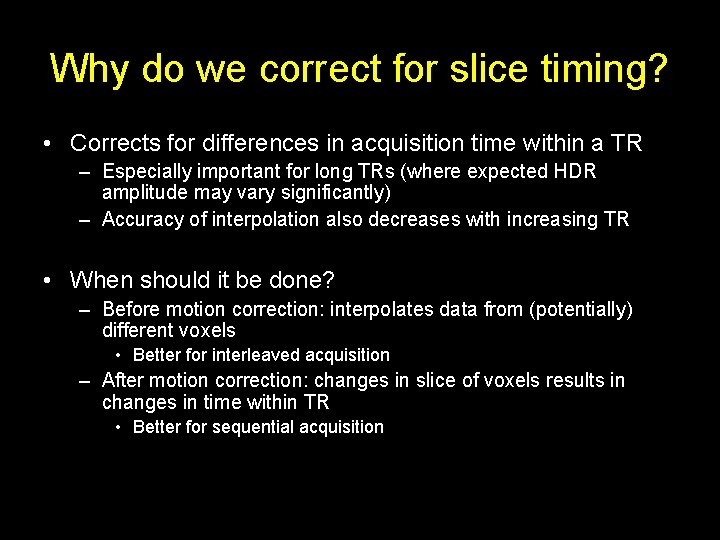
Why do we correct for slice timing? • Corrects for differences in acquisition time within a TR – Especially important for long TRs (where expected HDR amplitude may vary significantly) – Accuracy of interpolation also decreases with increasing TR • When should it be done? – Before motion correction: interpolates data from (potentially) different voxels • Better for interleaved acquisition – After motion correction: changes in slice of voxels results in changes in time within TR • Better for sequential acquisition
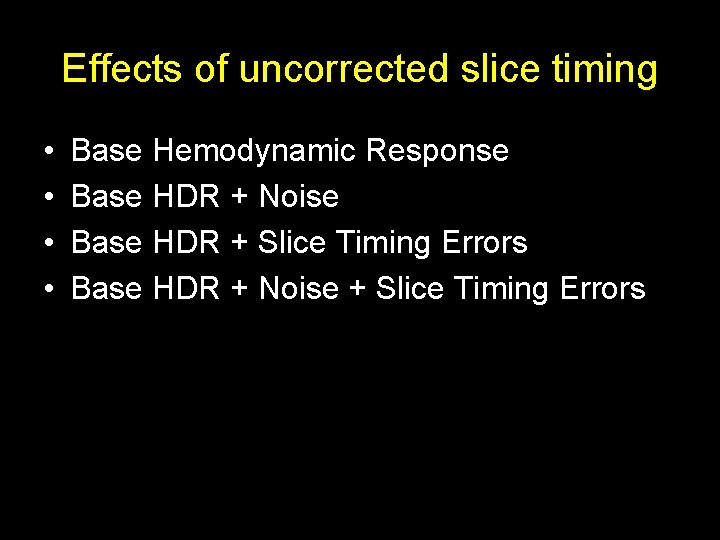
Effects of uncorrected slice timing • • Base Hemodynamic Response Base HDR + Noise Base HDR + Slice Timing Errors Base HDR + Noise + Slice Timing Errors
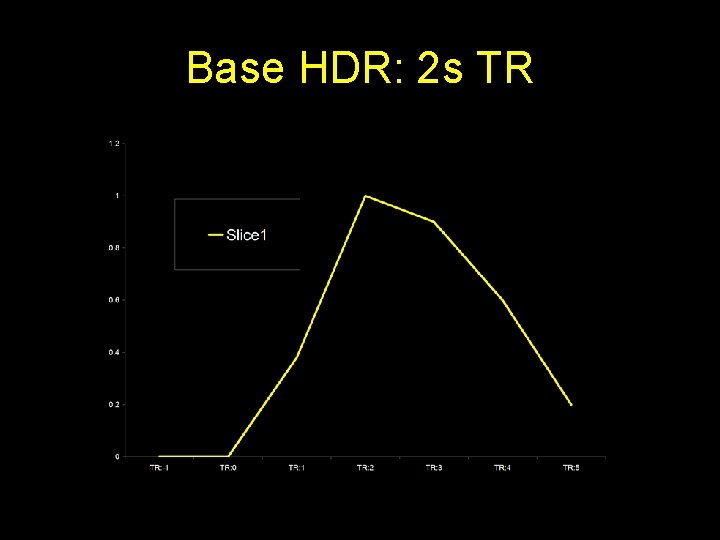
Base HDR: 2 s TR
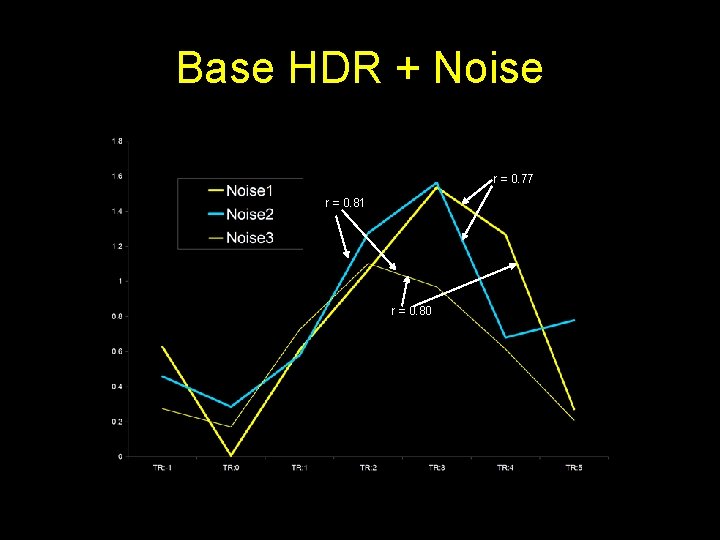
Base HDR + Noise r = 0. 77 r = 0. 81 r = 0. 80
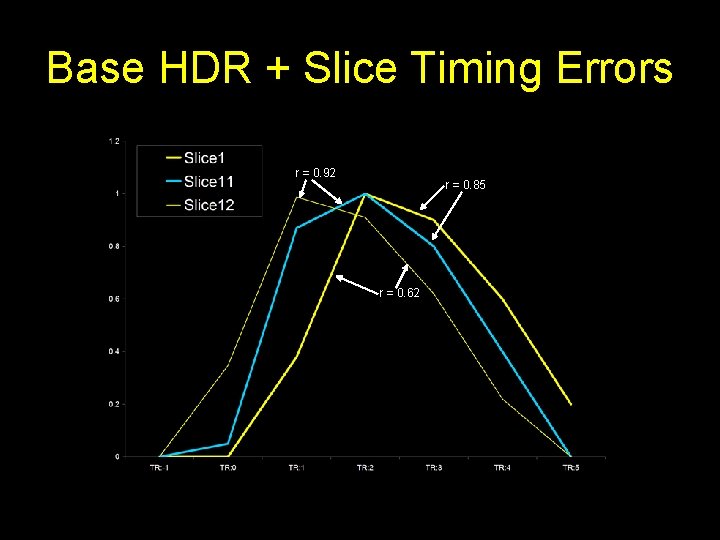
Base HDR + Slice Timing Errors r = 0. 92 r = 0. 85 r = 0. 62
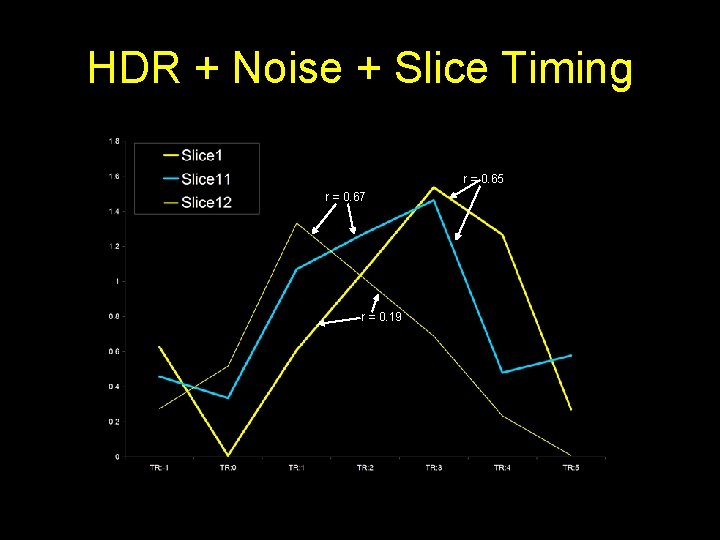
HDR + Noise + Slice Timing r = 0. 65 r = 0. 67 r = 0. 19
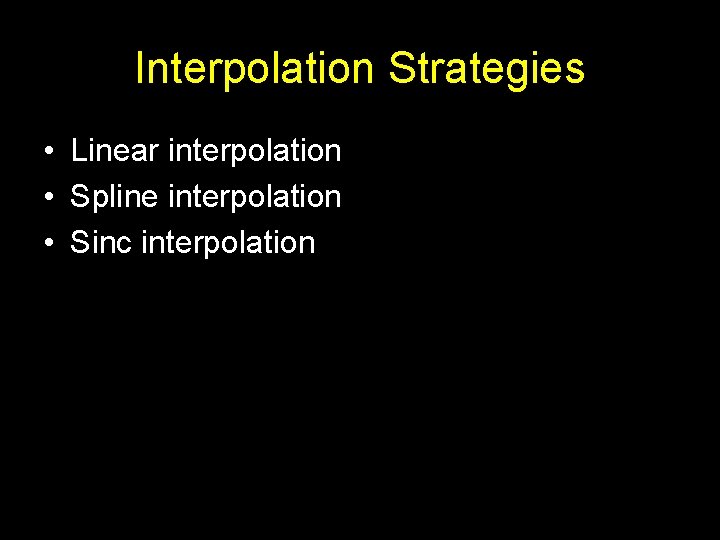
Interpolation Strategies • Linear interpolation • Spline interpolation • Sinc interpolation
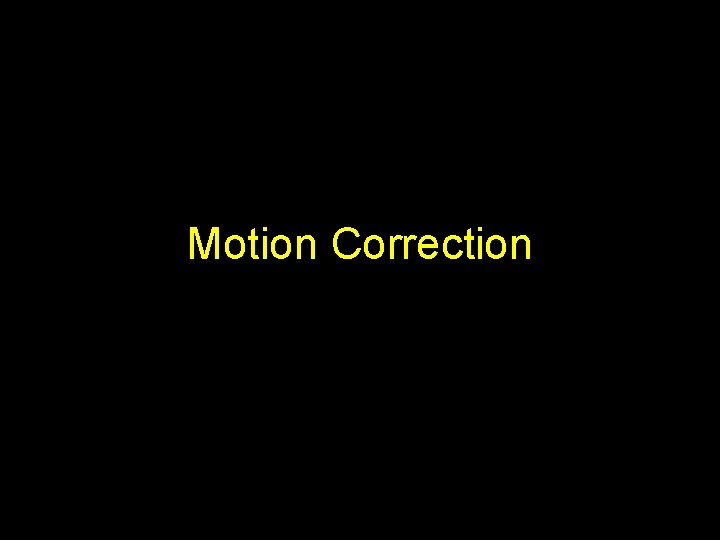
Motion Correction
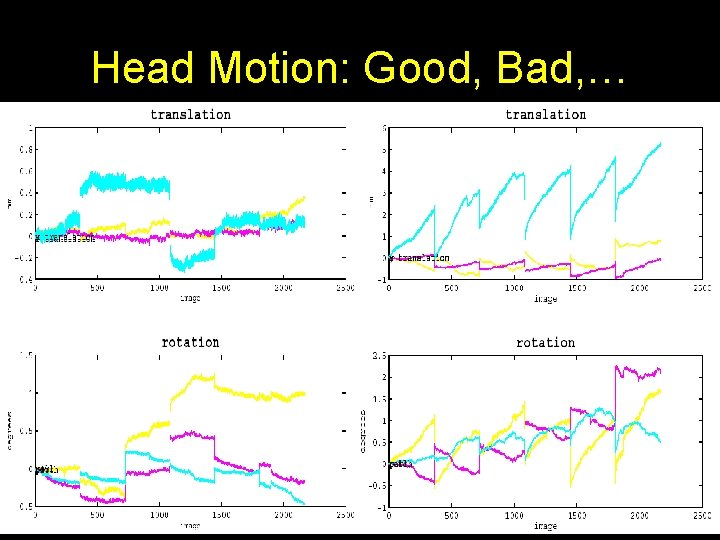
Head Motion: Good, Bad, …
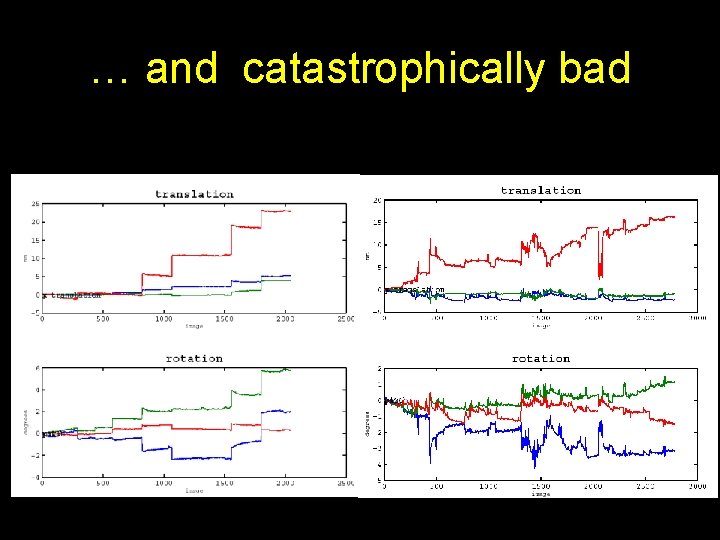
… and catastrophically bad
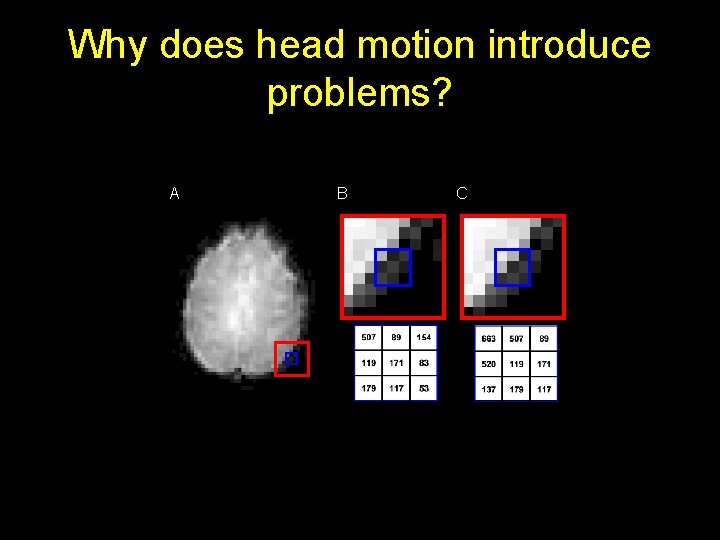
Why does head motion introduce problems? A B C
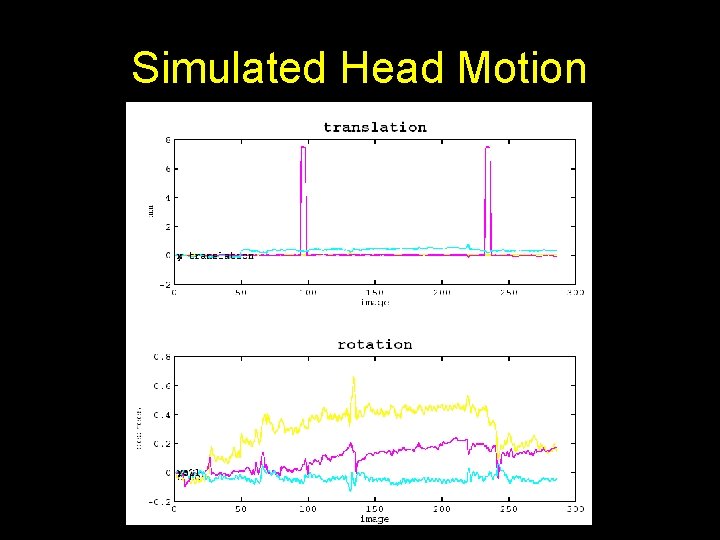
Simulated Head Motion
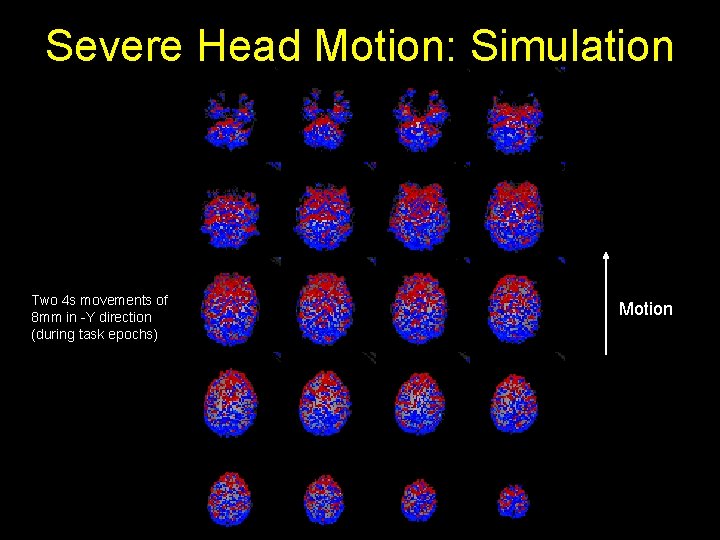
Severe Head Motion: Simulation Two 4 s movements of 8 mm in -Y direction (during task epochs) Motion
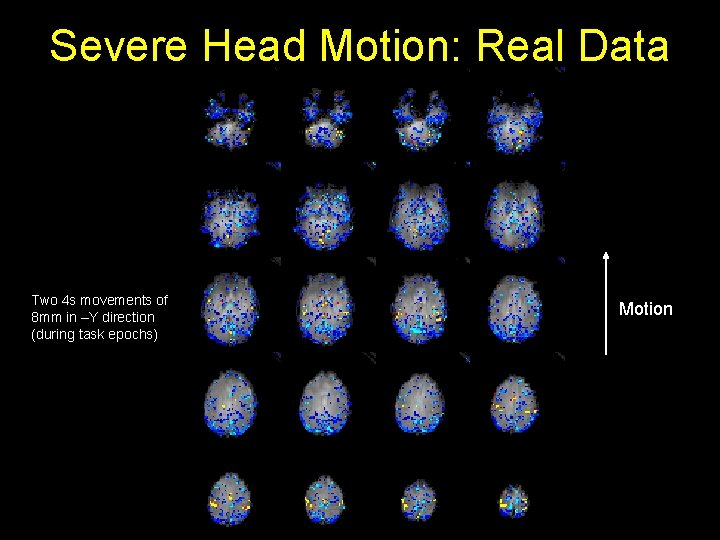
Severe Head Motion: Real Data Two 4 s movements of 8 mm in –Y direction (during task epochs) Motion
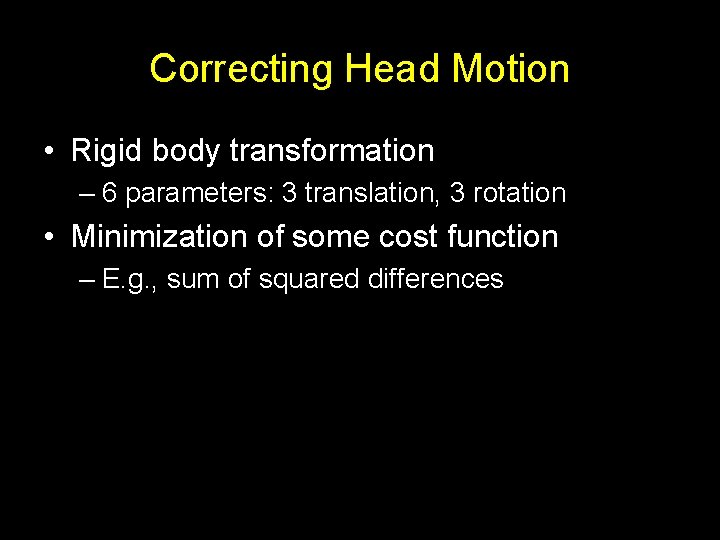
Correcting Head Motion • Rigid body transformation – 6 parameters: 3 translation, 3 rotation • Minimization of some cost function – E. g. , sum of squared differences
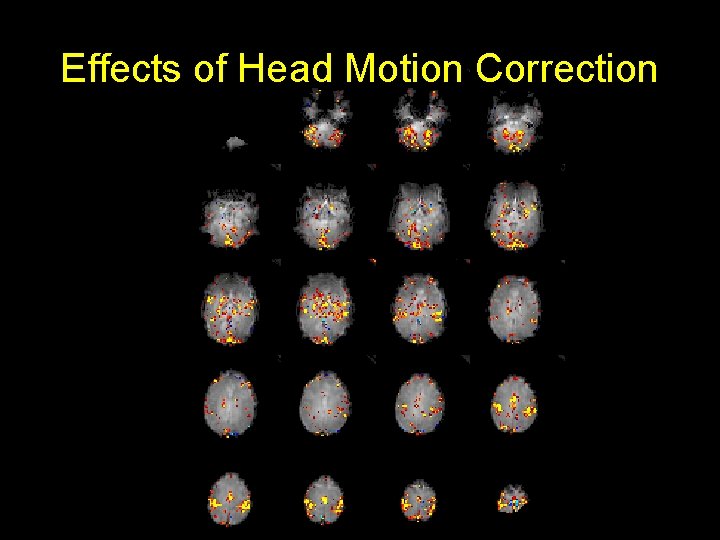
Effects of Head Motion Correction
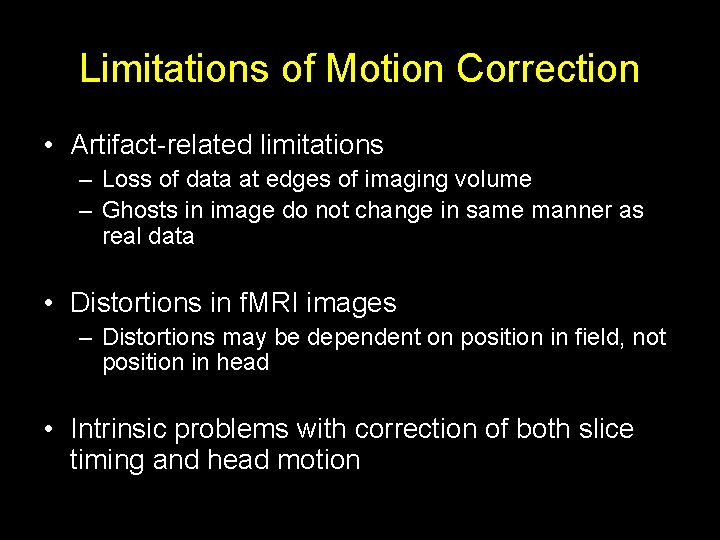
Limitations of Motion Correction • Artifact-related limitations – Loss of data at edges of imaging volume – Ghosts in image do not change in same manner as real data • Distortions in f. MRI images – Distortions may be dependent on position in field, not position in head • Intrinsic problems with correction of both slice timing and head motion
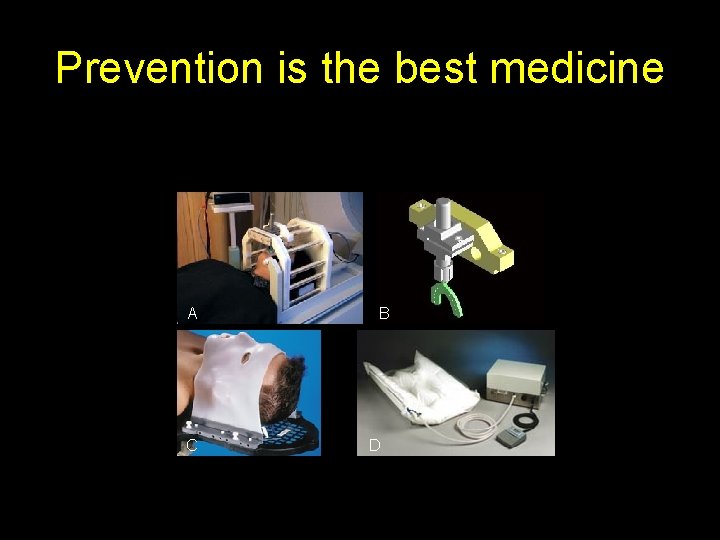
Prevention is the best medicine A C B D
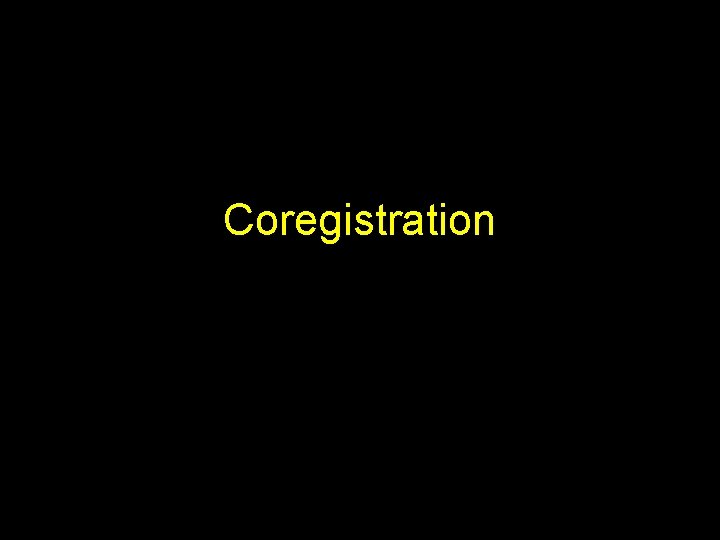
Coregistration
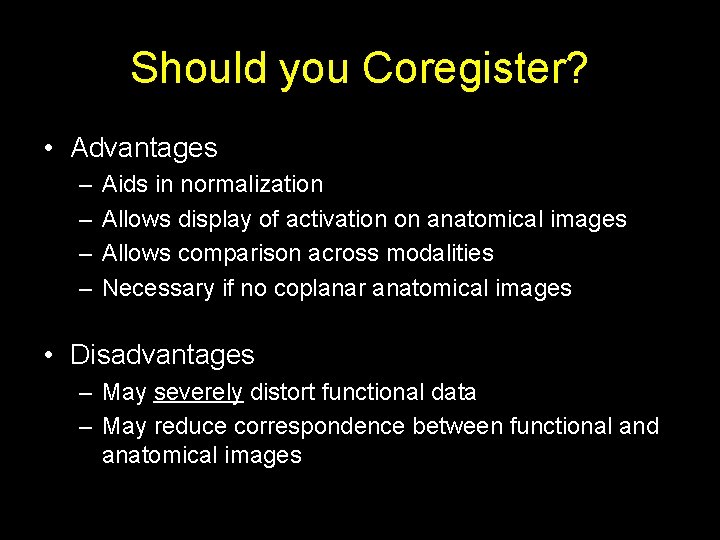
Should you Coregister? • Advantages – – Aids in normalization Allows display of activation on anatomical images Allows comparison across modalities Necessary if no coplanar anatomical images • Disadvantages – May severely distort functional data – May reduce correspondence between functional and anatomical images
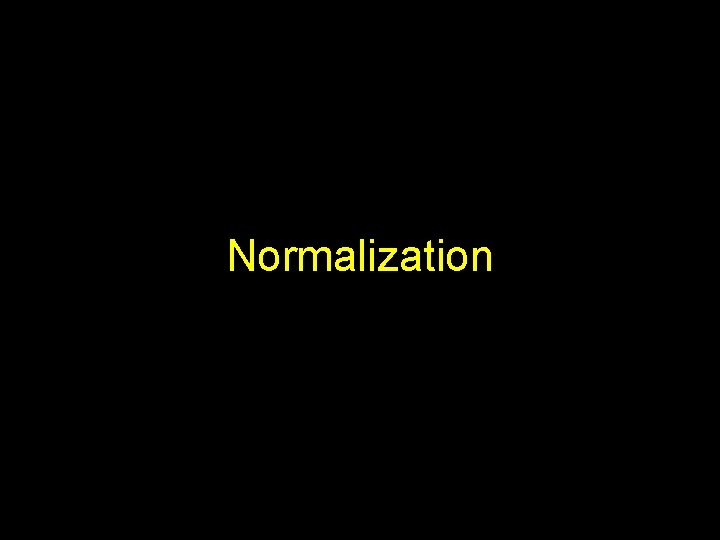
Normalization
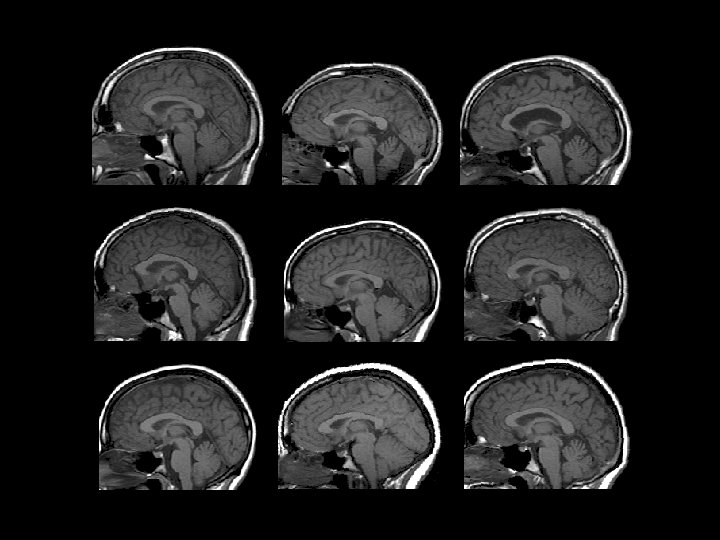
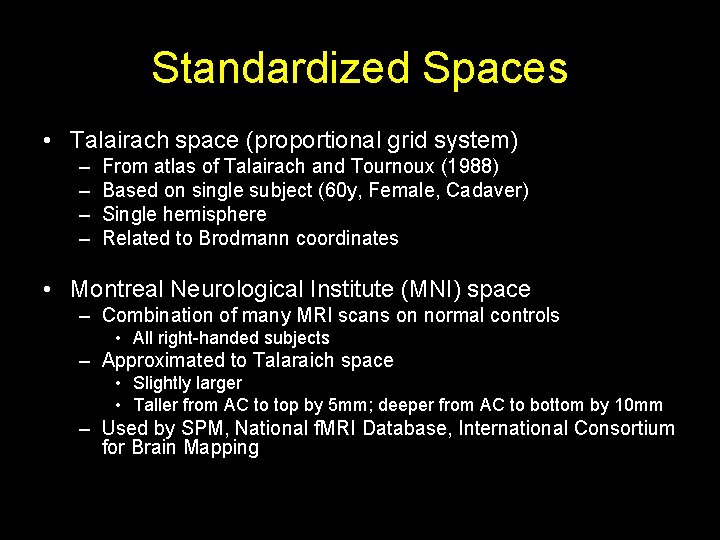
Standardized Spaces • Talairach space (proportional grid system) – – From atlas of Talairach and Tournoux (1988) Based on single subject (60 y, Female, Cadaver) Single hemisphere Related to Brodmann coordinates • Montreal Neurological Institute (MNI) space – Combination of many MRI scans on normal controls • All right-handed subjects – Approximated to Talaraich space • Slightly larger • Taller from AC to top by 5 mm; deeper from AC to bottom by 10 mm – Used by SPM, National f. MRI Database, International Consortium for Brain Mapping
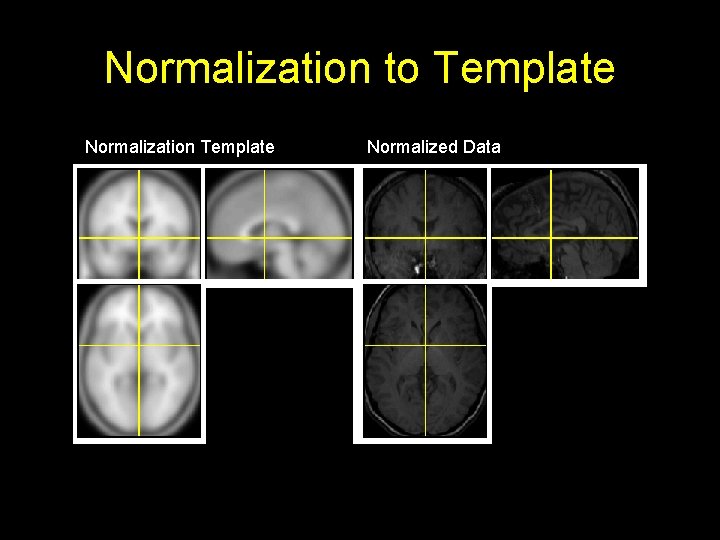
Normalization to Template Normalization Template Normalized Data
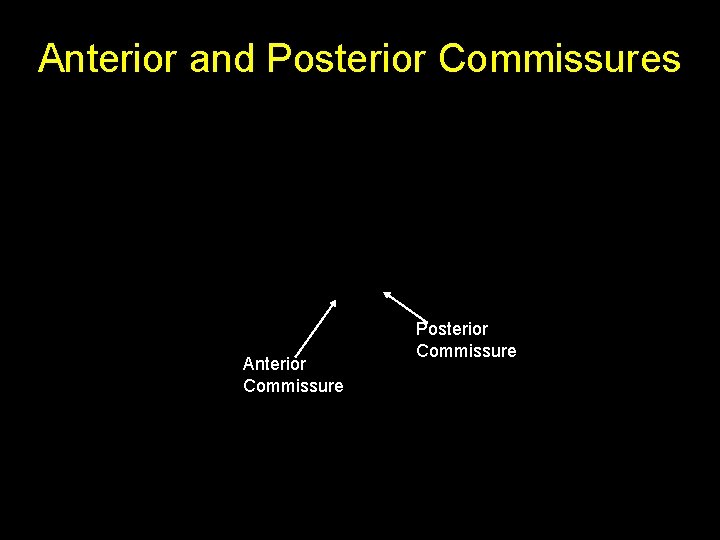
Anterior and Posterior Commissures Anterior Commissure Posterior Commissure
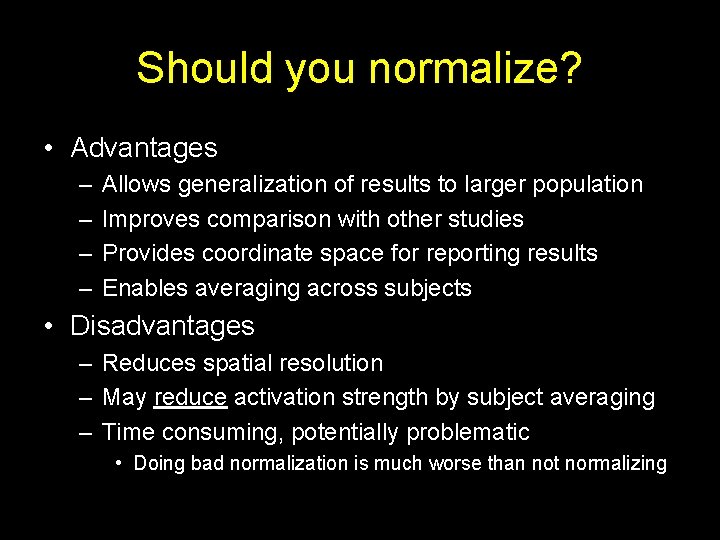
Should you normalize? • Advantages – – Allows generalization of results to larger population Improves comparison with other studies Provides coordinate space for reporting results Enables averaging across subjects • Disadvantages – Reduces spatial resolution – May reduce activation strength by subject averaging – Time consuming, potentially problematic • Doing bad normalization is much worse than not normalizing
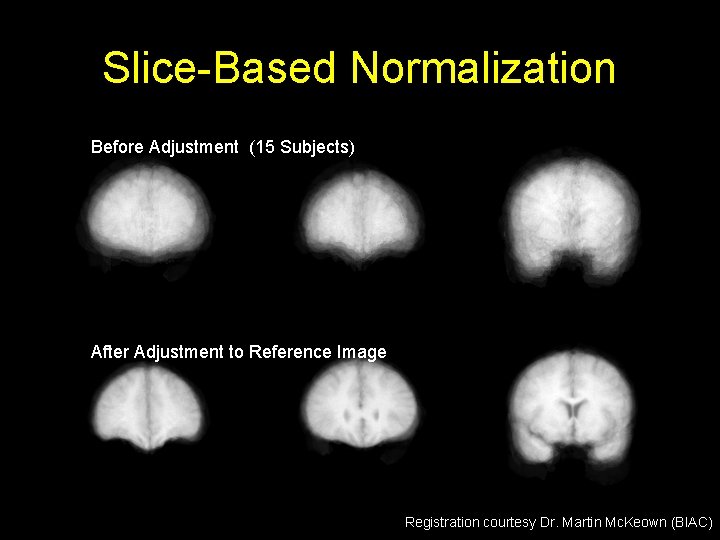
Slice-Based Normalization Before Adjustment (15 Subjects) After Adjustment to Reference Image Registration courtesy Dr. Martin Mc. Keown (BIAC)
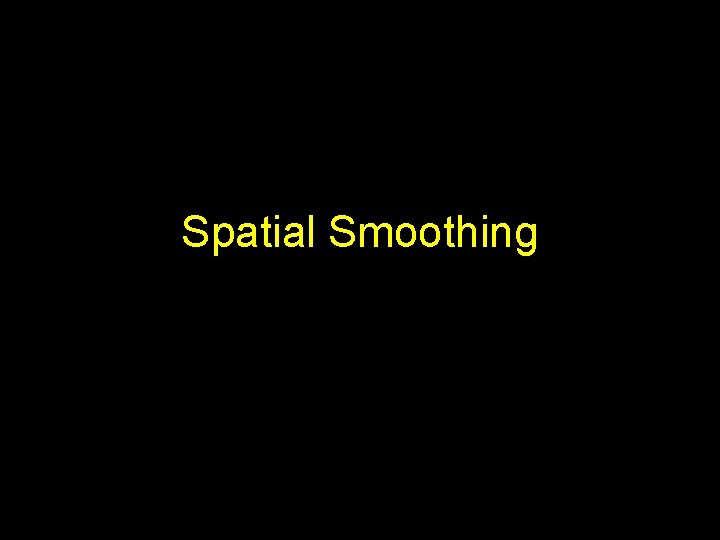
Spatial Smoothing
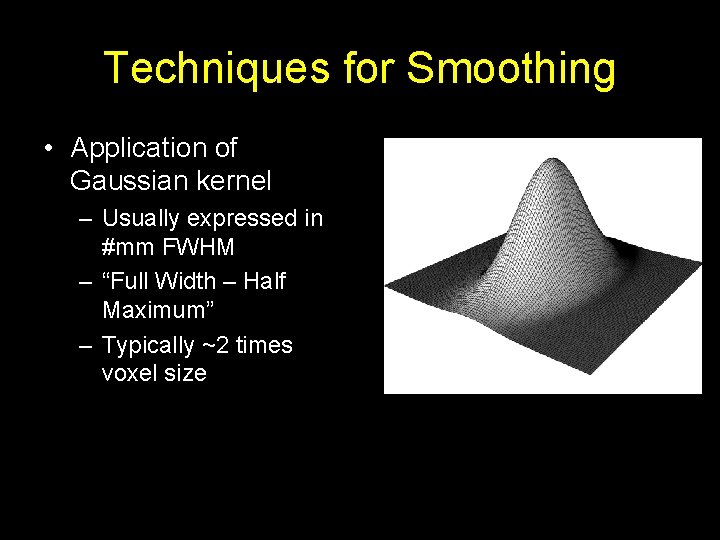
Techniques for Smoothing • Application of Gaussian kernel – Usually expressed in #mm FWHM – “Full Width – Half Maximum” – Typically ~2 times voxel size
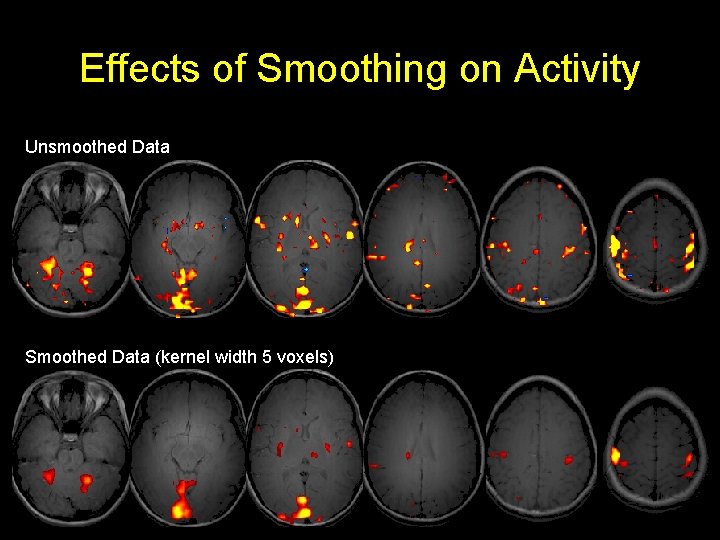
Effects of Smoothing on Activity Unsmoothed Data Smoothed Data (kernel width 5 voxels)
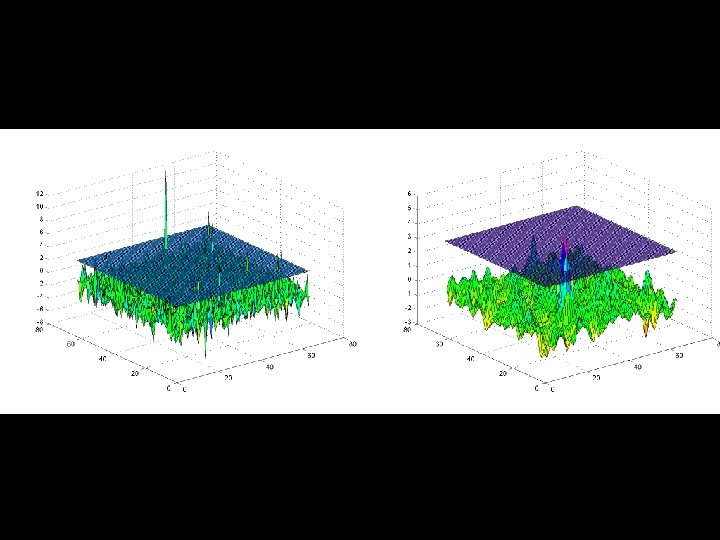
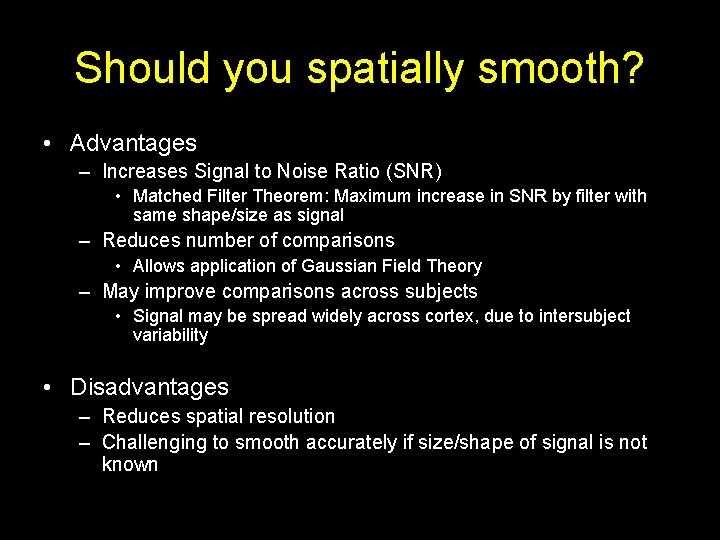
Should you spatially smooth? • Advantages – Increases Signal to Noise Ratio (SNR) • Matched Filter Theorem: Maximum increase in SNR by filter with same shape/size as signal – Reduces number of comparisons • Allows application of Gaussian Field Theory – May improve comparisons across subjects • Signal may be spread widely across cortex, due to intersubject variability • Disadvantages – Reduces spatial resolution – Challenging to smooth accurately if size/shape of signal is not known
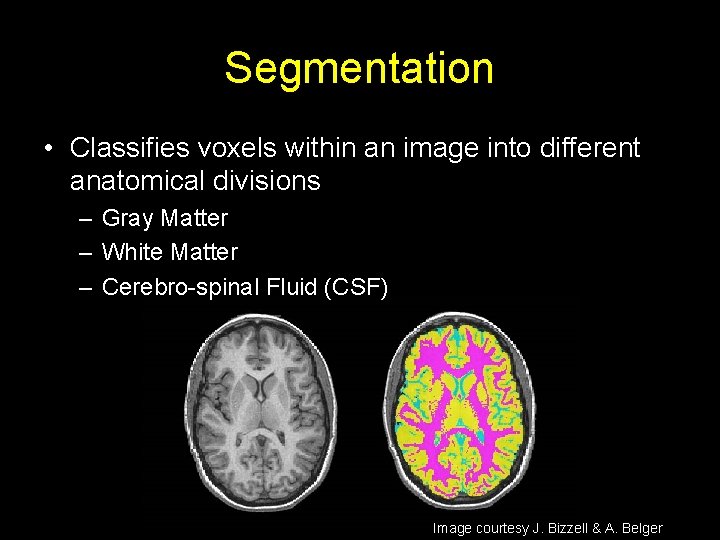
Segmentation • Classifies voxels within an image into different anatomical divisions – Gray Matter – White Matter – Cerebro-spinal Fluid (CSF) Image courtesy J. Bizzell & A. Belger
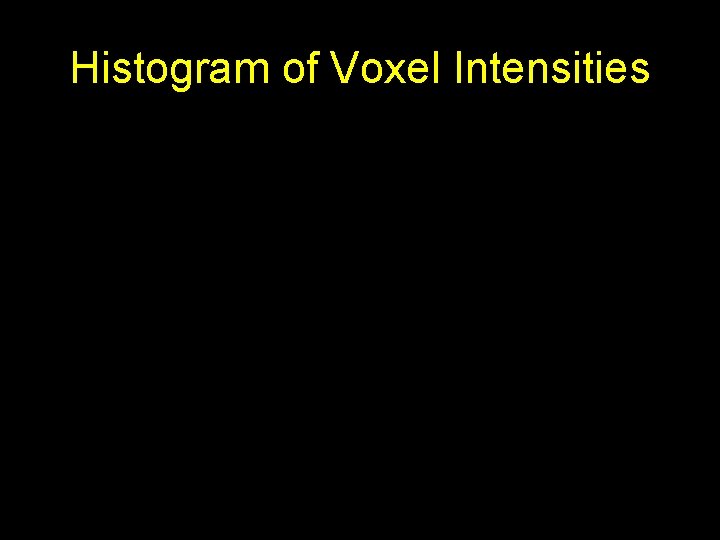
Histogram of Voxel Intensities
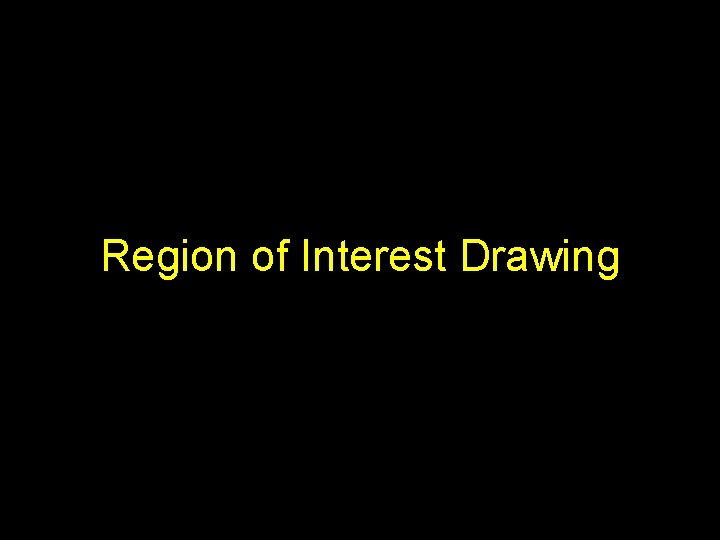
Region of Interest Drawing
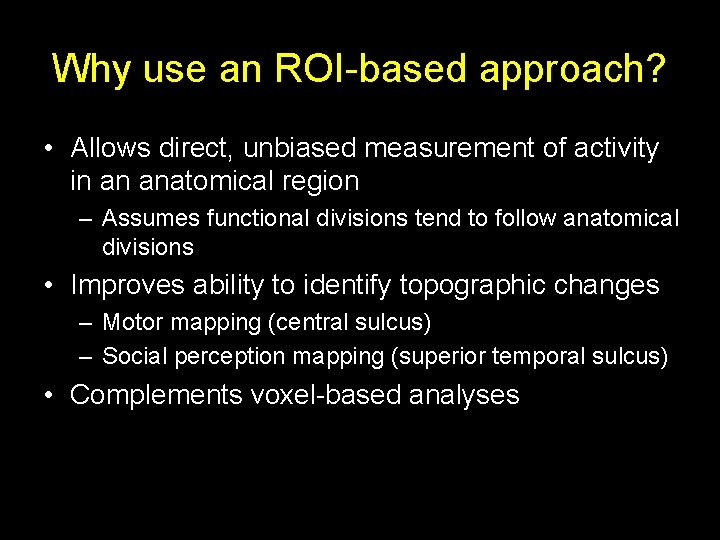
Why use an ROI-based approach? • Allows direct, unbiased measurement of activity in an anatomical region – Assumes functional divisions tend to follow anatomical divisions • Improves ability to identify topographic changes – Motor mapping (central sulcus) – Social perception mapping (superior temporal sulcus) • Complements voxel-based analyses
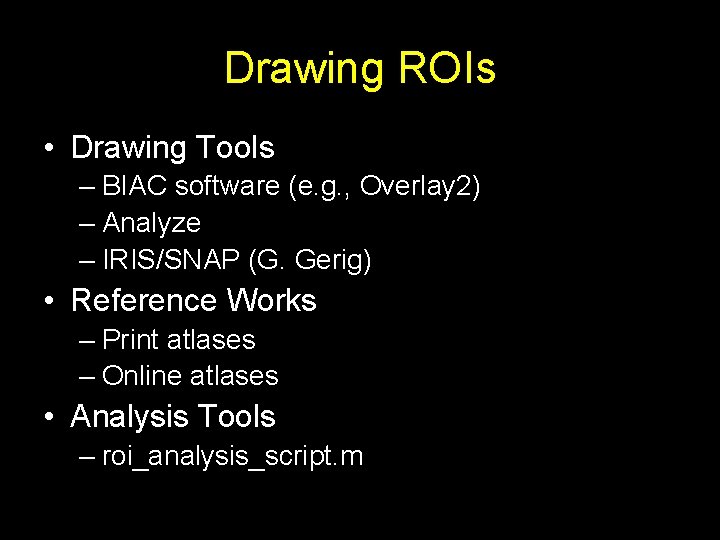
Drawing ROIs • Drawing Tools – BIAC software (e. g. , Overlay 2) – Analyze – IRIS/SNAP (G. Gerig) • Reference Works – Print atlases – Online atlases • Analysis Tools – roi_analysis_script. m
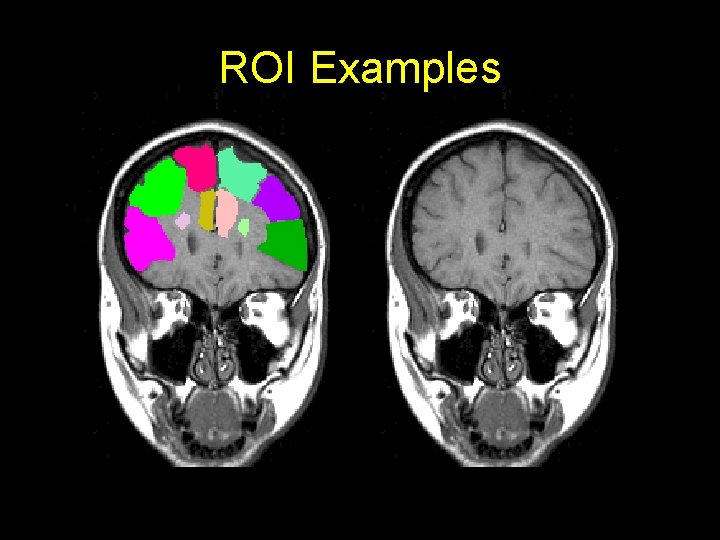
ROI Examples
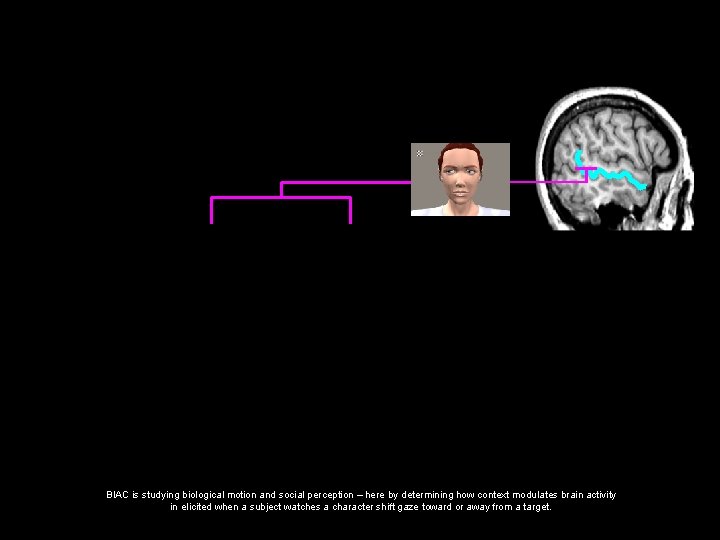
BIAC is studying biological motion and social perception – here by determining how context modulates brain activity in elicited when a subject watches a character shift gaze toward or away from a target.
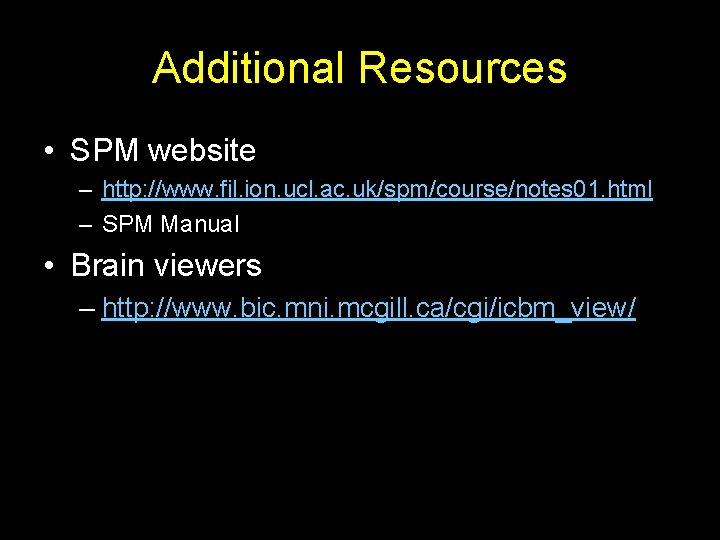
Additional Resources • SPM website – http: //www. fil. ion. ucl. ac. uk/spm/course/notes 01. html – SPM Manual • Brain viewers – http: //www. bic. mni. mcgill. ca/cgi/icbm_view/
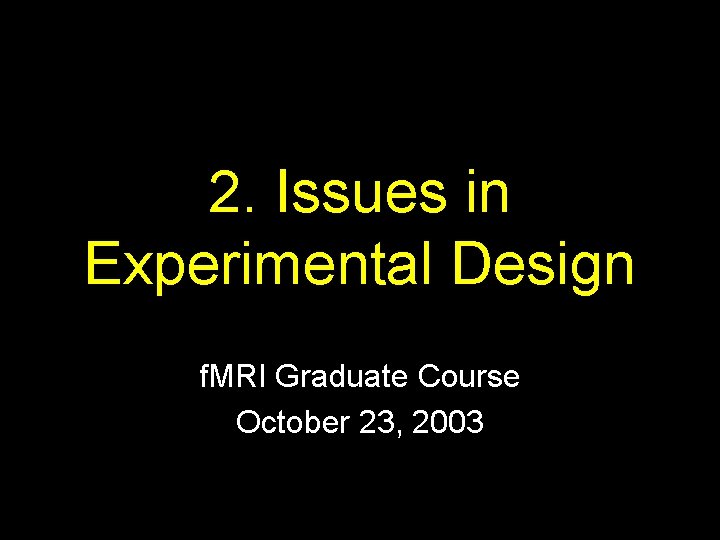
2. Issues in Experimental Design f. MRI Graduate Course October 23, 2003
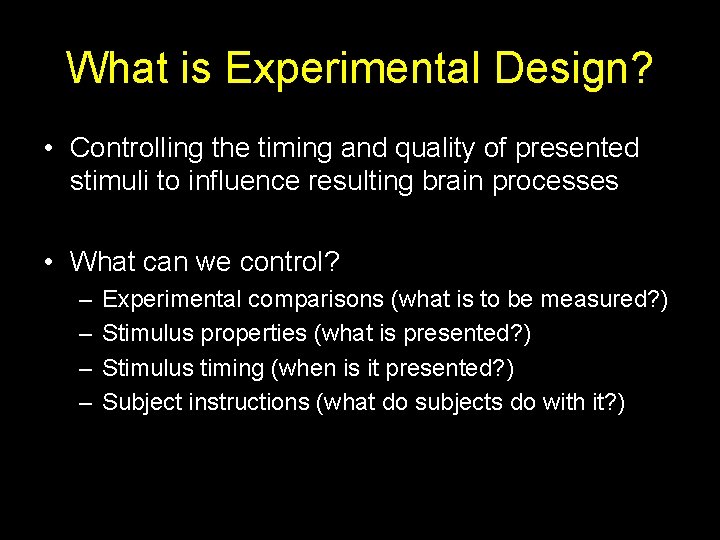
What is Experimental Design? • Controlling the timing and quality of presented stimuli to influence resulting brain processes • What can we control? – – Experimental comparisons (what is to be measured? ) Stimulus properties (what is presented? ) Stimulus timing (when is it presented? ) Subject instructions (what do subjects do with it? )
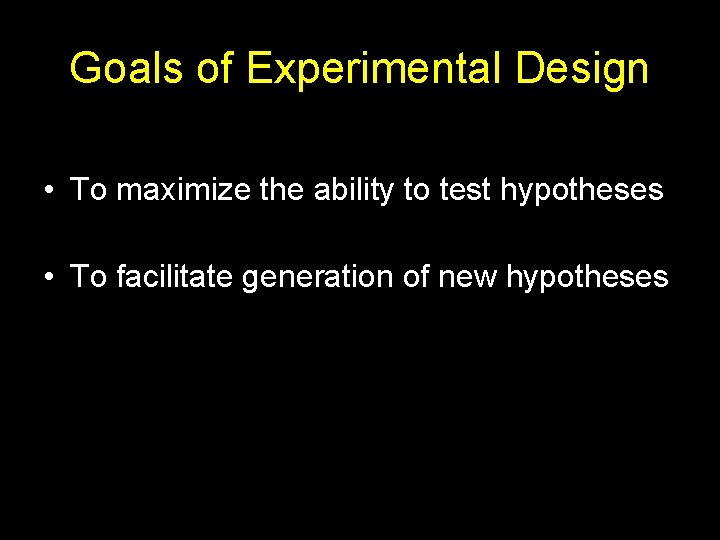
Goals of Experimental Design • To maximize the ability to test hypotheses • To facilitate generation of new hypotheses
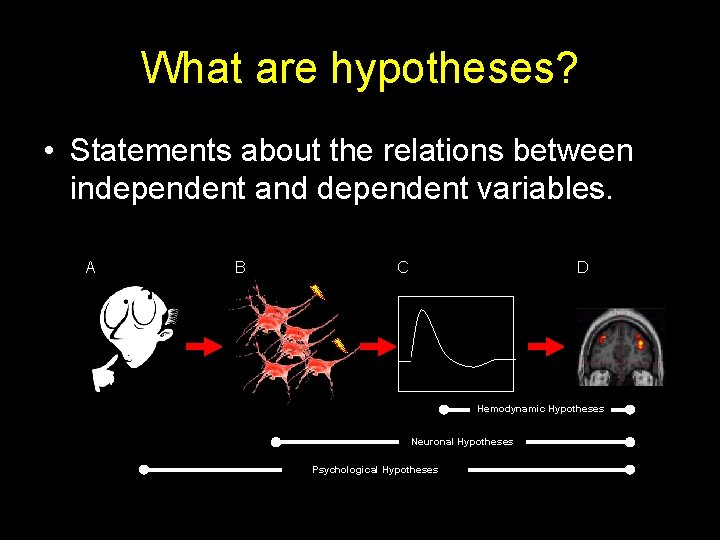
What are hypotheses? • Statements about the relations between independent and dependent variables. A B C D Hemodynamic Hypotheses Neuronal Hypotheses Psychological Hypotheses
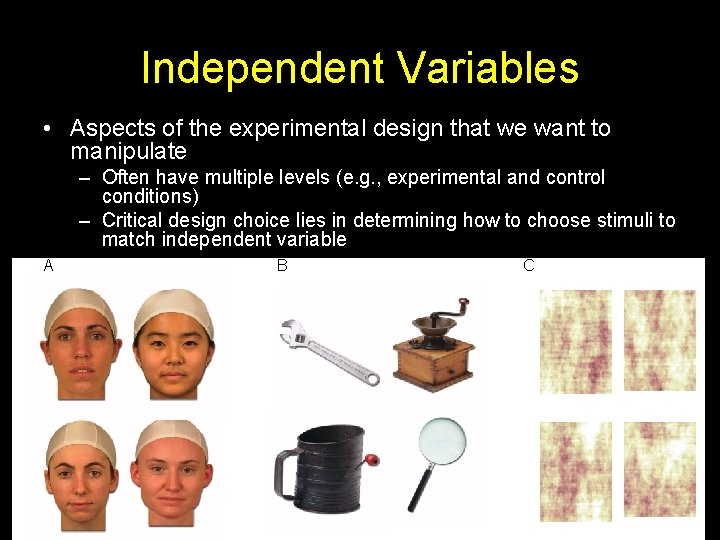
Independent Variables • Aspects of the experimental design that we want to manipulate – Often have multiple levels (e. g. , experimental and control conditions) – Critical design choice lies in determining how to choose stimuli to match independent variable A B C
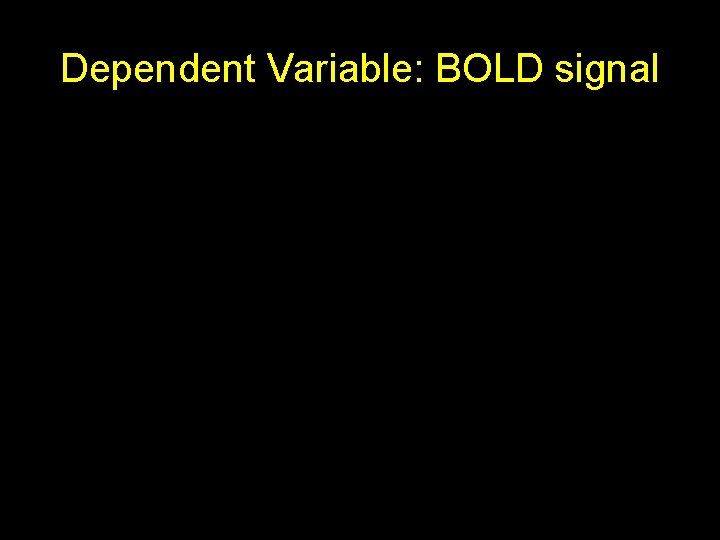
Dependent Variable: BOLD signal
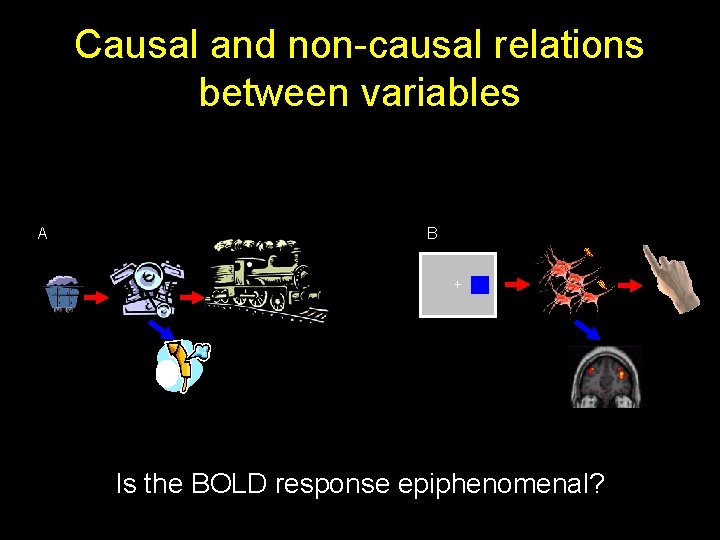
Causal and non-causal relations between variables A B Is the BOLD response epiphenomenal?
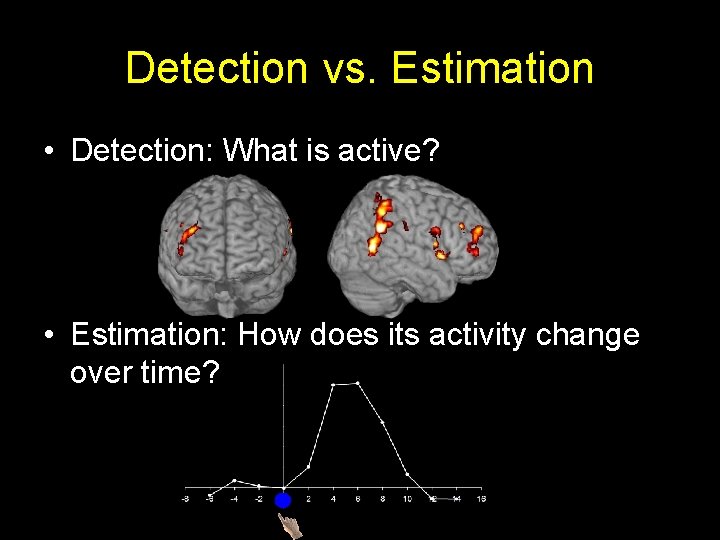
Detection vs. Estimation • Detection: What is active? • Estimation: How does its activity change over time?
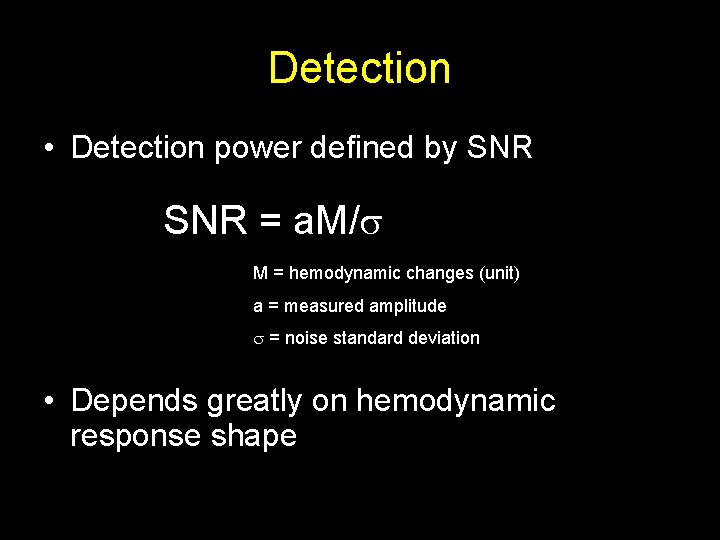
Detection • Detection power defined by SNR = a. M/ M = hemodynamic changes (unit) a = measured amplitude = noise standard deviation • Depends greatly on hemodynamic response shape
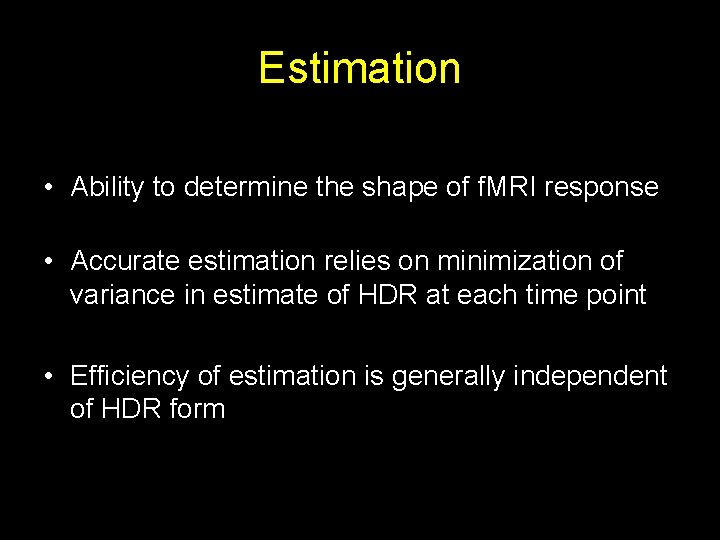
Estimation • Ability to determine the shape of f. MRI response • Accurate estimation relies on minimization of variance in estimate of HDR at each time point • Efficiency of estimation is generally independent of HDR form
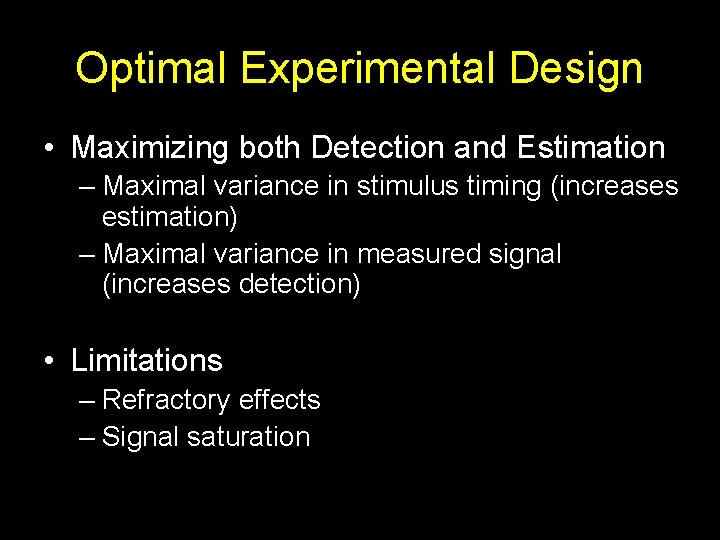
Optimal Experimental Design • Maximizing both Detection and Estimation – Maximal variance in stimulus timing (increases estimation) – Maximal variance in measured signal (increases detection) • Limitations – Refractory effects – Signal saturation
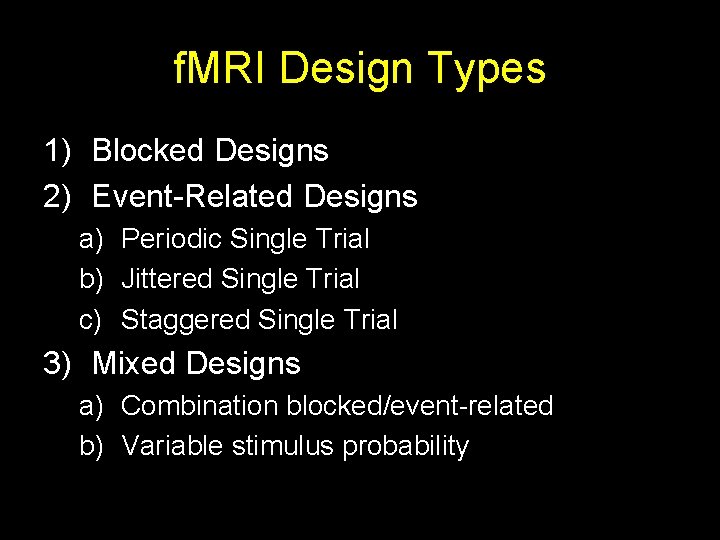
f. MRI Design Types 1) Blocked Designs 2) Event-Related Designs a) Periodic Single Trial b) Jittered Single Trial c) Staggered Single Trial 3) Mixed Designs a) Combination blocked/event-related b) Variable stimulus probability
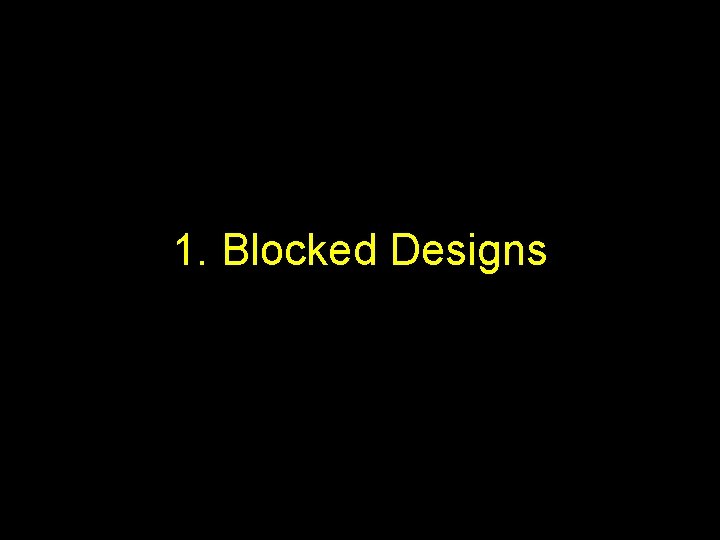
1. Blocked Designs
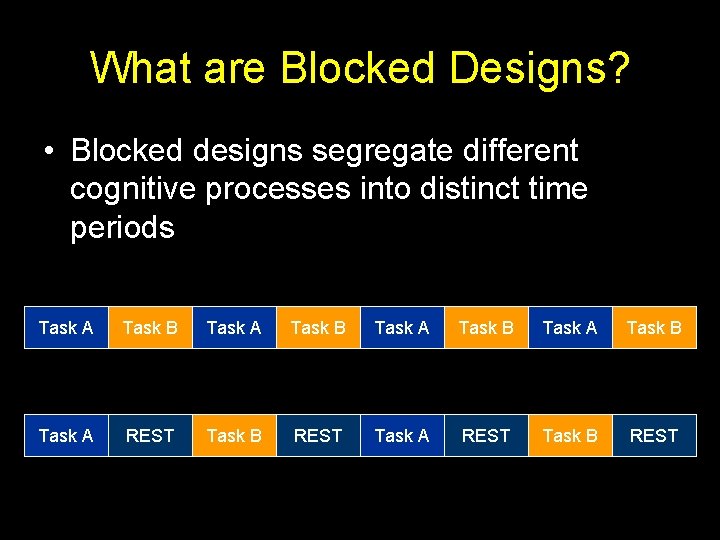
What are Blocked Designs? • Blocked designs segregate different cognitive processes into distinct time periods Task A Task B Task A REST Task B REST
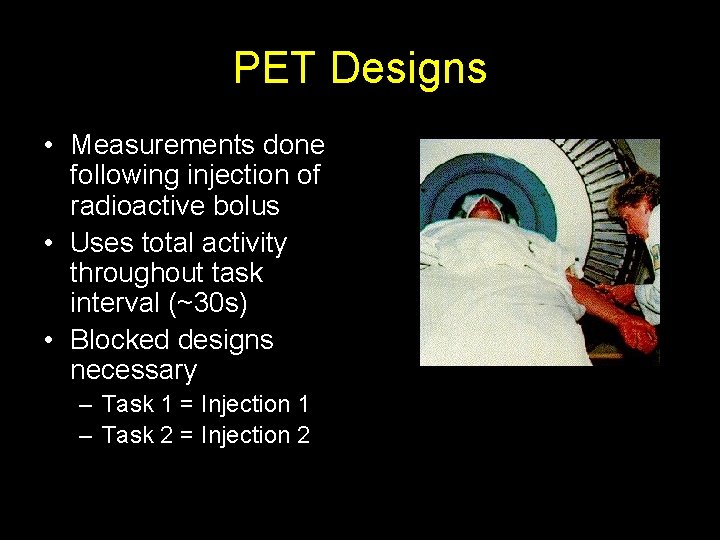
PET Designs • Measurements done following injection of radioactive bolus • Uses total activity throughout task interval (~30 s) • Blocked designs necessary – Task 1 = Injection 1 – Task 2 = Injection 2
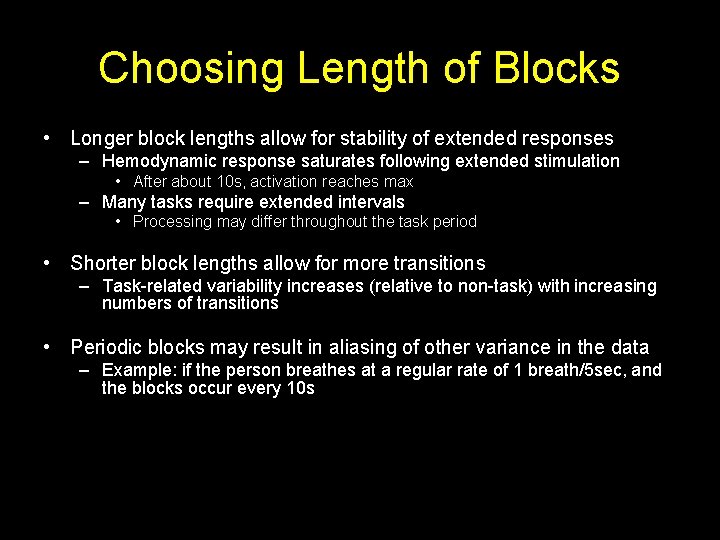
Choosing Length of Blocks • Longer block lengths allow for stability of extended responses – Hemodynamic response saturates following extended stimulation • After about 10 s, activation reaches max – Many tasks require extended intervals • Processing may differ throughout the task period • Shorter block lengths allow for more transitions – Task-related variability increases (relative to non-task) with increasing numbers of transitions • Periodic blocks may result in aliasing of other variance in the data – Example: if the person breathes at a regular rate of 1 breath/5 sec, and the blocks occur every 10 s
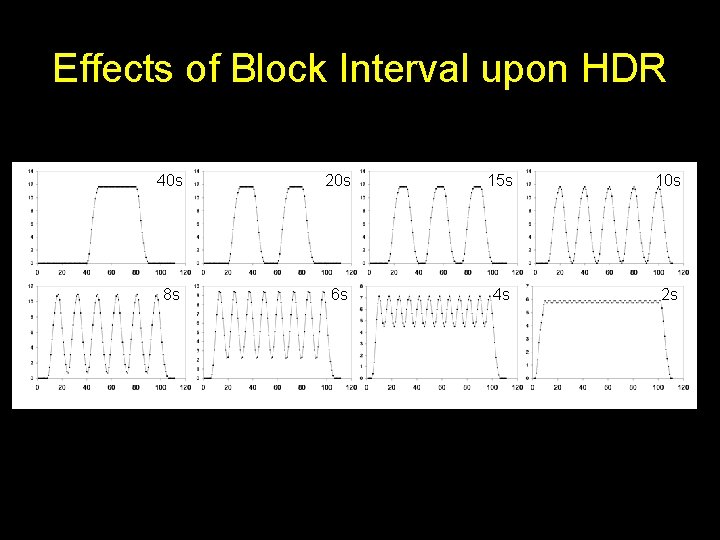
Effects of Block Interval upon HDR 40 s 20 s 15 s 10 s 8 s 6 s 4 s 2 s
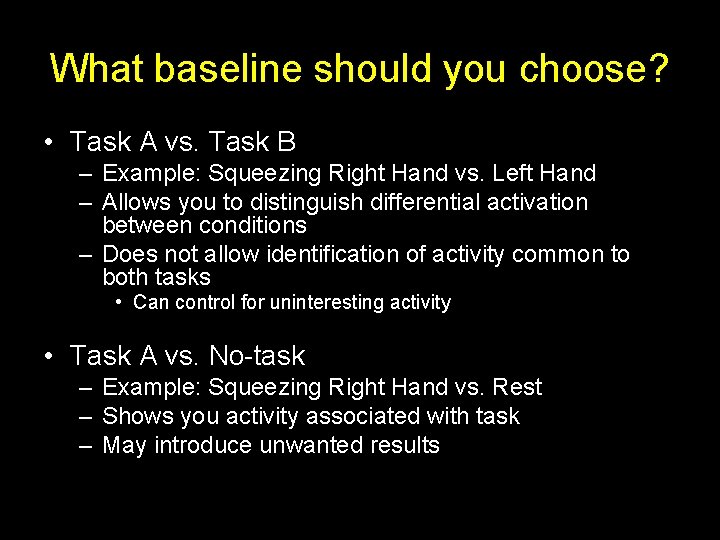
What baseline should you choose? • Task A vs. Task B – Example: Squeezing Right Hand vs. Left Hand – Allows you to distinguish differential activation between conditions – Does not allow identification of activity common to both tasks • Can control for uninteresting activity • Task A vs. No-task – Example: Squeezing Right Hand vs. Rest – Shows you activity associated with task – May introduce unwanted results
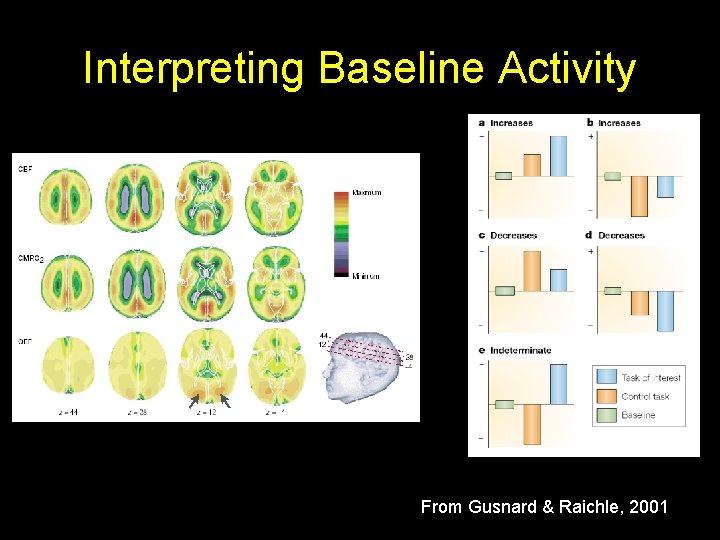
Interpreting Baseline Activity From Gusnard & Raichle, 2001
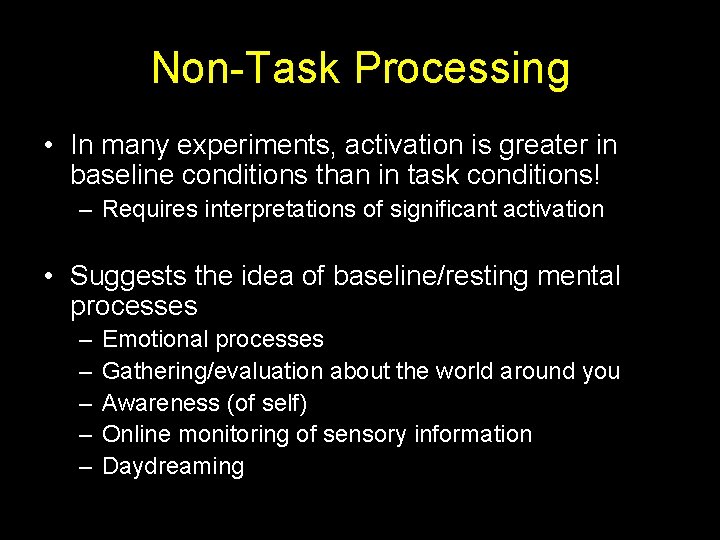
Non-Task Processing • In many experiments, activation is greater in baseline conditions than in task conditions! – Requires interpretations of significant activation • Suggests the idea of baseline/resting mental processes – – – Emotional processes Gathering/evaluation about the world around you Awareness (of self) Online monitoring of sensory information Daydreaming
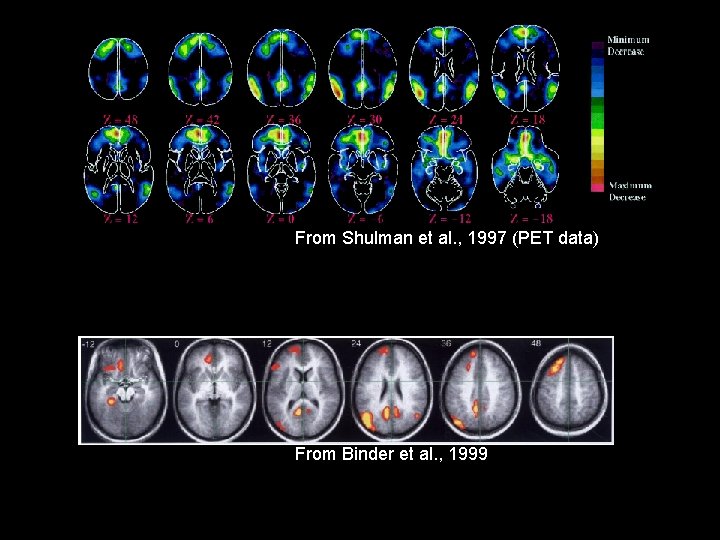
From Shulman et al. , 1997 (PET data) From Binder et al. , 1999
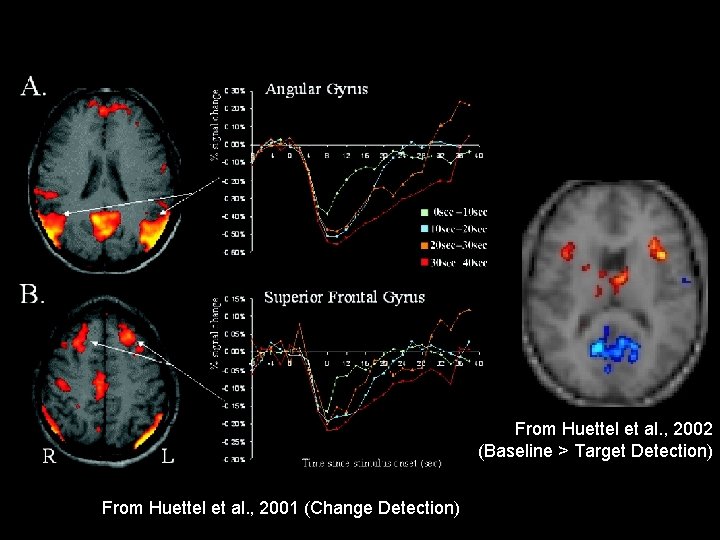
From Huettel et al. , 2002 (Baseline > Target Detection) From Huettel et al. , 2001 (Change Detection)
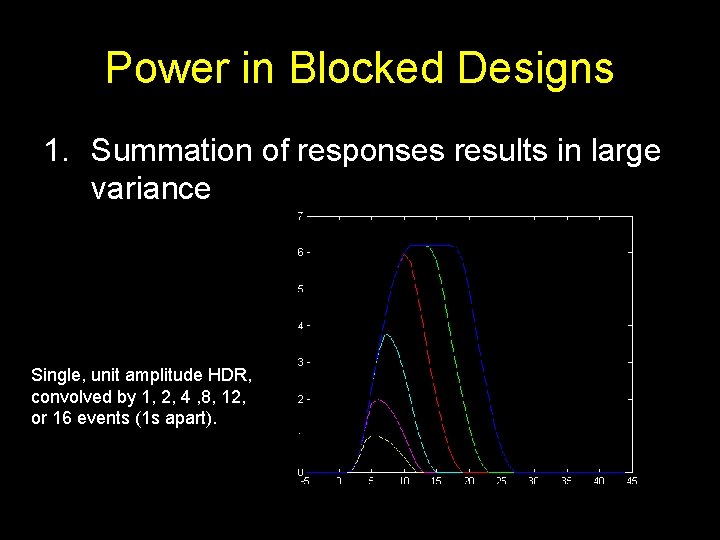
Power in Blocked Designs 1. Summation of responses results in large variance Single, unit amplitude HDR, convolved by 1, 2, 4 , 8, 12, or 16 events (1 s apart).
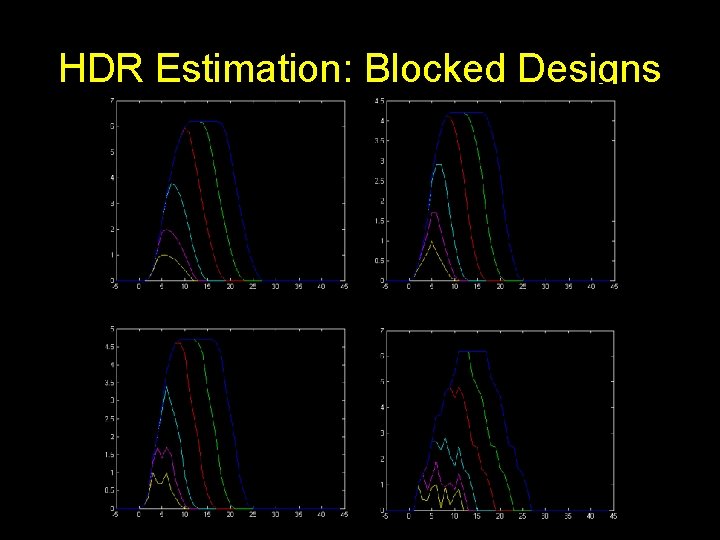
HDR Estimation: Blocked Designs
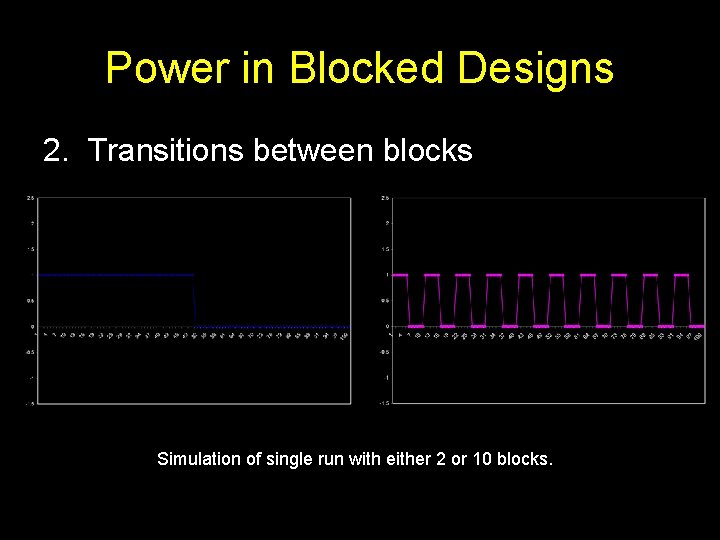
Power in Blocked Designs 2. Transitions between blocks Simulation of single run with either 2 or 10 blocks.
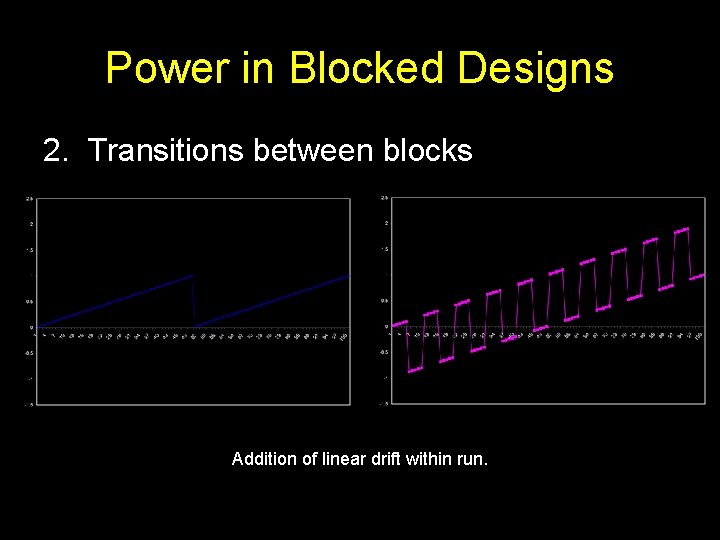
Power in Blocked Designs 2. Transitions between blocks Addition of linear drift within run.
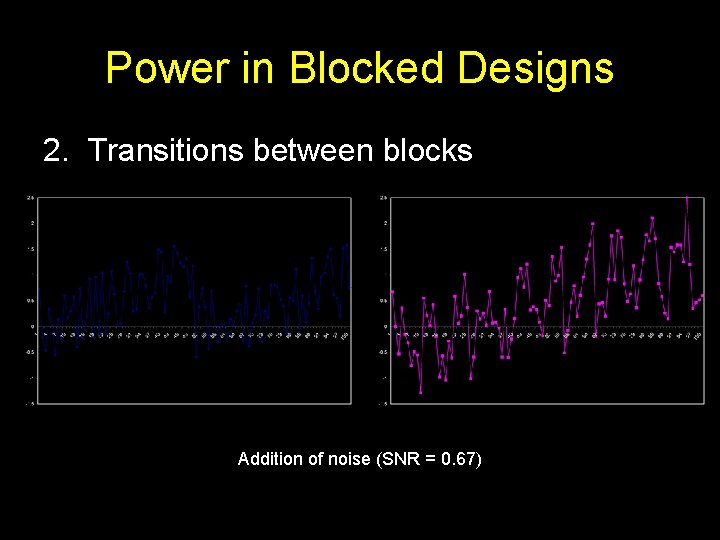
Power in Blocked Designs 2. Transitions between blocks Addition of noise (SNR = 0. 67)
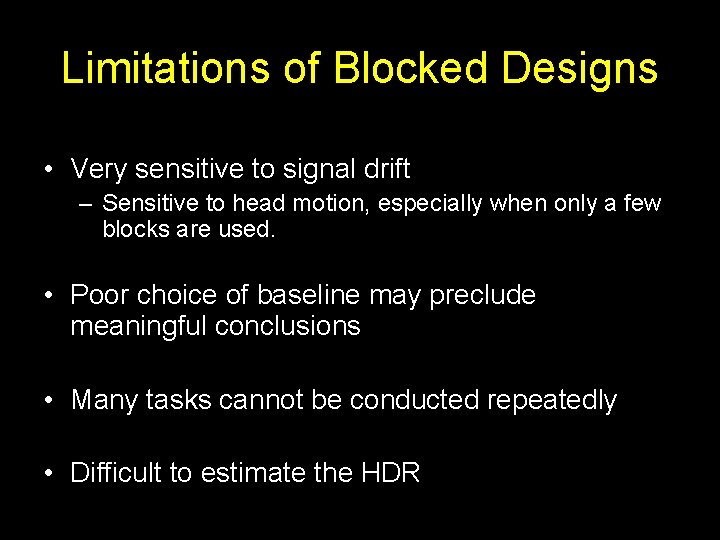
Limitations of Blocked Designs • Very sensitive to signal drift – Sensitive to head motion, especially when only a few blocks are used. • Poor choice of baseline may preclude meaningful conclusions • Many tasks cannot be conducted repeatedly • Difficult to estimate the HDR
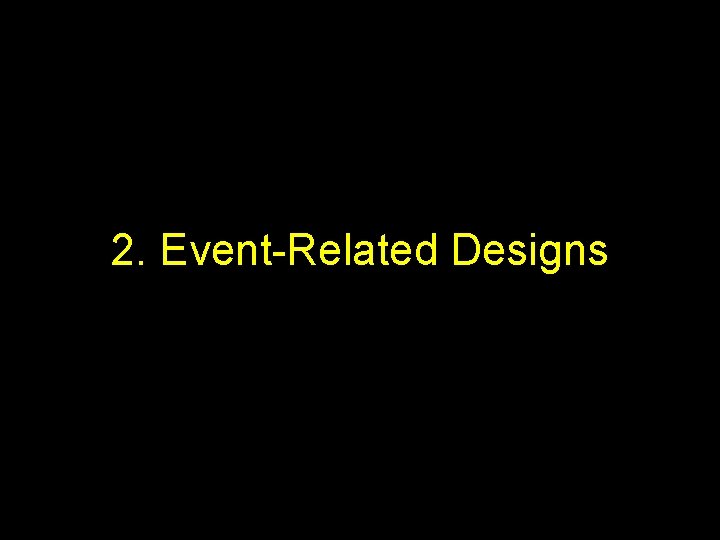
2. Event-Related Designs
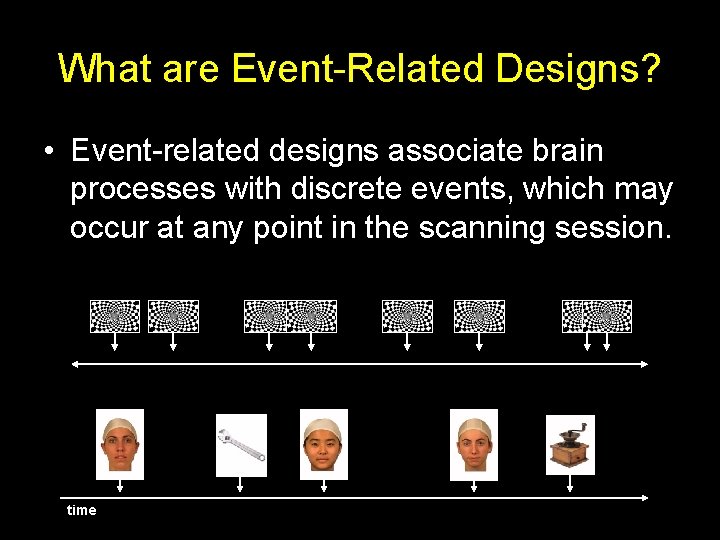
What are Event-Related Designs? • Event-related designs associate brain processes with discrete events, which may occur at any point in the scanning session. time
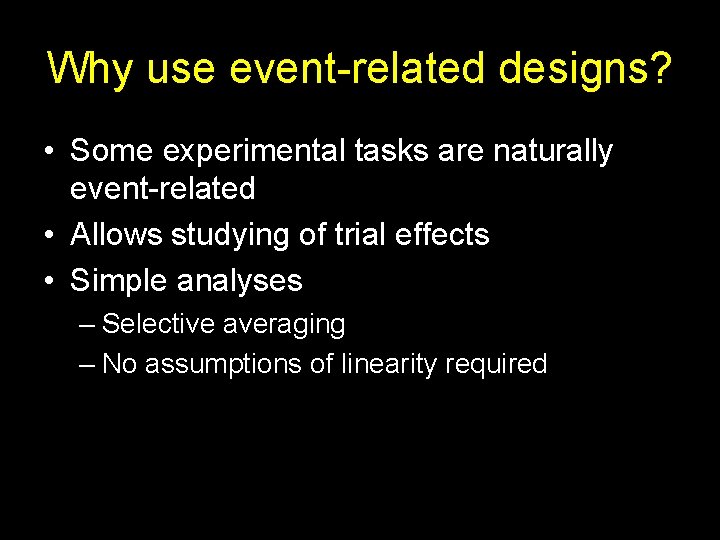
Why use event-related designs? • Some experimental tasks are naturally event-related • Allows studying of trial effects • Simple analyses – Selective averaging – No assumptions of linearity required
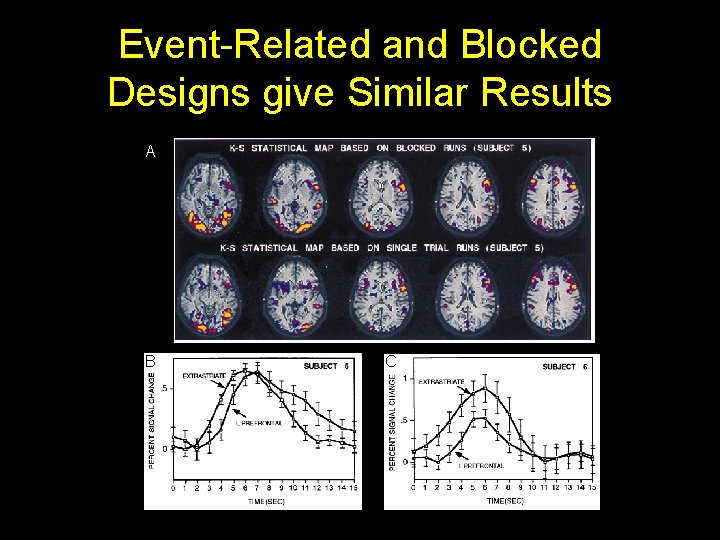
Event-Related and Blocked Designs give Similar Results A B C
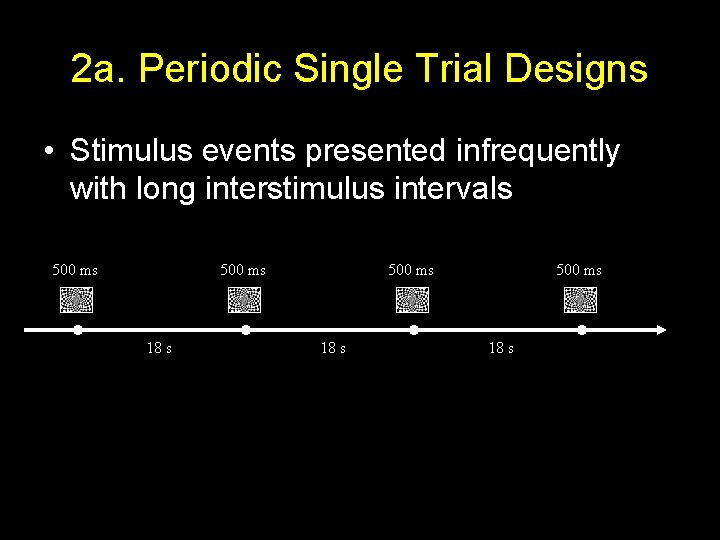
2 a. Periodic Single Trial Designs • Stimulus events presented infrequently with long interstimulus intervals 500 ms 18 s
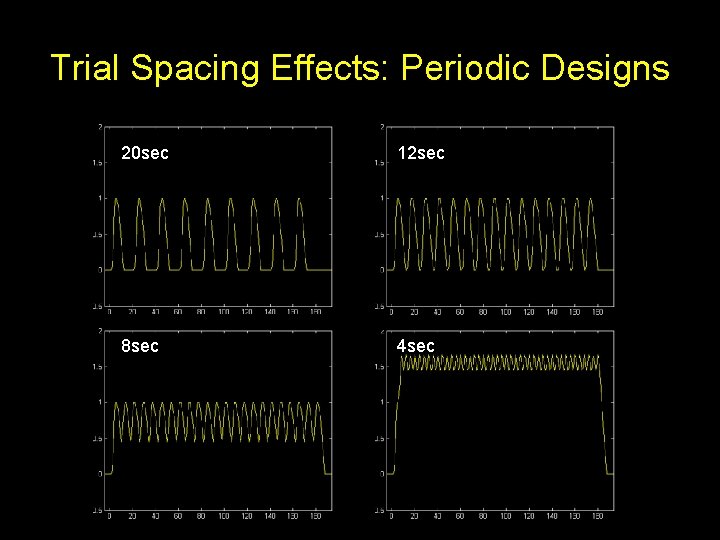
Trial Spacing Effects: Periodic Designs 20 sec 12 sec 8 sec 4 sec
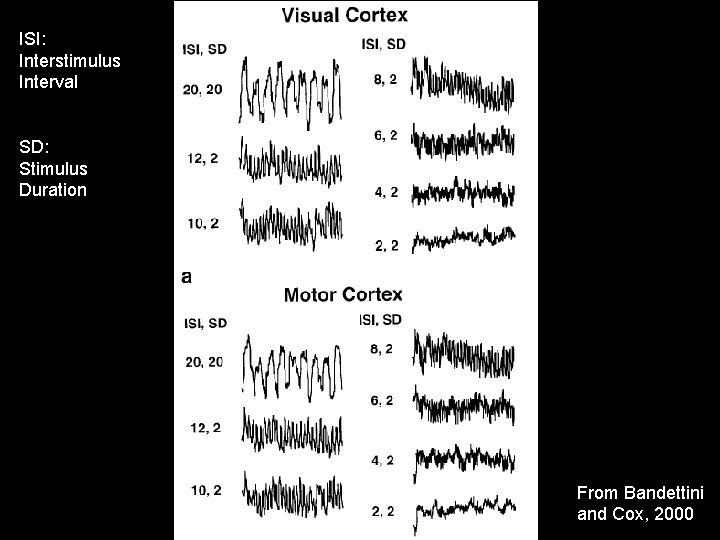
ISI: Interstimulus Interval SD: Stimulus Duration From Bandettini and Cox, 2000
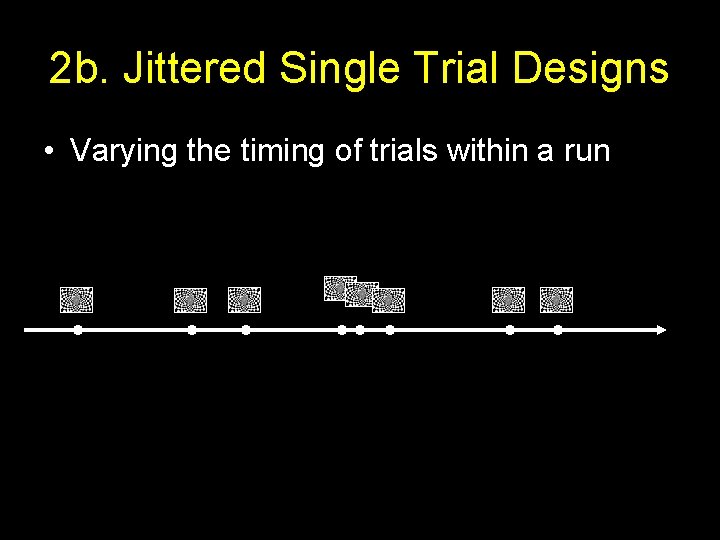
2 b. Jittered Single Trial Designs • Varying the timing of trials within a run
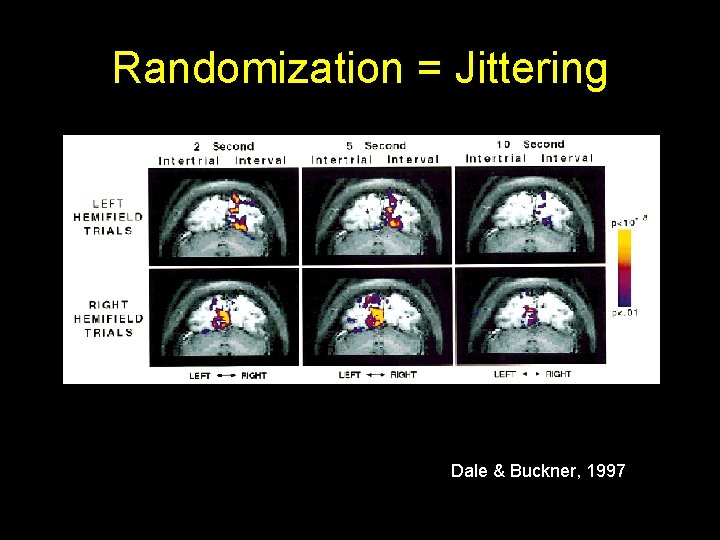
Randomization = Jittering Dale & Buckner, 1997
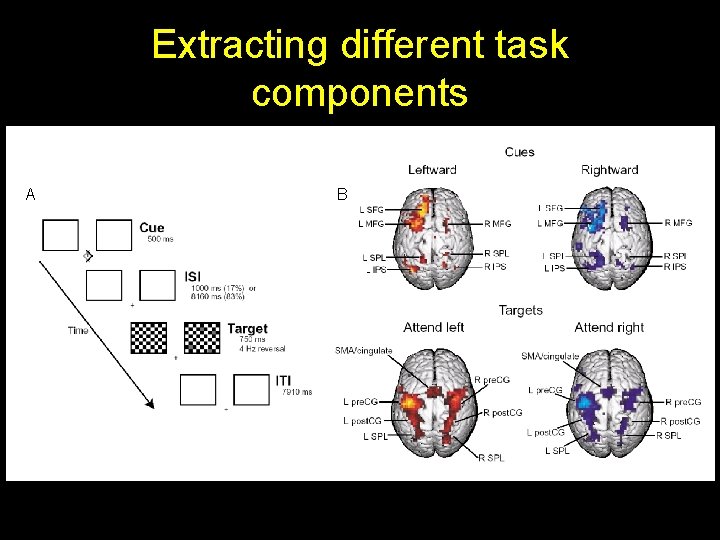
Extracting different task components A B
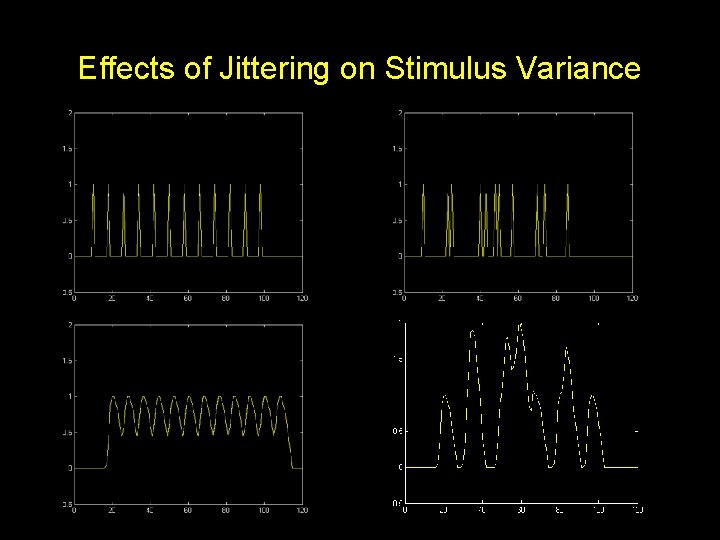
Effects of Jittering on Stimulus Variance
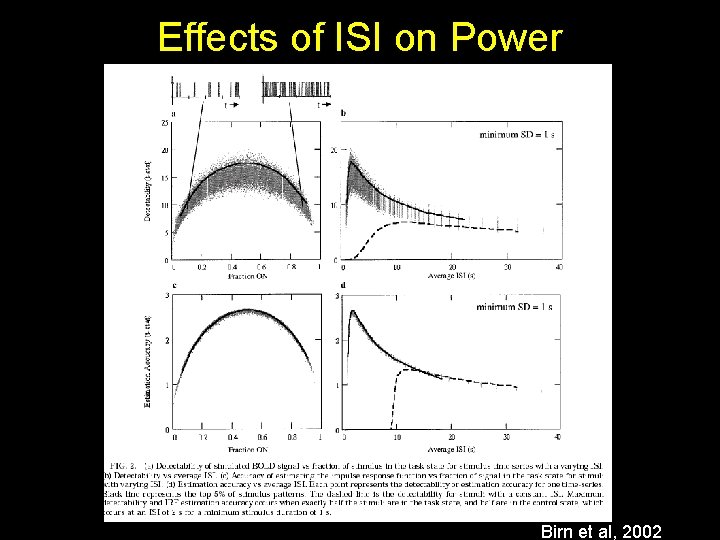
Effects of ISI on Power Birn et al, 2002
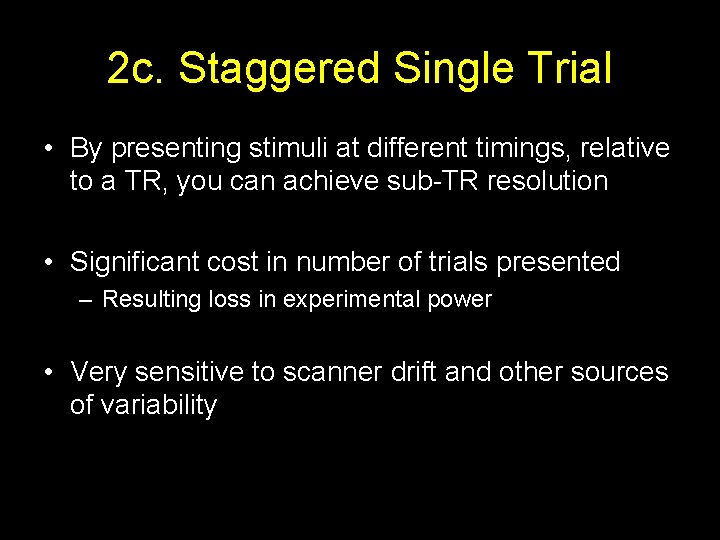
2 c. Staggered Single Trial • By presenting stimuli at different timings, relative to a TR, you can achieve sub-TR resolution • Significant cost in number of trials presented – Resulting loss in experimental power • Very sensitive to scanner drift and other sources of variability
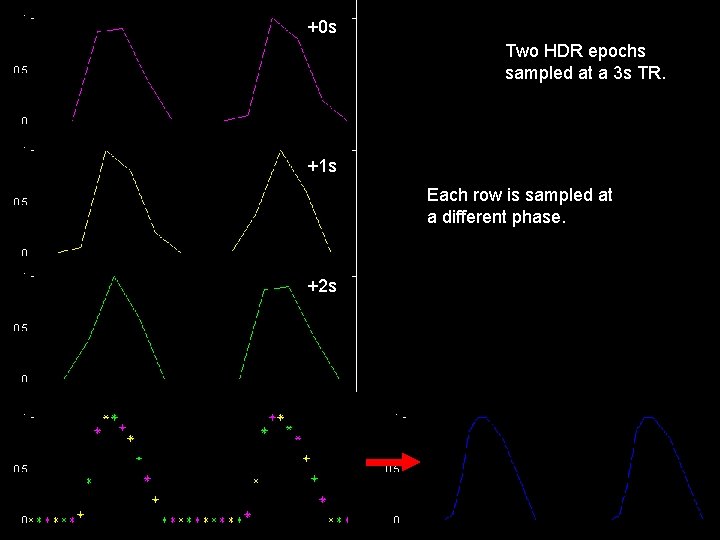
+0 s Two HDR epochs sampled at a 3 s TR. +1 s Each row is sampled at a different phase. +2 s
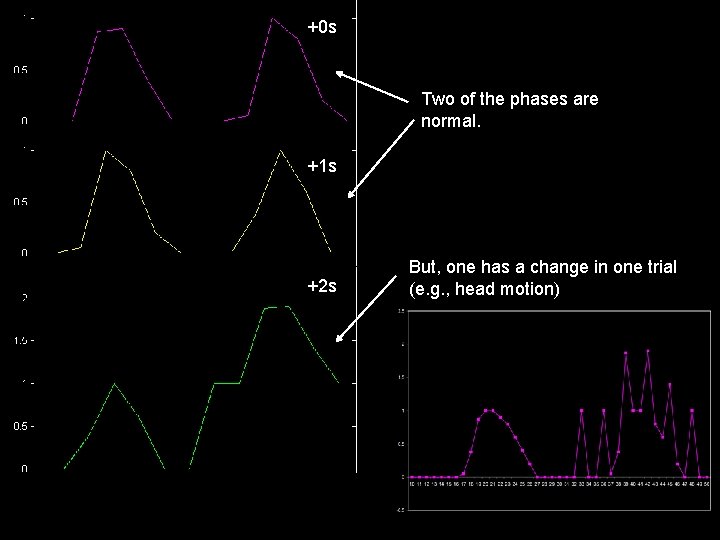
+0 s Two of the phases are normal. +1 s +2 s But, one has a change in one trial (e. g. , head motion)
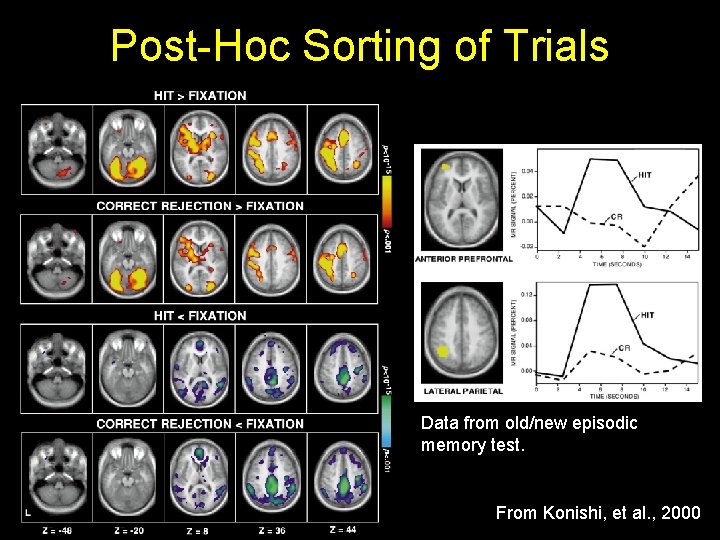
Post-Hoc Sorting of Trials Data from old/new episodic memory test. From Konishi, et al. , 2000
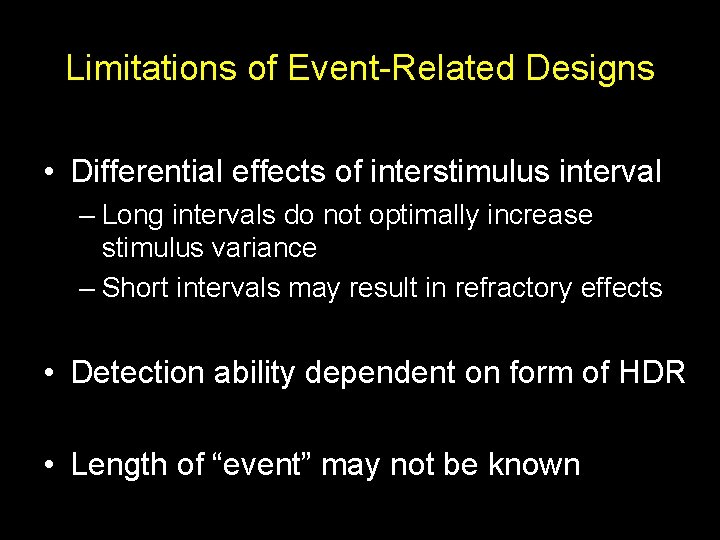
Limitations of Event-Related Designs • Differential effects of interstimulus interval – Long intervals do not optimally increase stimulus variance – Short intervals may result in refractory effects • Detection ability dependent on form of HDR • Length of “event” may not be known
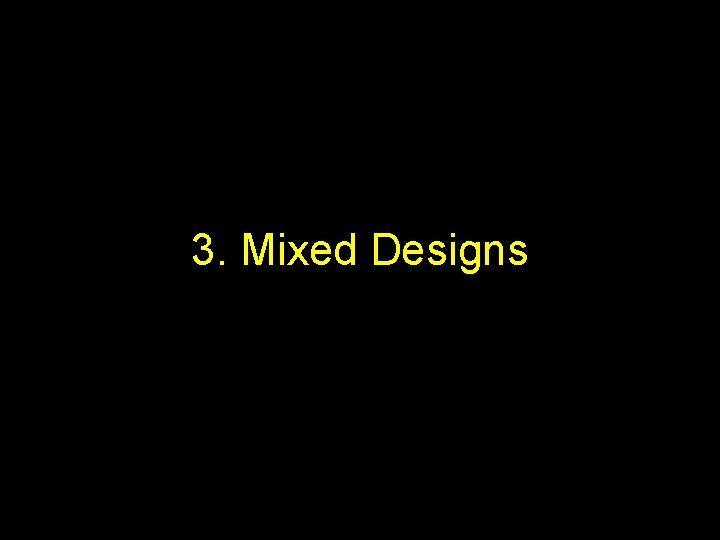
3. Mixed Designs
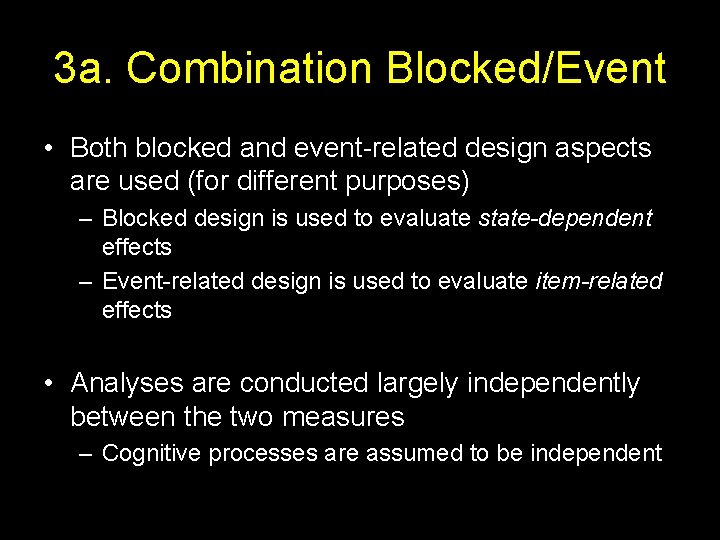
3 a. Combination Blocked/Event • Both blocked and event-related design aspects are used (for different purposes) – Blocked design is used to evaluate state-dependent effects – Event-related design is used to evaluate item-related effects • Analyses are conducted largely independently between the two measures – Cognitive processes are assumed to be independent
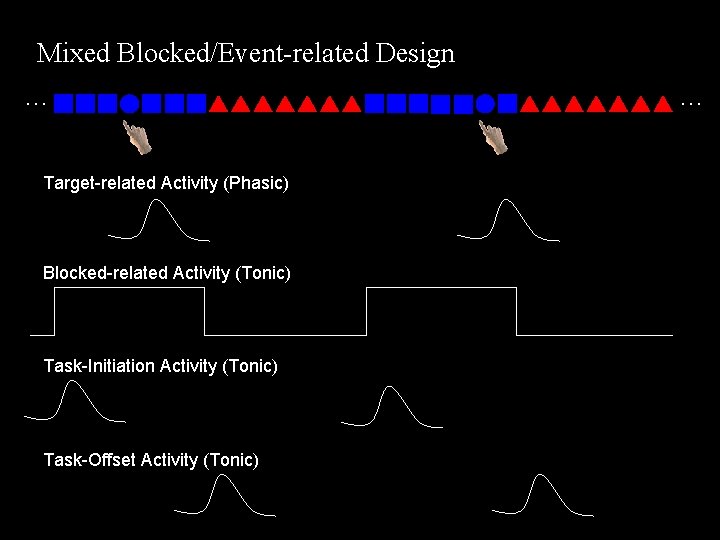
Mixed Blocked/Event-related Design … Target-related Activity (Phasic) Blocked-related Activity (Tonic) Task-Initiation Activity (Tonic) Task-Offset Activity (Tonic) …
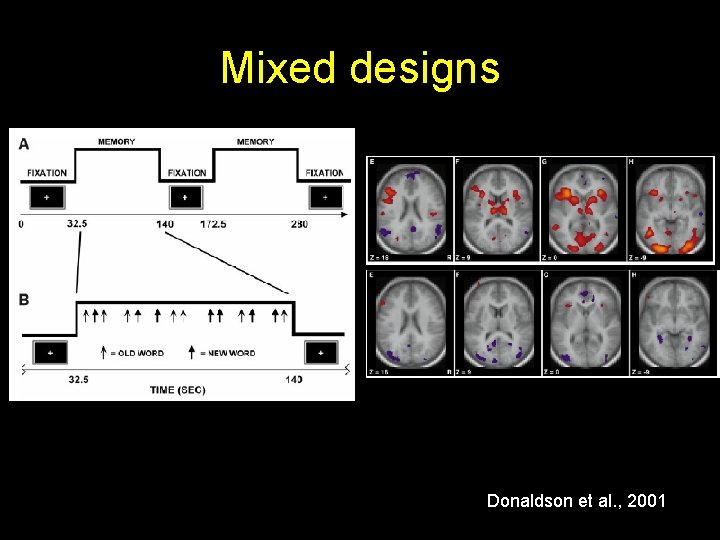
Mixed designs Donaldson et al. , 2001
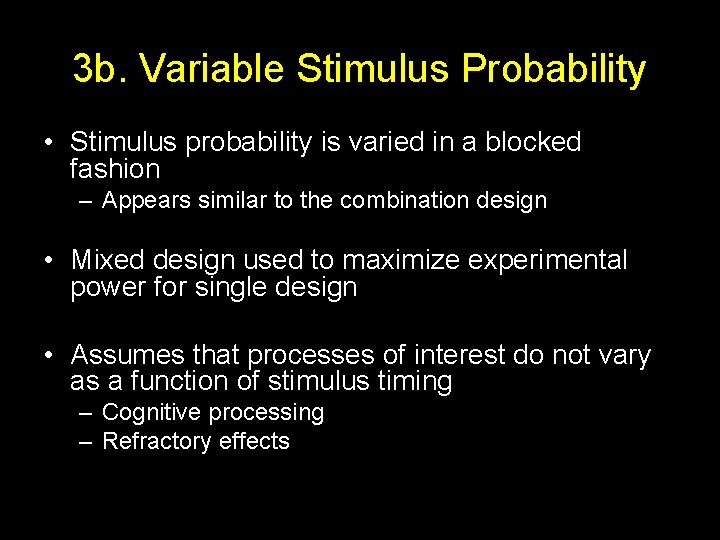
3 b. Variable Stimulus Probability • Stimulus probability is varied in a blocked fashion – Appears similar to the combination design • Mixed design used to maximize experimental power for single design • Assumes that processes of interest do not vary as a function of stimulus timing – Cognitive processing – Refractory effects
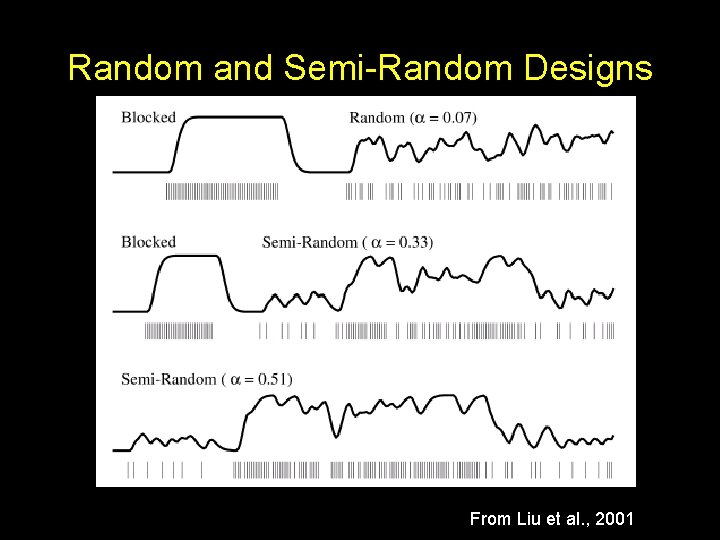
Random and Semi-Random Designs From Liu et al. , 2001
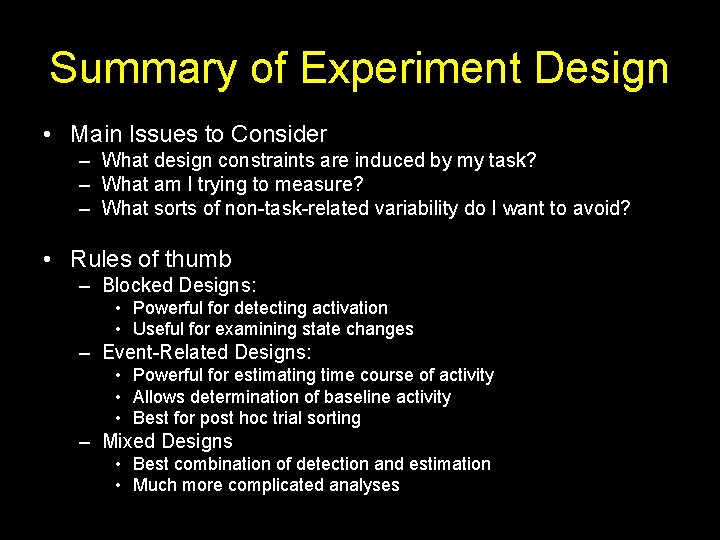
Summary of Experiment Design • Main Issues to Consider – What design constraints are induced by my task? – What am I trying to measure? – What sorts of non-task-related variability do I want to avoid? • Rules of thumb – Blocked Designs: • Powerful for detecting activation • Useful for examining state changes – Event-Related Designs: • Powerful for estimating time course of activity • Allows determination of baseline activity • Best for post hoc trial sorting – Mixed Designs • Best combination of detection and estimation • Much more complicated analyses
Data integration in data preprocessing
Entity identification problem in data integration
Afni fmri
Resting fmri
Huettel fmri
Fsl course data
Afni fmri
Outlier
Sequential feature selection
Data pretreatment
Neural network data preprocessing
Major tasks in data preprocessing
Text preprocessing steps
Document preprocessing
Finite element example
Image preprocessing
Preprocessing fem
Preprocessing in image processing
Password hashing and preprocessing
Password hashing and preprocessing
Dti preprocessing
Mri surf2surf
Mri indications gp
Ax t2 propeller mri
Mri image formation
Buford complex
How mri works
Mri hydrogen atoms
Frequency
Translate
Safetymri
Head of caudate blood supply
Mri energy consumption
Haghighat mri center
Nomics mri
Pregnancy mri
Mamdouh mahfouz
First mri image 1973
Mri hydrogen atoms
Gracilis muscle mri
Lesion ap psychology
First mri image 1973
Vertical mri
Pons radiology
Entry slice phenomenon mri
Foramen rotundum ct
Mri k space
Brainstem ct anatomy
Angular momentum mri
Atri meaning
Closterphibic
Scan image to text
Jt spencer death
Knee mri medicare
Disadvantage of mri
Koronala
Mri scanner
Fgatir ge mri
Mri question
Cmrr mri
Rakesh rao
Prosztata mr
Nsf mri
Andy rekito
Cnr mri
Cnr mri
Mri brain
Mri safety signage
Turners unwarping
Advances in mri
Mri scoliosis protocol
Mri safety
Homer simpson mri
Mri
Nmv mri
Carasil mri
Mri heat
Geometric distortion mri
Casoni test
Central disc protrusion mri
Dog mri hancock county
Dog mri hancock county
Fat signal mri
Mri heat
Mri position
Mri position
Mri position
Musculoskeletal mri anatomy
Mri brain
Abnormal mri
Slidetodoc.com
Parox fa
Pet/mri
Pituitary venous drainage
Mri baby face
Ct brain labelled
Mri safety training
Corpus callosum anatomy radiology
Absent bow tie sign
How mri works