On the Relation of Computing to the World
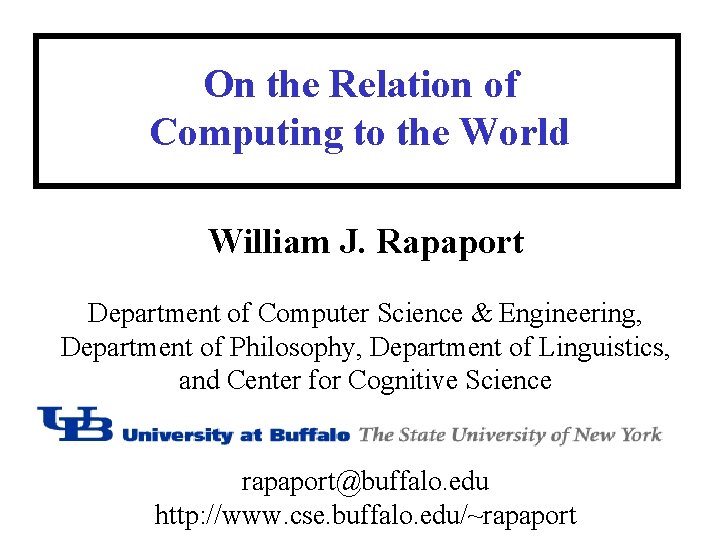
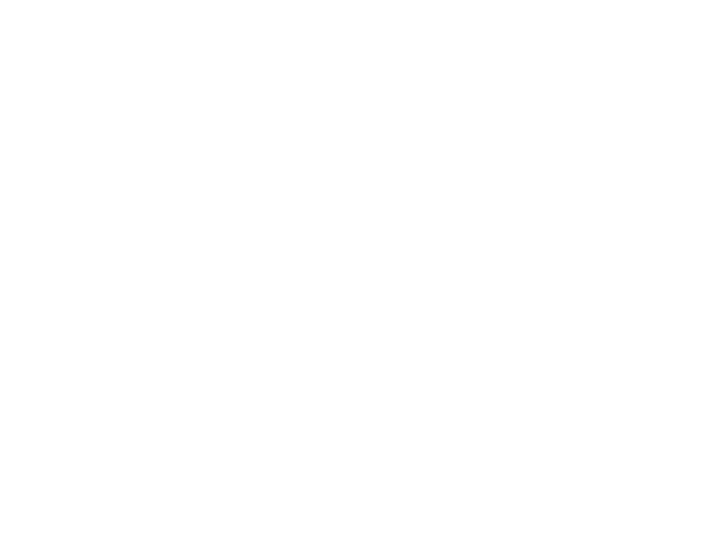
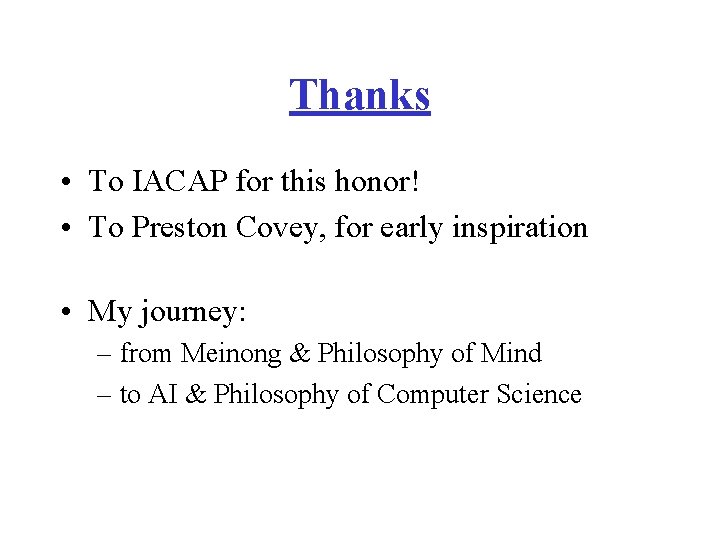
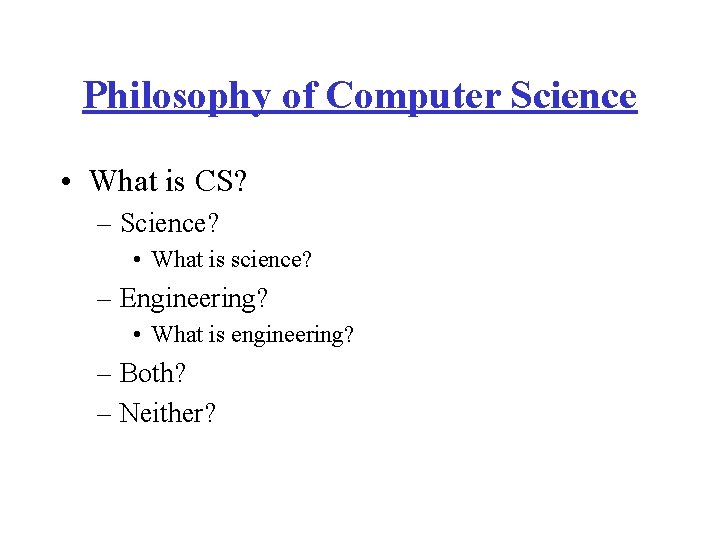
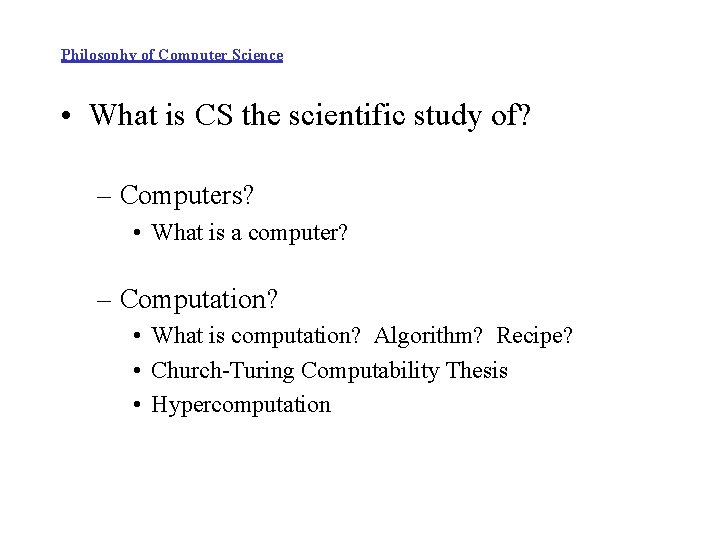
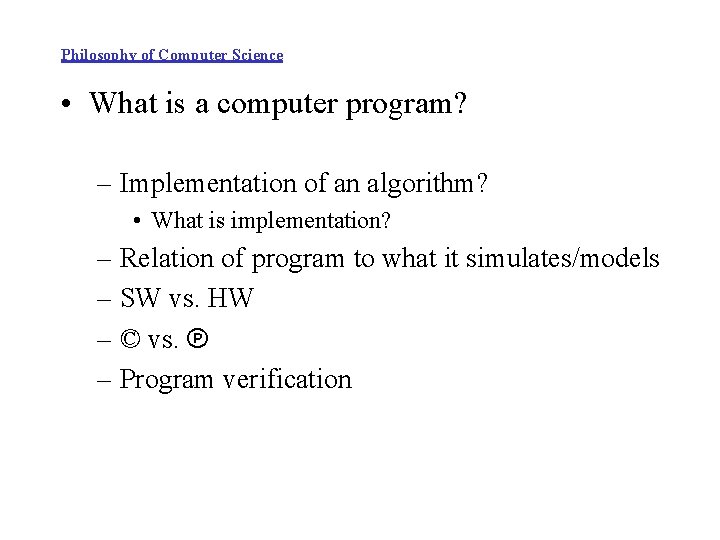
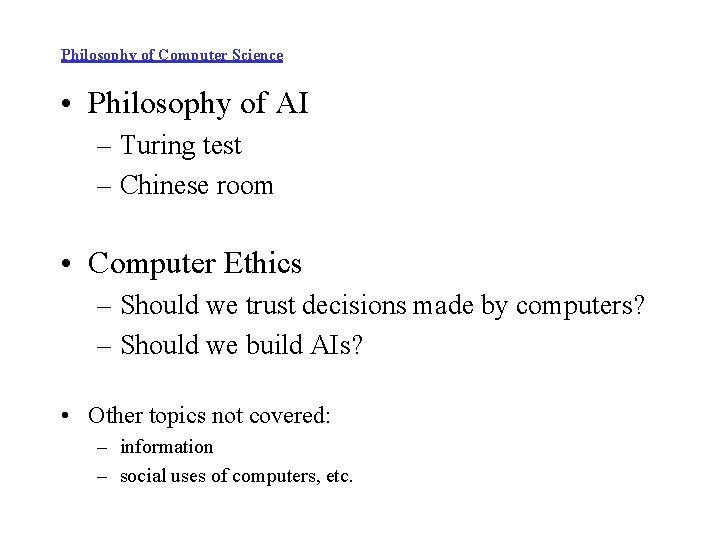
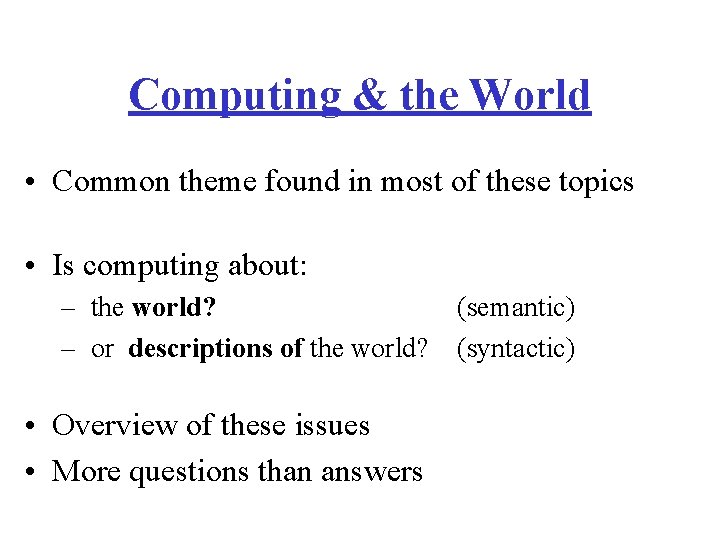
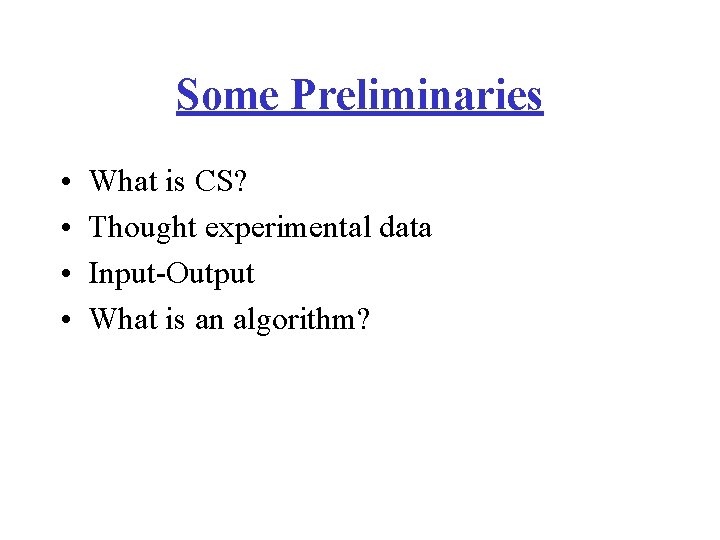
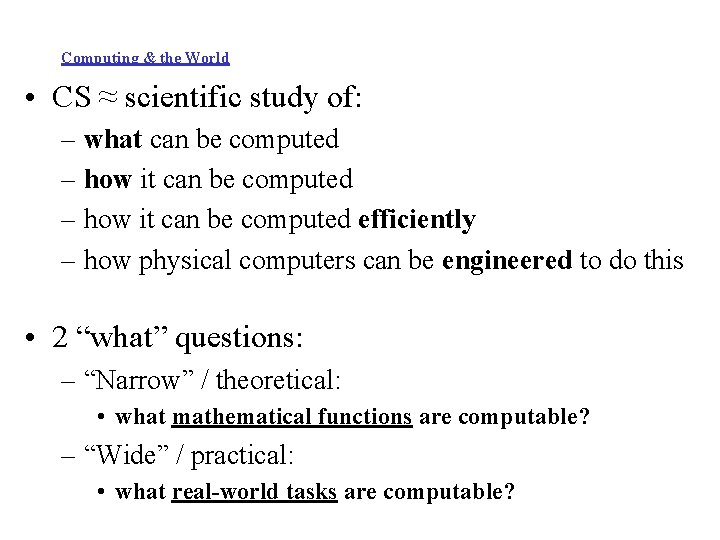
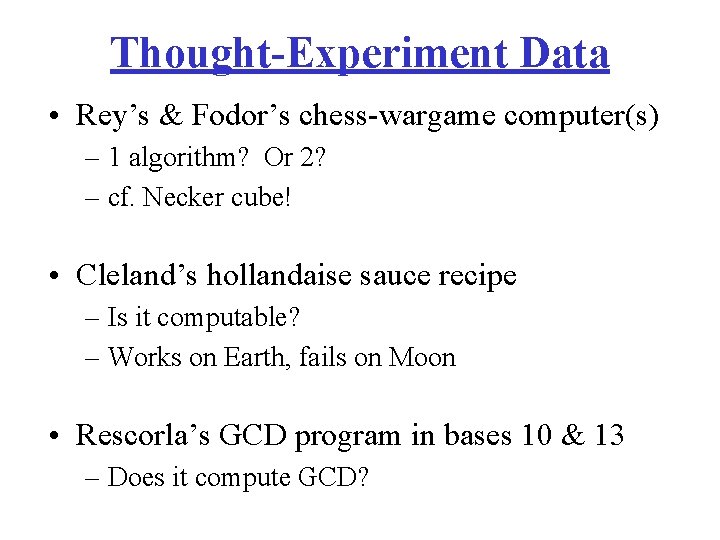
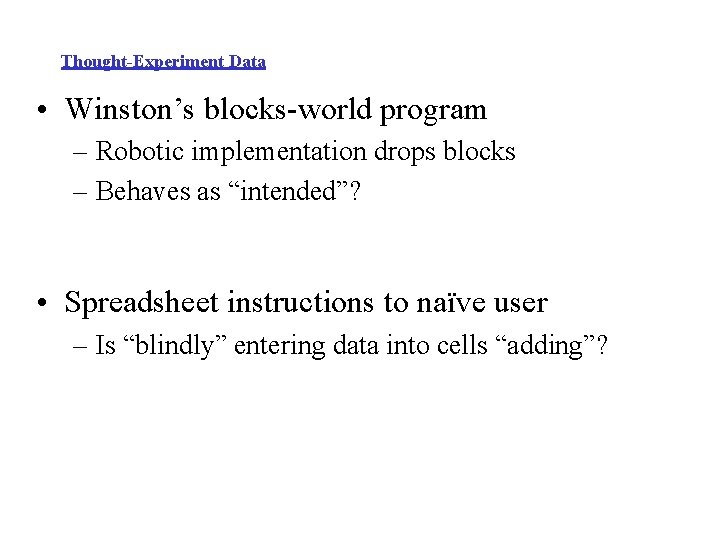
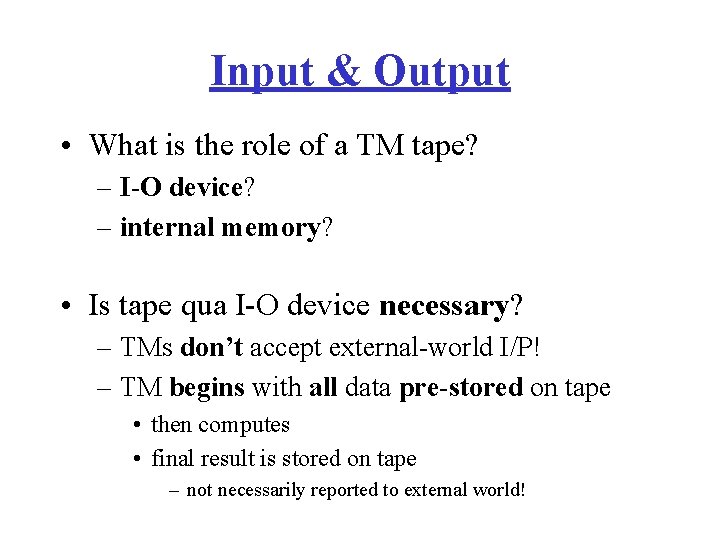
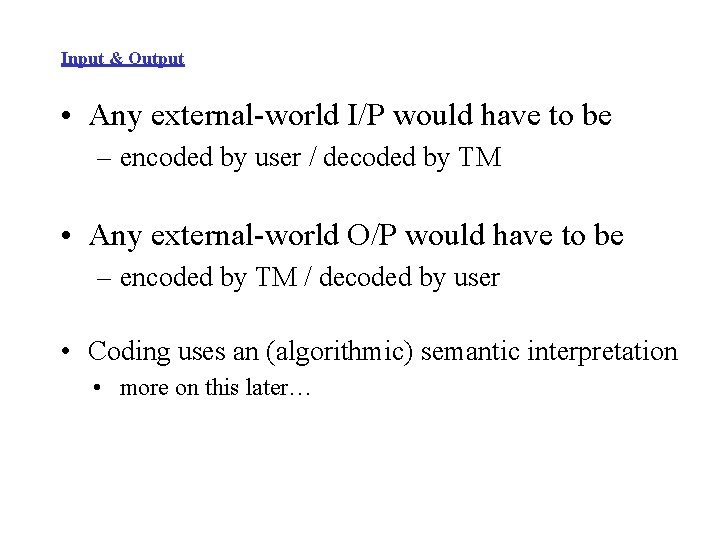
![What Is an Algorithm? • Algorithm (for E) [to accomplish G] is: – a What Is an Algorithm? • Algorithm (for E) [to accomplish G] is: – a](https://slidetodoc.com/presentation_image_h/01c4b80958f54371317578b53b8e5b15/image-15.jpg)
![What Is an Algorithm? • Algorithm (for E) [to accomplish G] is: – a What Is an Algorithm? • Algorithm (for E) [to accomplish G] is: – a](https://slidetodoc.com/presentation_image_h/01c4b80958f54371317578b53b8e5b15/image-16.jpg)
![What Is an Algorithm? • Algorithm (for E) [to accomplish G] is: – a What Is an Algorithm? • Algorithm (for E) [to accomplish G] is: – a](https://slidetodoc.com/presentation_image_h/01c4b80958f54371317578b53b8e5b15/image-17.jpg)
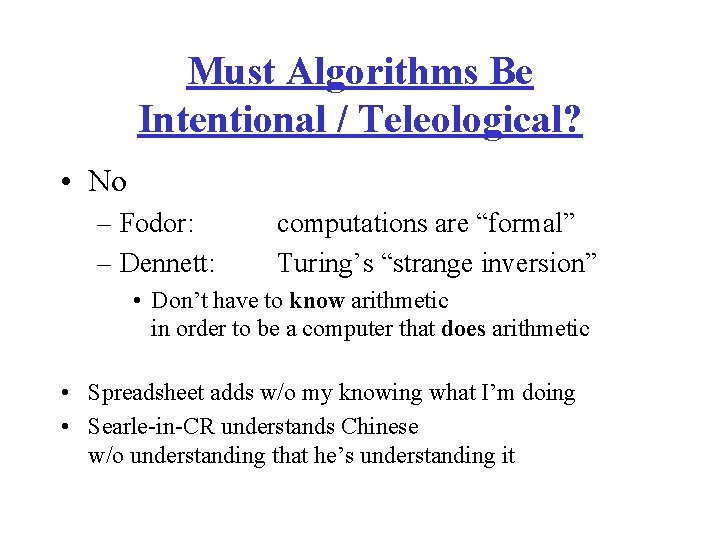
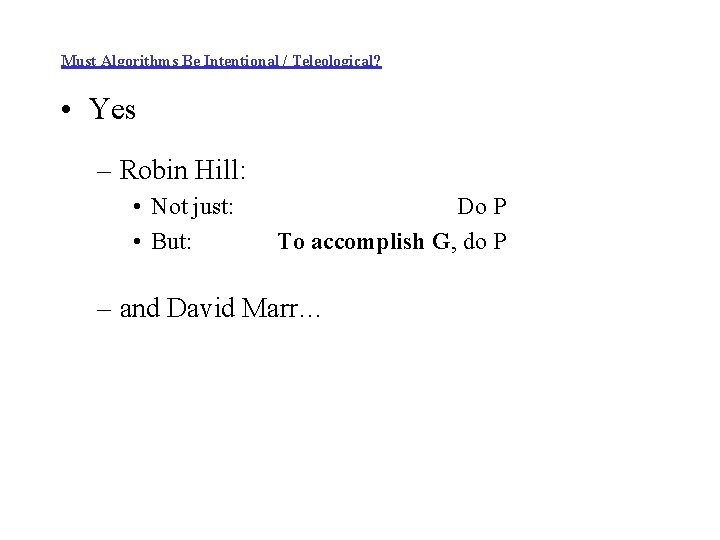
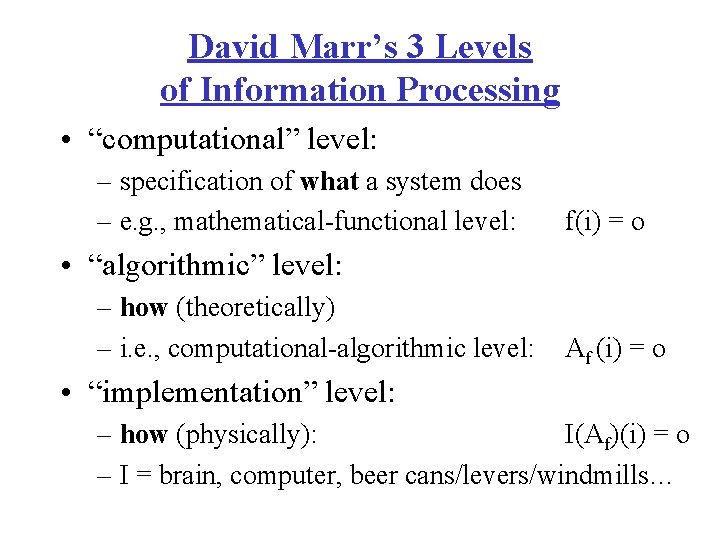
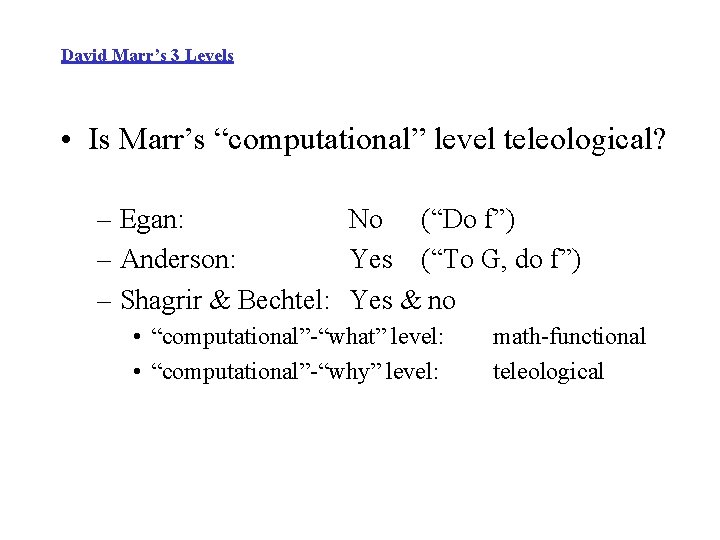
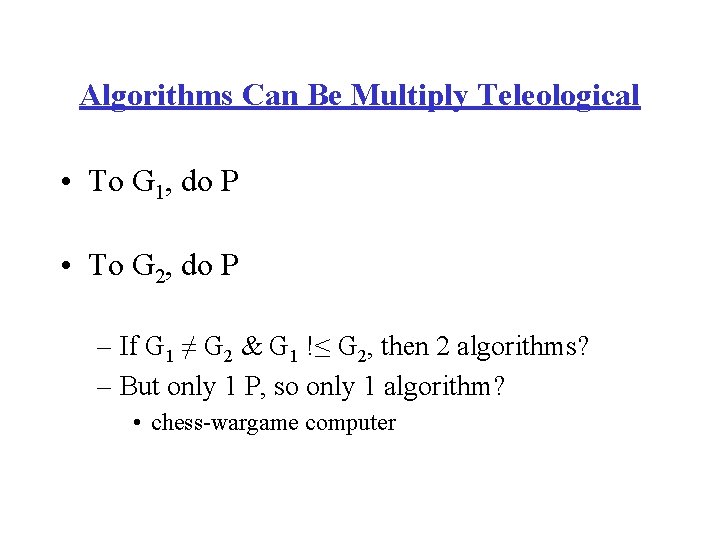
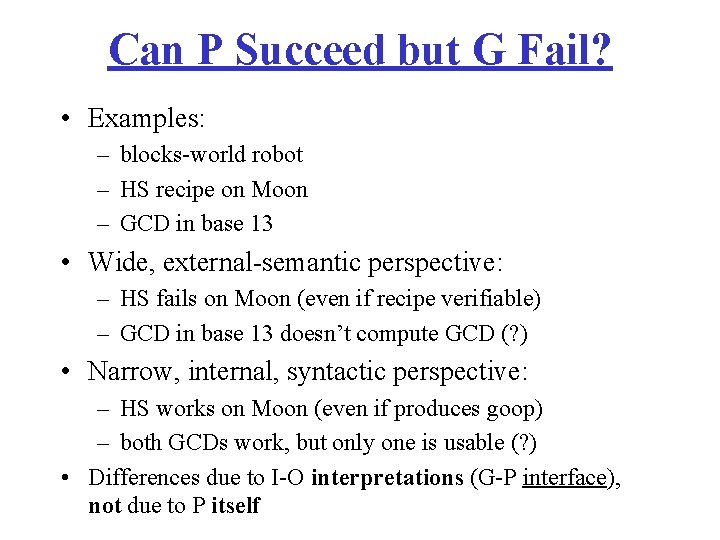
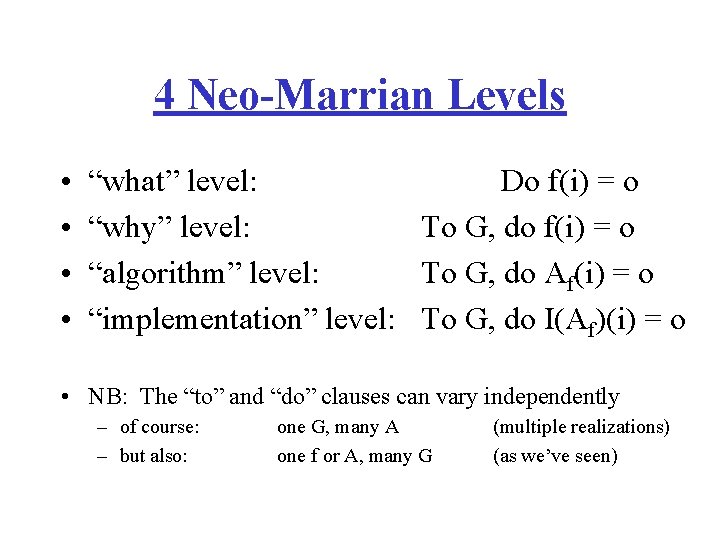
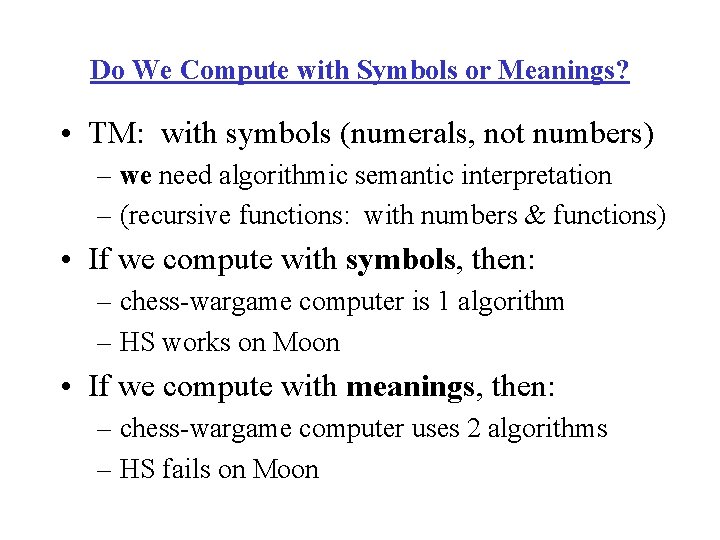
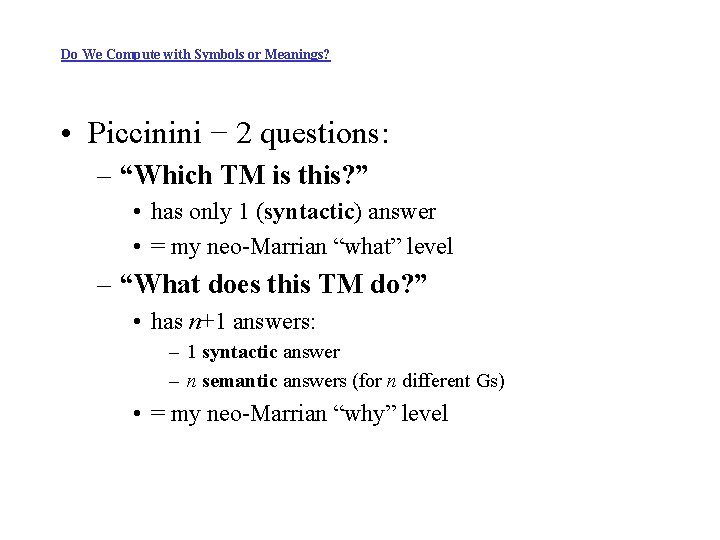
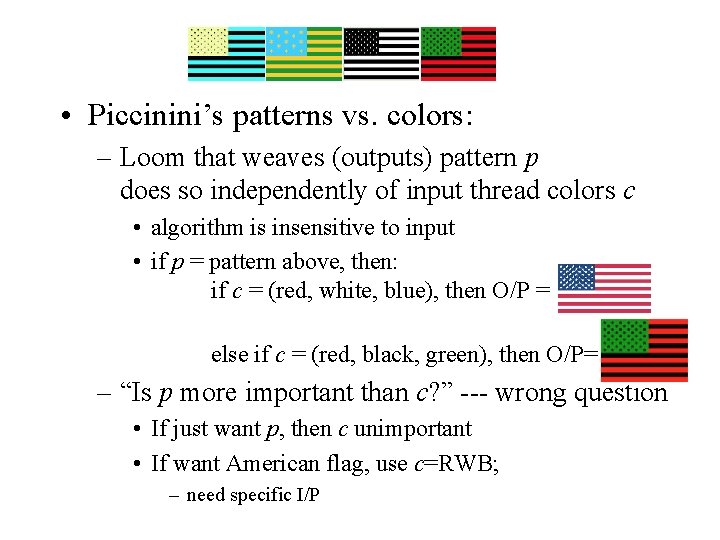
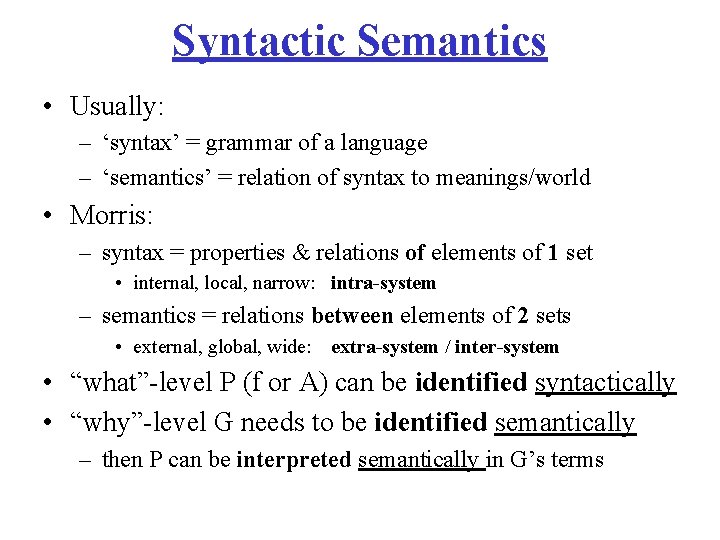
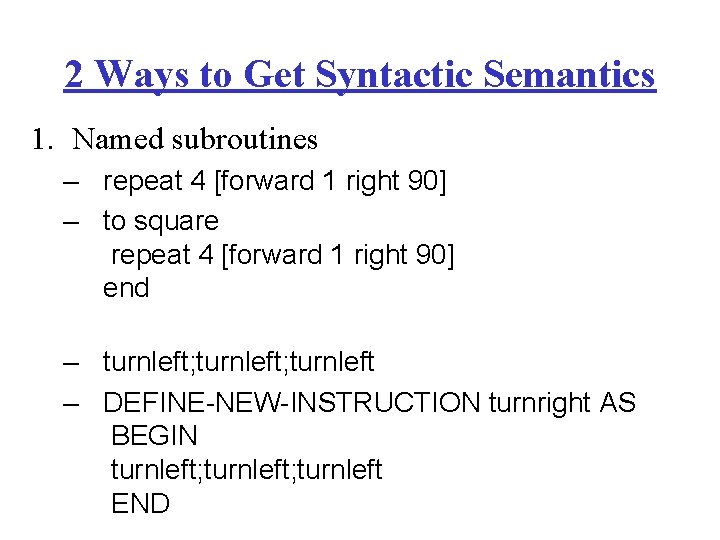
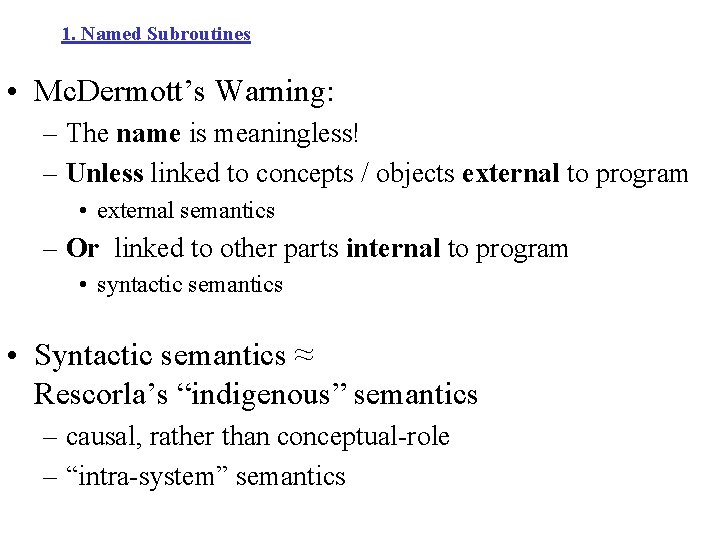
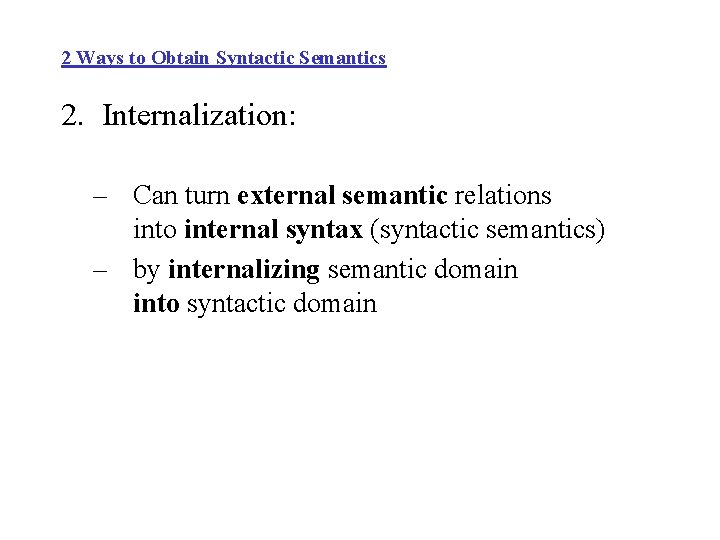
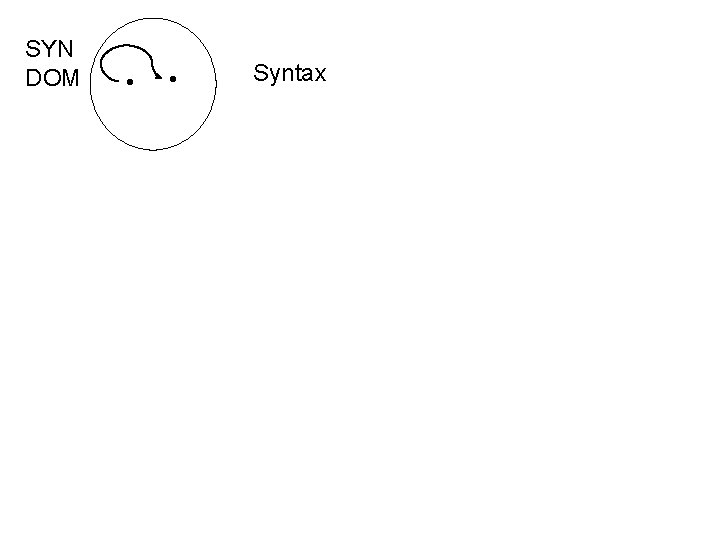
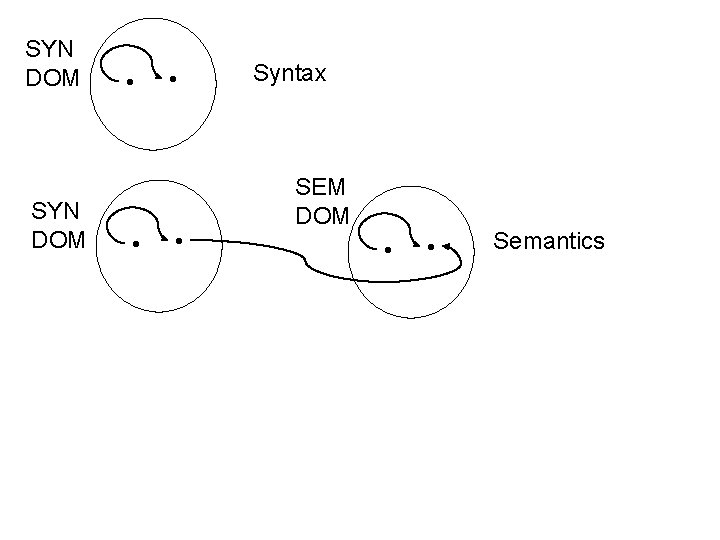
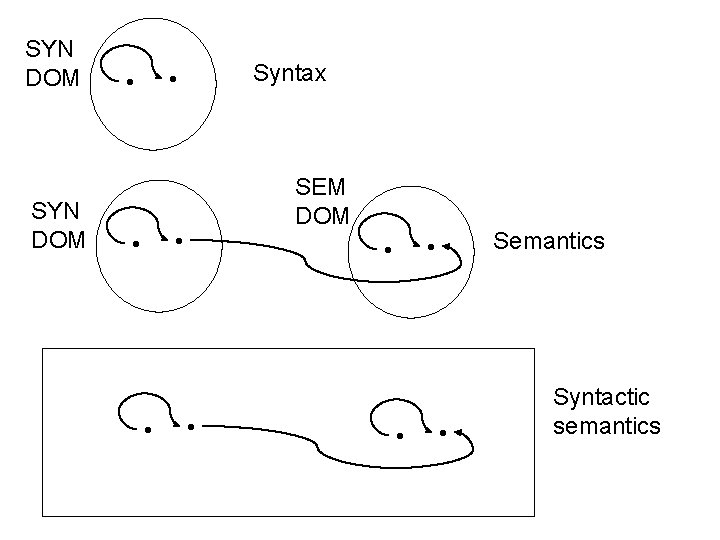
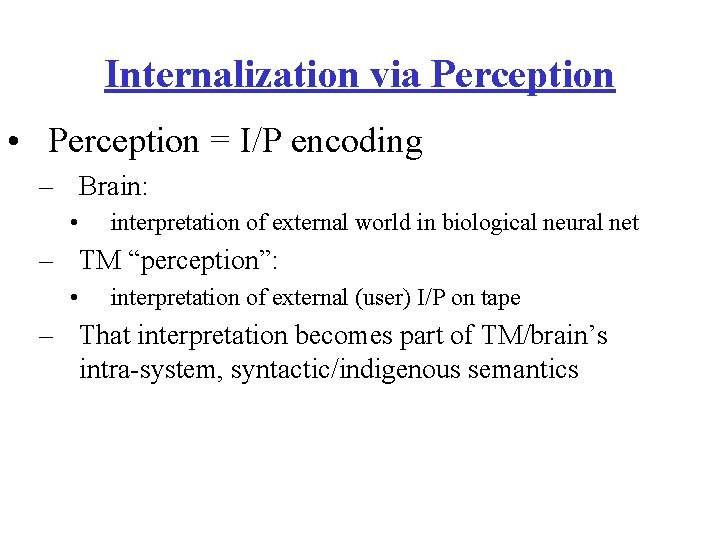
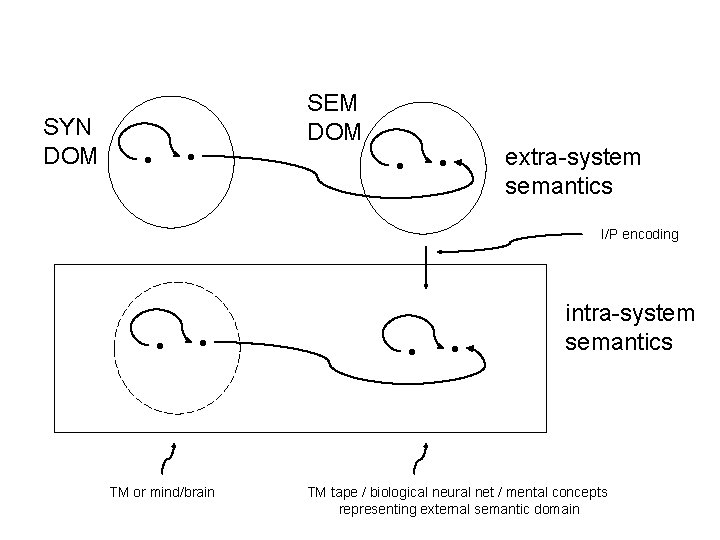
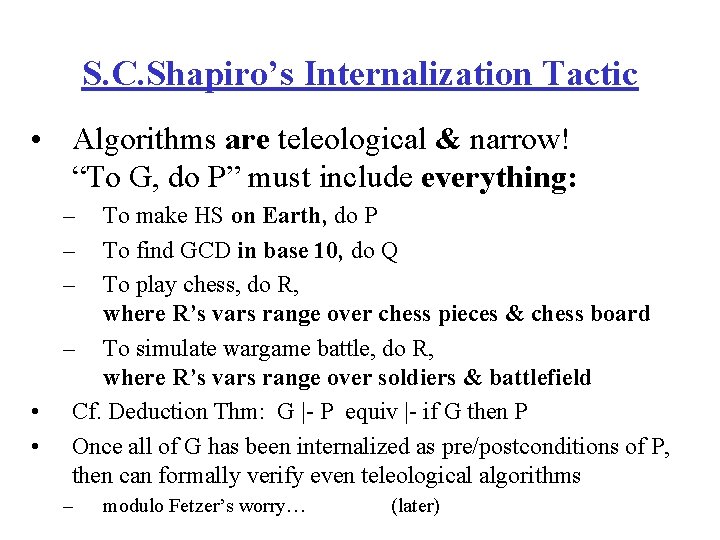
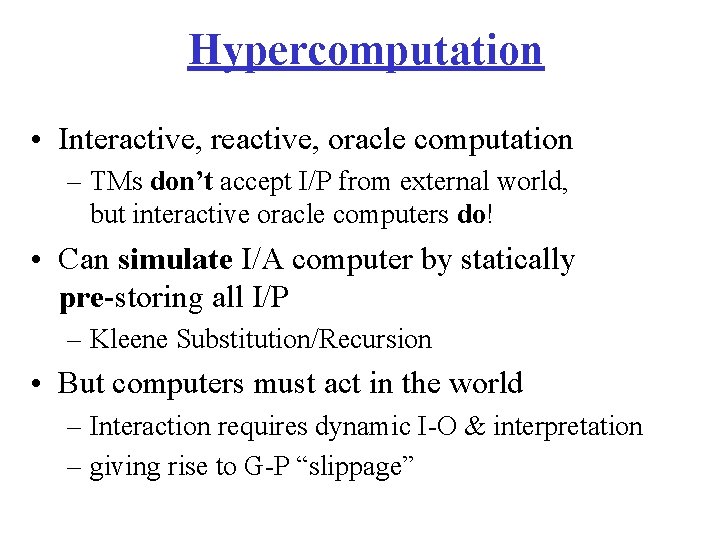
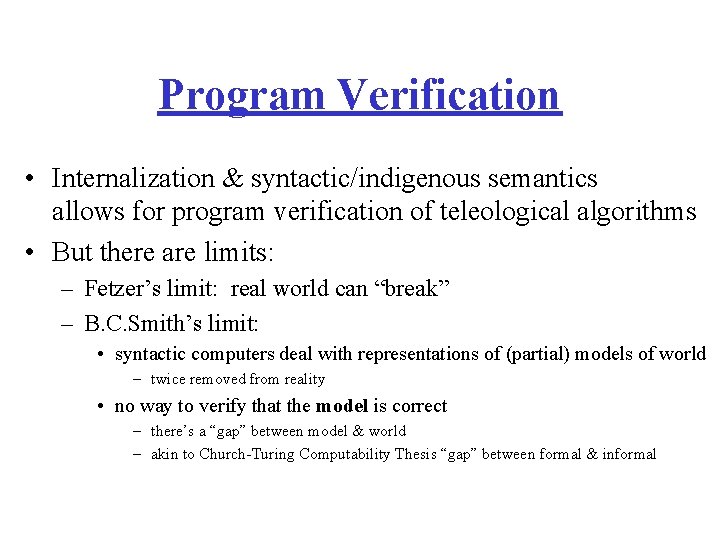
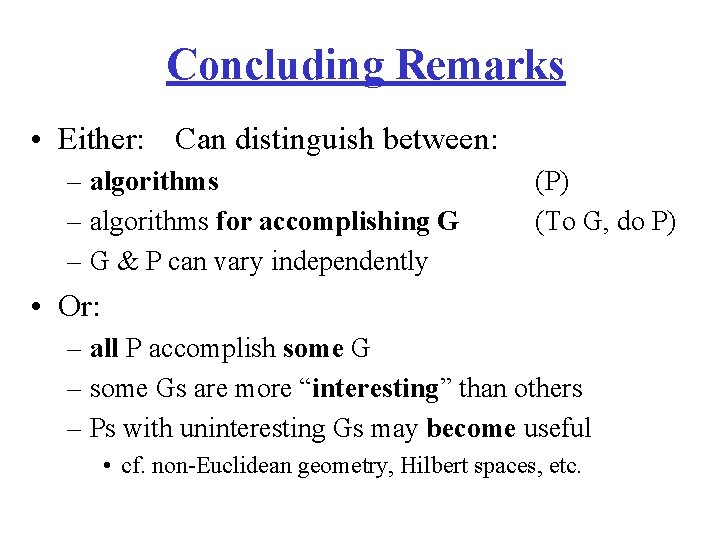
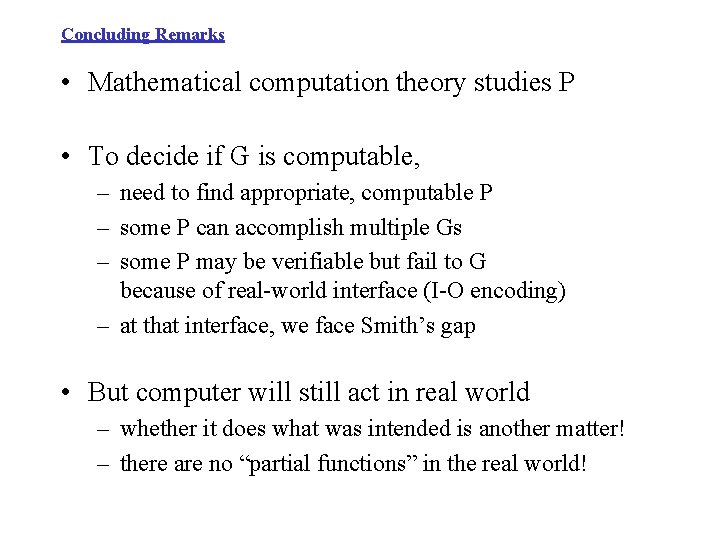
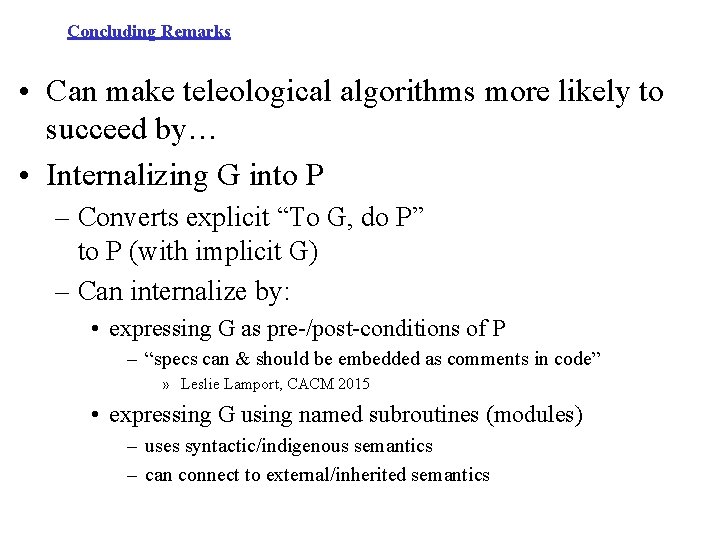
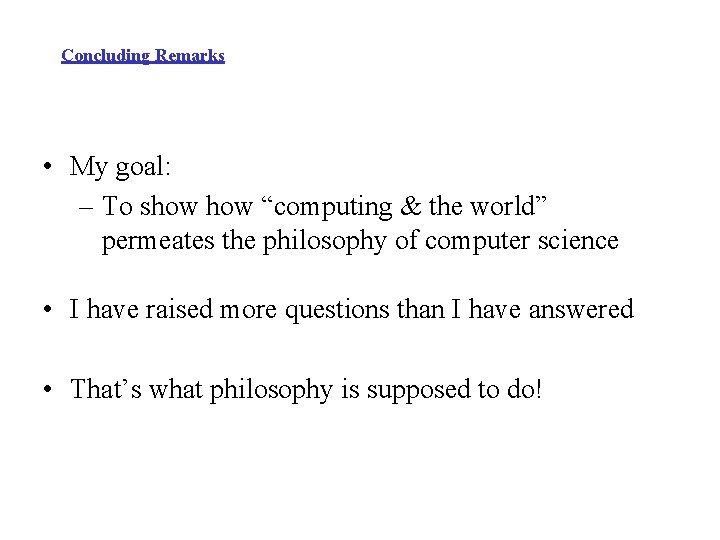
- Slides: 43
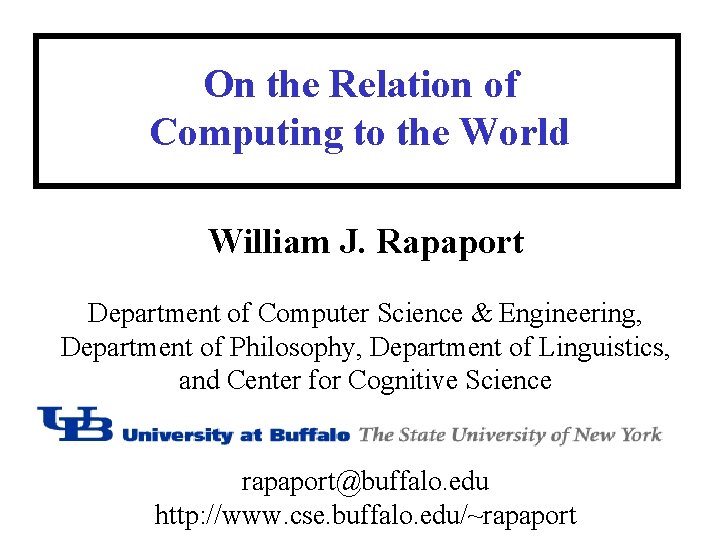
On the Relation of Computing to the World William J. Rapaport Department of Computer Science & Engineering, Department of Philosophy, Department of Linguistics, and Center for Cognitive Science rapaport@buffalo. edu http: //www. cse. buffalo. edu/~rapaport
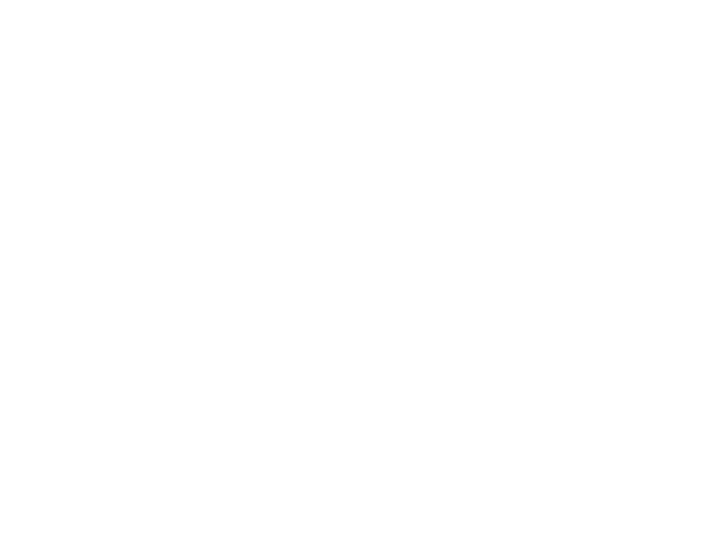
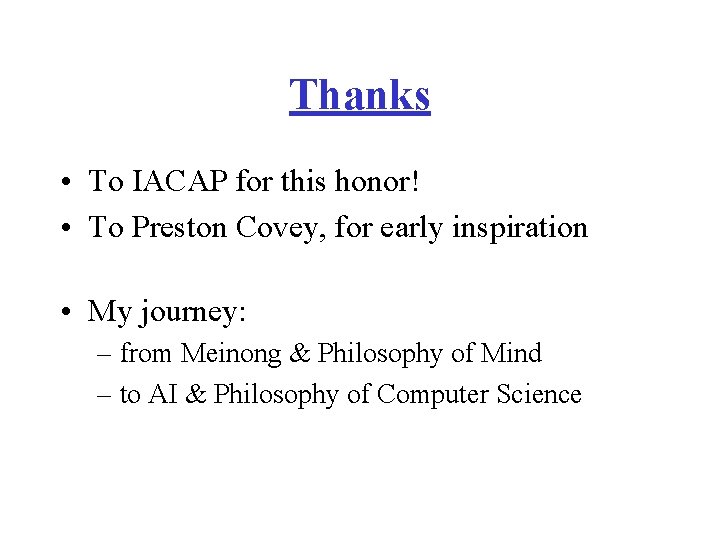
Thanks • To IACAP for this honor! • To Preston Covey, for early inspiration • My journey: – from Meinong & Philosophy of Mind – to AI & Philosophy of Computer Science
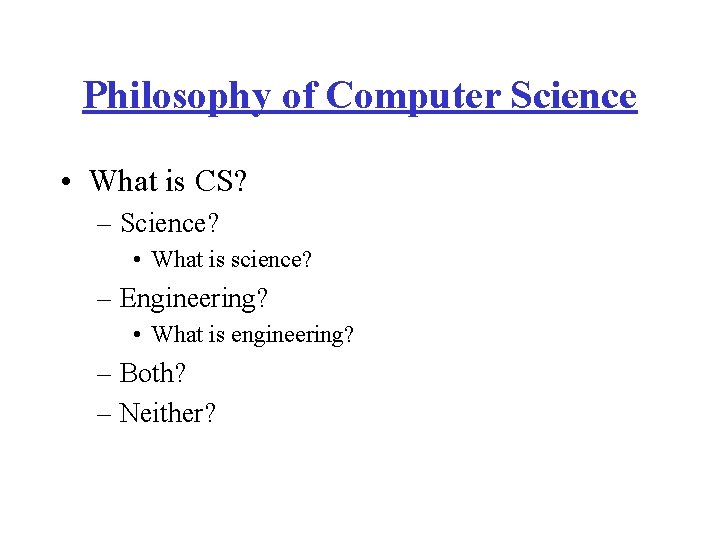
Philosophy of Computer Science • What is CS? – Science? • What is science? – Engineering? • What is engineering? – Both? – Neither?
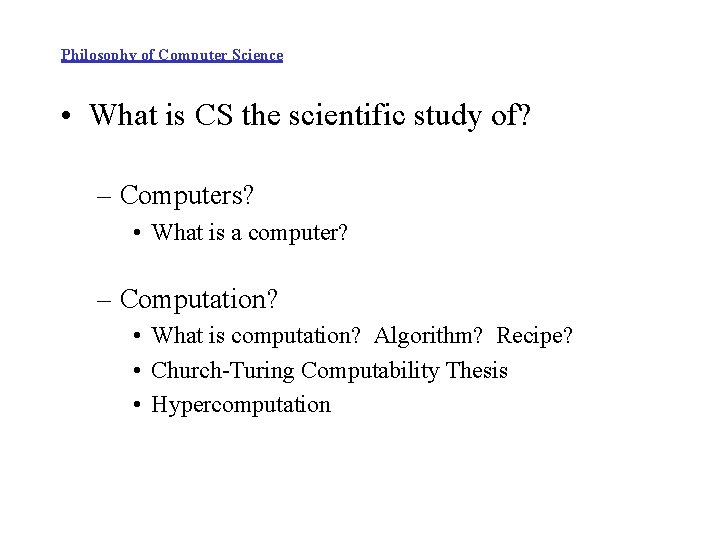
Philosophy of Computer Science • What is CS the scientific study of? – Computers? • What is a computer? – Computation? • What is computation? Algorithm? Recipe? • Church-Turing Computability Thesis • Hypercomputation
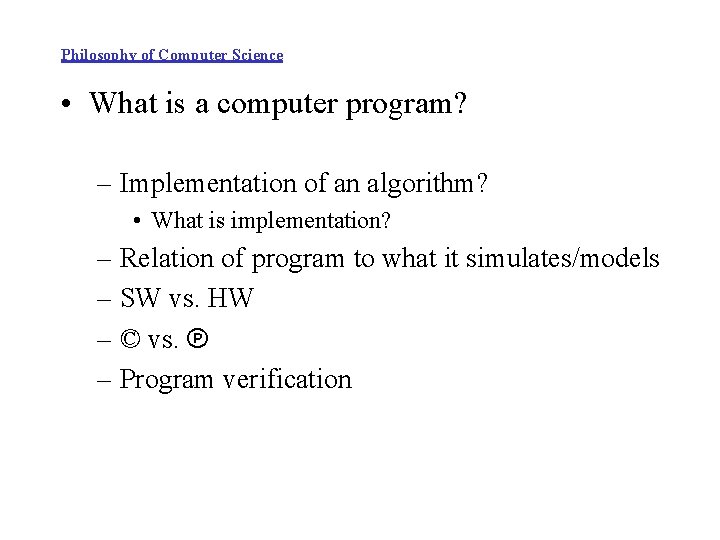
Philosophy of Computer Science • What is a computer program? – Implementation of an algorithm? • What is implementation? – Relation of program to what it simulates/models – SW vs. HW – © vs. ℗ – Program verification
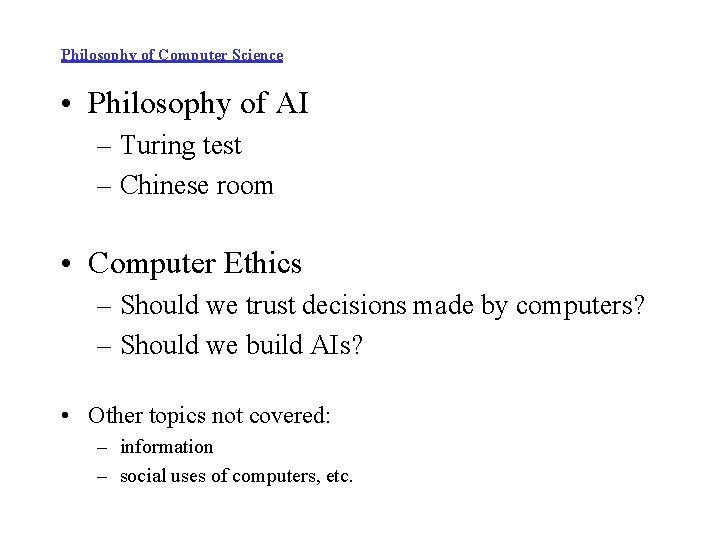
Philosophy of Computer Science • Philosophy of AI – Turing test – Chinese room • Computer Ethics – Should we trust decisions made by computers? – Should we build AIs? • Other topics not covered: – information – social uses of computers, etc.
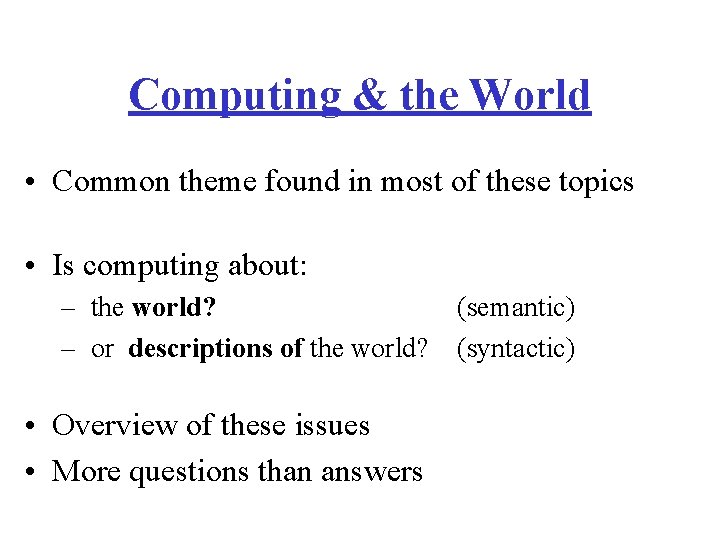
Computing & the World • Common theme found in most of these topics • Is computing about: – the world? (semantic) – or descriptions of the world? (syntactic) • Overview of these issues • More questions than answers
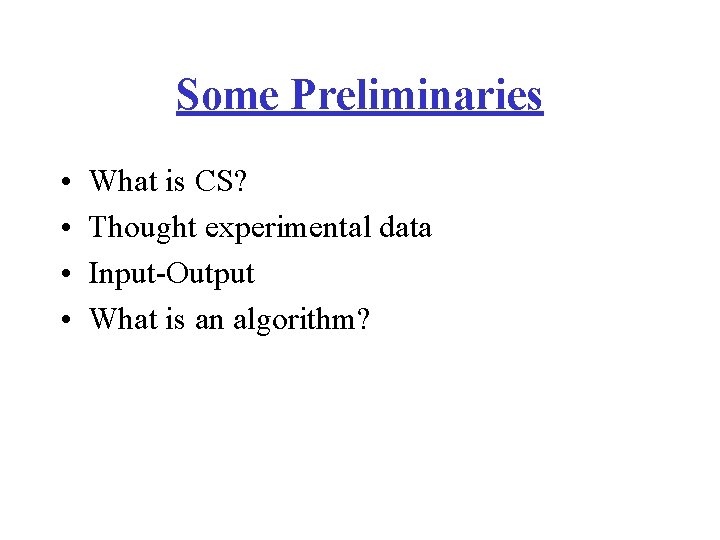
Some Preliminaries • • What is CS? Thought experimental data Input-Output What is an algorithm?
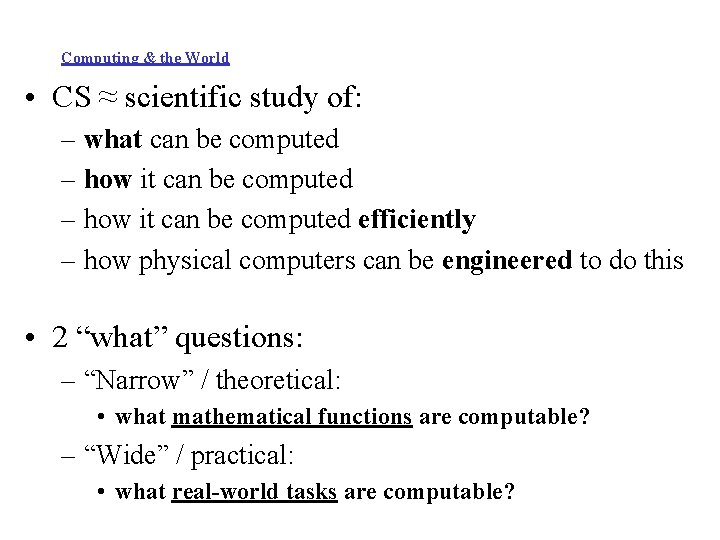
Computing & the World • CS ≈ scientific study of: – what can be computed – how it can be computed efficiently – how physical computers can be engineered to do this • 2 “what” questions: – “Narrow” / theoretical: • what mathematical functions are computable? – “Wide” / practical: • what real-world tasks are computable?
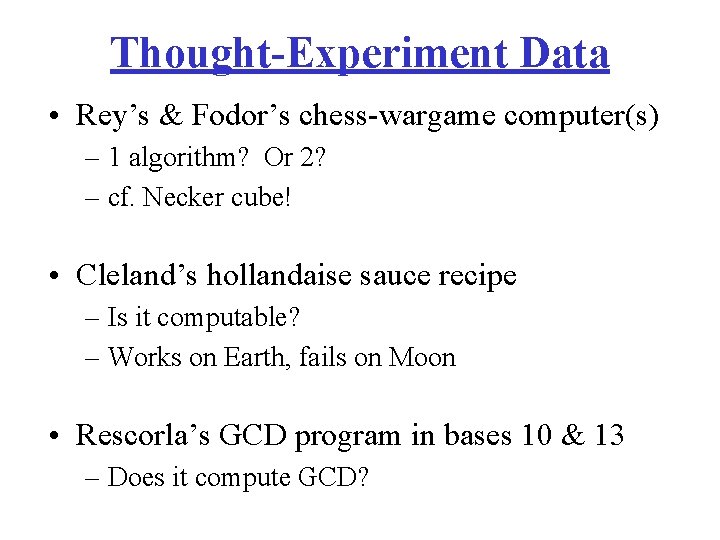
Thought-Experiment Data • Rey’s & Fodor’s chess-wargame computer(s) – 1 algorithm? Or 2? – cf. Necker cube! • Cleland’s hollandaise sauce recipe – Is it computable? – Works on Earth, fails on Moon • Rescorla’s GCD program in bases 10 & 13 – Does it compute GCD?
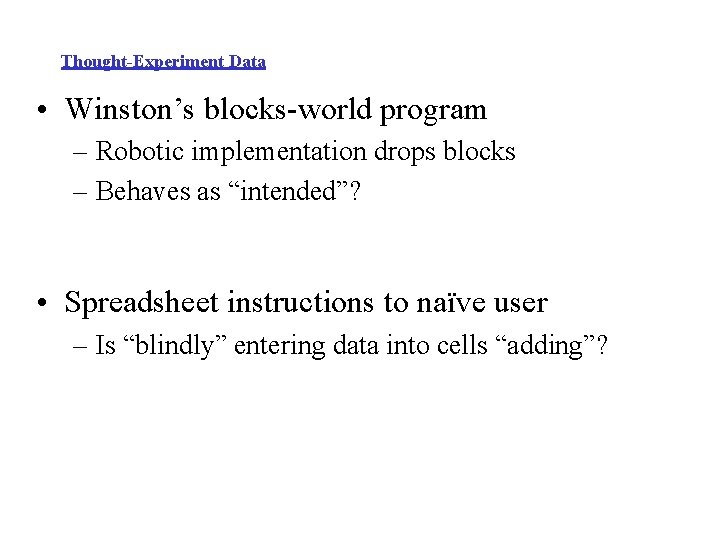
Thought-Experiment Data • Winston’s blocks-world program – Robotic implementation drops blocks – Behaves as “intended”? • Spreadsheet instructions to naïve user – Is “blindly” entering data into cells “adding”?
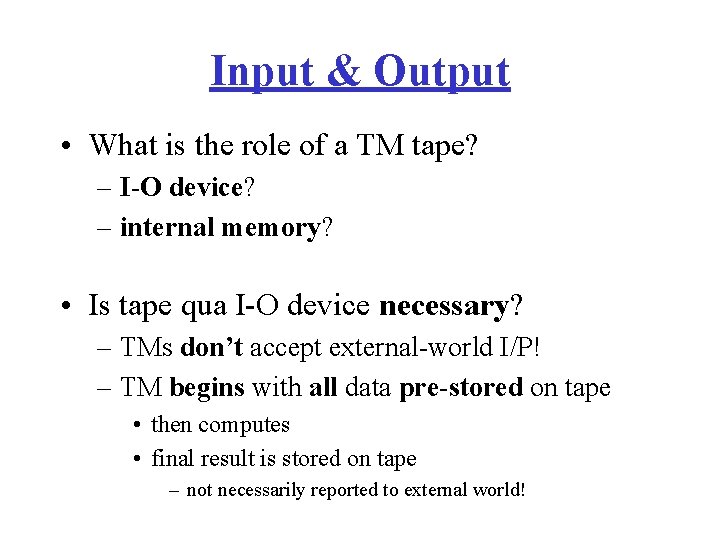
Input & Output • What is the role of a TM tape? – I-O device? – internal memory? • Is tape qua I-O device necessary? – TMs don’t accept external-world I/P! – TM begins with all data pre-stored on tape • then computes • final result is stored on tape – not necessarily reported to external world!
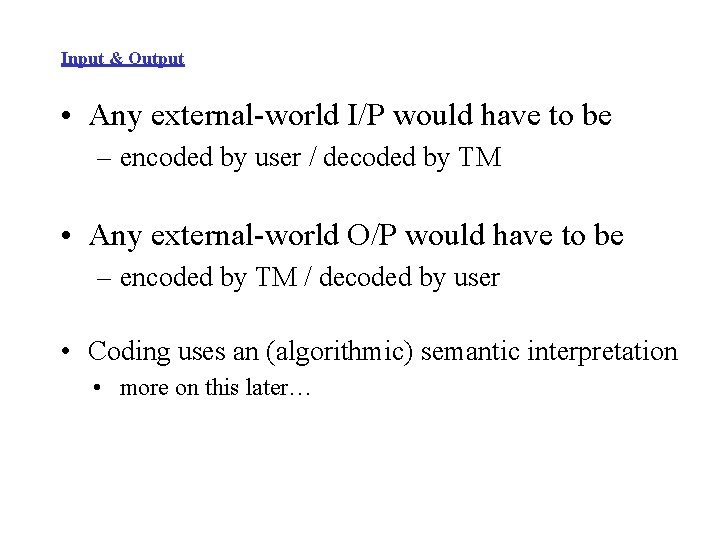
Input & Output • Any external-world I/P would have to be – encoded by user / decoded by TM • Any external-world O/P would have to be – encoded by TM / decoded by user • Coding uses an (algorithmic) semantic interpretation • more on this later…
![What Is an Algorithm Algorithm for E to accomplish G is a What Is an Algorithm? • Algorithm (for E) [to accomplish G] is: – a](https://slidetodoc.com/presentation_image_h/01c4b80958f54371317578b53b8e5b15/image-15.jpg)
What Is an Algorithm? • Algorithm (for E) [to accomplish G] is: – a procedure P • finite set of statements such that each S is: – composed of finite # of “symbols” from finite alphabet – unambiguous (for E) » (E “knows how” to do S » E can do S » S can be done in finite time » after doing S, E “knows” what to do next) – P takes finite time – [P ends with G accomplished]
![What Is an Algorithm Algorithm for E to accomplish G is a What Is an Algorithm? • Algorithm (for E) [to accomplish G] is: – a](https://slidetodoc.com/presentation_image_h/01c4b80958f54371317578b53b8e5b15/image-16.jpg)
What Is an Algorithm? • Algorithm (for E) [to accomplish G] is: – a procedure P • finite set of statements such that each S is: – composed of finite # of “symbols” from finite alphabet – unambiguous (for E) » (E “knows how” to do S » E can do S » S can be done in finite time » after doing S, E “knows” what to do next) – P takes finite time – [P ends with G accomplished]
![What Is an Algorithm Algorithm for E to accomplish G is a What Is an Algorithm? • Algorithm (for E) [to accomplish G] is: – a](https://slidetodoc.com/presentation_image_h/01c4b80958f54371317578b53b8e5b15/image-17.jpg)
What Is an Algorithm? • Algorithm (for E) [to accomplish G] is: – a procedure P • finite set of statements such that each S is: – composed of finite # of “symbols” from finite alphabet – unambiguous (for E) » (E “knows how” to do S » E can do S » S can be done in finite time » after doing S, E “knows” what to do next) – P takes finite time – [P ends with G accomplished]
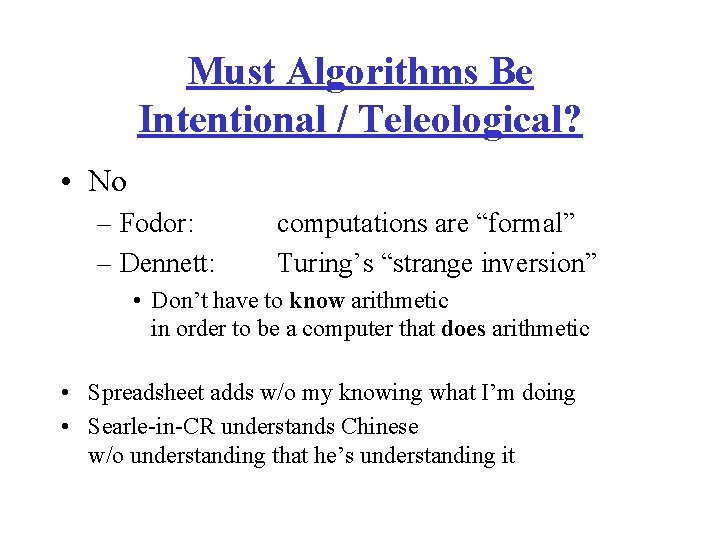
Must Algorithms Be Intentional / Teleological? • No – Fodor: – Dennett: computations are “formal” Turing’s “strange inversion” • Don’t have to know arithmetic in order to be a computer that does arithmetic • Spreadsheet adds w/o my knowing what I’m doing • Searle-in-CR understands Chinese w/o understanding that he’s understanding it
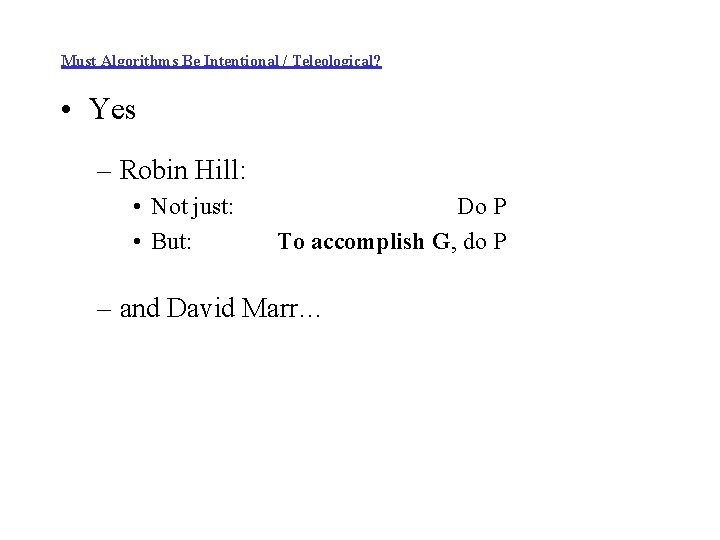
Must Algorithms Be Intentional / Teleological? • Yes – Robin Hill: • Not just: • But: Do P To accomplish G, do P – and David Marr…
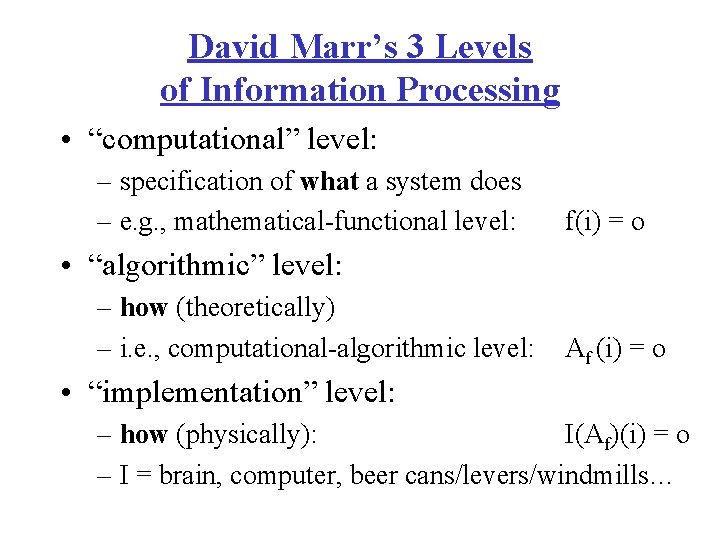
David Marr’s 3 Levels of Information Processing • “computational” level: – specification of what a system does – e. g. , mathematical-functional level: f(i) = o • “algorithmic” level: – how (theoretically) – i. e. , computational-algorithmic level: Af (i) = o • “implementation” level: – how (physically): I(Af)(i) = o – I = brain, computer, beer cans/levers/windmills…
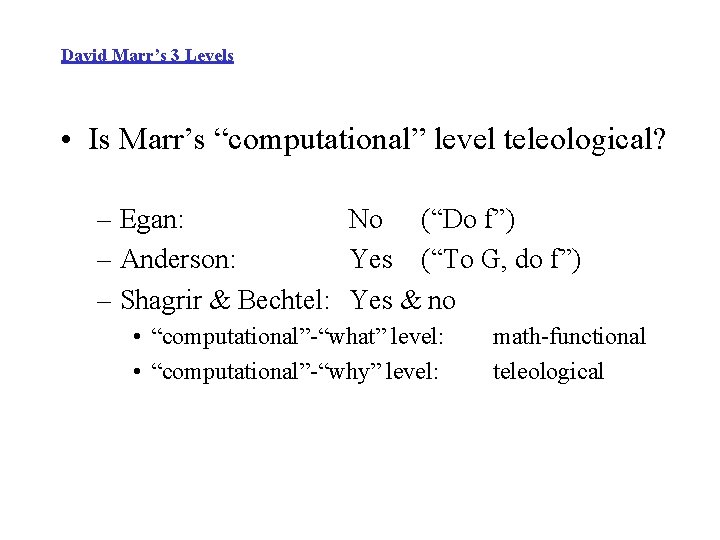
David Marr’s 3 Levels • Is Marr’s “computational” level teleological? – Egan: No (“Do f”) – Anderson: Yes (“To G, do f”) – Shagrir & Bechtel: Yes & no • “computational”-“what” level: • “computational”-“why” level: math-functional teleological
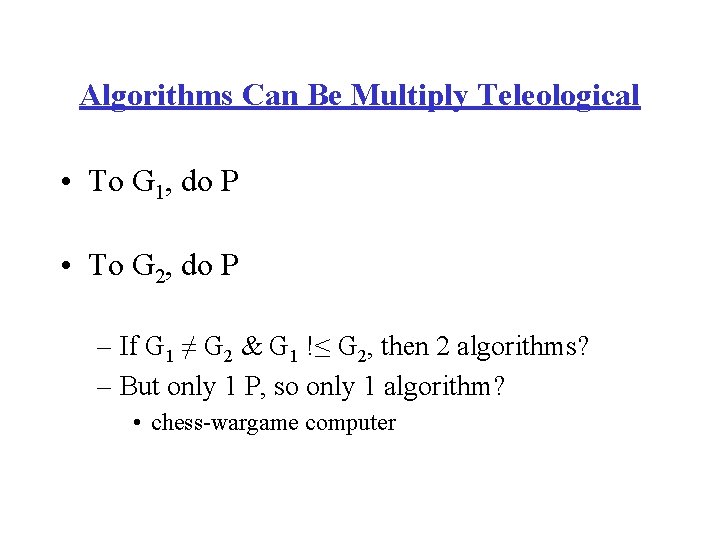
Algorithms Can Be Multiply Teleological • To G 1, do P • To G 2, do P – If G 1 ≠ G 2 & G 1 !≤ G 2, then 2 algorithms? – But only 1 P, so only 1 algorithm? • chess-wargame computer
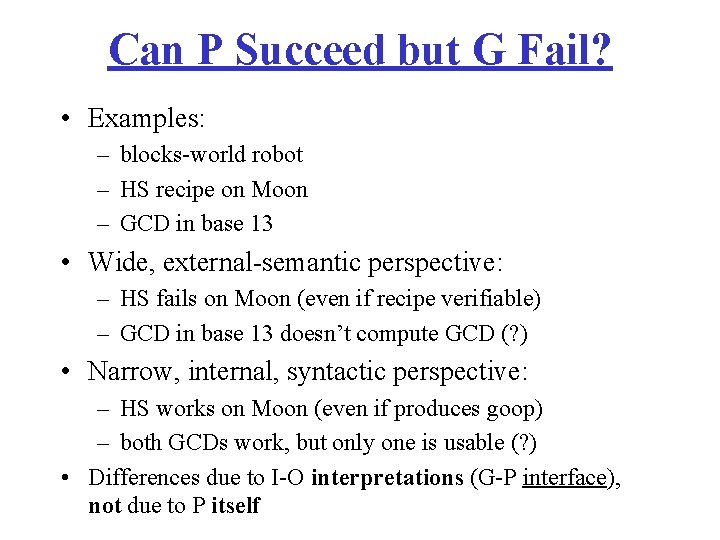
Can P Succeed but G Fail? • Examples: – blocks-world robot – HS recipe on Moon – GCD in base 13 • Wide, external-semantic perspective: – HS fails on Moon (even if recipe verifiable) – GCD in base 13 doesn’t compute GCD (? ) • Narrow, internal, syntactic perspective: – HS works on Moon (even if produces goop) – both GCDs work, but only one is usable (? ) • Differences due to I-O interpretations (G-P interface), not due to P itself
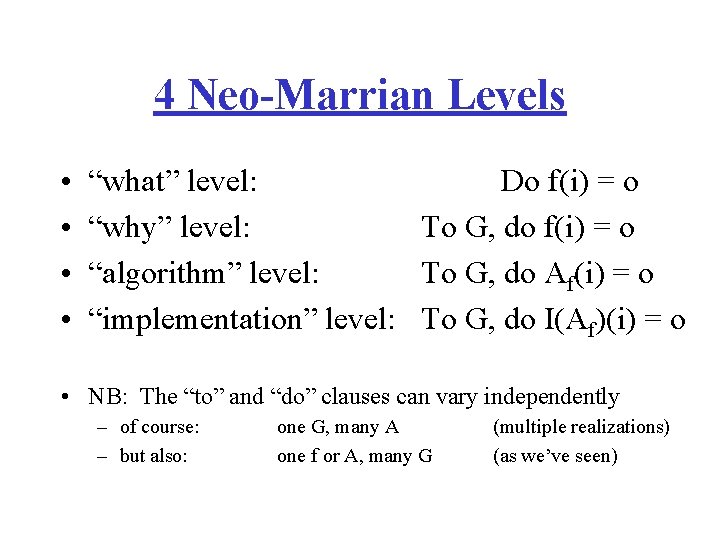
4 Neo-Marrian Levels • • “what” level: Do f(i) = o “why” level: To G, do f(i) = o “algorithm” level: To G, do Af(i) = o “implementation” level: To G, do I(Af)(i) = o • NB: The “to” and “do” clauses can vary independently – of course: – but also: one G, many A one f or A, many G (multiple realizations) (as we’ve seen)
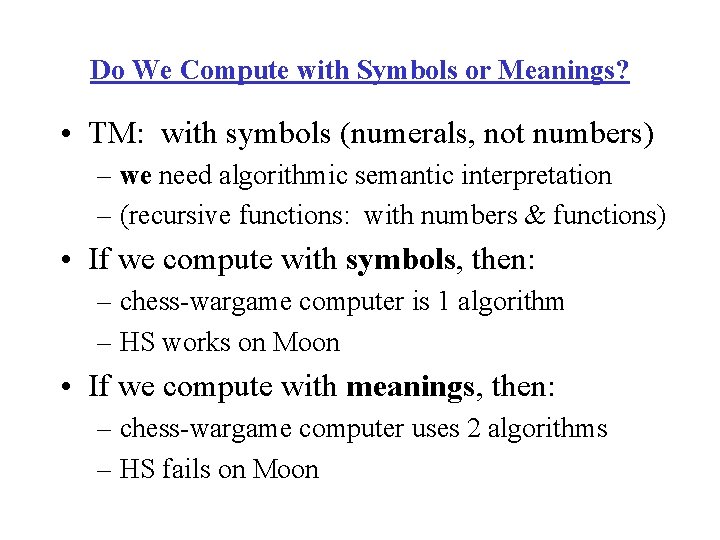
Do We Compute with Symbols or Meanings? • TM: with symbols (numerals, not numbers) – we need algorithmic semantic interpretation – (recursive functions: with numbers & functions) • If we compute with symbols, then: – chess-wargame computer is 1 algorithm – HS works on Moon • If we compute with meanings, then: – chess-wargame computer uses 2 algorithms – HS fails on Moon
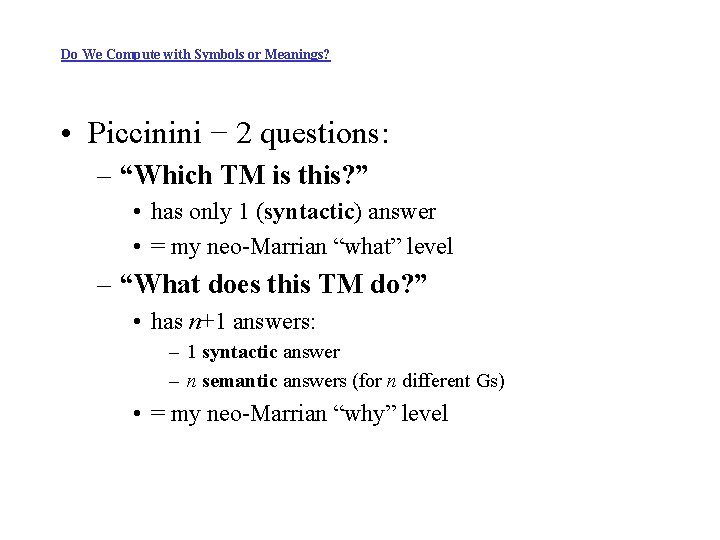
Do We Compute with Symbols or Meanings? • Piccinini − 2 questions: – “Which TM is this? ” • has only 1 (syntactic) answer • = my neo-Marrian “what” level – “What does this TM do? ” • has n+1 answers: – 1 syntactic answer – n semantic answers (for n different Gs) • = my neo-Marrian “why” level
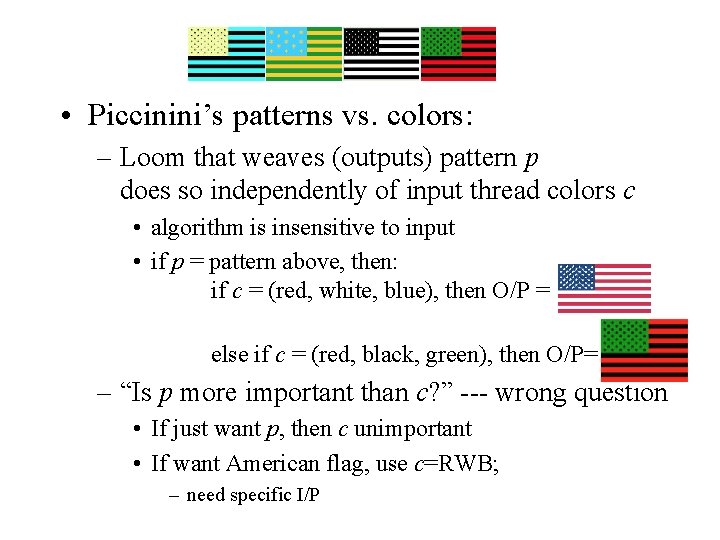
• Piccinini’s patterns vs. colors: – Loom that weaves (outputs) pattern p does so independently of input thread colors c • algorithm is insensitive to input • if p = pattern above, then: if c = (red, white, blue), then O/P = else if c = (red, black, green), then O/P= – “Is p more important than c? ” --- wrong question • If just want p, then c unimportant • If want American flag, use c=RWB; – need specific I/P
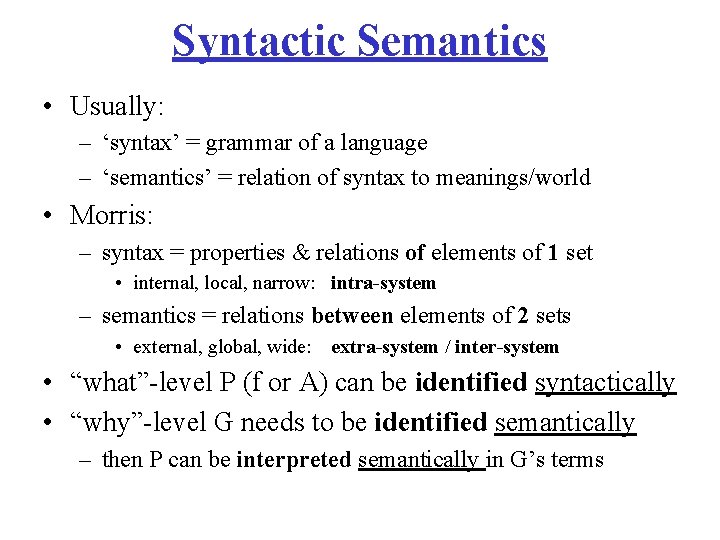
Syntactic Semantics • Usually: – ‘syntax’ = grammar of a language – ‘semantics’ = relation of syntax to meanings/world • Morris: – syntax = properties & relations of elements of 1 set • internal, local, narrow: intra-system – semantics = relations between elements of 2 sets • external, global, wide: extra-system / inter-system • “what”-level P (f or A) can be identified syntactically • “why”-level G needs to be identified semantically – then P can be interpreted semantically in G’s terms
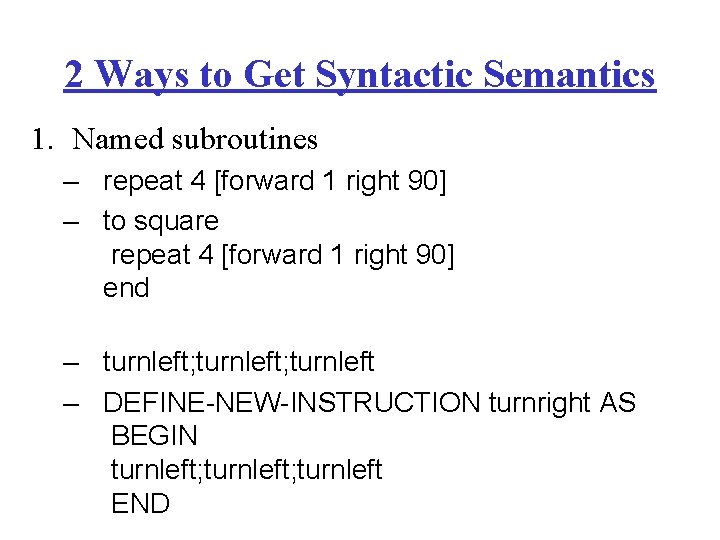
2 Ways to Get Syntactic Semantics 1. Named subroutines – repeat 4 [forward 1 right 90] – to square repeat 4 [forward 1 right 90] end – turnleft; turnleft – DEFINE-NEW-INSTRUCTION turnright AS BEGIN turnleft; turnleft END
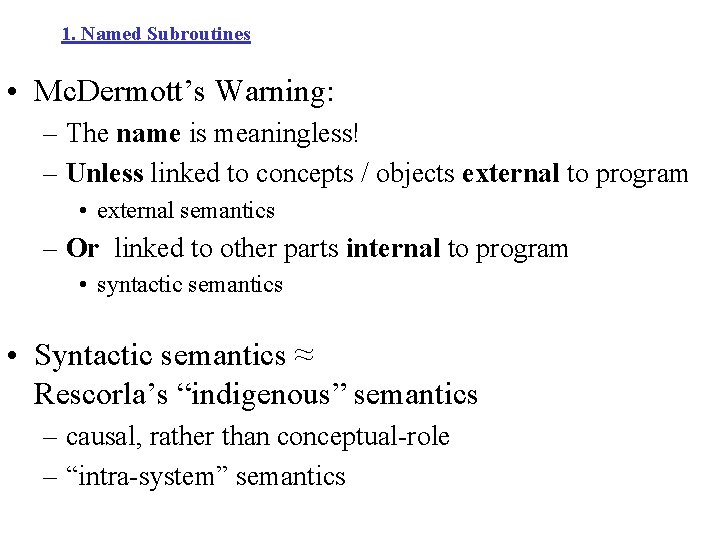
1. Named Subroutines • Mc. Dermott’s Warning: – The name is meaningless! – Unless linked to concepts / objects external to program • external semantics – Or linked to other parts internal to program • syntactic semantics • Syntactic semantics ≈ Rescorla’s “indigenous” semantics – causal, rather than conceptual-role – “intra-system” semantics
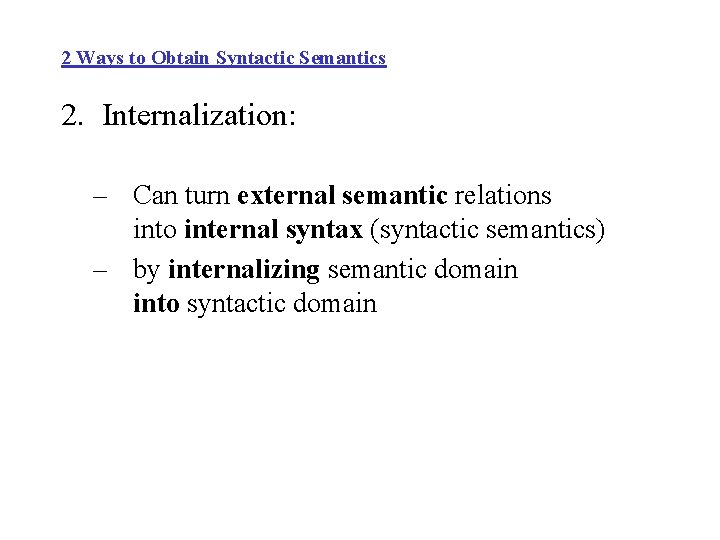
2 Ways to Obtain Syntactic Semantics 2. Internalization: – Can turn external semantic relations into internal syntax (syntactic semantics) – by internalizing semantic domain into syntactic domain
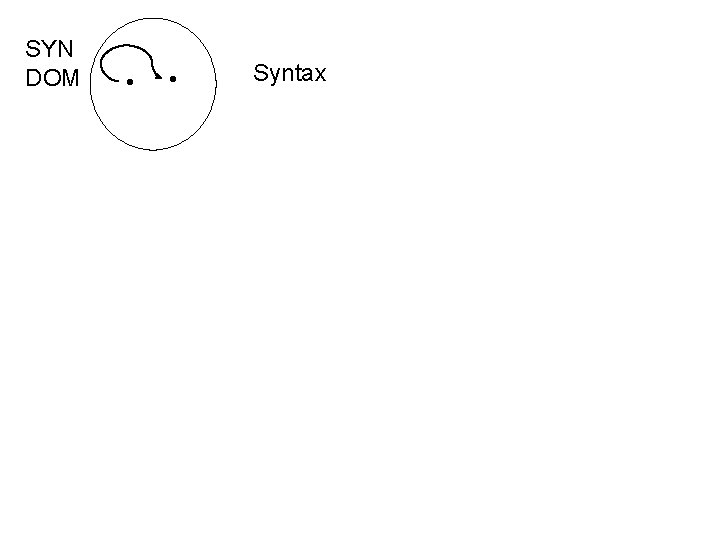
SYN DOM • • Syntax
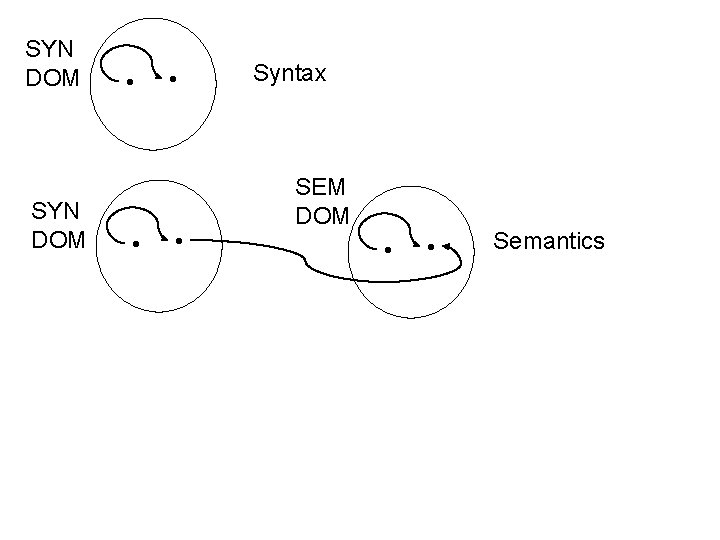
SYN DOM • • Syntax SEM DOM • • Semantics
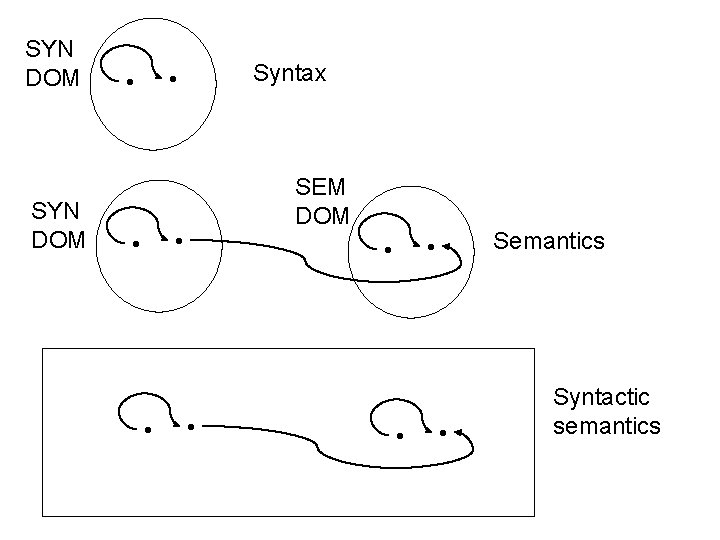
SYN DOM Syntax • • SEM DOM • • Semantics • • Syntactic semantics
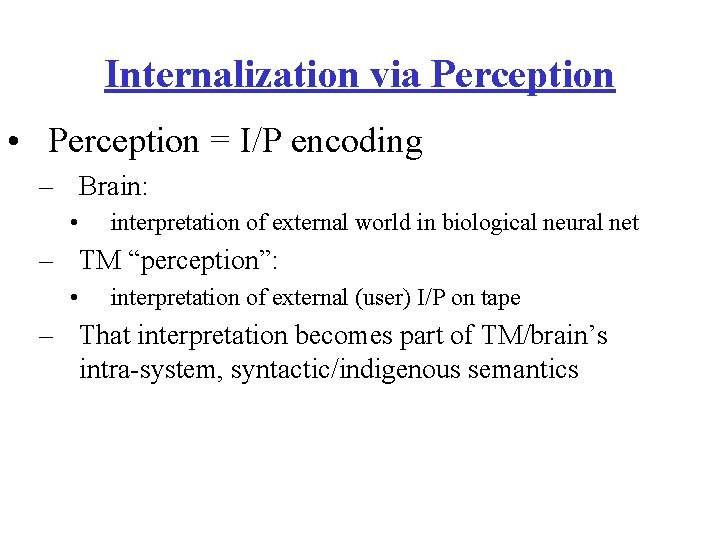
Internalization via Perception • Perception = I/P encoding – Brain: • interpretation of external world in biological neural net – TM “perception”: • interpretation of external (user) I/P on tape – That interpretation becomes part of TM/brain’s intra-system, syntactic/indigenous semantics
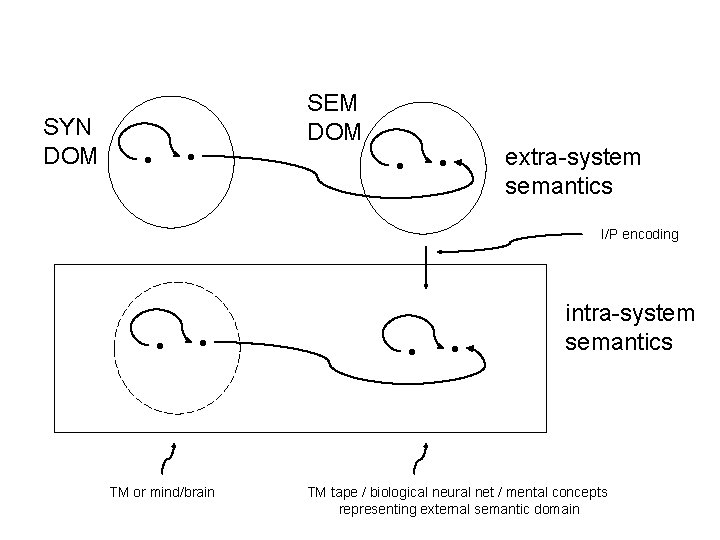
SYN DOM SEM DOM • • extra-system semantics • • I/P encoding • • TM or mind/brain • • intra-system semantics TM tape / biological neural net / mental concepts representing external semantic domain
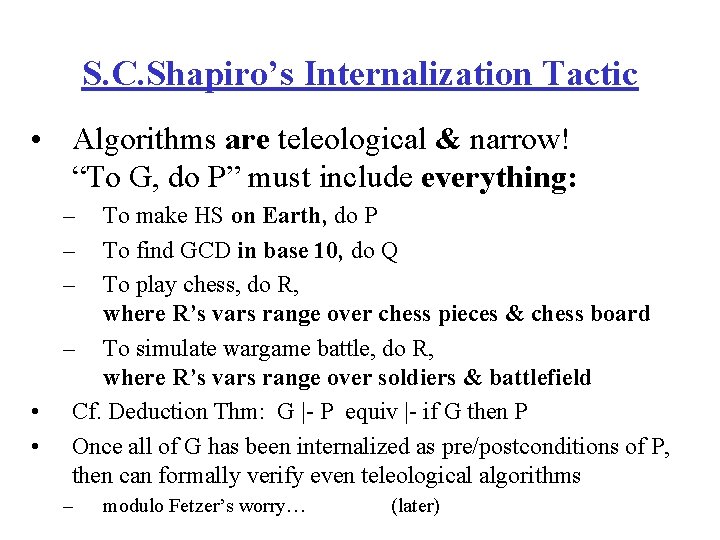
S. C. Shapiro’s Internalization Tactic • Algorithms are teleological & narrow! “To G, do P” must include everything: – – – To make HS on Earth, do P To find GCD in base 10, do Q To play chess, do R, where R’s vars range over chess pieces & chess board – To simulate wargame battle, do R, where R’s vars range over soldiers & battlefield • Cf. Deduction Thm: G |- P equiv |- if G then P • Once all of G has been internalized as pre/postconditions of P, then can formally verify even teleological algorithms – modulo Fetzer’s worry… (later)
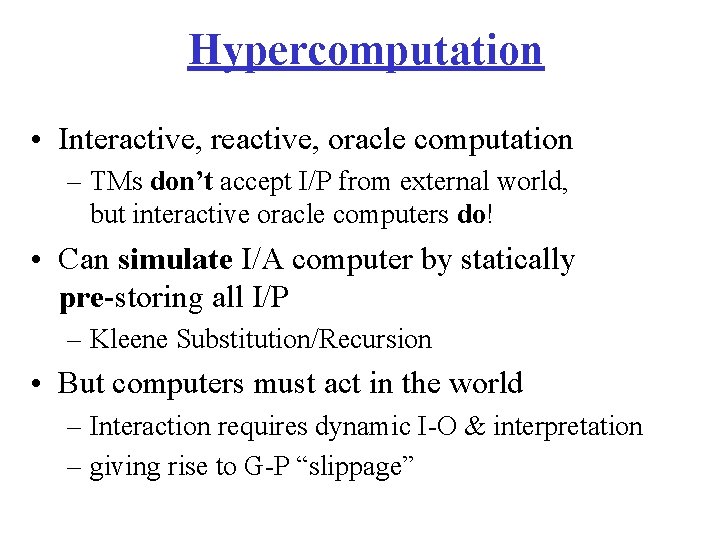
Hypercomputation • Interactive, reactive, oracle computation – TMs don’t accept I/P from external world, but interactive oracle computers do! • Can simulate I/A computer by statically pre-storing all I/P – Kleene Substitution/Recursion • But computers must act in the world – Interaction requires dynamic I-O & interpretation – giving rise to G-P “slippage”
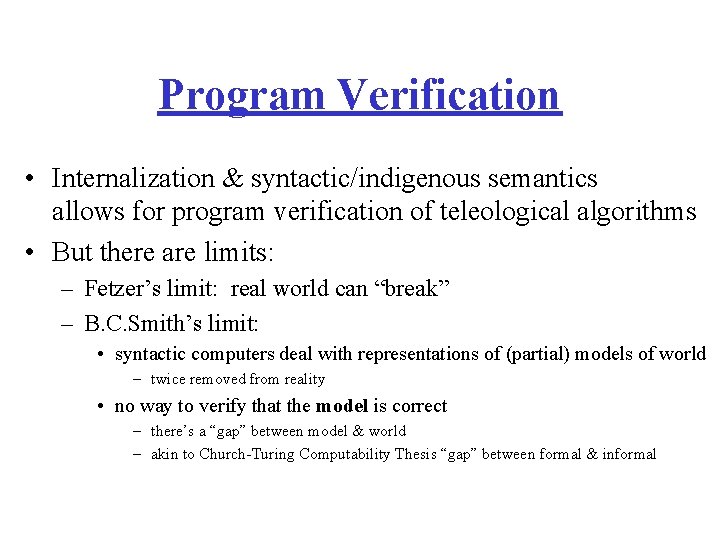
Program Verification • Internalization & syntactic/indigenous semantics allows for program verification of teleological algorithms • But there are limits: – Fetzer’s limit: real world can “break” – B. C. Smith’s limit: • syntactic computers deal with representations of (partial) models of world – twice removed from reality • no way to verify that the model is correct – there’s a “gap” between model & world – akin to Church-Turing Computability Thesis “gap” between formal & informal
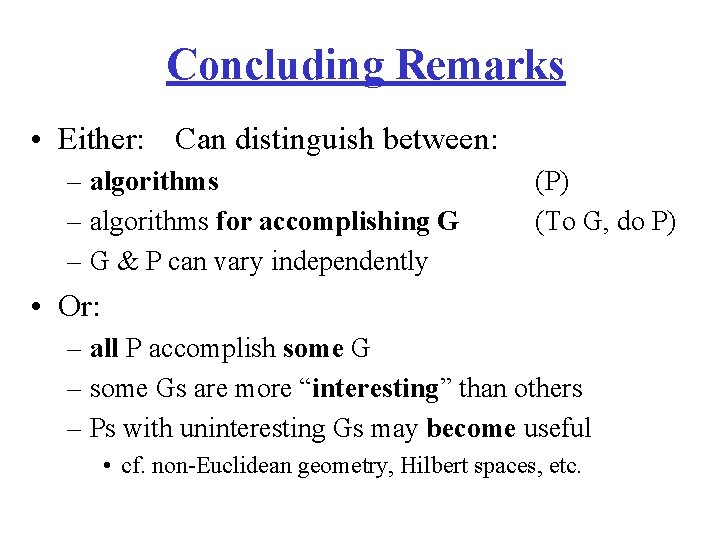
Concluding Remarks • Either: Can distinguish between: – algorithms for accomplishing G – G & P can vary independently (P) (To G, do P) • Or: – all P accomplish some G – some Gs are more “interesting” than others – Ps with uninteresting Gs may become useful • cf. non-Euclidean geometry, Hilbert spaces, etc.
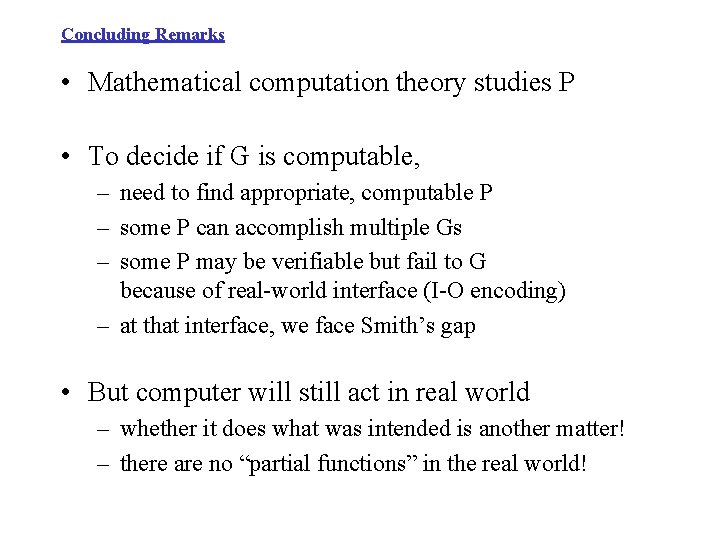
Concluding Remarks • Mathematical computation theory studies P • To decide if G is computable, – need to find appropriate, computable P – some P can accomplish multiple Gs – some P may be verifiable but fail to G because of real-world interface (I-O encoding) – at that interface, we face Smith’s gap • But computer will still act in real world – whether it does what was intended is another matter! – there are no “partial functions” in the real world!
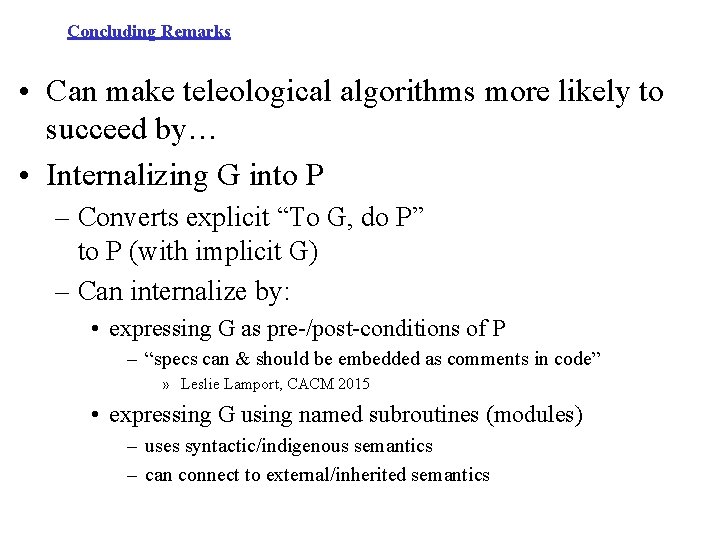
Concluding Remarks • Can make teleological algorithms more likely to succeed by… • Internalizing G into P – Converts explicit “To G, do P” to P (with implicit G) – Can internalize by: • expressing G as pre-/post-conditions of P – “specs can & should be embedded as comments in code” » Leslie Lamport, CACM 2015 • expressing G using named subroutines (modules) – uses syntactic/indigenous semantics – can connect to external/inherited semantics
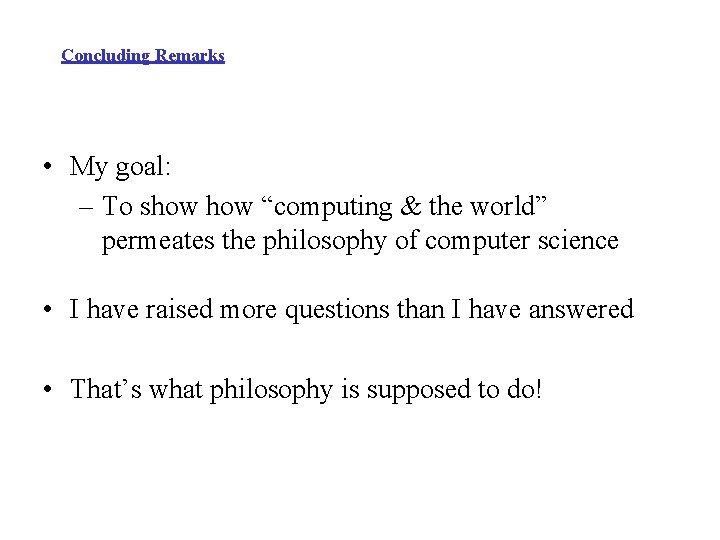
Concluding Remarks • My goal: – To show “computing & the world” permeates the philosophy of computer science • I have raised more questions than I have answered • That’s what philosophy is supposed to do!
Conventional computing and intelligent computing
Hình ảnh bộ gõ cơ thể búng tay
Bổ thể
Tỉ lệ cơ thể trẻ em
Chó sói
Tư thế worms-breton
Bài hát chúa yêu trần thế alleluia
Môn thể thao bắt đầu bằng từ chạy
Thế nào là hệ số cao nhất
Các châu lục và đại dương trên thế giới
Cong thức tính động năng
Trời xanh đây là của chúng ta thể thơ
Cách giải mật thư tọa độ
101012 bằng
độ dài liên kết
Các châu lục và đại dương trên thế giới
Thơ thất ngôn tứ tuyệt đường luật
Quá trình desamine hóa có thể tạo ra
Một số thể thơ truyền thống
Cái miệng nó xinh thế chỉ nói điều hay thôi
Vẽ hình chiếu vuông góc của vật thể sau
Thế nào là sự mỏi cơ
đặc điểm cơ thể của người tối cổ
Ví dụ về giọng cùng tên
Vẽ hình chiếu đứng bằng cạnh của vật thể
Phối cảnh
Thẻ vin
đại từ thay thế
điện thế nghỉ
Tư thế ngồi viết
Diễn thế sinh thái là
Các loại đột biến cấu trúc nhiễm sắc thể
Bảng số nguyên tố lớn hơn 1000
Tư thế ngồi viết
Lời thề hippocrates
Thiếu nhi thế giới liên hoan
ưu thế lai là gì
Sự nuôi và dạy con của hổ
Khi nào hổ mẹ dạy hổ con săn mồi
Sơ đồ cơ thể người
Từ ngữ thể hiện lòng nhân hậu
Thế nào là mạng điện lắp đặt kiểu nổi
Old world monkey vs new world monkey