The Monsoon Mission A mission mode project to
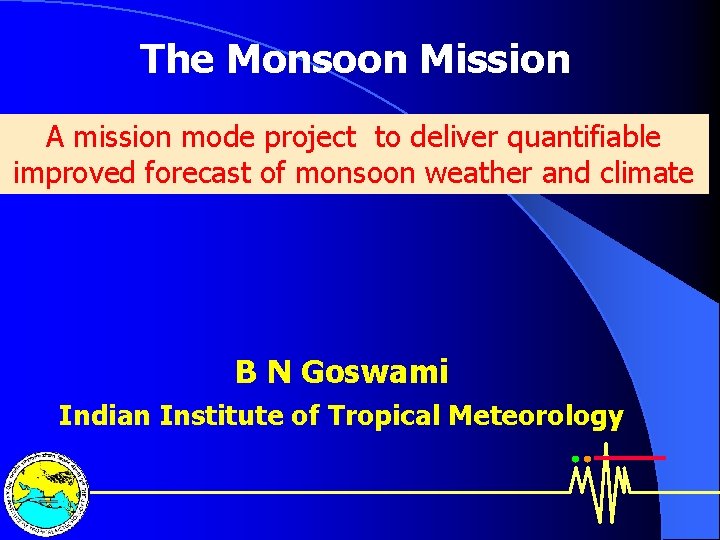
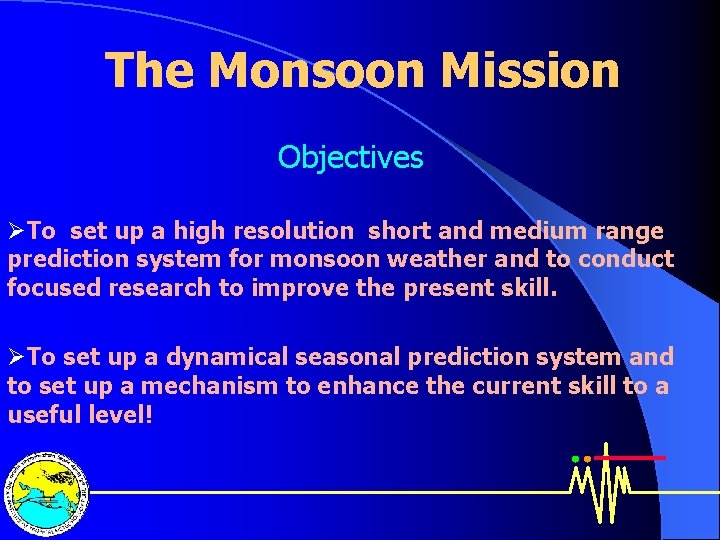
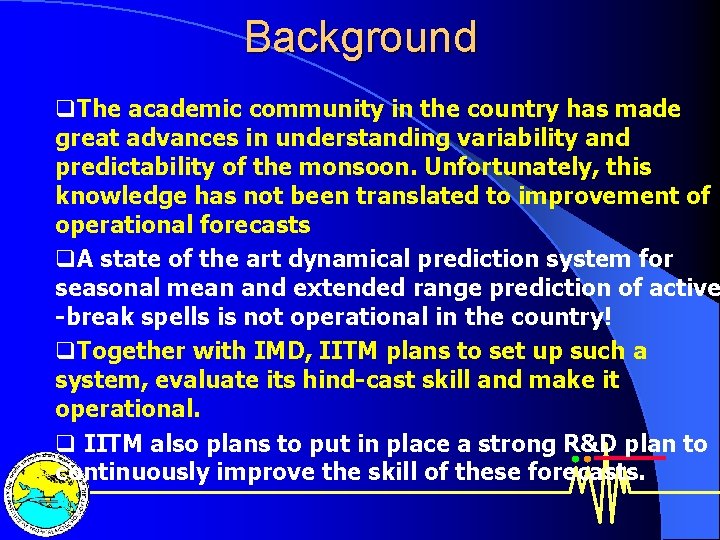
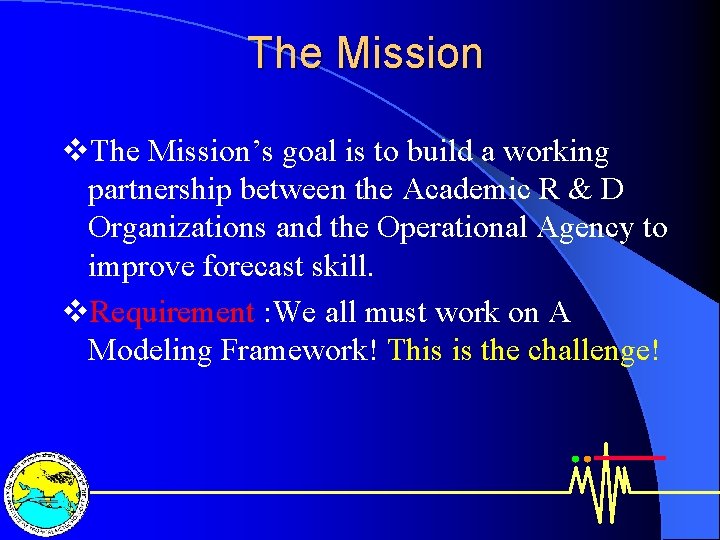
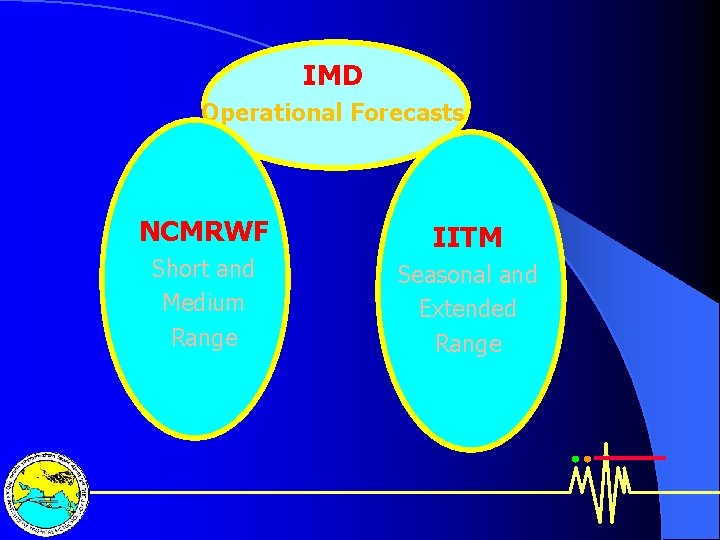
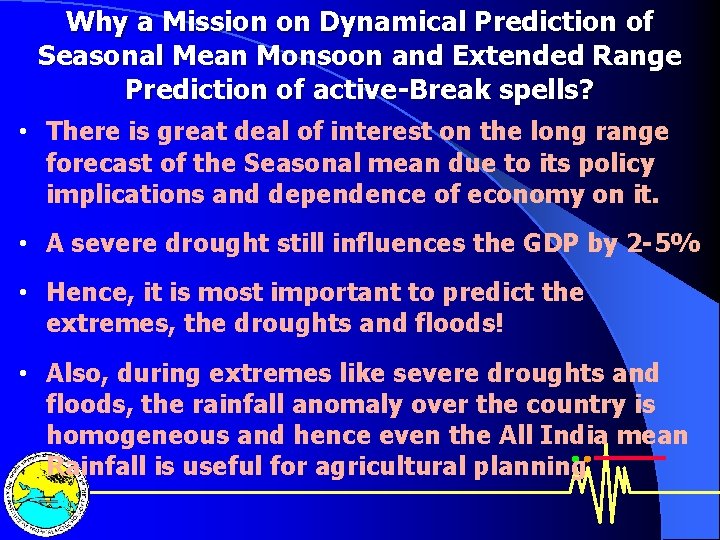
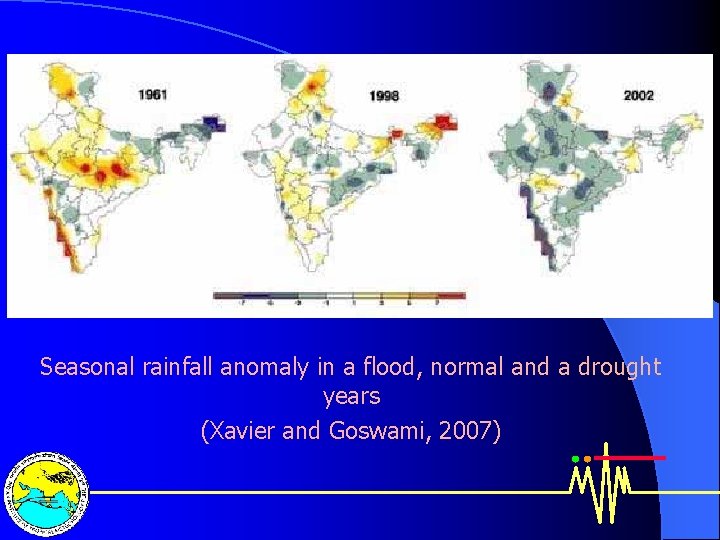
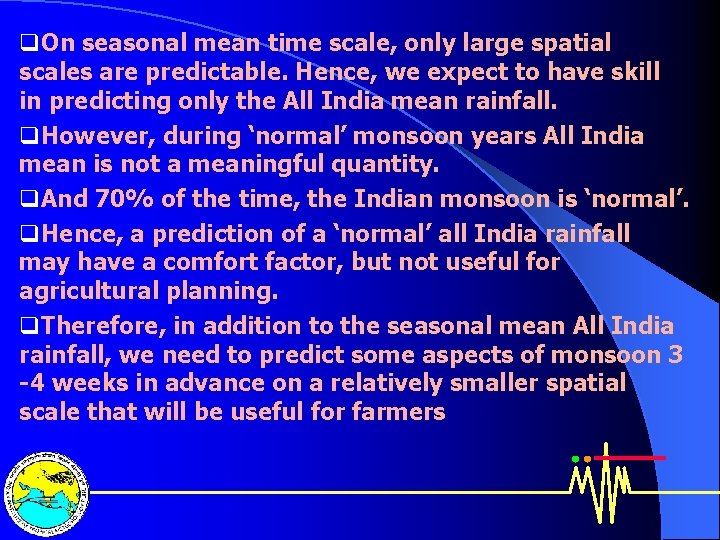
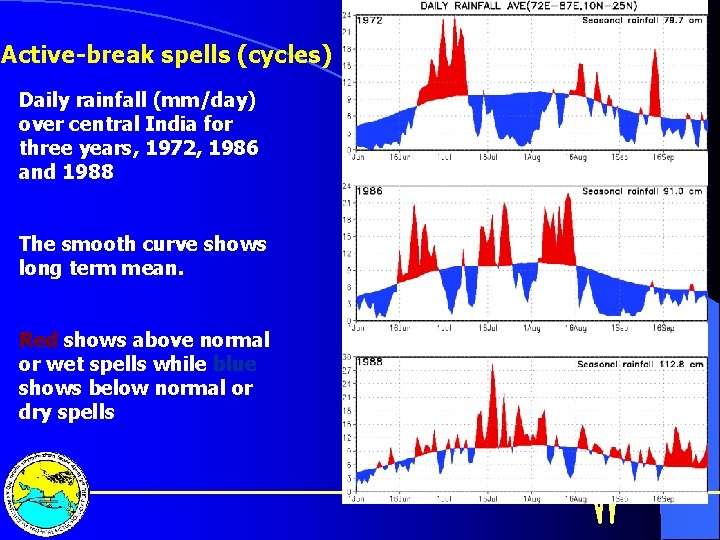
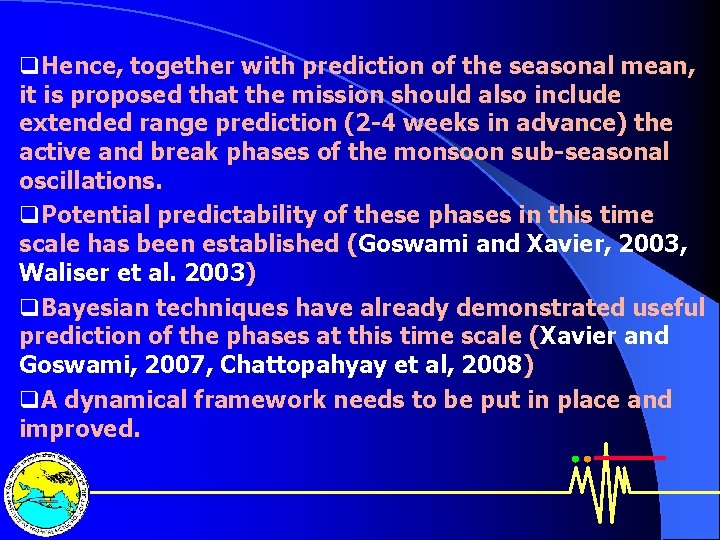
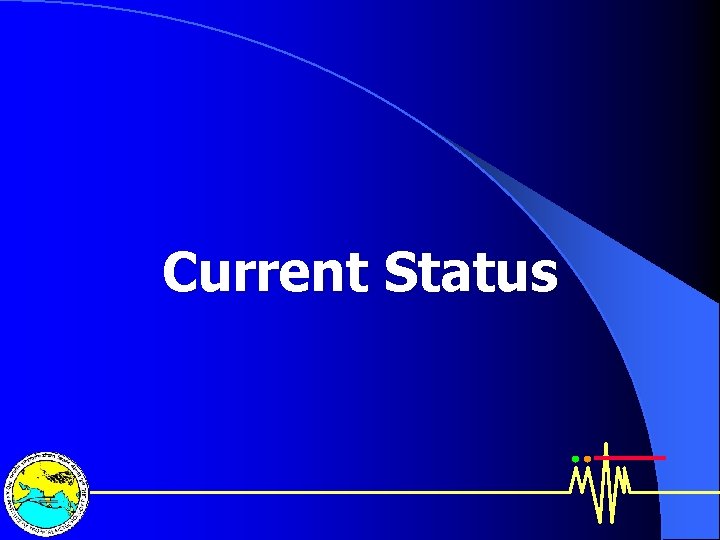
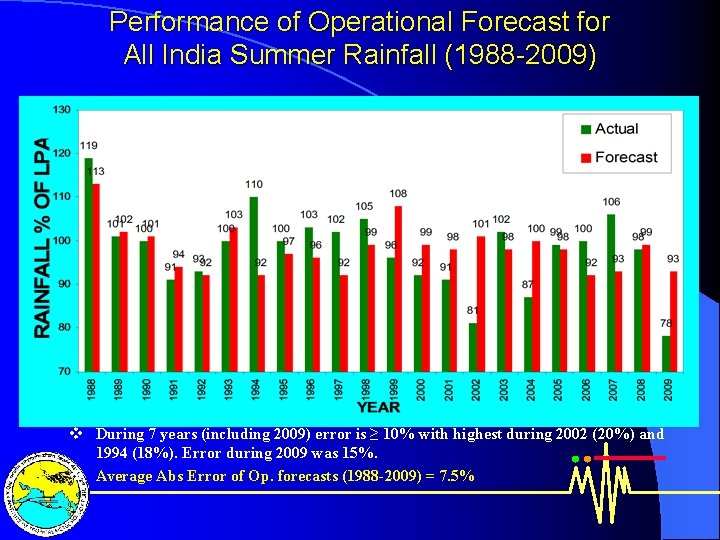
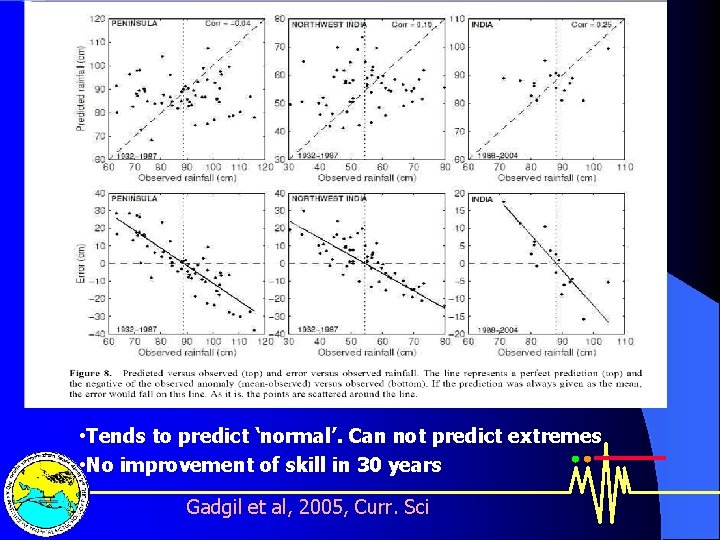
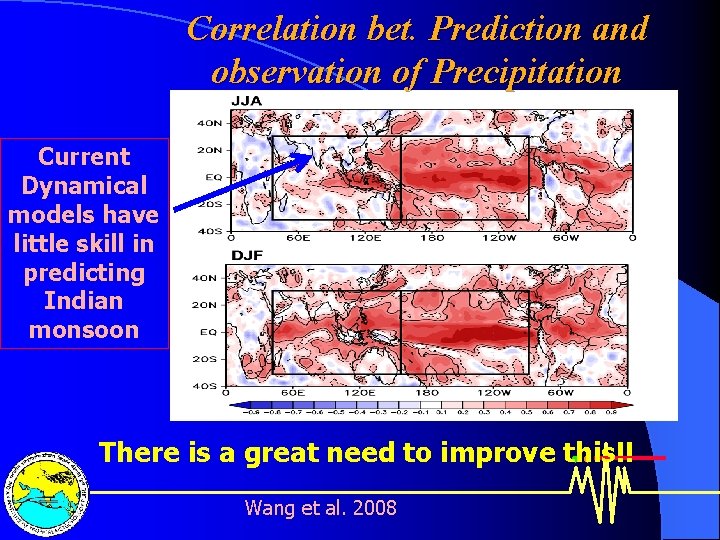
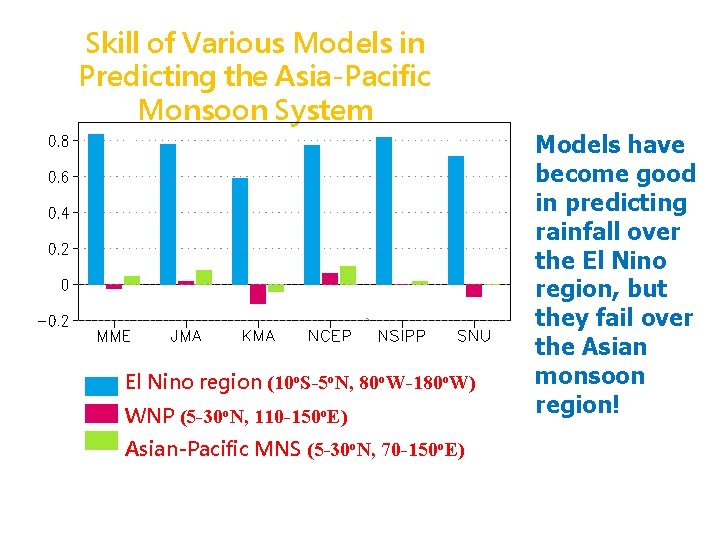
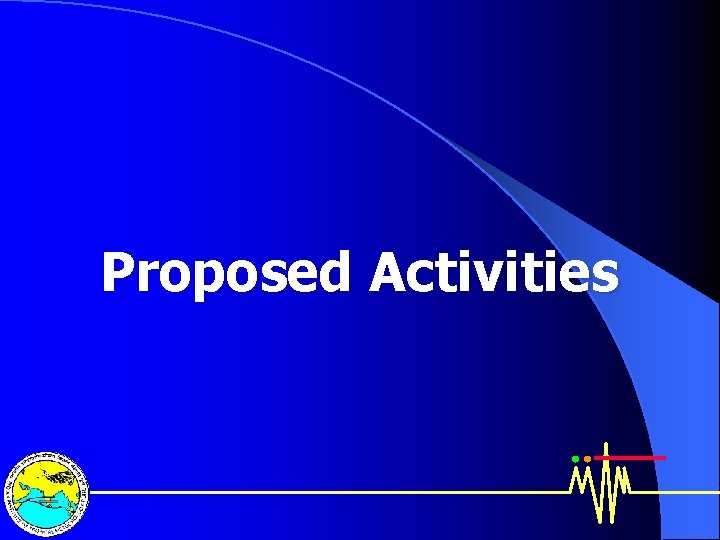
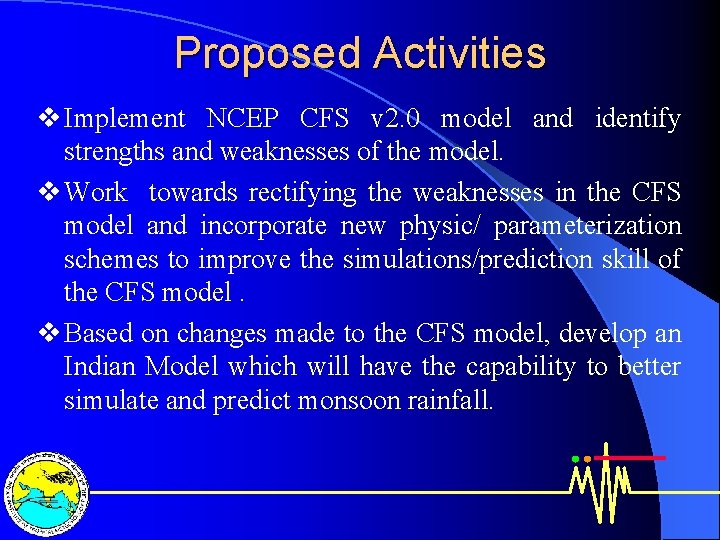
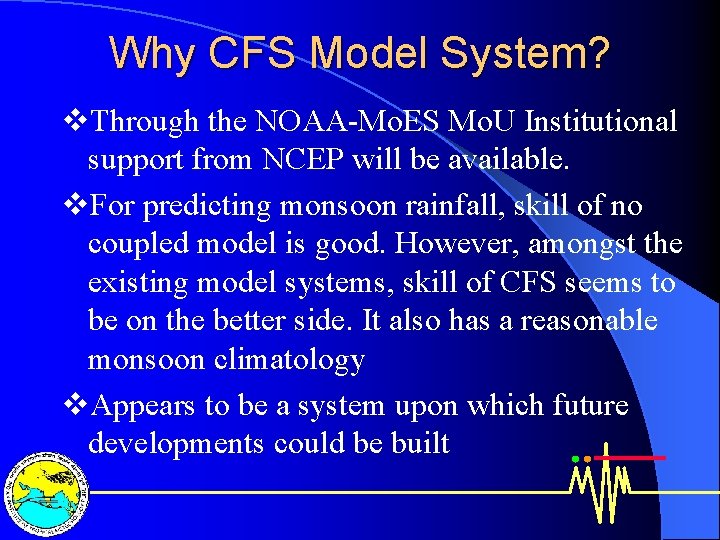
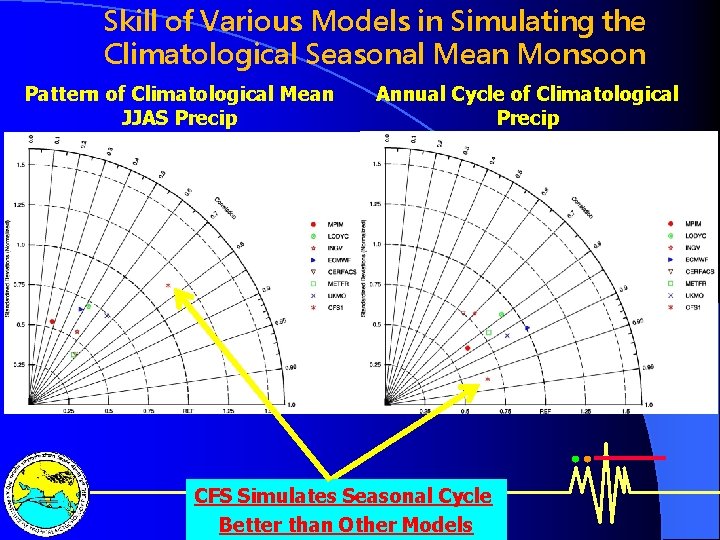
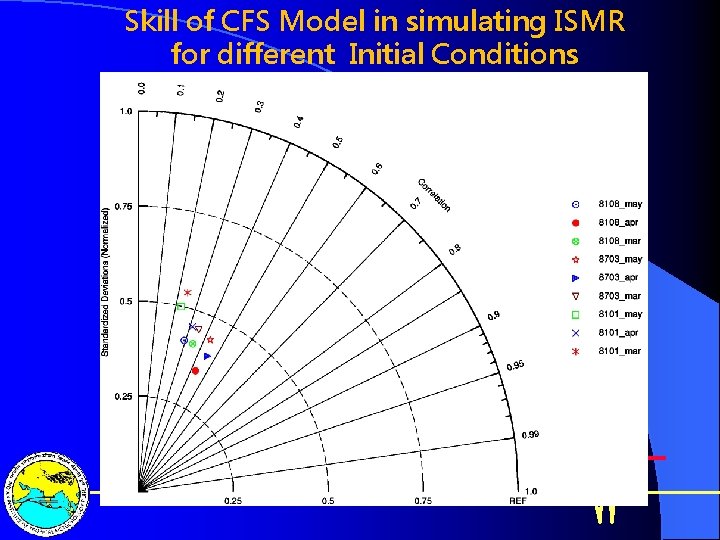
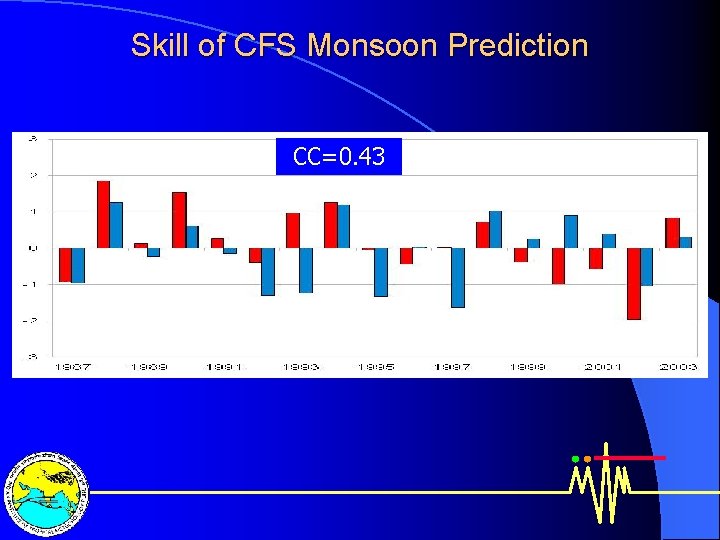
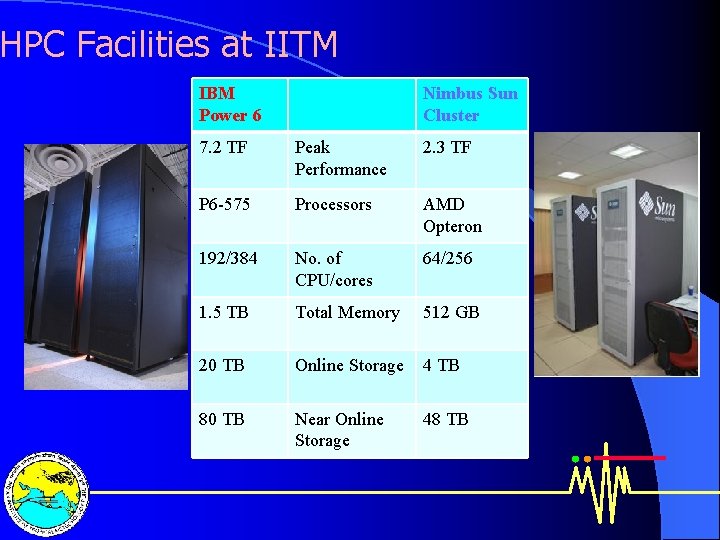
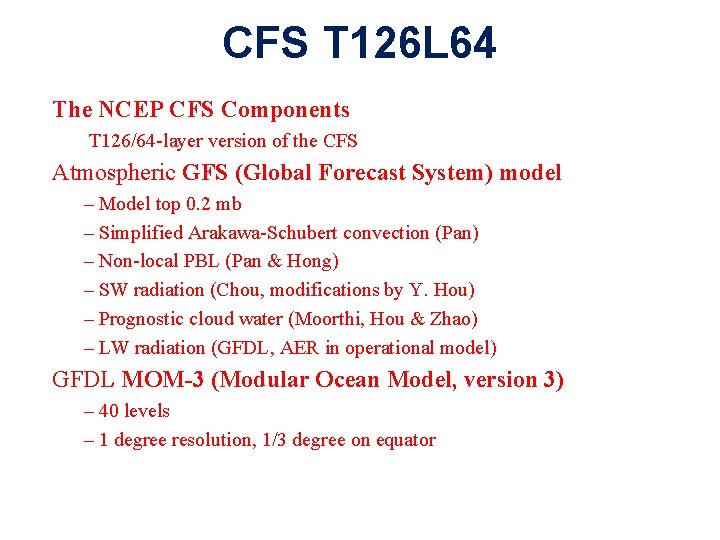
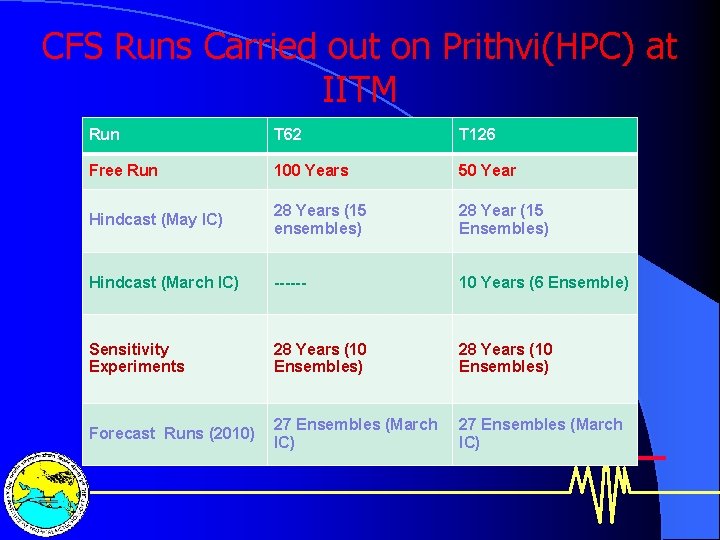
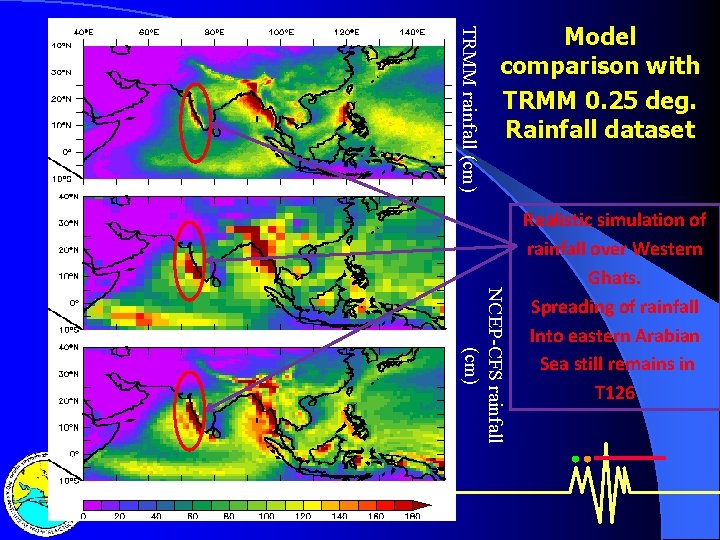
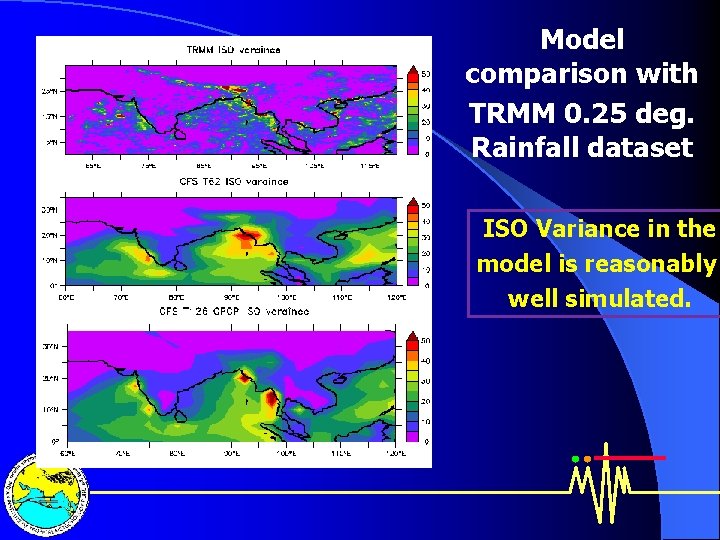
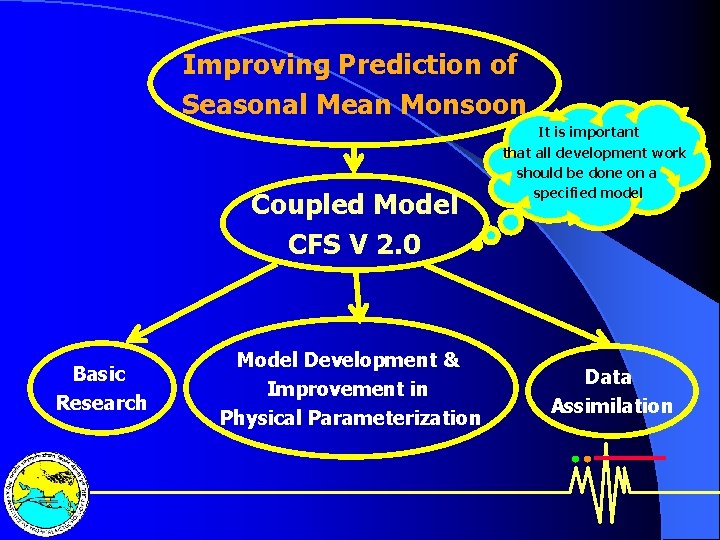
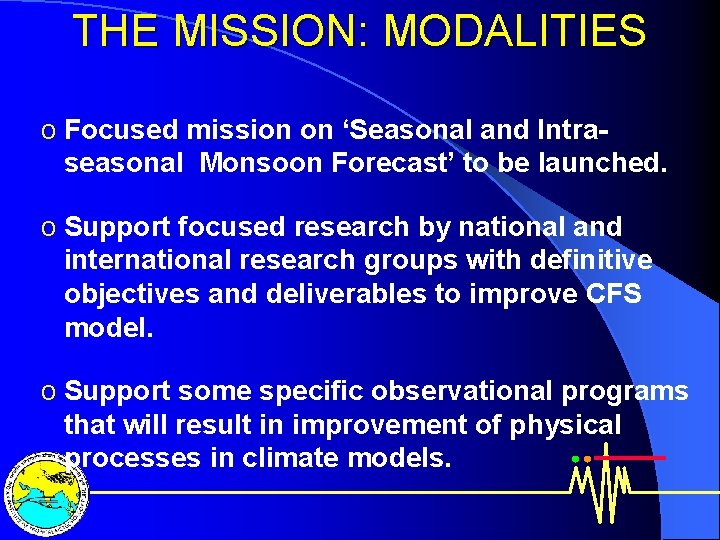
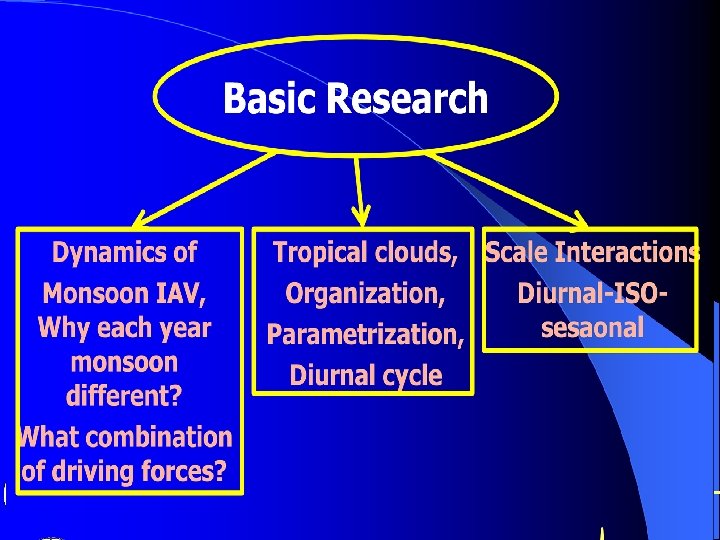
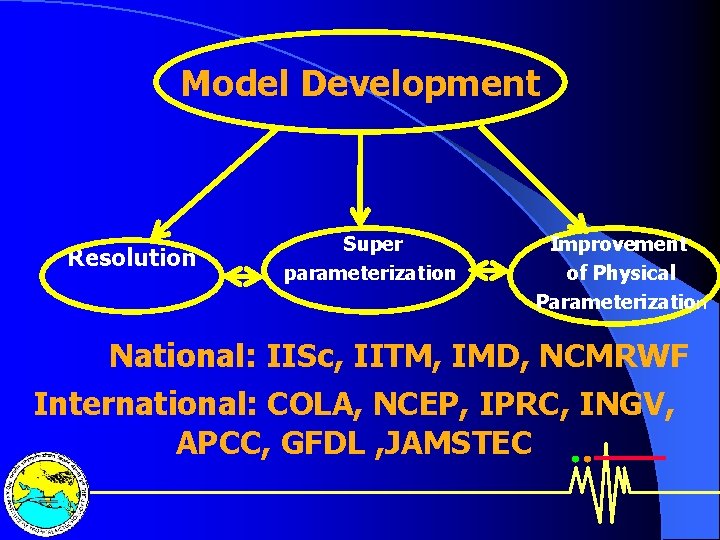
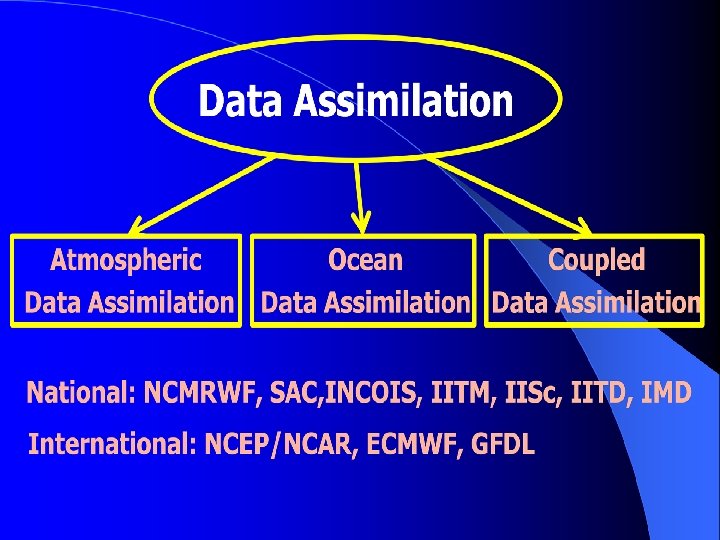
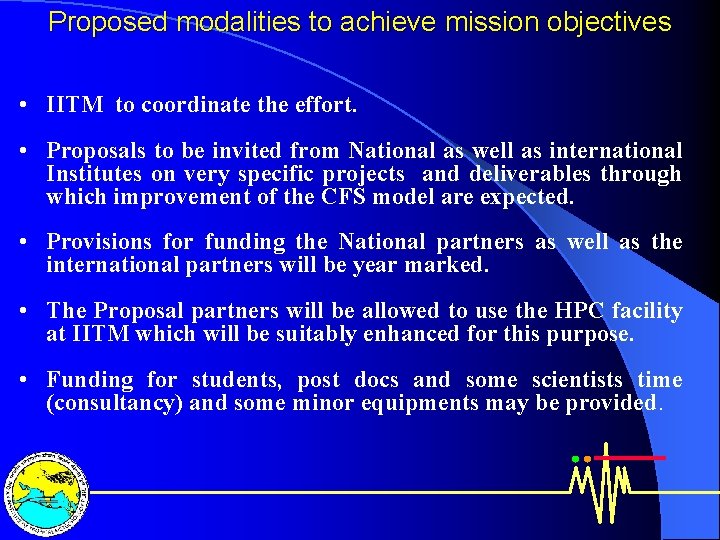
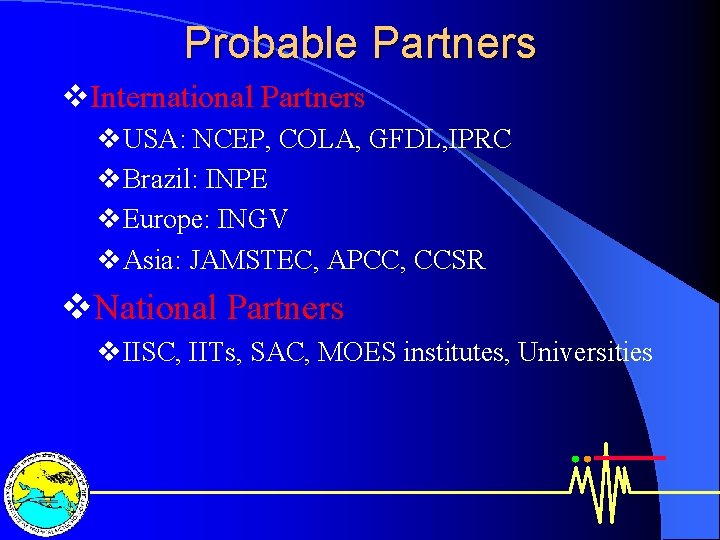
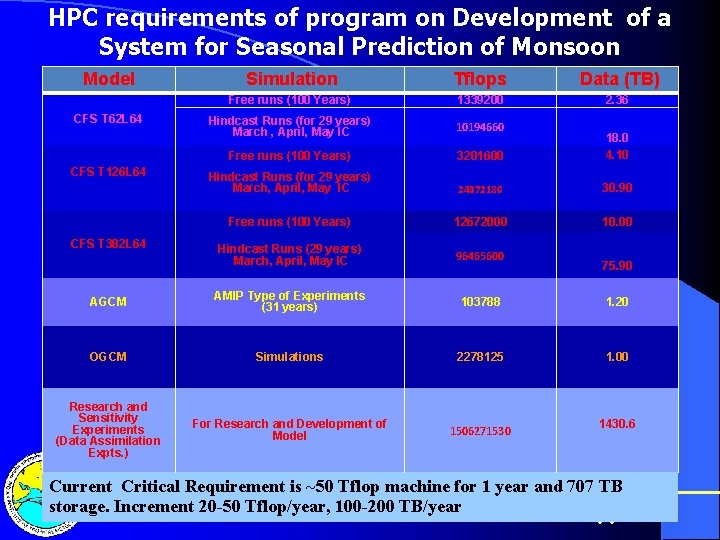
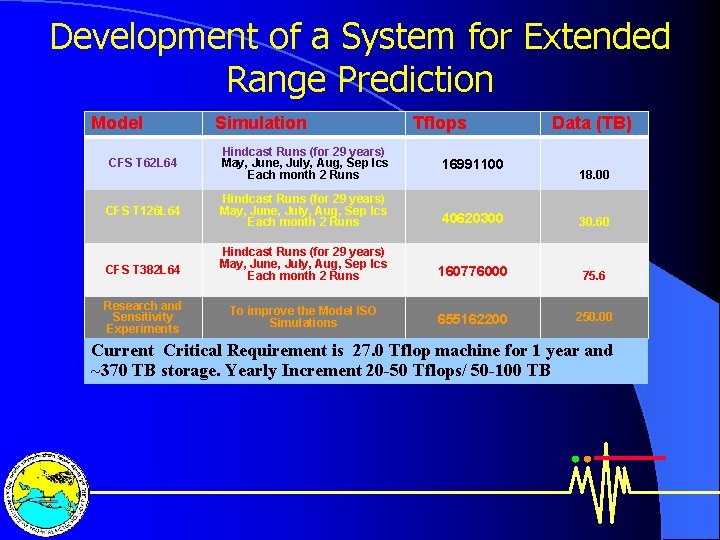
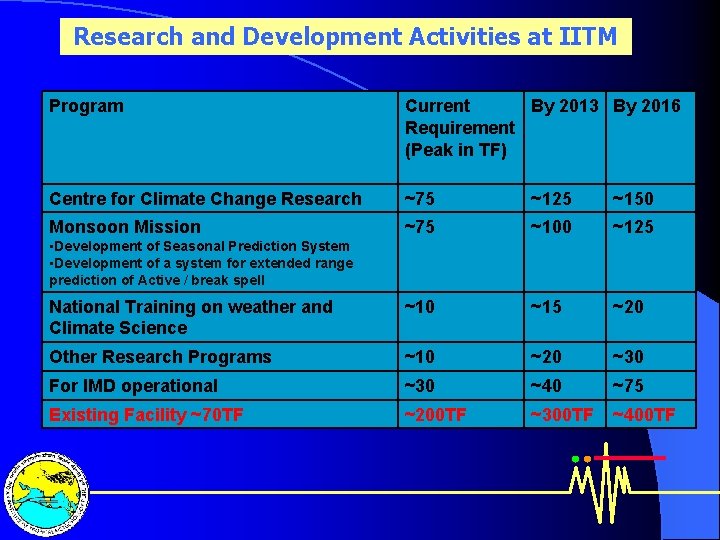
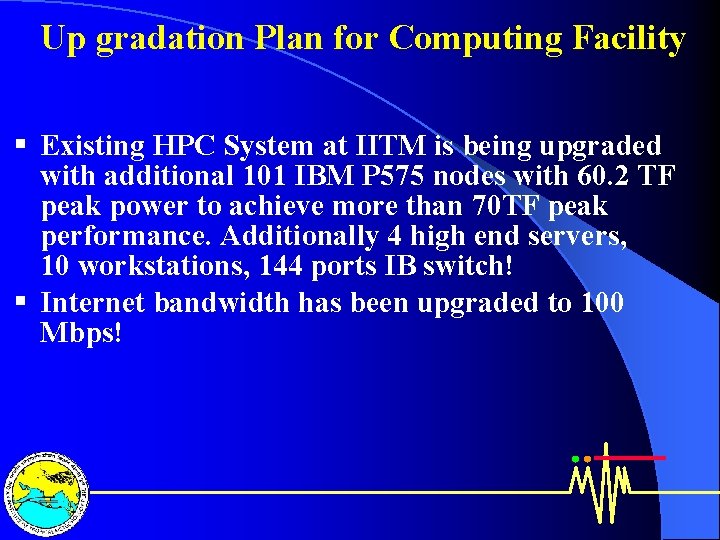
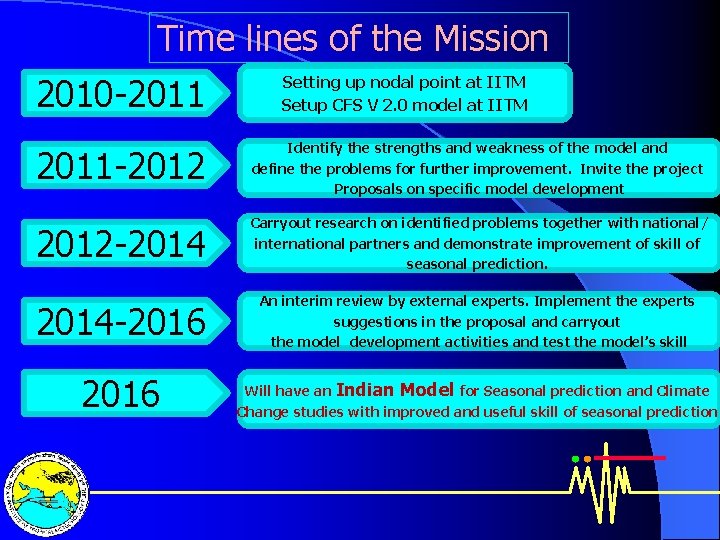
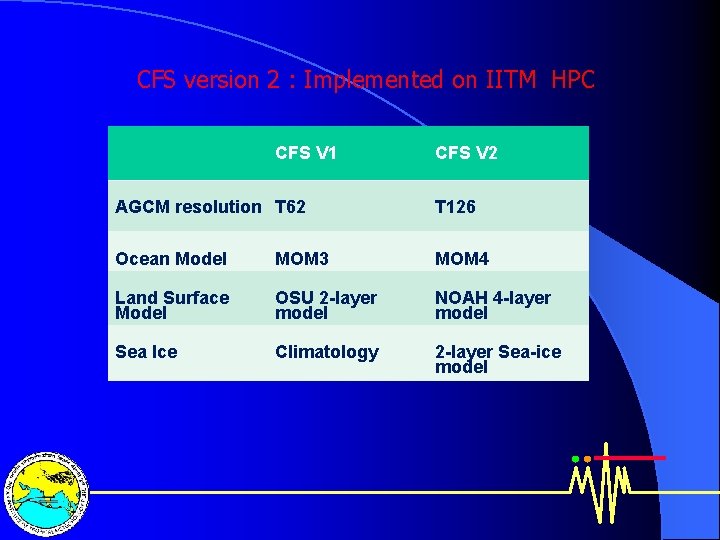
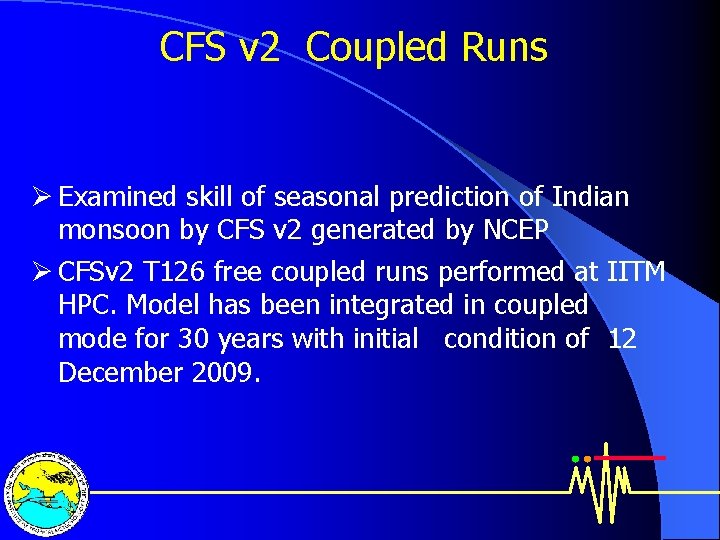
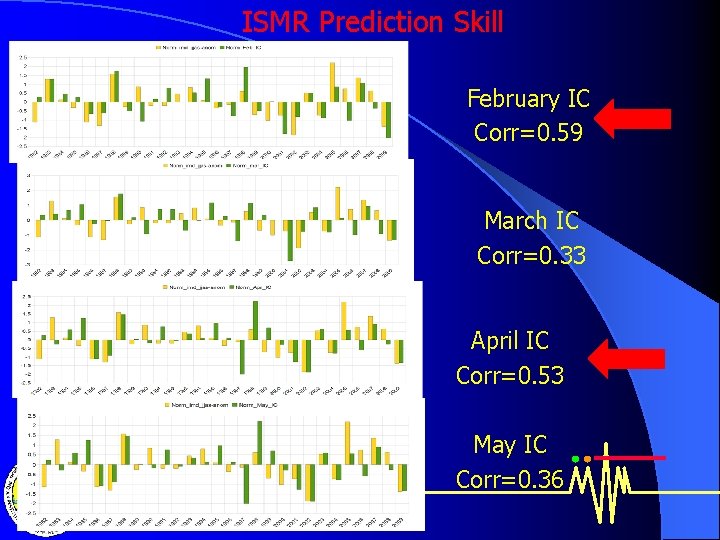
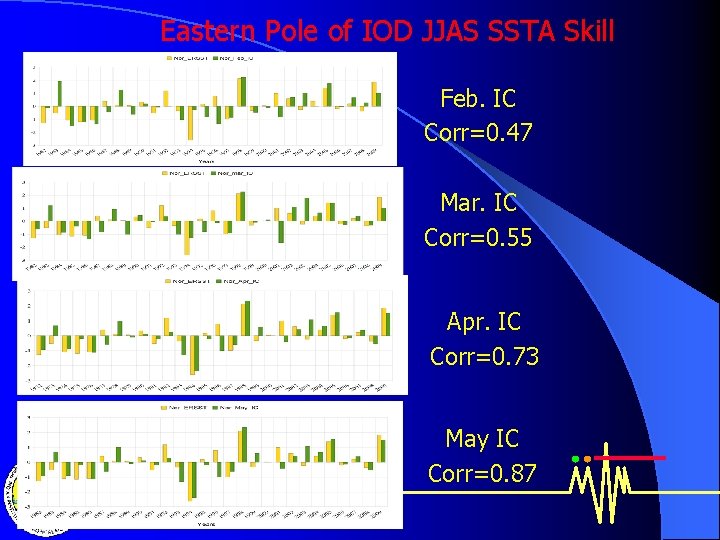
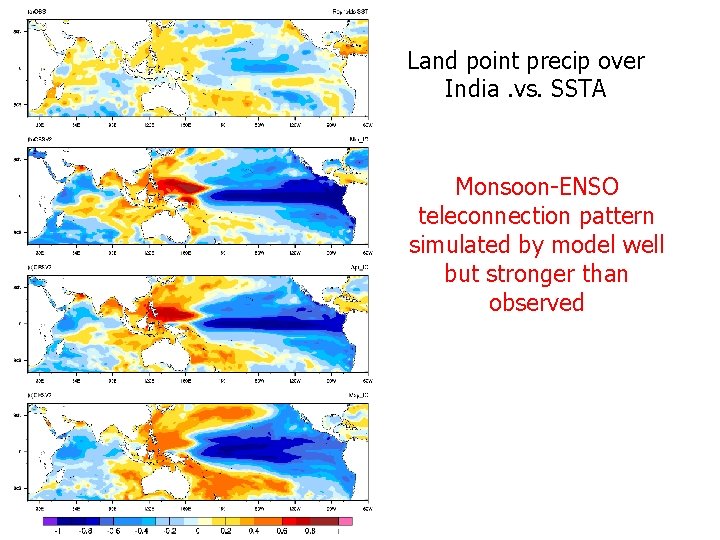
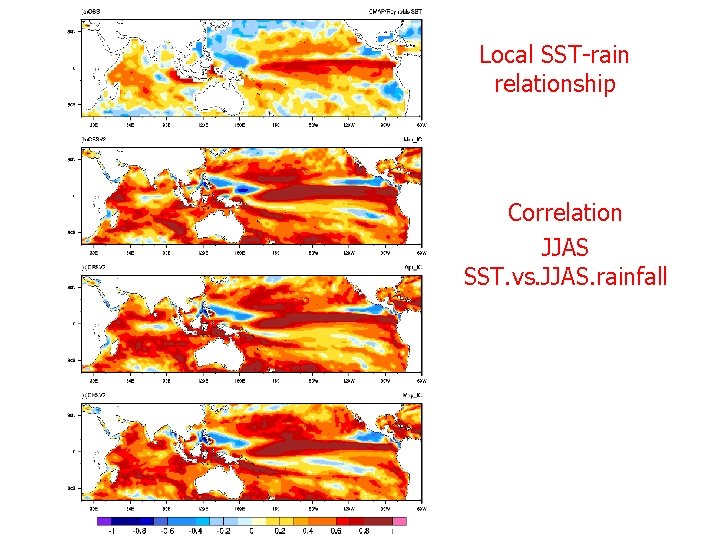
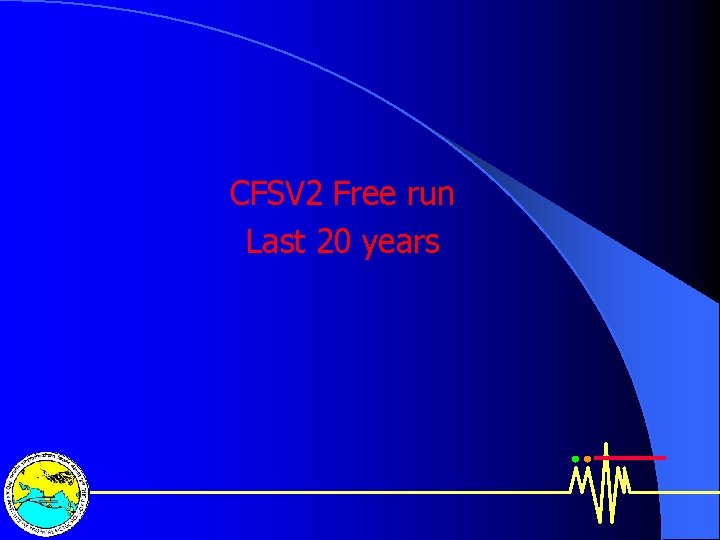
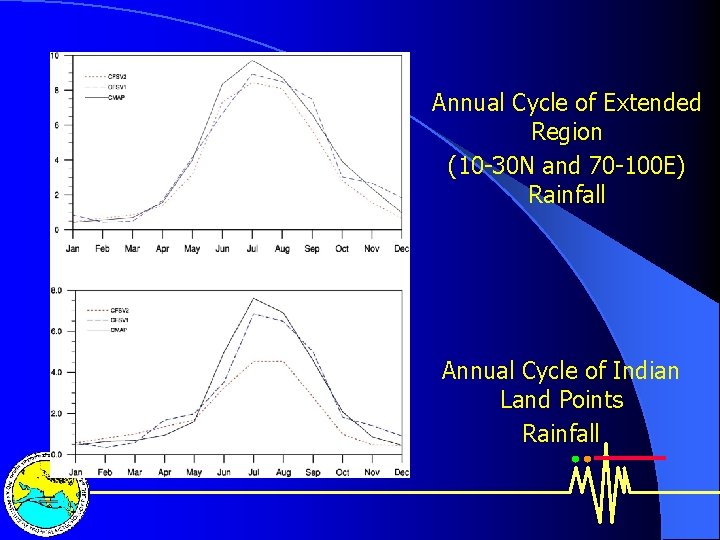
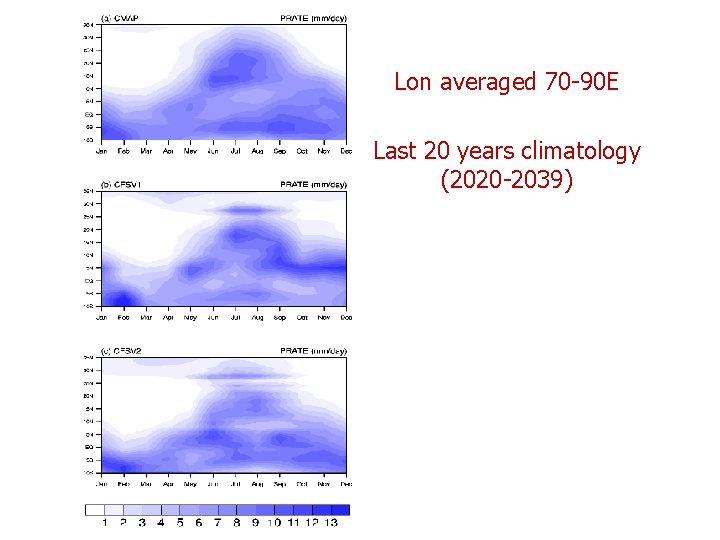
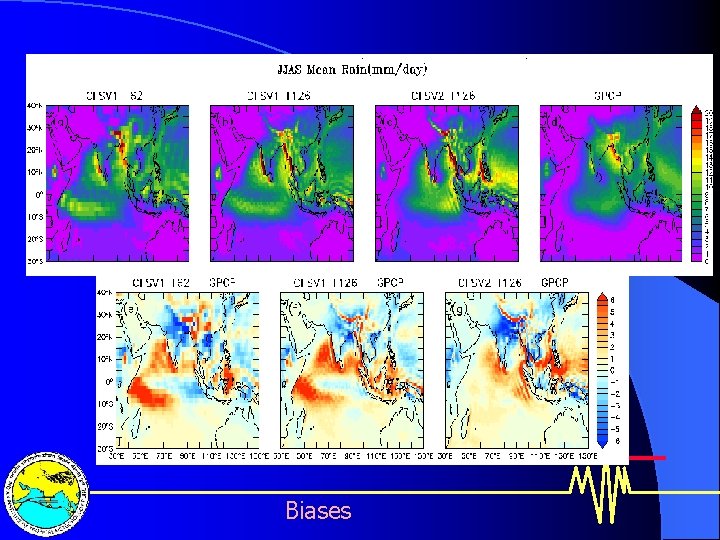
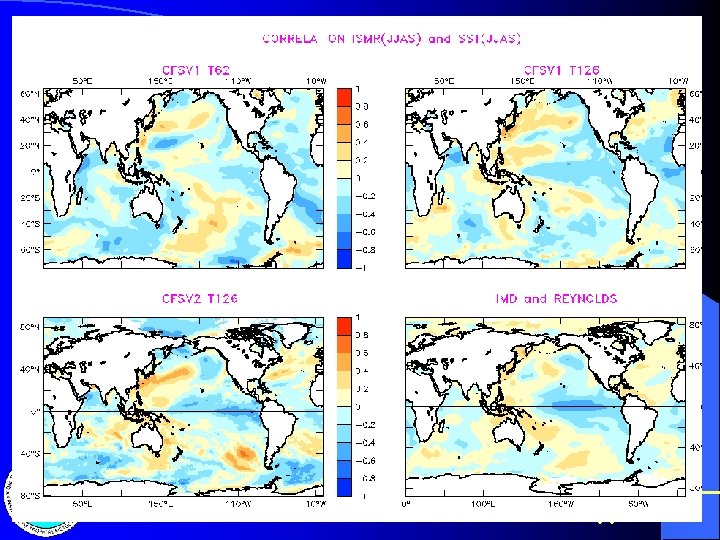
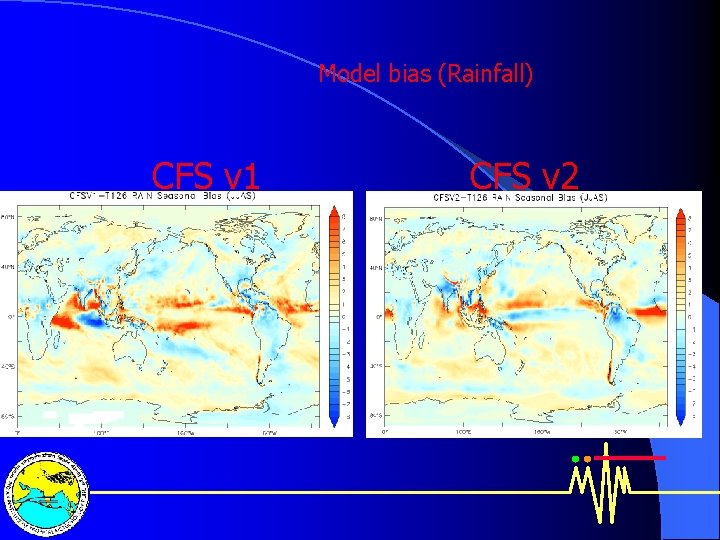
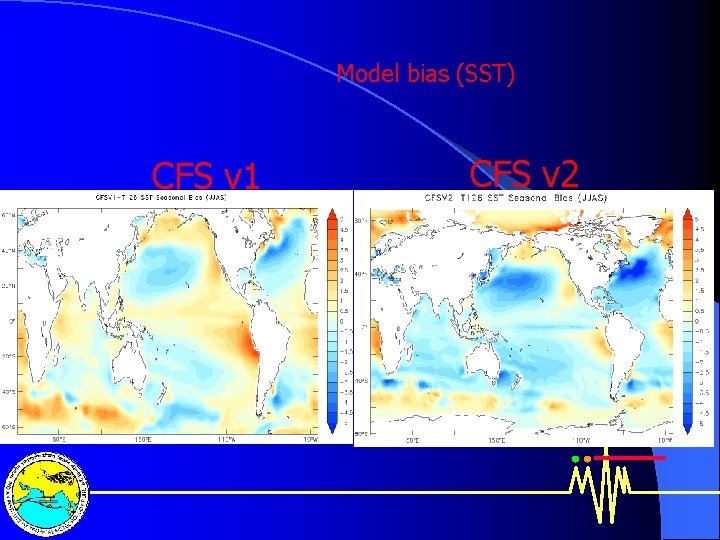
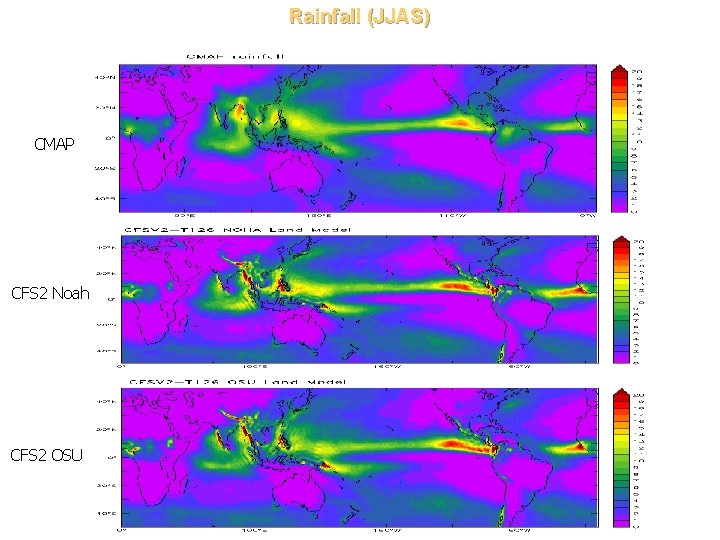
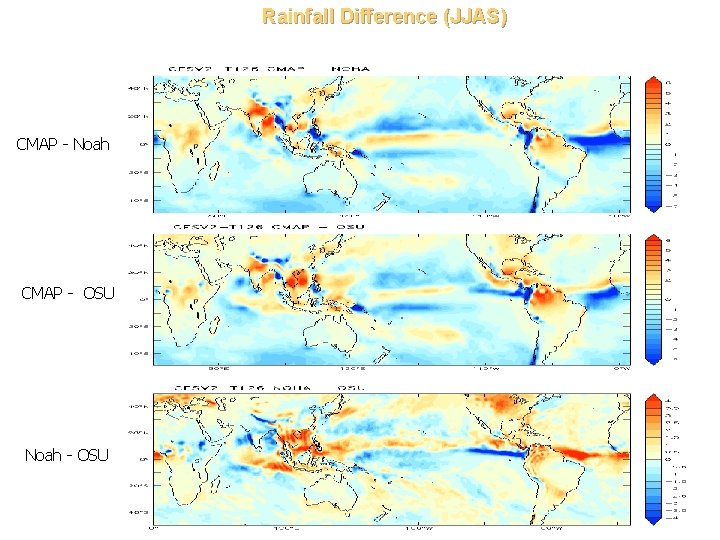
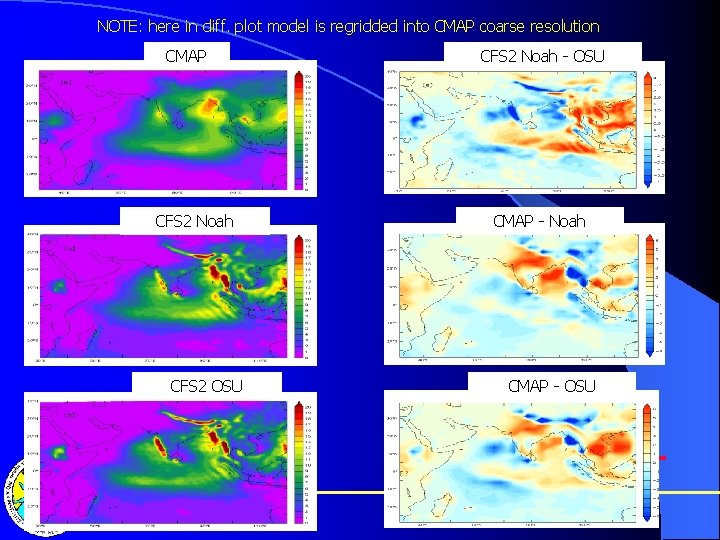
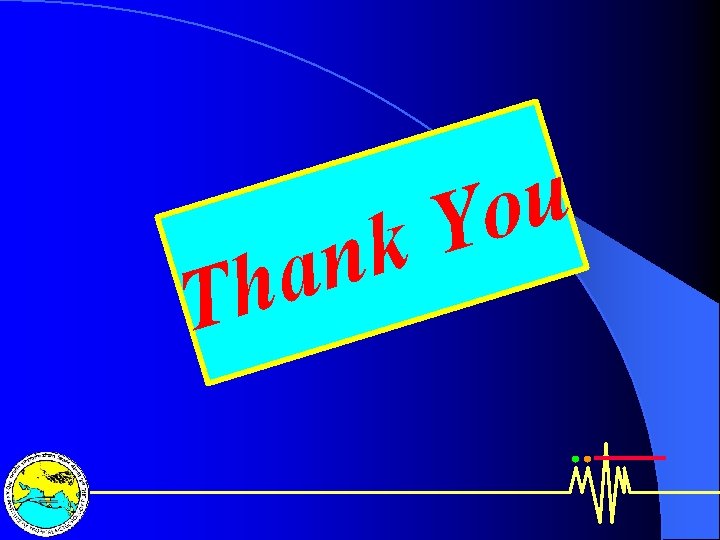
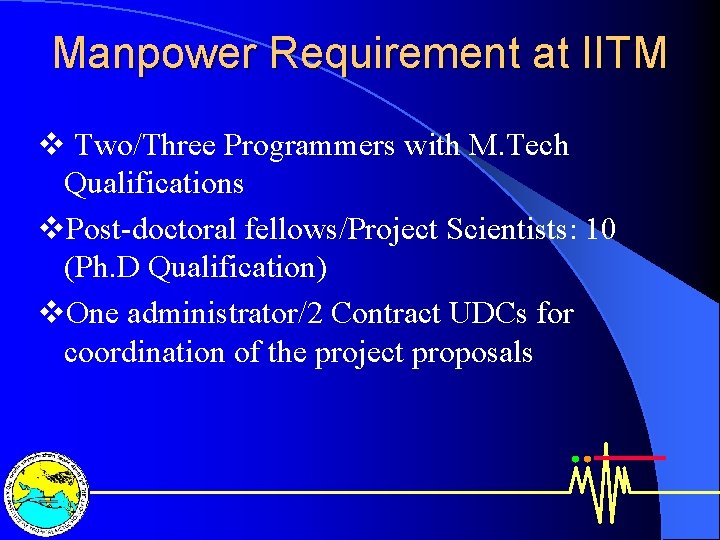
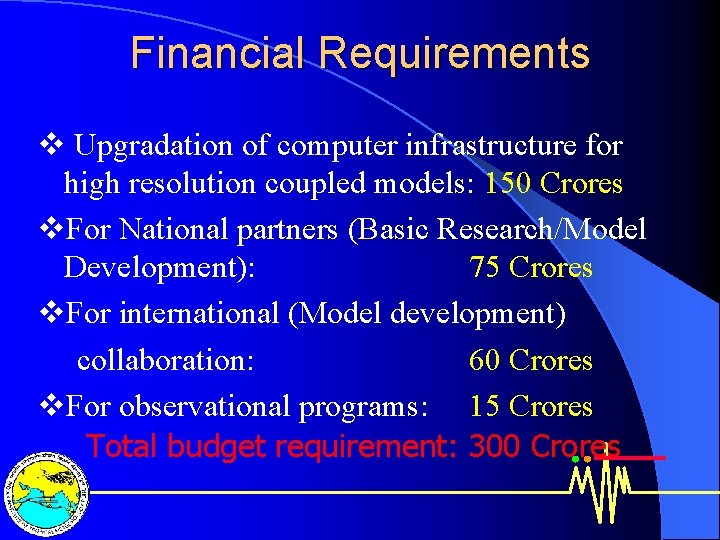
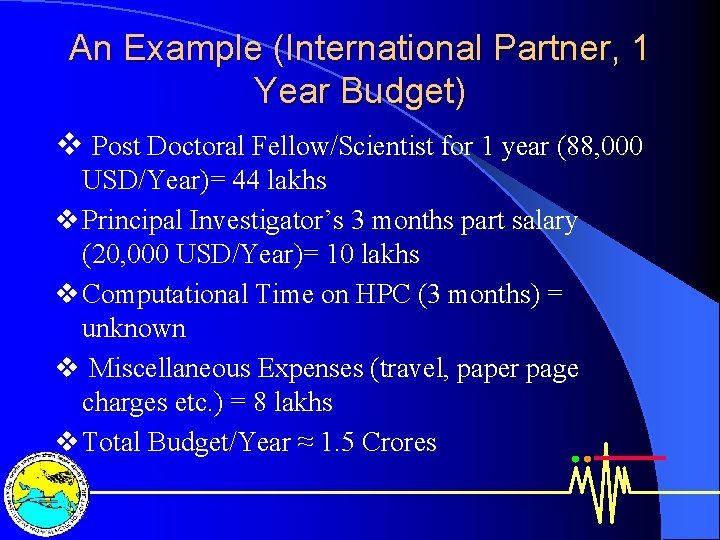
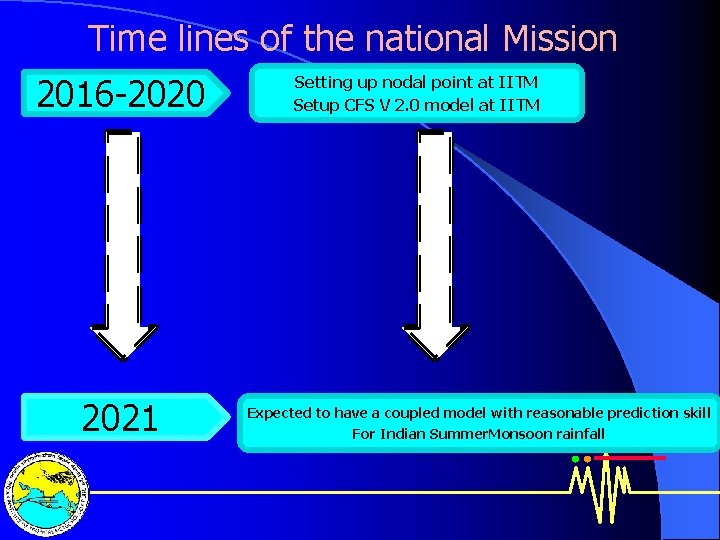
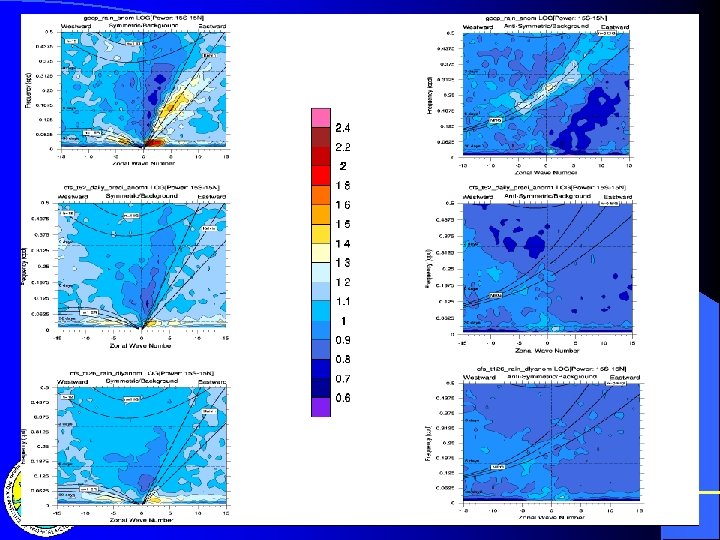
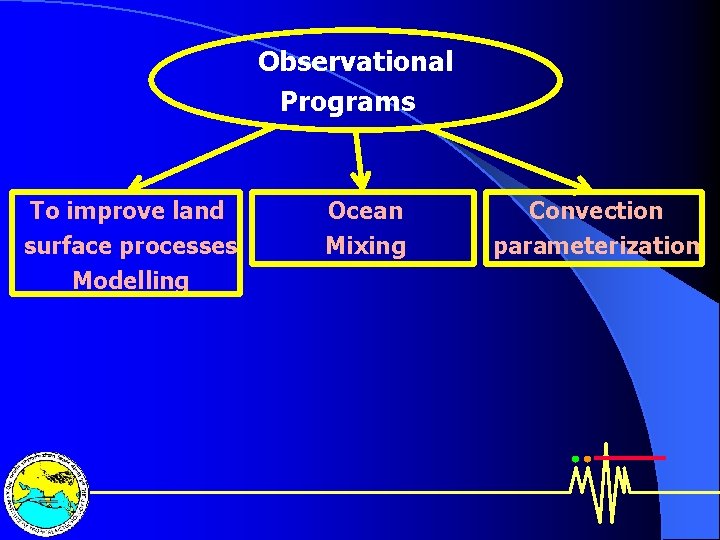
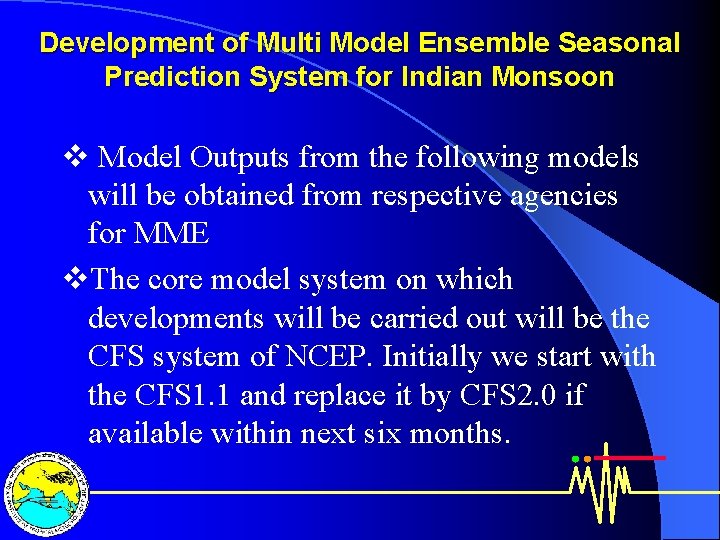
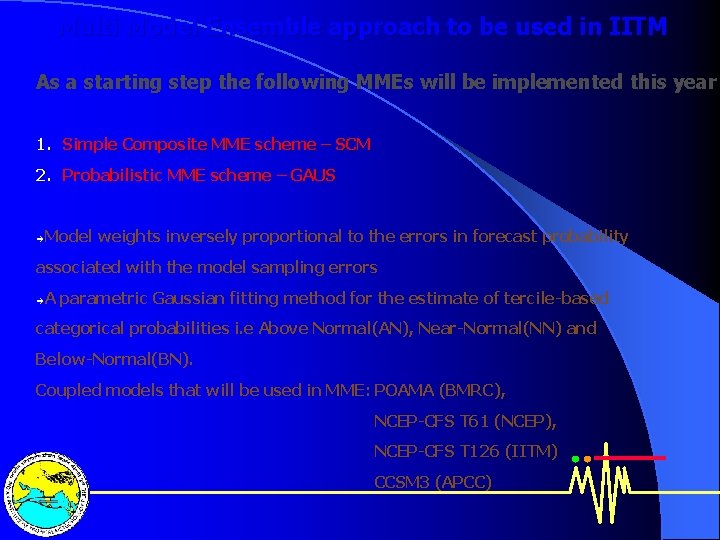
- Slides: 63
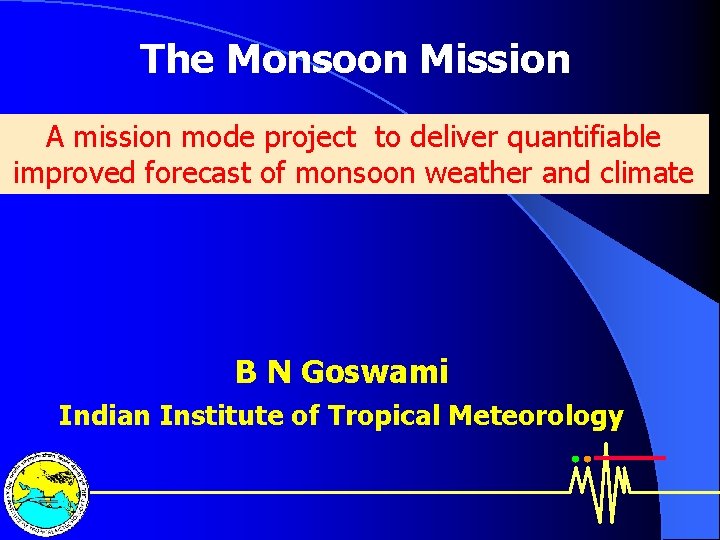
The Monsoon Mission A mission mode project to deliver quantifiable improved forecast of monsoon weather and climate B N Goswami Indian Institute of Tropical Meteorology
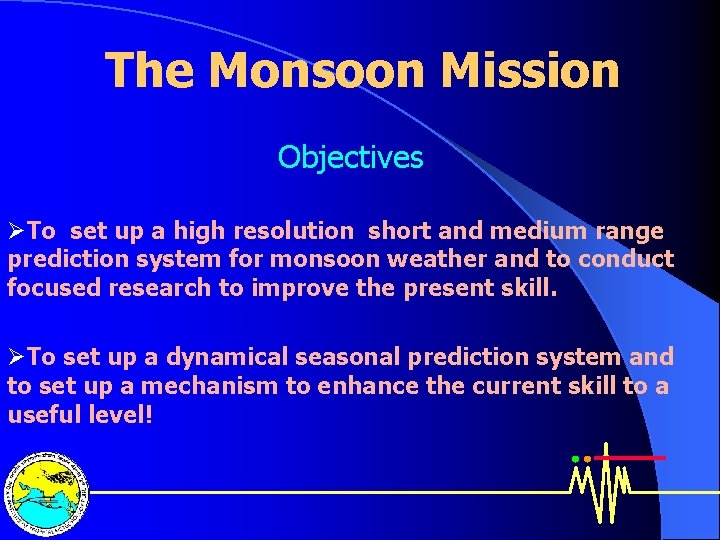
The Monsoon Mission Objectives ØTo set up a high resolution short and medium range prediction system for monsoon weather and to conduct focused research to improve the present skill. ØTo set up a dynamical seasonal prediction system and to set up a mechanism to enhance the current skill to a useful level!
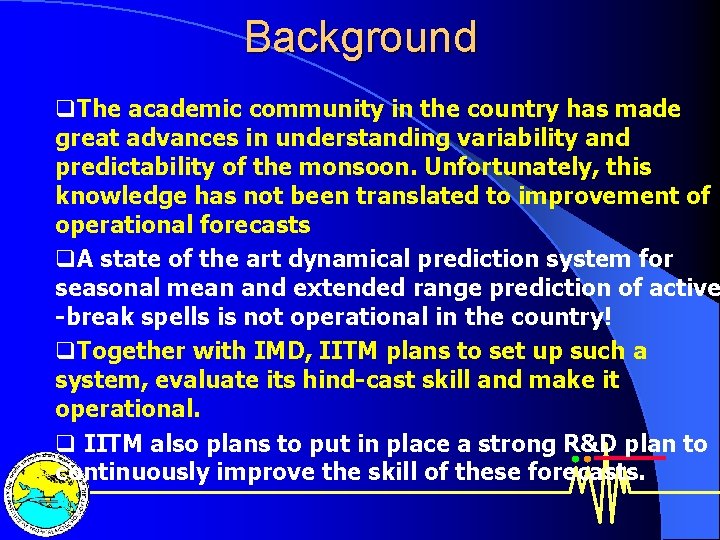
Background q. The academic community in the country has made great advances in understanding variability and predictability of the monsoon. Unfortunately, this knowledge has not been translated to improvement of operational forecasts q. A state of the art dynamical prediction system for seasonal mean and extended range prediction of active -break spells is not operational in the country! q. Together with IMD, IITM plans to set up such a system, evaluate its hind-cast skill and make it operational. q IITM also plans to put in place a strong R&D plan to continuously improve the skill of these forecasts.
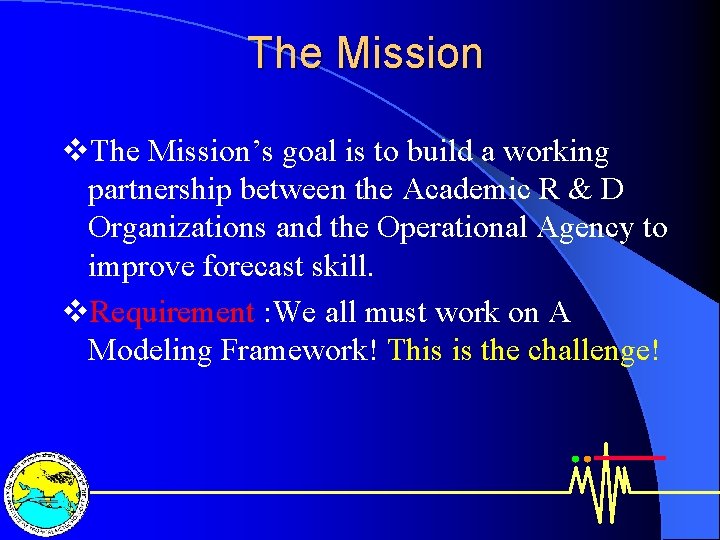
The Mission v. The Mission’s goal is to build a working partnership between the Academic R & D Organizations and the Operational Agency to improve forecast skill. v. Requirement : We all must work on A Modeling Framework! This is the challenge!
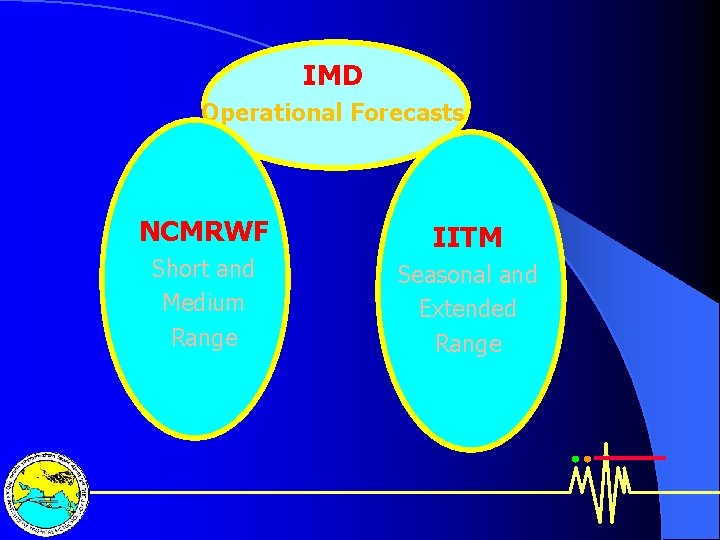
IMD Operational Forecasts NCMRWF Short and Medium Range IITM Seasonal and Extended Range
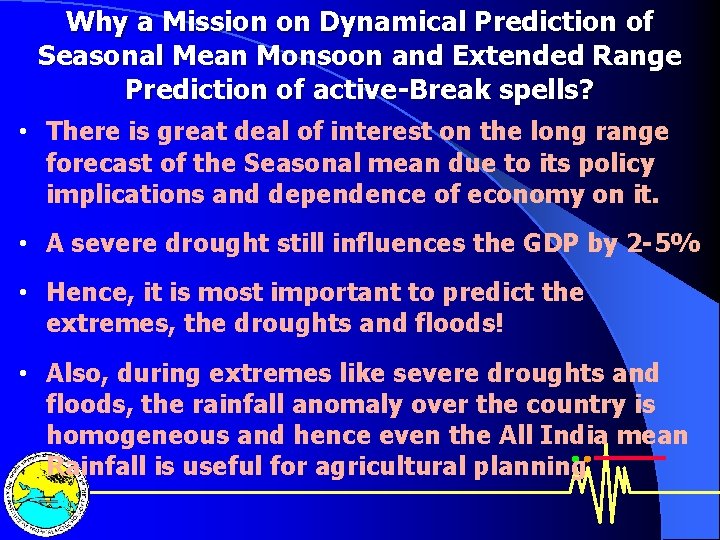
Why a Mission on Dynamical Prediction of Seasonal Mean Monsoon and Extended Range Prediction of active-Break spells? • There is great deal of interest on the long range forecast of the Seasonal mean due to its policy implications and dependence of economy on it. • A severe drought still influences the GDP by 2 -5% • Hence, it is most important to predict the extremes, the droughts and floods! • Also, during extremes like severe droughts and floods, the rainfall anomaly over the country is homogeneous and hence even the All India mean Rainfall is useful for agricultural planning
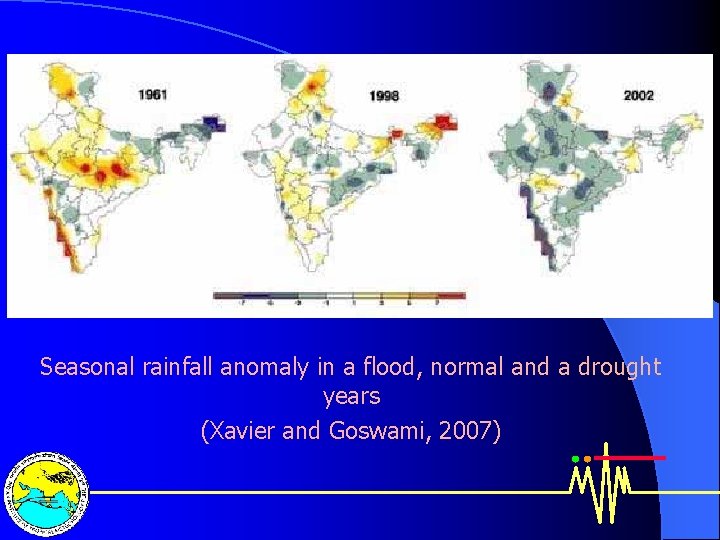
Seasonal rainfall anomaly in a flood, normal and a drought years (Xavier and Goswami, 2007)
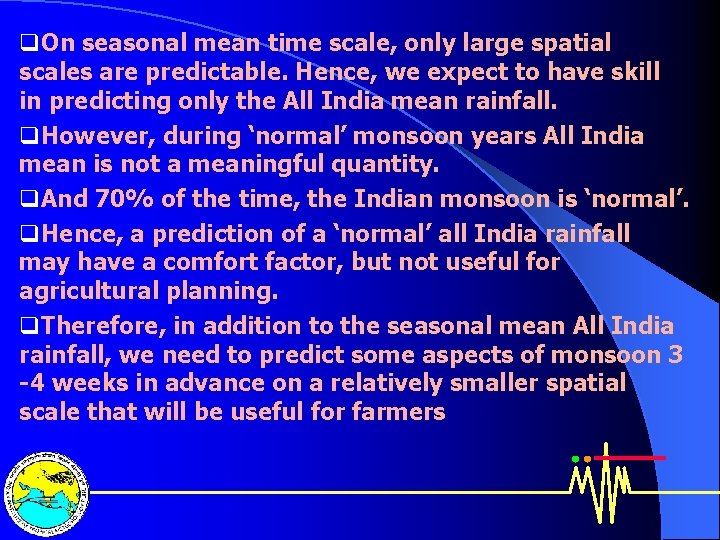
q. On seasonal mean time scale, only large spatial scales are predictable. Hence, we expect to have skill in predicting only the All India mean rainfall. q. However, during ‘normal’ monsoon years All India mean is not a meaningful quantity. q. And 70% of the time, the Indian monsoon is ‘normal’. q. Hence, a prediction of a ‘normal’ all India rainfall may have a comfort factor, but not useful for agricultural planning. q. Therefore, in addition to the seasonal mean All India rainfall, we need to predict some aspects of monsoon 3 -4 weeks in advance on a relatively smaller spatial scale that will be useful for farmers
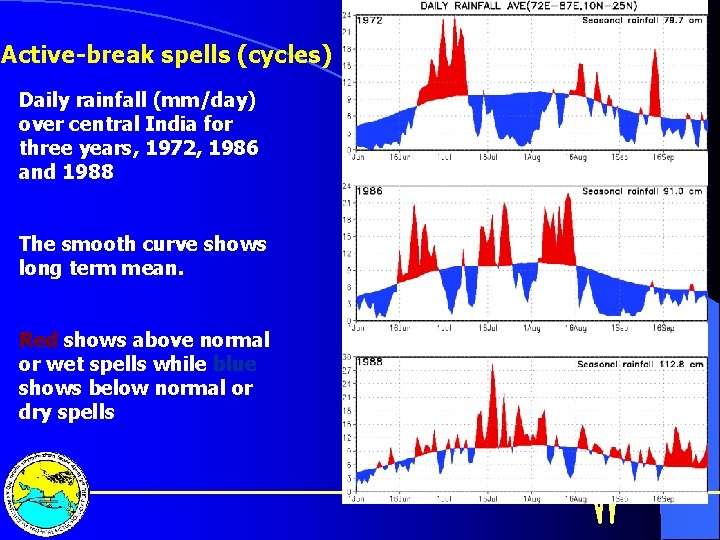
Active-break spells (cycles) Daily rainfall (mm/day) over central India for three years, 1972, 1986 and 1988 The smooth curve shows long term mean. Red shows above normal or wet spells while blue shows below normal or dry spells
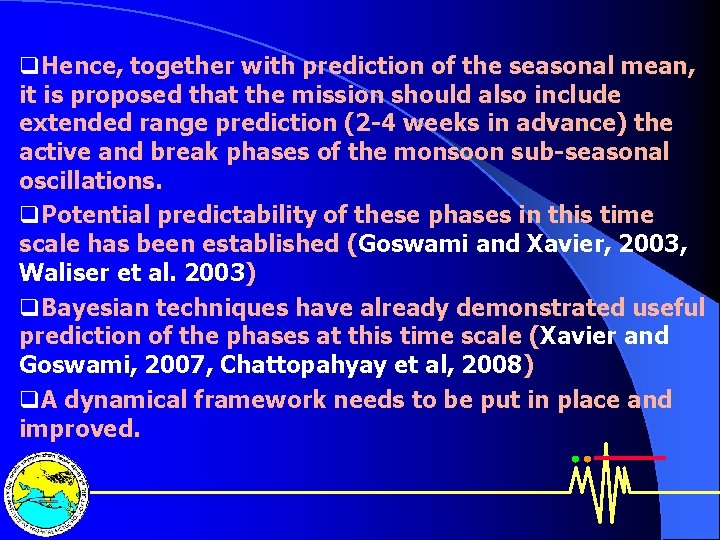
q. Hence, together with prediction of the seasonal mean, it is proposed that the mission should also include extended range prediction (2 -4 weeks in advance) the active and break phases of the monsoon sub-seasonal oscillations. q. Potential predictability of these phases in this time scale has been established (Goswami and Xavier, 2003, Waliser et al. 2003) q. Bayesian techniques have already demonstrated useful prediction of the phases at this time scale (Xavier and Goswami, 2007, Chattopahyay et al, 2008) q. A dynamical framework needs to be put in place and improved.
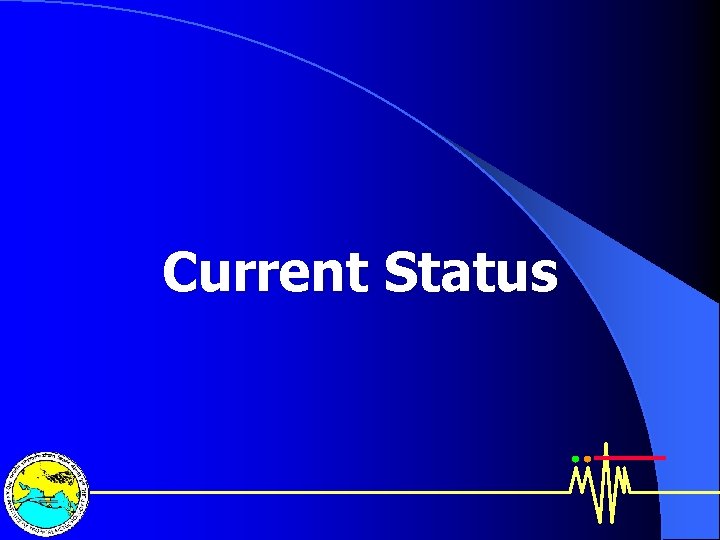
Current Status
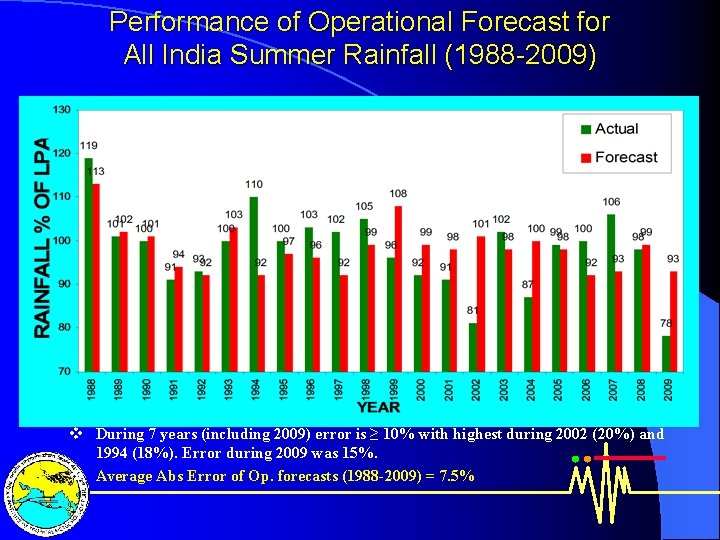
Performance of Operational Forecast for All India Summer Rainfall (1988 -2009) v During 7 years (including 2009) error is ≥ 10% with highest during 2002 (20%) and 1994 (18%). Error during 2009 was 15%. v Average Abs Error of Op. forecasts (1988 -2009) = 7. 5%
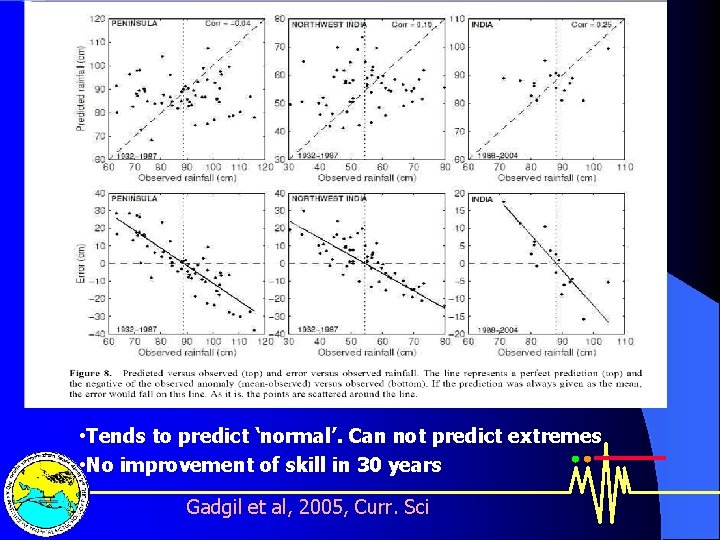
• Tends to predict ‘normal’. Can not predict extremes • No improvement of skill in 30 years Gadgil et al, 2005, Curr. Sci
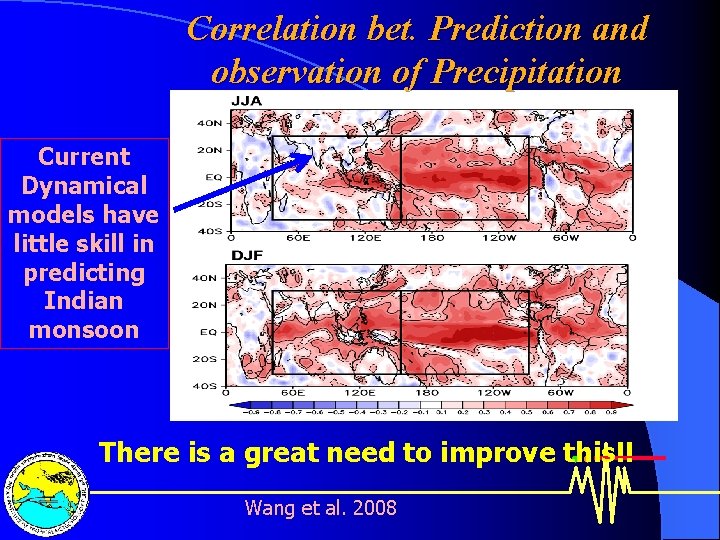
Correlation bet. Prediction and observation of Precipitation Current Dynamical models have little skill in predicting Indian monsoon There is a great need to improve this!! Wang et al. 2008
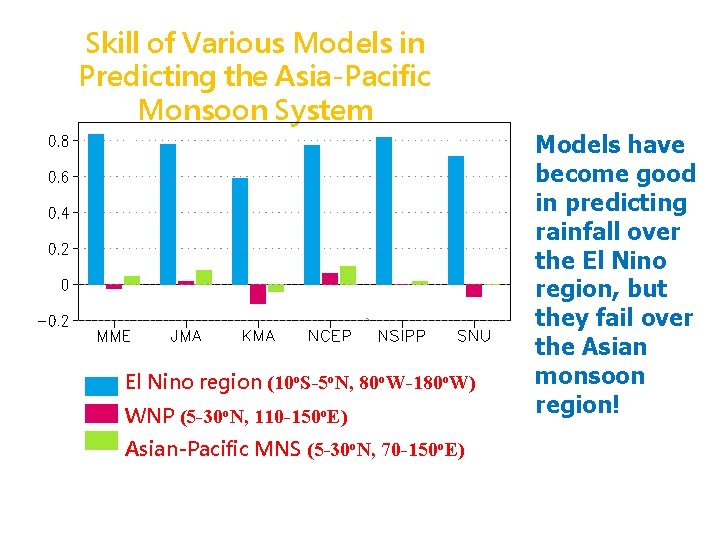
Skill of Various Models in Predicting the Asia-Pacific Monsoon System El Nino region (10 o. S-5 o. N, 80 o. W-180 o. W) WNP (5 -30 o. N, 110 -150 o. E) Asian-Pacific MNS (5 -30 o. N, 70 -150 o. E) Models have become good in predicting rainfall over the El Nino region, but they fail over the Asian monsoon region!
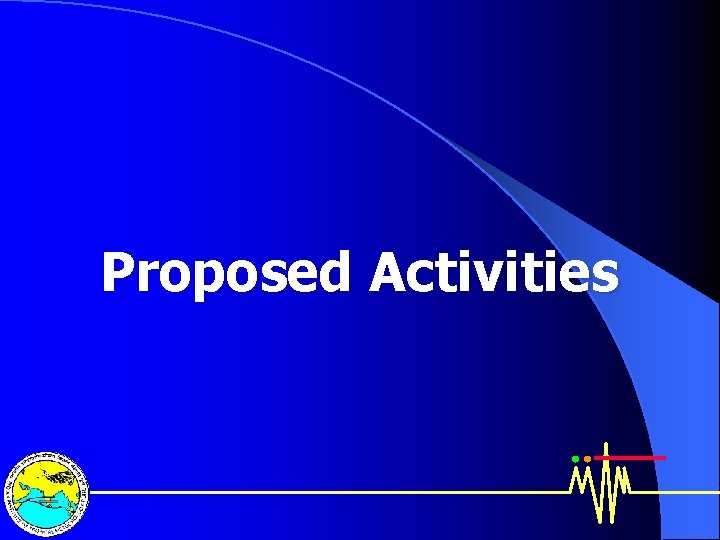
Proposed Activities
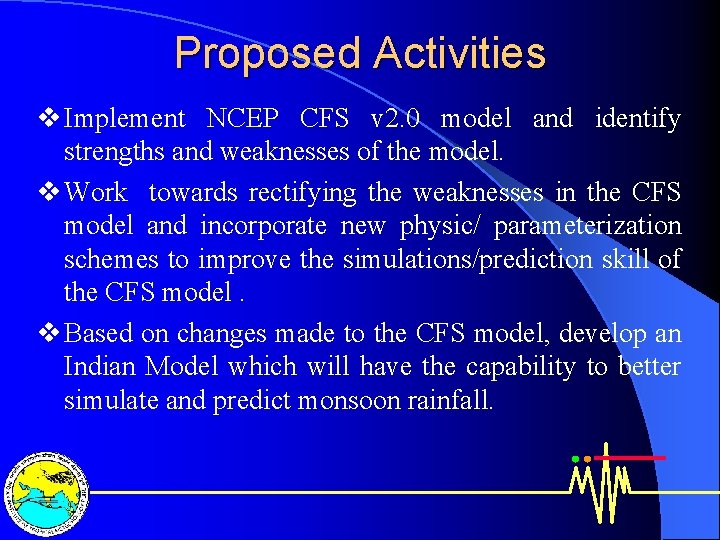
Proposed Activities v Implement NCEP CFS v 2. 0 model and identify strengths and weaknesses of the model. v Work towards rectifying the weaknesses in the CFS model and incorporate new physic/ parameterization schemes to improve the simulations/prediction skill of the CFS model. v Based on changes made to the CFS model, develop an Indian Model which will have the capability to better simulate and predict monsoon rainfall.
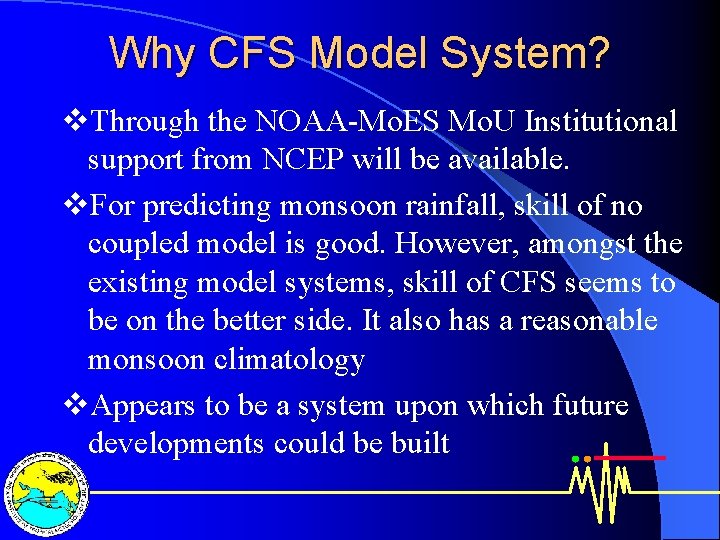
Why CFS Model System? v. Through the NOAA-Mo. ES Mo. U Institutional support from NCEP will be available. v. For predicting monsoon rainfall, skill of no coupled model is good. However, amongst the existing model systems, skill of CFS seems to be on the better side. It also has a reasonable monsoon climatology v. Appears to be a system upon which future developments could be built
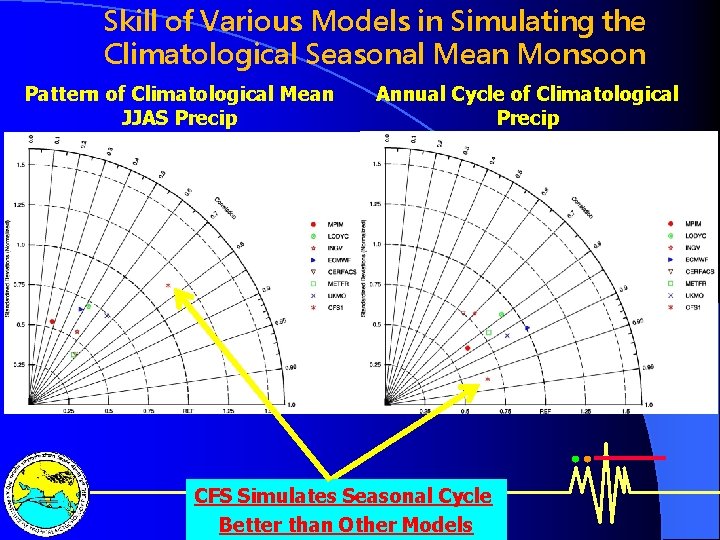
Skill of Various Models in Simulating the Climatological Seasonal Mean Monsoon Pattern of Climatological Mean JJAS Precip Annual Cycle of Climatological Precip CFS Simulates Seasonal Cycle Better than Other Models
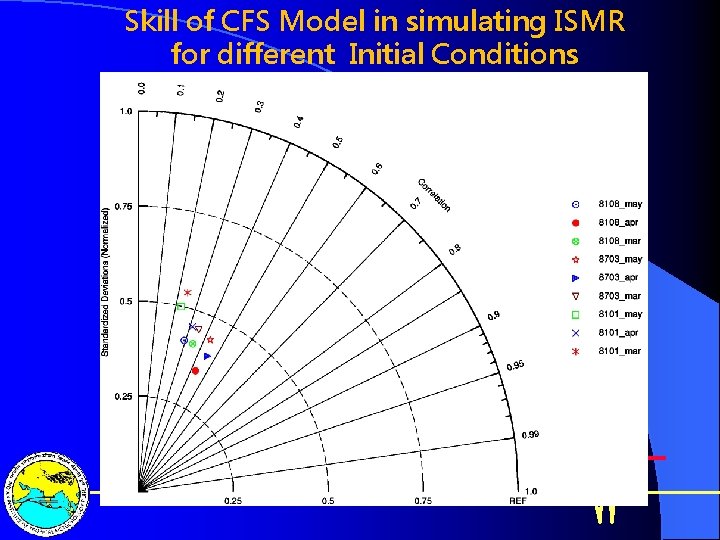
Skill of CFS Model in simulating ISMR for different Initial Conditions
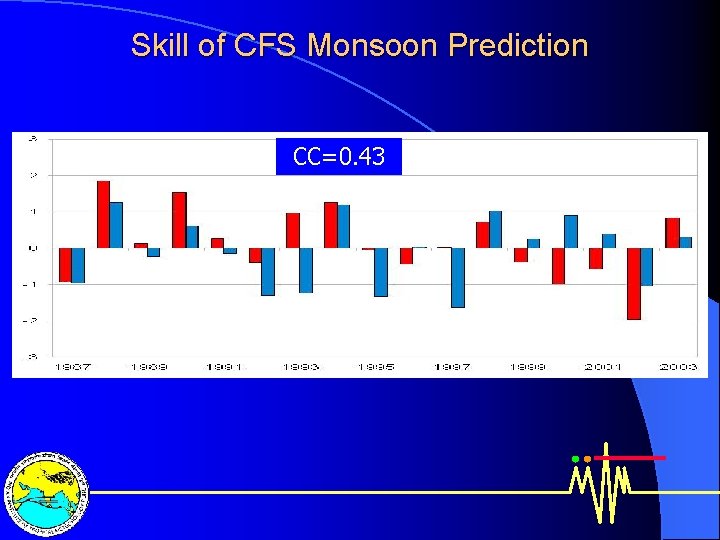
Skill of CFS Monsoon Prediction CC=0. 43
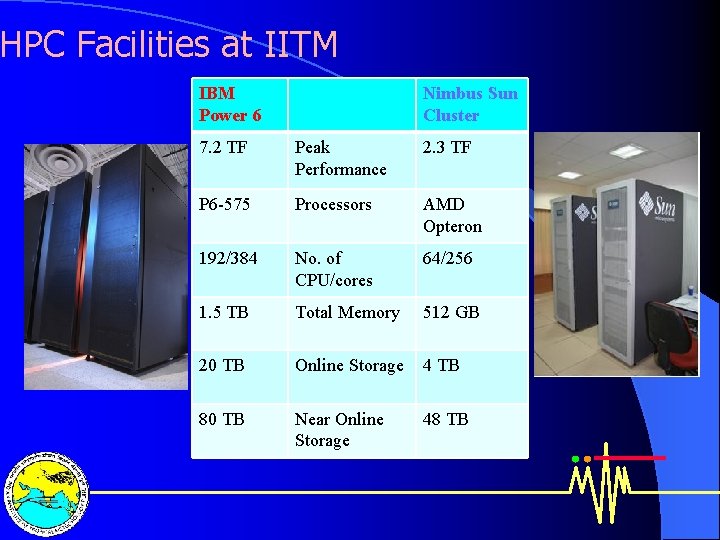
HPC Facilities at IITM IBM Power 6 Nimbus Sun Cluster 7. 2 TF Peak Performance 2. 3 TF P 6 -575 Processors AMD Opteron 192/384 No. of CPU/cores 64/256 1. 5 TB Total Memory 512 GB 20 TB Online Storage 4 TB 80 TB Near Online Storage 48 TB
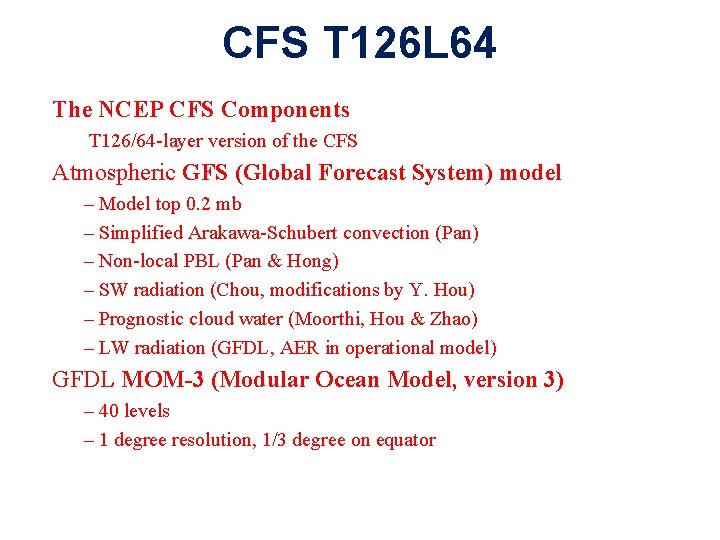
CFS T 126 L 64 • The NCEP CFS Components – T 126/64 -layer version of the CFS • Atmospheric GFS (Global Forecast System) model – – – – Model top 0. 2 mb – Simplified Arakawa-Schubert convection (Pan) – Non-local PBL (Pan & Hong) – SW radiation (Chou, modifications by Y. Hou) – Prognostic cloud water (Moorthi, Hou & Zhao) – LW radiation (GFDL, AER in operational model) • GFDL MOM-3 (Modular Ocean Model, version 3) – – 40 levels – – 1 degree resolution, 1/3 degree on equator
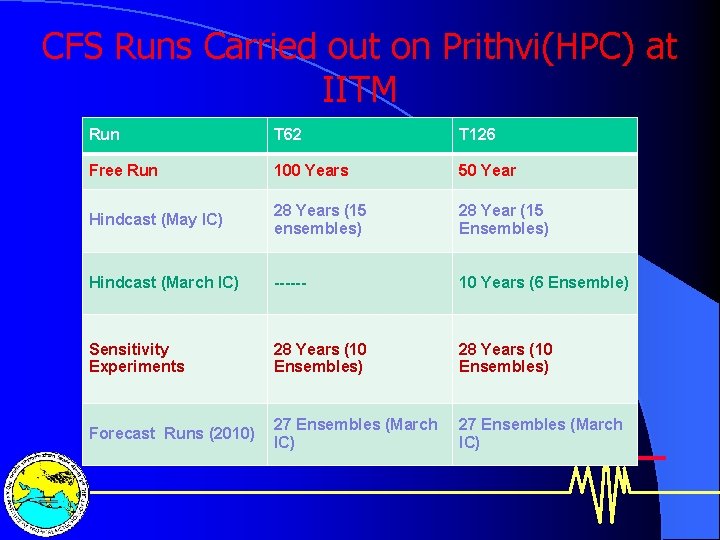
CFS Runs Carried out on Prithvi(HPC) at IITM Run T 62 T 126 Free Run 100 Years 50 Year Hindcast (May IC) 28 Years (15 ensembles) 28 Year (15 Ensembles) Hindcast (March IC) ------ 10 Years (6 Ensemble) Sensitivity Experiments 28 Years (10 Ensembles) Forecast Runs (2010) 27 Ensembles (March IC)
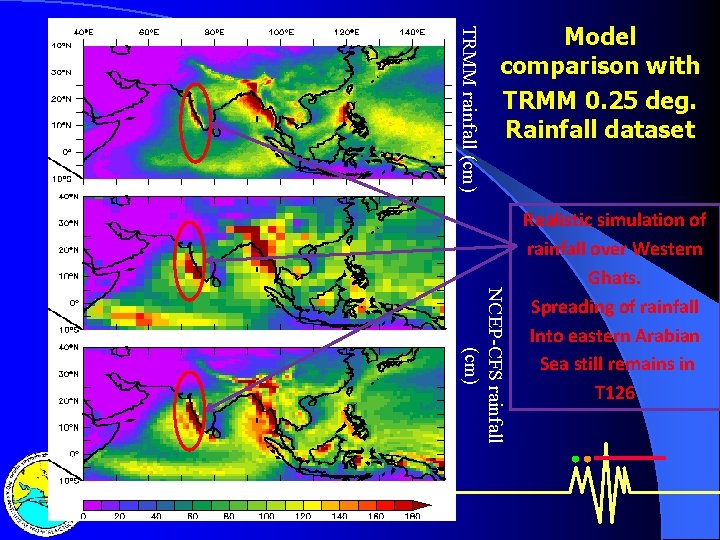
TRMM rainfall (cm) Model comparison with TRMM 0. 25 deg. Rainfall dataset NCEP-CFS rainfall (cm) Realistic simulation of rainfall over Western Ghats. Spreading of rainfall Into eastern Arabian Sea still remains in T 126
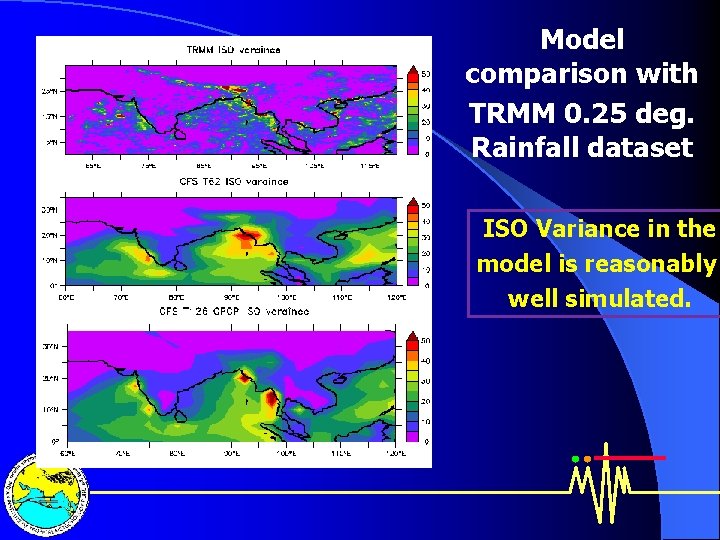
Model comparison with TRMM 0. 25 deg. Rainfall dataset ISO Variance in the model is reasonably well simulated.
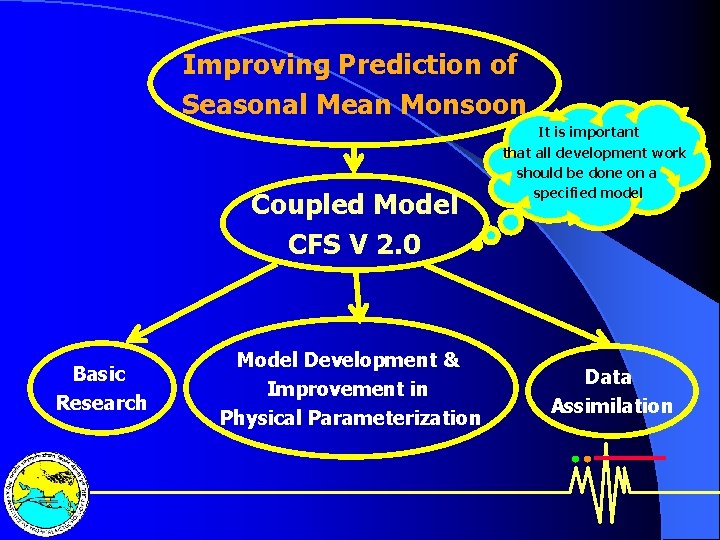
Improving Prediction of Seasonal Mean Monsoon Coupled Model CFS V 2. 0 Basic Research Model Development & Improvement in Physical Parameterization It is important that all development work should be done on a specified model Data Assimilation
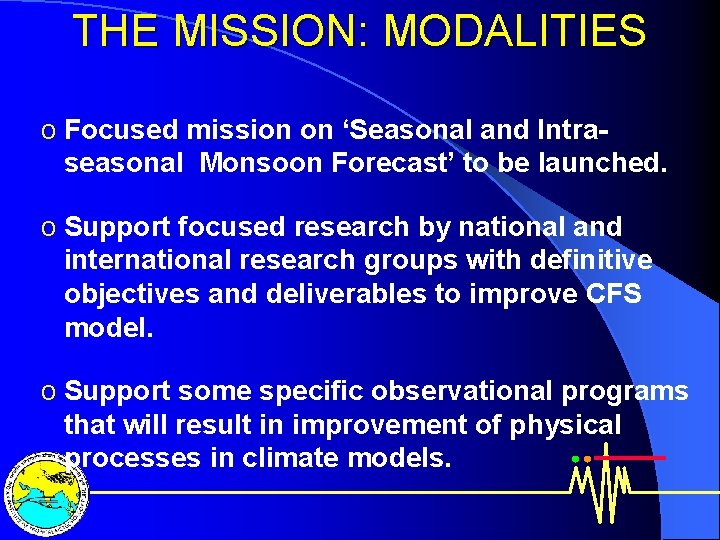
THE MISSION: MODALITIES o Focused mission on ‘Seasonal and Intraseasonal Monsoon Forecast’ to be launched. o Support focused research by national and international research groups with definitive objectives and deliverables to improve CFS model. o Support some specific observational programs that will result in improvement of physical processes in climate models.
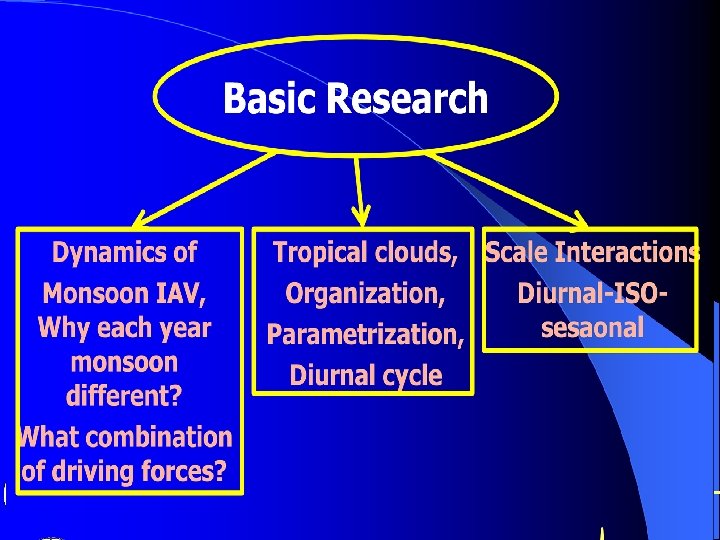
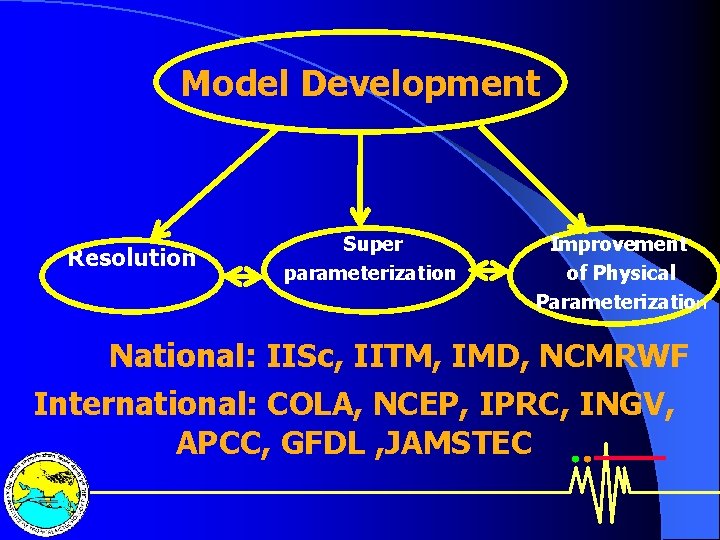
Model Development Resolution Super parameterization Improvement of Physical Parameterization National: IISc, IITM, IMD, NCMRWF International: COLA, NCEP, IPRC, INGV, APCC, GFDL , JAMSTEC
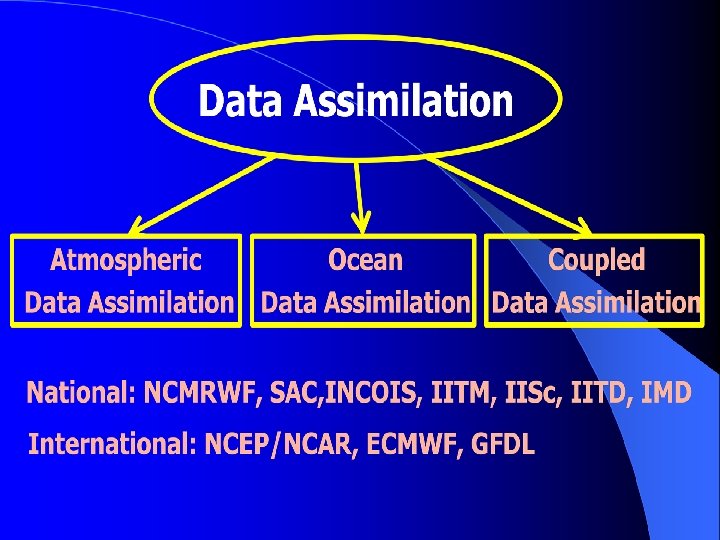
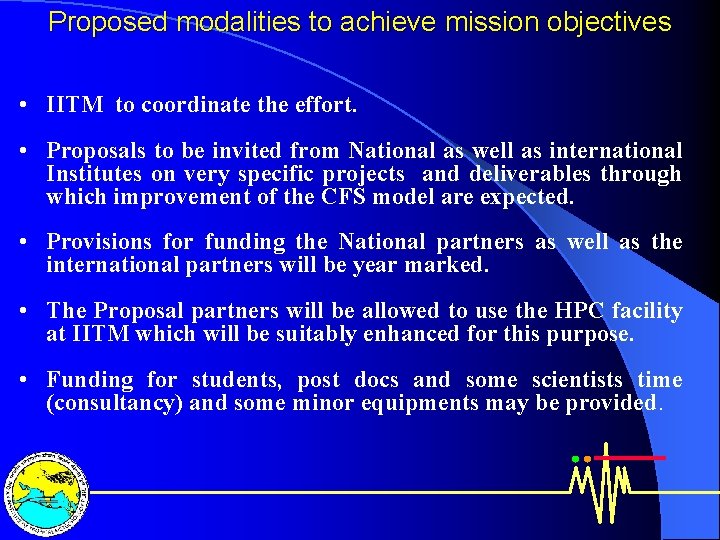
Proposed modalities to achieve mission objectives • IITM to coordinate the effort. • Proposals to be invited from National as well as international Institutes on very specific projects and deliverables through which improvement of the CFS model are expected. • Provisions for funding the National partners as well as the international partners will be year marked. • The Proposal partners will be allowed to use the HPC facility at IITM which will be suitably enhanced for this purpose. • Funding for students, post docs and some scientists time (consultancy) and some minor equipments may be provided.
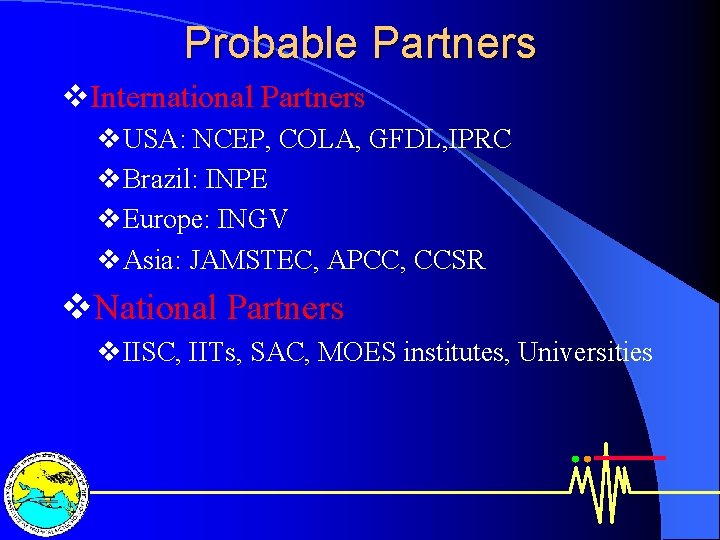
Probable Partners v. International Partners v. USA: NCEP, COLA, GFDL, IPRC v. Brazil: INPE v. Europe: INGV v. Asia: JAMSTEC, APCC, CCSR v. National Partners v. IISC, IITs, SAC, MOES institutes, Universities
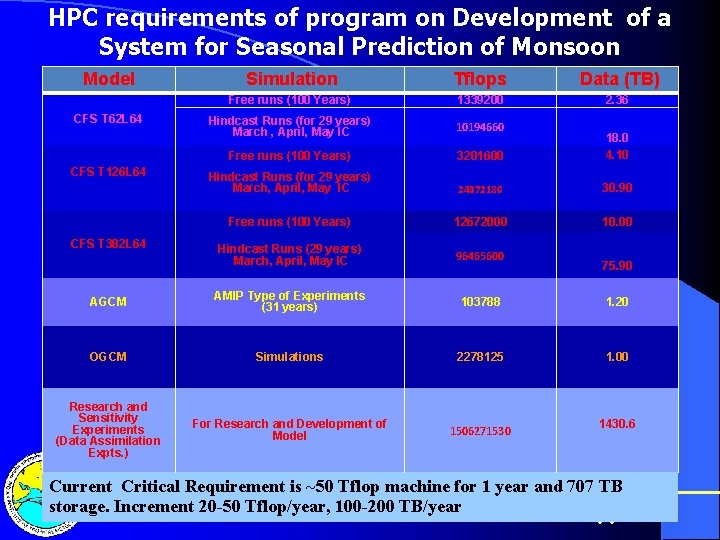
HPC requirements of program on Development of a System for Seasonal Prediction of Monsoon Model Simulation Tflops Data (TB) Free runs (100 Years) 1339200 2. 36 Hindcast Runs (for 29 years) March , April, May IC 10194660 Free runs (100 Years) 3201600 18. 0 4. 10 Hindcast Runs (for 29 years) March, April, May IC 24372180 30. 90 Free runs (100 Years) 12672000 10. 00 Hindcast Runs (29 years) March, April, May IC 96465600 AGCM AMIP Type of Experiments (31 years) 103788 1. 20 OGCM Simulations 2278125 1. 00 Research and Sensitivity Experiments (Data Assimilation Expts. ) For Research and Development of Model 1506271530 CFS T 62 L 64 CFS T 126 L 64 CFS T 382 L 64 75. 90 1430. 6 Current Critical Requirement is ~50 Tflop machine for 1 year and 707 TB storage. Increment 20 -50 Tflop/year, 100 -200 TB/year
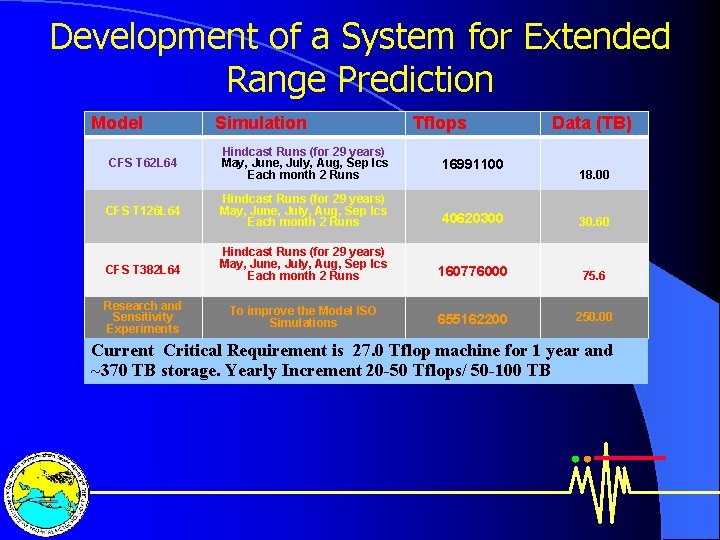
Development of a System for Extended Range Prediction Model Simulation Tflops Data (TB) CFS T 62 L 64 Hindcast Runs (for 29 years) May, June, July, Aug, Sep Ics Each month 2 Runs CFS T 126 L 64 Hindcast Runs (for 29 years) May, June, July, Aug, Sep Ics Each month 2 Runs 40620300 30. 60 CFS T 382 L 64 Hindcast Runs (for 29 years) May, June, July, Aug, Sep Ics Each month 2 Runs 160776000 75. 6 Research and Sensitivity Experiments To improve the Model ISO Simulations 655162200 250. 00 16991100 18. 00 Current Critical Requirement is 27. 0 Tflop machine for 1 year and ~370 TB storage. Yearly Increment 20 -50 Tflops/ 50 -100 TB
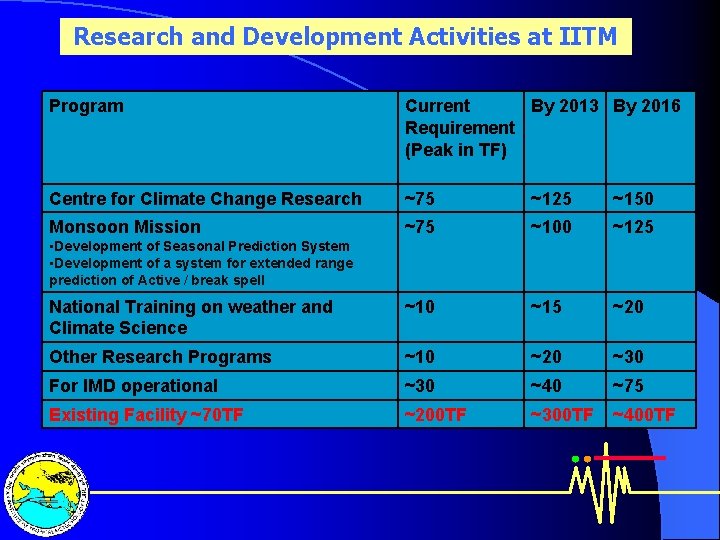
Research and Development Activities at IITM Program Current By 2013 By 2016 Requirement (Peak in TF) Centre for Climate Change Research ~75 ~125 ~150 Monsoon Mission ~75 ~100 ~125 National Training on weather and Climate Science ~10 ~15 ~20 Other Research Programs ~10 ~20 ~30 For IMD operational ~30 ~40 ~75 Existing Facility ~70 TF ~200 TF ~300 TF ~400 TF • Development of Seasonal Prediction System • Development of a system for extended range prediction of Active / break spell
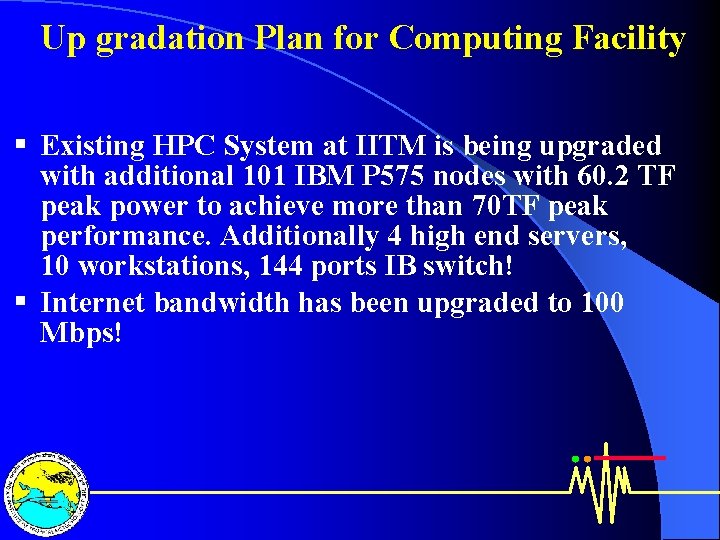
Up gradation Plan for Computing Facility § Existing HPC System at IITM is being upgraded with additional 101 IBM P 575 nodes with 60. 2 TF peak power to achieve more than 70 TF peak performance. Additionally 4 high end servers, 10 workstations, 144 ports IB switch! § Internet bandwidth has been upgraded to 100 Mbps!
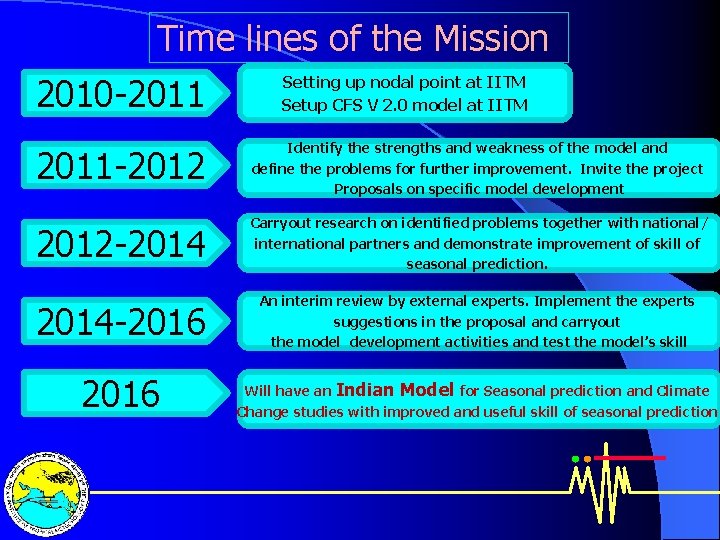
Time lines of the Mission 2010 -2011 Setting up nodal point at IITM Setup CFS V 2. 0 model at IITM 2011 -2012 Identify the strengths and weakness of the model and define the problems for further improvement. Invite the project Proposals on specific model development 2012 -2014 Carryout research on identified problems together with national/ international partners and demonstrate improvement of skill of seasonal prediction. 2014 -2016 An interim review by external experts. Implement the experts suggestions in the proposal and carryout the model development activities and test the model’s skill 2016 Will have an Indian Model for Seasonal prediction and Climate Change studies with improved and useful skill of seasonal prediction
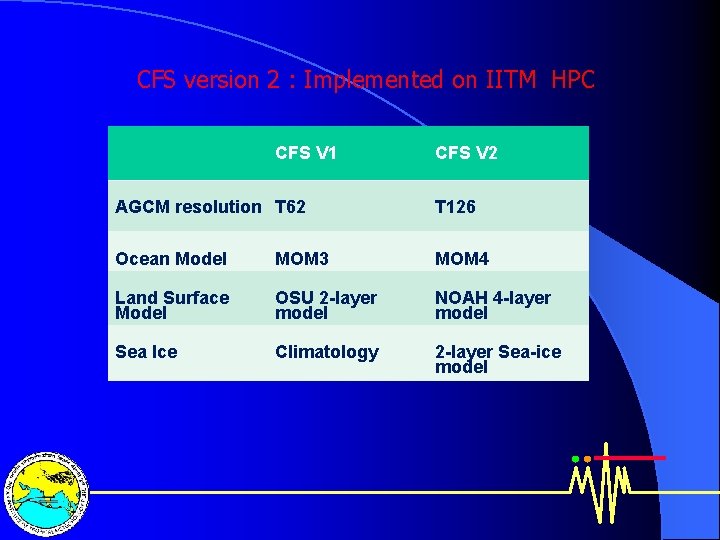
CFS version 2 : Implemented on IITM HPC CFS V 1 CFS V 2 AGCM resolution T 62 T 126 Ocean Model MOM 3 MOM 4 Land Surface Model OSU 2 -layer model NOAH 4 -layer model Sea Ice Climatology 2 -layer Sea-ice model
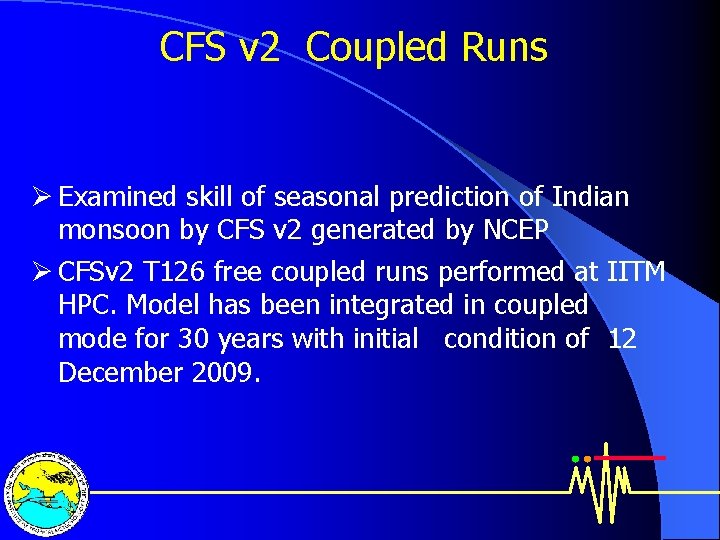
CFS v 2 Coupled Runs Ø Examined skill of seasonal prediction of Indian monsoon by CFS v 2 generated by NCEP Ø CFSv 2 T 126 free coupled runs performed at IITM HPC. Model has been integrated in coupled mode for 30 years with initial condition of 12 December 2009.
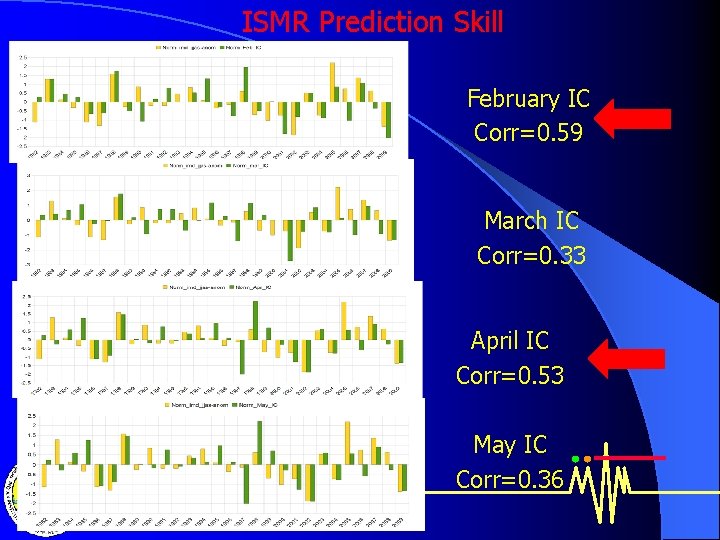
ISMR Prediction Skill February IC Corr=0. 59 March IC Corr=0. 33 April IC Corr=0. 53 May IC Corr=0. 36
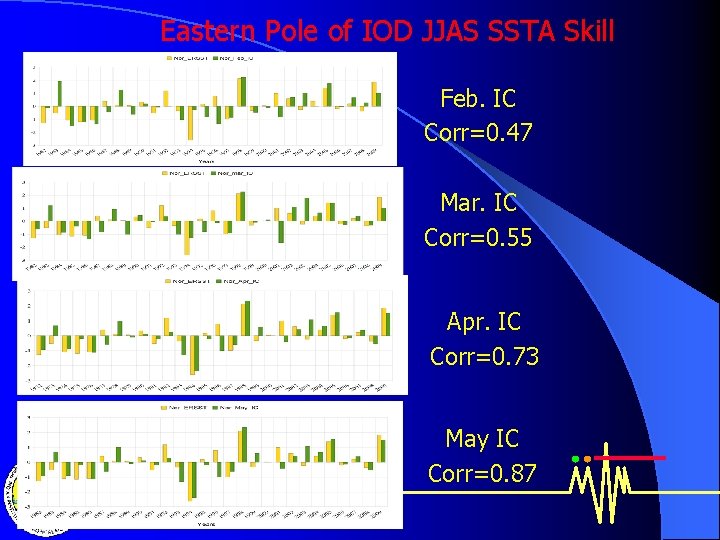
Eastern Pole of IOD JJAS SSTA Skill Feb. IC Corr=0. 47 Mar. IC Corr=0. 55 Apr. IC Corr=0. 73 May IC Corr=0. 87
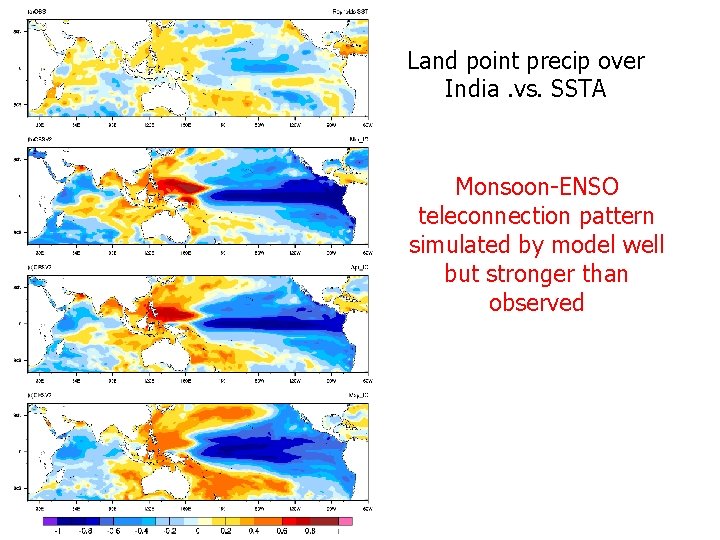
Land point precip over India. vs. SSTA Monsoon-ENSO teleconnection pattern simulated by model well but stronger than observed
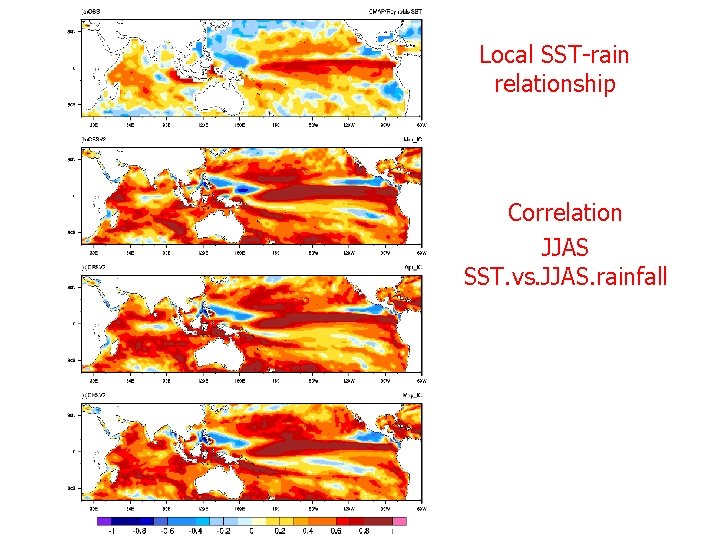
Local SST-rain relationship Correlation JJAS SST. vs. JJAS. rainfall
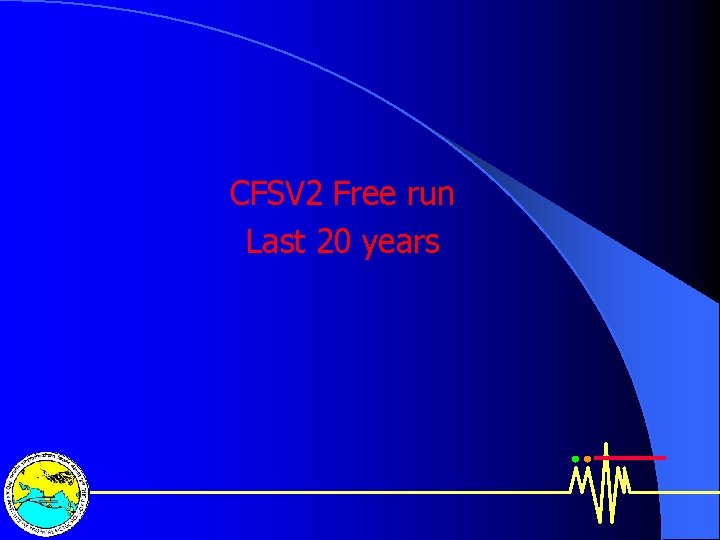
CFSV 2 Free run Last 20 years
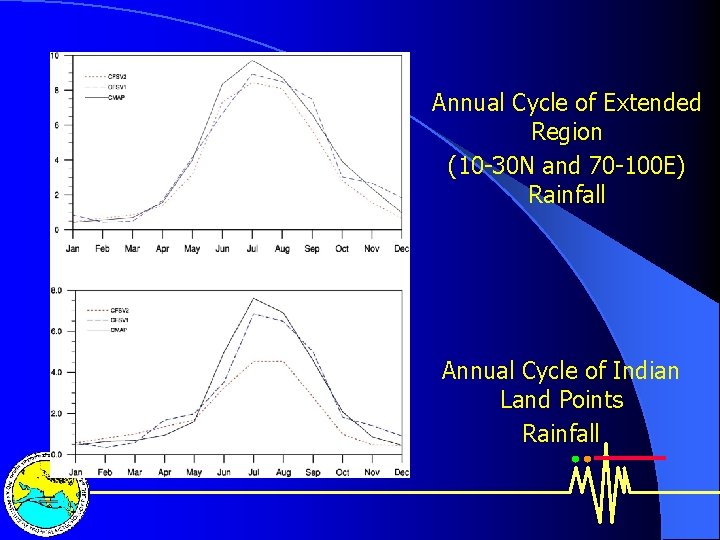
Annual Cycle of Extended Region (10 -30 N and 70 -100 E) Rainfall Annual Cycle of Indian Land Points Rainfall
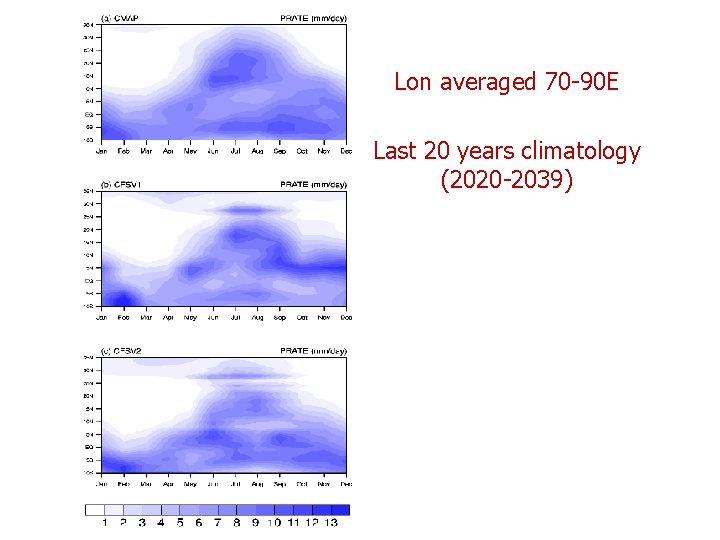
Lon averaged 70 -90 E Last 20 years climatology (2020 -2039)
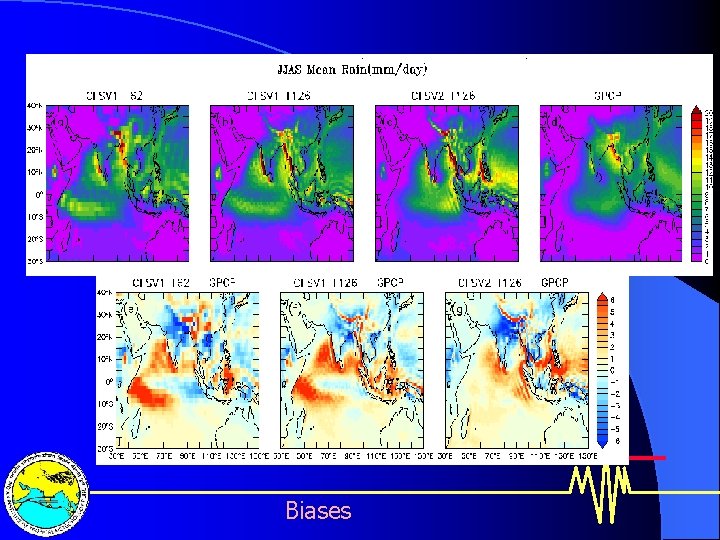
Biases
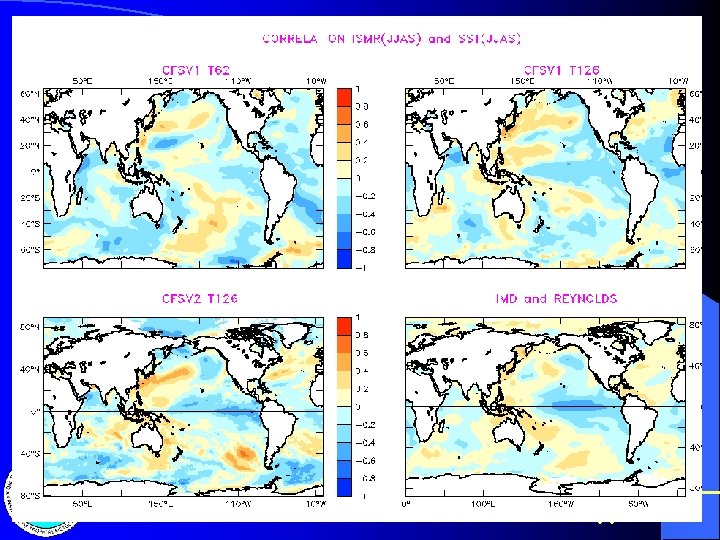
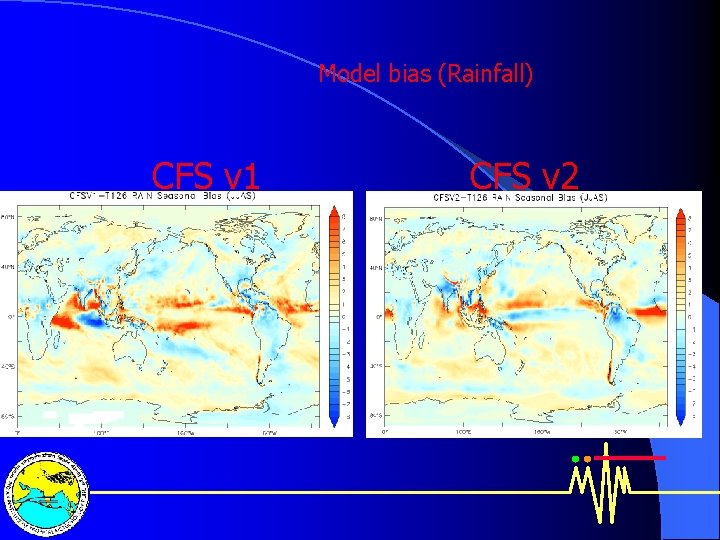
Model bias (Rainfall) CFS v 1 CFS v 2
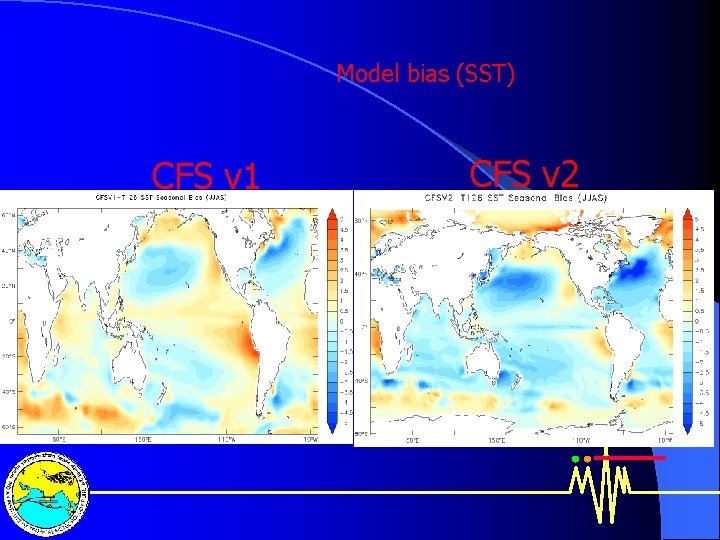
Model bias (SST) CFS v 1 CFS v 2
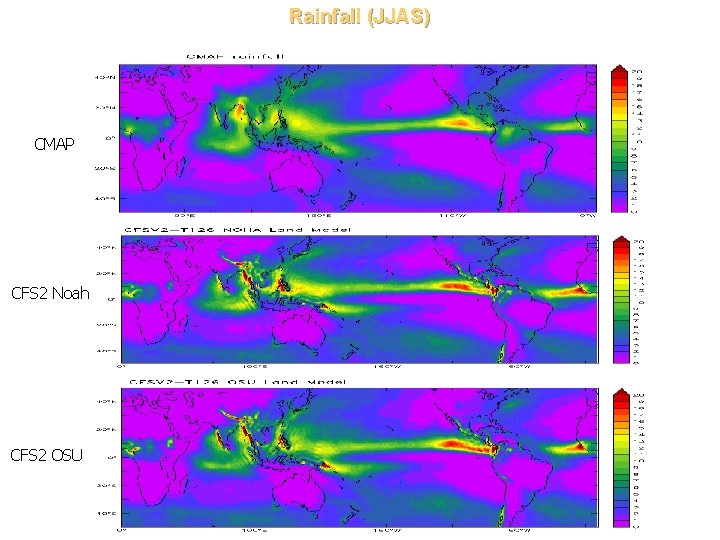
Rainfall (JJAS) CMAP CFS 2 Noah CFS 2 OSU
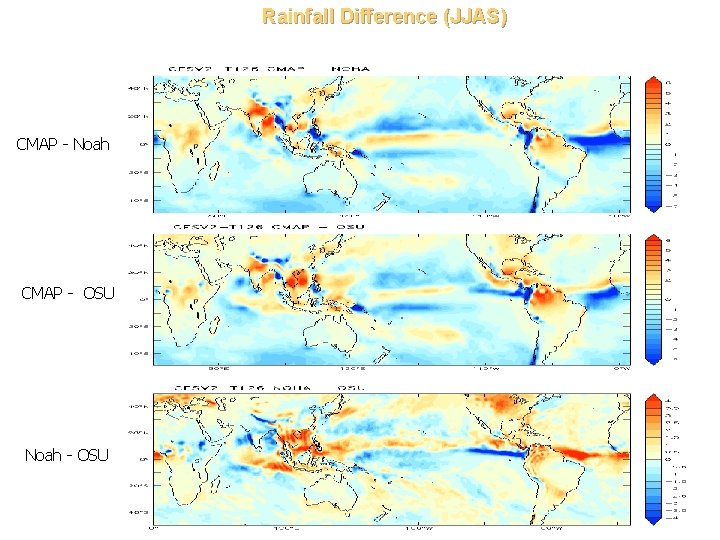
Rainfall Difference (JJAS) CMAP - Noah CMAP - OSU Noah - OSU
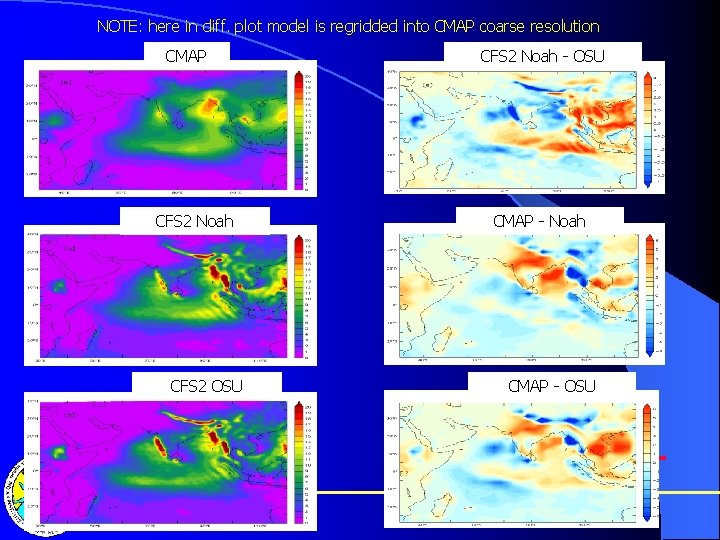
NOTE: here in diff. plot model is regridded into CMAP coarse resolution CMAP CFS 2 Noah CFS 2 OSU CFS 2 Noah - OSU CMAP - Noah CMAP - OSU
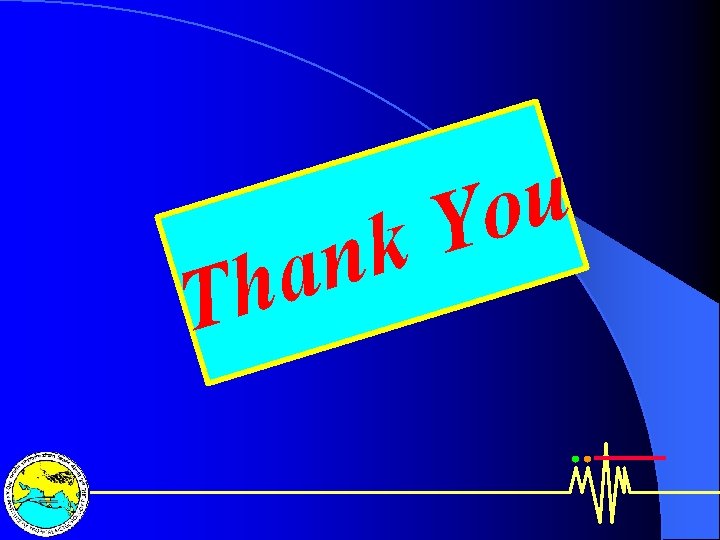
k n a h T u o Y
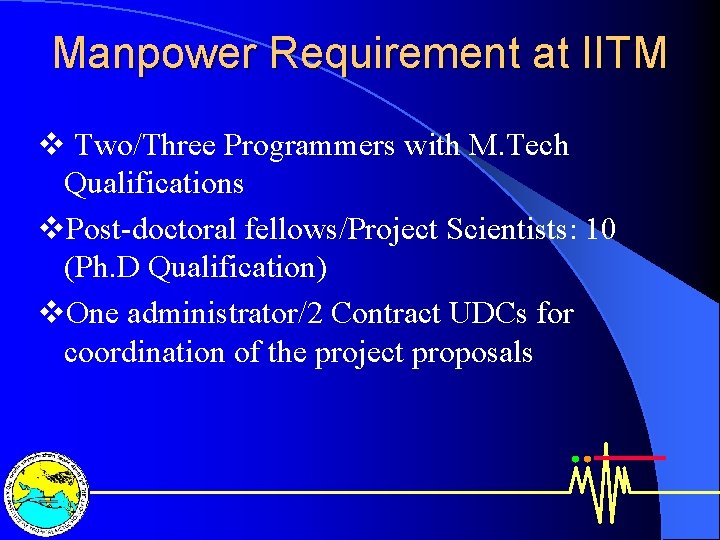
Manpower Requirement at IITM v Two/Three Programmers with M. Tech Qualifications v. Post-doctoral fellows/Project Scientists: 10 (Ph. D Qualification) v. One administrator/2 Contract UDCs for coordination of the project proposals
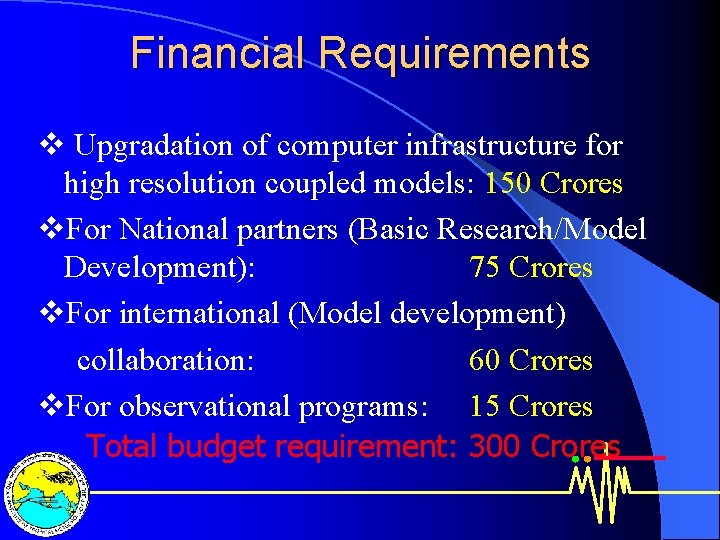
Financial Requirements v Upgradation of computer infrastructure for high resolution coupled models: 150 Crores v. For National partners (Basic Research/Model Development): 75 Crores v. For international (Model development) collaboration: 60 Crores v. For observational programs: 15 Crores Total budget requirement: 300 Crores
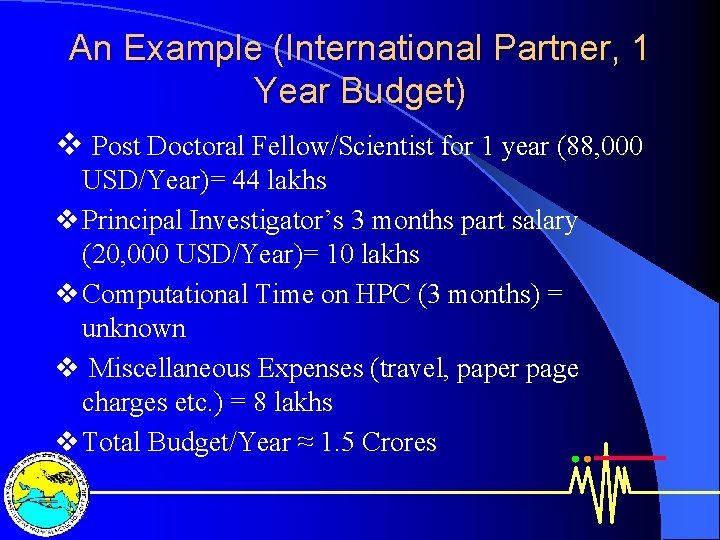
An Example (International Partner, 1 Year Budget) v Post Doctoral Fellow/Scientist for 1 year (88, 000 USD/Year)= 44 lakhs v Principal Investigator’s 3 months part salary (20, 000 USD/Year)= 10 lakhs v Computational Time on HPC (3 months) = unknown v Miscellaneous Expenses (travel, paper page charges etc. ) = 8 lakhs v Total Budget/Year ≈ 1. 5 Crores
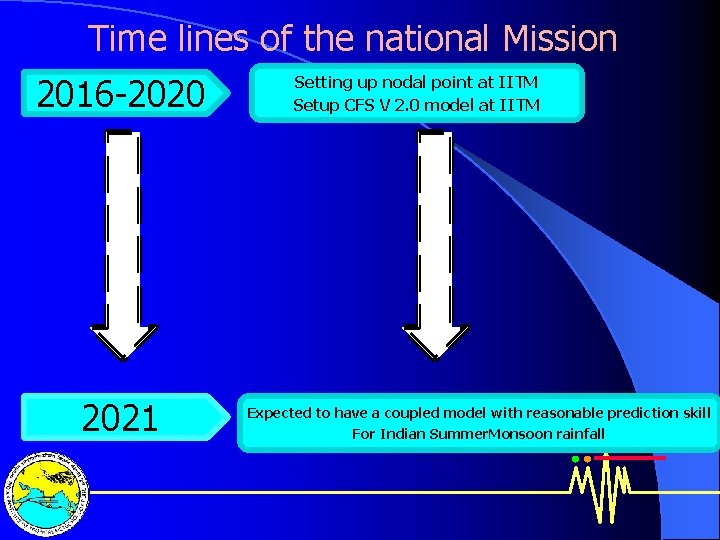
Time lines of the national Mission 2016 -2020 2021 Setting up nodal point at IITM Setup CFS V 2. 0 model at IITM Expected to have a coupled model with reasonable prediction skill For Indian Summer. Monsoon rainfall
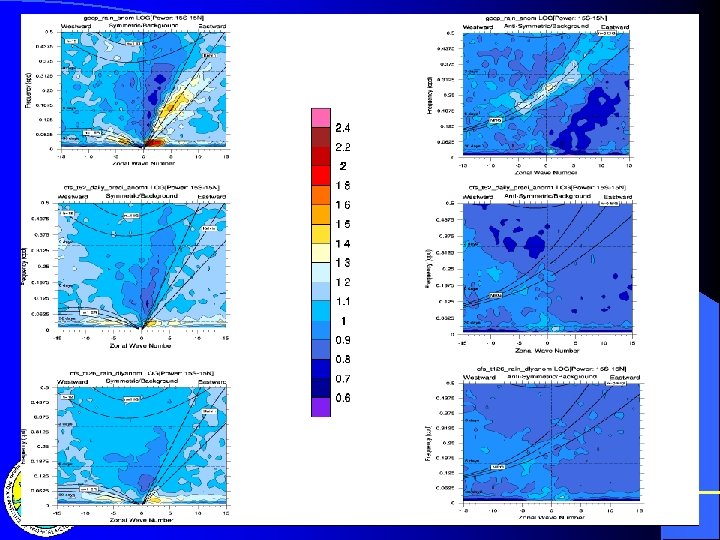
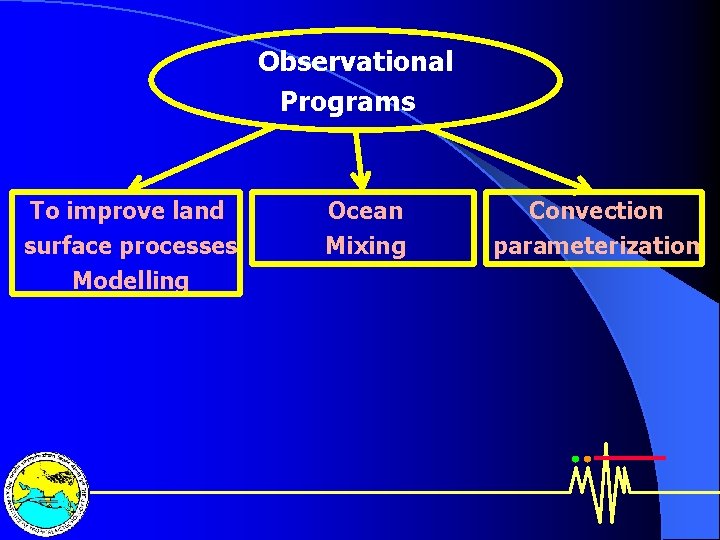
Observational Programs To improve land surface processes Modelling Ocean Mixing Convection parameterization
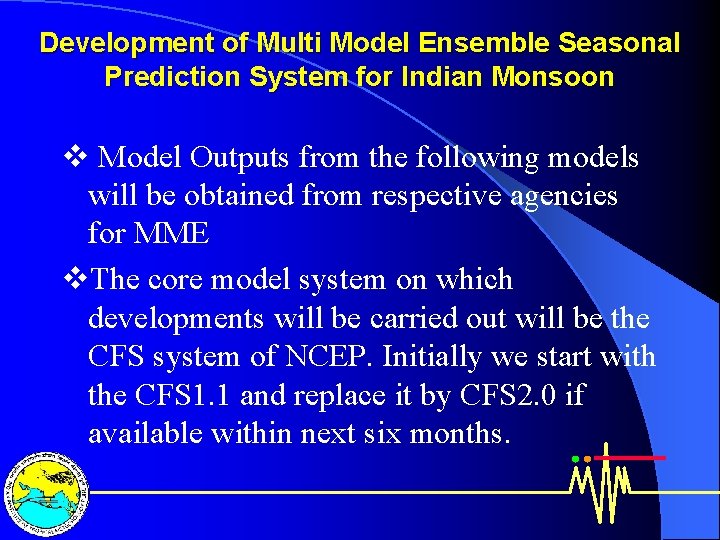
Development of Multi Model Ensemble Seasonal Prediction System for Indian Monsoon v Model Outputs from the following models will be obtained from respective agencies for MME v. The core model system on which developments will be carried out will be the CFS system of NCEP. Initially we start with the CFS 1. 1 and replace it by CFS 2. 0 if available within next six months.
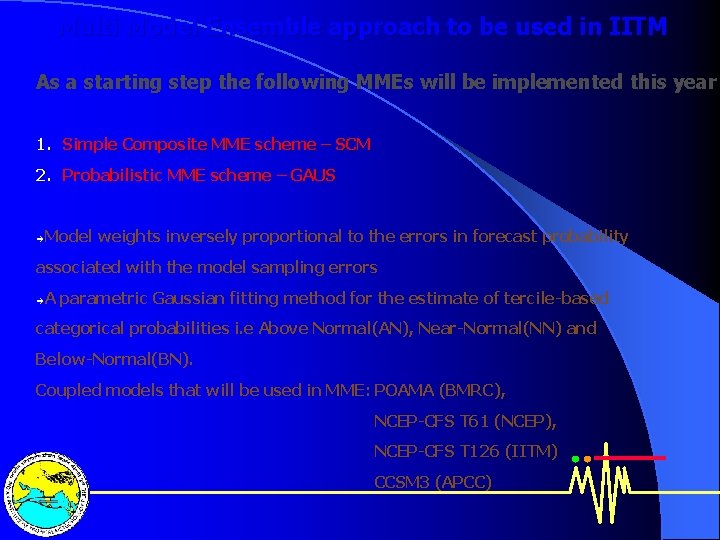
Multi Model Ensemble approach to be used in IITM As a starting step the following MMEs will be implemented this year 1. Simple Composite MME scheme – SCM 2. Probabilistic MME scheme – GAUS Model weights inversely proportional to the errors in forecast probability associated with the model sampling errors A parametric Gaussian fitting method for the estimate of tercile-based categorical probabilities i. e Above Normal(AN), Near-Normal(NN) and Below-Normal(BN). Coupled models that will be used in MME: POAMA (BMRC), NCEP-CFS T 61 (NCEP), NCEP-CFS T 126 (IITM) CCSM 3 (APCC)
A mode b mode m mode
National monsoon mission
Focus mode and diffuse mode
Mode địa chỉ tức thì là mode
Difference between real protected and virtual mode of 80386
Different modes of operation of timer in 8051
Gartner mode 1 mode 2
Perbedaan (planning mode) dan (evolutionary mode)
8086 vs 8088
Monsoon forest
Monsoon marketplace pricing
Arid climograph
African monsoon
Monoplane occlusion definition
Asia seasonal forecast
Monsoon
Monsoon expedition monex
Monsoon desk 2008
Monsoon desk
Southwest monsoon
Monsoon climate characteristics
Southwest monsoon singapore
Pathra dathuwa
Monsoon boys
The climate of india is strongly influenced by dash
Primate space
Stone edge software
Hát kết hợp bộ gõ cơ thể
Ng-html
Bổ thể
Tỉ lệ cơ thể trẻ em
Gấu đi như thế nào
Tư thế worm breton là gì
Chúa sống lại
Các môn thể thao bắt đầu bằng tiếng bóng
Thế nào là hệ số cao nhất
Các châu lục và đại dương trên thế giới
Công thức tính thế năng
Trời xanh đây là của chúng ta thể thơ
Mật thư anh em như thể tay chân
Làm thế nào để 102-1=99
độ dài liên kết
Các châu lục và đại dương trên thế giới
Thơ thất ngôn tứ tuyệt đường luật
Quá trình desamine hóa có thể tạo ra
Một số thể thơ truyền thống
Cái miệng bé xinh thế chỉ nói điều hay thôi
Vẽ hình chiếu vuông góc của vật thể sau
Thế nào là sự mỏi cơ
đặc điểm cơ thể của người tối cổ
V. c c
Vẽ hình chiếu đứng bằng cạnh của vật thể
Tia chieu sa te
Thẻ vin
đại từ thay thế
điện thế nghỉ
Tư thế ngồi viết
Diễn thế sinh thái là
Các loại đột biến cấu trúc nhiễm sắc thể
So nguyen to
Tư thế ngồi viết
Lời thề hippocrates
Thiếu nhi thế giới liên hoan
ưu thế lai là gì