Toward an Understanding of the Economics of Apologies
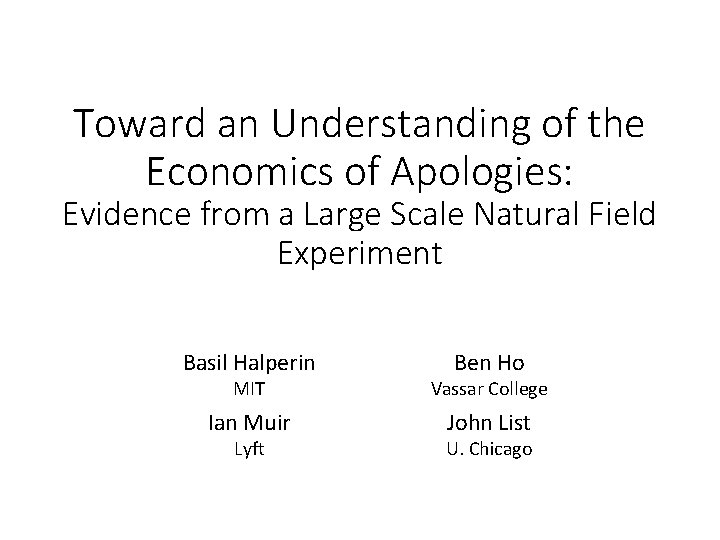
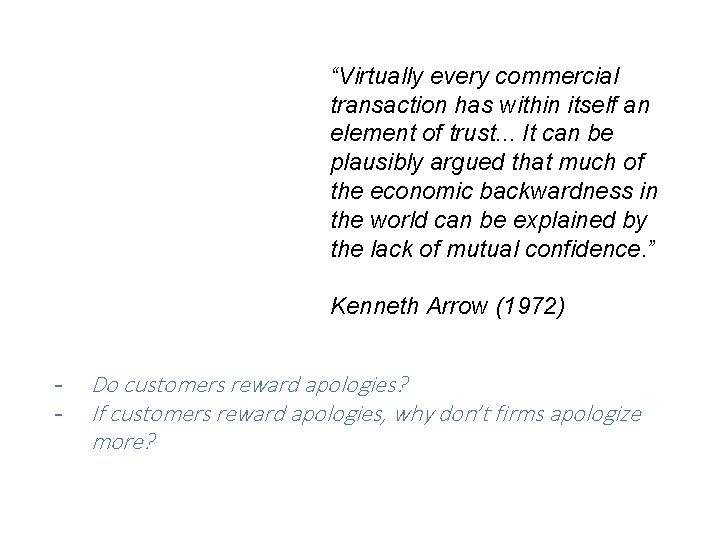
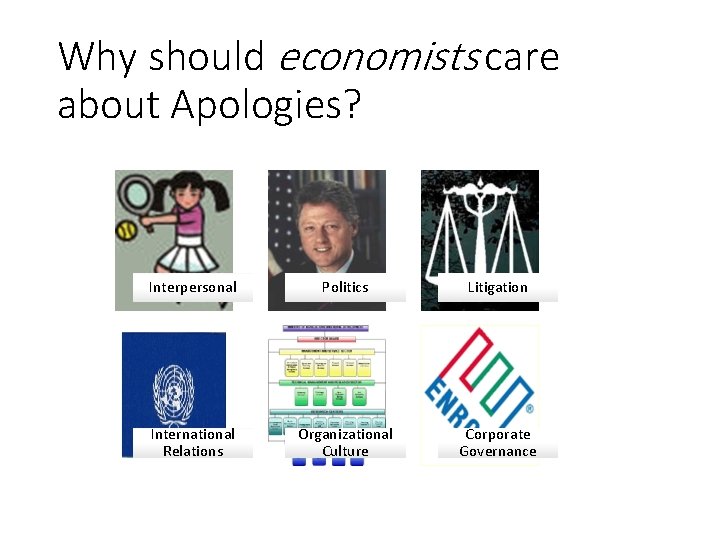
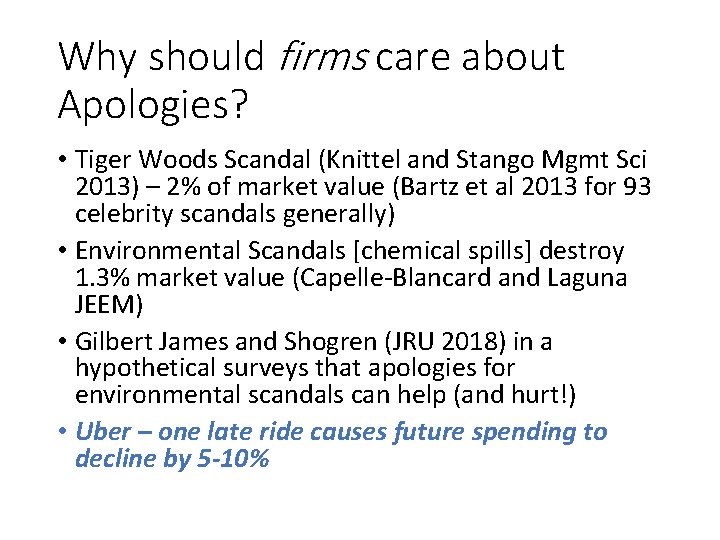
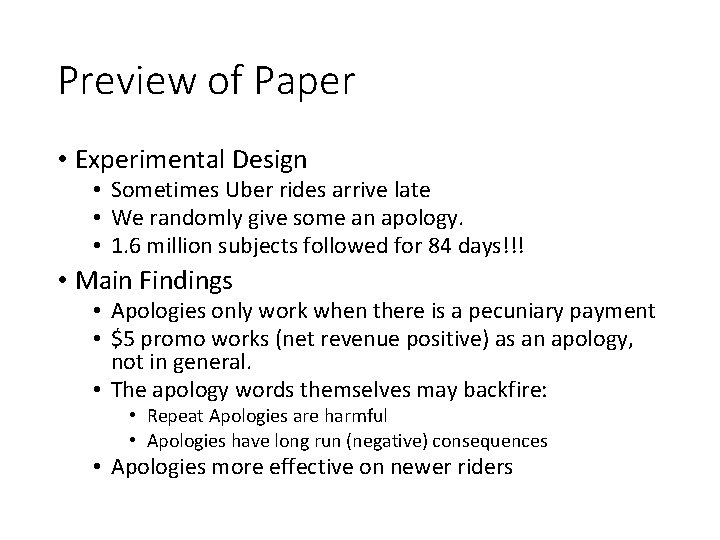
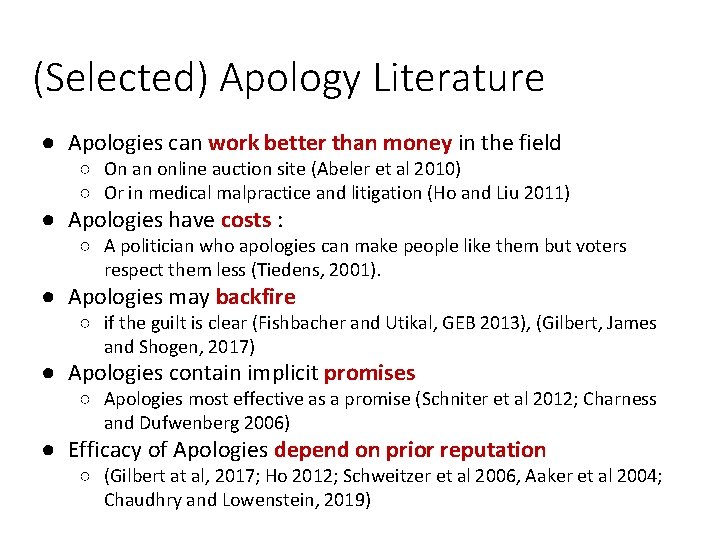
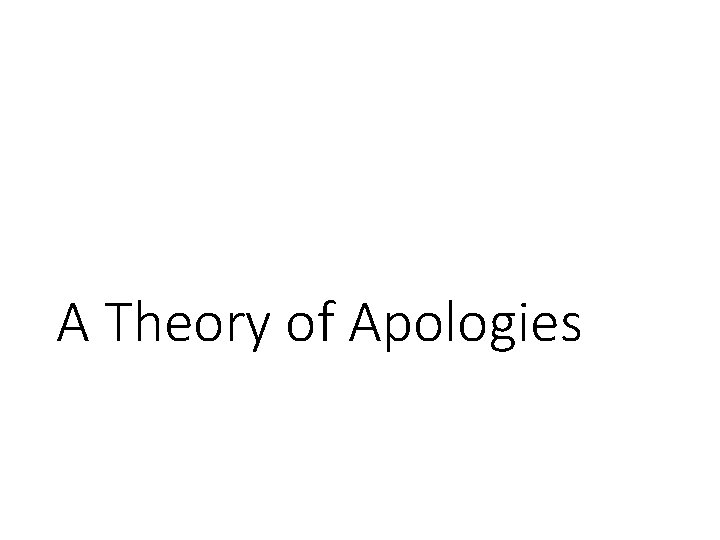
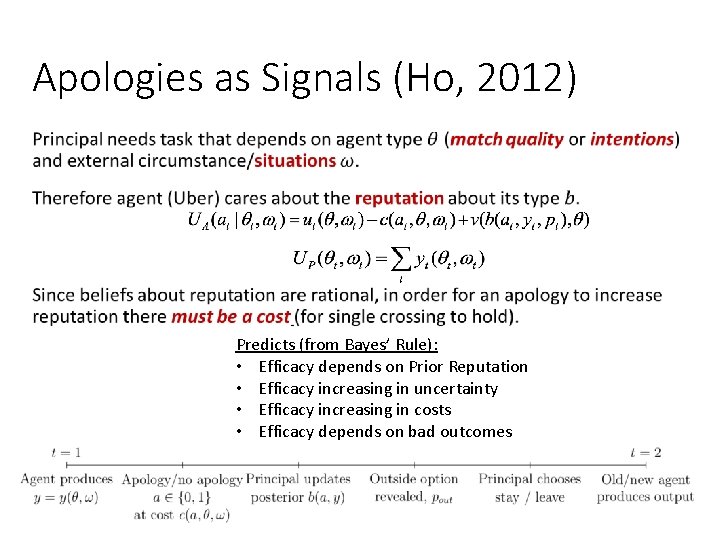
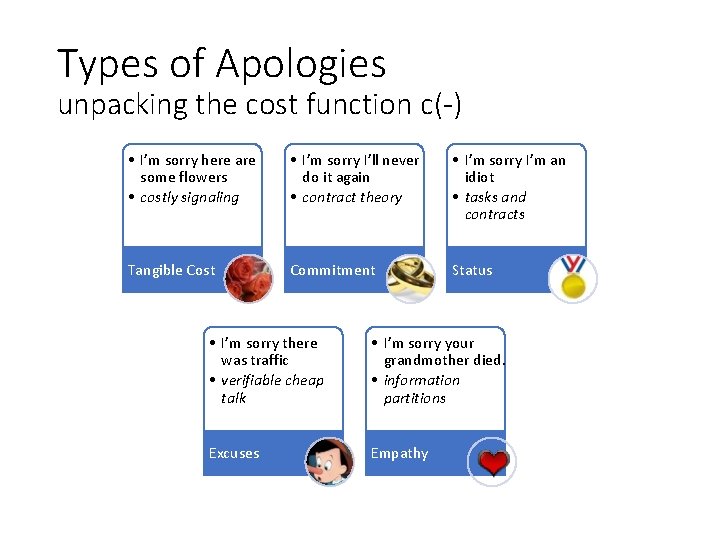
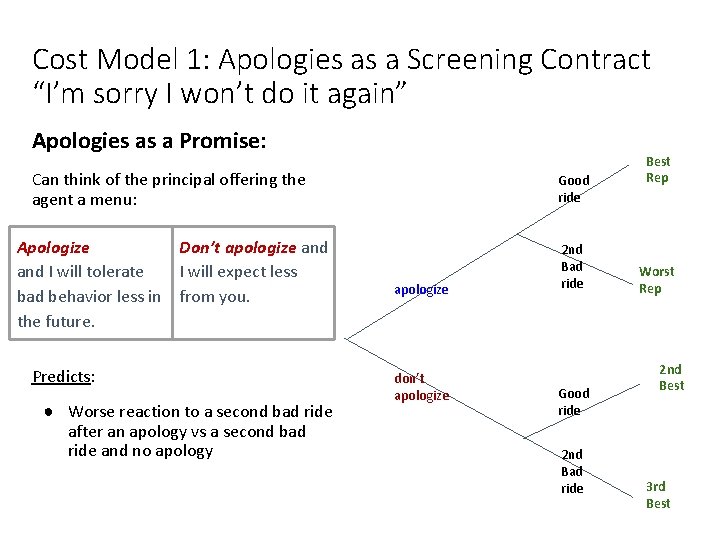
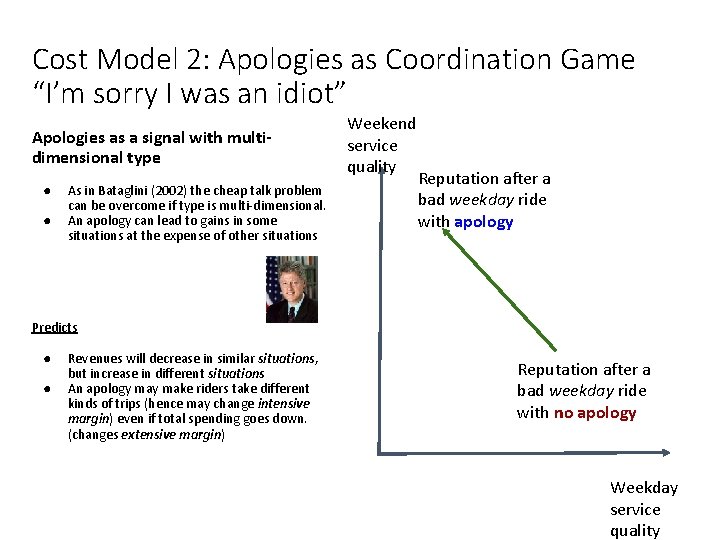
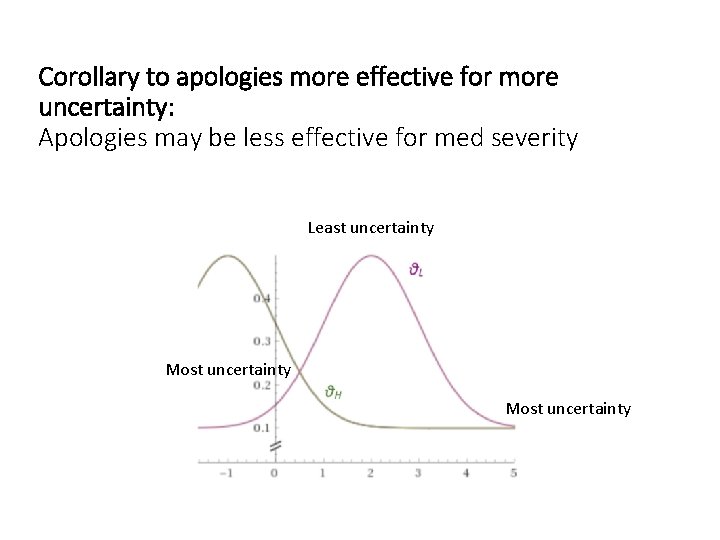
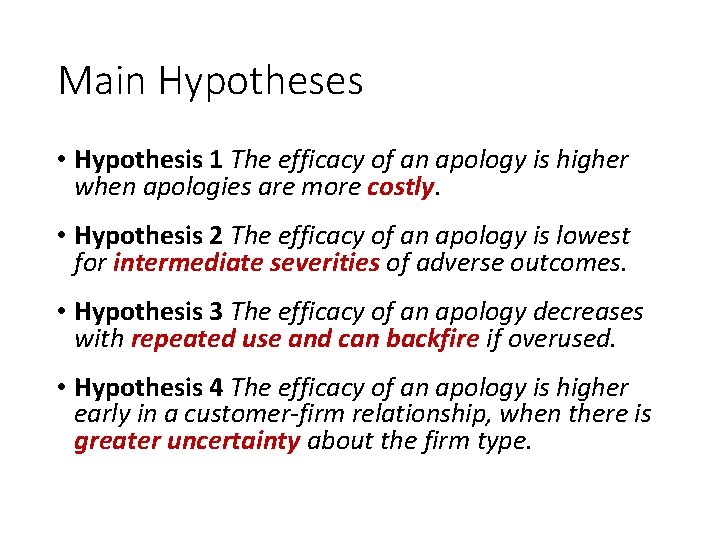
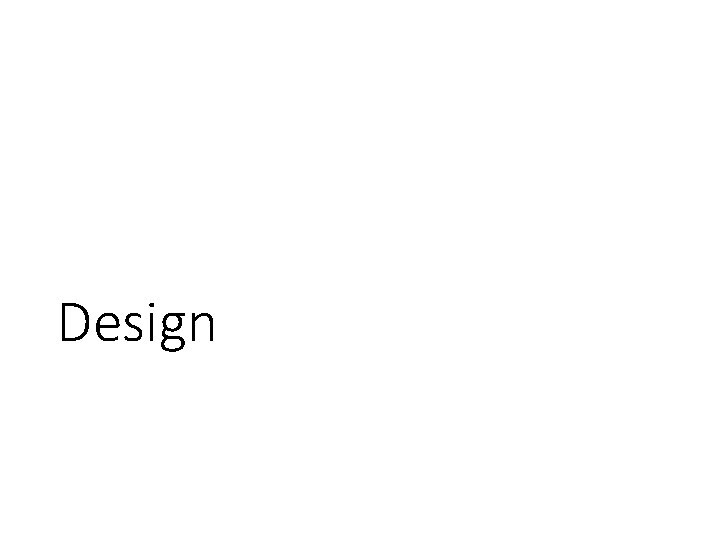
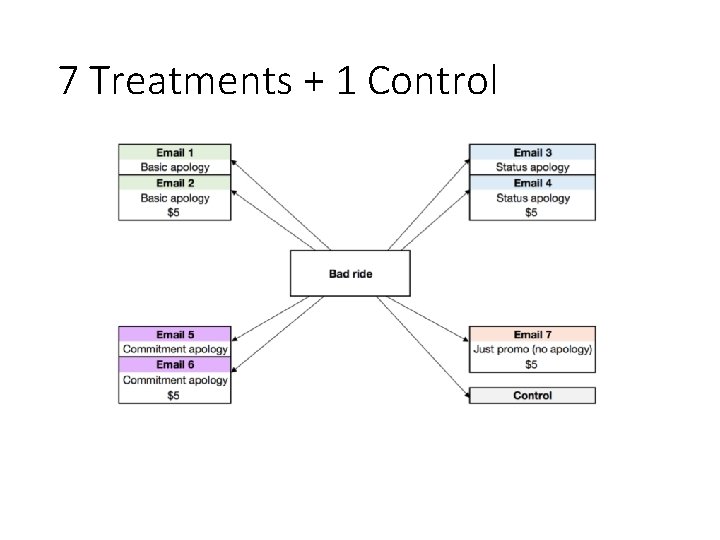
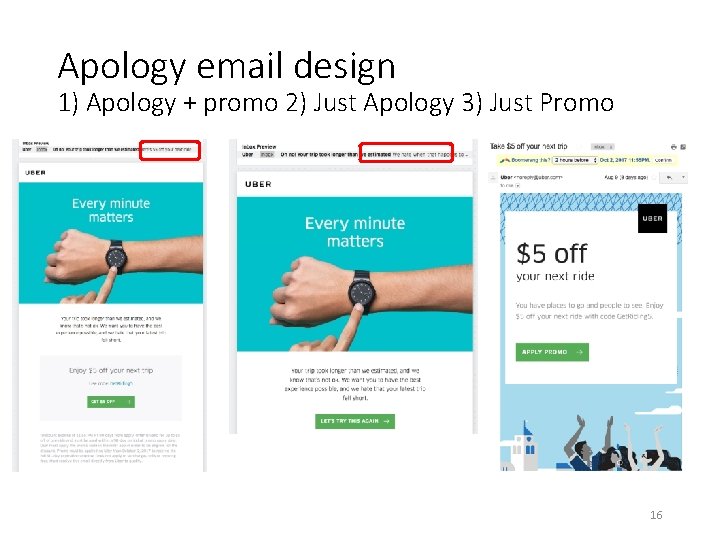
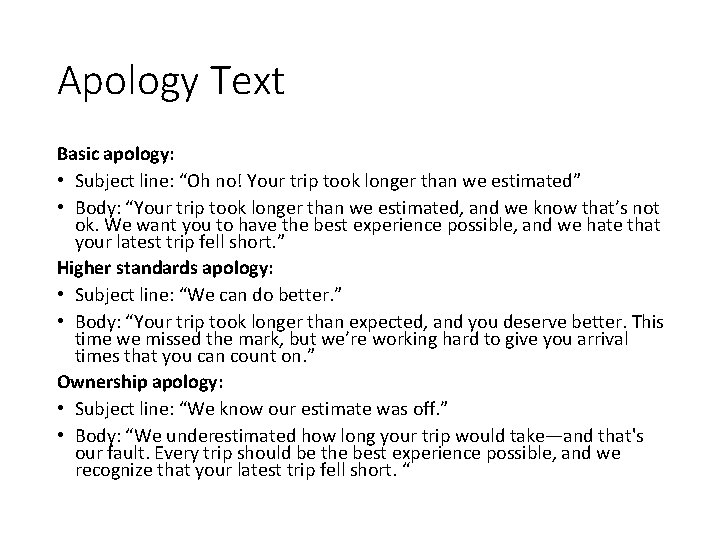
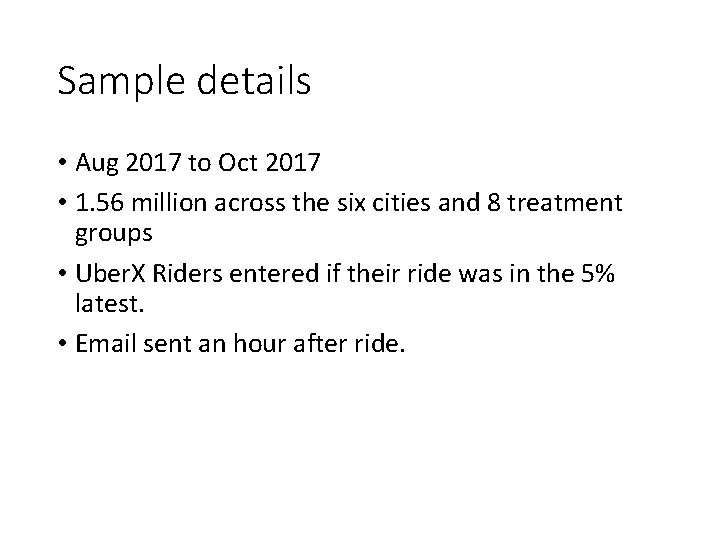
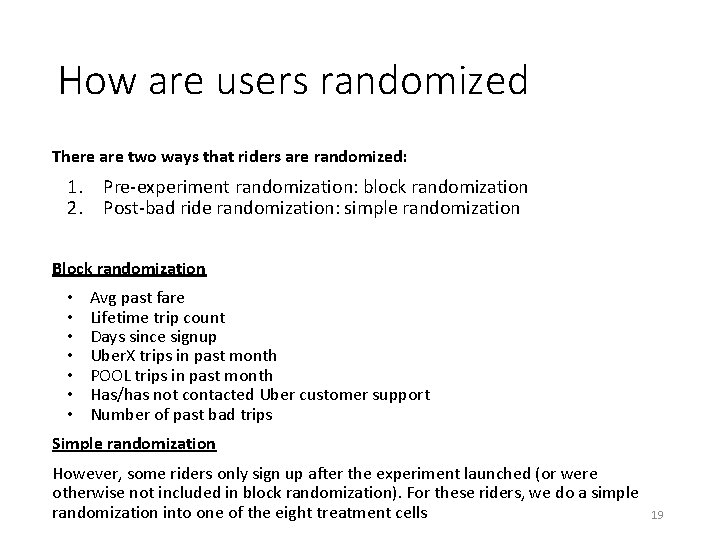
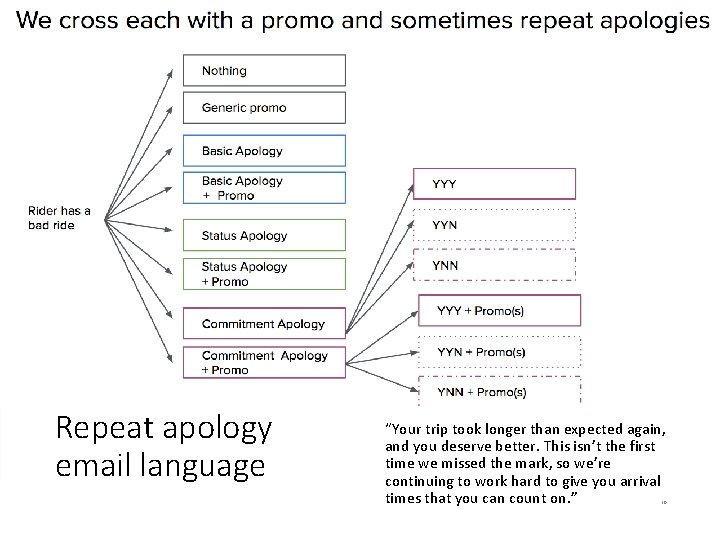
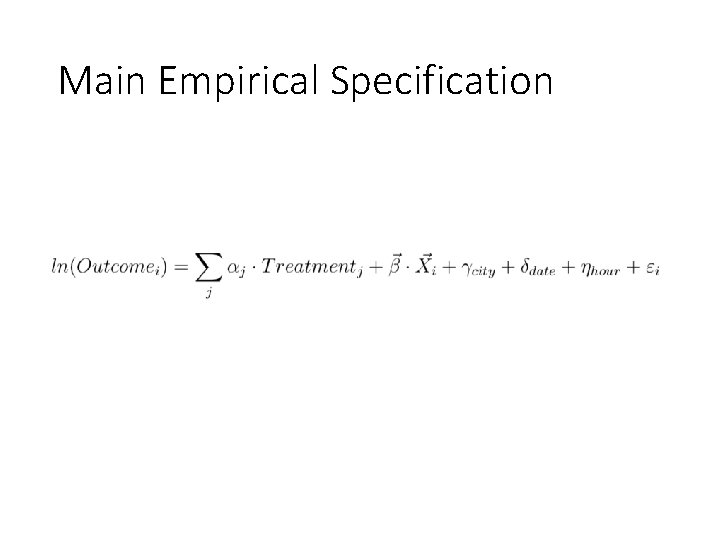
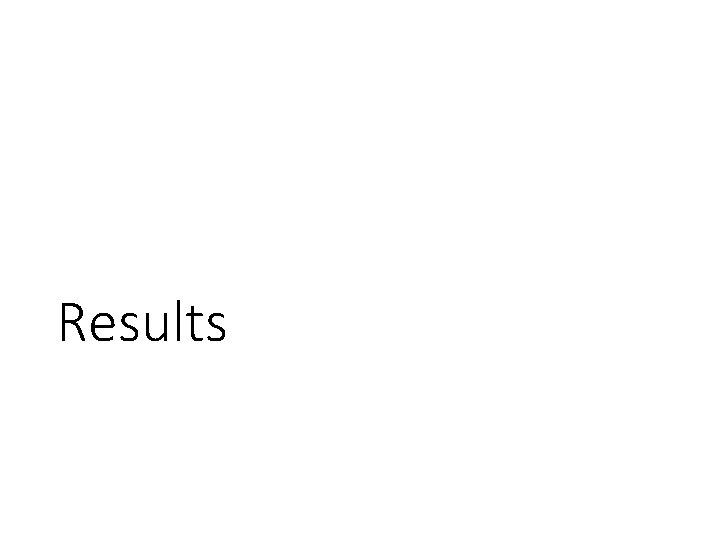
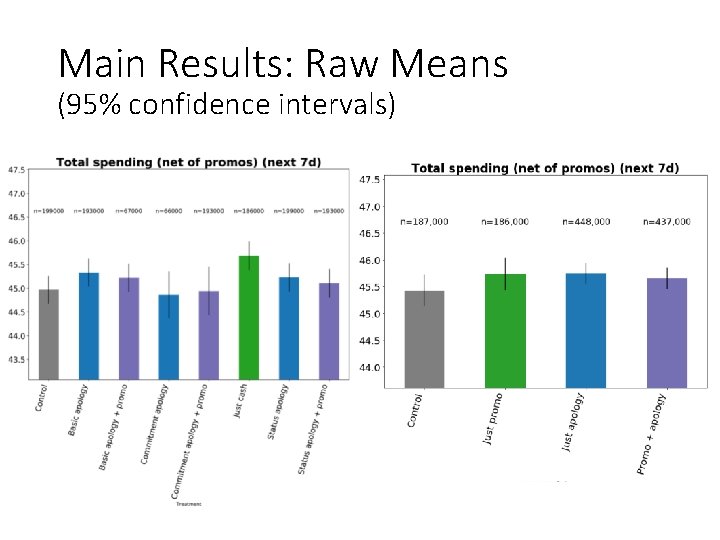
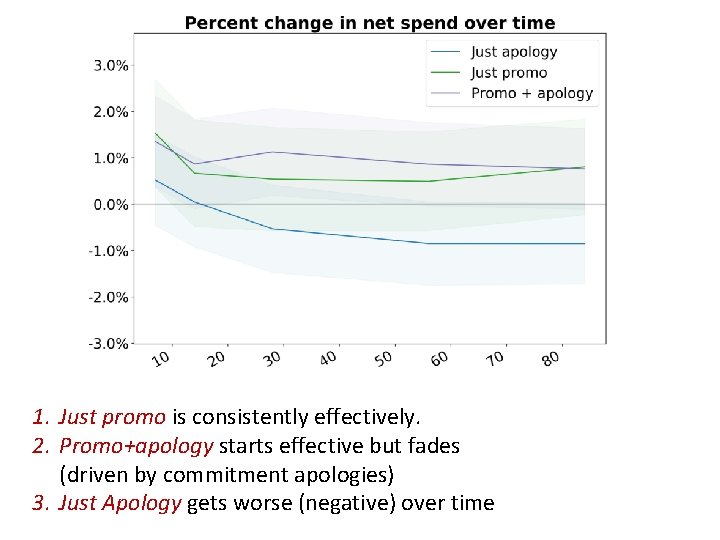
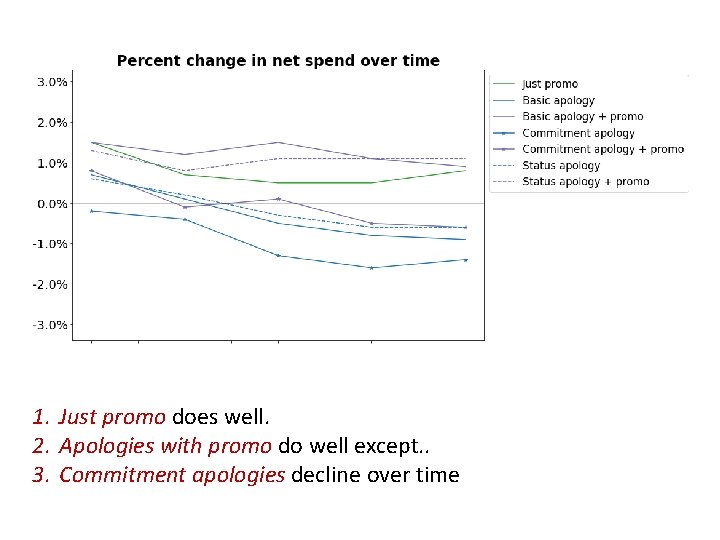
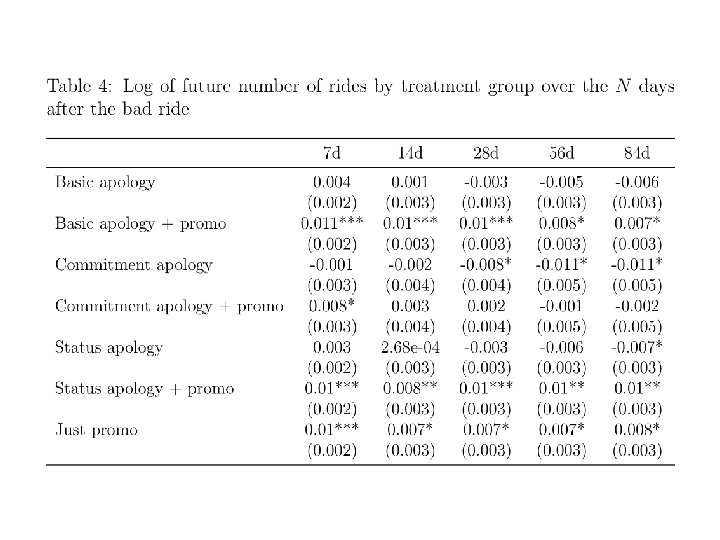
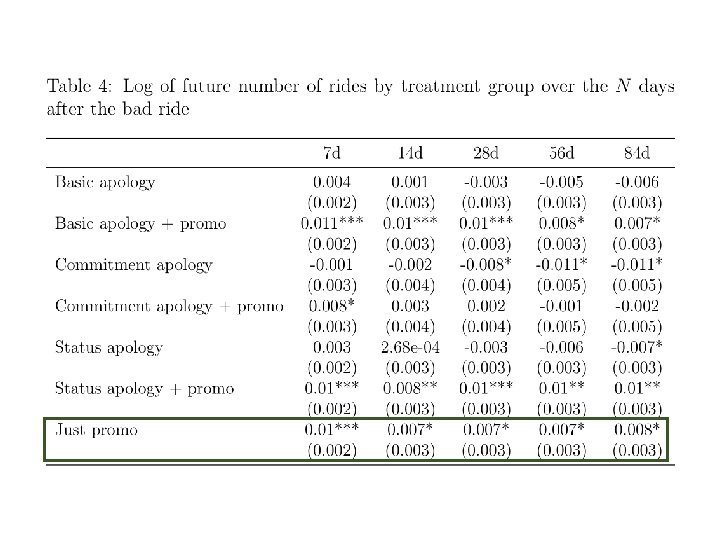
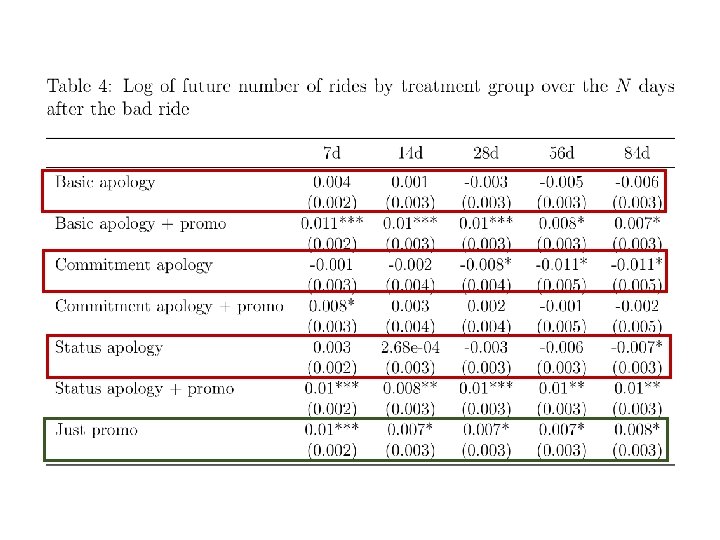
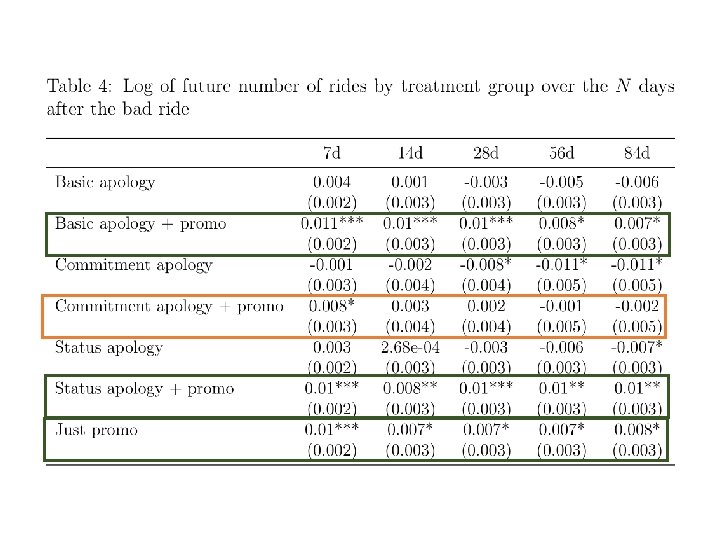
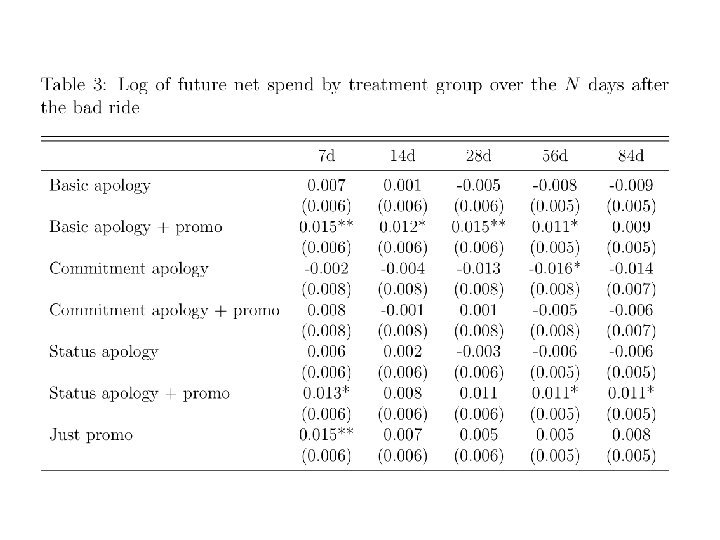
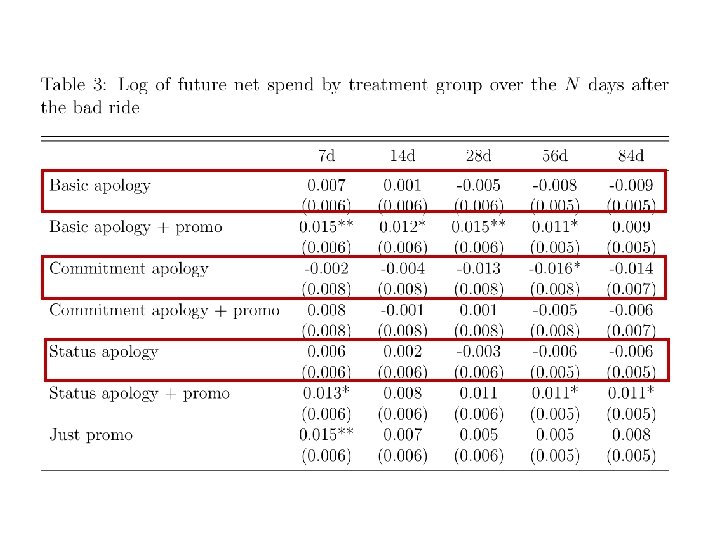
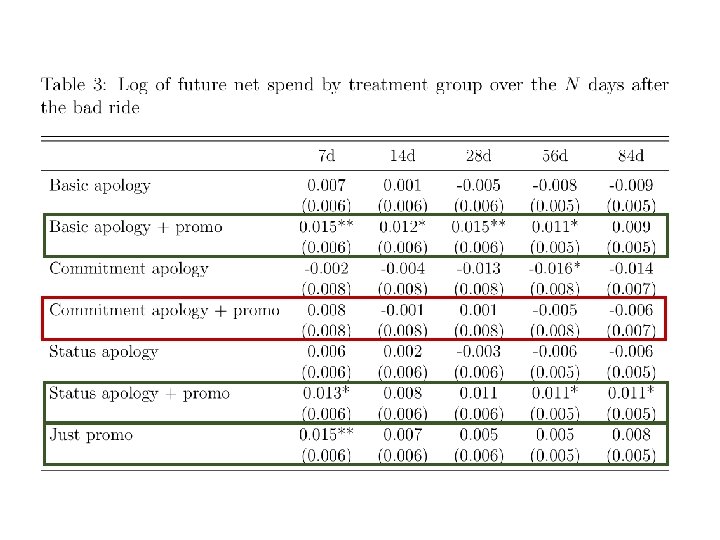
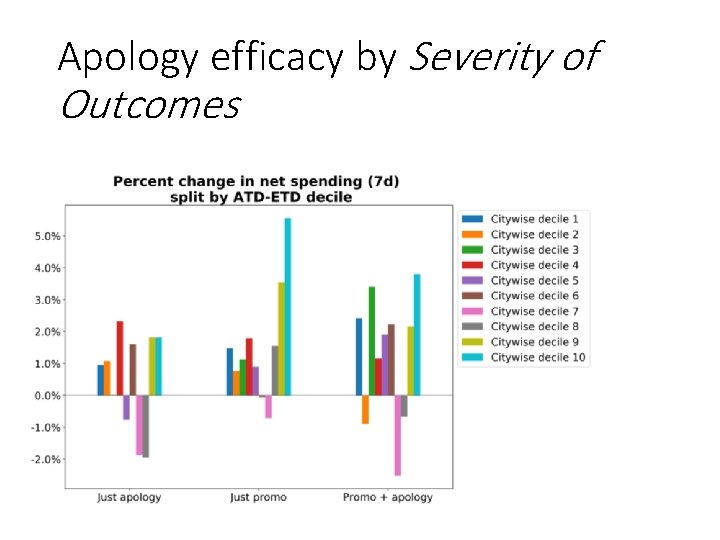
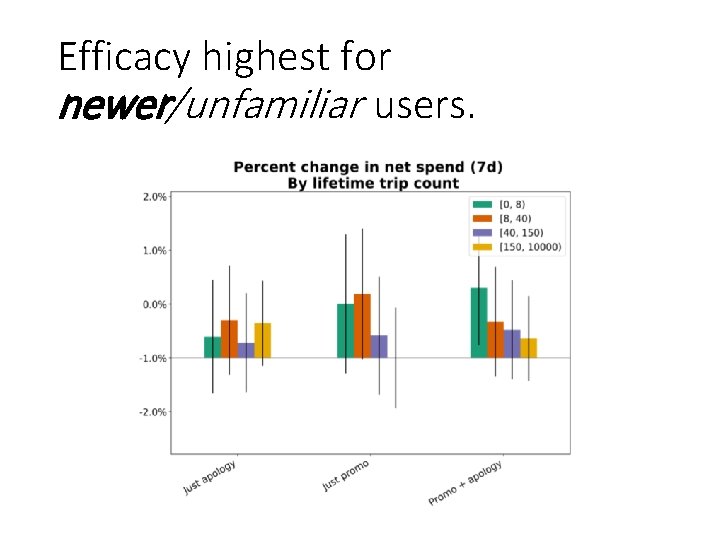
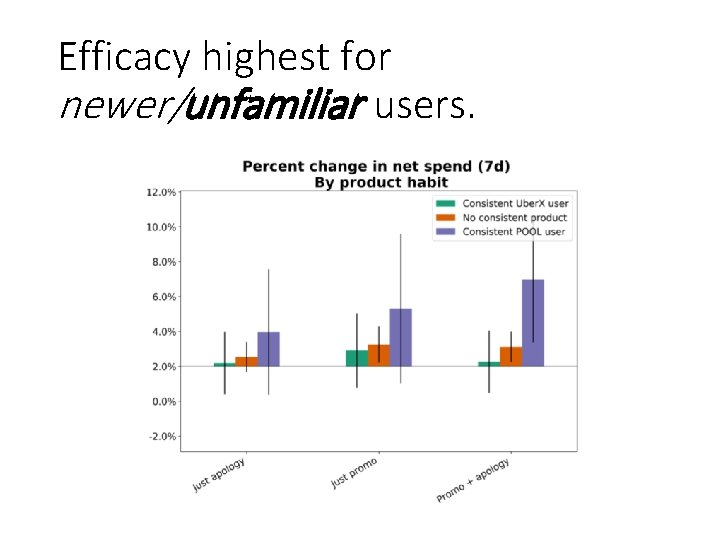
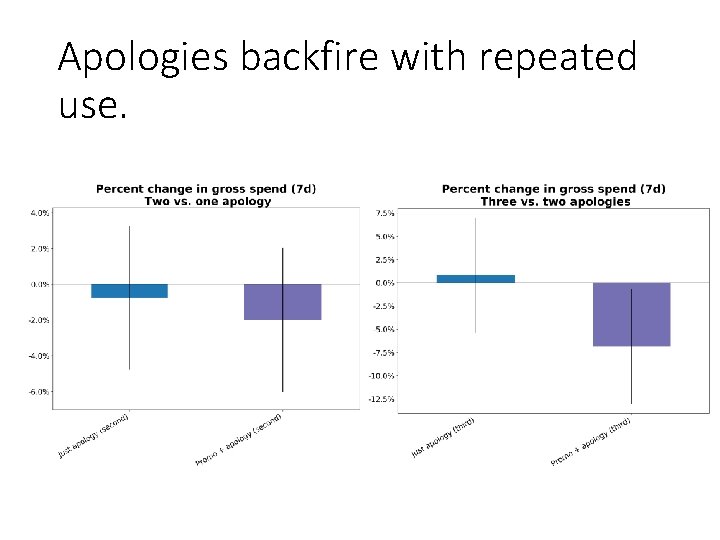
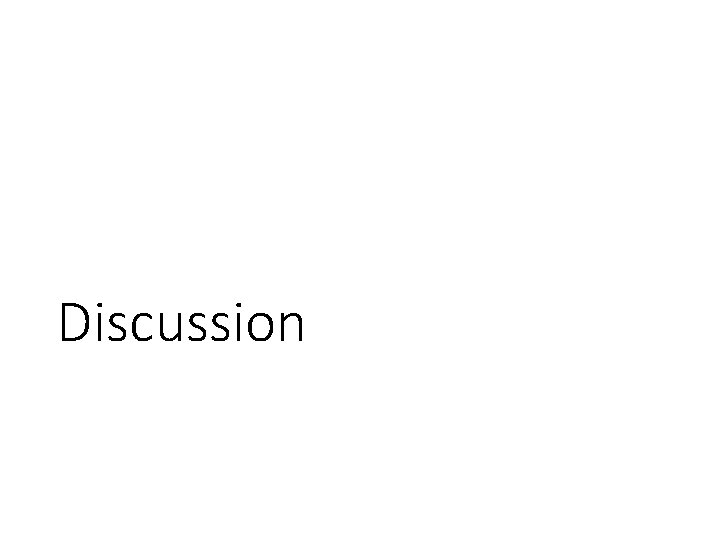
![Placebo Test: Generic $5 coupon (small sample [6000] of similar customers in Miami) Placebo Test: Generic $5 coupon (small sample [6000] of similar customers in Miami)](https://slidetodoc.com/presentation_image_h/82e53bf2226ff3481230e03f4ec9d414/image-38.jpg)
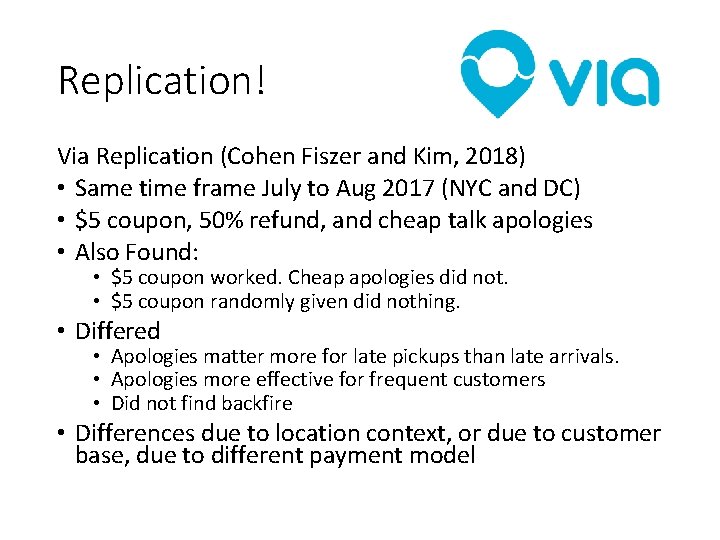
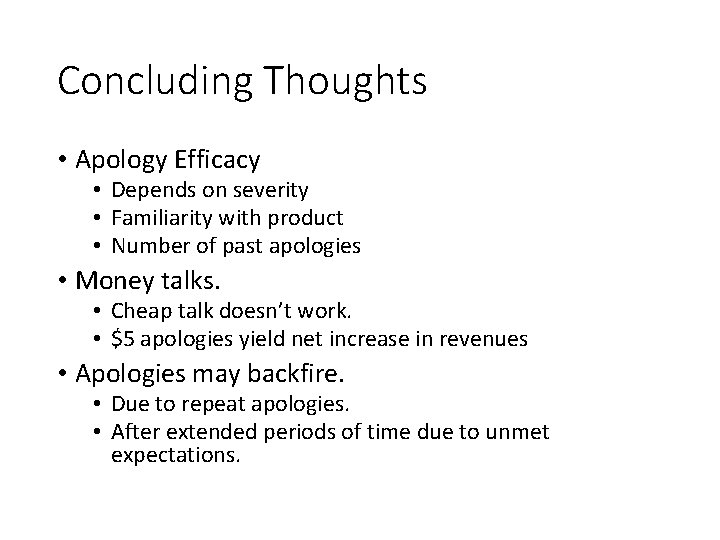
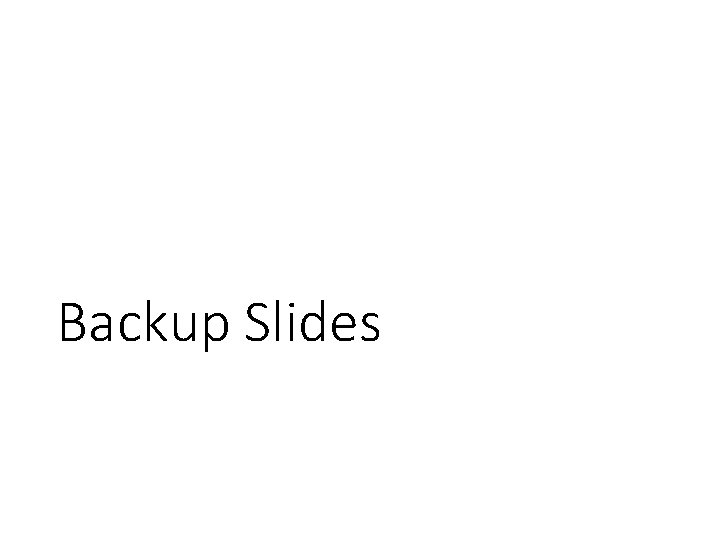
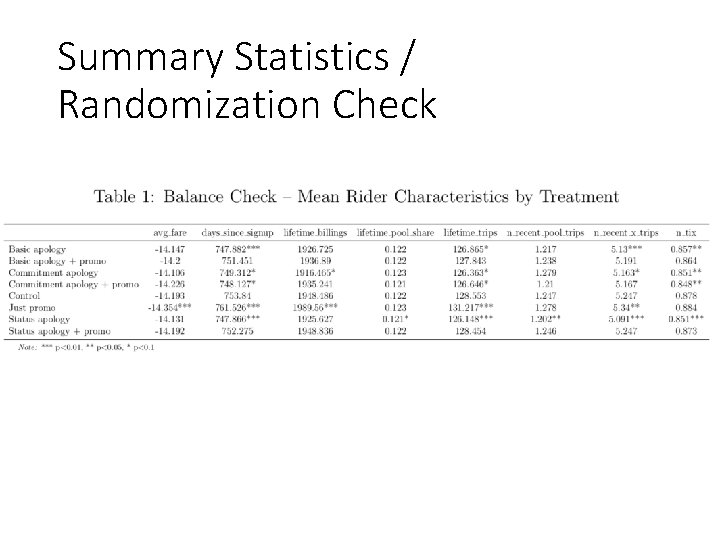
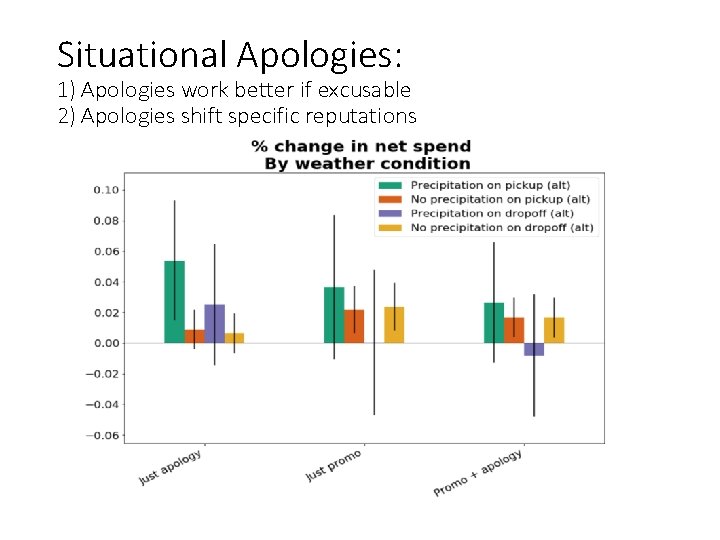
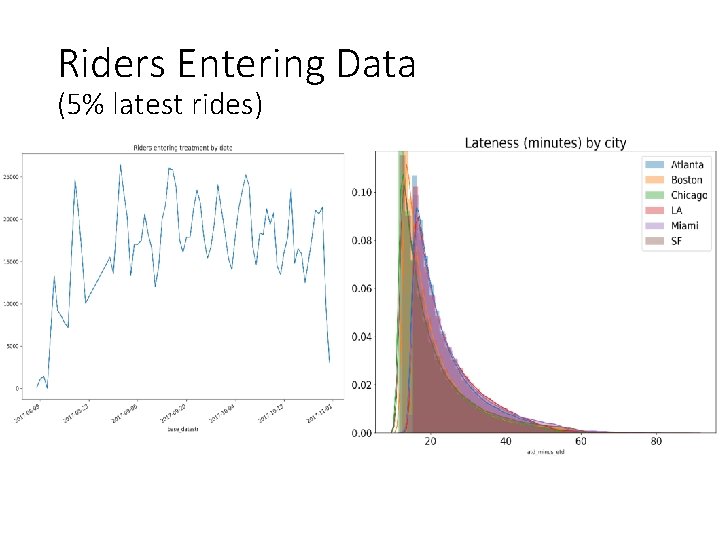
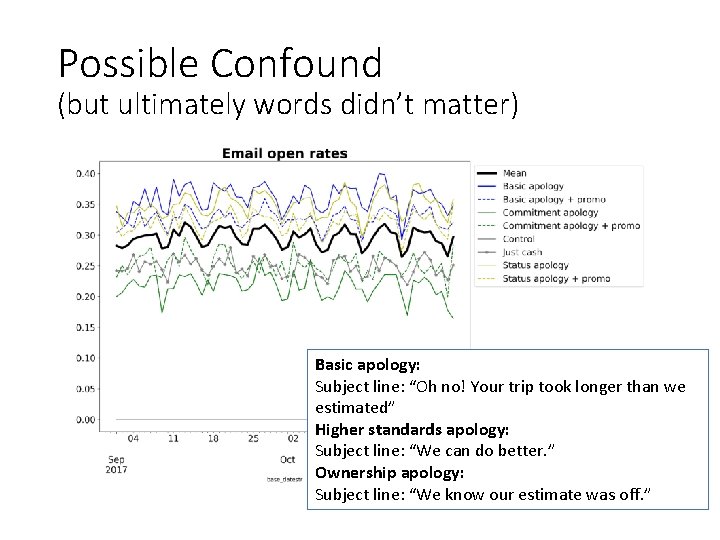
- Slides: 45
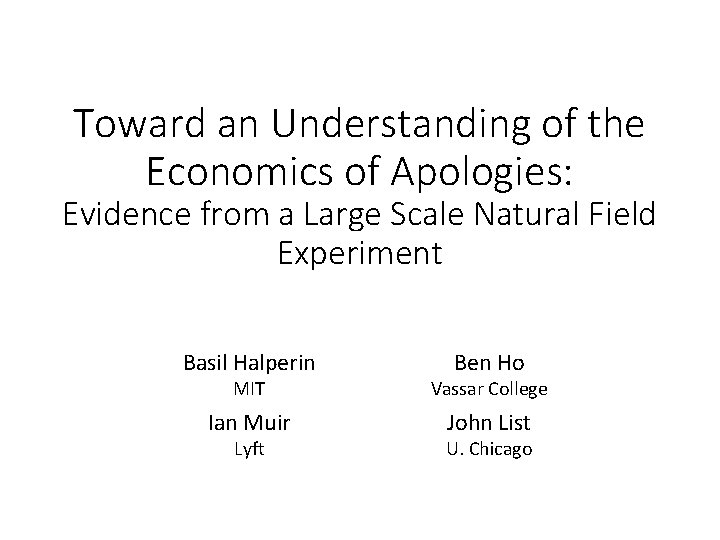
Toward an Understanding of the Economics of Apologies: Evidence from a Large Scale Natural Field Experiment Basil Halperin Ben Ho MIT Vassar College Ian Muir John List Lyft U. Chicago
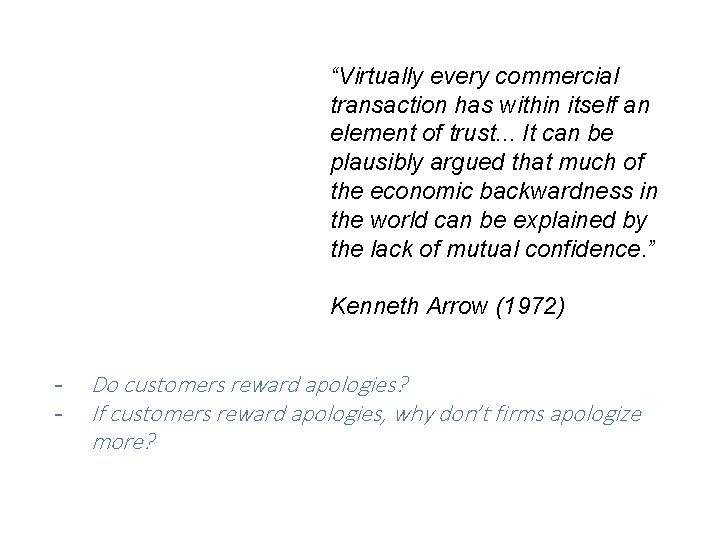
“Virtually every commercial transaction has within itself an element of trust. . . It can be plausibly argued that much of the economic backwardness in the world can be explained by the lack of mutual confidence. ” Kenneth Arrow (1972) - Do customers reward apologies? If customers reward apologies, why don’t firms apologize more?
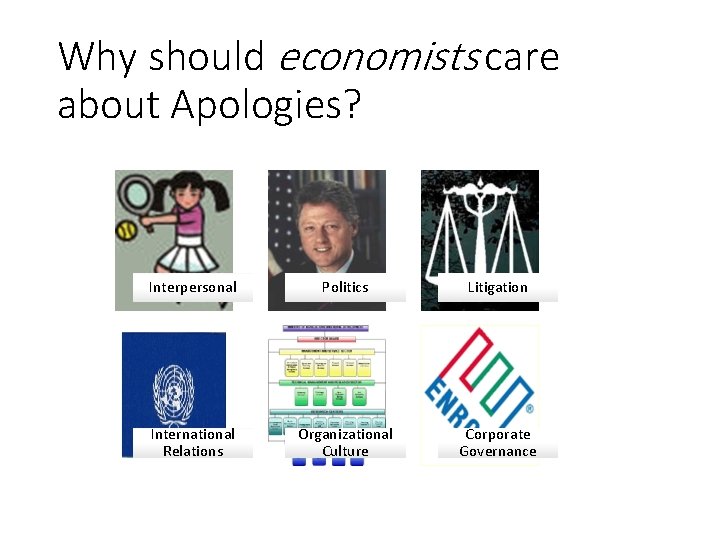
Why should economists care about Apologies? Interpersonal Politics Litigation International Relations Organizational Culture Corporate Governance
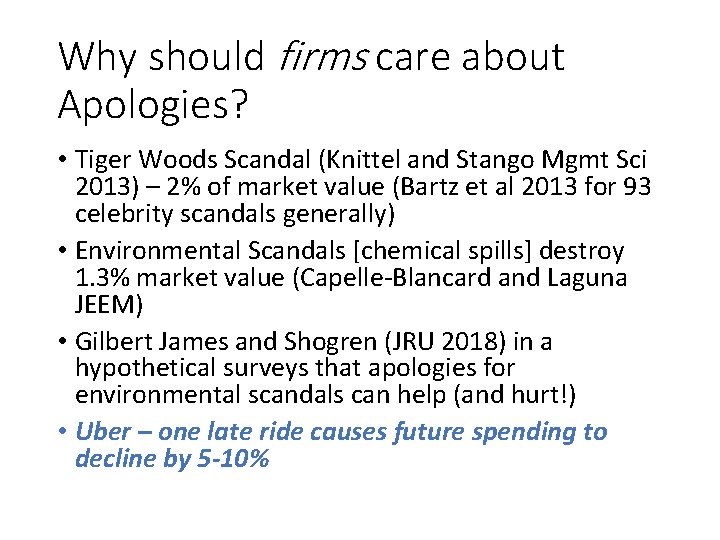
Why should firms care about Apologies? • Tiger Woods Scandal (Knittel and Stango Mgmt Sci 2013) – 2% of market value (Bartz et al 2013 for 93 celebrity scandals generally) • Environmental Scandals [chemical spills] destroy 1. 3% market value (Capelle-Blancard and Laguna JEEM) • Gilbert James and Shogren (JRU 2018) in a hypothetical surveys that apologies for environmental scandals can help (and hurt!) • Uber – one late ride causes future spending to decline by 5 -10%
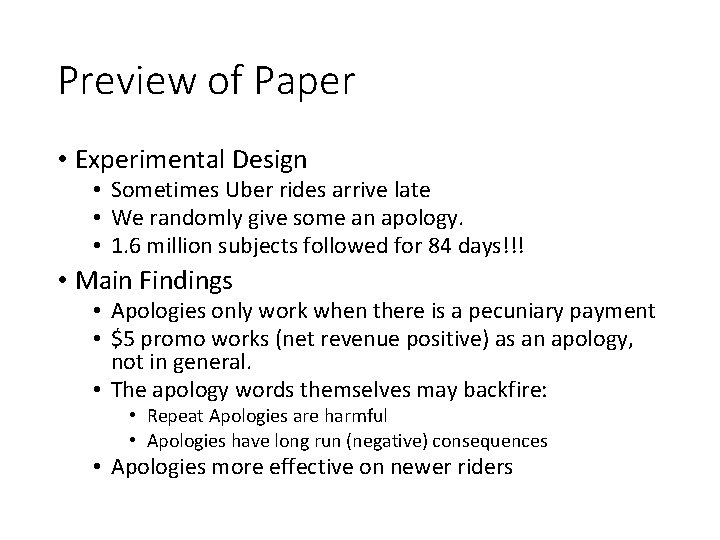
Preview of Paper • Experimental Design • Sometimes Uber rides arrive late • We randomly give some an apology. • 1. 6 million subjects followed for 84 days!!! • Main Findings • Apologies only work when there is a pecuniary payment • $5 promo works (net revenue positive) as an apology, not in general. • The apology words themselves may backfire: • Repeat Apologies are harmful • Apologies have long run (negative) consequences • Apologies more effective on newer riders
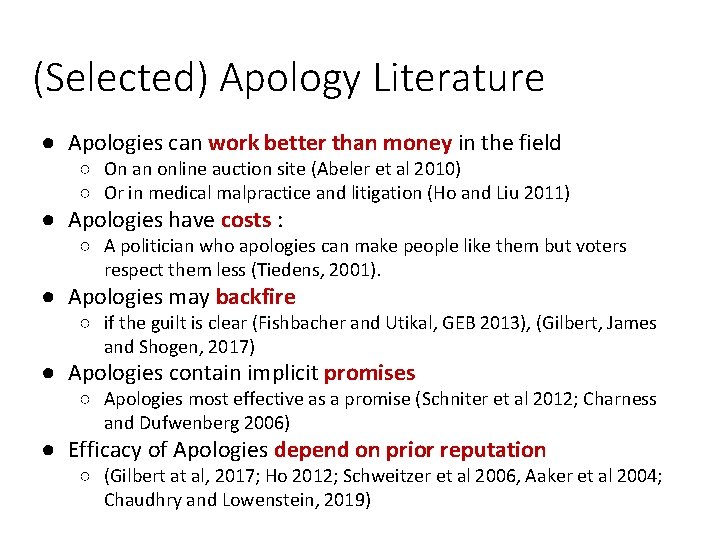
(Selected) Apology Literature ● Apologies can work better than money in the field ○ On an online auction site (Abeler et al 2010) ○ Or in medical malpractice and litigation (Ho and Liu 2011) ● Apologies have costs : ○ A politician who apologies can make people like them but voters respect them less (Tiedens, 2001). ● Apologies may backfire ○ if the guilt is clear (Fishbacher and Utikal, GEB 2013), (Gilbert, James and Shogen, 2017) ● Apologies contain implicit promises ○ Apologies most effective as a promise (Schniter et al 2012; Charness and Dufwenberg 2006) ● Efficacy of Apologies depend on prior reputation ○ (Gilbert at al, 2017; Ho 2012; Schweitzer et al 2006, Aaker et al 2004; Chaudhry and Lowenstein, 2019)
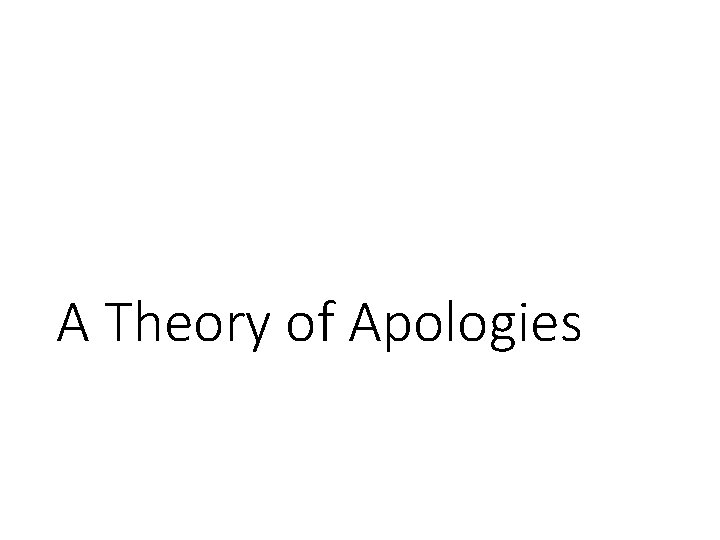
A Theory of Apologies
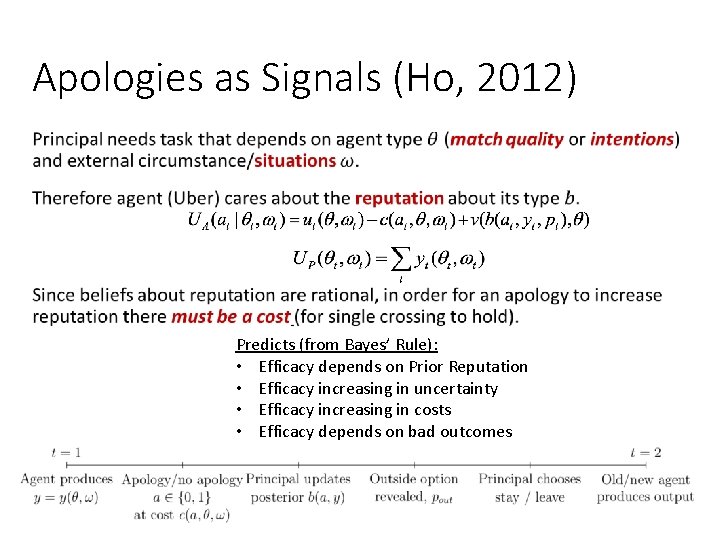
Apologies as Signals (Ho, 2012) ● Predicts (from Bayes’ Rule): • Efficacy depends on Prior Reputation • Efficacy increasing in uncertainty • Efficacy increasing in costs • Efficacy depends on bad outcomes
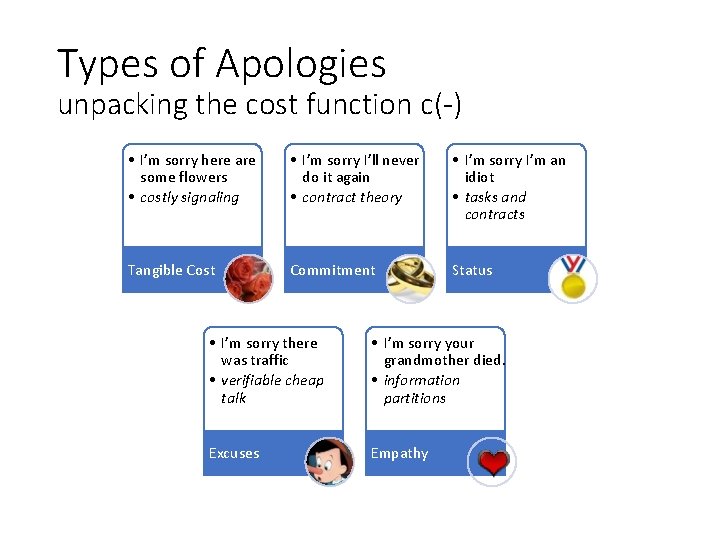
Types of Apologies unpacking the cost function c(-) • I’m sorry here are some flowers • costly signaling • I’m sorry I’ll never do it again • contract theory • I’m sorry I’m an idiot • tasks and contracts Tangible Cost Commitment Status • I’m sorry there was traffic • verifiable cheap talk • I’m sorry your grandmother died. • information partitions Excuses Empathy
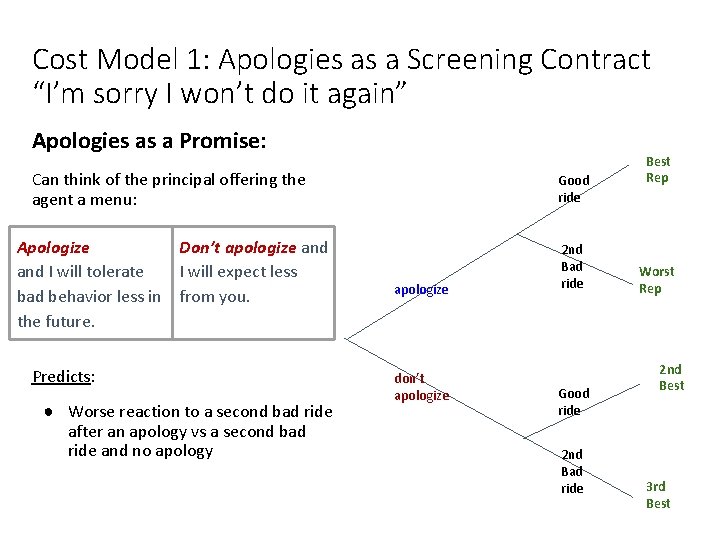
Cost Model 1: Apologies as a Screening Contract “I’m sorry I won’t do it again” Apologies as a Promise: Can think of the principal offering the agent a menu: Good ride Apologize Don’t apologize and I will tolerate I will expect less bad behavior less in from you. the future. 2 nd Bad ride Predicts: ● Worse reaction to a second bad ride after an apology vs a second bad ride and no apology apologize don’t apologize Good ride 2 nd Bad ride Best Rep Worst Rep 2 nd Best 3 rd Best
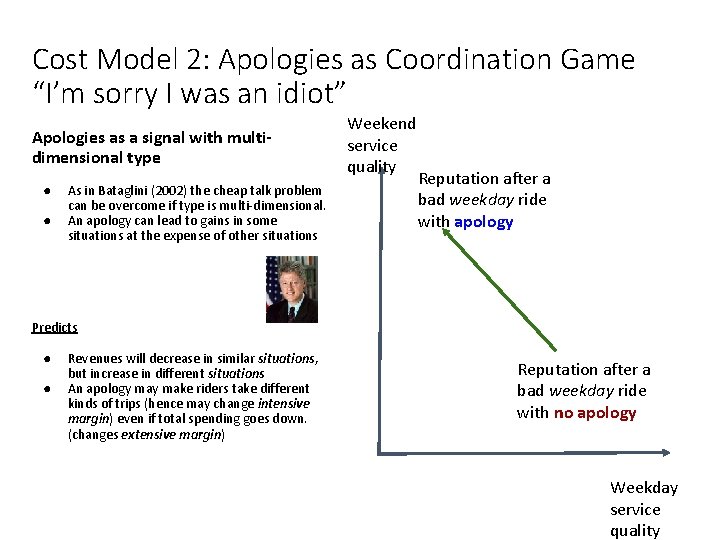
Cost Model 2: Apologies as Coordination Game “I’m sorry I was an idiot” Apologies as a signal with multidimensional type ● ● As in Bataglini (2002) the cheap talk problem can be overcome if type is multi-dimensional. An apology can lead to gains in some situations at the expense of other situations Weekend service quality Reputation after a bad weekday ride with apology Predicts ● ● Revenues will decrease in similar situations, but increase in different situations An apology make riders take different kinds of trips (hence may change intensive margin) even if total spending goes down. (changes extensive margin) Reputation after a bad weekday ride with no apology Weekday service quality
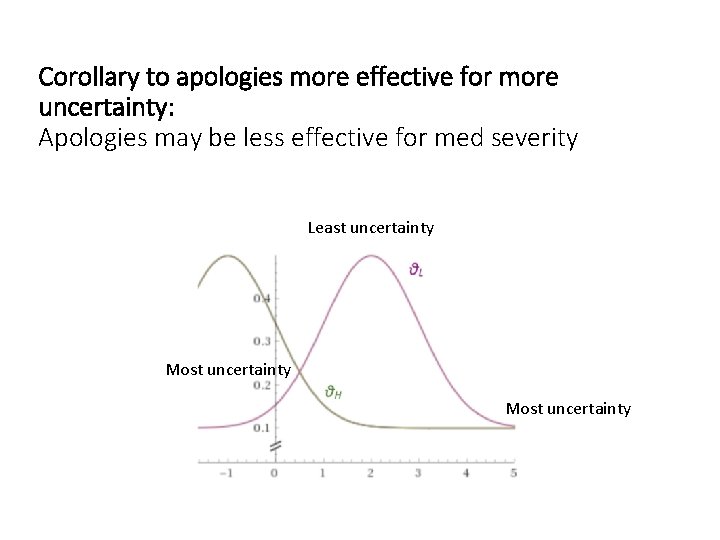
Corollary to apologies more effective for more uncertainty: Apologies may be less effective for med severity Least uncertainty Most uncertainty
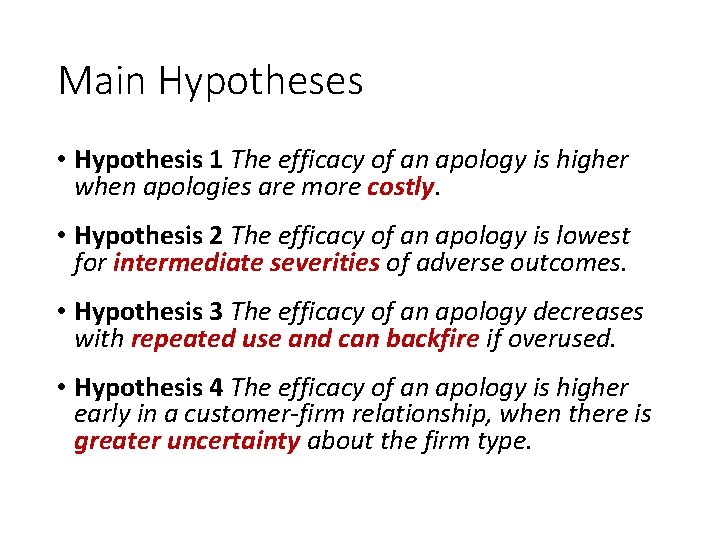
Main Hypotheses • Hypothesis 1 The efficacy of an apology is higher when apologies are more costly. • Hypothesis 2 The efficacy of an apology is lowest for intermediate severities of adverse outcomes. • Hypothesis 3 The efficacy of an apology decreases with repeated use and can backfire if overused. • Hypothesis 4 The efficacy of an apology is higher early in a customer-firm relationship, when there is greater uncertainty about the firm type.
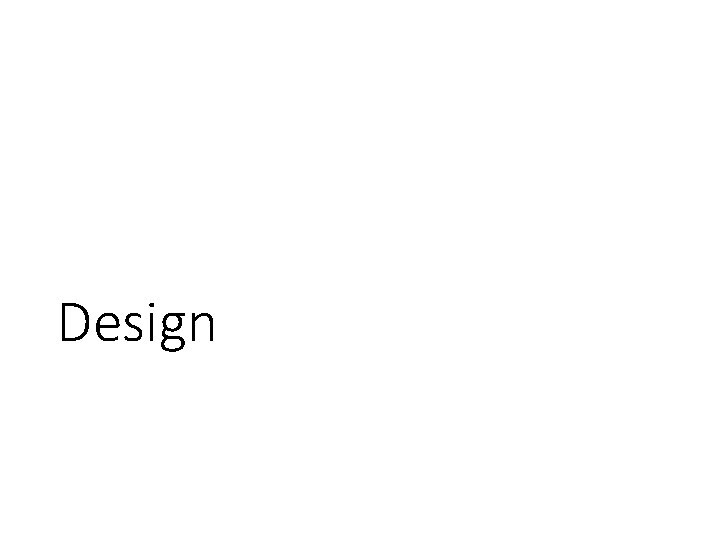
Design
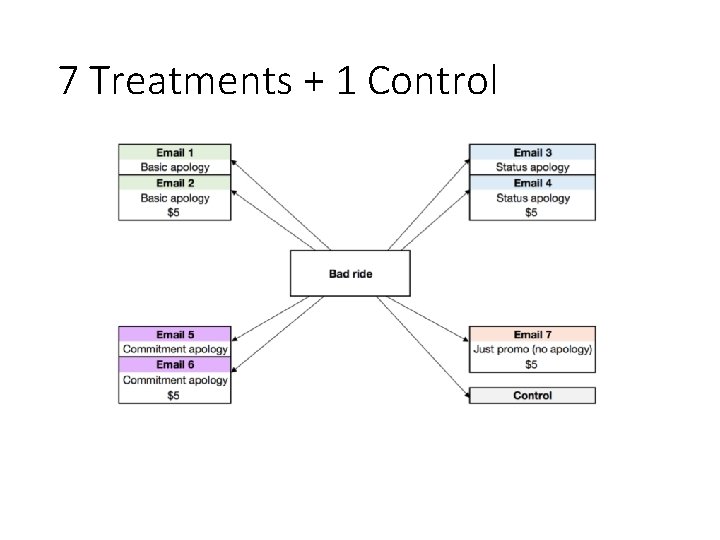
7 Treatments + 1 Control
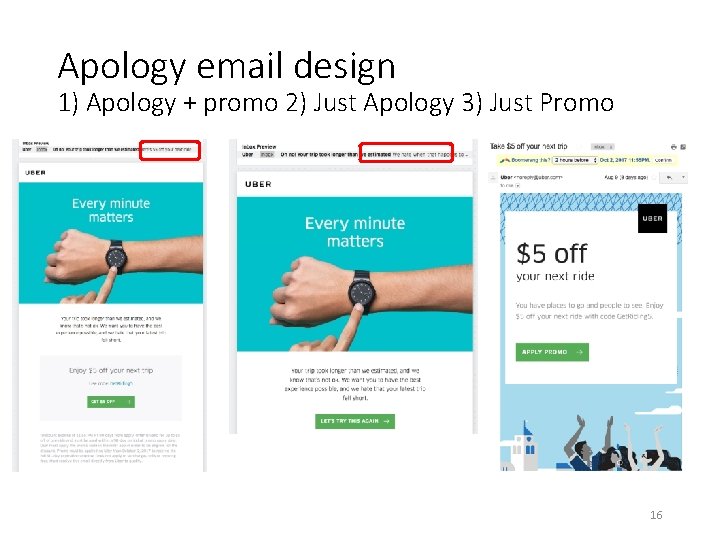
Apology email design 1) Apology + promo 2) Just Apology 3) Just Promo 16
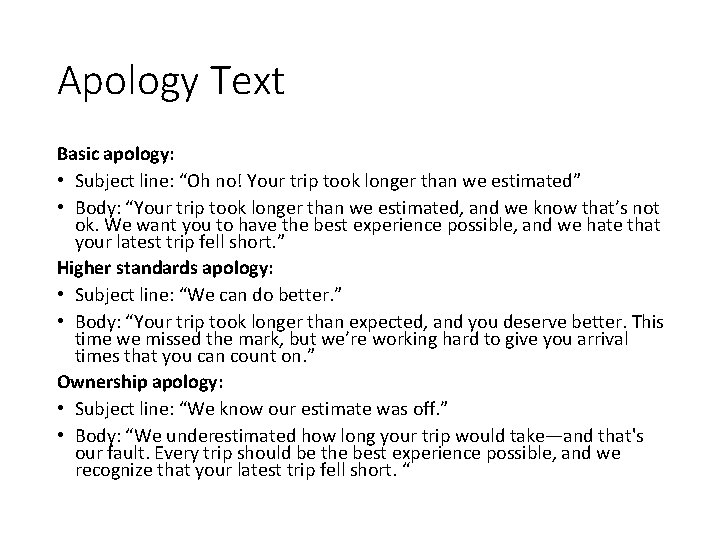
Apology Text Basic apology: • Subject line: “Oh no! Your trip took longer than we estimated” • Body: “Your trip took longer than we estimated, and we know that’s not ok. We want you to have the best experience possible, and we hate that your latest trip fell short. ” Higher standards apology: • Subject line: “We can do better. ” • Body: “Your trip took longer than expected, and you deserve better. This time we missed the mark, but we’re working hard to give you arrival times that you can count on. ” Ownership apology: • Subject line: “We know our estimate was off. ” • Body: “We underestimated how long your trip would take—and that's our fault. Every trip should be the best experience possible, and we recognize that your latest trip fell short. “
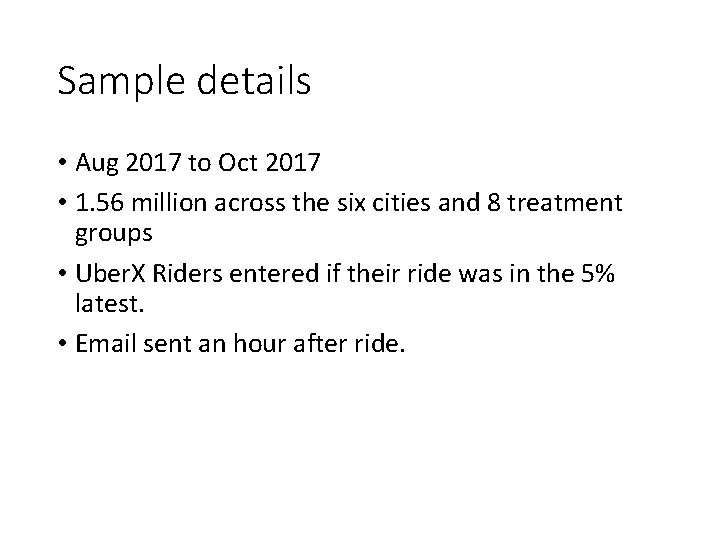
Sample details • Aug 2017 to Oct 2017 • 1. 56 million across the six cities and 8 treatment groups • Uber. X Riders entered if their ride was in the 5% latest. • Email sent an hour after ride.
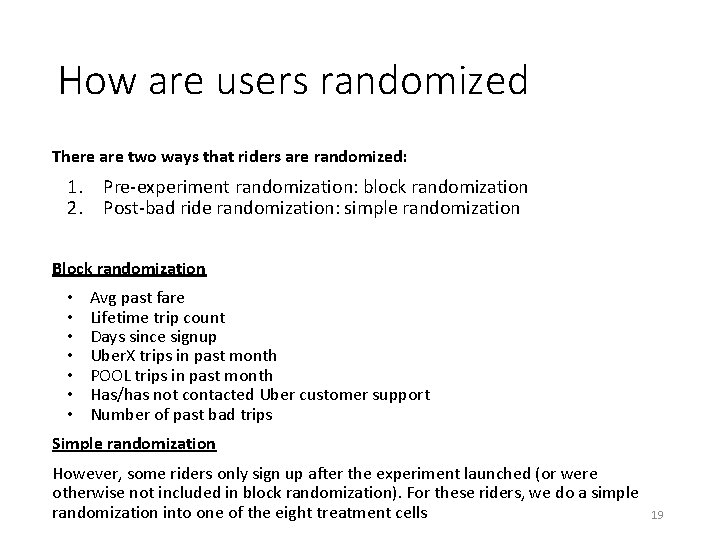
How are users randomized There are two ways that riders are randomized: 1. Pre-experiment randomization: block randomization 2. Post-bad ride randomization: simple randomization Block randomization • • Avg past fare Lifetime trip count Days since signup Uber. X trips in past month POOL trips in past month Has/has not contacted Uber customer support Number of past bad trips Simple randomization However, some riders only sign up after the experiment launched (or were otherwise not included in block randomization). For these riders, we do a simple randomization into one of the eight treatment cells 19
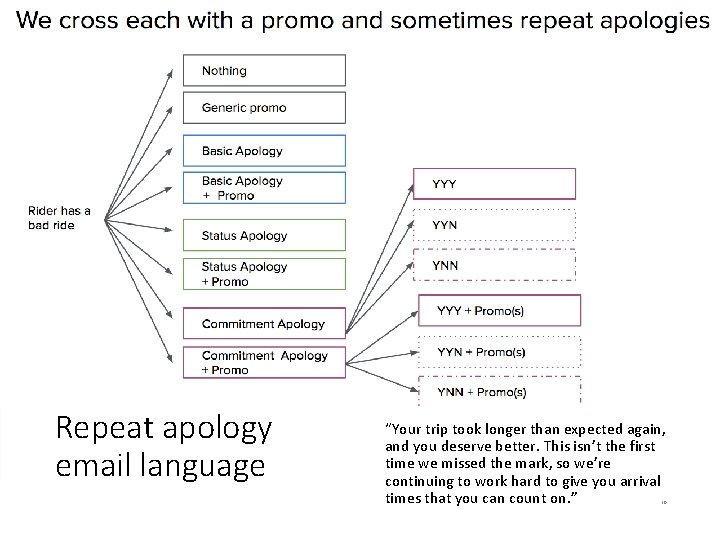
Repeat apology email language “Your trip took longer than expected again, and you deserve better. This isn’t the first time we missed the mark, so we’re continuing to work hard to give you arrival times that you can count on. ” 20
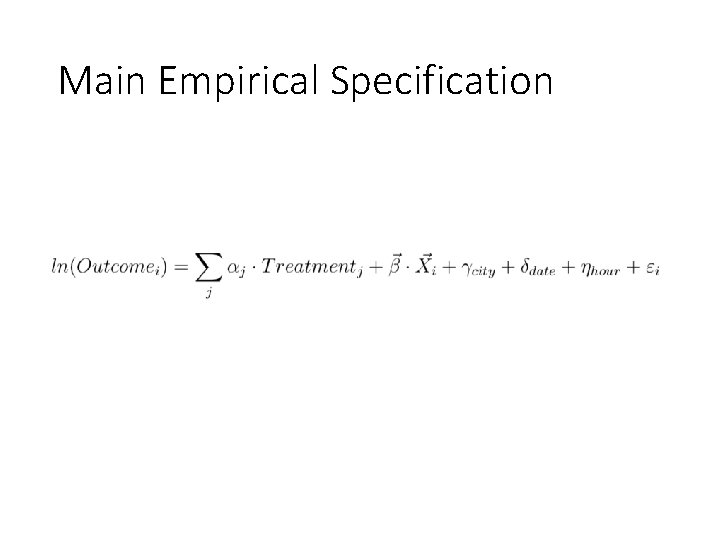
Main Empirical Specification
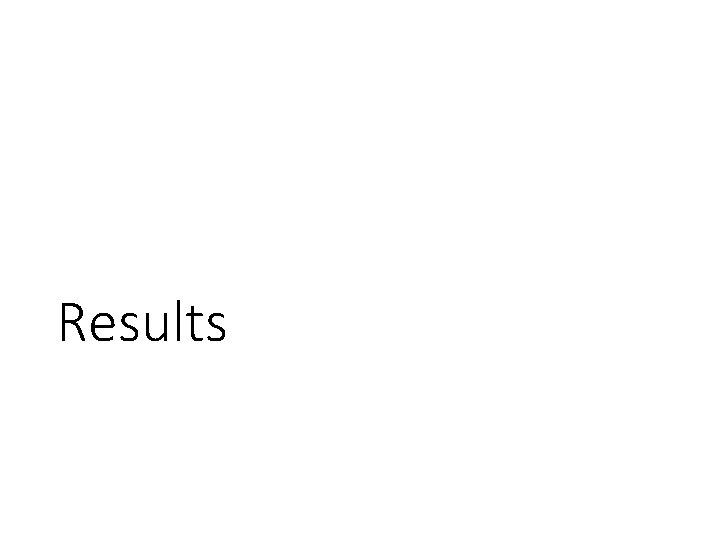
Results
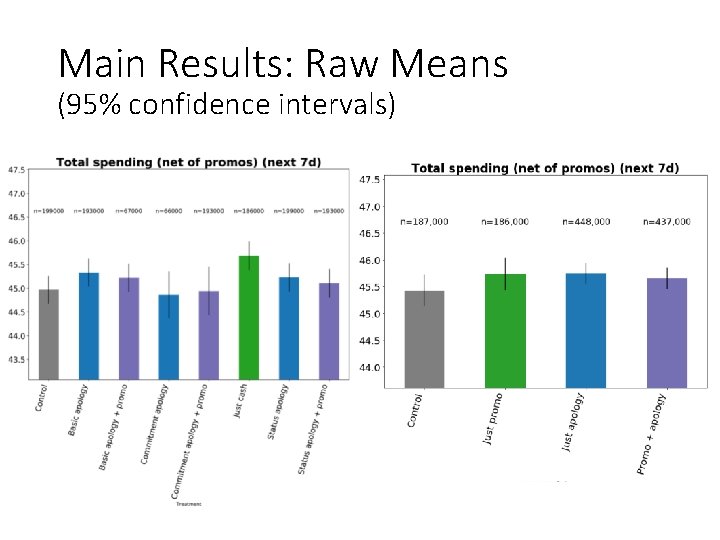
Main Results: Raw Means (95% confidence intervals)
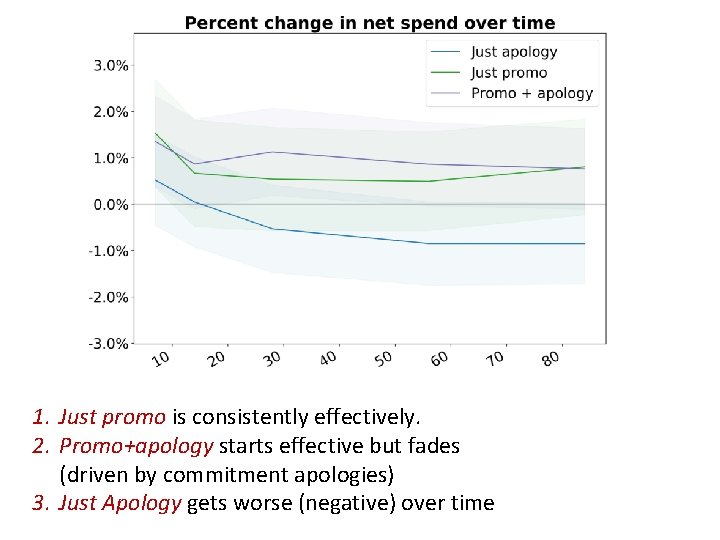
1. Just promo is consistently effectively. 2. Promo+apology starts effective but fades (driven by commitment apologies) 3. Just Apology gets worse (negative) over time
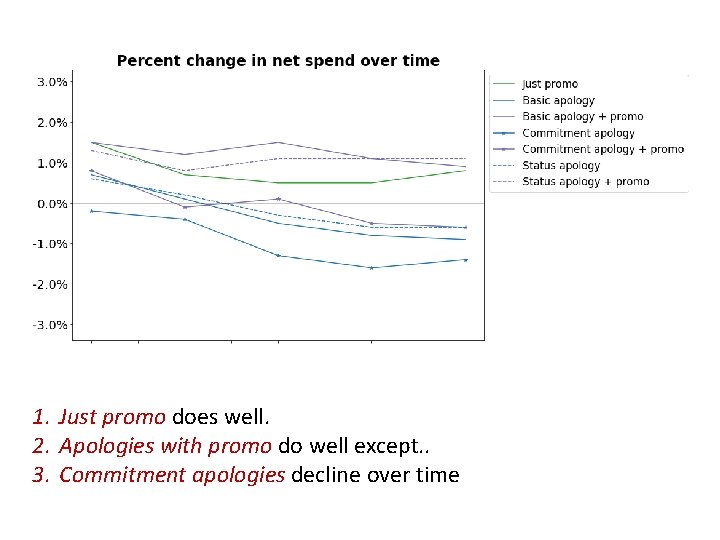
1. Just promo does well. 2. Apologies with promo do well except. . 3. Commitment apologies decline over time
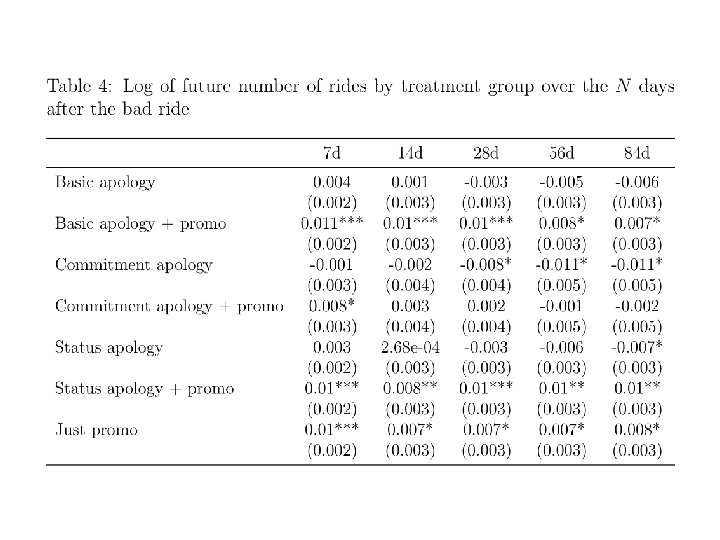
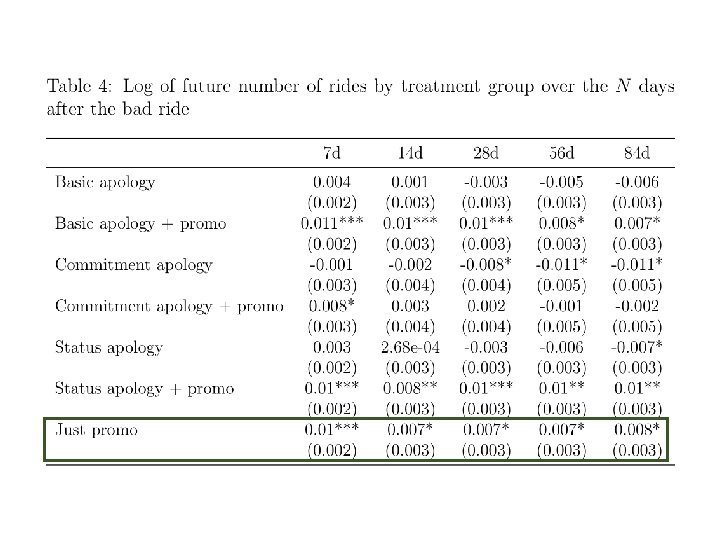
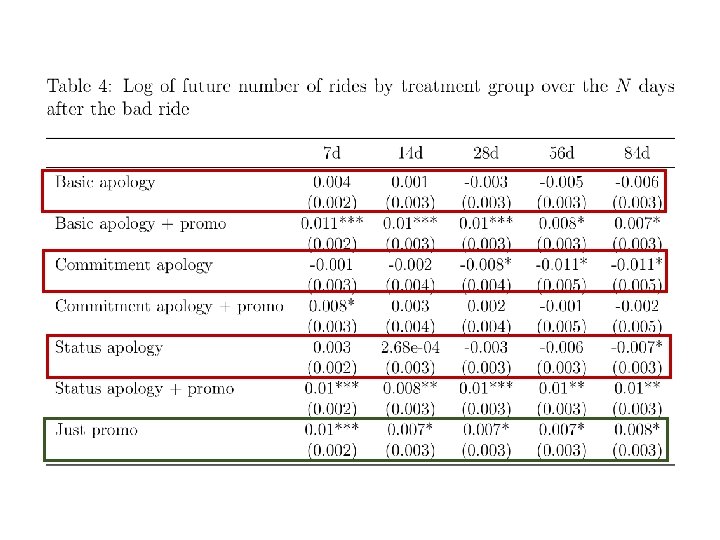
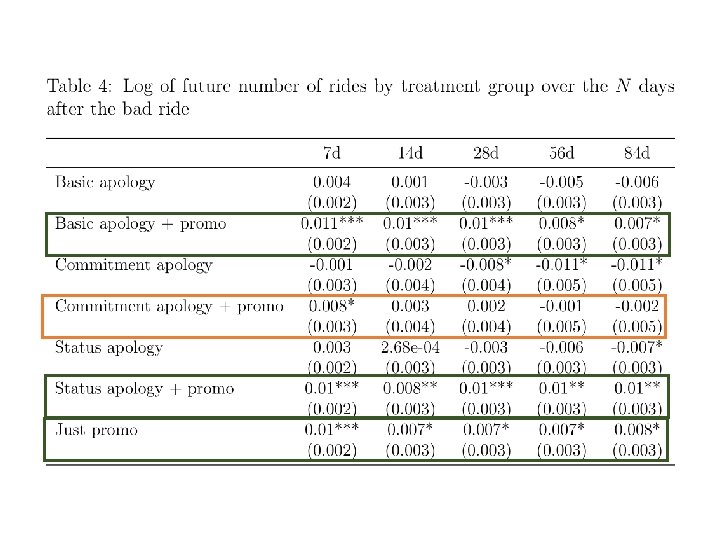
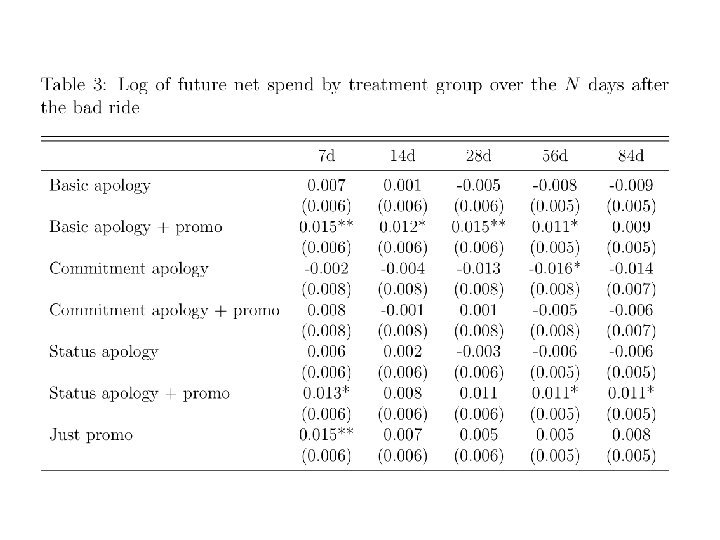
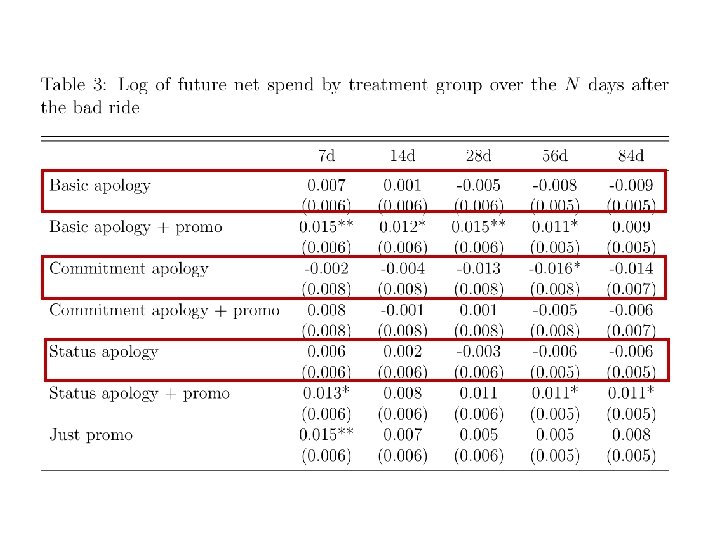
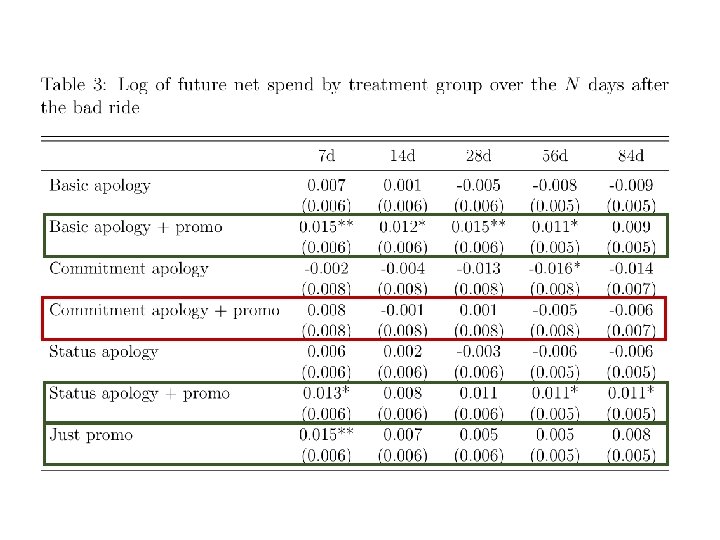
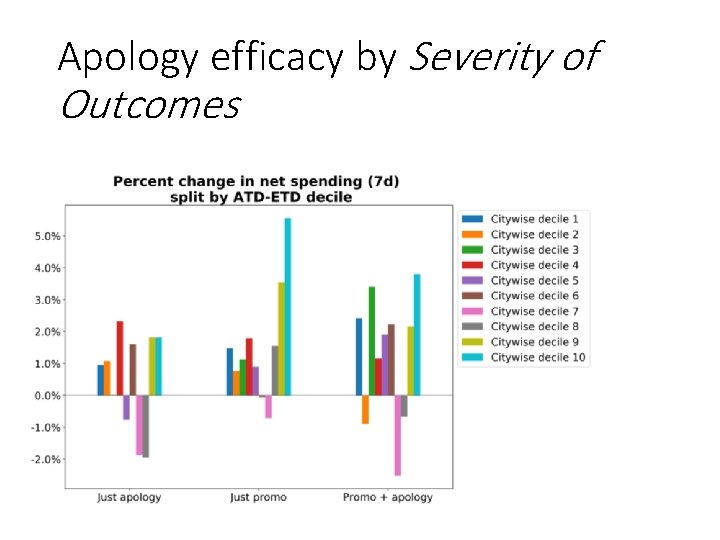
Apology efficacy by Severity of Outcomes
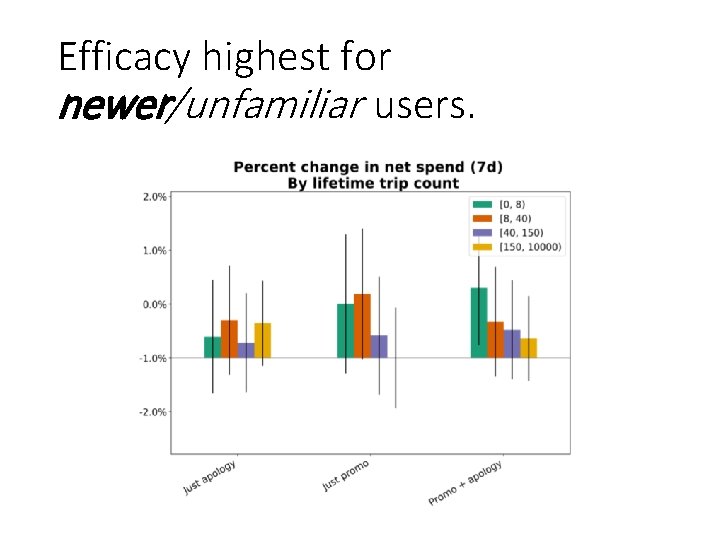
Efficacy highest for newer/unfamiliar users.
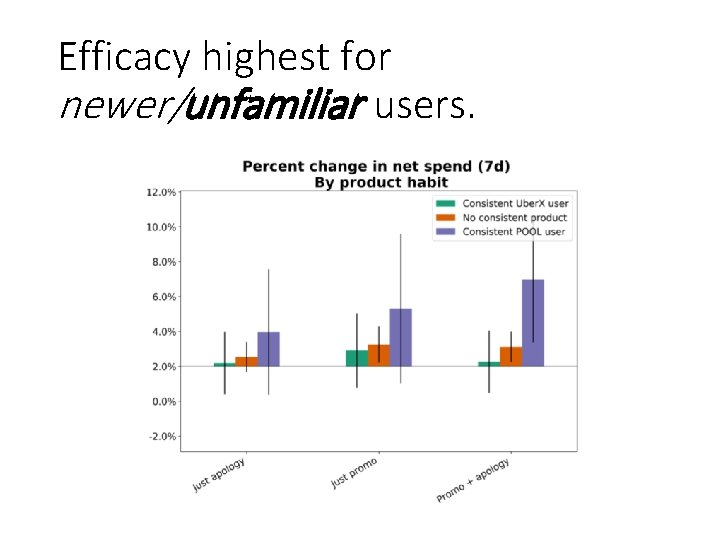
Efficacy highest for newer/unfamiliar users.
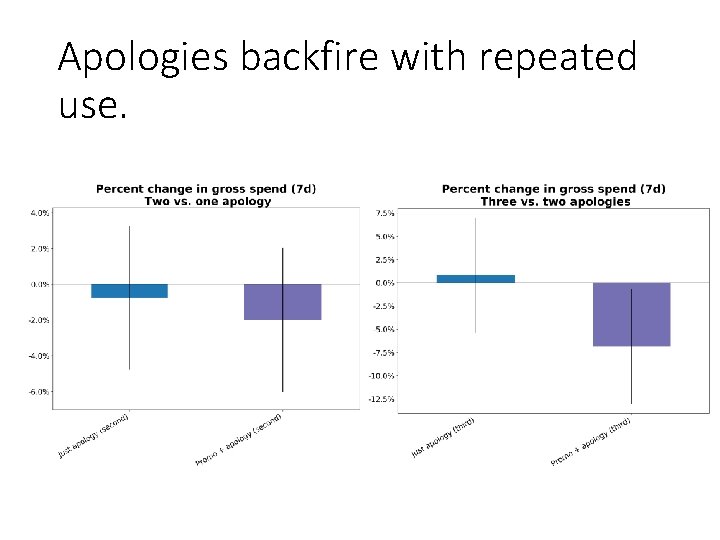
Apologies backfire with repeated use.
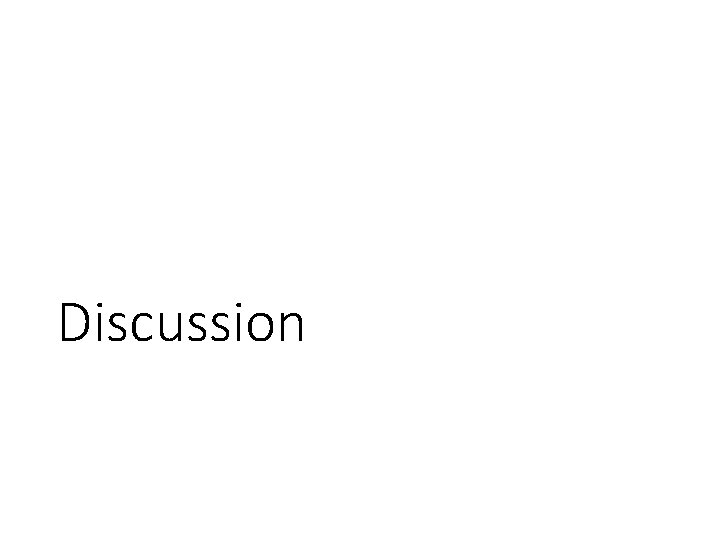
Discussion
![Placebo Test Generic 5 coupon small sample 6000 of similar customers in Miami Placebo Test: Generic $5 coupon (small sample [6000] of similar customers in Miami)](https://slidetodoc.com/presentation_image_h/82e53bf2226ff3481230e03f4ec9d414/image-38.jpg)
Placebo Test: Generic $5 coupon (small sample [6000] of similar customers in Miami)
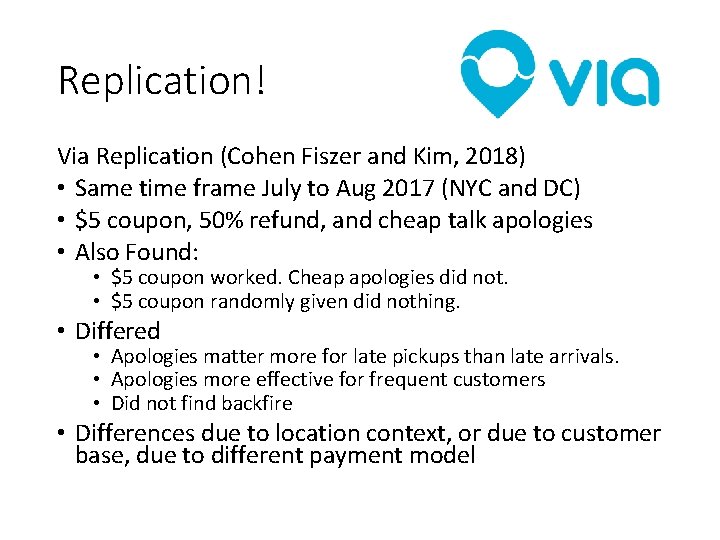
Replication! Via Replication (Cohen Fiszer and Kim, 2018) • Same time frame July to Aug 2017 (NYC and DC) • $5 coupon, 50% refund, and cheap talk apologies • Also Found: • $5 coupon worked. Cheap apologies did not. • $5 coupon randomly given did nothing. • Differed • Apologies matter more for late pickups than late arrivals. • Apologies more effective for frequent customers • Did not find backfire • Differences due to location context, or due to customer base, due to different payment model
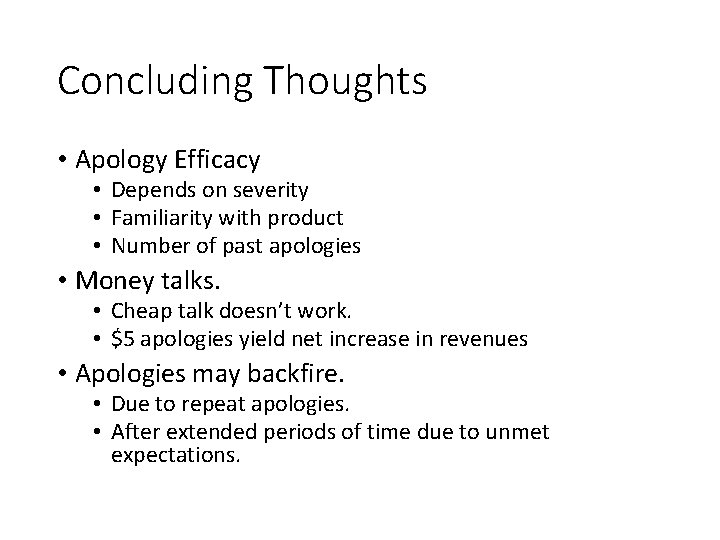
Concluding Thoughts • Apology Efficacy • Depends on severity • Familiarity with product • Number of past apologies • Money talks. • Cheap talk doesn’t work. • $5 apologies yield net increase in revenues • Apologies may backfire. • Due to repeat apologies. • After extended periods of time due to unmet expectations.
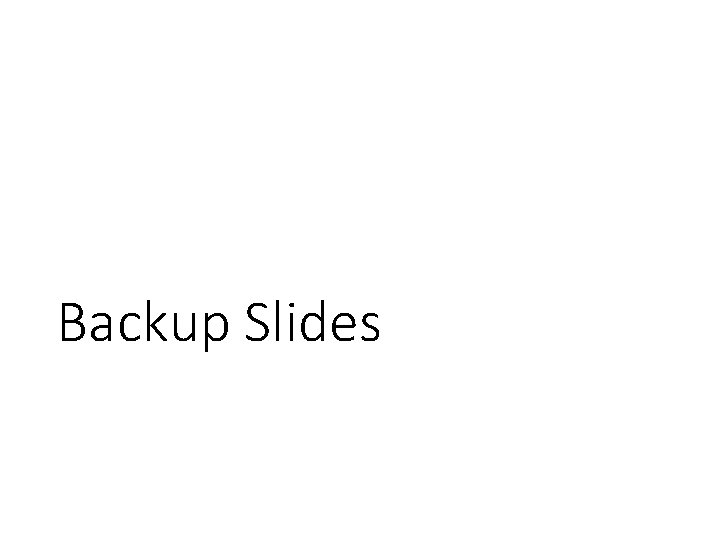
Backup Slides
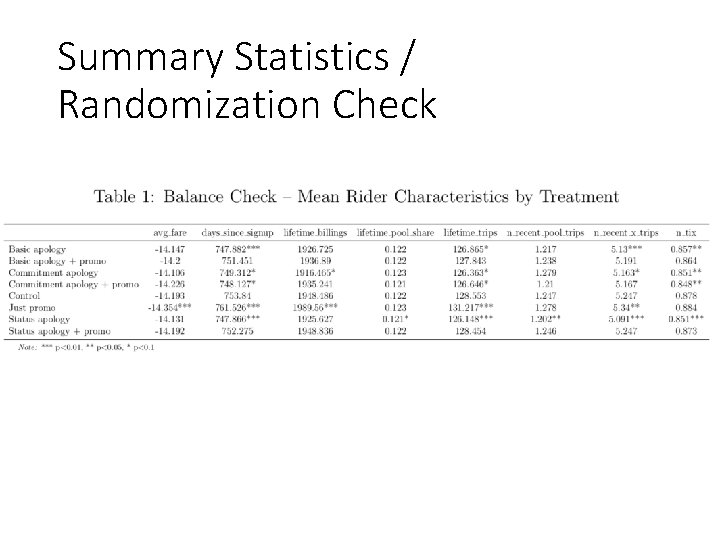
Summary Statistics / Randomization Check
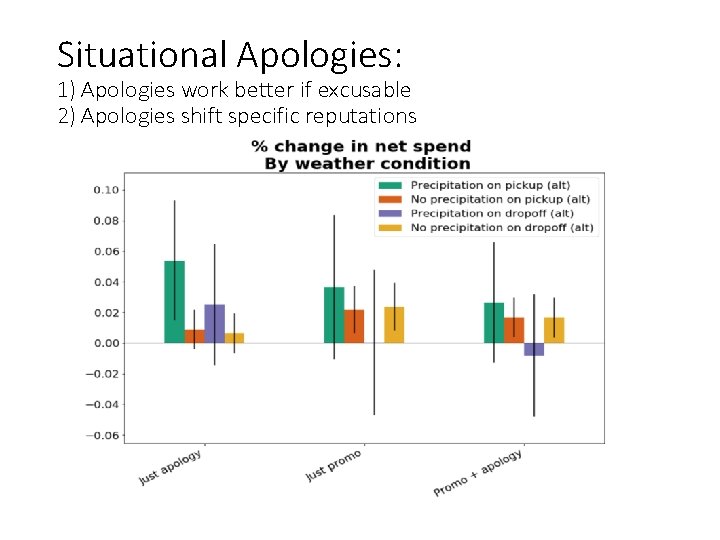
Situational Apologies: 1) Apologies work better if excusable 2) Apologies shift specific reputations
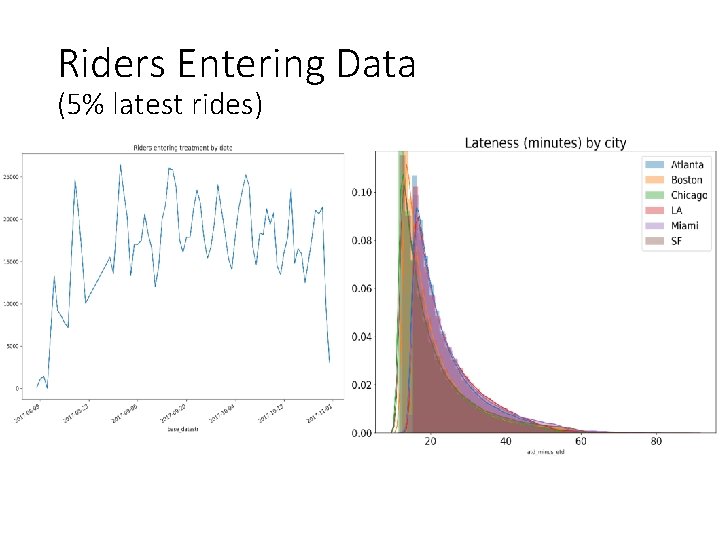
Riders Entering Data (5% latest rides)
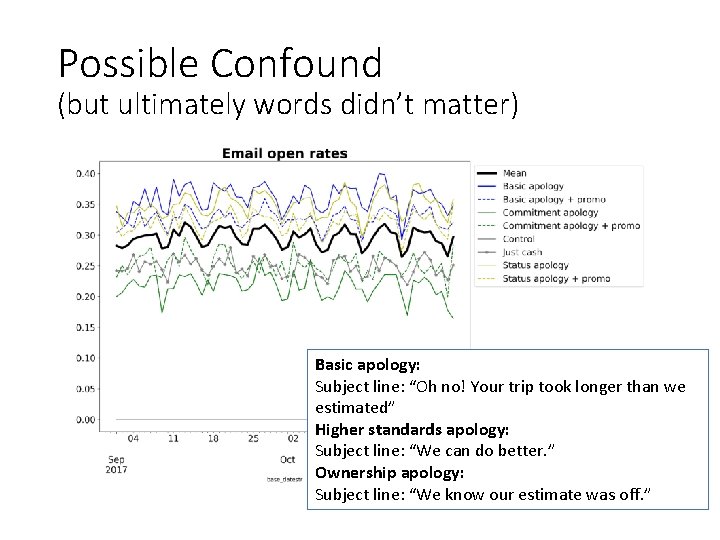
Possible Confound (but ultimately words didn’t matter) Basic apology: Subject line: “Oh no! Your trip took longer than we estimated” Higher standards apology: Subject line: “We can do better. ” Ownership apology: Subject line: “We know our estimate was off. ”
I owe you apologies
Yoshiko imamoto
Economics chapter 4 section 1 understanding demand answers
Economics and business economics maastricht
Elements of mathematical economics
Mật thư tọa độ 5x5
Tư thế ngồi viết
Giọng cùng tên là
Voi kéo gỗ như thế nào
Thẻ vin
Thơ thất ngôn tứ tuyệt đường luật
Hổ đẻ mỗi lứa mấy con
Từ ngữ thể hiện lòng nhân hậu
Thế nào là hệ số cao nhất
Diễn thế sinh thái là
Slidetodoc
Vẽ hình chiếu vuông góc của vật thể sau
Làm thế nào để 102-1=99
Lời thề hippocrates
Vẽ hình chiếu đứng bằng cạnh của vật thể
Tư thế worms-breton
đại từ thay thế
Quá trình desamine hóa có thể tạo ra
Công của trọng lực
Sự nuôi và dạy con của hổ
Thế nào là mạng điện lắp đặt kiểu nổi
Các châu lục và đại dương trên thế giới
Dot
Bổ thể
Thế nào là sự mỏi cơ
độ dài liên kết
Thiếu nhi thế giới liên hoan
Chúa yêu trần thế alleluia
điện thế nghỉ
Một số thể thơ truyền thống
Trời xanh đây là của chúng ta thể thơ
Sơ đồ cơ thể người
Số nguyên tố là gì
đặc điểm cơ thể của người tối cổ
Tỉ lệ cơ thể trẻ em
Vẽ hình chiếu vuông góc của vật thể sau
Các châu lục và đại dương trên thế giới
ưu thế lai là gì
Tư thế ngồi viết
Môn thể thao bắt đầu bằng từ chạy
Hình ảnh bộ gõ cơ thể búng tay