IS 4800 Empirical Research Methods for Information Science
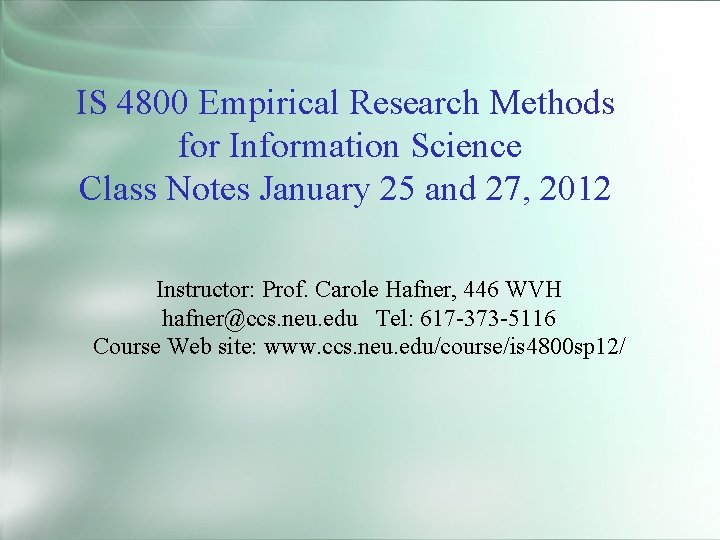
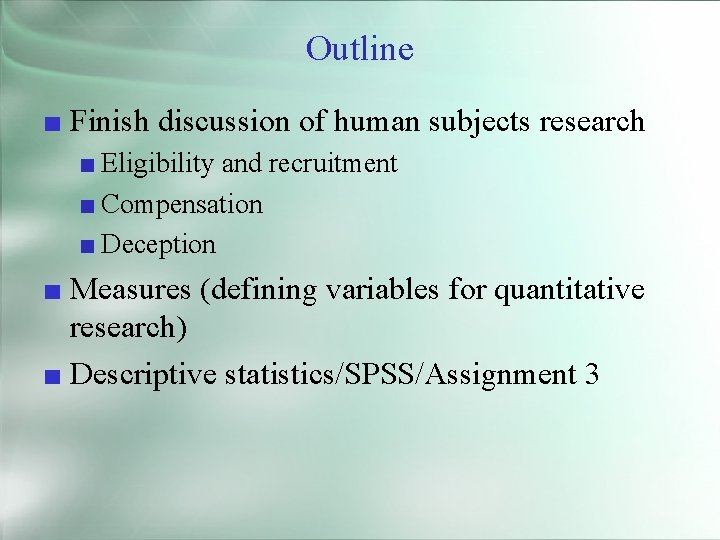
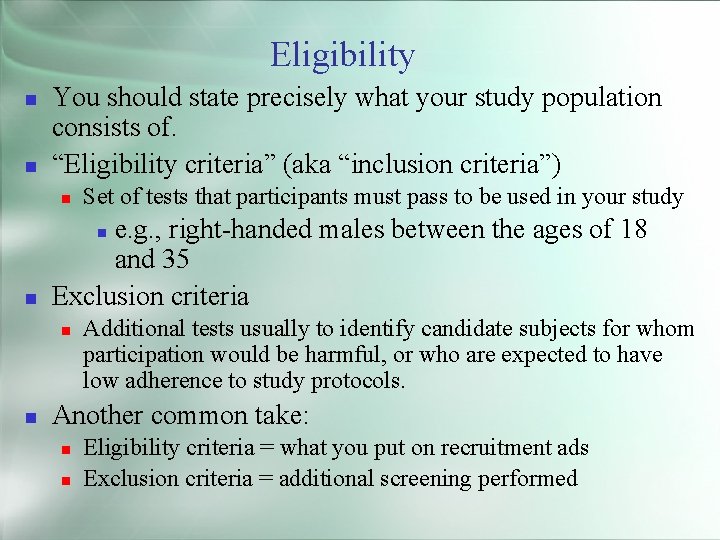
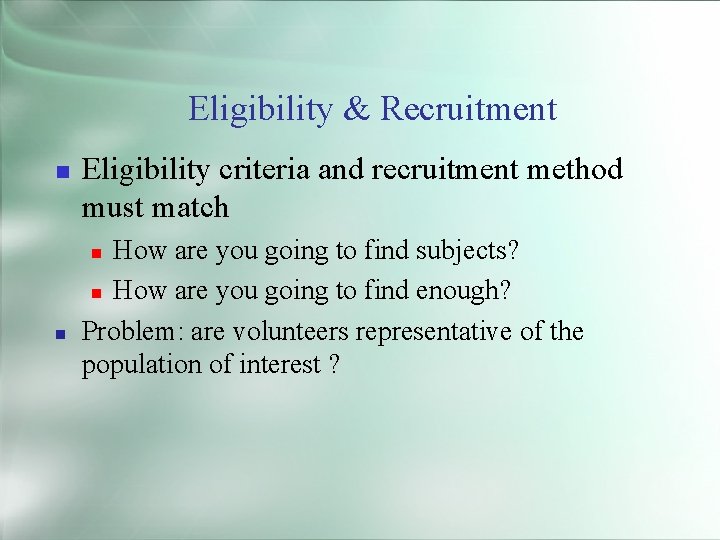
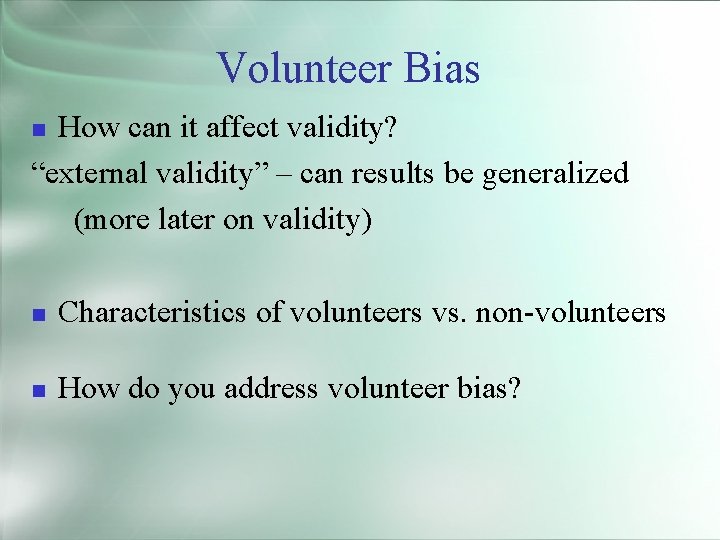
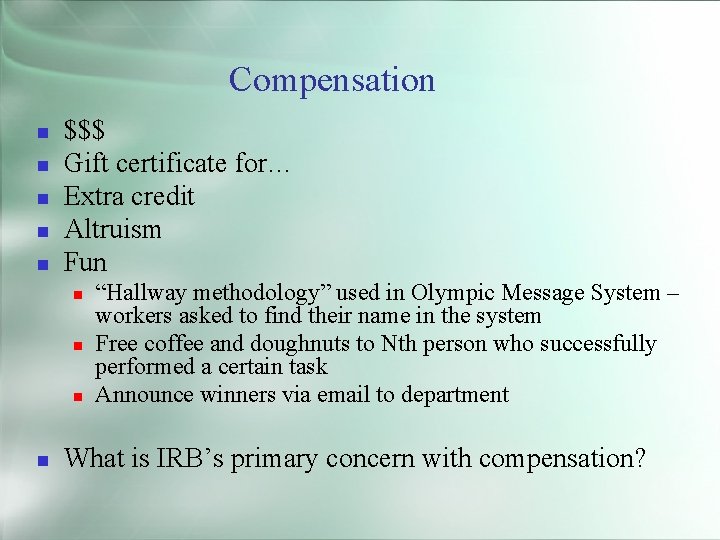
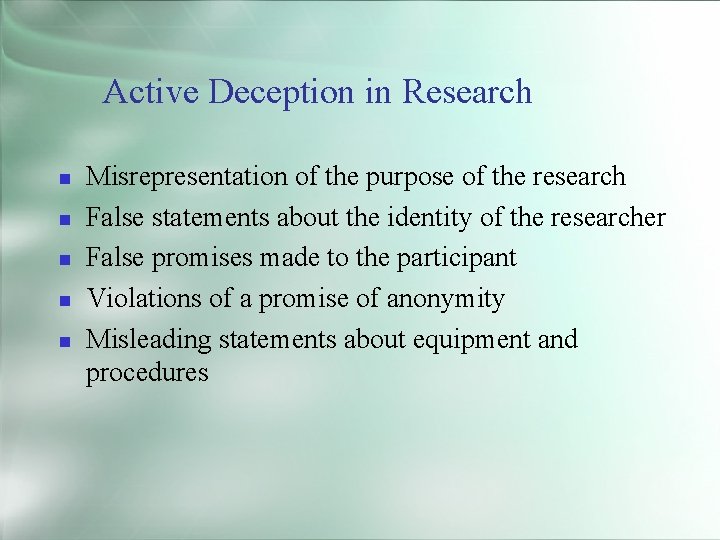
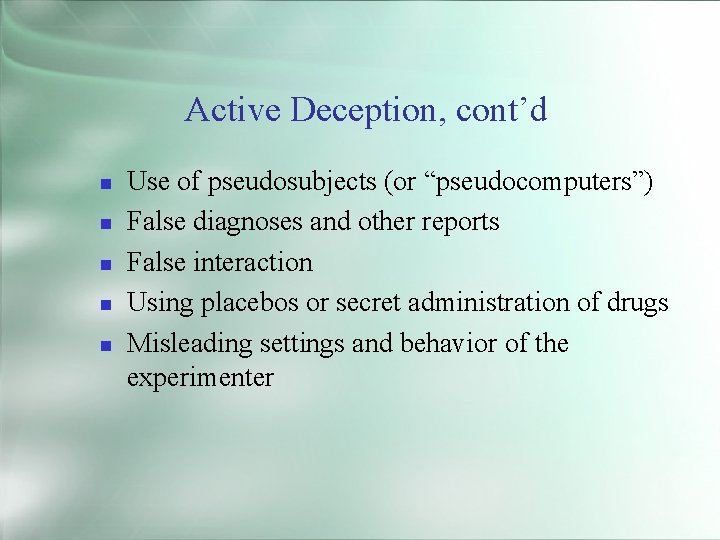
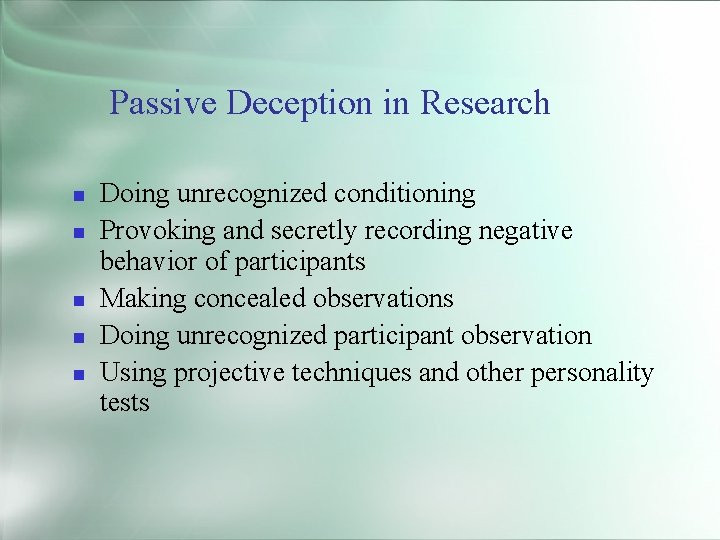
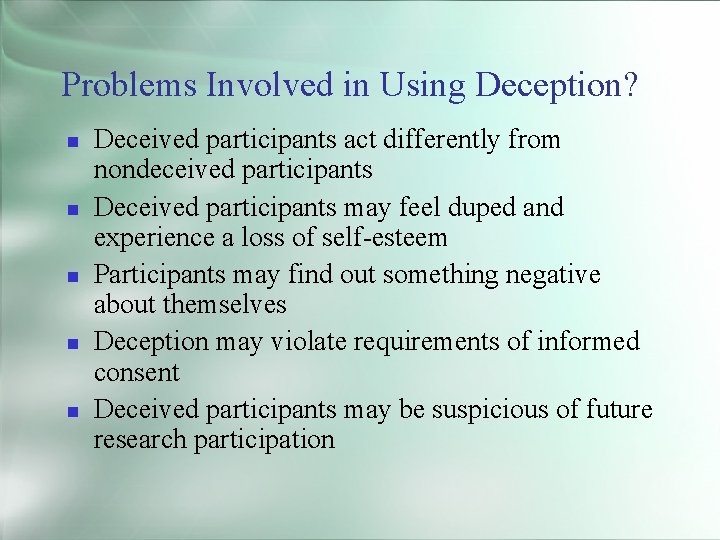
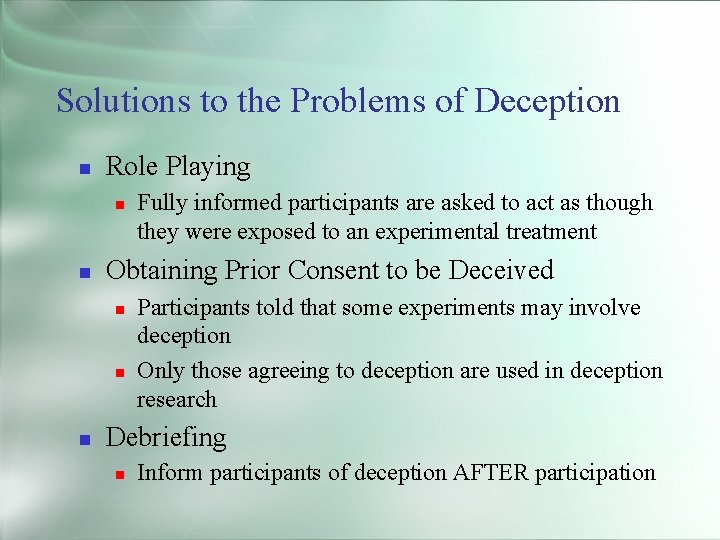
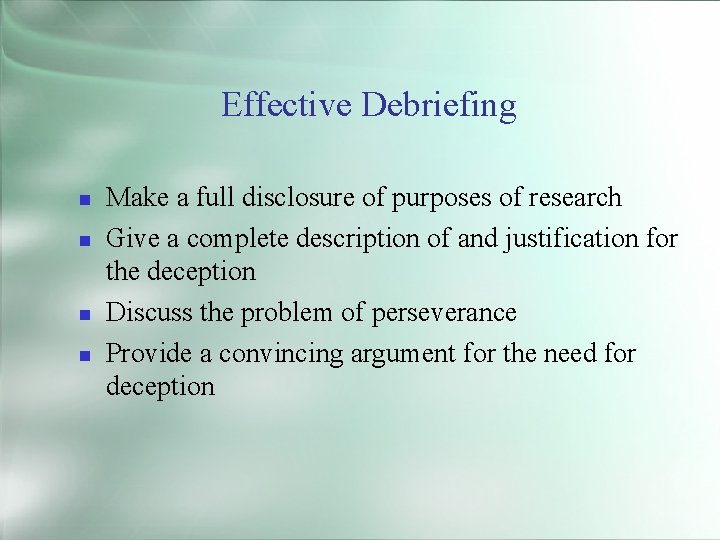
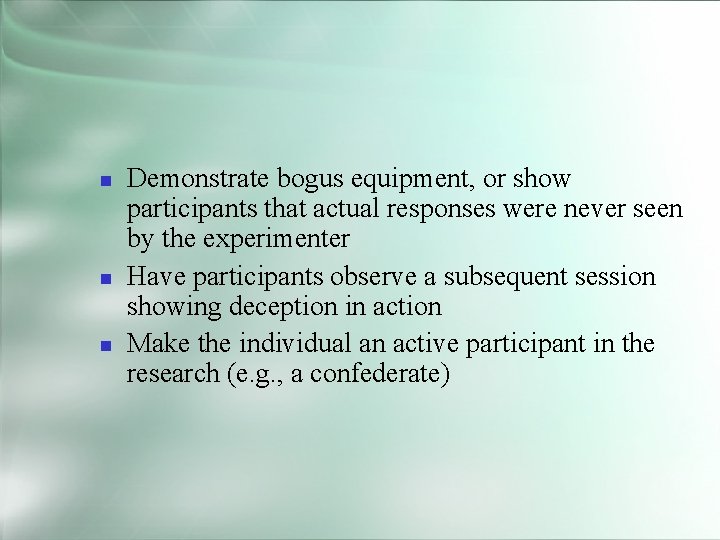
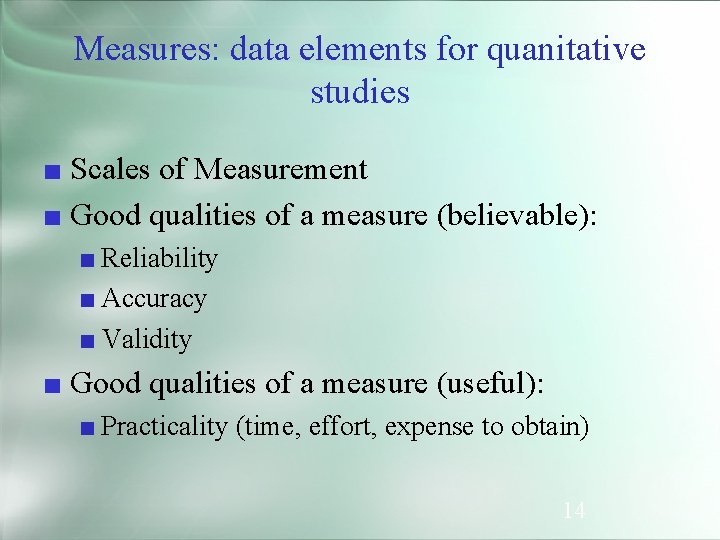
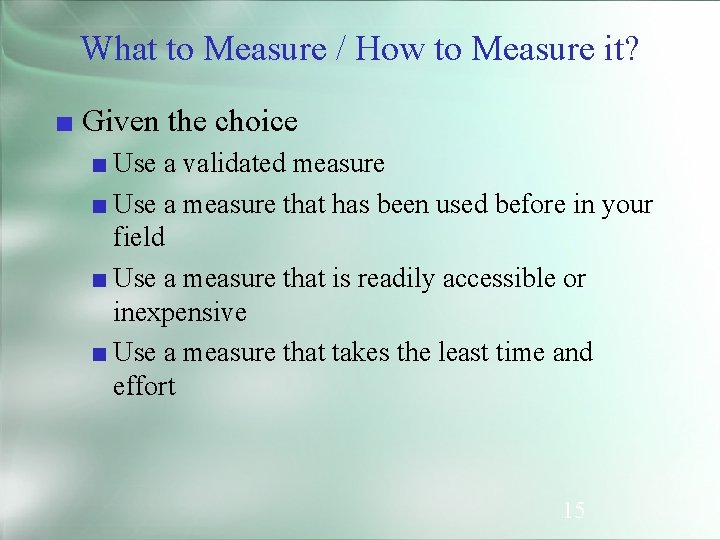
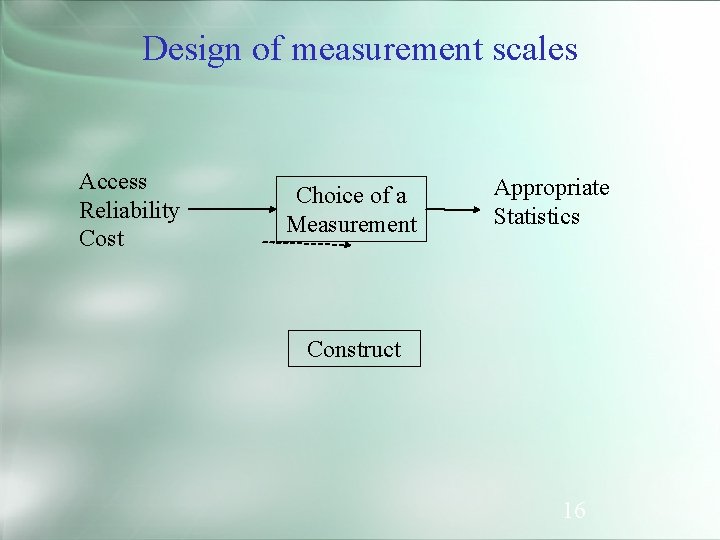
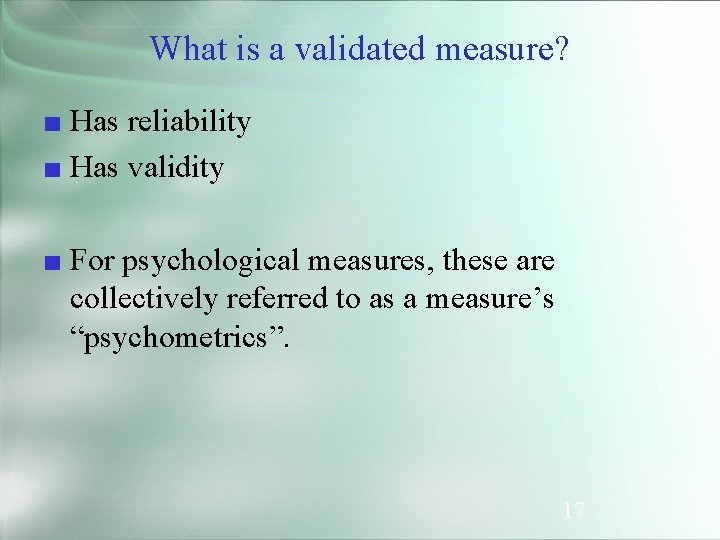
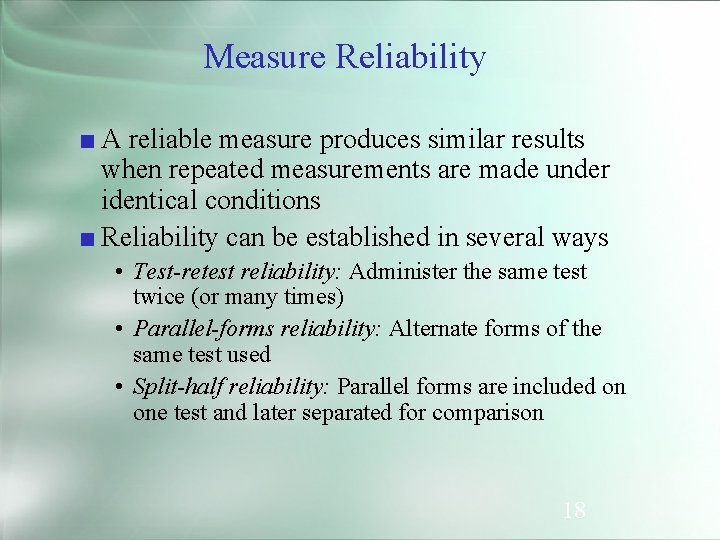
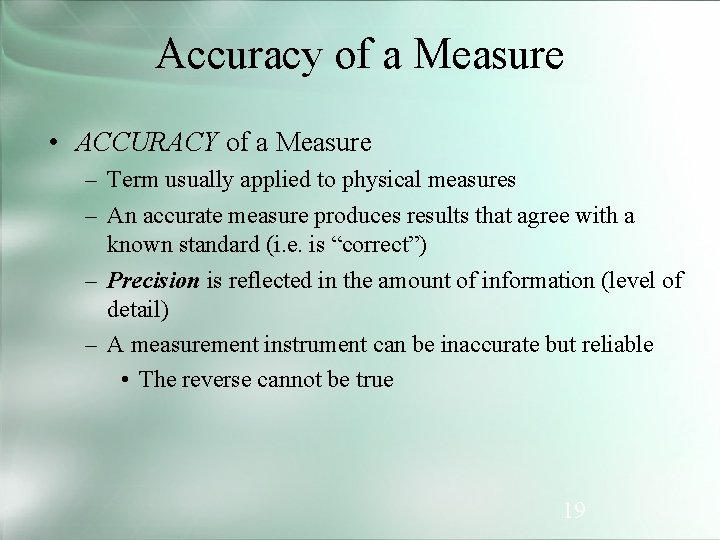
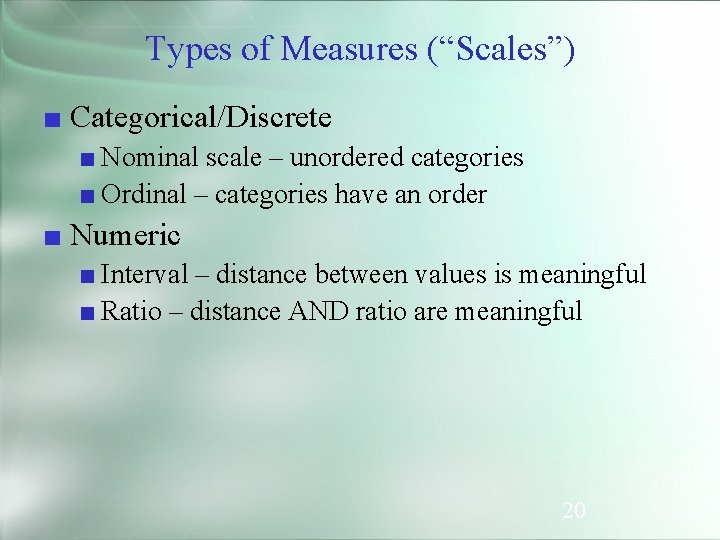
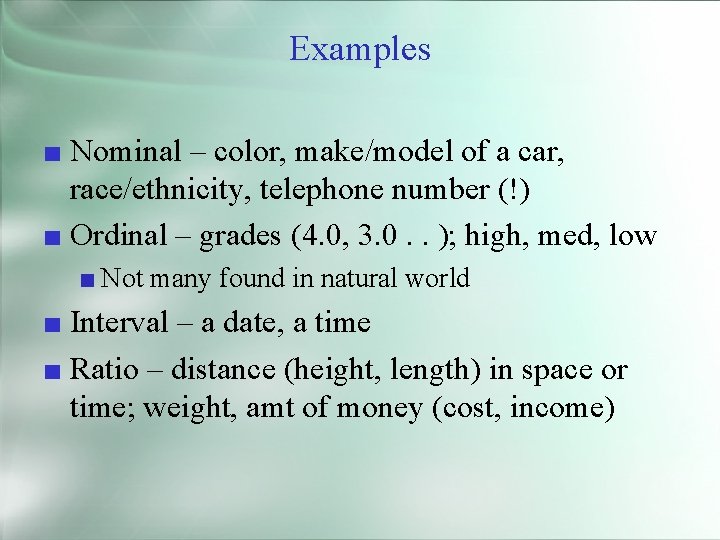
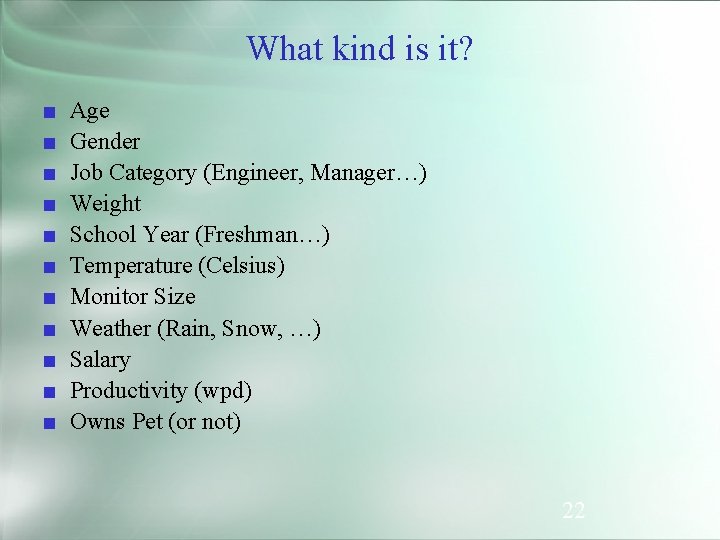
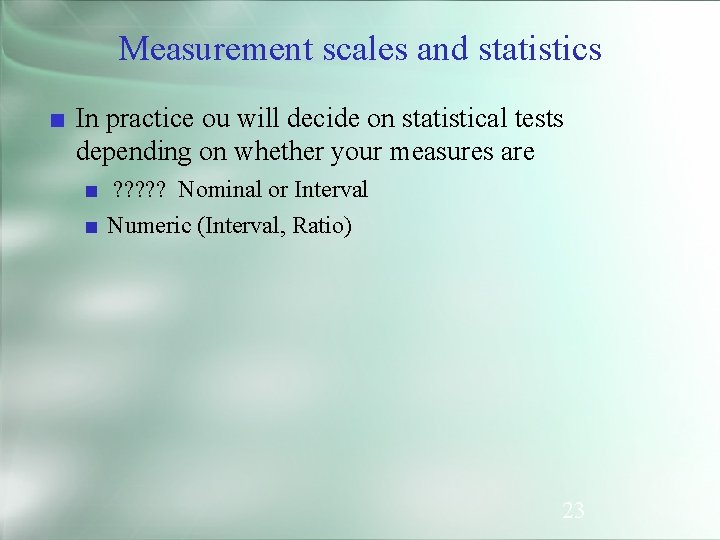
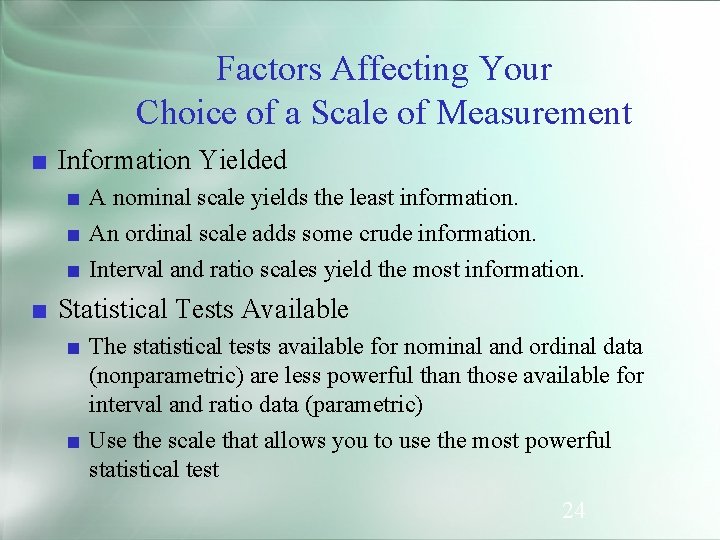
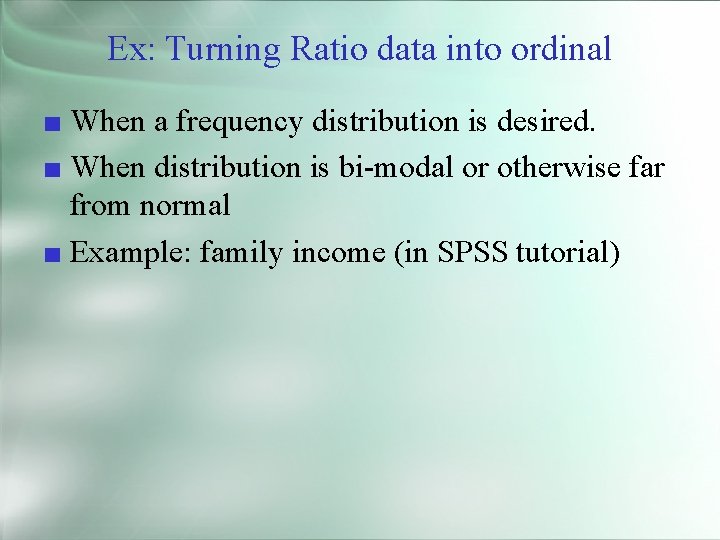
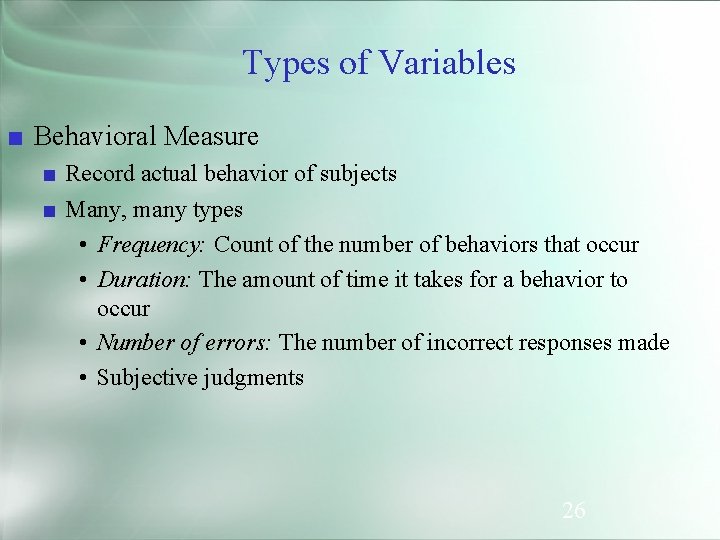
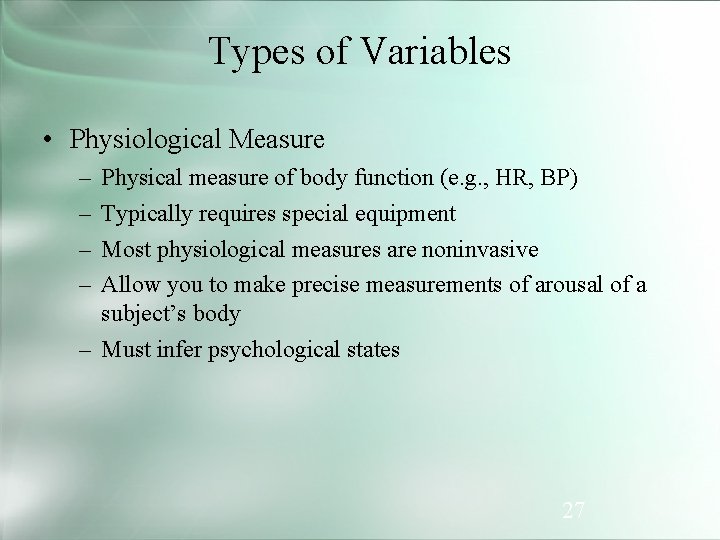
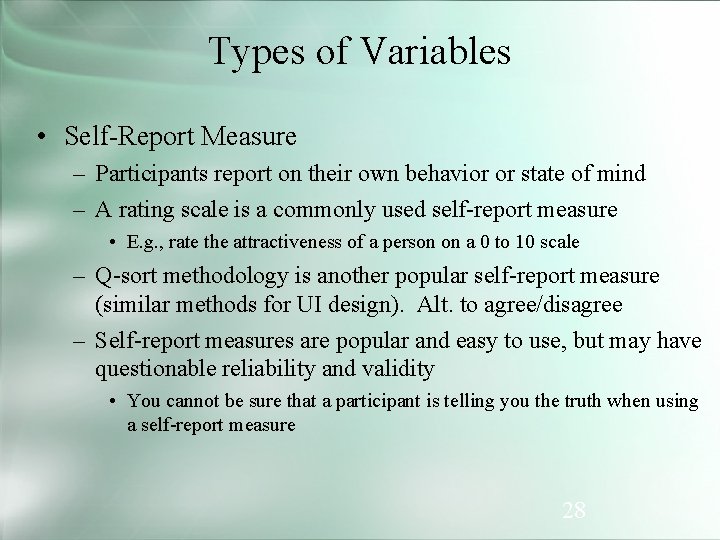
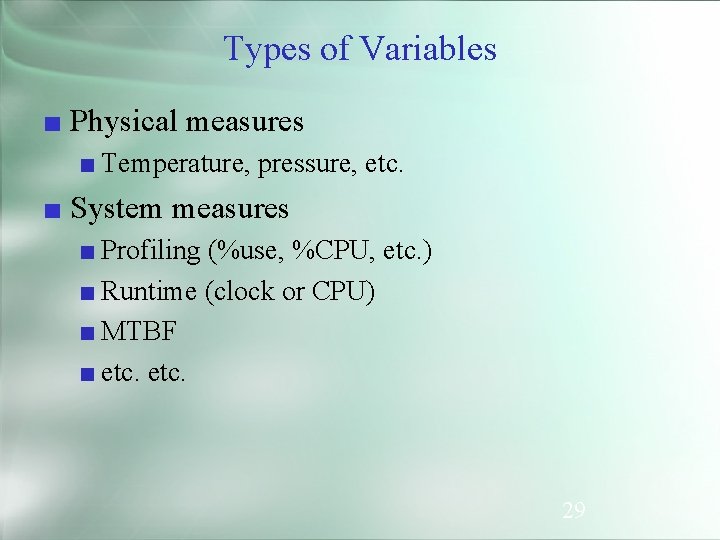
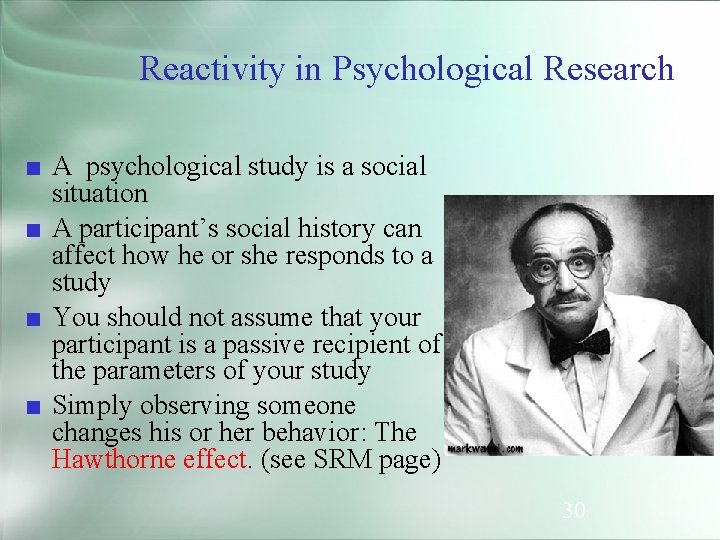
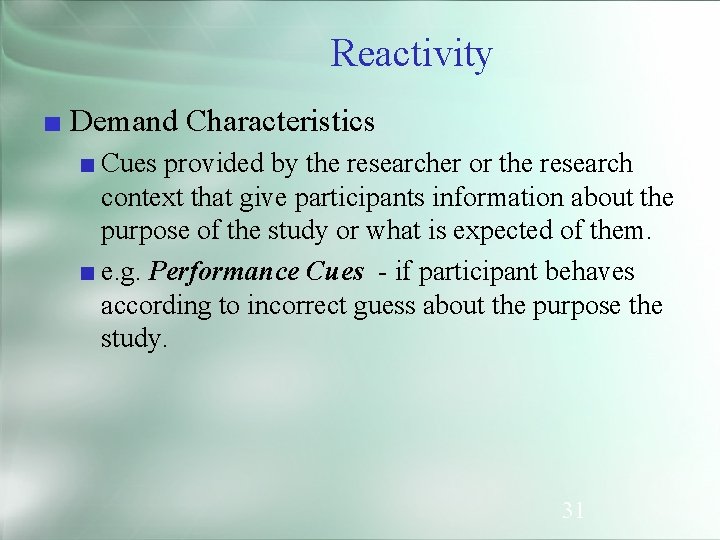
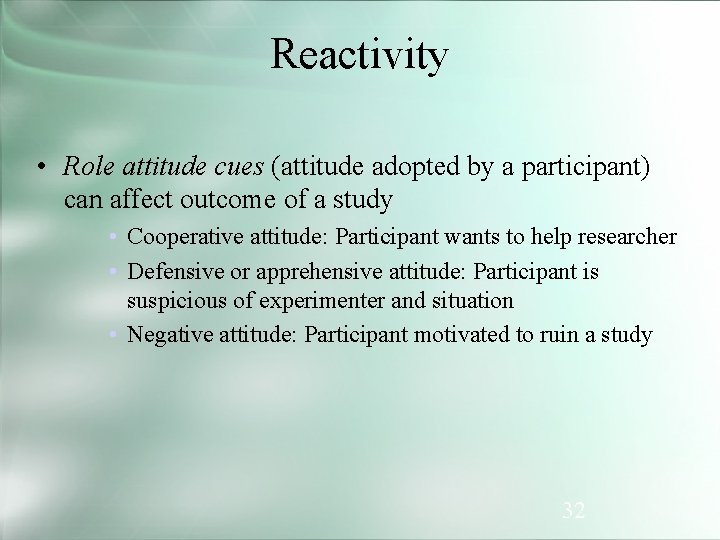
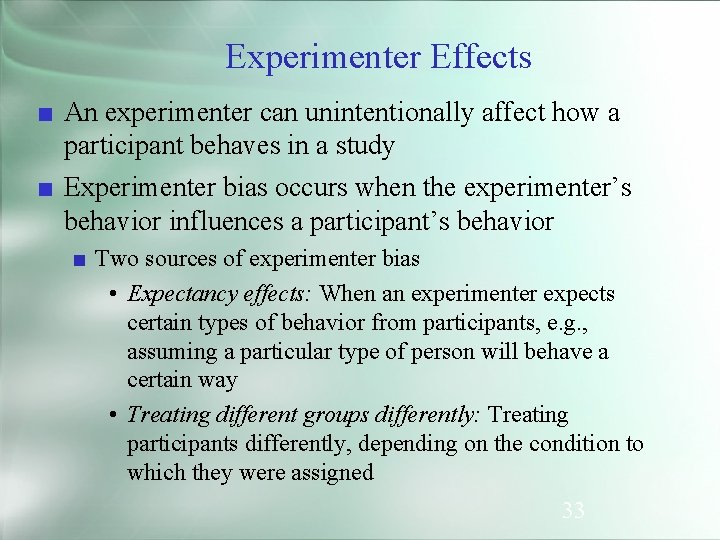
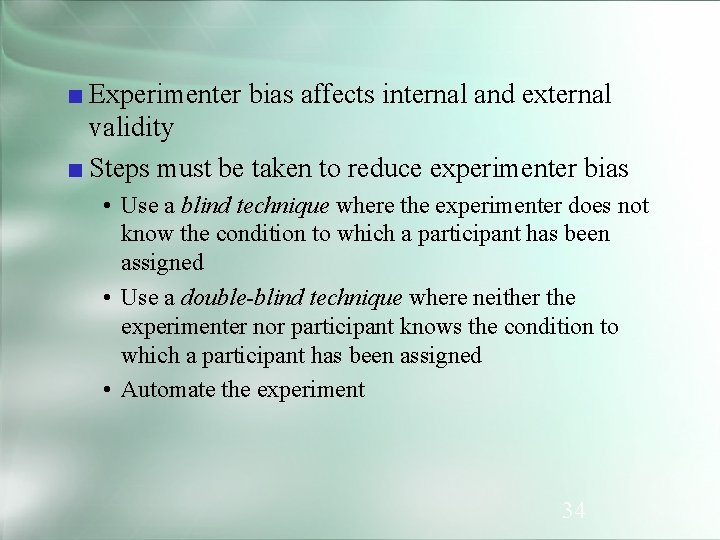
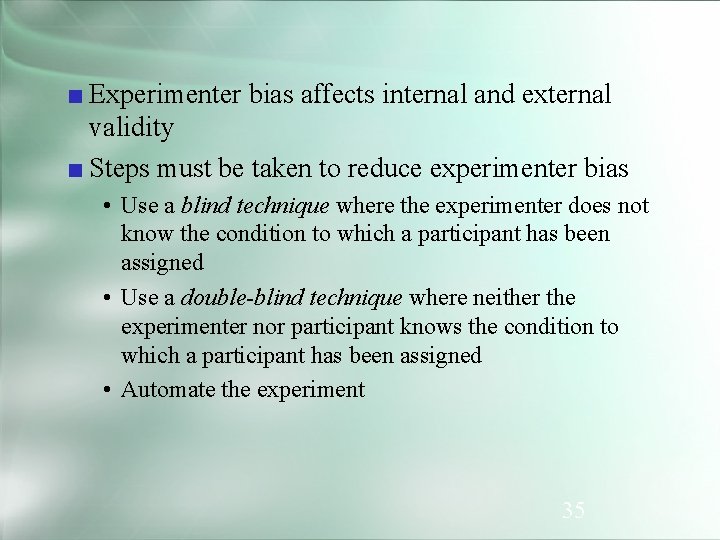
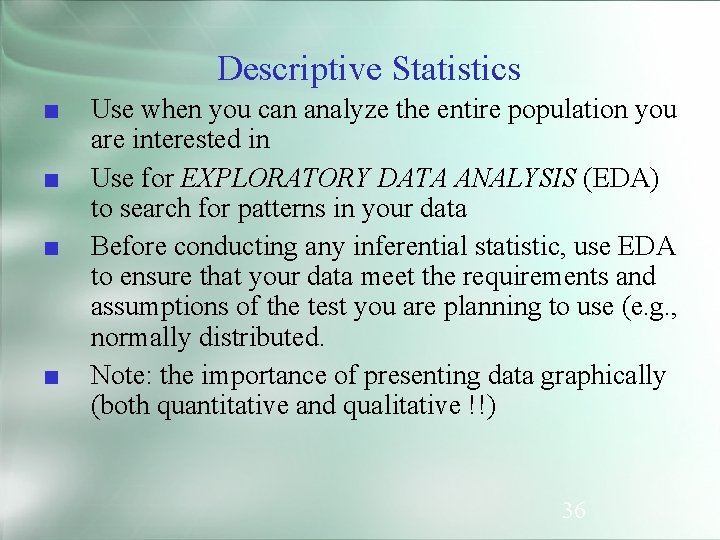
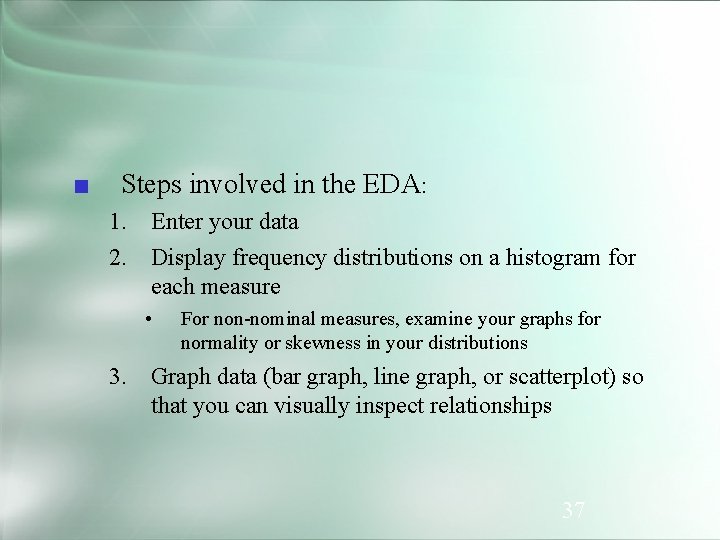
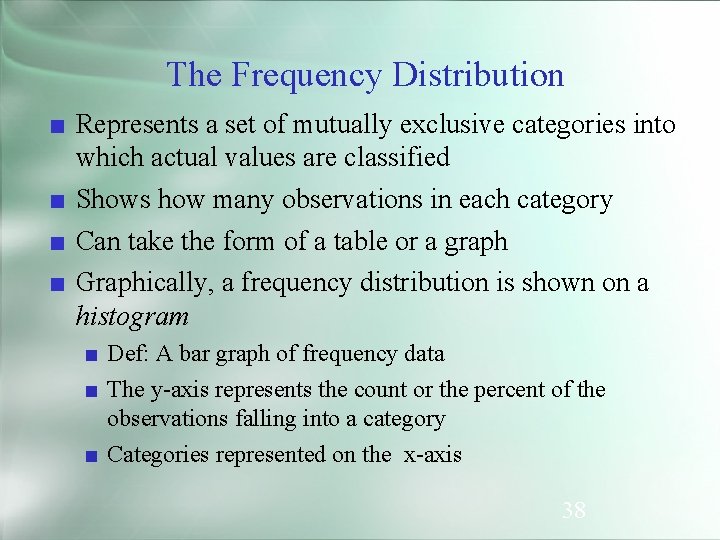
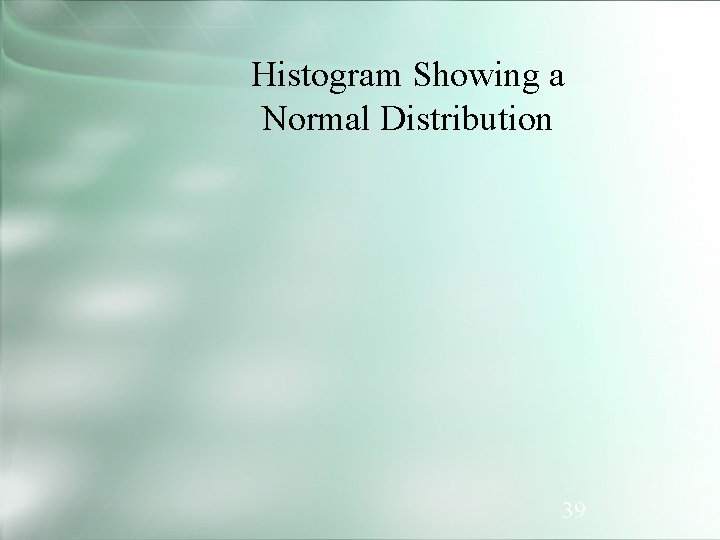
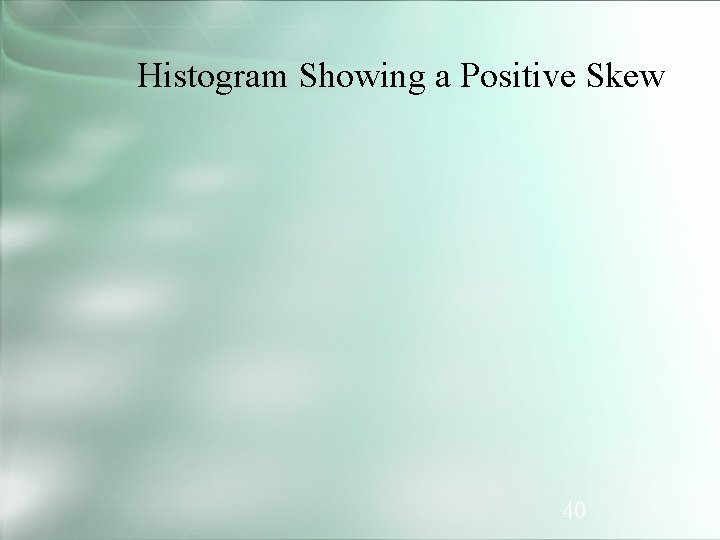
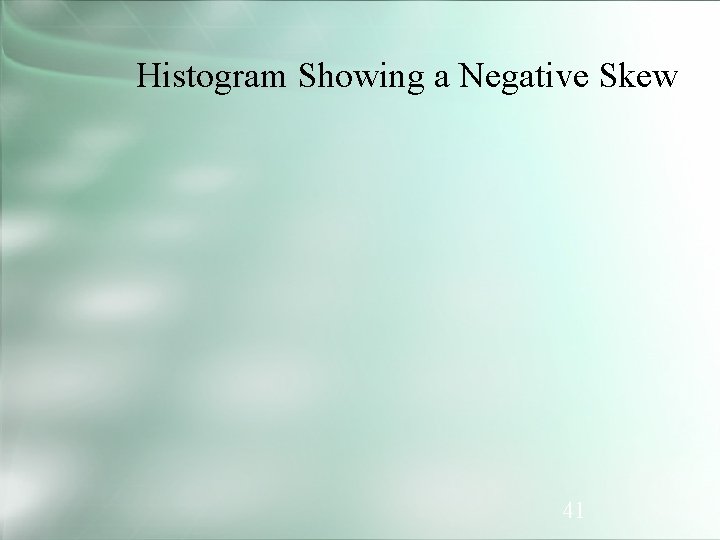
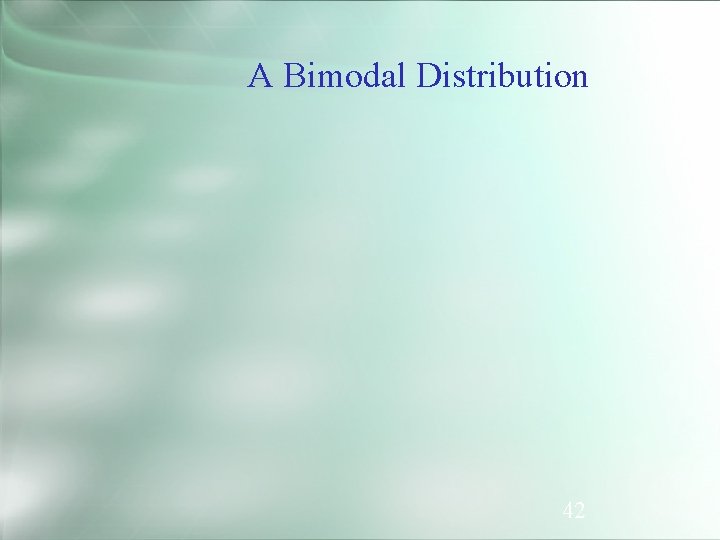
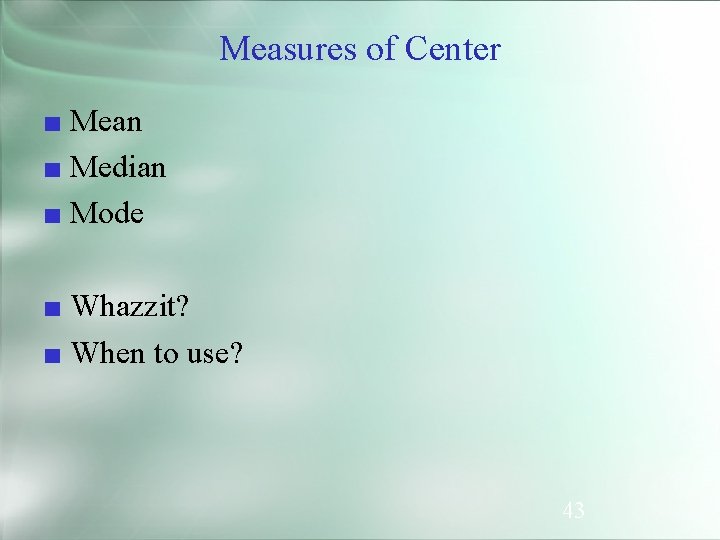
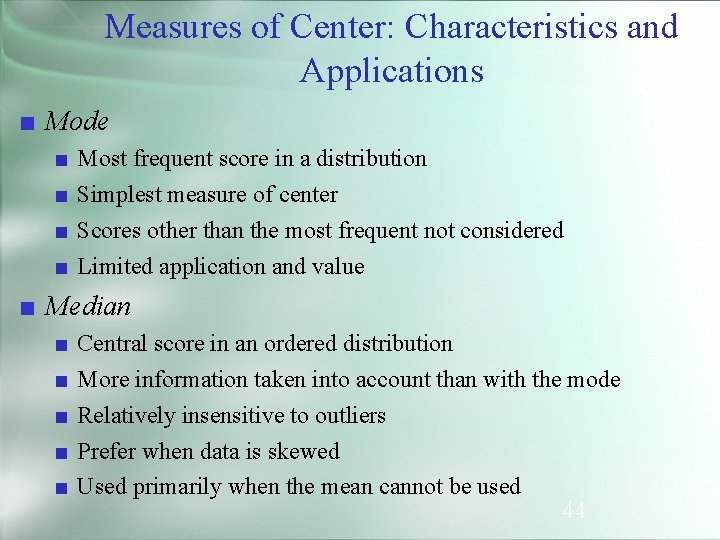
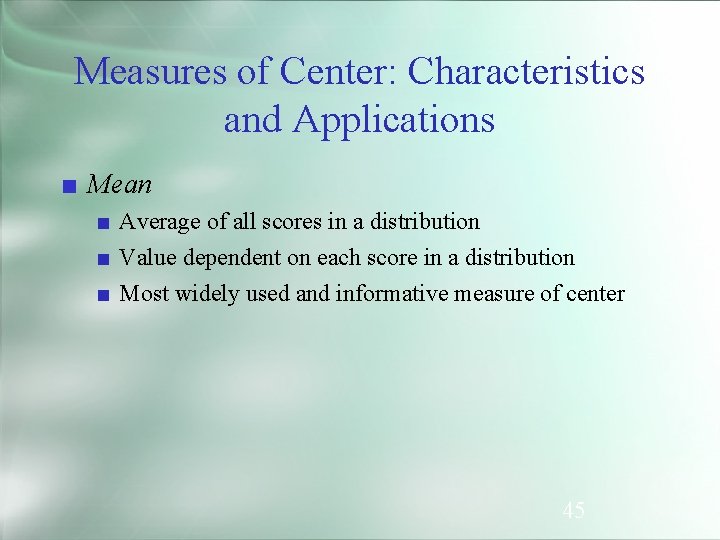
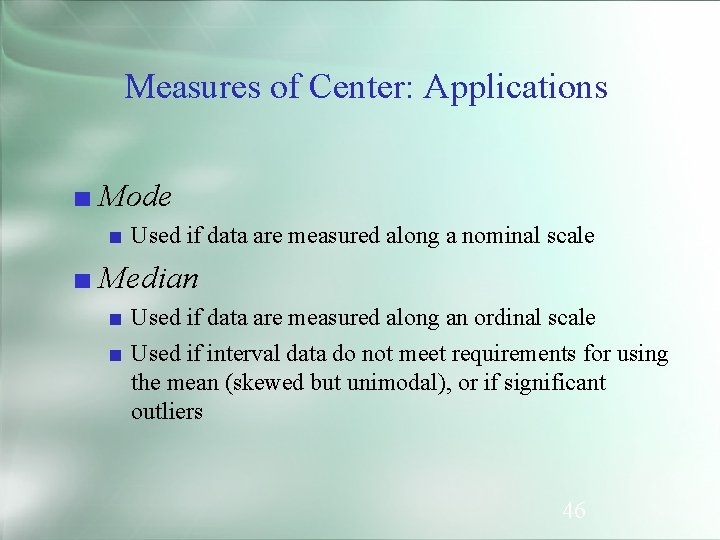
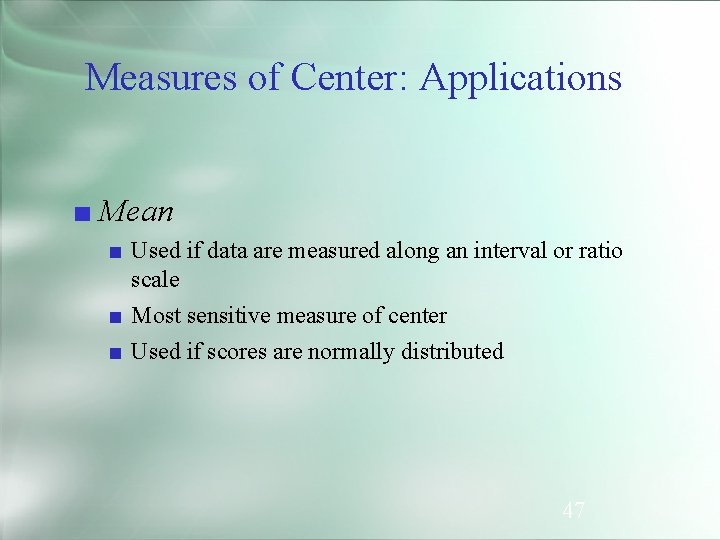
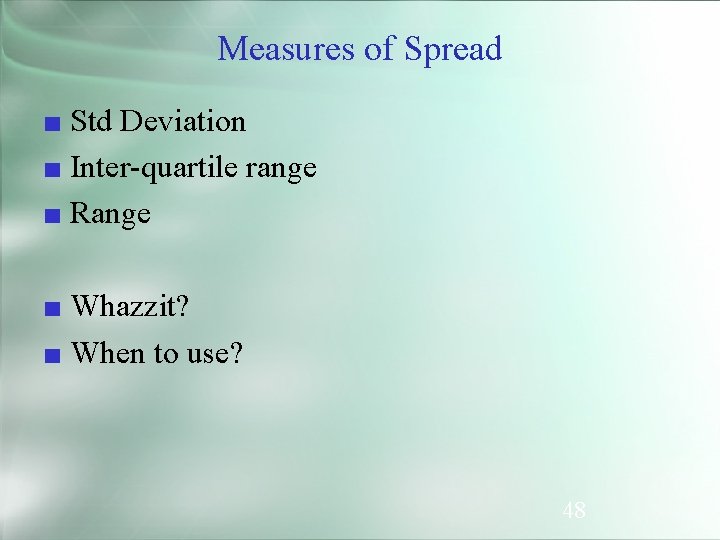
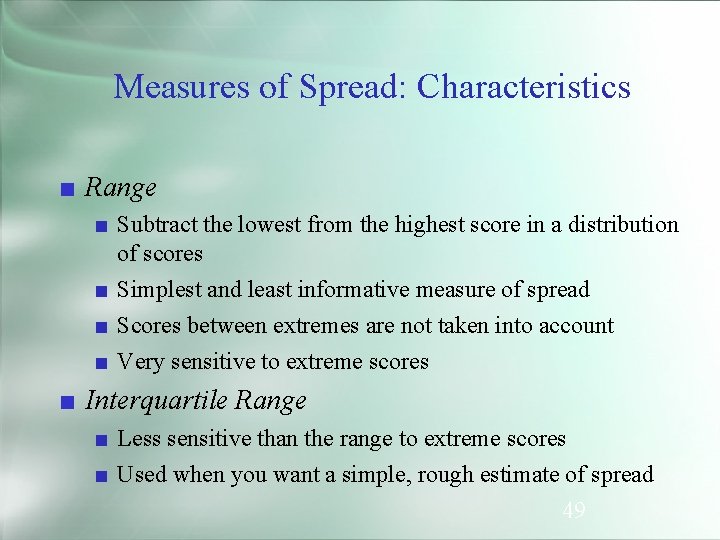
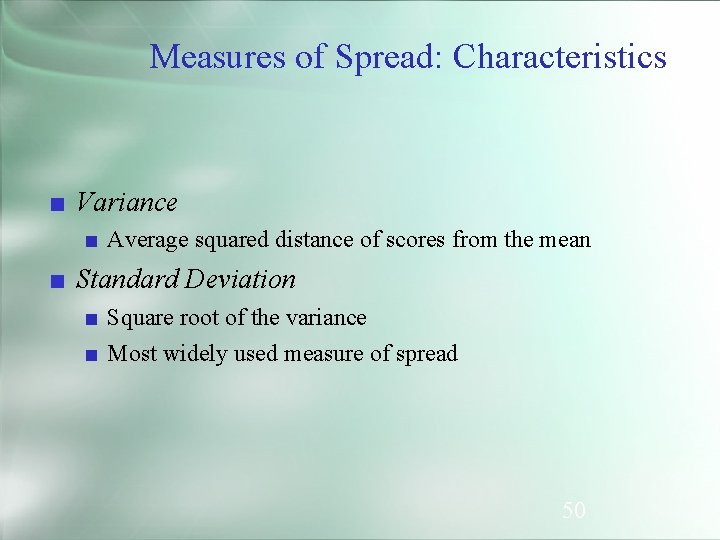
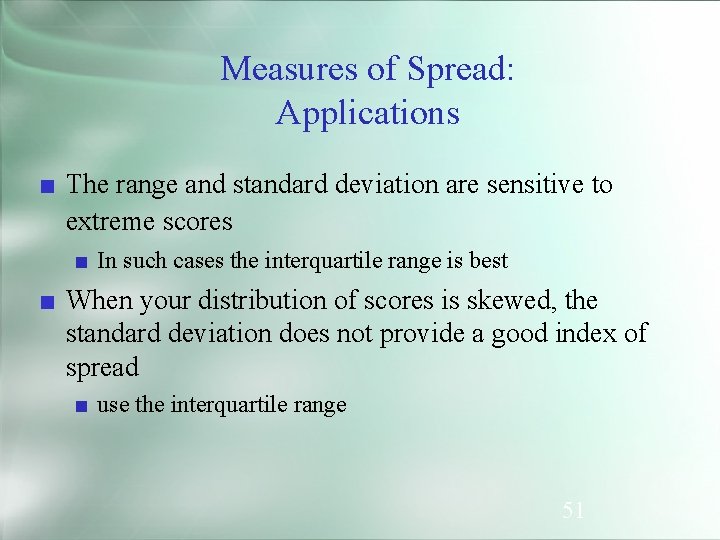
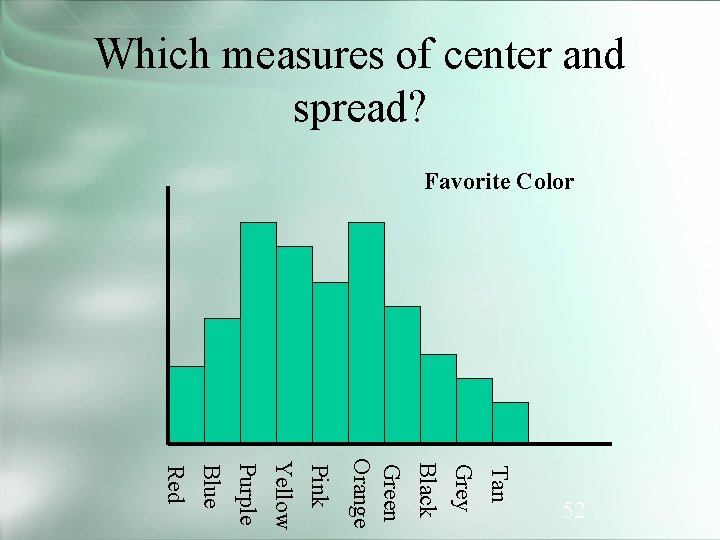
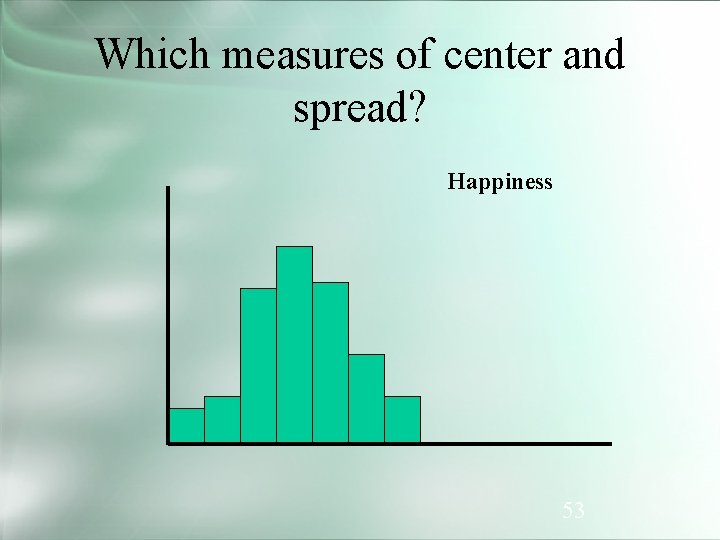
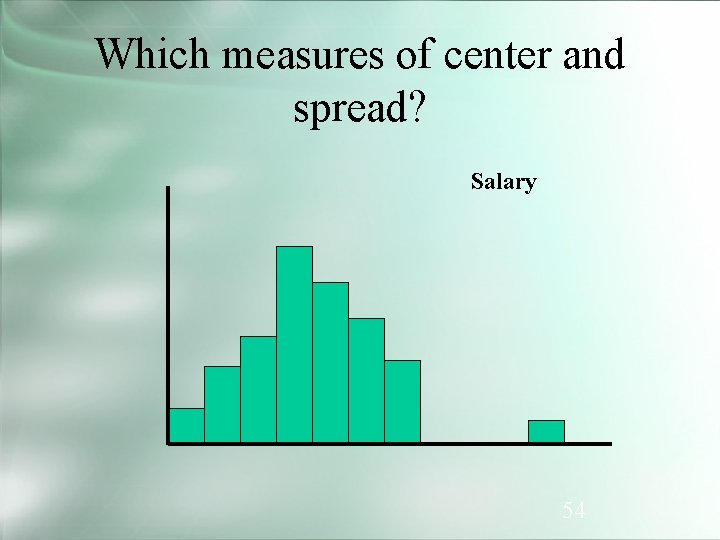
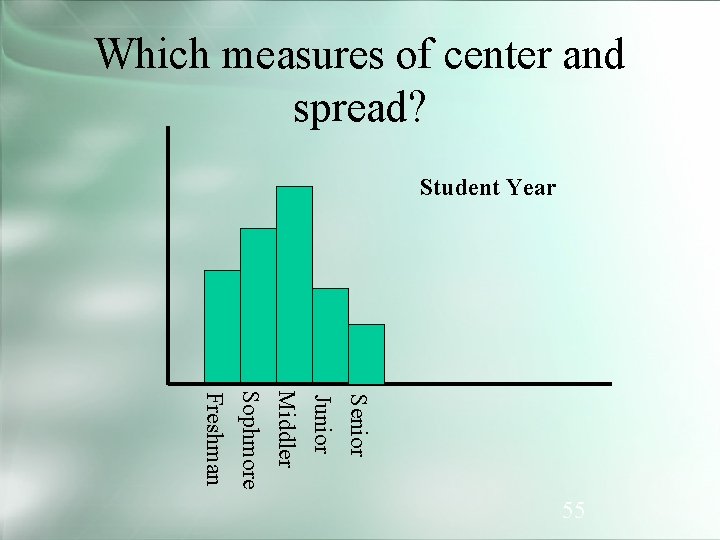
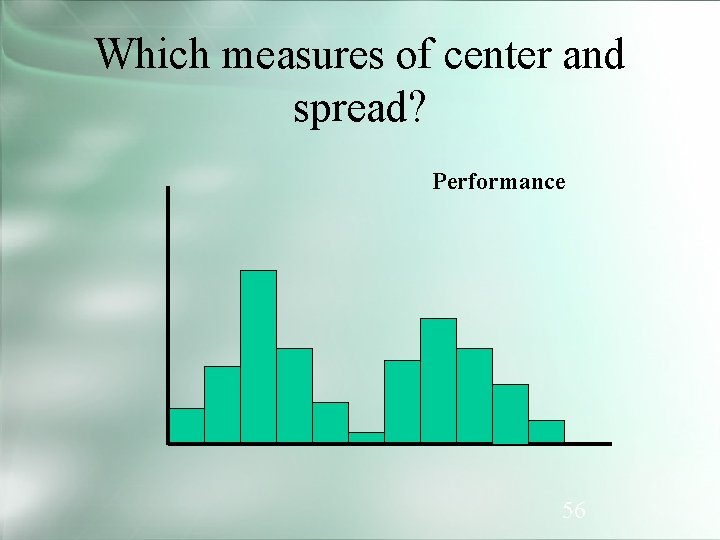
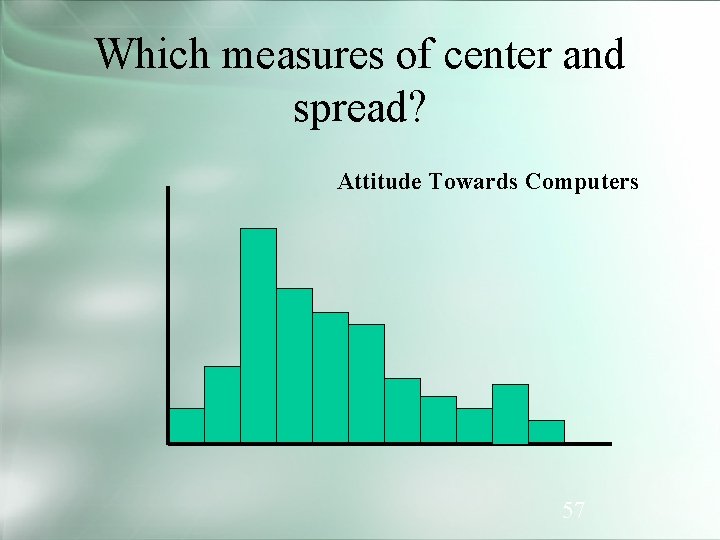
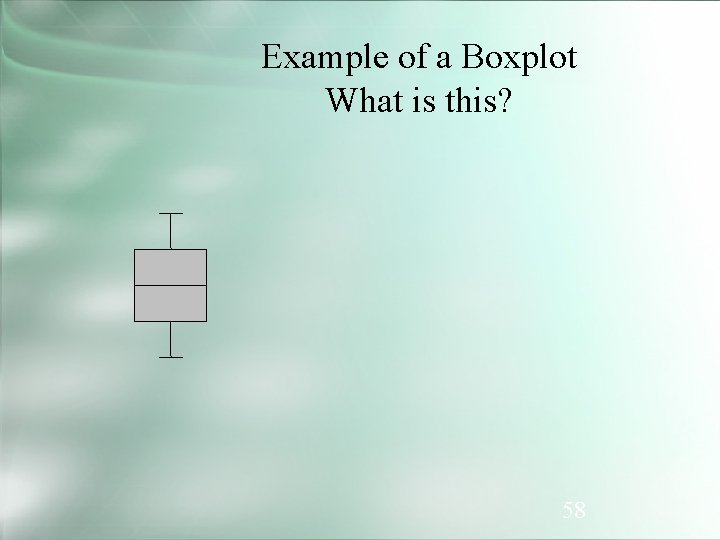
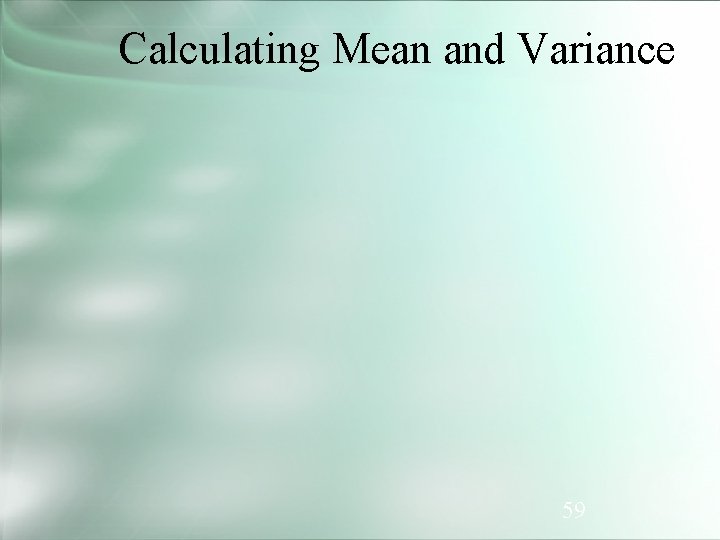
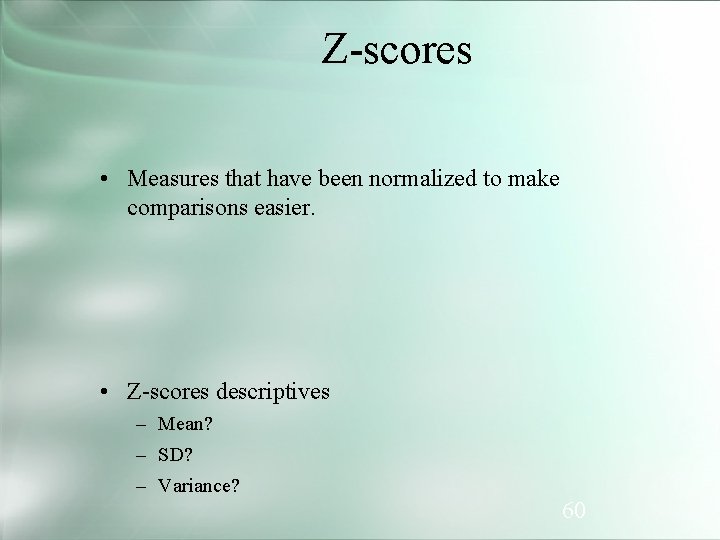
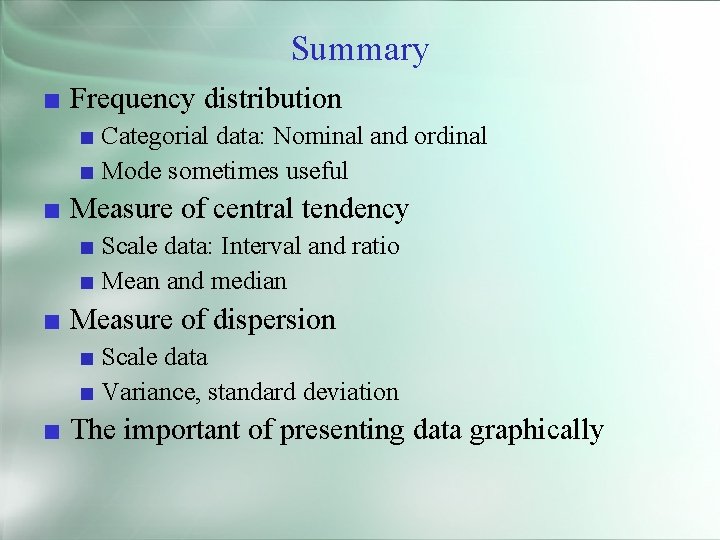
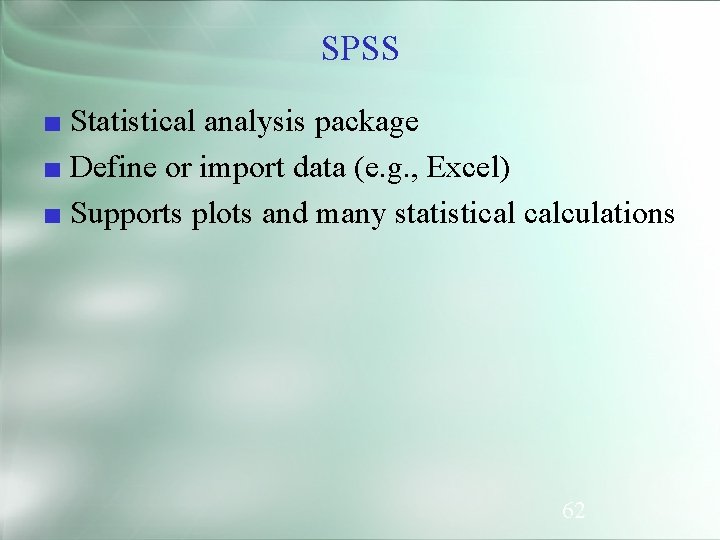
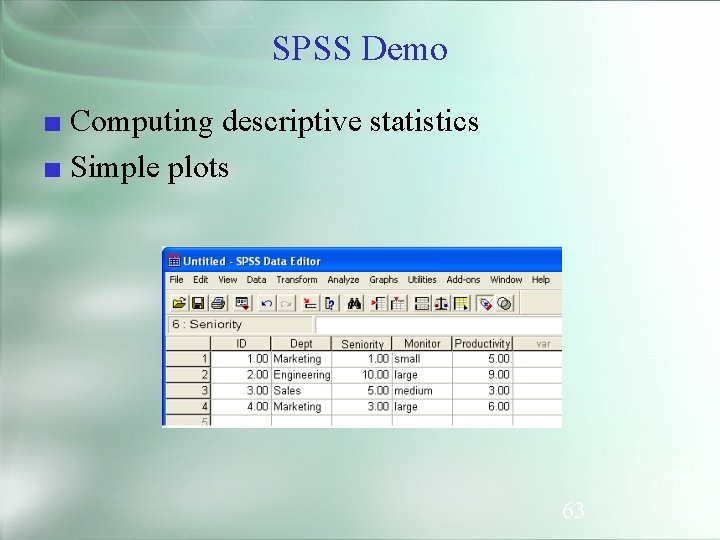
- Slides: 63
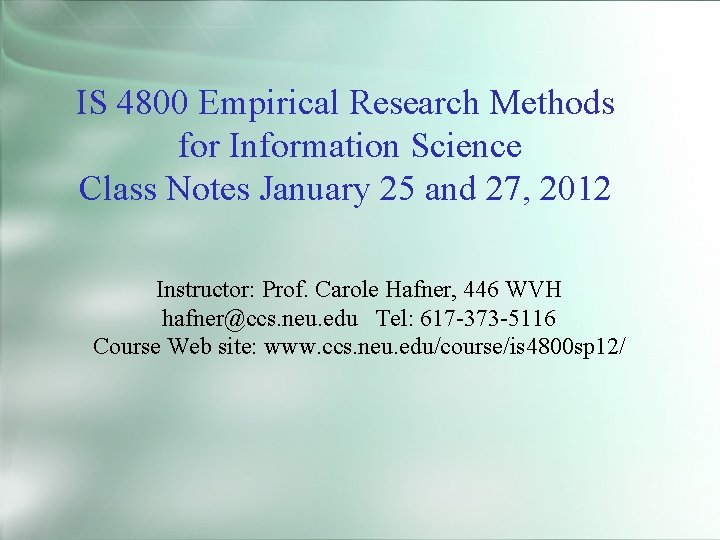
IS 4800 Empirical Research Methods for Information Science Class Notes January 25 and 27, 2012 Instructor: Prof. Carole Hafner, 446 WVH hafner@ccs. neu. edu Tel: 617 -373 -5116 Course Web site: www. ccs. neu. edu/course/is 4800 sp 12/
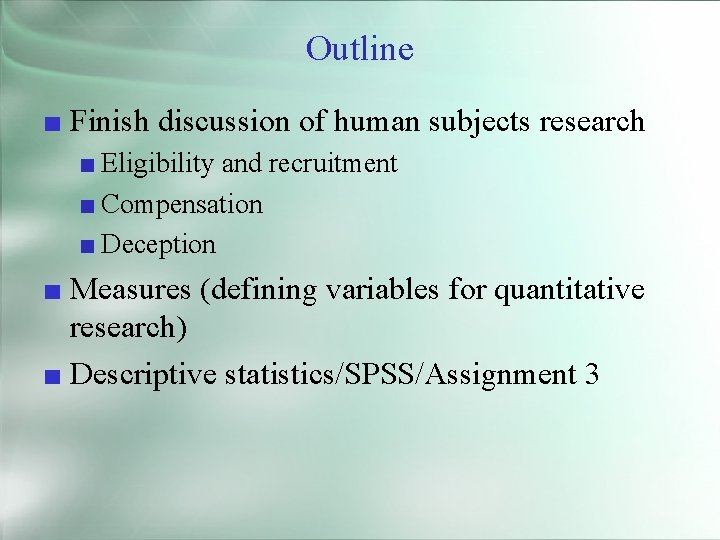
Outline ■ Finish discussion of human subjects research ■ Eligibility and recruitment ■ Compensation ■ Deception ■ Measures (defining variables for quantitative research) ■ Descriptive statistics/SPSS/Assignment 3
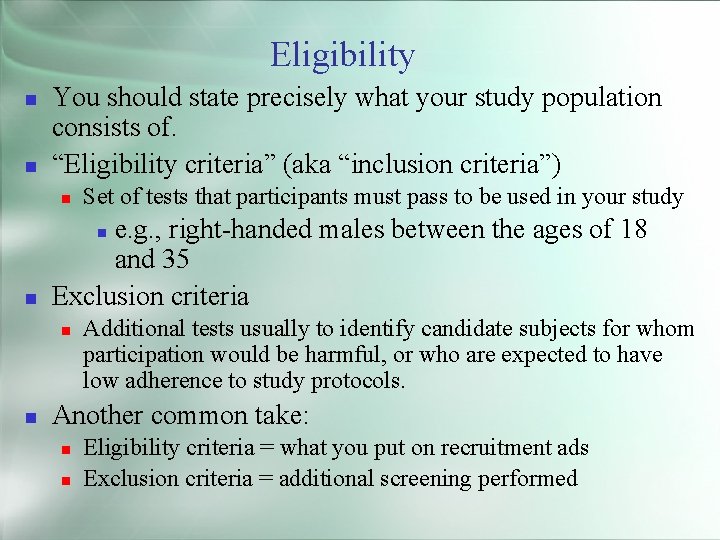
Eligibility You should state precisely what your study population consists of. “Eligibility criteria” (aka “inclusion criteria”) Set of tests that participants must pass to be used in your study e. g. , right-handed males between the ages of 18 and 35 Exclusion criteria Additional tests usually to identify candidate subjects for whom participation would be harmful, or who are expected to have low adherence to study protocols. Another common take: Eligibility criteria = what you put on recruitment ads Exclusion criteria = additional screening performed
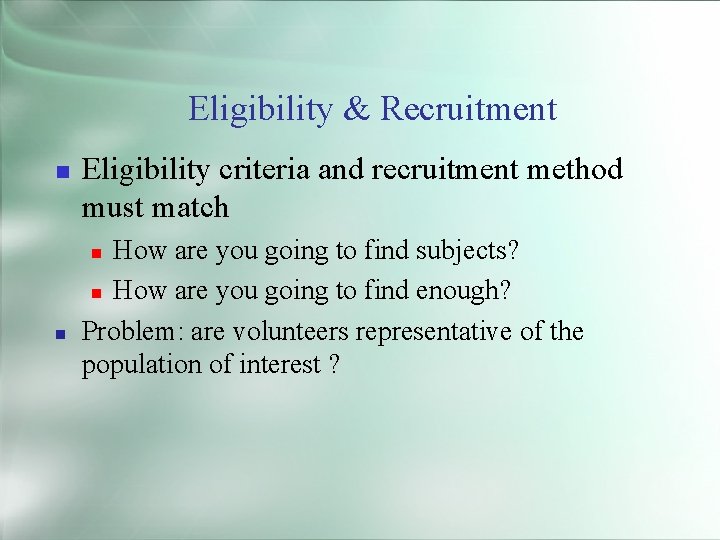
Eligibility & Recruitment Eligibility criteria and recruitment method must match How are you going to find subjects? How are you going to find enough? Problem: are volunteers representative of the population of interest ?
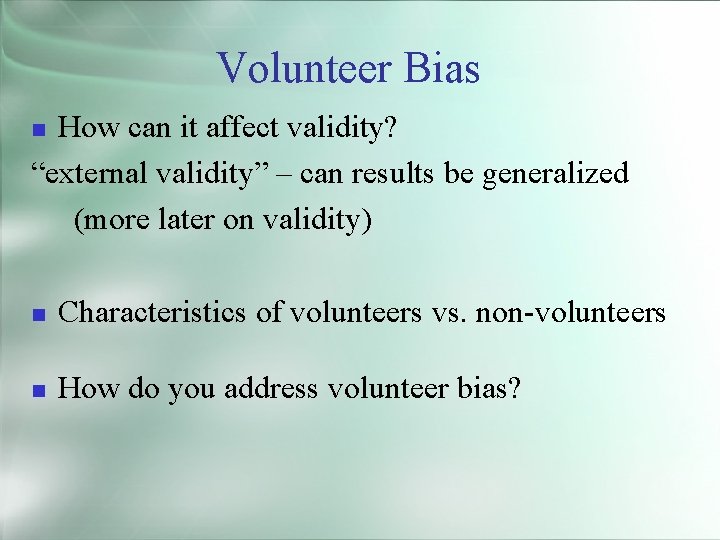
Volunteer Bias How can it affect validity? “external validity” – can results be generalized (more later on validity) Characteristics of volunteers vs. non-volunteers How do you address volunteer bias?
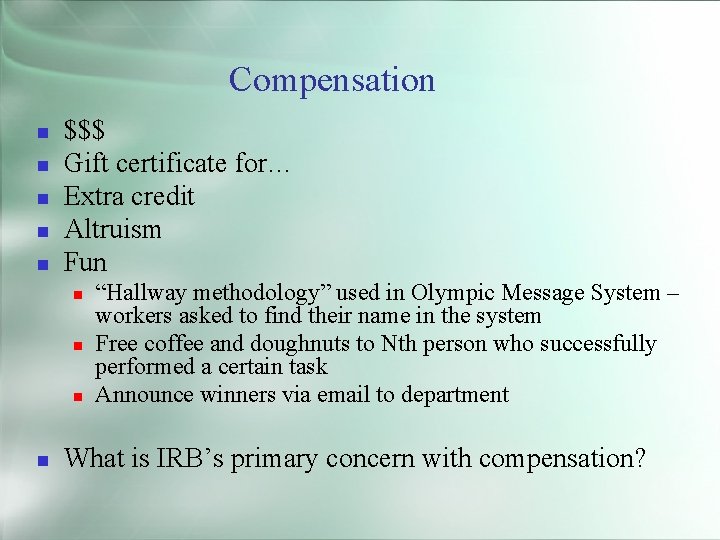
Compensation $$$ Gift certificate for… Extra credit Altruism Fun “Hallway methodology” used in Olympic Message System – workers asked to find their name in the system Free coffee and doughnuts to Nth person who successfully performed a certain task Announce winners via email to department What is IRB’s primary concern with compensation?
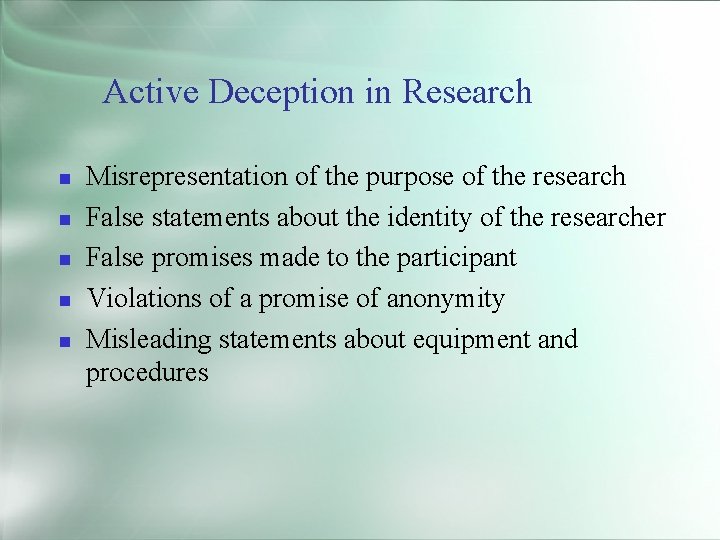
Active Deception in Research Misrepresentation of the purpose of the research False statements about the identity of the researcher False promises made to the participant Violations of a promise of anonymity Misleading statements about equipment and procedures
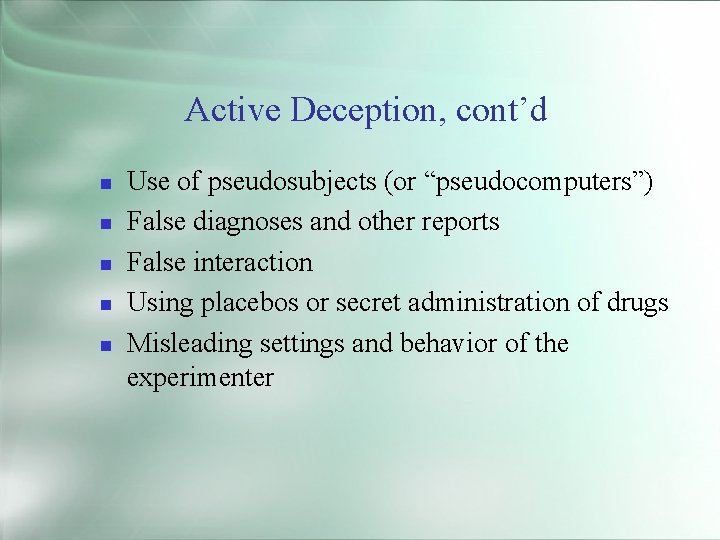
Active Deception, cont’d Use of pseudosubjects (or “pseudocomputers”) False diagnoses and other reports False interaction Using placebos or secret administration of drugs Misleading settings and behavior of the experimenter
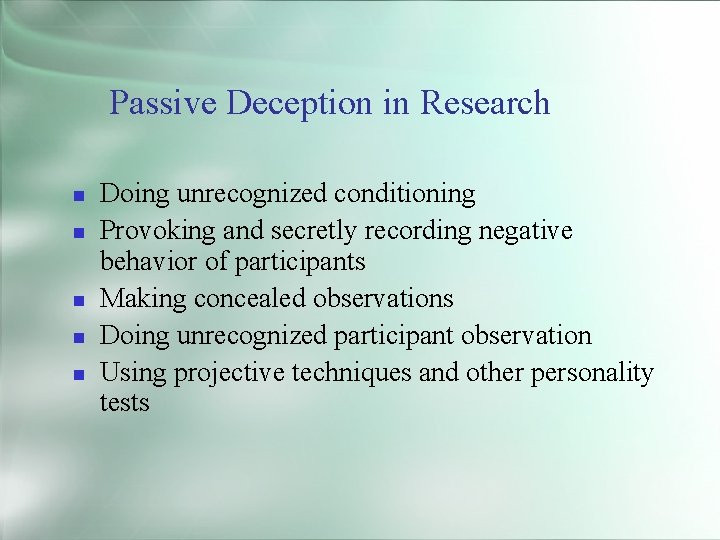
Passive Deception in Research Doing unrecognized conditioning Provoking and secretly recording negative behavior of participants Making concealed observations Doing unrecognized participant observation Using projective techniques and other personality tests
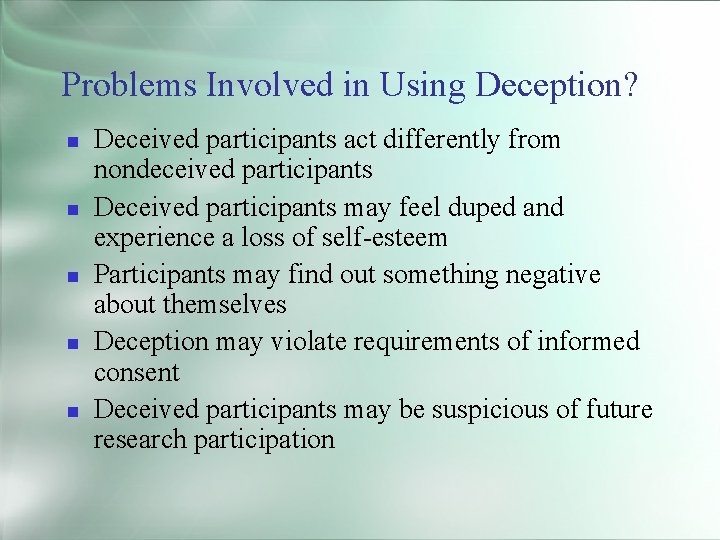
Problems Involved in Using Deception? Deceived participants act differently from nondeceived participants Deceived participants may feel duped and experience a loss of self-esteem Participants may find out something negative about themselves Deception may violate requirements of informed consent Deceived participants may be suspicious of future research participation
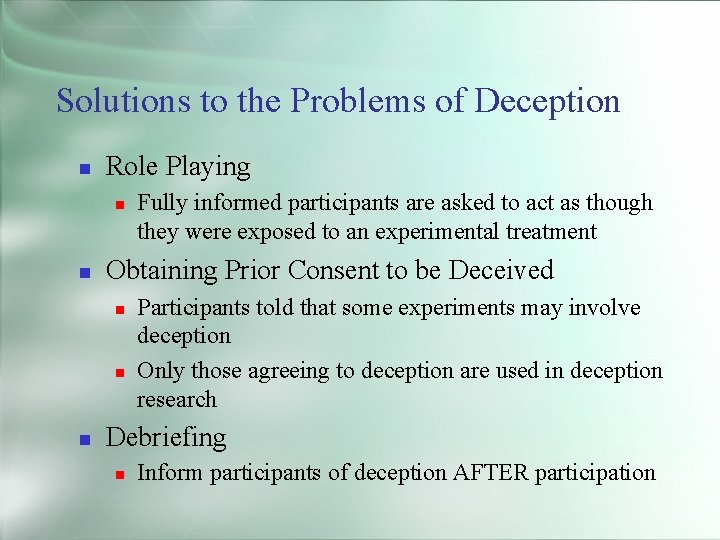
Solutions to the Problems of Deception Role Playing Obtaining Prior Consent to be Deceived Fully informed participants are asked to act as though they were exposed to an experimental treatment Participants told that some experiments may involve deception Only those agreeing to deception are used in deception research Debriefing Inform participants of deception AFTER participation
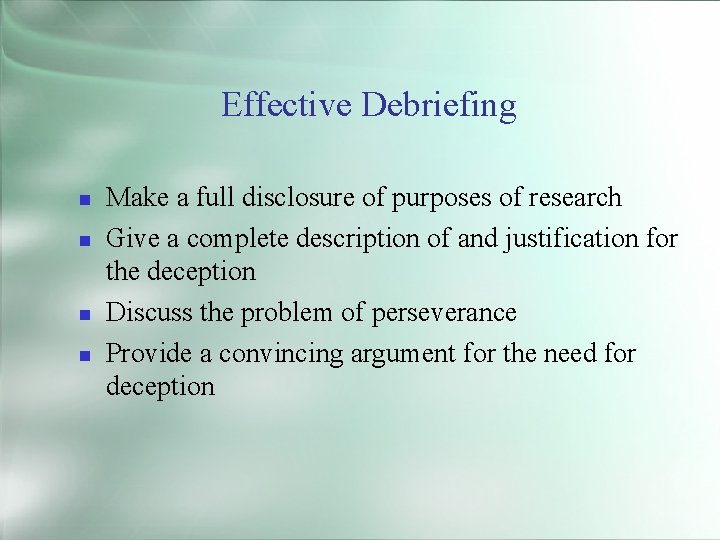
Effective Debriefing Make a full disclosure of purposes of research Give a complete description of and justification for the deception Discuss the problem of perseverance Provide a convincing argument for the need for deception
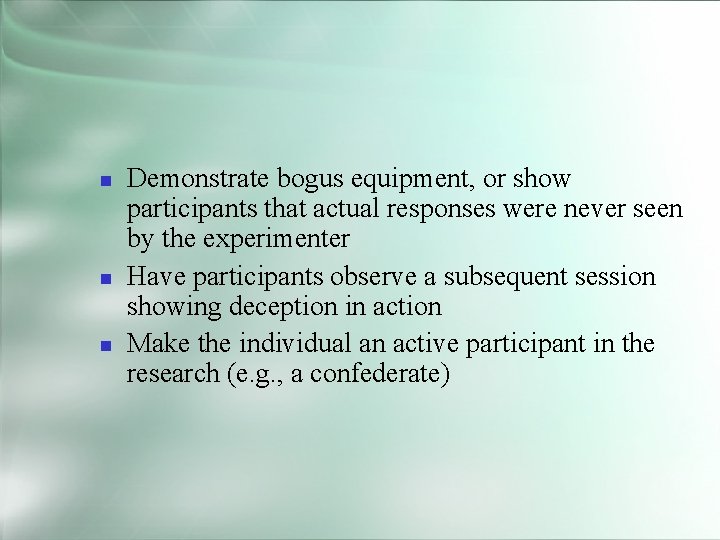
Demonstrate bogus equipment, or show participants that actual responses were never seen by the experimenter Have participants observe a subsequent session showing deception in action Make the individual an active participant in the research (e. g. , a confederate)
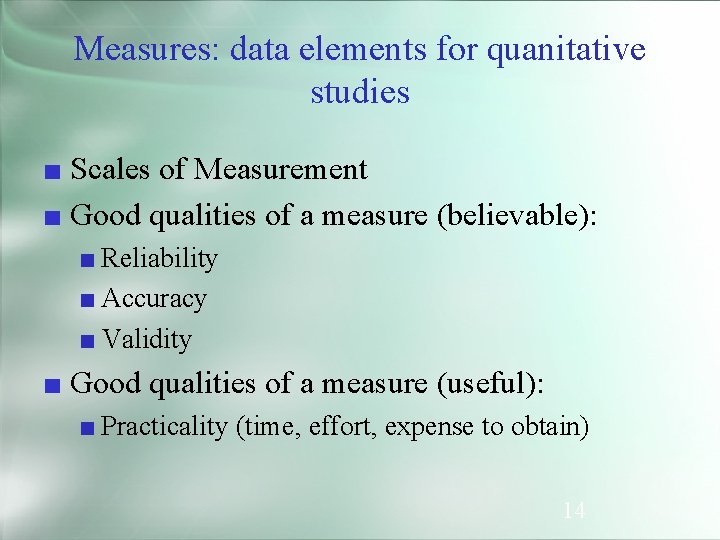
Measures: data elements for quanitative studies ■ Scales of Measurement ■ Good qualities of a measure (believable): ■ Reliability ■ Accuracy ■ Validity ■ Good qualities of a measure (useful): ■ Practicality (time, effort, expense to obtain) 14
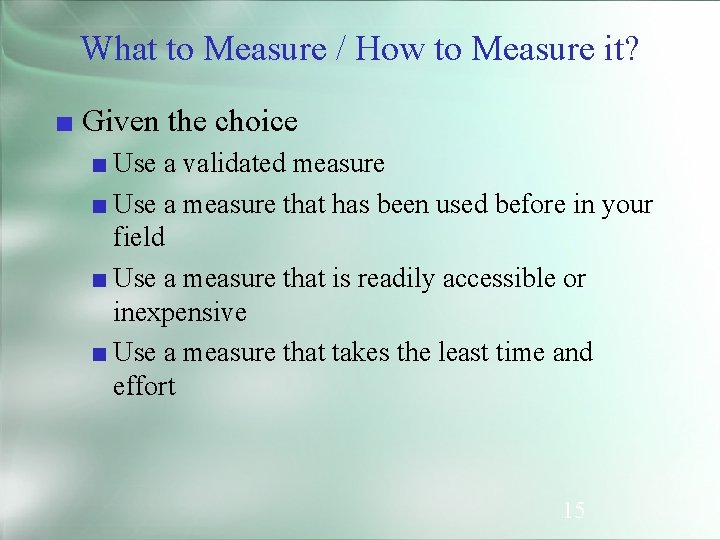
What to Measure / How to Measure it? ■ Given the choice ■ Use a validated measure ■ Use a measure that has been used before in your field ■ Use a measure that is readily accessible or inexpensive ■ Use a measure that takes the least time and effort 15
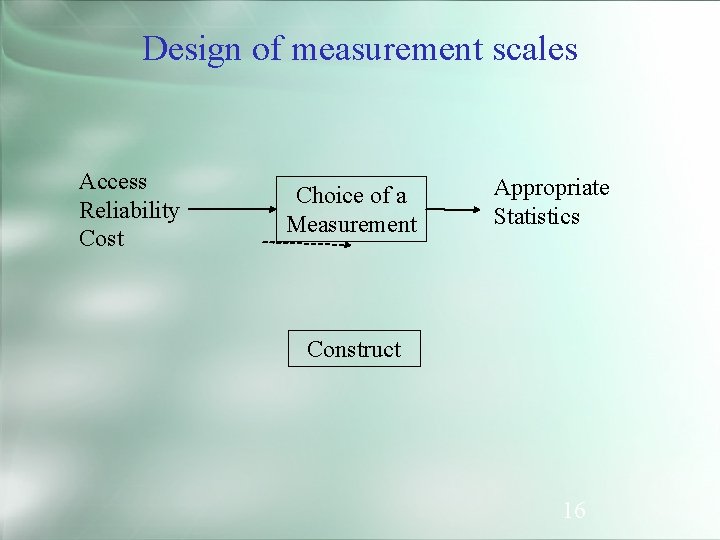
Design of measurement scales Access Reliability Cost Choice of a Measurement Appropriate Statistics Construct 16
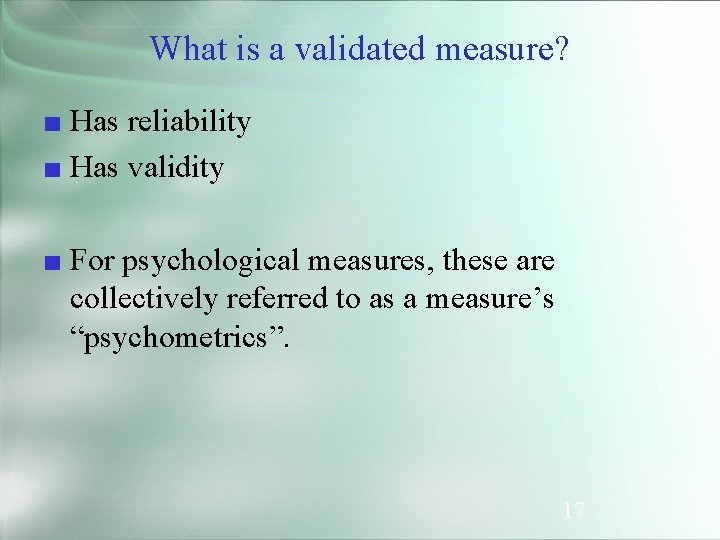
What is a validated measure? ■ Has reliability ■ Has validity ■ For psychological measures, these are collectively referred to as a measure’s “psychometrics”. 17
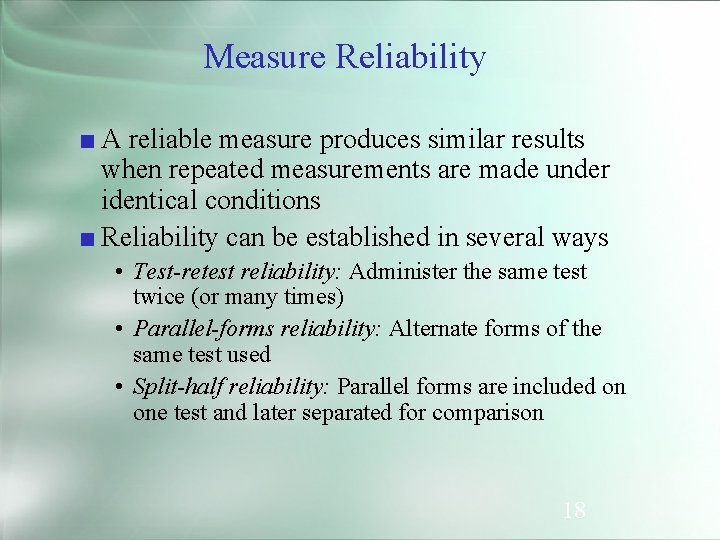
Measure Reliability ■ A reliable measure produces similar results when repeated measurements are made under identical conditions ■ Reliability can be established in several ways • Test-retest reliability: Administer the same test twice (or many times) • Parallel-forms reliability: Alternate forms of the same test used • Split-half reliability: Parallel forms are included on one test and later separated for comparison 18
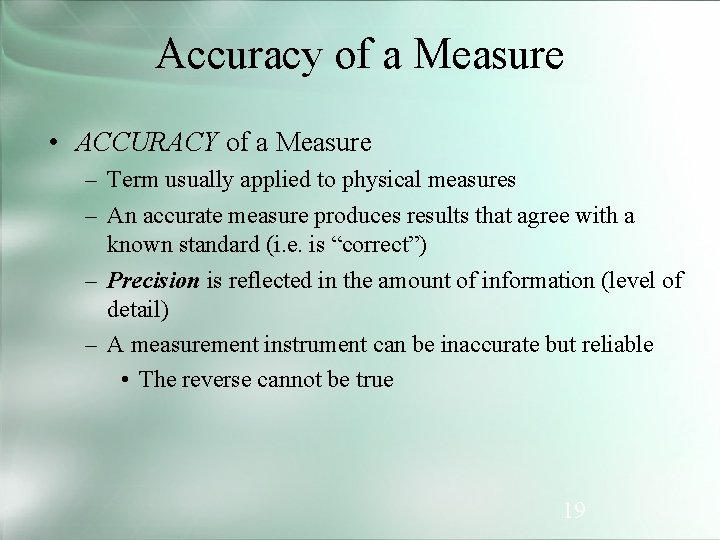
Accuracy of a Measure • ACCURACY of a Measure – Term usually applied to physical measures – An accurate measure produces results that agree with a known standard (i. e. is “correct”) – Precision is reflected in the amount of information (level of detail) – A measurement instrument can be inaccurate but reliable • The reverse cannot be true 19
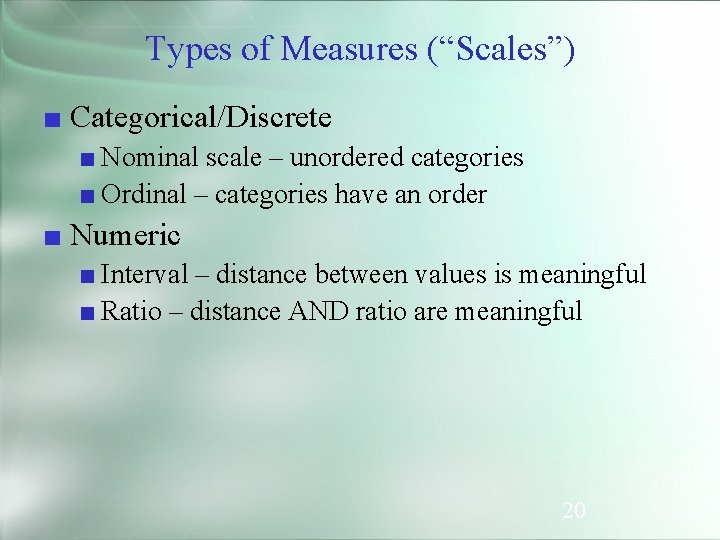
Types of Measures (“Scales”) ■ Categorical/Discrete ■ Nominal scale – unordered categories ■ Ordinal – categories have an order ■ Numeric ■ Interval – distance between values is meaningful ■ Ratio – distance AND ratio are meaningful 20
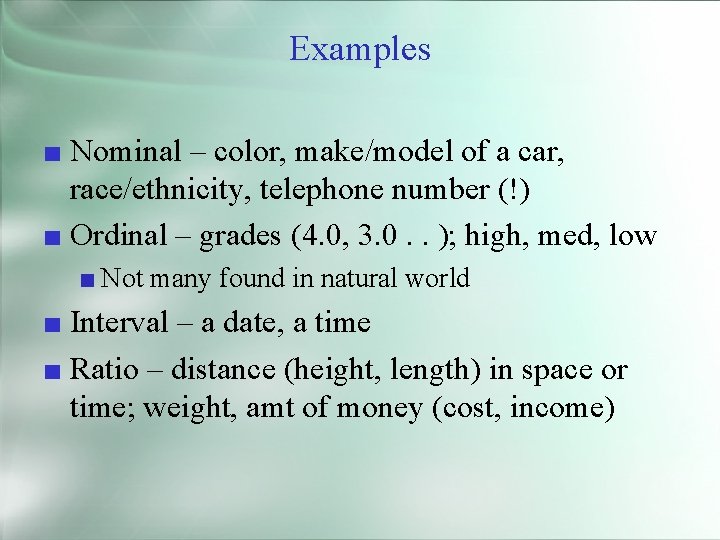
Examples ■ Nominal – color, make/model of a car, race/ethnicity, telephone number (!) ■ Ordinal – grades (4. 0, 3. 0. . ); high, med, low ■ Not many found in natural world ■ Interval – a date, a time ■ Ratio – distance (height, length) in space or time; weight, amt of money (cost, income)
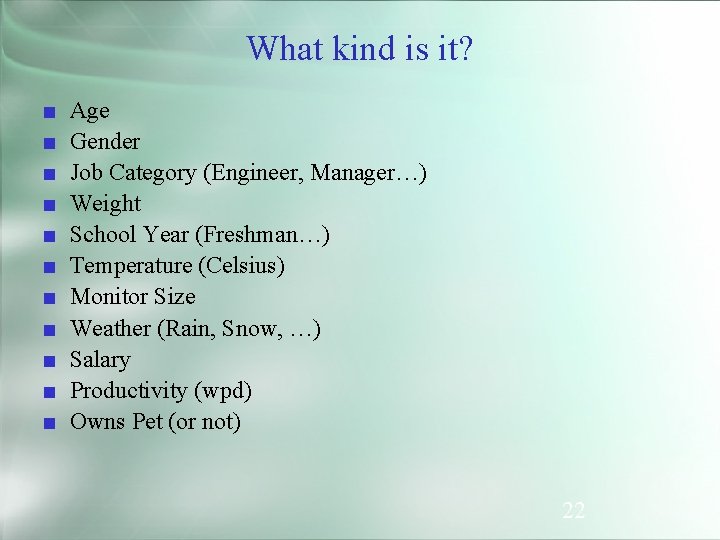
What kind is it? ■ ■ ■ Age Gender Job Category (Engineer, Manager…) Weight School Year (Freshman…) Temperature (Celsius) Monitor Size Weather (Rain, Snow, …) Salary Productivity (wpd) Owns Pet (or not) 22
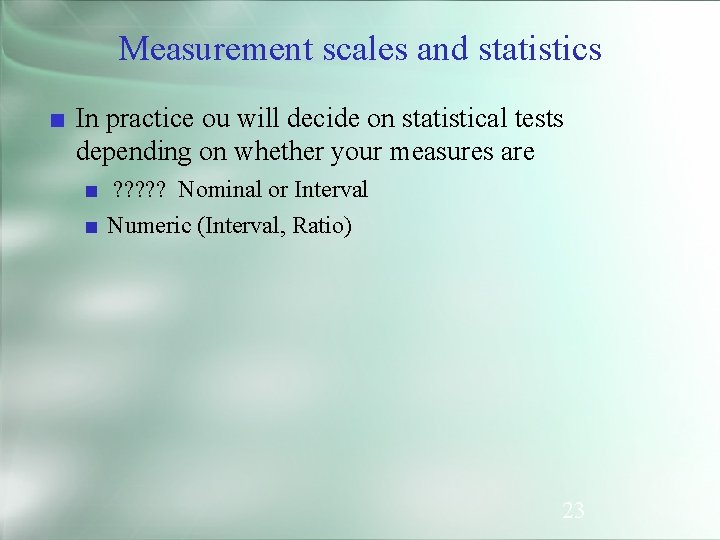
Measurement scales and statistics ■ In practice ou will decide on statistical tests depending on whether your measures are ■ ? ? ? Nominal or Interval ■ Numeric (Interval, Ratio) 23
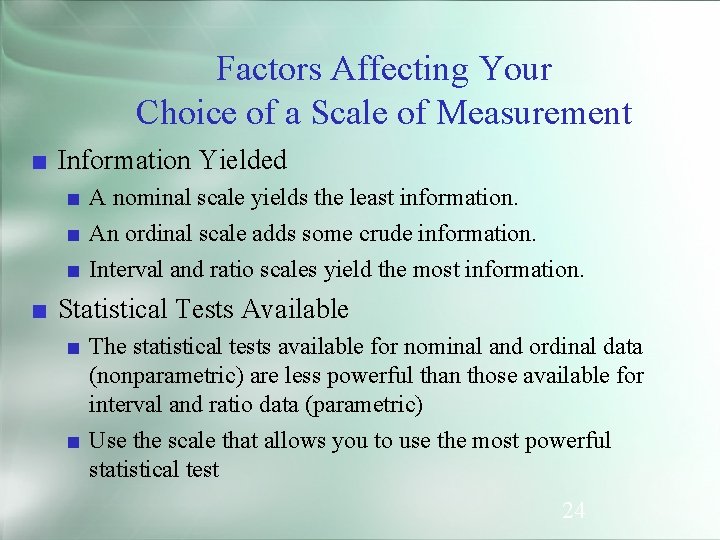
Factors Affecting Your Choice of a Scale of Measurement ■ Information Yielded ■ A nominal scale yields the least information. ■ An ordinal scale adds some crude information. ■ Interval and ratio scales yield the most information. ■ Statistical Tests Available ■ The statistical tests available for nominal and ordinal data (nonparametric) are less powerful than those available for interval and ratio data (parametric) ■ Use the scale that allows you to use the most powerful statistical test 24
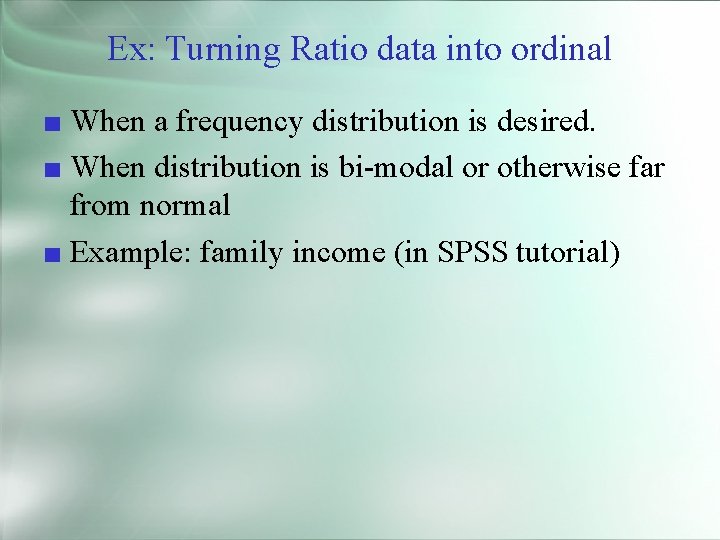
Ex: Turning Ratio data into ordinal ■ When a frequency distribution is desired. ■ When distribution is bi-modal or otherwise far from normal ■ Example: family income (in SPSS tutorial)
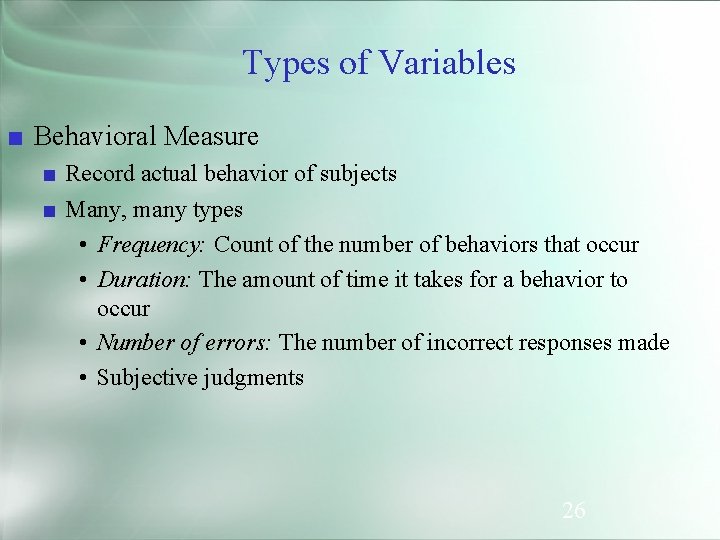
Types of Variables ■ Behavioral Measure ■ Record actual behavior of subjects ■ Many, many types • Frequency: Count of the number of behaviors that occur • Duration: The amount of time it takes for a behavior to occur • Number of errors: The number of incorrect responses made • Subjective judgments 26
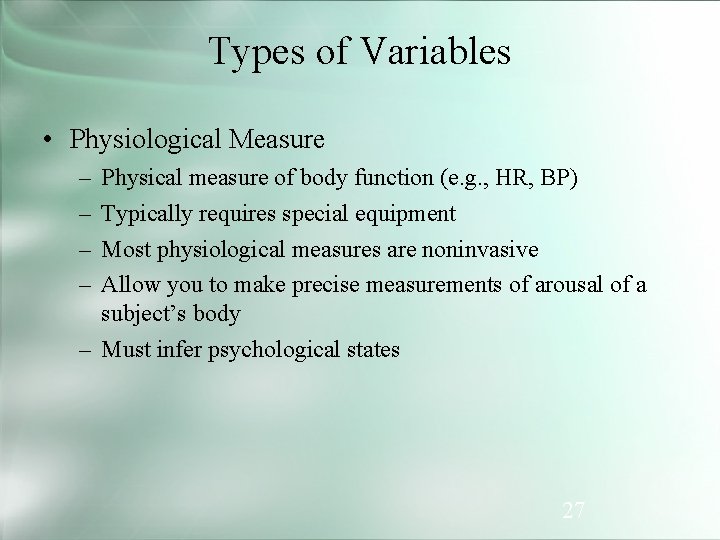
Types of Variables • Physiological Measure – – Physical measure of body function (e. g. , HR, BP) Typically requires special equipment Most physiological measures are noninvasive Allow you to make precise measurements of arousal of a subject’s body – Must infer psychological states 27
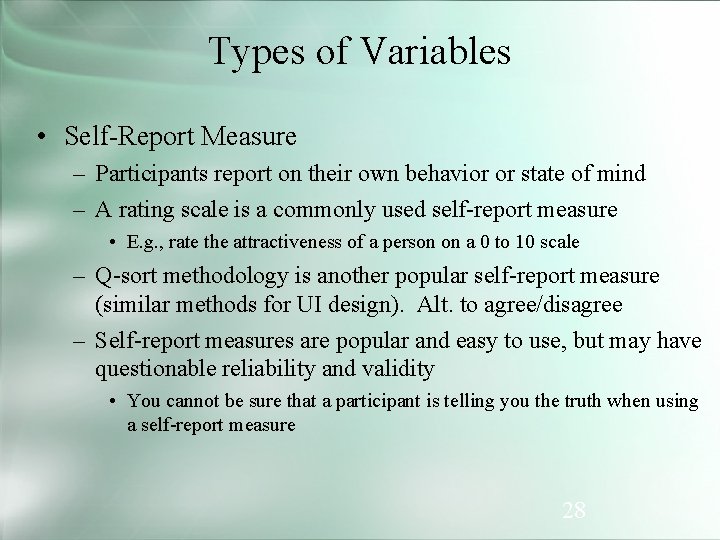
Types of Variables • Self-Report Measure – Participants report on their own behavior or state of mind – A rating scale is a commonly used self-report measure • E. g. , rate the attractiveness of a person on a 0 to 10 scale – Q-sort methodology is another popular self-report measure (similar methods for UI design). Alt. to agree/disagree – Self-report measures are popular and easy to use, but may have questionable reliability and validity • You cannot be sure that a participant is telling you the truth when using a self-report measure 28
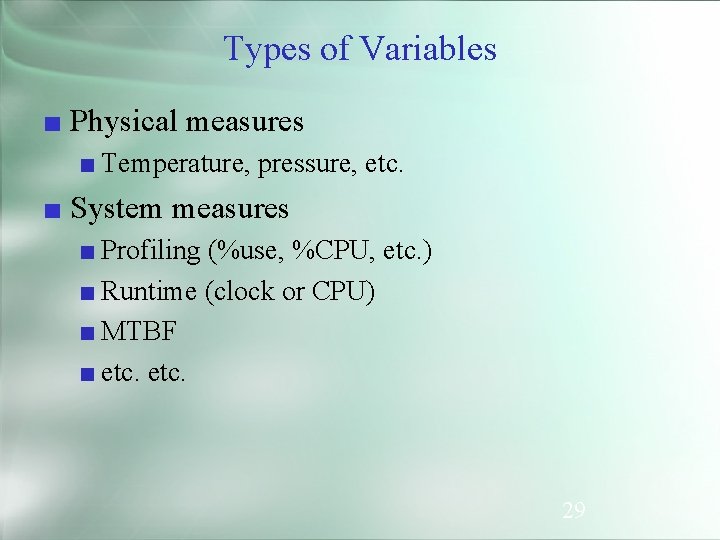
Types of Variables ■ Physical measures ■ Temperature, pressure, etc. ■ System measures ■ Profiling (%use, %CPU, etc. ) ■ Runtime (clock or CPU) ■ MTBF ■ etc. 29
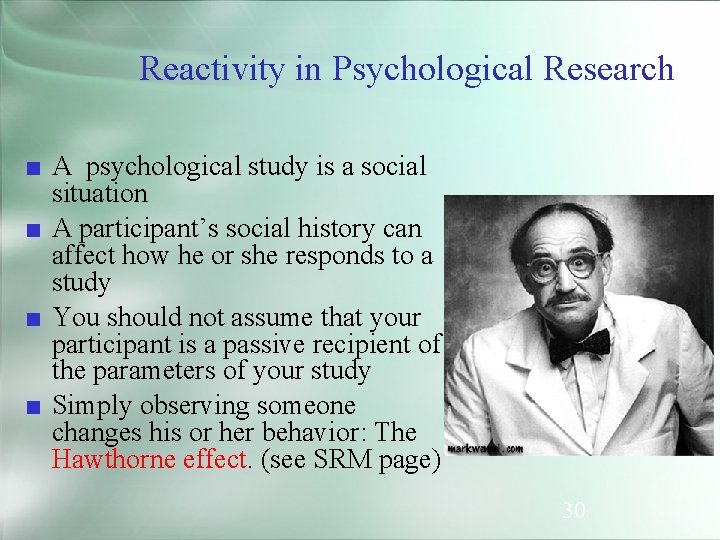
Reactivity in Psychological Research ■ A psychological study is a social situation ■ A participant’s social history can affect how he or she responds to a study ■ You should not assume that your participant is a passive recipient of the parameters of your study ■ Simply observing someone changes his or her behavior: The Hawthorne effect. (see SRM page) 30
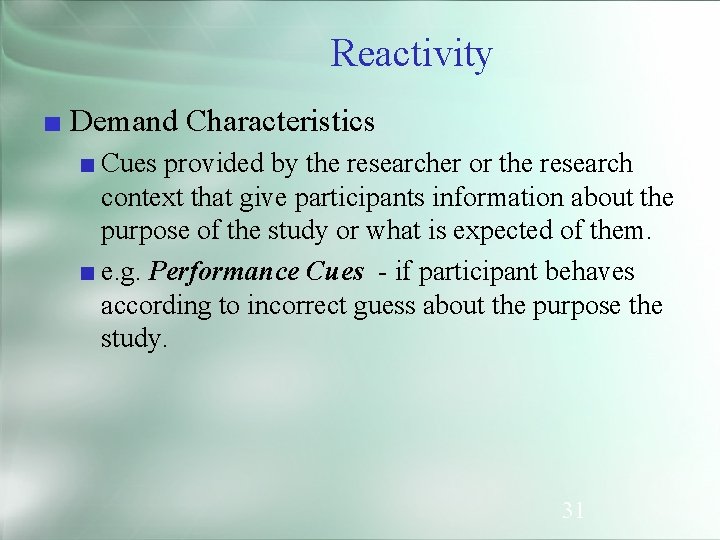
Reactivity ■ Demand Characteristics ■ Cues provided by the researcher or the research context that give participants information about the purpose of the study or what is expected of them. ■ e. g. Performance Cues - if participant behaves according to incorrect guess about the purpose the study. 31
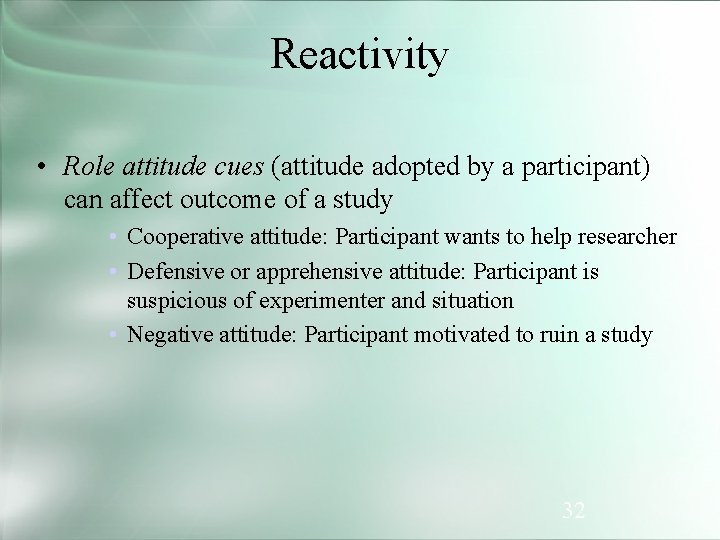
Reactivity • Role attitude cues (attitude adopted by a participant) can affect outcome of a study • Cooperative attitude: Participant wants to help researcher • Defensive or apprehensive attitude: Participant is suspicious of experimenter and situation • Negative attitude: Participant motivated to ruin a study 32
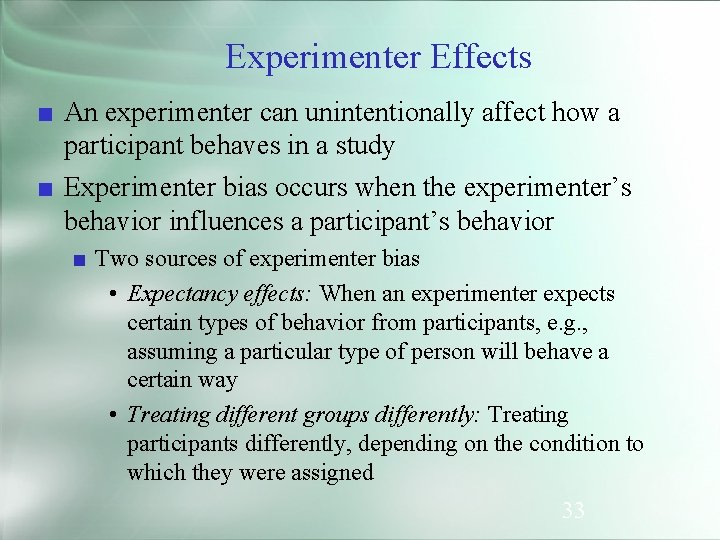
Experimenter Effects ■ An experimenter can unintentionally affect how a participant behaves in a study ■ Experimenter bias occurs when the experimenter’s behavior influences a participant’s behavior ■ Two sources of experimenter bias • Expectancy effects: When an experimenter expects certain types of behavior from participants, e. g. , assuming a particular type of person will behave a certain way • Treating different groups differently: Treating participants differently, depending on the condition to which they were assigned 33
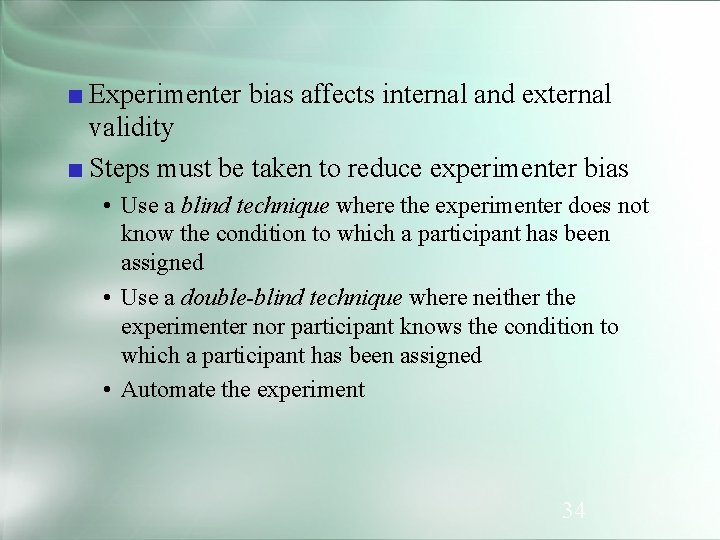
■ Experimenter bias affects internal and external validity ■ Steps must be taken to reduce experimenter bias • Use a blind technique where the experimenter does not know the condition to which a participant has been assigned • Use a double-blind technique where neither the experimenter nor participant knows the condition to which a participant has been assigned • Automate the experiment 34
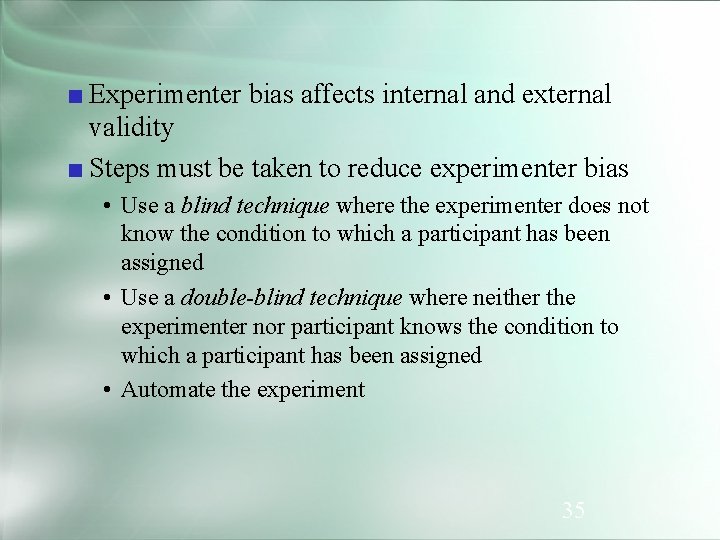
■ Experimenter bias affects internal and external validity ■ Steps must be taken to reduce experimenter bias • Use a blind technique where the experimenter does not know the condition to which a participant has been assigned • Use a double-blind technique where neither the experimenter nor participant knows the condition to which a participant has been assigned • Automate the experiment 35
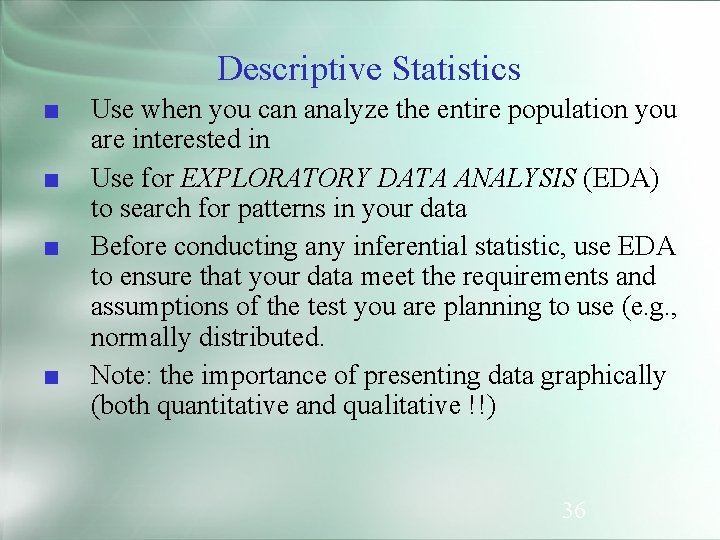
Descriptive Statistics ■ ■ Use when you can analyze the entire population you are interested in Use for EXPLORATORY DATA ANALYSIS (EDA) to search for patterns in your data Before conducting any inferential statistic, use EDA to ensure that your data meet the requirements and assumptions of the test you are planning to use (e. g. , normally distributed. Note: the importance of presenting data graphically (both quantitative and qualitative !!) 36
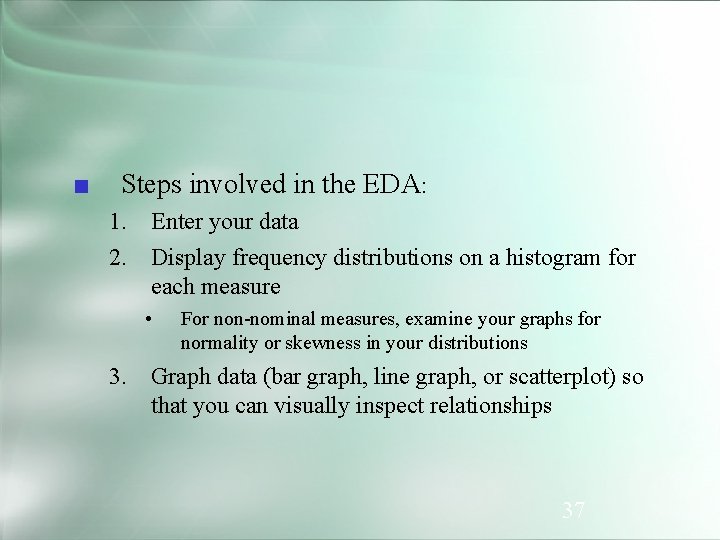
■ Steps involved in the EDA: 1. Enter your data 2. Display frequency distributions on a histogram for each measure • For non-nominal measures, examine your graphs for normality or skewness in your distributions 3. Graph data (bar graph, line graph, or scatterplot) so that you can visually inspect relationships 37
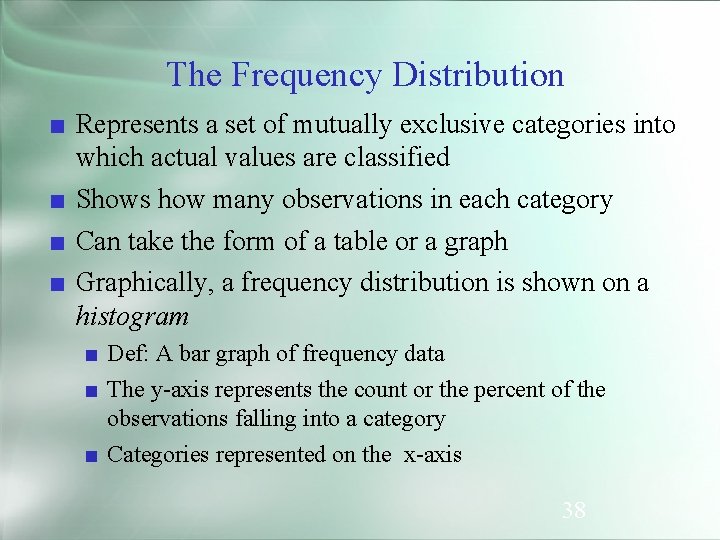
The Frequency Distribution ■ Represents a set of mutually exclusive categories into which actual values are classified ■ Shows how many observations in each category ■ Can take the form of a table or a graph ■ Graphically, a frequency distribution is shown on a histogram ■ Def: A bar graph of frequency data ■ The y-axis represents the count or the percent of the observations falling into a category ■ Categories represented on the x-axis 38
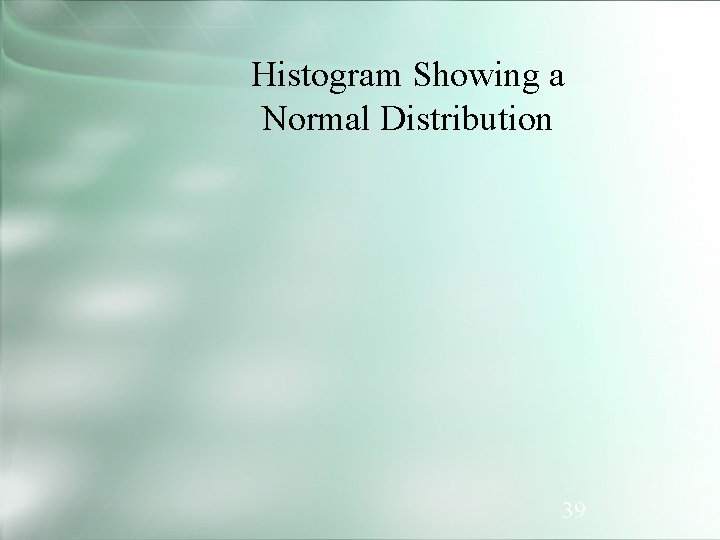
Histogram Showing a Normal Distribution 39
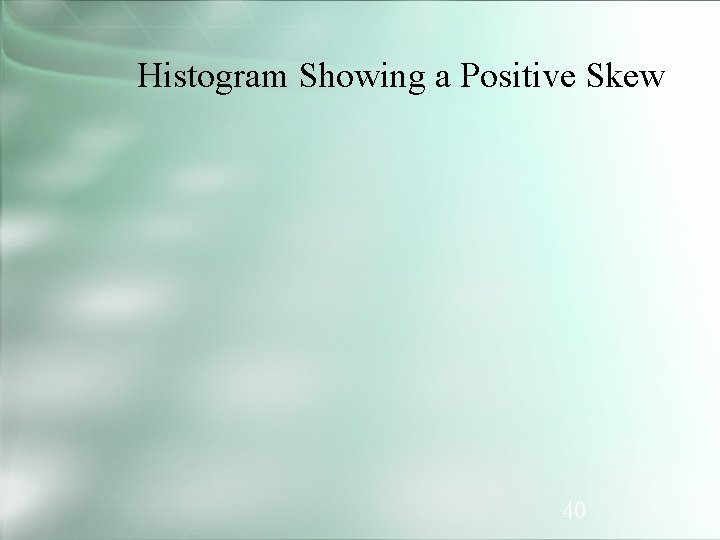
Histogram Showing a Positive Skew 40
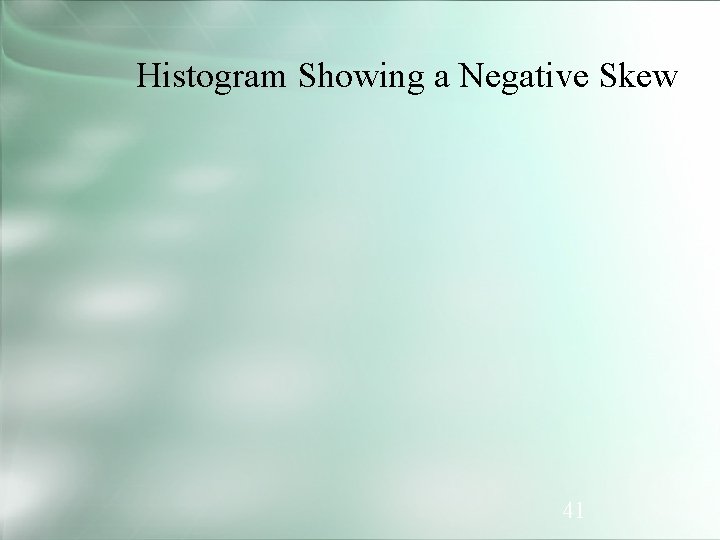
Histogram Showing a Negative Skew 41
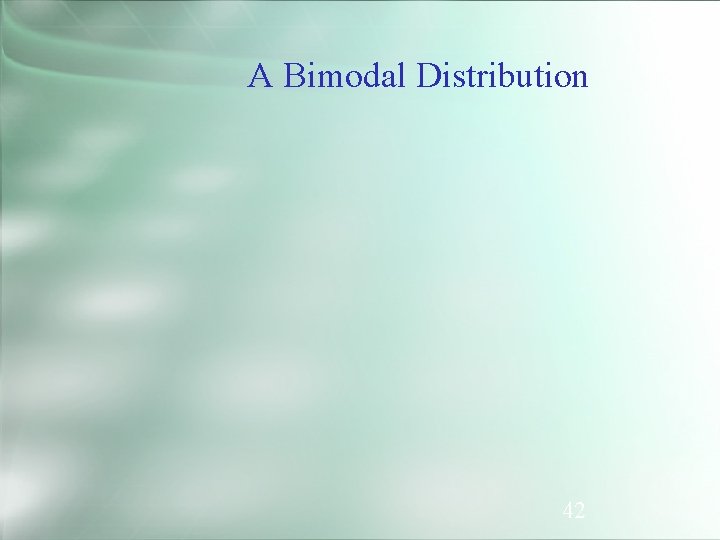
A Bimodal Distribution 42
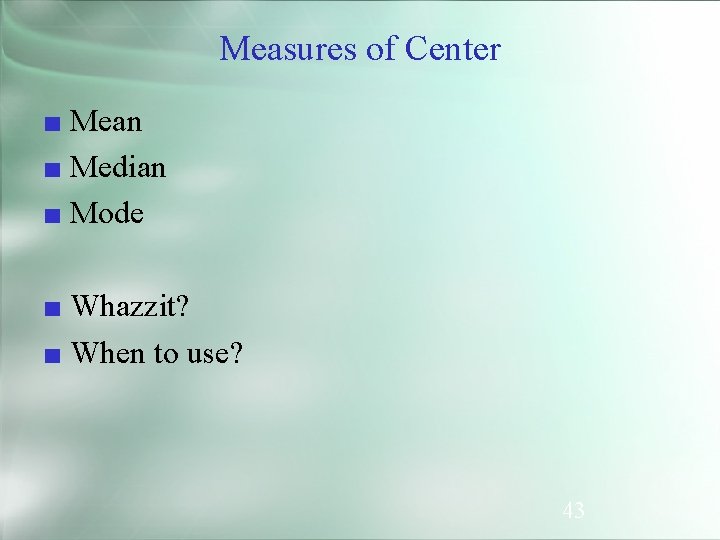
Measures of Center ■ Mean ■ Median ■ Mode ■ Whazzit? ■ When to use? 43
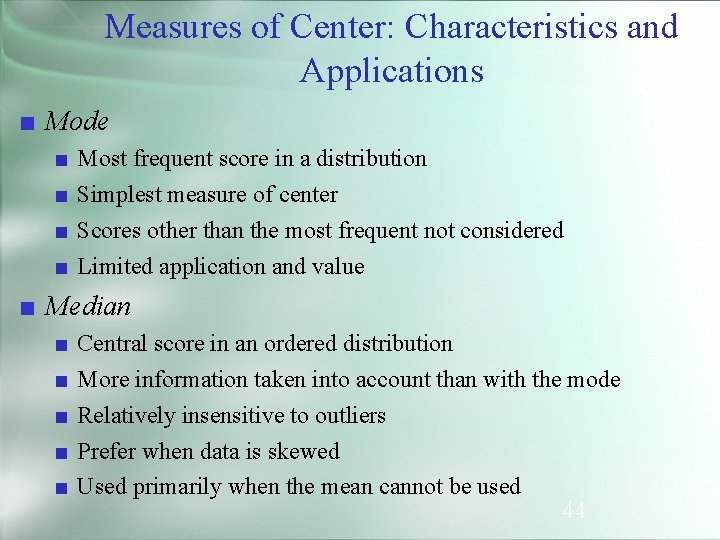
Measures of Center: Characteristics and Applications ■ Mode ■ ■ Most frequent score in a distribution Simplest measure of center Scores other than the most frequent not considered Limited application and value ■ Median ■ ■ ■ Central score in an ordered distribution More information taken into account than with the mode Relatively insensitive to outliers Prefer when data is skewed Used primarily when the mean cannot be used 44
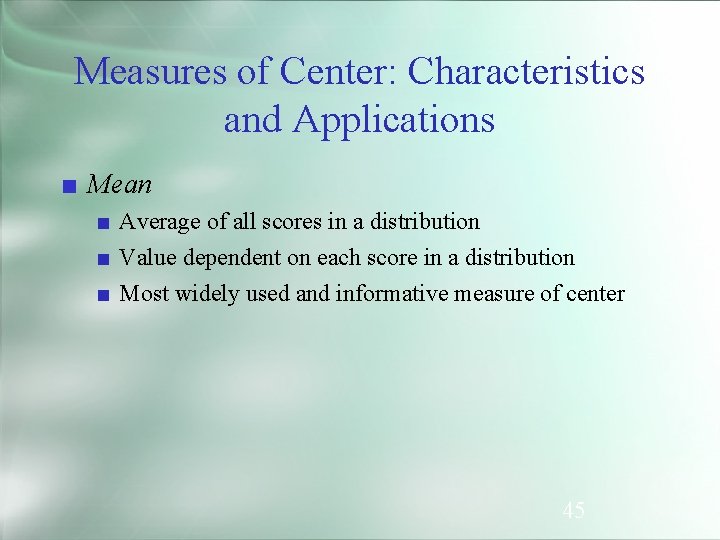
Measures of Center: Characteristics and Applications ■ Mean ■ Average of all scores in a distribution ■ Value dependent on each score in a distribution ■ Most widely used and informative measure of center 45
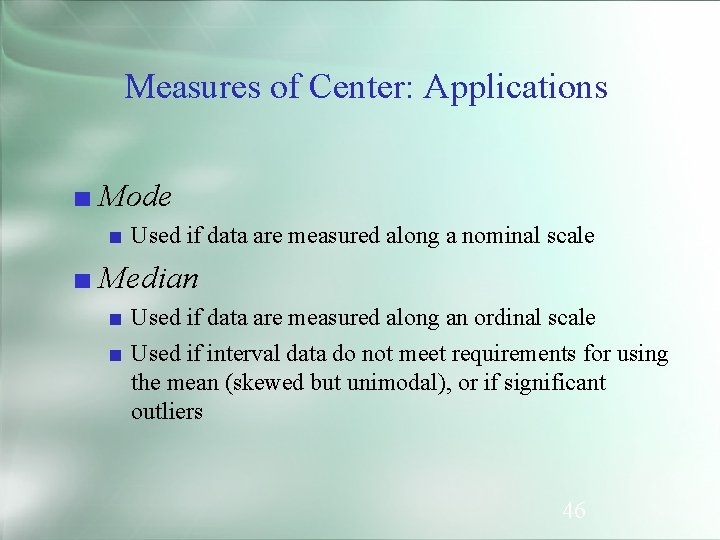
Measures of Center: Applications ■ Mode ■ Used if data are measured along a nominal scale ■ Median ■ Used if data are measured along an ordinal scale ■ Used if interval data do not meet requirements for using the mean (skewed but unimodal), or if significant outliers 46
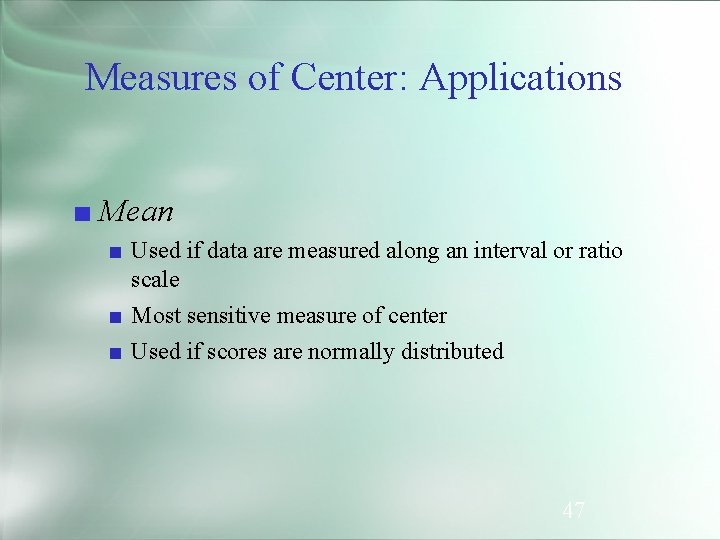
Measures of Center: Applications ■ Mean ■ Used if data are measured along an interval or ratio scale ■ Most sensitive measure of center ■ Used if scores are normally distributed 47
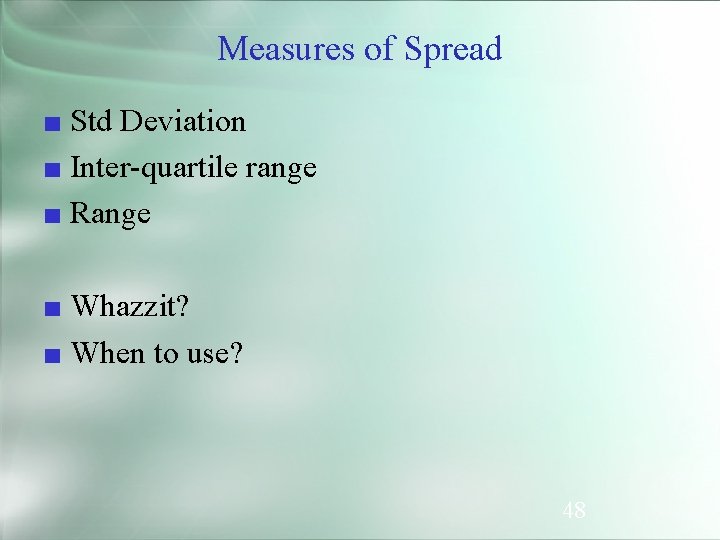
Measures of Spread ■ Std Deviation ■ Inter-quartile range ■ Range ■ Whazzit? ■ When to use? 48
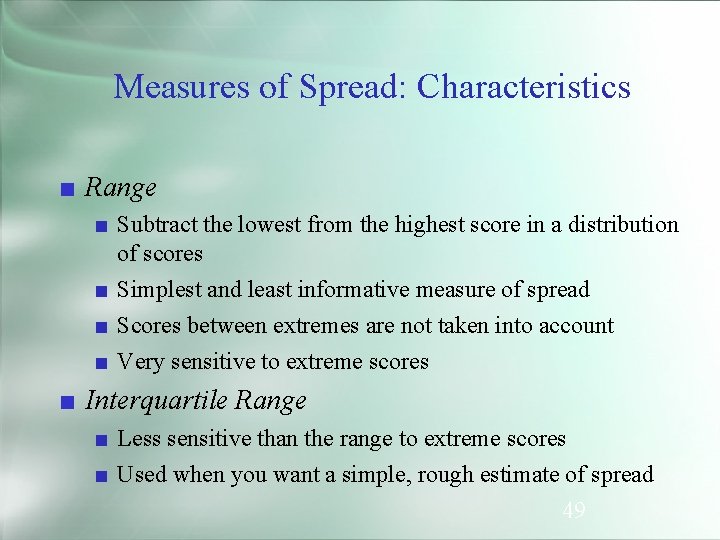
Measures of Spread: Characteristics ■ Range ■ Subtract the lowest from the highest score in a distribution of scores ■ Simplest and least informative measure of spread ■ Scores between extremes are not taken into account ■ Very sensitive to extreme scores ■ Interquartile Range ■ Less sensitive than the range to extreme scores ■ Used when you want a simple, rough estimate of spread 49
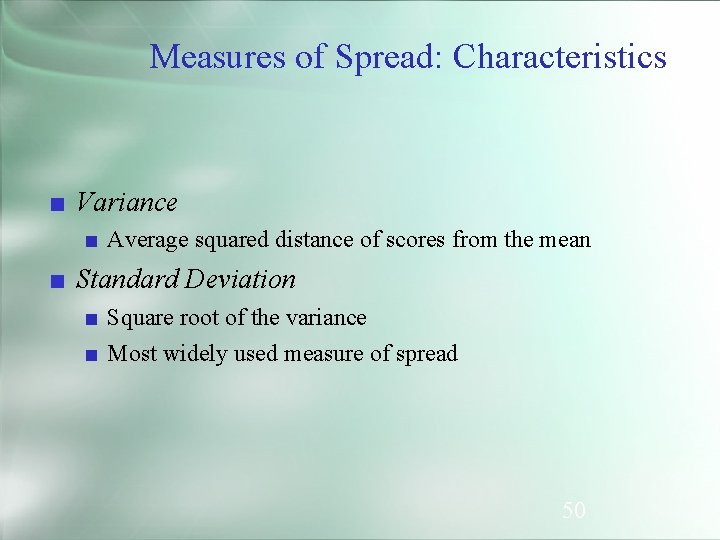
Measures of Spread: Characteristics ■ Variance ■ Average squared distance of scores from the mean ■ Standard Deviation ■ Square root of the variance ■ Most widely used measure of spread 50
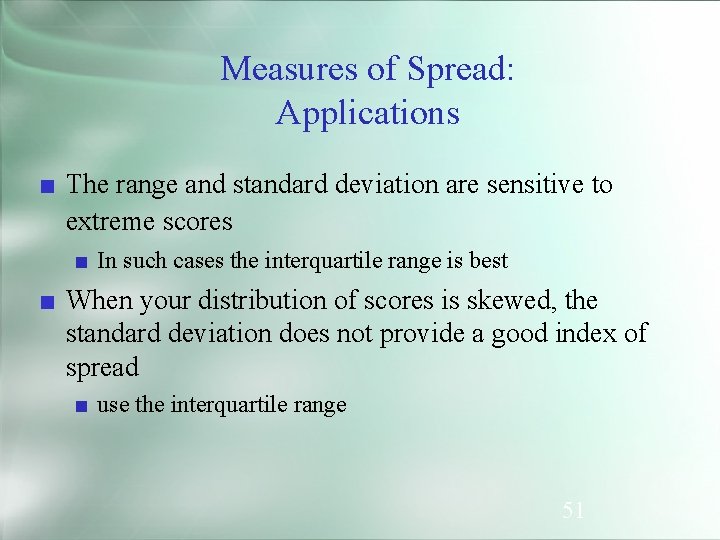
Measures of Spread: Applications ■ The range and standard deviation are sensitive to extreme scores ■ In such cases the interquartile range is best ■ When your distribution of scores is skewed, the standard deviation does not provide a good index of spread ■ use the interquartile range 51
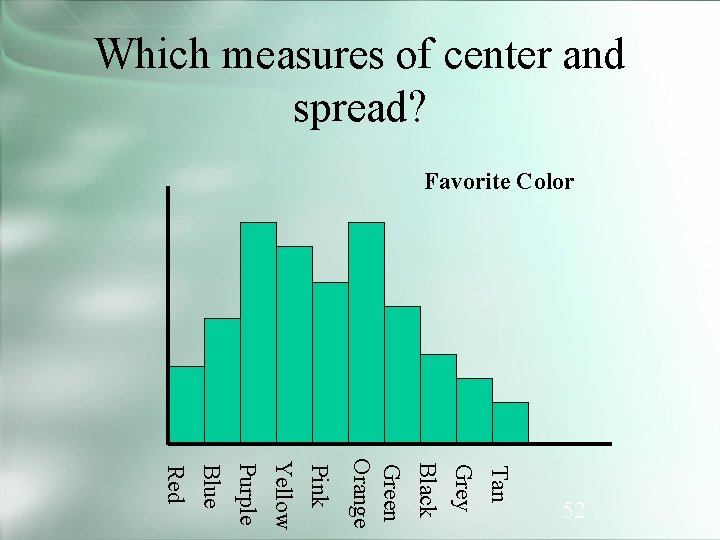
Which measures of center and spread? Favorite Color Tan Grey Black Green Orange Pink Yellow Purple Blue Red 52
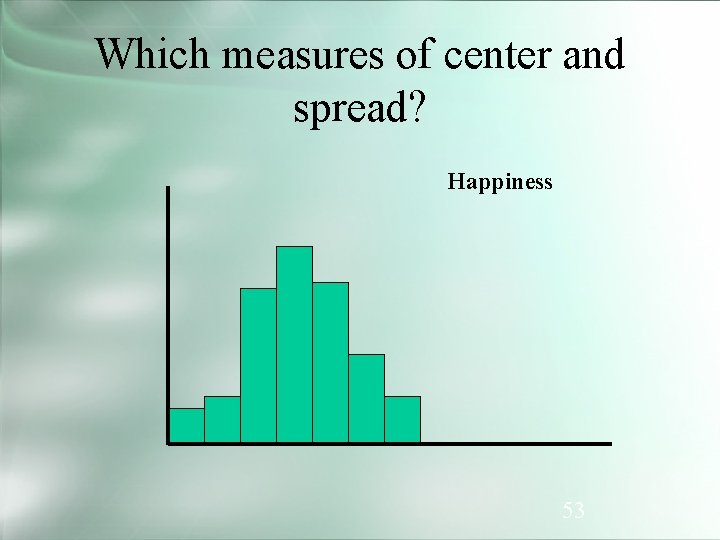
Which measures of center and spread? Happiness 53
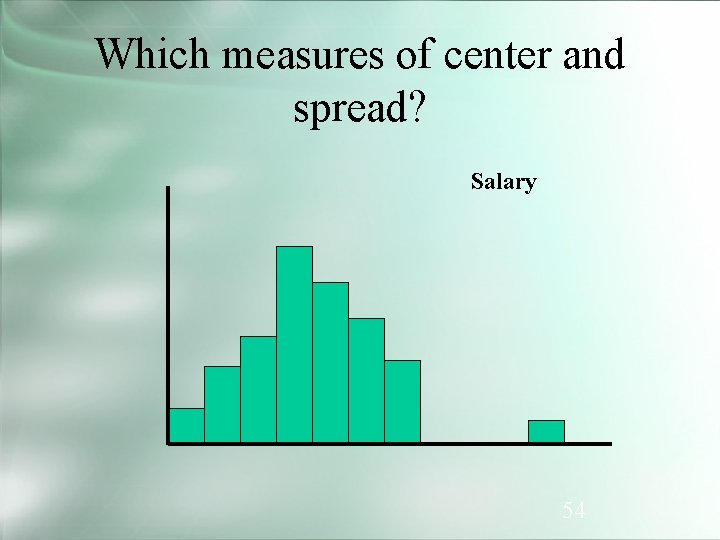
Which measures of center and spread? Salary 54
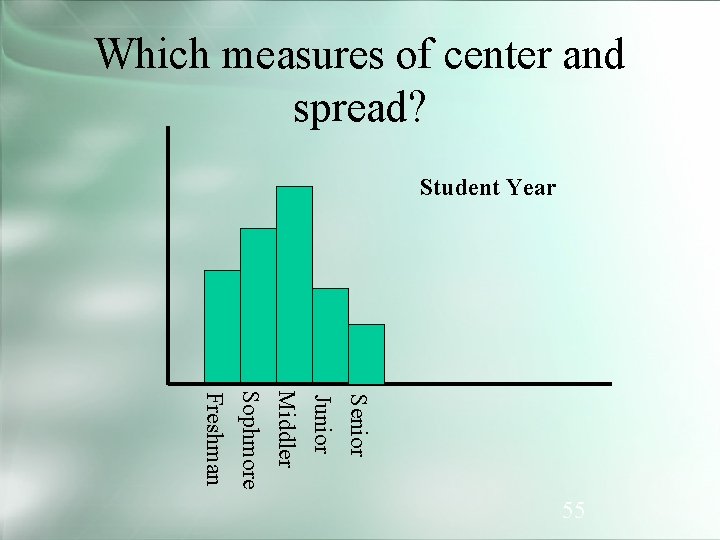
Which measures of center and spread? Student Year Senior Junior Middler Sophmore Freshman 55
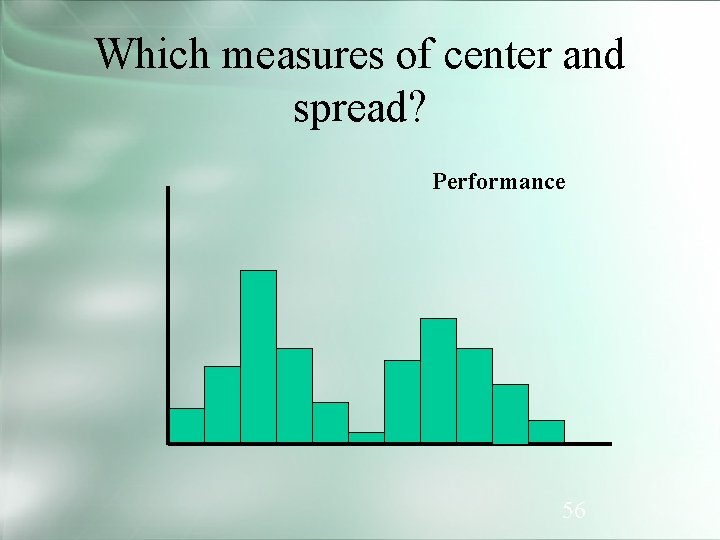
Which measures of center and spread? Performance 56
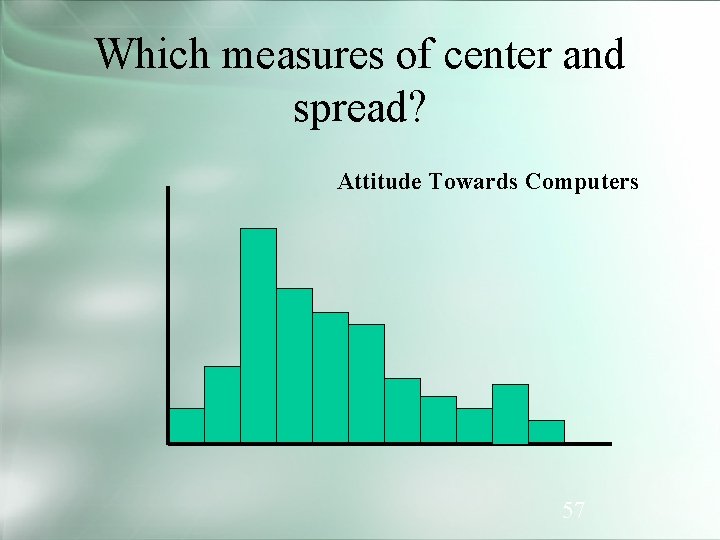
Which measures of center and spread? Attitude Towards Computers 57
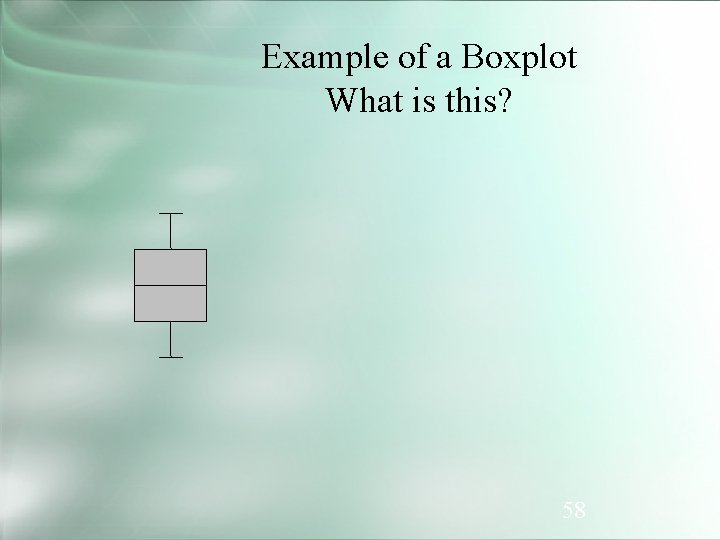
Example of a Boxplot What is this? 58
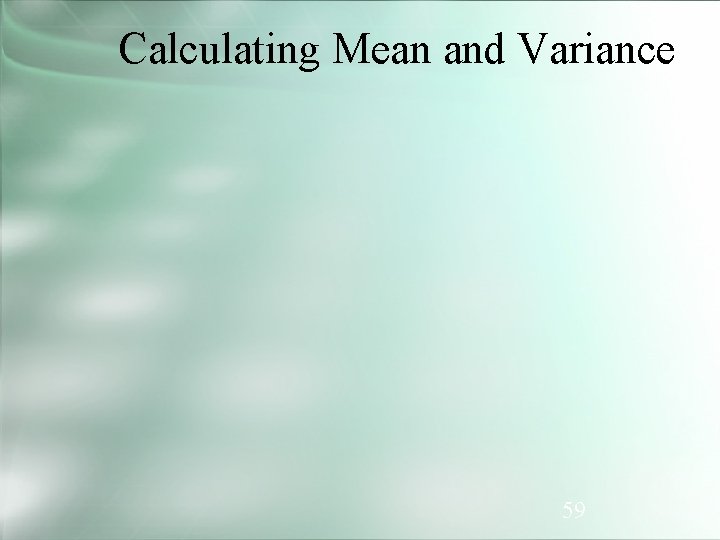
Calculating Mean and Variance 59
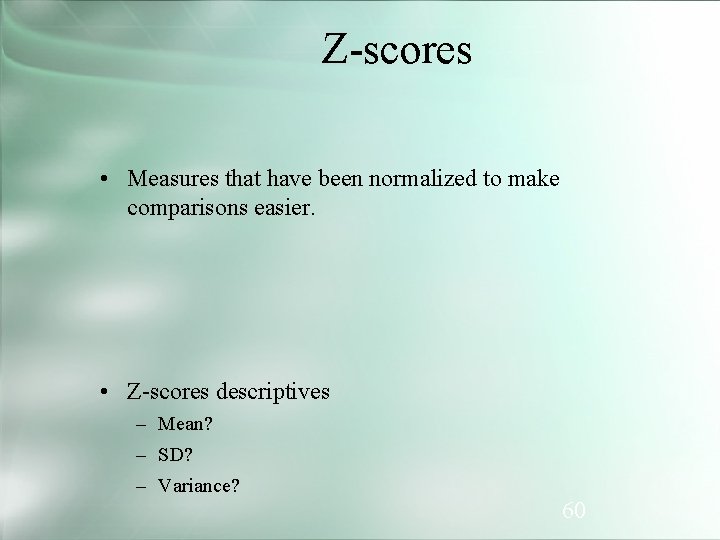
Z-scores • Measures that have been normalized to make comparisons easier. • Z-scores descriptives – Mean? – SD? – Variance? 60
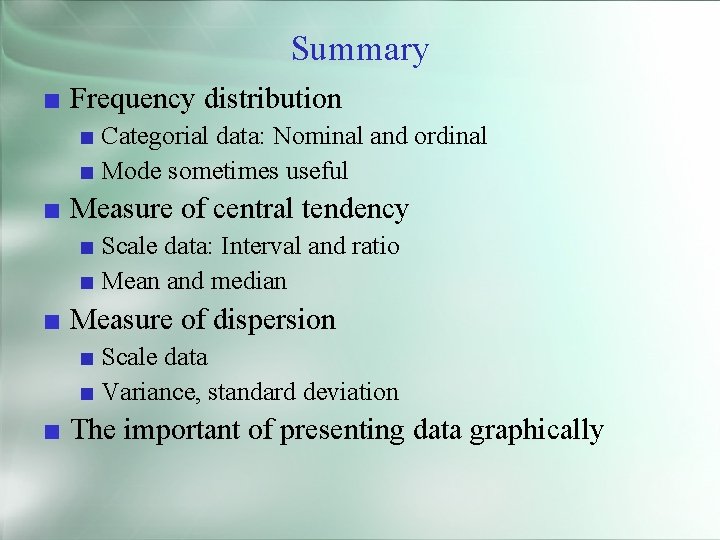
Summary ■ Frequency distribution ■ Categorial data: Nominal and ordinal ■ Mode sometimes useful ■ Measure of central tendency ■ Scale data: Interval and ratio ■ Mean and median ■ Measure of dispersion ■ Scale data ■ Variance, standard deviation ■ The important of presenting data graphically
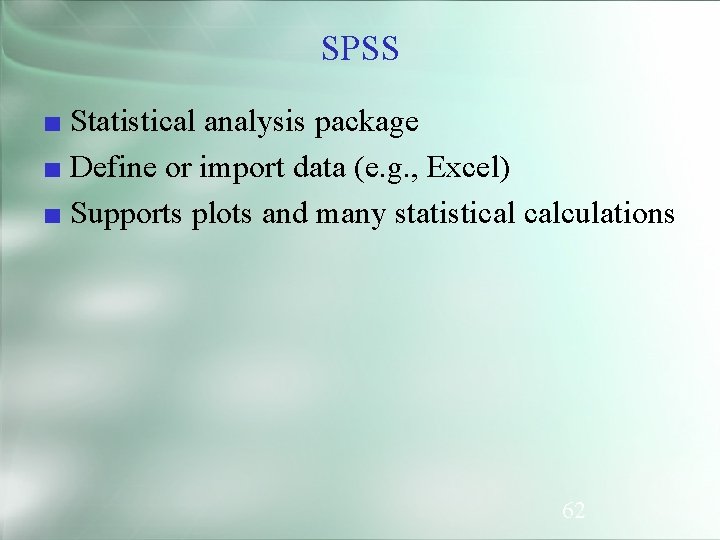
SPSS ■ Statistical analysis package ■ Define or import data (e. g. , Excel) ■ Supports plots and many statistical calculations 62
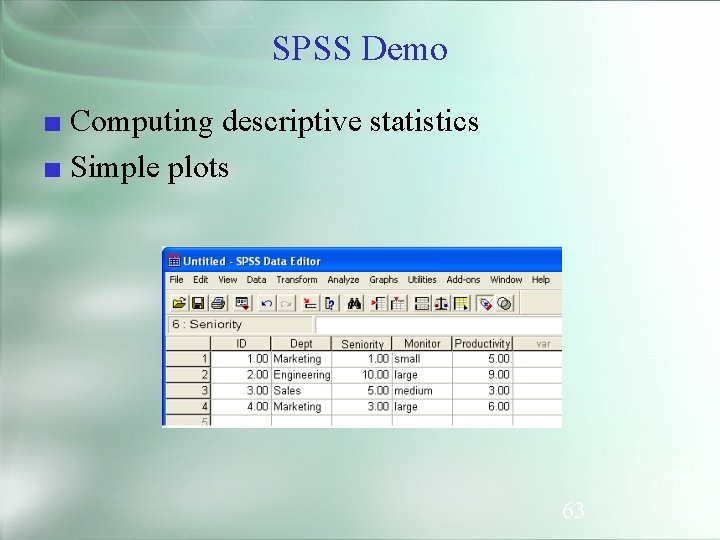
SPSS Demo ■ Computing descriptive statistics ■ Simple plots 63
4800 oak grove drive pasadena
4800 oak grove drive
Early beginnings of tourism
Fermax 4800 esquema
Checkpoint 12600
Mazak fh 4800
Political science methodology
Research methods in exercise science
My favorite subject is
Fabrication of wax pattern
Types of empirical research
Difference between empirical and conceptual research
Applied vs fundamental research
Kontinuitetshantering
Typiska novell drag
Tack för att ni lyssnade bild
Ekologiskt fotavtryck
Varför kallas perioden 1918-1939 för mellankrigstiden?
En lathund för arbete med kontinuitetshantering
Kassaregister ideell förening
Personlig tidbok för yrkesförare
Sura för anatom
Vad är densitet
Datorkunskap för nybörjare
Stig kerman
Mall debattartikel
Delegerande ledarskap
Nyckelkompetenser för livslångt lärande
Påbyggnader för flakfordon
Arkimedes princip formel
Publik sektor
Bo bergman jag fryser om dina händer
Presentera för publik crossboss
Argument för teckenspråk som minoritetsspråk
Plats för toran ark
Klassificeringsstruktur för kommunala verksamheter
Luftstrupen för medicinare
Claes martinsson
Centrum för kunskap och säkerhet
Programskede byggprocessen
Mat för unga idrottare
Verktyg för automatisering av utbetalningar
Rutin för avvikelsehantering
Smärtskolan kunskap för livet
Ministerstyre för och nackdelar
Tack för att ni har lyssnat
Hur ser ett referat ut
Redogör för vad psykologi är
Matematisk modellering eksempel
Tack för att ni har lyssnat
Borra hål för knoppar
Vilken grundregel finns det för tronföljden i sverige?
Formel standardavvikelse
Tack för att ni har lyssnat
Rita perspektiv
Ledningssystem för verksamhetsinformation
Tobinskatten för och nackdelar
Blomman för dagen drog
Modell för handledningsprocess
Egg för emanuel
Elektronik för barn
Kvinnlig mantel i antikens rom
Strategi för svensk viltförvaltning
Kung dog 1611