ONTOLOGY LEARNING DEPARTING FROM THE ONTOLOGY LAYER CAKE
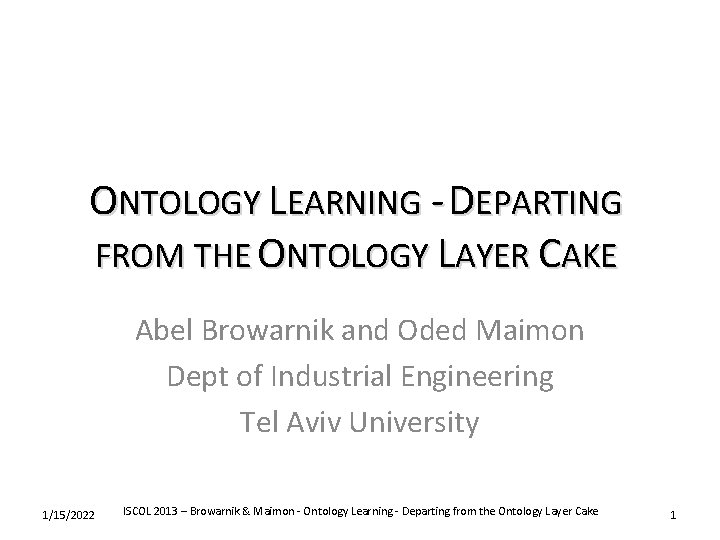
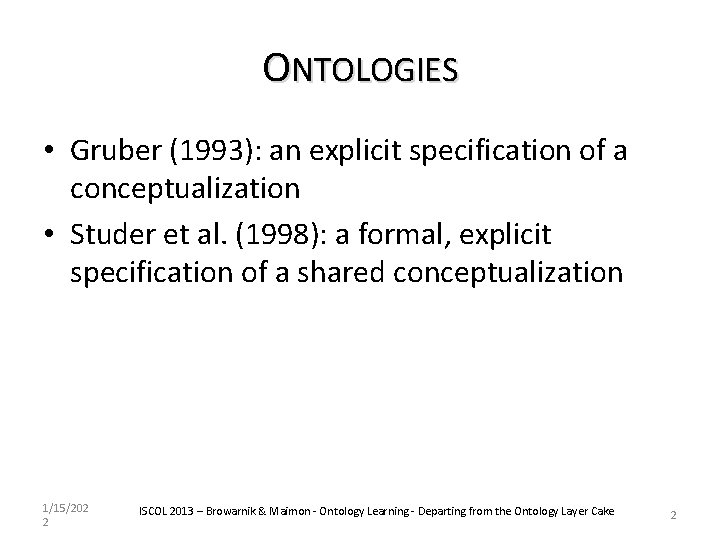
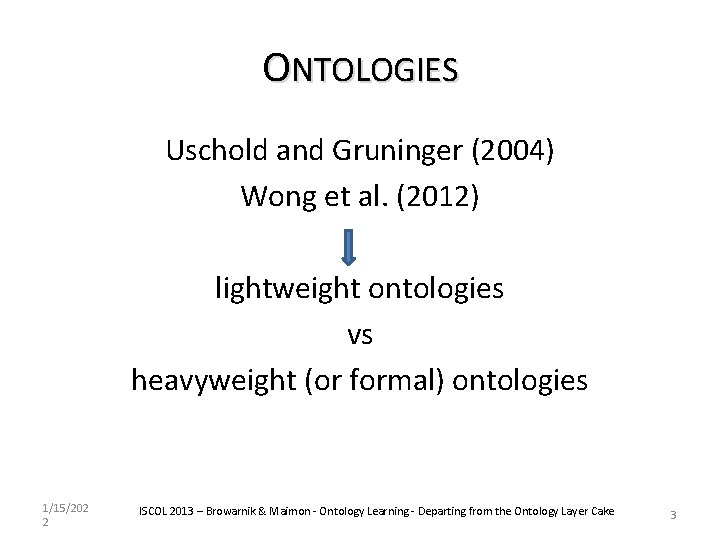
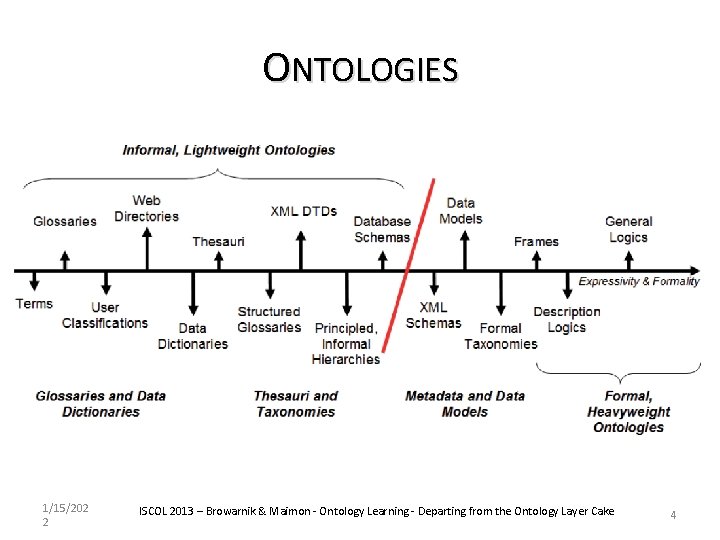
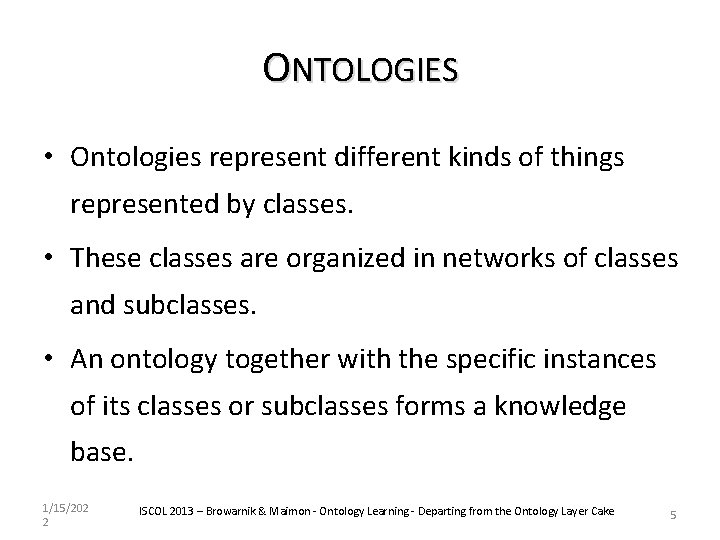
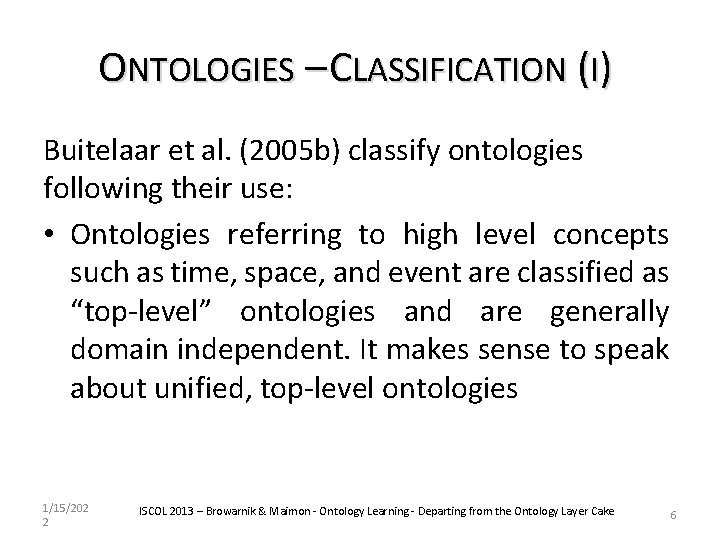
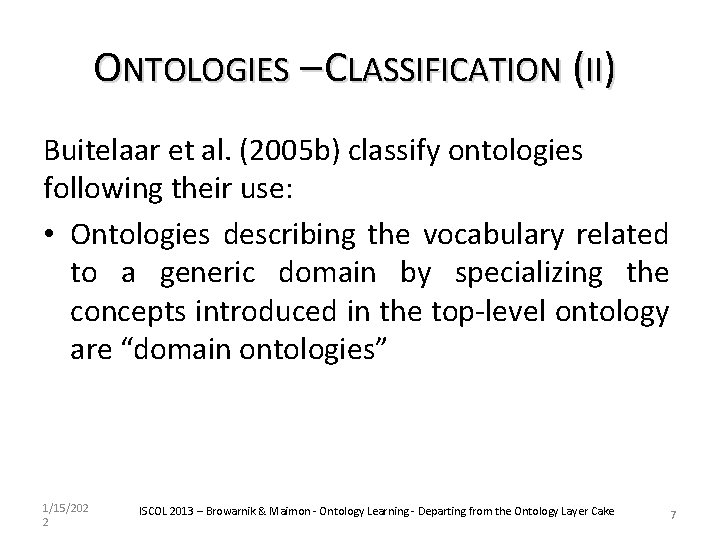
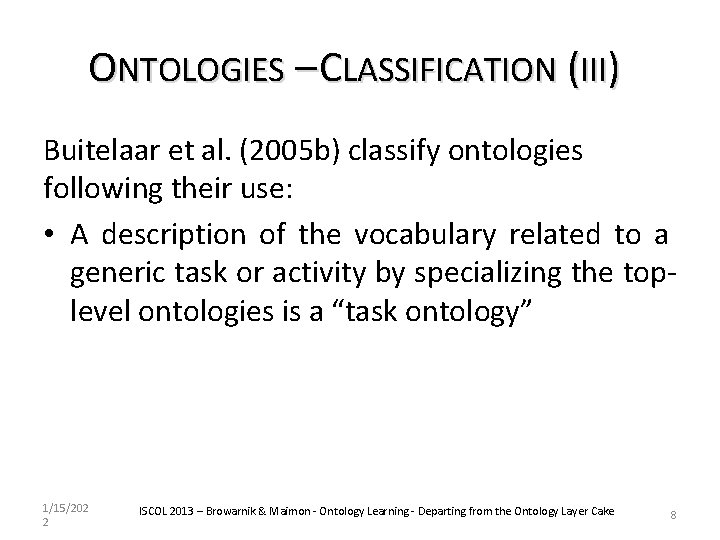
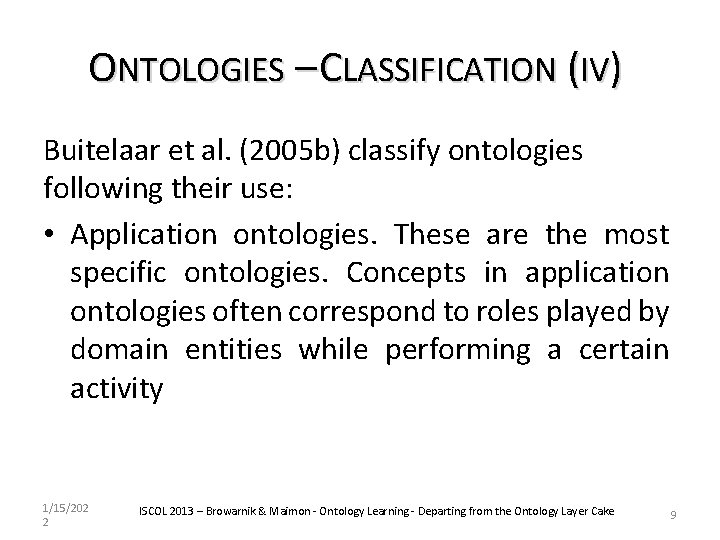
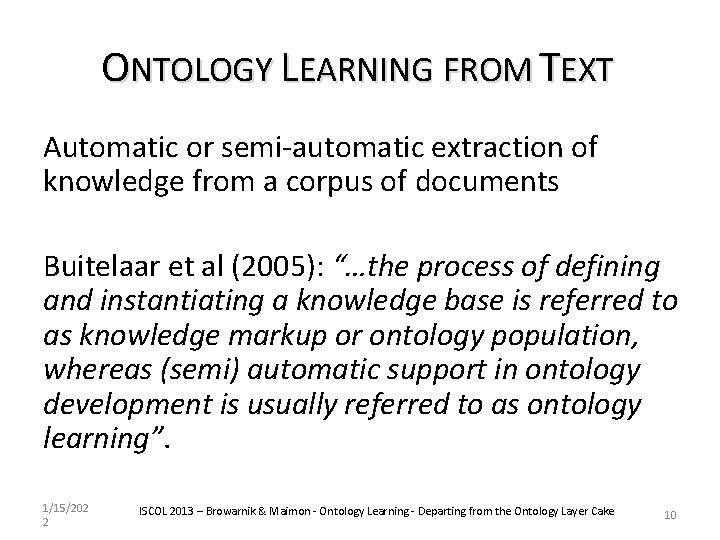
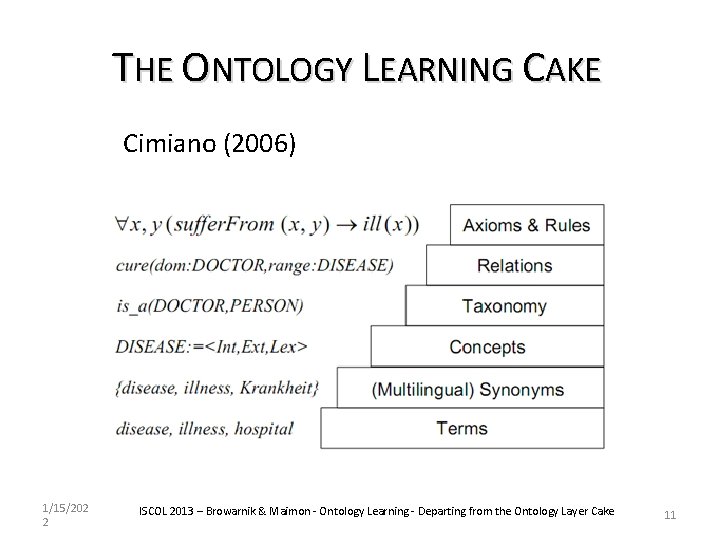
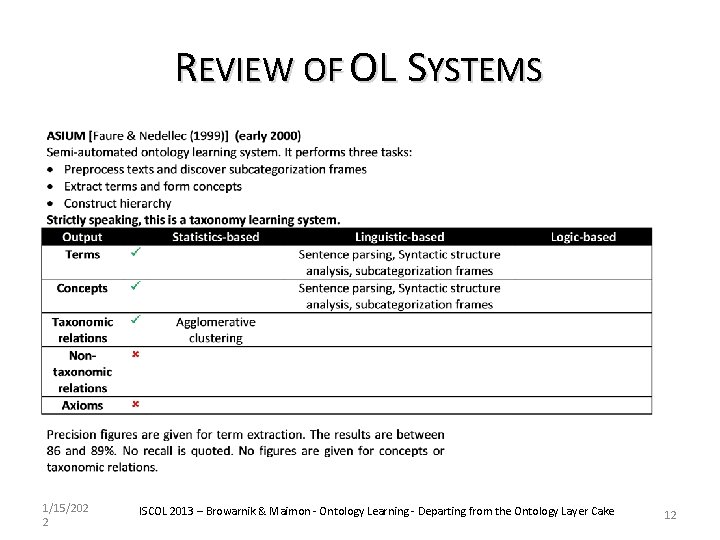
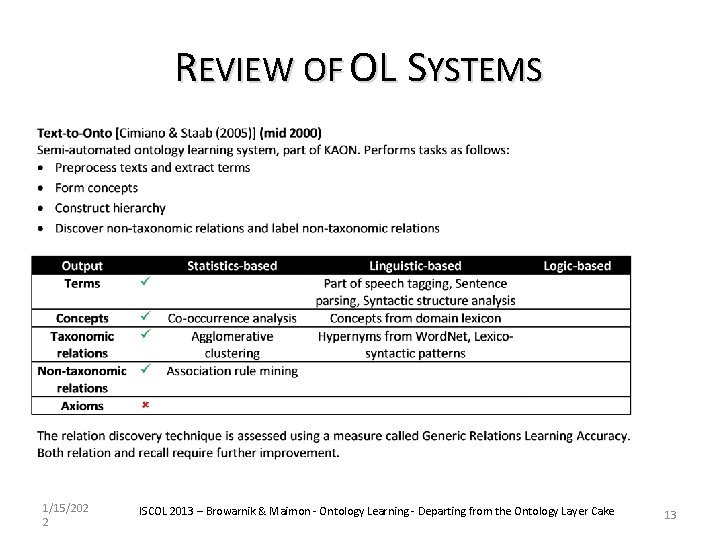
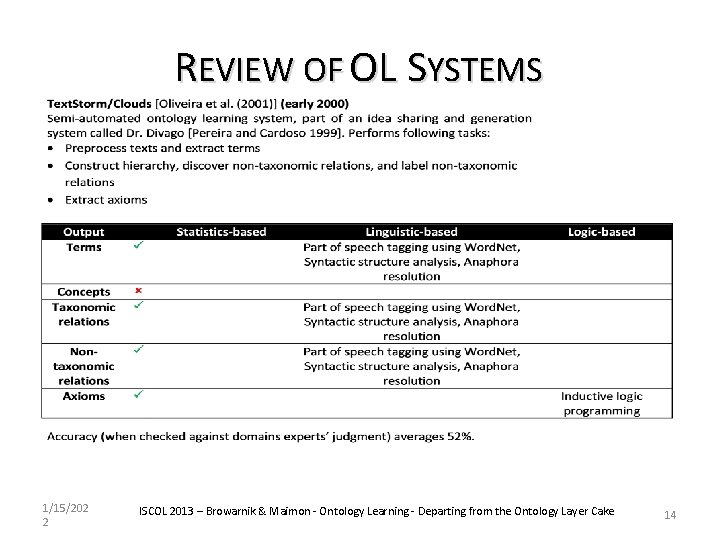
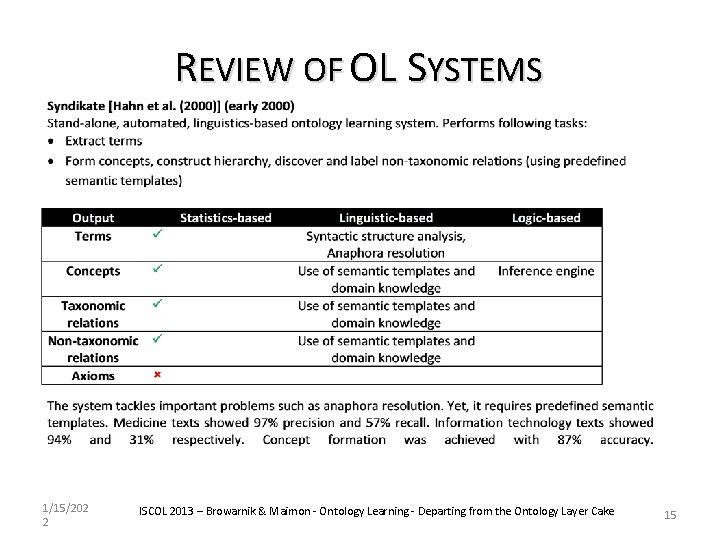
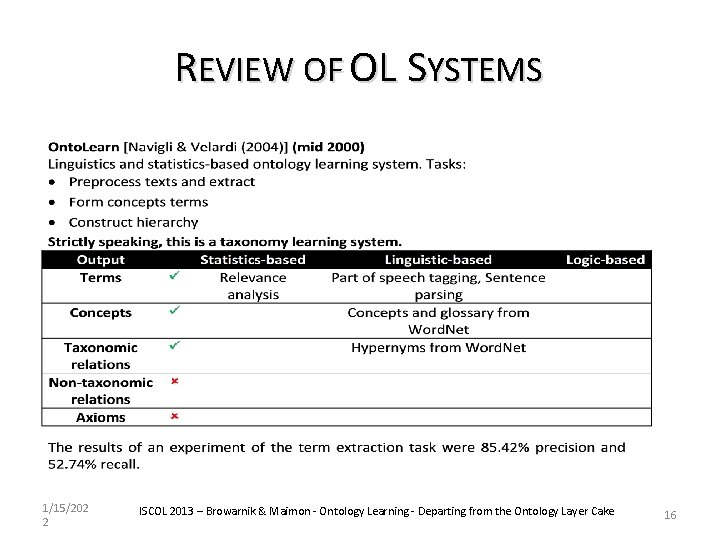
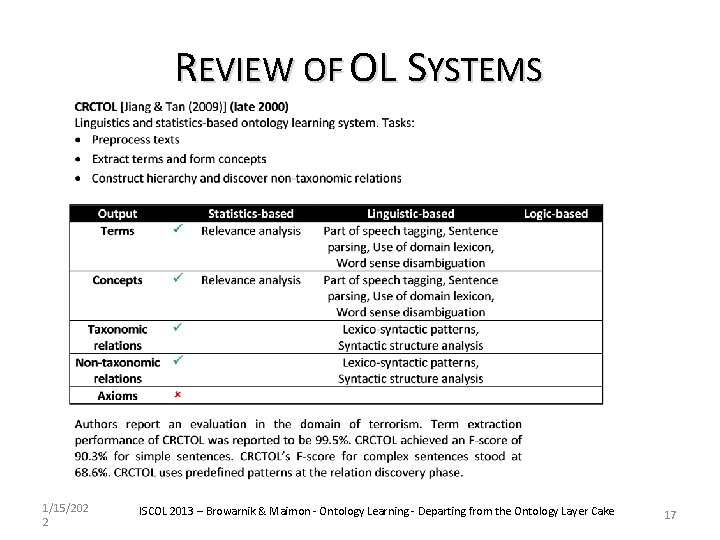
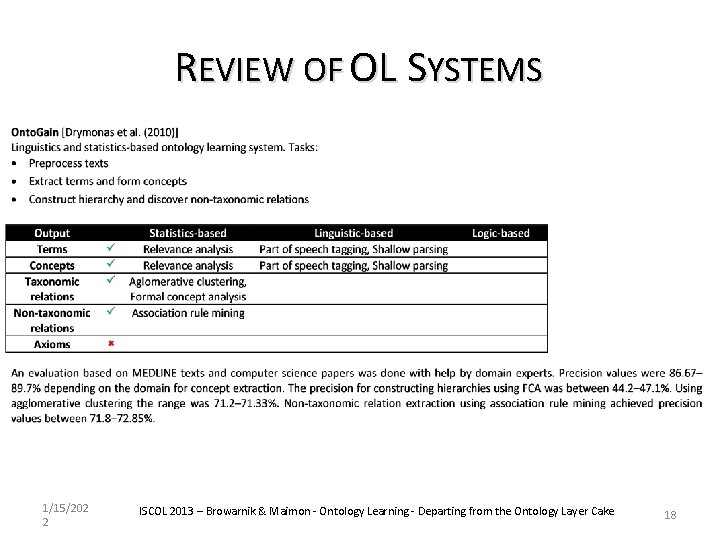
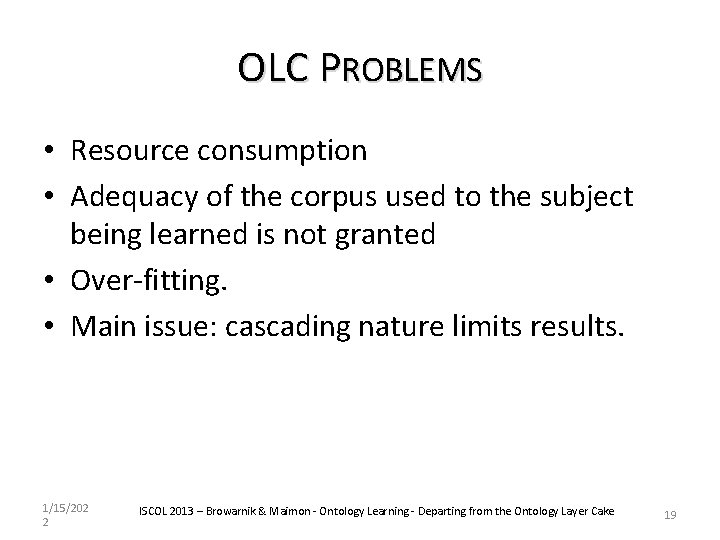
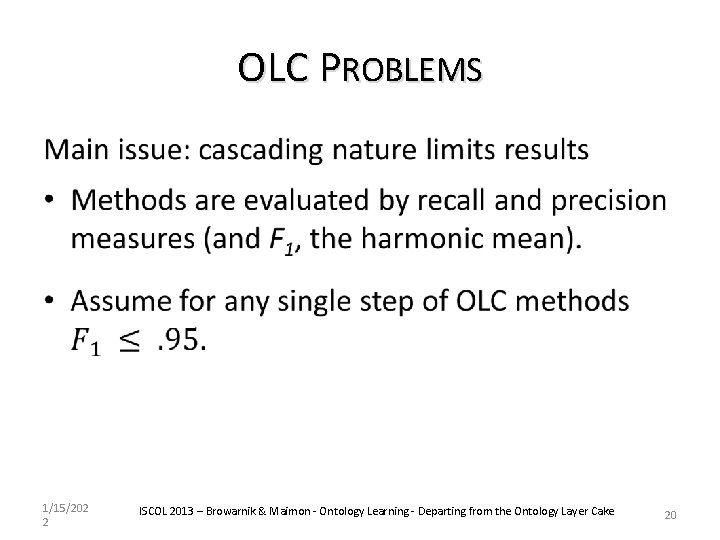
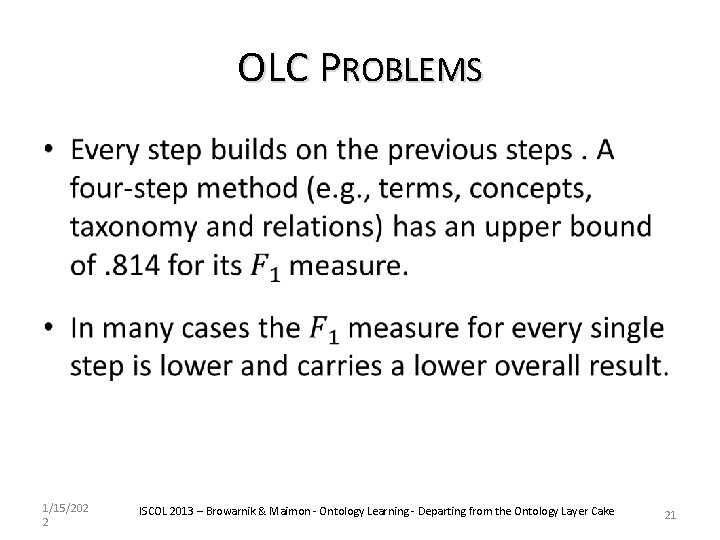
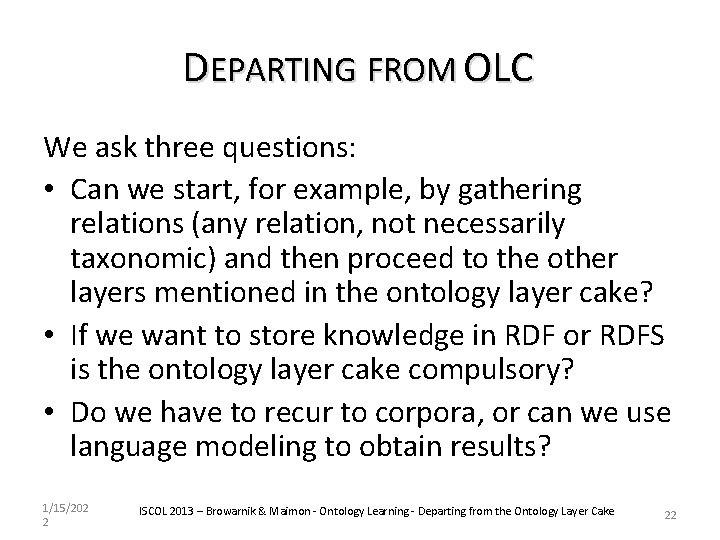
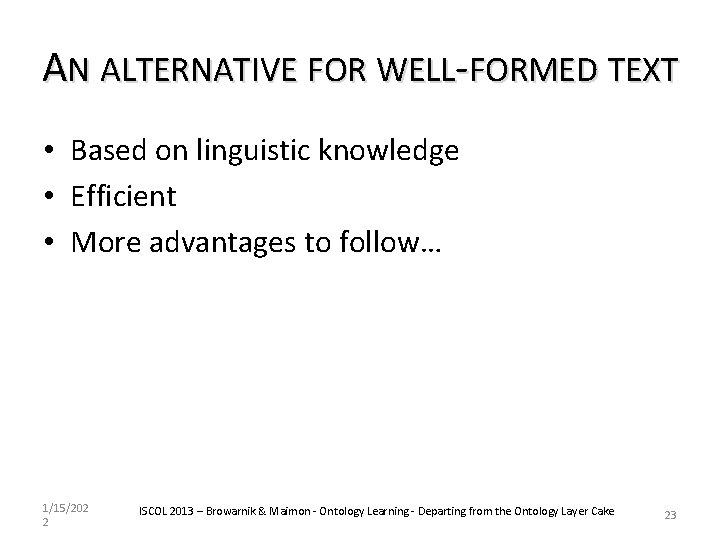
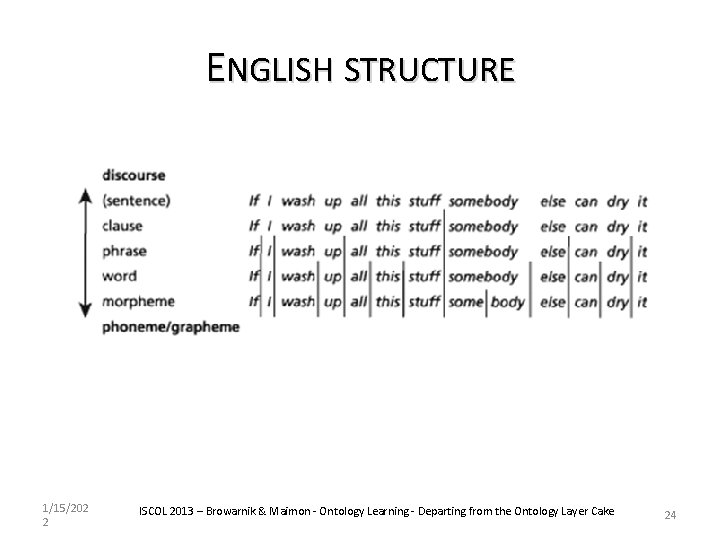
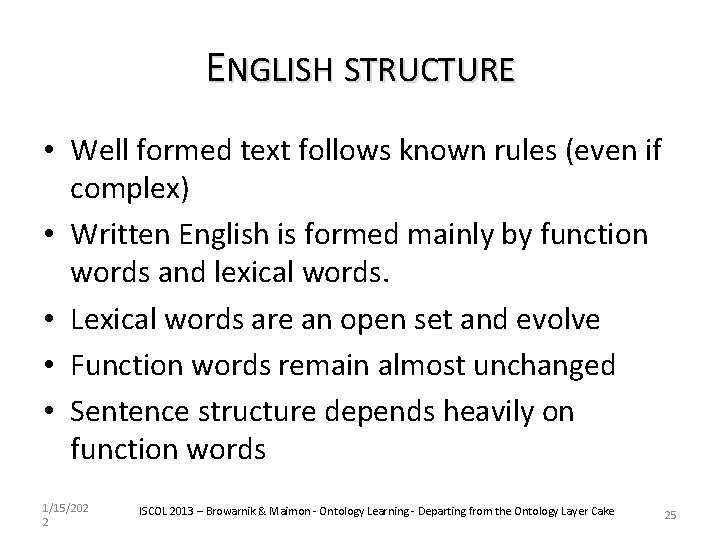
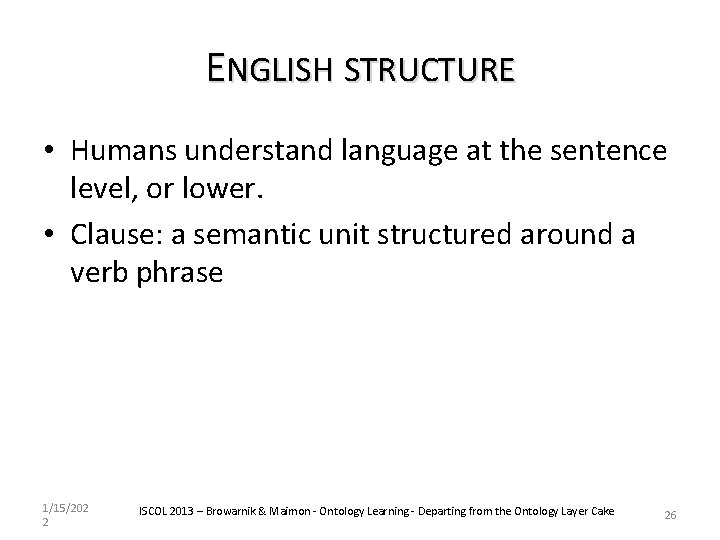
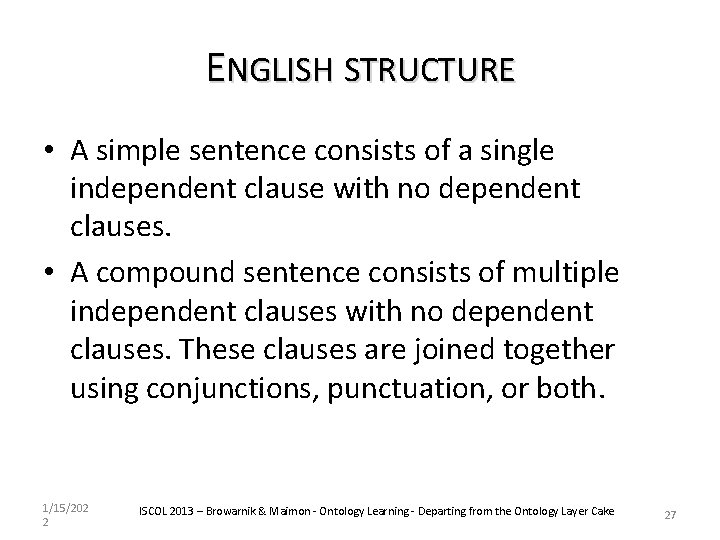
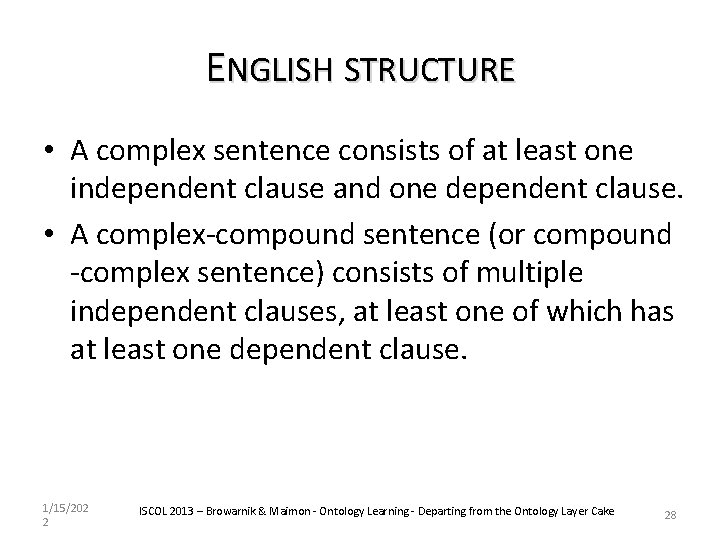
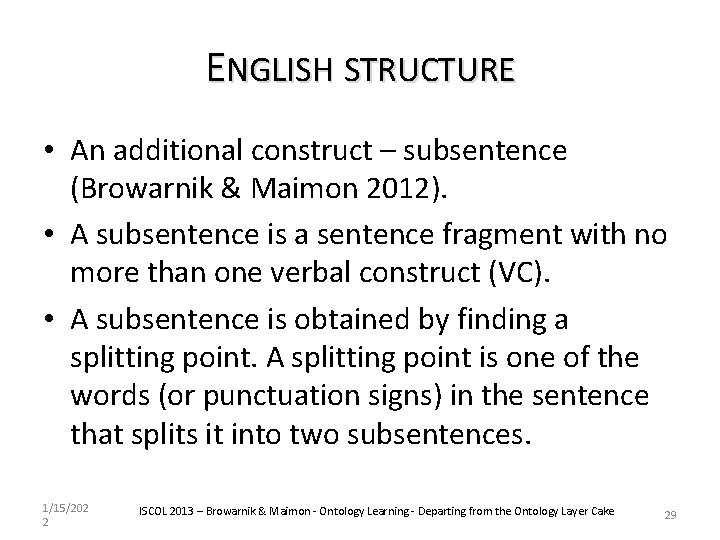
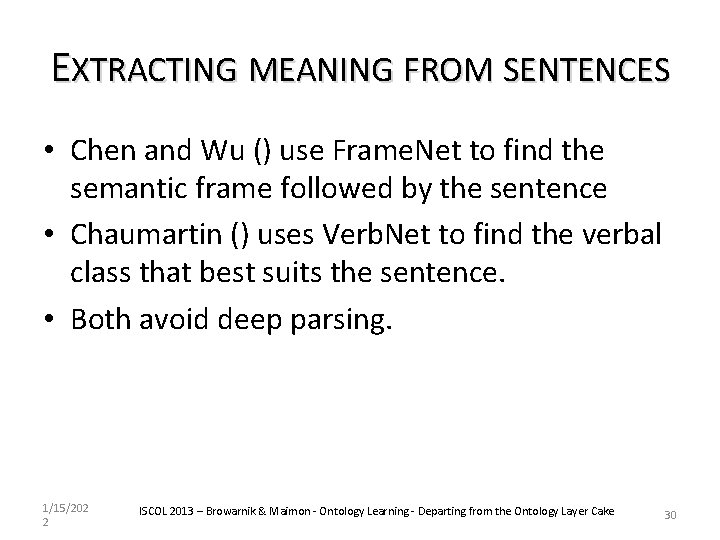
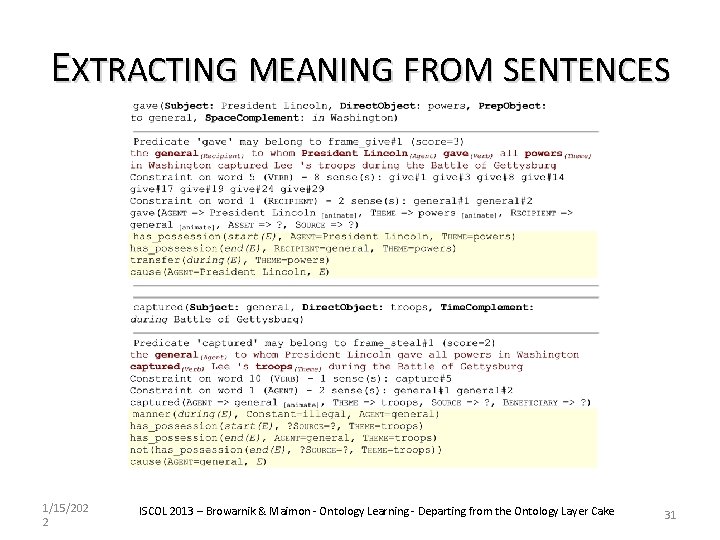
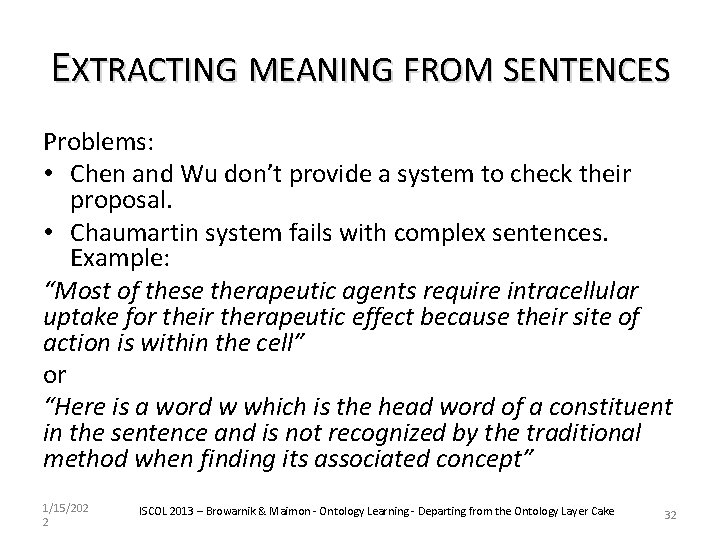
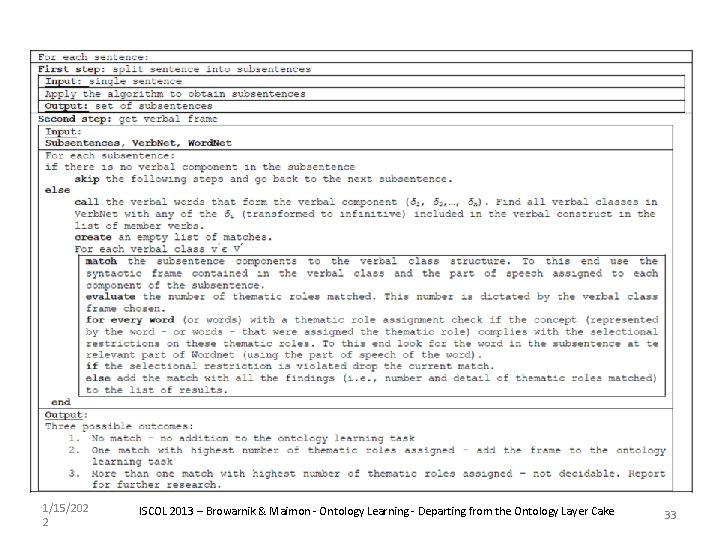
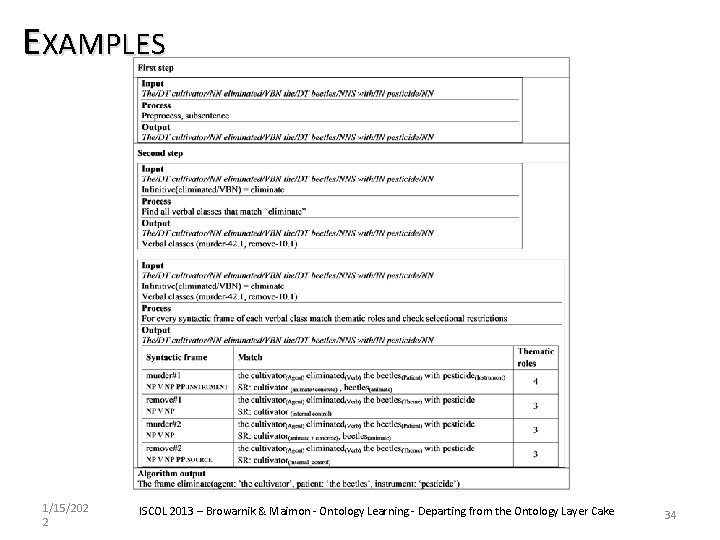
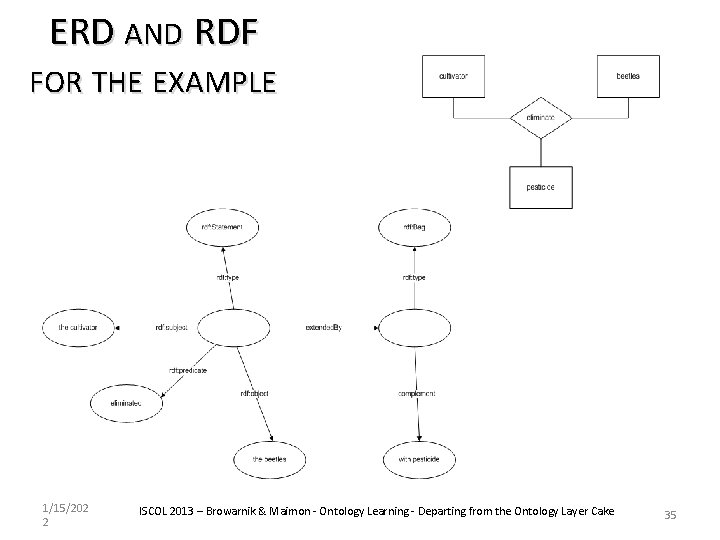
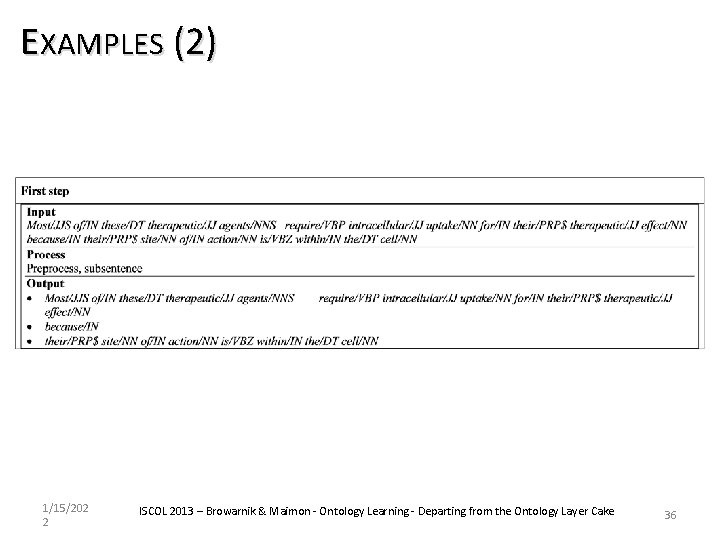
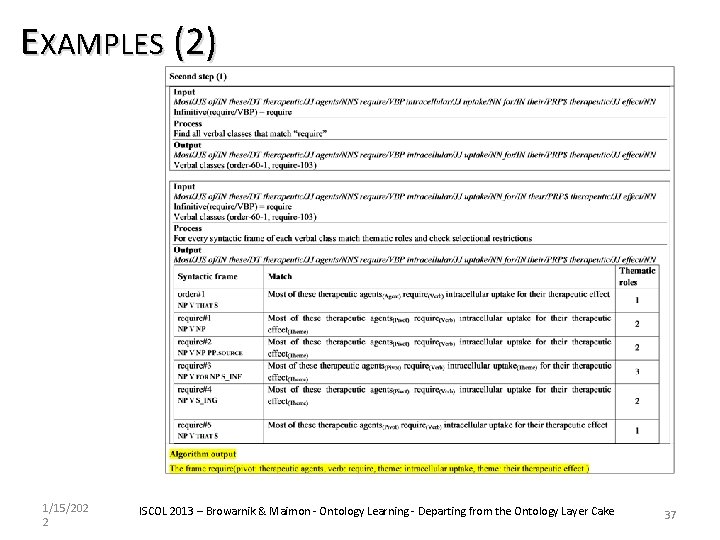
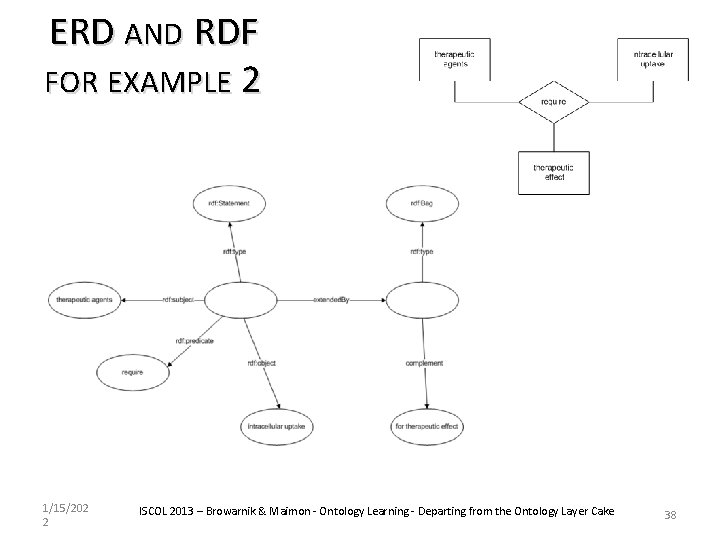
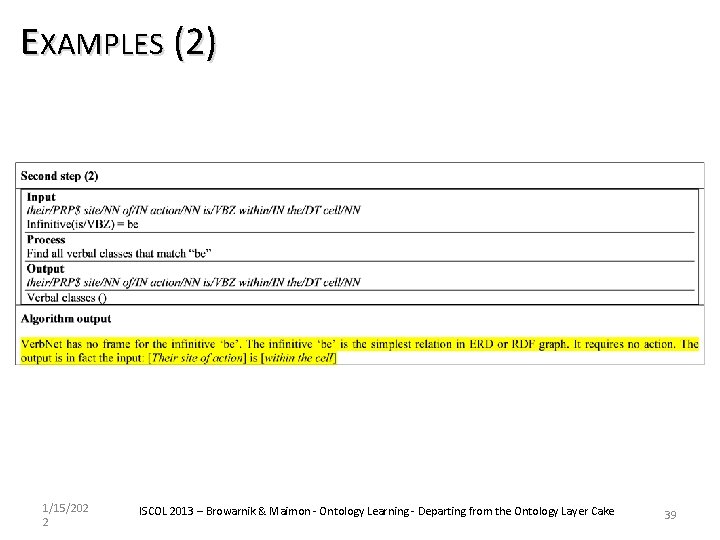
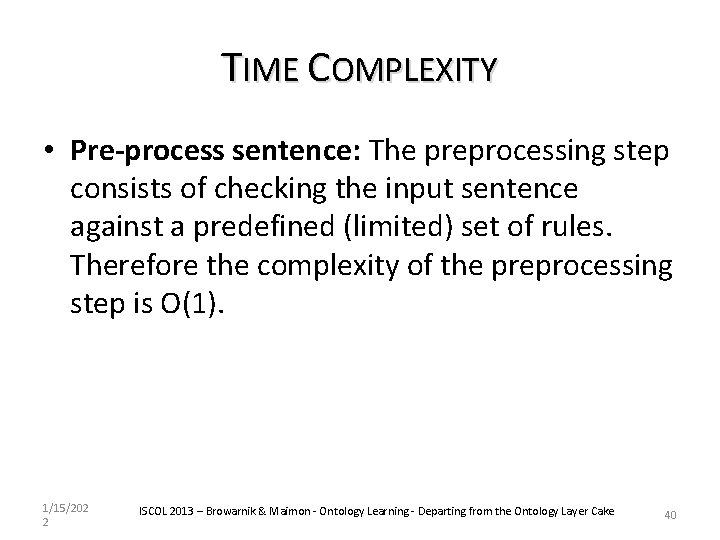
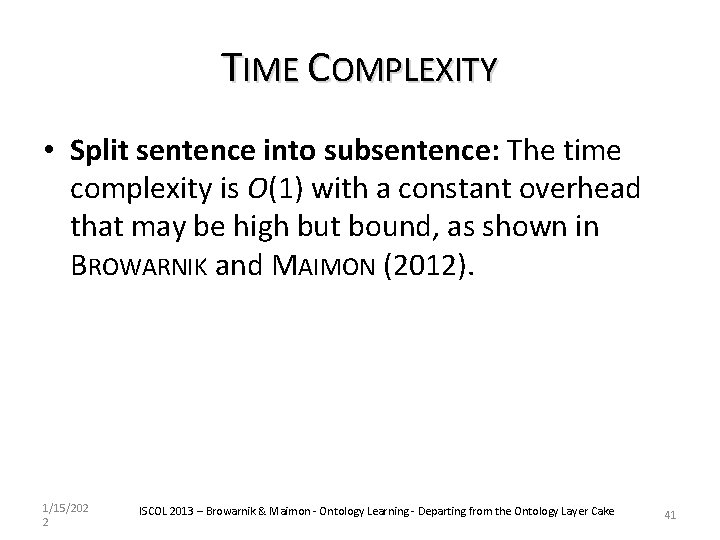
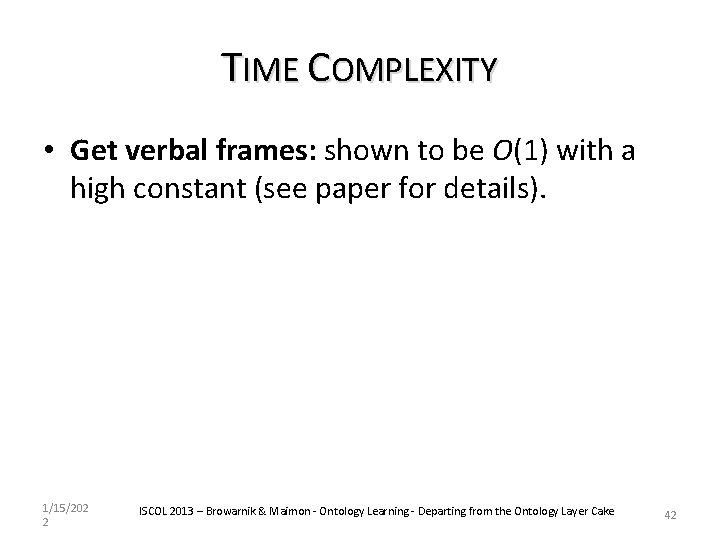
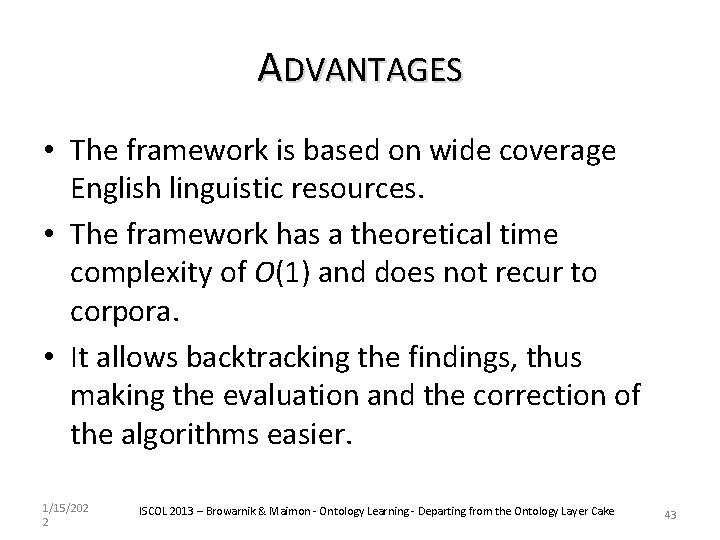
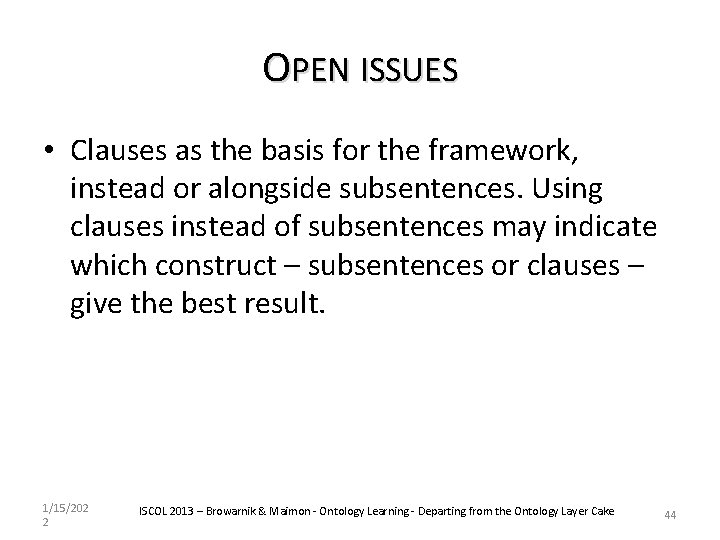
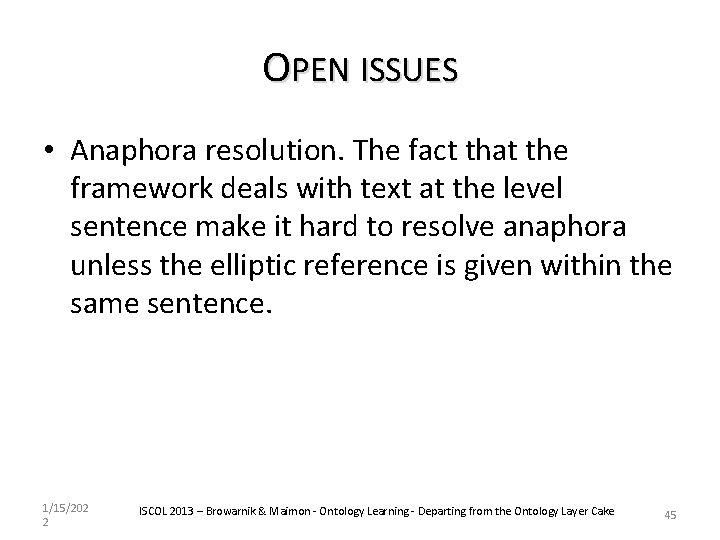
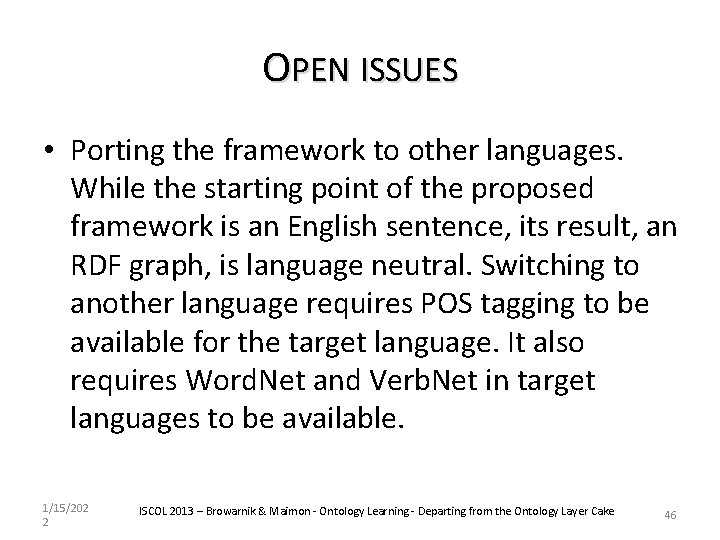
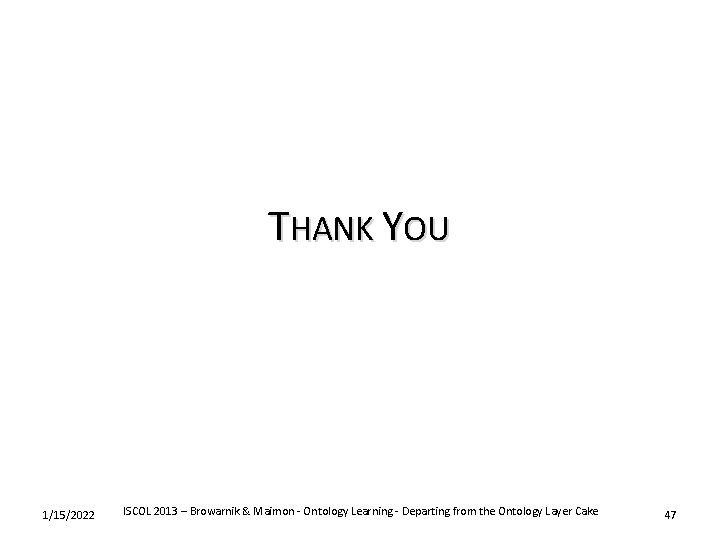
- Slides: 47
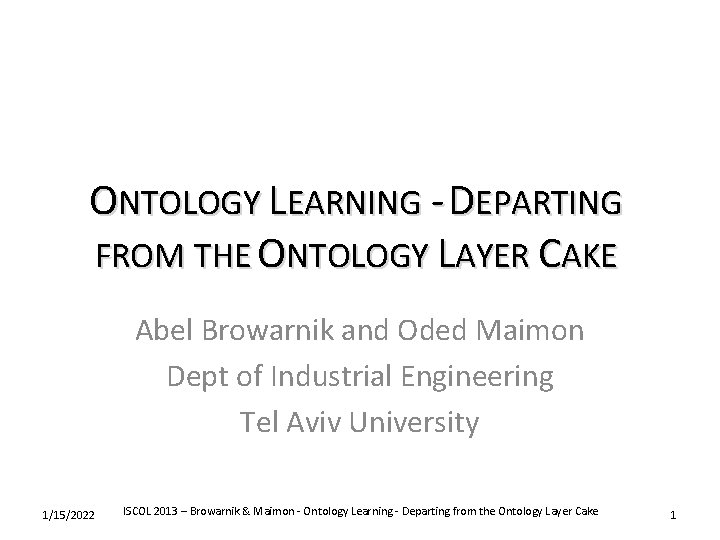
ONTOLOGY LEARNING - DEPARTING FROM THE ONTOLOGY LAYER CAKE Abel Browarnik and Oded Maimon Dept of Industrial Engineering Tel Aviv University 1/15/2022 ISCOL 2013 – Browarnik & Maimon - Ontology Learning - Departing from the Ontology Layer Cake 1
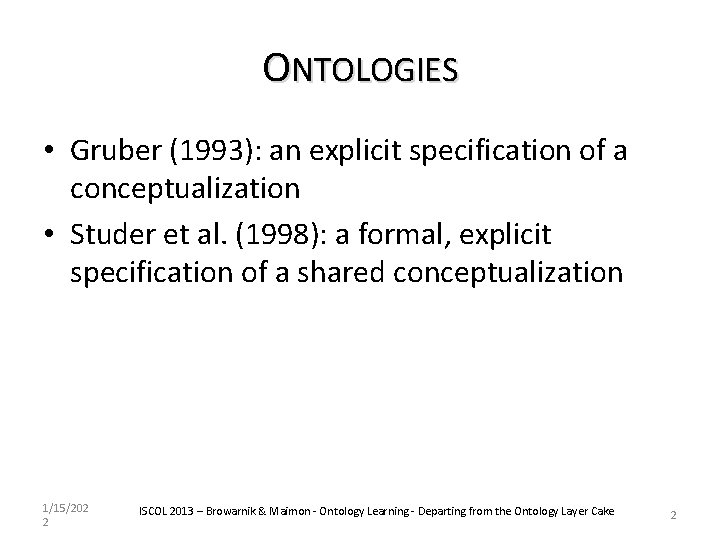
ONTOLOGIES • Gruber (1993): an explicit specification of a conceptualization • Studer et al. (1998): a formal, explicit specification of a shared conceptualization 1/15/202 2 ISCOL 2013 – Browarnik & Maimon - Ontology Learning - Departing from the Ontology Layer Cake 2
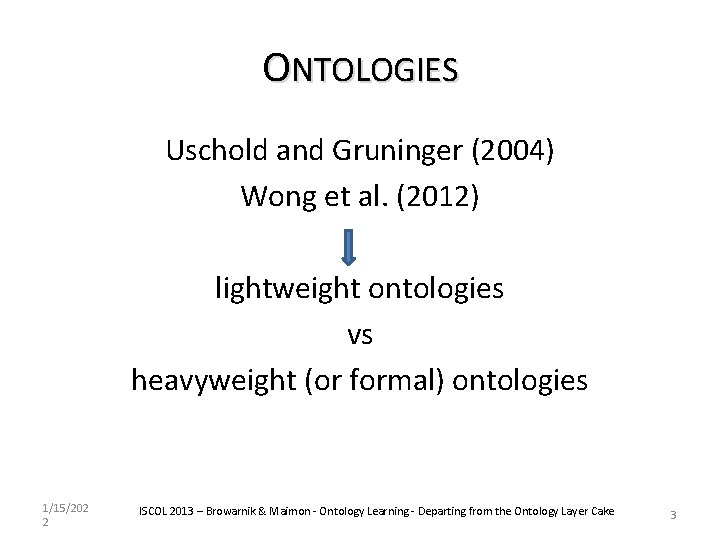
ONTOLOGIES Uschold and Gruninger (2004) Wong et al. (2012) lightweight ontologies vs heavyweight (or formal) ontologies 1/15/202 2 ISCOL 2013 – Browarnik & Maimon - Ontology Learning - Departing from the Ontology Layer Cake 3
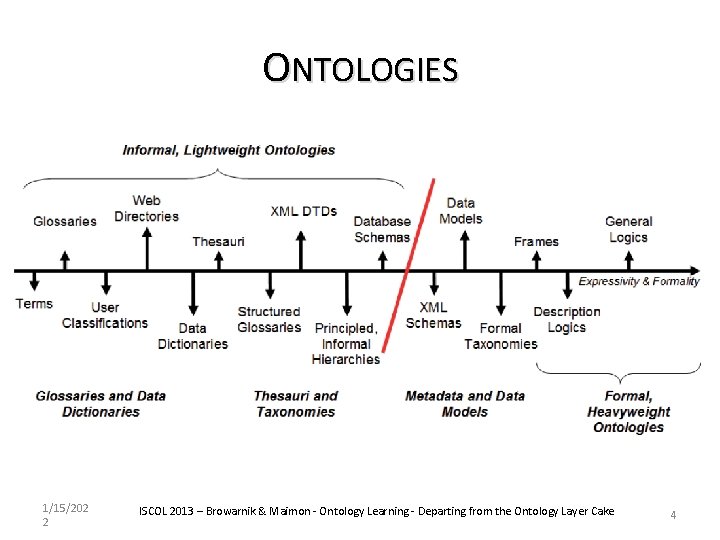
ONTOLOGIES 1/15/202 2 ISCOL 2013 – Browarnik & Maimon - Ontology Learning - Departing from the Ontology Layer Cake 4
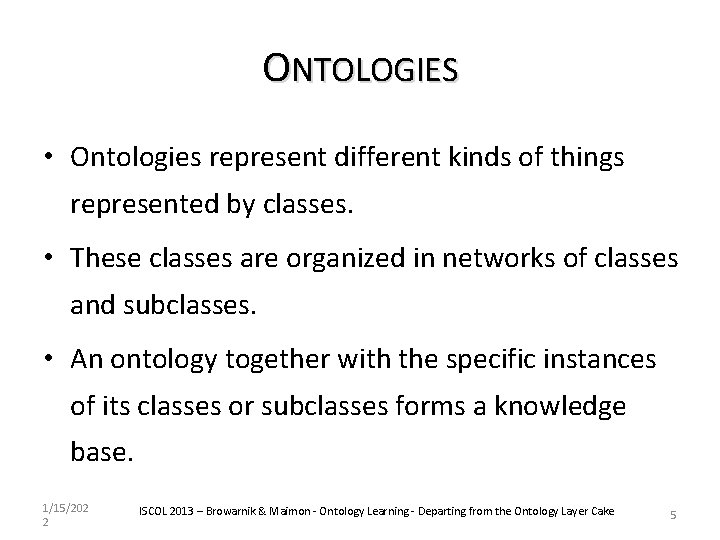
ONTOLOGIES • Ontologies represent different kinds of things represented by classes. • These classes are organized in networks of classes and subclasses. • An ontology together with the specific instances of its classes or subclasses forms a knowledge base. 1/15/202 2 ISCOL 2013 – Browarnik & Maimon - Ontology Learning - Departing from the Ontology Layer Cake 5
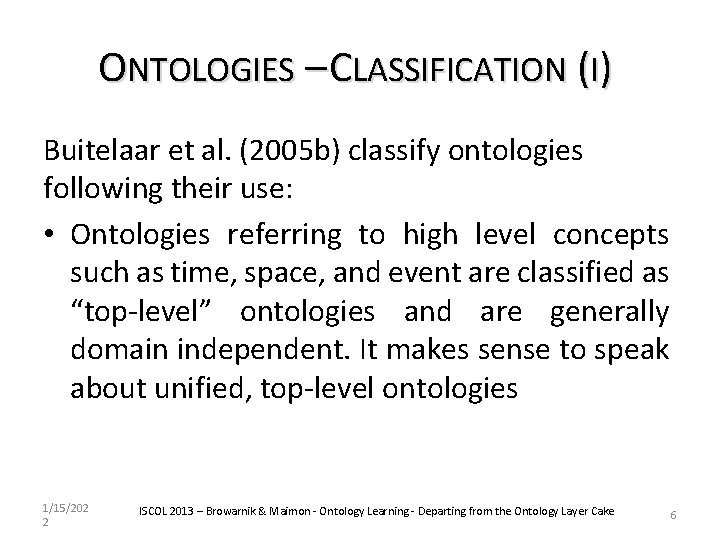
ONTOLOGIES – CLASSIFICATION (I) Buitelaar et al. (2005 b) classify ontologies following their use: • Ontologies referring to high level concepts such as time, space, and event are classified as “top-level” ontologies and are generally domain independent. It makes sense to speak about unified, top-level ontologies 1/15/202 2 ISCOL 2013 – Browarnik & Maimon - Ontology Learning - Departing from the Ontology Layer Cake 6
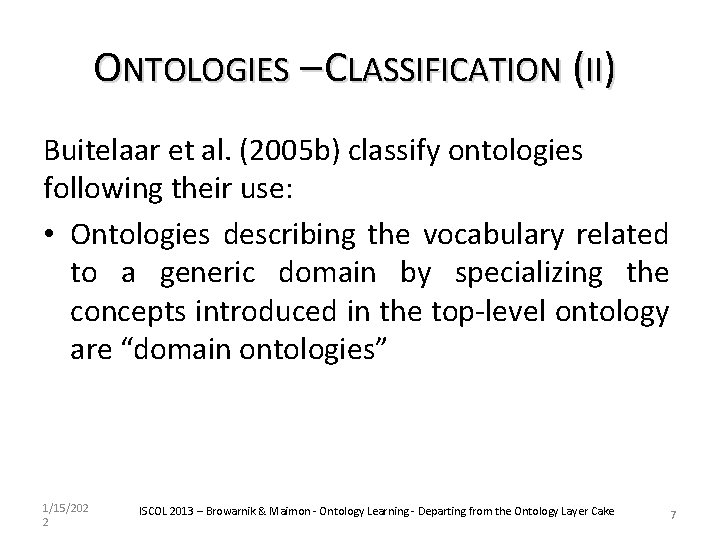
ONTOLOGIES – CLASSIFICATION (II) Buitelaar et al. (2005 b) classify ontologies following their use: • Ontologies describing the vocabulary related to a generic domain by specializing the concepts introduced in the top-level ontology are “domain ontologies” 1/15/202 2 ISCOL 2013 – Browarnik & Maimon - Ontology Learning - Departing from the Ontology Layer Cake 7
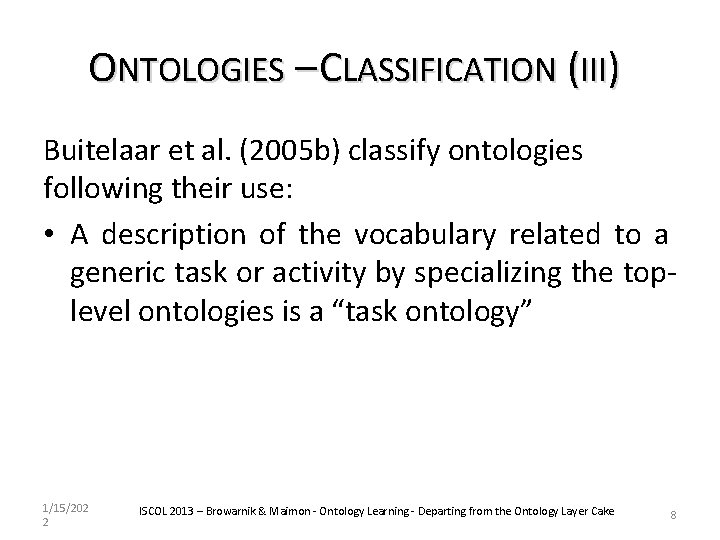
ONTOLOGIES – CLASSIFICATION (III) Buitelaar et al. (2005 b) classify ontologies following their use: • A description of the vocabulary related to a generic task or activity by specializing the toplevel ontologies is a “task ontology” 1/15/202 2 ISCOL 2013 – Browarnik & Maimon - Ontology Learning - Departing from the Ontology Layer Cake 8
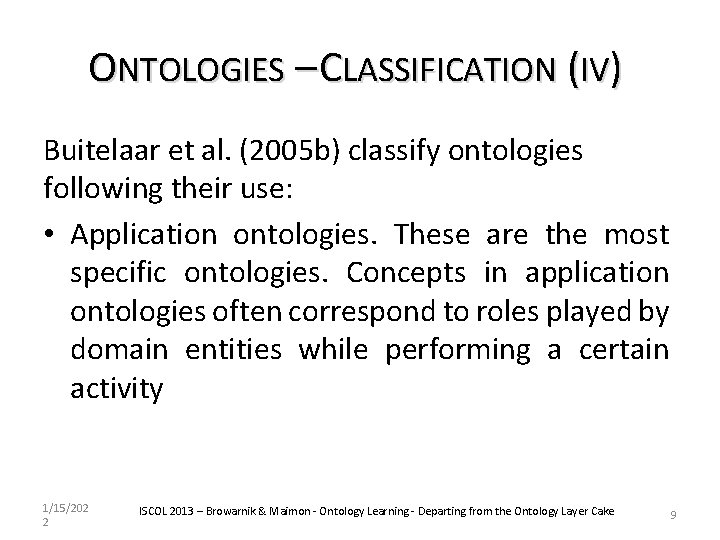
ONTOLOGIES – CLASSIFICATION (IV) Buitelaar et al. (2005 b) classify ontologies following their use: • Application ontologies. These are the most specific ontologies. Concepts in application ontologies often correspond to roles played by domain entities while performing a certain activity 1/15/202 2 ISCOL 2013 – Browarnik & Maimon - Ontology Learning - Departing from the Ontology Layer Cake 9
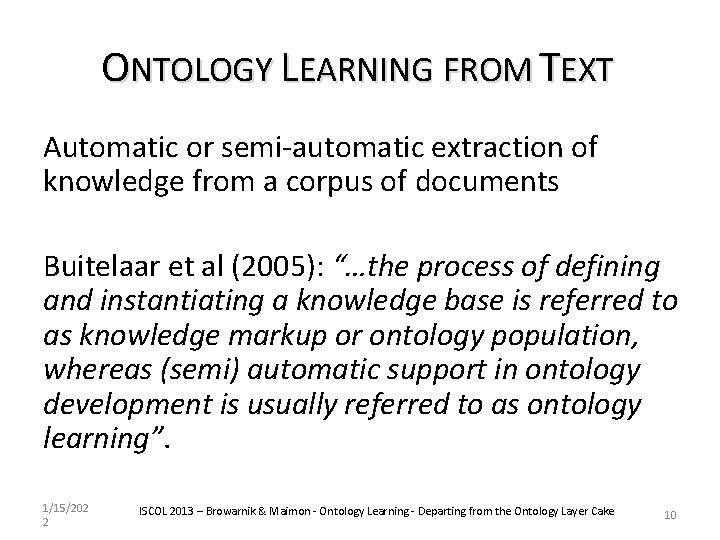
ONTOLOGY LEARNING FROM TEXT Automatic or semi-automatic extraction of knowledge from a corpus of documents Buitelaar et al (2005): “…the process of defining and instantiating a knowledge base is referred to as knowledge markup or ontology population, whereas (semi) automatic support in ontology development is usually referred to as ontology learning”. 1/15/202 2 ISCOL 2013 – Browarnik & Maimon - Ontology Learning - Departing from the Ontology Layer Cake 10
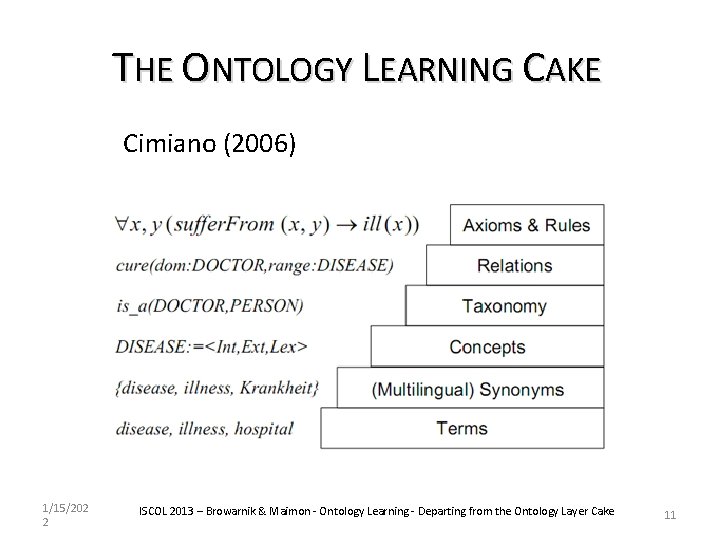
THE ONTOLOGY LEARNING CAKE Cimiano (2006) 1/15/202 2 ISCOL 2013 – Browarnik & Maimon - Ontology Learning - Departing from the Ontology Layer Cake 11
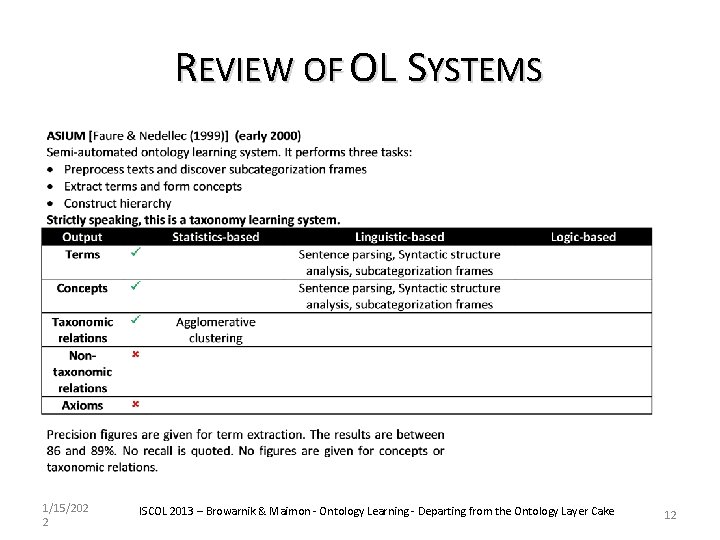
REVIEW OF OL SYSTEMS 1/15/202 2 ISCOL 2013 – Browarnik & Maimon - Ontology Learning - Departing from the Ontology Layer Cake 12
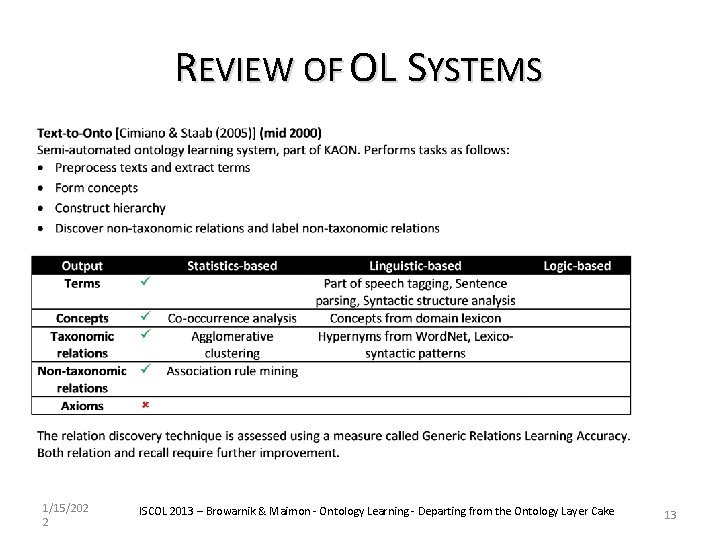
REVIEW OF OL SYSTEMS 1/15/202 2 ISCOL 2013 – Browarnik & Maimon - Ontology Learning - Departing from the Ontology Layer Cake 13
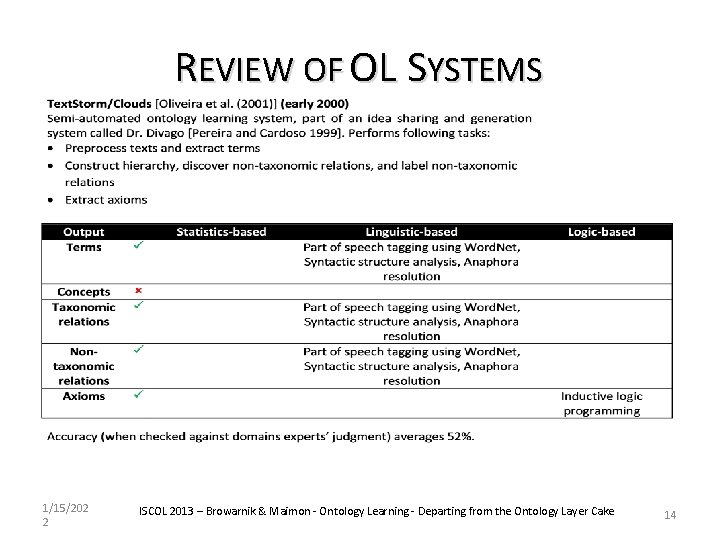
REVIEW OF OL SYSTEMS 1/15/202 2 ISCOL 2013 – Browarnik & Maimon - Ontology Learning - Departing from the Ontology Layer Cake 14
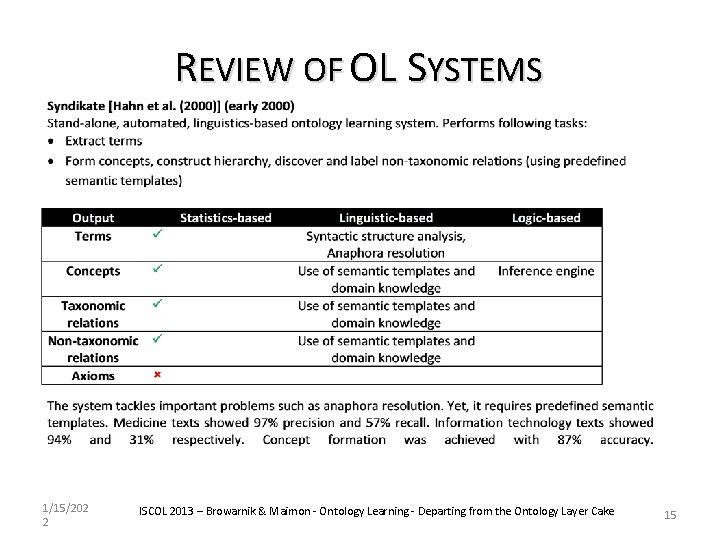
REVIEW OF OL SYSTEMS 1/15/202 2 ISCOL 2013 – Browarnik & Maimon - Ontology Learning - Departing from the Ontology Layer Cake 15
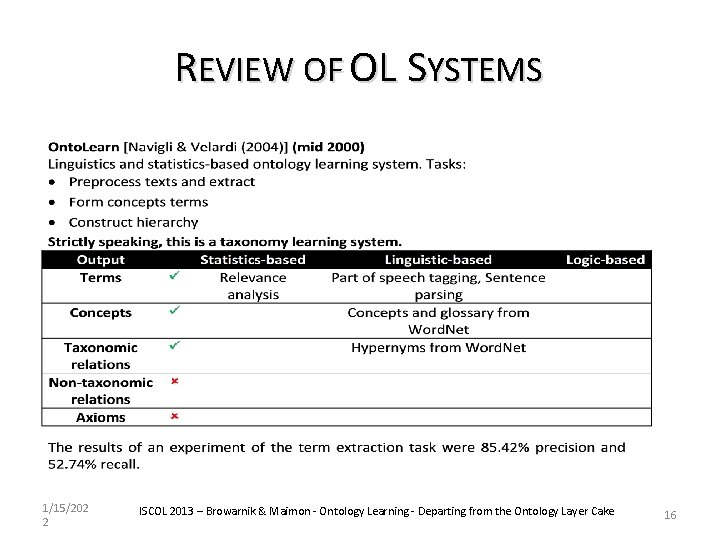
REVIEW OF OL SYSTEMS 1/15/202 2 ISCOL 2013 – Browarnik & Maimon - Ontology Learning - Departing from the Ontology Layer Cake 16
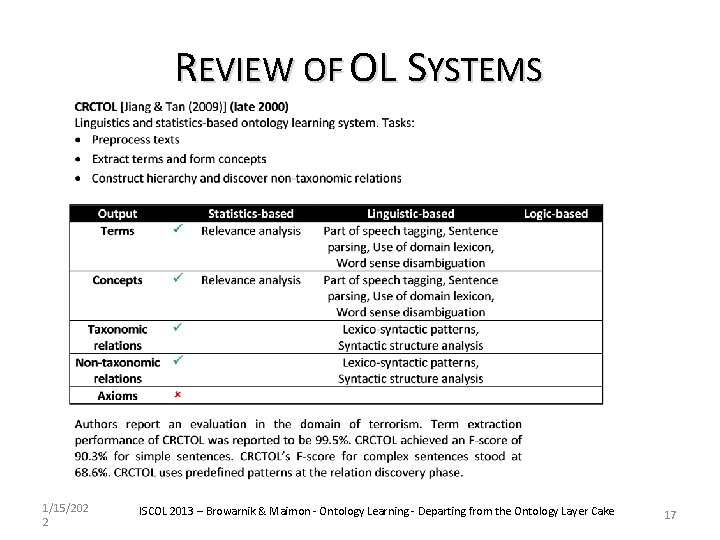
REVIEW OF OL SYSTEMS 1/15/202 2 ISCOL 2013 – Browarnik & Maimon - Ontology Learning - Departing from the Ontology Layer Cake 17
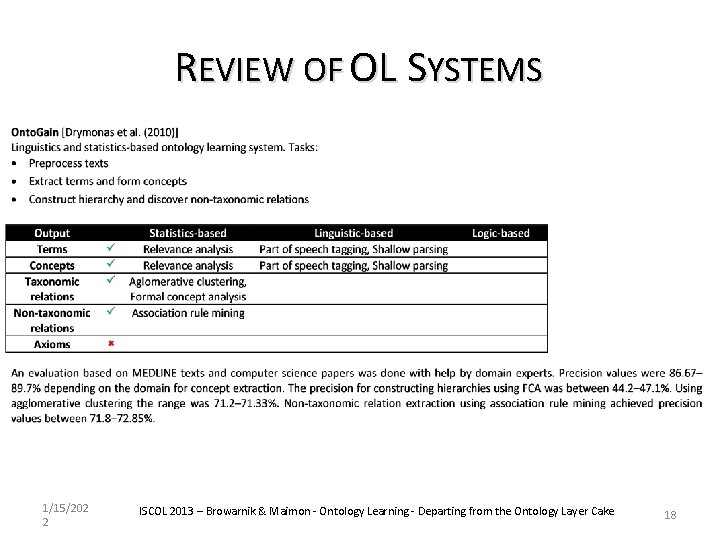
REVIEW OF OL SYSTEMS 1/15/202 2 ISCOL 2013 – Browarnik & Maimon - Ontology Learning - Departing from the Ontology Layer Cake 18
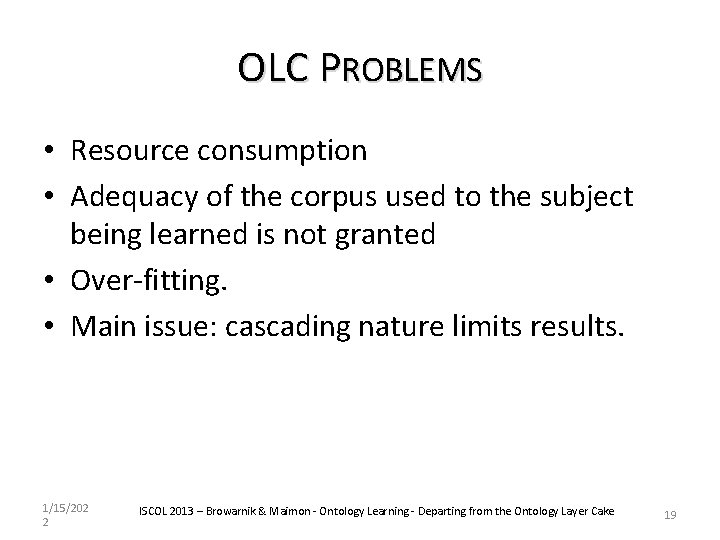
OLC PROBLEMS • Resource consumption • Adequacy of the corpus used to the subject being learned is not granted • Over-fitting. • Main issue: cascading nature limits results. 1/15/202 2 ISCOL 2013 – Browarnik & Maimon - Ontology Learning - Departing from the Ontology Layer Cake 19
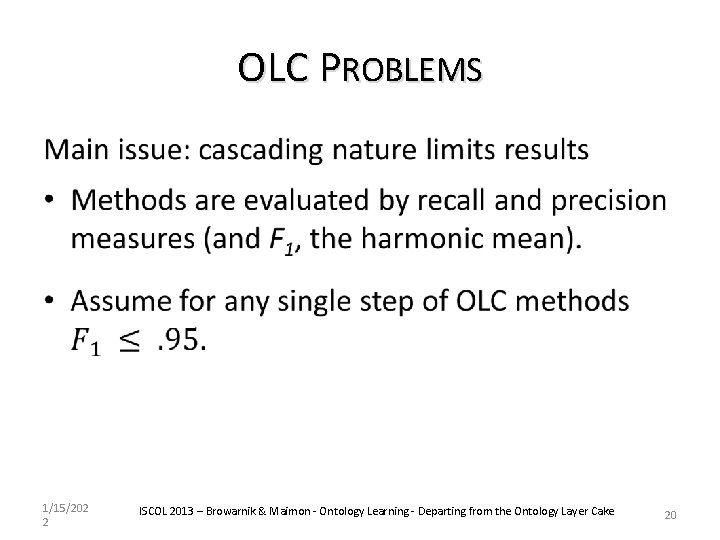
OLC PROBLEMS • 1/15/202 2 ISCOL 2013 – Browarnik & Maimon - Ontology Learning - Departing from the Ontology Layer Cake 20
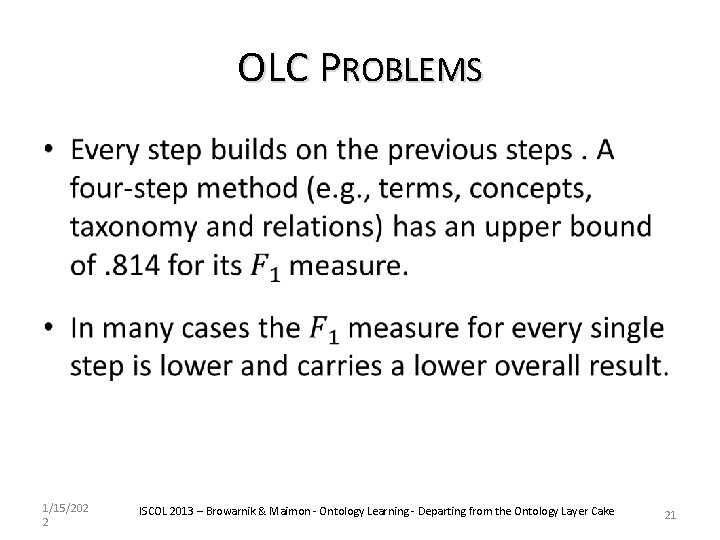
OLC PROBLEMS • 1/15/202 2 ISCOL 2013 – Browarnik & Maimon - Ontology Learning - Departing from the Ontology Layer Cake 21
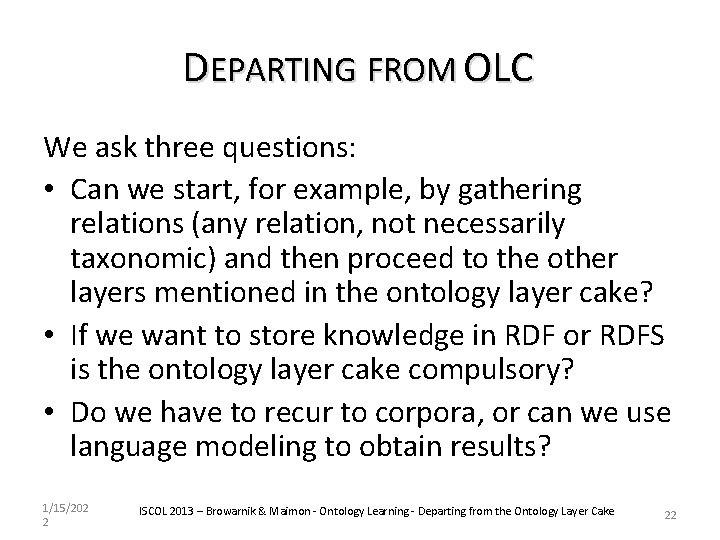
DEPARTING FROM OLC We ask three questions: • Can we start, for example, by gathering relations (any relation, not necessarily taxonomic) and then proceed to the other layers mentioned in the ontology layer cake? • If we want to store knowledge in RDF or RDFS is the ontology layer cake compulsory? • Do we have to recur to corpora, or can we use language modeling to obtain results? 1/15/202 2 ISCOL 2013 – Browarnik & Maimon - Ontology Learning - Departing from the Ontology Layer Cake 22
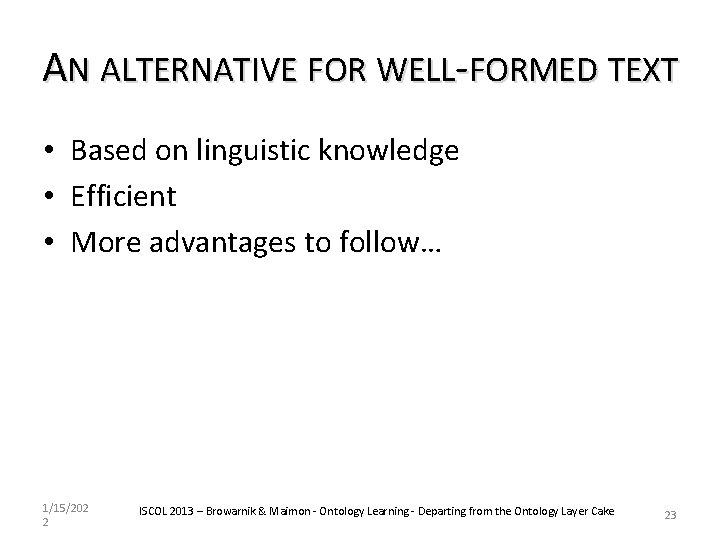
AN ALTERNATIVE FOR WELL-FORMED TEXT • Based on linguistic knowledge • Efficient • More advantages to follow… 1/15/202 2 ISCOL 2013 – Browarnik & Maimon - Ontology Learning - Departing from the Ontology Layer Cake 23
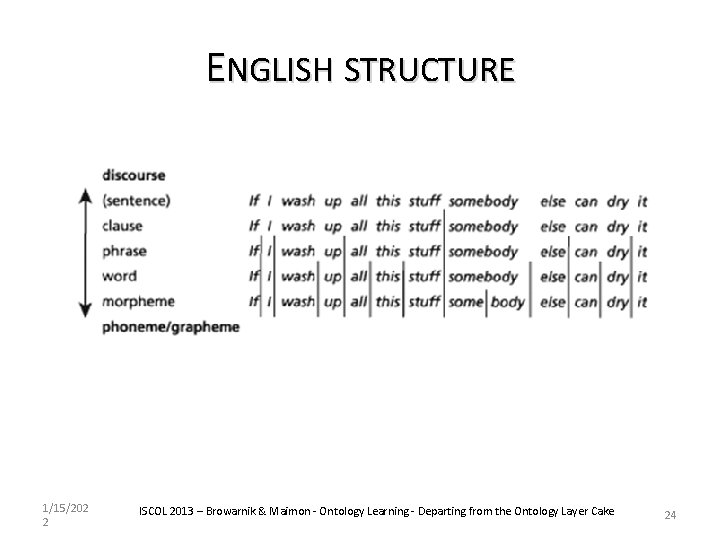
ENGLISH STRUCTURE 1/15/202 2 ISCOL 2013 – Browarnik & Maimon - Ontology Learning - Departing from the Ontology Layer Cake 24
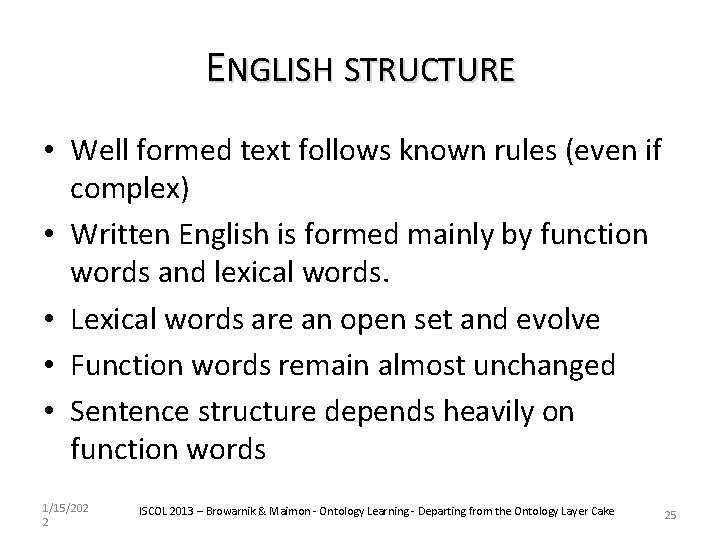
ENGLISH STRUCTURE • Well formed text follows known rules (even if complex) • Written English is formed mainly by function words and lexical words. • Lexical words are an open set and evolve • Function words remain almost unchanged • Sentence structure depends heavily on function words 1/15/202 2 ISCOL 2013 – Browarnik & Maimon - Ontology Learning - Departing from the Ontology Layer Cake 25
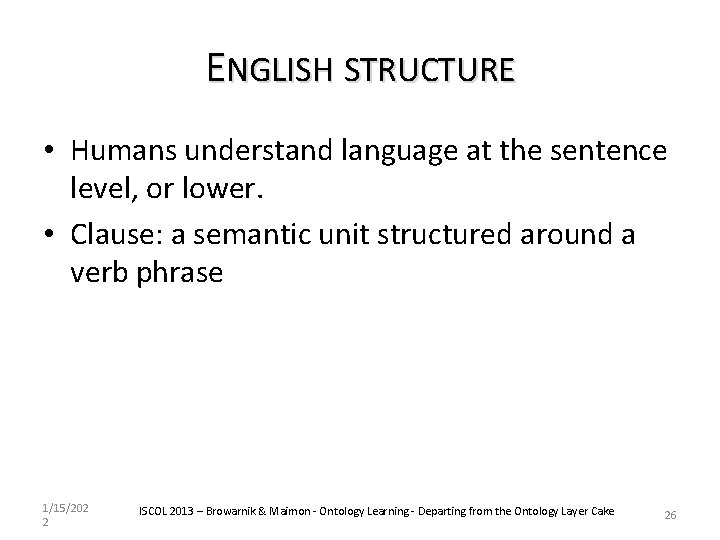
ENGLISH STRUCTURE • Humans understand language at the sentence level, or lower. • Clause: a semantic unit structured around a verb phrase 1/15/202 2 ISCOL 2013 – Browarnik & Maimon - Ontology Learning - Departing from the Ontology Layer Cake 26
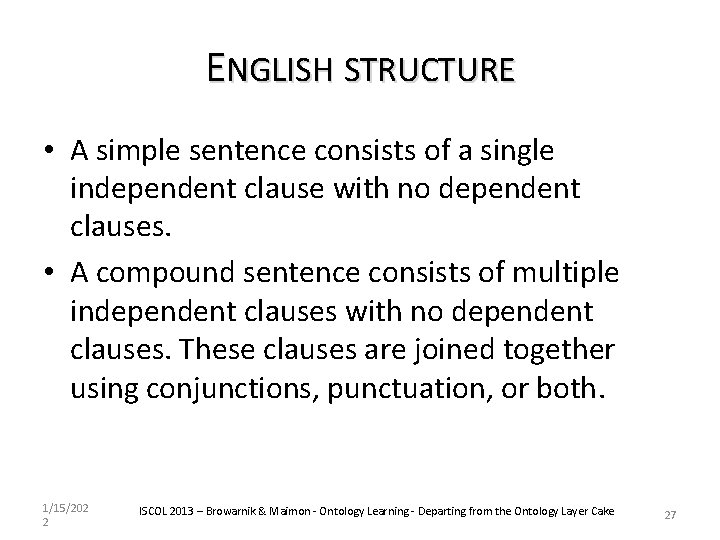
ENGLISH STRUCTURE • A simple sentence consists of a single independent clause with no dependent clauses. • A compound sentence consists of multiple independent clauses with no dependent clauses. These clauses are joined together using conjunctions, punctuation, or both. 1/15/202 2 ISCOL 2013 – Browarnik & Maimon - Ontology Learning - Departing from the Ontology Layer Cake 27
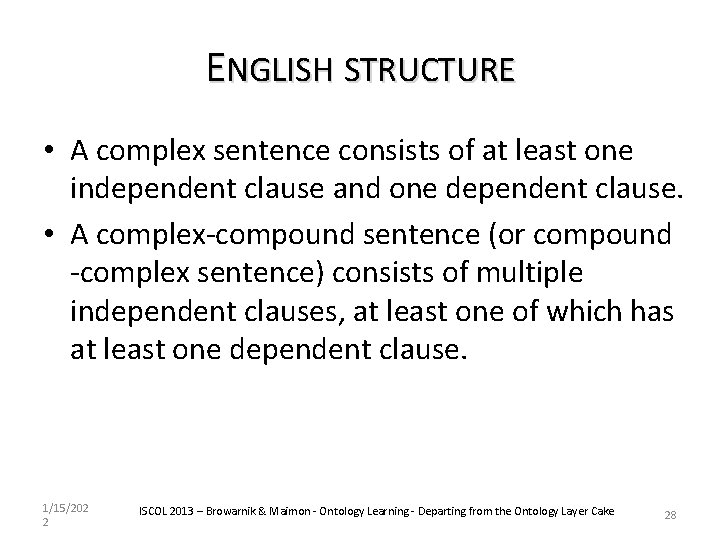
ENGLISH STRUCTURE • A complex sentence consists of at least one independent clause and one dependent clause. • A complex-compound sentence (or compound -complex sentence) consists of multiple independent clauses, at least one of which has at least one dependent clause. 1/15/202 2 ISCOL 2013 – Browarnik & Maimon - Ontology Learning - Departing from the Ontology Layer Cake 28
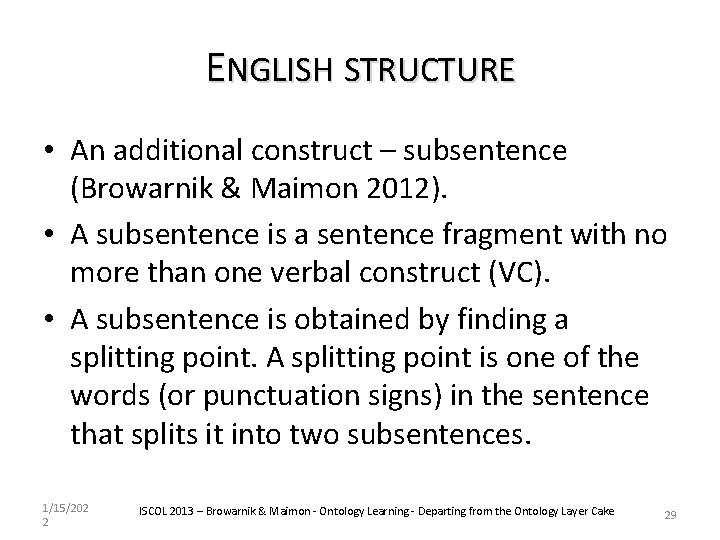
ENGLISH STRUCTURE • An additional construct – subsentence (Browarnik & Maimon 2012). • A subsentence is a sentence fragment with no more than one verbal construct (VC). • A subsentence is obtained by finding a splitting point. A splitting point is one of the words (or punctuation signs) in the sentence that splits it into two subsentences. 1/15/202 2 ISCOL 2013 – Browarnik & Maimon - Ontology Learning - Departing from the Ontology Layer Cake 29
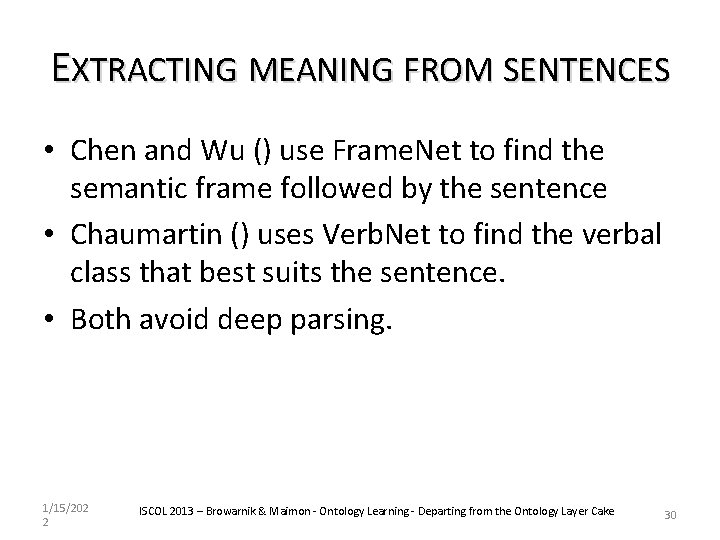
EXTRACTING MEANING FROM SENTENCES • Chen and Wu () use Frame. Net to find the semantic frame followed by the sentence • Chaumartin () uses Verb. Net to find the verbal class that best suits the sentence. • Both avoid deep parsing. 1/15/202 2 ISCOL 2013 – Browarnik & Maimon - Ontology Learning - Departing from the Ontology Layer Cake 30
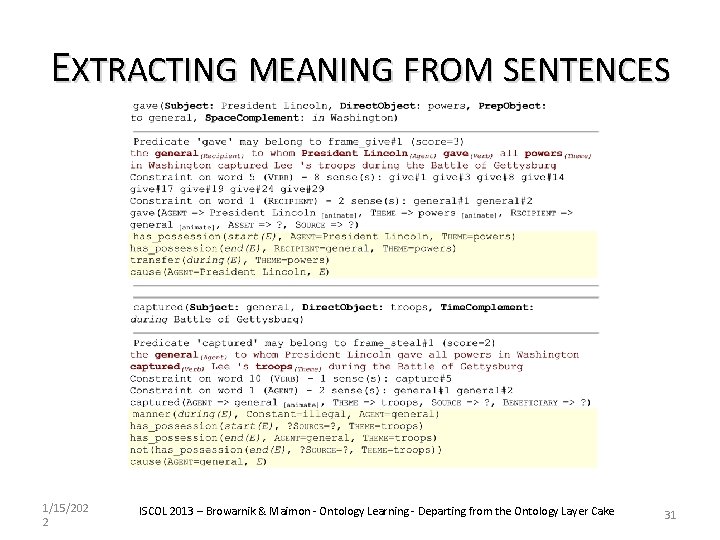
EXTRACTING MEANING FROM SENTENCES 1/15/202 2 ISCOL 2013 – Browarnik & Maimon - Ontology Learning - Departing from the Ontology Layer Cake 31
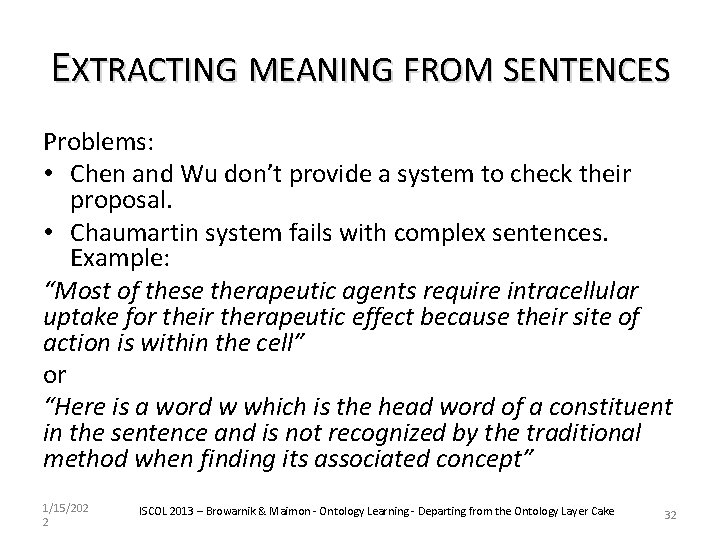
EXTRACTING MEANING FROM SENTENCES Problems: • Chen and Wu don’t provide a system to check their proposal. • Chaumartin system fails with complex sentences. Example: “Most of these therapeutic agents require intracellular uptake for their therapeutic effect because their site of action is within the cell” or “Here is a word w which is the head word of a constituent in the sentence and is not recognized by the traditional method when finding its associated concept” 1/15/202 2 ISCOL 2013 – Browarnik & Maimon - Ontology Learning - Departing from the Ontology Layer Cake 32
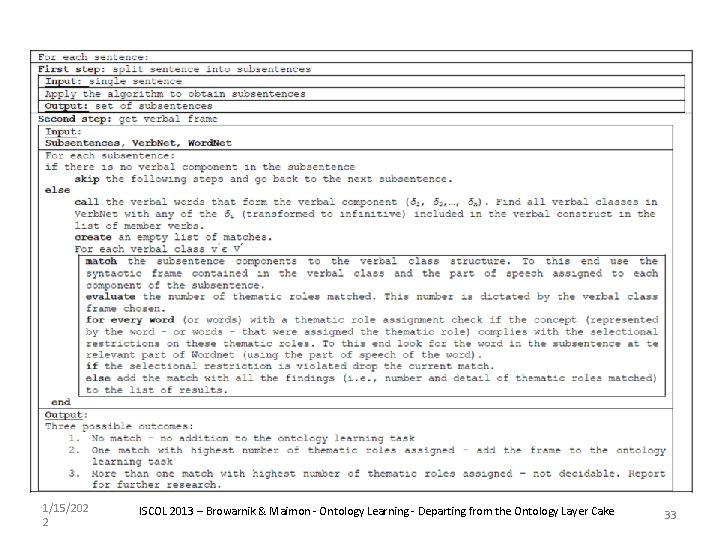
THE PROPOSED OL FRAMEWORK 1/15/202 2 ISCOL 2013 – Browarnik & Maimon - Ontology Learning - Departing from the Ontology Layer Cake 33
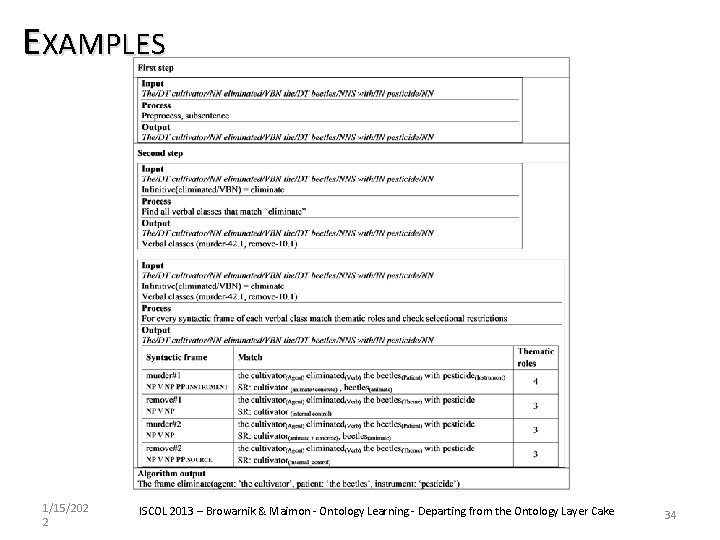
EXAMPLES 1/15/202 2 ISCOL 2013 – Browarnik & Maimon - Ontology Learning - Departing from the Ontology Layer Cake 34
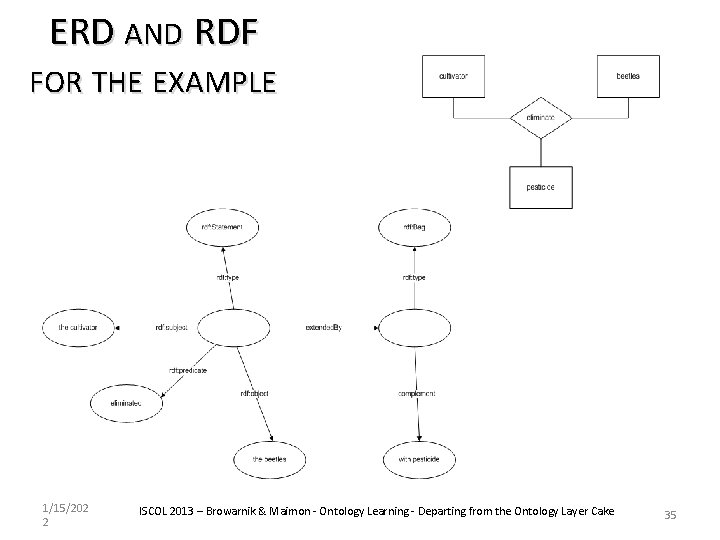
ERD AND RDF FOR THE EXAMPLE 1/15/202 2 ISCOL 2013 – Browarnik & Maimon - Ontology Learning - Departing from the Ontology Layer Cake 35
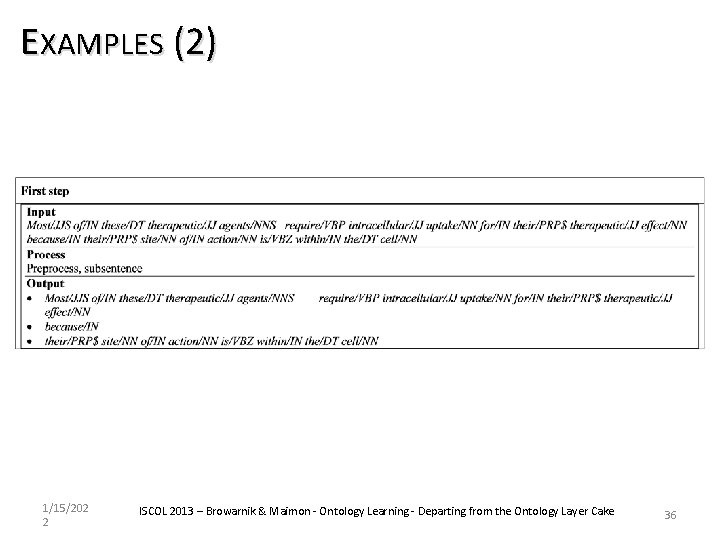
EXAMPLES (2) 1/15/202 2 ISCOL 2013 – Browarnik & Maimon - Ontology Learning - Departing from the Ontology Layer Cake 36
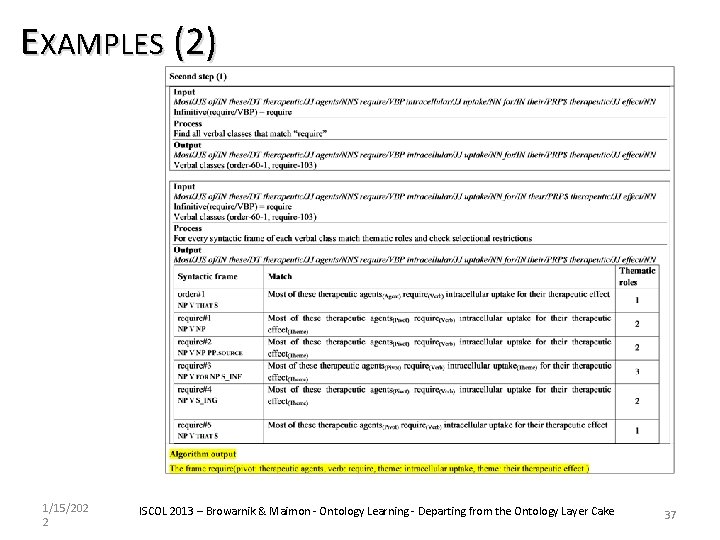
EXAMPLES (2) 1/15/202 2 ISCOL 2013 – Browarnik & Maimon - Ontology Learning - Departing from the Ontology Layer Cake 37
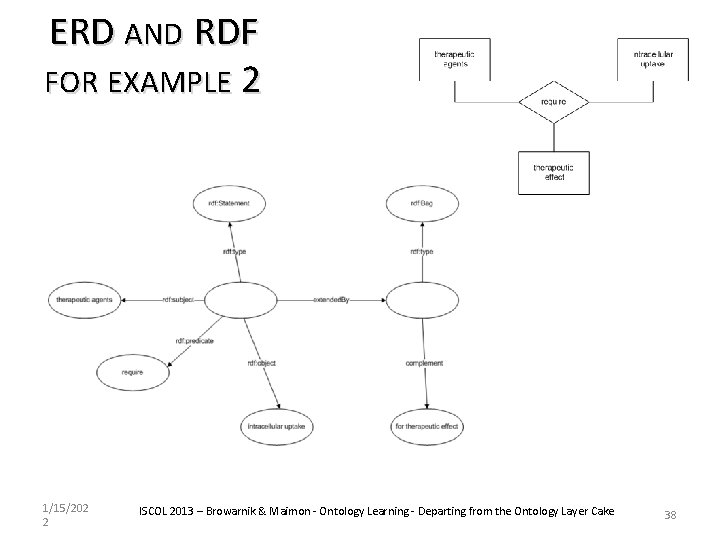
ERD AND RDF FOR EXAMPLE 2 1/15/202 2 ISCOL 2013 – Browarnik & Maimon - Ontology Learning - Departing from the Ontology Layer Cake 38
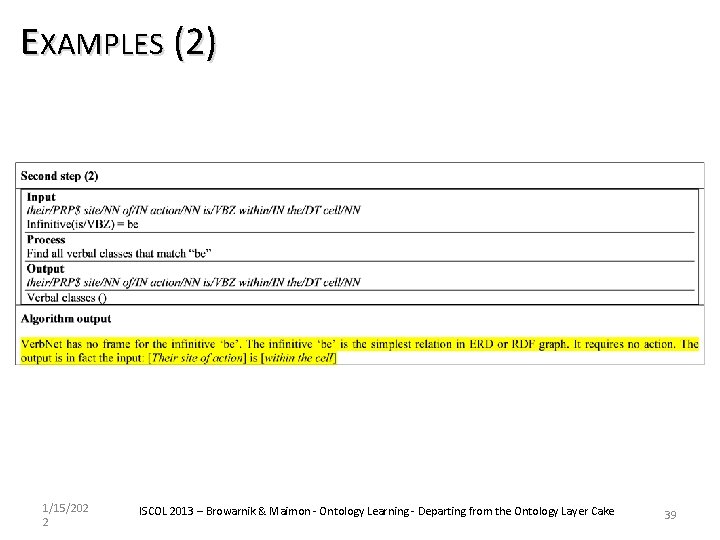
EXAMPLES (2) 1/15/202 2 ISCOL 2013 – Browarnik & Maimon - Ontology Learning - Departing from the Ontology Layer Cake 39
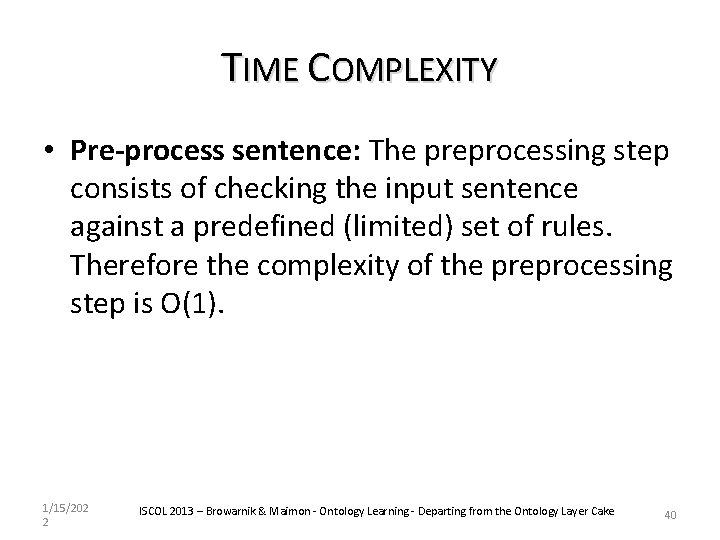
TIME COMPLEXITY • Pre-process sentence: The preprocessing step consists of checking the input sentence against a predefined (limited) set of rules. Therefore the complexity of the preprocessing step is O(1). 1/15/202 2 ISCOL 2013 – Browarnik & Maimon - Ontology Learning - Departing from the Ontology Layer Cake 40
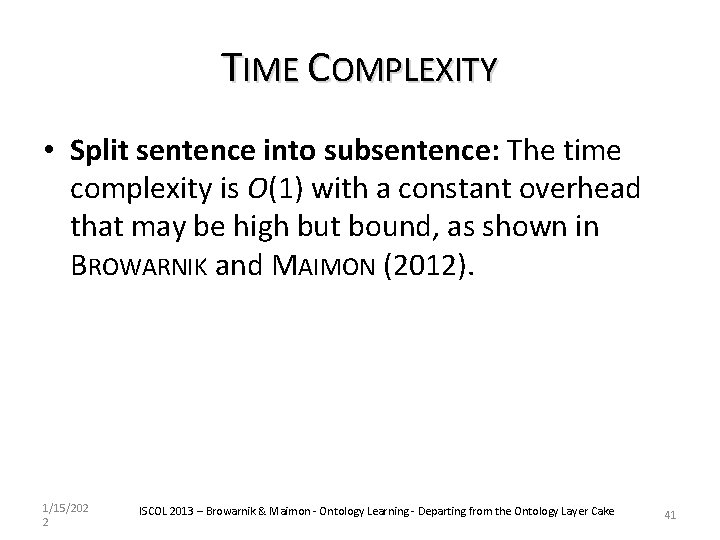
TIME COMPLEXITY • Split sentence into subsentence: The time complexity is O(1) with a constant overhead that may be high but bound, as shown in BROWARNIK and MAIMON (2012). 1/15/202 2 ISCOL 2013 – Browarnik & Maimon - Ontology Learning - Departing from the Ontology Layer Cake 41
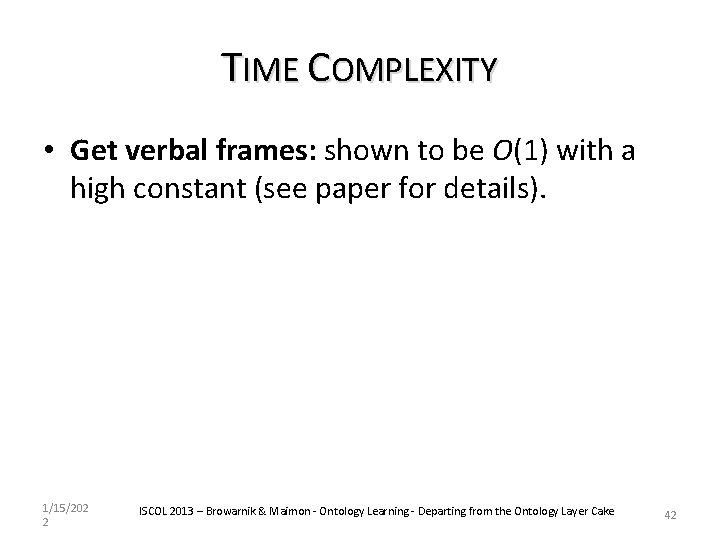
TIME COMPLEXITY • Get verbal frames: shown to be O(1) with a high constant (see paper for details). 1/15/202 2 ISCOL 2013 – Browarnik & Maimon - Ontology Learning - Departing from the Ontology Layer Cake 42
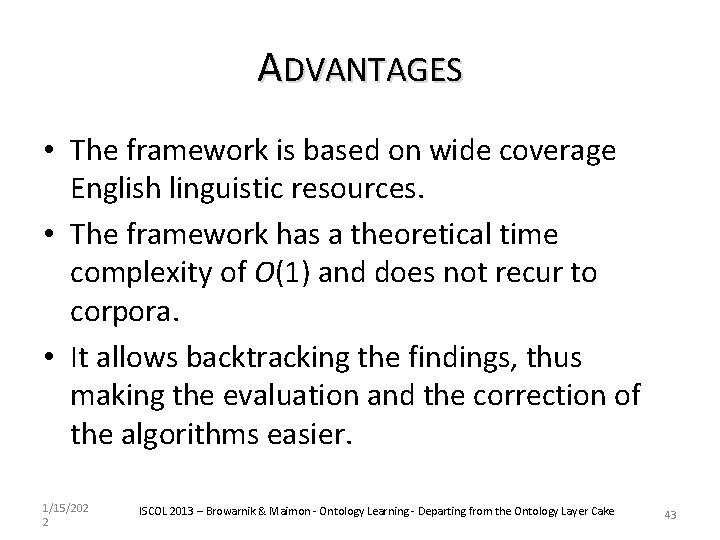
ADVANTAGES • The framework is based on wide coverage English linguistic resources. • The framework has a theoretical time complexity of O(1) and does not recur to corpora. • It allows backtracking the findings, thus making the evaluation and the correction of the algorithms easier. 1/15/202 2 ISCOL 2013 – Browarnik & Maimon - Ontology Learning - Departing from the Ontology Layer Cake 43
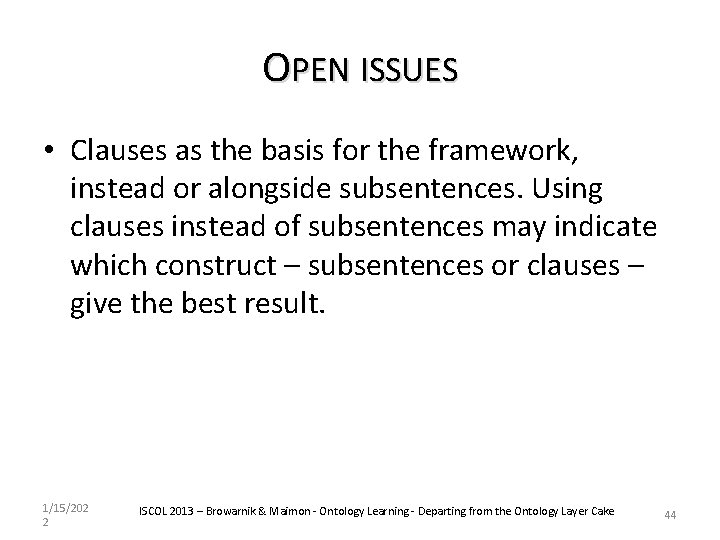
OPEN ISSUES • Clauses as the basis for the framework, instead or alongside subsentences. Using clauses instead of subsentences may indicate which construct – subsentences or clauses – give the best result. 1/15/202 2 ISCOL 2013 – Browarnik & Maimon - Ontology Learning - Departing from the Ontology Layer Cake 44
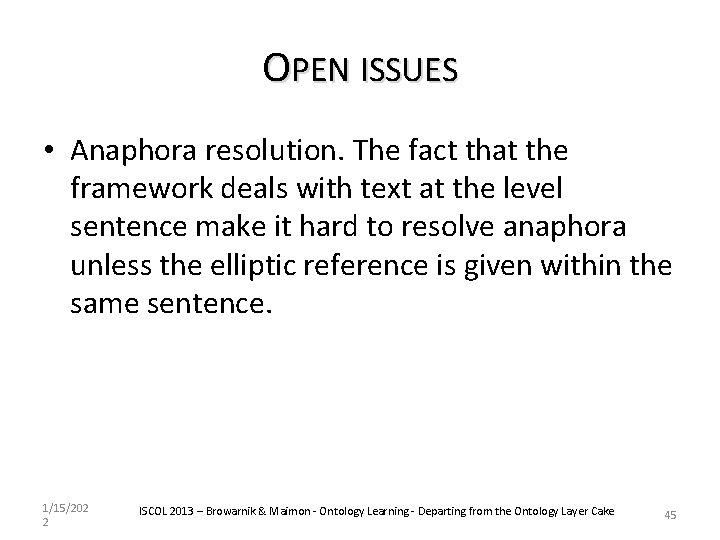
OPEN ISSUES • Anaphora resolution. The fact that the framework deals with text at the level sentence make it hard to resolve anaphora unless the elliptic reference is given within the same sentence. 1/15/202 2 ISCOL 2013 – Browarnik & Maimon - Ontology Learning - Departing from the Ontology Layer Cake 45
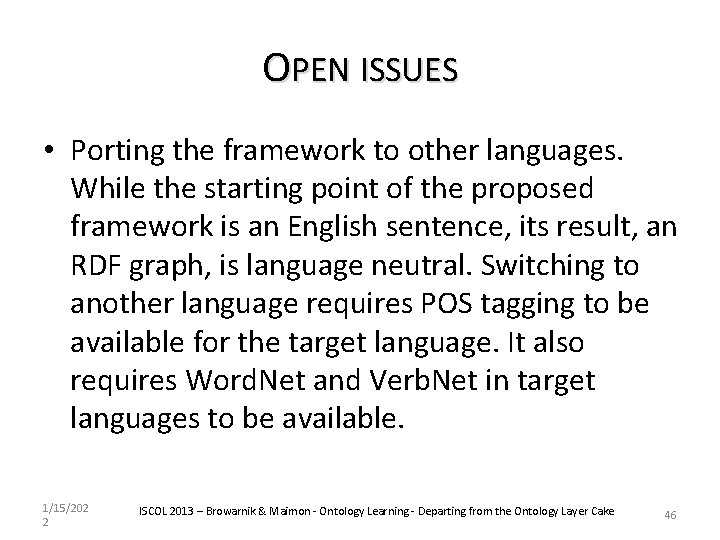
OPEN ISSUES • Porting the framework to other languages. While the starting point of the proposed framework is an English sentence, its result, an RDF graph, is language neutral. Switching to another language requires POS tagging to be available for the target language. It also requires Word. Net and Verb. Net in target languages to be available. 1/15/202 2 ISCOL 2013 – Browarnik & Maimon - Ontology Learning - Departing from the Ontology Layer Cake 46
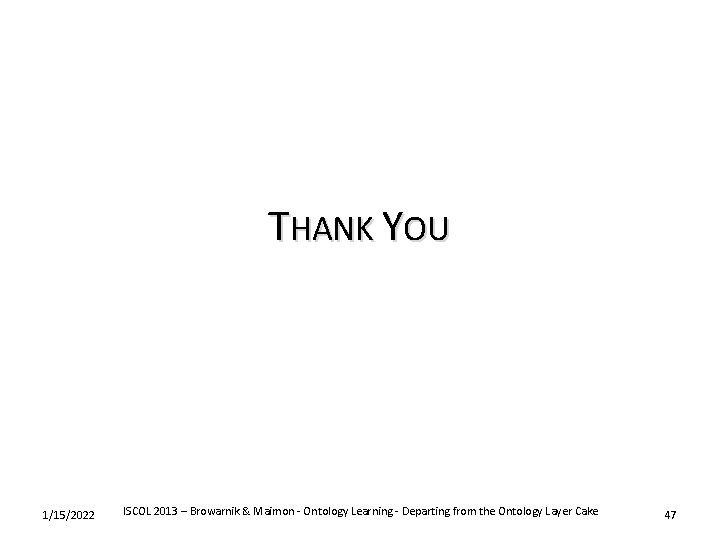
THANK YOU 1/15/2022 ISCOL 2013 – Browarnik & Maimon - Ontology Learning - Departing from the Ontology Layer Cake 47
Shortened cake and unshortened cake
Semantic web layer cake
Semantic web layer cake
Script
Semantic web layer cake
Pigmented layer and neural layer
Parietal layer and visceral layer
Secure socket layer and transport layer security
Layer 6 presentation layer
Secure socket layer and transport layer security
Secure socket layer and transport layer security
Secure socket layer and transport layer security
Layer 2 e layer 3
Layer-by-layer assembly
Layer 2 vs layer 3 bitstream
Cuadro comparativo e-learning y b-learning
Hình ảnh bộ gõ cơ thể búng tay
Frameset trong html5
Bổ thể
Tỉ lệ cơ thể trẻ em
Voi kéo gỗ như thế nào
Tư thế worm breton là gì
Chúa sống lại
Kể tên các môn thể thao
Thế nào là hệ số cao nhất
Các châu lục và đại dương trên thế giới
Công của trọng lực
Trời xanh đây là của chúng ta thể thơ
Mật thư anh em như thể tay chân
Phép trừ bù
độ dài liên kết
Các châu lục và đại dương trên thế giới
Thể thơ truyền thống
Quá trình desamine hóa có thể tạo ra
Một số thể thơ truyền thống
Cái miệng xinh xinh thế chỉ nói điều hay thôi
Vẽ hình chiếu vuông góc của vật thể sau
Thế nào là sự mỏi cơ
đặc điểm cơ thể của người tối cổ
Thế nào là giọng cùng tên?
Vẽ hình chiếu đứng bằng cạnh của vật thể
Tia chieu sa te
Thẻ vin
đại từ thay thế
điện thế nghỉ
Tư thế ngồi viết
Diễn thế sinh thái là
Các loại đột biến cấu trúc nhiễm sắc thể