Sampling Design Small Samples Can Enlighten The proof
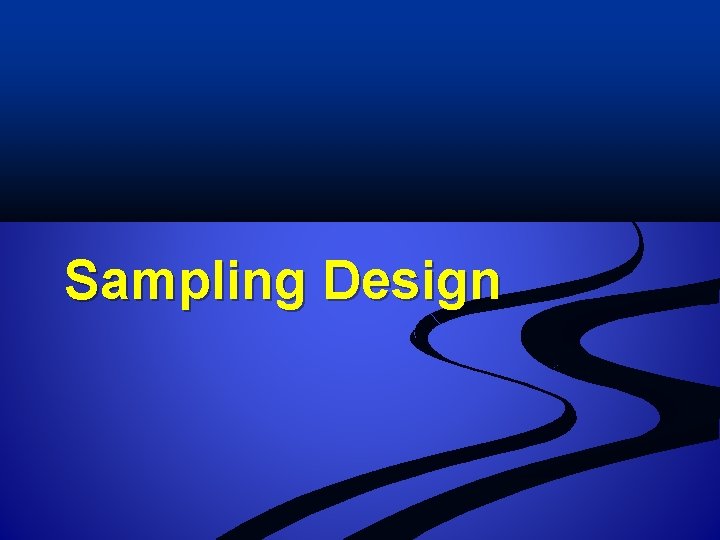
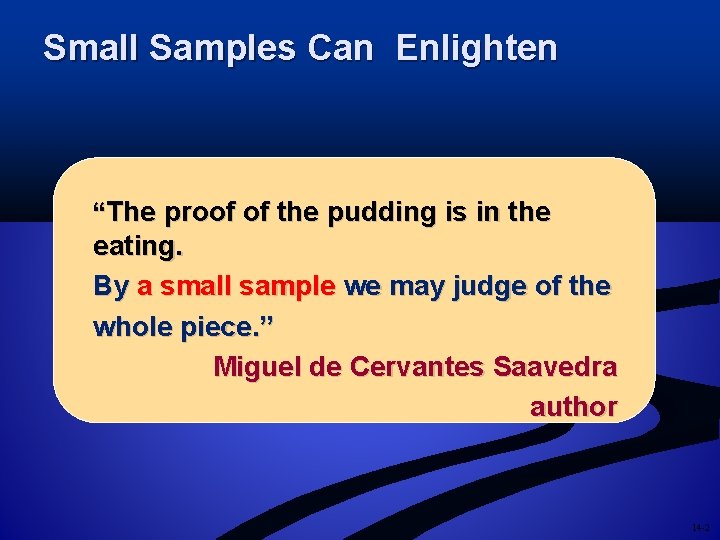
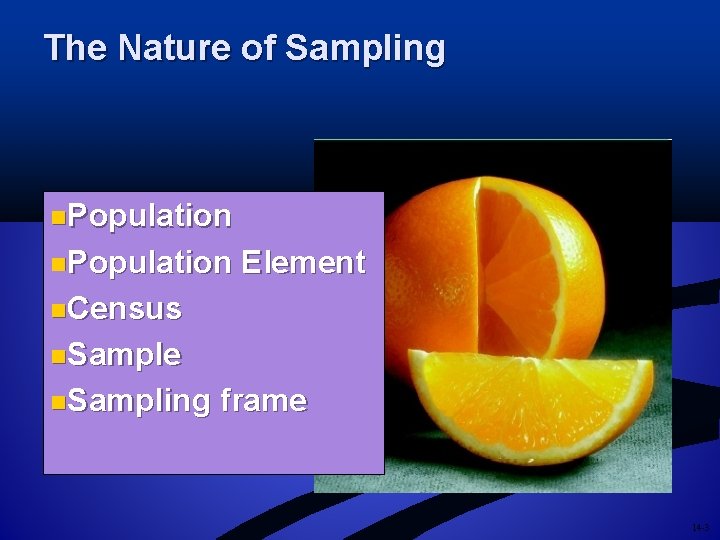
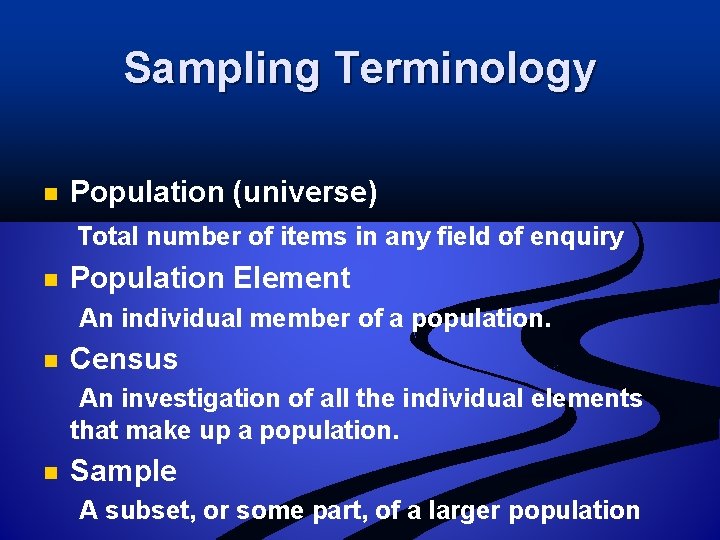
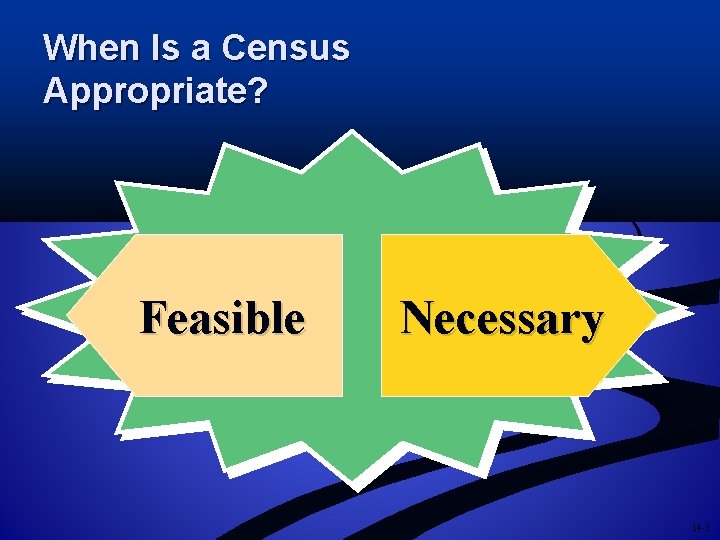
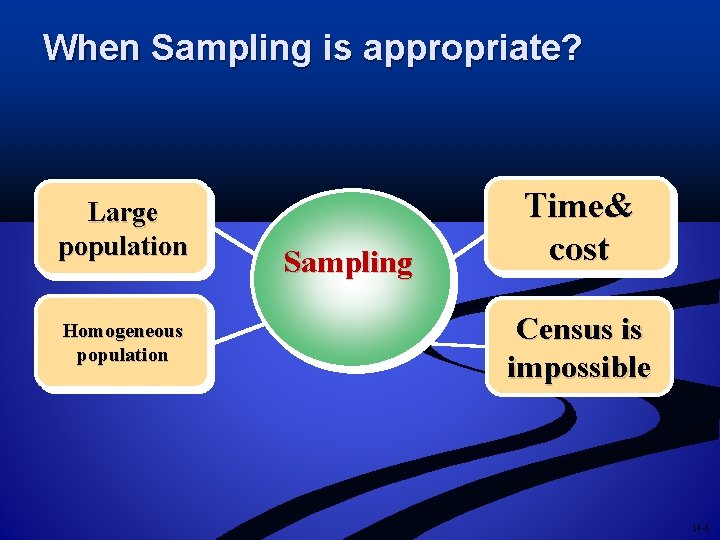
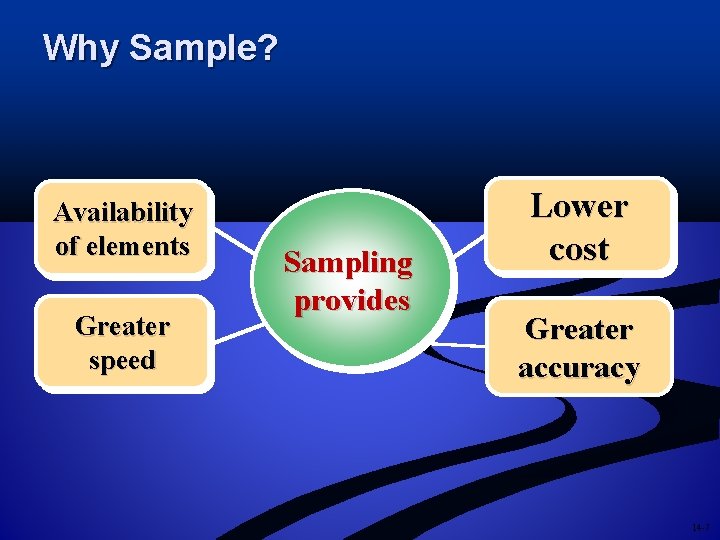
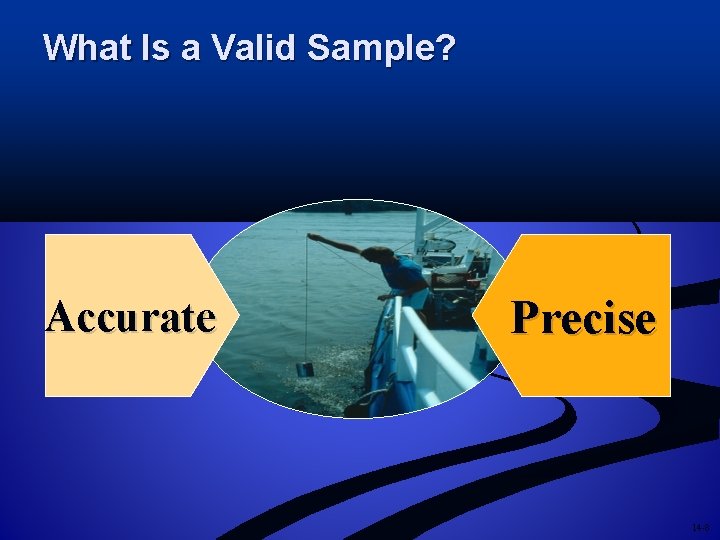
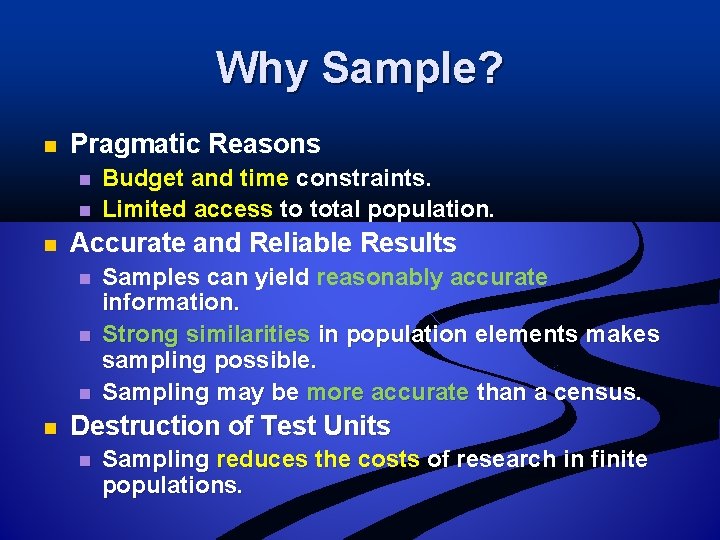
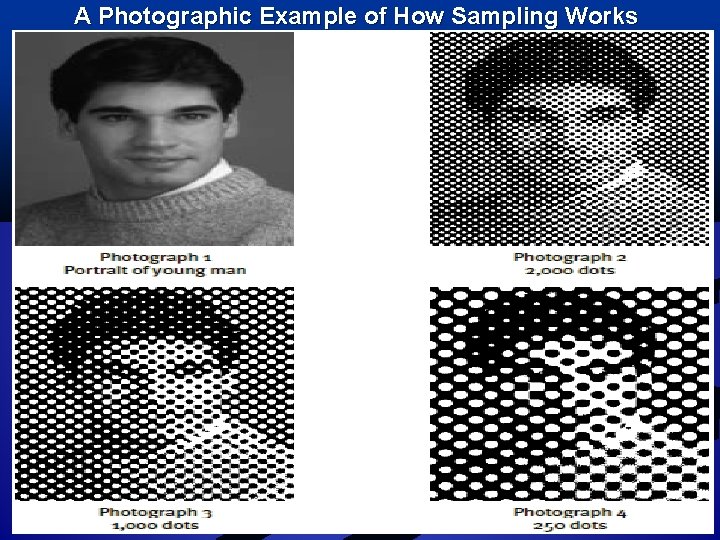
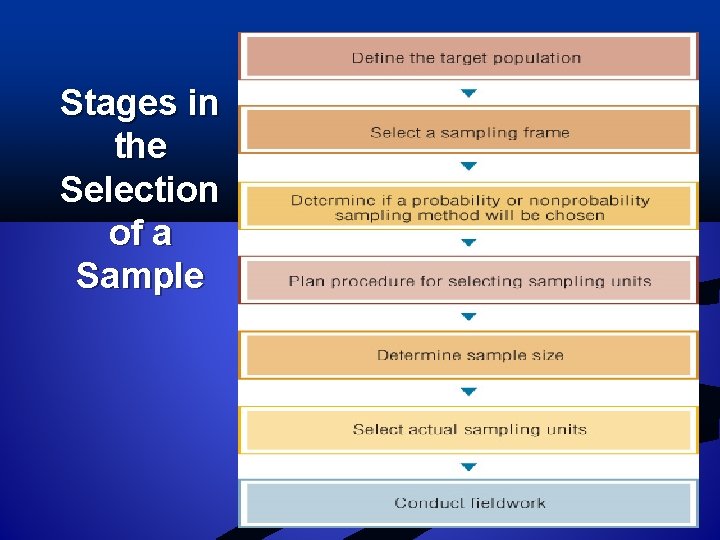
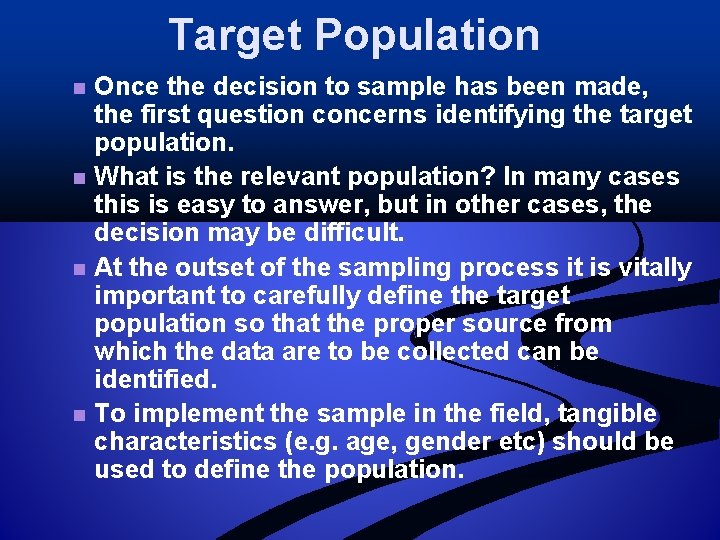
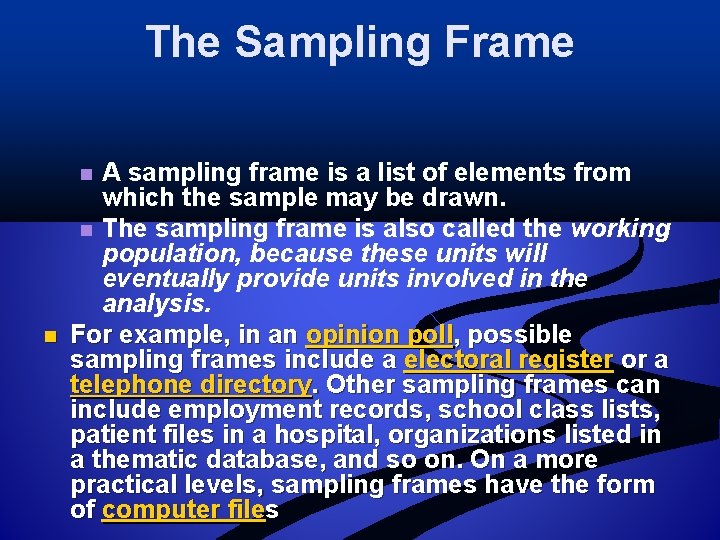
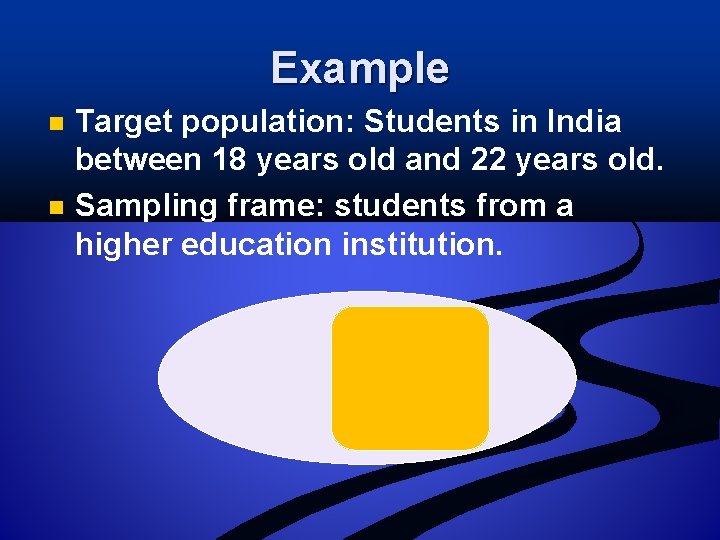
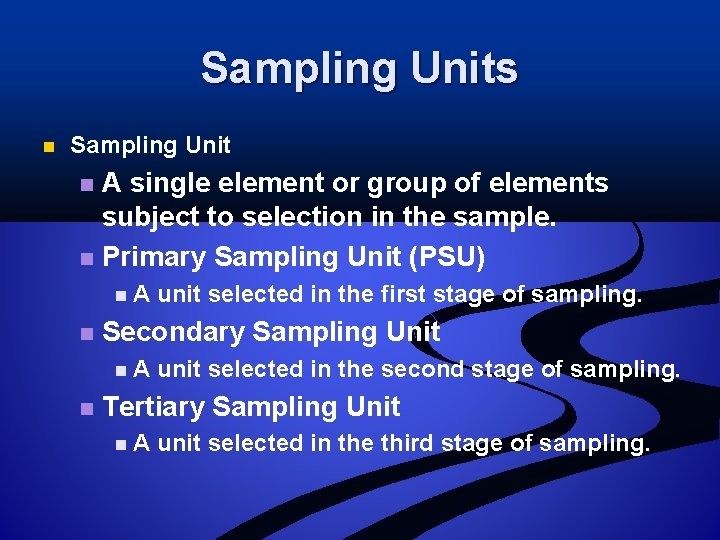
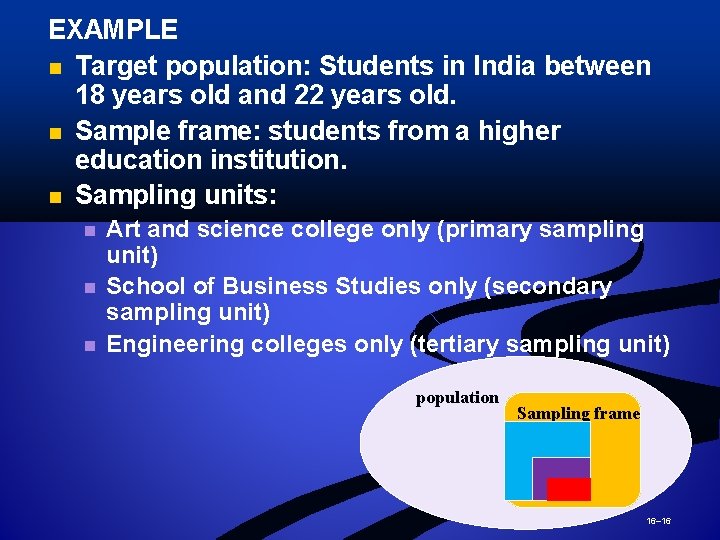
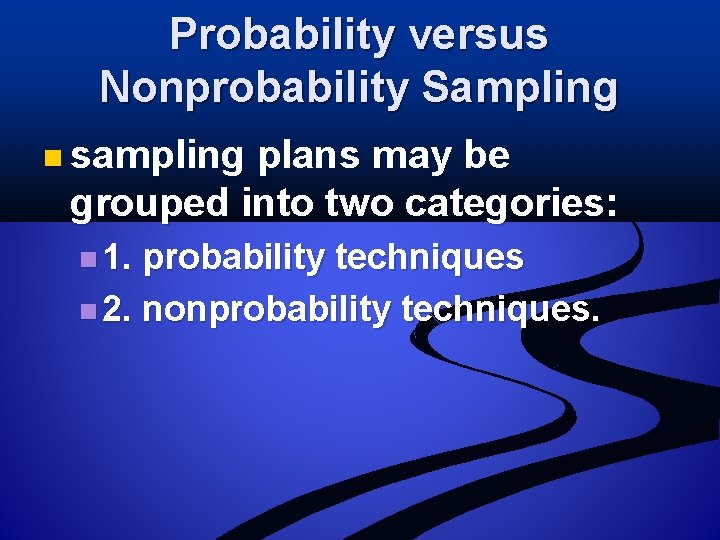
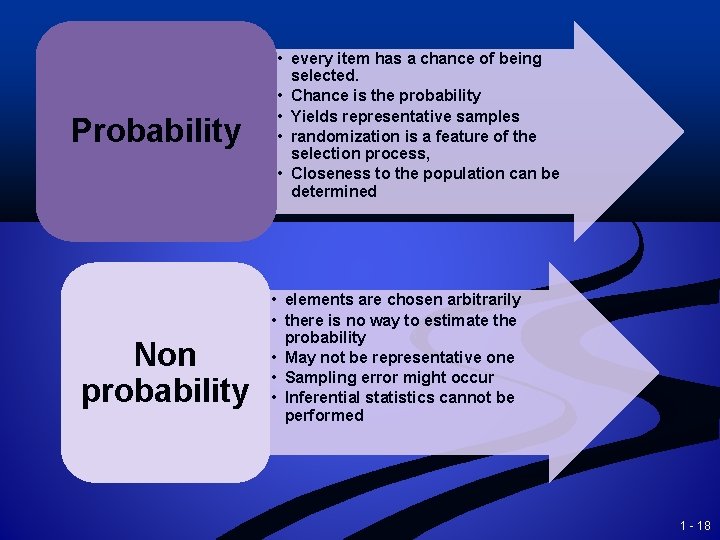
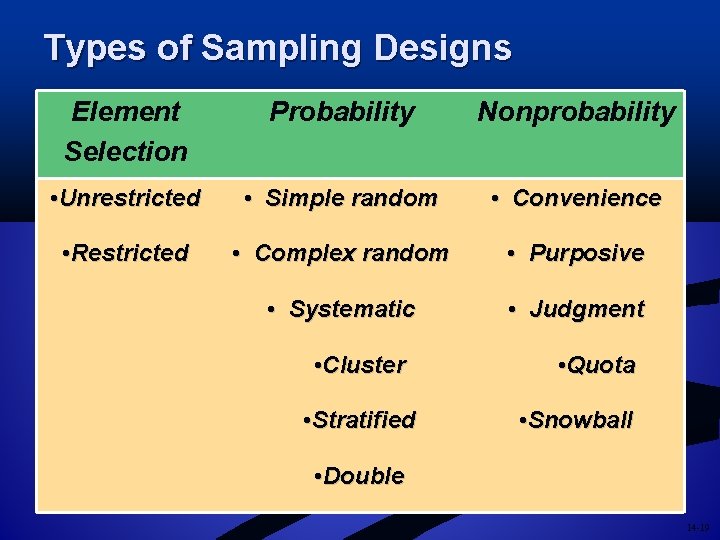
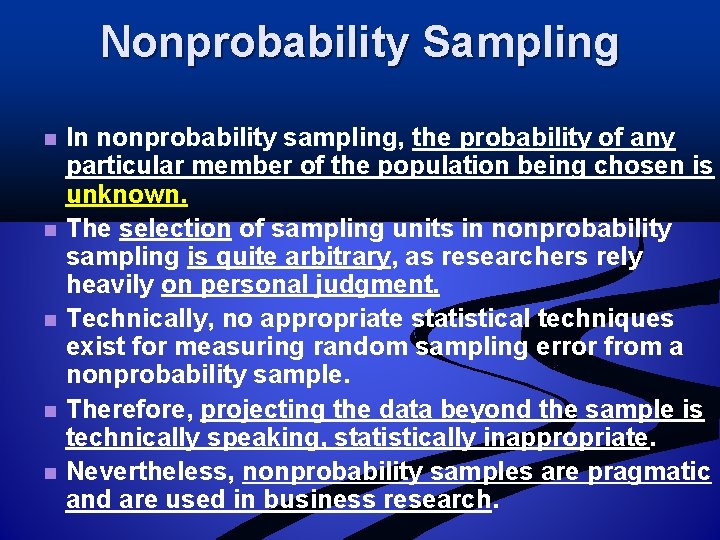
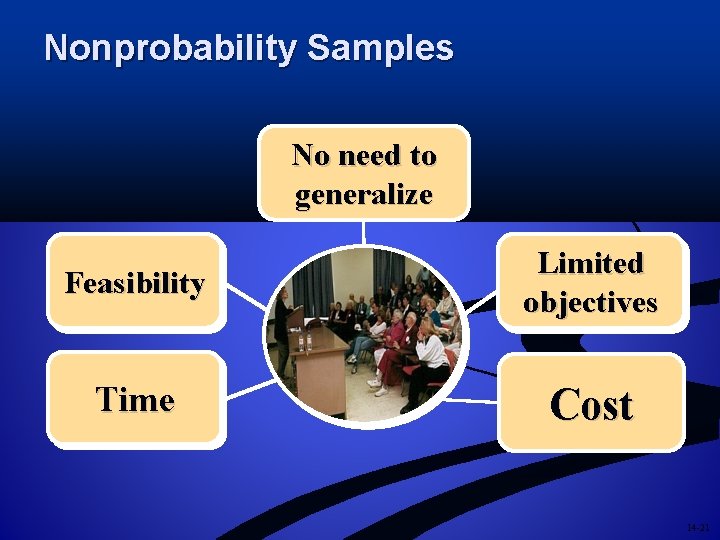
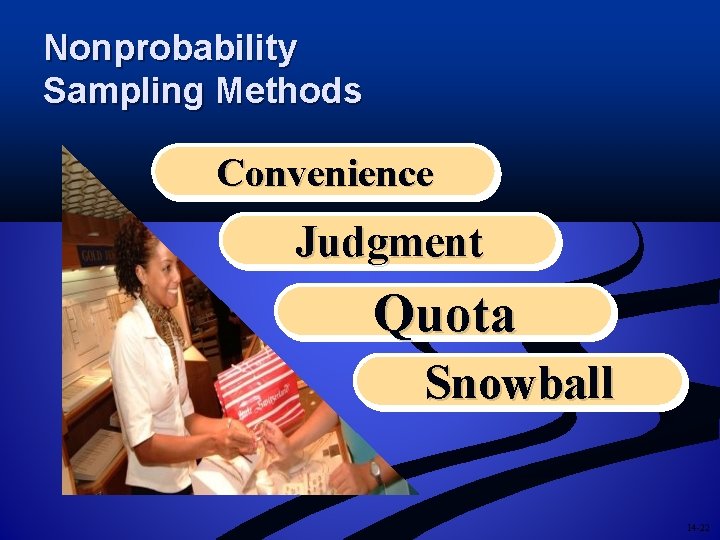
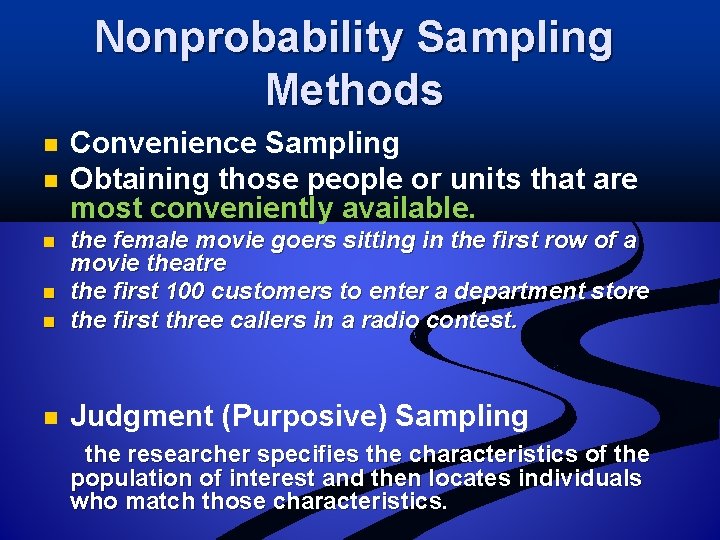
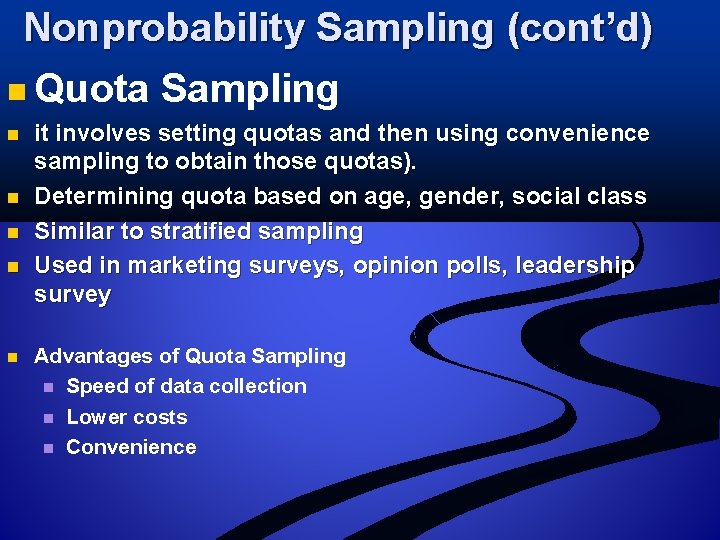
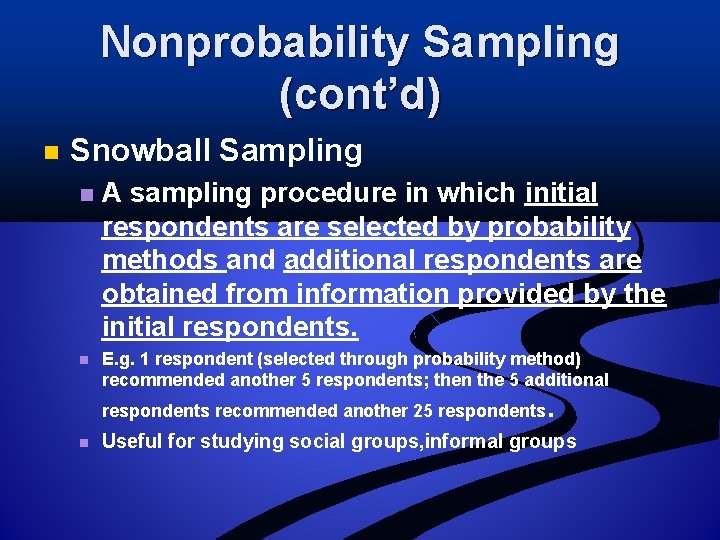
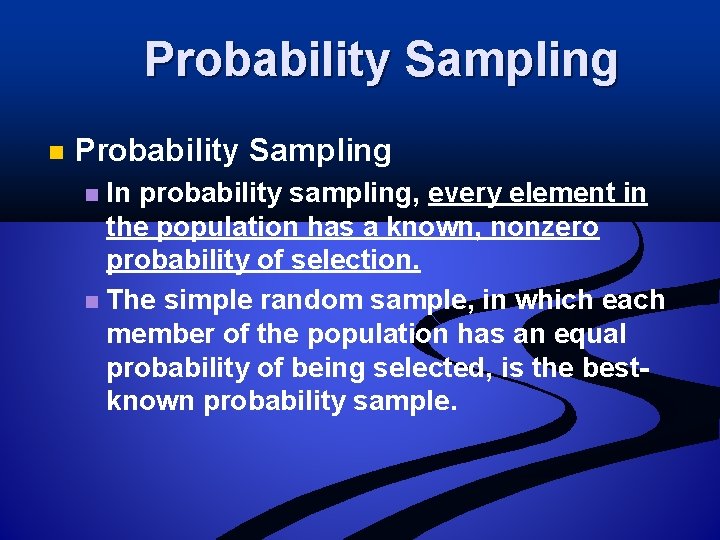
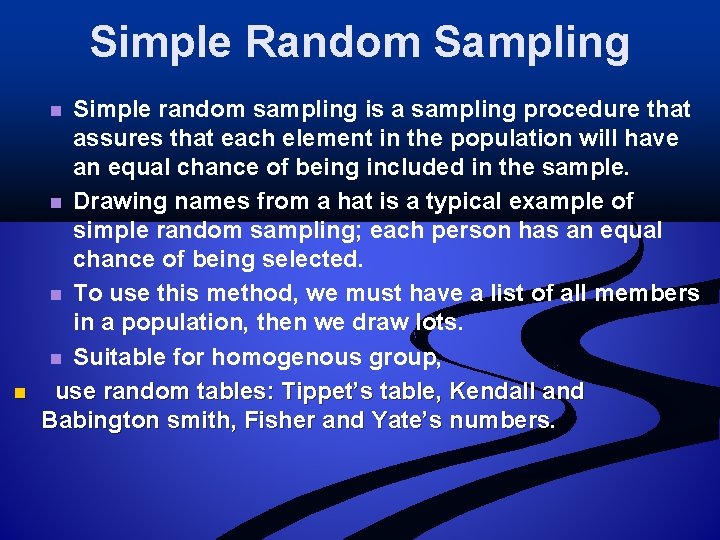
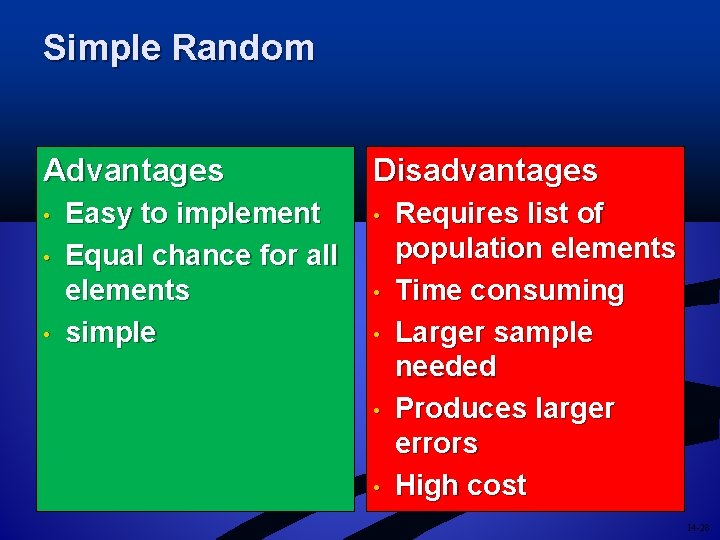
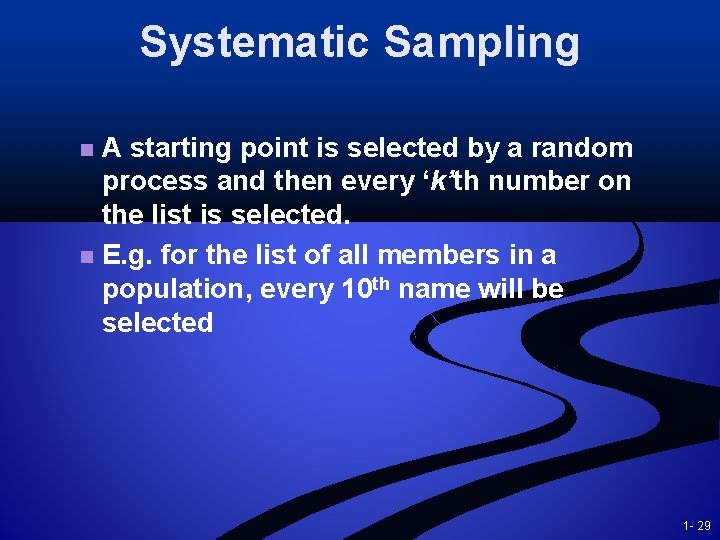
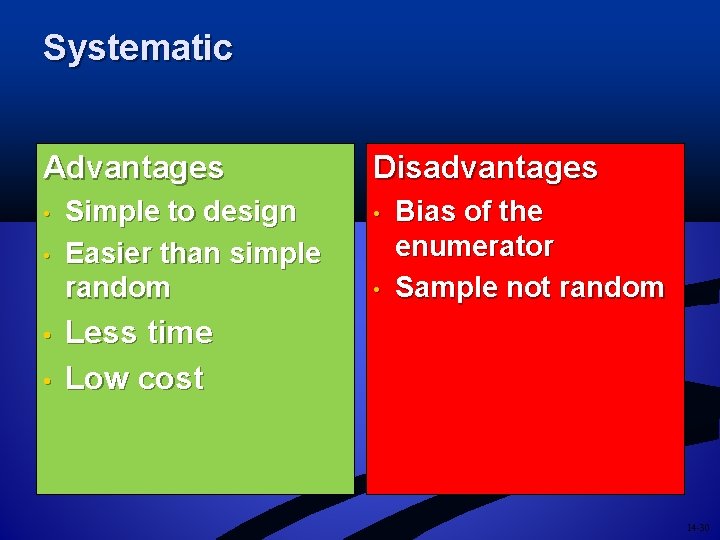
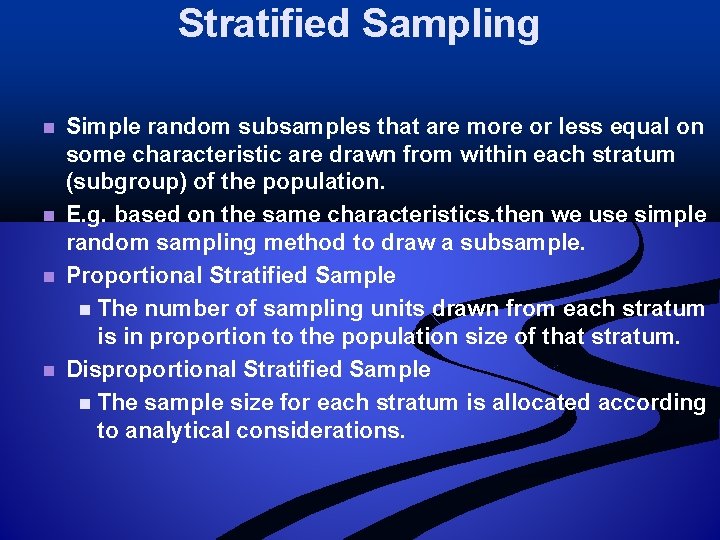
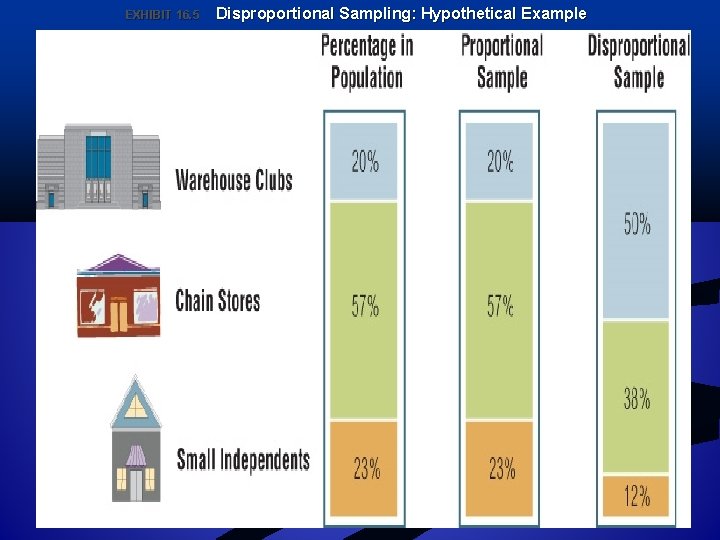
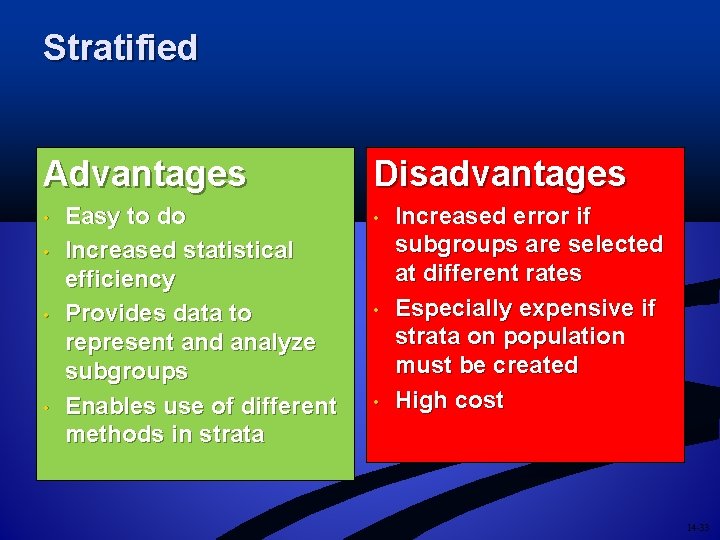
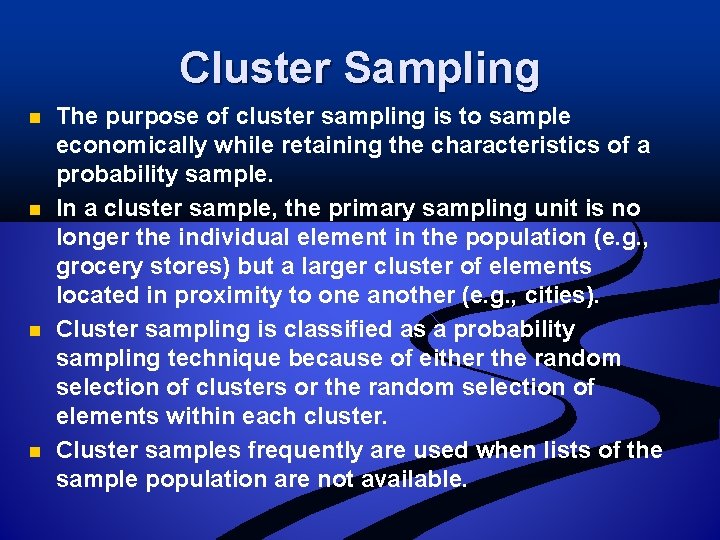
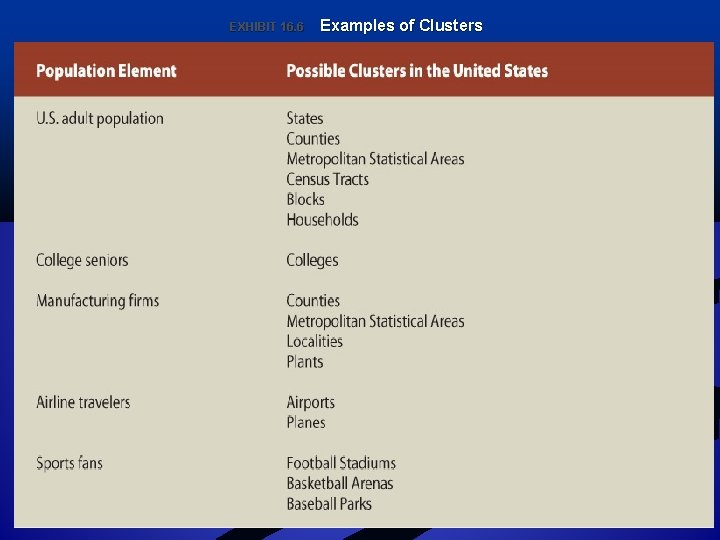
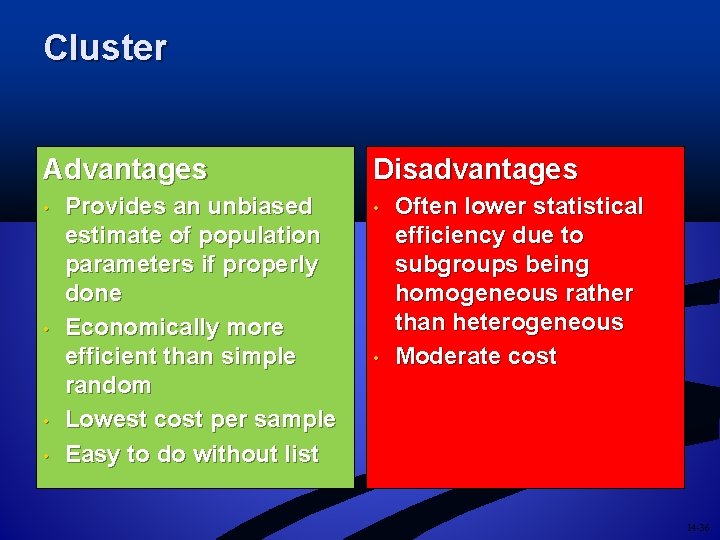
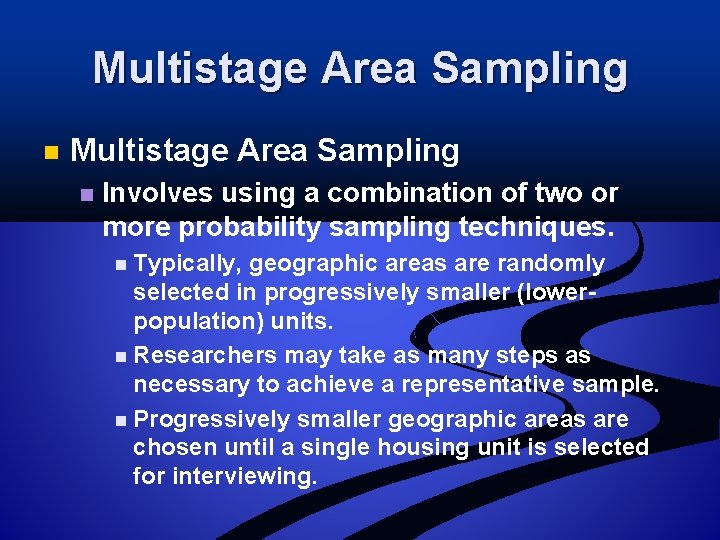
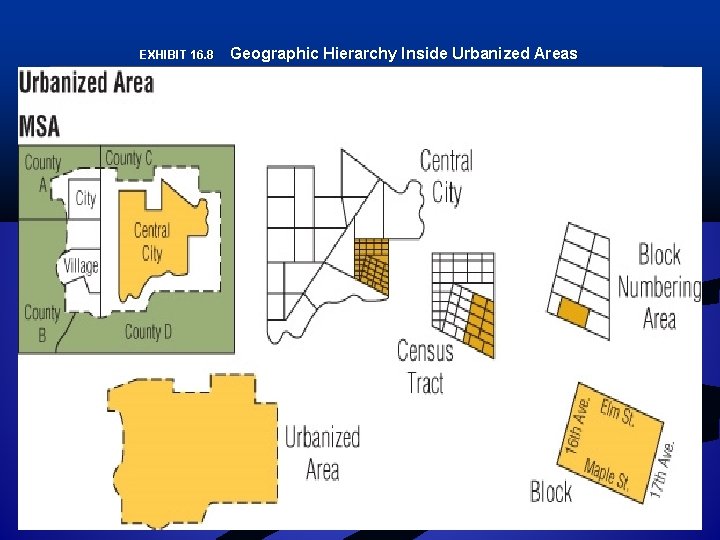
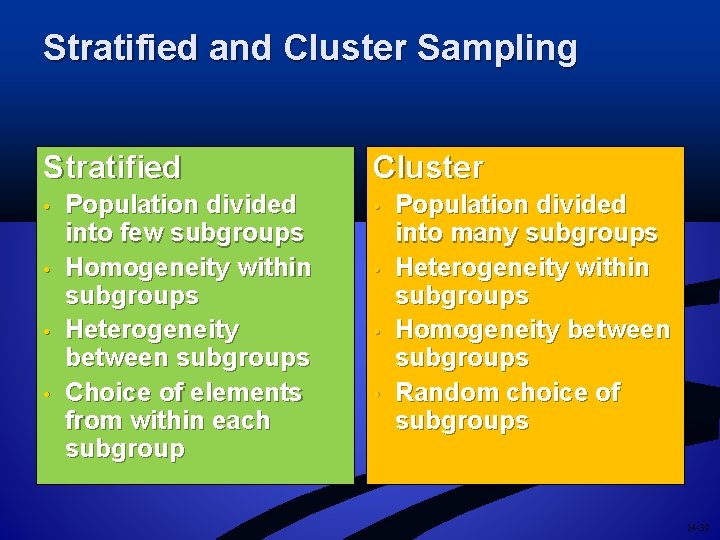
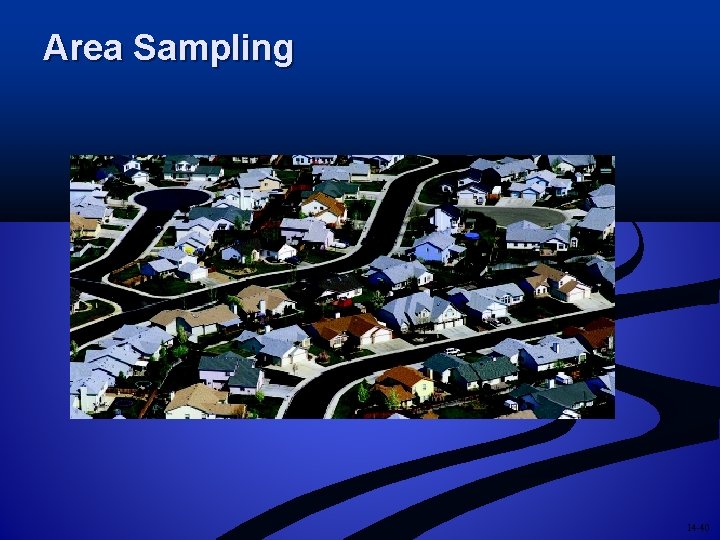
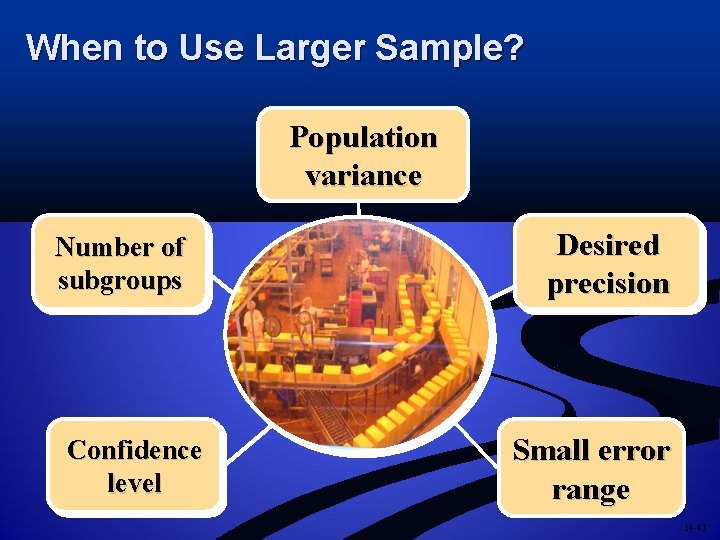
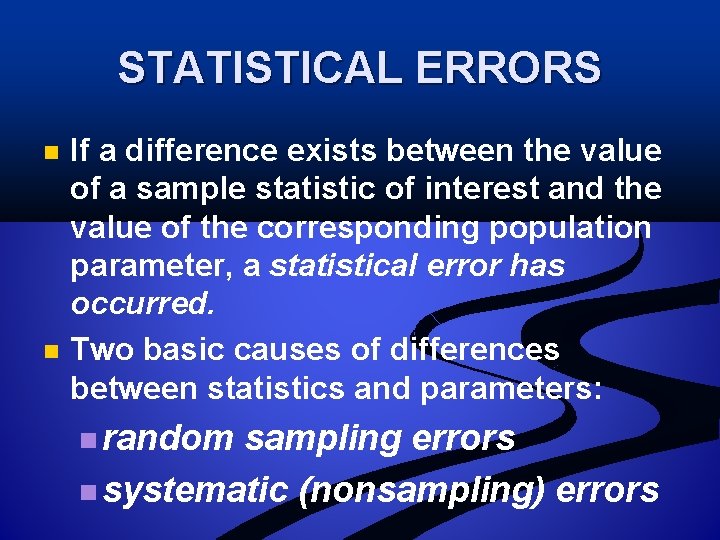
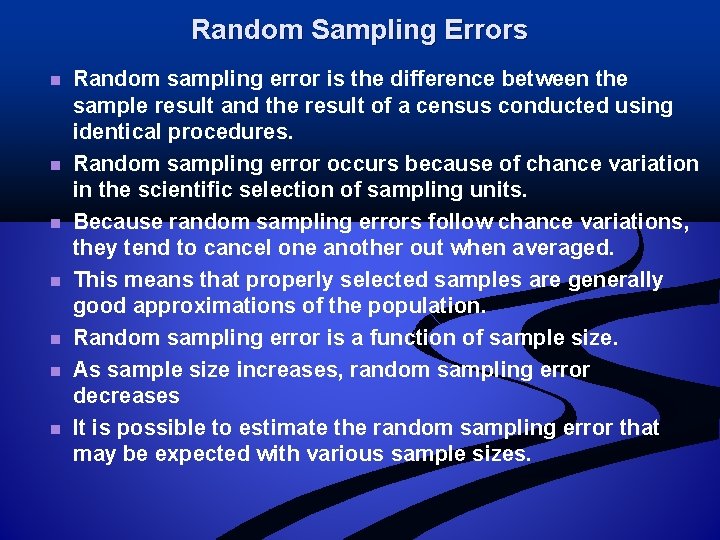
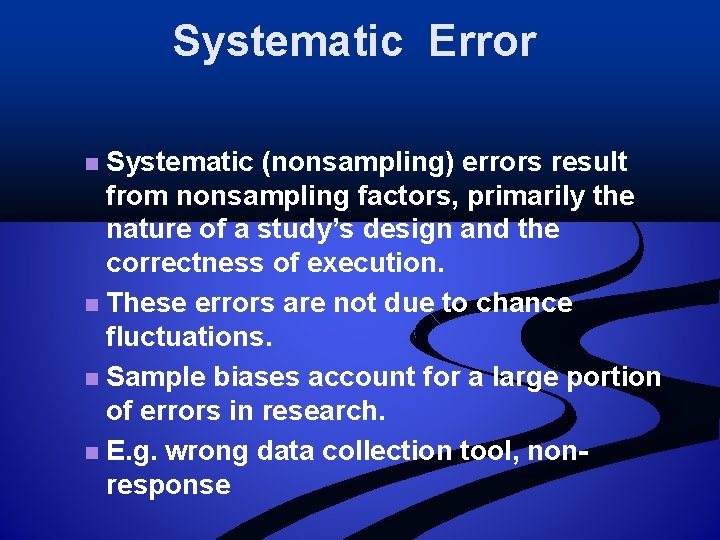
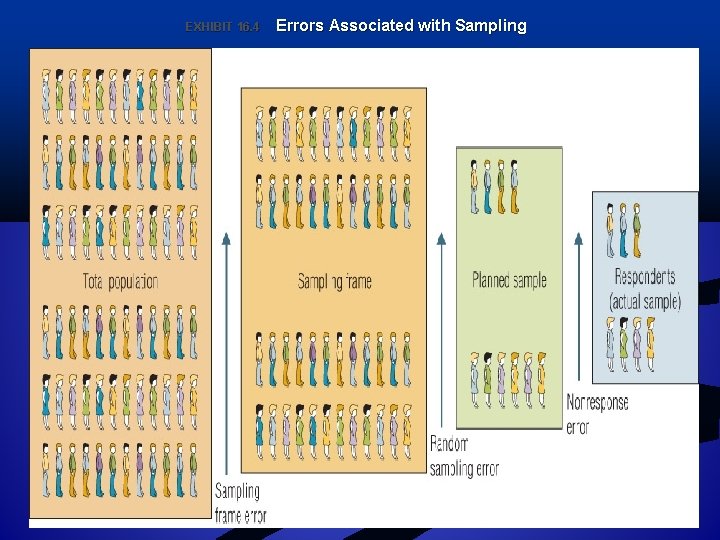
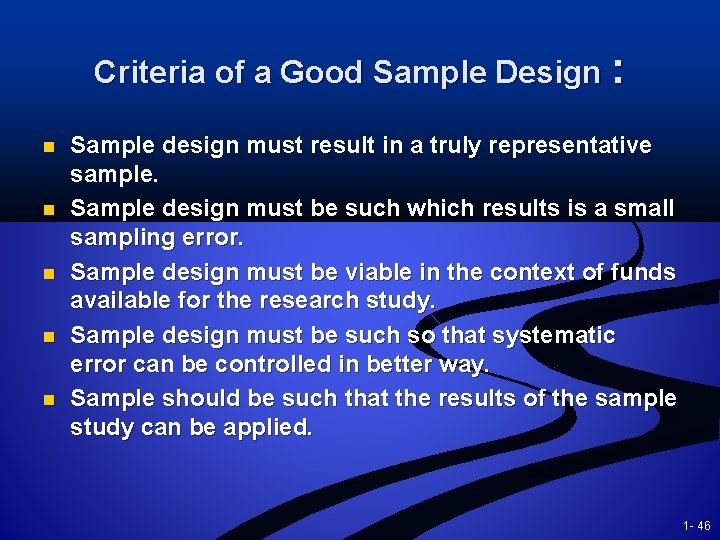
- Slides: 46
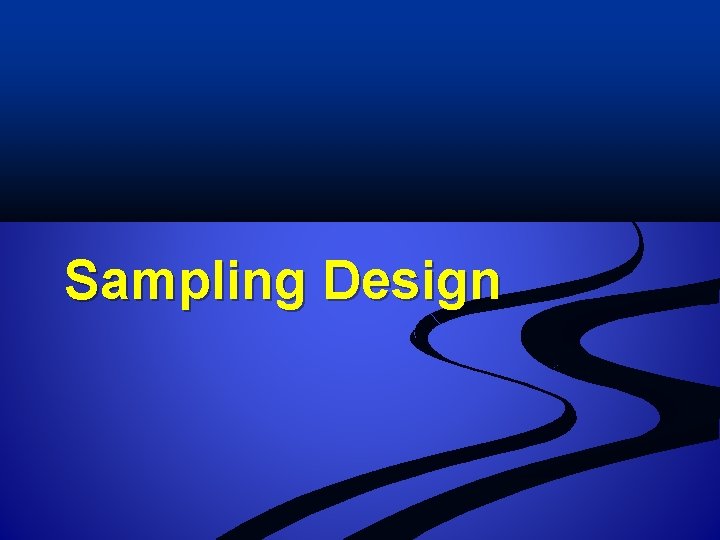
Sampling Design
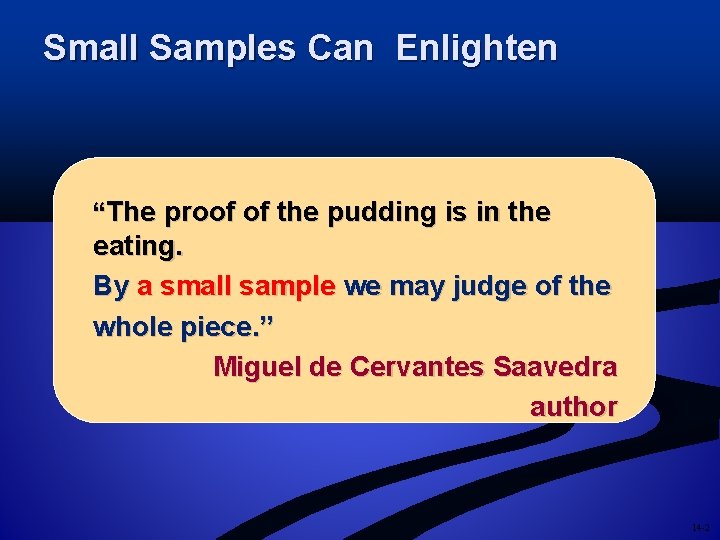
Small Samples Can Enlighten “The proof of the pudding is in the eating. By a small sample we may judge of the whole piece. ” Miguel de Cervantes Saavedra author 14 -2
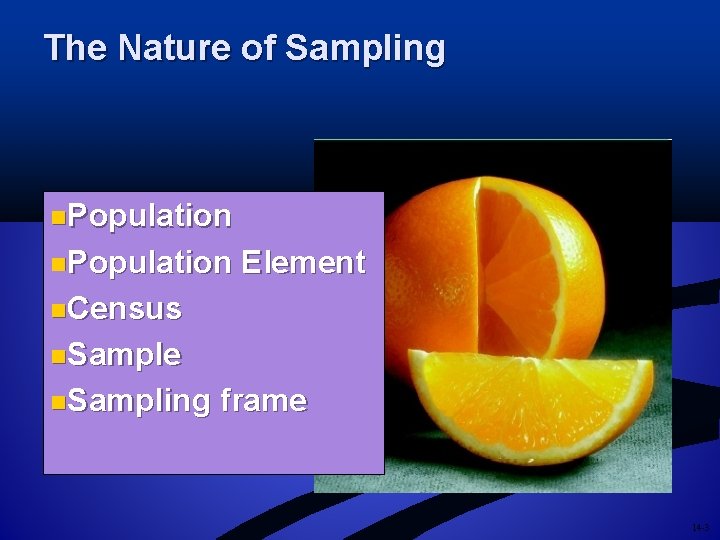
The Nature of Sampling n. Population Element n. Census n. Sample n. Sampling frame 14 -3
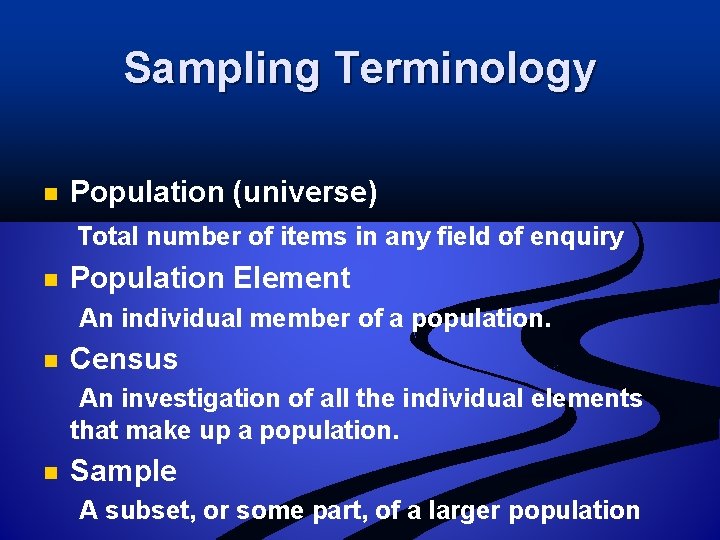
Sampling Terminology n Population (universe) Total number of items in any field of enquiry n Population Element An individual member of a population. n Census An investigation of all the individual elements that make up a population. n Sample A subset, or some part, of a larger population
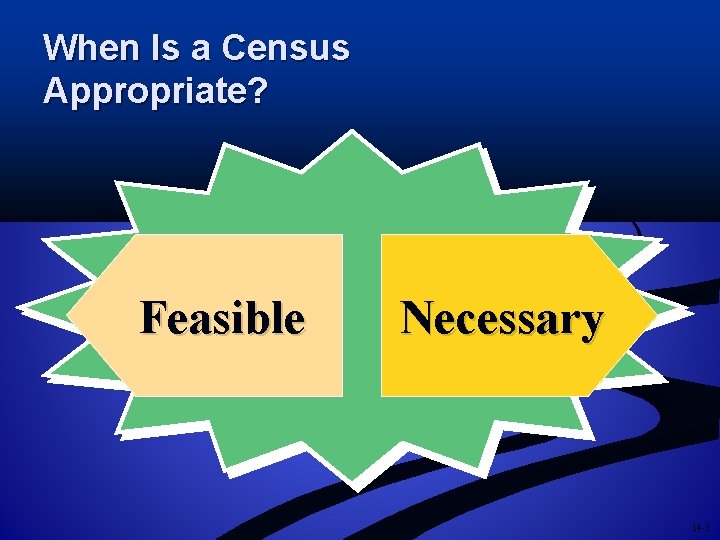
When Is a Census Appropriate? Feasible Necessary 14 -5
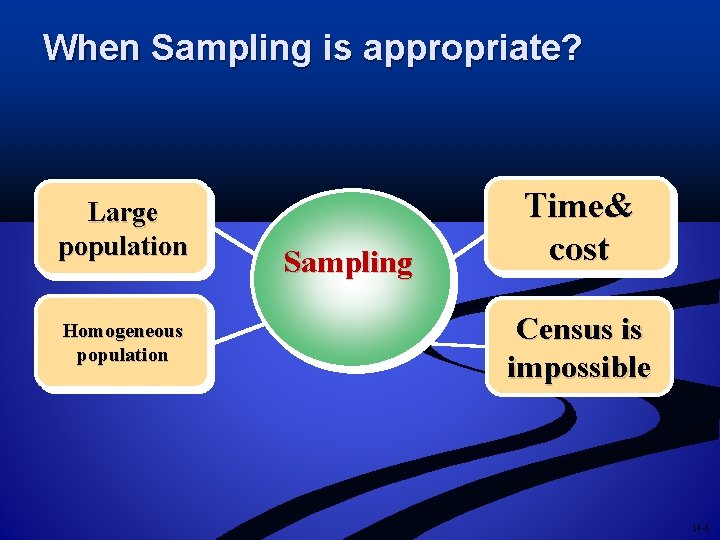
When Sampling is appropriate? Large population Homogeneous population Sampling Time& cost Census is impossible 14 -6
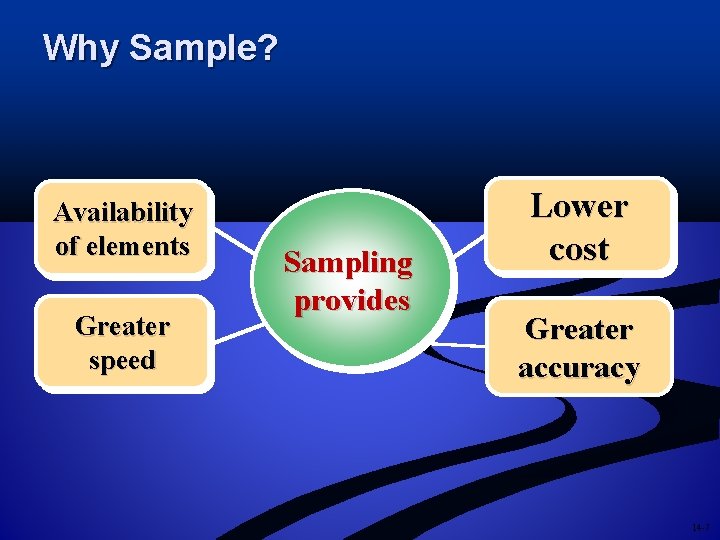
Why Sample? Availability of elements Greater speed Sampling provides Lower cost Greater accuracy 14 -7
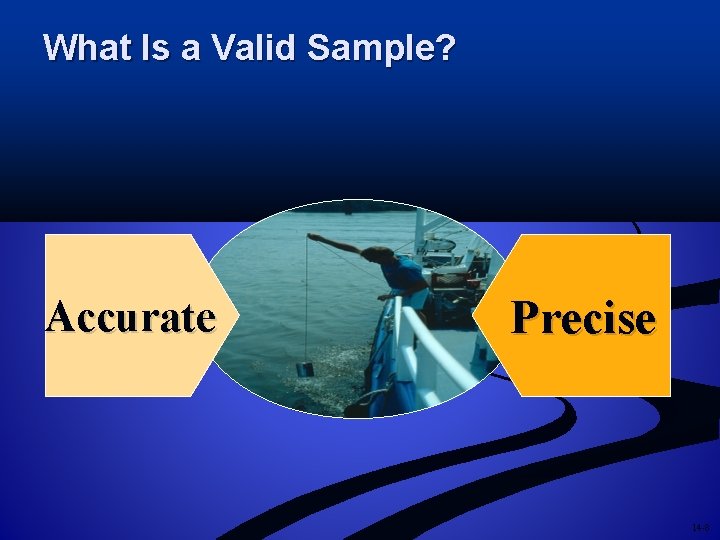
What Is a Valid Sample? Accurate Precise 14 -8
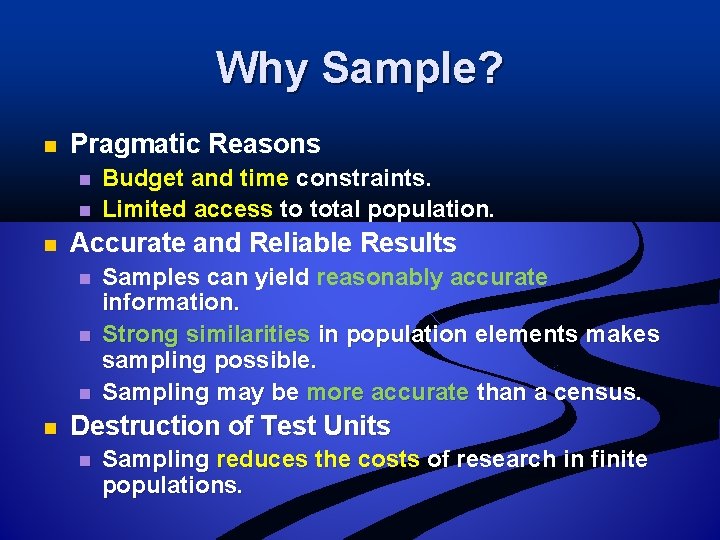
Why Sample? n Pragmatic Reasons n n n Accurate and Reliable Results n n Budget and time constraints. Limited access to total population. Samples can yield reasonably accurate information. Strong similarities in population elements makes sampling possible. Sampling may be more accurate than a census. Destruction of Test Units n Sampling reduces the costs of research in finite populations.
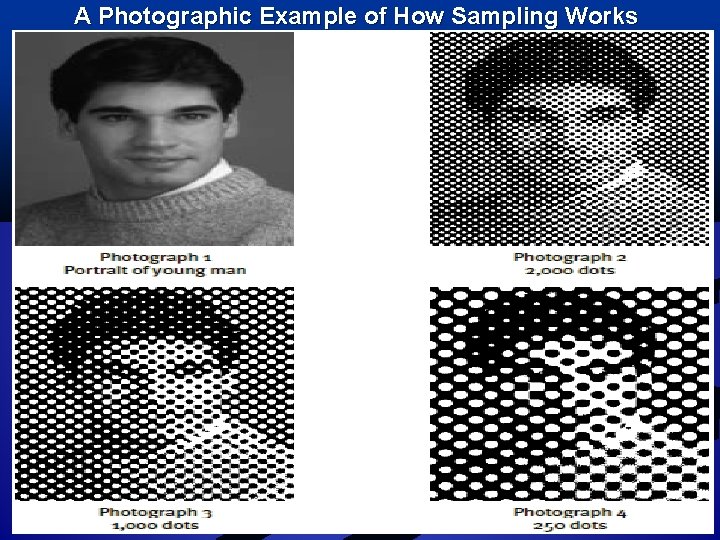
A Photographic Example of How Sampling Works
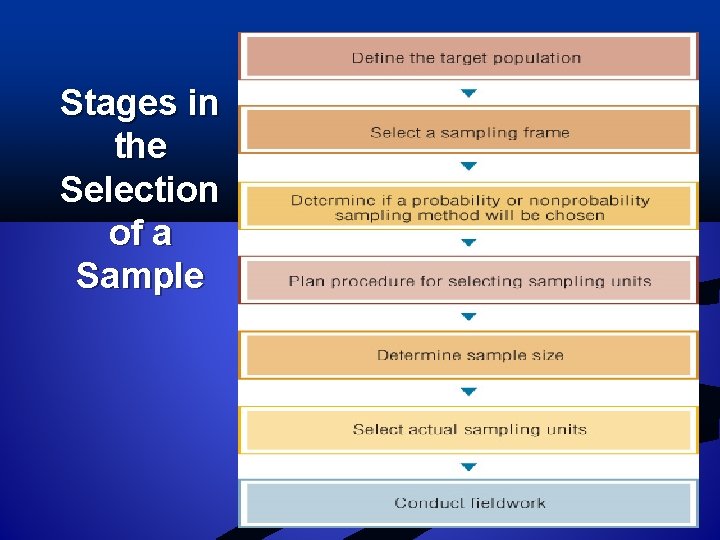
Stages in the Selection of a Sample 16– 11
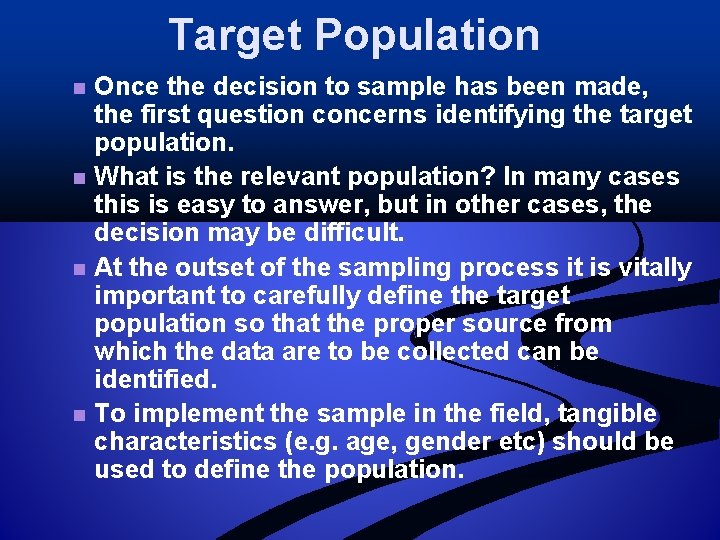
Target Population n n Once the decision to sample has been made, the first question concerns identifying the target population. What is the relevant population? In many cases this is easy to answer, but in other cases, the decision may be difficult. At the outset of the sampling process it is vitally important to carefully define the target population so that the proper source from which the data are to be collected can be identified. To implement the sample in the field, tangible characteristics (e. g. age, gender etc) should be used to define the population.
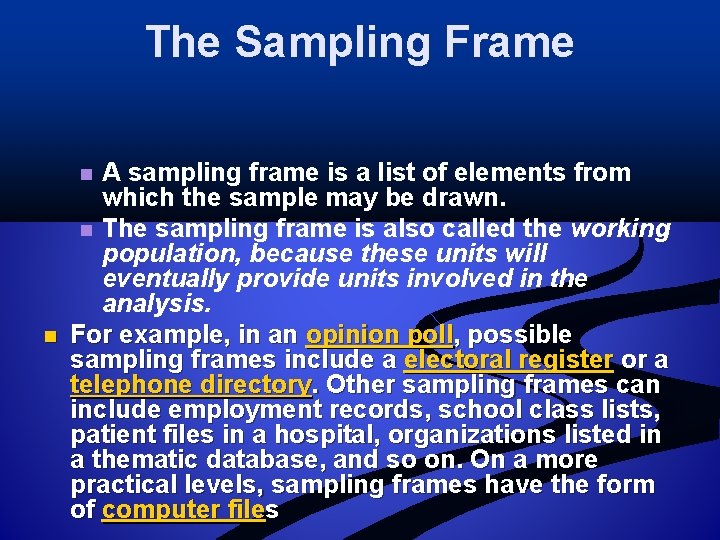
The Sampling Frame A sampling frame is a list of elements from which the sample may be drawn. n The sampling frame is also called the working population, because these units will eventually provide units involved in the analysis. For example, in an opinion poll, possible sampling frames include a electoral register or a telephone directory. Other sampling frames can include employment records, school class lists, patient files in a hospital, organizations listed in a thematic database, and so on. On a more practical levels, sampling frames have the form of computer files n n
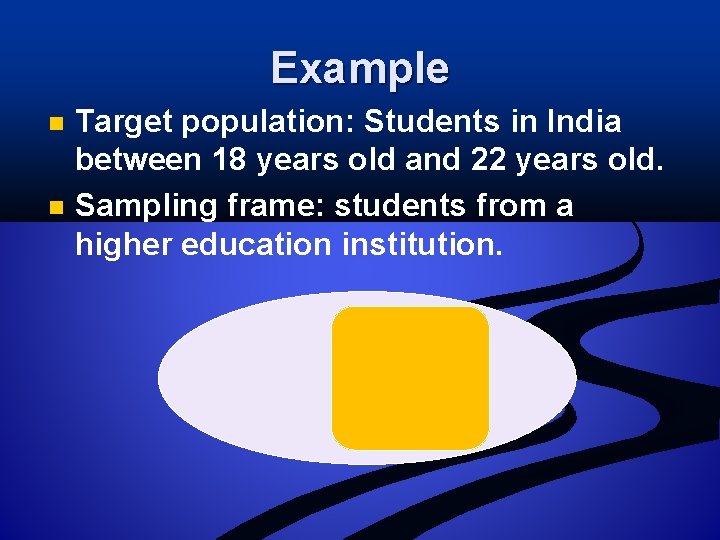
Example n n Target population: Students in India between 18 years old and 22 years old. Sampling frame: students from a higher education institution.
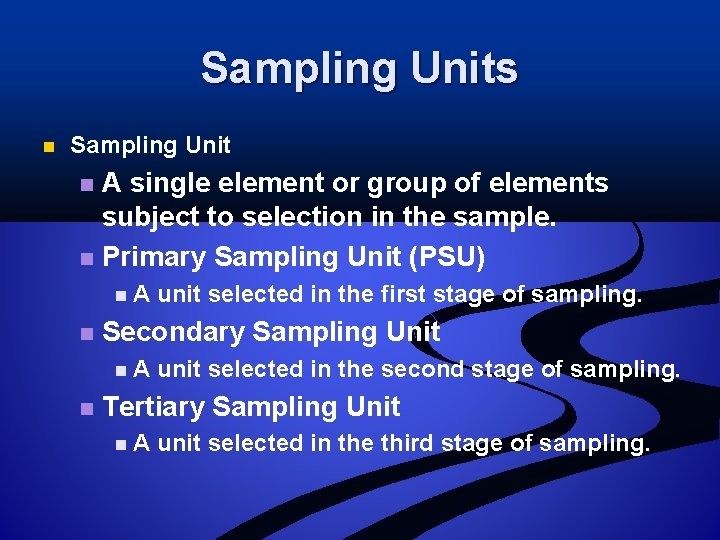
Sampling Units n Sampling Unit A single element or group of elements subject to selection in the sample. n Primary Sampling Unit (PSU) n n. A n Secondary Sampling Unit n. A n unit selected in the first stage of sampling. unit selected in the second stage of sampling. Tertiary Sampling Unit n. A unit selected in the third stage of sampling.
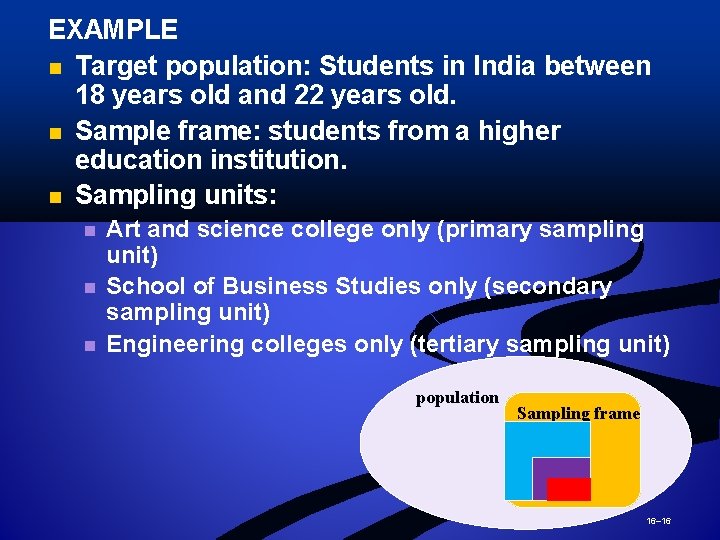
EXAMPLE n Target population: Students in India between 18 years old and 22 years old. n Sample frame: students from a higher education institution. n Sampling units: n n n Art and science college only (primary sampling unit) School of Business Studies only (secondary sampling unit) Engineering colleges only (tertiary sampling unit) population Sampling frame 16– 16
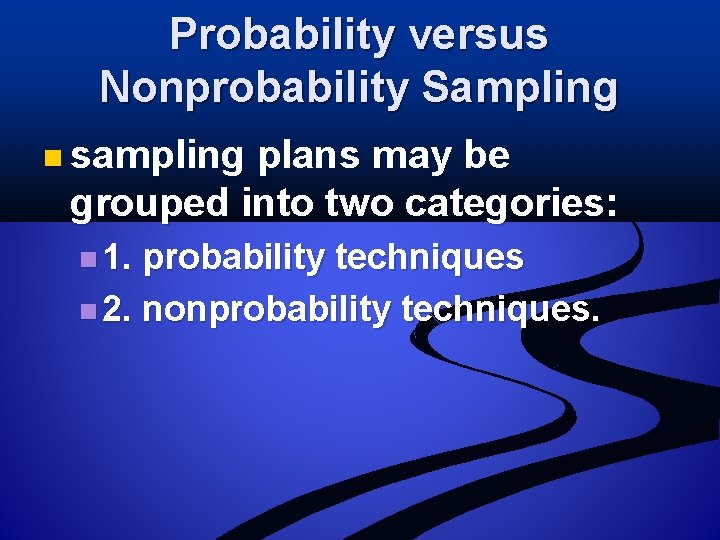
Probability versus Nonprobability Sampling n sampling plans may be grouped into two categories: n 1. probability techniques n 2. nonprobability techniques.
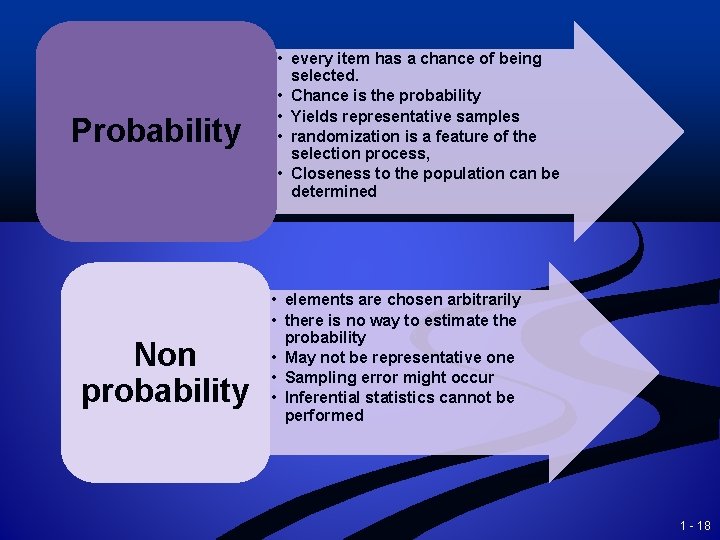
Probability Non probability • every item has a chance of being selected. • Chance is the probability • Yields representative samples • randomization is a feature of the selection process, • Closeness to the population can be determined • elements are chosen arbitrarily • there is no way to estimate the probability • May not be representative one • Sampling error might occur • Inferential statistics cannot be performed 1 - 18
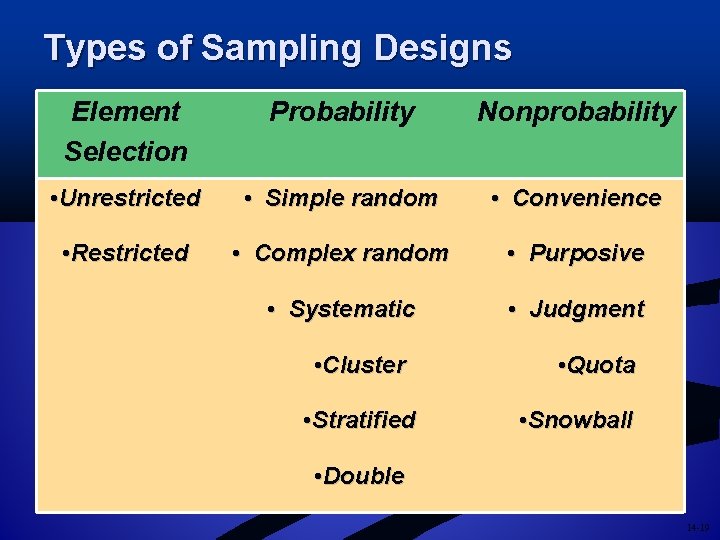
Types of Sampling Designs Element Selection Probability Nonprobability • Unrestricted • Simple random • Convenience • Restricted • Complex random • Purposive • Systematic • Judgment • Cluster • Stratified • Quota • Snowball • Double 14 -19
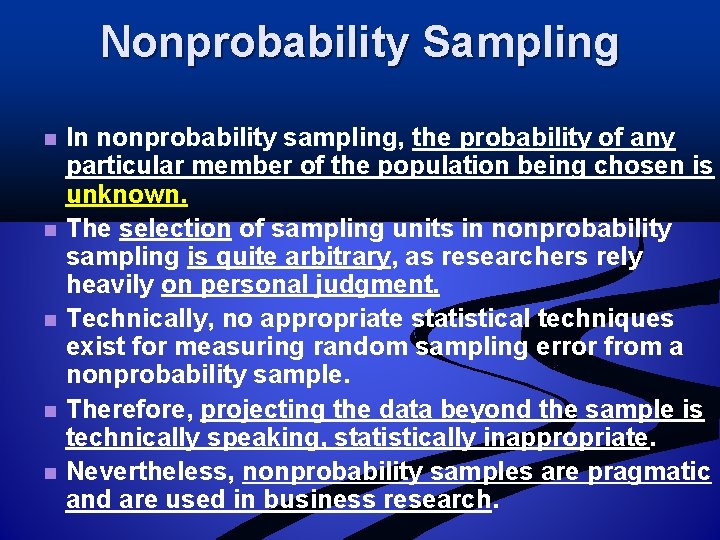
Nonprobability Sampling n n n In nonprobability sampling, the probability of any particular member of the population being chosen is unknown. The selection of sampling units in nonprobability sampling is quite arbitrary, as researchers rely heavily on personal judgment. Technically, no appropriate statistical techniques exist for measuring random sampling error from a nonprobability sample. Therefore, projecting the data beyond the sample is technically speaking, statistically inappropriate. Nevertheless, nonprobability samples are pragmatic and are used in business research.
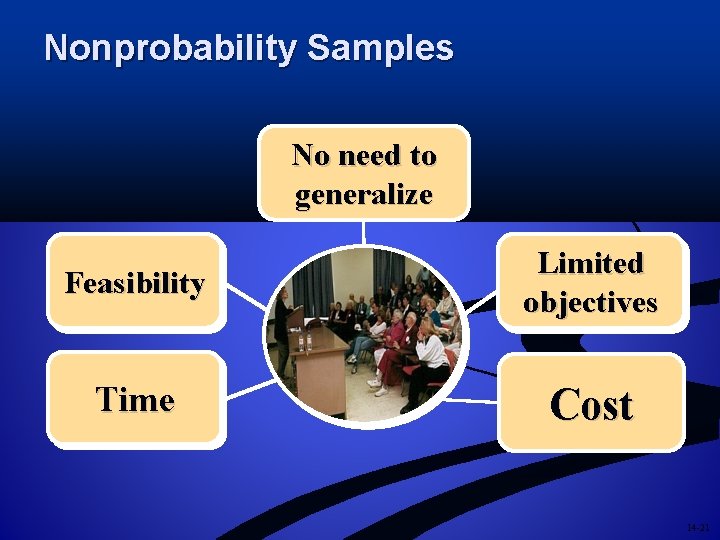
Nonprobability Samples No need to generalize Feasibility Limited objectives Time Cost 14 -21
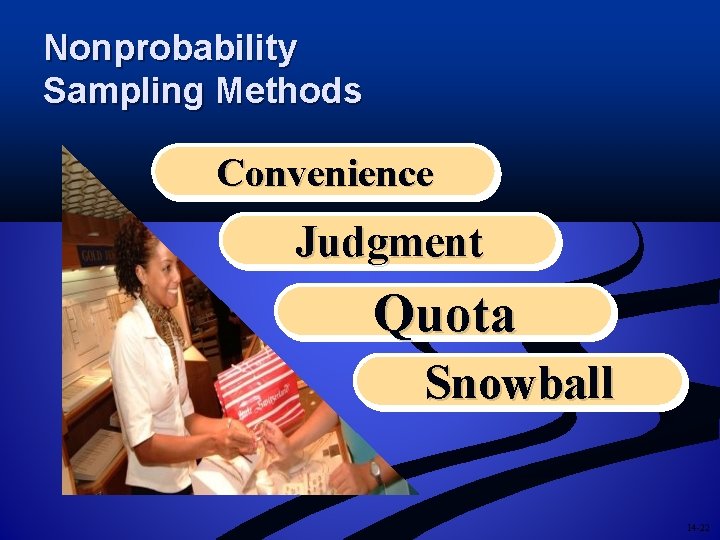
Nonprobability Sampling Methods Convenience Judgment Quota Snowball 14 -22
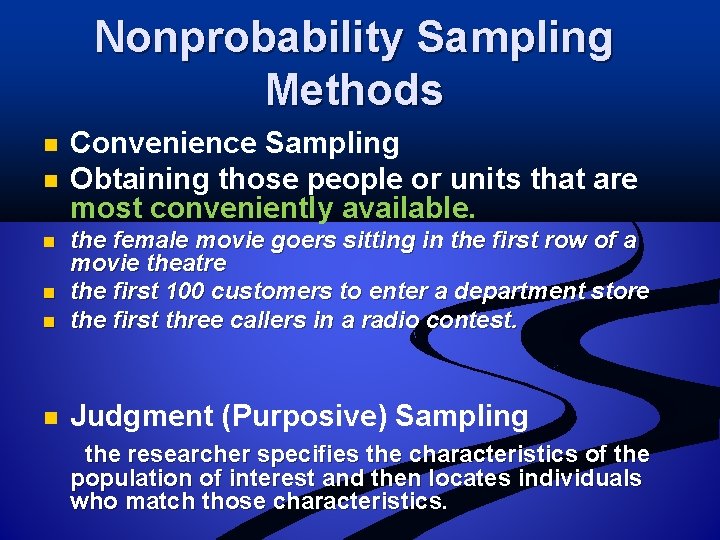
Nonprobability Sampling Methods n n Convenience Sampling Obtaining those people or units that are most conveniently available. n the female movie goers sitting in the first row of a movie theatre the first 100 customers to enter a department store the first three callers in a radio contest. n Judgment (Purposive) Sampling n n the researcher specifies the characteristics of the population of interest and then locates individuals who match those characteristics.
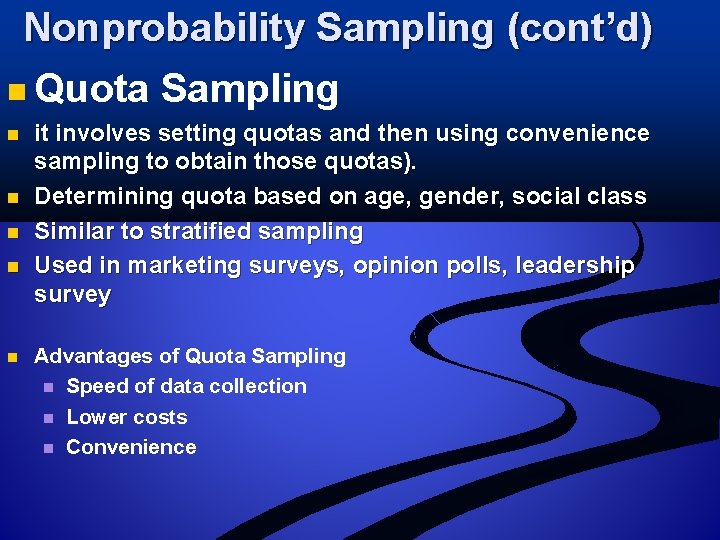
Nonprobability Sampling (cont’d) n Quota Sampling n n n it involves setting quotas and then using convenience sampling to obtain those quotas). Determining quota based on age, gender, social class Similar to stratified sampling Used in marketing surveys, opinion polls, leadership survey Advantages of Quota Sampling n Speed of data collection n Lower costs n Convenience
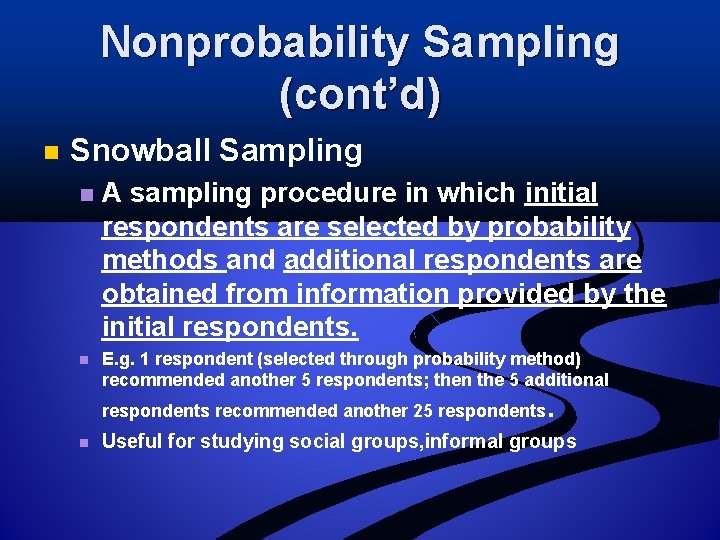
Nonprobability Sampling (cont’d) n Snowball Sampling n n A sampling procedure in which initial respondents are selected by probability methods and additional respondents are obtained from information provided by the initial respondents. E. g. 1 respondent (selected through probability method) recommended another 5 respondents; then the 5 additional respondents recommended another 25 respondents. n Useful for studying social groups, informal groups
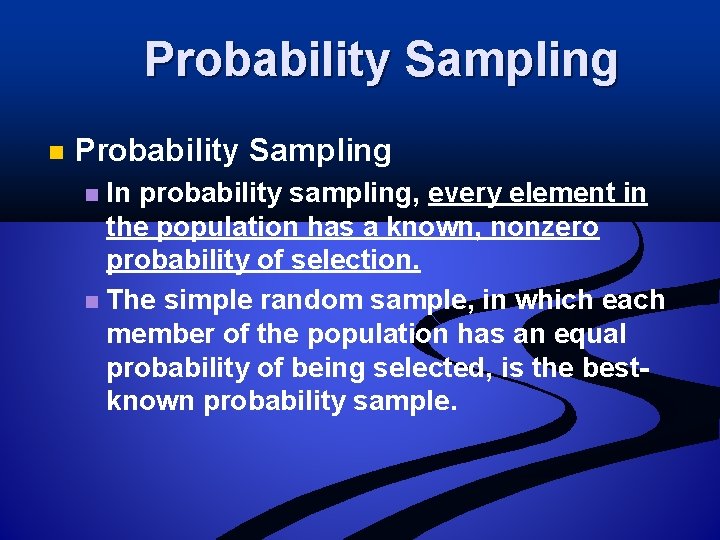
Probability Sampling n Probability Sampling In probability sampling, every element in the population has a known, nonzero probability of selection. n The simple random sample, in which each member of the population has an equal probability of being selected, is the bestknown probability sample. n
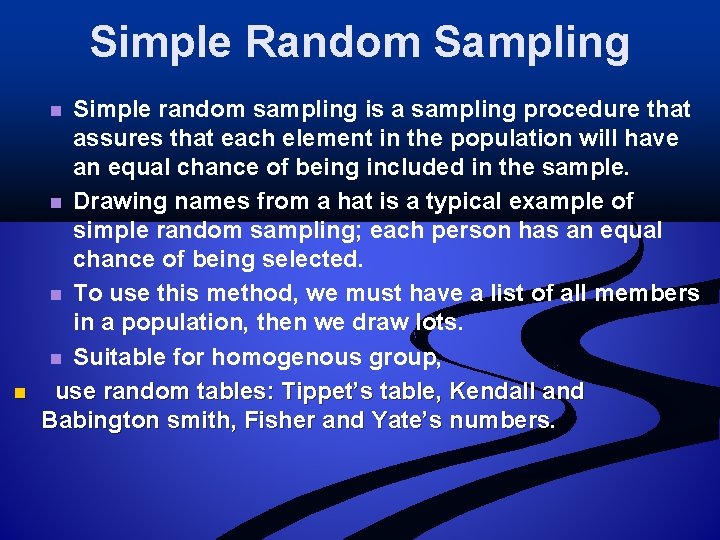
Simple Random Sampling Simple random sampling is a sampling procedure that assures that each element in the population will have an equal chance of being included in the sample. n Drawing names from a hat is a typical example of simple random sampling; each person has an equal chance of being selected. n To use this method, we must have a list of all members in a population, then we draw lots. n Suitable for homogenous group, use random tables: Tippet’s table, Kendall and Babington smith, Fisher and Yate’s numbers. n n
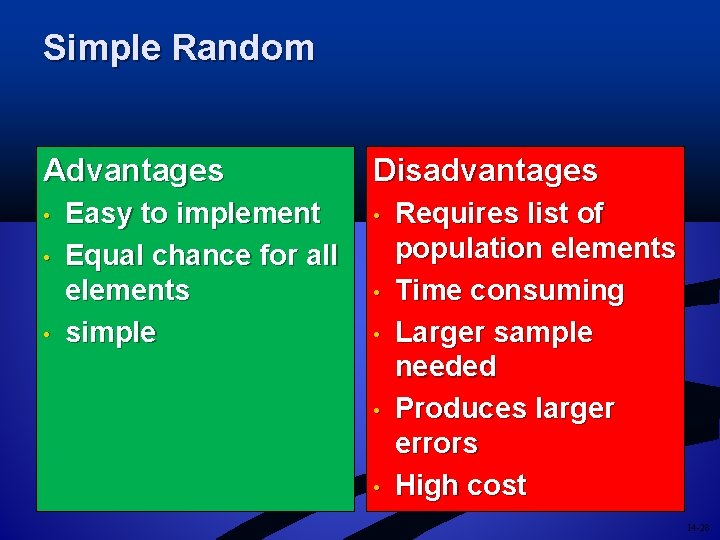
Simple Random Advantages • • • Easy to implement Equal chance for all elements simple Disadvantages • • • Requires list of population elements Time consuming Larger sample needed Produces larger errors High cost 14 -28
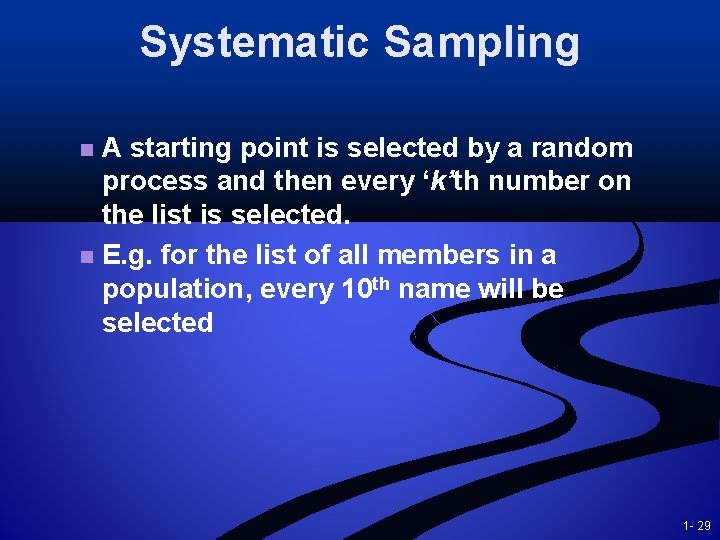
Systematic Sampling A starting point is selected by a random process and then every ‘k’th number on the list is selected. n E. g. for the list of all members in a population, every 10 th name will be selected n 1 - 29
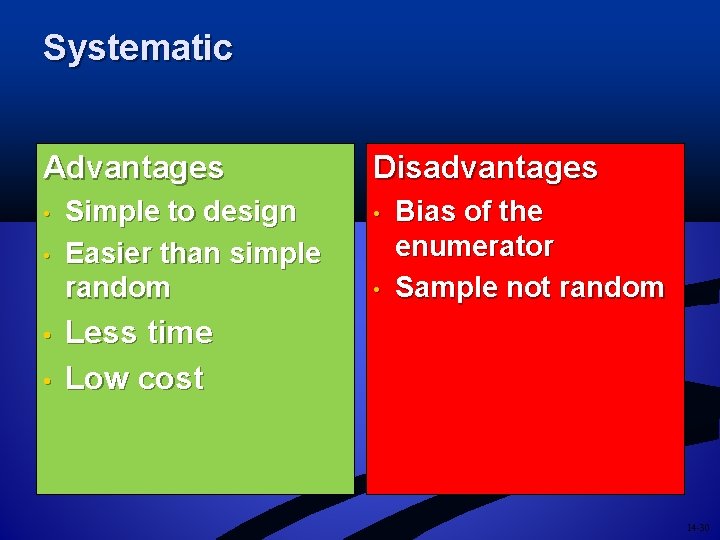
Systematic Advantages • • Simple to design Easier than simple random Disadvantages • • Bias of the enumerator Sample not random Less time Low cost 14 -30
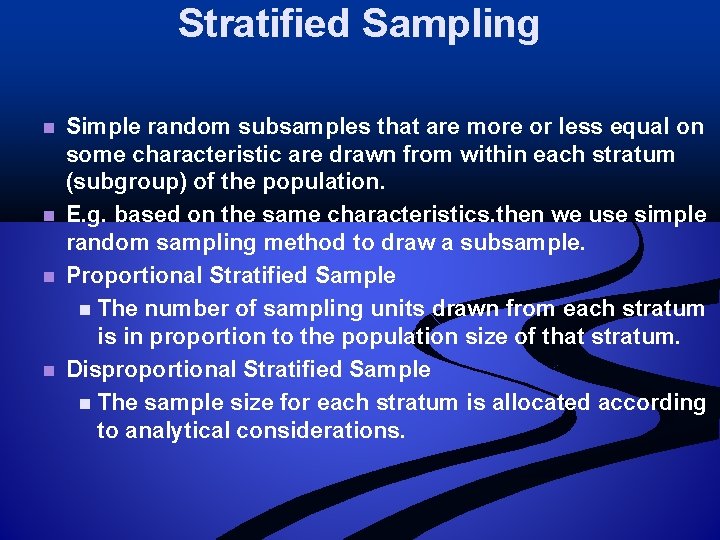
Stratified Sampling n n Simple random subsamples that are more or less equal on some characteristic are drawn from within each stratum (subgroup) of the population. E. g. based on the same characteristics. then we use simple random sampling method to draw a subsample. Proportional Stratified Sample n The number of sampling units drawn from each stratum is in proportion to the population size of that stratum. Disproportional Stratified Sample n The sample size for each stratum is allocated according to analytical considerations.
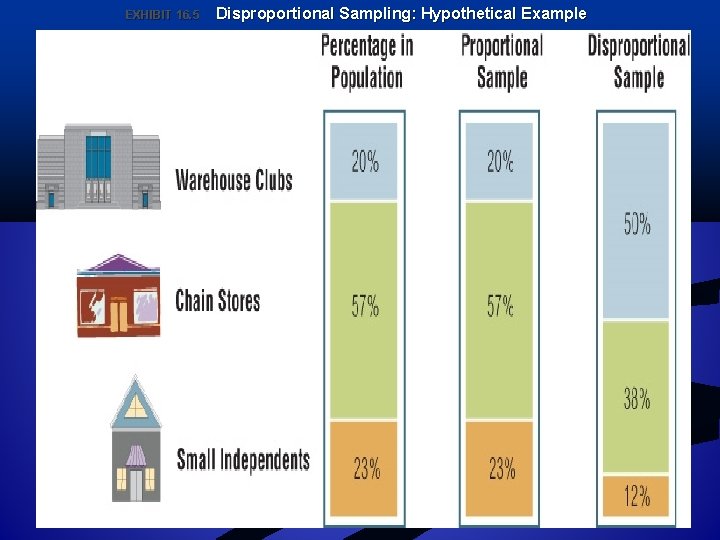
EXHIBIT 16. 5 Disproportional Sampling: Hypothetical Example
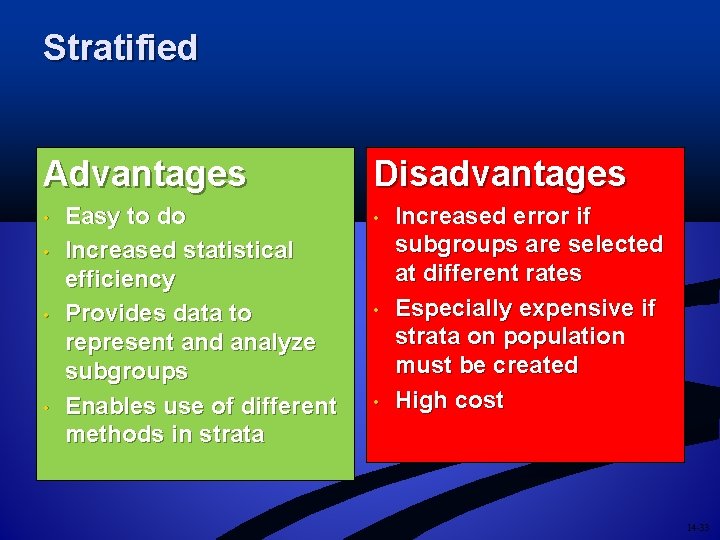
Stratified Advantages • • Easy to do Increased statistical efficiency Provides data to represent and analyze subgroups Enables use of different methods in strata Disadvantages • • • Increased error if subgroups are selected at different rates Especially expensive if strata on population must be created High cost 14 -33
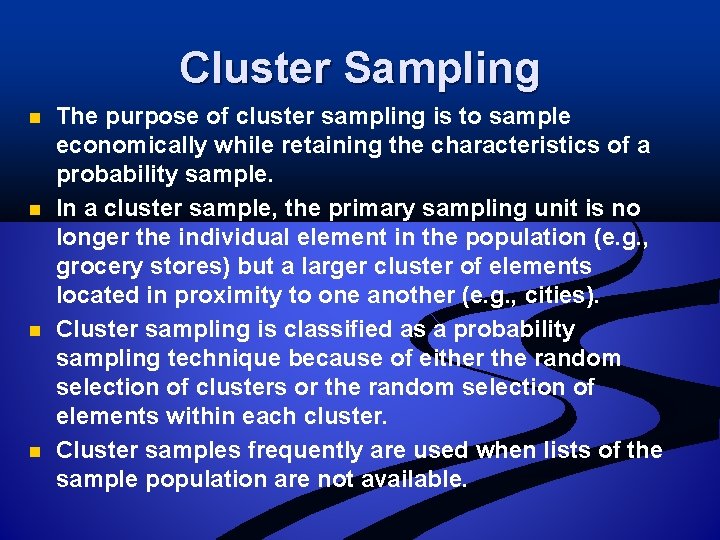
Cluster Sampling n n The purpose of cluster sampling is to sample economically while retaining the characteristics of a probability sample. In a cluster sample, the primary sampling unit is no longer the individual element in the population (e. g. , grocery stores) but a larger cluster of elements located in proximity to one another (e. g. , cities). Cluster sampling is classified as a probability sampling technique because of either the random selection of clusters or the random selection of elements within each cluster. Cluster samples frequently are used when lists of the sample population are not available.
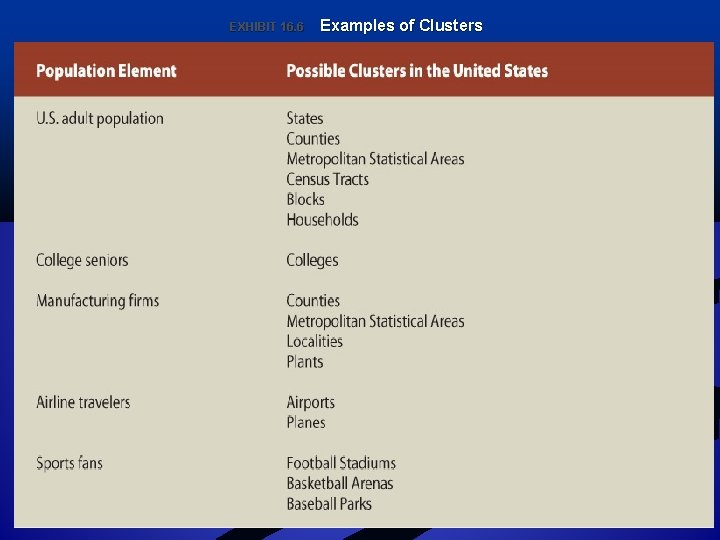
EXHIBIT 16. 6 Examples of Clusters
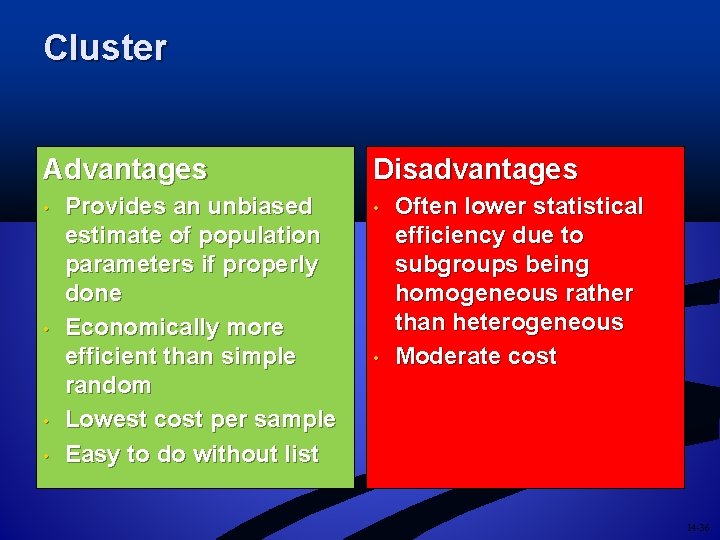
Cluster Advantages • • Provides an unbiased estimate of population parameters if properly done Economically more efficient than simple random Lowest cost per sample Easy to do without list Disadvantages • • Often lower statistical efficiency due to subgroups being homogeneous rather than heterogeneous Moderate cost 14 -36
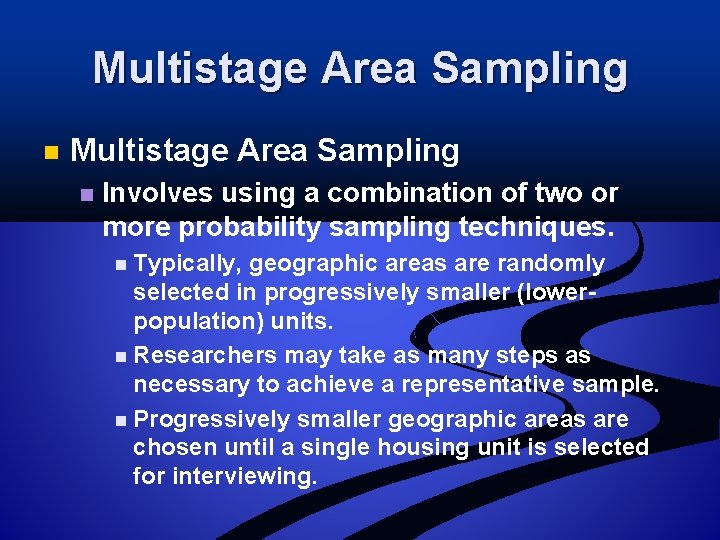
Multistage Area Sampling n Involves using a combination of two or more probability sampling techniques. n Typically, geographic areas are randomly selected in progressively smaller (lowerpopulation) units. n Researchers may take as many steps as necessary to achieve a representative sample. n Progressively smaller geographic areas are chosen until a single housing unit is selected for interviewing.
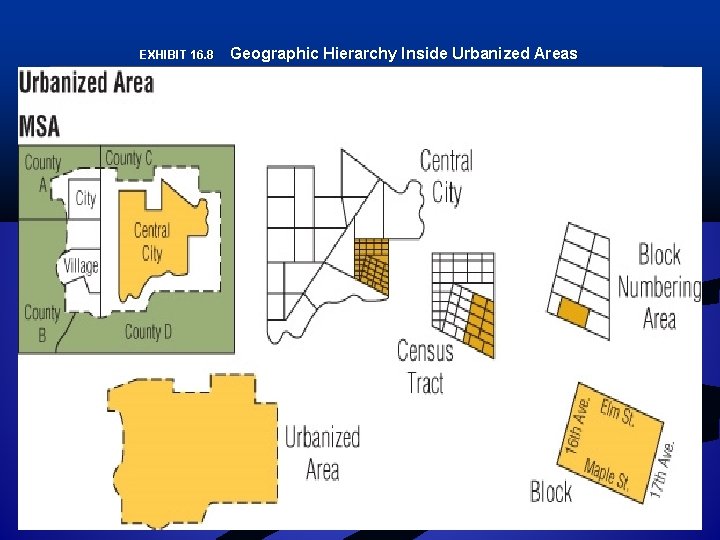
EXHIBIT 16. 8 Geographic Hierarchy Inside Urbanized Areas
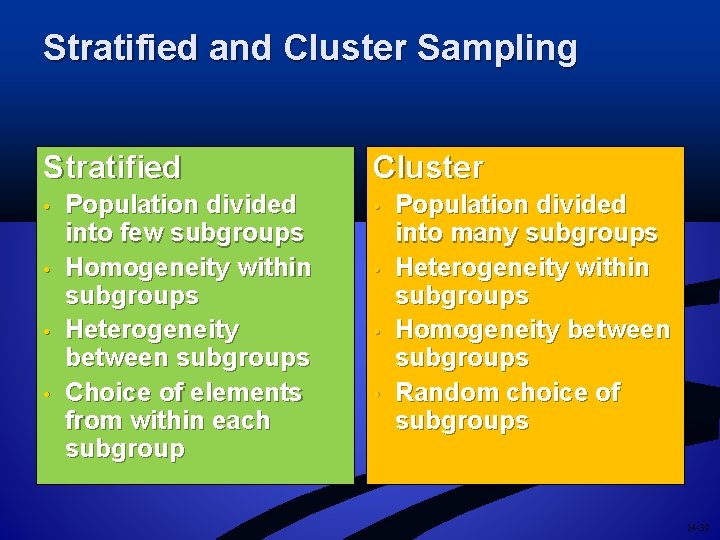
Stratified and Cluster Sampling Stratified • • Population divided into few subgroups Homogeneity within subgroups Heterogeneity between subgroups Choice of elements from within each subgroup Cluster • • Population divided into many subgroups Heterogeneity within subgroups Homogeneity between subgroups Random choice of subgroups 14 -39
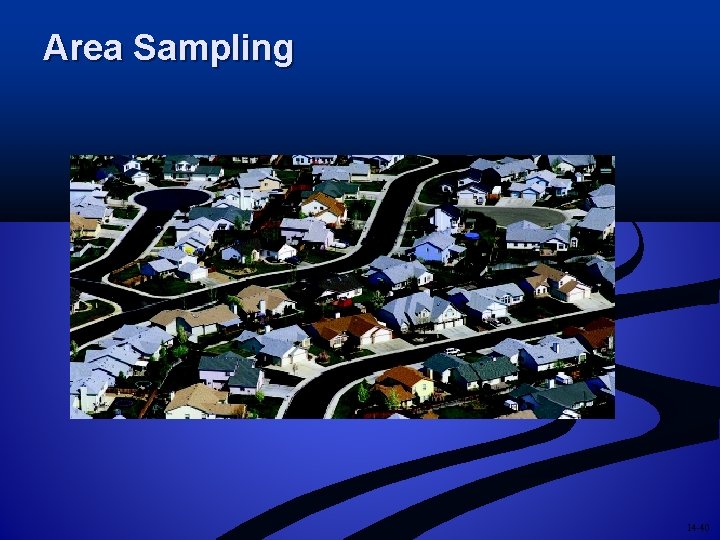
Area Sampling 14 -40
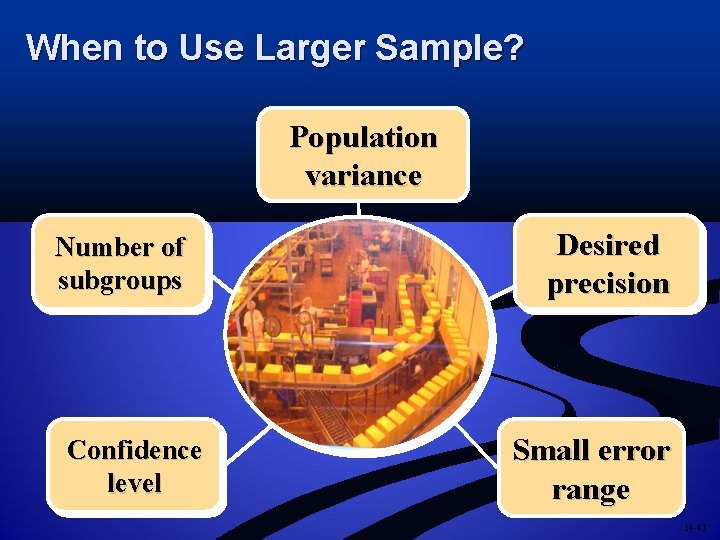
When to Use Larger Sample? Population variance Number of subgroups Confidence level Desired precision Small error range 14 -41`
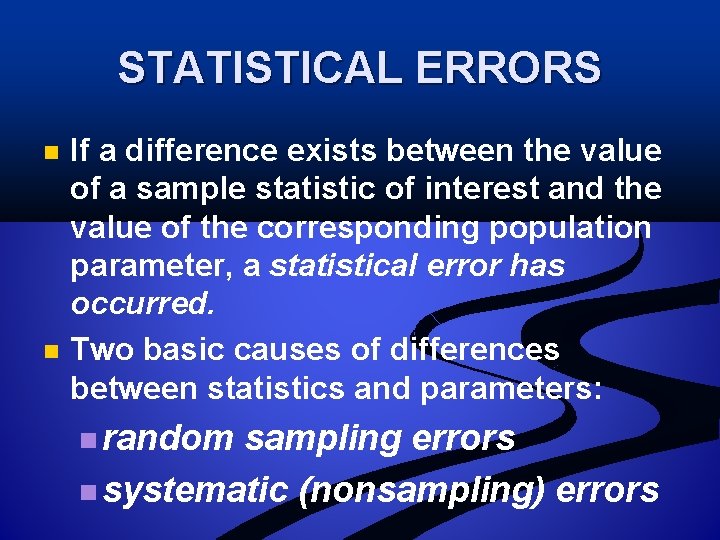
STATISTICAL ERRORS n n If a difference exists between the value of a sample statistic of interest and the value of the corresponding population parameter, a statistical error has occurred. Two basic causes of differences between statistics and parameters: n random sampling errors n systematic (nonsampling) errors
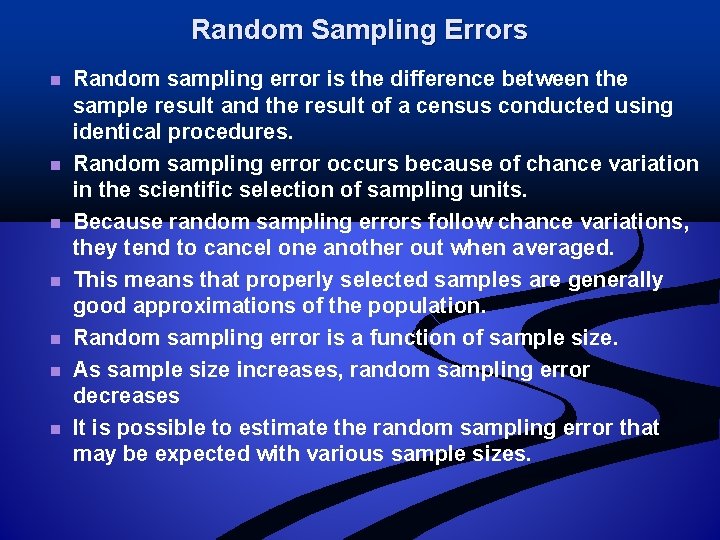
Random Sampling Errors n n n n Random sampling error is the difference between the sample result and the result of a census conducted using identical procedures. Random sampling error occurs because of chance variation in the scientific selection of sampling units. Because random sampling errors follow chance variations, they tend to cancel one another out when averaged. This means that properly selected samples are generally good approximations of the population. Random sampling error is a function of sample size. As sample size increases, random sampling error decreases It is possible to estimate the random sampling error that may be expected with various sample sizes.
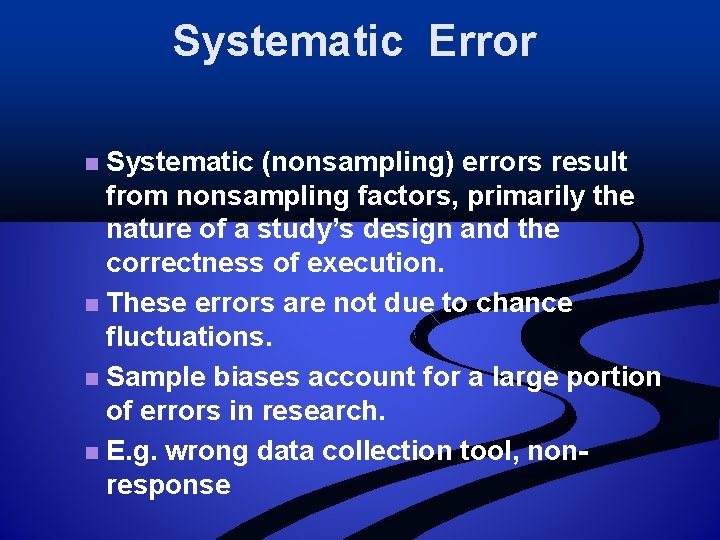
Systematic Error Systematic (nonsampling) errors result from nonsampling factors, primarily the nature of a study’s design and the correctness of execution. n These errors are not due to chance fluctuations. n Sample biases account for a large portion of errors in research. n E. g. wrong data collection tool, nonresponse n
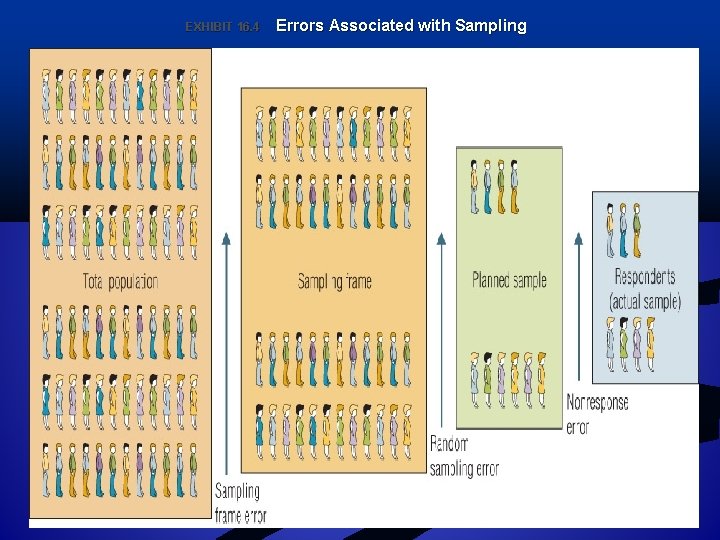
EXHIBIT 16. 4 Errors Associated with Sampling
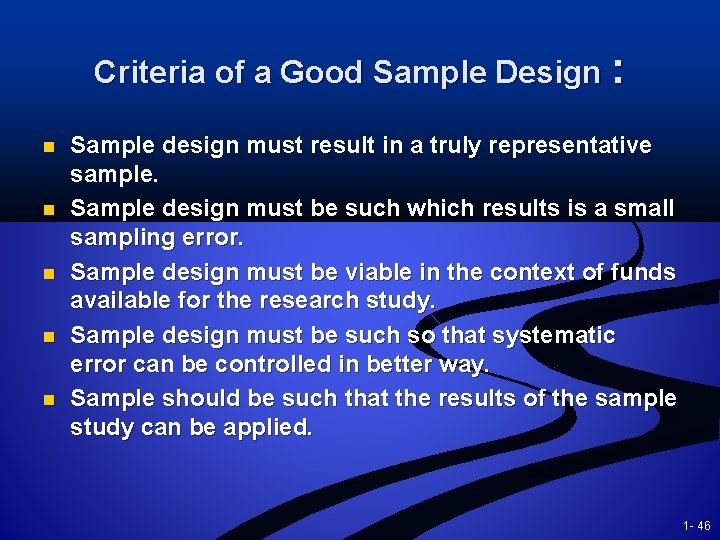
Criteria of a Good Sample Design : n n n Sample design must result in a truly representative sample. Sample design must be such which results is a small sampling error. Sample design must be viable in the context of funds available for the research study. Sample design must be such so that systematic error can be controlled in better way. Sample should be such that the results of the sample study can be applied. 1 - 46
Entertain persuade inform
Enlighten about image noise and restoration
Enlighten the darkness of my heart
Graphs that enlighten and graphs that deceive
Indirect proof assumption
Direct proof and indirect proof
What is indirect proof
Direct proof and indirect proof
Unit 2 homework 6 algebraic proof
2-5 algebraic proof
Probability vs non probability sampling
Cluster sampling vs stratified random sampling
Difference between cluster and strata
Contoh checklist observasi psikologi
Cluster sampling vs stratified sampling
Research sampling definition
Natural sampling vs flat top sampling
Successive independent samples design
Matched samples design
Hình ảnh bộ gõ cơ thể búng tay
Ng-html
Bổ thể
Tỉ lệ cơ thể trẻ em
Gấu đi như thế nào
Chụp phim tư thế worms-breton
Chúa yêu trần thế alleluia
Môn thể thao bắt đầu bằng từ đua
Thế nào là hệ số cao nhất
Các châu lục và đại dương trên thế giới
Công thức tính thế năng
Trời xanh đây là của chúng ta thể thơ
Mật thư tọa độ 5x5
101012 bằng
độ dài liên kết
Các châu lục và đại dương trên thế giới
Thể thơ truyền thống
Quá trình desamine hóa có thể tạo ra
Một số thể thơ truyền thống
Bàn tay mà dây bẩn
Vẽ hình chiếu vuông góc của vật thể sau
Thế nào là sự mỏi cơ
đặc điểm cơ thể của người tối cổ
Thứ tự các dấu thăng giáng ở hóa biểu
Vẽ hình chiếu đứng bằng cạnh của vật thể
Vẽ hình chiếu vuông góc của vật thể sau
Thẻ vin
đại từ thay thế