SAMPLING DESIGN 7 1 Reasons for sampling 7
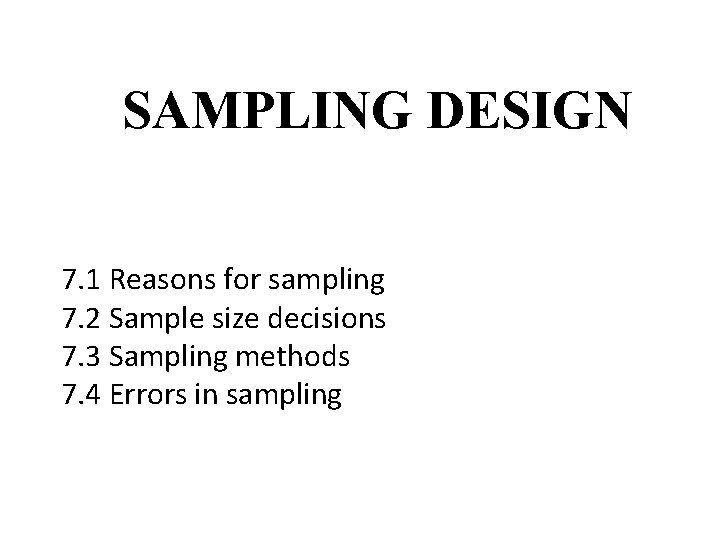
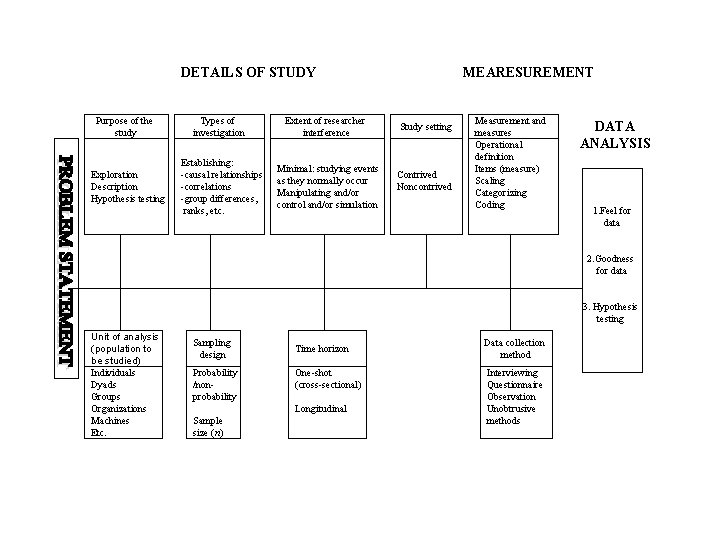
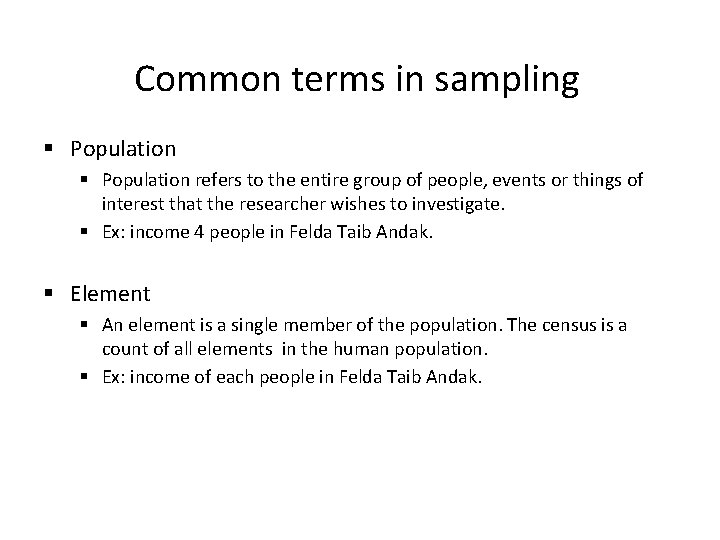
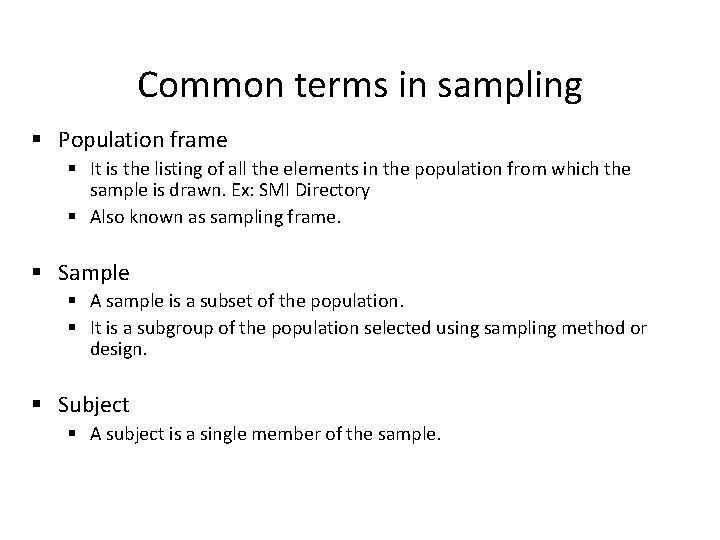
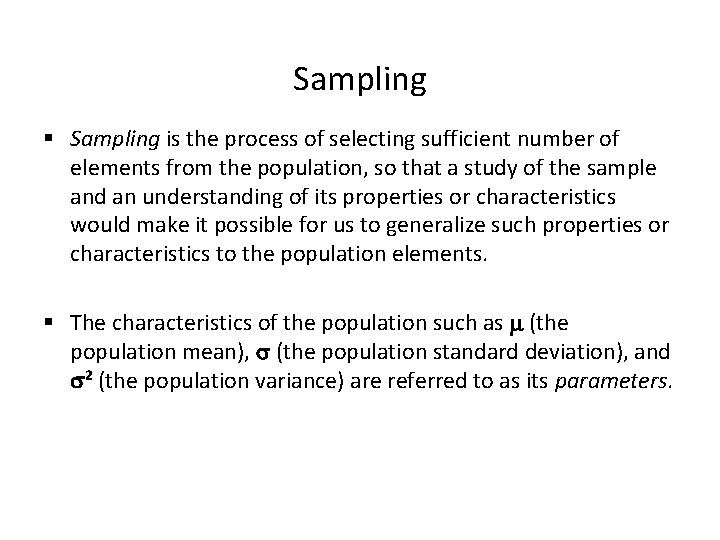
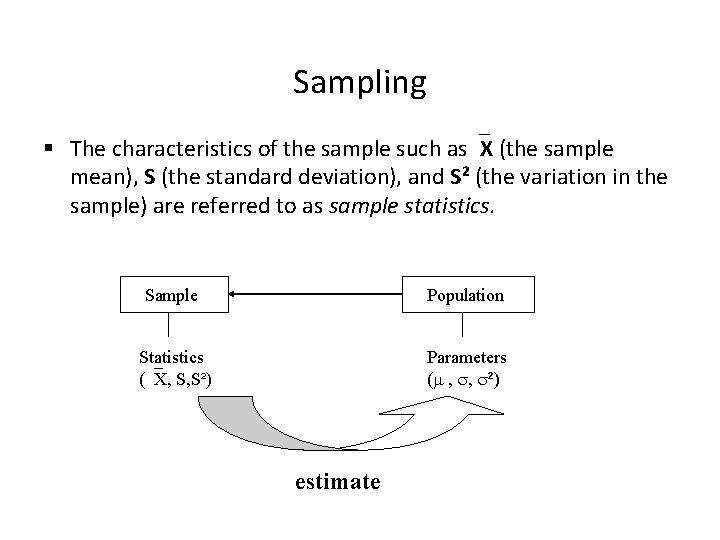
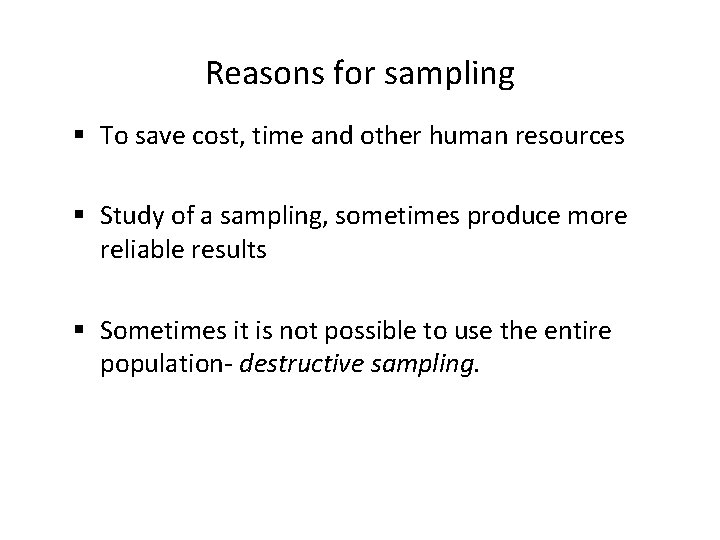
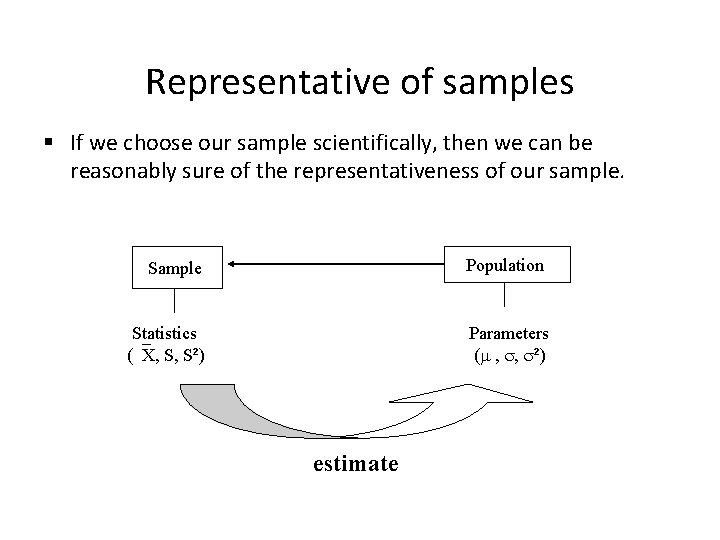
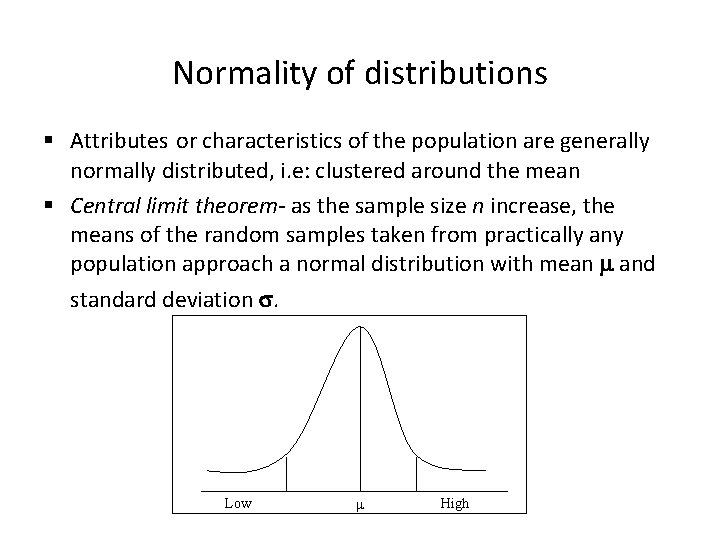
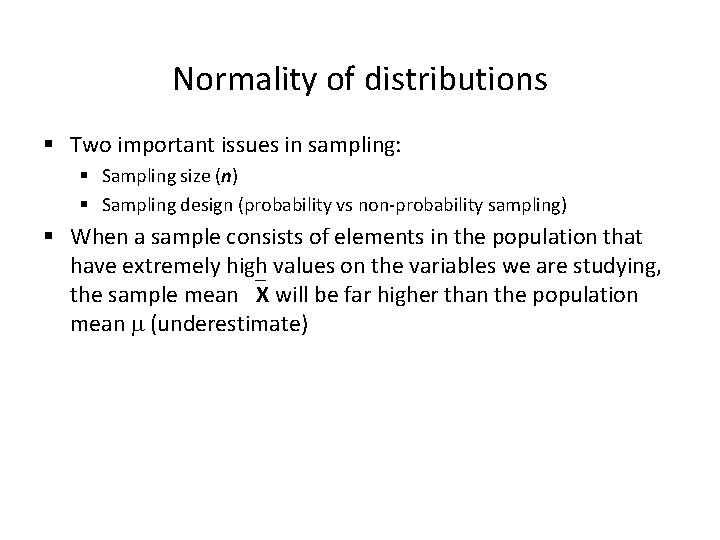
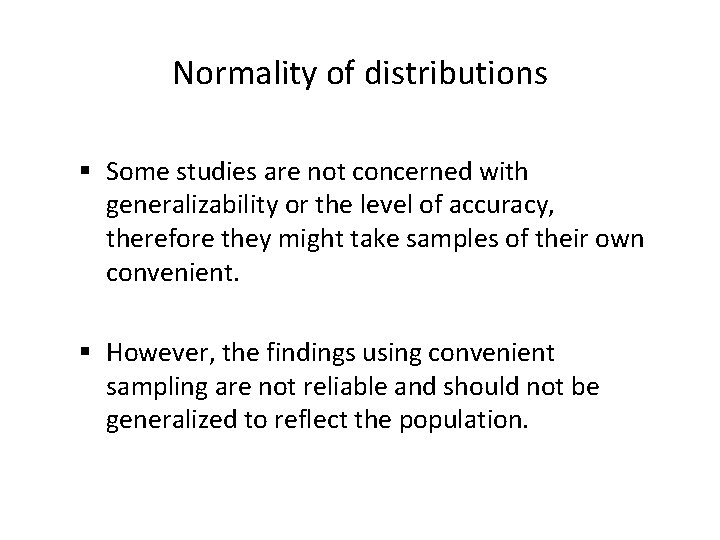
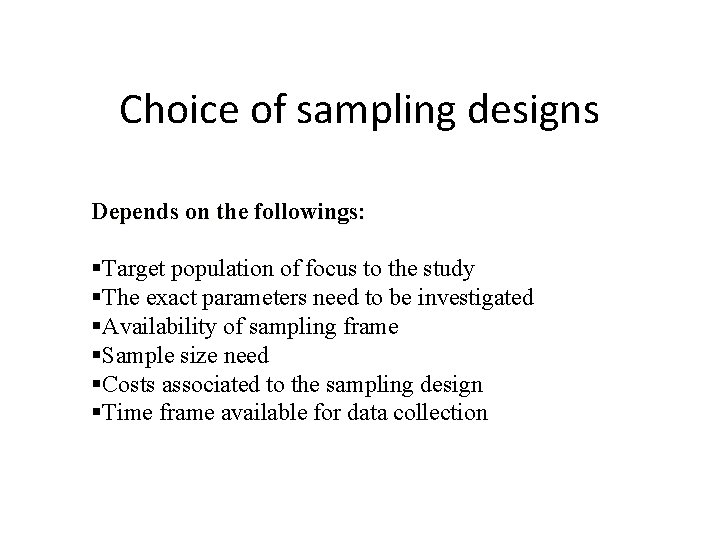
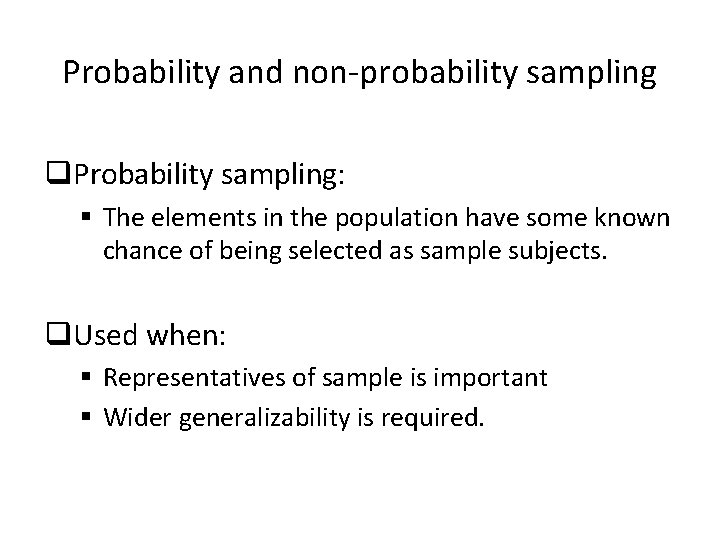
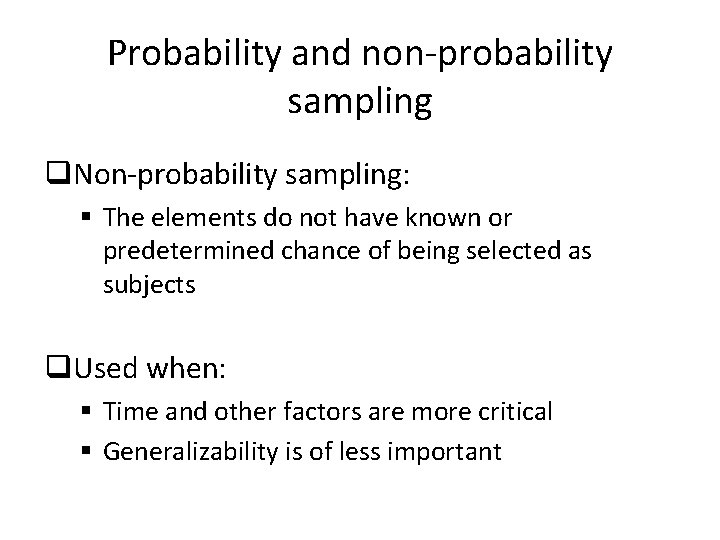
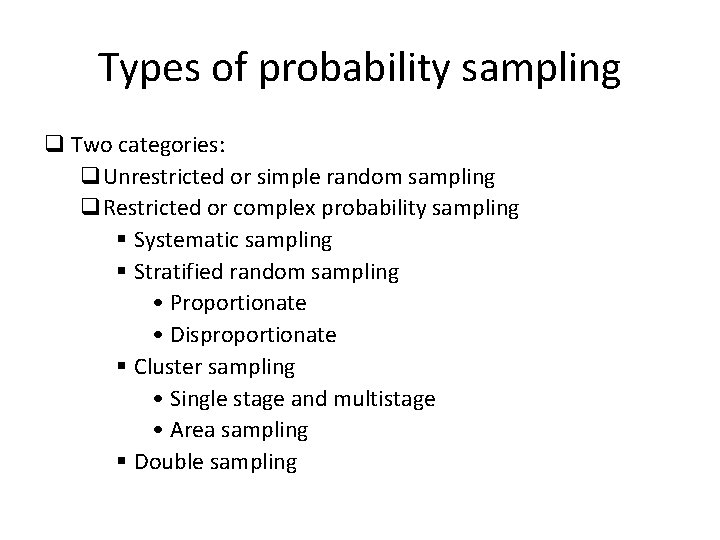
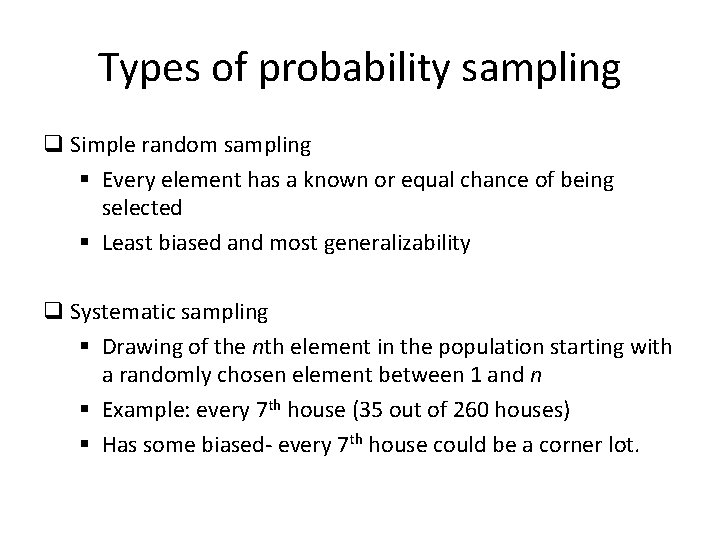
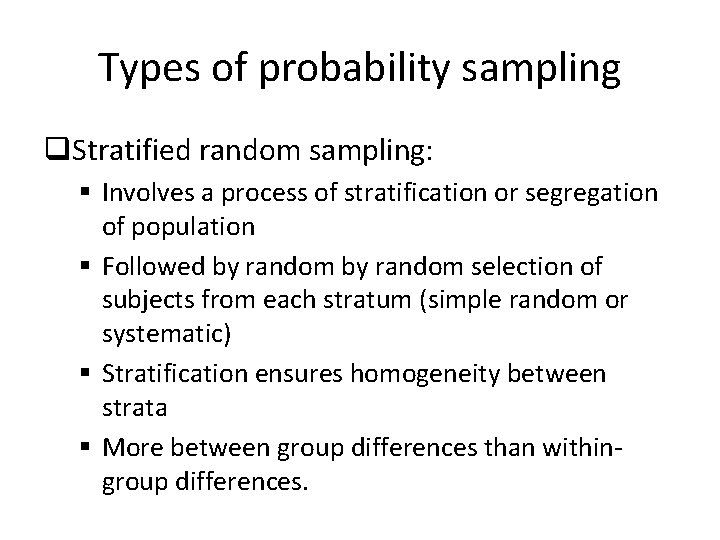
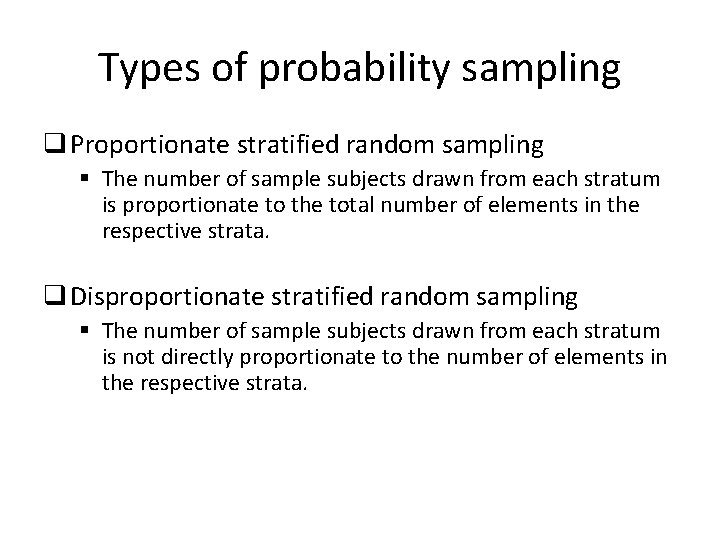
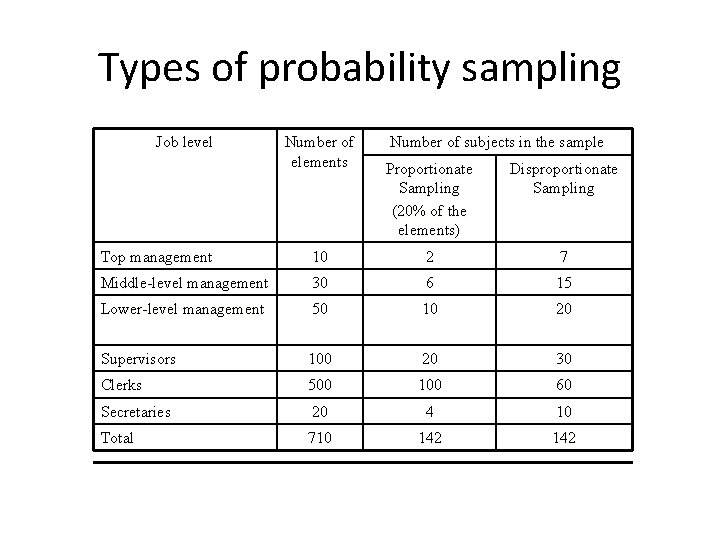
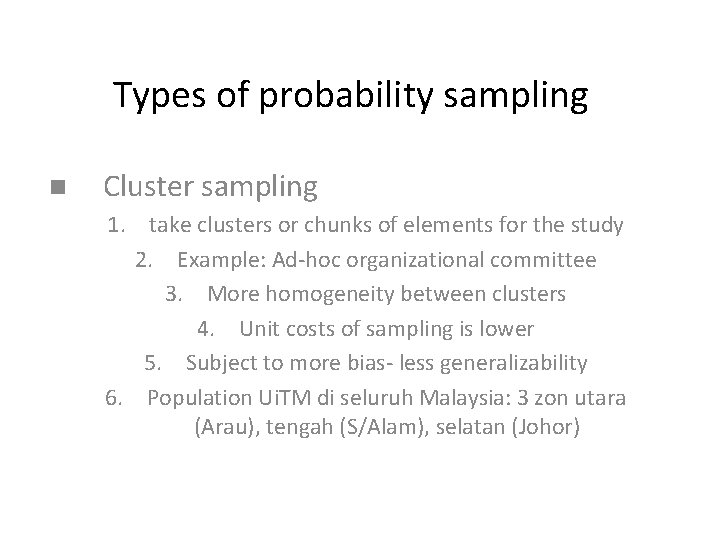
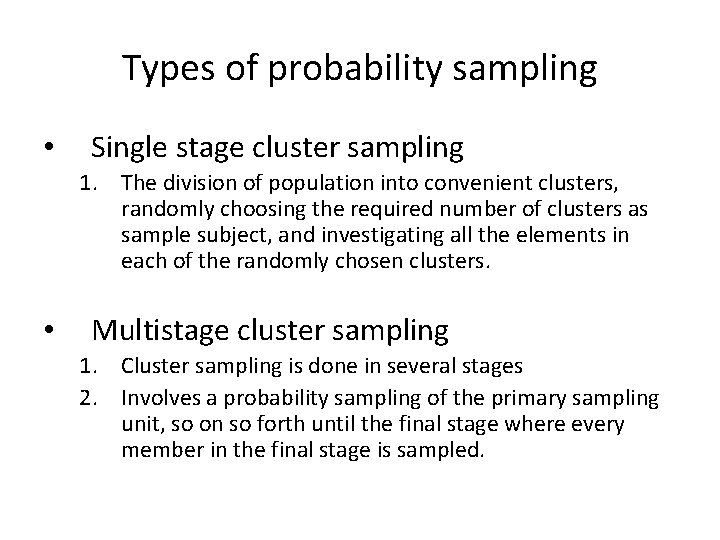
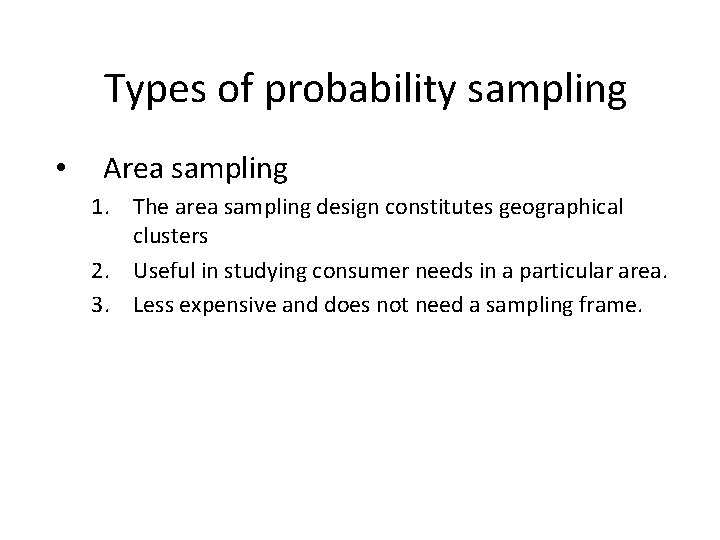
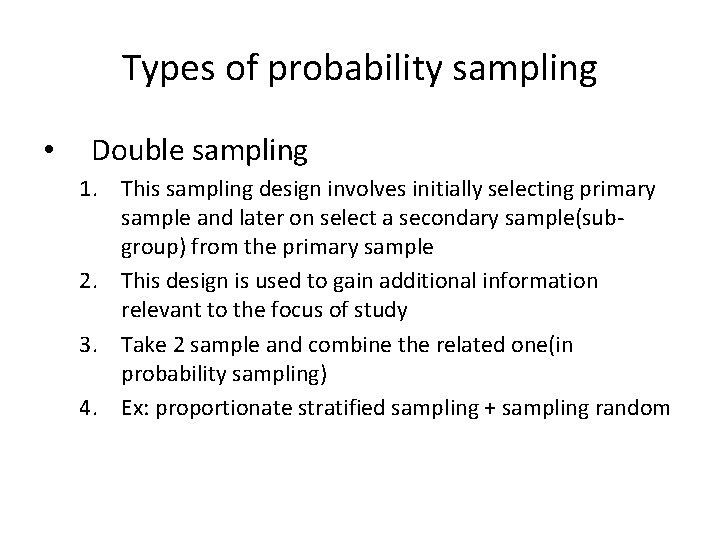
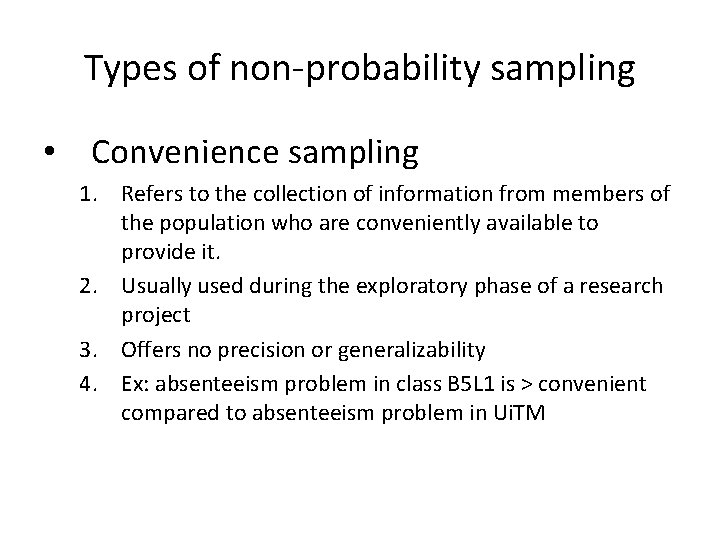
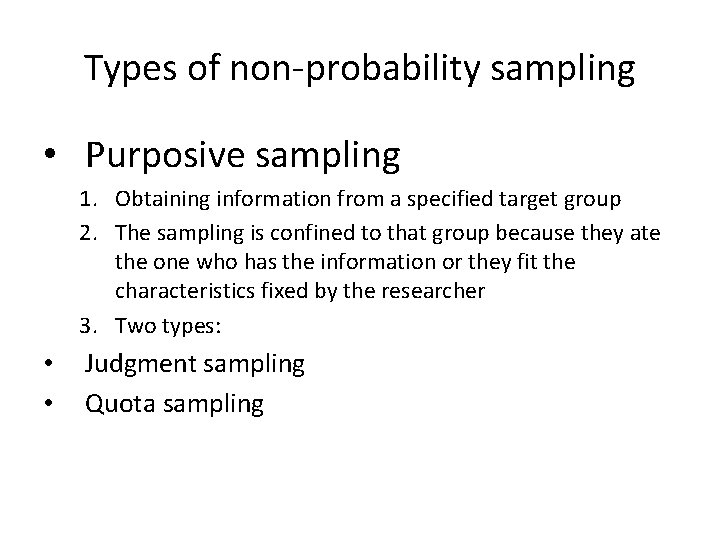
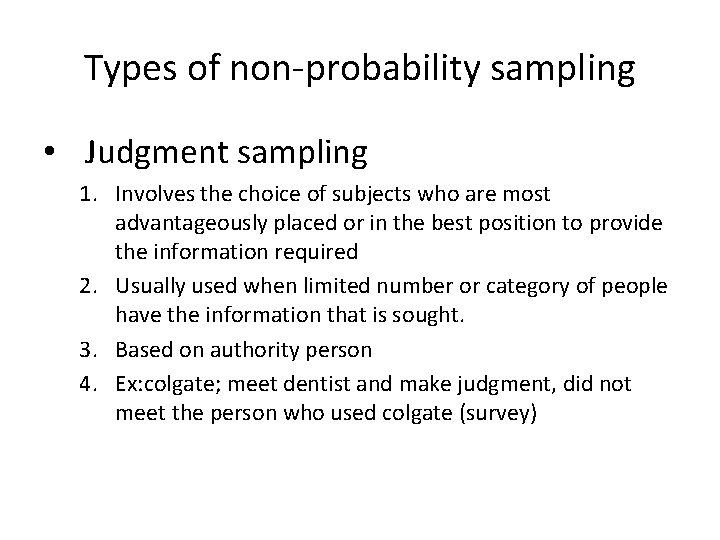
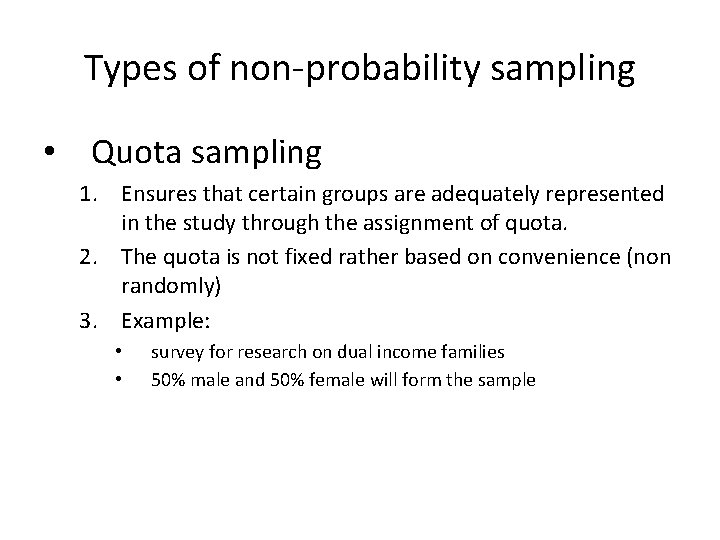
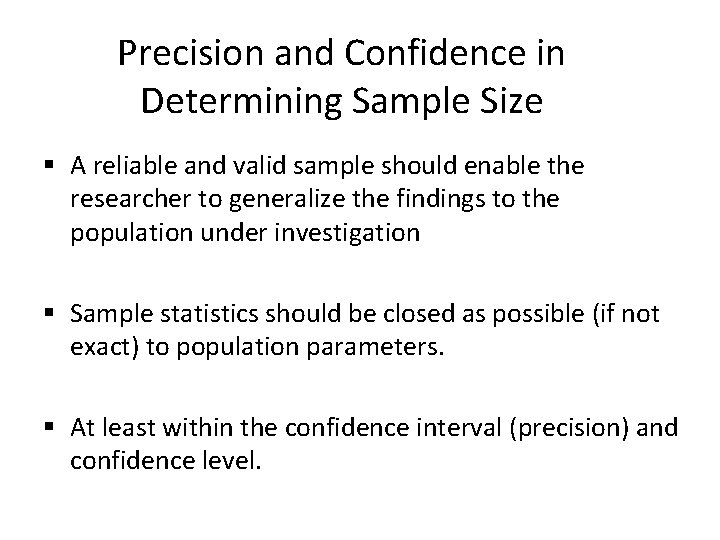
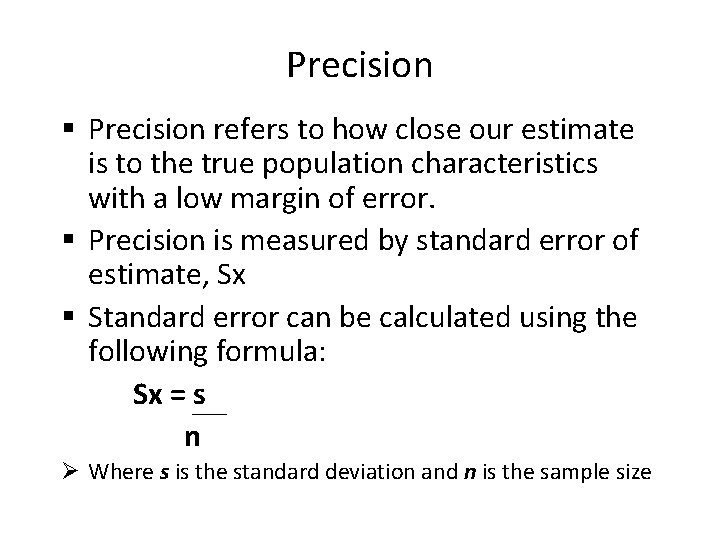
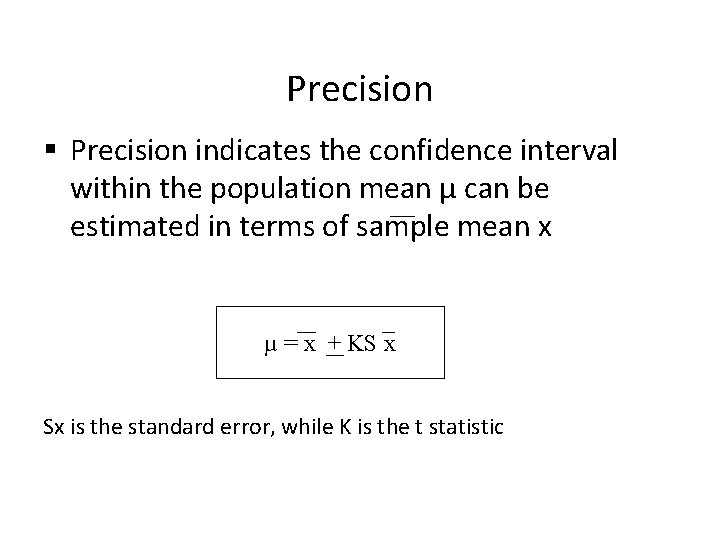
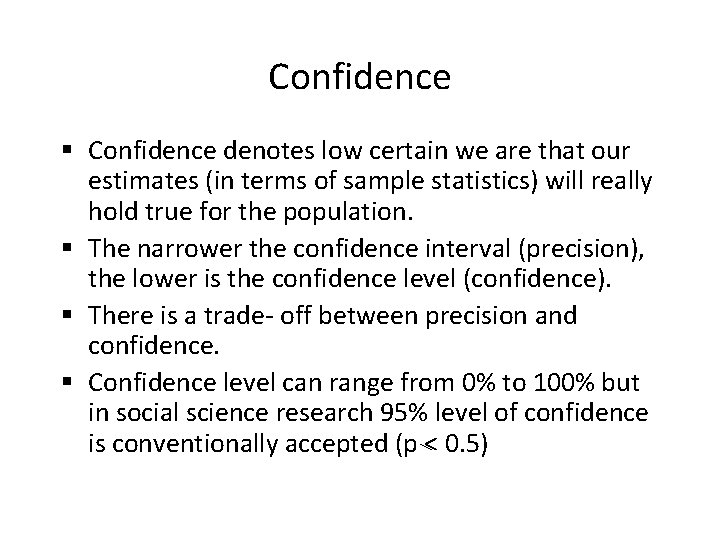
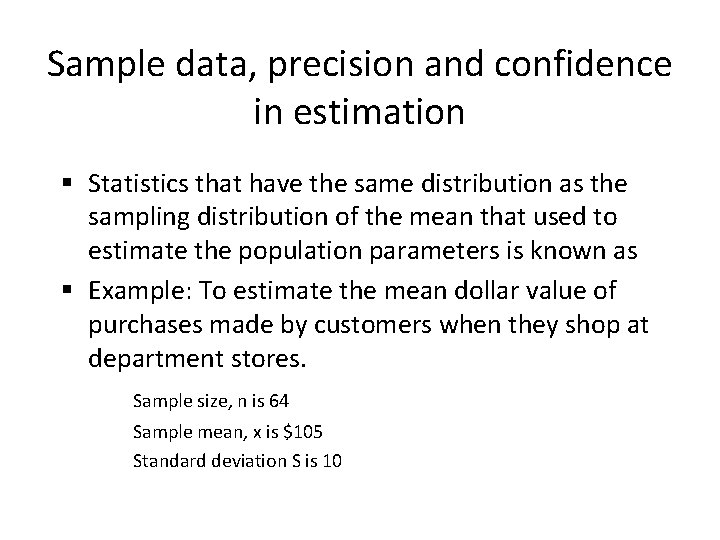
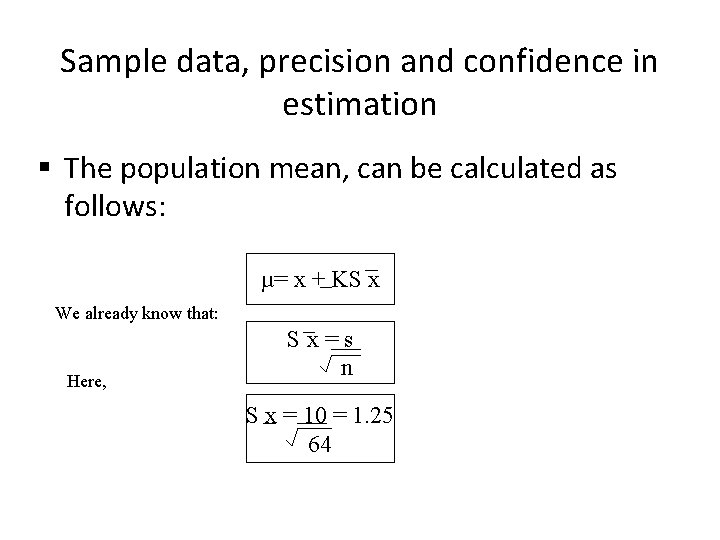
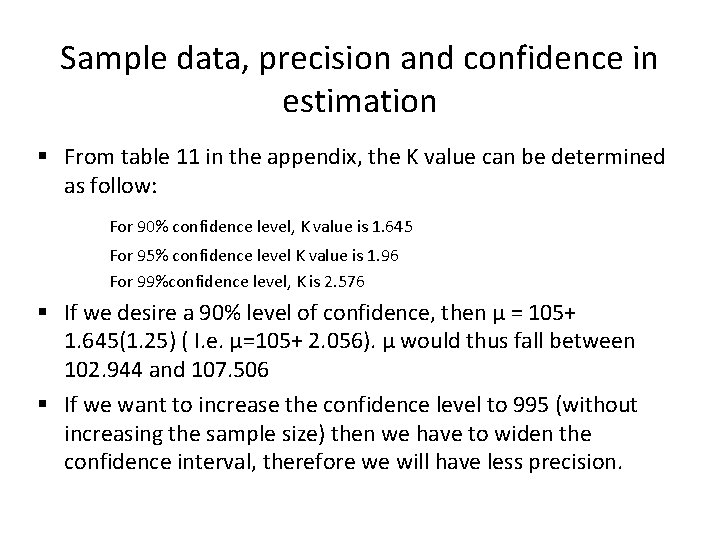
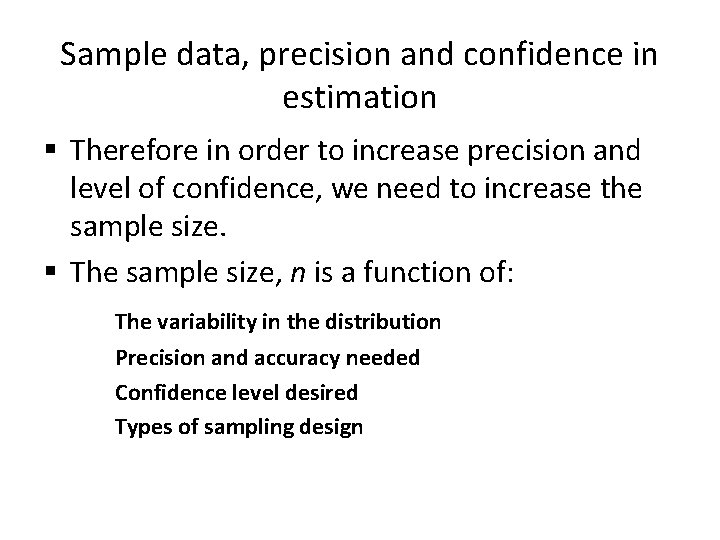
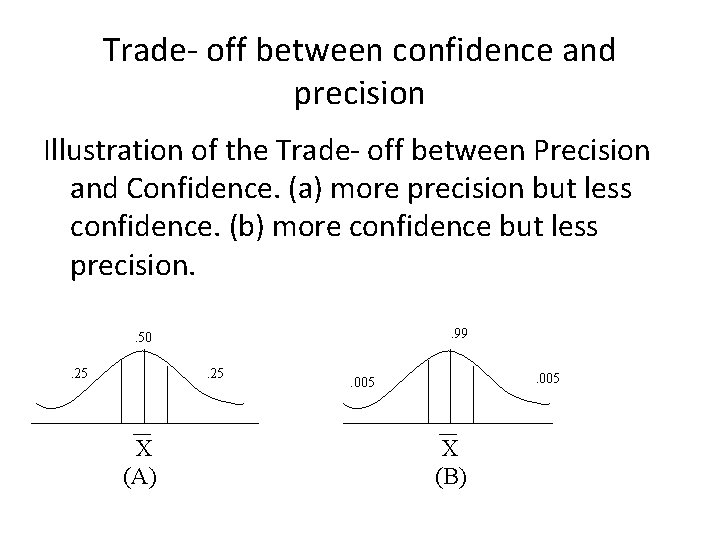
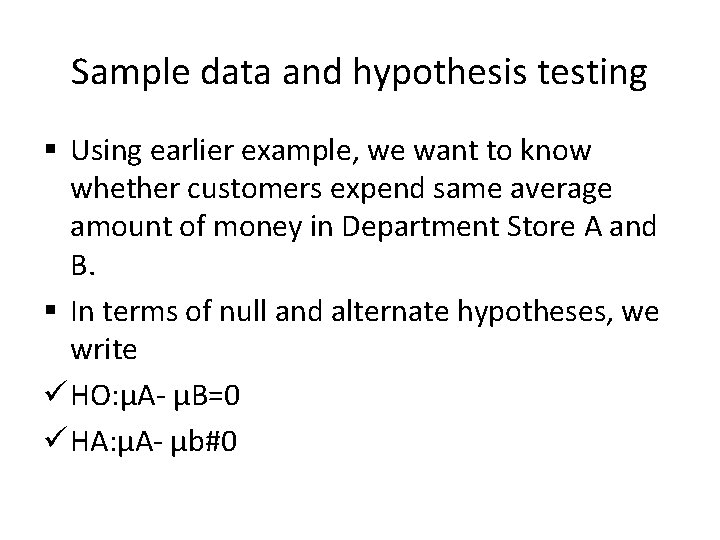
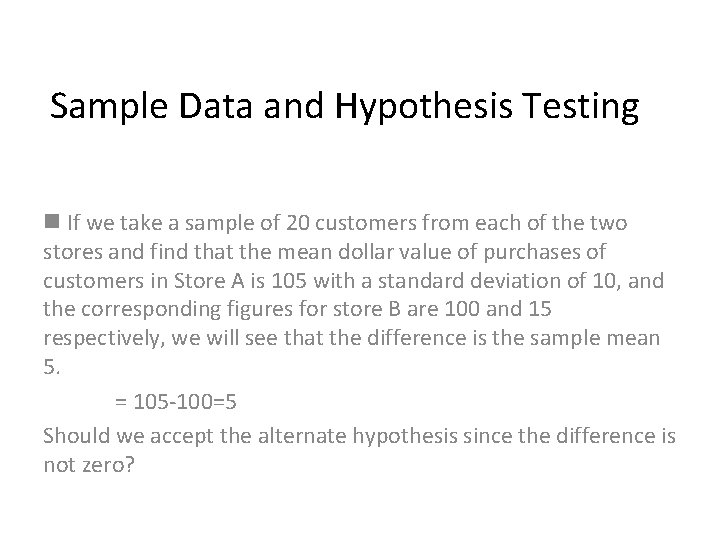
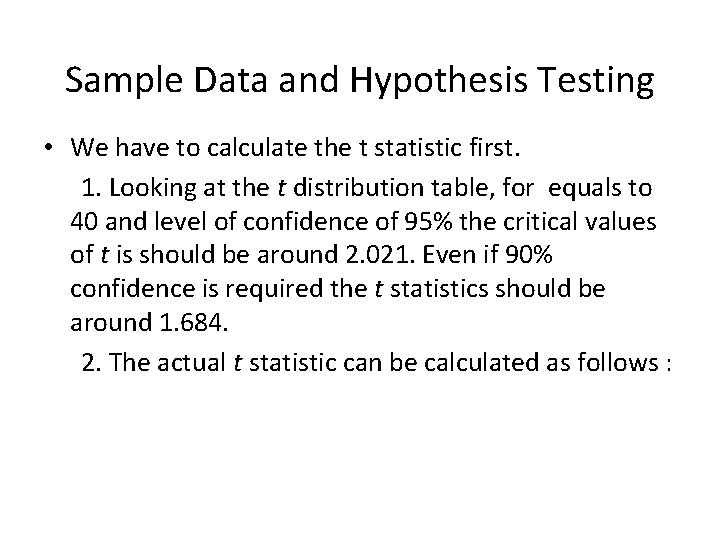
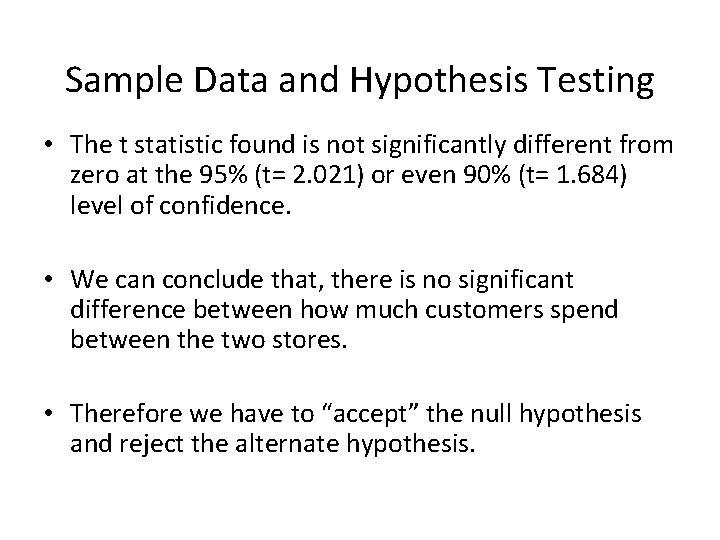
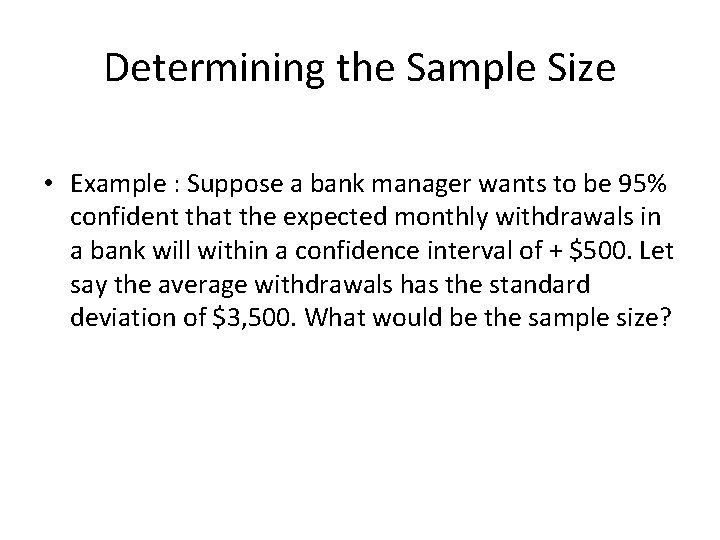
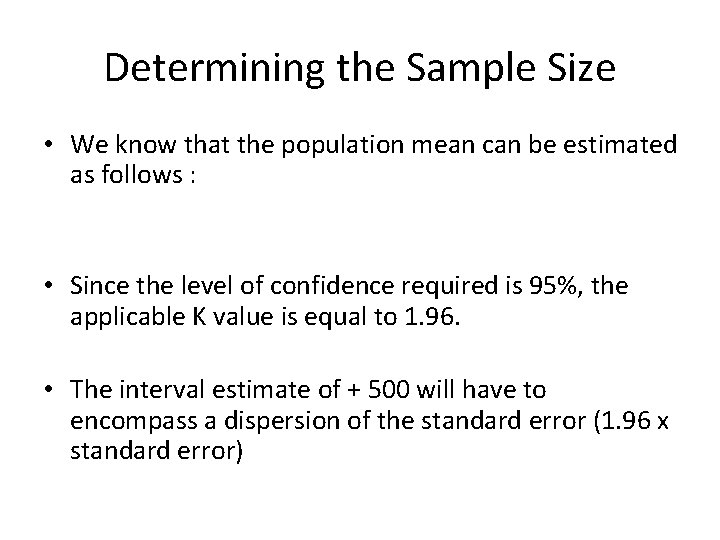
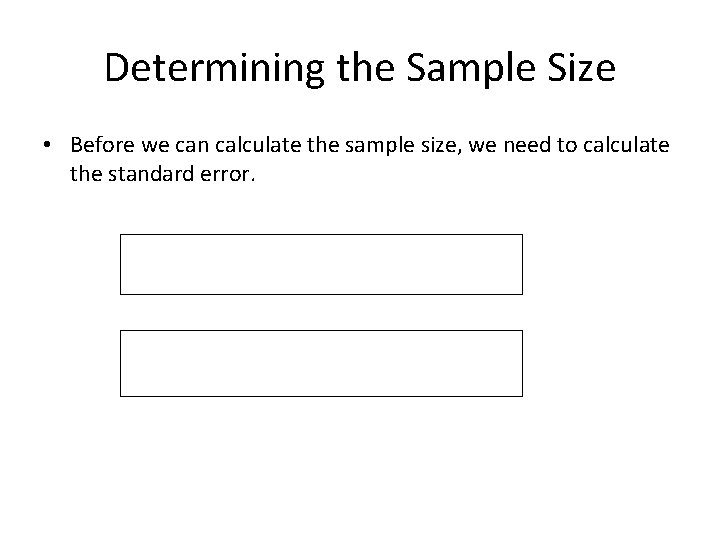
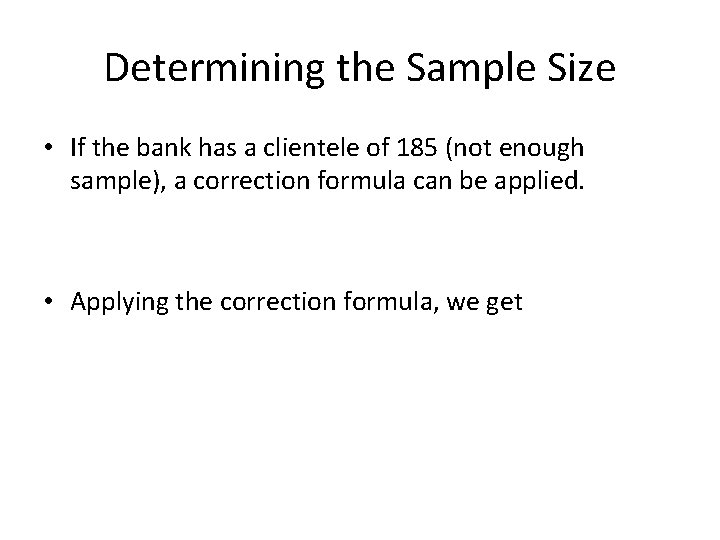
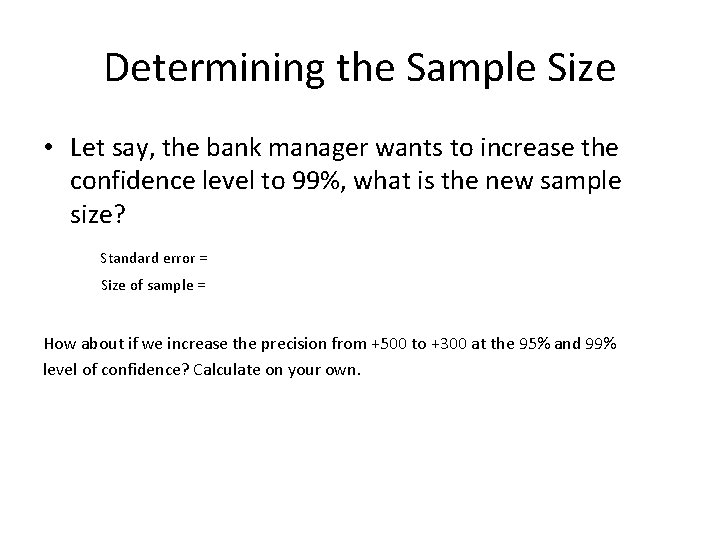
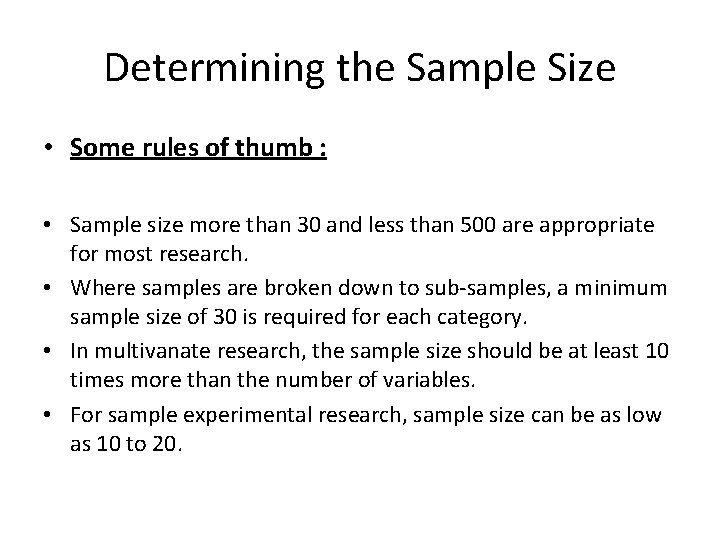
- Slides: 46
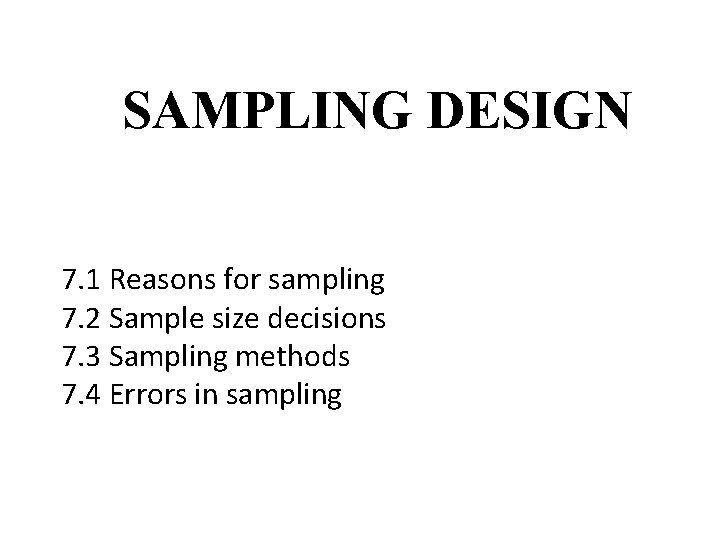
SAMPLING DESIGN 7. 1 Reasons for sampling 7. 2 Sample size decisions 7. 3 Sampling methods 7. 4 Errors in sampling
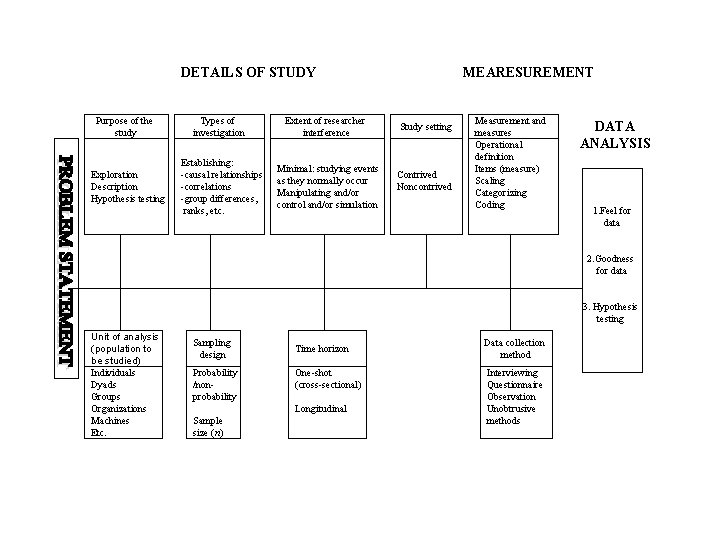
DETAILS OF STUDY Purpose of the study Exploration Description Hypothesis testing Types of investigation Establishing: -causal relationships -correlations -group differences, ranks, etc. Extent of researcher interference Minimal: studying events as they normally occur Manipulating and/or control and/or simulation MEARESUREMENT Study setting Contrived Noncontrived Measurement and measures Operational definition Items (measure) Scaling Categorizing Coding DATA ANALYSIS 1. Feel for data 2. Goodness for data 3. Hypothesis testing Unit of analysis (population to be studied) Individuals Dyads Groups Organizations Machines Etc. Sampling design Probability /nonprobability Time horizon One-shot (cross-sectional) Longitudinal Sample size (n) Data collection method Interviewing Questionnaire Observation Unobtrusive methods
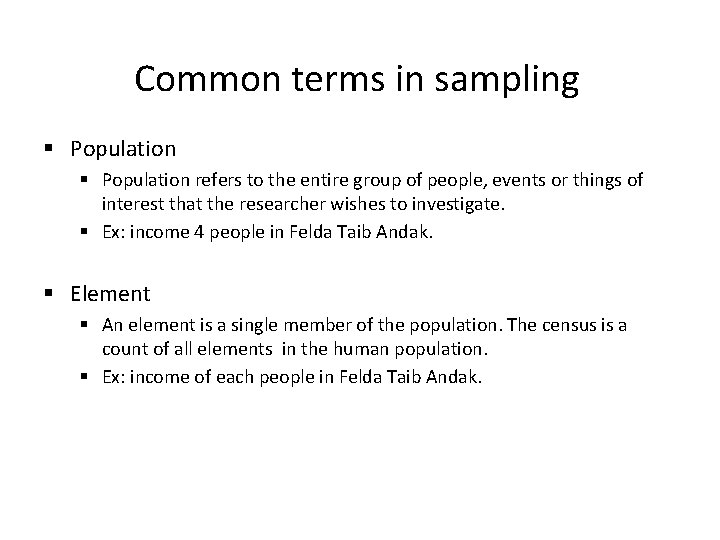
Common terms in sampling § Population refers to the entire group of people, events or things of interest that the researcher wishes to investigate. § Ex: income 4 people in Felda Taib Andak. § Element § An element is a single member of the population. The census is a count of all elements in the human population. § Ex: income of each people in Felda Taib Andak.
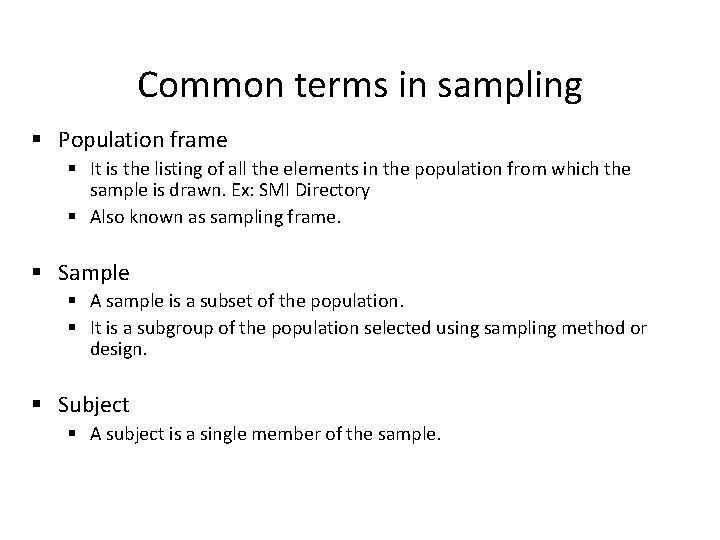
Common terms in sampling § Population frame § It is the listing of all the elements in the population from which the sample is drawn. Ex: SMI Directory § Also known as sampling frame. § Sample § A sample is a subset of the population. § It is a subgroup of the population selected using sampling method or design. § Subject § A subject is a single member of the sample.
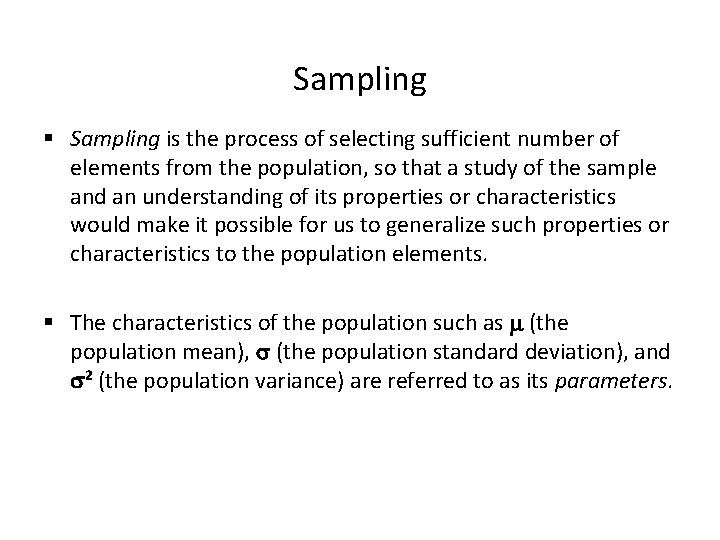
Sampling § Sampling is the process of selecting sufficient number of elements from the population, so that a study of the sample and an understanding of its properties or characteristics would make it possible for us to generalize such properties or characteristics to the population elements. § The characteristics of the population such as (the population mean), (the population standard deviation), and ² (the population variance) are referred to as its parameters.
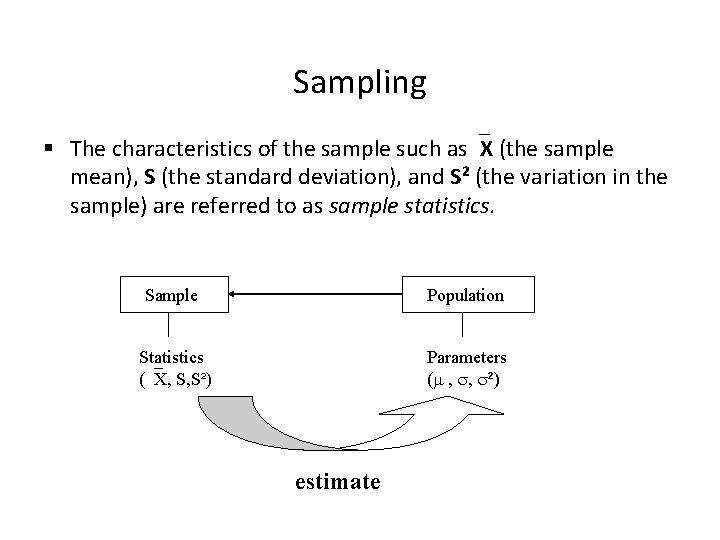
Sampling § The characteristics of the sample such as X (the sample mean), S (the standard deviation), and S² (the variation in the sample) are referred to as sample statistics. Sample Population Statistics ( X, S, S²) Parameters ( , , ²) estimate
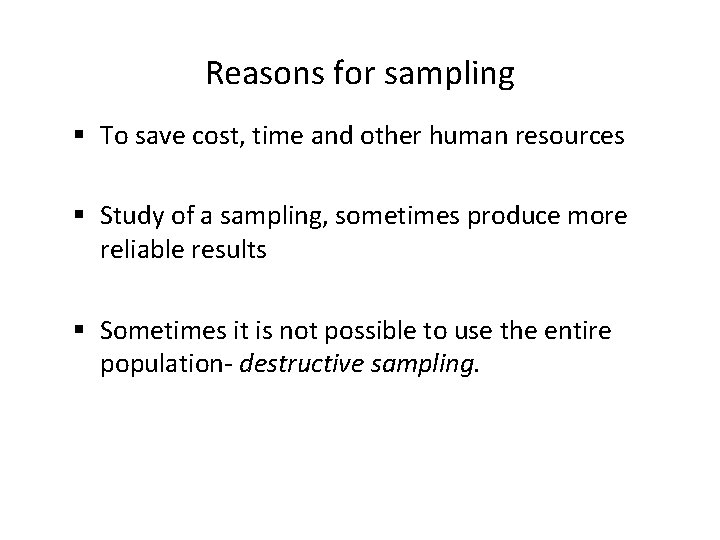
Reasons for sampling § To save cost, time and other human resources § Study of a sampling, sometimes produce more reliable results § Sometimes it is not possible to use the entire population- destructive sampling.
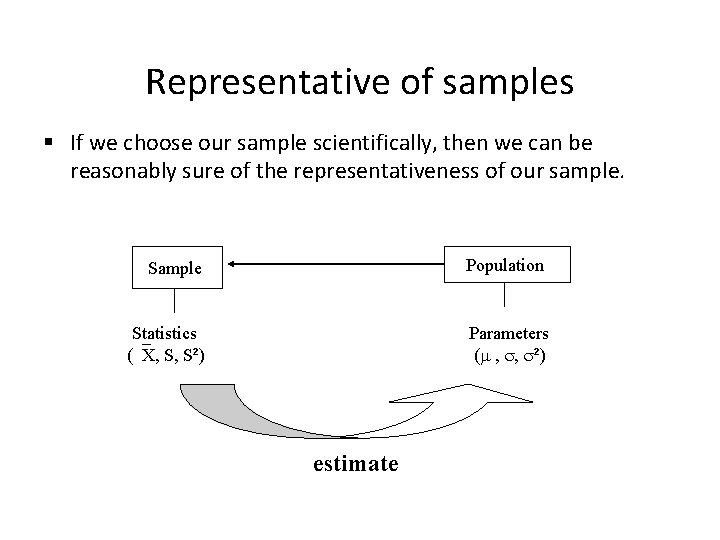
Representative of samples § If we choose our sample scientifically, then we can be reasonably sure of the representativeness of our sample. Sample Population Statistics ( X, S, S²) Parameters ( , , ²) estimate
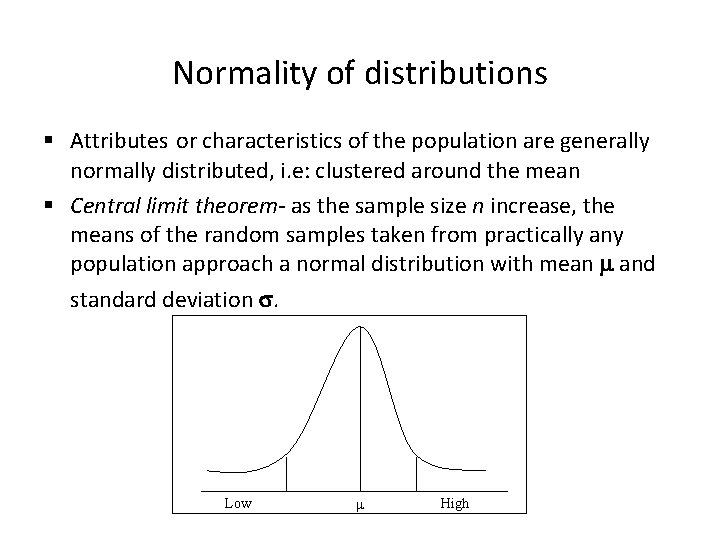
Normality of distributions § Attributes or characteristics of the population are generally normally distributed, i. e: clustered around the mean § Central limit theorem- as the sample size n increase, the means of the random samples taken from practically any population approach a normal distribution with mean and standard deviation . Low High
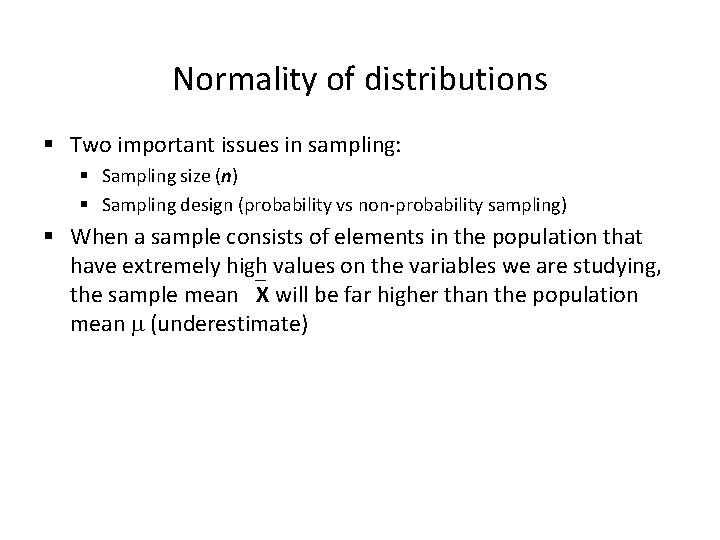
Normality of distributions § Two important issues in sampling: § Sampling size (n) § Sampling design (probability vs non-probability sampling) § When a sample consists of elements in the population that have extremely high values on the variables we are studying, the sample mean X will be far higher than the population mean (underestimate)
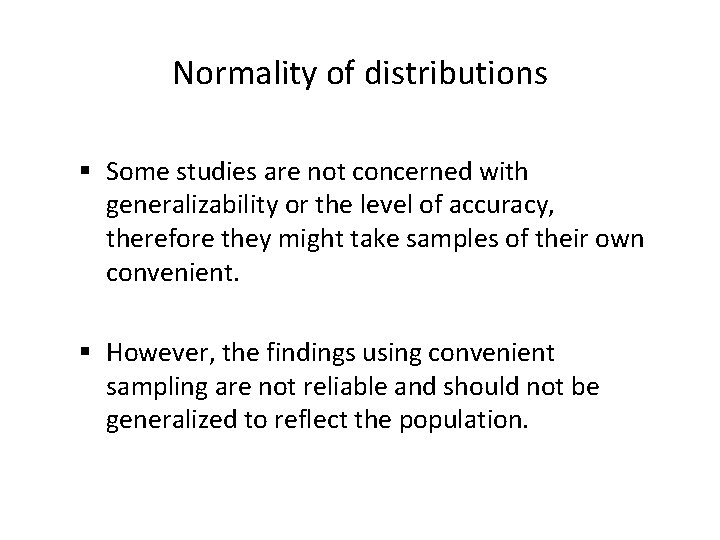
Normality of distributions § Some studies are not concerned with generalizability or the level of accuracy, therefore they might take samples of their own convenient. § However, the findings using convenient sampling are not reliable and should not be generalized to reflect the population.
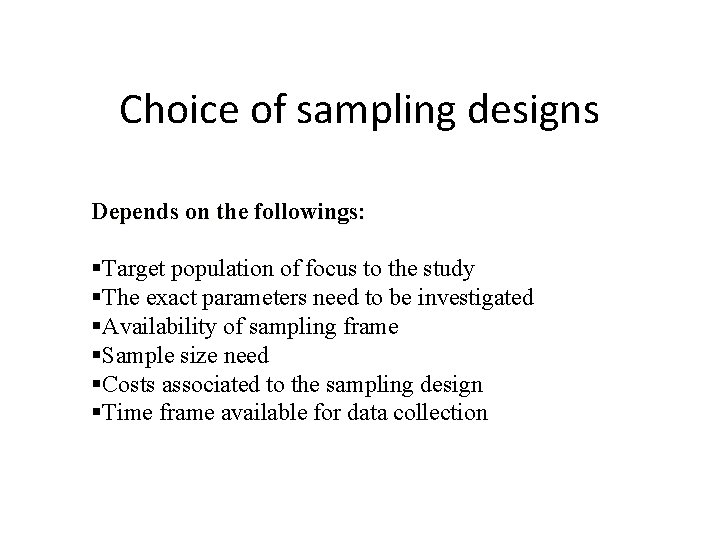
Choice of sampling designs Depends on the followings: §Target population of focus to the study §The exact parameters need to be investigated §Availability of sampling frame §Sample size need §Costs associated to the sampling design §Time frame available for data collection
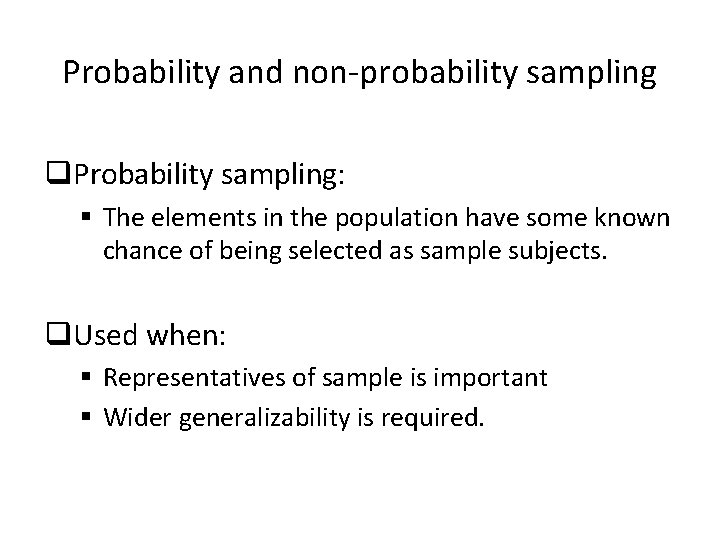
Probability and non-probability sampling q. Probability sampling: § The elements in the population have some known chance of being selected as sample subjects. q. Used when: § Representatives of sample is important § Wider generalizability is required.
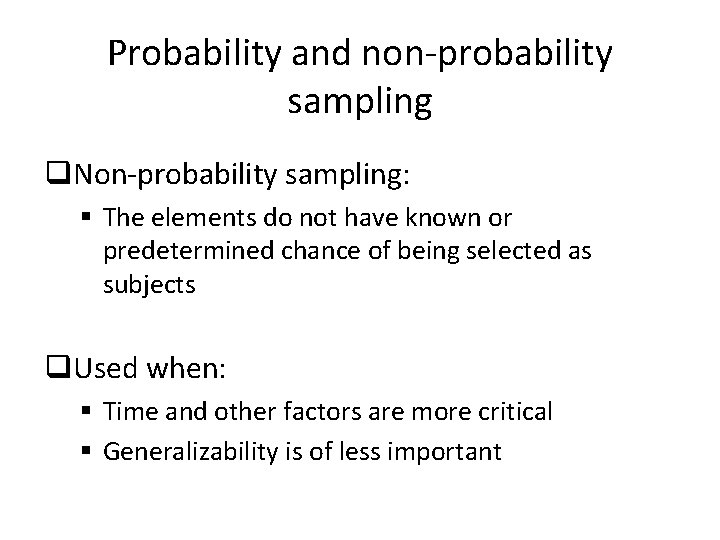
Probability and non-probability sampling q. Non-probability sampling: § The elements do not have known or predetermined chance of being selected as subjects q. Used when: § Time and other factors are more critical § Generalizability is of less important
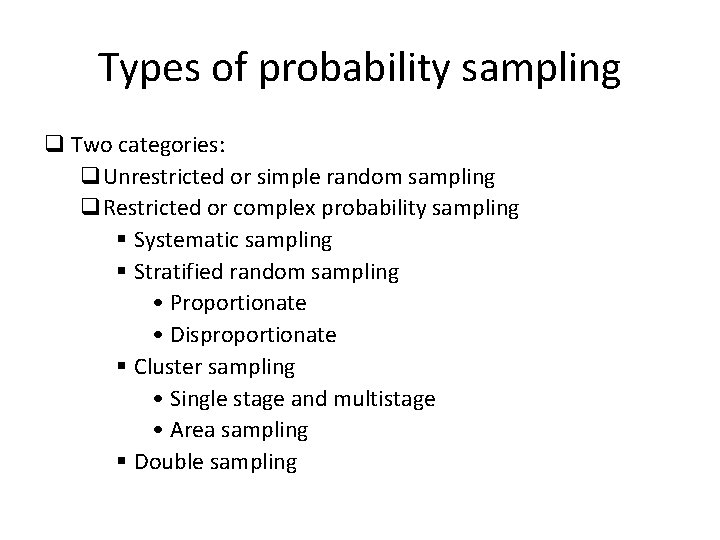
Types of probability sampling q Two categories: q. Unrestricted or simple random sampling q. Restricted or complex probability sampling § Systematic sampling § Stratified random sampling • Proportionate • Disproportionate § Cluster sampling • Single stage and multistage • Area sampling § Double sampling
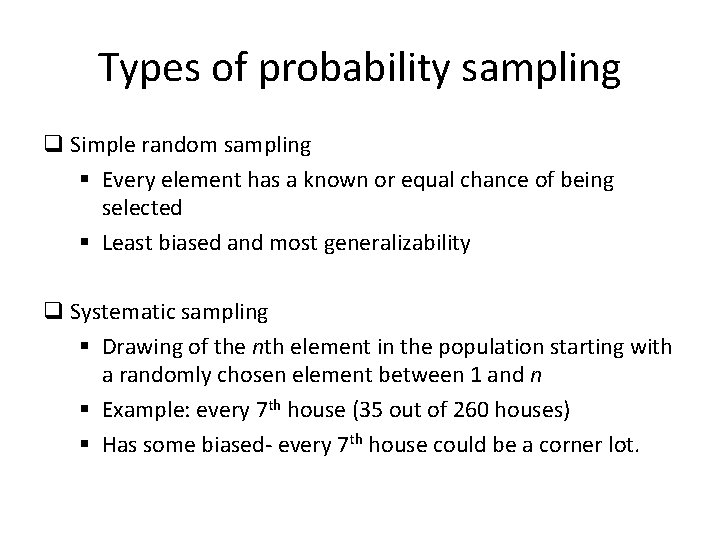
Types of probability sampling q Simple random sampling § Every element has a known or equal chance of being selected § Least biased and most generalizability q Systematic sampling § Drawing of the nth element in the population starting with a randomly chosen element between 1 and n § Example: every 7 th house (35 out of 260 houses) § Has some biased- every 7 th house could be a corner lot.
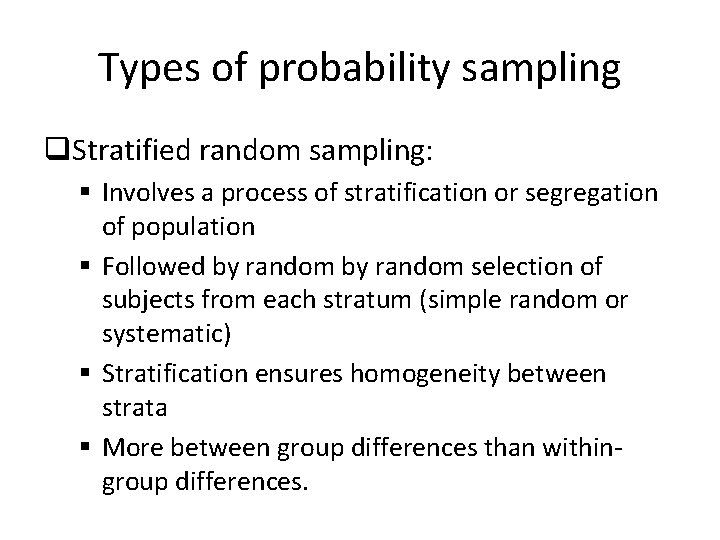
Types of probability sampling q. Stratified random sampling: § Involves a process of stratification or segregation of population § Followed by random selection of subjects from each stratum (simple random or systematic) § Stratification ensures homogeneity between strata § More between group differences than withingroup differences.
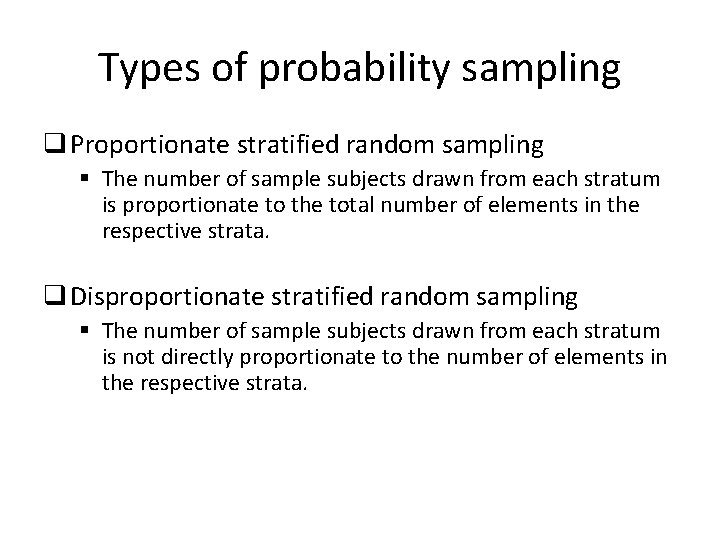
Types of probability sampling q Proportionate stratified random sampling § The number of sample subjects drawn from each stratum is proportionate to the total number of elements in the respective strata. q Disproportionate stratified random sampling § The number of sample subjects drawn from each stratum is not directly proportionate to the number of elements in the respective strata.
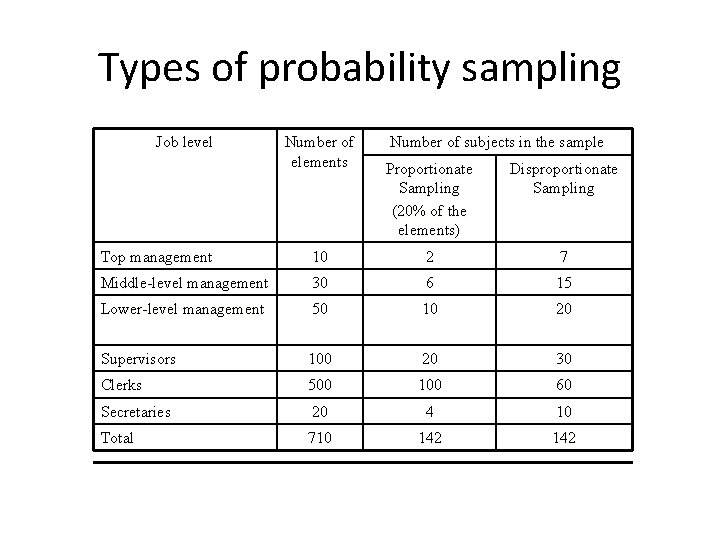
Types of probability sampling Job level Number of elements Number of subjects in the sample Proportionate Sampling (20% of the elements) Disproportionate Sampling Top management 10 2 7 Middle-level management 30 6 15 Lower-level management 50 10 20 Supervisors 100 20 30 Clerks 500 100 60 Secretaries 20 4 10 Total 710 142
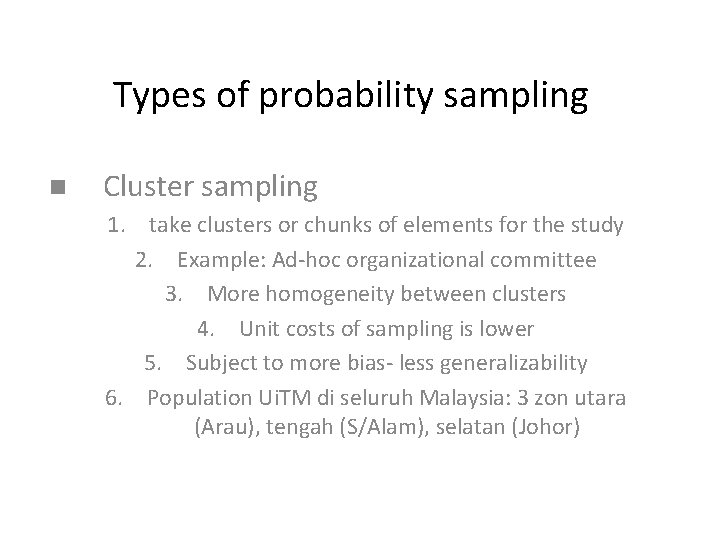
Types of probability sampling n Cluster sampling 1. take clusters or chunks of elements for the study 2. Example: Ad-hoc organizational committee 3. More homogeneity between clusters 4. Unit costs of sampling is lower 5. Subject to more bias- less generalizability 6. Population Ui. TM di seluruh Malaysia: 3 zon utara (Arau), tengah (S/Alam), selatan (Johor)
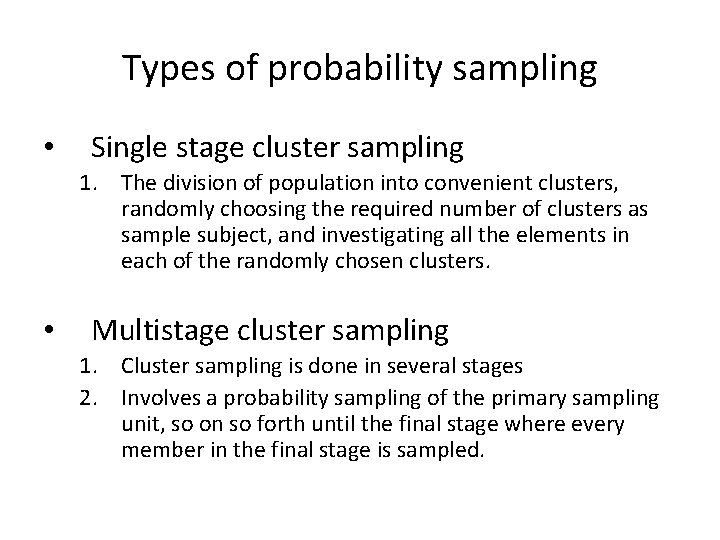
Types of probability sampling • Single stage cluster sampling 1. The division of population into convenient clusters, randomly choosing the required number of clusters as sample subject, and investigating all the elements in each of the randomly chosen clusters. • Multistage cluster sampling 1. Cluster sampling is done in several stages 2. Involves a probability sampling of the primary sampling unit, so on so forth until the final stage where every member in the final stage is sampled.
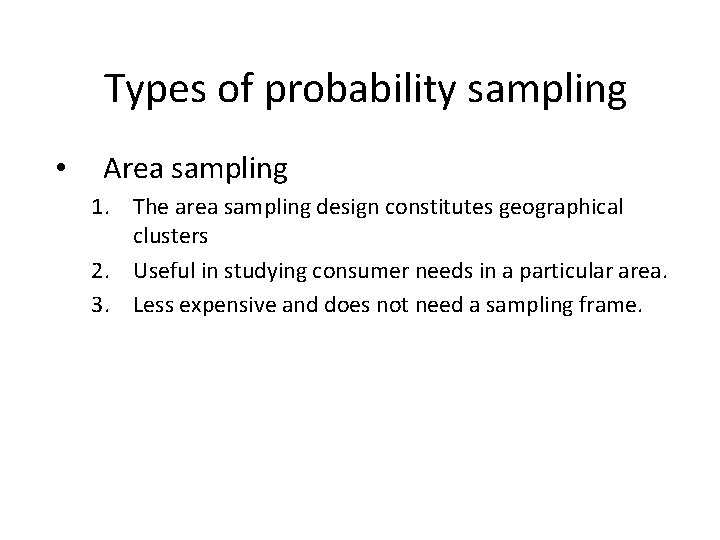
Types of probability sampling • Area sampling 1. The area sampling design constitutes geographical clusters 2. Useful in studying consumer needs in a particular area. 3. Less expensive and does not need a sampling frame.
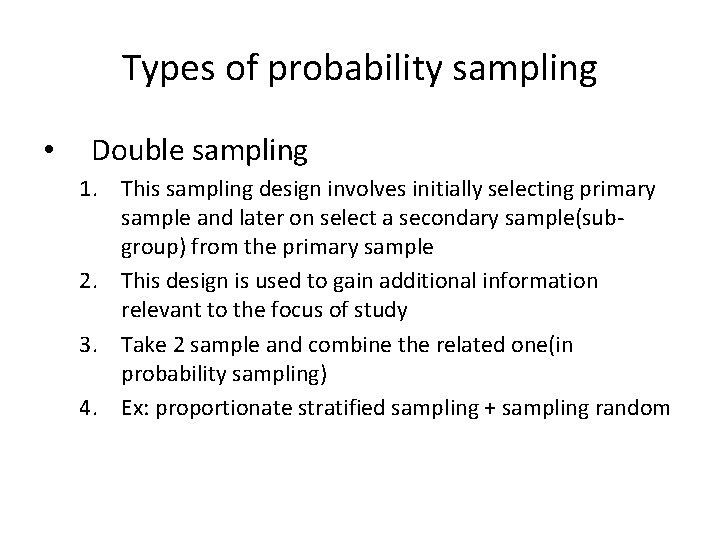
Types of probability sampling • Double sampling 1. This sampling design involves initially selecting primary sample and later on select a secondary sample(subgroup) from the primary sample 2. This design is used to gain additional information relevant to the focus of study 3. Take 2 sample and combine the related one(in probability sampling) 4. Ex: proportionate stratified sampling + sampling random
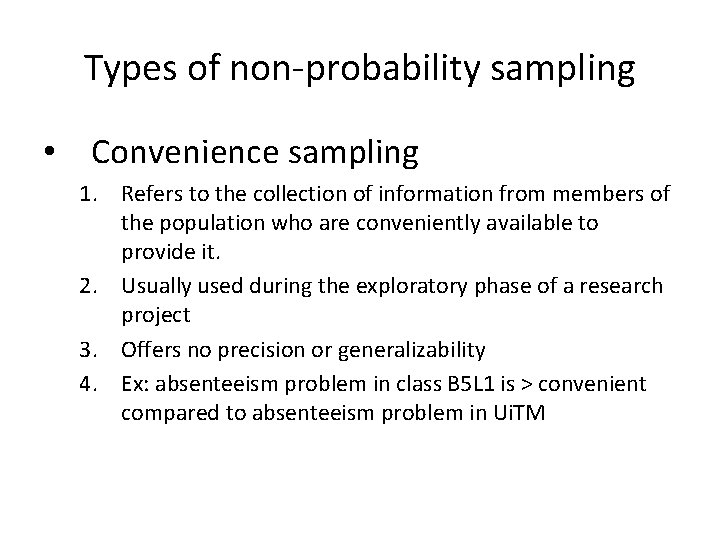
Types of non-probability sampling • Convenience sampling 1. Refers to the collection of information from members of the population who are conveniently available to provide it. 2. Usually used during the exploratory phase of a research project 3. Offers no precision or generalizability 4. Ex: absenteeism problem in class B 5 L 1 is > convenient compared to absenteeism problem in Ui. TM
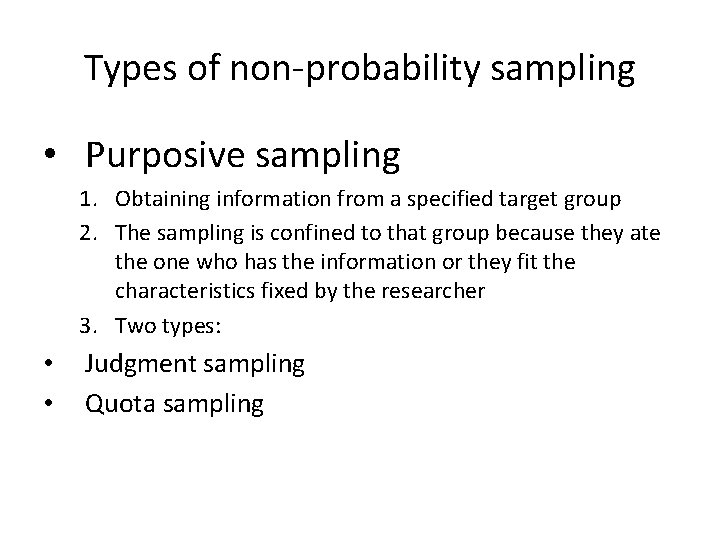
Types of non-probability sampling • Purposive sampling 1. Obtaining information from a specified target group 2. The sampling is confined to that group because they ate the one who has the information or they fit the characteristics fixed by the researcher 3. Two types: • • Judgment sampling Quota sampling
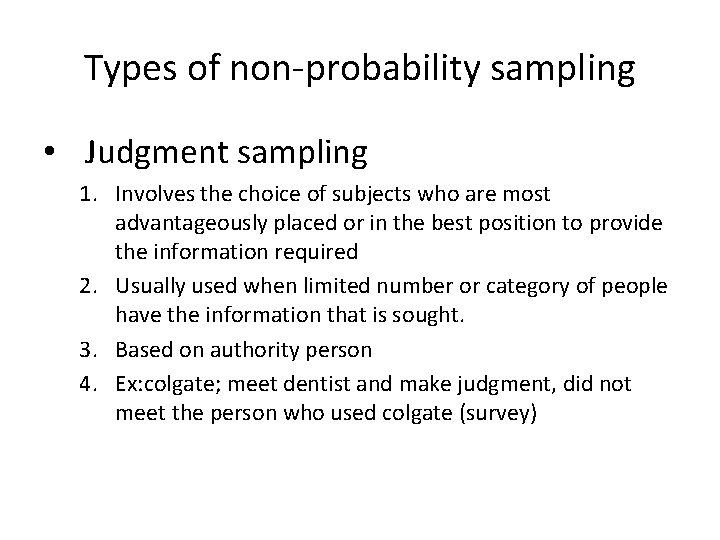
Types of non-probability sampling • Judgment sampling 1. Involves the choice of subjects who are most advantageously placed or in the best position to provide the information required 2. Usually used when limited number or category of people have the information that is sought. 3. Based on authority person 4. Ex: colgate; meet dentist and make judgment, did not meet the person who used colgate (survey)
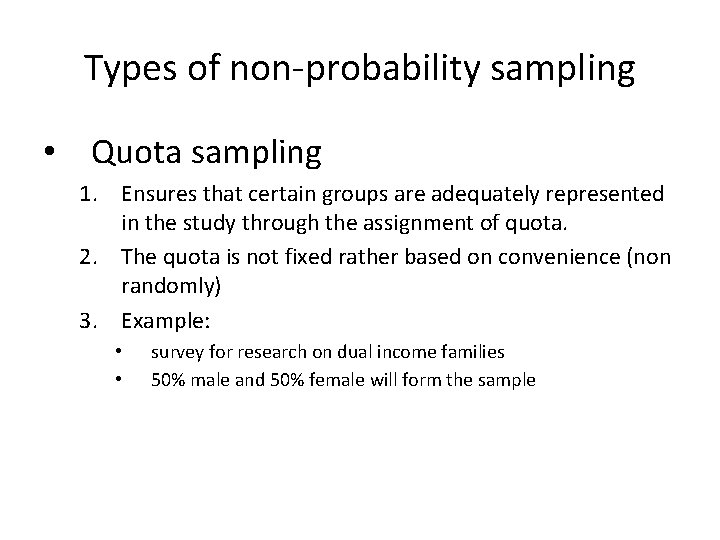
Types of non-probability sampling • Quota sampling 1. Ensures that certain groups are adequately represented in the study through the assignment of quota. 2. The quota is not fixed rather based on convenience (non randomly) 3. Example: • • survey for research on dual income families 50% male and 50% female will form the sample
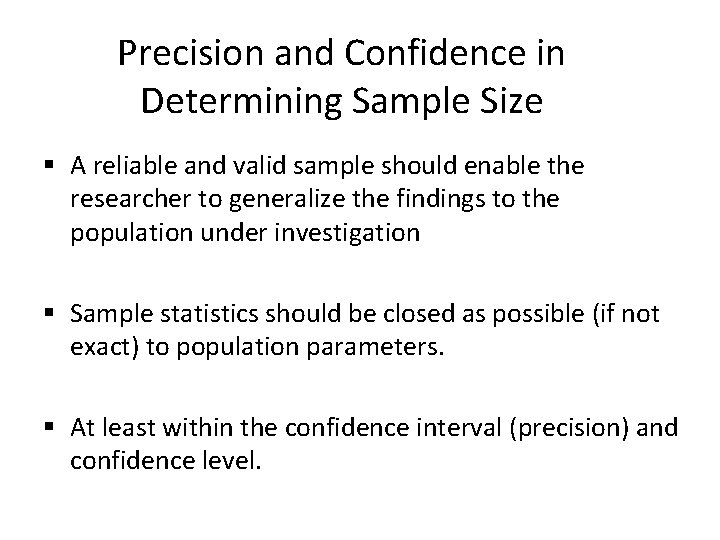
Precision and Confidence in Determining Sample Size § A reliable and valid sample should enable the researcher to generalize the findings to the population under investigation § Sample statistics should be closed as possible (if not exact) to population parameters. § At least within the confidence interval (precision) and confidence level.
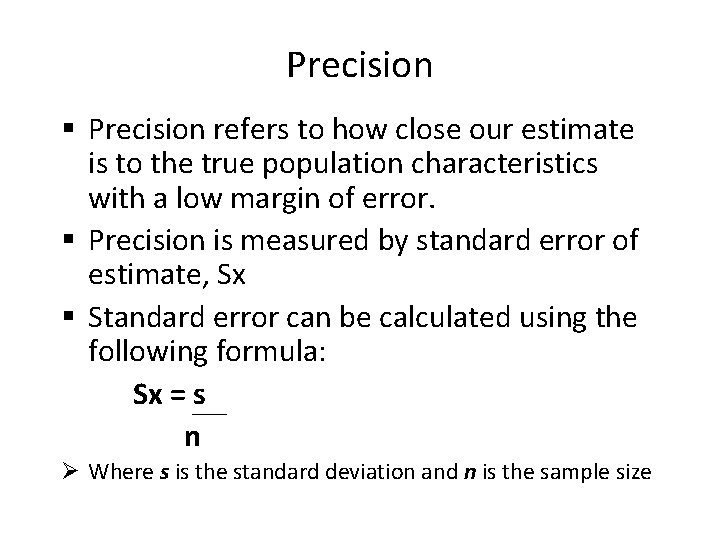
Precision § Precision refers to how close our estimate is to the true population characteristics with a low margin of error. § Precision is measured by standard error of estimate, Sx § Standard error can be calculated using the following formula: Sx = s n Ø Where s is the standard deviation and n is the sample size
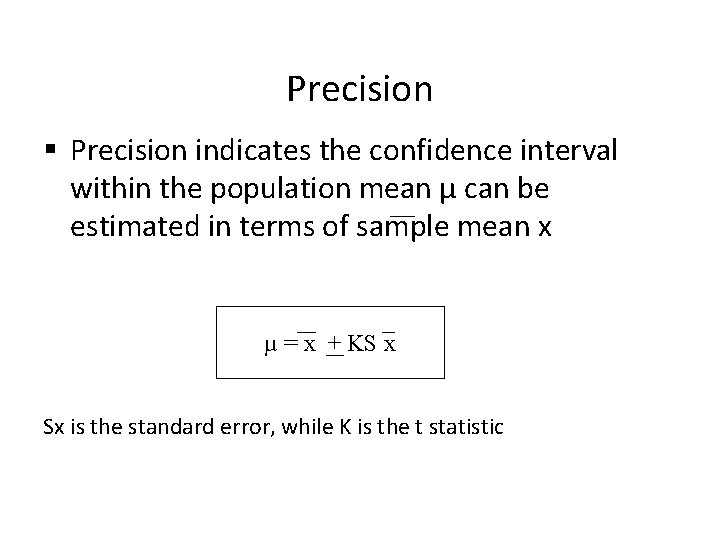
Precision § Precision indicates the confidence interval within the population mean µ can be estimated in terms of sample mean x µ = x + KS x Sx is the standard error, while K is the t statistic
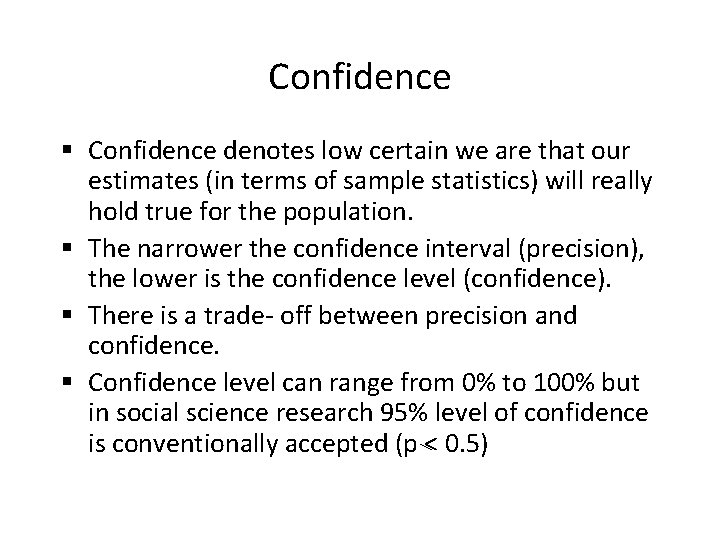
Confidence § Confidence denotes low certain we are that our estimates (in terms of sample statistics) will really hold true for the population. § The narrower the confidence interval (precision), the lower is the confidence level (confidence). § There is a trade- off between precision and confidence. § Confidence level can range from 0% to 100% but in social science research 95% level of confidence is conventionally accepted (p < 0. 5)
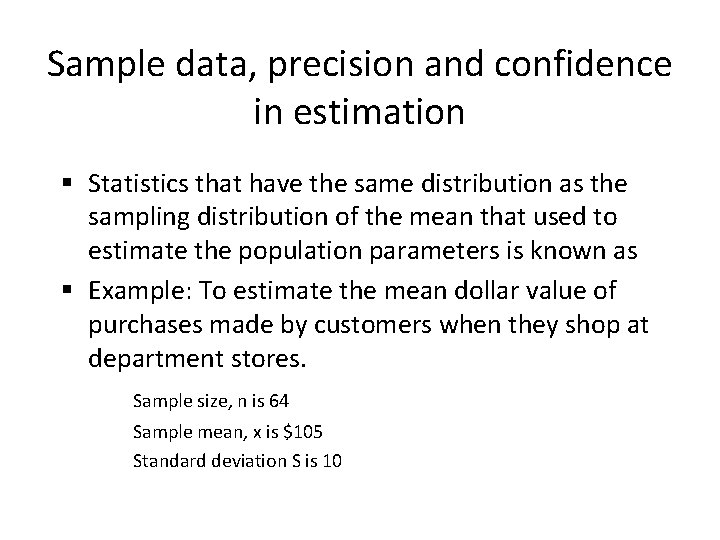
Sample data, precision and confidence in estimation § Statistics that have the same distribution as the sampling distribution of the mean that used to estimate the population parameters is known as § Example: To estimate the mean dollar value of purchases made by customers when they shop at department stores. Sample size, n is 64 Sample mean, x is $105 Standard deviation S is 10
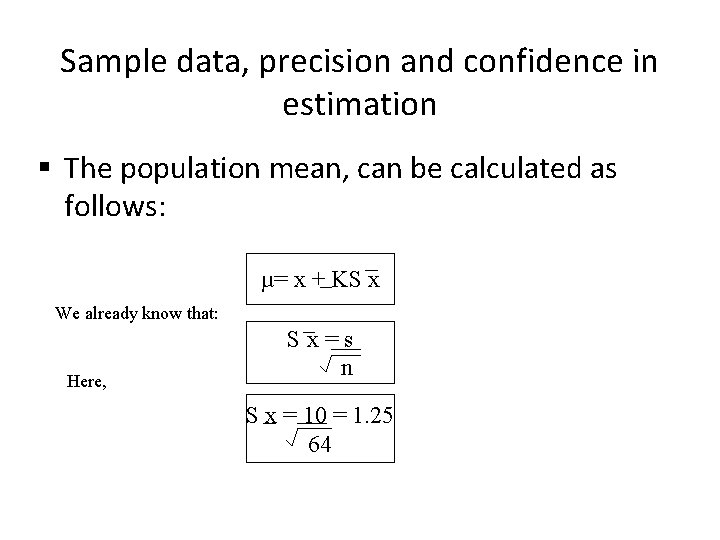
Sample data, precision and confidence in estimation § The population mean, can be calculated as follows: µ= x + KS x We already know that: Here, Sx=s n S x = 10 = 1. 25 64
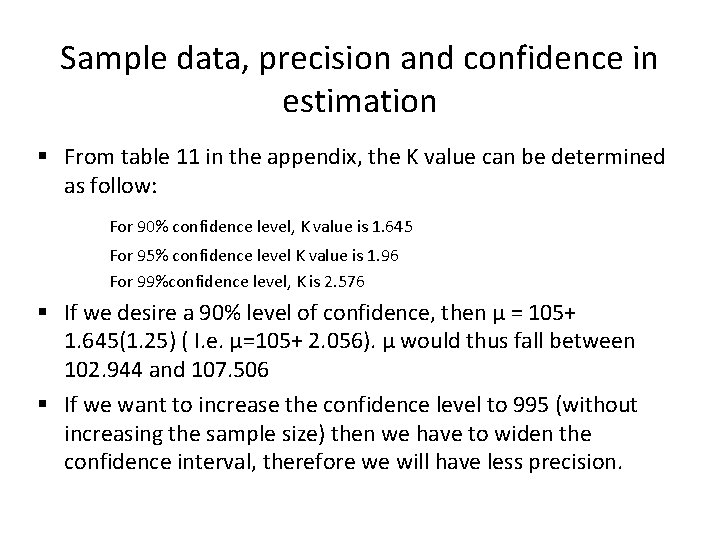
Sample data, precision and confidence in estimation § From table 11 in the appendix, the K value can be determined as follow: For 90% confidence level, K value is 1. 645 For 95% confidence level K value is 1. 96 For 99%confidence level, K is 2. 576 § If we desire a 90% level of confidence, then µ = 105+ 1. 645(1. 25) ( I. e. µ=105+ 2. 056). µ would thus fall between 102. 944 and 107. 506 § If we want to increase the confidence level to 995 (without increasing the sample size) then we have to widen the confidence interval, therefore we will have less precision.
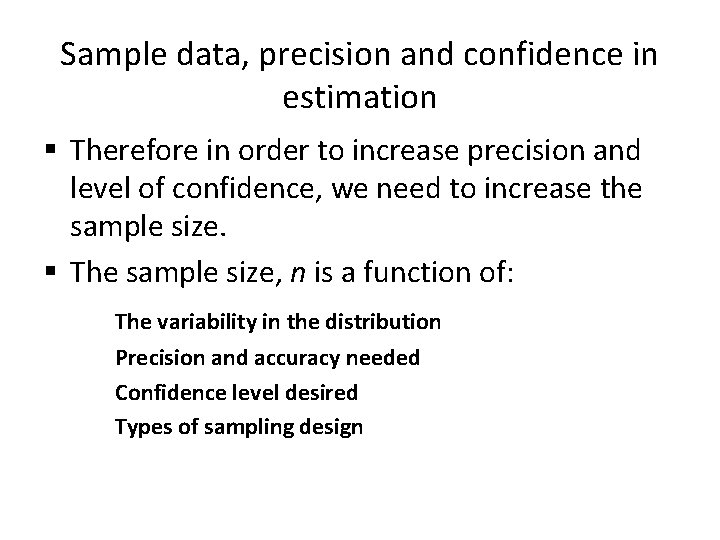
Sample data, precision and confidence in estimation § Therefore in order to increase precision and level of confidence, we need to increase the sample size. § The sample size, n is a function of: The variability in the distribution Precision and accuracy needed Confidence level desired Types of sampling design
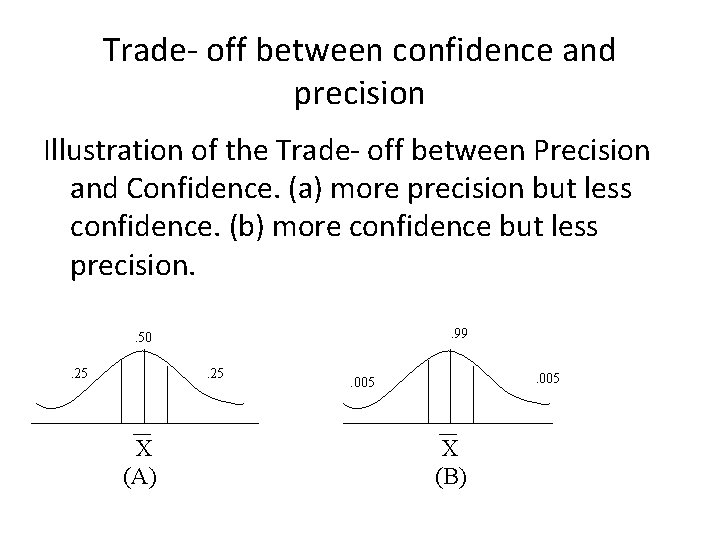
Trade- off between confidence and precision Illustration of the Trade- off between Precision and Confidence. (a) more precision but less confidence. (b) more confidence but less precision. . 99 . 50. 25 X (A) . 005 X (B)
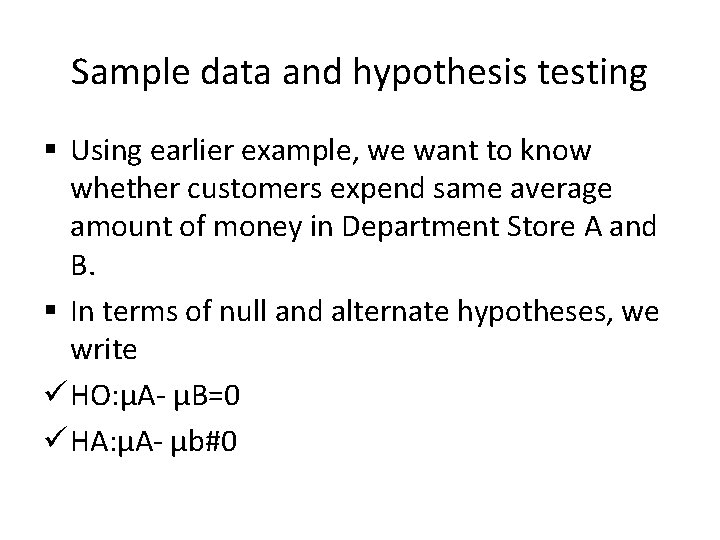
Sample data and hypothesis testing § Using earlier example, we want to know whether customers expend same average amount of money in Department Store A and B. § In terms of null and alternate hypotheses, we write ü HO: µA- µB=0 ü HA: µA- µb#0
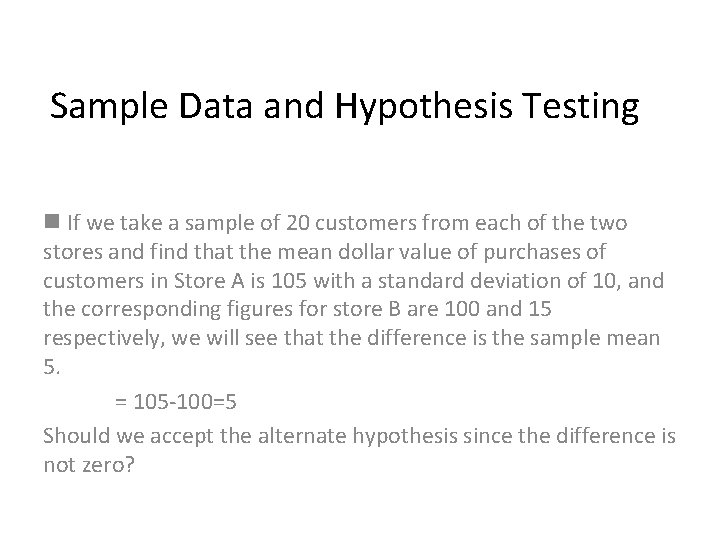
Sample Data and Hypothesis Testing n If we take a sample of 20 customers from each of the two stores and find that the mean dollar value of purchases of customers in Store A is 105 with a standard deviation of 10, and the corresponding figures for store B are 100 and 15 respectively, we will see that the difference is the sample mean 5. = 105 -100=5 Should we accept the alternate hypothesis since the difference is not zero?
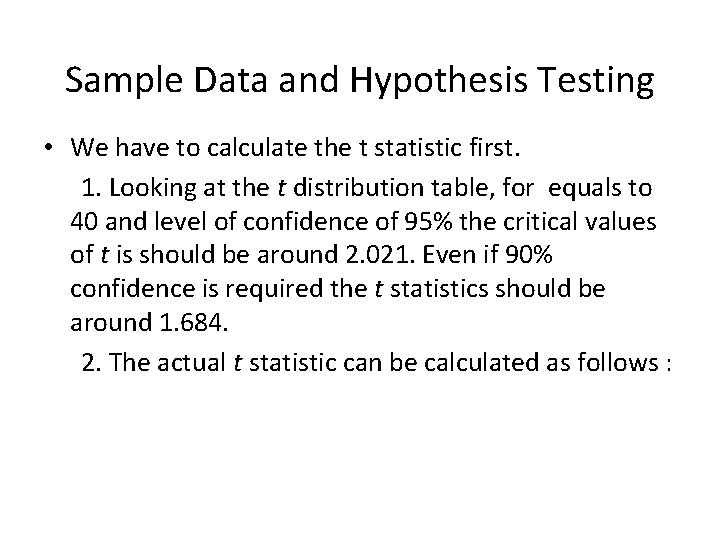
Sample Data and Hypothesis Testing • We have to calculate the t statistic first. 1. Looking at the t distribution table, for equals to 40 and level of confidence of 95% the critical values of t is should be around 2. 021. Even if 90% confidence is required the t statistics should be around 1. 684. 2. The actual t statistic can be calculated as follows :
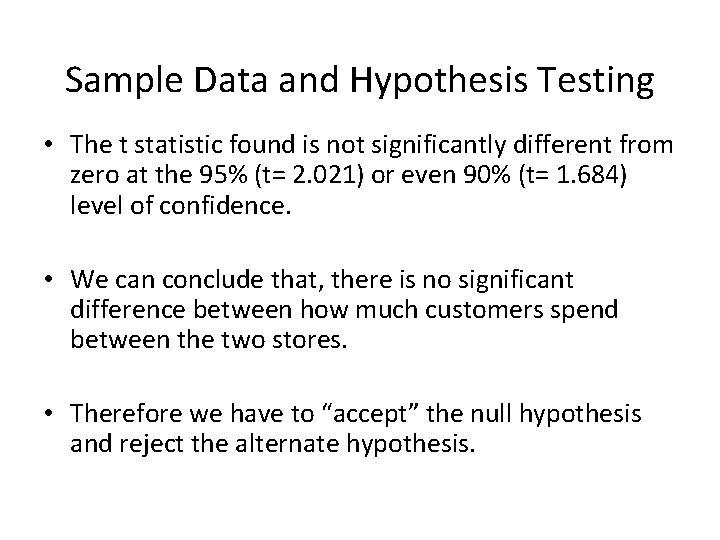
Sample Data and Hypothesis Testing • The t statistic found is not significantly different from zero at the 95% (t= 2. 021) or even 90% (t= 1. 684) level of confidence. • We can conclude that, there is no significant difference between how much customers spend between the two stores. • Therefore we have to “accept” the null hypothesis and reject the alternate hypothesis.
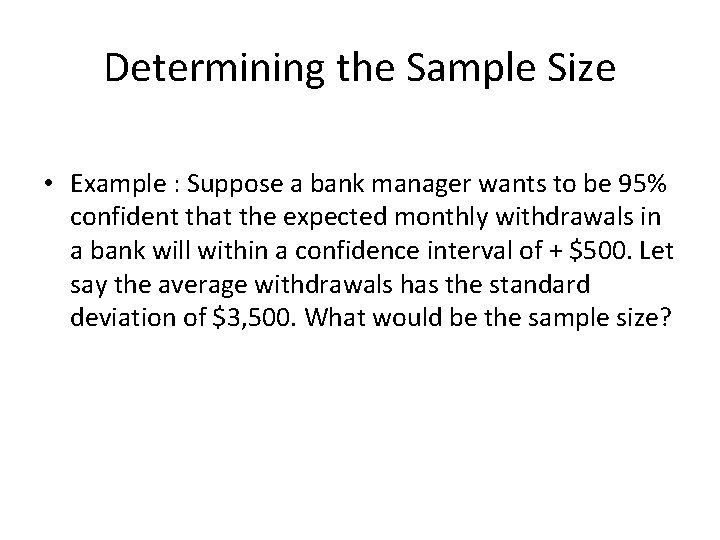
Determining the Sample Size • Example : Suppose a bank manager wants to be 95% confident that the expected monthly withdrawals in a bank will within a confidence interval of + $500. Let say the average withdrawals has the standard deviation of $3, 500. What would be the sample size?
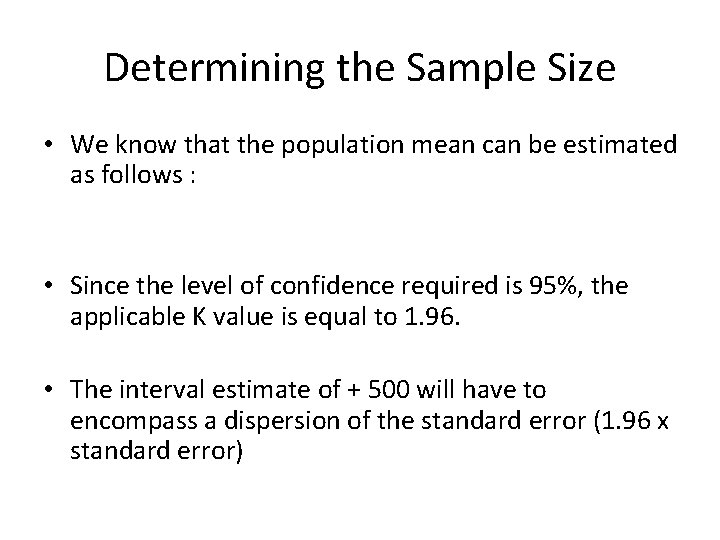
Determining the Sample Size • We know that the population mean can be estimated as follows : • Since the level of confidence required is 95%, the applicable K value is equal to 1. 96. • The interval estimate of + 500 will have to encompass a dispersion of the standard error (1. 96 x standard error)
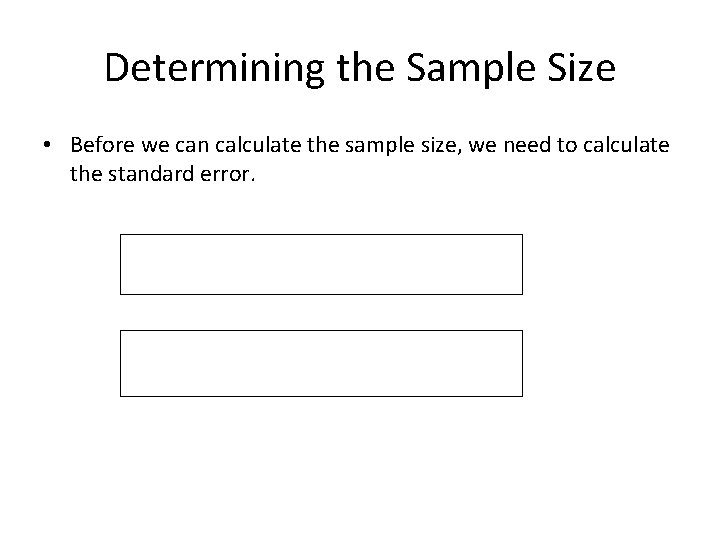
Determining the Sample Size • Before we can calculate the sample size, we need to calculate the standard error.
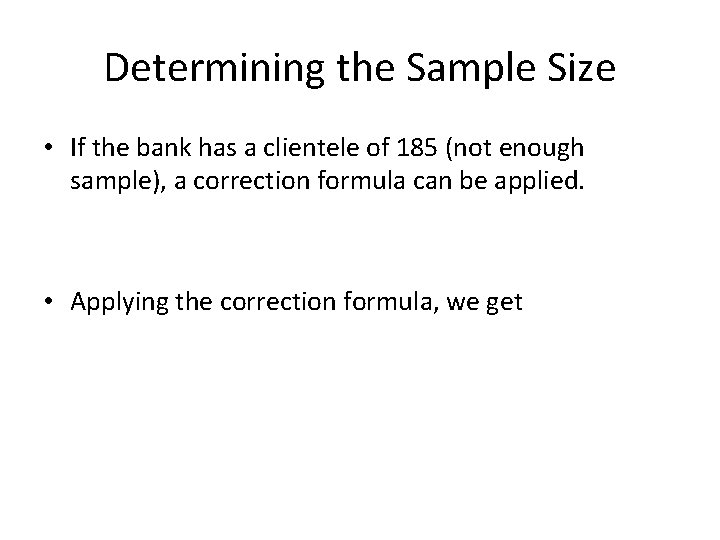
Determining the Sample Size • If the bank has a clientele of 185 (not enough sample), a correction formula can be applied. • Applying the correction formula, we get
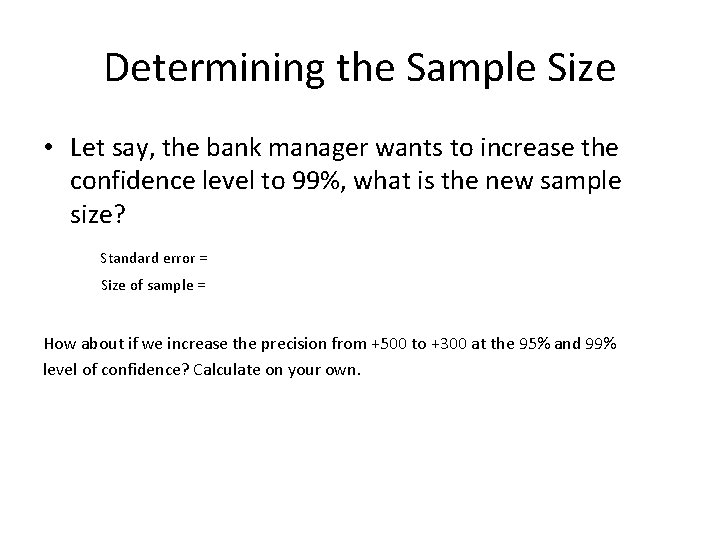
Determining the Sample Size • Let say, the bank manager wants to increase the confidence level to 99%, what is the new sample size? Standard error = Size of sample = How about if we increase the precision from +500 to +300 at the 95% and 99% level of confidence? Calculate on your own.
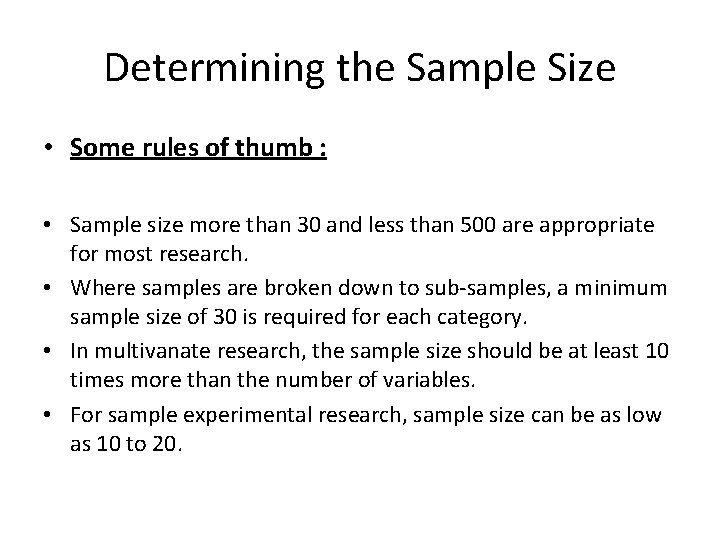
Determining the Sample Size • Some rules of thumb : • Sample size more than 30 and less than 500 are appropriate for most research. • Where samples are broken down to sub-samples, a minimum sample size of 30 is required for each category. • In multivanate research, the sample size should be at least 10 times more than the number of variables. • For sample experimental research, sample size can be as low as 10 to 20.
Sampling method in research
Limitations of systematic sampling
What is stratified sampling
Contoh observasi event sampling
Cluster sampling vs stratified sampling
Example of convenience sampling in statistics
Natural sampling vs flat top sampling
Iso 22301 utbildning
Typiska novell drag
Nationell inriktning för artificiell intelligens
Returpilarna
Varför kallas perioden 1918-1939 för mellankrigstiden
En lathund för arbete med kontinuitetshantering
Personalliggare bygg undantag
Vilotidsbok
A gastrica
Vad är densitet
Datorkunskap för nybörjare
Boverket ka
Debattartikel struktur
Magnetsjukhus
Nyckelkompetenser för livslångt lärande
Påbyggnader för flakfordon
Formel för lufttryck
Svenskt ramverk för digital samverkan
Lyckans minut erik lindorm analys
Presentera för publik crossboss
Teckenspråk minoritetsspråk argument
Kanaans land
Treserva lathund
Fimbrietratt
Claes martinsson
Cks
Byggprocessen steg för steg
Mat för idrottare
Verktyg för automatisering av utbetalningar
Rutin för avvikelsehantering
Smärtskolan kunskap för livet
Ministerstyre för och nackdelar
Tack för att ni har lyssnat
Referat mall
Redogör för vad psykologi är
Stål för stötfångarsystem
Atmosfr
Borra hål för knoppar
Vilken grundregel finns det för tronföljden i sverige?
Fr formel