Judea Pearlprofessor of Computer Science and Statistics and
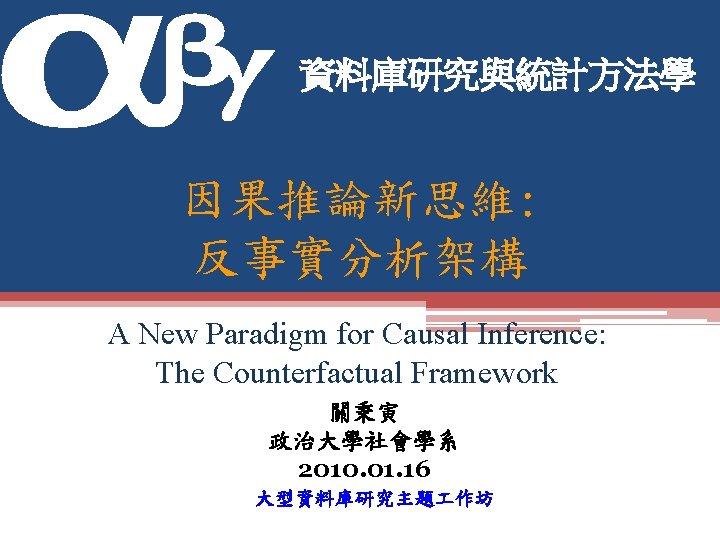
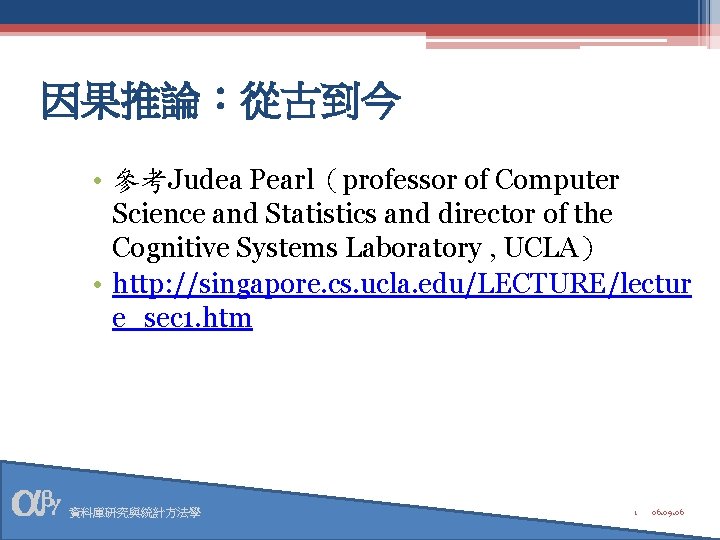
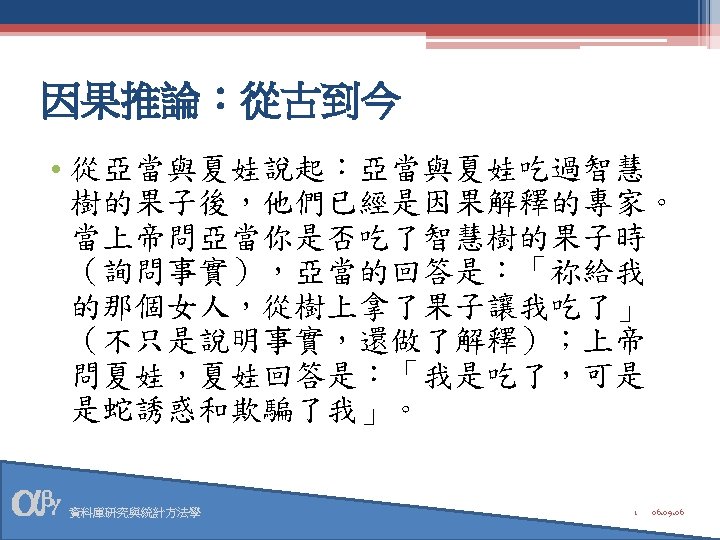
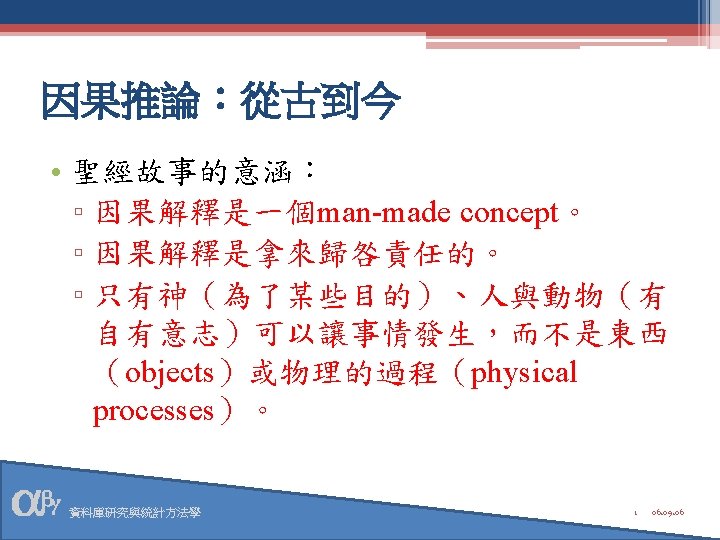
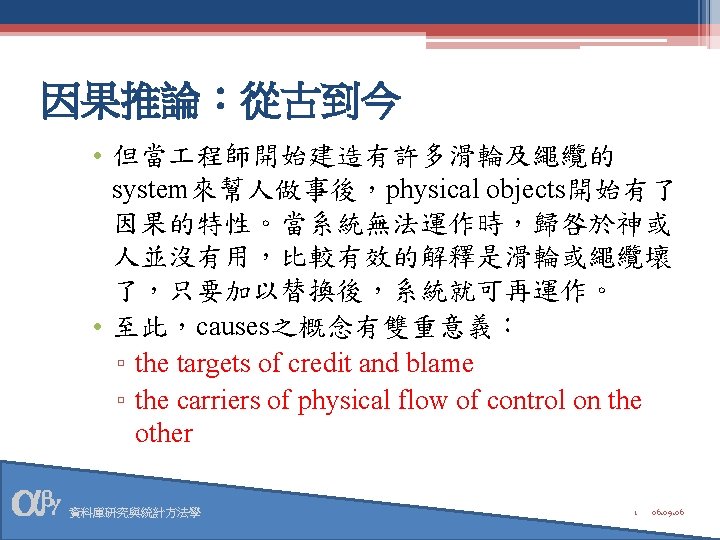
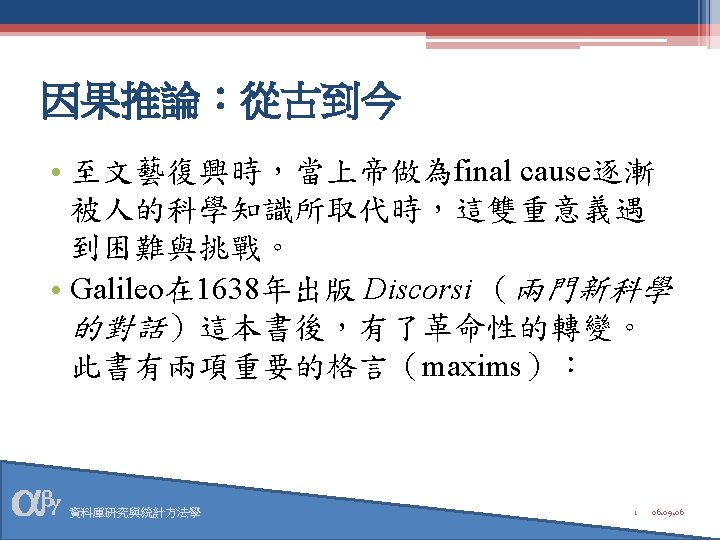
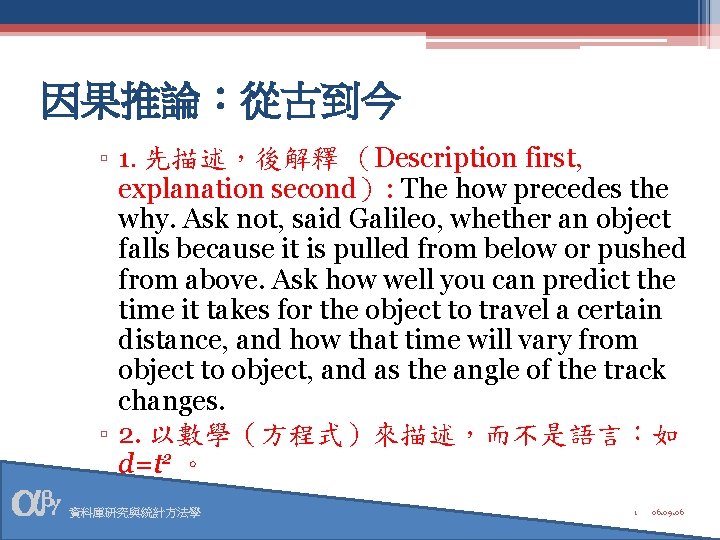
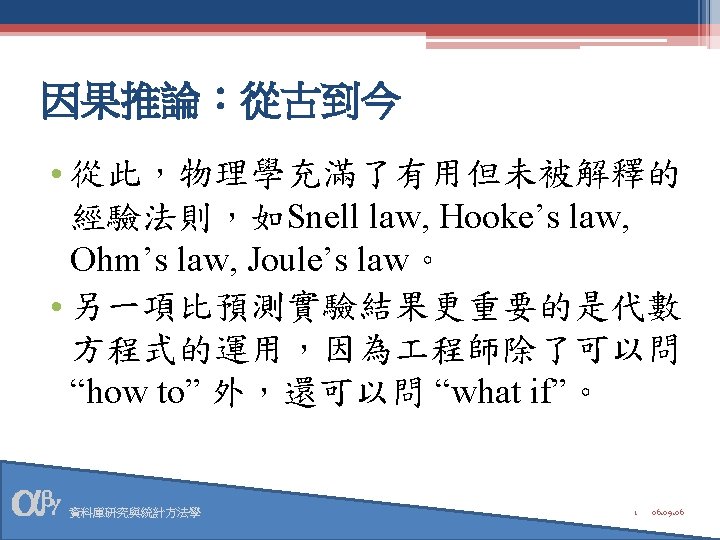
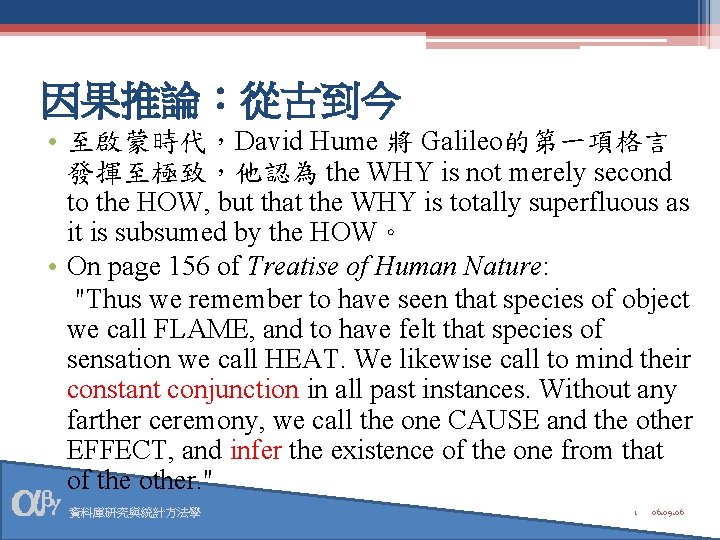
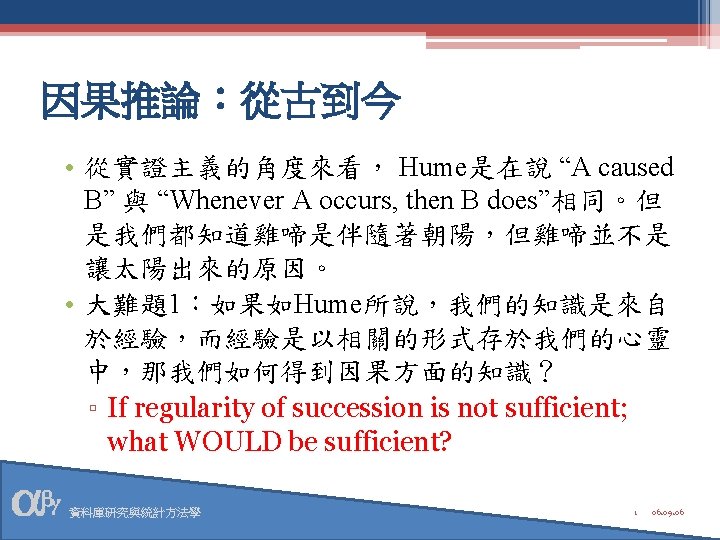
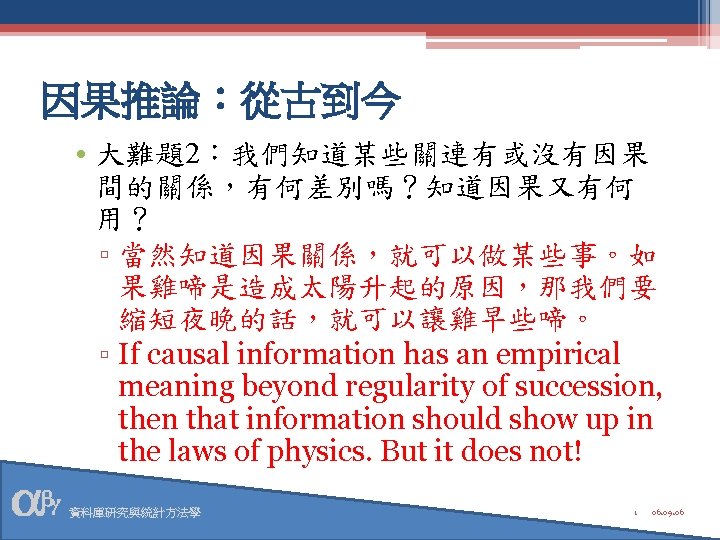
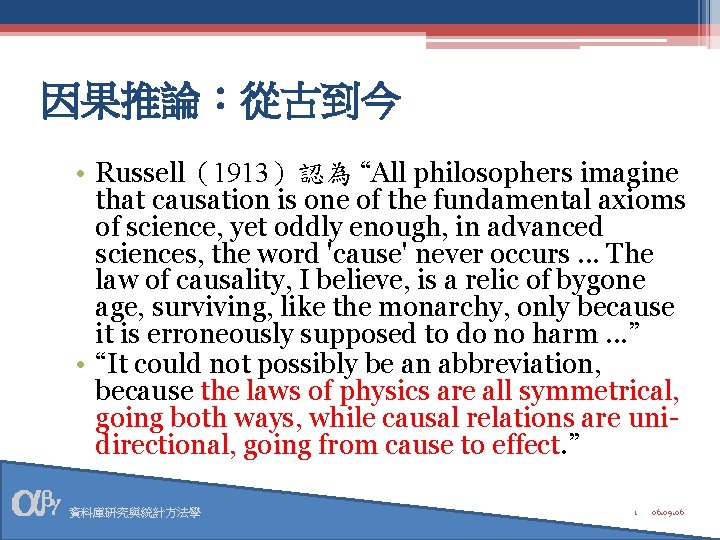
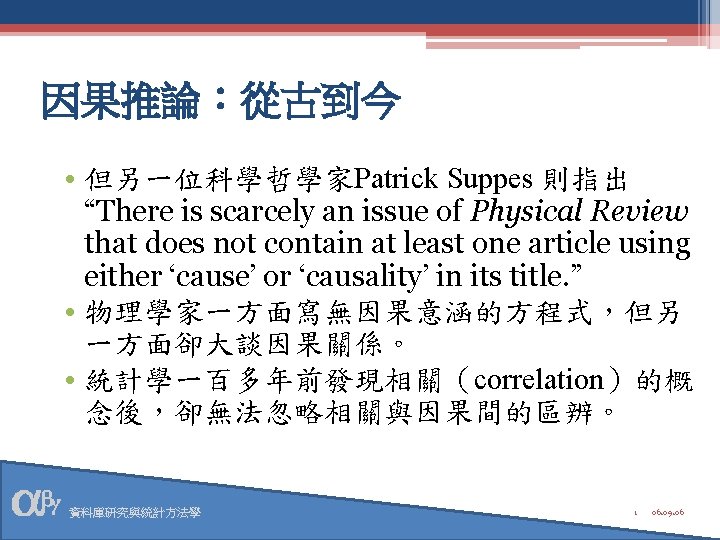
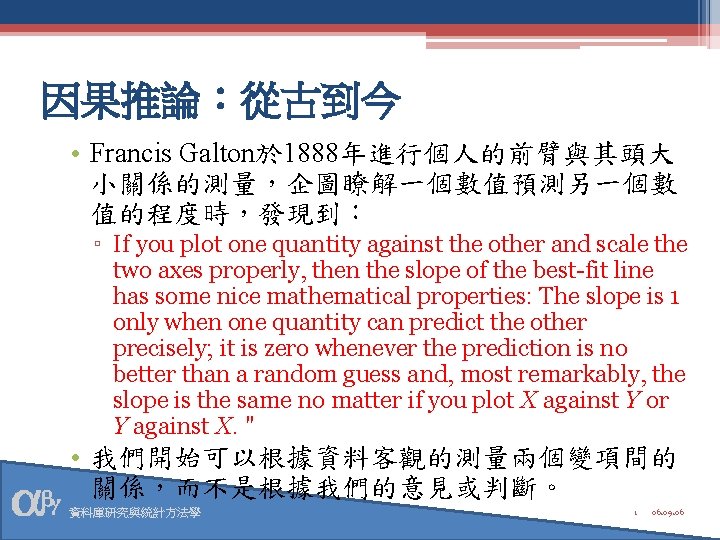
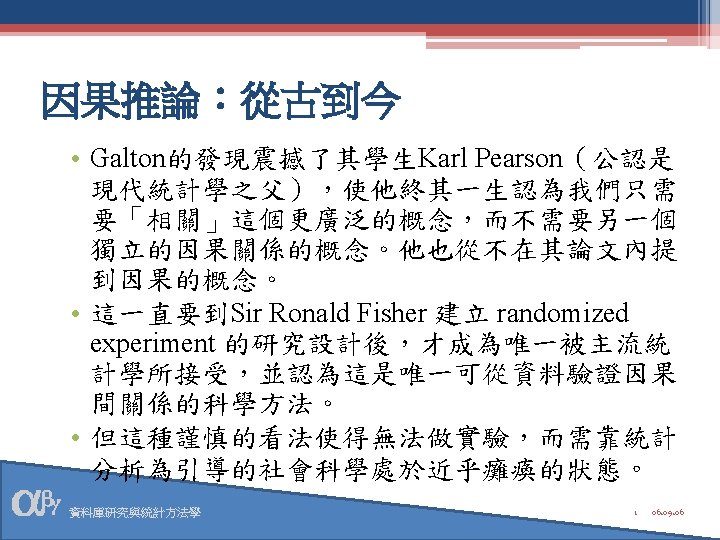
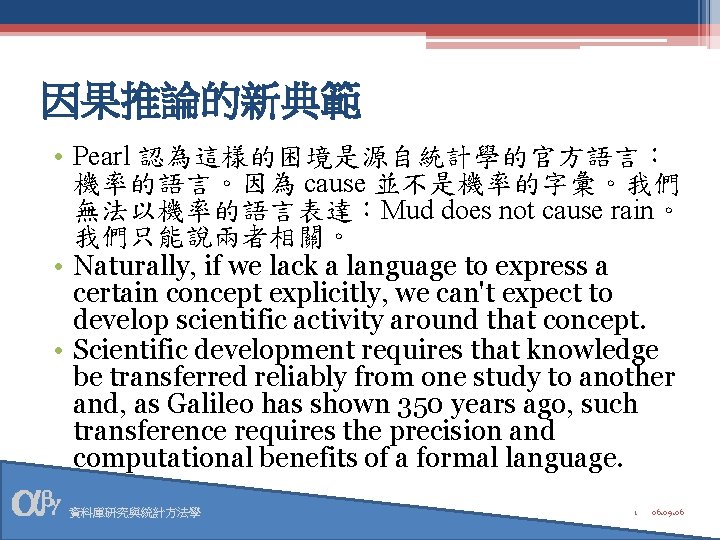
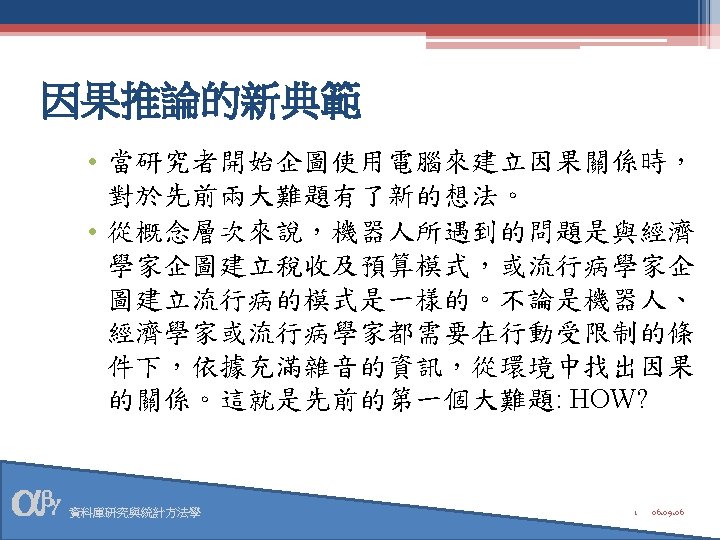
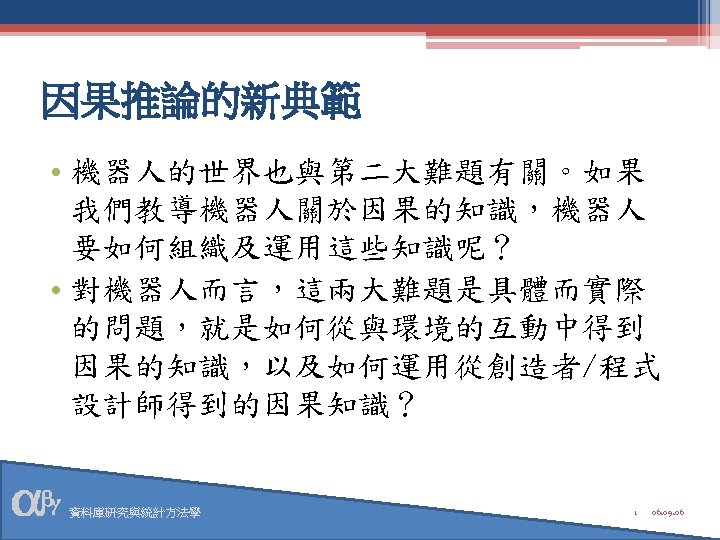
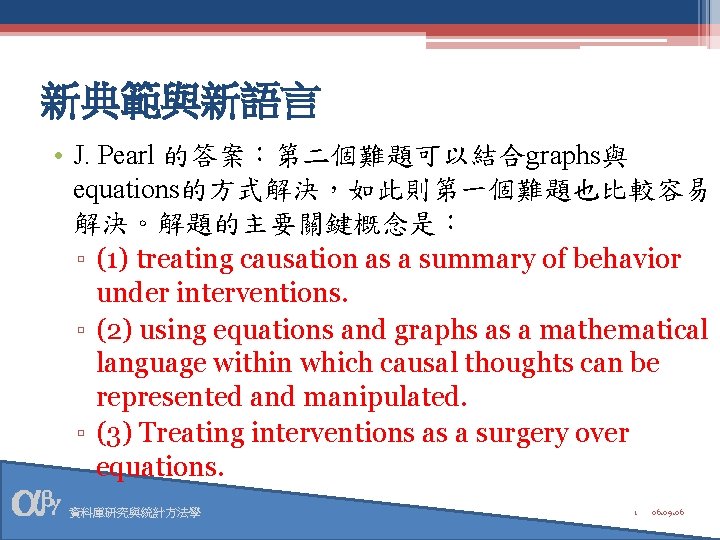
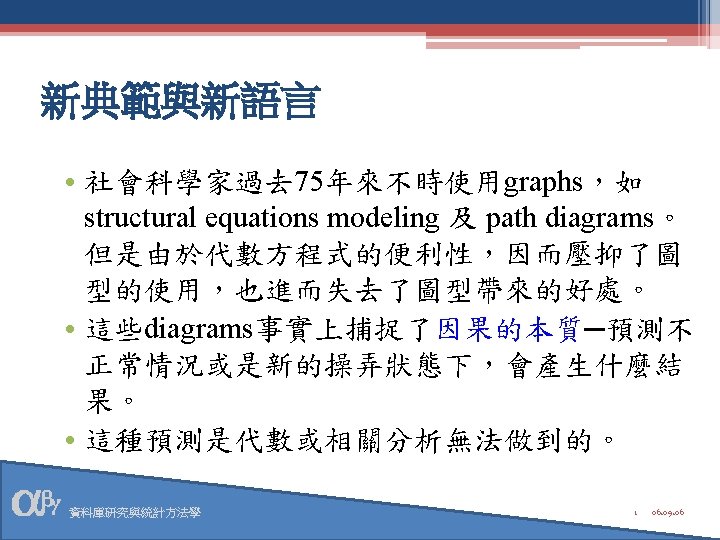
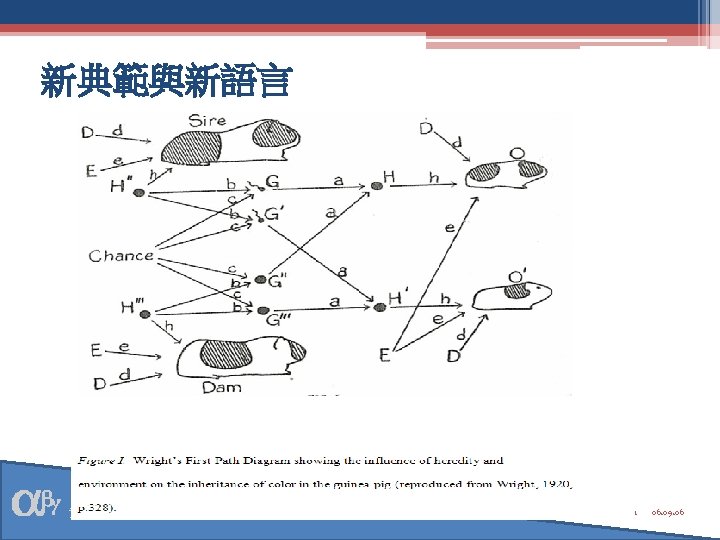
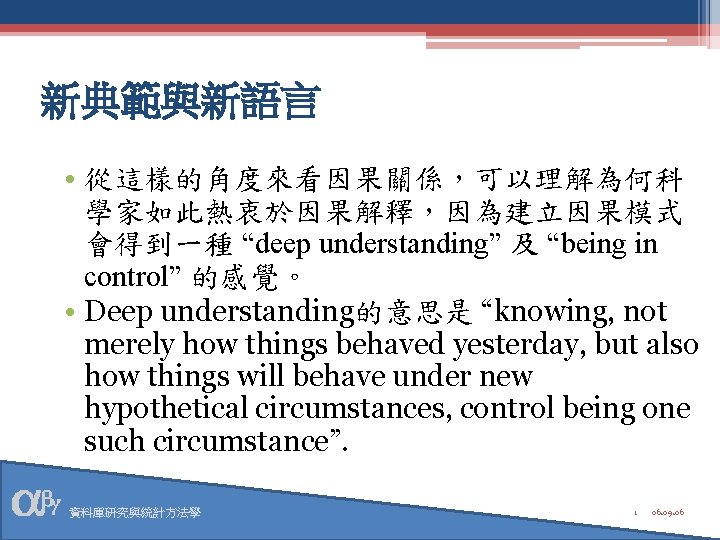
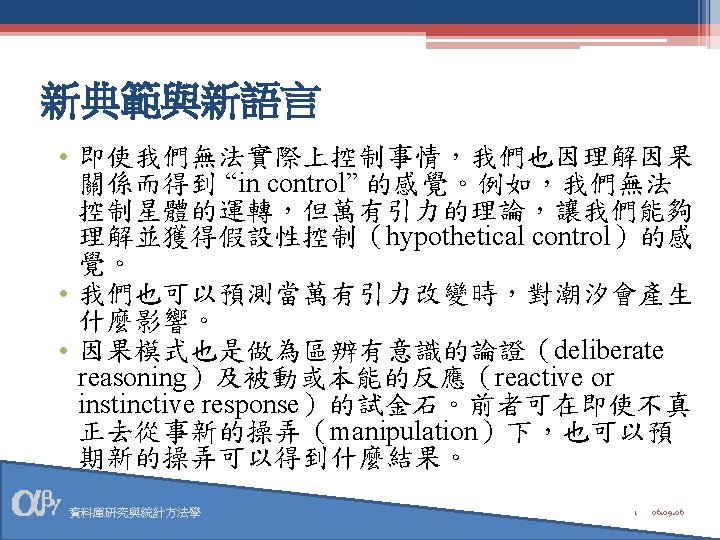
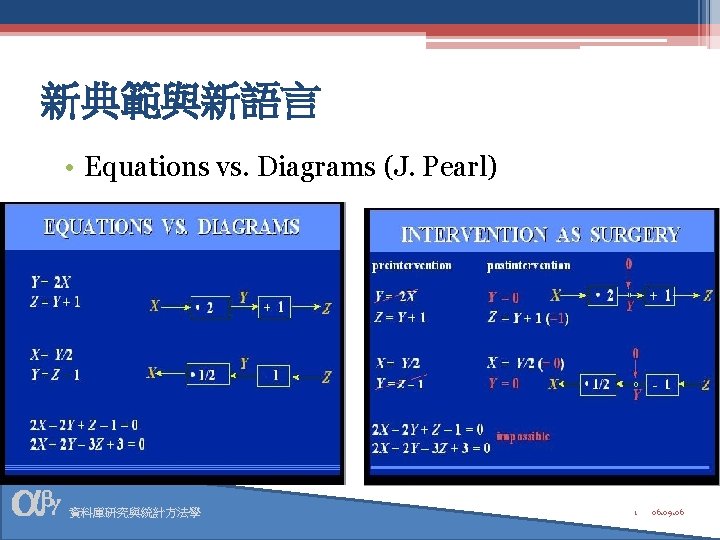
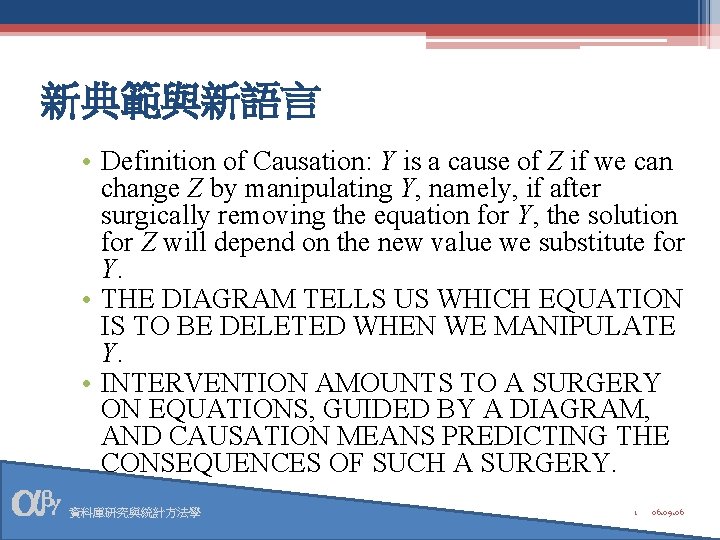
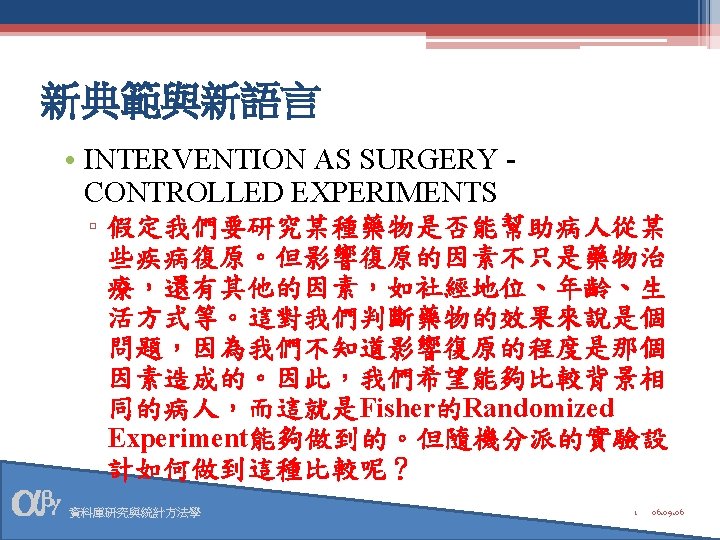
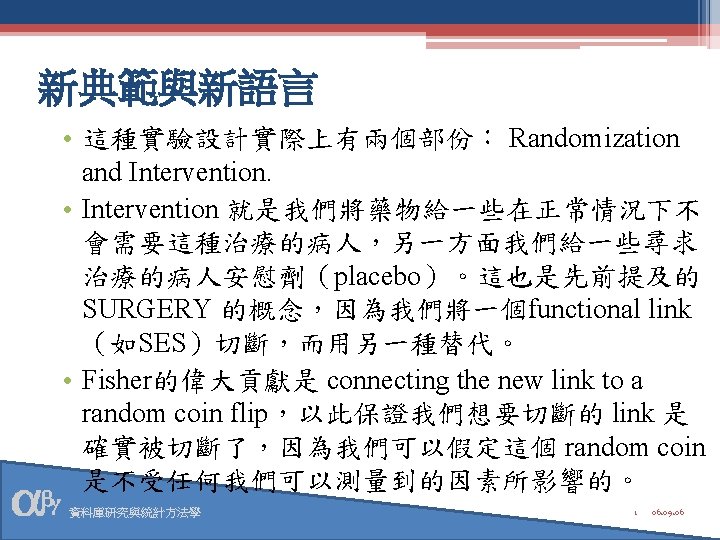
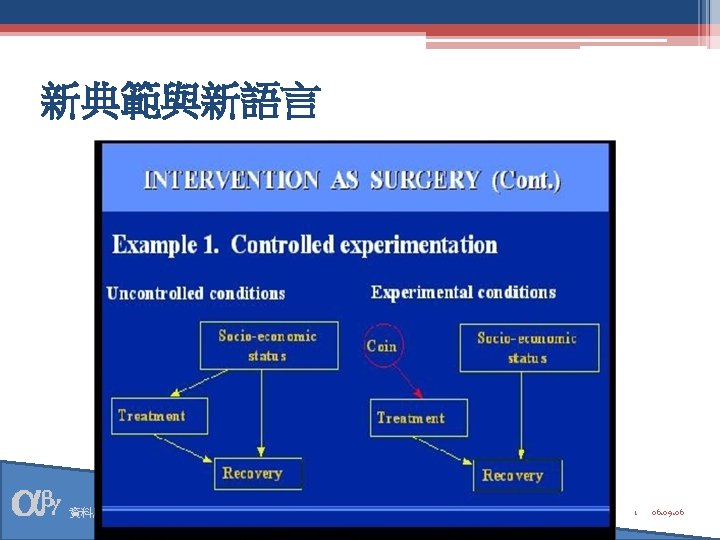
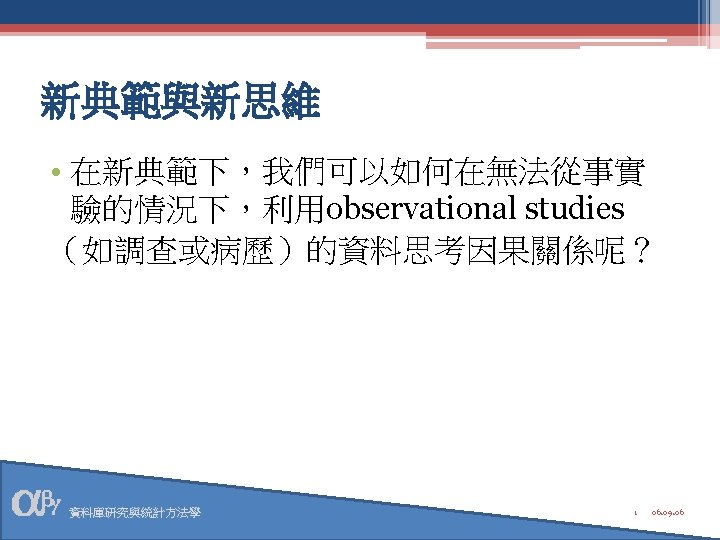
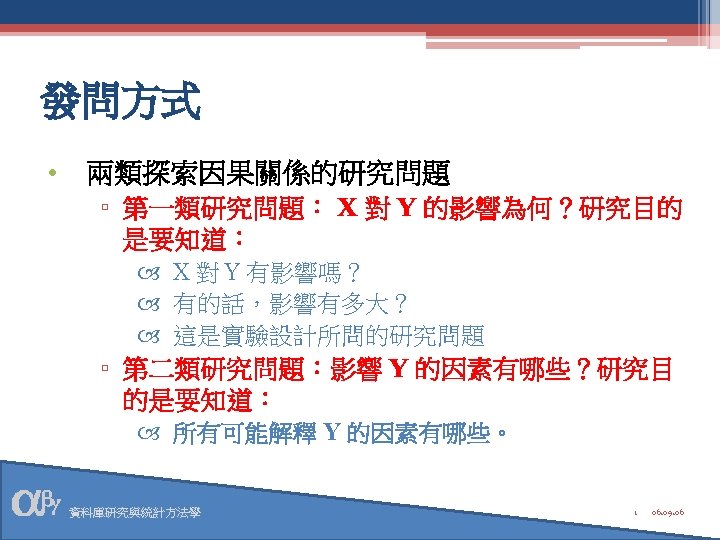
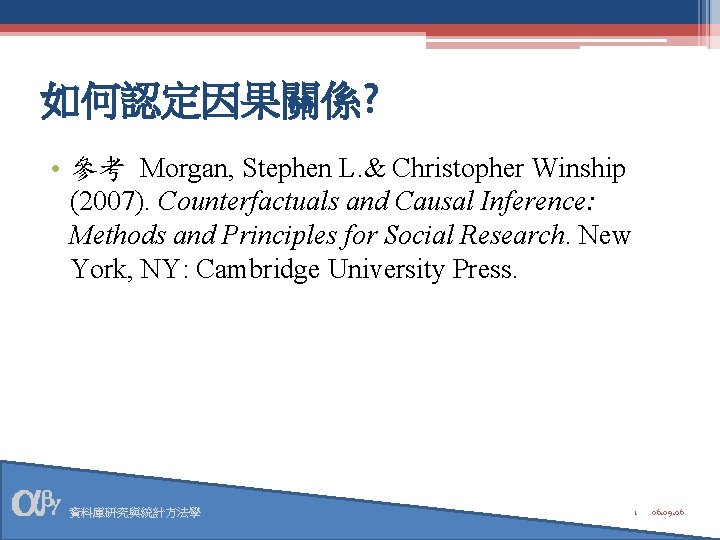
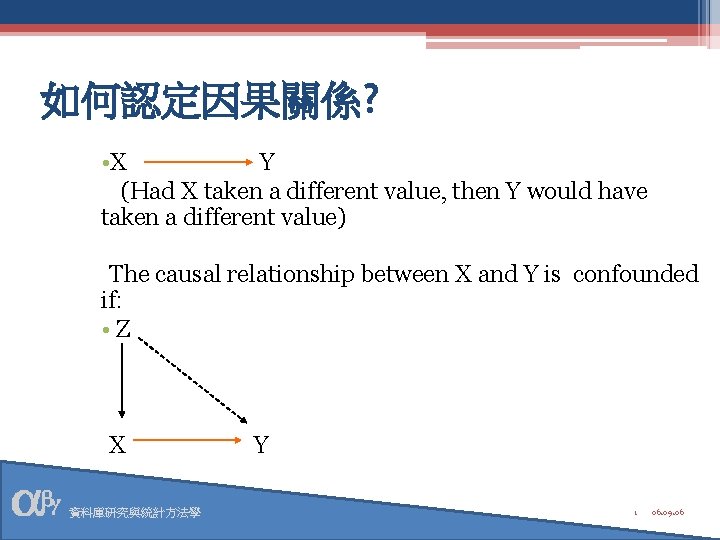
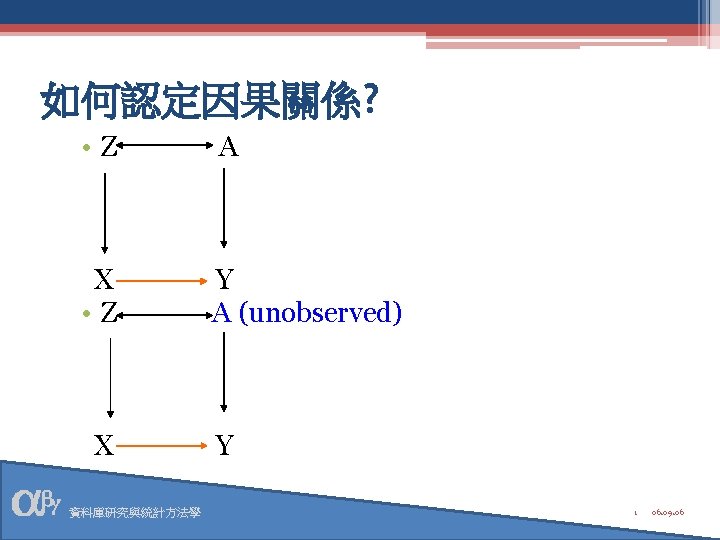
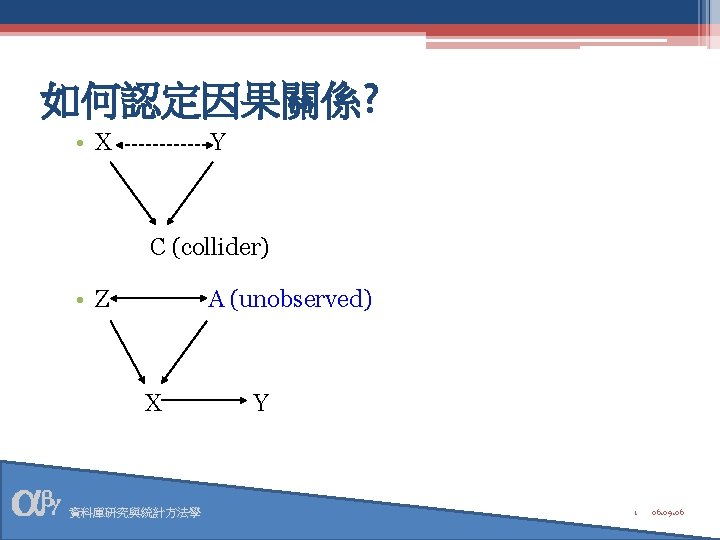
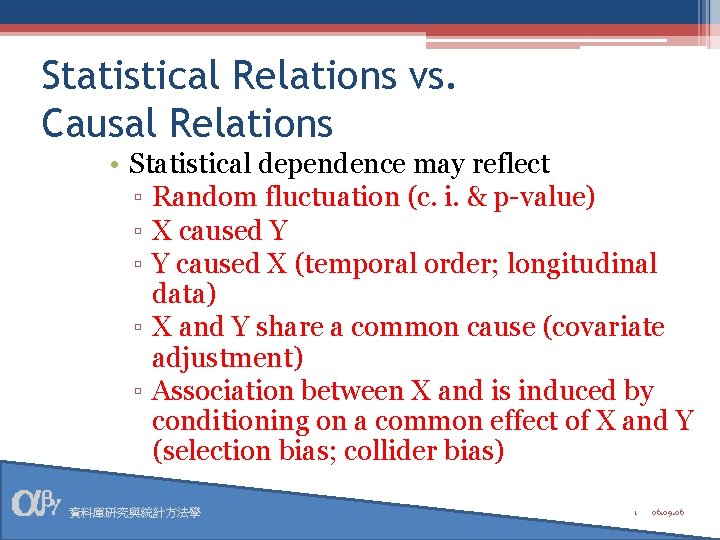
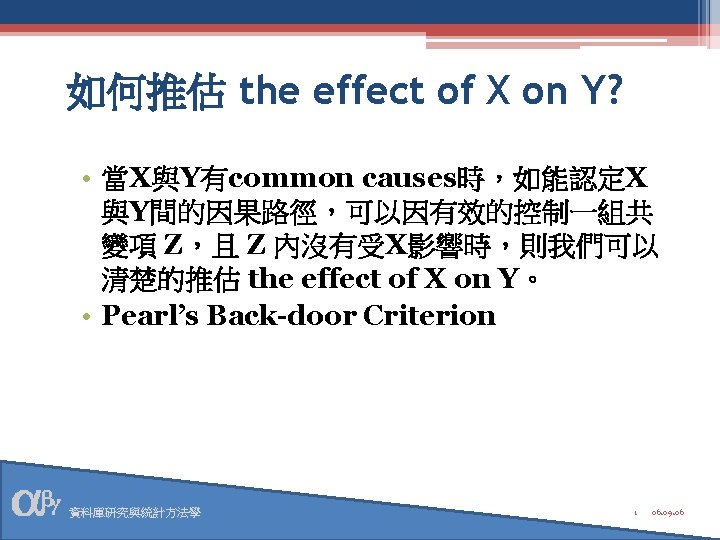
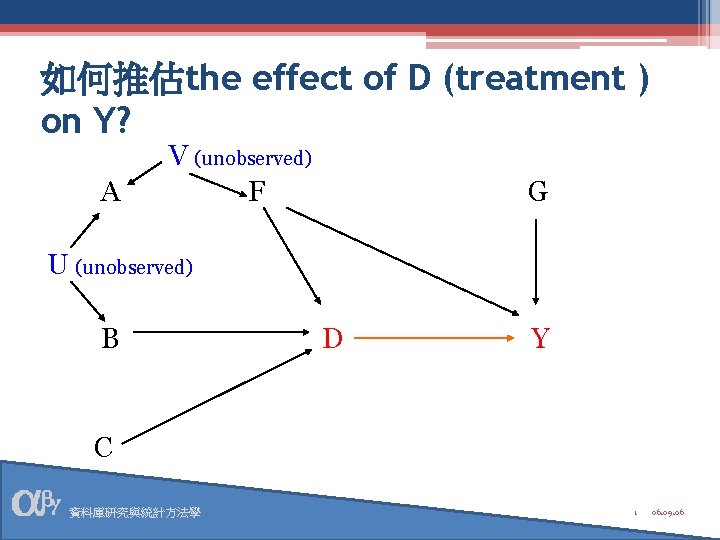
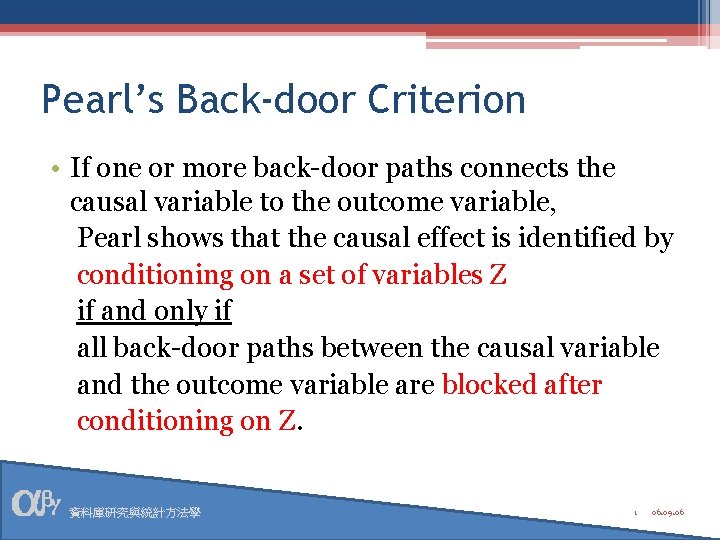
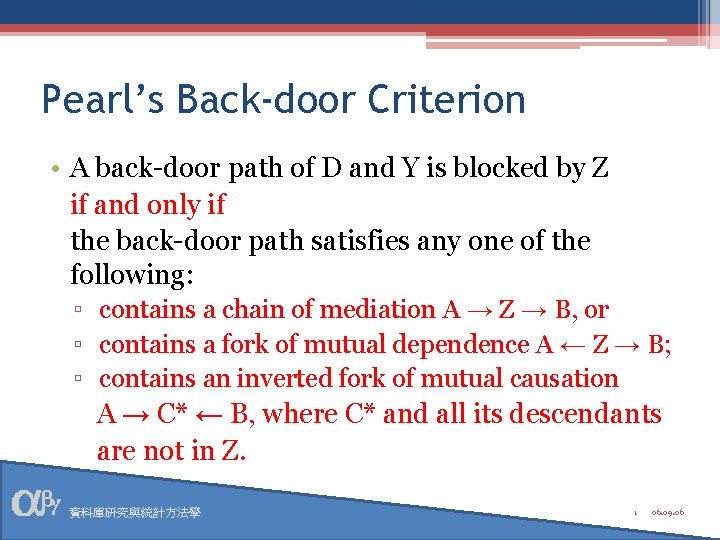
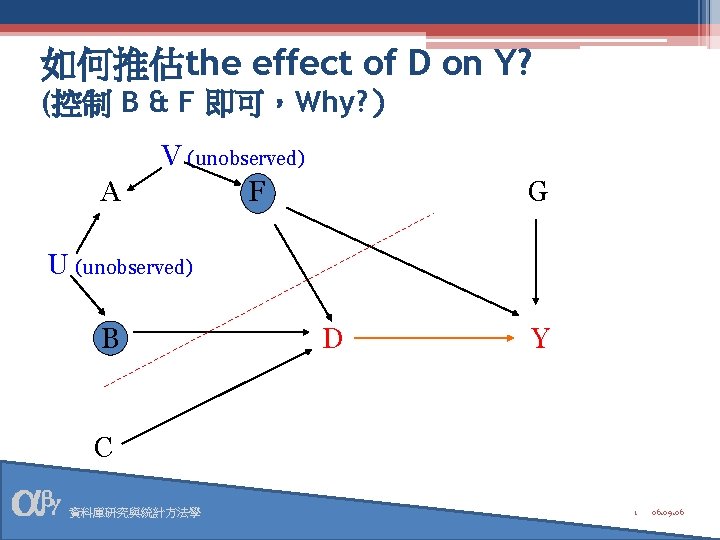
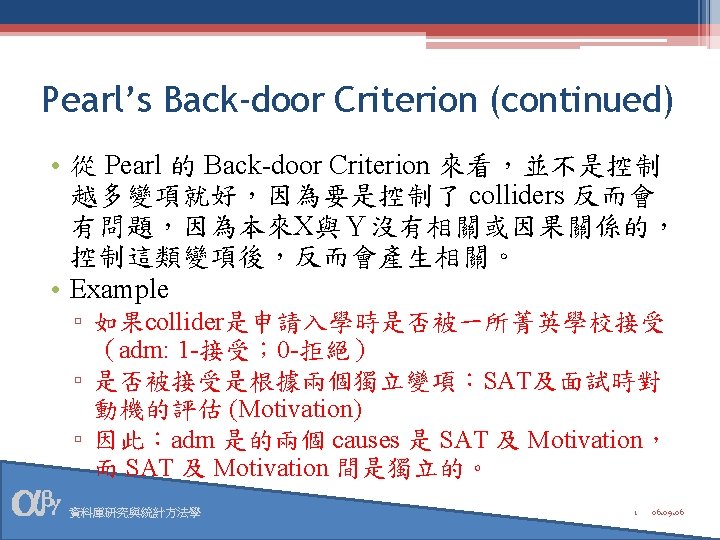
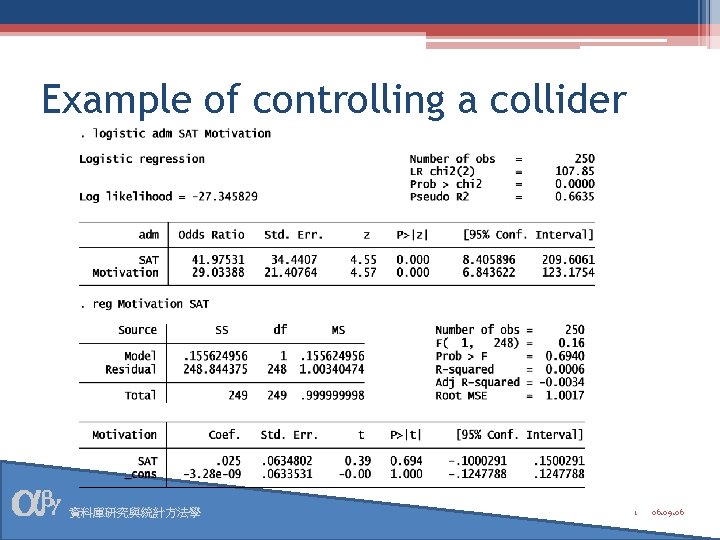
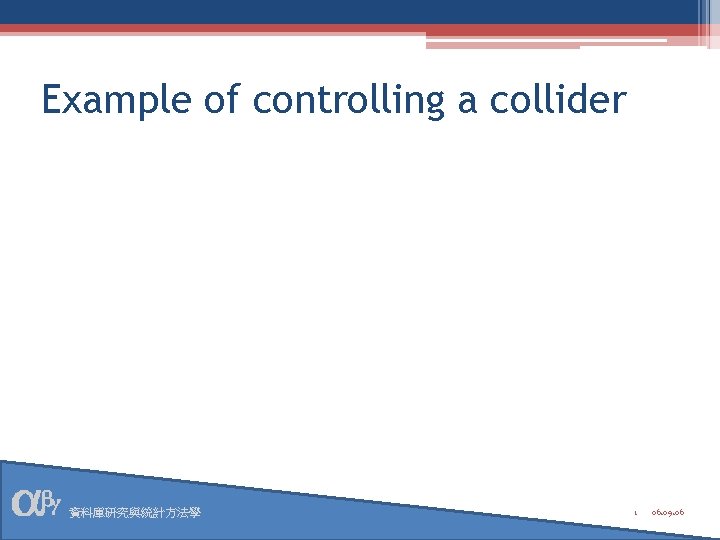
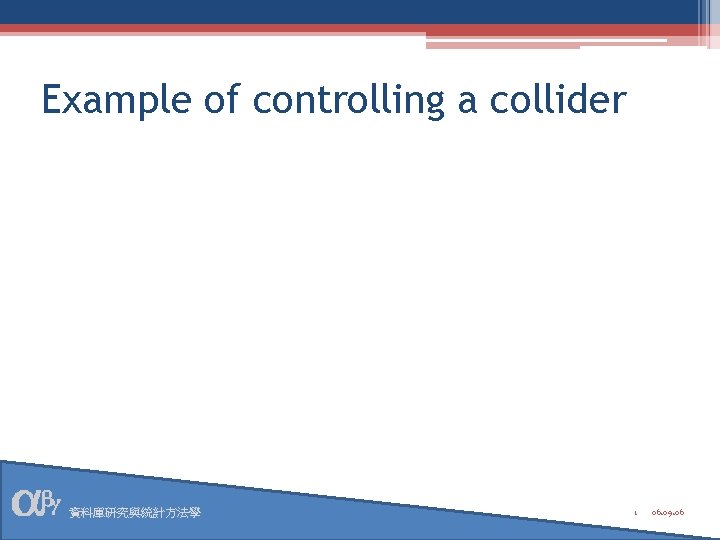
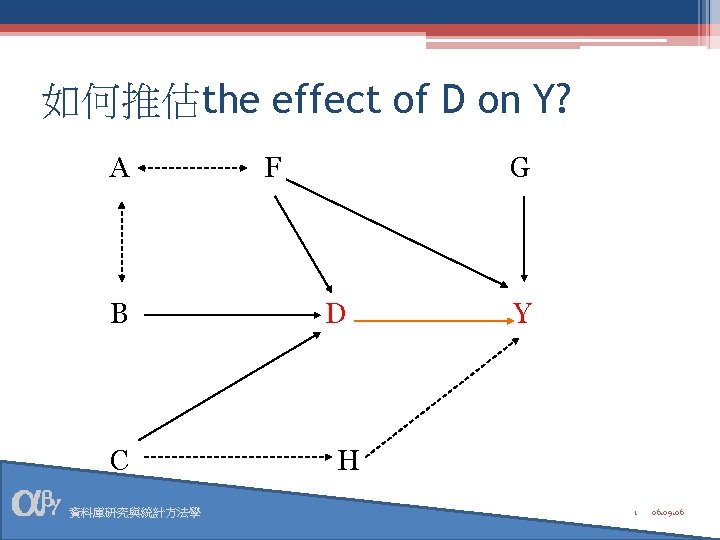
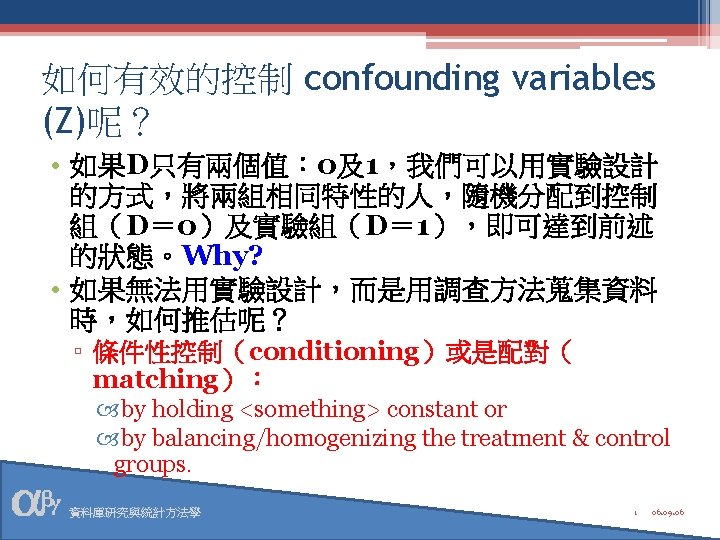
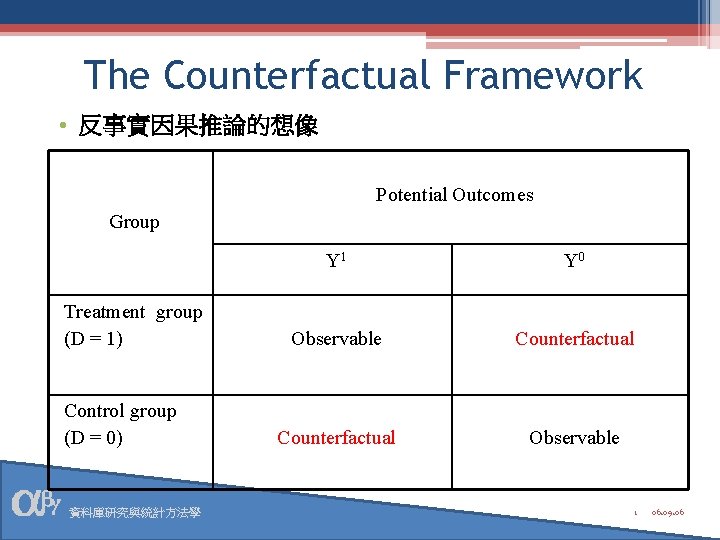
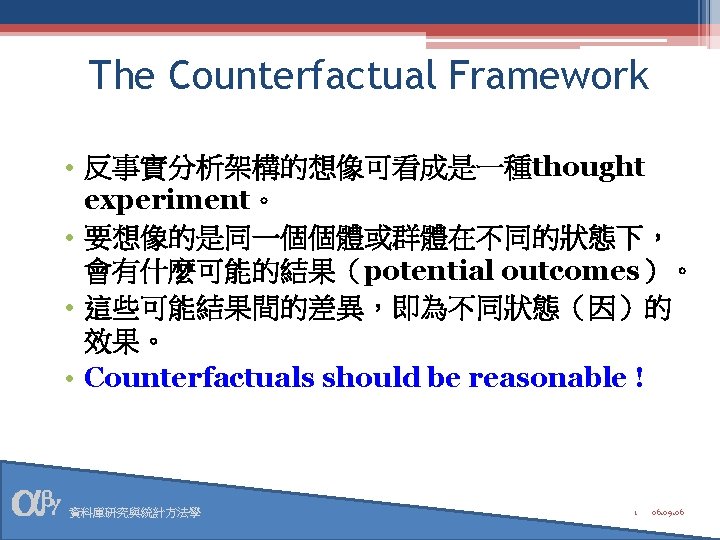
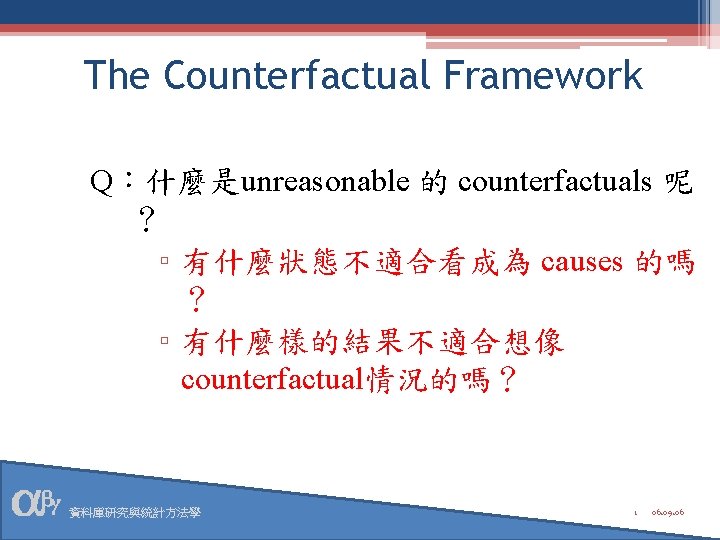
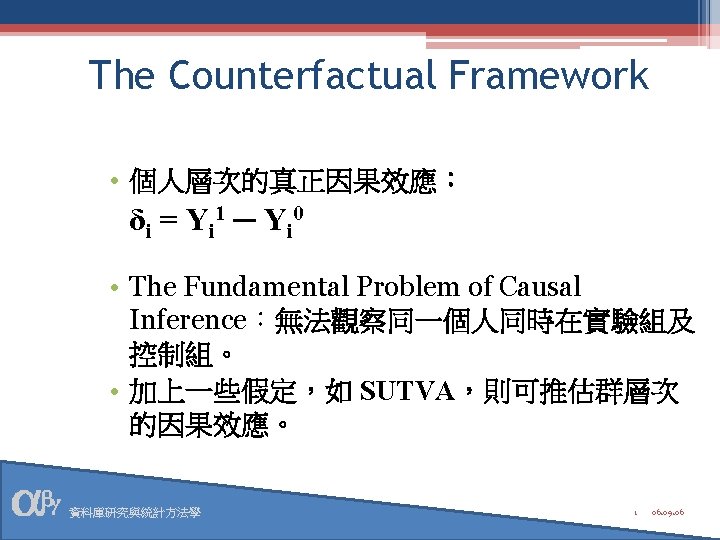
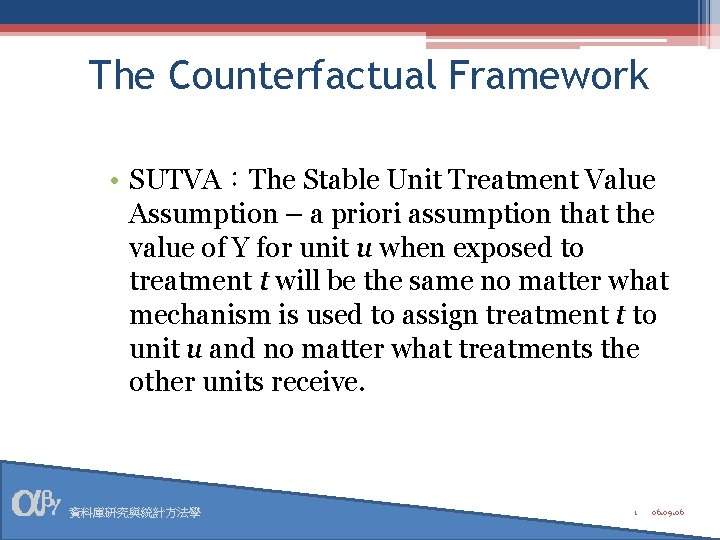
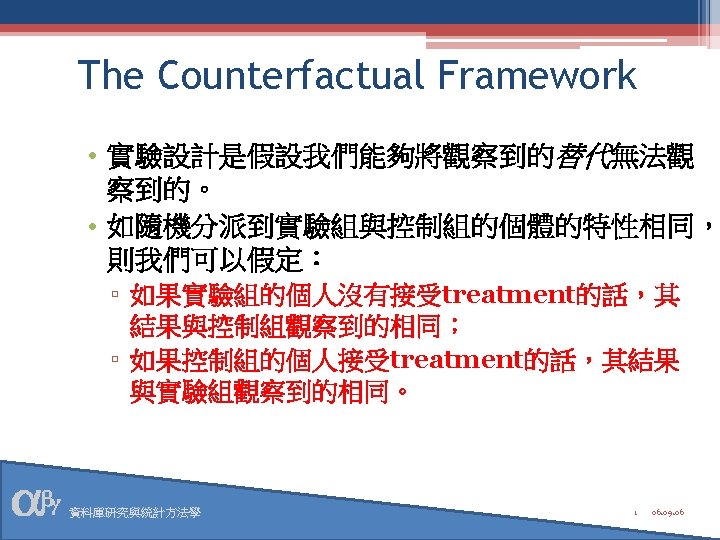
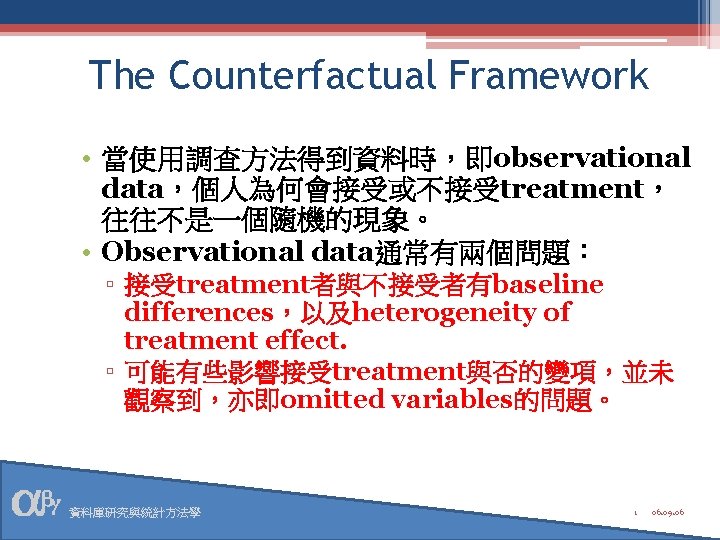
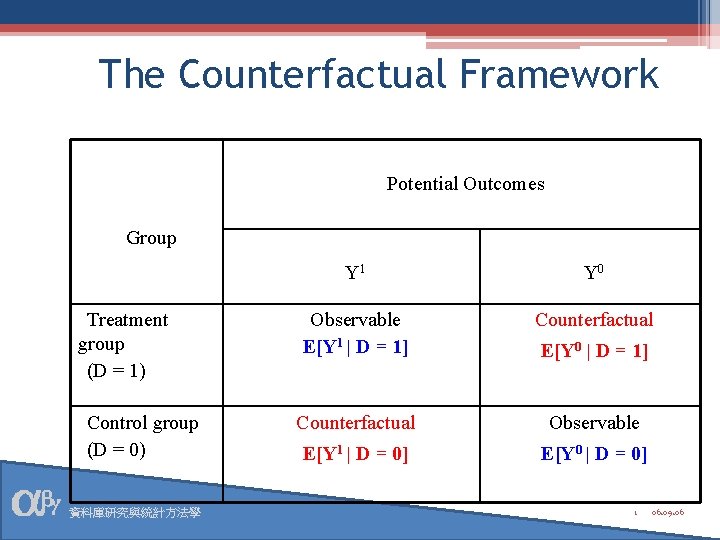
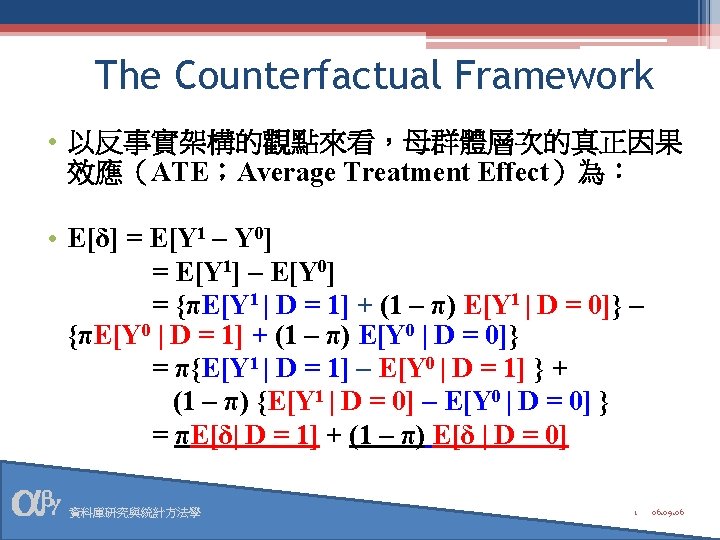
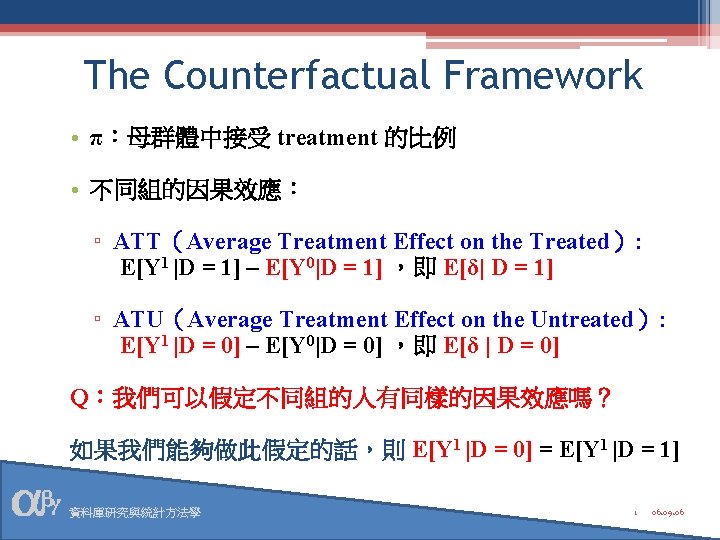
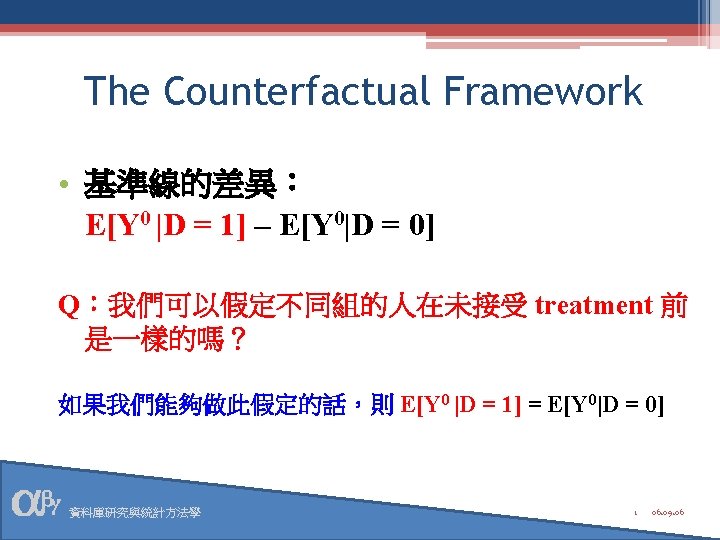
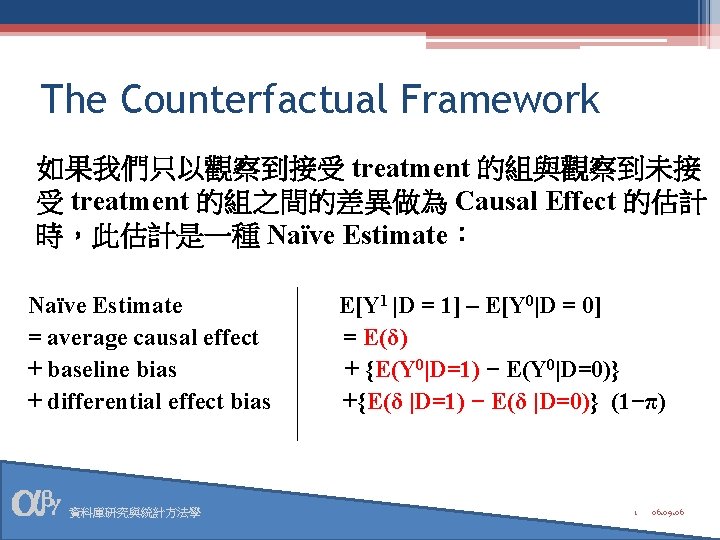
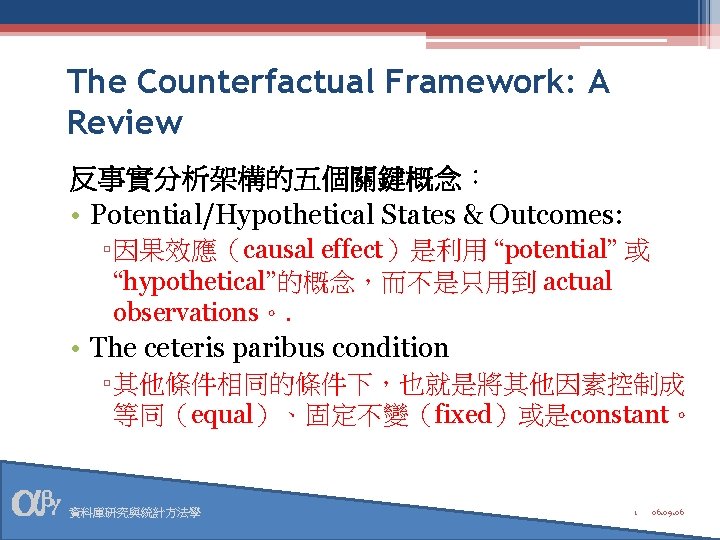
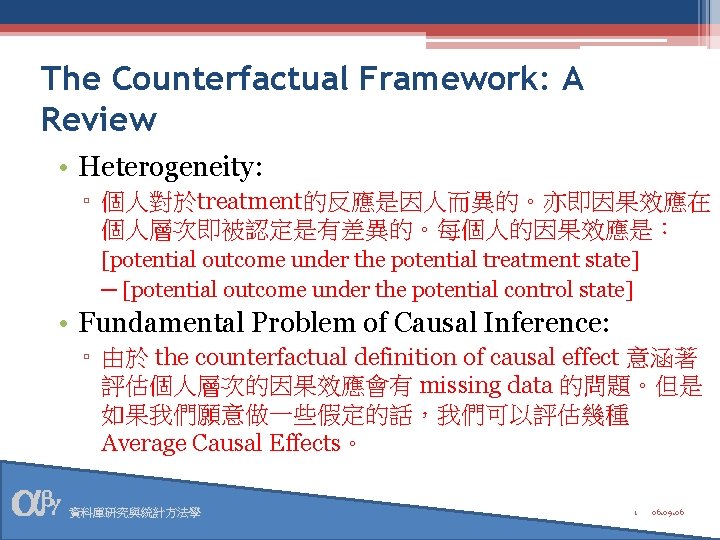
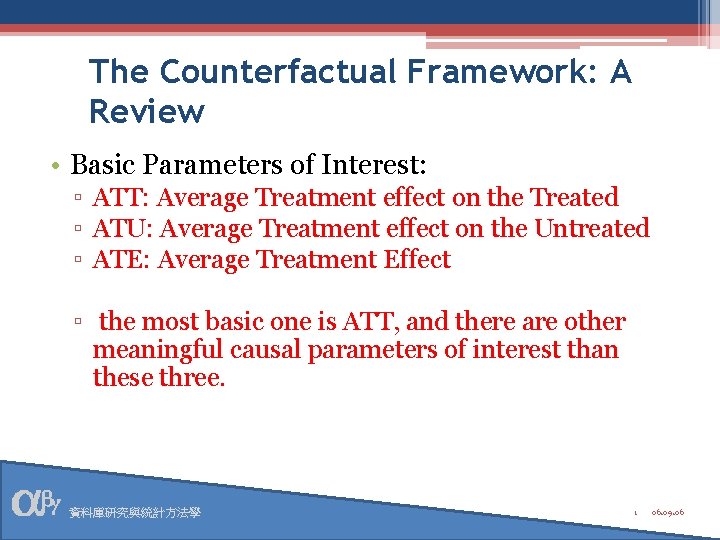
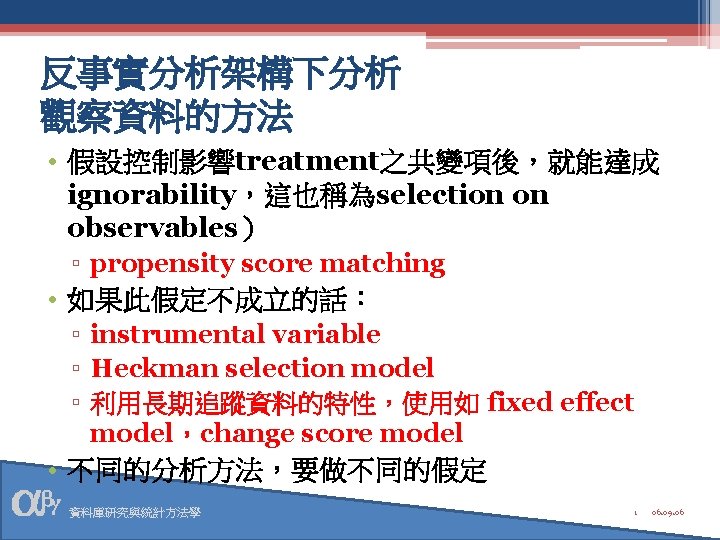
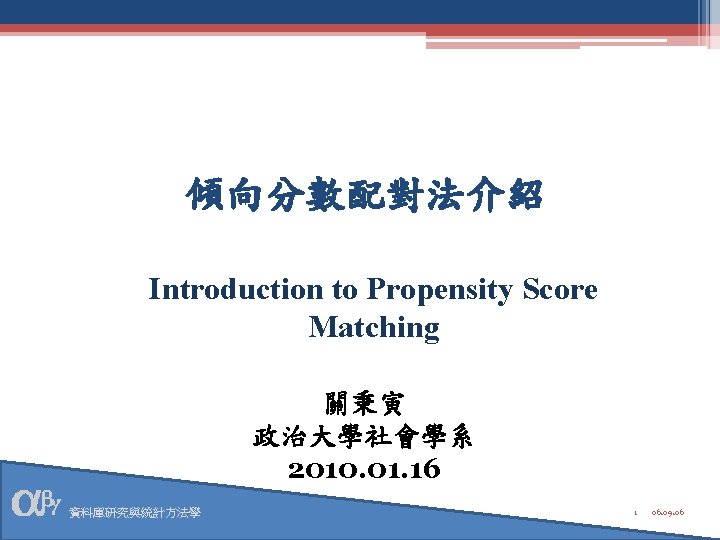
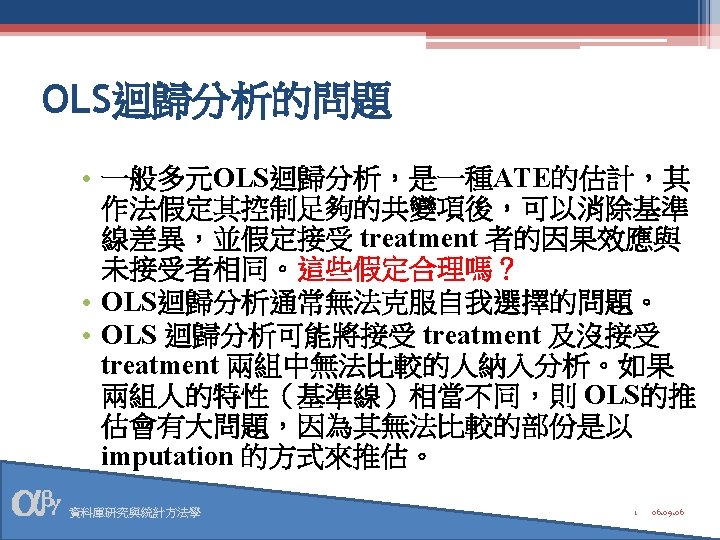
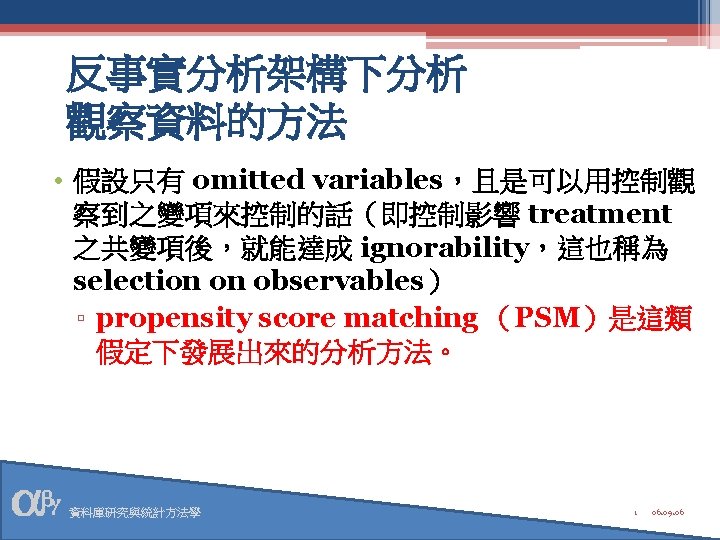
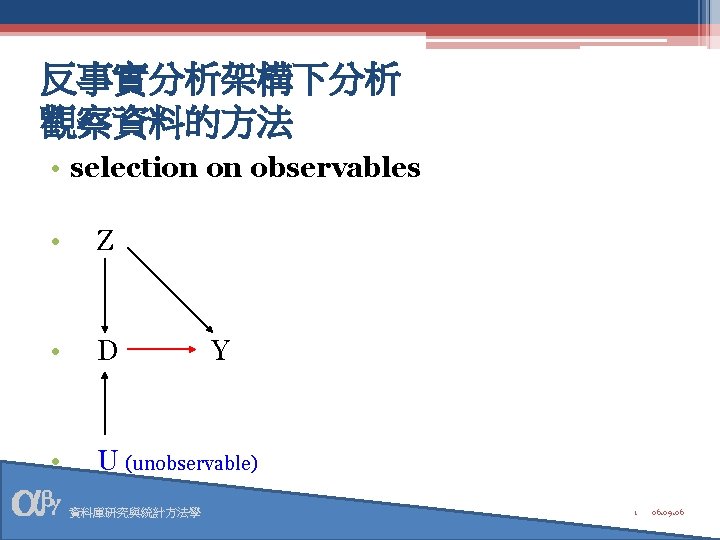
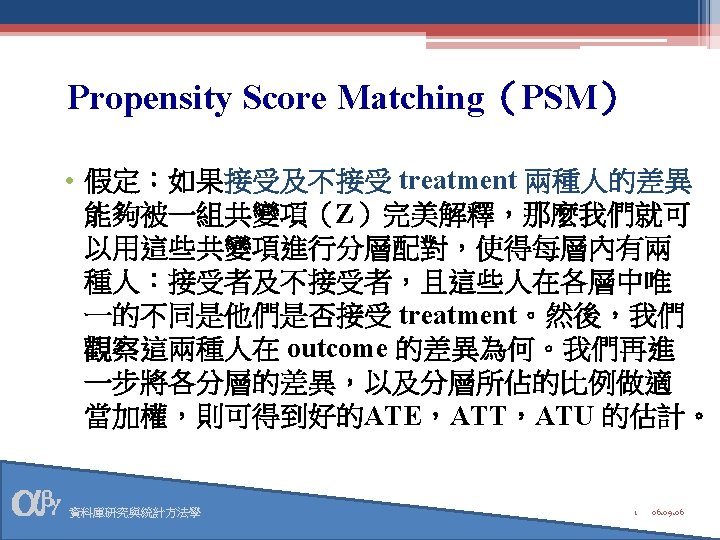
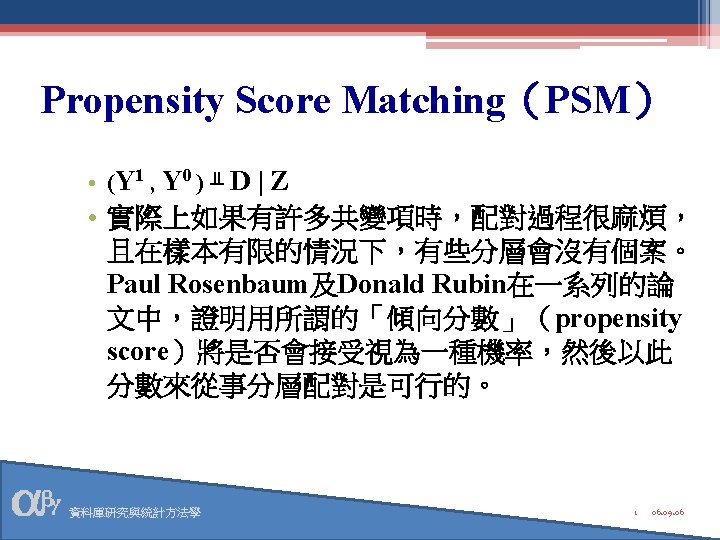
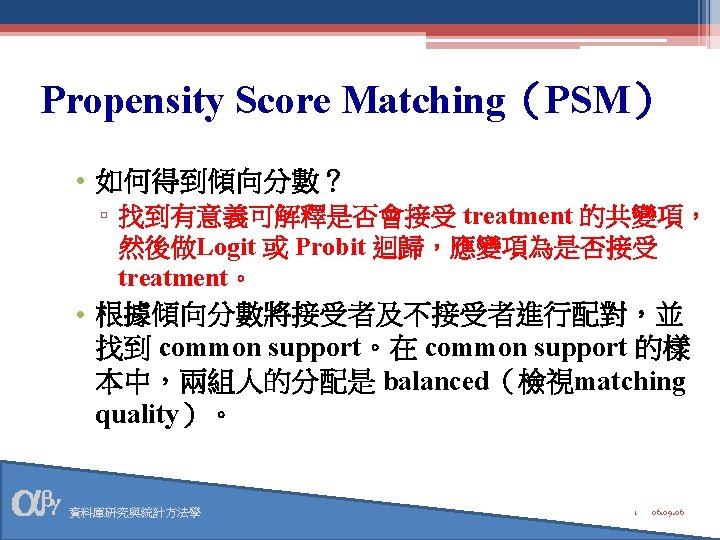
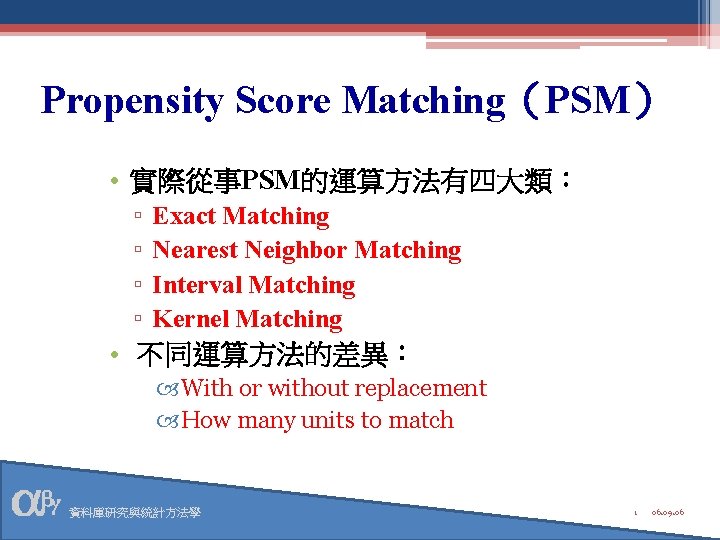
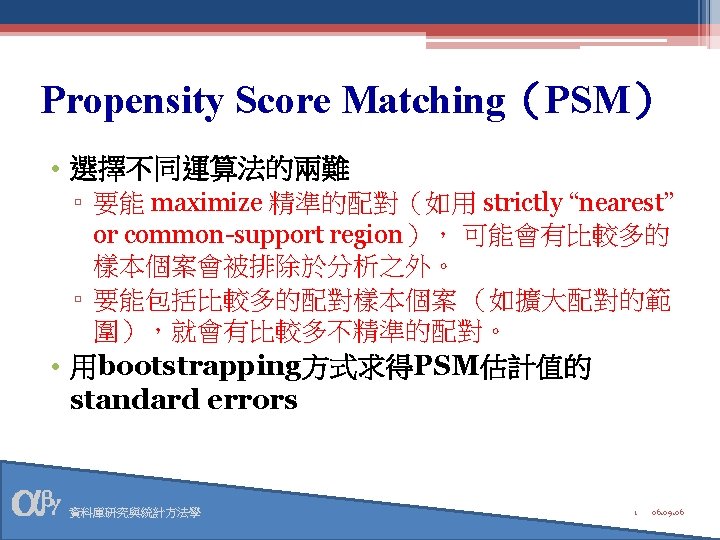
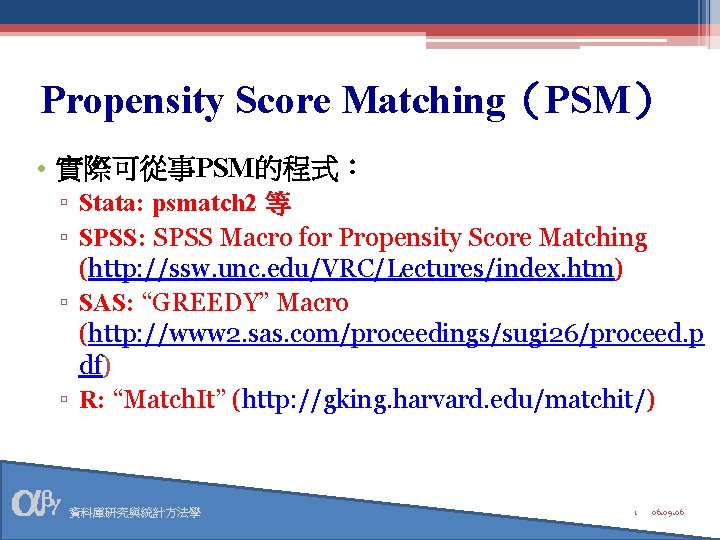
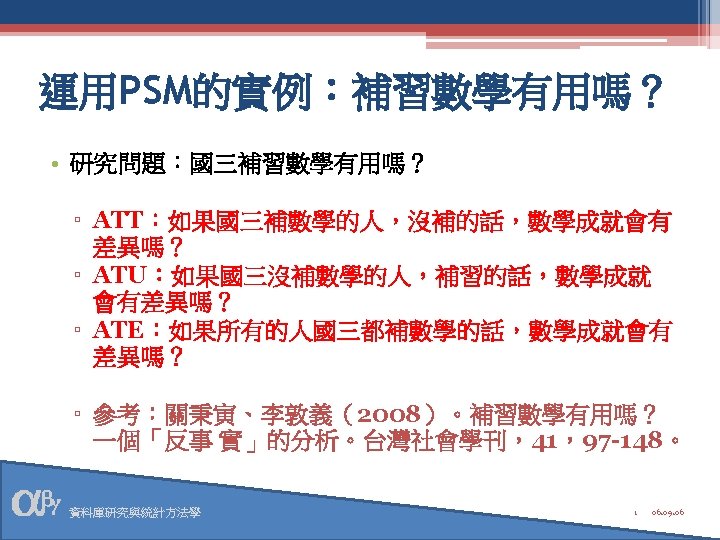
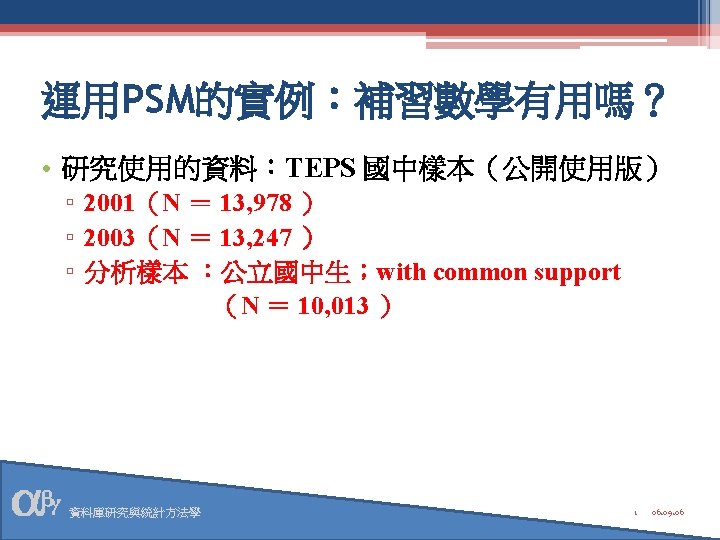
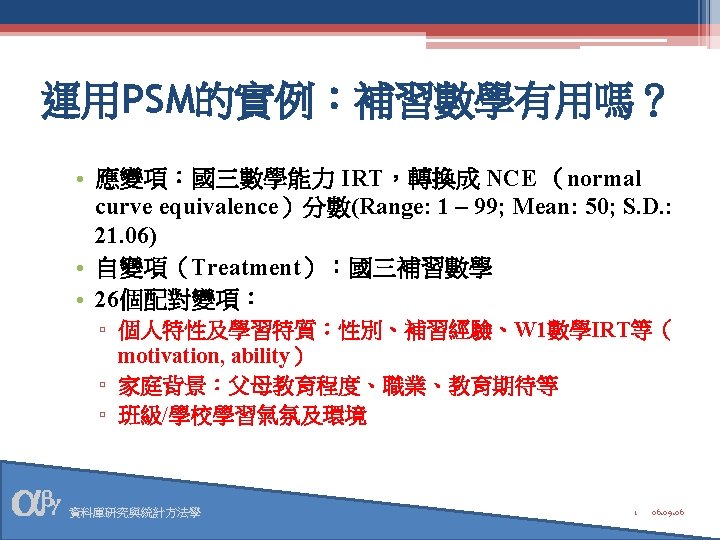
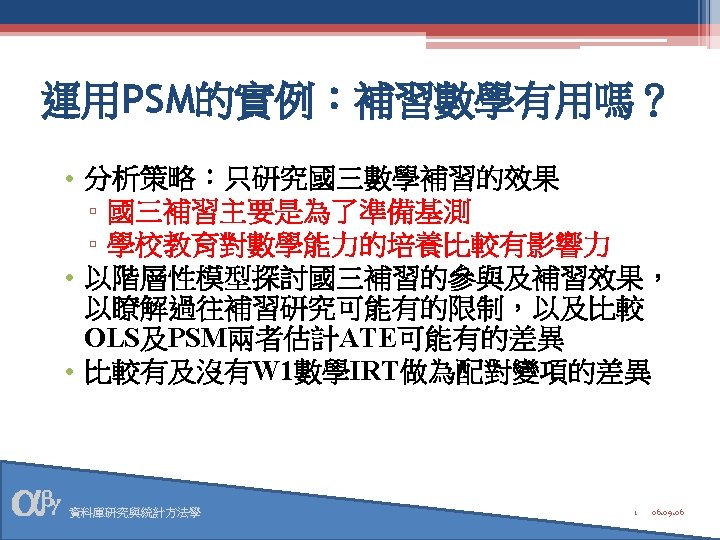
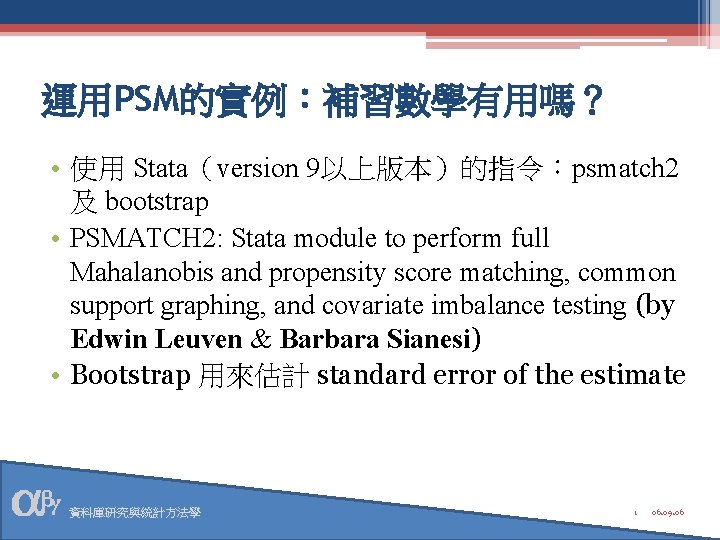
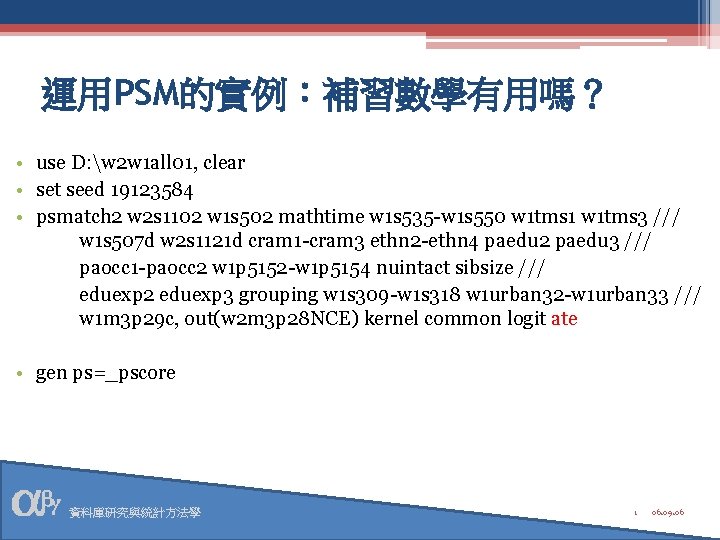
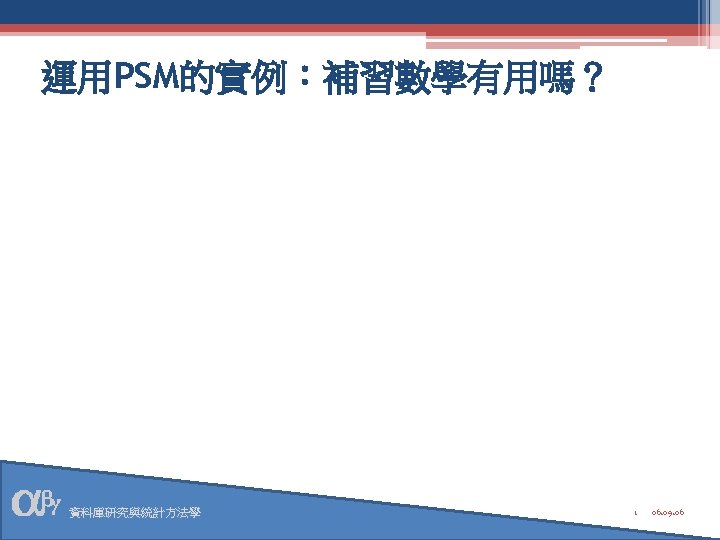
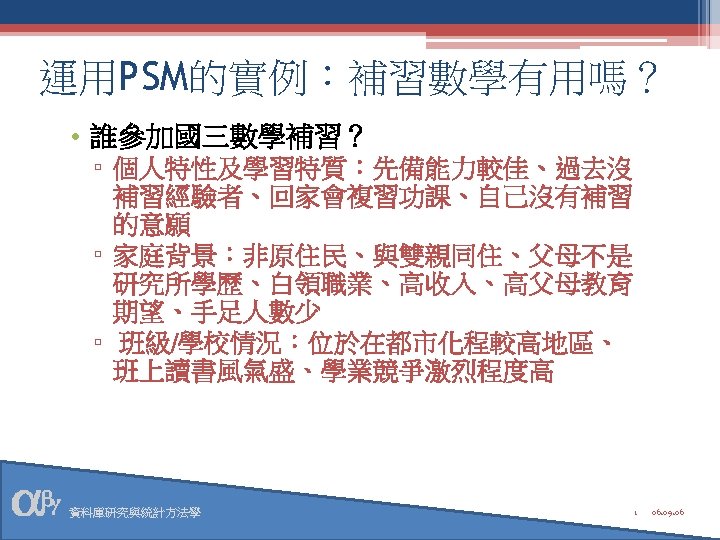
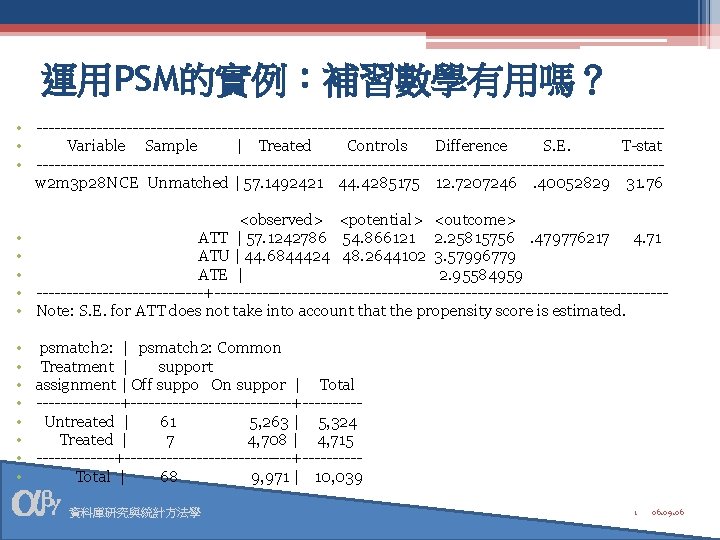
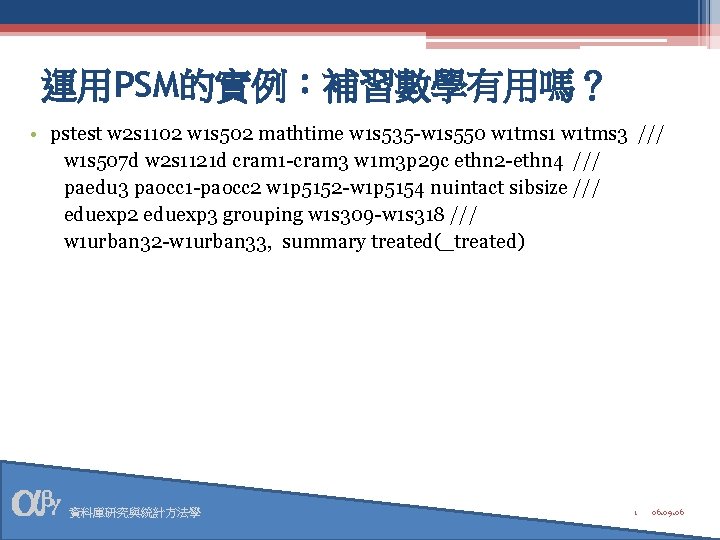
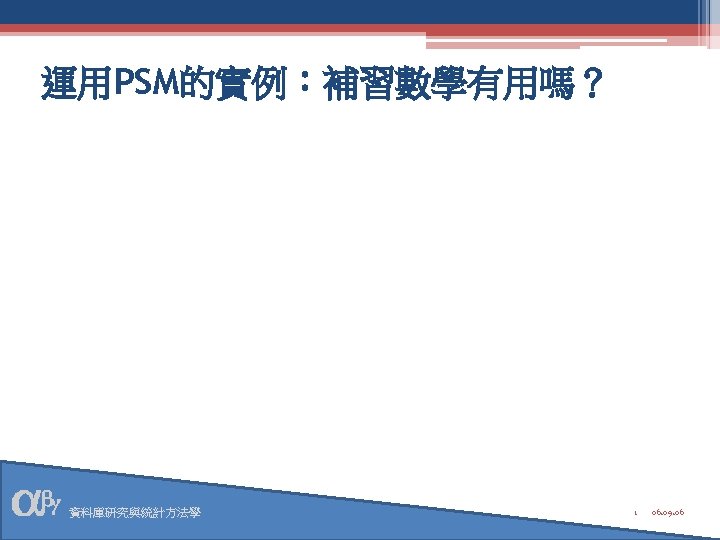
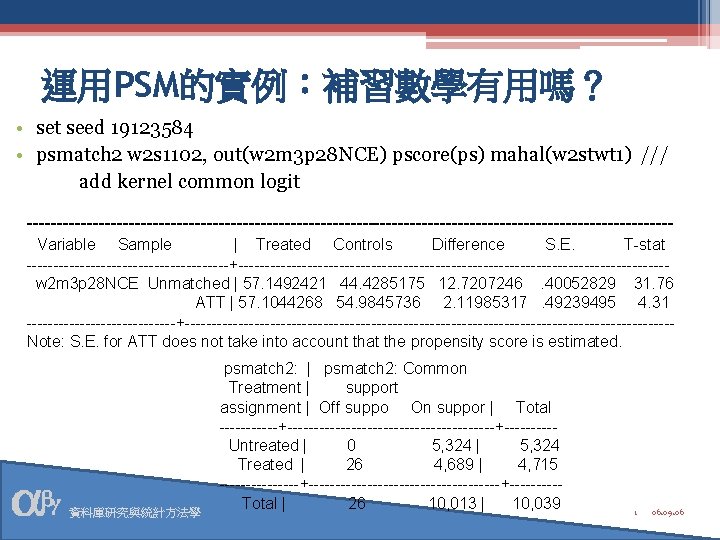
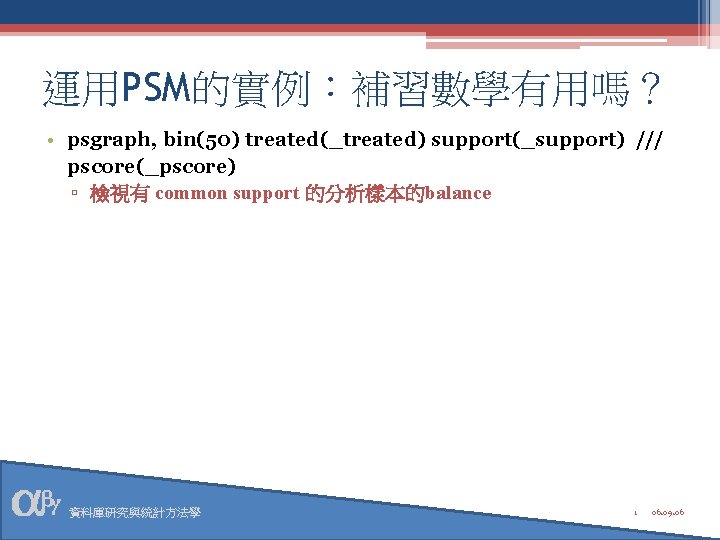
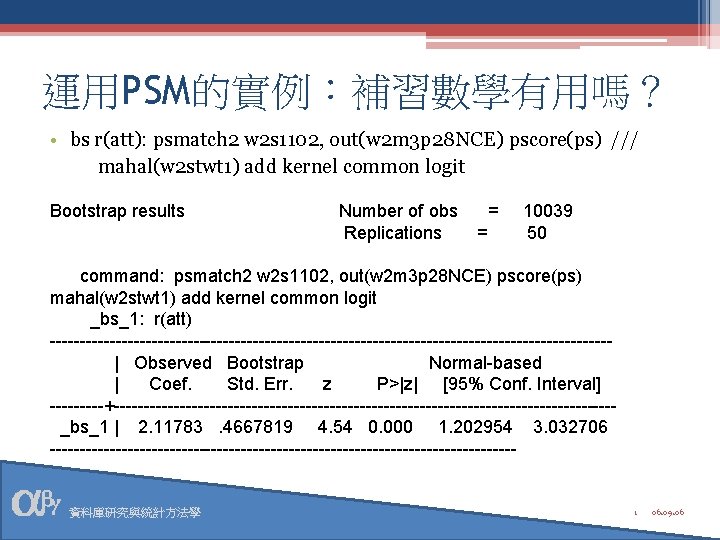
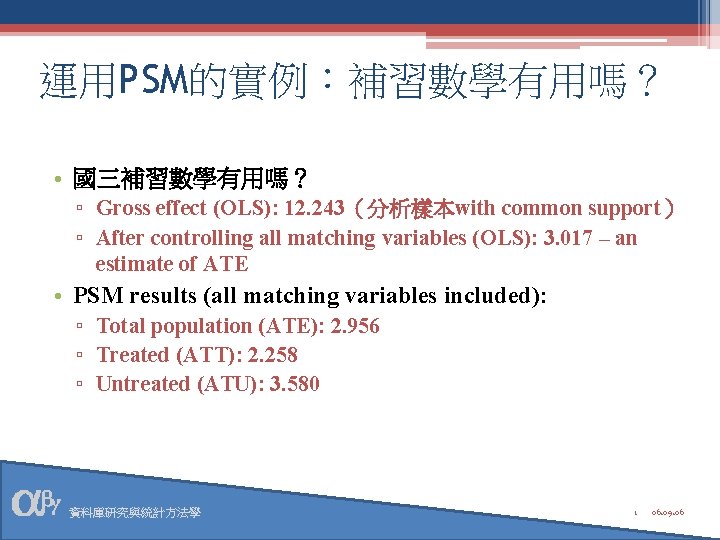
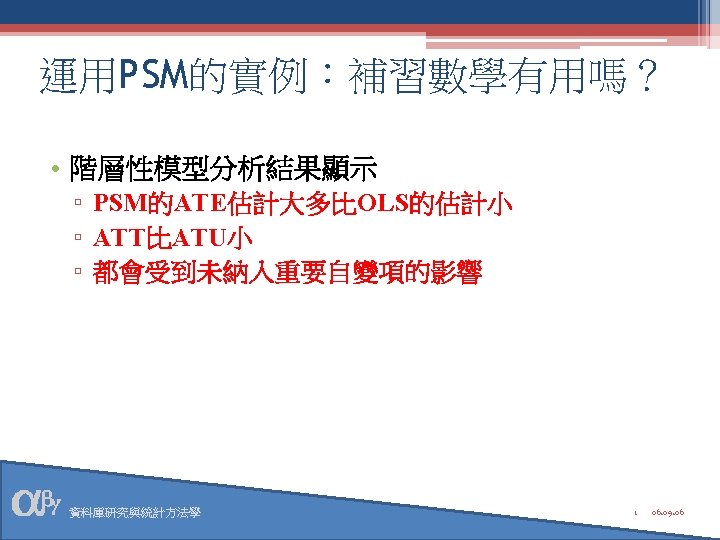
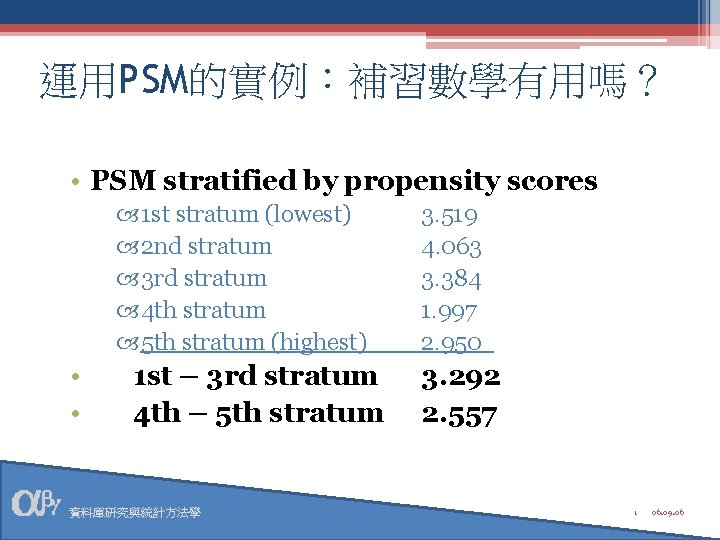
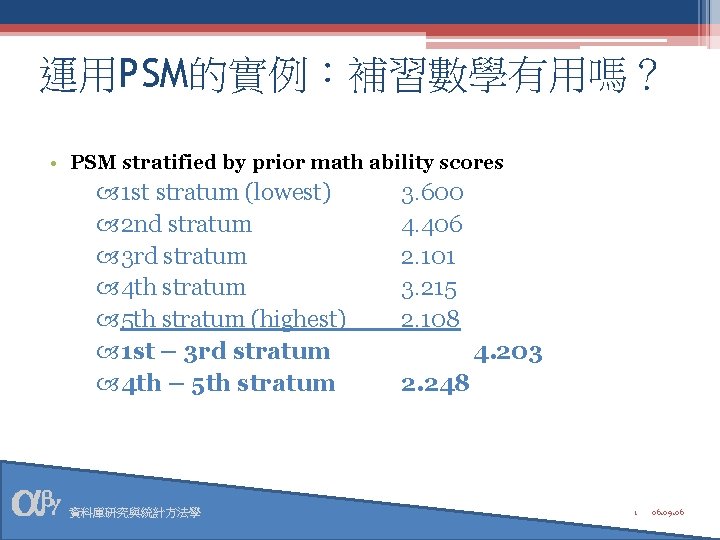
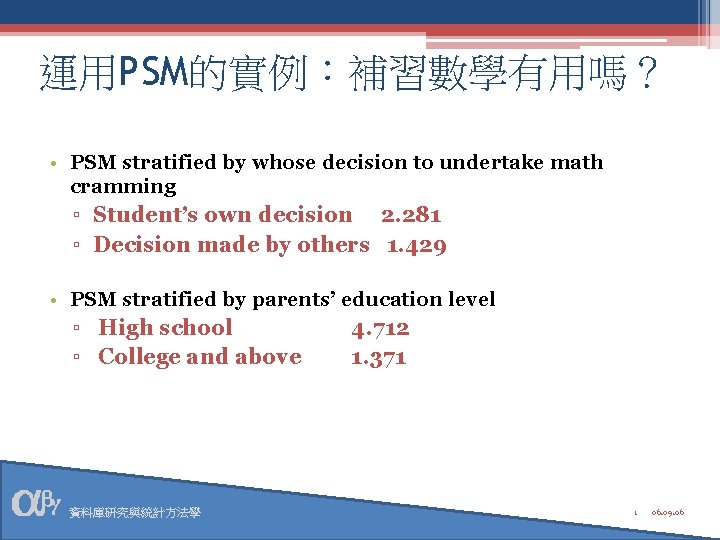
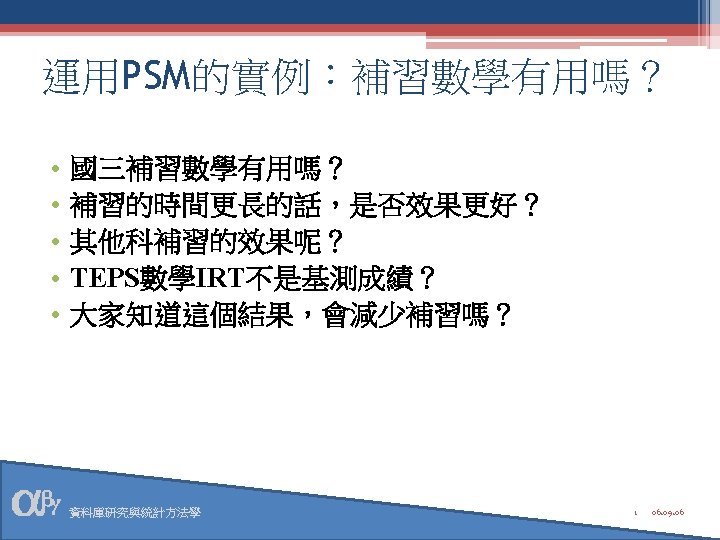
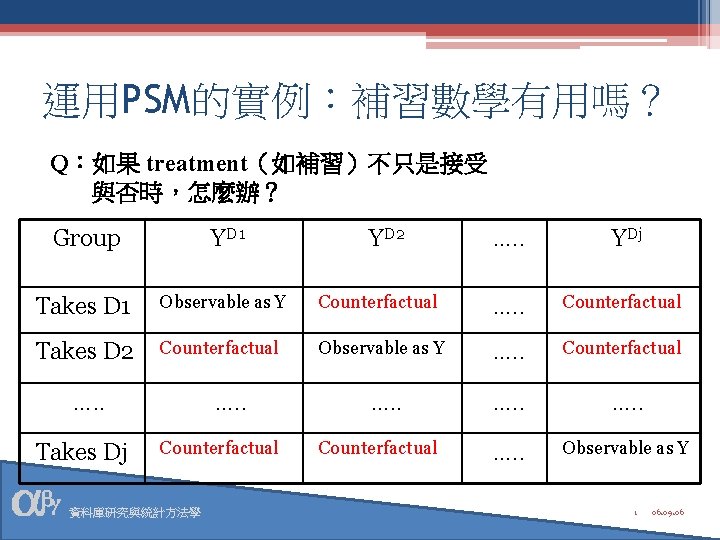
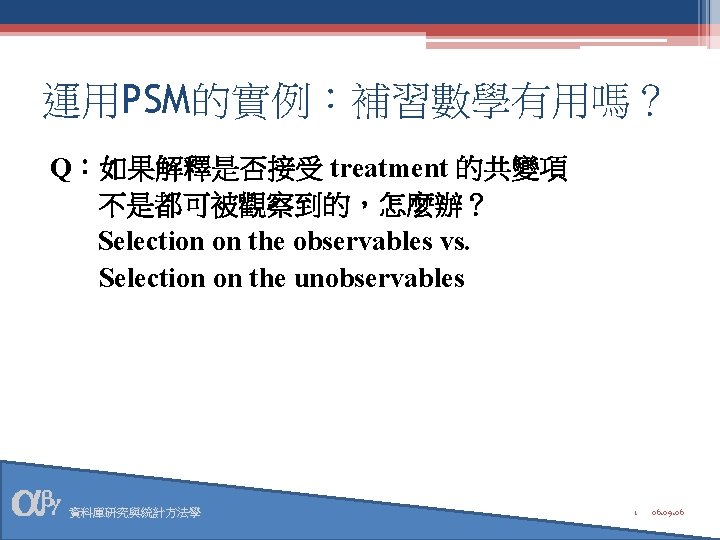
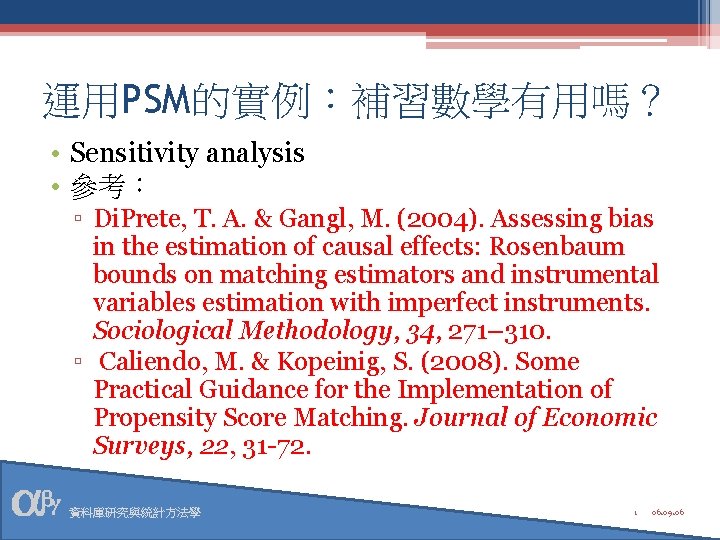
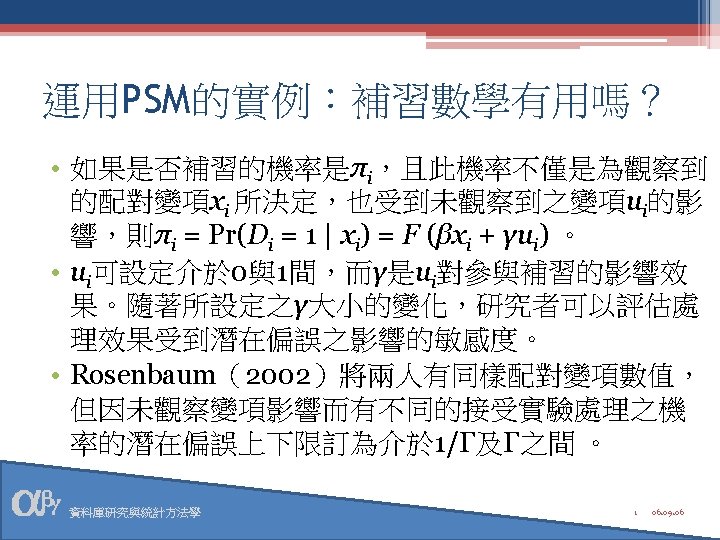
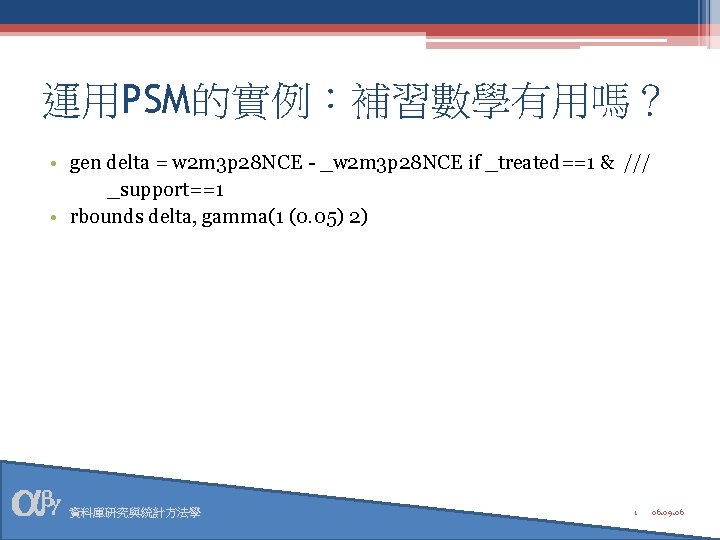
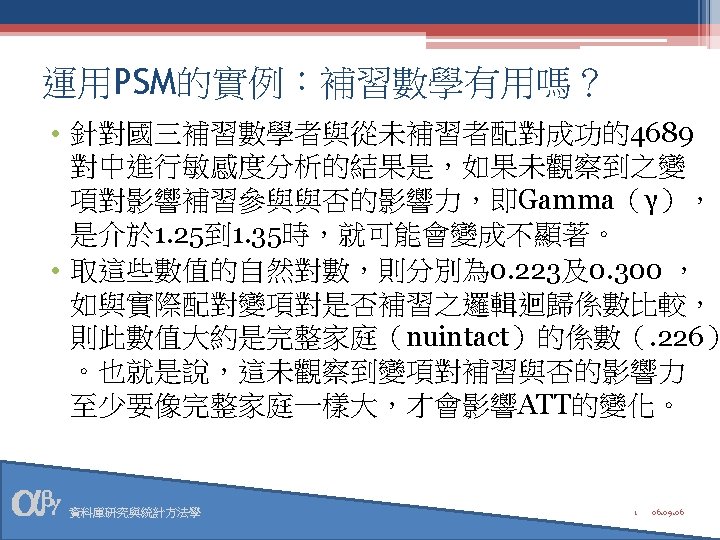
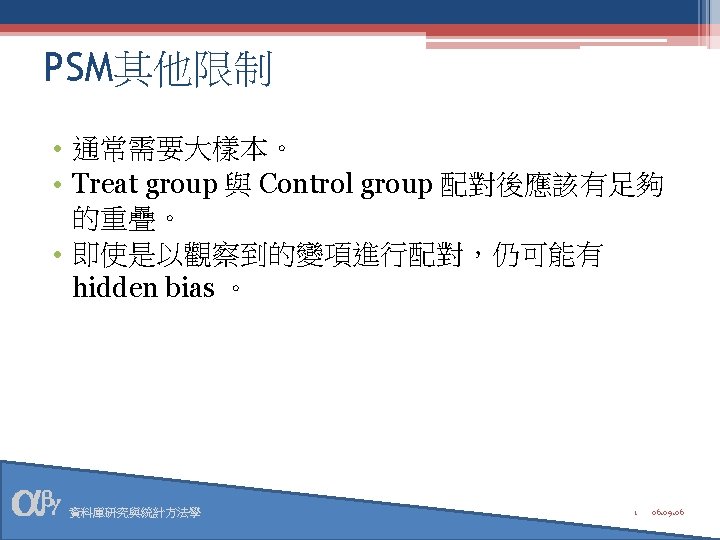
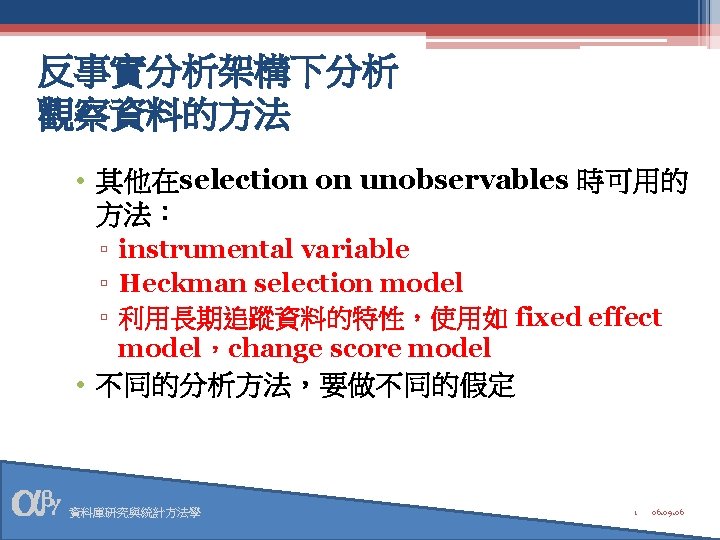
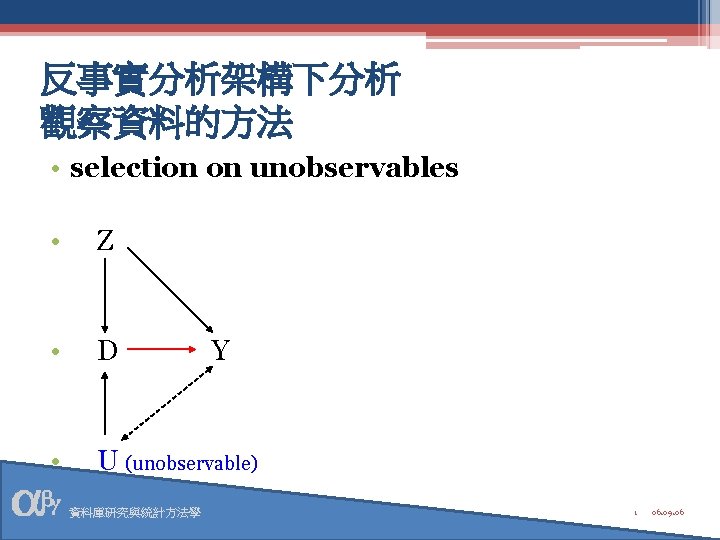
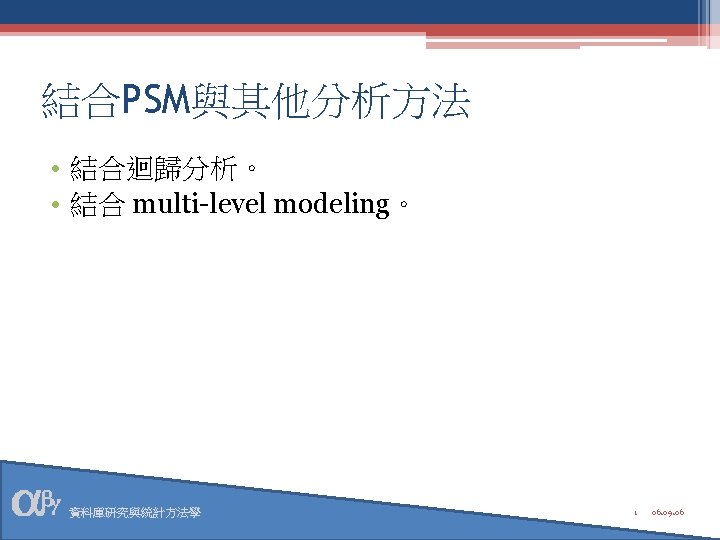
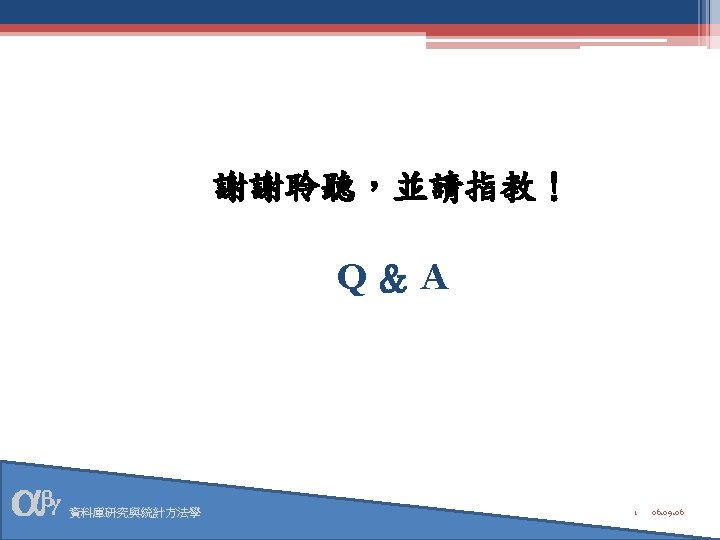
- Slides: 103
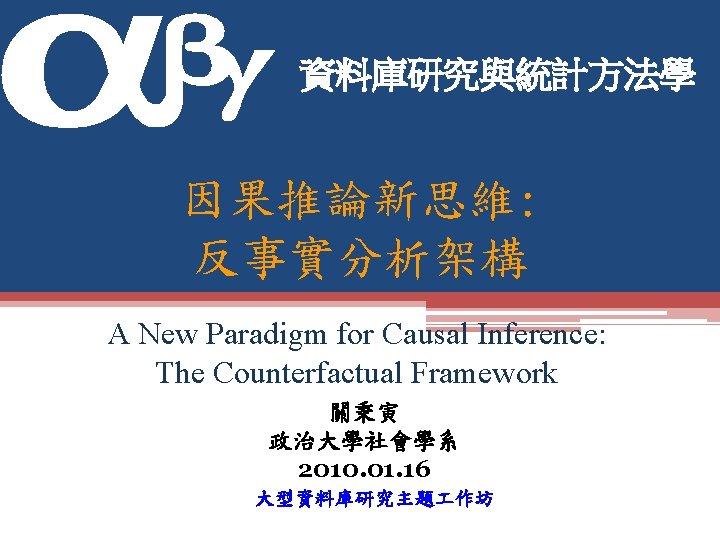
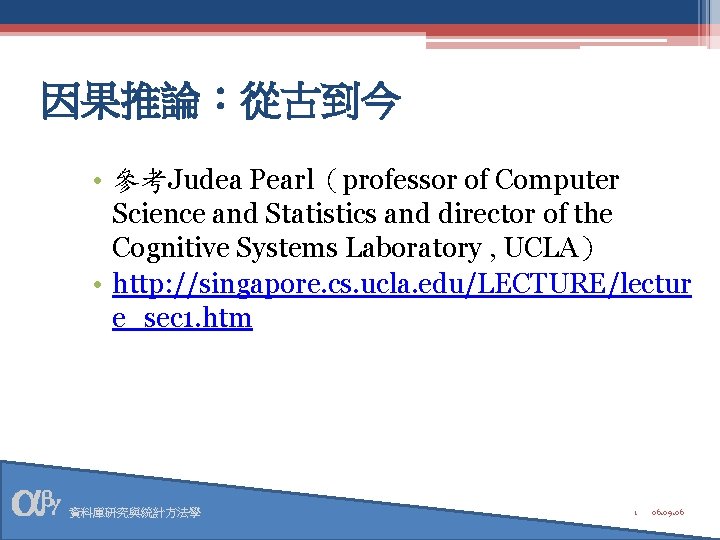
因果推論:從古到今 • 參考Judea Pearl(professor of Computer Science and Statistics and director of the Cognitive Systems Laboratory , UCLA) • http: //singapore. cs. ucla. edu/LECTURE/lectur e_sec 1. htm 資料庫研究與統計方法學 1 06. 09. 06
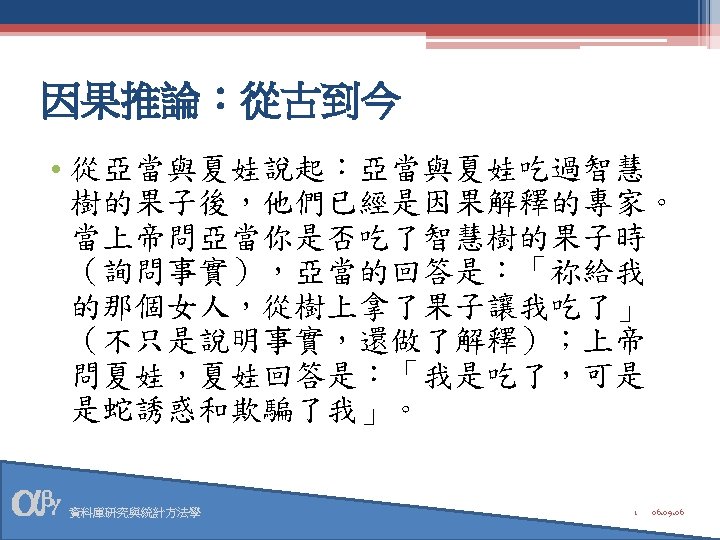
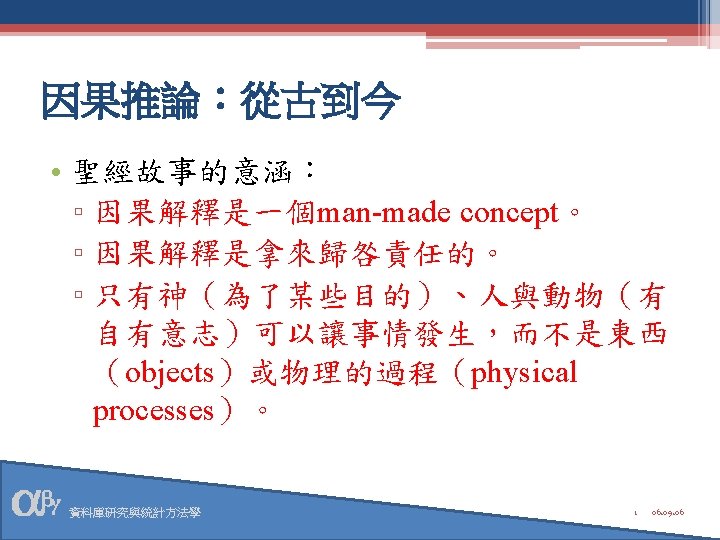
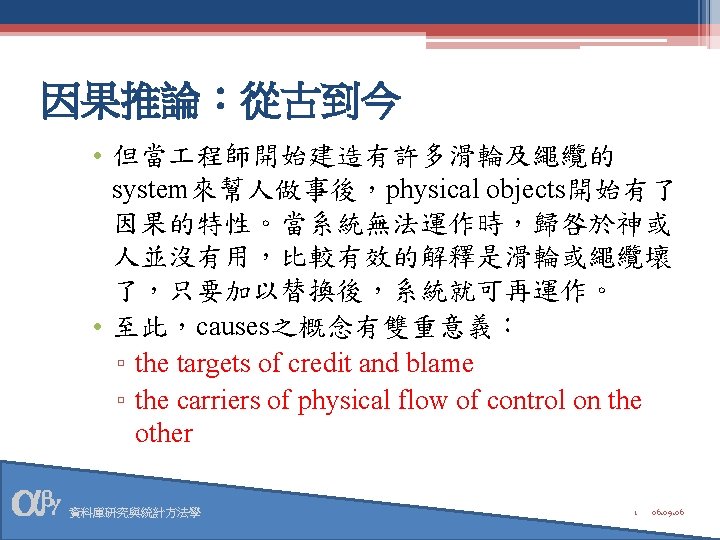
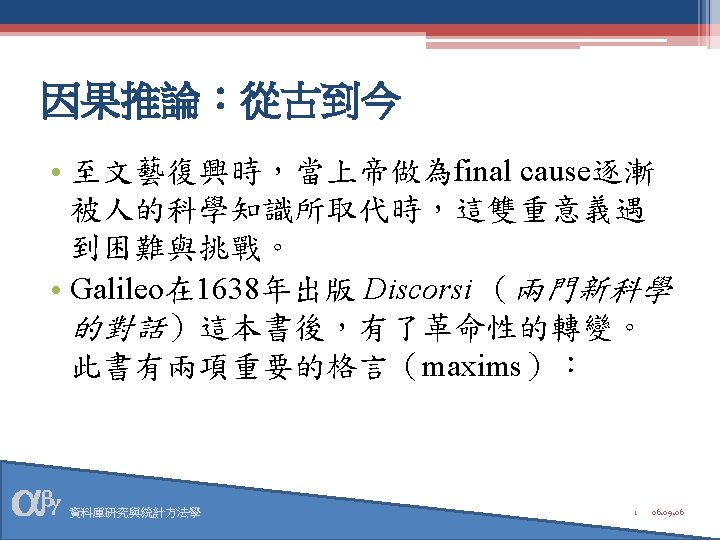
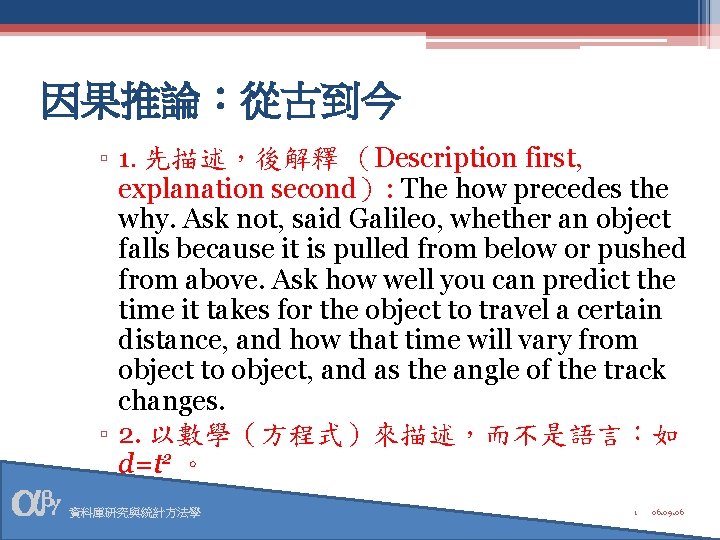
因果推論:從古到今 ▫ 1. 先描述,後解釋 (Description first, explanation second): The how precedes the why. Ask not, said Galileo, whether an object falls because it is pulled from below or pushed from above. Ask how well you can predict the time it takes for the object to travel a certain distance, and how that time will vary from object to object, and as the angle of the track changes. ▫ 2. 以數學(方程式)來描述,而不是語言:如 d=t 2 。 資料庫研究與統計方法學 1 06. 09. 06
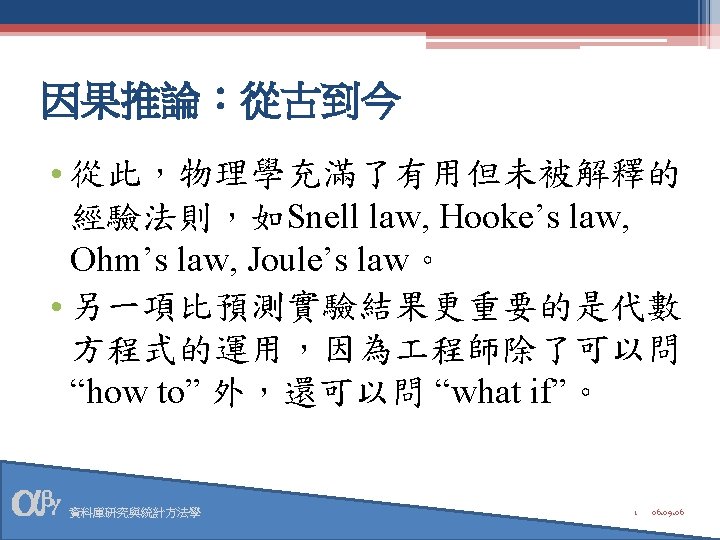
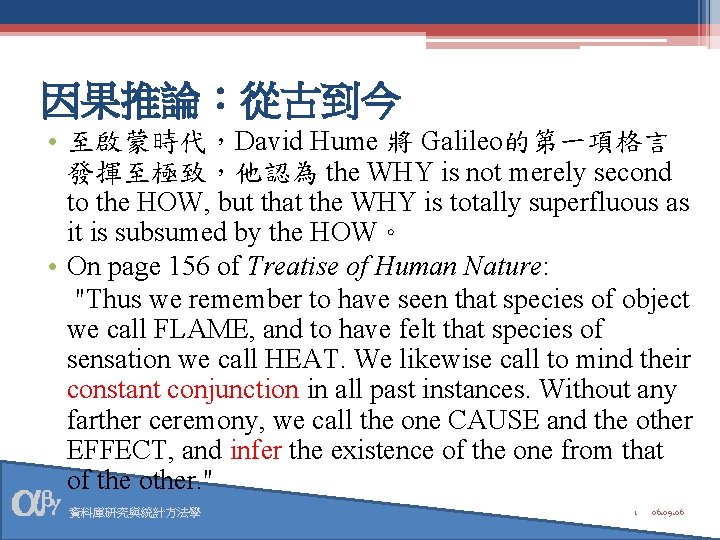
因果推論:從古到今 • 至啟蒙時代,David Hume 將 Galileo的第一項格言 發揮至極致,他認為 the WHY is not merely second to the HOW, but that the WHY is totally superfluous as it is subsumed by the HOW。 • On page 156 of Treatise of Human Nature: "Thus we remember to have seen that species of object we call FLAME, and to have felt that species of sensation we call HEAT. We likewise call to mind their constant conjunction in all past instances. Without any farther ceremony, we call the one CAUSE and the other EFFECT, and infer the existence of the one from that of the other. " 資料庫研究與統計方法學 1 06. 09. 06
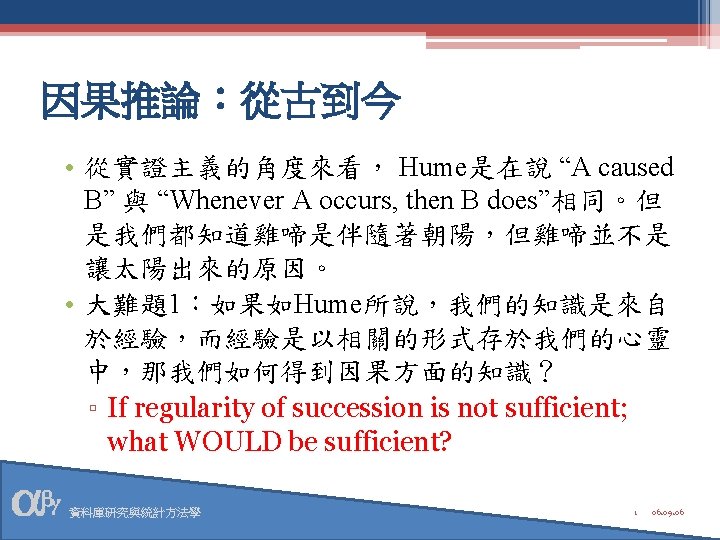
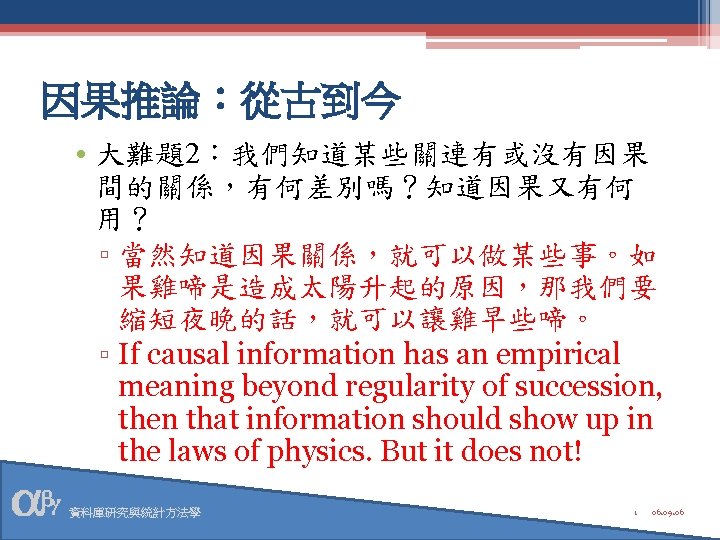
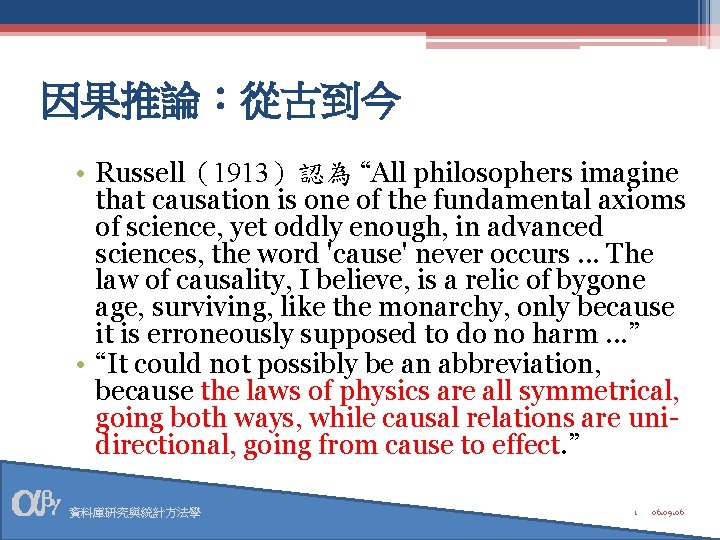
因果推論:從古到今 • Russell(1913)認為 “All philosophers imagine that causation is one of the fundamental axioms of science, yet oddly enough, in advanced sciences, the word 'cause' never occurs. . . The law of causality, I believe, is a relic of bygone age, surviving, like the monarchy, only because it is erroneously supposed to do no harm. . . ” • “It could not possibly be an abbreviation, because the laws of physics are all symmetrical, going both ways, while causal relations are unidirectional, going from cause to effect. ” 資料庫研究與統計方法學 1 06. 09. 06
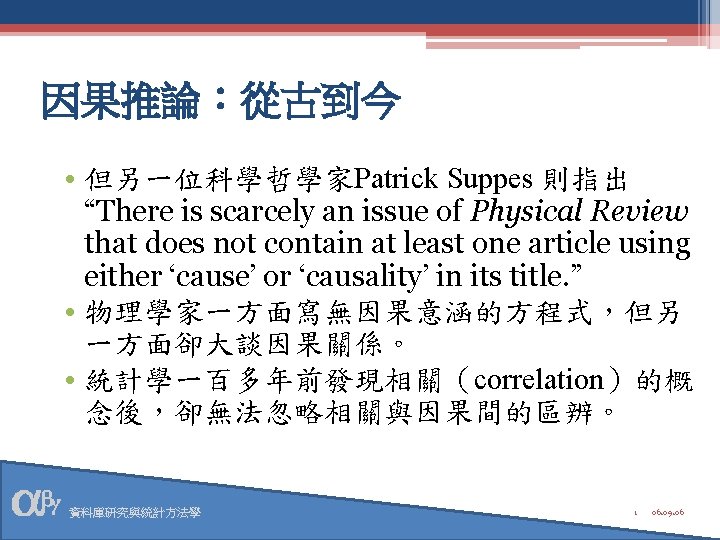
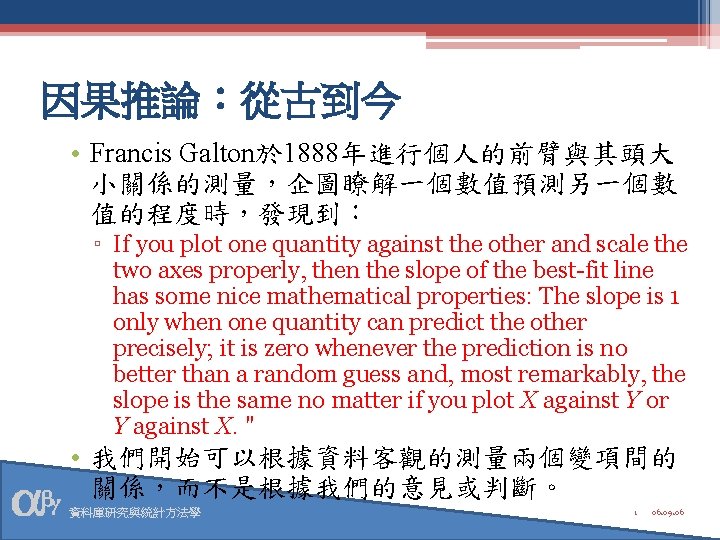
因果推論:從古到今 • Francis Galton於 1888年進行個人的前臂與其頭大 小關係的測量,企圖瞭解一個數值預測另一個數 值的程度時,發現到: ▫ If you plot one quantity against the other and scale the two axes properly, then the slope of the best-fit line has some nice mathematical properties: The slope is 1 only when one quantity can predict the other precisely; it is zero whenever the prediction is no better than a random guess and, most remarkably, the slope is the same no matter if you plot X against Y or Y against X. " • 我們開始可以根據資料客觀的測量兩個變項間的 關係,而不是根據我們的意見或判斷。 資料庫研究與統計方法學 1 06. 09. 06
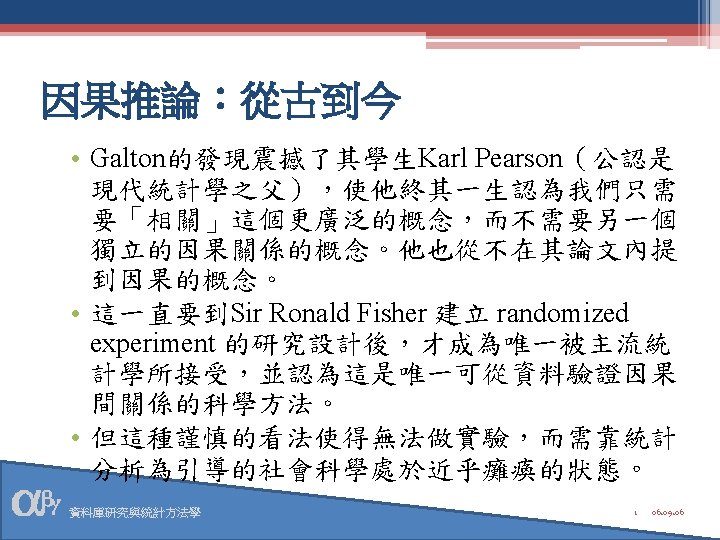
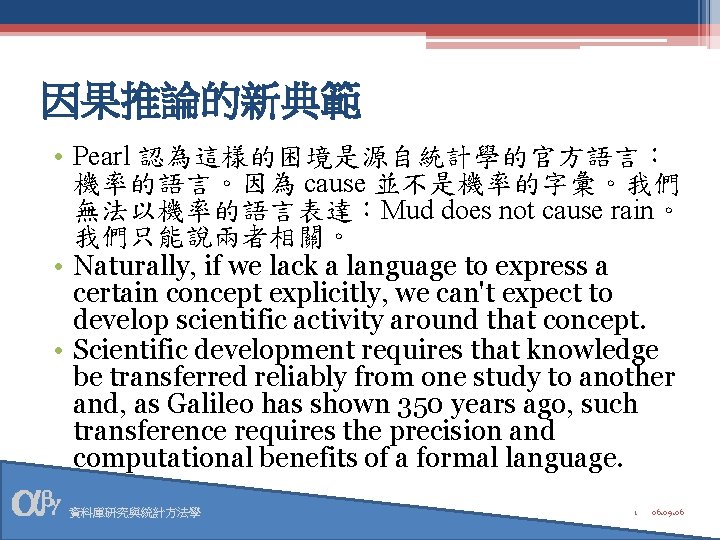
因果推論的新典範 • Pearl 認為這樣的困境是源自統計學的官方語言: 機率的語言。因為 cause 並不是機率的字彙。我們 無法以機率的語言表達:Mud does not cause rain。 我們只能說兩者相關。 • Naturally, if we lack a language to express a certain concept explicitly, we can't expect to develop scientific activity around that concept. • Scientific development requires that knowledge be transferred reliably from one study to another and, as Galileo has shown 350 years ago, such transference requires the precision and computational benefits of a formal language. 資料庫研究與統計方法學 1 06. 09. 06
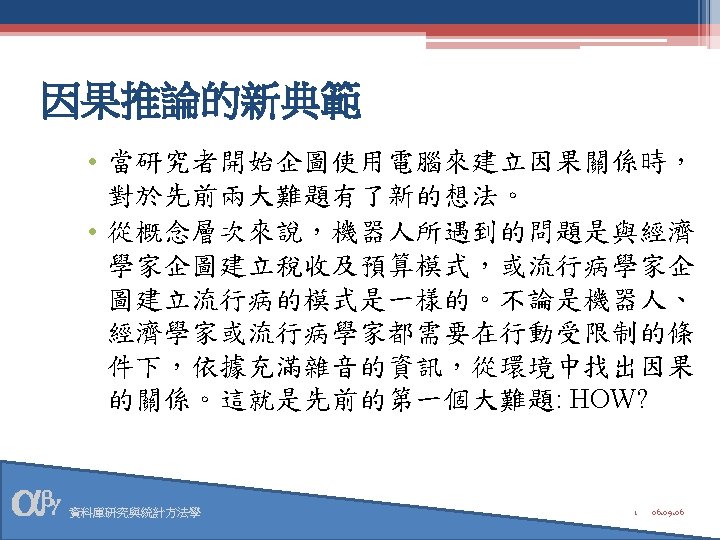
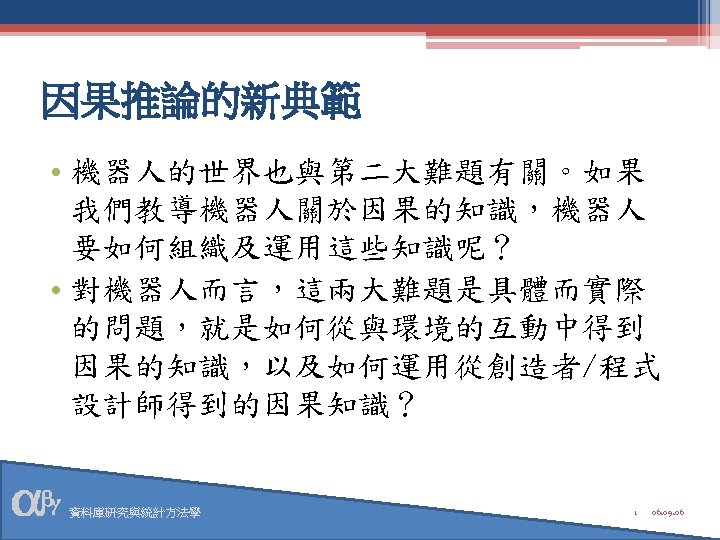
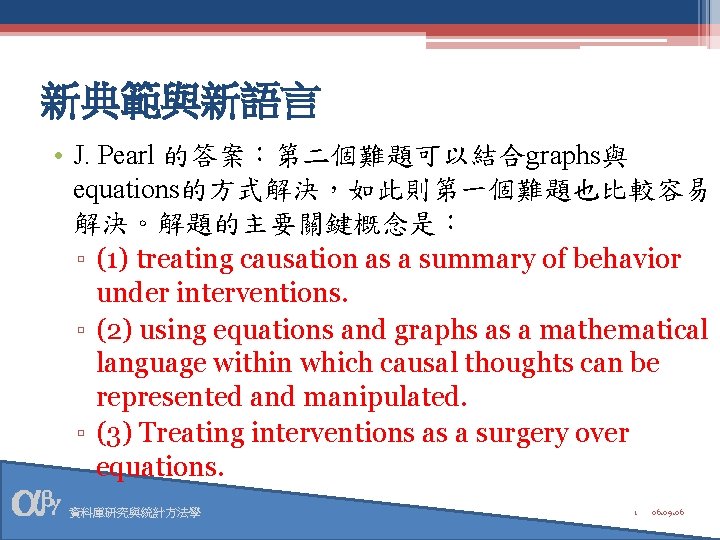
新典範與新語言 • J. Pearl 的答案:第二個難題可以結合graphs與 equations的方式解決,如此則第一個難題也比較容易 解決。解題的主要關鍵概念是: ▫ (1) treating causation as a summary of behavior under interventions. ▫ (2) using equations and graphs as a mathematical language within which causal thoughts can be represented and manipulated. ▫ (3) Treating interventions as a surgery over equations. 資料庫研究與統計方法學 1 06. 09. 06
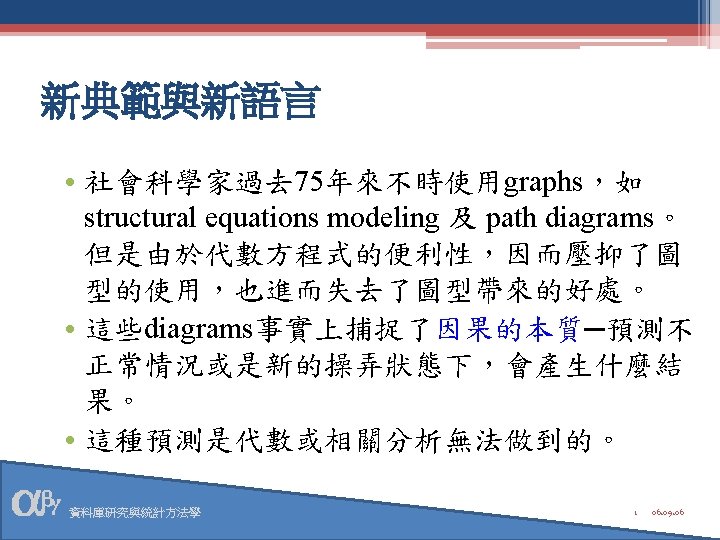
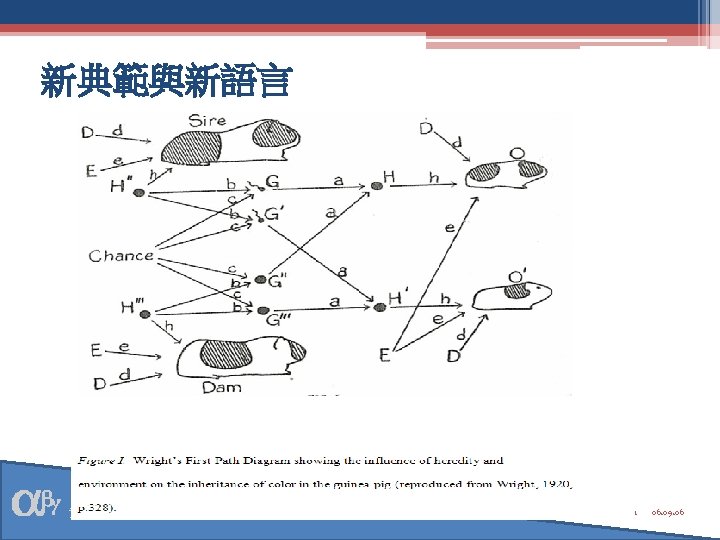
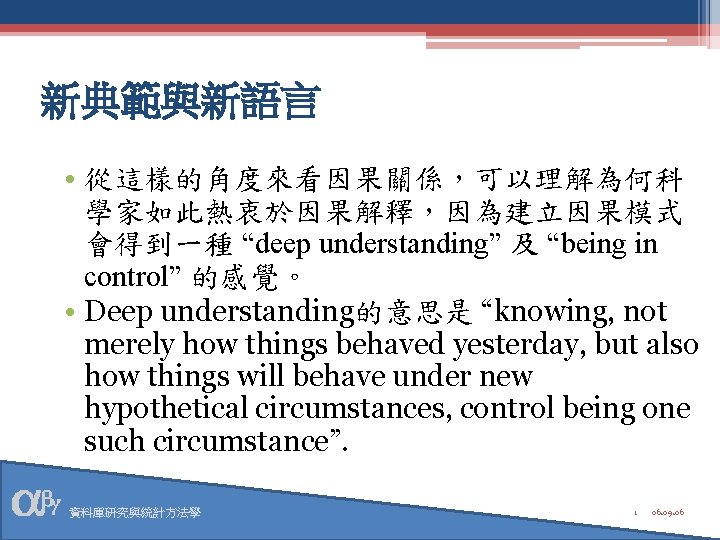
新典範與新語言 • 從這樣的角度來看因果關係,可以理解為何科 學家如此熱衷於因果解釋,因為建立因果模式 會得到一種 “deep understanding” 及 “being in control” 的感覺。 • Deep understanding的意思是 “knowing, not merely how things behaved yesterday, but also how things will behave under new hypothetical circumstances, control being one such circumstance”. 資料庫研究與統計方法學 1 06. 09. 06
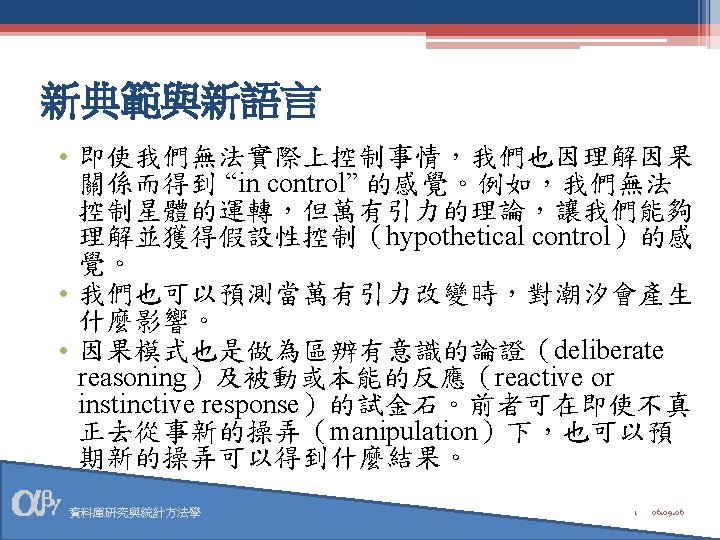
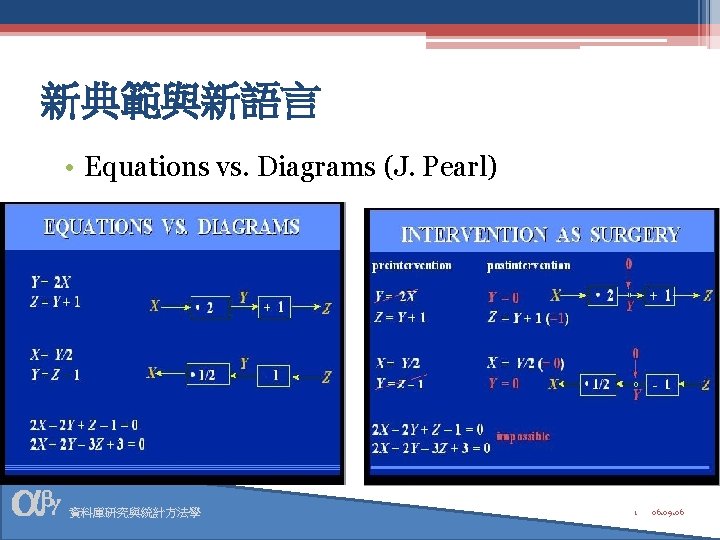
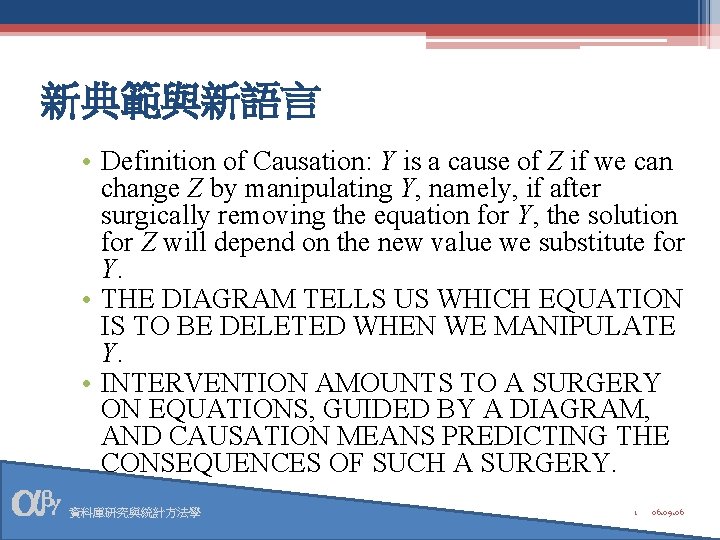
新典範與新語言 • Definition of Causation: Y is a cause of Z if we can change Z by manipulating Y, namely, if after surgically removing the equation for Y, the solution for Z will depend on the new value we substitute for Y. • THE DIAGRAM TELLS US WHICH EQUATION IS TO BE DELETED WHEN WE MANIPULATE Y. • INTERVENTION AMOUNTS TO A SURGERY ON EQUATIONS, GUIDED BY A DIAGRAM, AND CAUSATION MEANS PREDICTING THE CONSEQUENCES OF SUCH A SURGERY. 資料庫研究與統計方法學 1 06. 09. 06
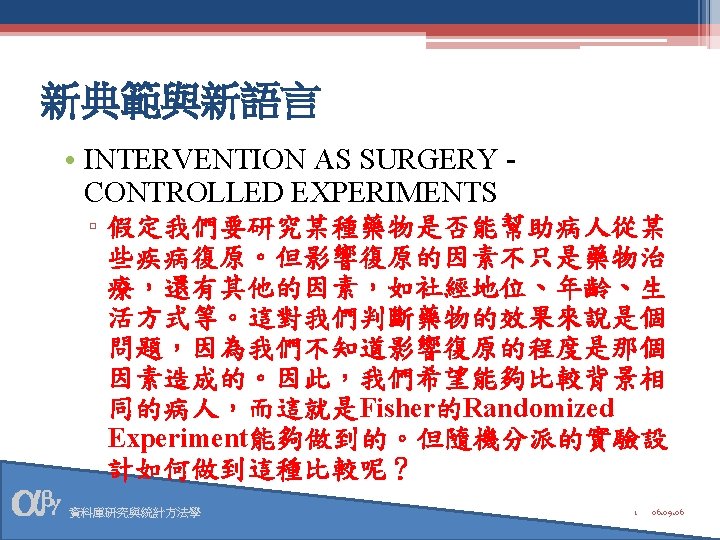
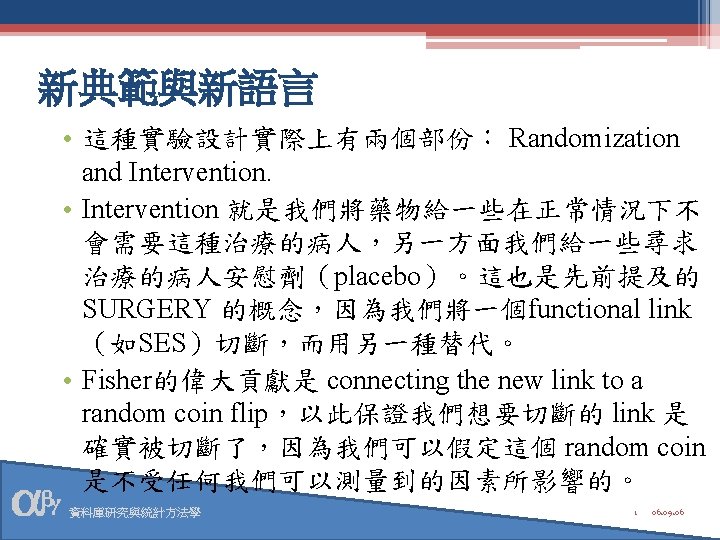
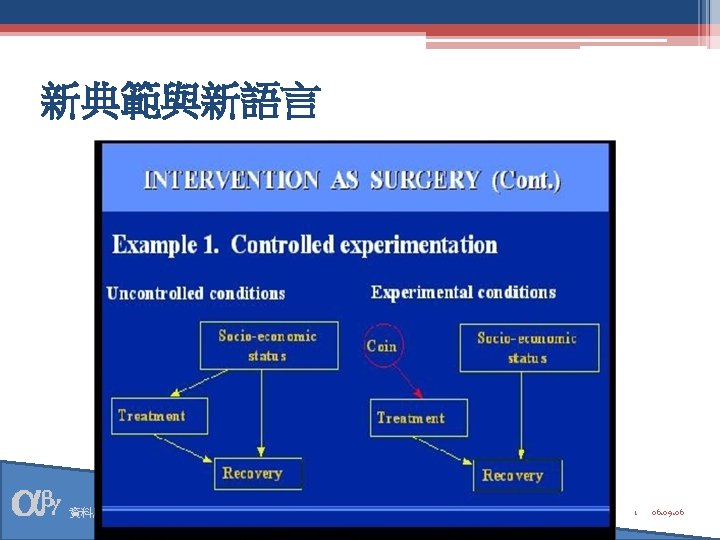
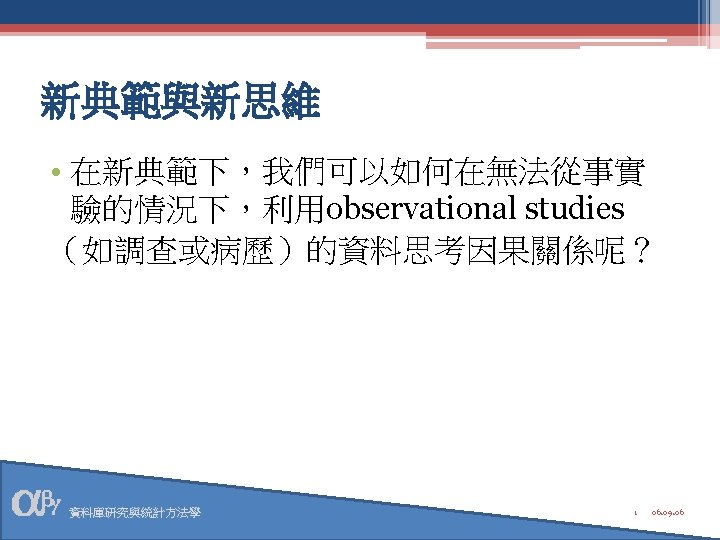
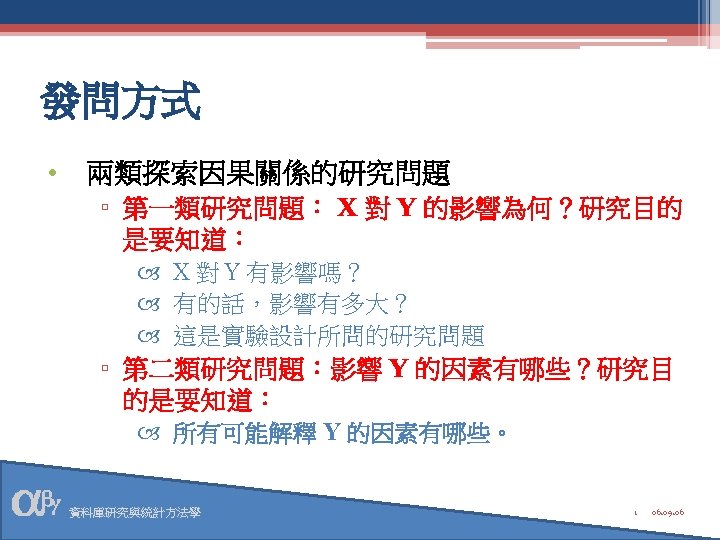
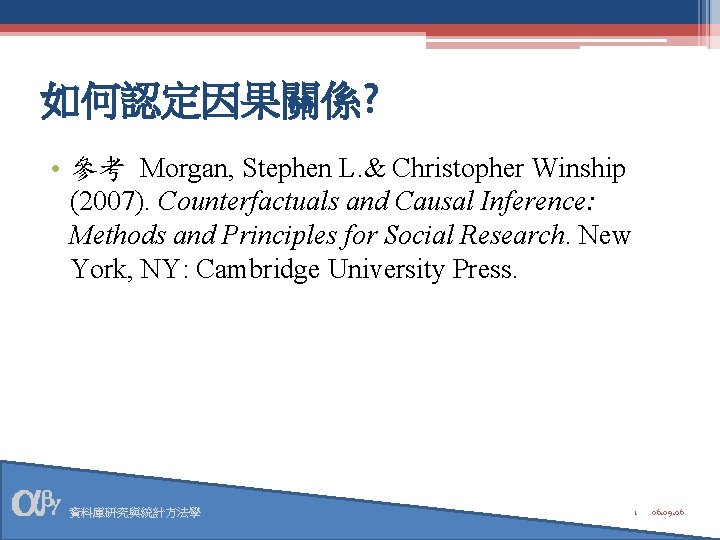
如何認定因果關係? • 參考 Morgan, Stephen L. & Christopher Winship (2007). Counterfactuals and Causal Inference: Methods and Principles for Social Research. New York, NY: Cambridge University Press. 資料庫研究與統計方法學 1 06. 09. 06
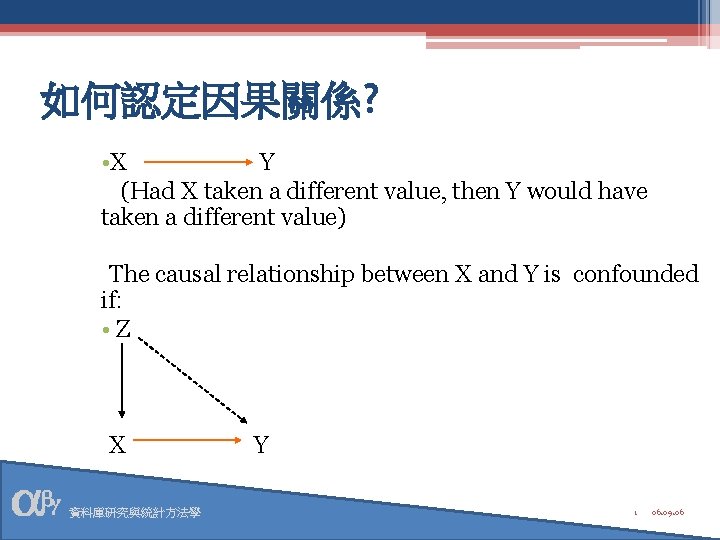
如何認定因果關係? • X Y (Had X taken a different value, then Y would have taken a different value) The causal relationship between X and Y is confounded if: • Z X 資料庫研究與統計方法學 Y 1 06. 09. 06
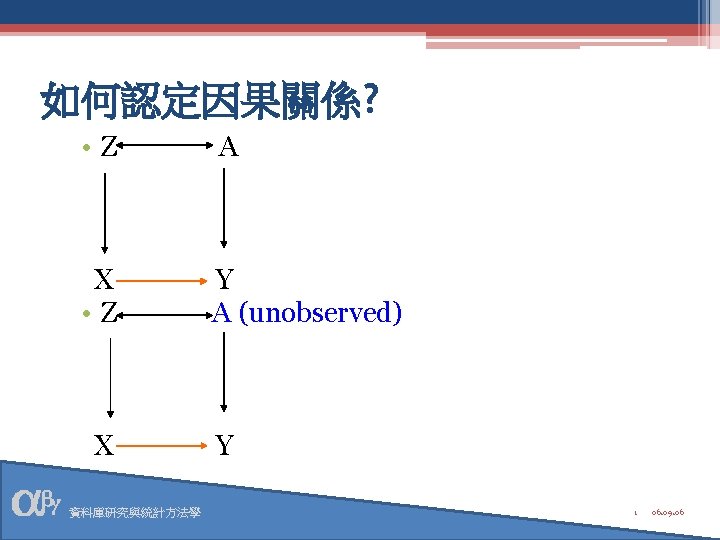
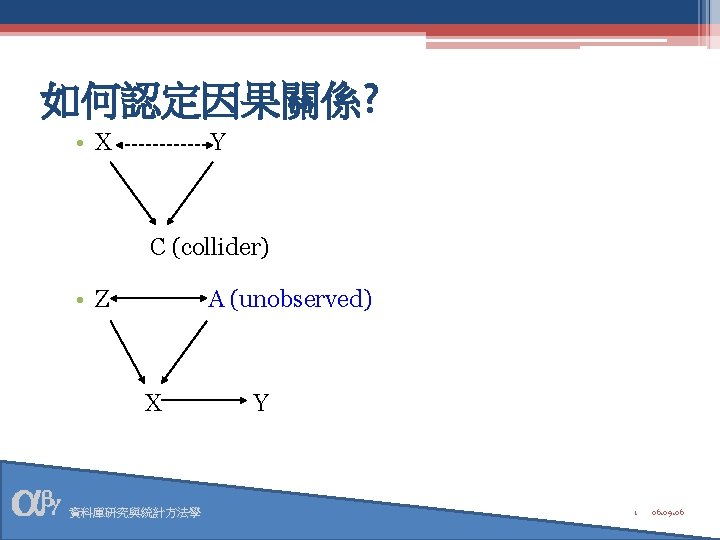
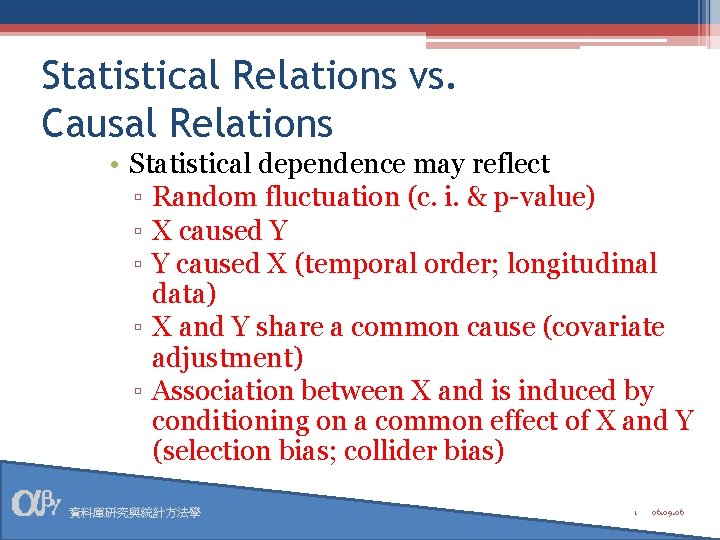
Statistical Relations vs. Causal Relations • Statistical dependence may reflect ▫ Random fluctuation (c. i. & p-value) ▫ X caused Y ▫ Y caused X (temporal order; longitudinal data) ▫ X and Y share a common cause (covariate adjustment) ▫ Association between X and is induced by conditioning on a common effect of X and Y (selection bias; collider bias) 資料庫研究與統計方法學 1 06. 09. 06
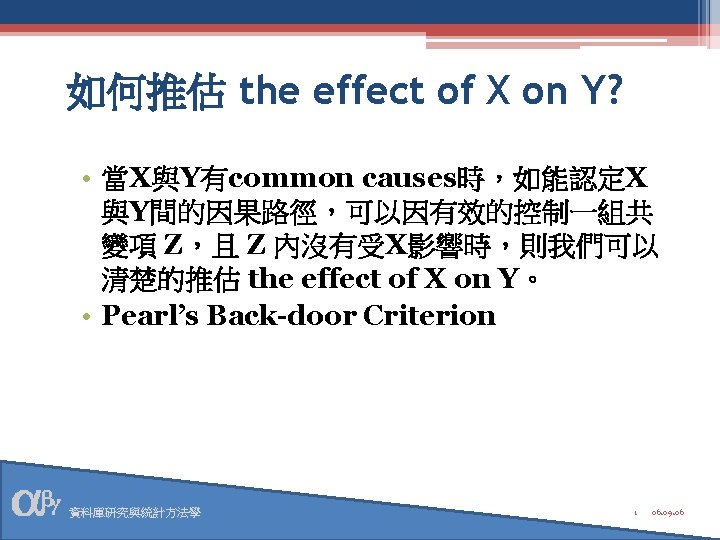
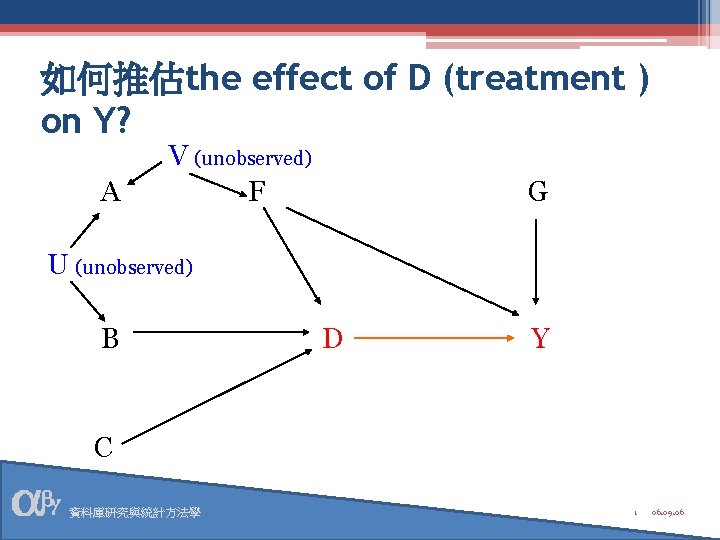
如何推估the effect of D (treatment ) on Y? A V (unobserved) F G U (unobserved) B D Y C 資料庫研究與統計方法學 1 06. 09. 06
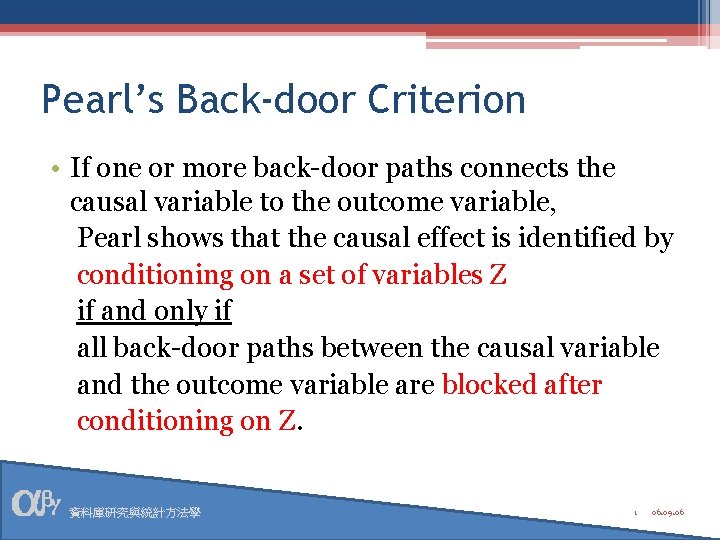
Pearl’s Back-door Criterion • If one or more back-door paths connects the causal variable to the outcome variable, Pearl shows that the causal effect is identified by conditioning on a set of variables Z if and only if all back-door paths between the causal variable and the outcome variable are blocked after conditioning on Z. 資料庫研究與統計方法學 1 06. 09. 06
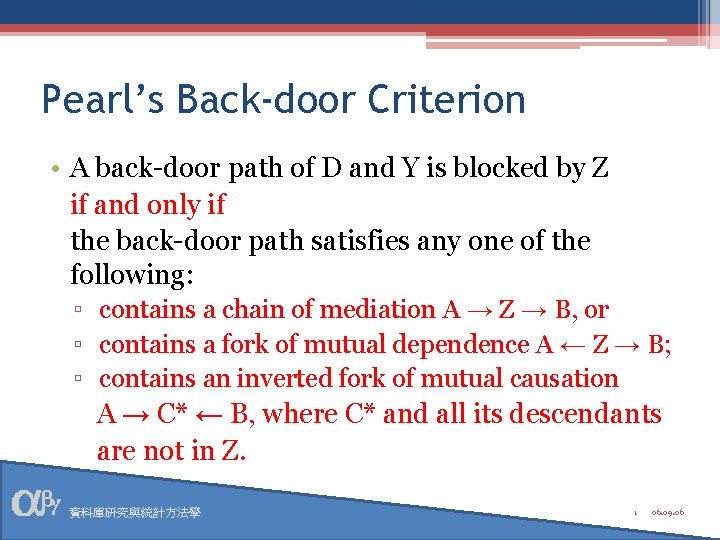
Pearl’s Back-door Criterion • A back-door path of D and Y is blocked by Z if and only if the back-door path satisfies any one of the following: ▫ contains a chain of mediation A → Z → B, or ▫ contains a fork of mutual dependence A ← Z → B; ▫ contains an inverted fork of mutual causation A → C* ← B, where C* and all its descendants are not in Z. 資料庫研究與統計方法學 1 06. 09. 06
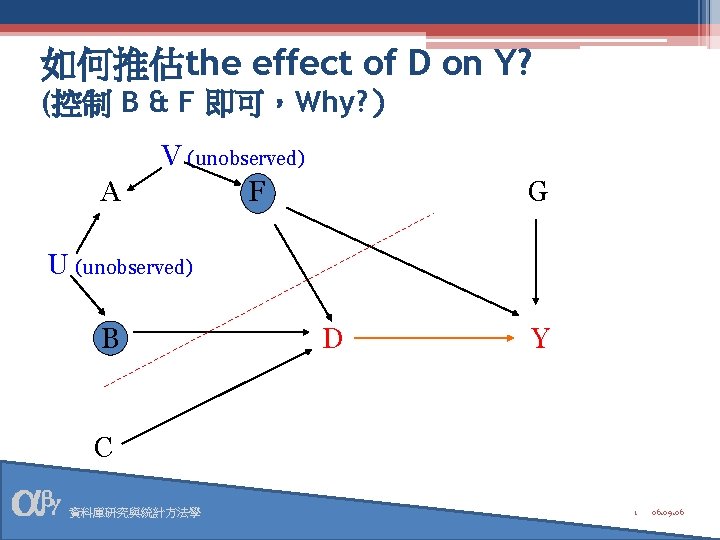
如何推估the effect of D on Y? (控制 B & F 即可,Why? ) A V (unobserved) F G U (unobserved) B D Y C 資料庫研究與統計方法學 1 06. 09. 06
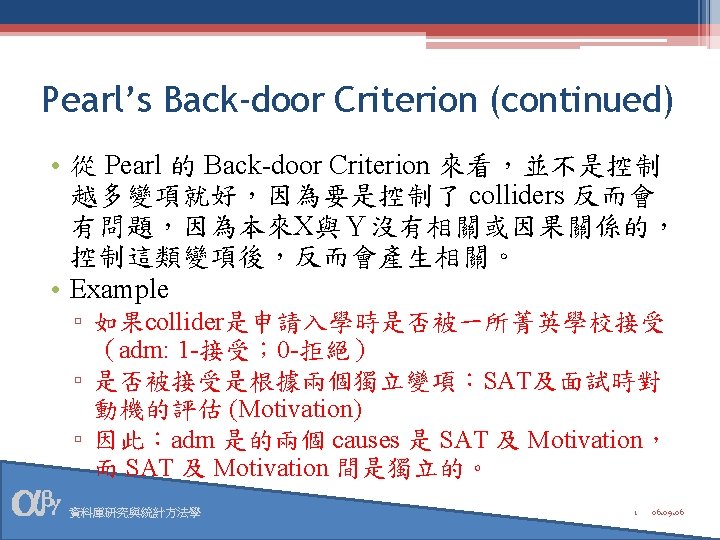
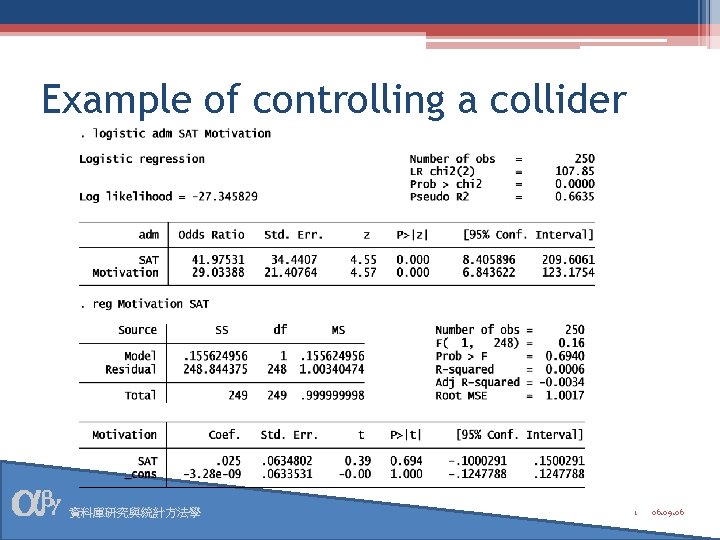
Example of controlling a collider 資料庫研究與統計方法學 1 06. 09. 06
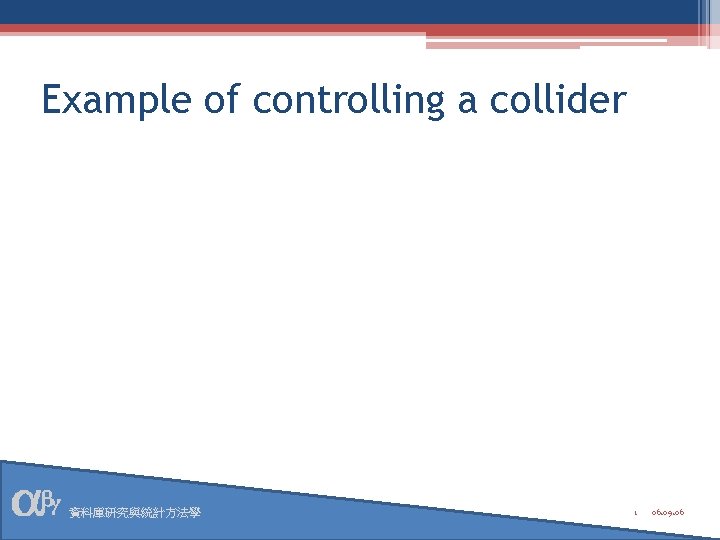
Example of controlling a collider 資料庫研究與統計方法學 1 06. 09. 06
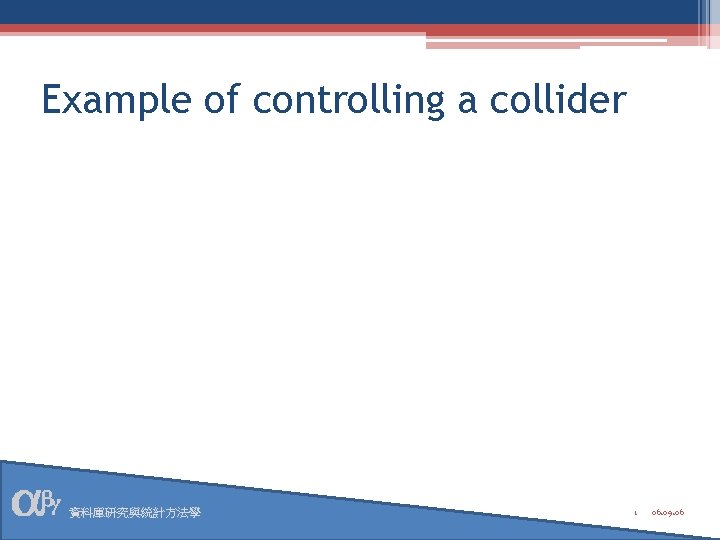
Example of controlling a collider 資料庫研究與統計方法學 1 06. 09. 06
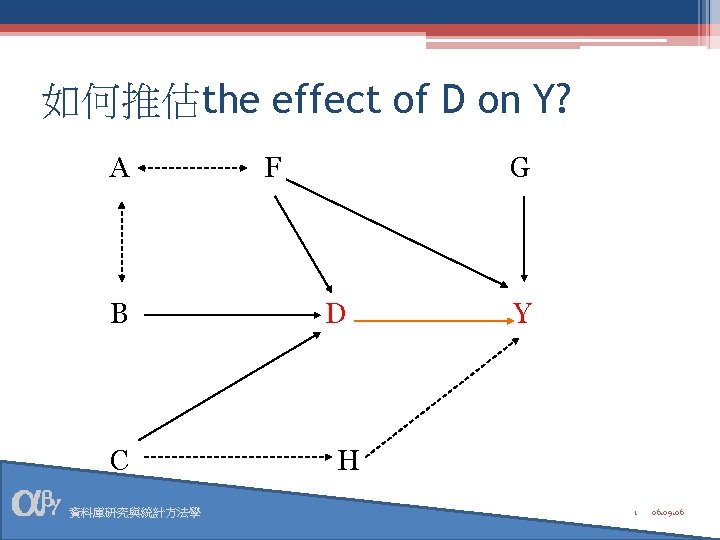
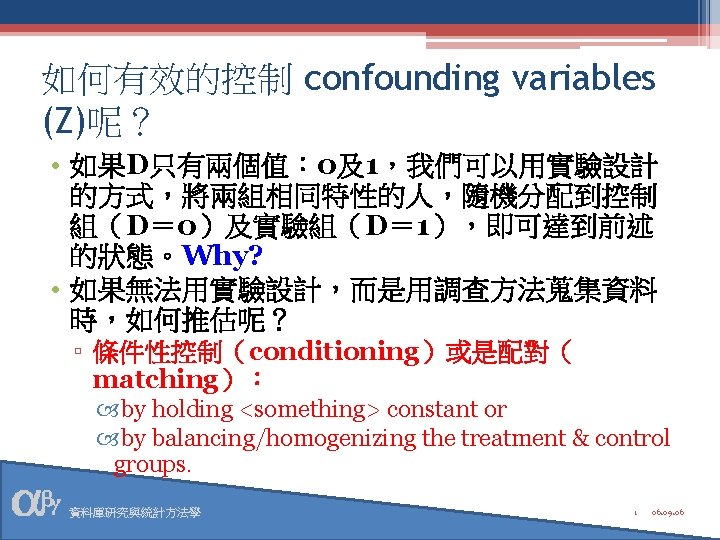
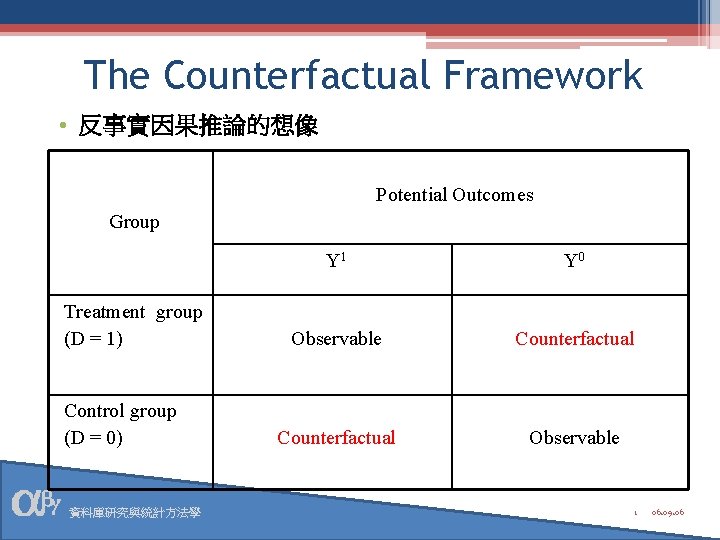
The Counterfactual Framework • 反事實因果推論的想像 Potential Outcomes Group Treatment group (D = 1) Control group (D = 0) 資料庫研究與統計方法學 Y 1 Y 0 Observable Counterfactual Observable 1 06. 09. 06
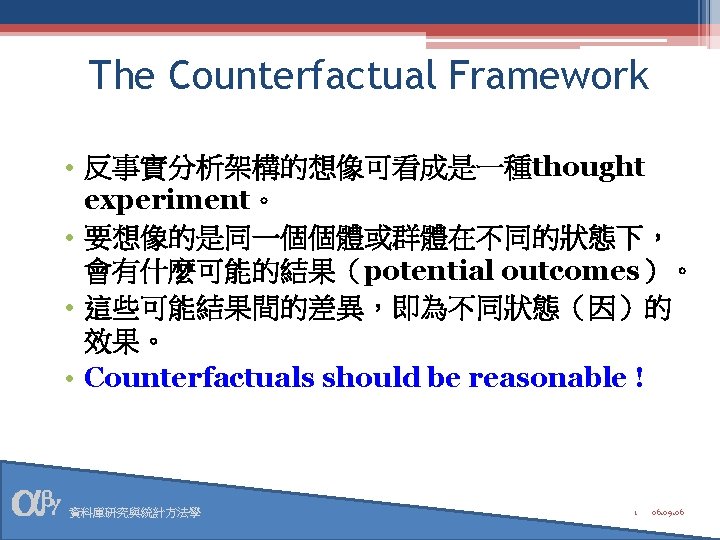
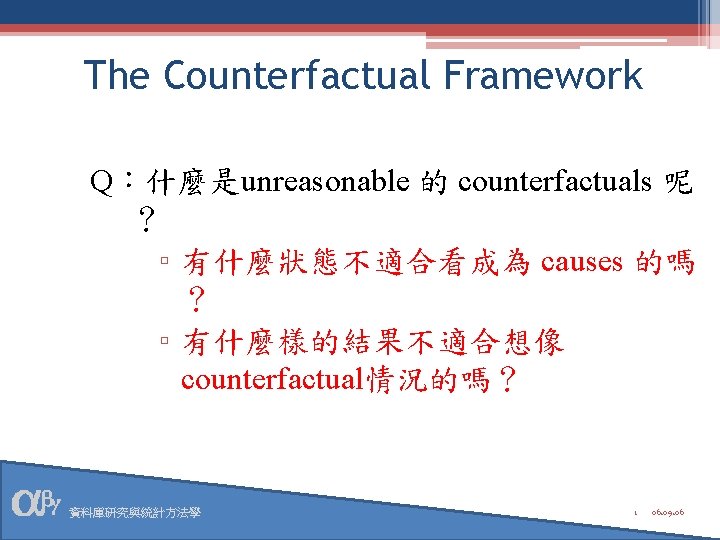
The Counterfactual Framework Q:什麼是unreasonable 的 counterfactuals 呢 ? ▫ 有什麼狀態不適合看成為 causes 的嗎 ? ▫ 有什麼樣的結果不適合想像 counterfactual情況的嗎? 資料庫研究與統計方法學 1 06. 09. 06
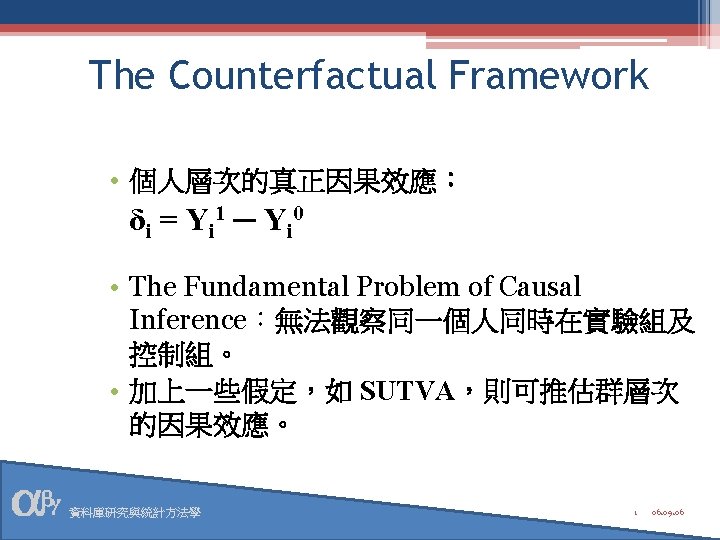
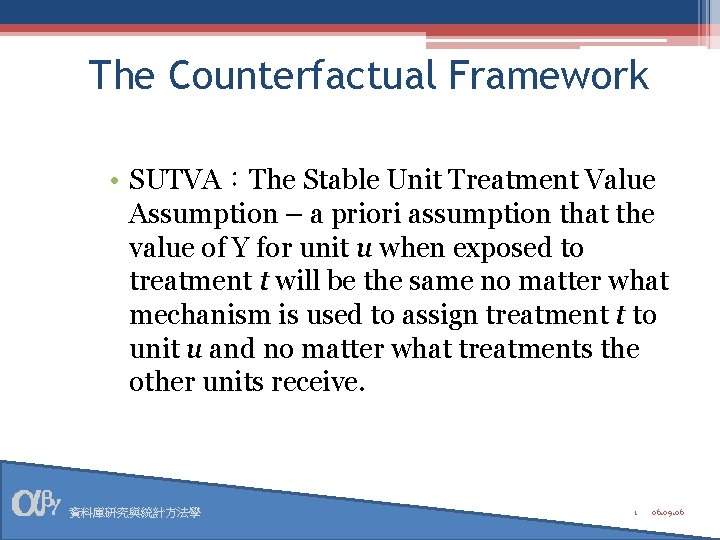
The Counterfactual Framework • SUTVA:The Stable Unit Treatment Value Assumption – a priori assumption that the value of Y for unit u when exposed to treatment t will be the same no matter what mechanism is used to assign treatment t to unit u and no matter what treatments the other units receive. 資料庫研究與統計方法學 1 06. 09. 06
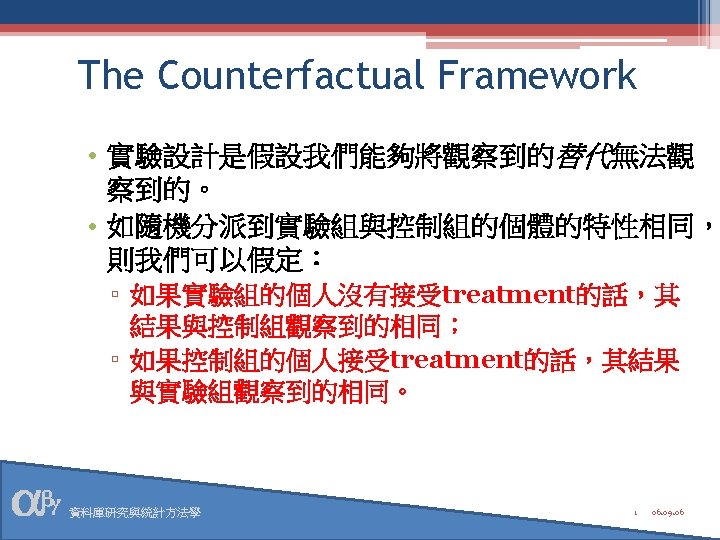
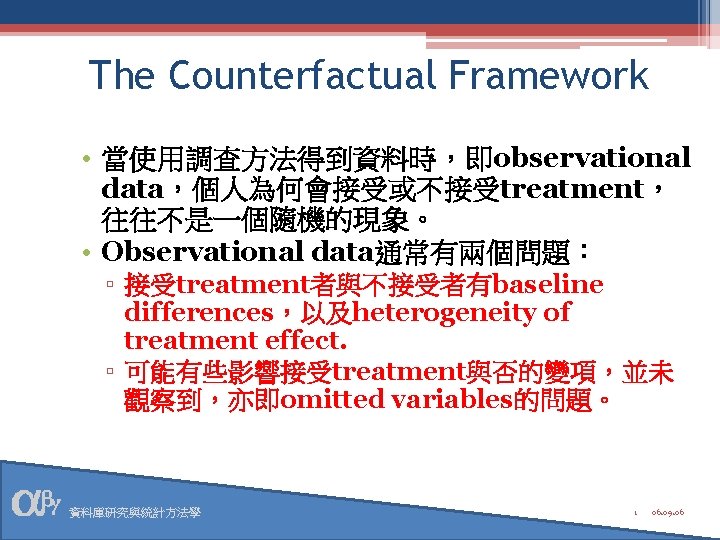
The Counterfactual Framework • 當使用調查方法得到資料時,即observational data,個人為何會接受或不接受treatment, 往往不是一個隨機的現象。 • Observational data通常有兩個問題: ▫ 接受treatment者與不接受者有baseline differences,以及heterogeneity of treatment effect. ▫ 可能有些影響接受treatment與否的變項,並未 觀察到,亦即omitted variables的問題。 資料庫研究與統計方法學 1 06. 09. 06
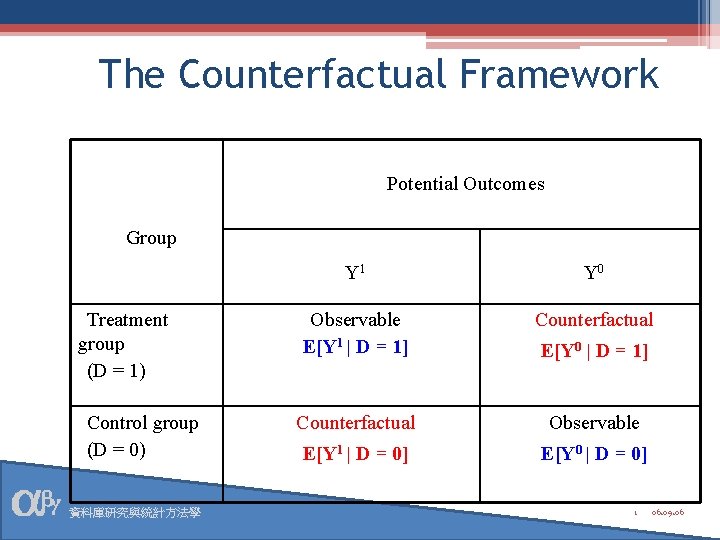
The Counterfactual Framework Potential Outcomes Group Treatment group (D = 1) Control group (D = 0) 資料庫研究與統計方法學 Y 1 Y 0 Observable E[Y 1 | D = 1] Counterfactual Observable E[Y 1 | D = 0] E[Y 0 | D = 1] 1 06. 09. 06
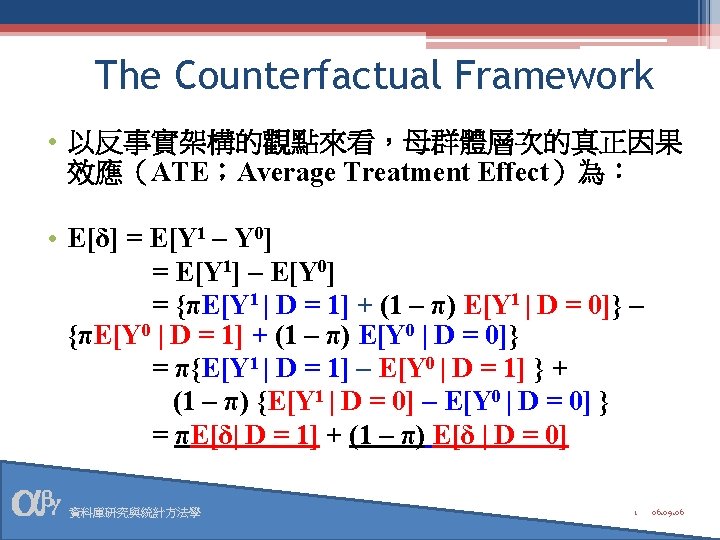
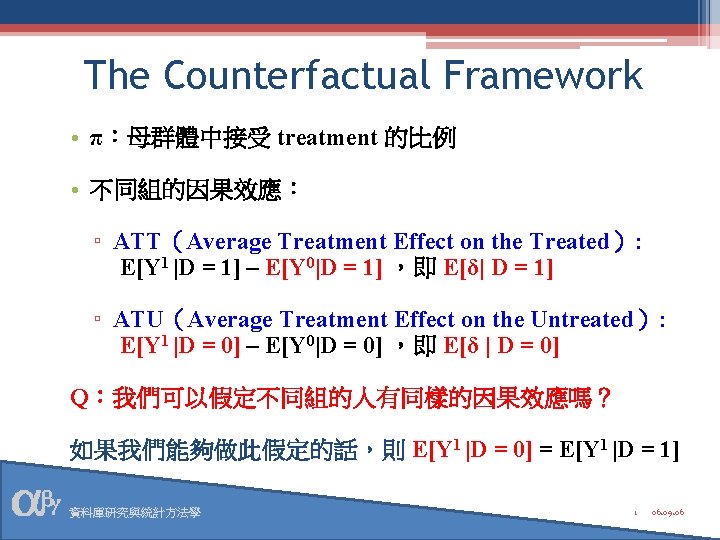
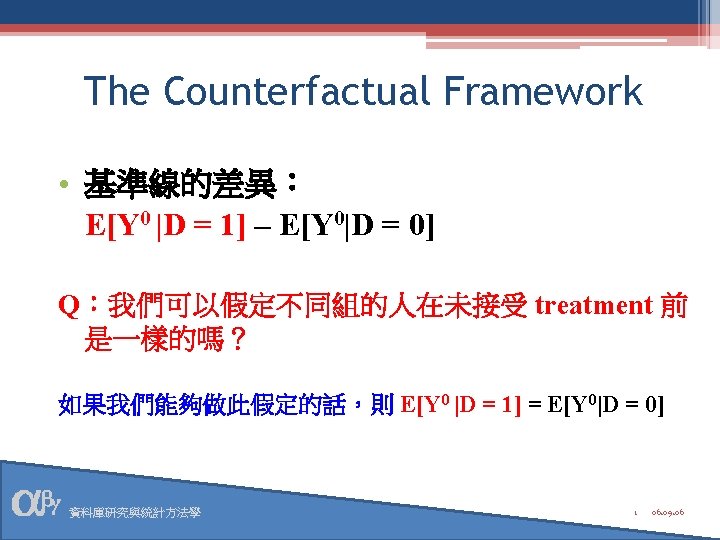
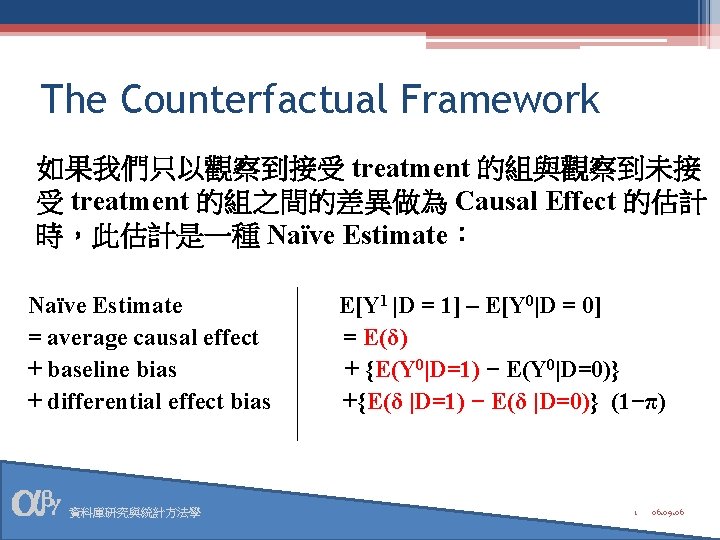
The Counterfactual Framework 如果我們只以觀察到接受 treatment 的組與觀察到未接 受 treatment 的組之間的差異做為 Causal Effect 的估計 時,此估計是一種 Naïve Estimate: Naïve Estimate = average causal effect + baseline bias + differential effect bias 資料庫研究與統計方法學 E[Y 1 |D = 1] – E[Y 0|D = 0] = E(δ) + {E(Y 0|D=1) − E(Y 0|D=0)} +{E(δ |D=1) − E(δ |D=0)} (1−π) 1 06. 09. 06
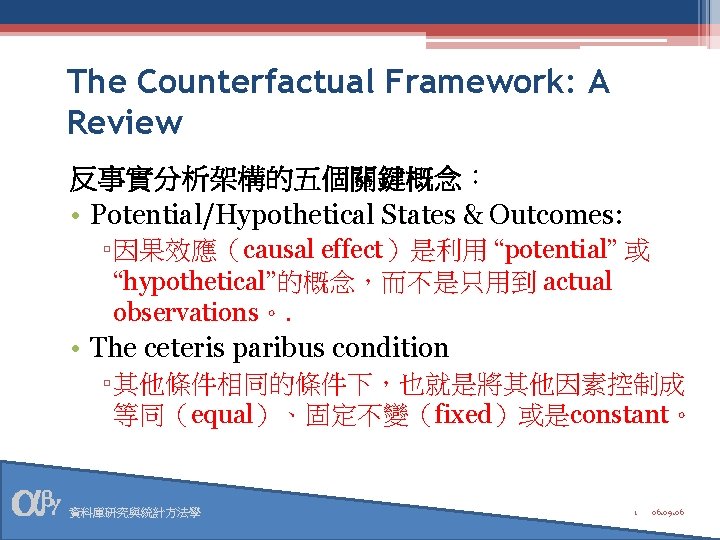
The Counterfactual Framework: A Review 反事實分析架構的五個關鍵概念: • Potential/Hypothetical States & Outcomes: ▫ 因果效應(causal effect)是利用 “potential” 或 “hypothetical”的概念,而不是只用到 actual observations。. • The ceteris paribus condition ▫ 其他條件相同的條件下,也就是將其他因素控制成 等同(equal)、固定不變(fixed)或是constant。 資料庫研究與統計方法學 1 06. 09. 06
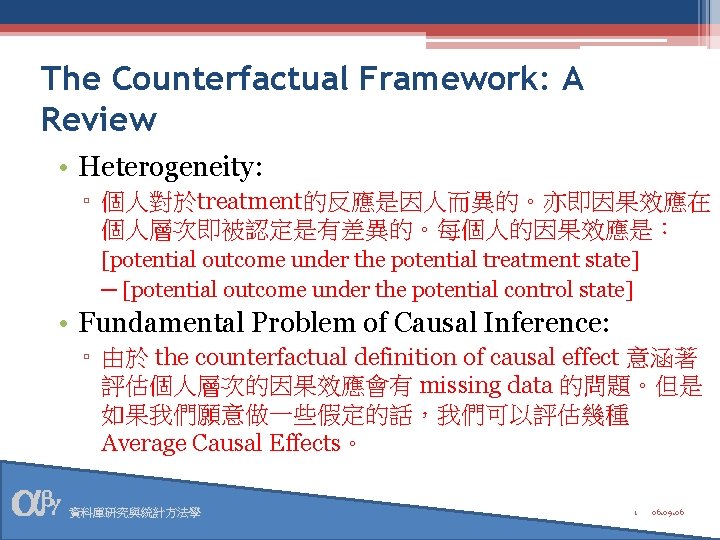
The Counterfactual Framework: A Review • Heterogeneity: ▫ 個人對於treatment的反應是因人而異的。亦即因果效應在 個人層次即被認定是有差異的。每個人的因果效應是: [potential outcome under the potential treatment state] ─ [potential outcome under the potential control state] • Fundamental Problem of Causal Inference: ▫ 由於 the counterfactual definition of causal effect 意涵著 評估個人層次的因果效應會有 missing data 的問題。但是 如果我們願意做一些假定的話,我們可以評估幾種 Average Causal Effects。 資料庫研究與統計方法學 1 06. 09. 06
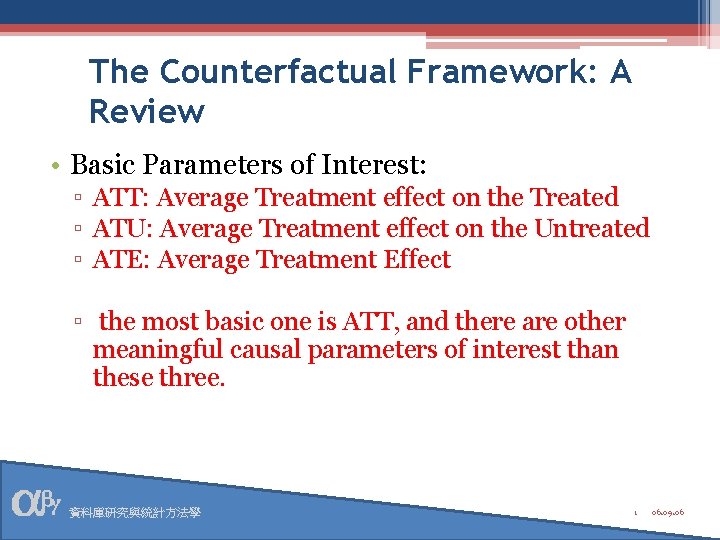
The Counterfactual Framework: A Review • Basic Parameters of Interest: ▫ ATT: Average Treatment effect on the Treated ▫ ATU: Average Treatment effect on the Untreated ▫ ATE: Average Treatment Effect ▫ the most basic one is ATT, and there are other meaningful causal parameters of interest than these three. 資料庫研究與統計方法學 1 06. 09. 06
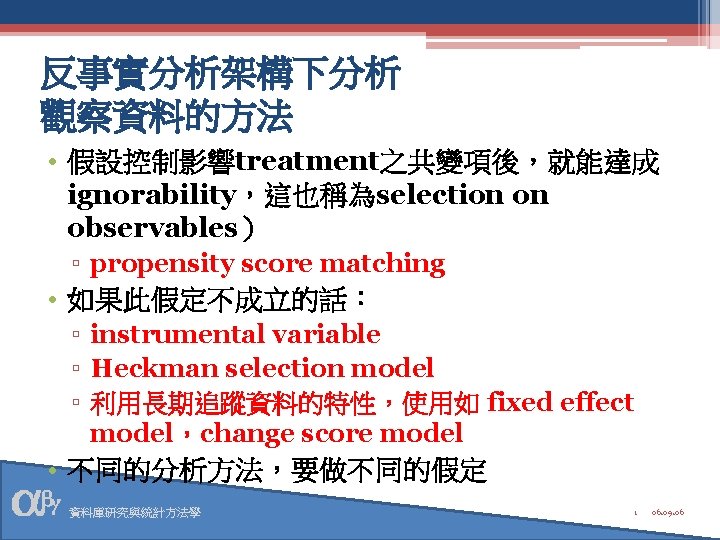
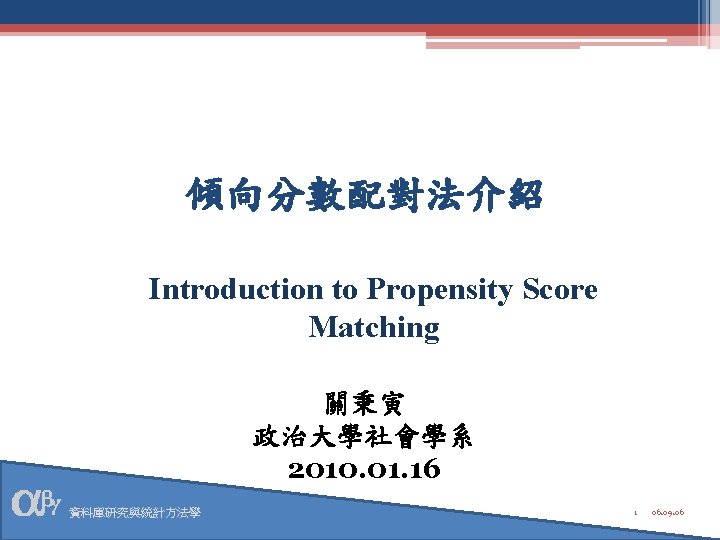
傾向分數配對法介紹 Introduction to Propensity Score Matching 關秉寅 政治大學社會學系 2010. 01. 16 資料庫研究與統計方法學 1 06. 09. 06
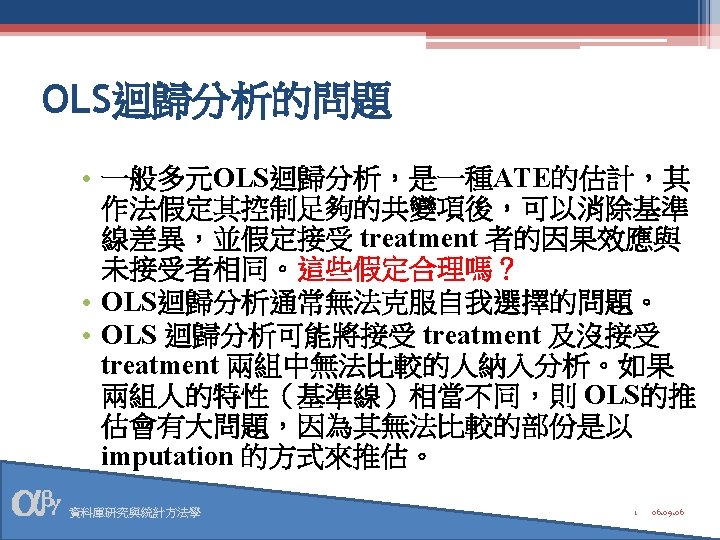
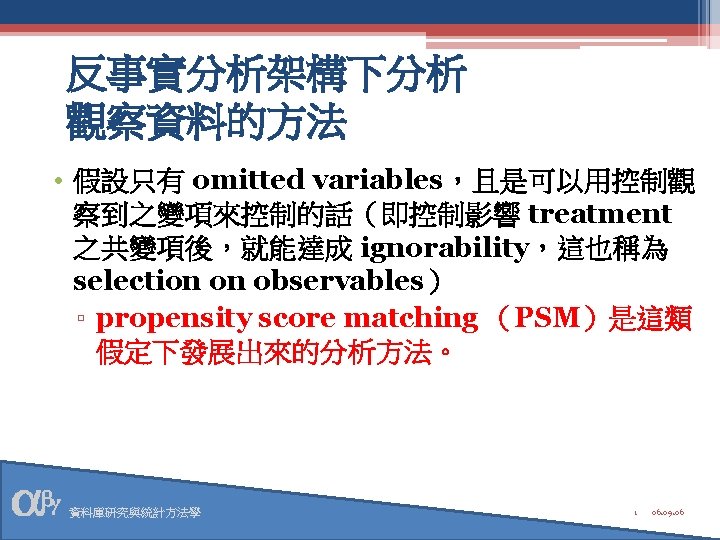
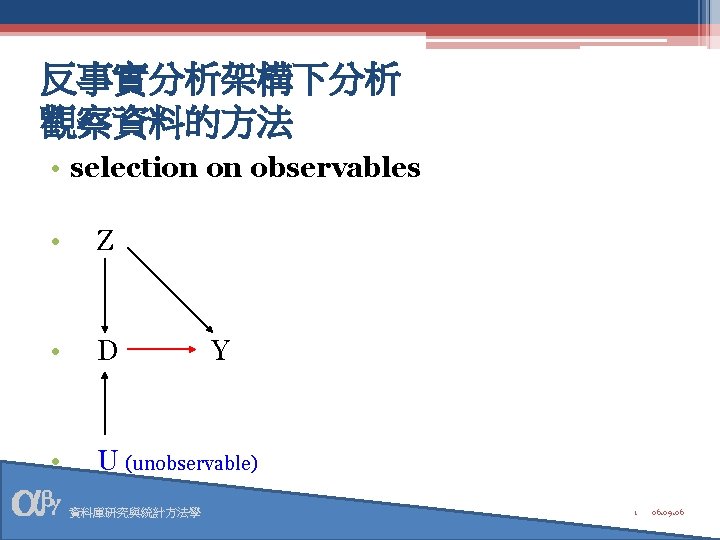
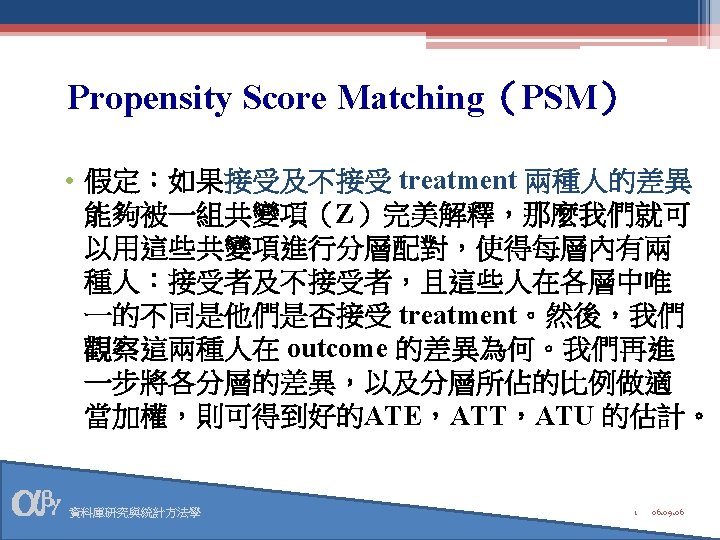
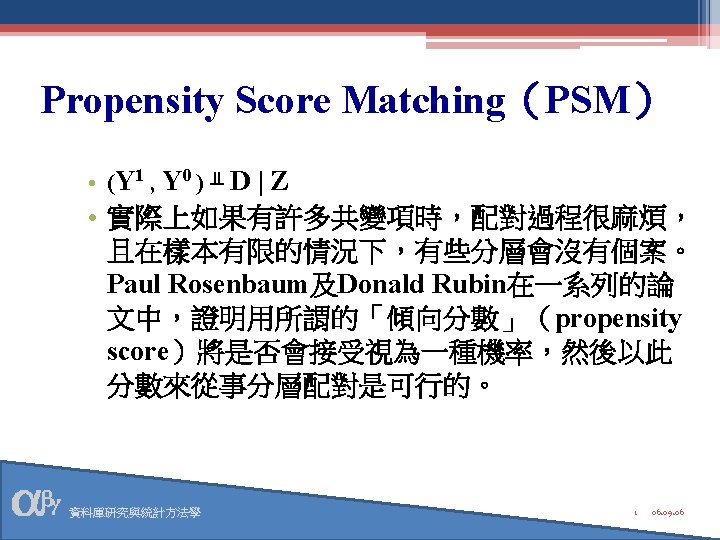
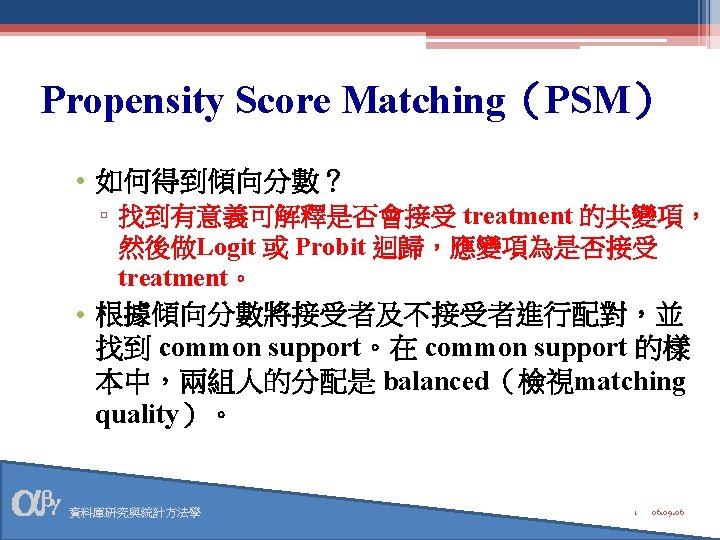
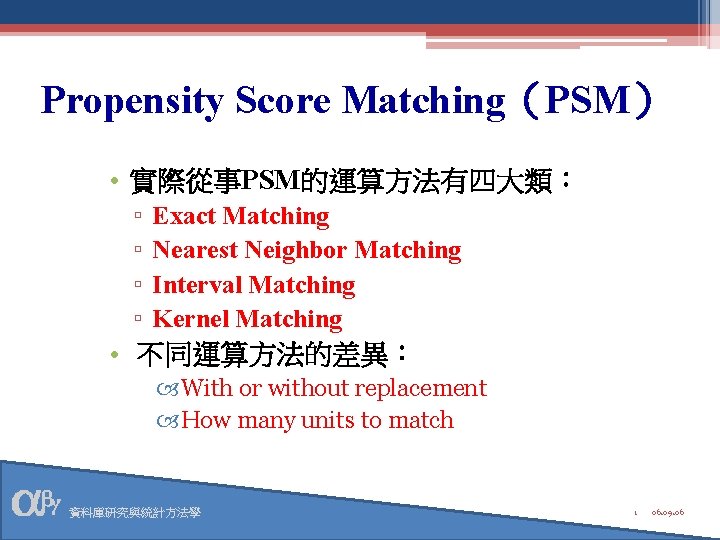
Propensity Score Matching(PSM) • 實際從事PSM的運算方法有四大類: ▫ ▫ Exact Matching Nearest Neighbor Matching Interval Matching Kernel Matching • 不同運算方法的差異: With or without replacement How many units to match 資料庫研究與統計方法學 1 06. 09. 06
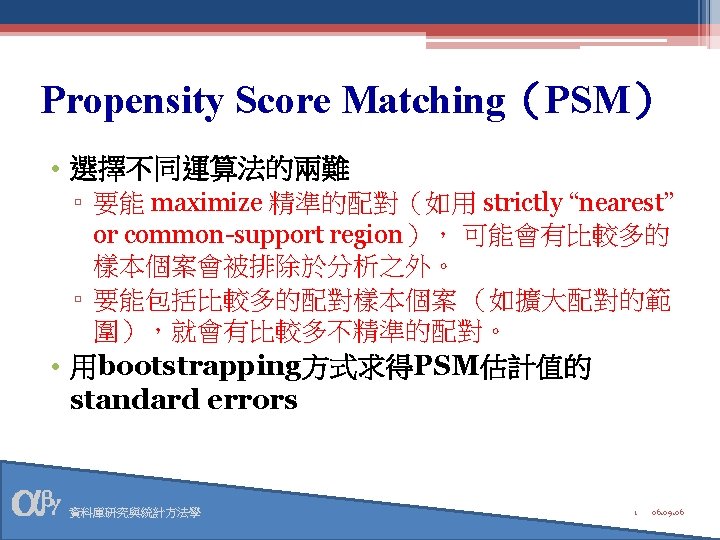
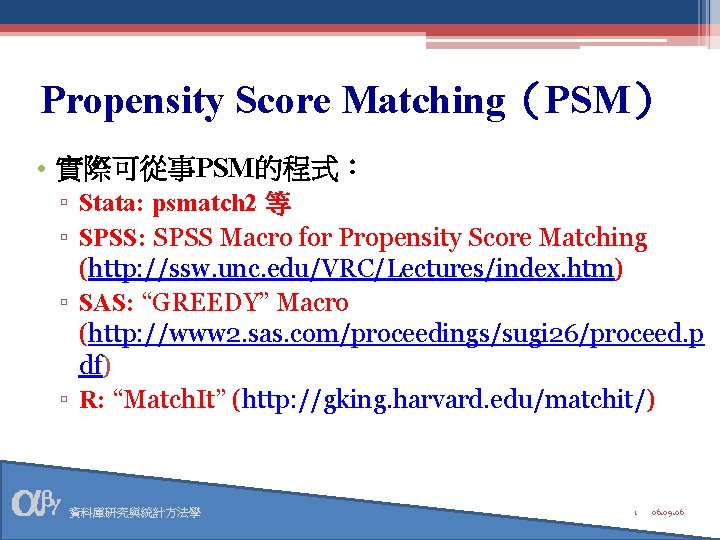
Propensity Score Matching(PSM) • 實際可從事PSM的程式: ▫ Stata: psmatch 2 等 ▫ SPSS: SPSS Macro for Propensity Score Matching (http: //ssw. unc. edu/VRC/Lectures/index. htm) ▫ SAS: “GREEDY” Macro (http: //www 2. sas. com/proceedings/sugi 26/proceed. p df) ▫ R: “Match. It” (http: //gking. harvard. edu/matchit/) 資料庫研究與統計方法學 1 06. 09. 06
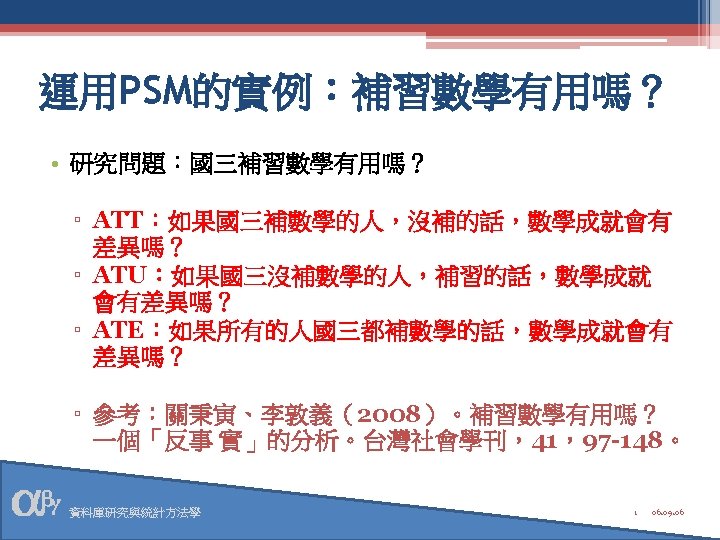
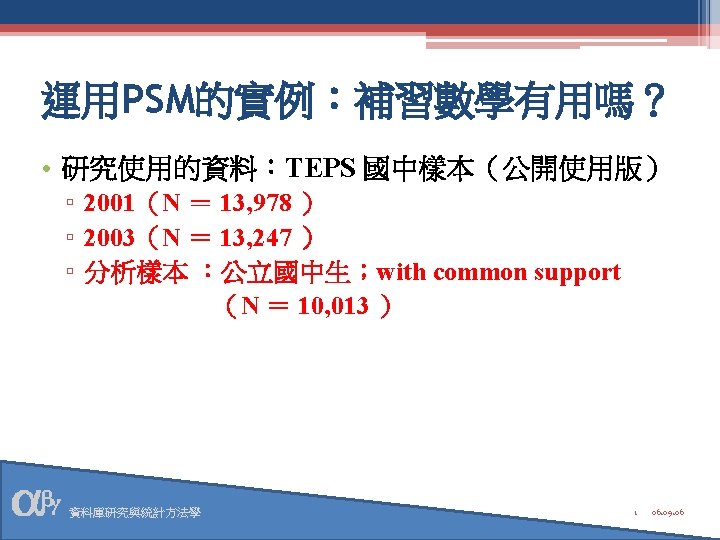
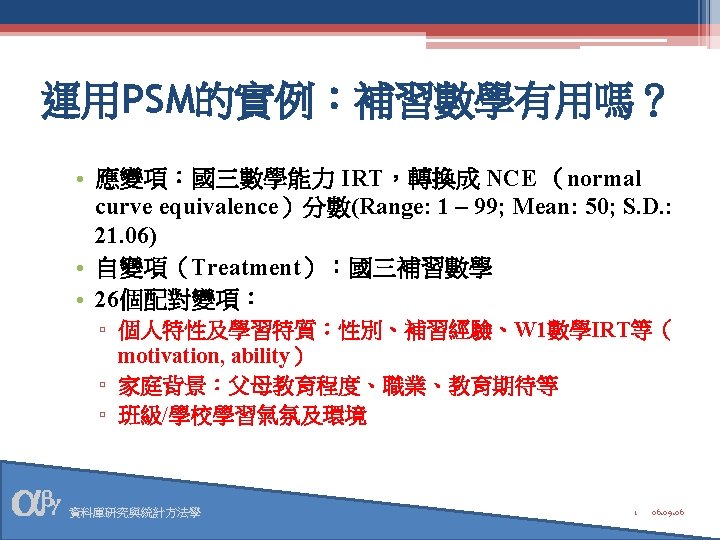
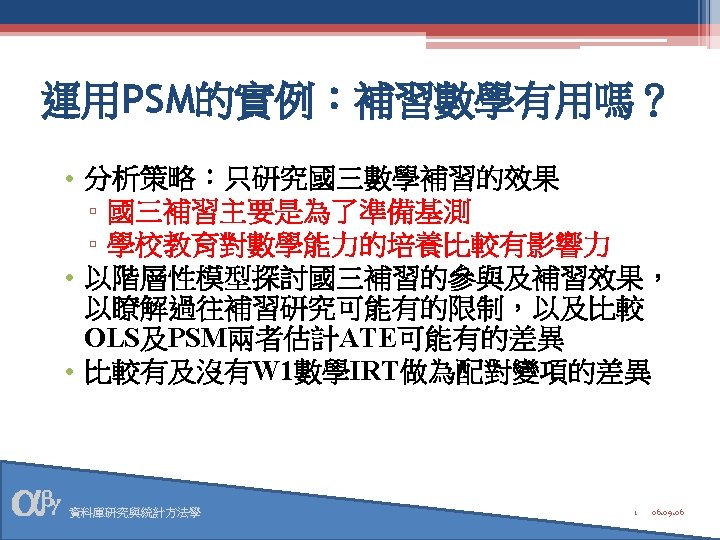
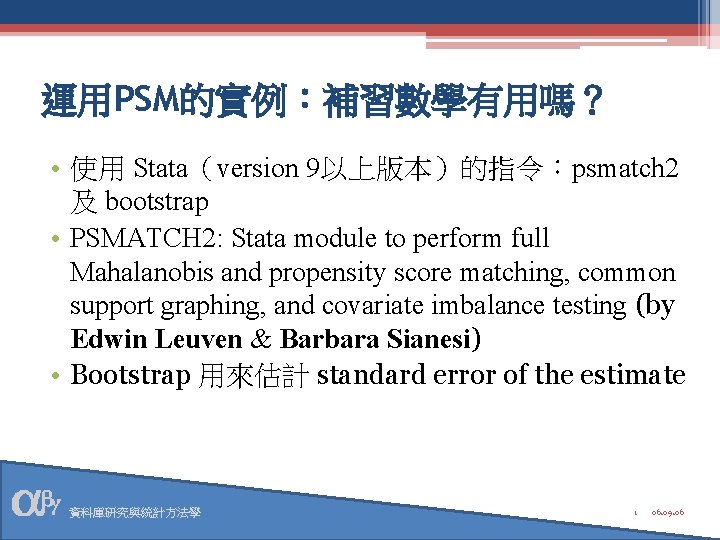
運用PSM的實例:補習數學有用嗎? • 使用 Stata(version 9以上版本)的指令:psmatch 2 及 bootstrap • PSMATCH 2: Stata module to perform full Mahalanobis and propensity score matching, common support graphing, and covariate imbalance testing (by Edwin Leuven & Barbara Sianesi) • Bootstrap 用來估計 standard error of the estimate 資料庫研究與統計方法學 1 06. 09. 06
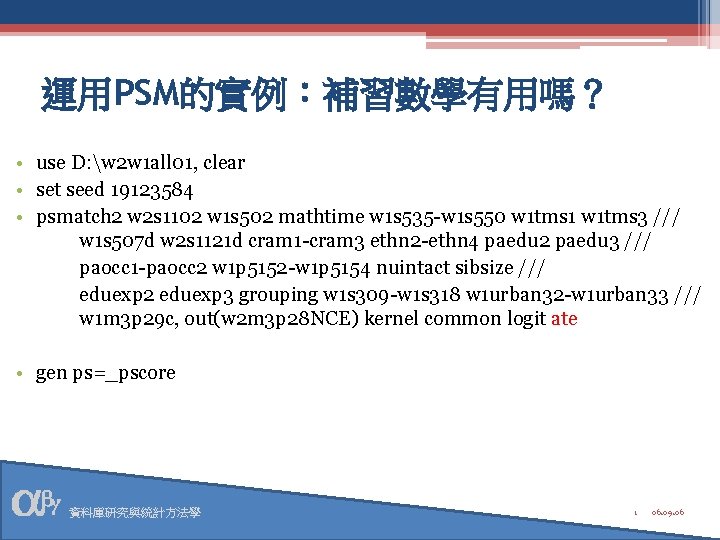
運用PSM的實例:補習數學有用嗎? • use D: w 2 w 1 all 01, clear • set seed 19123584 • psmatch 2 w 2 s 1102 w 1 s 502 mathtime w 1 s 535 -w 1 s 550 w 1 tms 1 w 1 tms 3 /// w 1 s 507 d w 2 s 1121 d cram 1 -cram 3 ethn 2 -ethn 4 paedu 2 paedu 3 /// paocc 1 -paocc 2 w 1 p 5152 -w 1 p 5154 nuintact sibsize /// eduexp 2 eduexp 3 grouping w 1 s 309 -w 1 s 318 w 1 urban 32 -w 1 urban 33 /// w 1 m 3 p 29 c, out(w 2 m 3 p 28 NCE) kernel common logit ate • gen ps=_pscore 資料庫研究與統計方法學 1 06. 09. 06
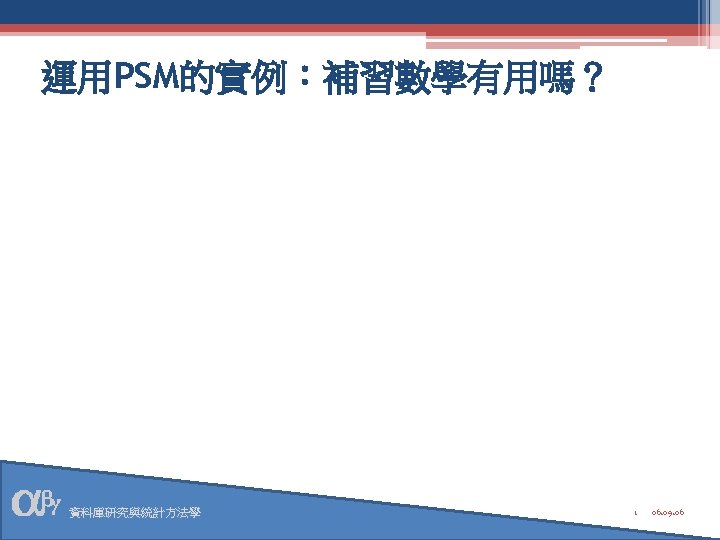
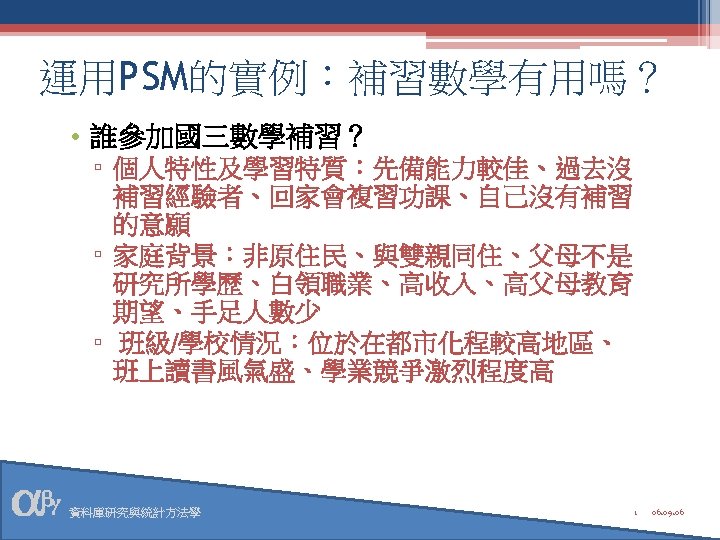
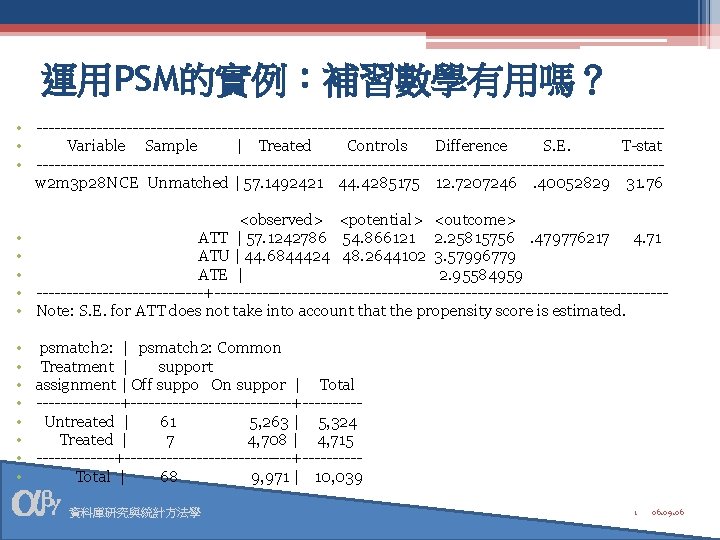
運用PSM的實例:補習數學有用嗎? • ---------------------------------------------------- • Variable Sample | Treated Controls Difference S. E. T-stat • ----------------------------------------------------w 2 m 3 p 28 NCE Unmatched | 57. 1492421 44. 4285175 12. 7207246. 40052829 31. 76 • • • <observed> <potential> <outcome> ATT | 57. 1242786 54. 866121 2. 25815756. 479776217 4. 71 ATU | 44. 6844424 48. 2644102 3. 57996779 ATE | 2. 95584959 --------------+--------------------------------------Note: S. E. for ATT does not take into account that the propensity score is estimated. • psmatch 2: | psmatch 2: Common • Treatment | support • assignment | Off suppo On suppor | Total • -------+--------------+----- • Untreated | 61 5, 263 | 5, 324 • Treated | 7 4, 708 | 4, 715 • -------+--------------+----- • Total | 68 9, 971 | 10, 039 資料庫研究與統計方法學 1 06. 09. 06
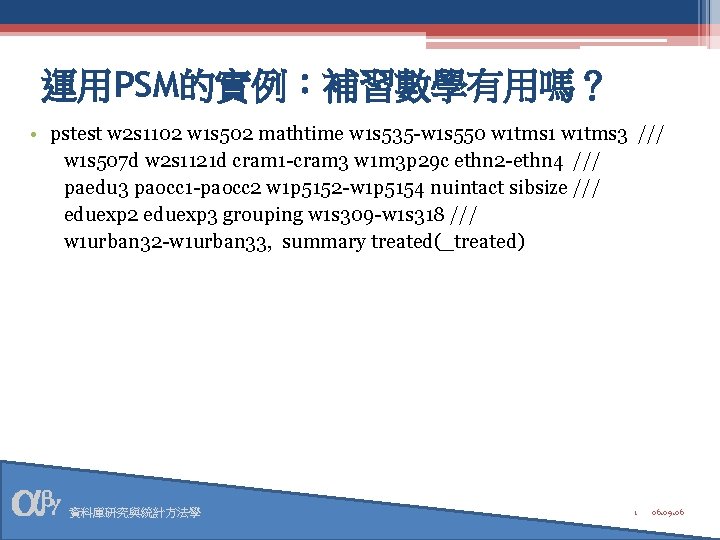
運用PSM的實例:補習數學有用嗎? • pstest w 2 s 1102 w 1 s 502 mathtime w 1 s 535 -w 1 s 550 w 1 tms 1 w 1 tms 3 /// w 1 s 507 d w 2 s 1121 d cram 1 -cram 3 w 1 m 3 p 29 c ethn 2 -ethn 4 /// paedu 3 paocc 1 -paocc 2 w 1 p 5152 -w 1 p 5154 nuintact sibsize /// eduexp 2 eduexp 3 grouping w 1 s 309 -w 1 s 318 /// w 1 urban 32 -w 1 urban 33, summary treated(_treated) 資料庫研究與統計方法學 1 06. 09. 06
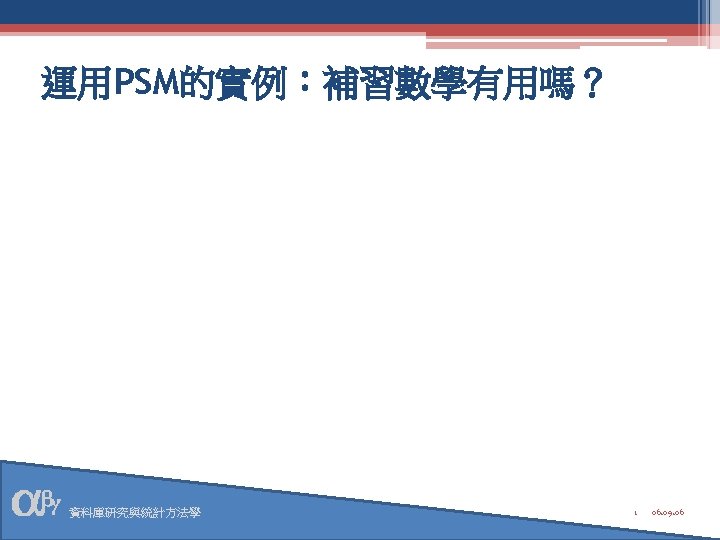
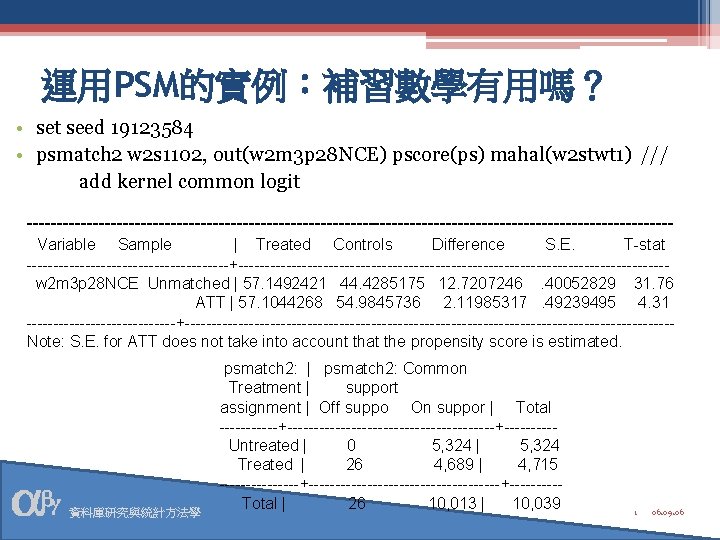
運用PSM的實例:補習數學有用嗎? • set seed 19123584 • psmatch 2 w 2 s 1102, out(w 2 m 3 p 28 NCE) pscore(ps) mahal(w 2 stwt 1) /// add kernel common logit ------------------------------------------------------Variable Sample | Treated Controls Difference S. E. T-stat -------------------+----------------------------------------w 2 m 3 p 28 NCE Unmatched | 57. 1492421 44. 4285175 12. 7207246. 40052829 31. 76 ATT | 57. 1044268 54. 9845736 2. 11985317. 49239495 4. 31 --------------+----------------------------------------------Note: S. E. for ATT does not take into account that the propensity score is estimated. 資料庫研究與統計方法學 psmatch 2: | psmatch 2: Common Treatment | support assignment | Off suppo On suppor | Total ------+--------------------+-----Untreated | 0 5, 324 | 5, 324 Treated | 26 4, 689 | 4, 715 --------+------------------+-----Total | 26 10, 013 | 10, 039 1 06. 09. 06
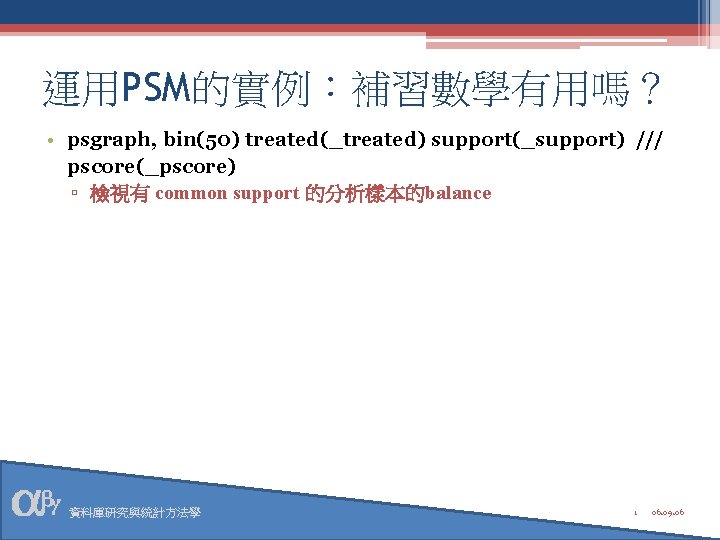
運用PSM的實例:補習數學有用嗎? • psgraph, bin(50) treated(_treated) support(_support) /// pscore(_pscore) ▫ 檢視有 common support 的分析樣本的balance 資料庫研究與統計方法學 1 06. 09. 06
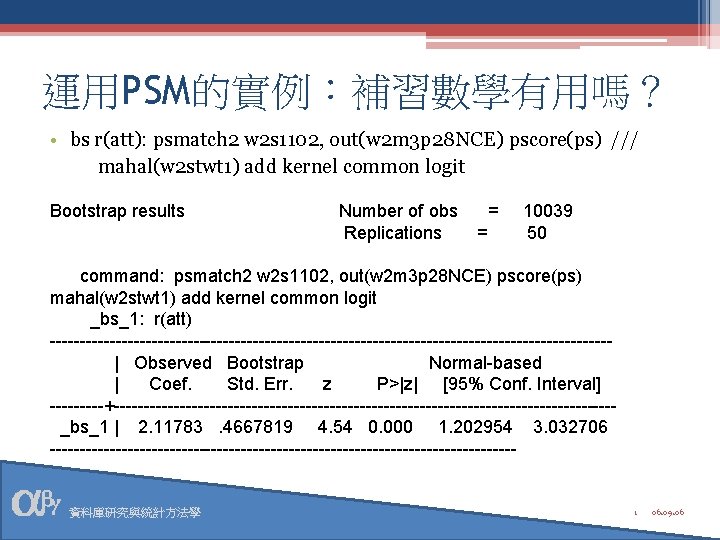
運用PSM的實例:補習數學有用嗎? • bs r(att): psmatch 2 w 2 s 1102, out(w 2 m 3 p 28 NCE) pscore(ps) /// mahal(w 2 stwt 1) add kernel common logit Bootstrap results Number of obs Replications = = 10039 50 command: psmatch 2 w 2 s 1102, out(w 2 m 3 p 28 NCE) pscore(ps) mahal(w 2 stwt 1) add kernel common logit _bs_1: r(att) -----------------------------------------------| Observed Bootstrap Normal-based | Coef. Std. Err. z P>|z| [95% Conf. Interval] -----+------------------------------------------_bs_1 | 2. 11783. 4667819 4. 54 0. 000 1. 202954 3. 032706 --------------------------------------- 資料庫研究與統計方法學 1 06. 09. 06
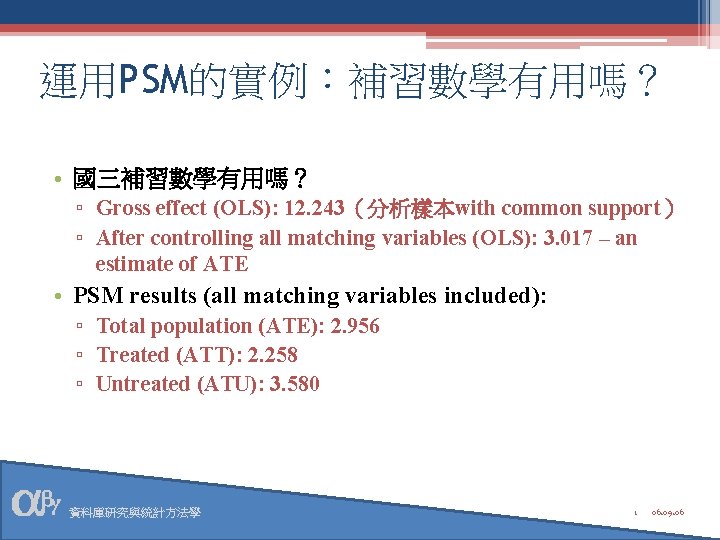
運用PSM的實例:補習數學有用嗎? • 國三補習數學有用嗎? ▫ Gross effect (OLS): 12. 243(分析樣本with common support) ▫ After controlling all matching variables (OLS): 3. 017 – an estimate of ATE • PSM results (all matching variables included): ▫ Total population (ATE): 2. 956 ▫ Treated (ATT): 2. 258 ▫ Untreated (ATU): 3. 580 資料庫研究與統計方法學 1 06. 09. 06
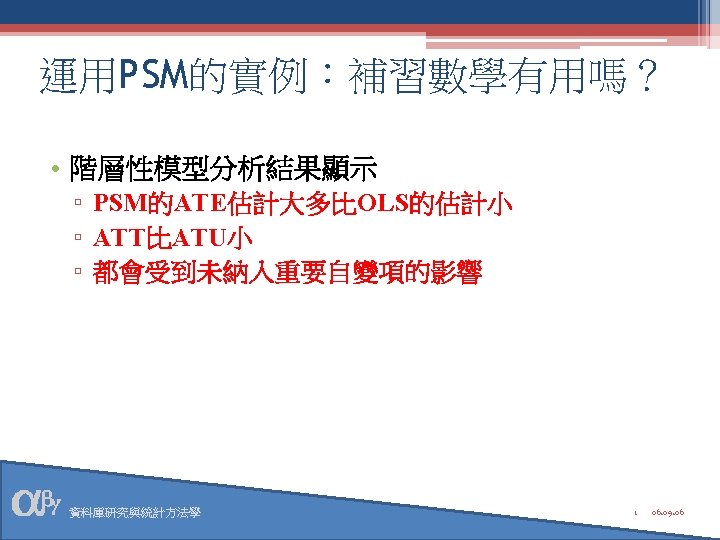
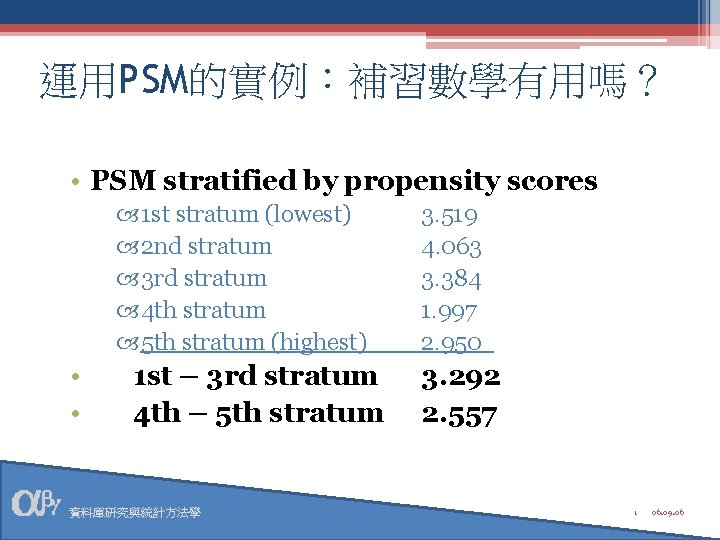
運用PSM的實例:補習數學有用嗎? • PSM stratified by propensity scores 1 st stratum (lowest) 2 nd stratum 3 rd stratum 4 th stratum 5 th stratum (highest) • • 1 st – 3 rd stratum 4 th – 5 th stratum 資料庫研究與統計方法學 3. 519 4. 063 3. 384 1. 997 2. 950 3. 292 2. 557 1 06. 09. 06
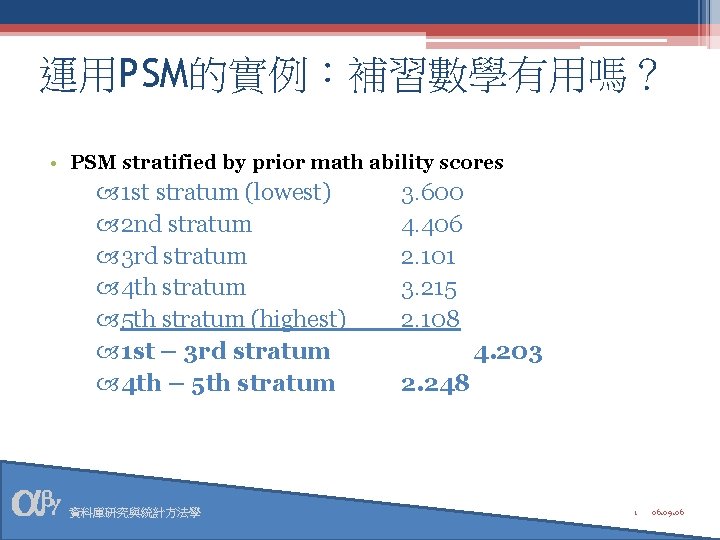
運用PSM的實例:補習數學有用嗎? • PSM stratified by prior math ability scores 1 st stratum (lowest) 2 nd stratum 3 rd stratum 4 th stratum 5 th stratum (highest) 1 st – 3 rd stratum 4 th – 5 th stratum 資料庫研究與統計方法學 3. 600 4. 406 2. 101 3. 215 2. 108 4. 203 2. 248 1 06. 09. 06
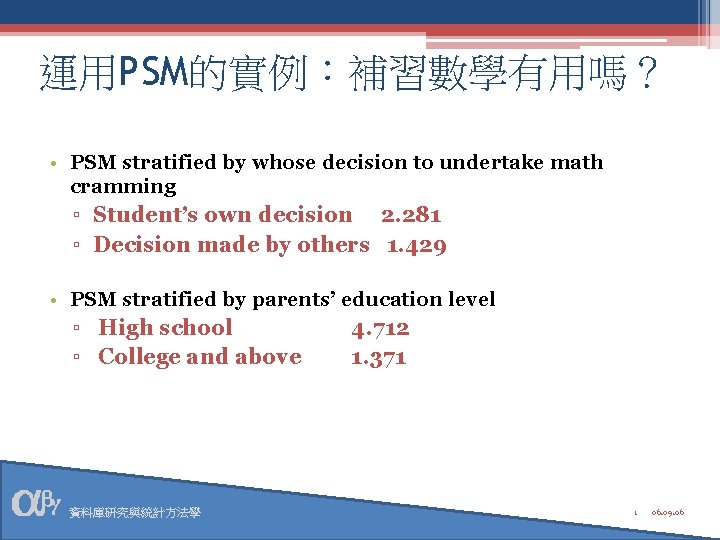
運用PSM的實例:補習數學有用嗎? • PSM stratified by whose decision to undertake math cramming ▫ Student’s own decision 2. 281 ▫ Decision made by others 1. 429 • PSM stratified by parents’ education level ▫ High school ▫ College and above 資料庫研究與統計方法學 4. 712 1. 371 1 06. 09. 06
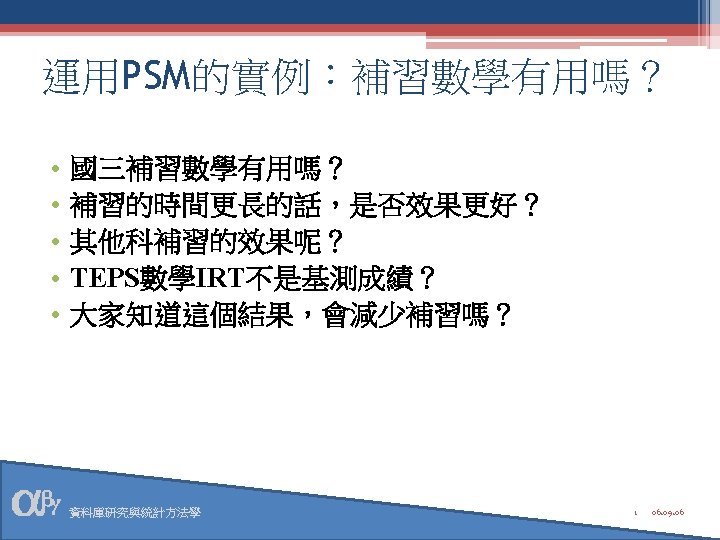
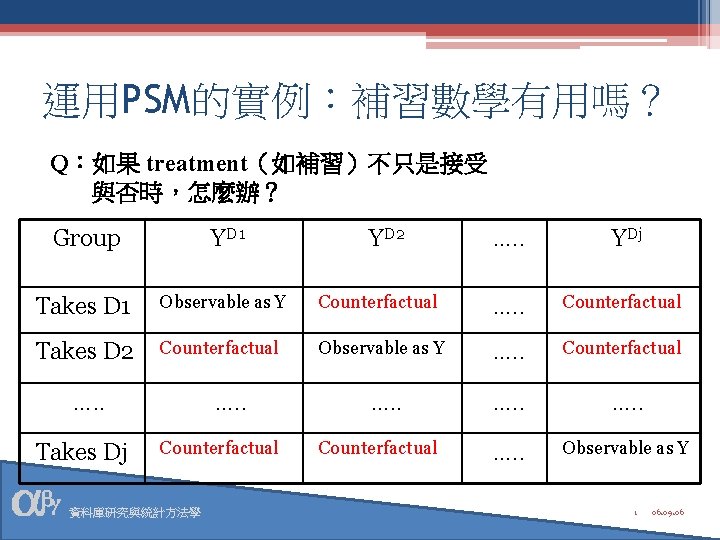
運用PSM的實例:補習數學有用嗎? Q:如果 treatment(如補習)不只是接受 與否時,怎麼辦? Group YD 1 Takes D 1 Observable as Y Counterfactual …. . Counterfactual Takes D 2 Counterfactual Observable as Y …. . Counterfactual …. . Observable as Y …. . Takes Dj …. . Counterfactual 資料庫研究與統計方法學 YD 2 Counterfactual …. . YDj 1 06. 09. 06
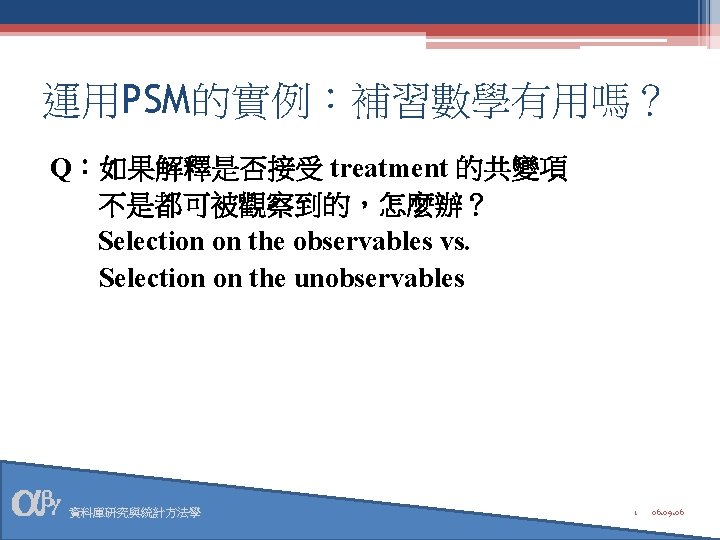
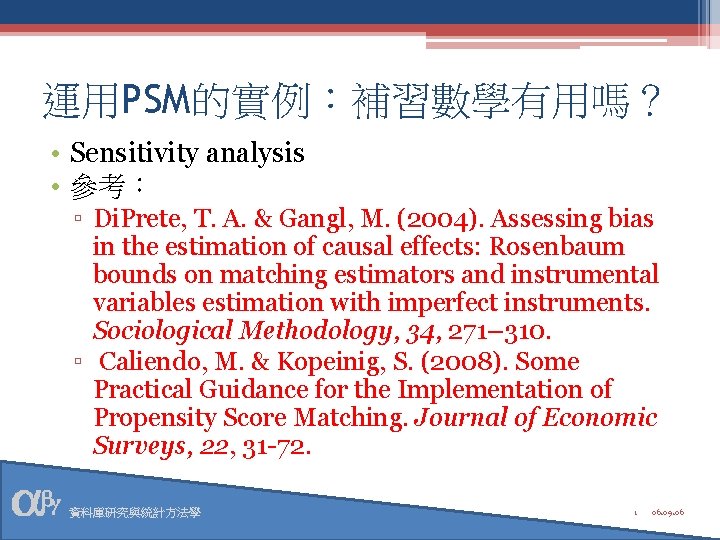
運用PSM的實例:補習數學有用嗎? • Sensitivity analysis • 參考: ▫ Di. Prete, T. A. & Gangl, M. (2004). Assessing bias in the estimation of causal effects: Rosenbaum bounds on matching estimators and instrumental variables estimation with imperfect instruments. Sociological Methodology, 34, 271– 310. ▫ Caliendo, M. & Kopeinig, S. (2008). Some Practical Guidance for the Implementation of Propensity Score Matching. Journal of Economic Surveys, 22, 31 -72. 資料庫研究與統計方法學 1 06. 09. 06
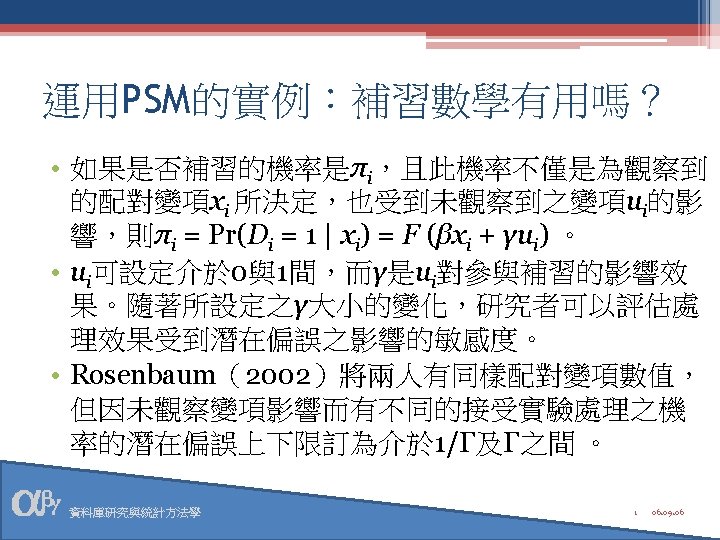
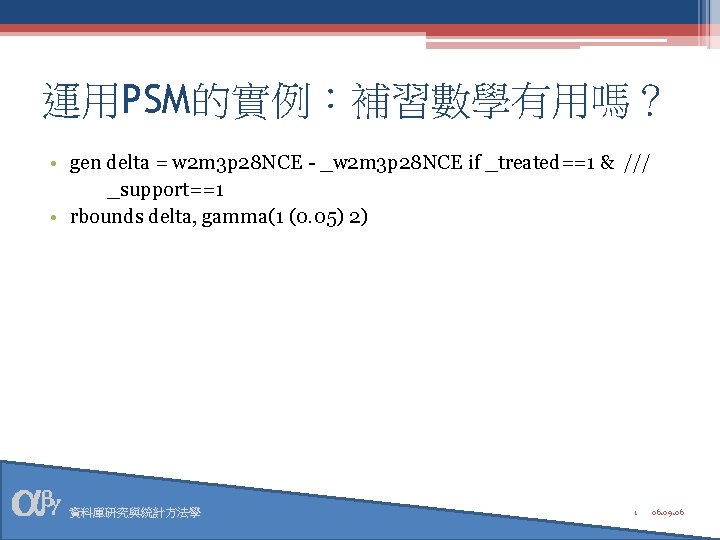
運用PSM的實例:補習數學有用嗎? • gen delta = w 2 m 3 p 28 NCE - _w 2 m 3 p 28 NCE if _treated==1 & /// _support==1 • rbounds delta, gamma(1 (0. 05) 2) 資料庫研究與統計方法學 1 06. 09. 06
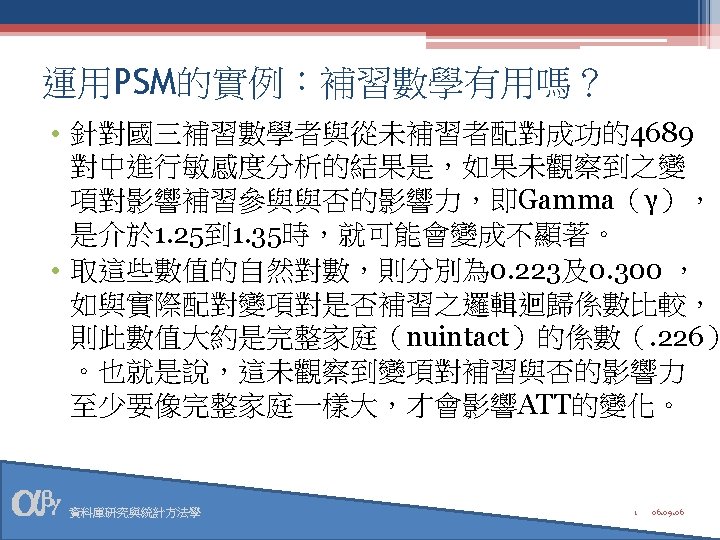
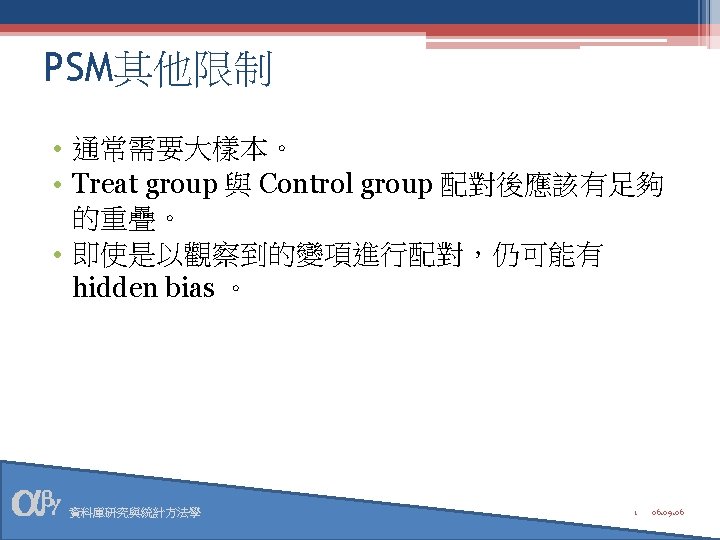
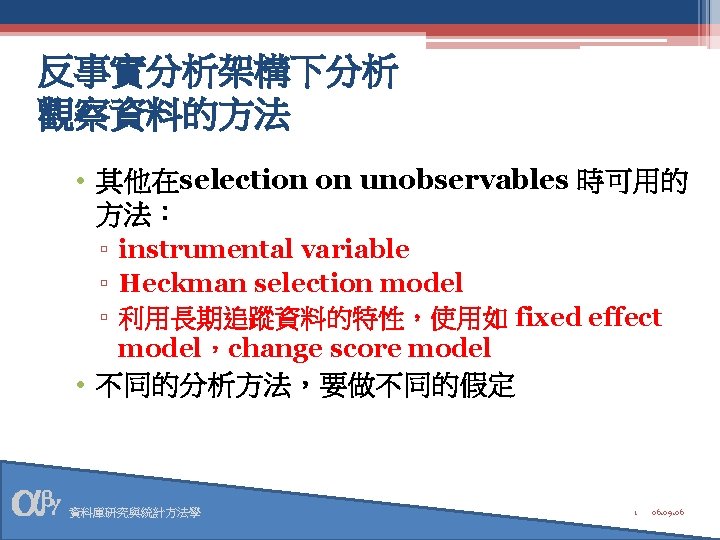
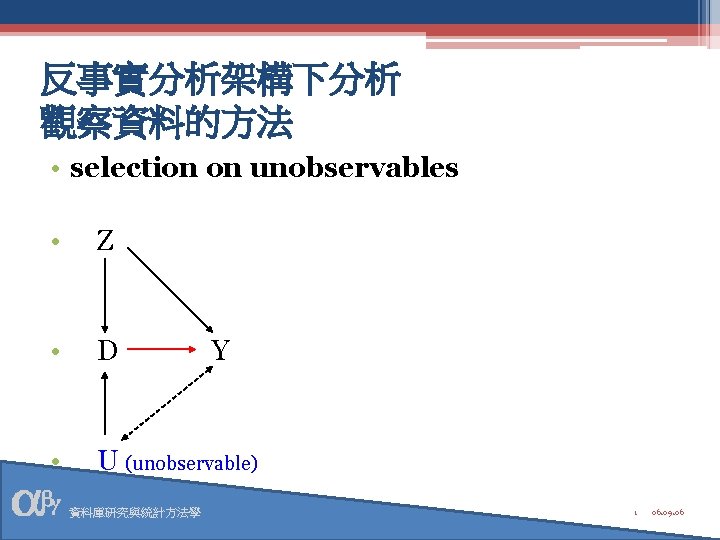
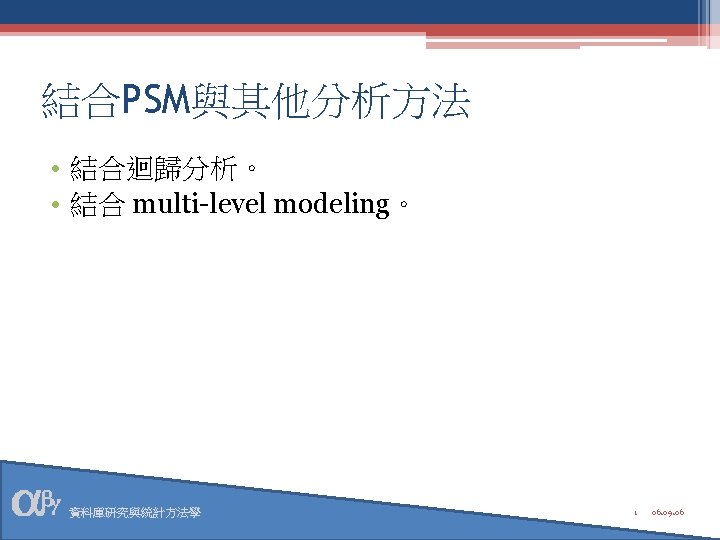
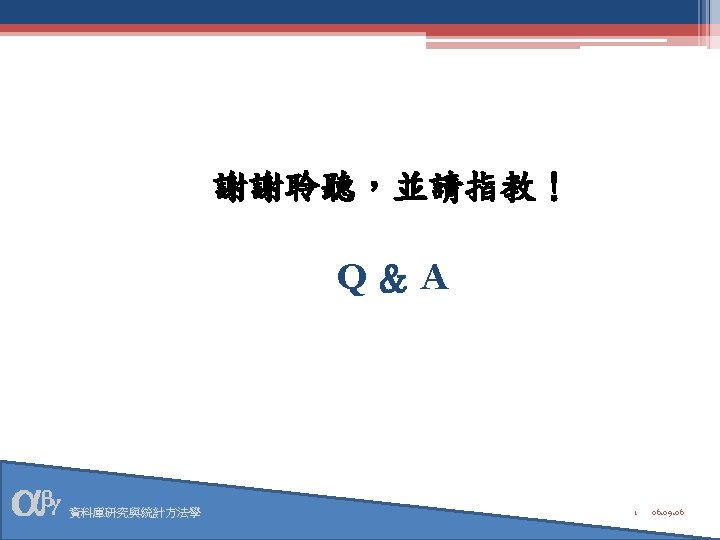
Jerusalem judea samaria and the ends of the earth
Judea to samaria
Donde queda belen de judea
Jerusalem judea samaria
Lukas 10:1-11
Judea pearl causality
My favourite subject maths for class 4
Introduction to statistics what is statistics
Statistics is the science of conducting studies to:
Statistics is science or art
Statistics for social science
Natural science and social science similarities
Science fusion introduction to science and technology
Hard and soft science
Computer science input and output
Difference between ba and bs in computer science
Ucf college of engineering and computer science
Erik jonsson school of engineering and computer science
Computer science and engineering unr
Eecs ucla
Pltw
Erik jonsson school of engineering and computer science
Utd erik jonsson school of engineering
General objectives of computer
What is computer organization
The large program that controls how the cpu communicates
Computer organization and architecture difference
Natural vs social science
What are the branches of natural science
Natural science vs physical science
Applied science vs pure science
Why environmental science is an interdisciplinary science
Julie lundquist
Wjec gcse computer science
Phoenix online computer science university
How many fields in computer science
Example of procedural abstraction
Computer science unsolved problems
University of bridgeport engineering
Computer science tutor bridgeport
Sequencing ap csp
Brad karp ucl
Ucl computer science interview
Casting computer science
Predicate computer science
Computer science illuminated (doc or html) file
Konsep dasar logika himpunan
Yonsei computer science
Sat in computer science
Ib computer science topic 6
Data representation computer science
Recursion apcsa
Recurrence relation computer science
Push down
Computer science component 1
Ocr gcse computer science algorithm questions
Electrical engineering northwestern
Parse computer science
Undecidable problems in computer science
Otterbein computer science
What is iteration in computer science
Computer science vu
Computer science polymorphism
Heuristic
Best fs algorithm
Theoretical computer science
Mch fsu
Computer science experiments
A level computer science exemplar candidate work
Elevens lab ap computer science
Wpi ece
Software engineering vs computer science
Edexcel igcse computer science
Computer science department rutgers
Cs 3304
The definition of computer science
What is ib computer science
Parameter computer science
File handling computer science
Cryptography computer science
Chomsky computer science
Science haiku
Domains of computer science
Computer science flowchart symbols
Iteration definition computer science
K state computer science
Ib computer science topic 1 questions
How many fields in computer science
Abstraction gcse computer science
Basic concepts of computer science
Algorithm definition computer science
Hexadecimal to binary
Ai is a branch of computer science
Array computer science
Undecidable problems in computer science
Computer science growth rate
Computer science flowchart symbols
Morgans computers
Efi arazi school of computer science
Abstraction computer science
Presentation greeting example
Lightboard frq
Great theoretical ideas in computer science
York university computer science