Correlation Regression The Data http core ecu edupsycwuenschkSPSS
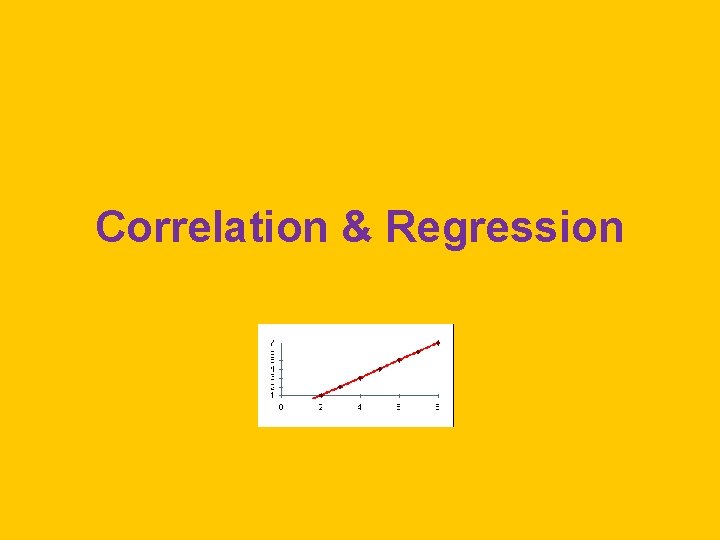
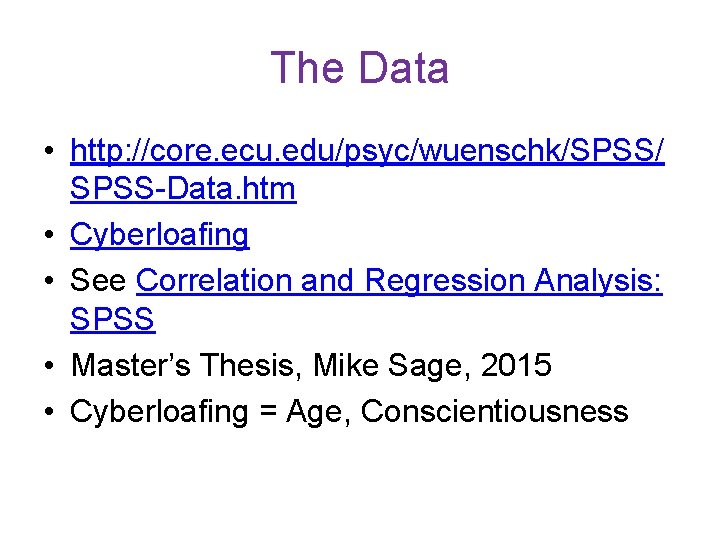
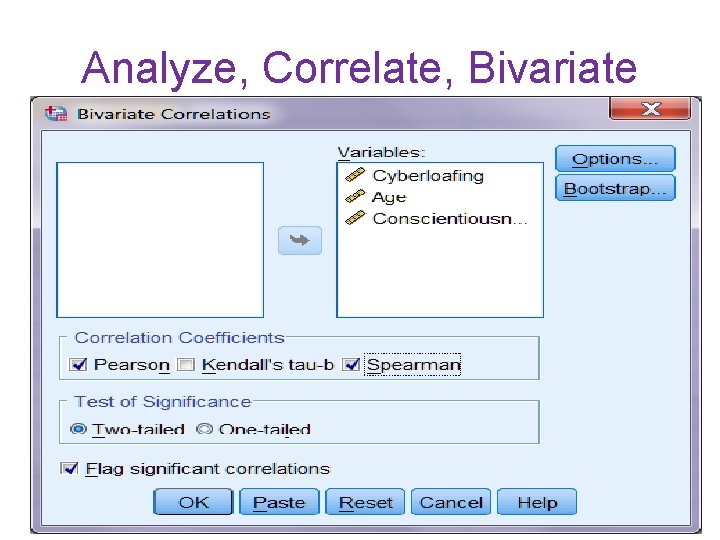
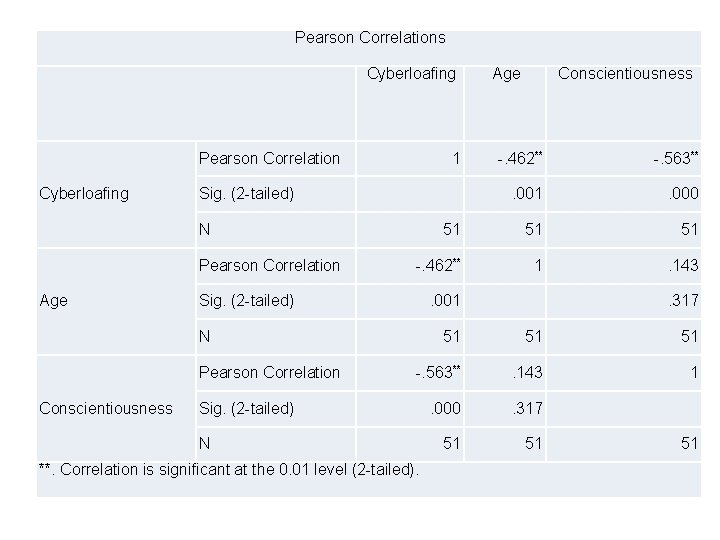
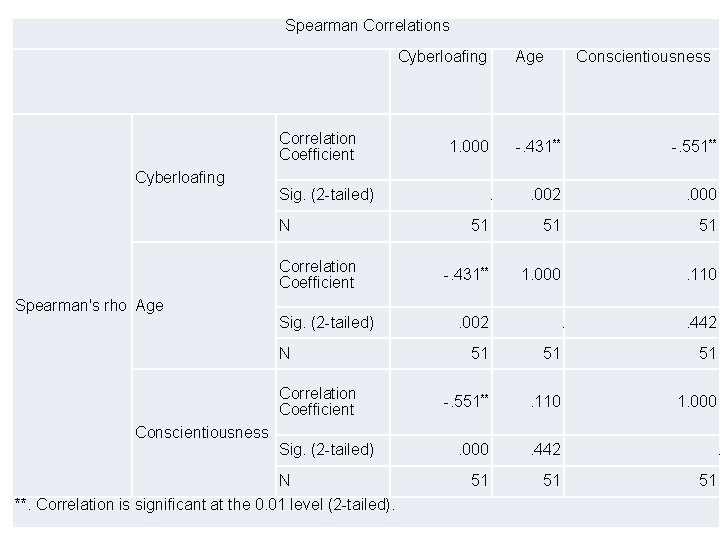
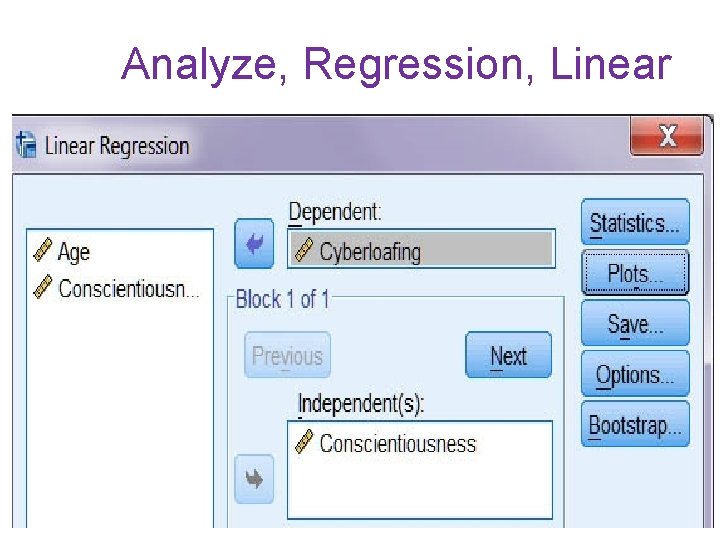
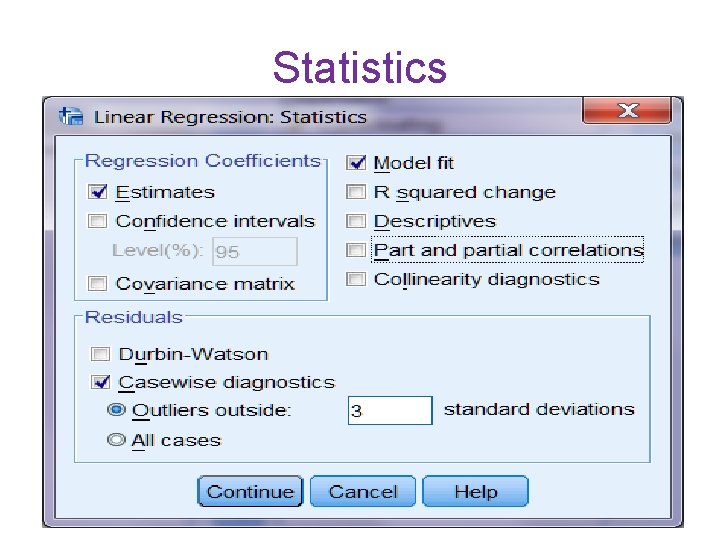
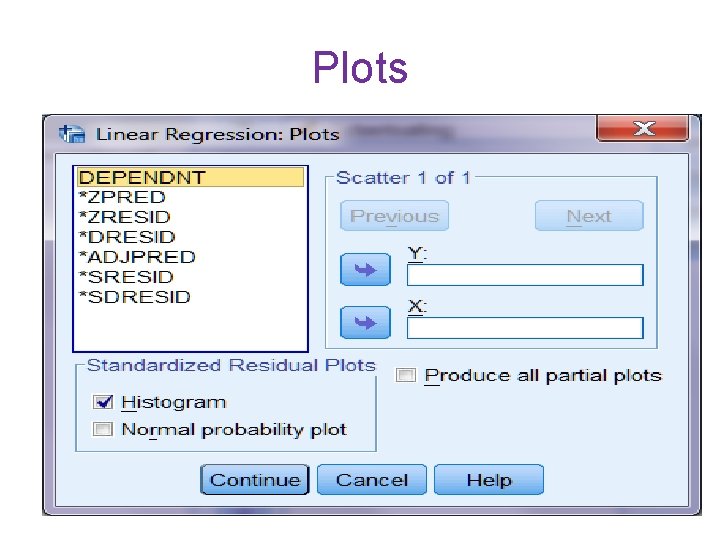
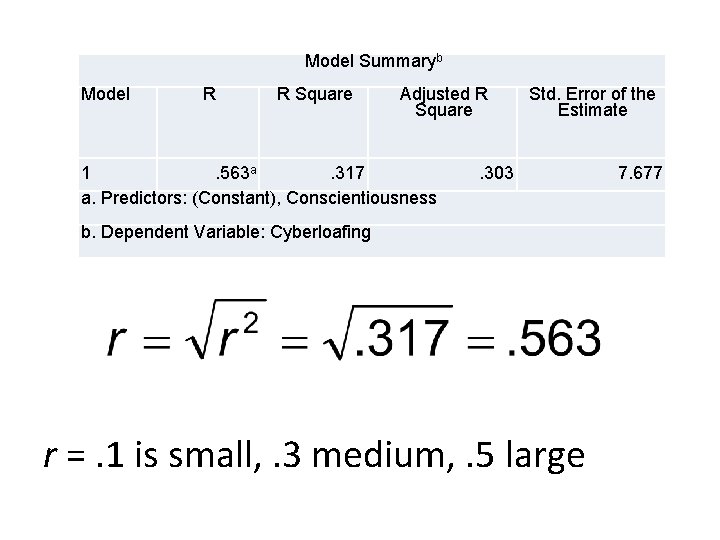
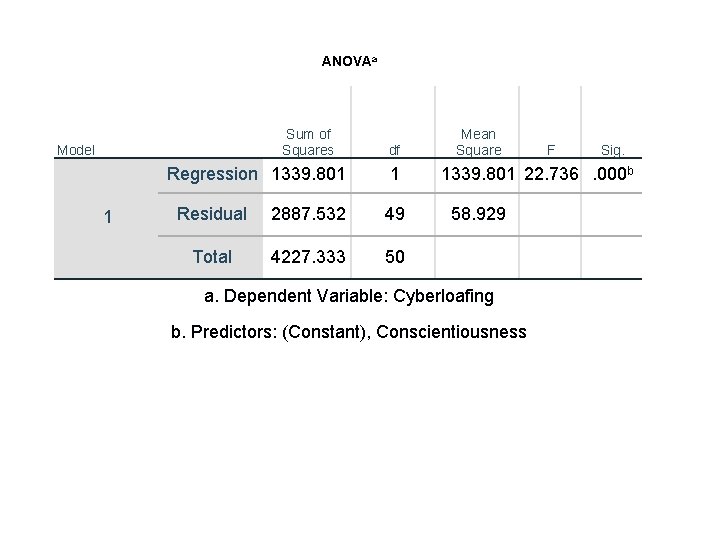
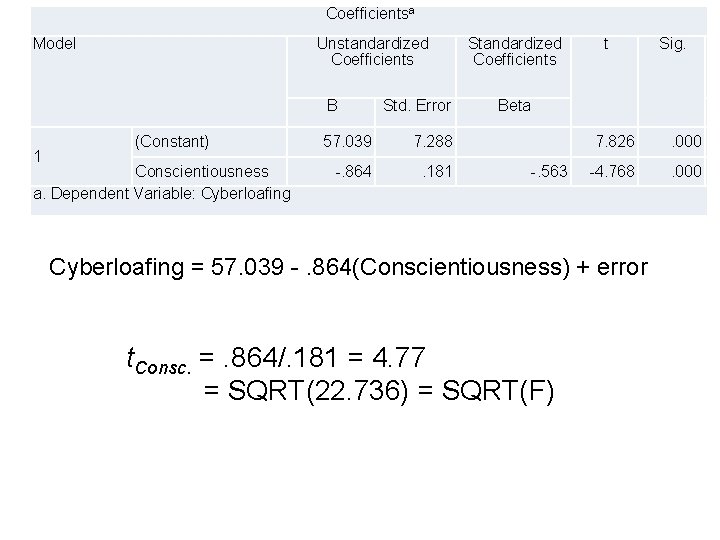
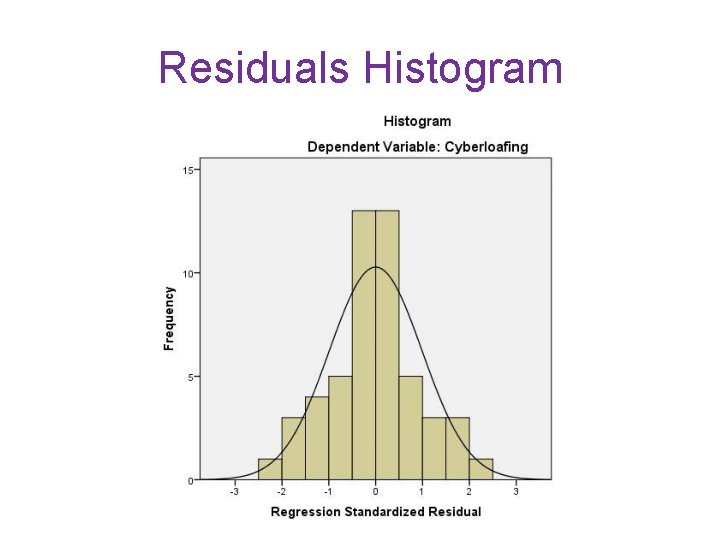
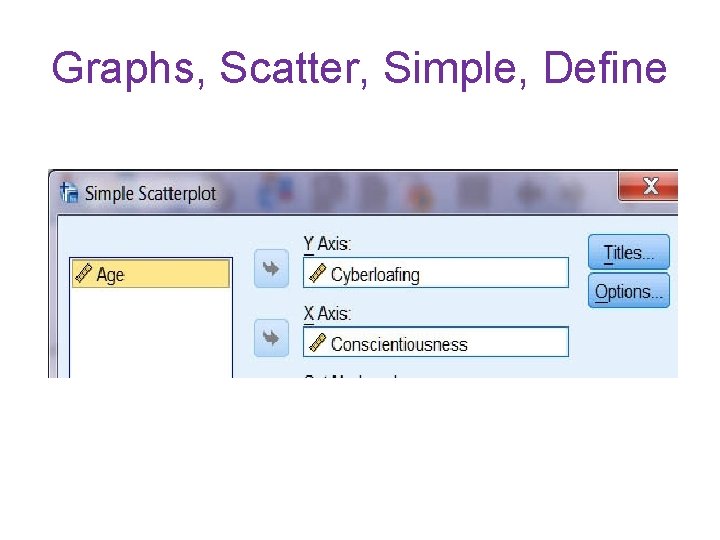
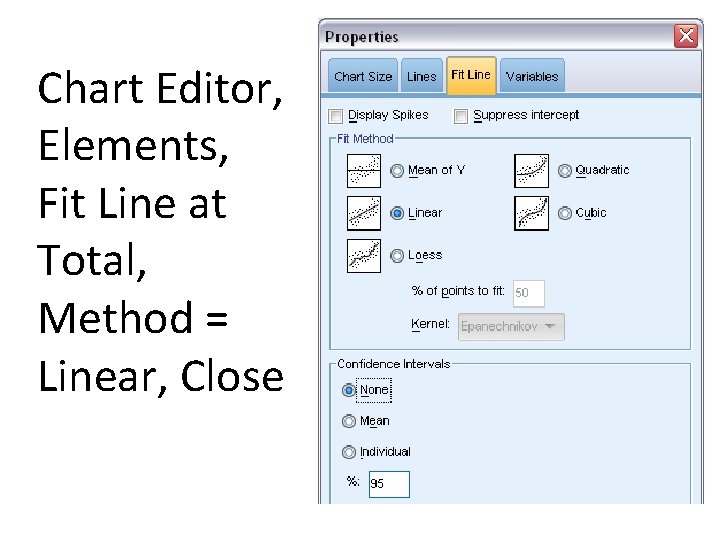
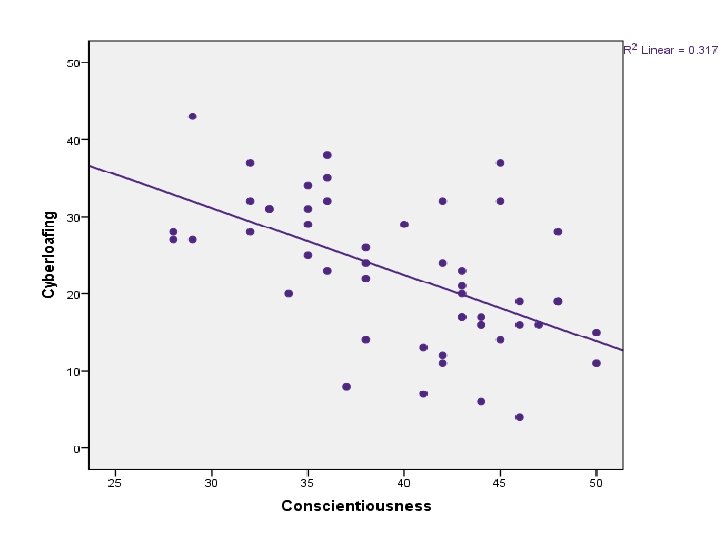
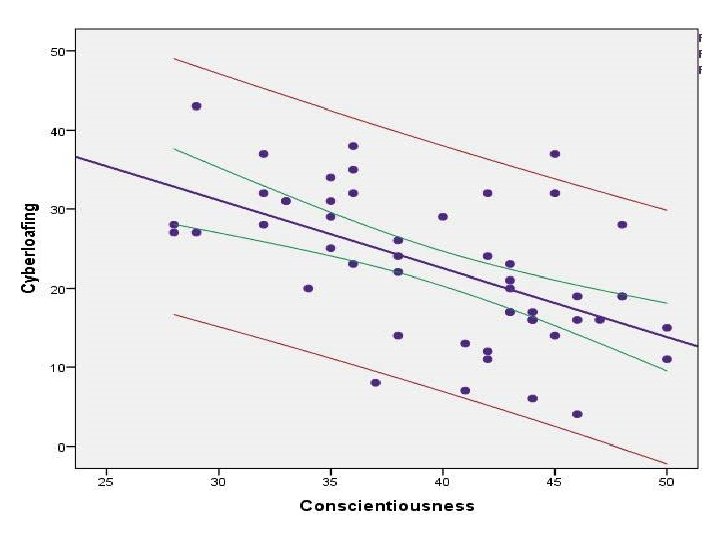
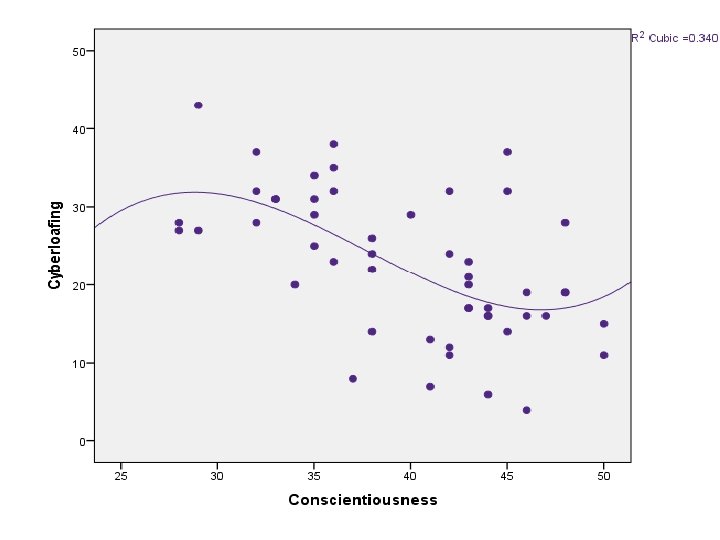
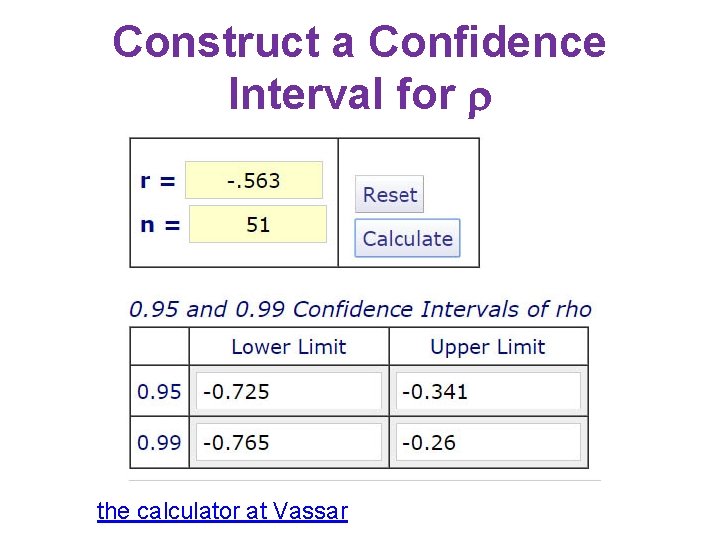
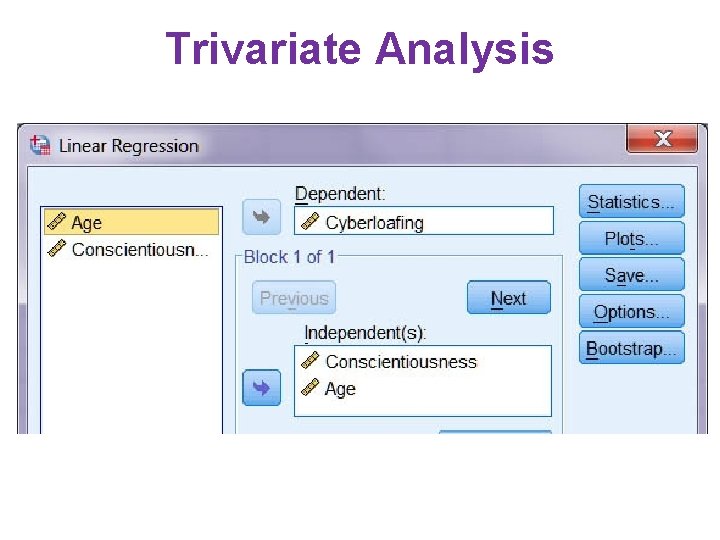
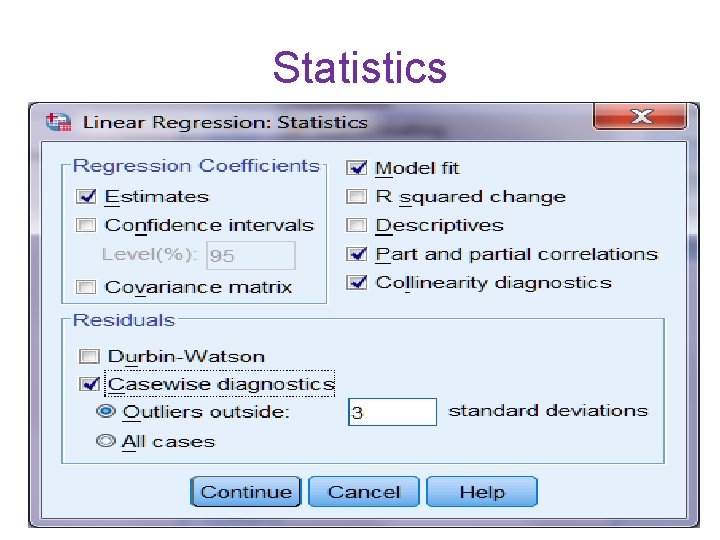
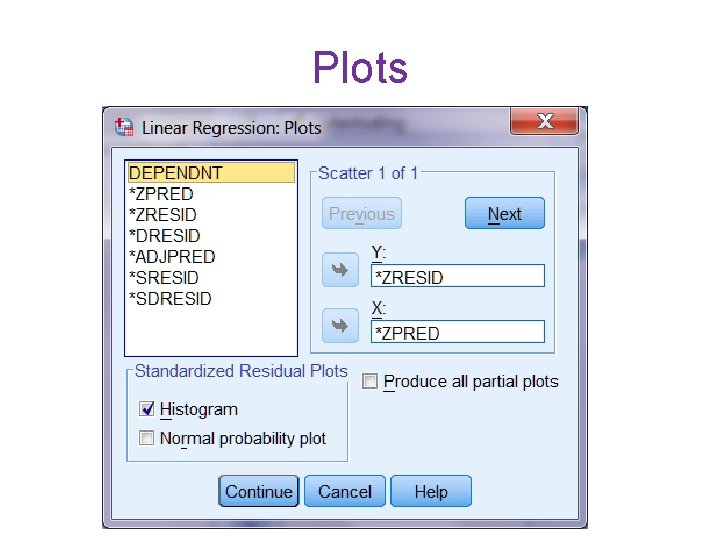
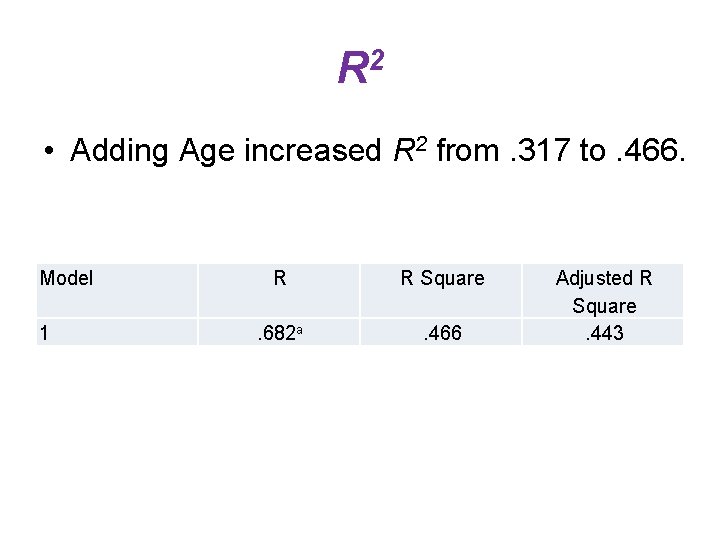
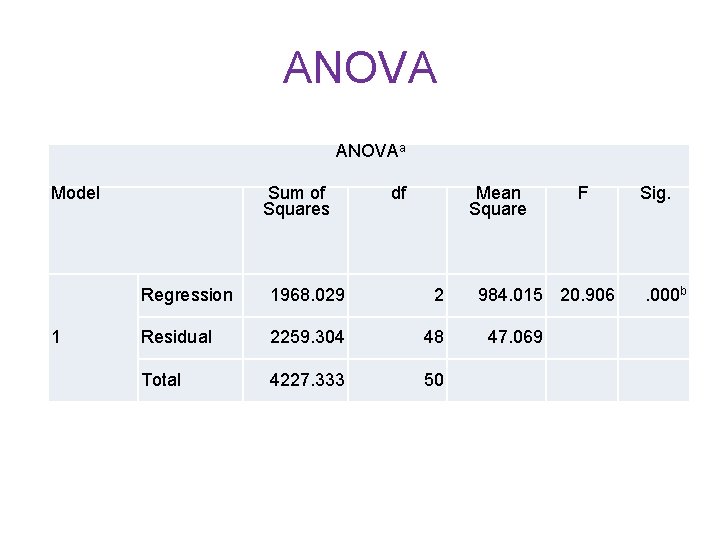
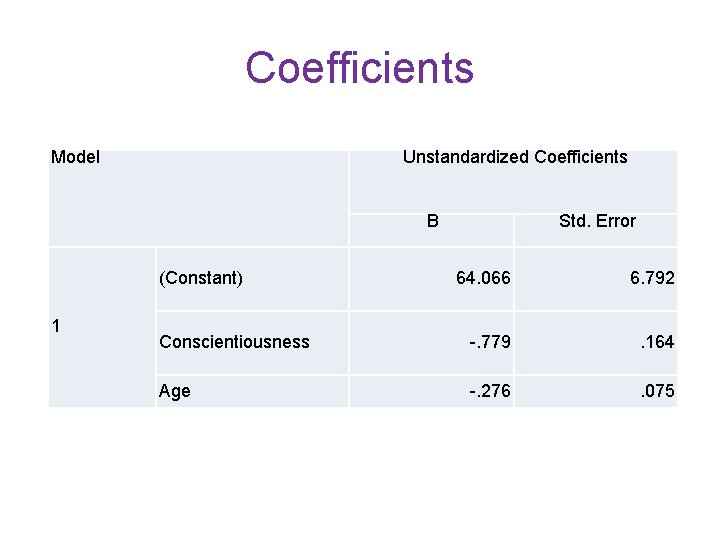
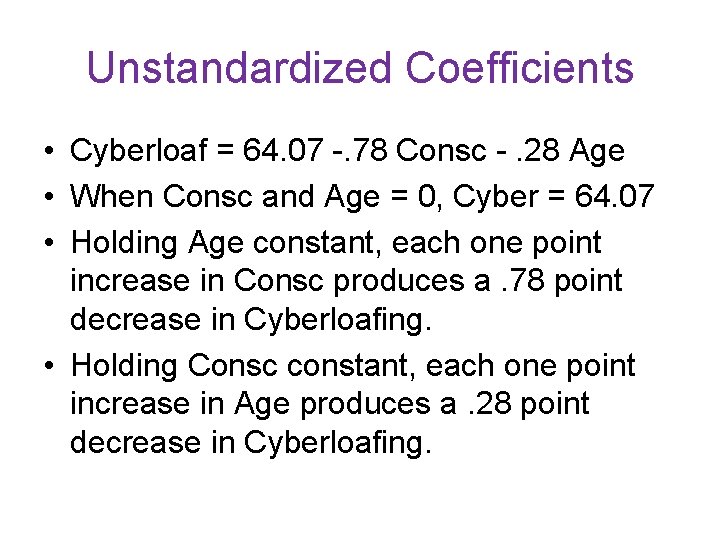
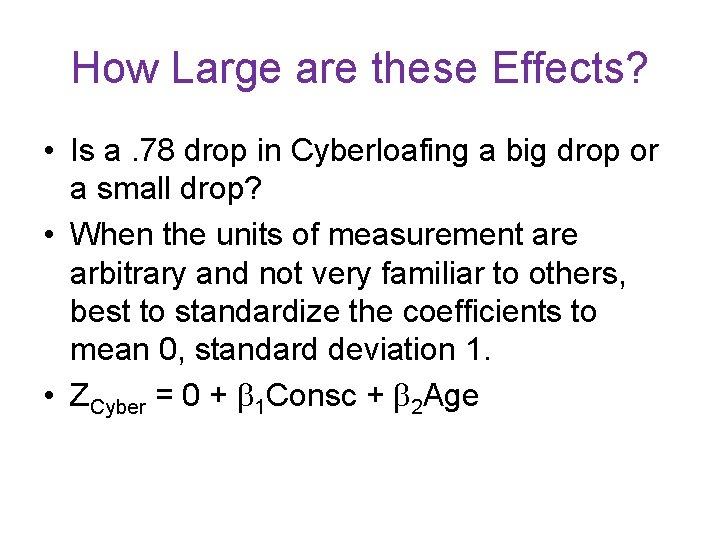
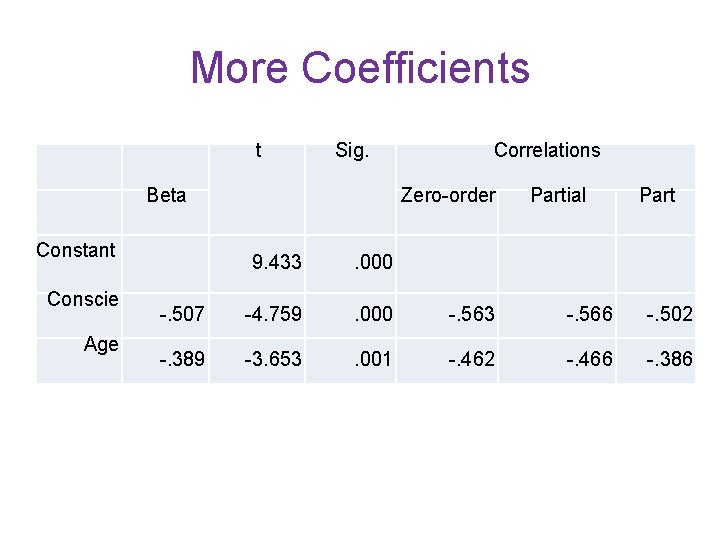
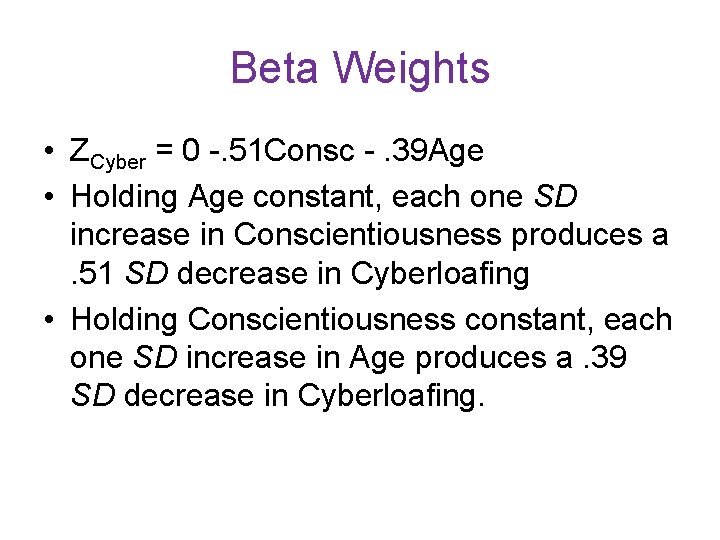
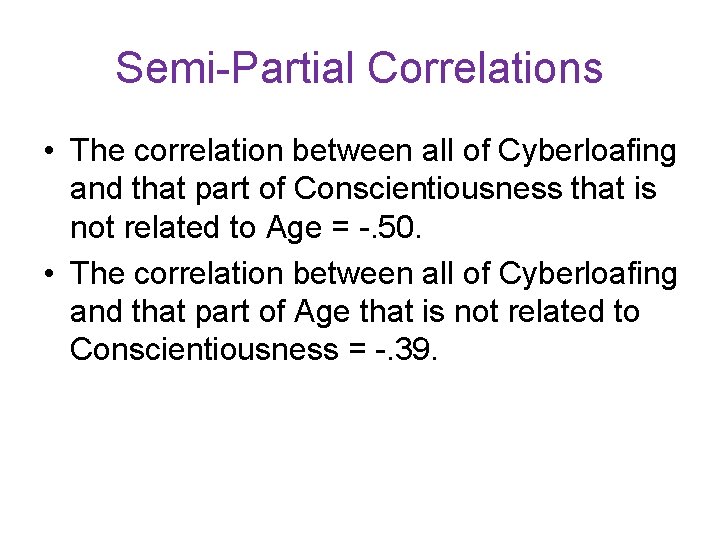
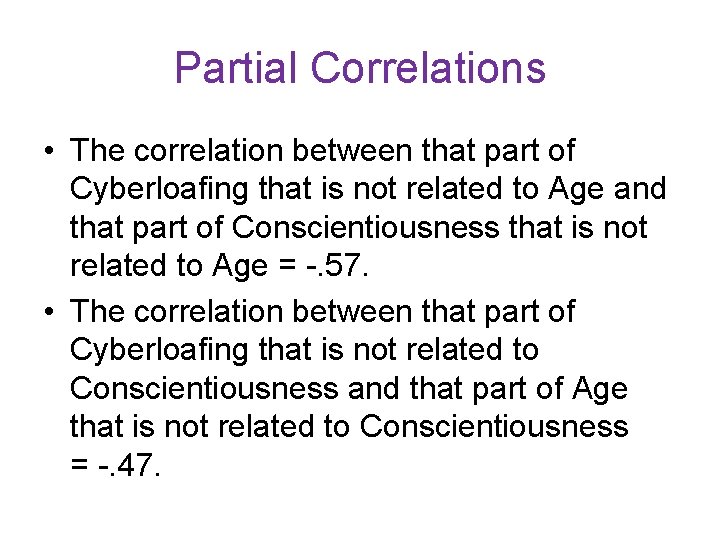
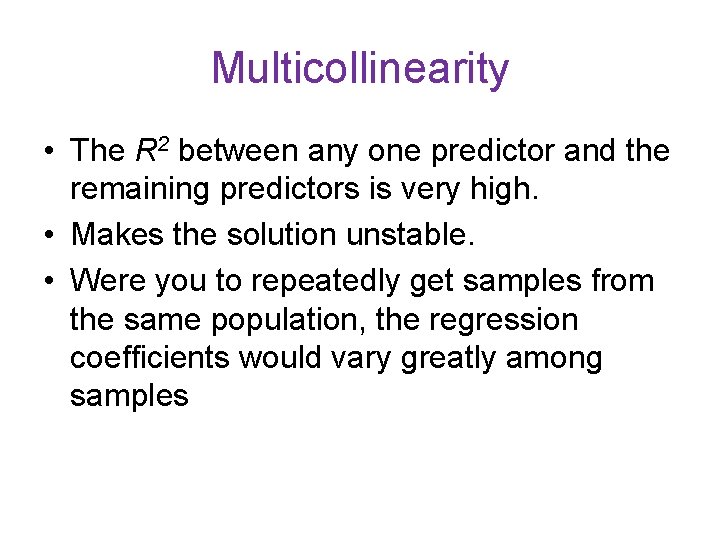
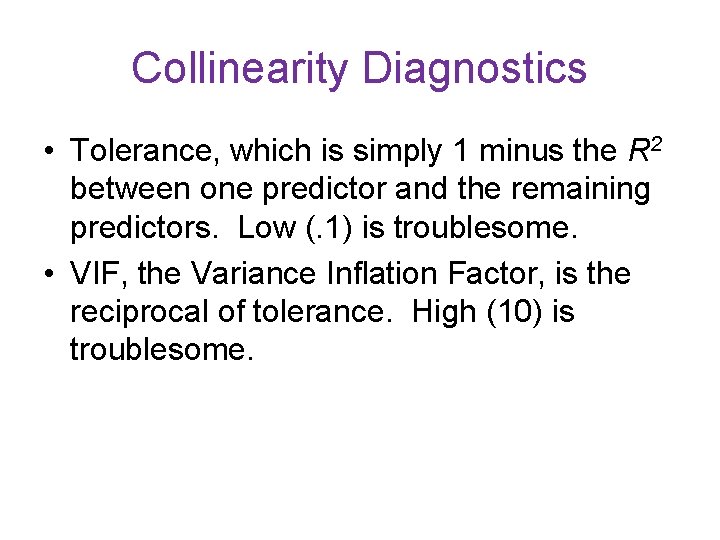
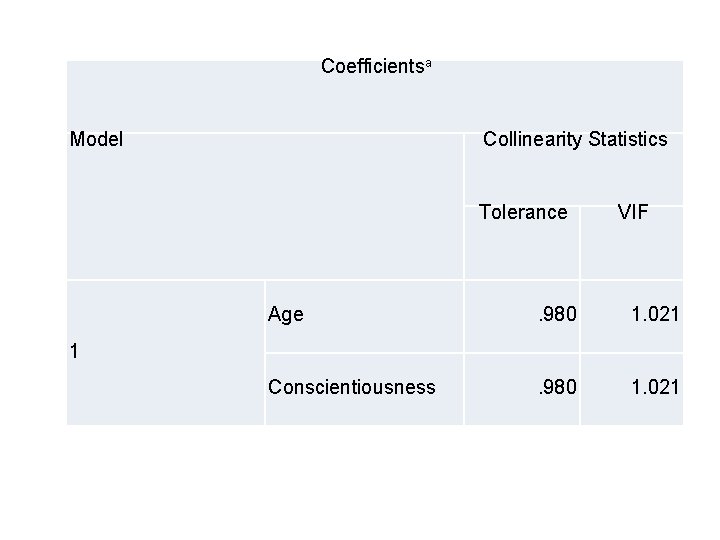
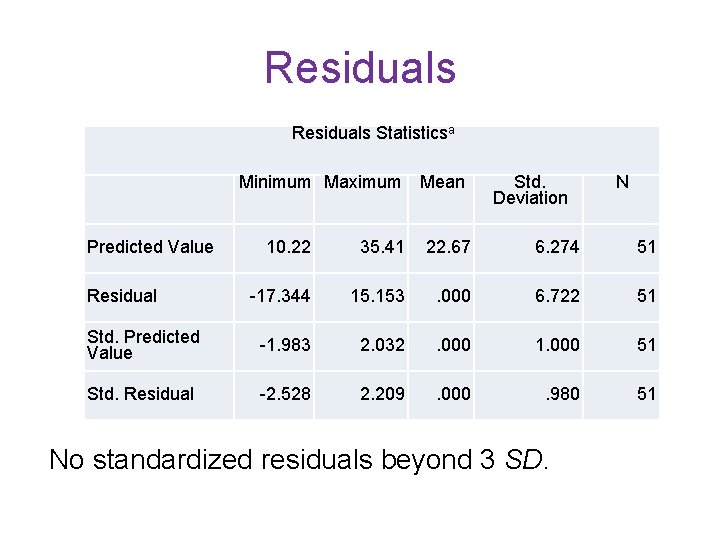
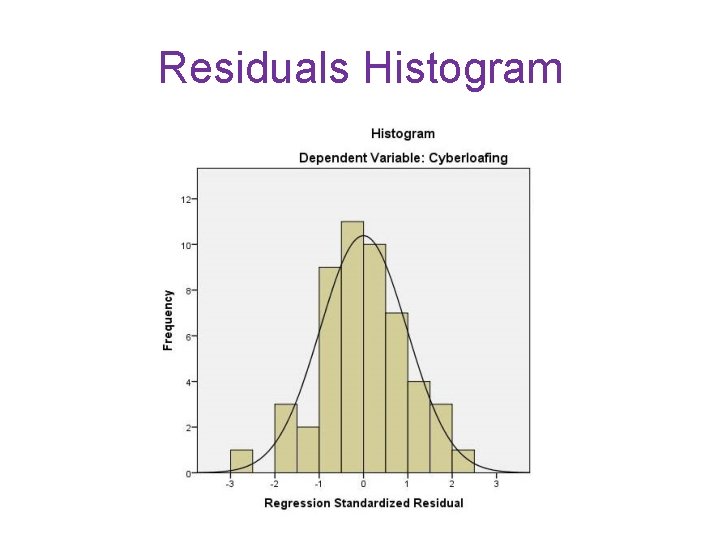
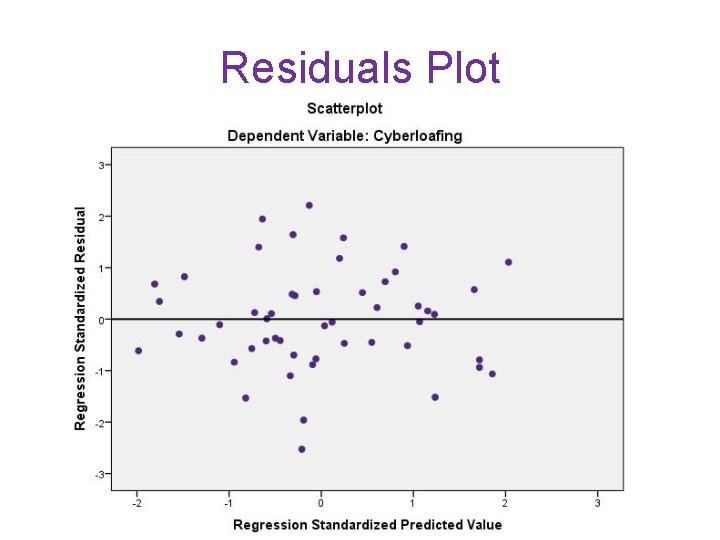
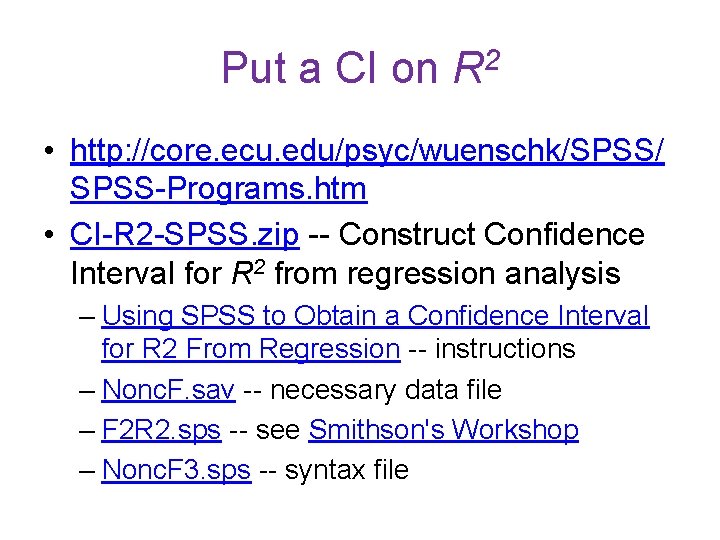
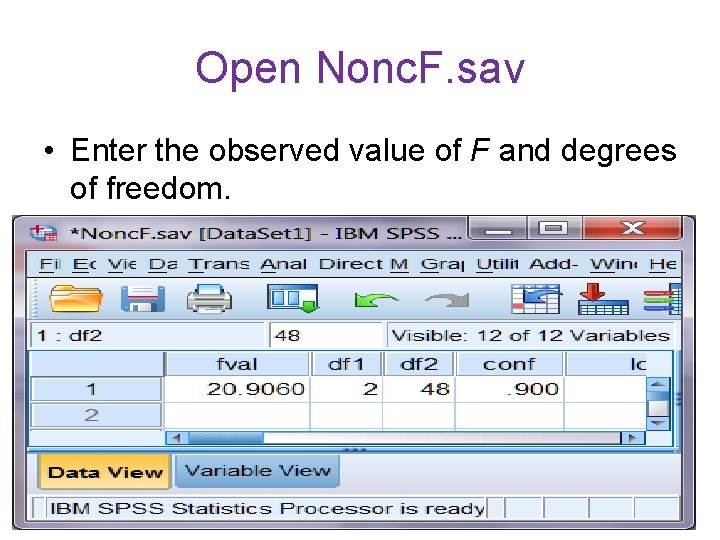
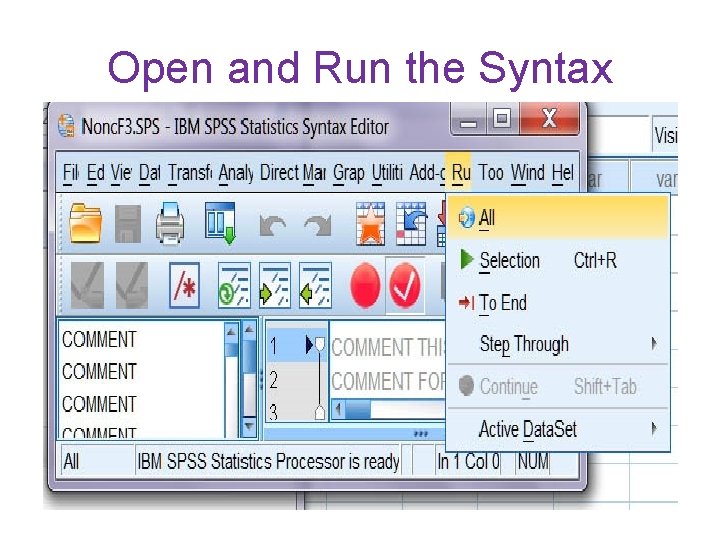
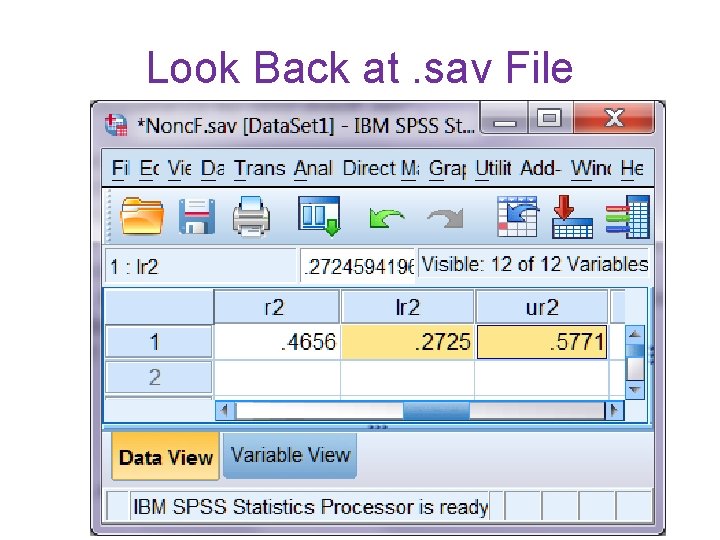
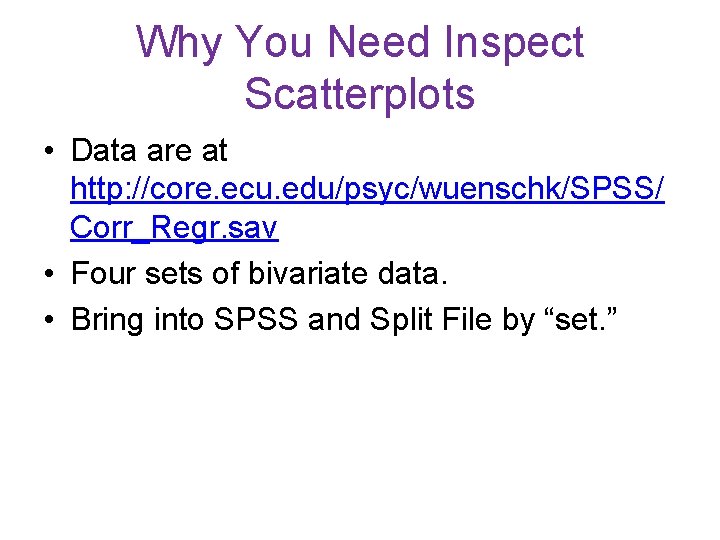
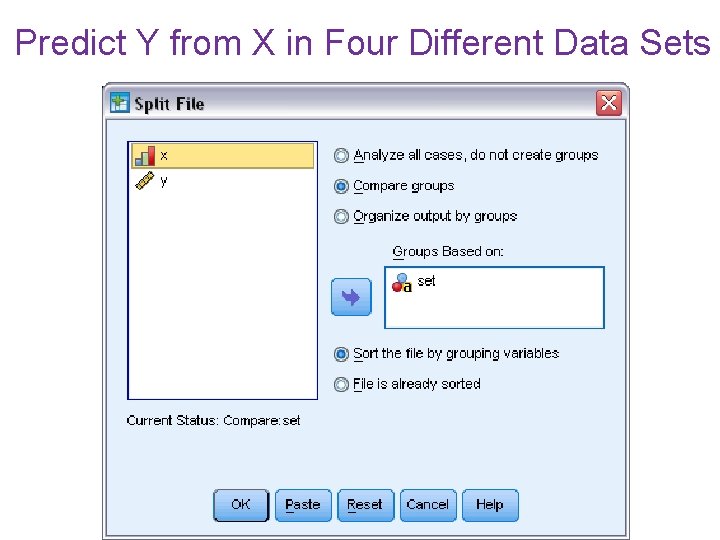
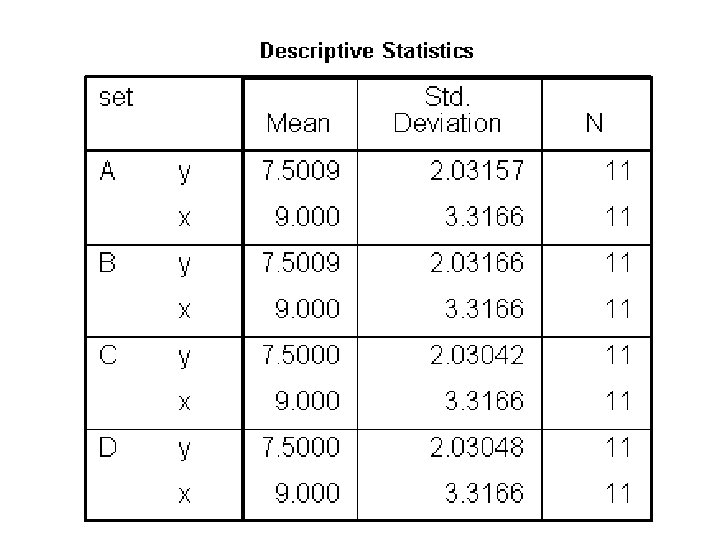
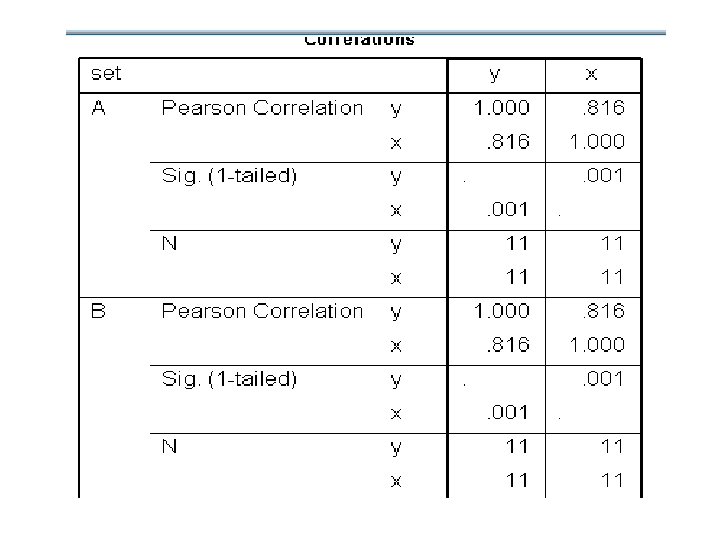
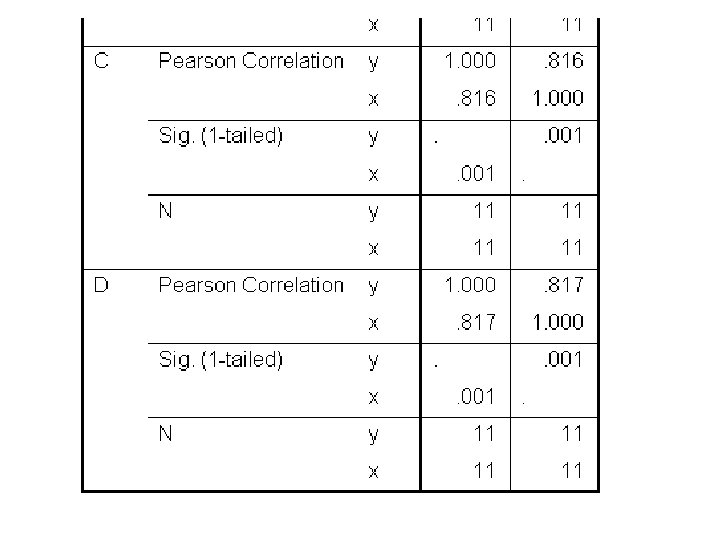
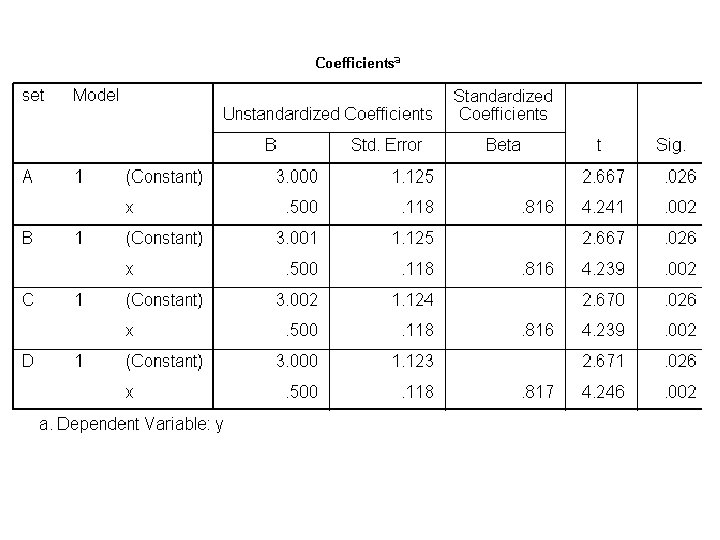
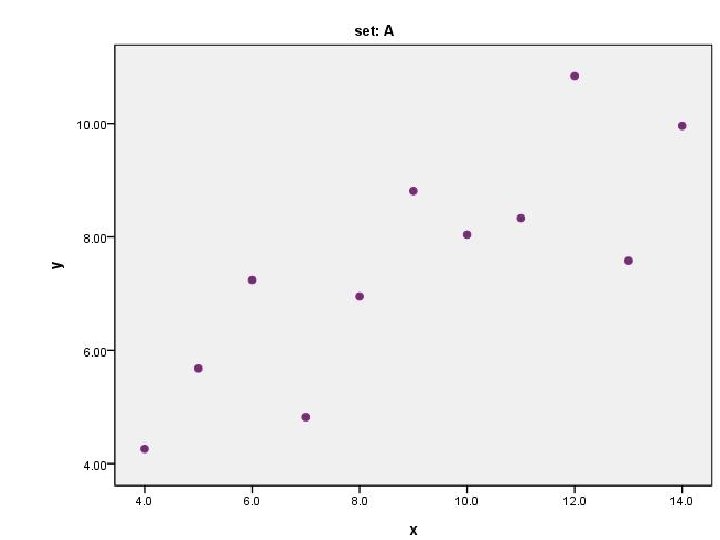
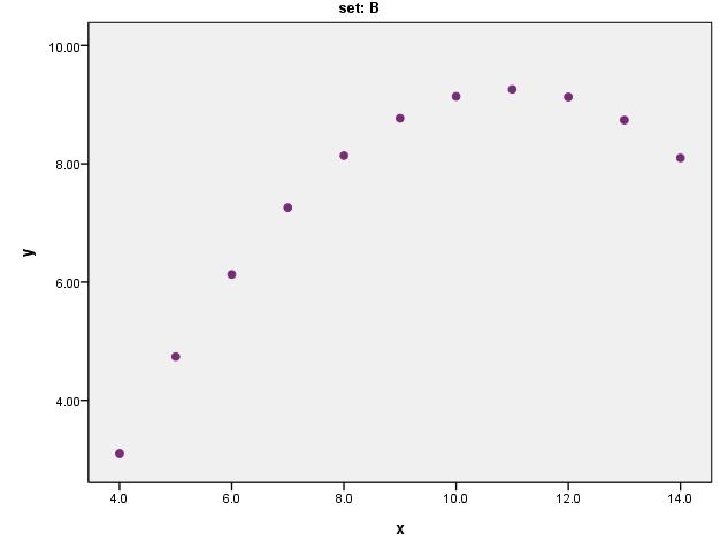
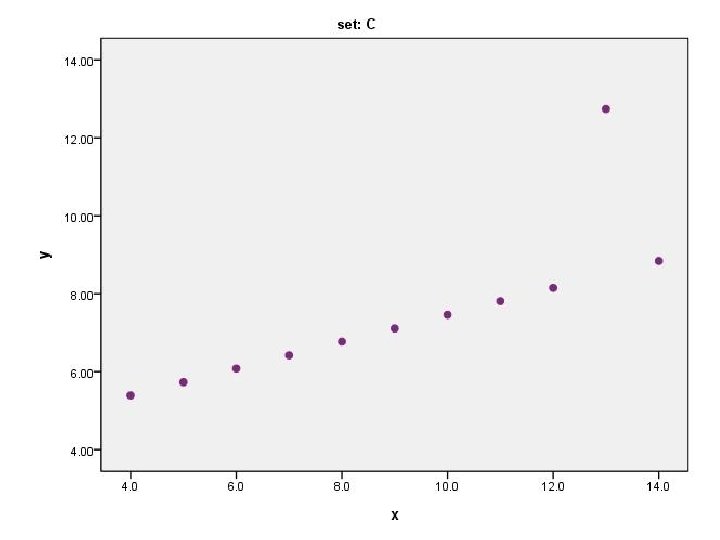
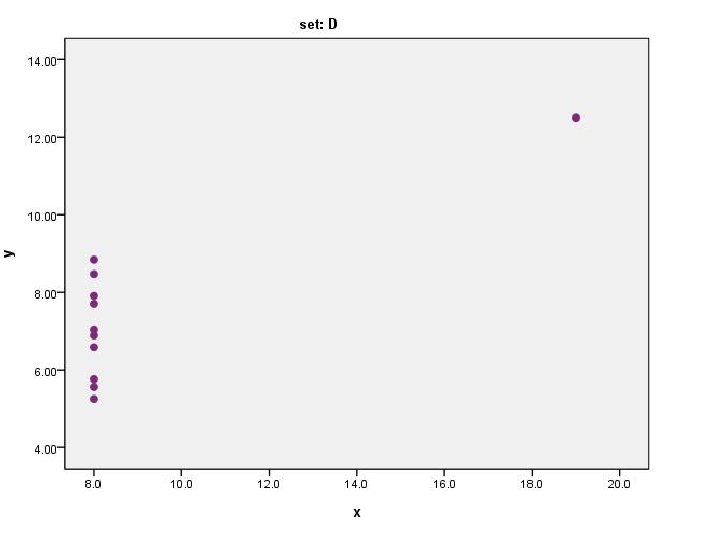
- Slides: 50
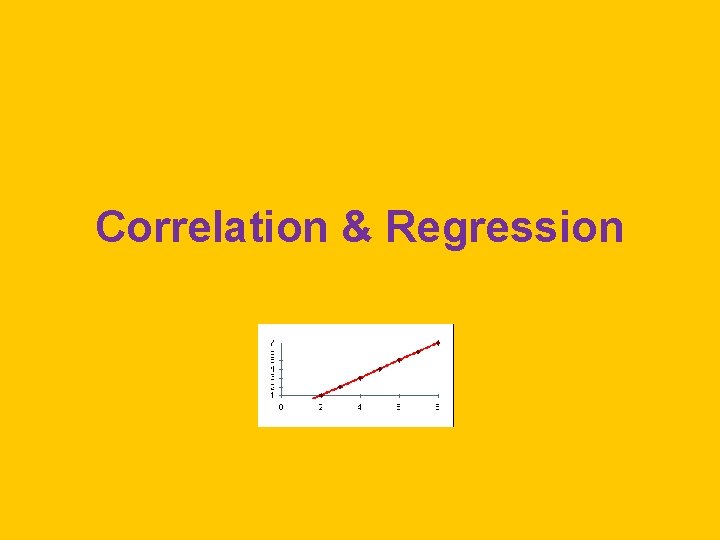
Correlation & Regression
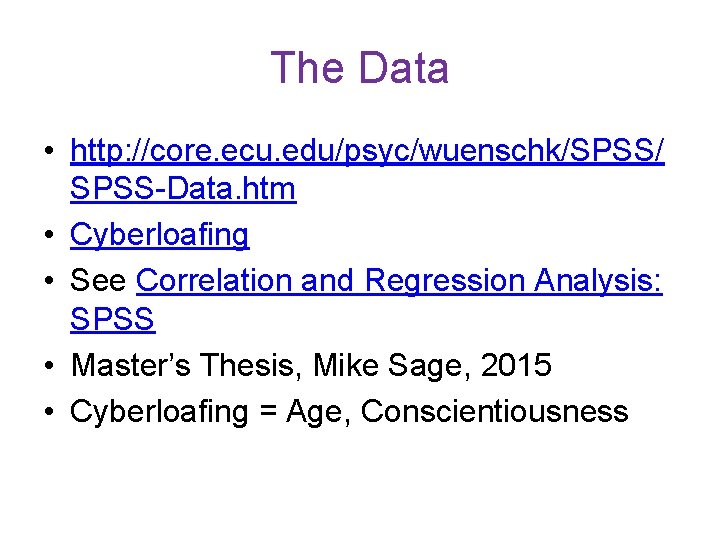
The Data • http: //core. ecu. edu/psyc/wuenschk/SPSS/ SPSS-Data. htm • Cyberloafing • See Correlation and Regression Analysis: SPSS • Master’s Thesis, Mike Sage, 2015 • Cyberloafing = Age, Conscientiousness
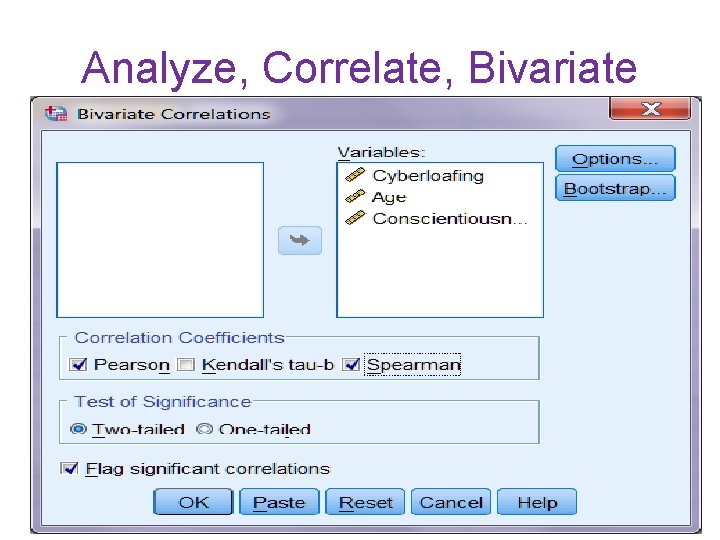
Analyze, Correlate, Bivariate
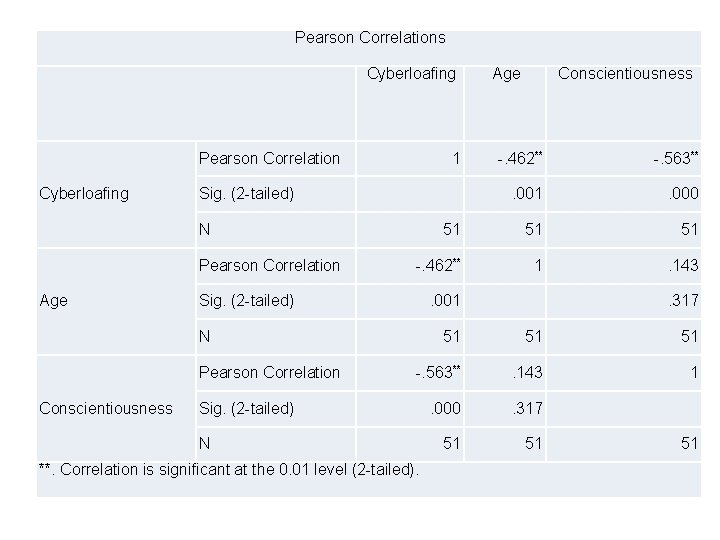
Pearson Correlations Cyberloafing Pearson Correlation Cyberloafing Sig. (2 -tailed) 1 Age -. 563** . 001 . 000 51 51 51 -. 462** 1 . 143 . 001 Sig. (2 -tailed) N Pearson Correlation Conscientiousness -. 462** N Pearson Correlation Age . 317 51 51 51 -. 563** . 143 1 Sig. (2 -tailed) N **. Correlation is significant at the 0. 01 level (2 -tailed). . 000 51 . 317 51 51
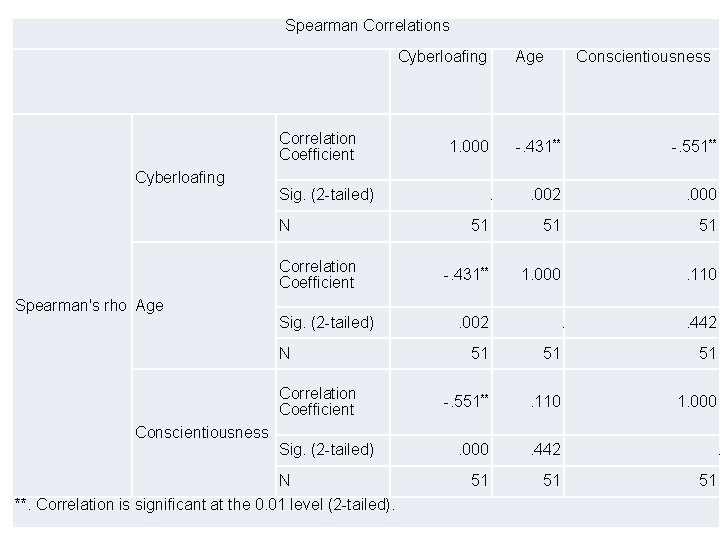
Spearman Correlations Cyberloafing Correlation Coefficient Spearman's rho Age Sig. (2 -tailed) N Correlation Coefficient Conscientiousness 1. 000 Sig. (2 -tailed) N **. Correlation is significant at the 0. 01 level (2 -tailed). Conscientiousness -. 431** -. 551** . 002 . 000 51 51 51 -. 431** 1. 000 . 110 Sig. (2 -tailed) N Age . . 002 . . 442 51 51 51 -. 551** . 110 1. 000 . 442 51 51
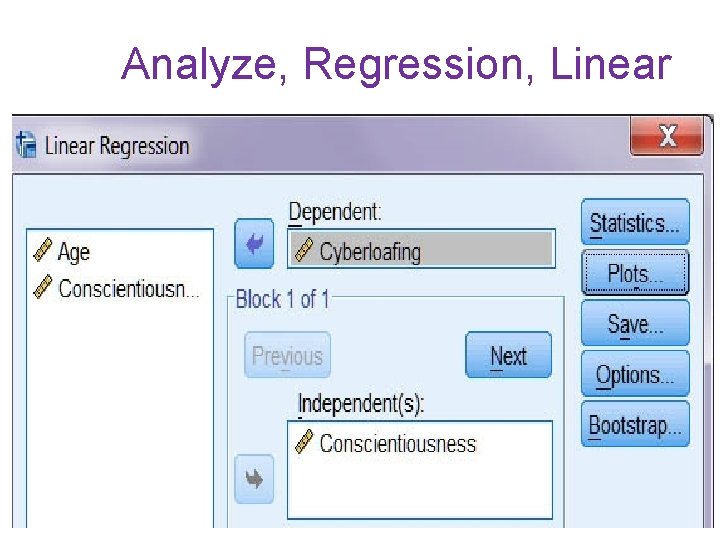
Analyze, Regression, Linear
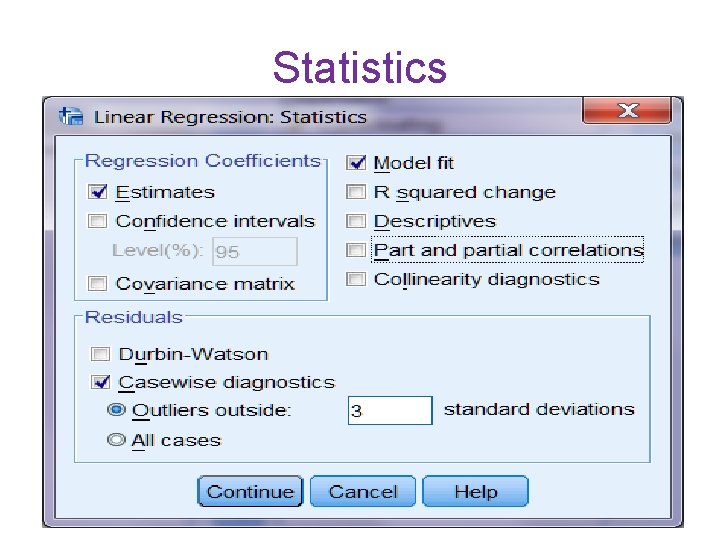
Statistics
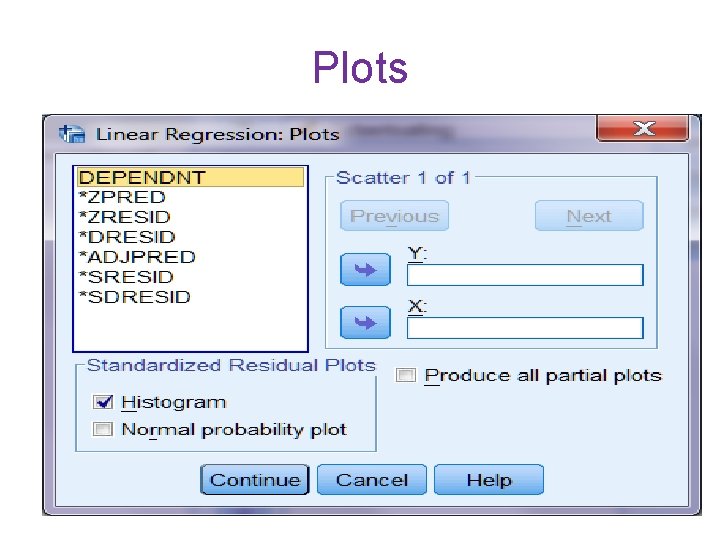
Plots
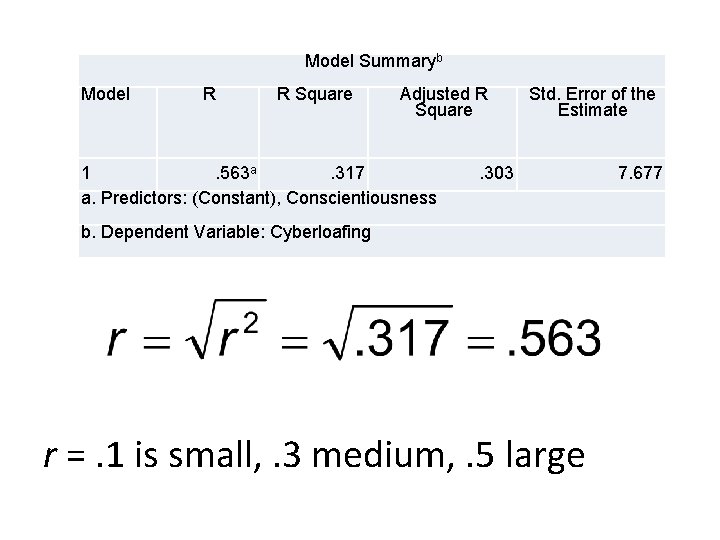
Model Summaryb Model R R Square Adjusted R Square 1. 563 a. 317 a. Predictors: (Constant), Conscientiousness Std. Error of the Estimate . 303 b. Dependent Variable: Cyberloafing r =. 1 is small, . 3 medium, . 5 large 7. 677
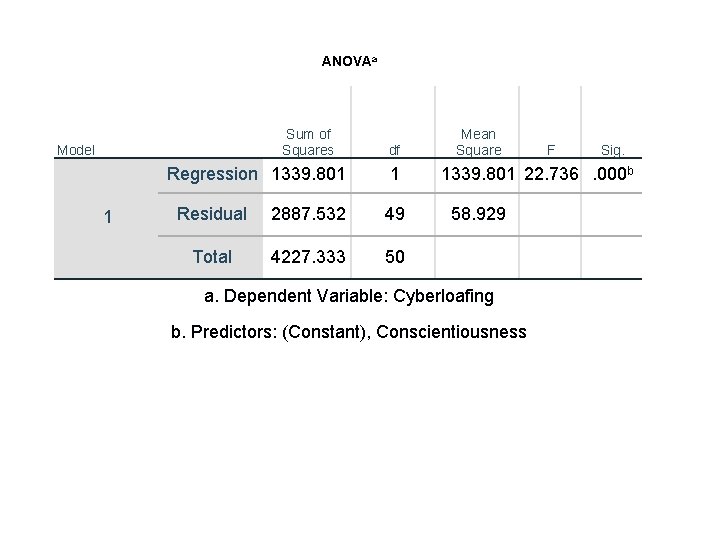
ANOVAa Sum of Squares Model Regression 1339. 801 1 df 1 Mean Square F Sig. 1339. 801 22. 736. 000 b Residual 2887. 532 49 58. 929 Total 4227. 333 50 a. Dependent Variable: Cyberloafing b. Predictors: (Constant), Conscientiousness
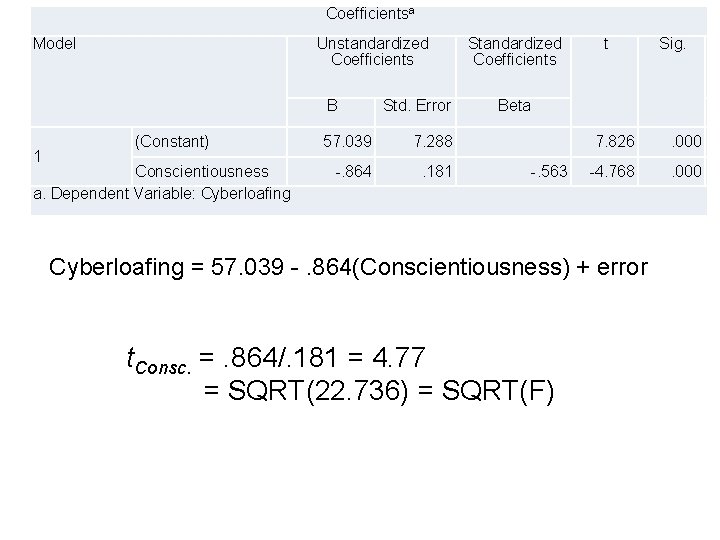
Coefficientsa Model Unstandardized Coefficients B 1 (Constant) Conscientiousness a. Dependent Variable: Cyberloafing 57. 039 -. 864 Std. Error Standardized Coefficients -. 563 7. 826 . 000 -4. 768 . 000 Cyberloafing = 57. 039 -. 864(Conscientiousness) + error t. Consc. =. 864/. 181 = 4. 77 = SQRT(22. 736) = SQRT(F) Sig. Beta 7. 288 . 181 t
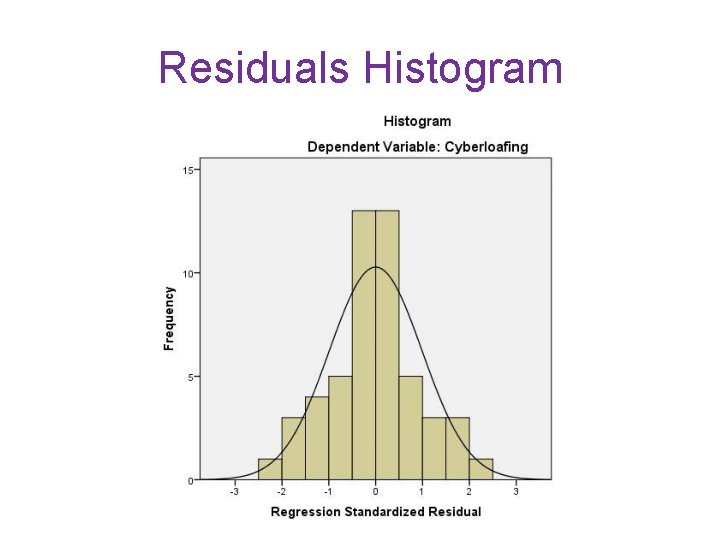
Residuals Histogram
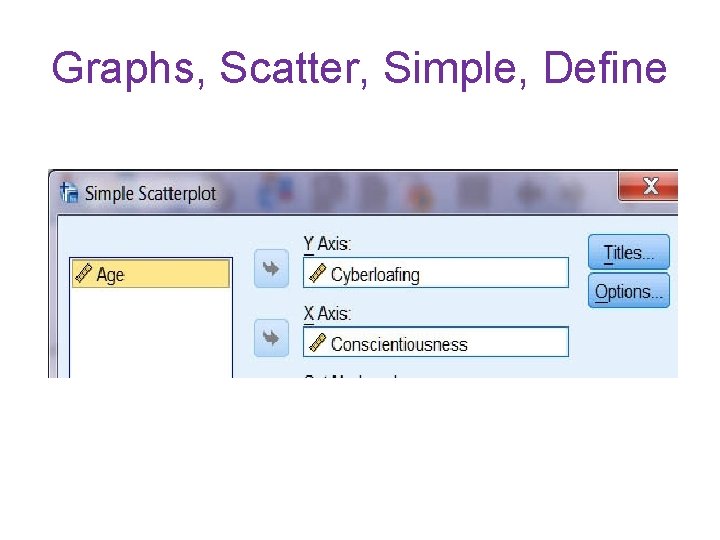
Graphs, Scatter, Simple, Define
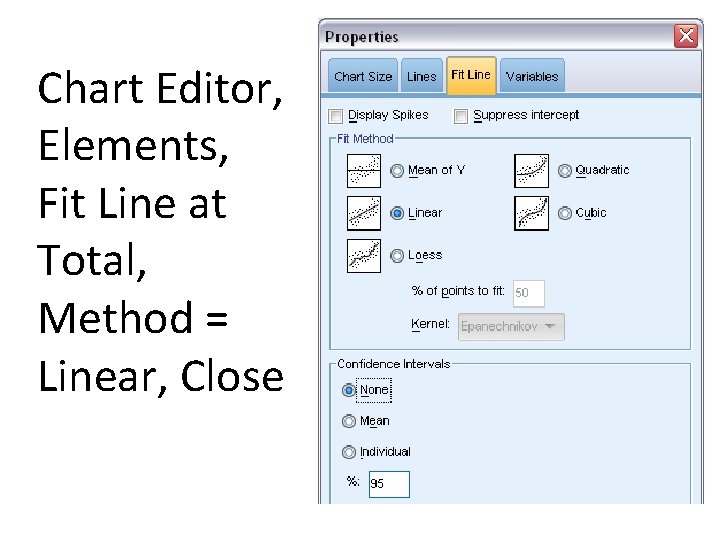
Chart Editor, Elements, Fit Line at Total, Method = Linear, Close
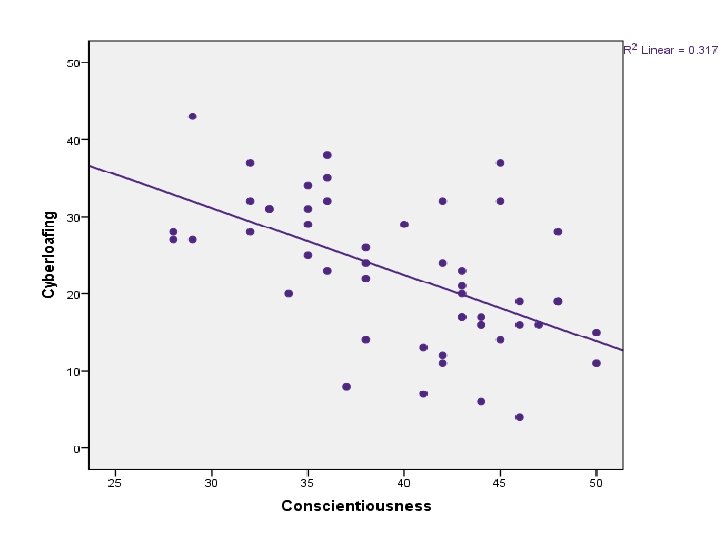
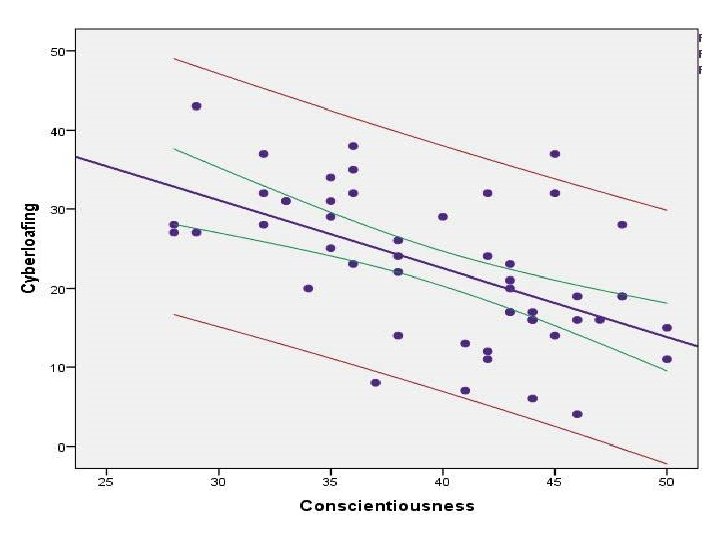
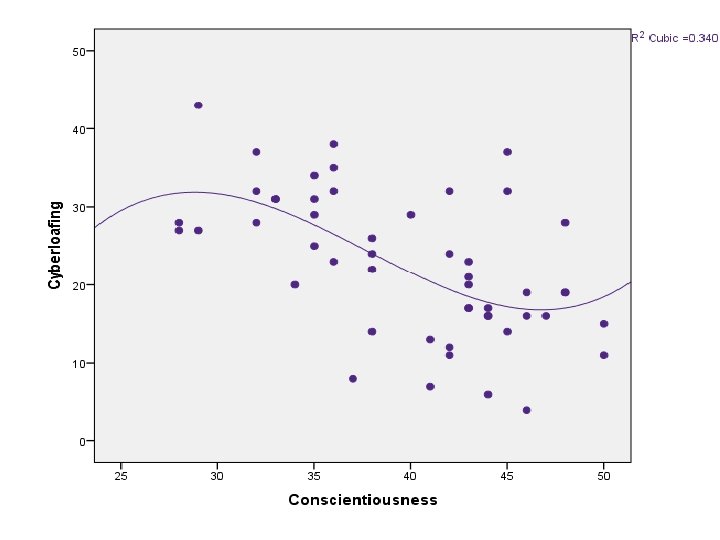
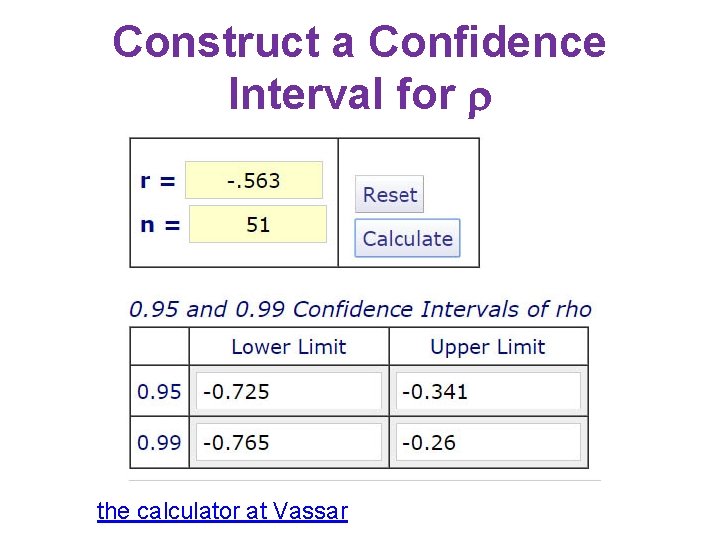
Construct a Confidence Interval for the calculator at Vassar
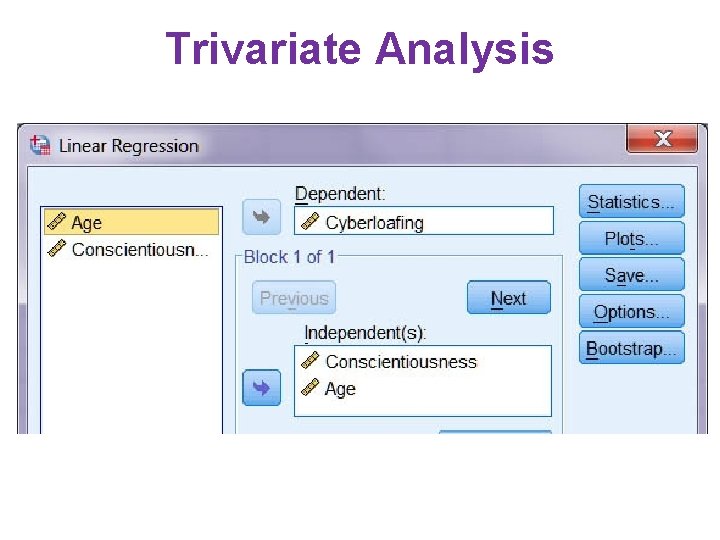
Trivariate Analysis
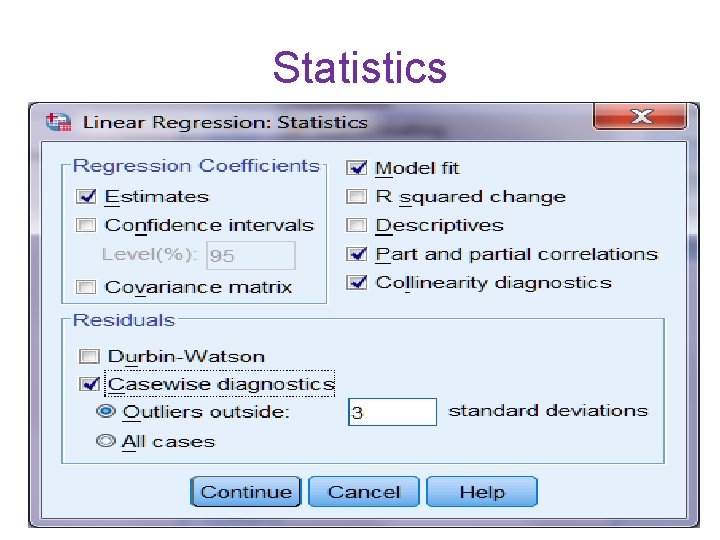
Statistics
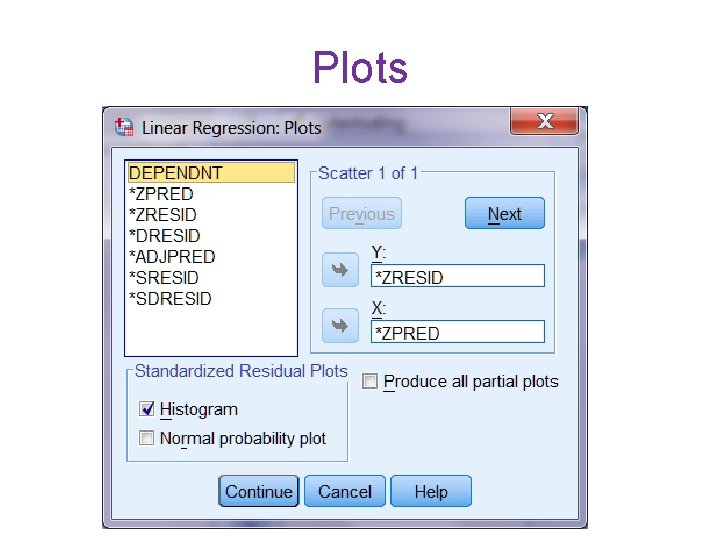
Plots
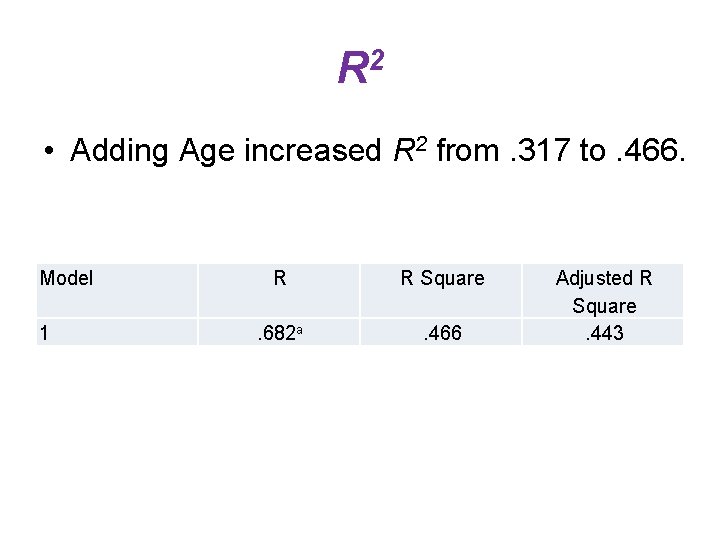
R 2 • Adding Age increased R 2 from. 317 to. 466. Model 1 R R Square . 682 a . 466 Adjusted R Square. 443
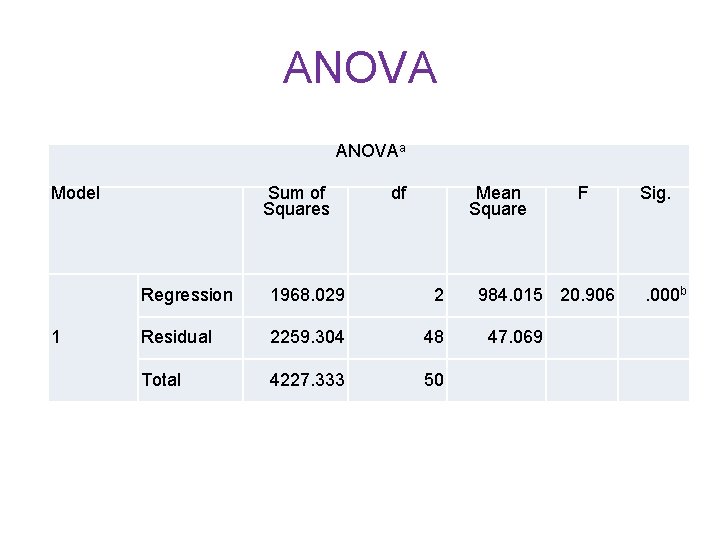
ANOVAa Model 1 Sum of Squares df Mean Square Regression 1968. 029 2 Residual 2259. 304 48 Total 4227. 333 50 F Sig. 984. 015 20. 906 . 000 b 47. 069
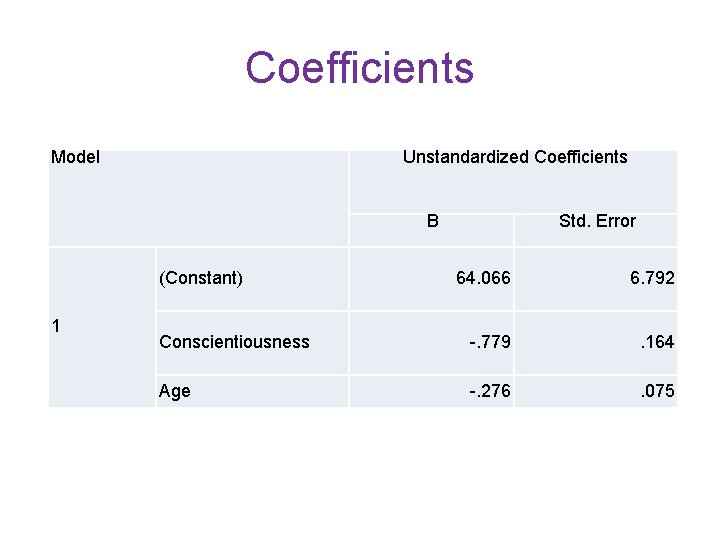
Coefficients Model Unstandardized Coefficients B (Constant) 1 Std. Error 64. 066 6. 792 Conscientiousness -. 779 . 164 Age -. 276 . 075
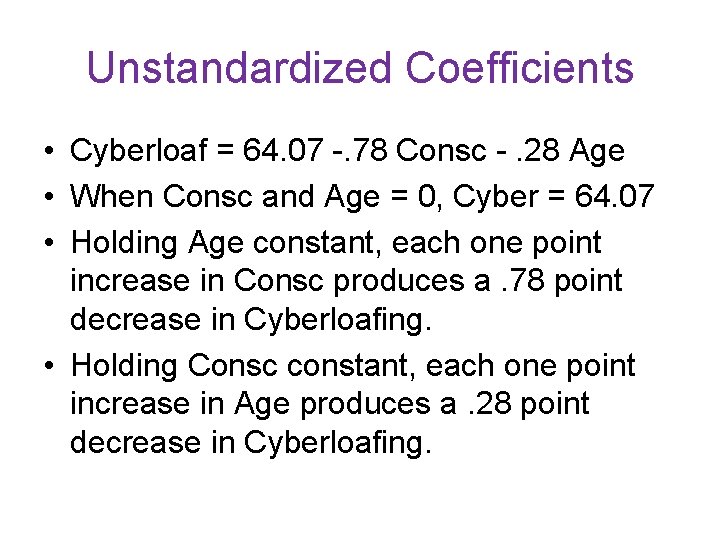
Unstandardized Coefficients • Cyberloaf = 64. 07 -. 78 Consc -. 28 Age • When Consc and Age = 0, Cyber = 64. 07 • Holding Age constant, each one point increase in Consc produces a. 78 point decrease in Cyberloafing. • Holding Consc constant, each one point increase in Age produces a. 28 point decrease in Cyberloafing.
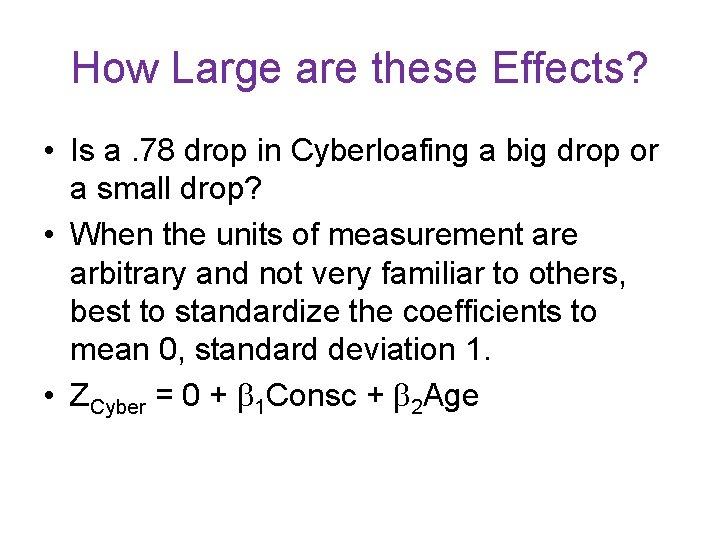
How Large are these Effects? • Is a. 78 drop in Cyberloafing a big drop or a small drop? • When the units of measurement are arbitrary and not very familiar to others, best to standardize the coefficients to mean 0, standard deviation 1. • ZCyber = 0 + 1 Consc + 2 Age
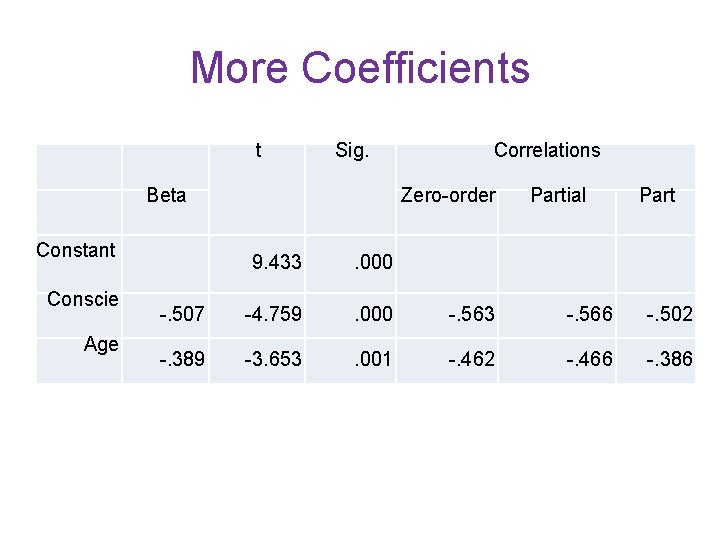
More Coefficients Beta Constant Conscie Age t Sig. Correlations Zero-order Partial Part 9. 433 . 000 -. 507 -4. 759 . 000 -. 563 -. 566 -. 502 -. 389 -3. 653 . 001 -. 462 -. 466 -. 386
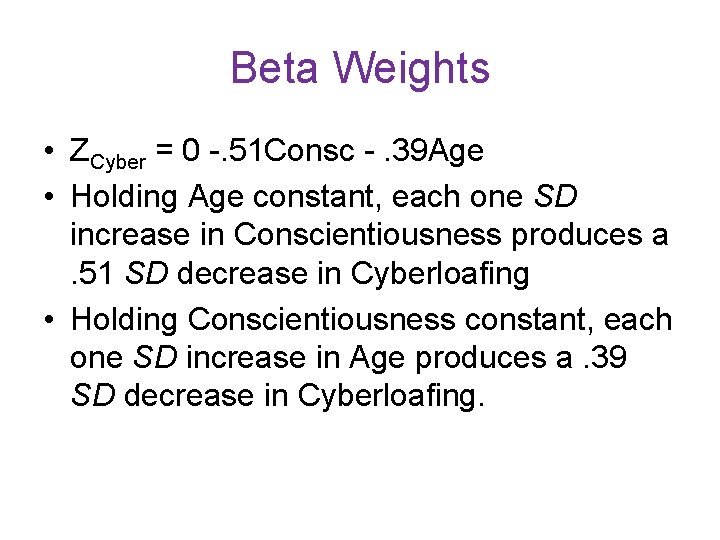
Beta Weights • ZCyber = 0 -. 51 Consc -. 39 Age • Holding Age constant, each one SD increase in Conscientiousness produces a . 51 SD decrease in Cyberloafing • Holding Conscientiousness constant, each one SD increase in Age produces a. 39 SD decrease in Cyberloafing.
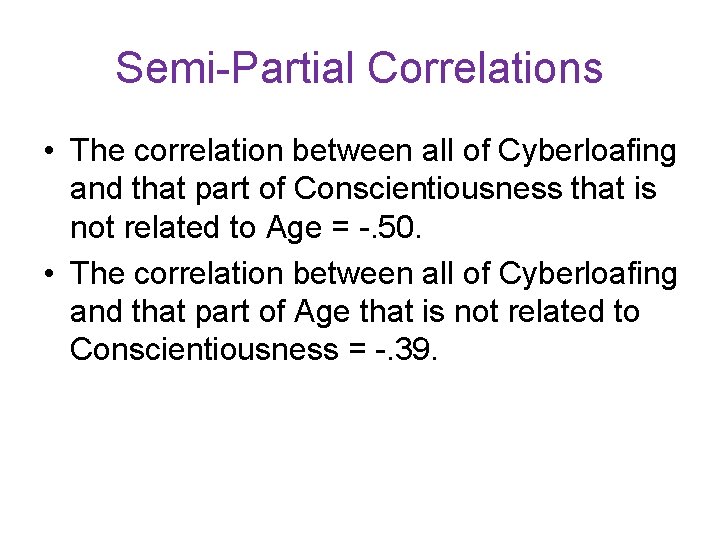
Semi-Partial Correlations • The correlation between all of Cyberloafing and that part of Conscientiousness that is not related to Age = -. 50. • The correlation between all of Cyberloafing and that part of Age that is not related to Conscientiousness = -. 39.
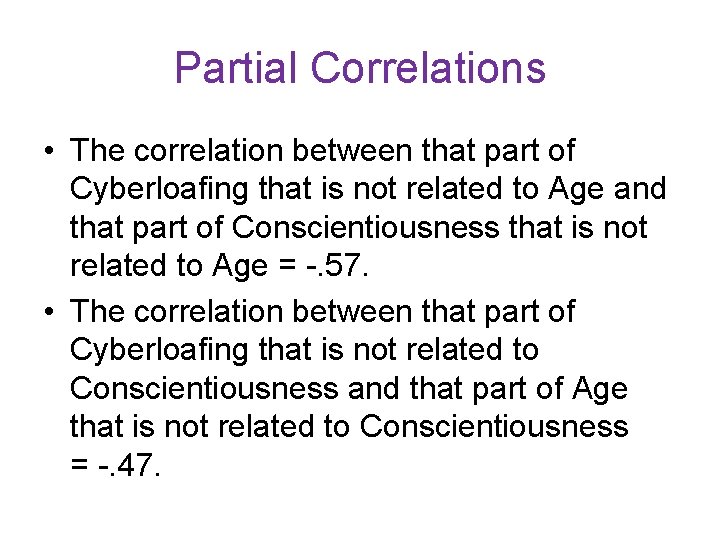
Partial Correlations • The correlation between that part of Cyberloafing that is not related to Age and that part of Conscientiousness that is not related to Age = -. 57. • The correlation between that part of Cyberloafing that is not related to Conscientiousness and that part of Age that is not related to Conscientiousness = -. 47.
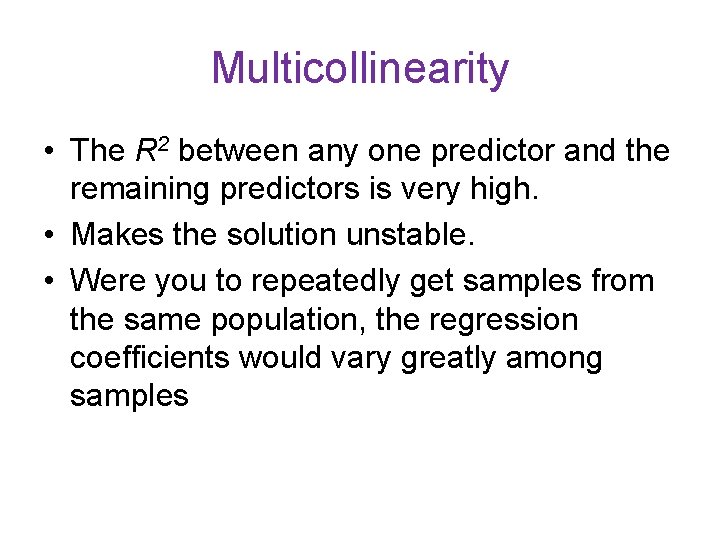
Multicollinearity • The R 2 between any one predictor and the remaining predictors is very high. • Makes the solution unstable. • Were you to repeatedly get samples from the same population, the regression coefficients would vary greatly among samples
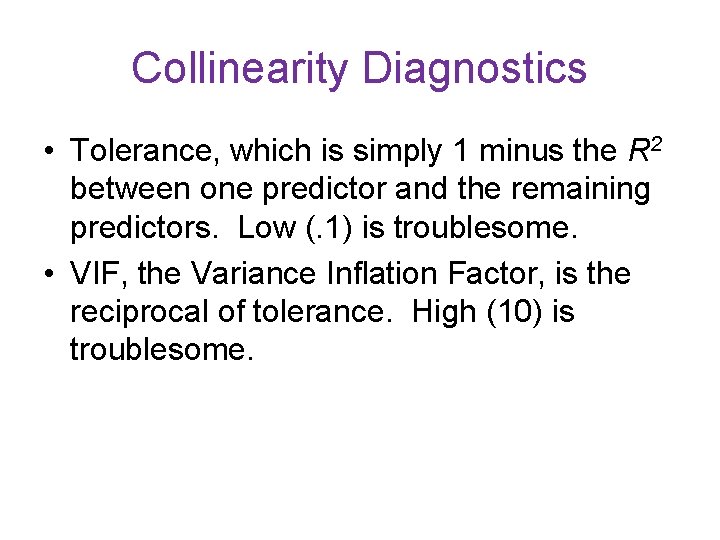
Collinearity Diagnostics • Tolerance, which is simply 1 minus the R 2 between one predictor and the remaining predictors. Low (. 1) is troublesome. • VIF, the Variance Inflation Factor, is the reciprocal of tolerance. High (10) is troublesome.
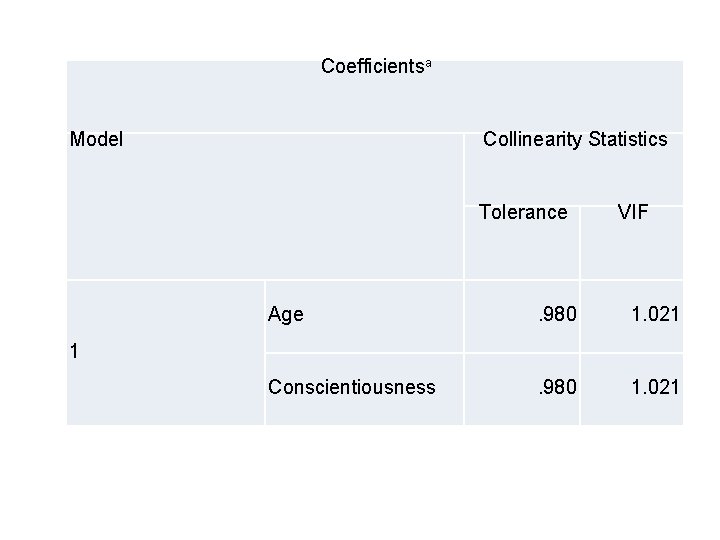
Coefficientsa Model Collinearity Statistics Tolerance VIF Age . 980 1. 021 Conscientiousness . 980 1. 021 1
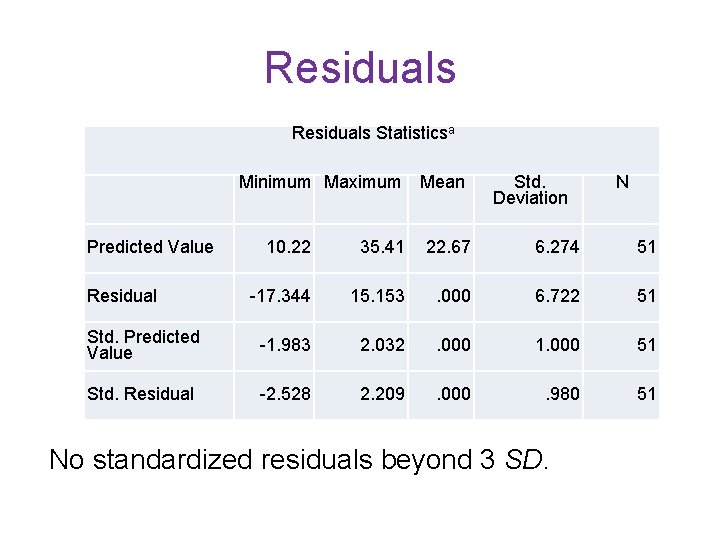
Residuals Statisticsa Predicted Value Minimum Maximum Mean Std. Deviation N 10. 22 35. 41 22. 67 6. 274 51 -17. 344 15. 153 . 000 6. 722 51 Std. Predicted Value -1. 983 2. 032 . 000 1. 000 51 Std. Residual -2. 528 2. 209 . 000 . 980 51 Residual No standardized residuals beyond 3 SD.
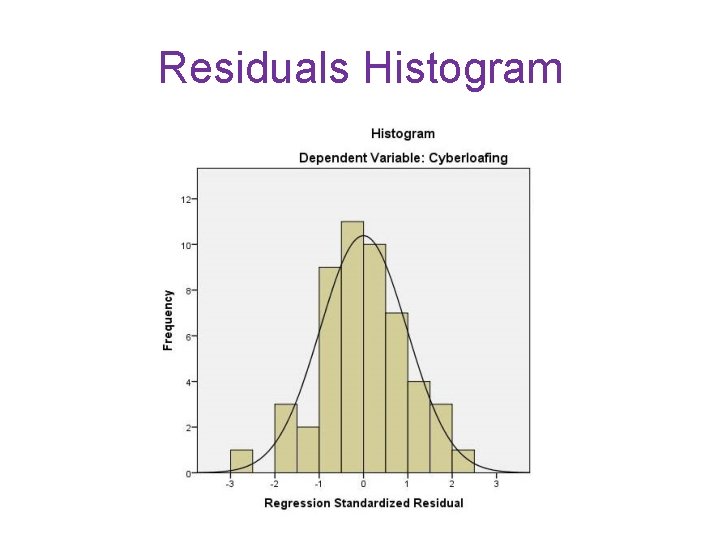
Residuals Histogram
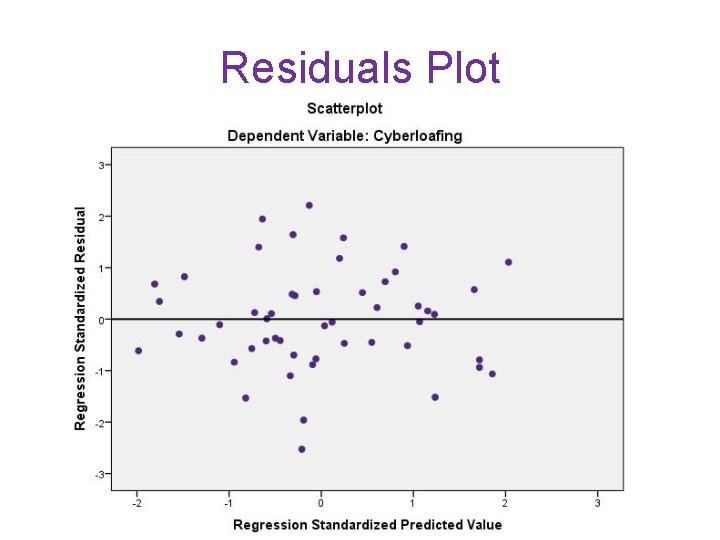
Residuals Plot
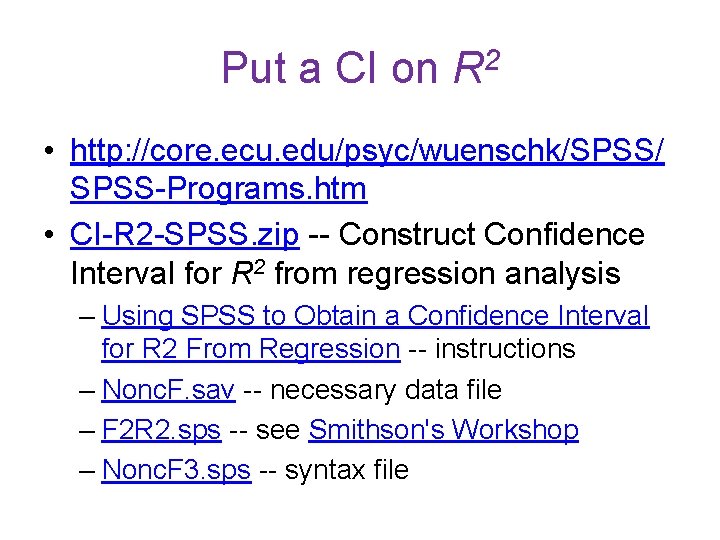
Put a CI on R 2 • http: //core. ecu. edu/psyc/wuenschk/SPSS/ SPSS-Programs. htm • CI-R 2 -SPSS. zip -- Construct Confidence Interval for R 2 from regression analysis – Using SPSS to Obtain a Confidence Interval for R 2 From Regression -- instructions – Nonc. F. sav -- necessary data file – F 2 R 2. sps -- see Smithson's Workshop – Nonc. F 3. sps -- syntax file
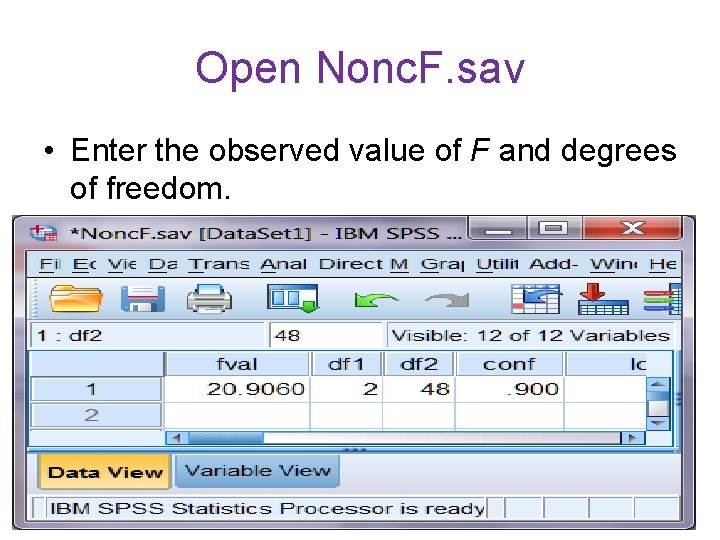
Open Nonc. F. sav • Enter the observed value of F and degrees of freedom.
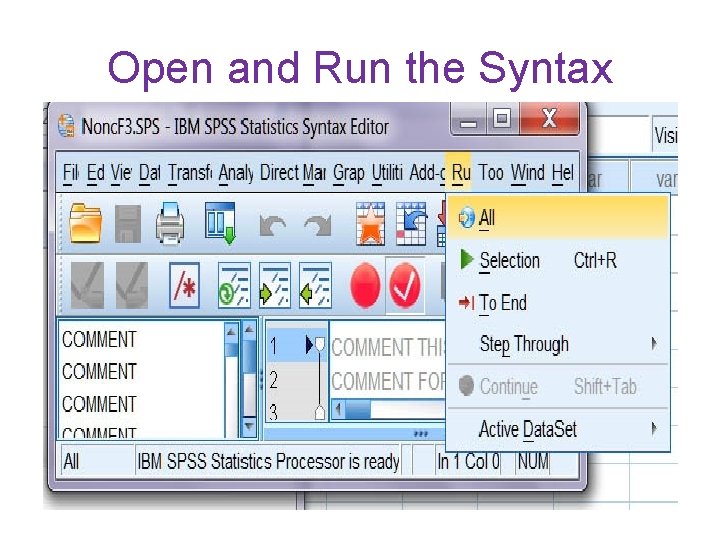
Open and Run the Syntax
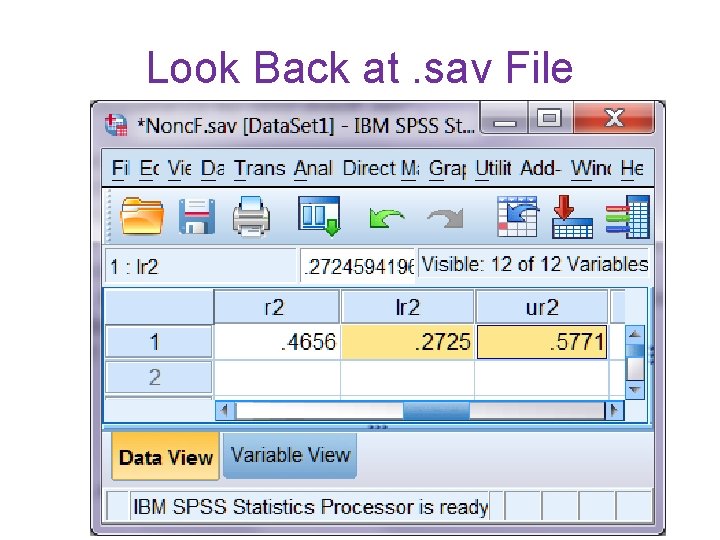
Look Back at. sav File
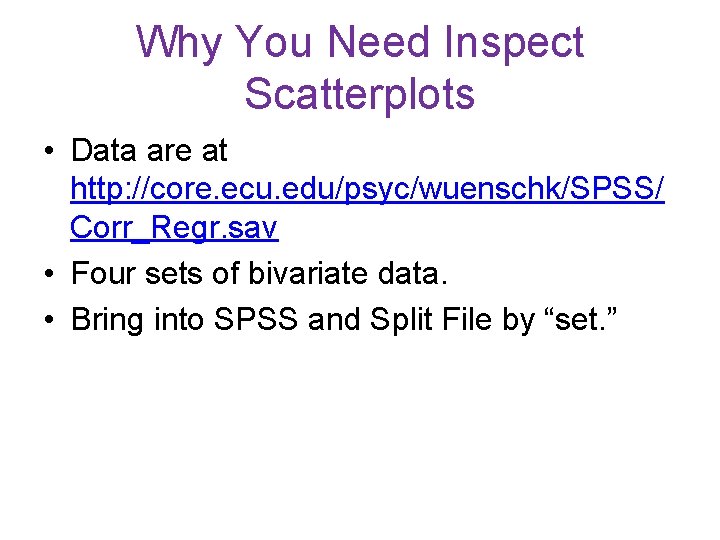
Why You Need Inspect Scatterplots • Data are at http: //core. ecu. edu/psyc/wuenschk/SPSS/ Corr_Regr. sav • Four sets of bivariate data. • Bring into SPSS and Split File by “set. ”
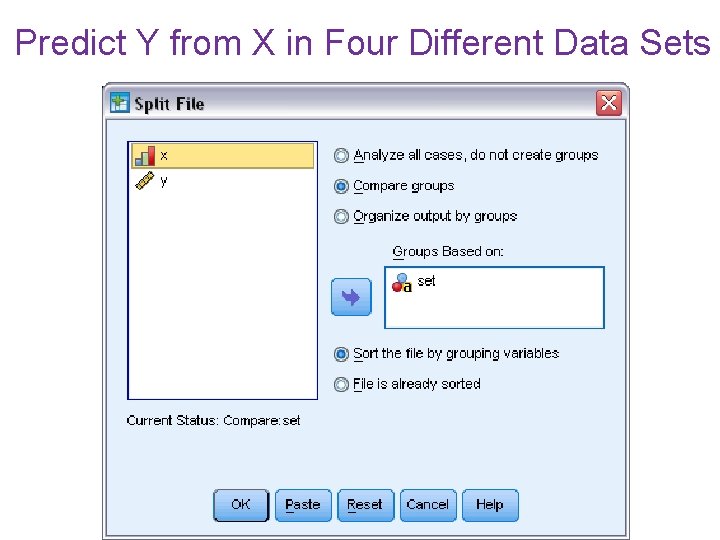
Predict Y from X in Four Different Data Sets
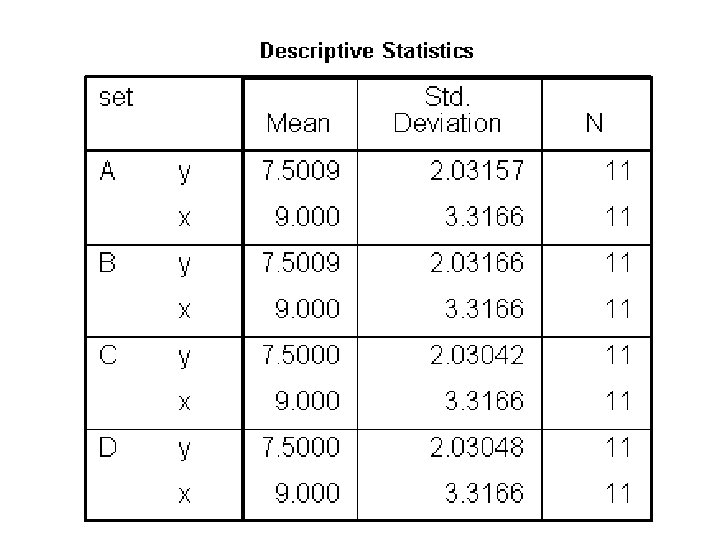
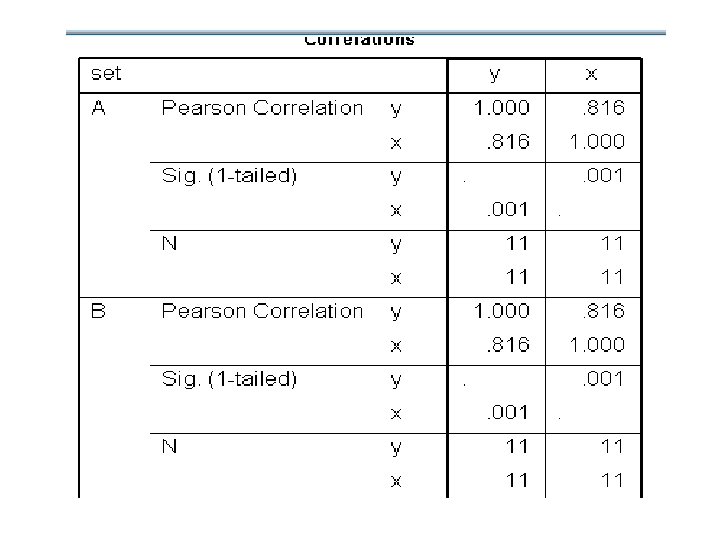
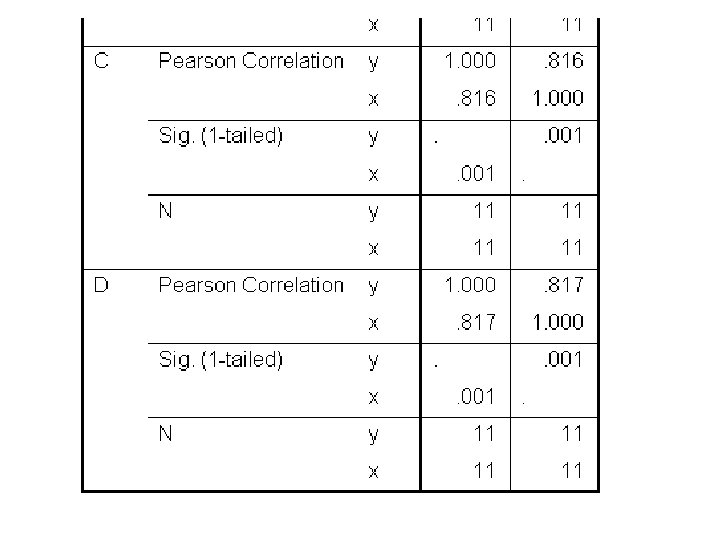
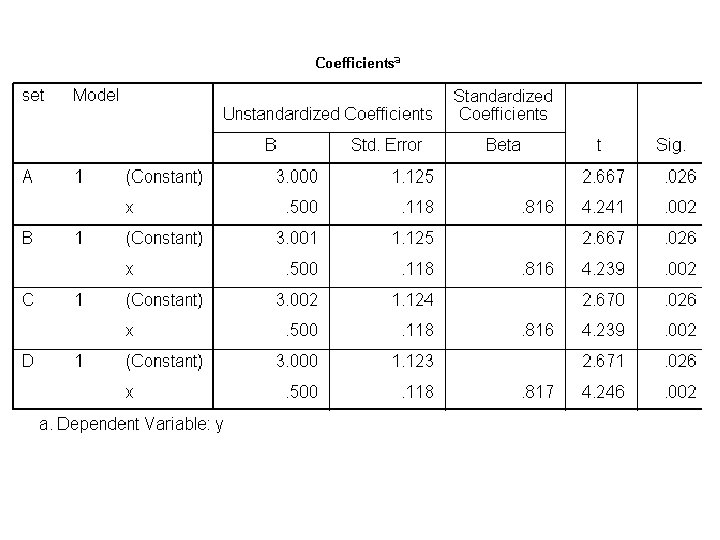
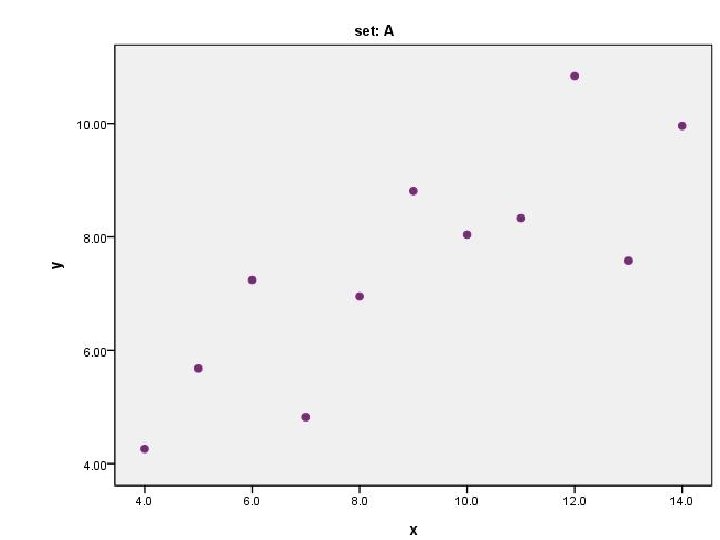
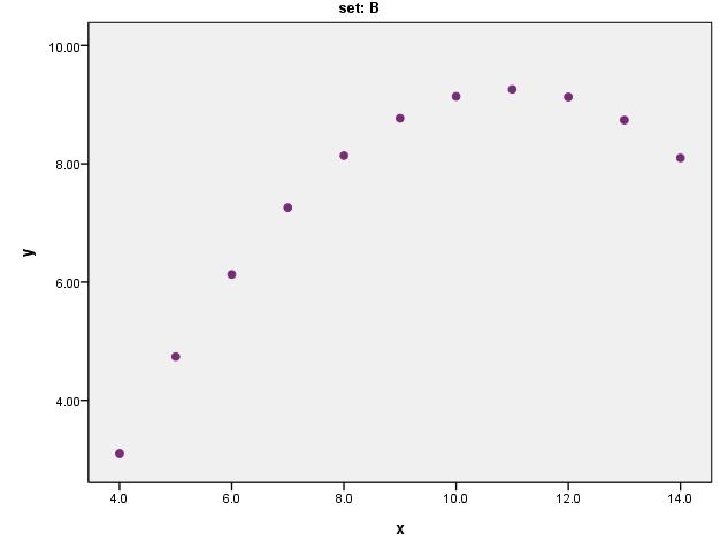
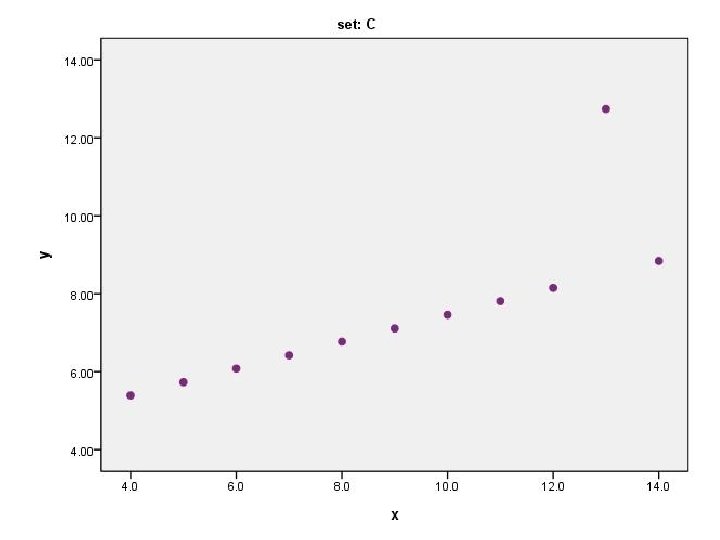
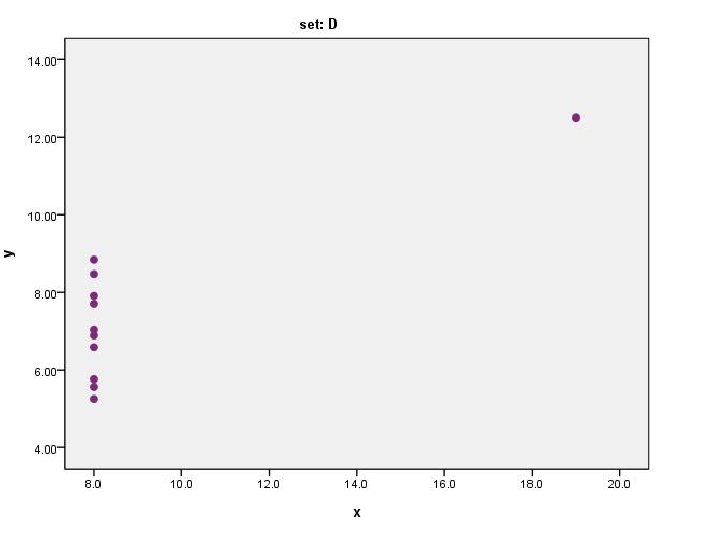
Positive correlation versus negative correlation
Positive and negative correlation
Difference between regression and correlation
Correlation and regression
Difference between correlation and regression
Regression vs correlation
Coefficient of correlation
Difference between regression and correlation
How to calculate sst in regression
Difference between correlation and regression
Bivariate vs multivariate
Contoh soal persamaan regresi dari data
Simple linear regression and multiple linear regression
Multiple linear regression
Logistic regression vs linear regression
Logistic regression vs linear regression
Correlation hypothesis example
Correlation data mining
Probable error uses the following formula
Winperl
The brittle, rocky outer layer of earth
Inner core and outer core
What are the 3 main layers of the earth? *
Purpose of paradox
Ecu weight loss surgery
Ecu weight loss surgery
Synerject fuel injection
Synerject llc
Ecu nowforce
Hayabusa ecu pinout
Extreme wide shot
Camera framing
Ecu faculty manual
Ecu campus map
Skid control ecu with actuator
Ecu itcs
East carolina pool and spa
Degreeworks ecu
Ecu talk
Ecu joyner
Pebblepad ecu
Mg hv battery shut off
Ecu eduroam
Ken robinson ecu
Monkeyrunner python script examples
Ecu cu mcu ms ws ews
Ecu environmental health
Ecu reu
Http //mbs.meb.gov.tr/ http //www.alantercihleri.com
Http //pelatihan tik.ung.ac.id
Logistic regression data mining