Clusters in data Common Statistical Task Find Clusters
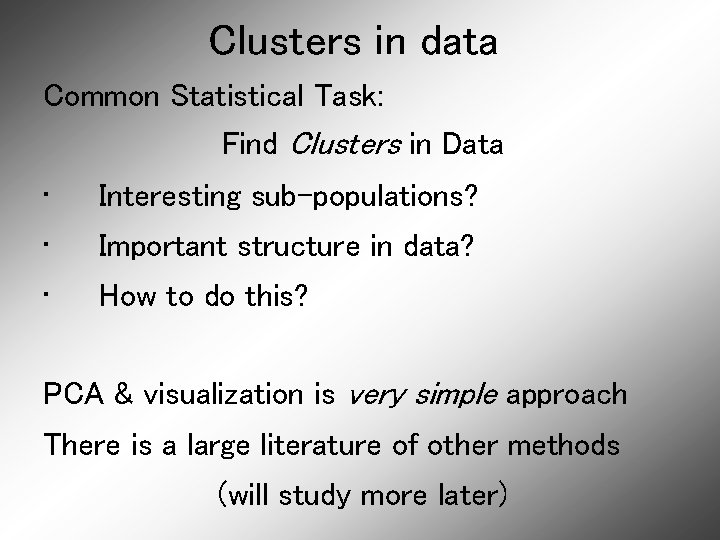
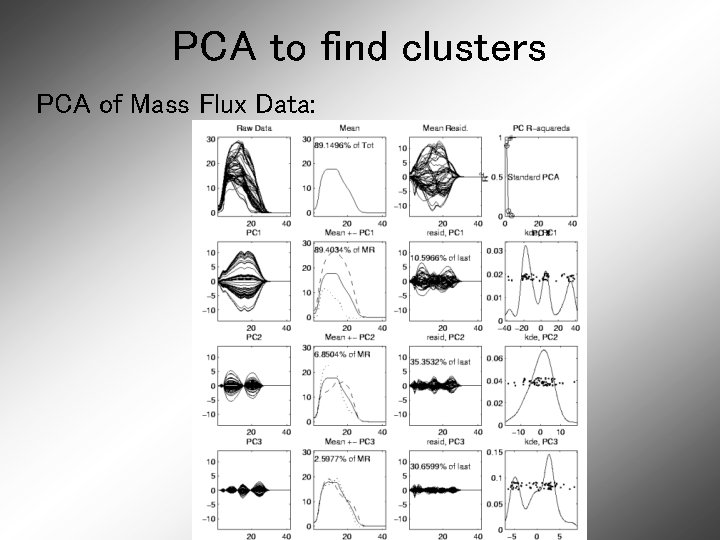
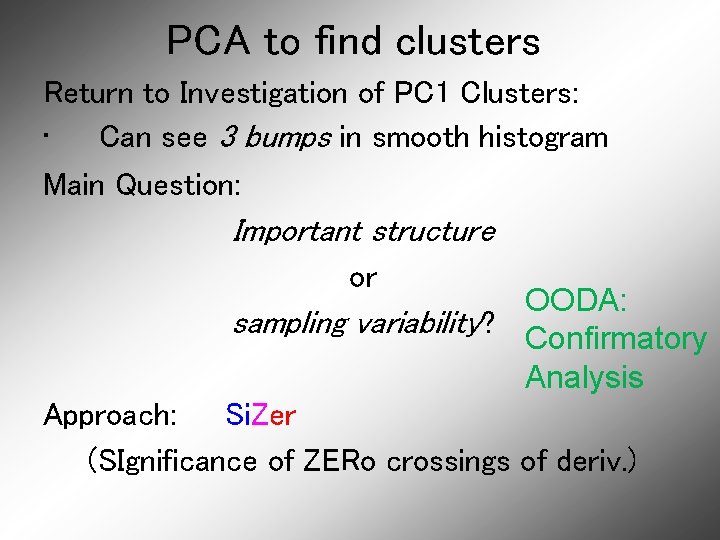
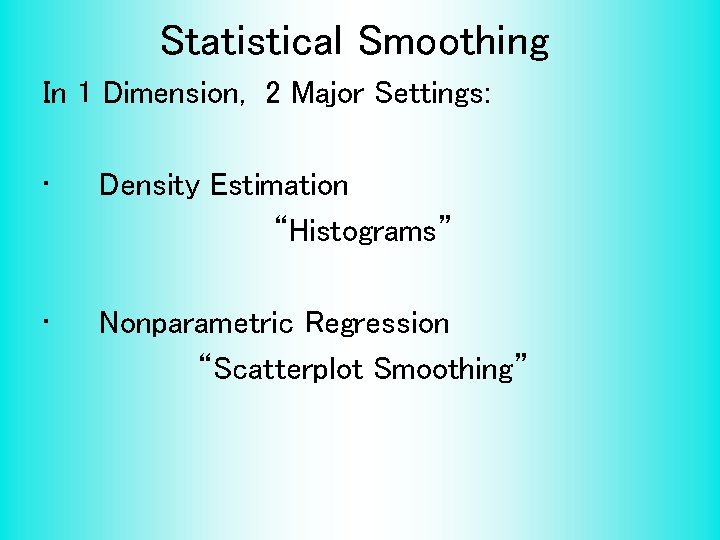
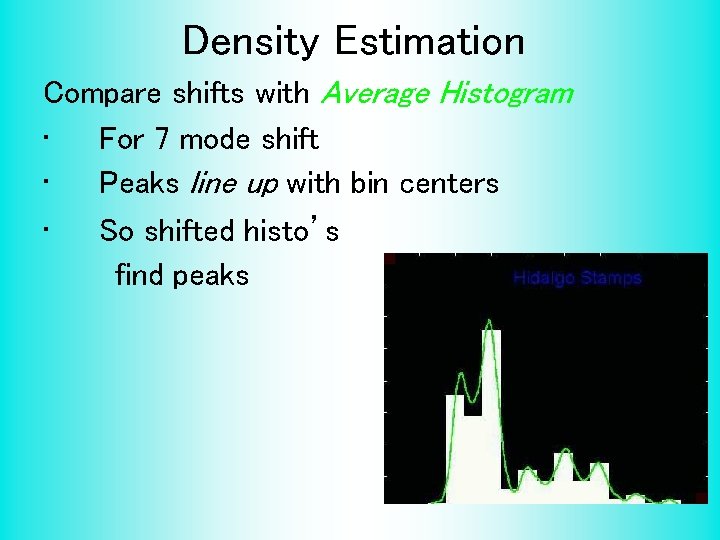
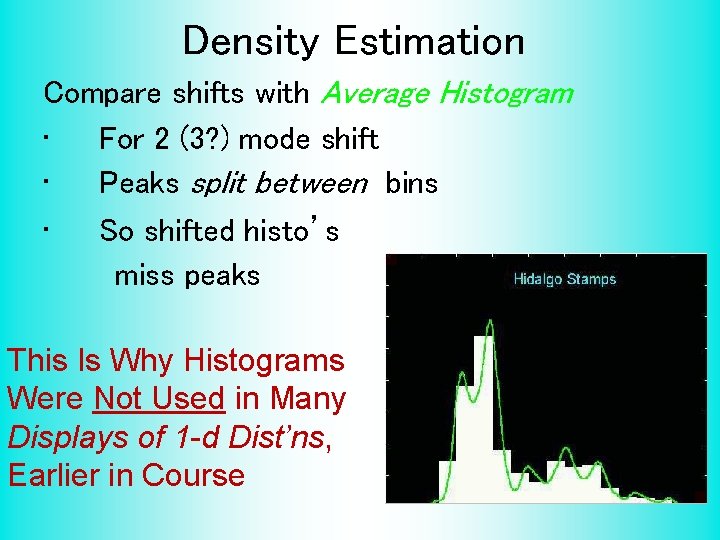
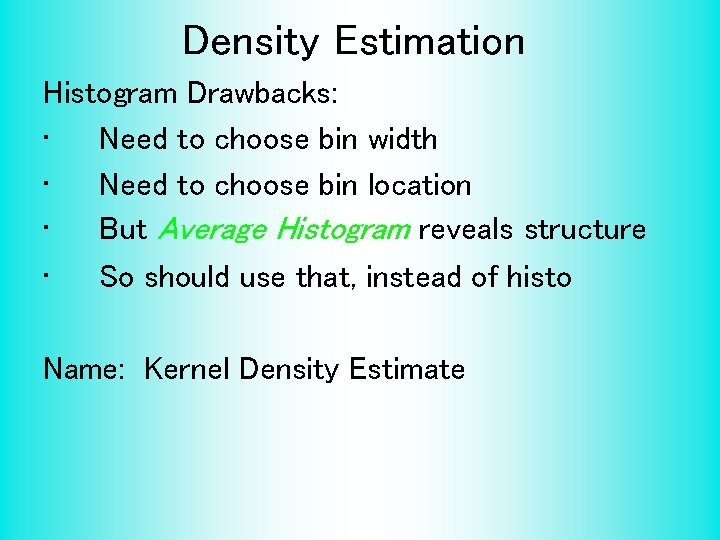
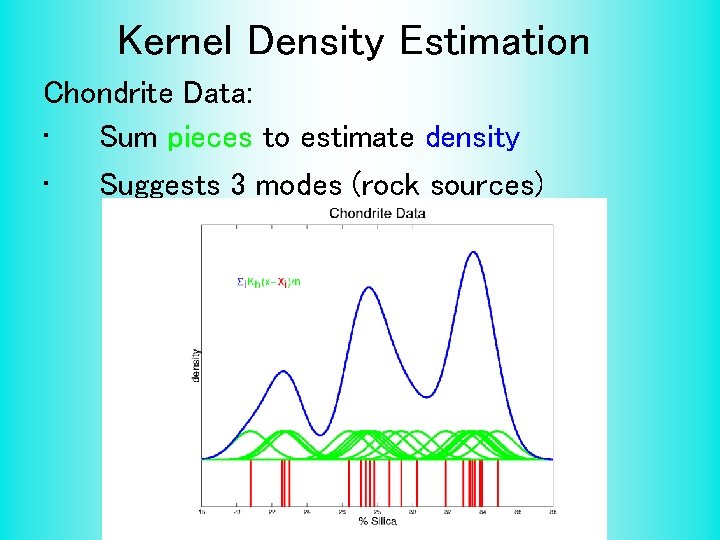
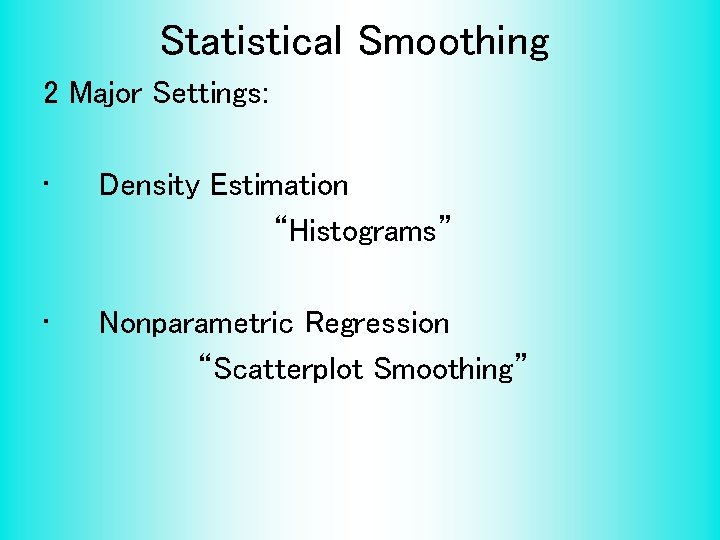
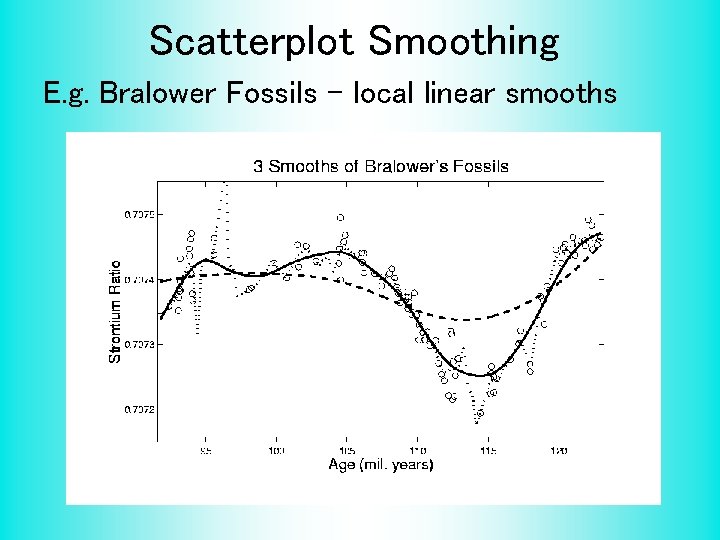
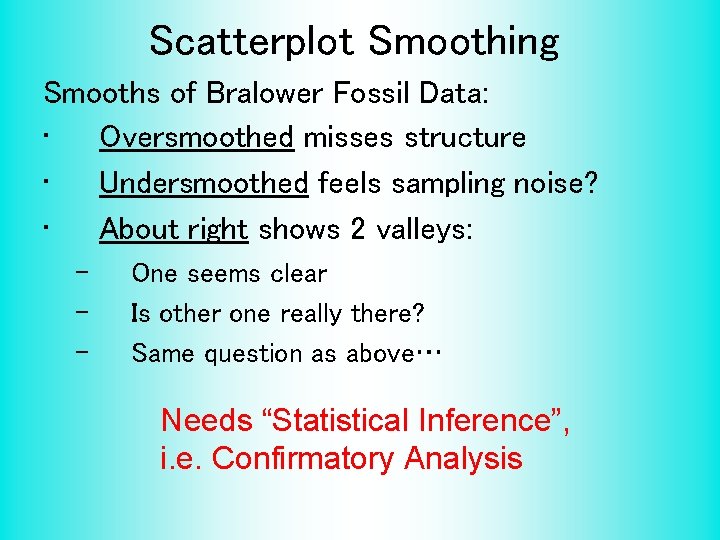
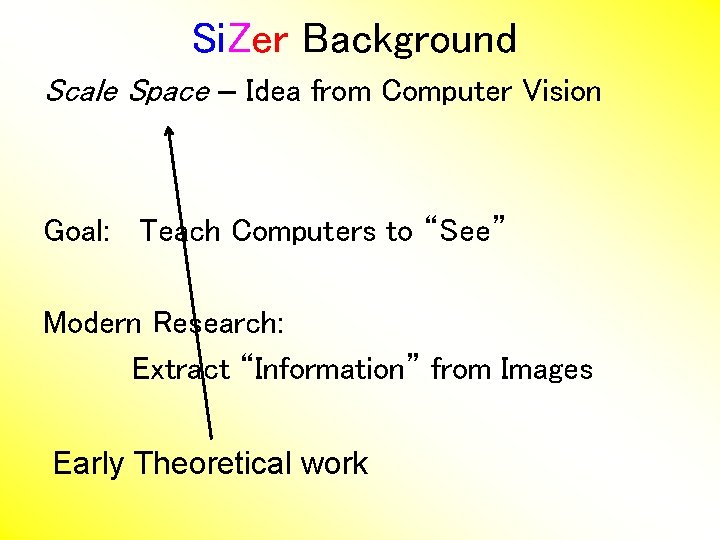
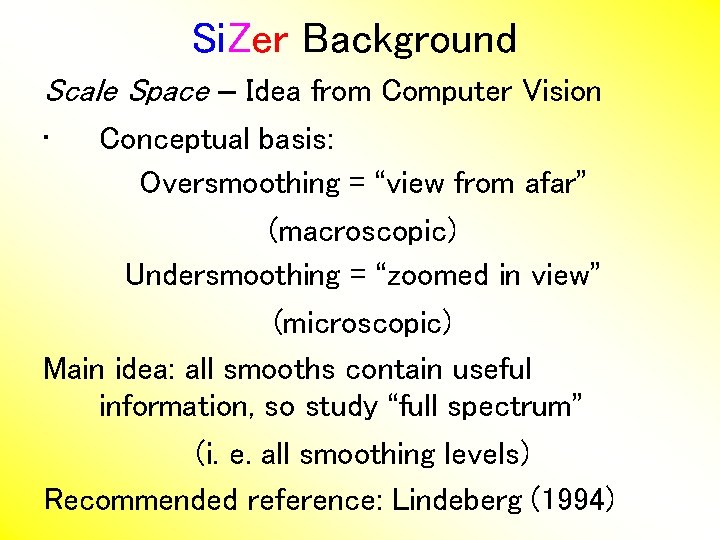
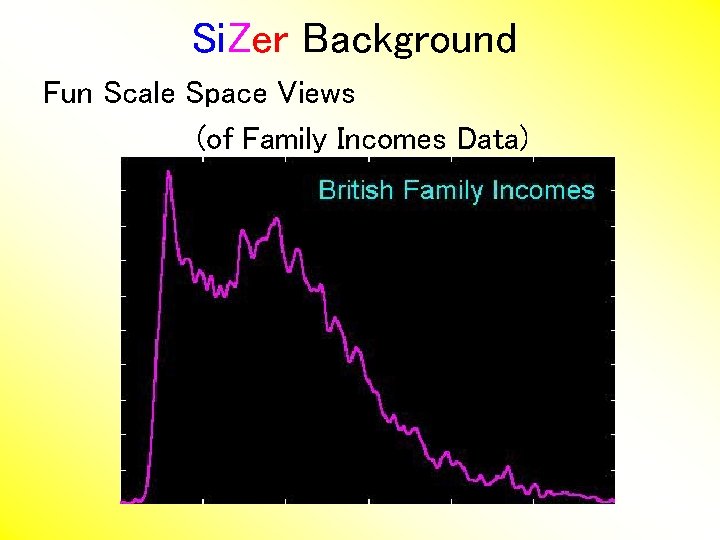
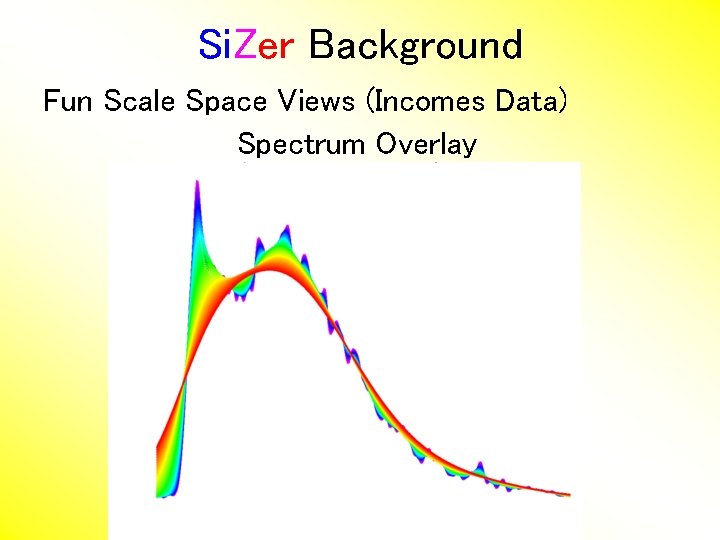
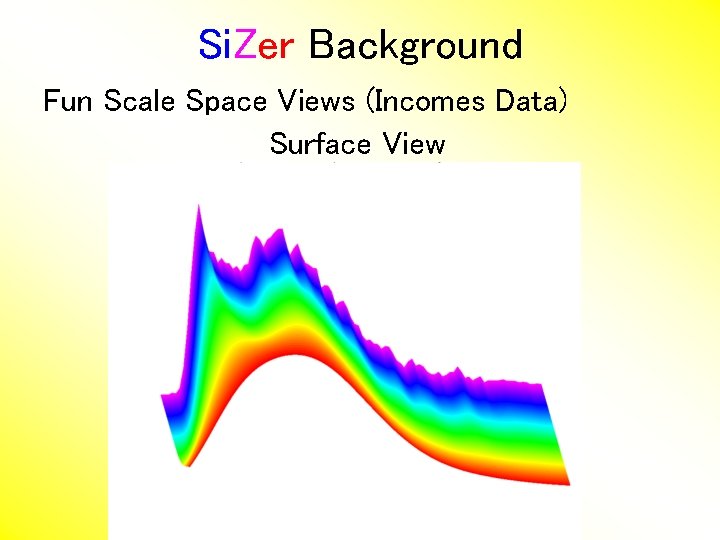
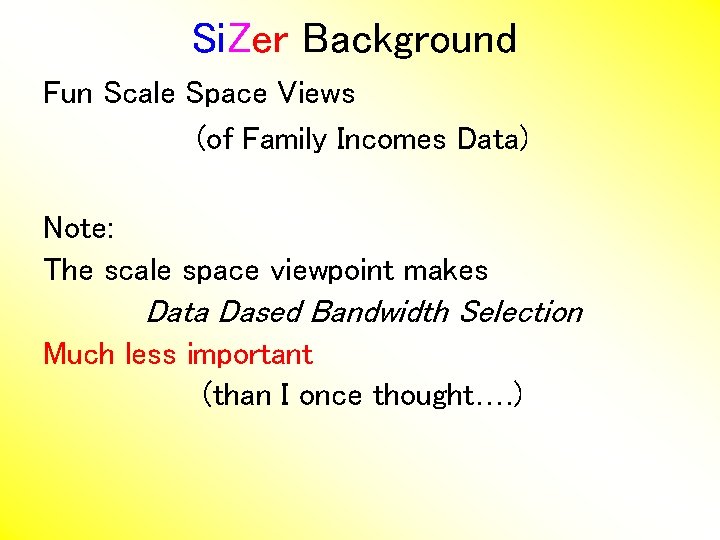
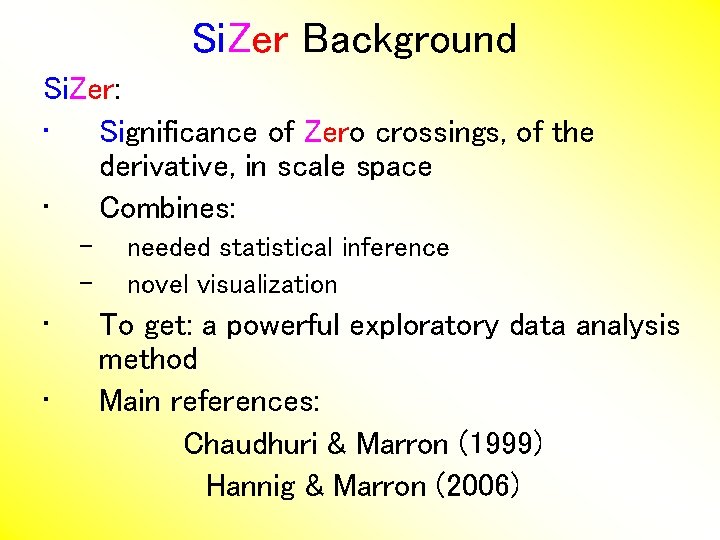
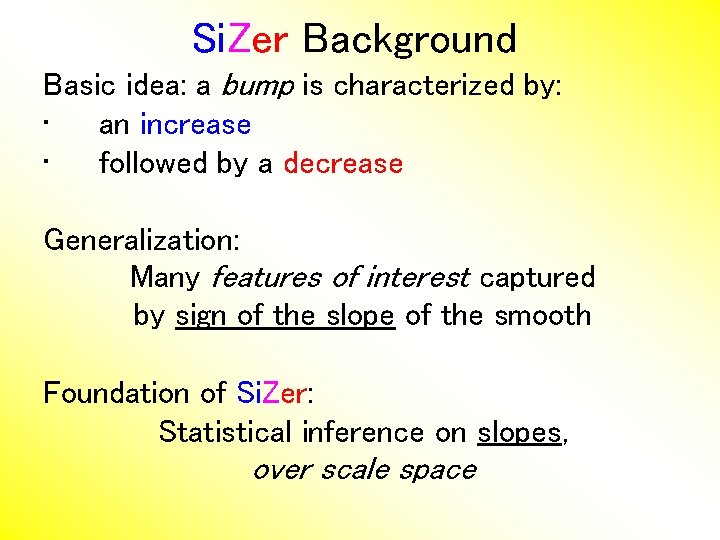
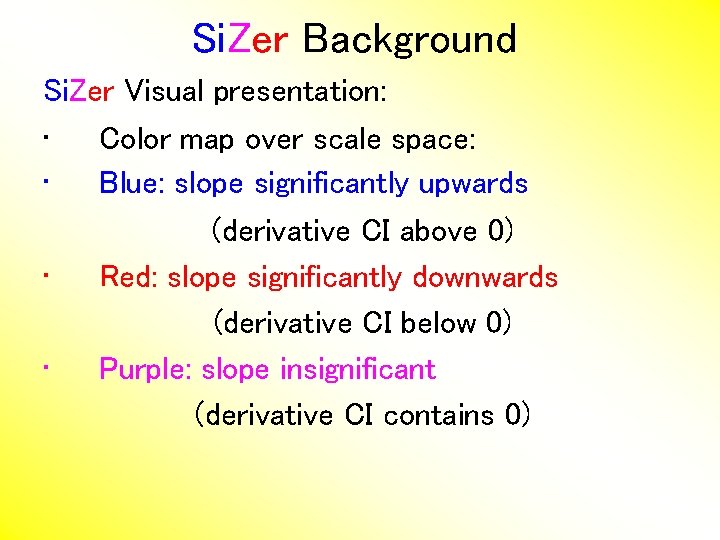
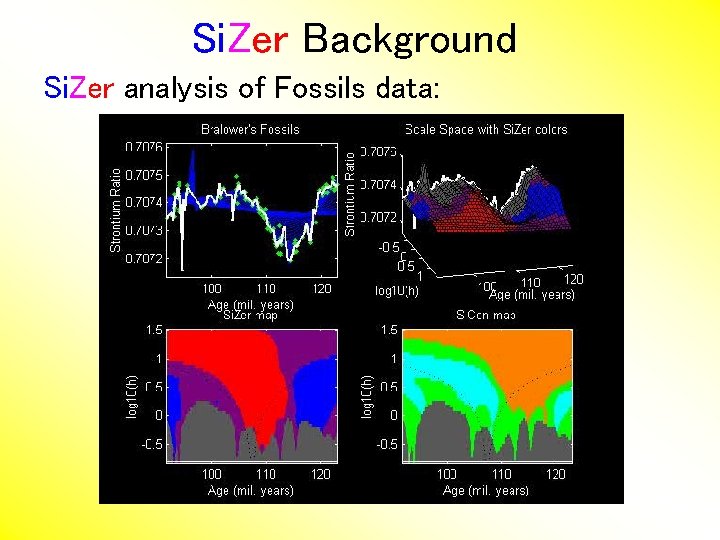
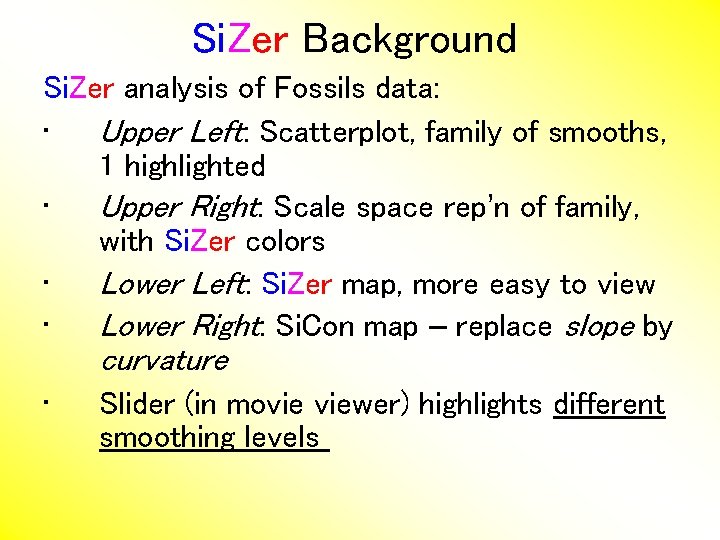
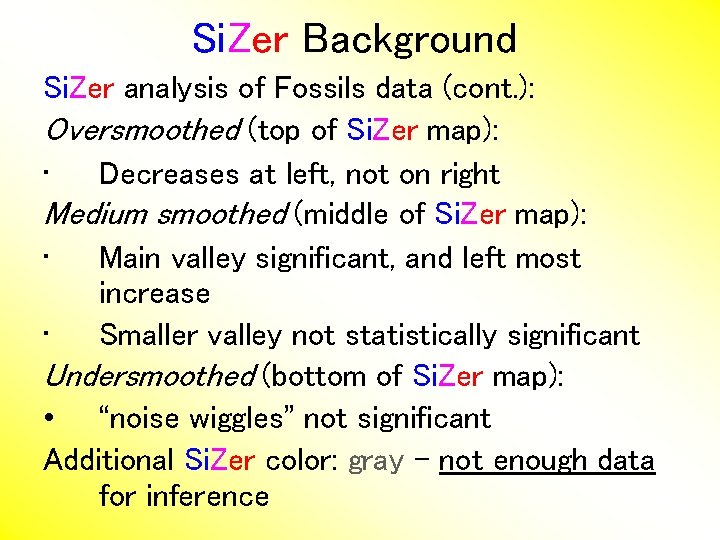
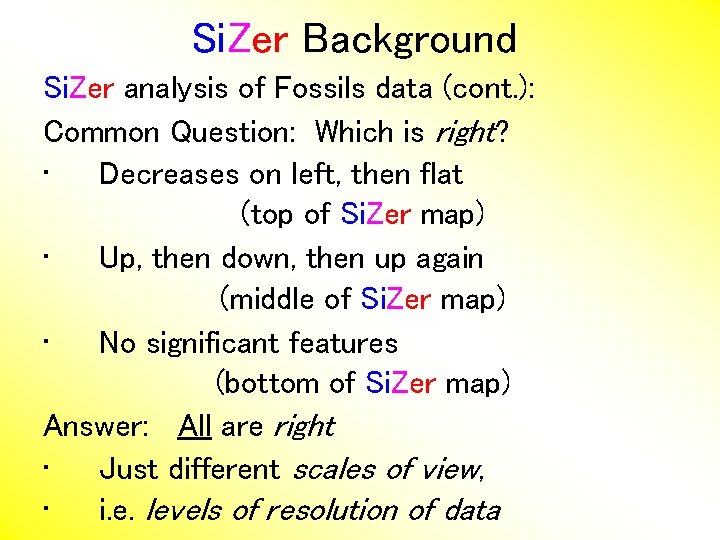
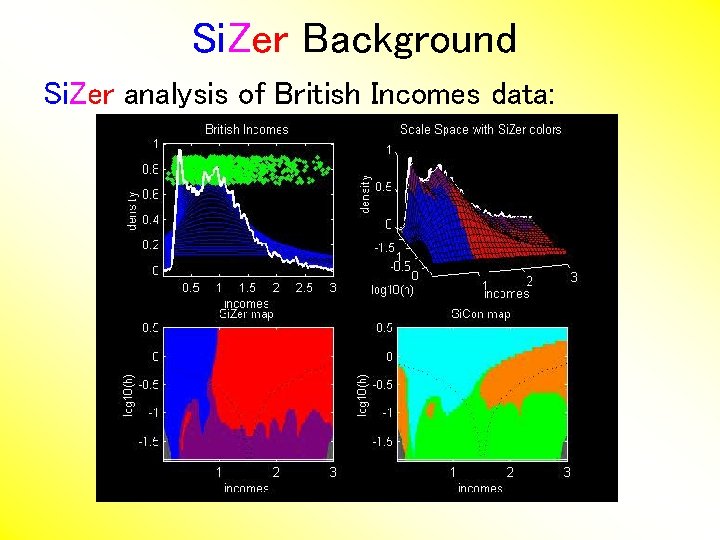
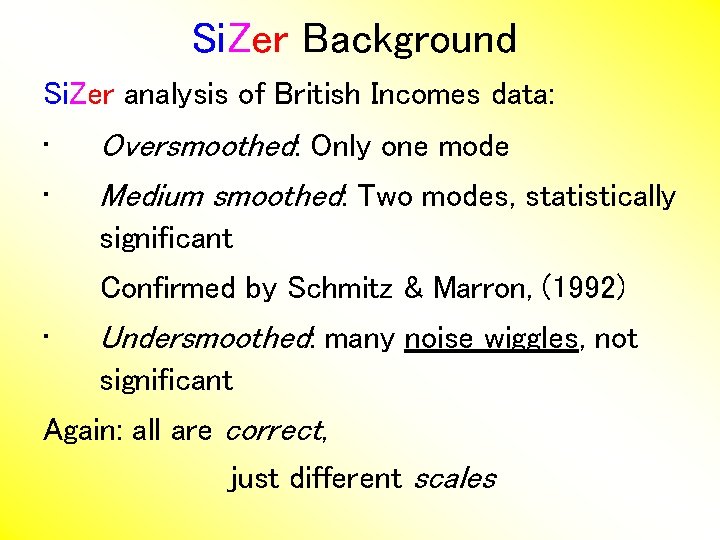
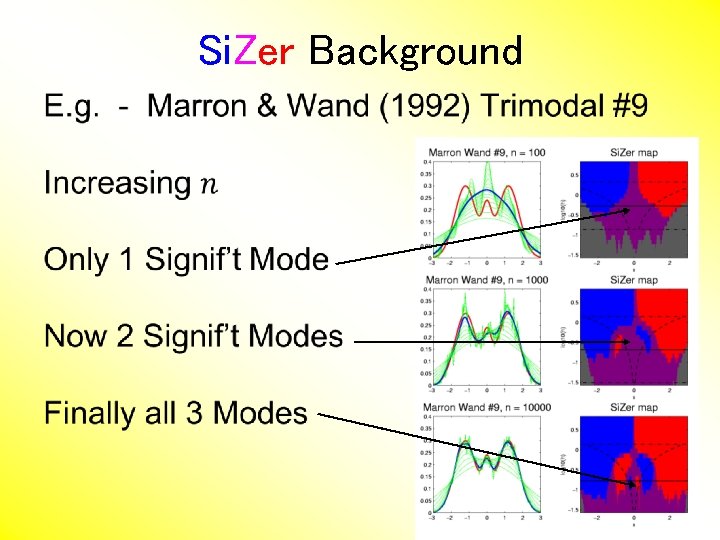
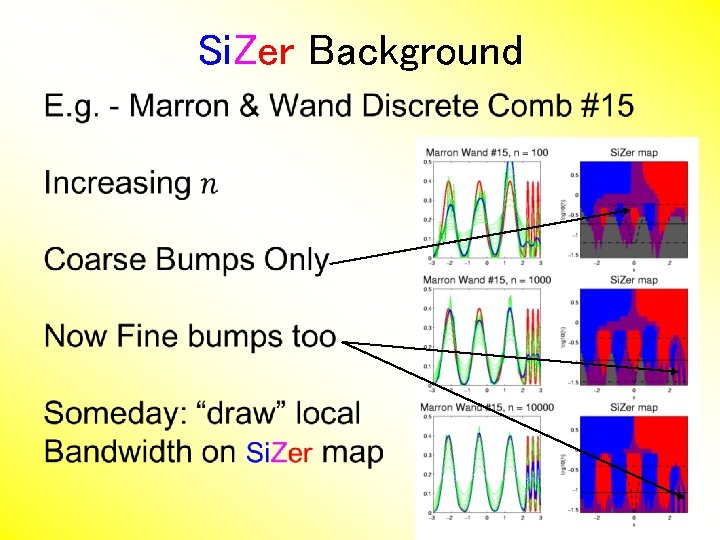
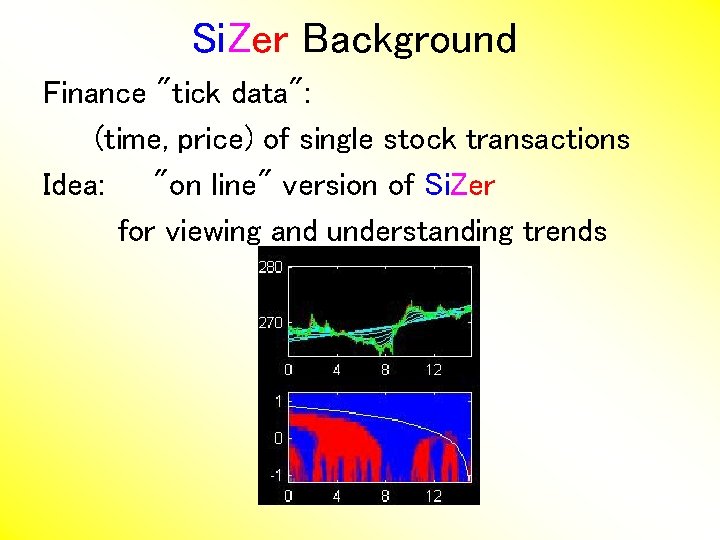
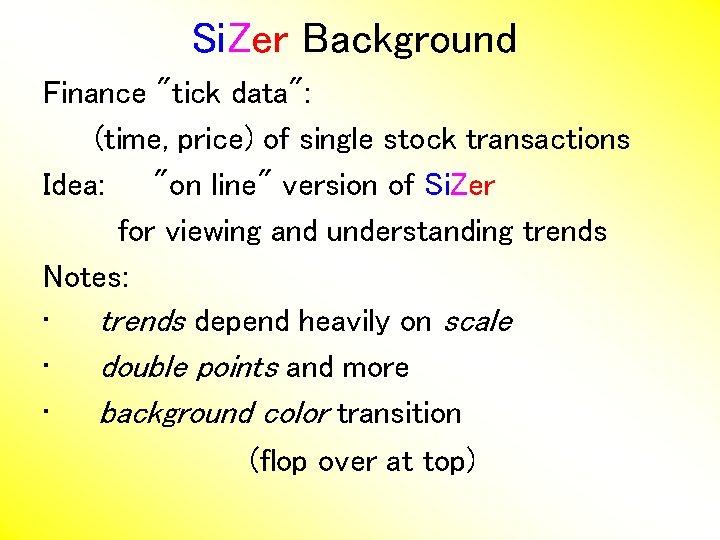
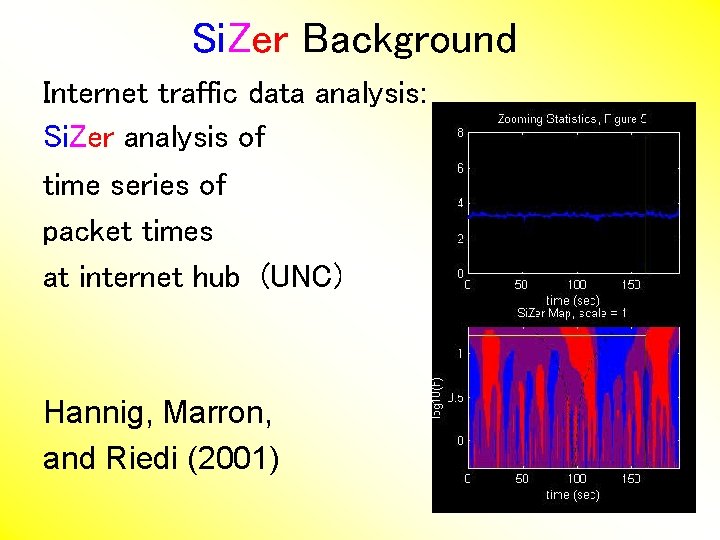
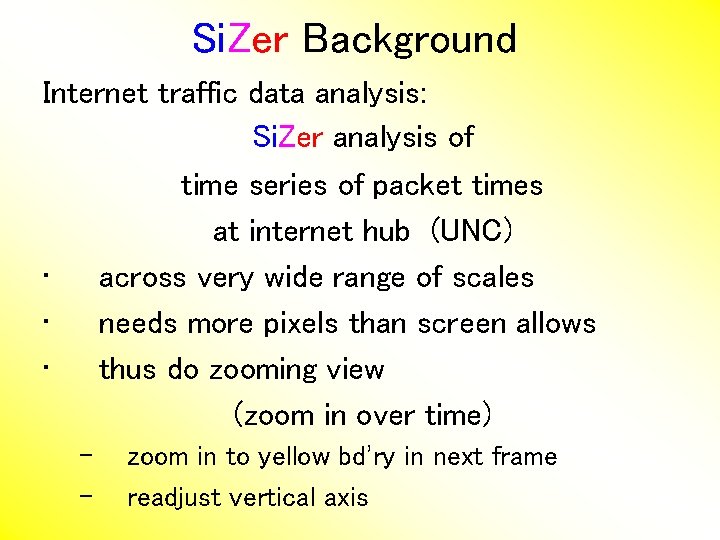
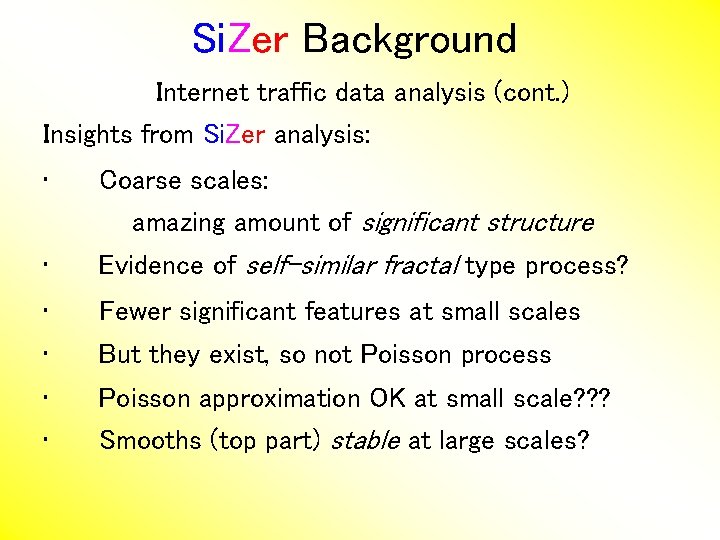
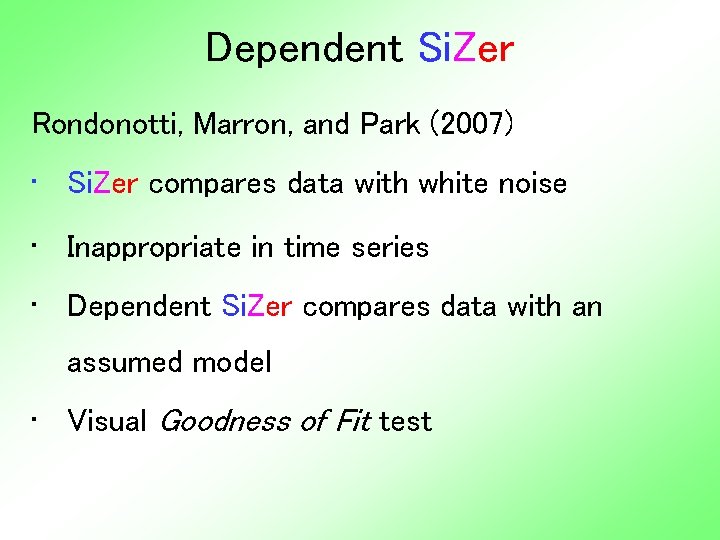
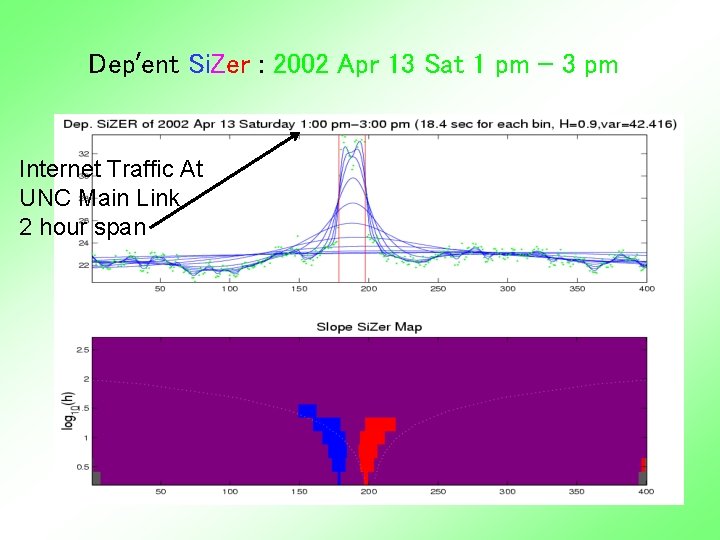
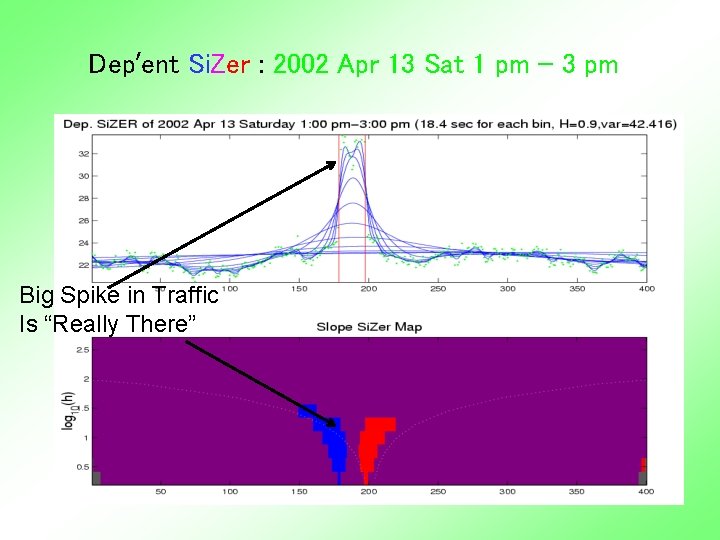
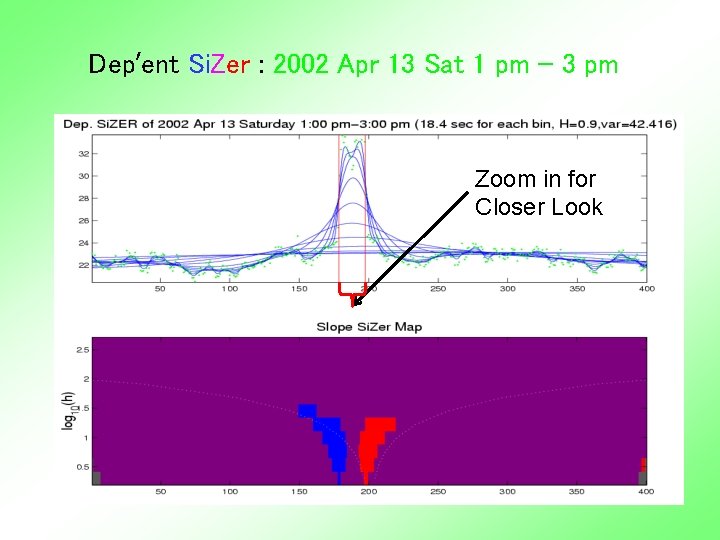
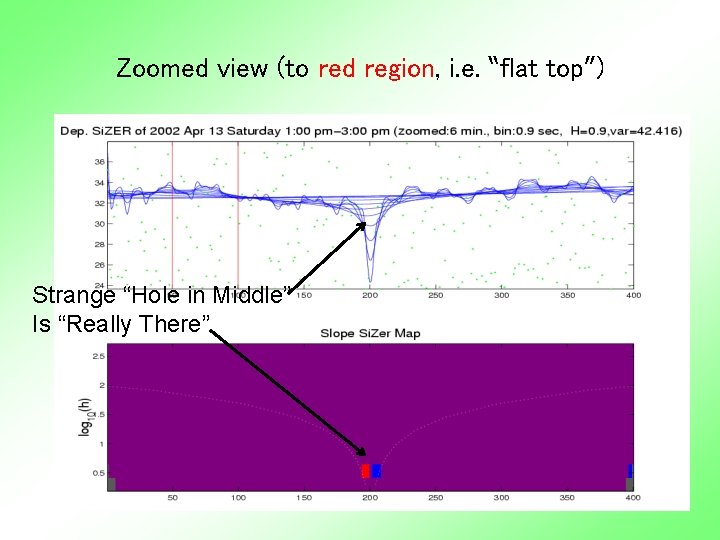
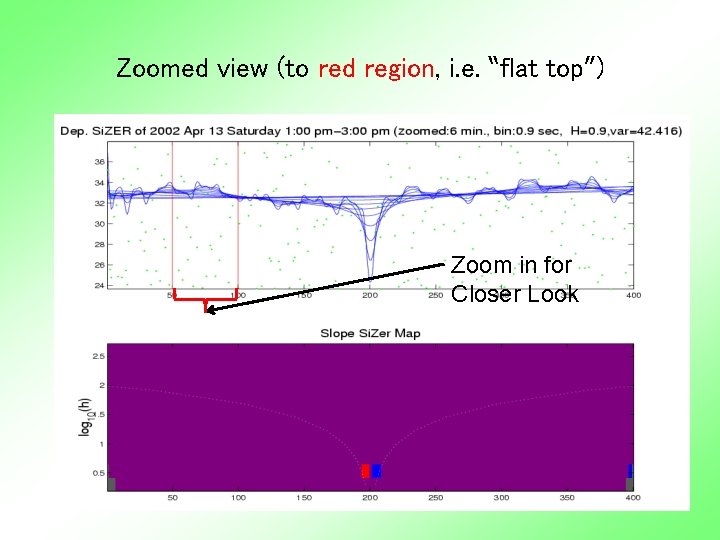
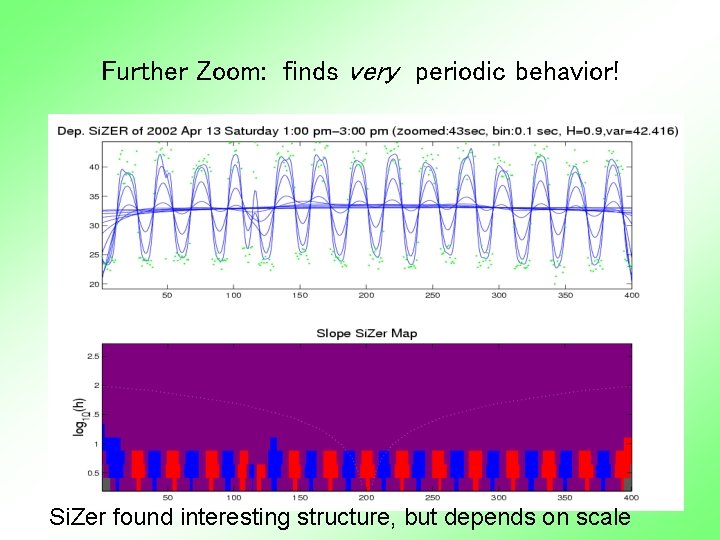
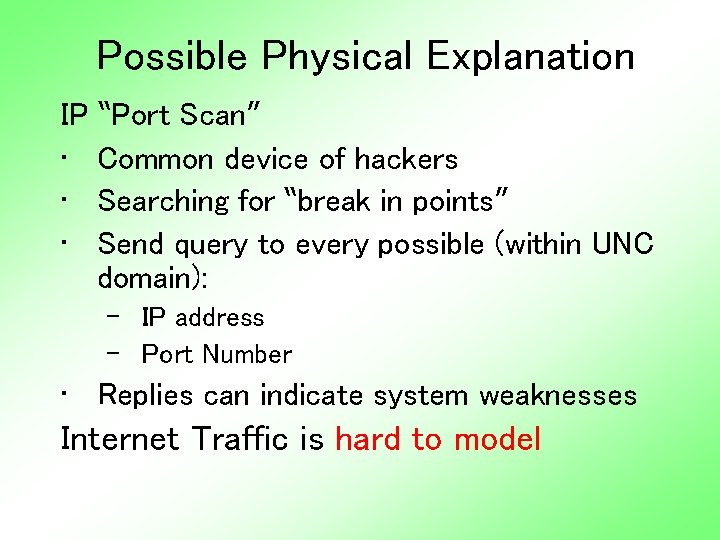
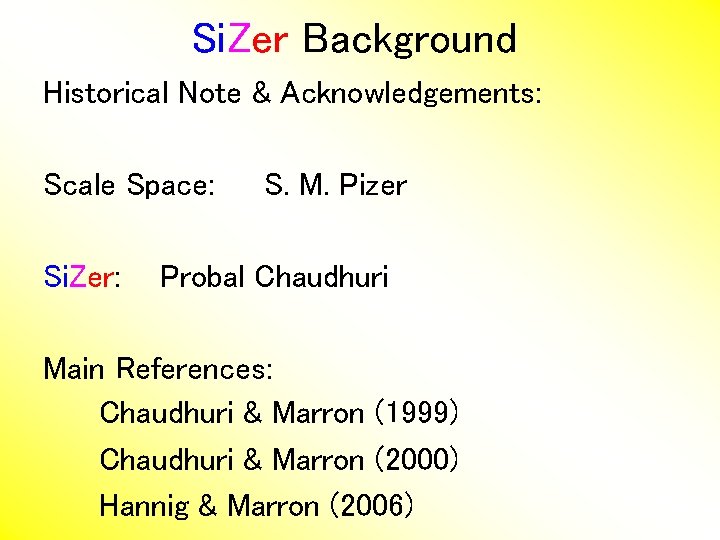
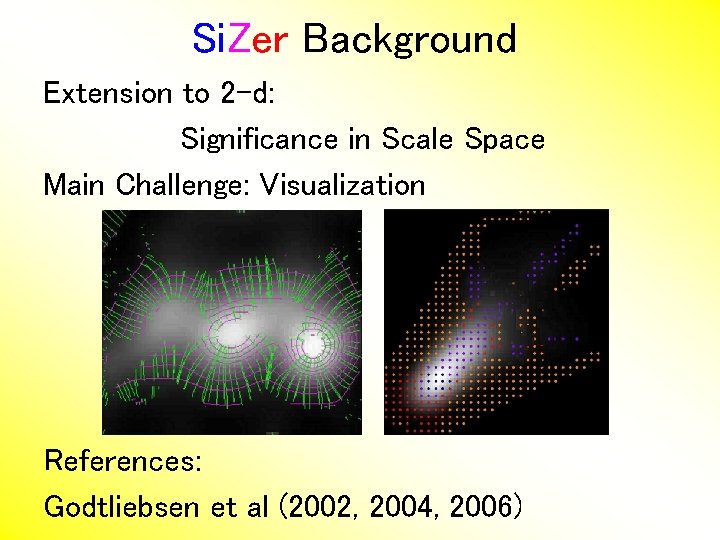
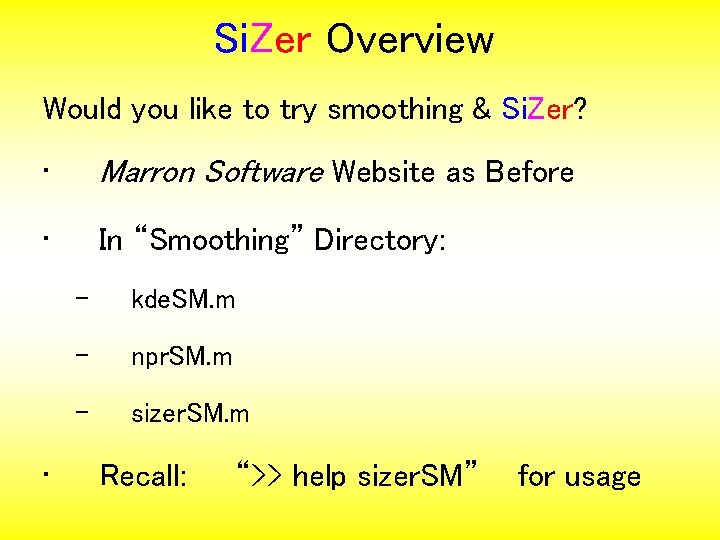
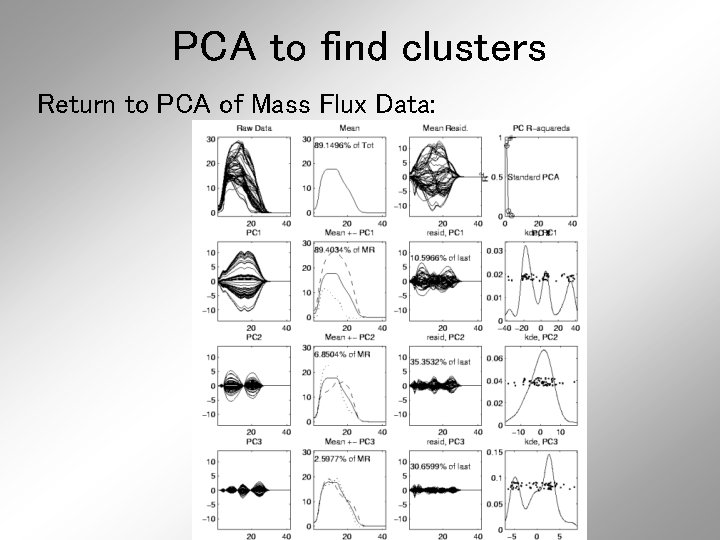
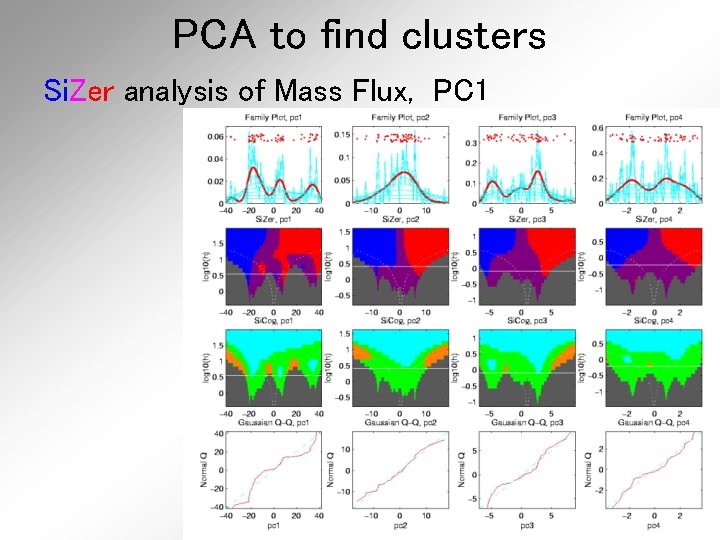
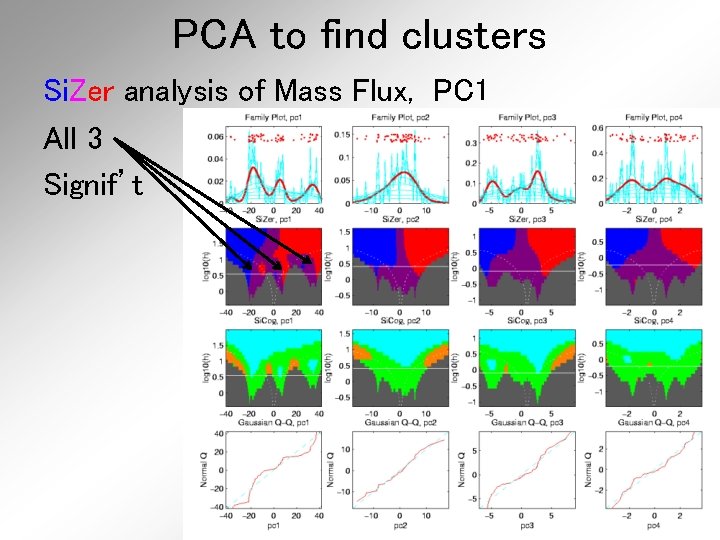
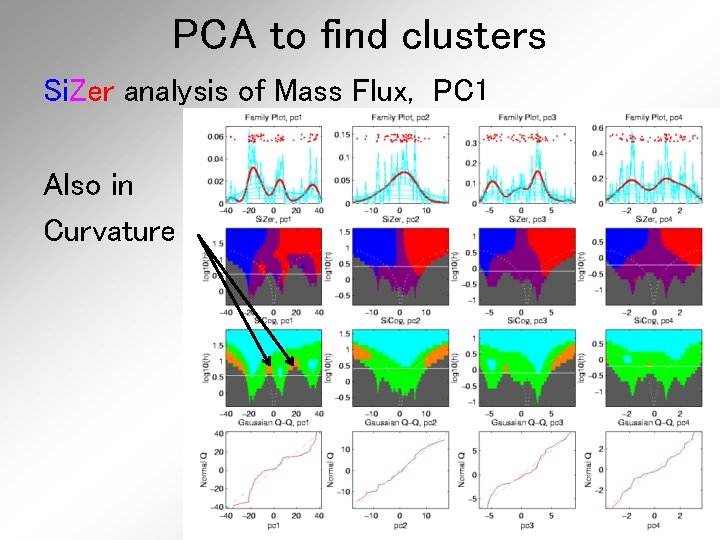
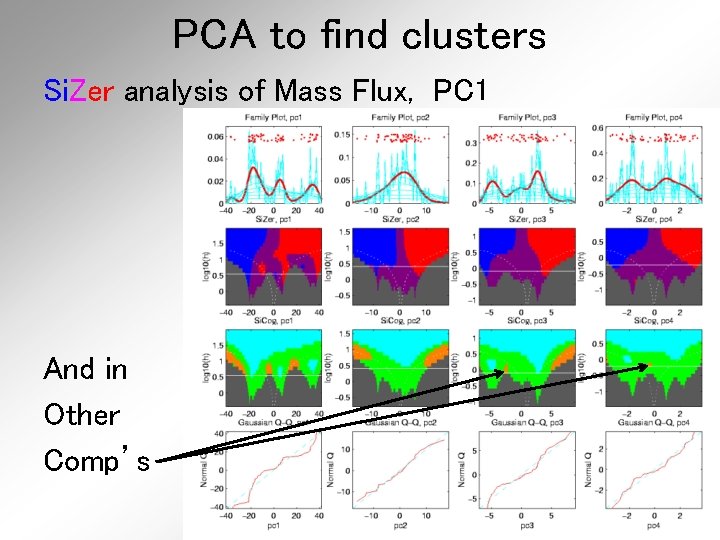
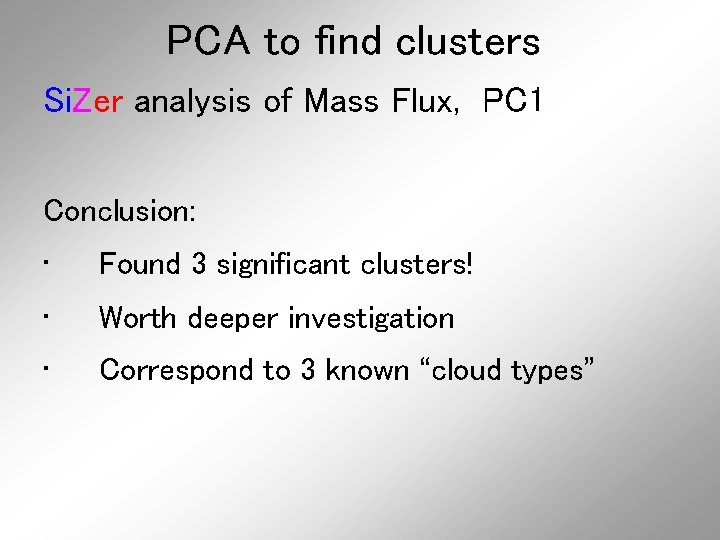
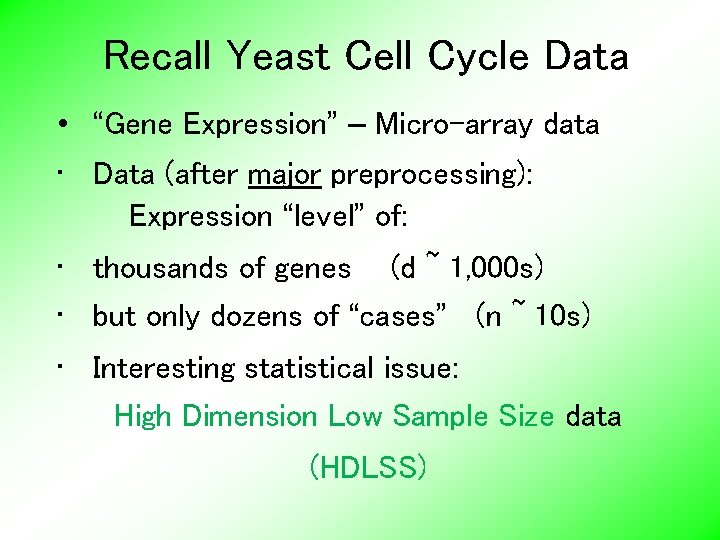
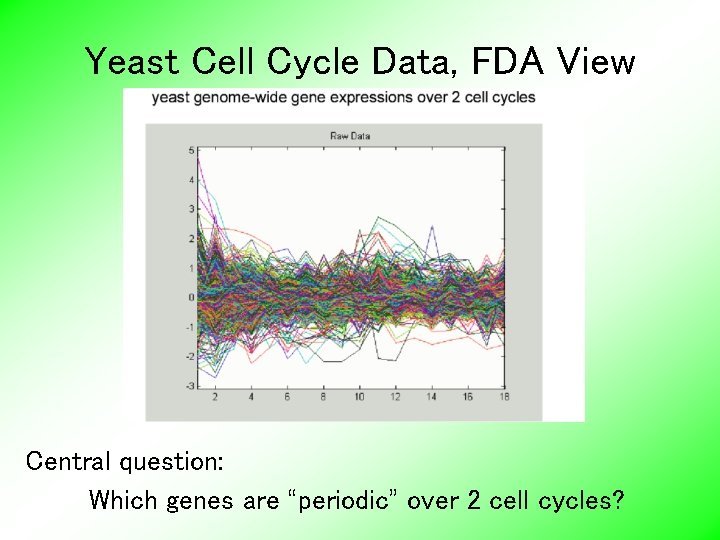
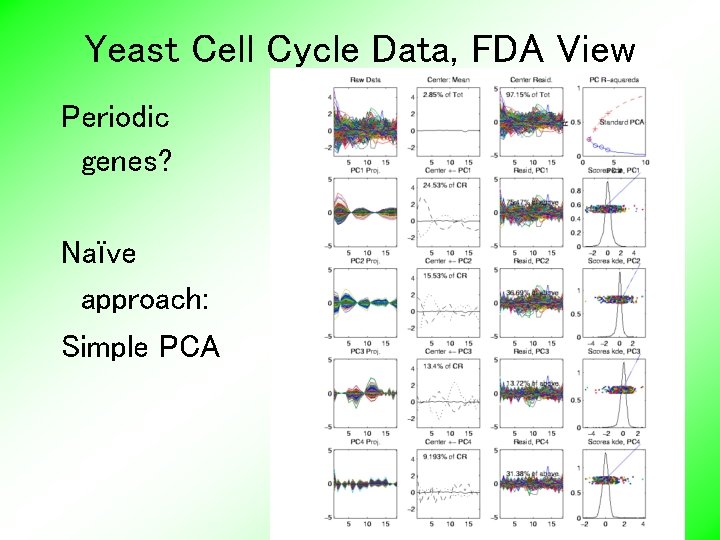
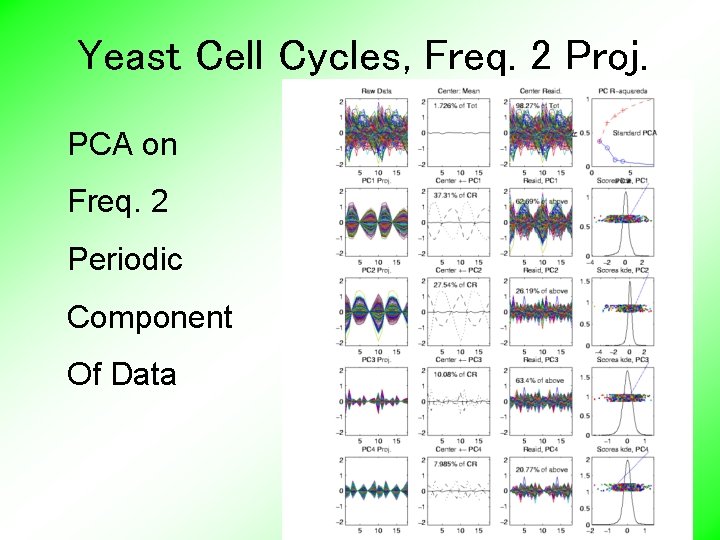
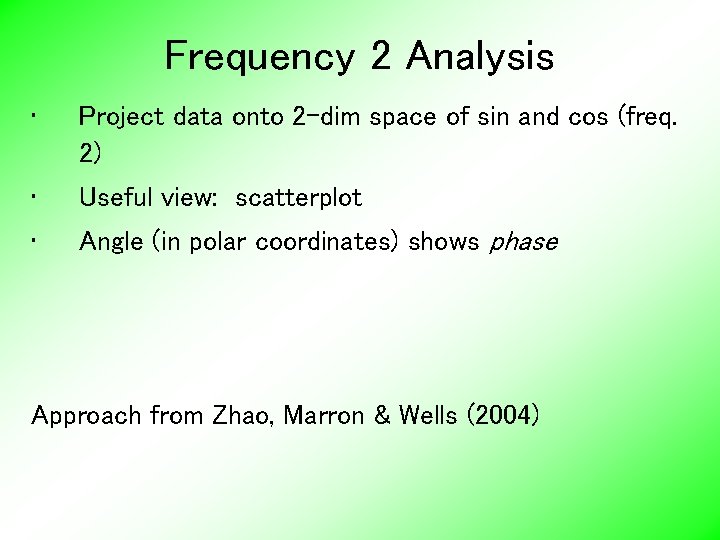
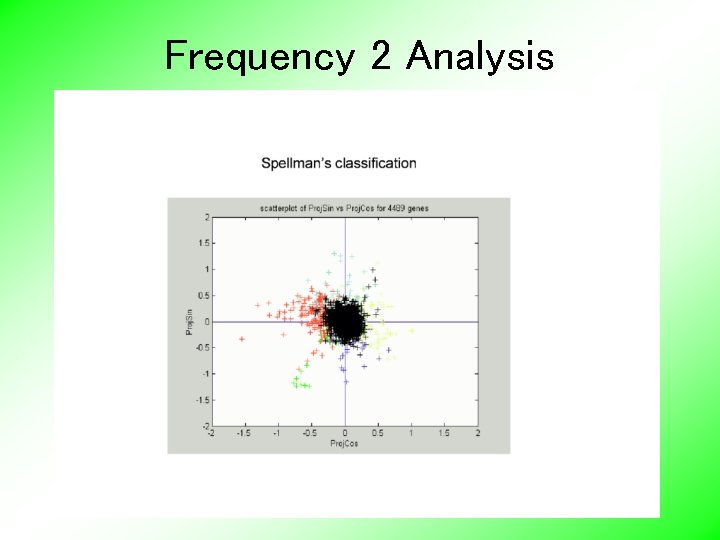
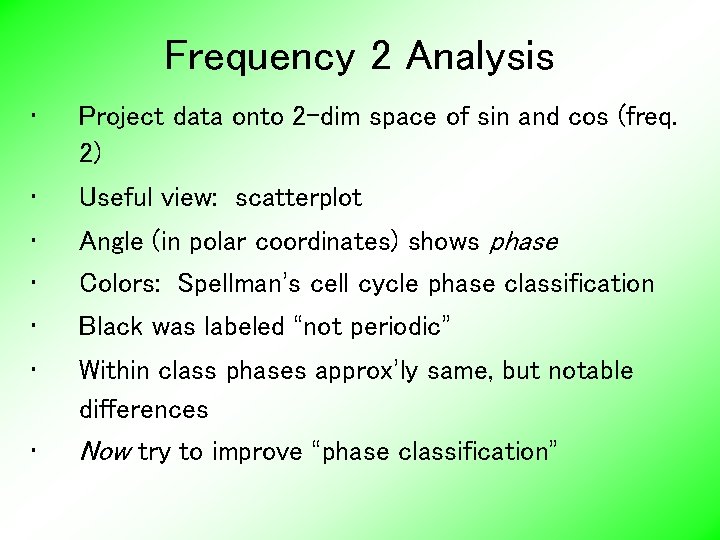
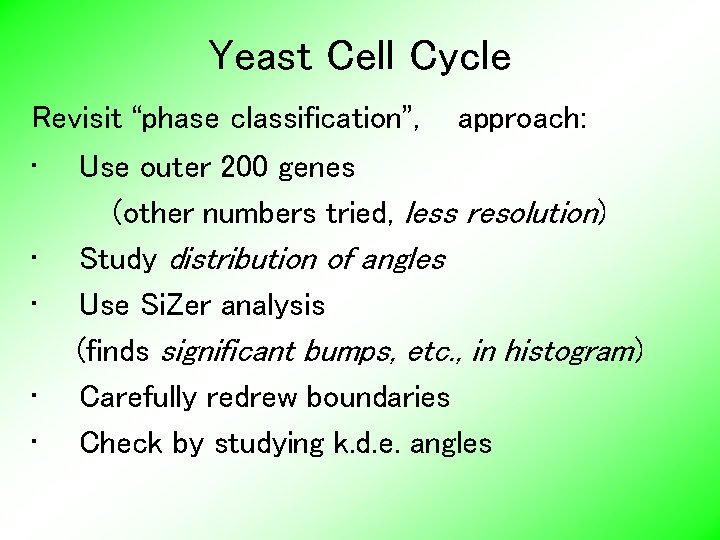
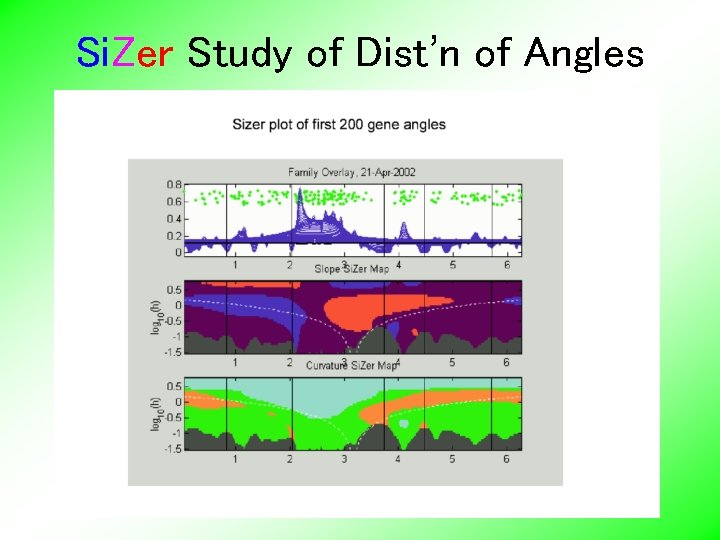
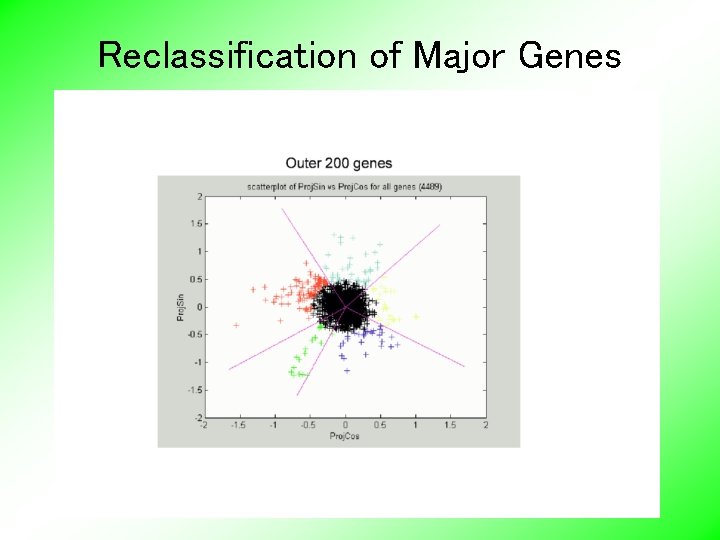
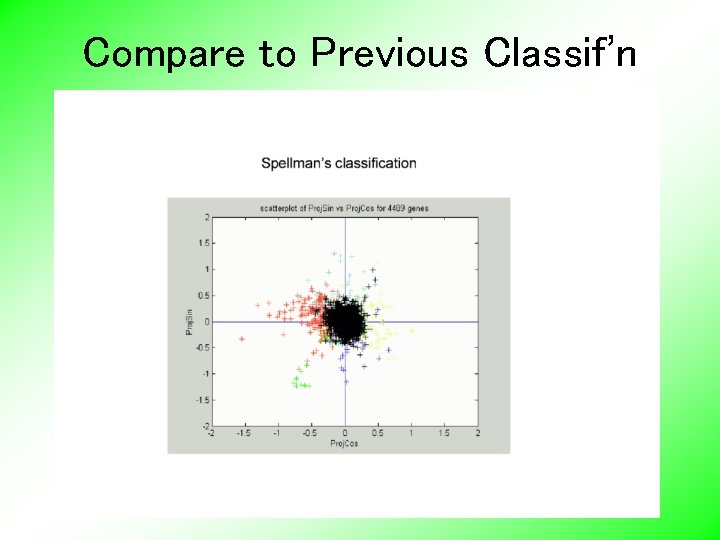
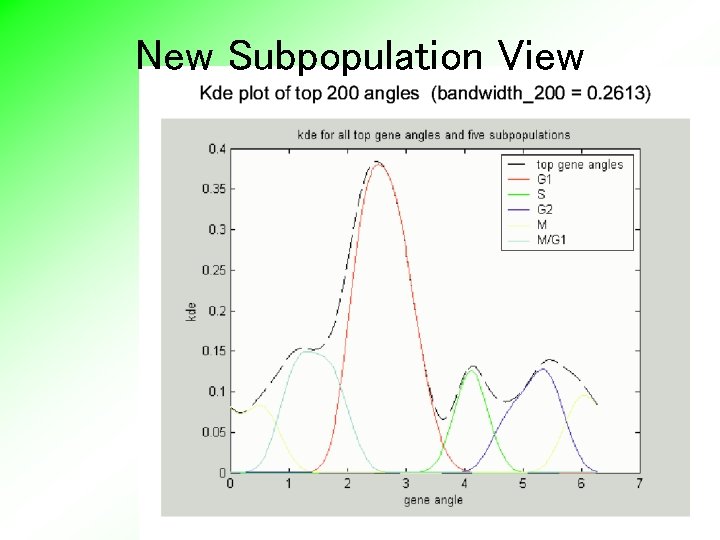
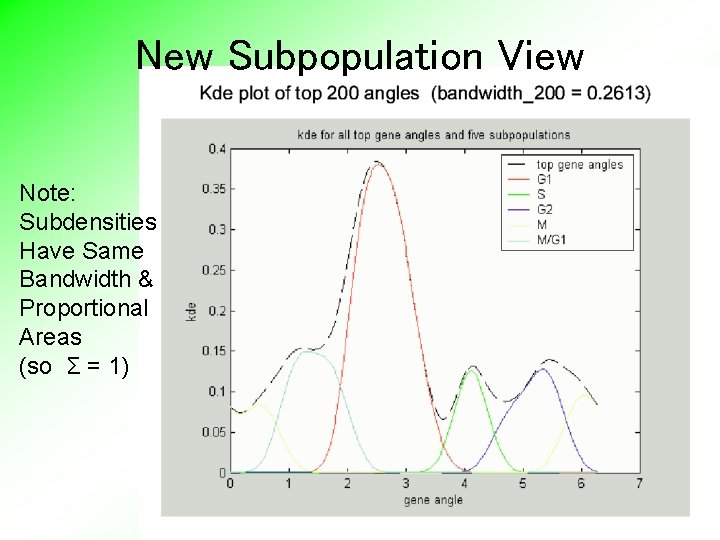
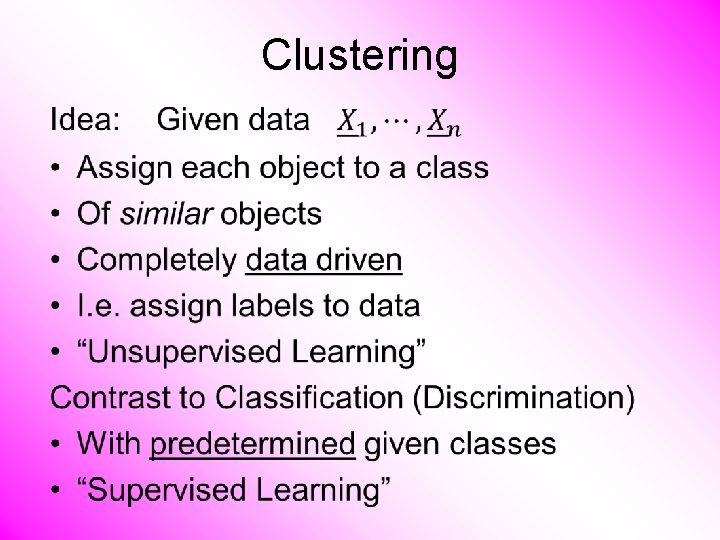
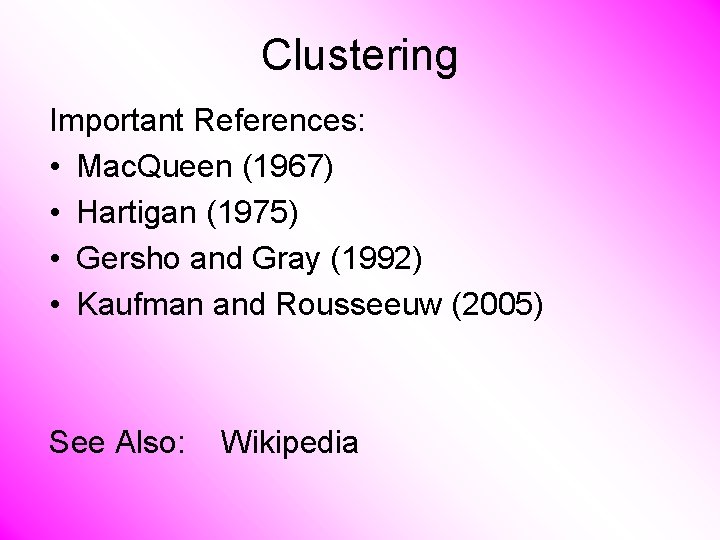
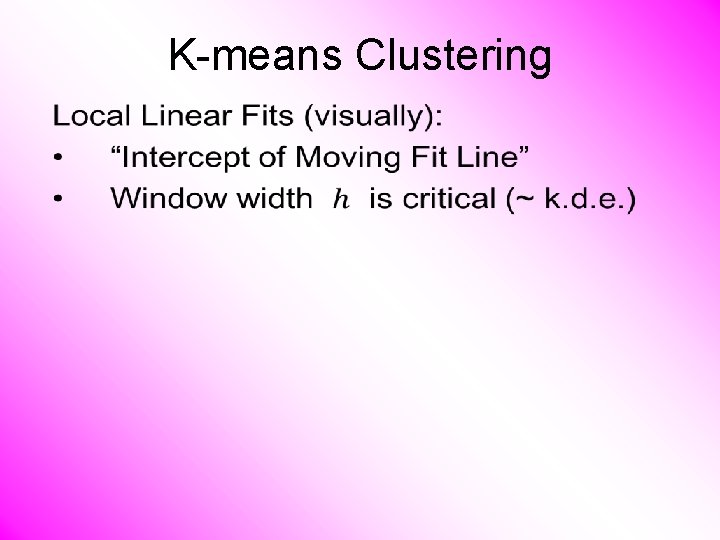
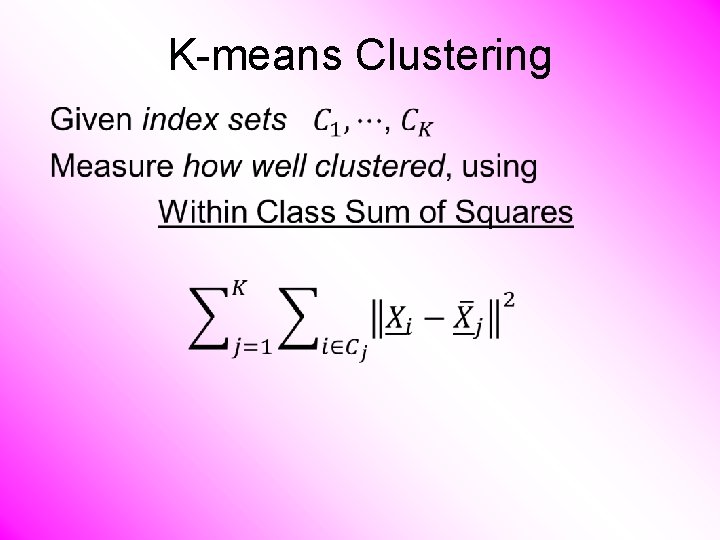
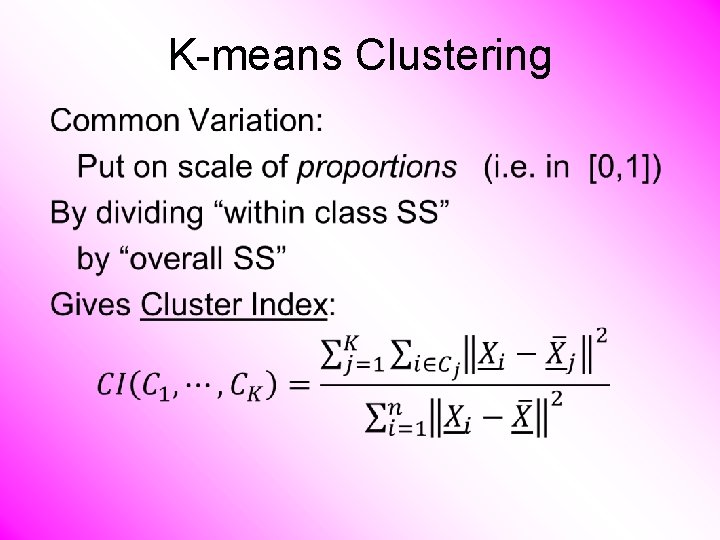
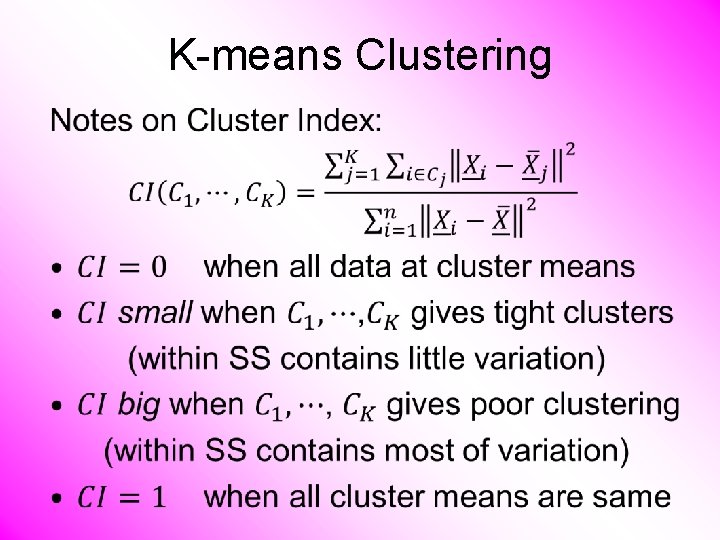
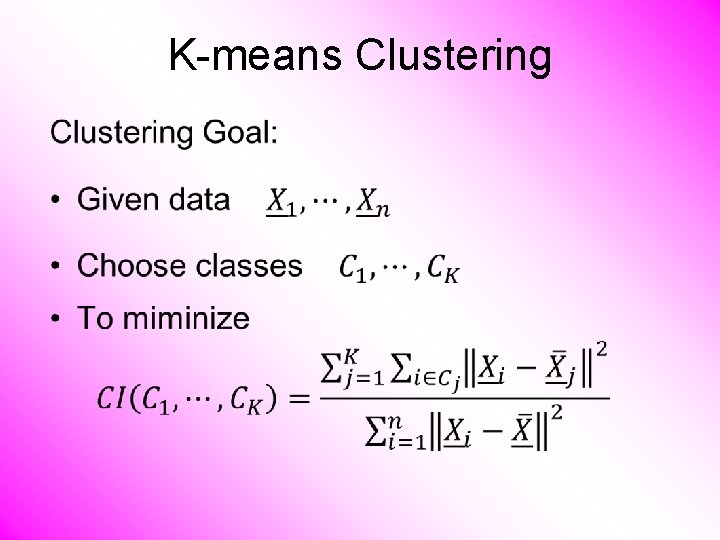
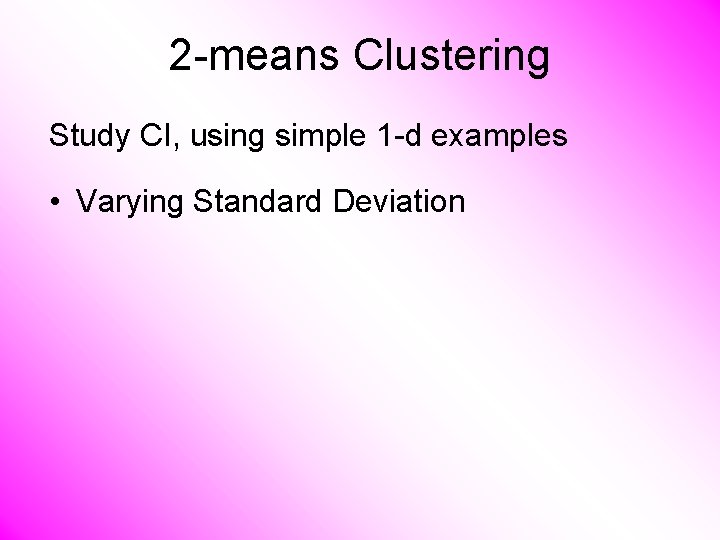
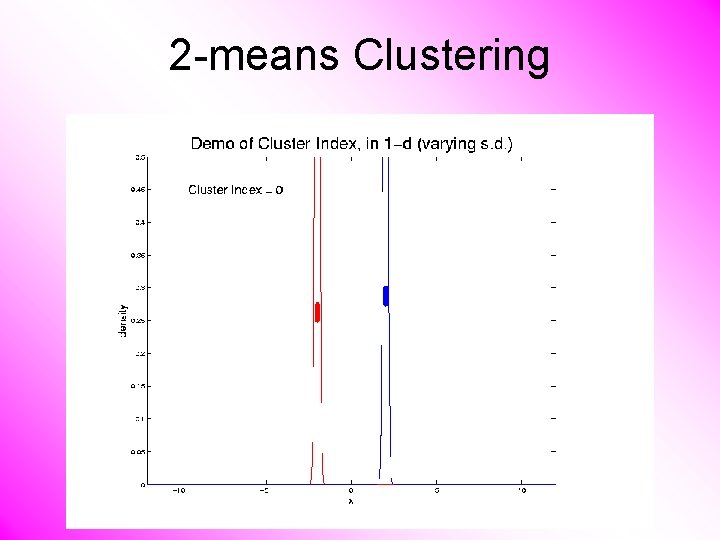
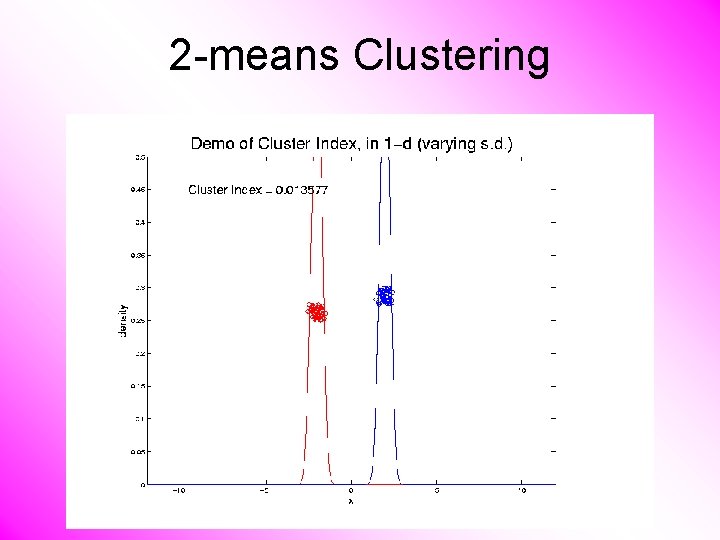
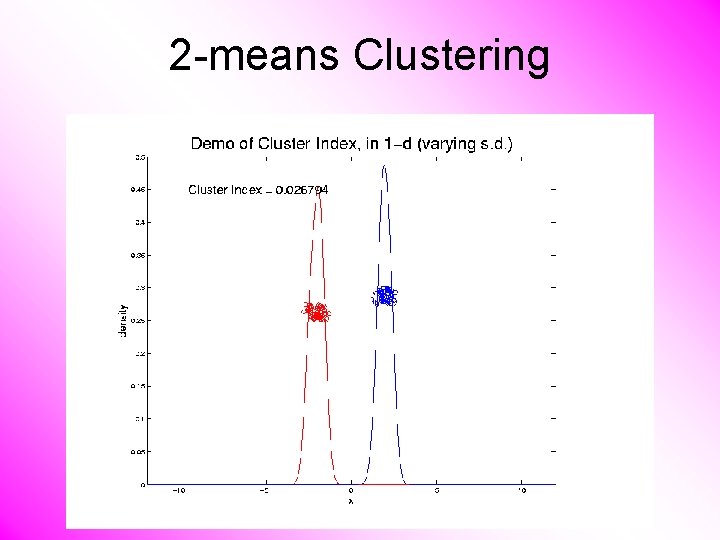
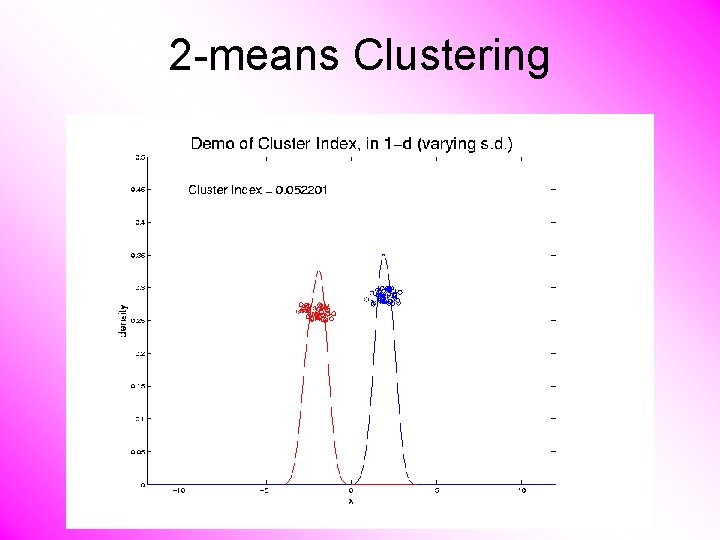
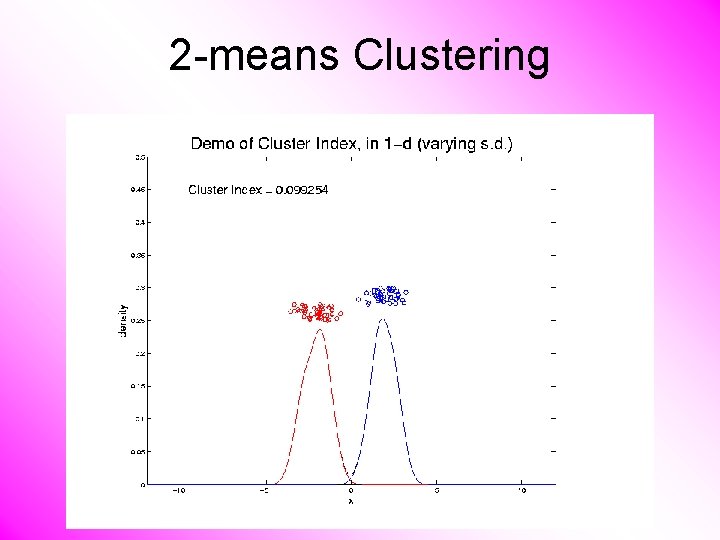
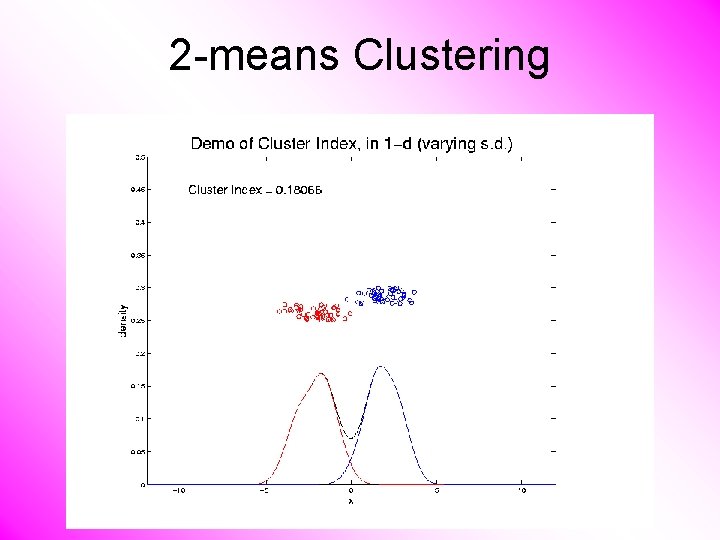
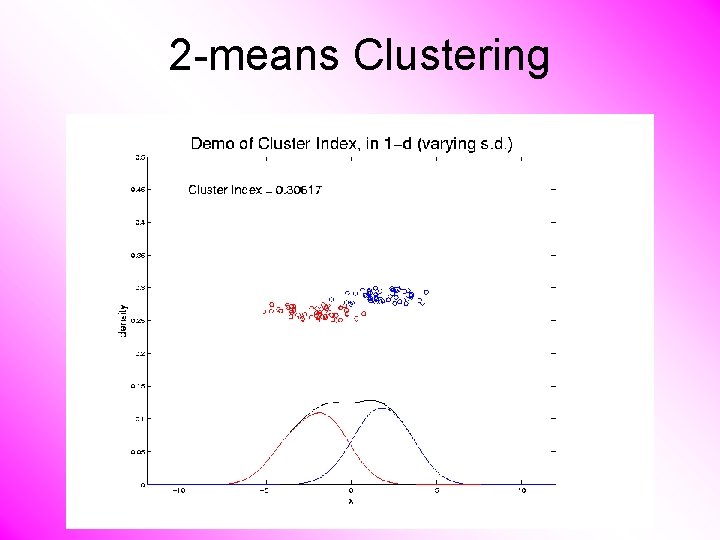
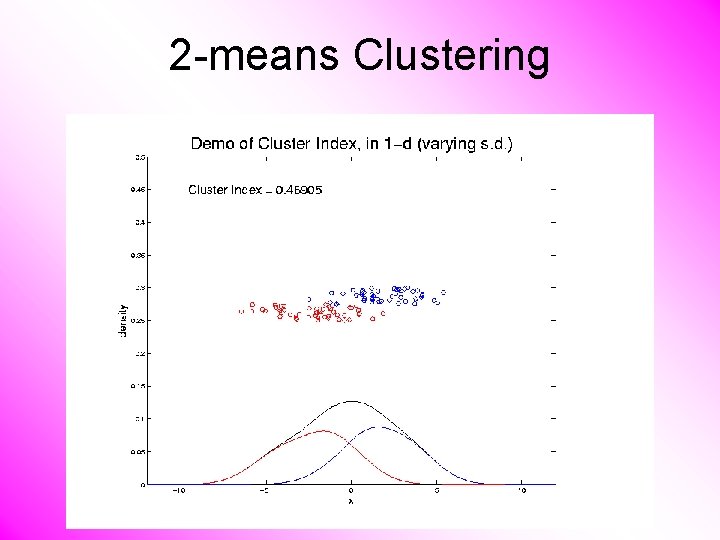
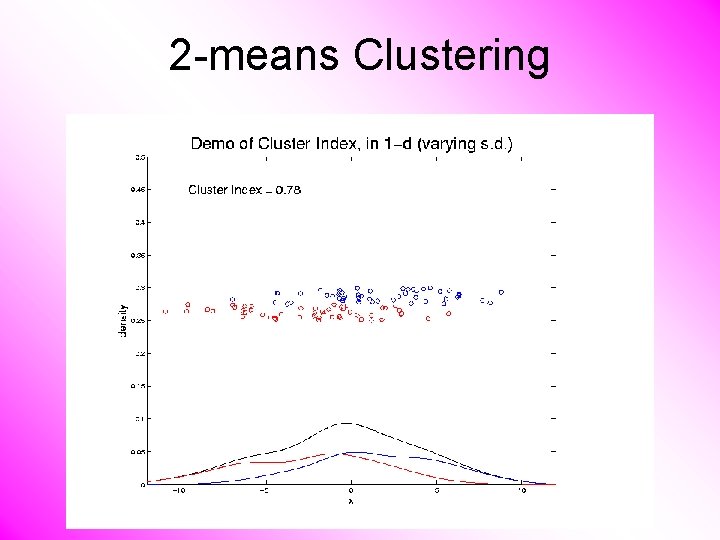
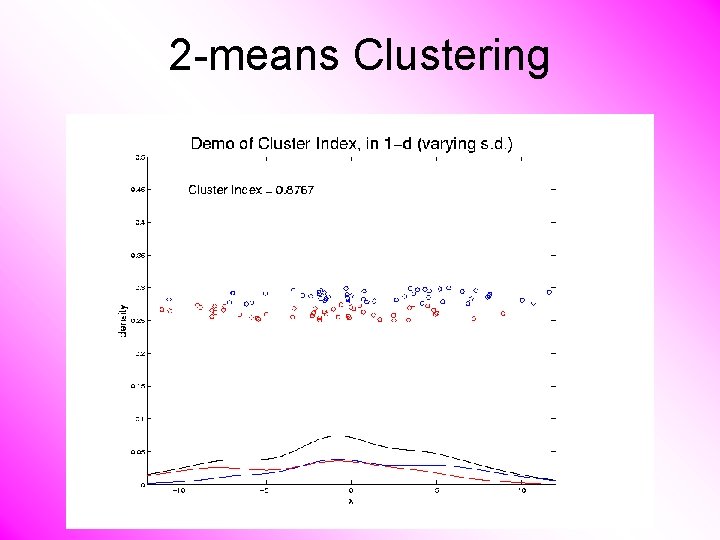
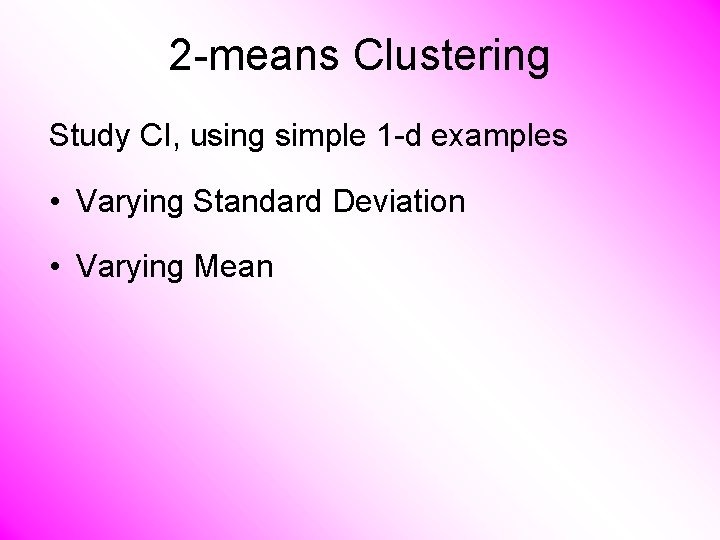
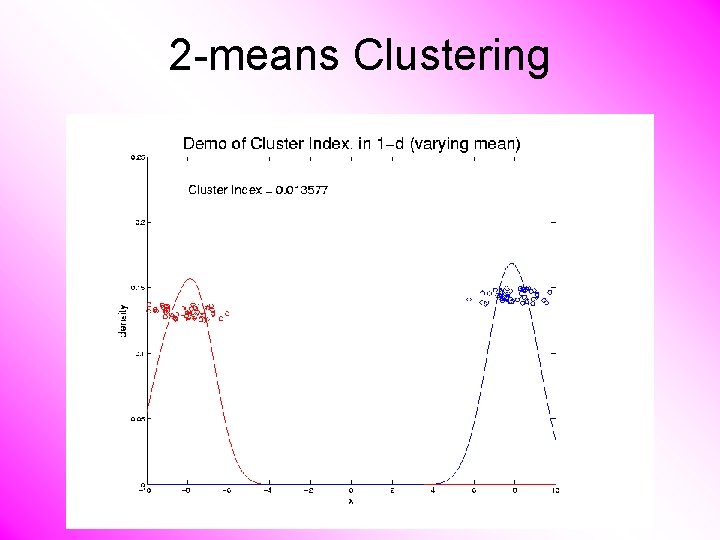
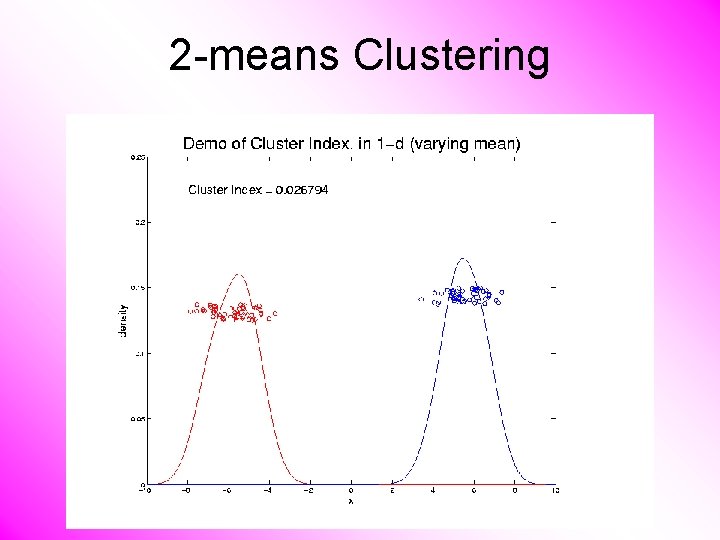
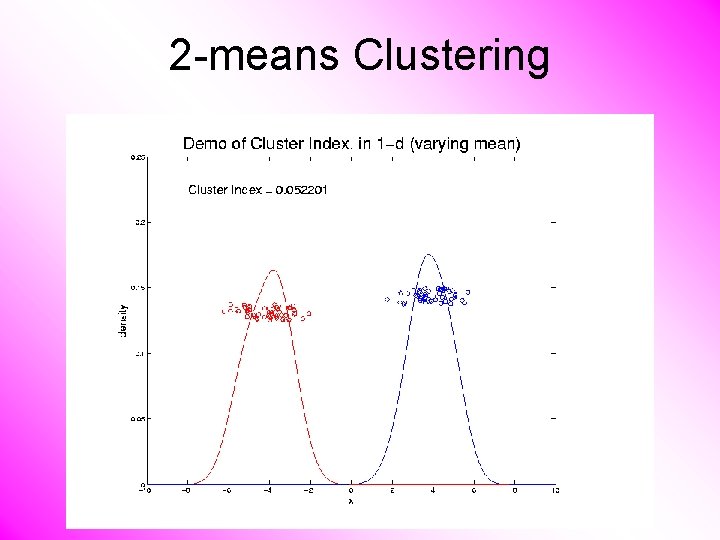
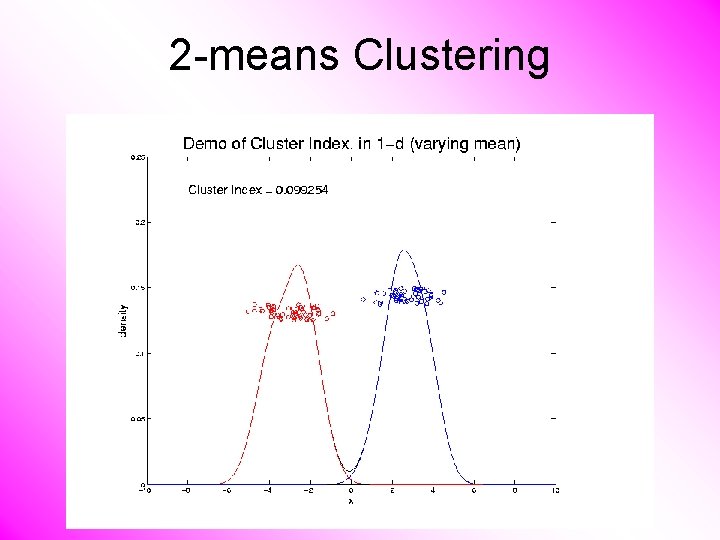
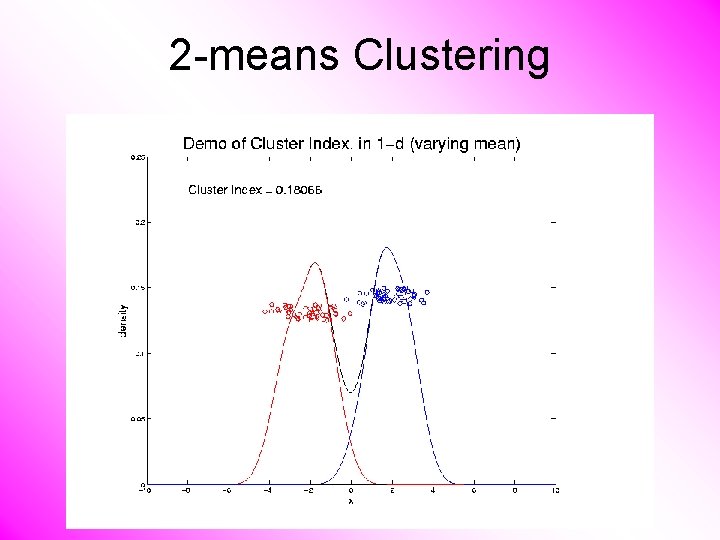
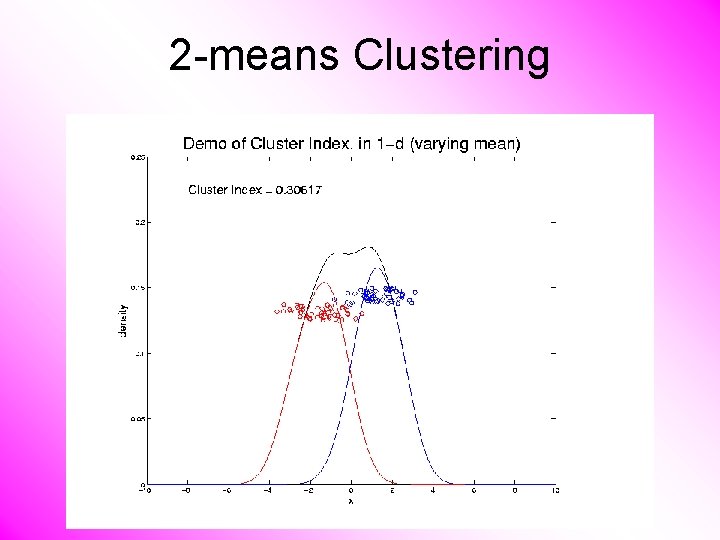
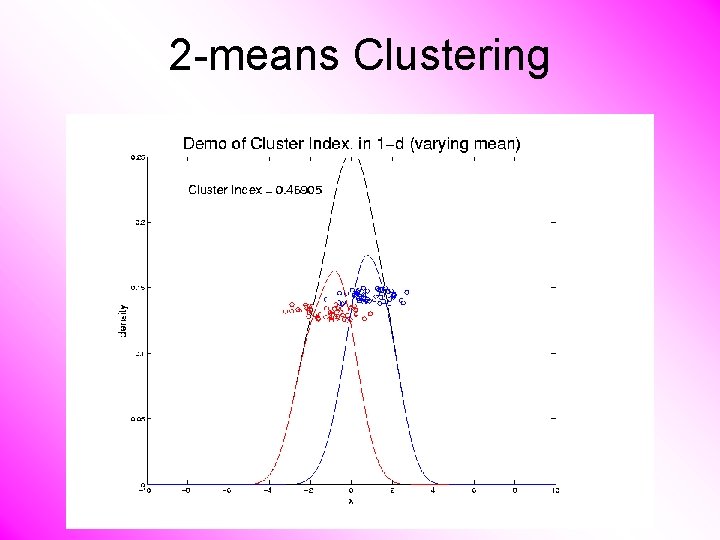
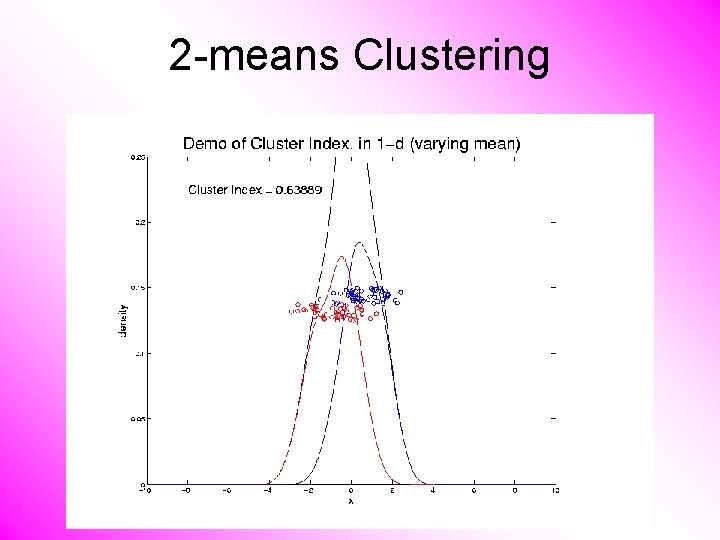
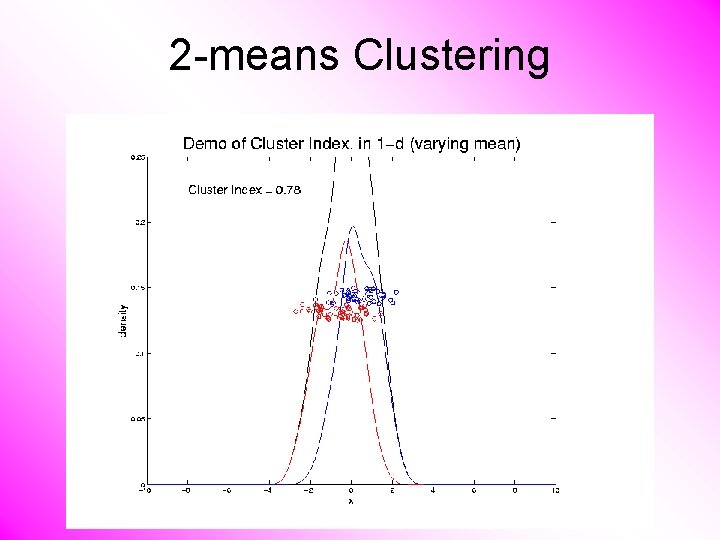
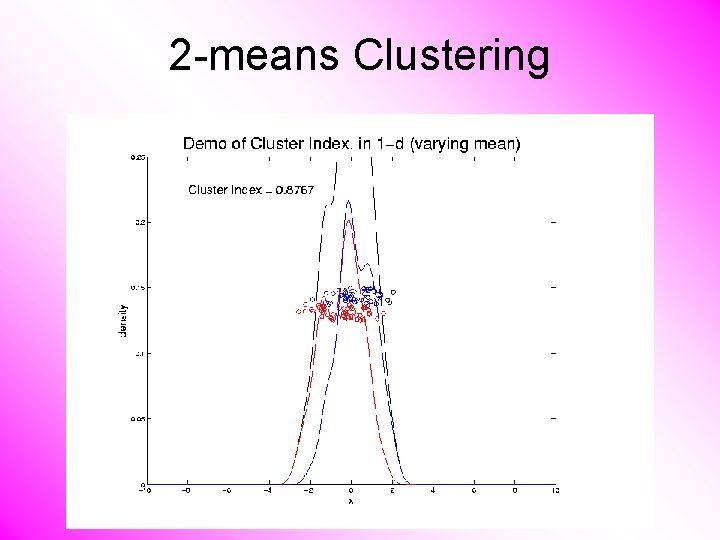
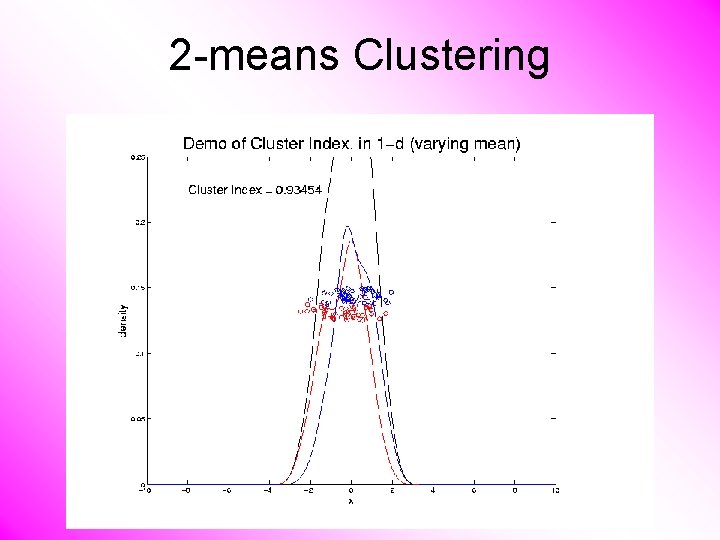
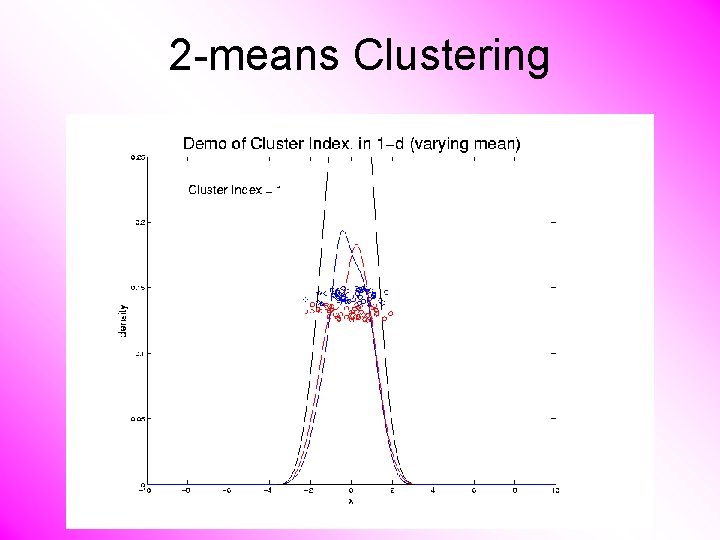
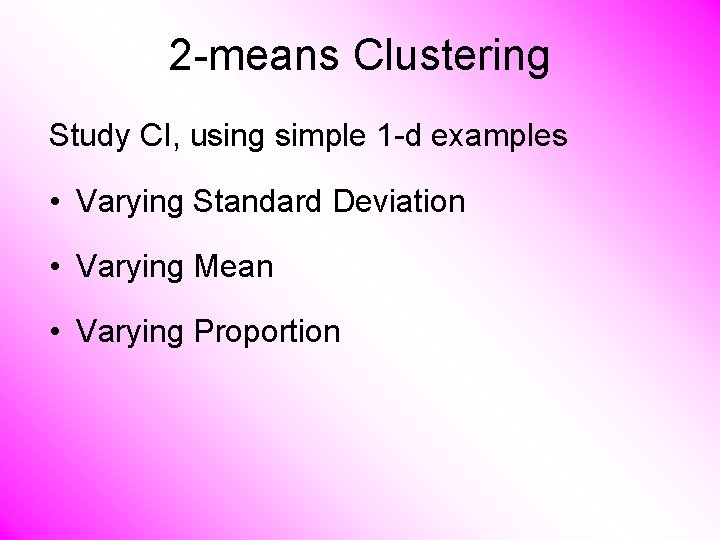
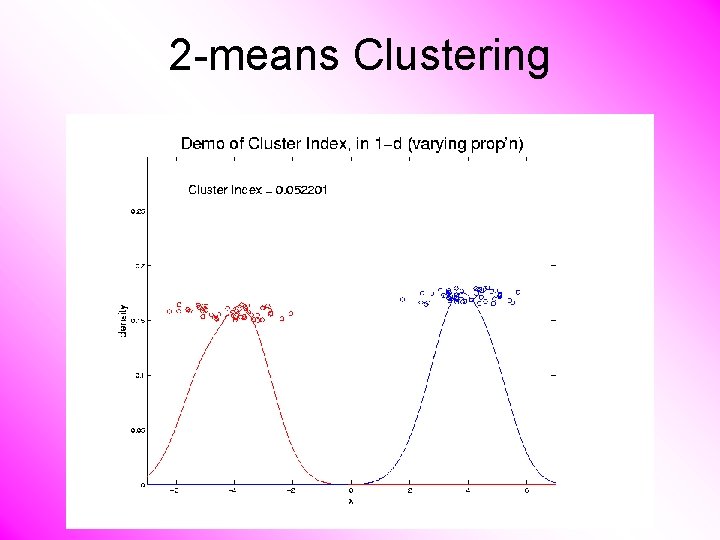
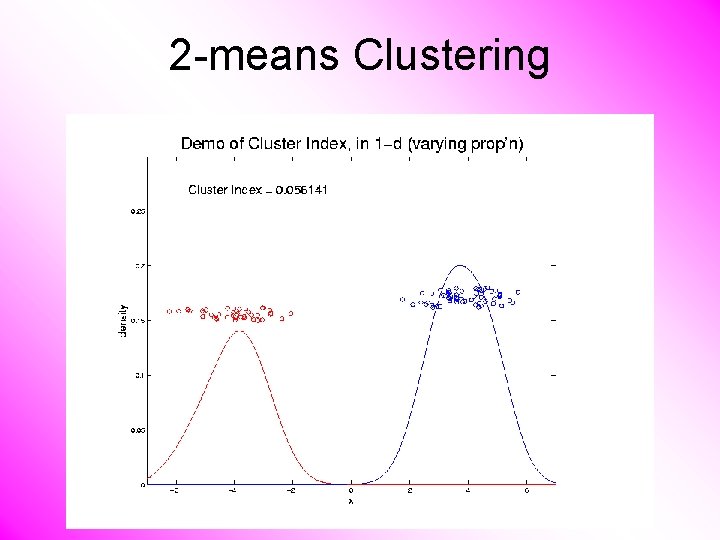
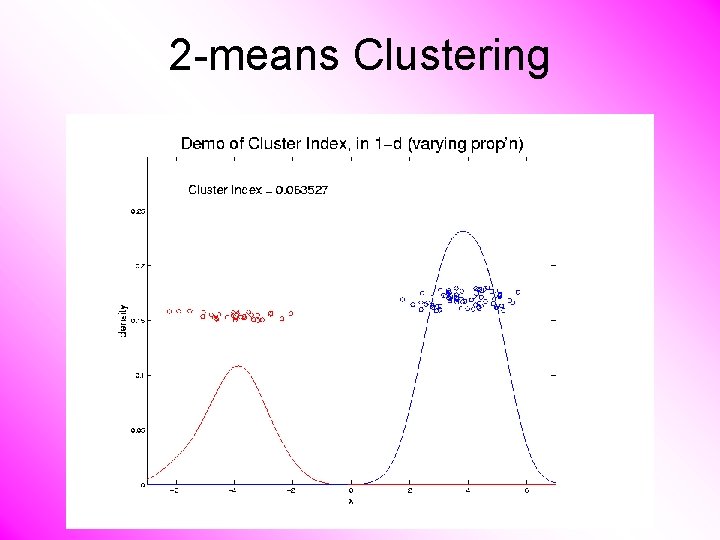
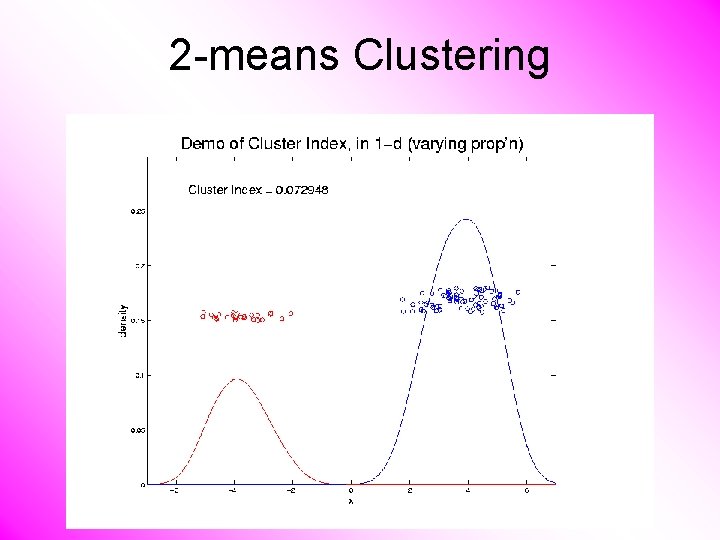
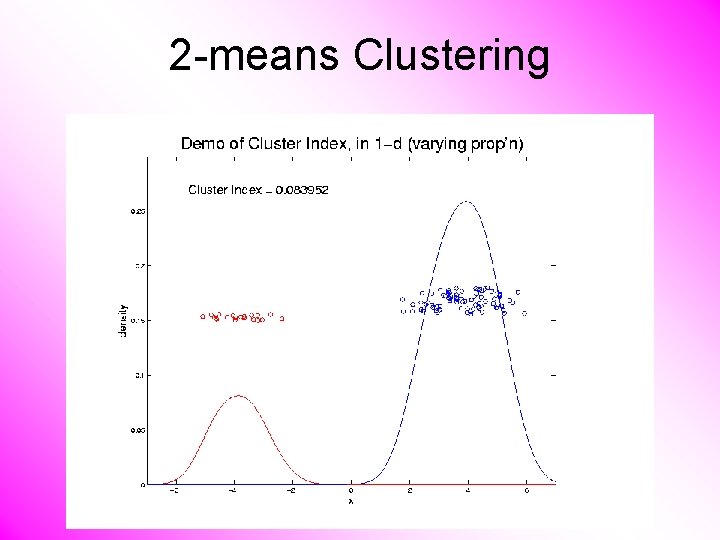
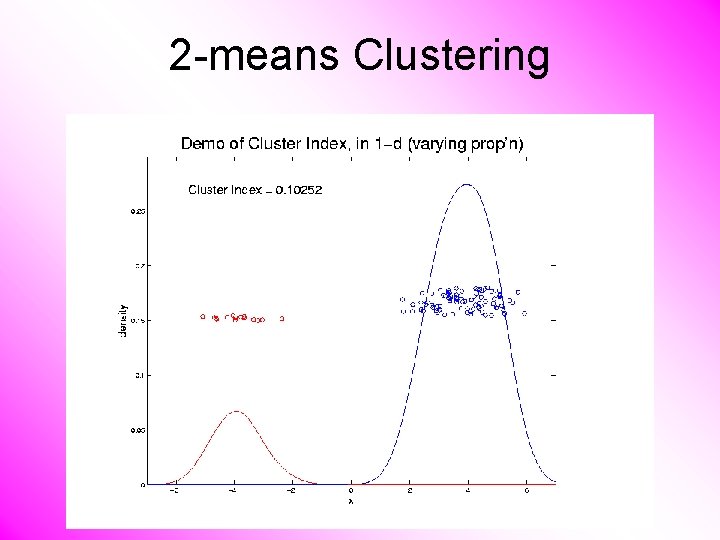
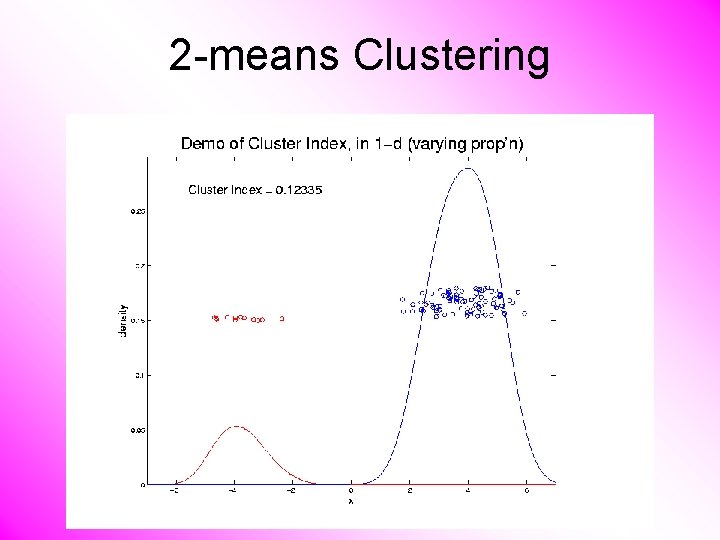
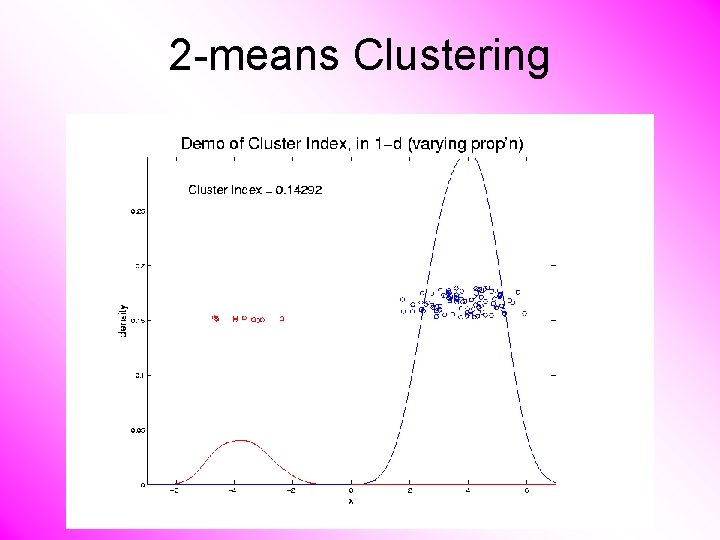
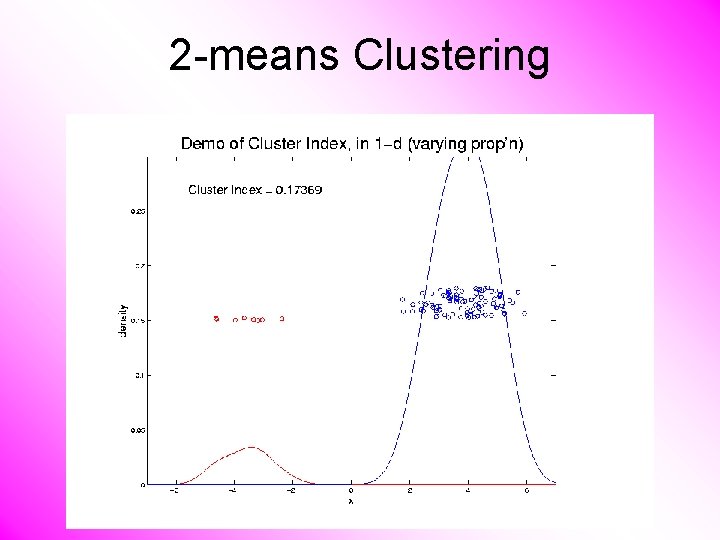
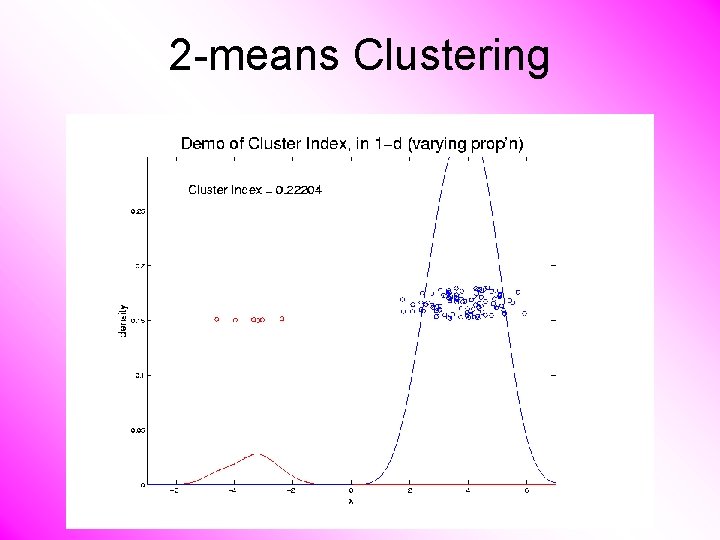
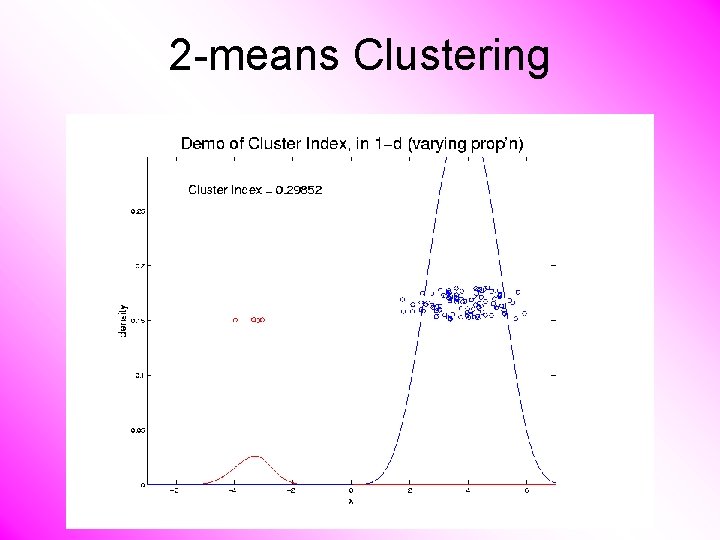
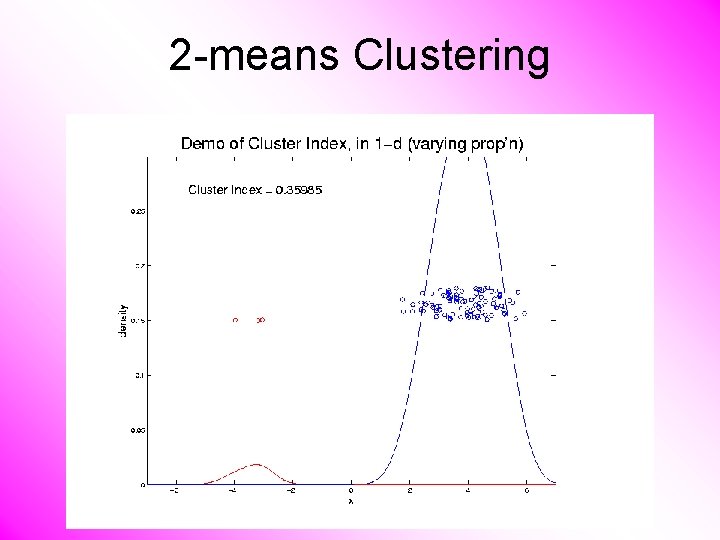
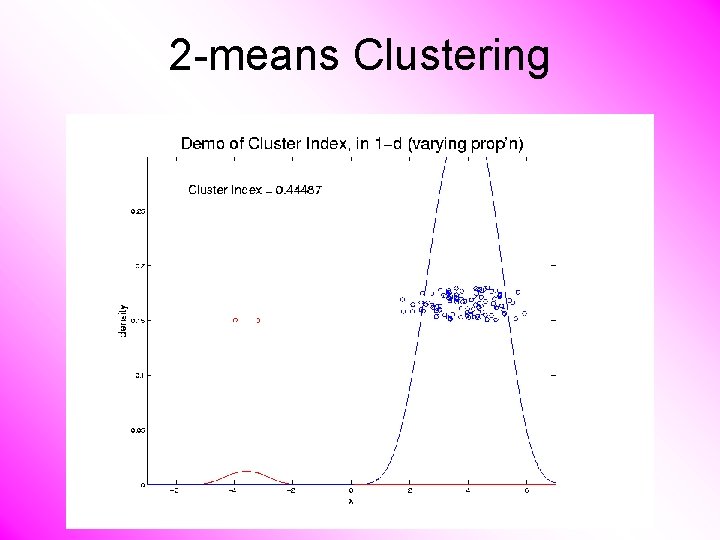
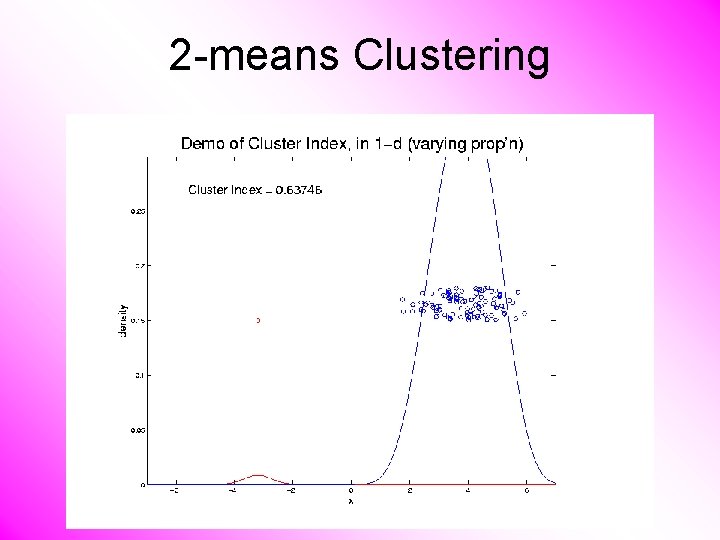
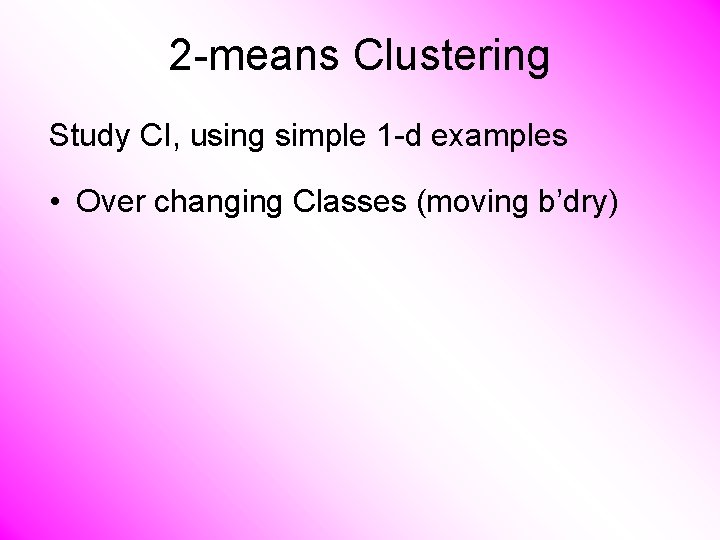
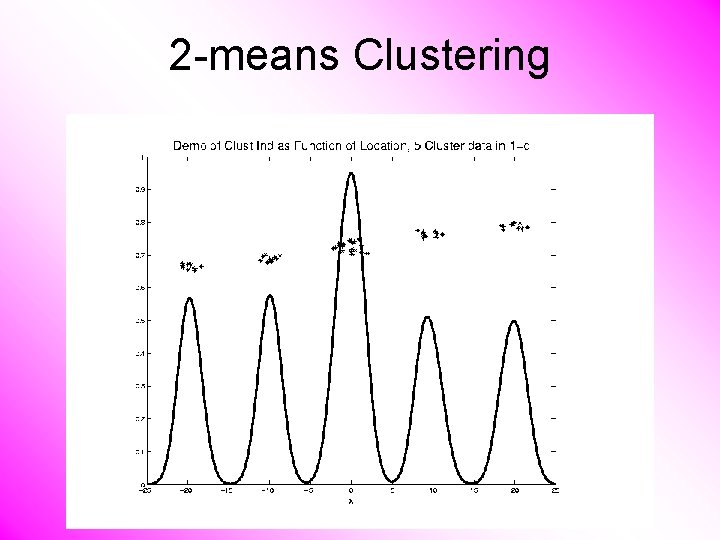
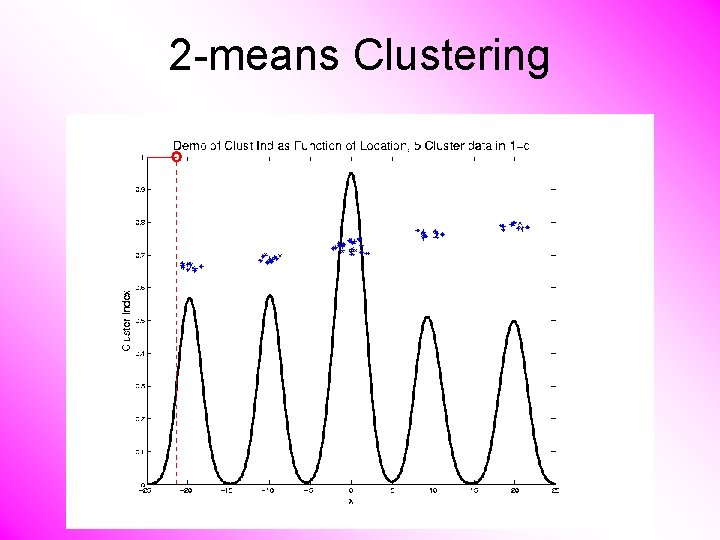
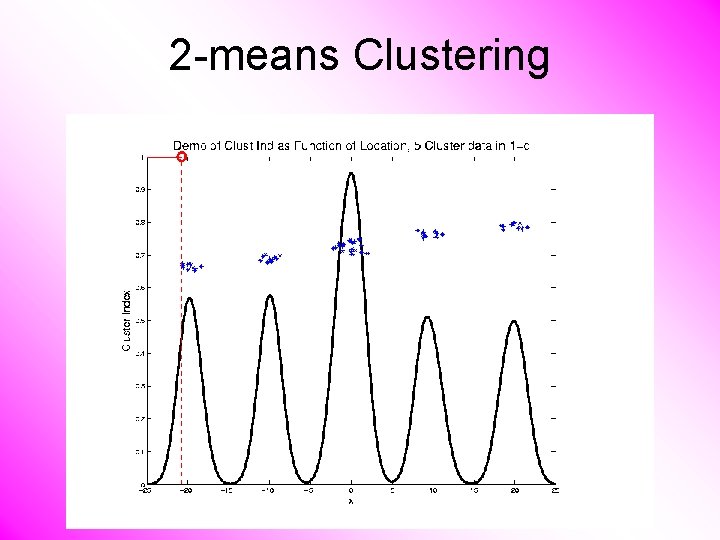
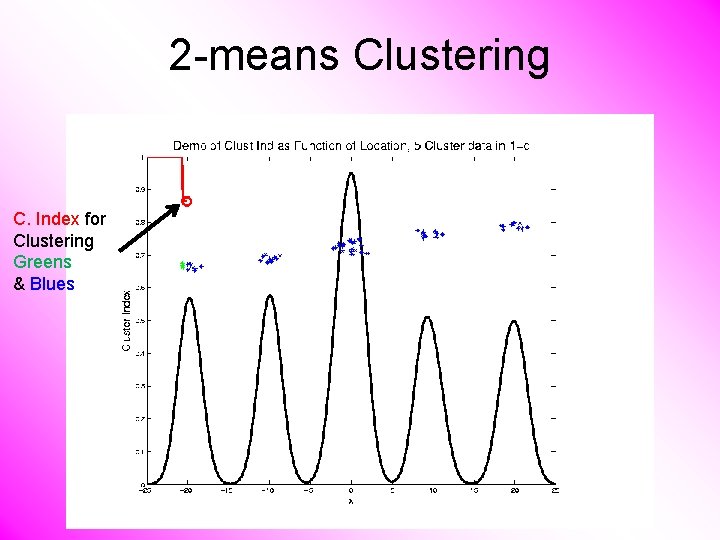
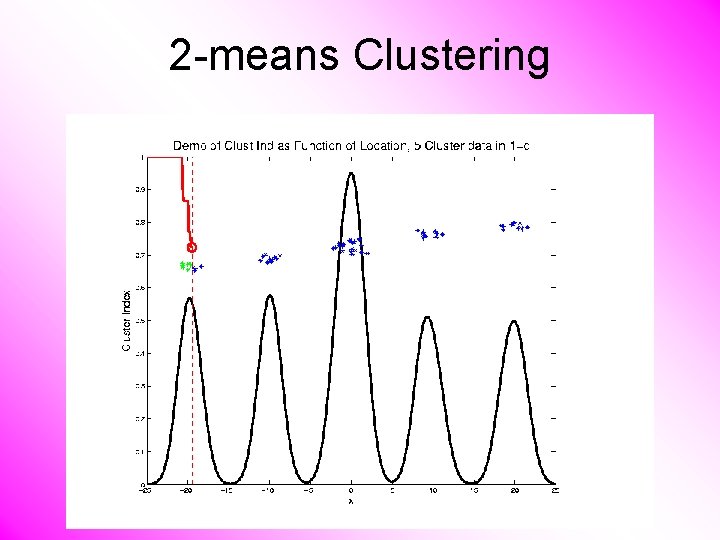
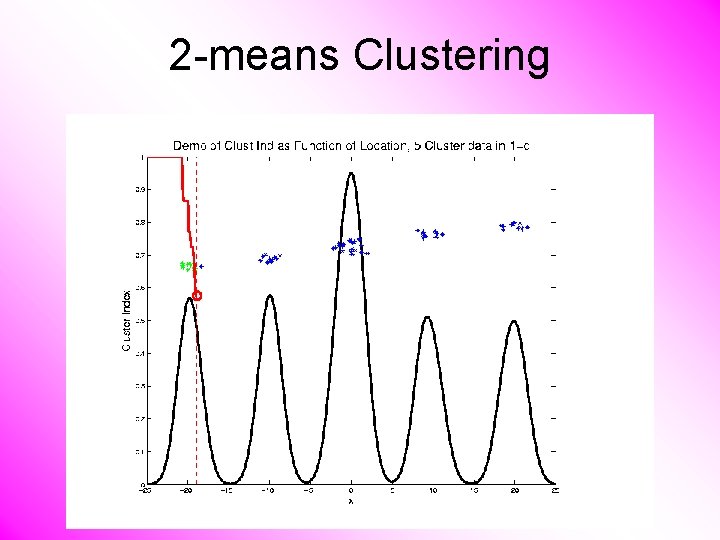
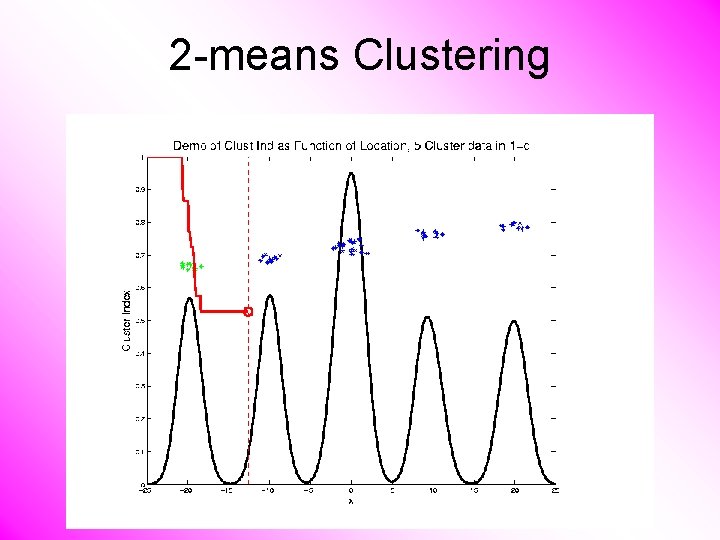
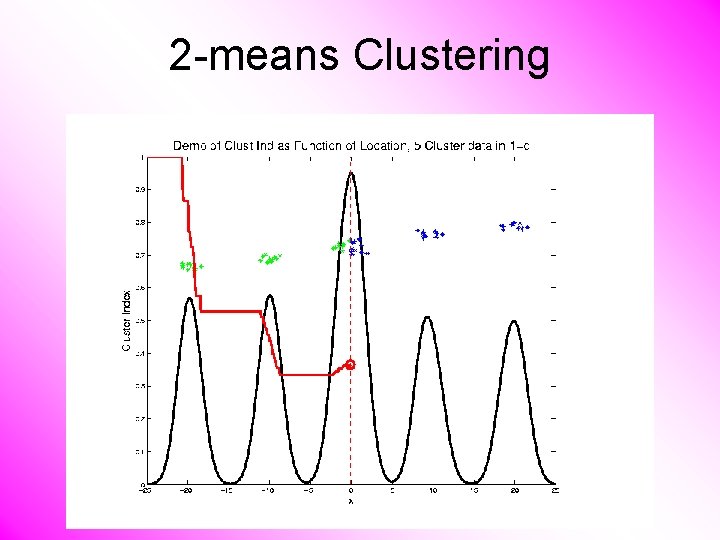
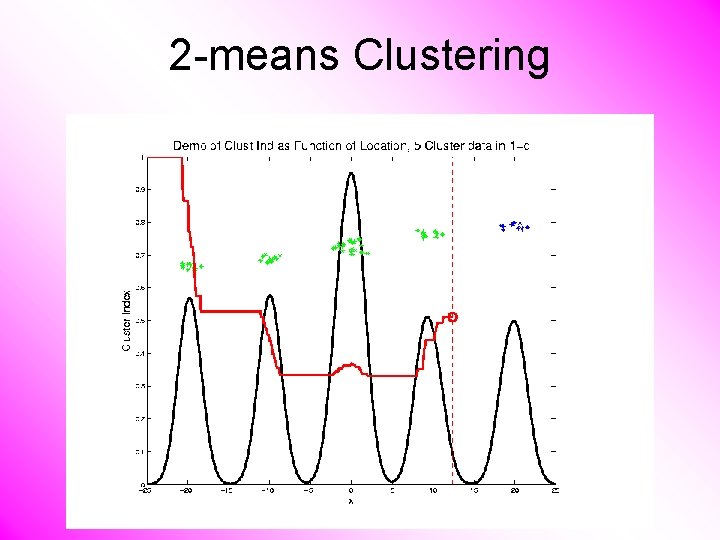
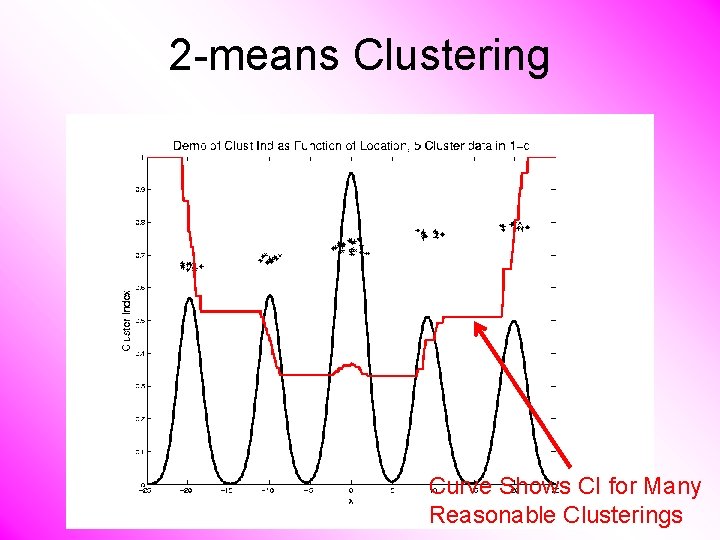
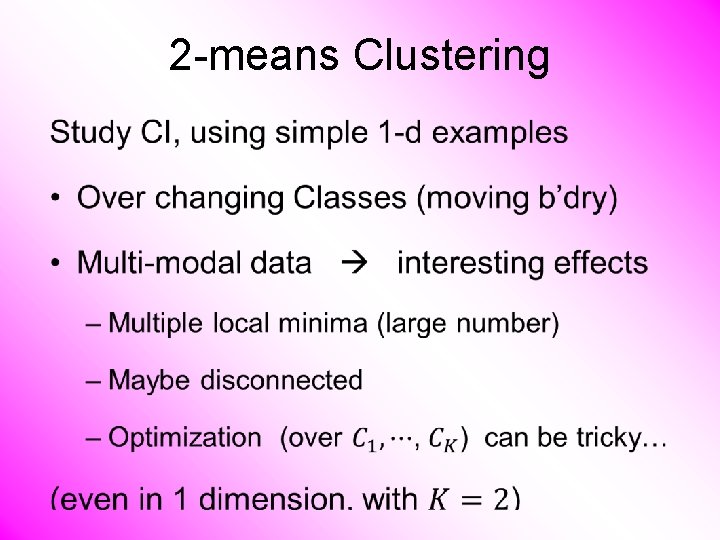
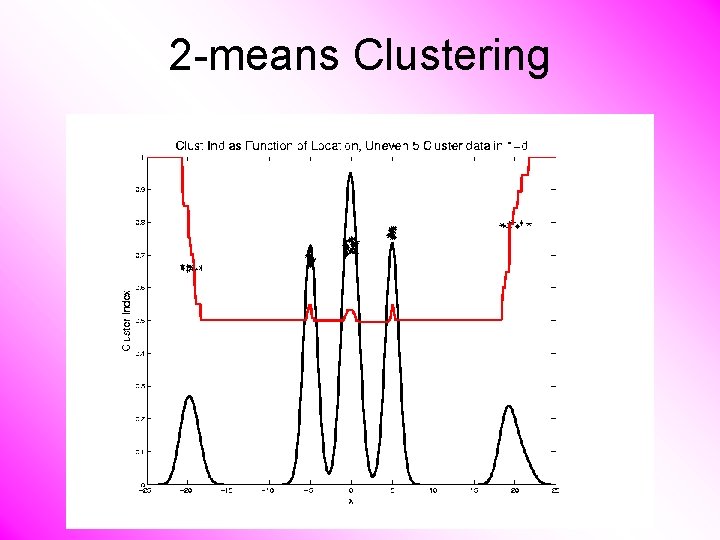
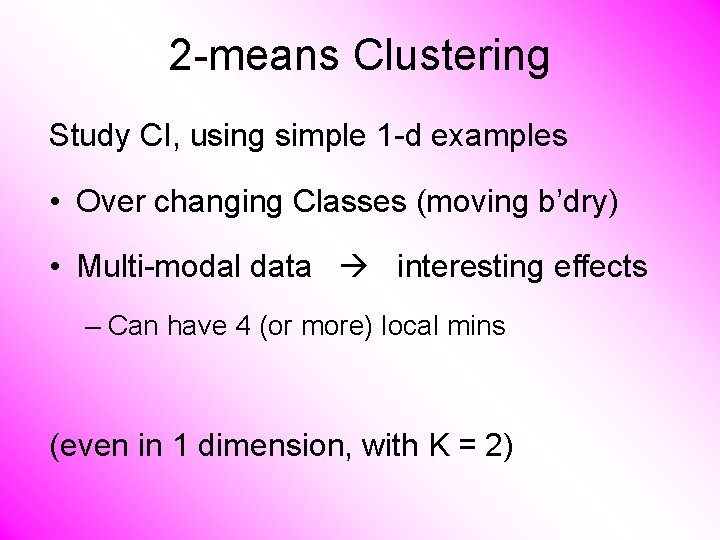
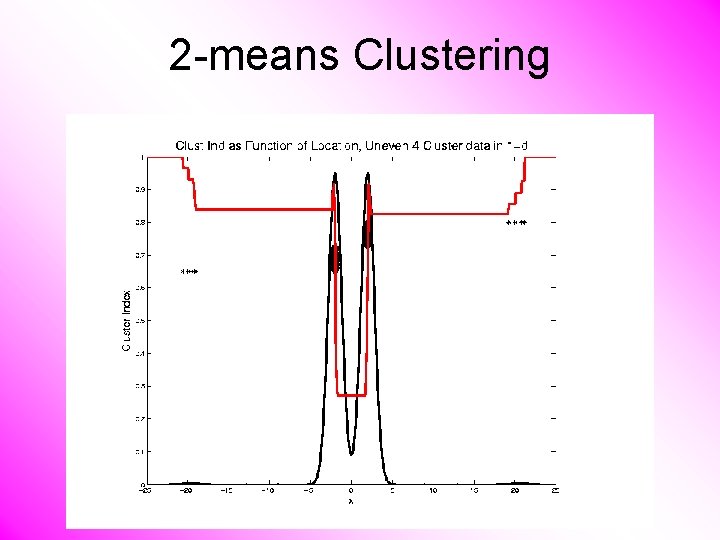
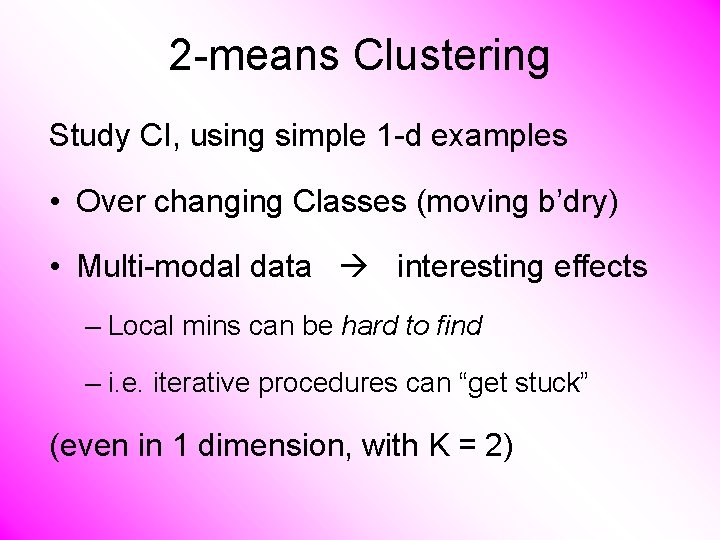
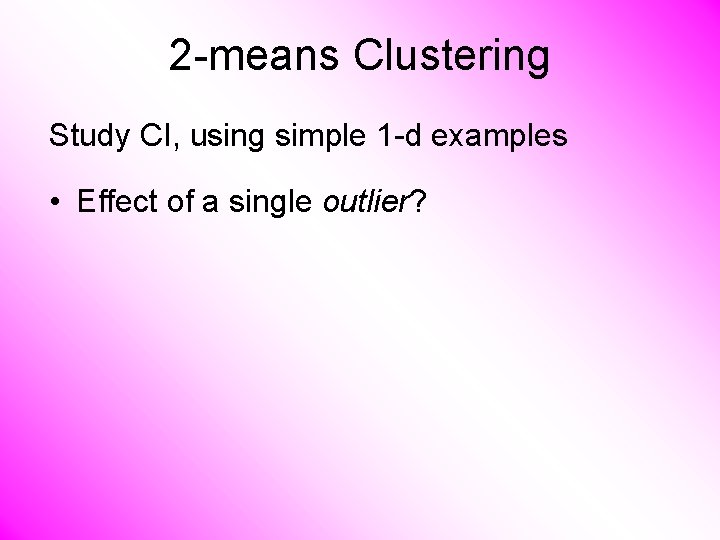
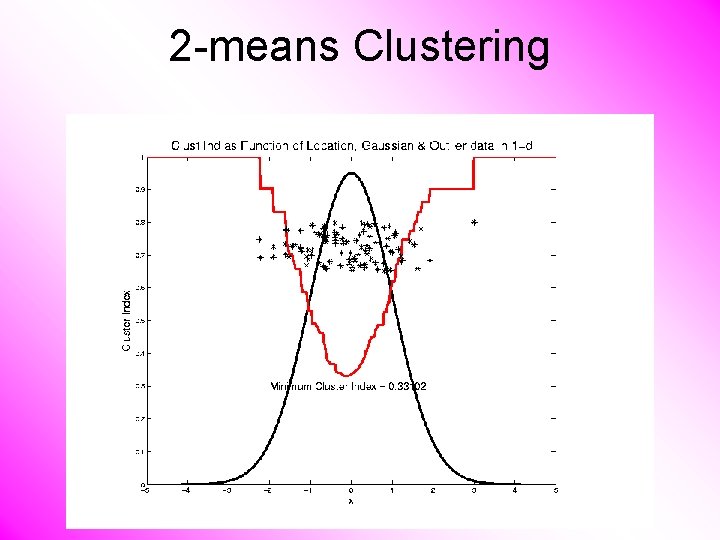
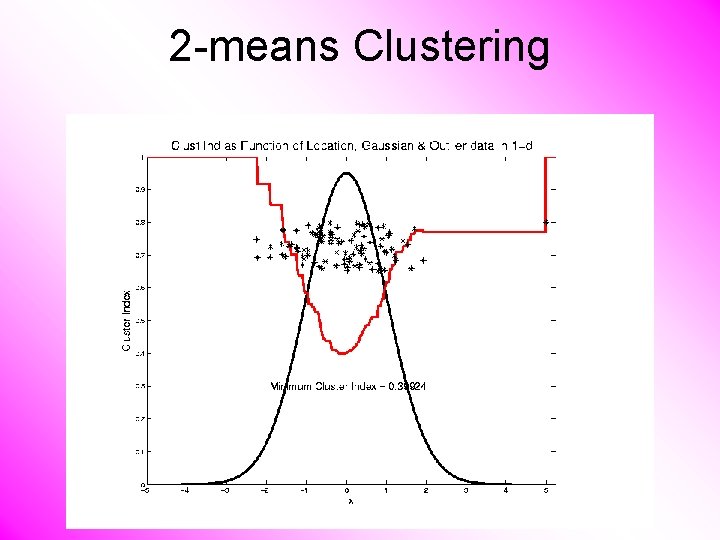
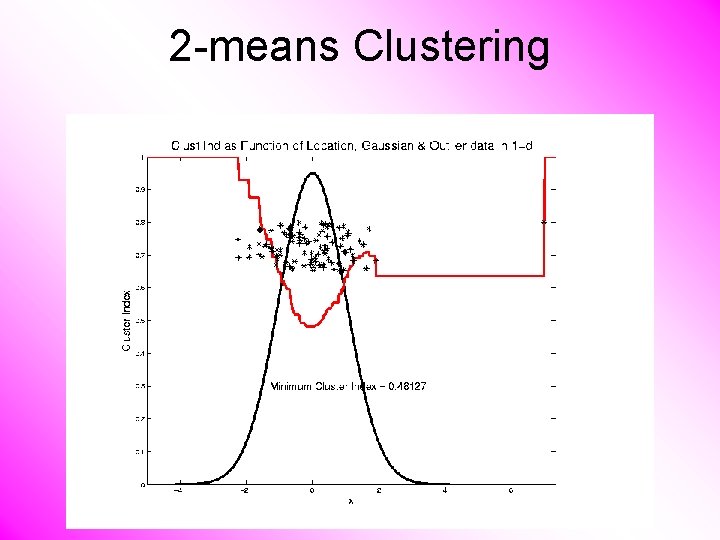
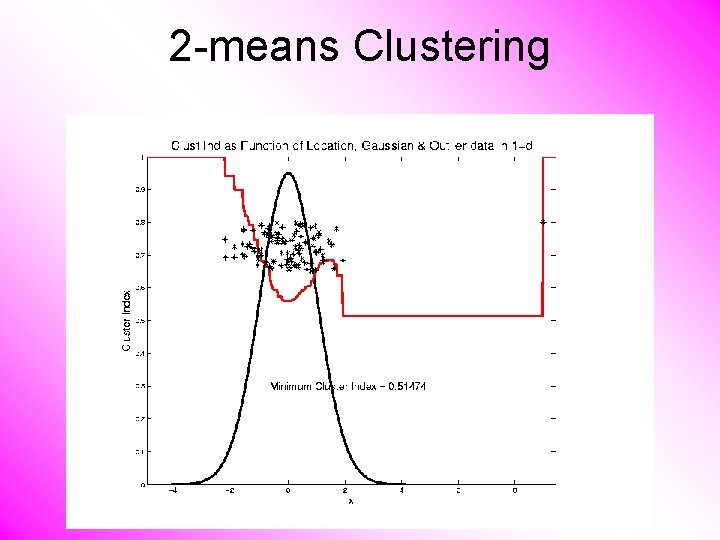
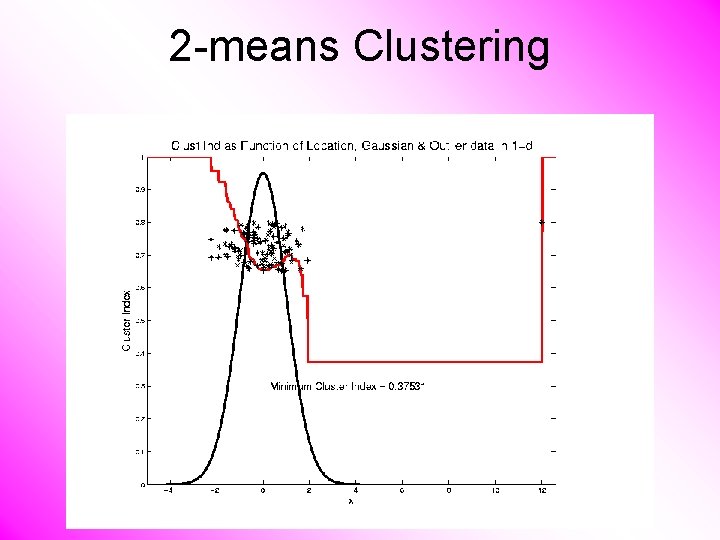
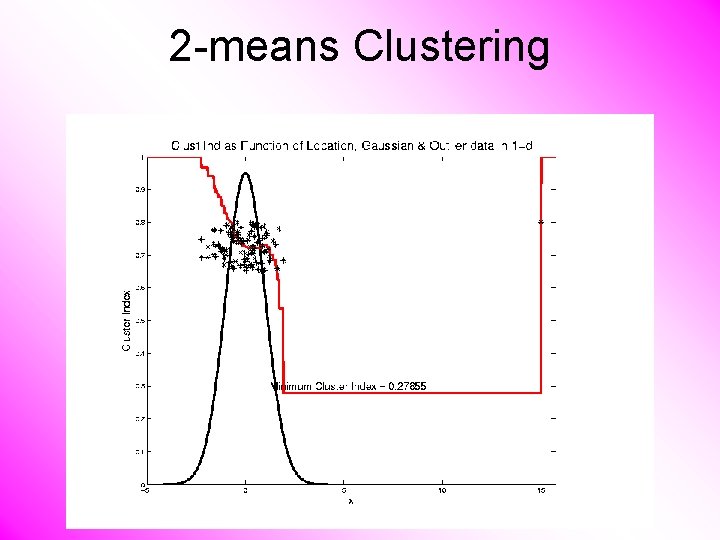
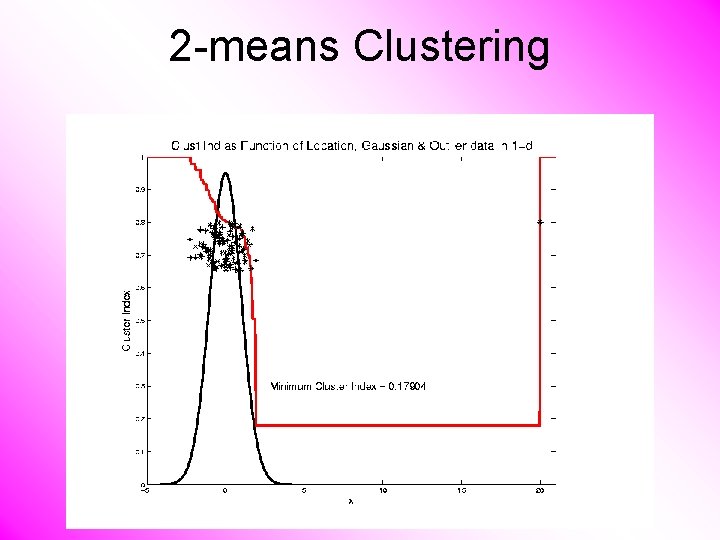
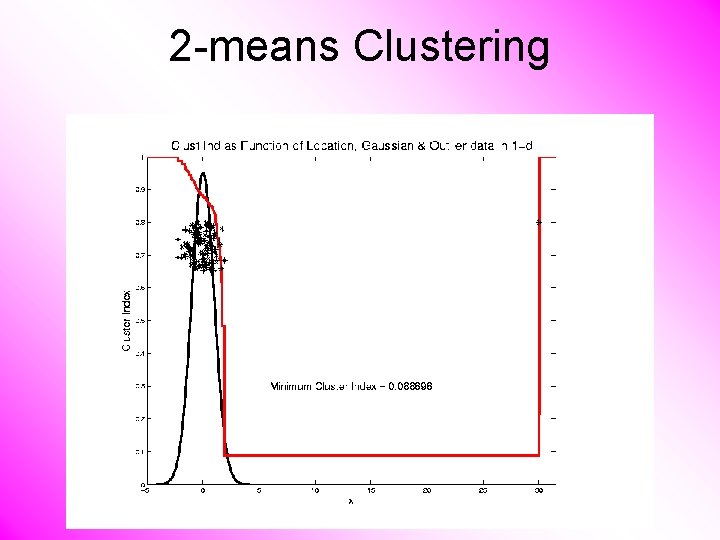
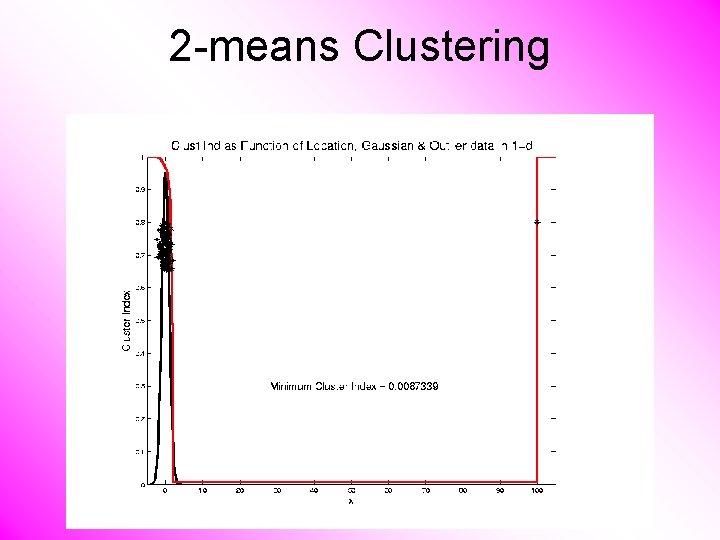
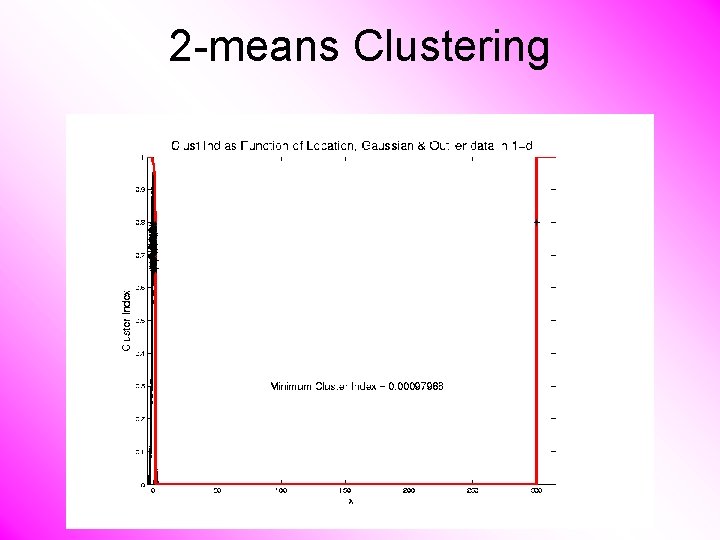
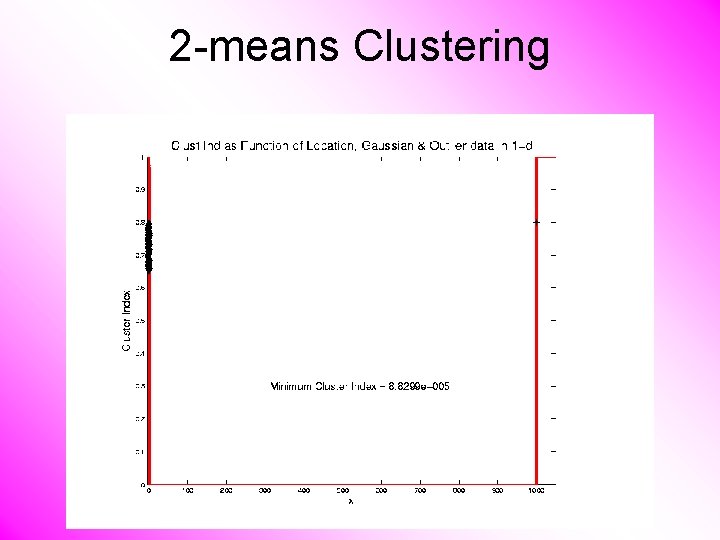
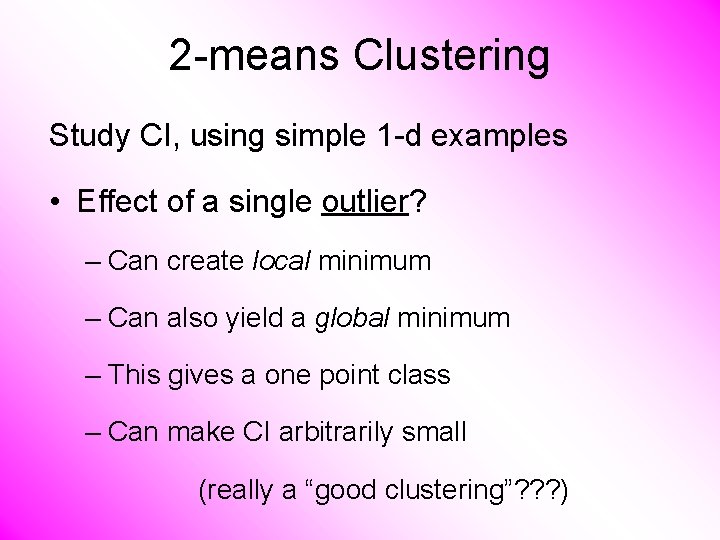
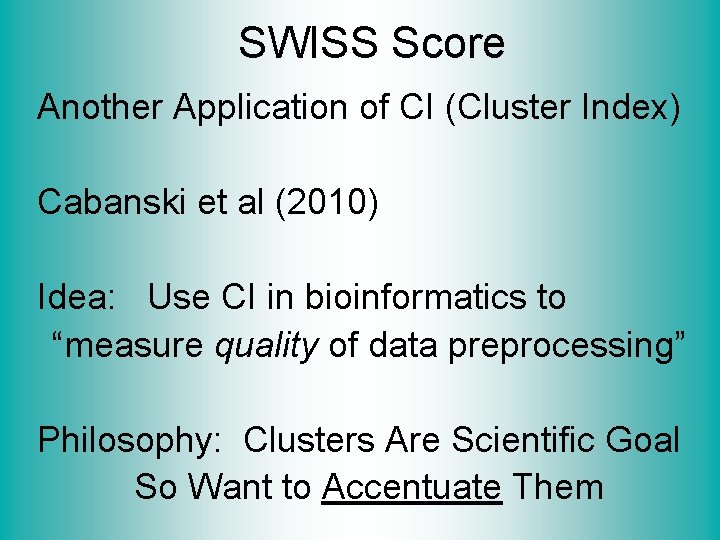
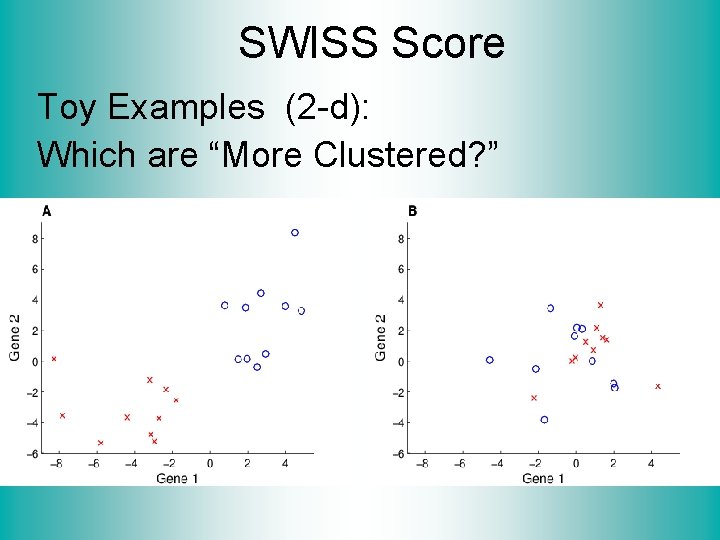
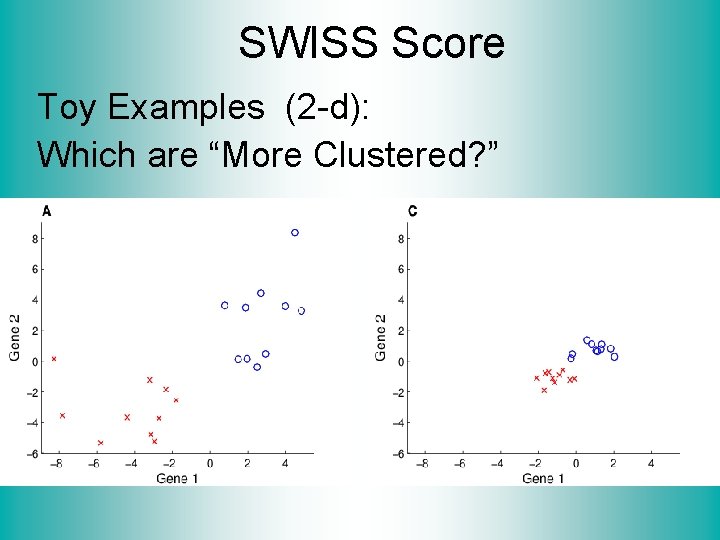
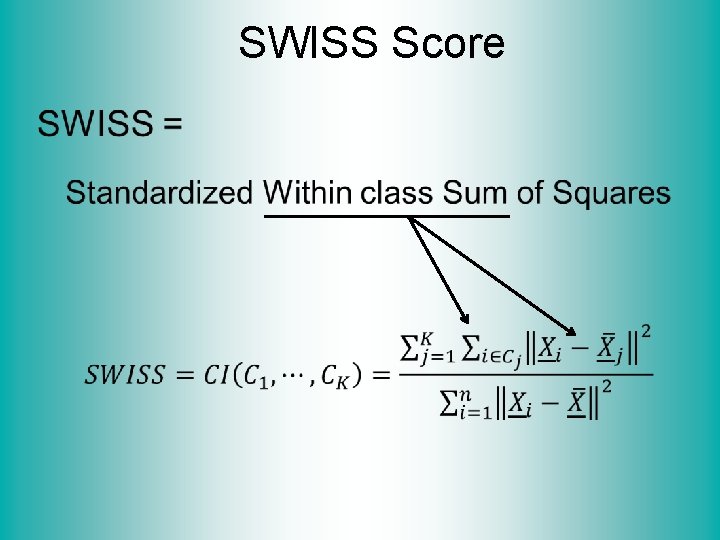
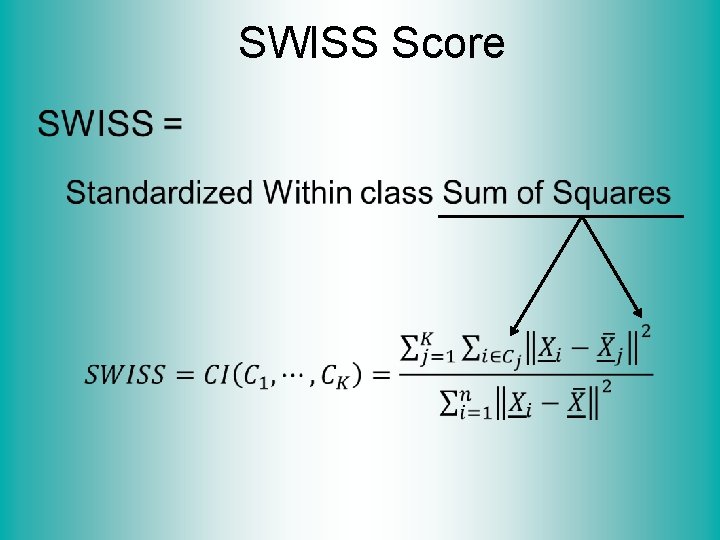
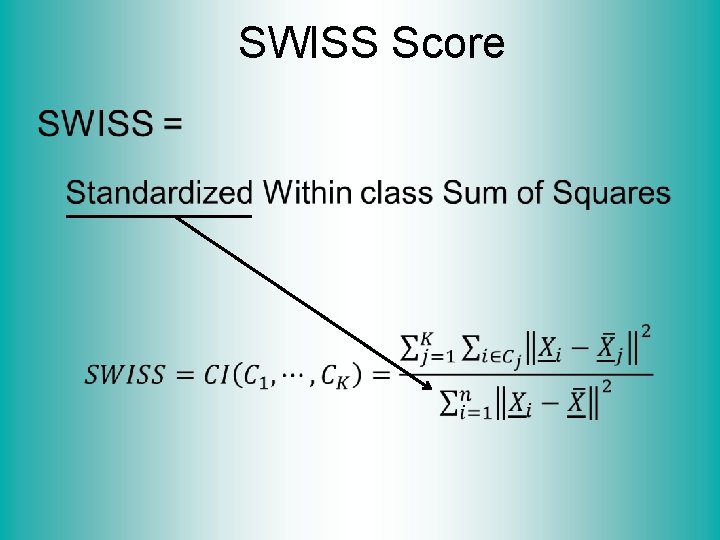
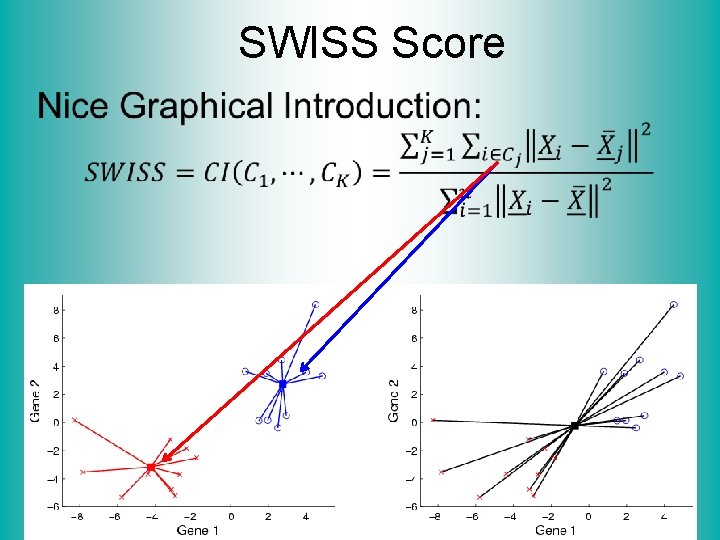
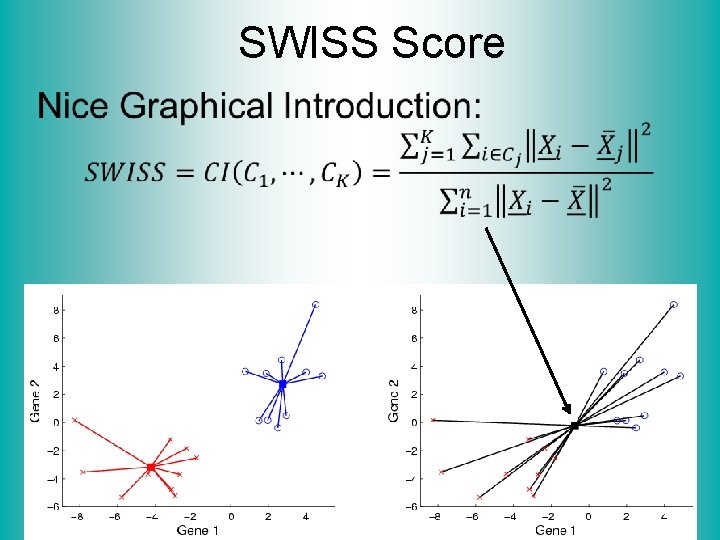
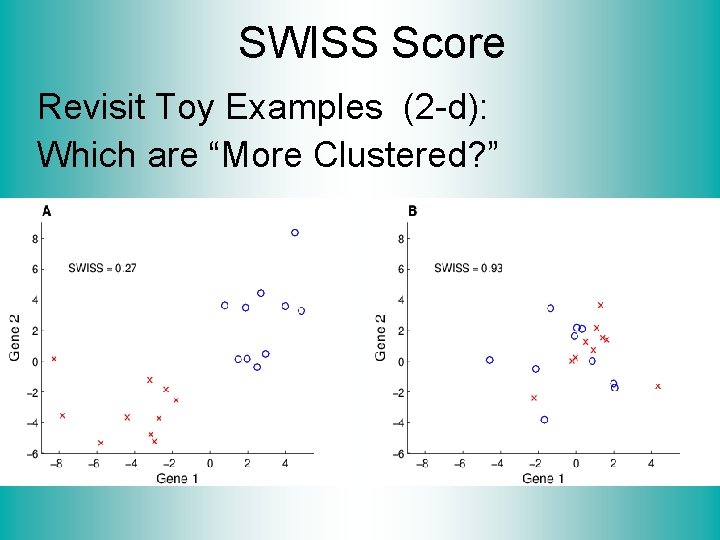
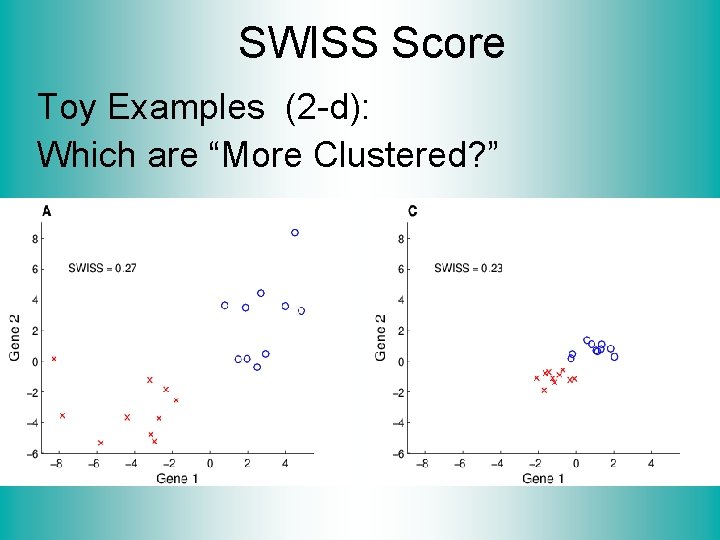
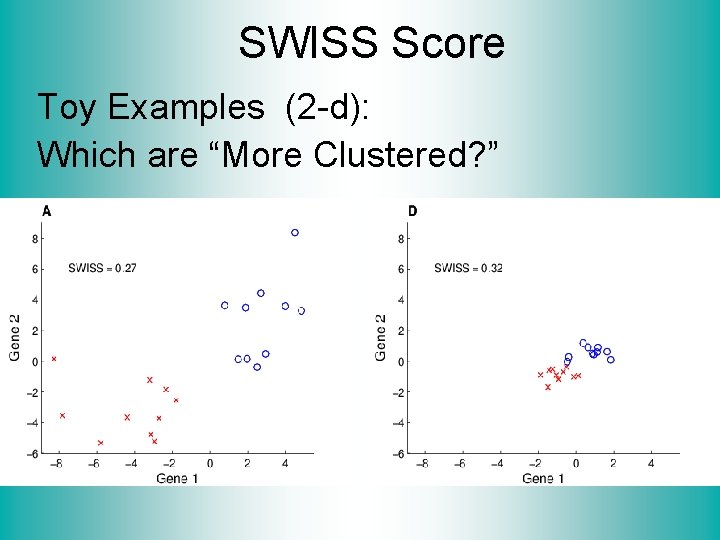
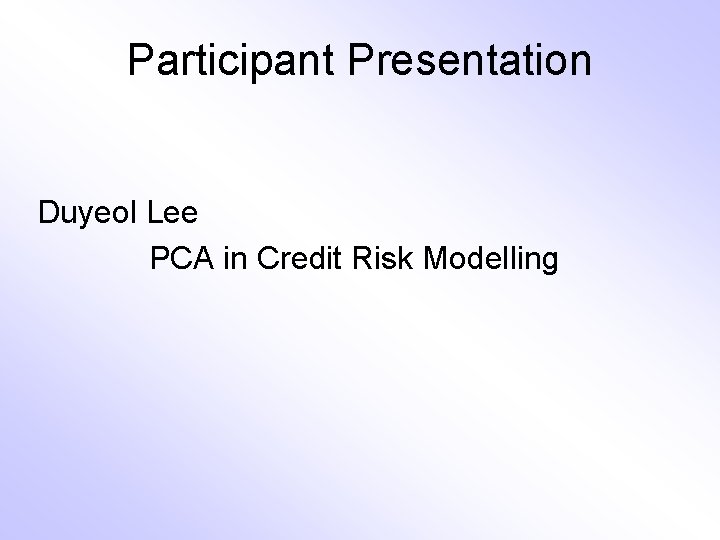
- Slides: 150
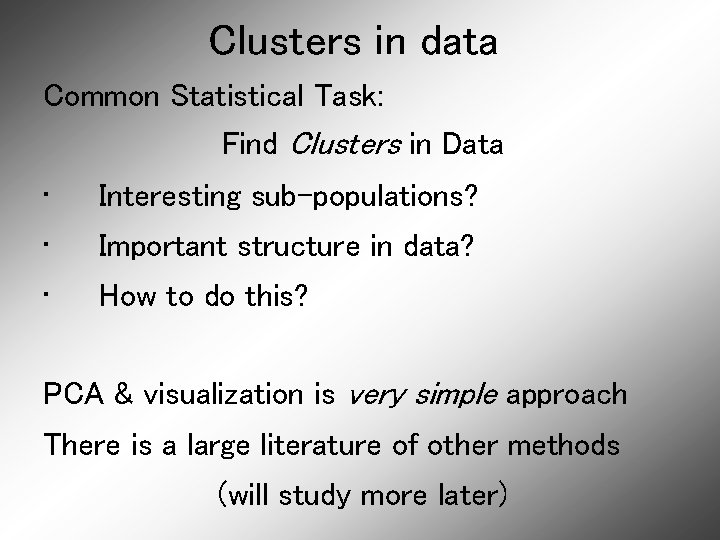
Clusters in data Common Statistical Task: Find Clusters in Data • Interesting sub-populations? • Important structure in data? • How to do this? PCA & visualization is very simple approach There is a large literature of other methods (will study more later)
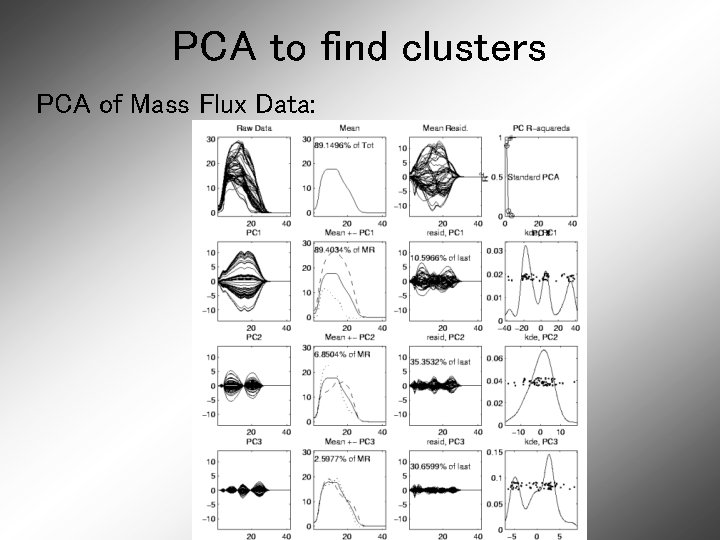
PCA to find clusters PCA of Mass Flux Data:
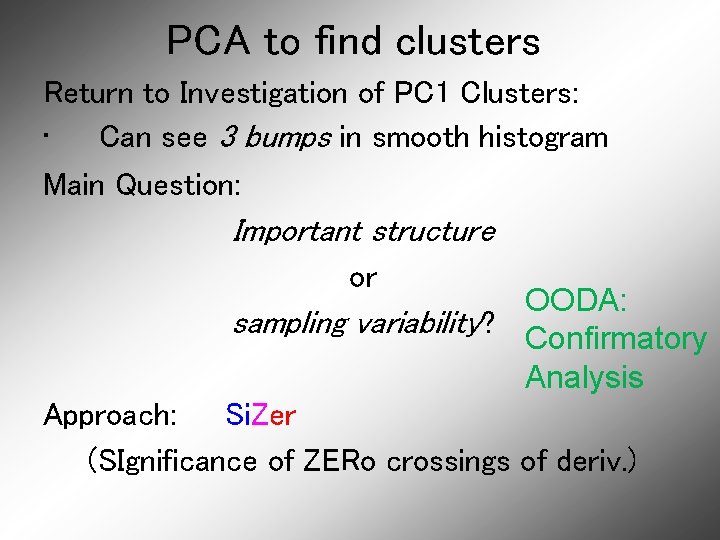
PCA to find clusters Return to Investigation of PC 1 Clusters: • Can see 3 bumps in smooth histogram Main Question: Important structure or OODA: sampling variability? Confirmatory Analysis Approach: Si. Zer (SIgnificance of ZERo crossings of deriv. )
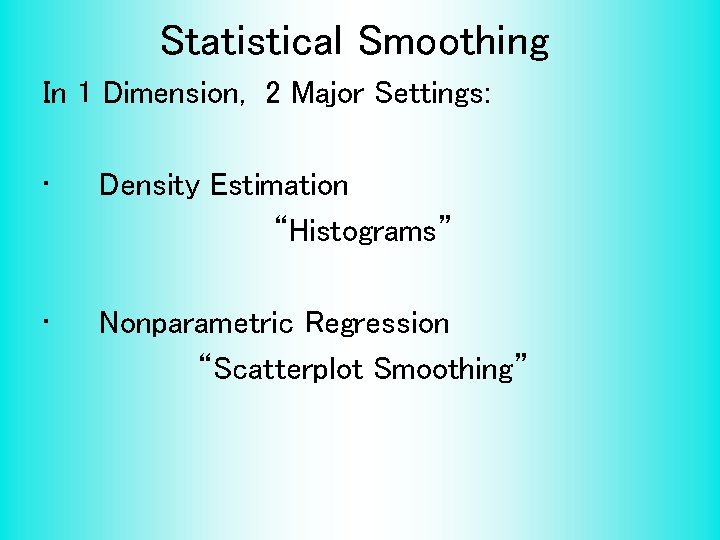
Statistical Smoothing In 1 Dimension, 2 Major Settings: • Density Estimation “Histograms” • Nonparametric Regression “Scatterplot Smoothing”
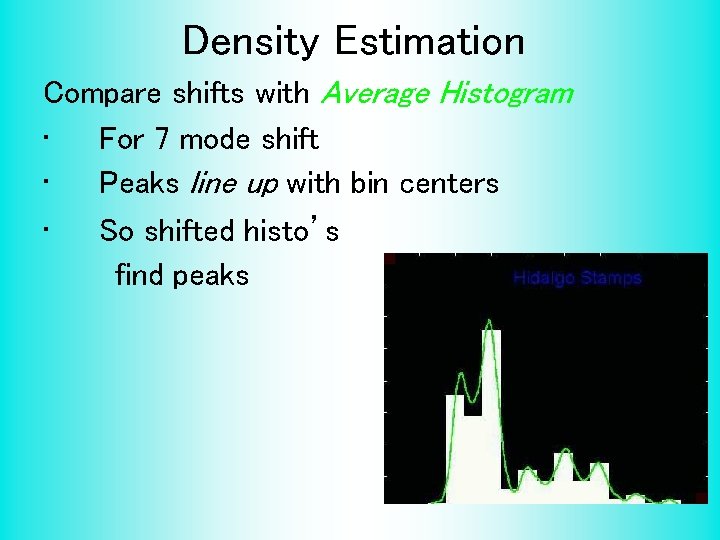
Density Estimation Compare shifts with Average Histogram • For 7 mode shift • Peaks line up with bin centers • So shifted histo’s find peaks
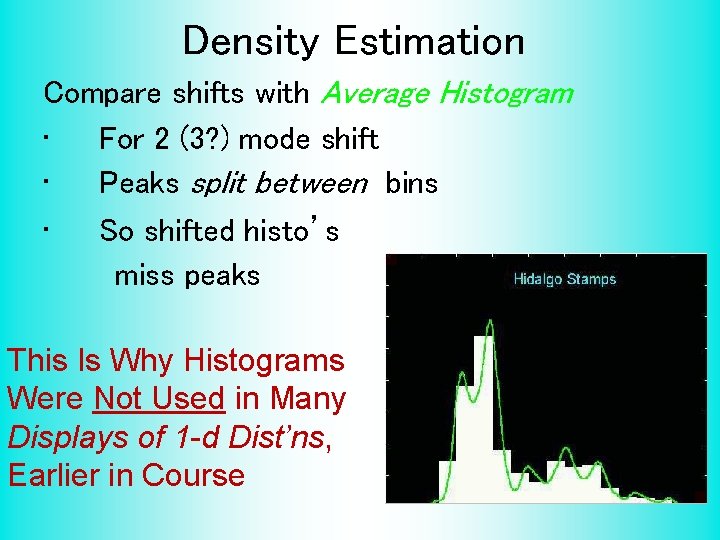
Density Estimation Compare shifts with Average Histogram • For 2 (3? ) mode shift • Peaks split between bins • So shifted histo’s miss peaks This Is Why Histograms Were Not Used in Many Displays of 1 -d Dist’ns, Earlier in Course
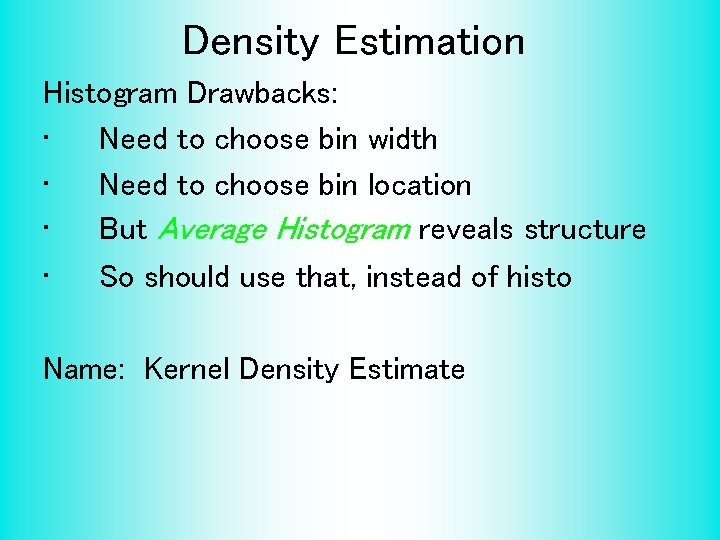
Density Estimation Histogram Drawbacks: • Need to choose bin width • Need to choose bin location • But Average Histogram reveals structure • So should use that, instead of histo Name: Kernel Density Estimate
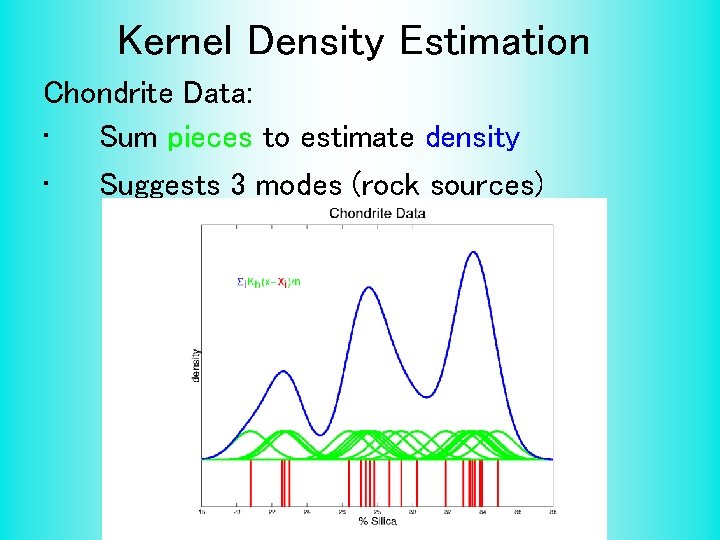
Kernel Density Estimation Chondrite Data: • Sum pieces to estimate density • Suggests 3 modes (rock sources)
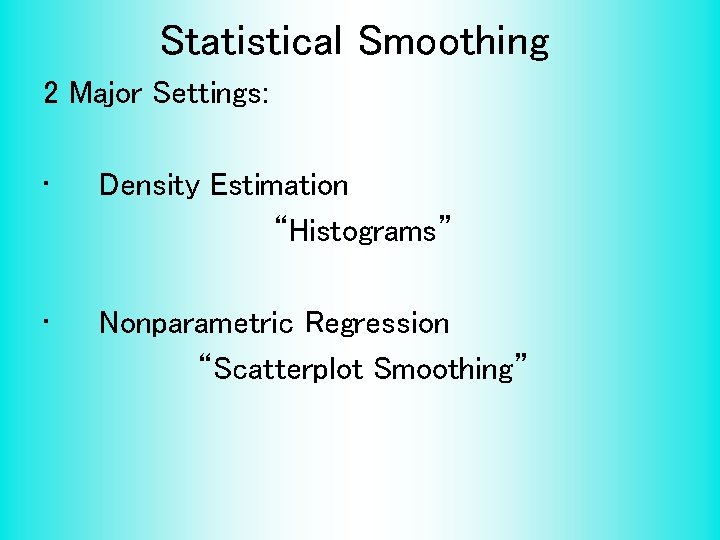
Statistical Smoothing 2 Major Settings: • Density Estimation “Histograms” • Nonparametric Regression “Scatterplot Smoothing”
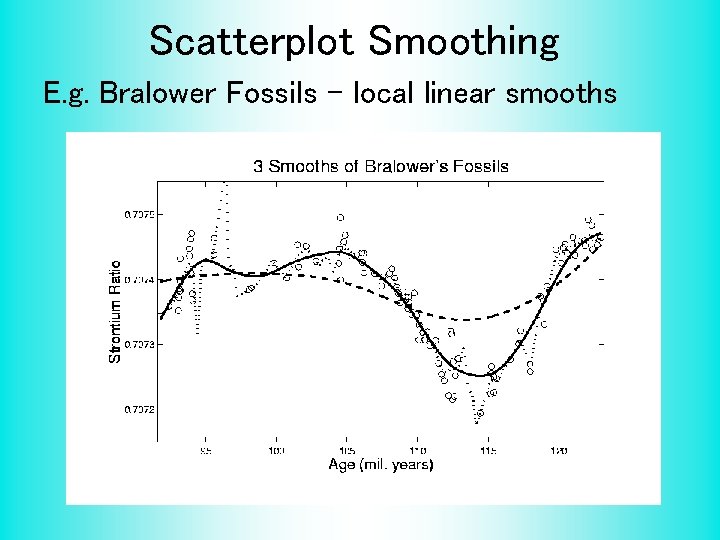
Scatterplot Smoothing E. g. Bralower Fossils – local linear smooths
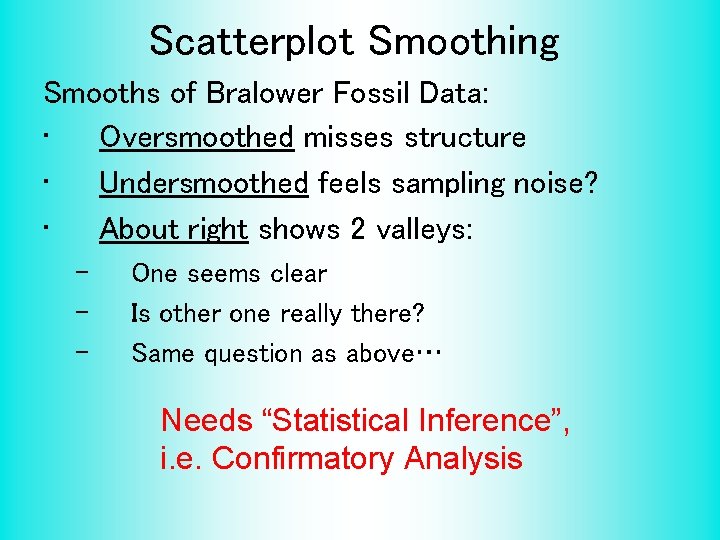
Scatterplot Smoothing Smooths of Bralower Fossil Data: • Oversmoothed misses structure • Undersmoothed feels sampling noise? • About right shows 2 valleys: – – – One seems clear Is other one really there? Same question as above… Needs “Statistical Inference”, i. e. Confirmatory Analysis
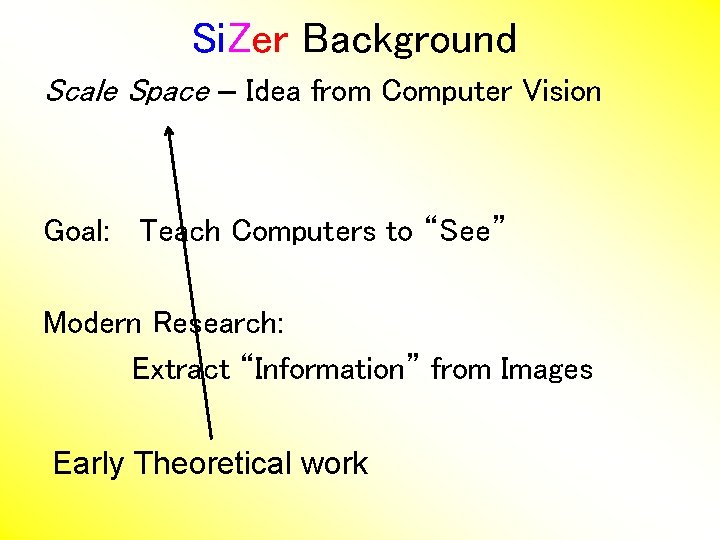
Si. Zer Background Scale Space – Idea from Computer Vision Goal: Teach Computers to “See” Modern Research: Extract “Information” from Images Early Theoretical work
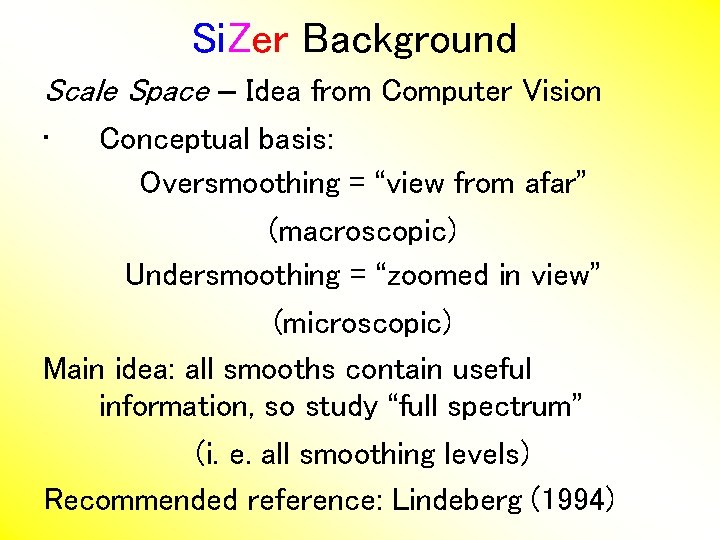
Si. Zer Background Scale Space – Idea from Computer Vision • Conceptual basis: Oversmoothing = “view from afar” (macroscopic) Undersmoothing = “zoomed in view” (microscopic) Main idea: all smooths contain useful information, so study “full spectrum” (i. e. all smoothing levels) Recommended reference: Lindeberg (1994)
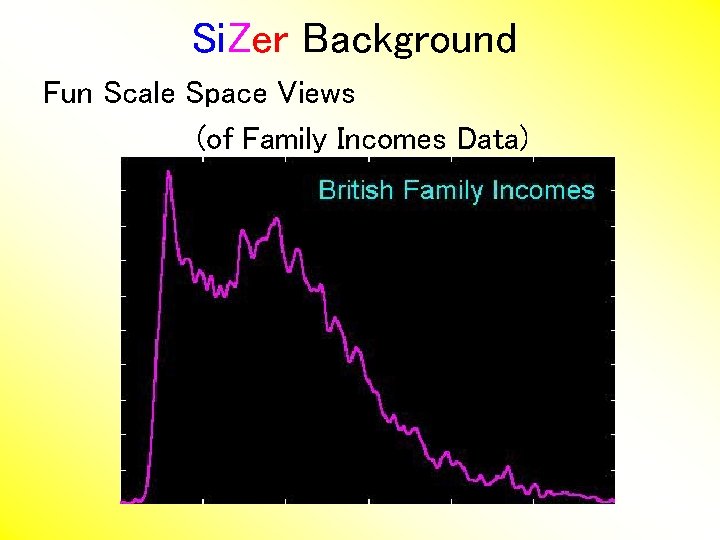
Si. Zer Background Fun Scale Space Views (of Family Incomes Data)
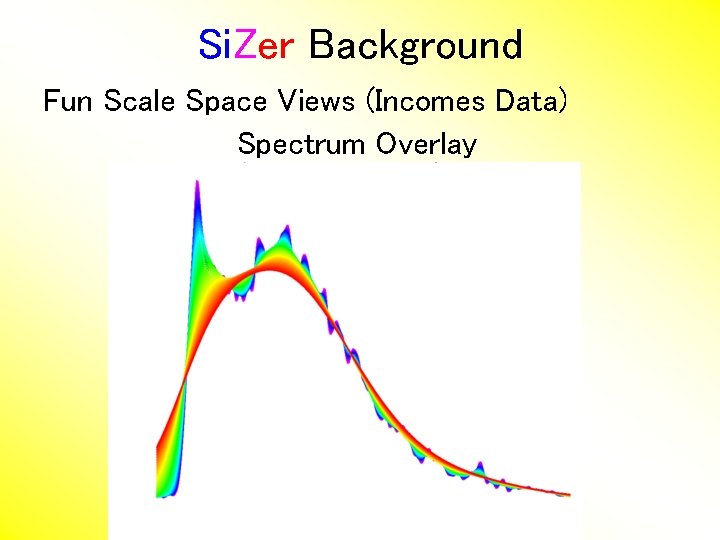
Si. Zer Background Fun Scale Space Views (Incomes Data) Spectrum Overlay
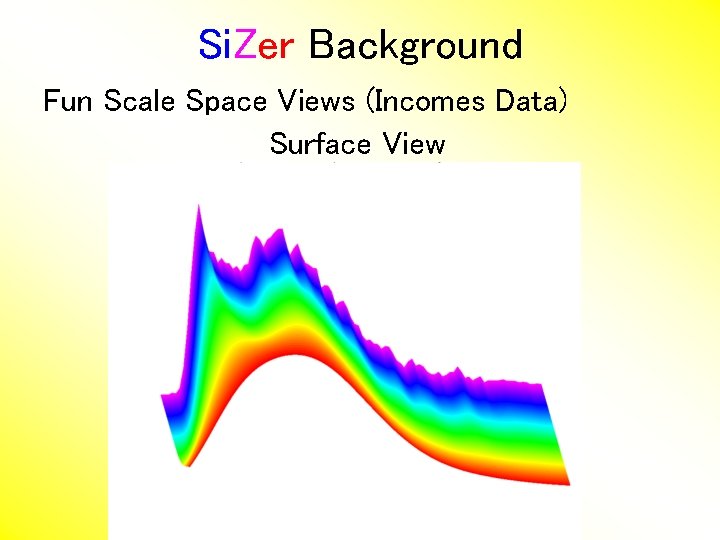
Si. Zer Background Fun Scale Space Views (Incomes Data) Surface View
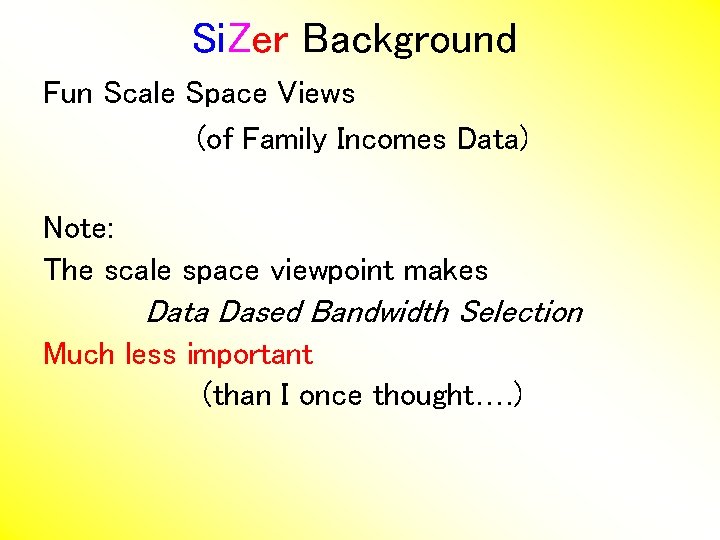
Si. Zer Background Fun Scale Space Views (of Family Incomes Data) Note: The scale space viewpoint makes Data Dased Bandwidth Selection Much less important (than I once thought…. )
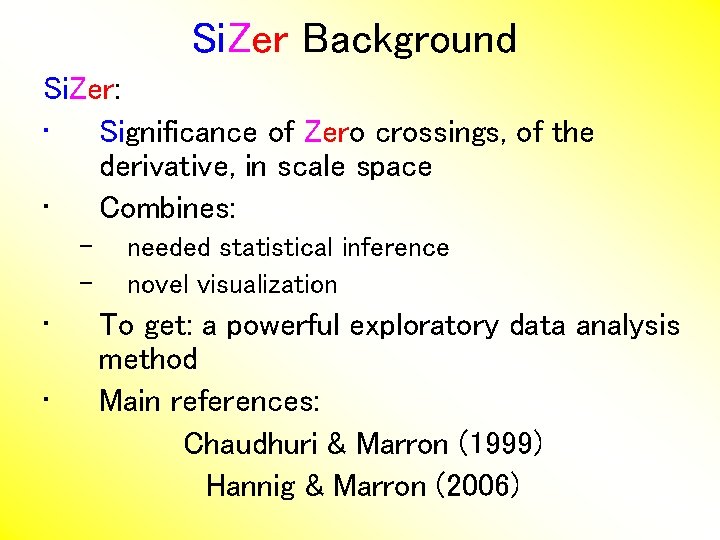
Si. Zer Background Si. Zer: • Significance of Zero crossings, of the derivative, in scale space • Combines: – – • • needed statistical inference novel visualization To get: a powerful exploratory data analysis method Main references: Chaudhuri & Marron (1999) Hannig & Marron (2006)
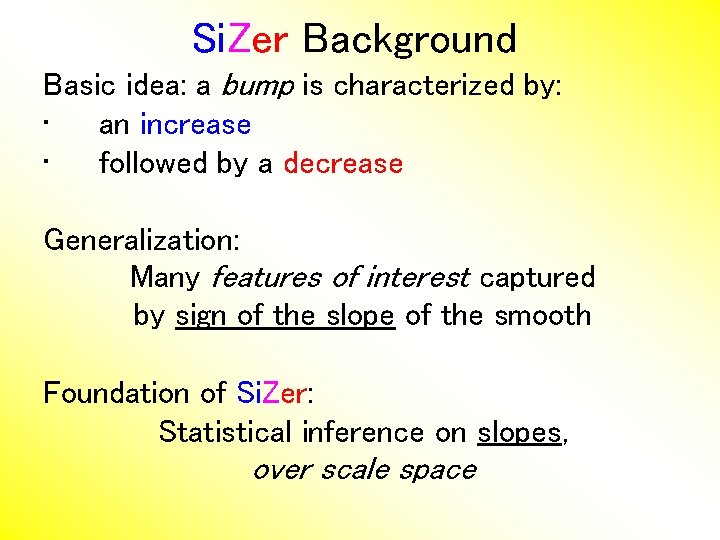
Si. Zer Background Basic idea: a bump is characterized by: • an increase • followed by a decrease Generalization: Many features of interest captured by sign of the slope of the smooth Foundation of Si. Zer: Statistical inference on slopes, over scale space
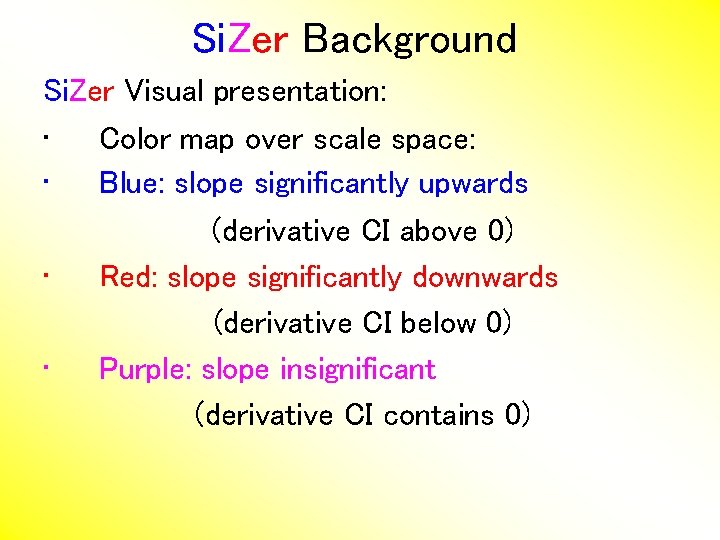
Si. Zer Background Si. Zer Visual presentation: • Color map over scale space: • Blue: slope significantly upwards (derivative CI above 0) • Red: slope significantly downwards (derivative CI below 0) • Purple: slope insignificant (derivative CI contains 0)
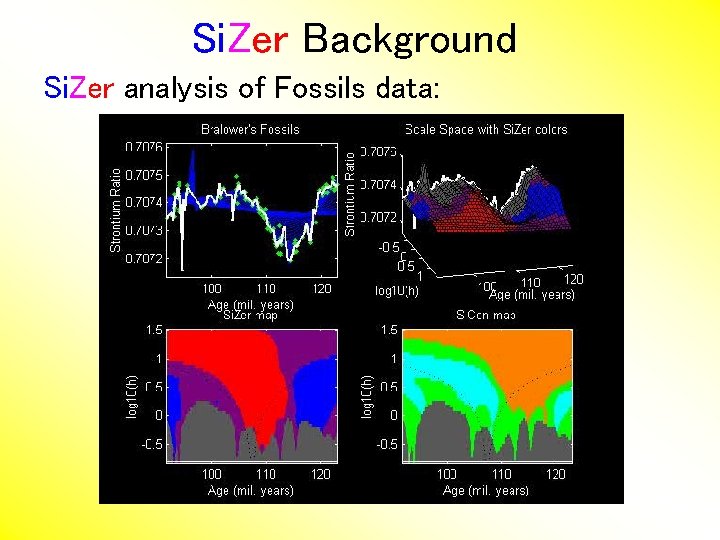
Si. Zer Background Si. Zer analysis of Fossils data:
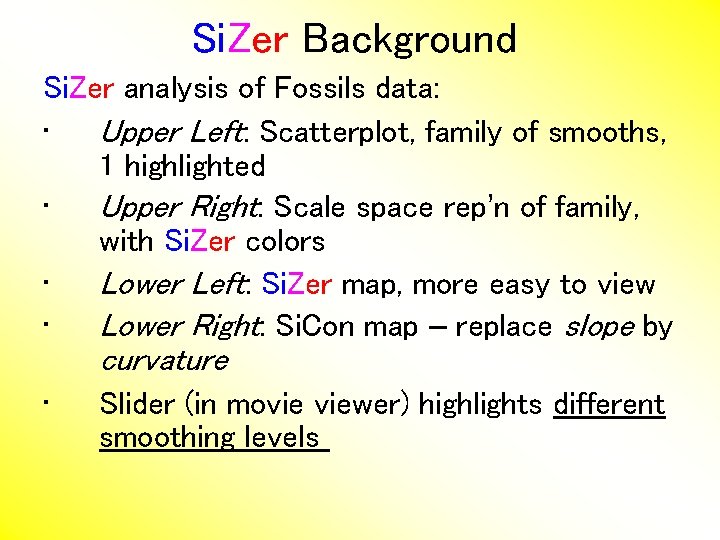
Si. Zer Background Si. Zer analysis of Fossils data: • Upper Left: Scatterplot, family of smooths, 1 highlighted • Upper Right: Scale space rep’n of family, with Si. Zer colors • Lower Left: Si. Zer map, more easy to view • Lower Right: Si. Con map – replace slope by curvature • Slider (in movie viewer) highlights different smoothing levels
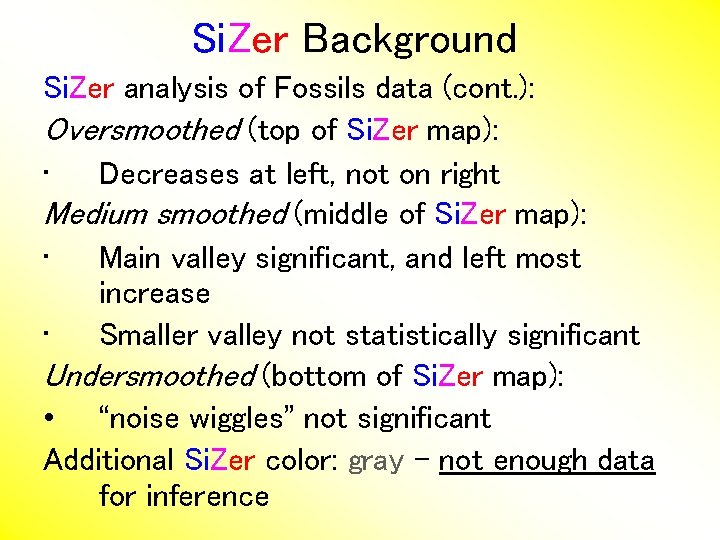
Si. Zer Background Si. Zer analysis of Fossils data (cont. ): Oversmoothed (top of Si. Zer map): • Decreases at left, not on right Medium smoothed (middle of Si. Zer map): • Main valley significant, and left most increase • Smaller valley not statistically significant Undersmoothed (bottom of Si. Zer map): • “noise wiggles” not significant Additional Si. Zer color: gray - not enough data for inference
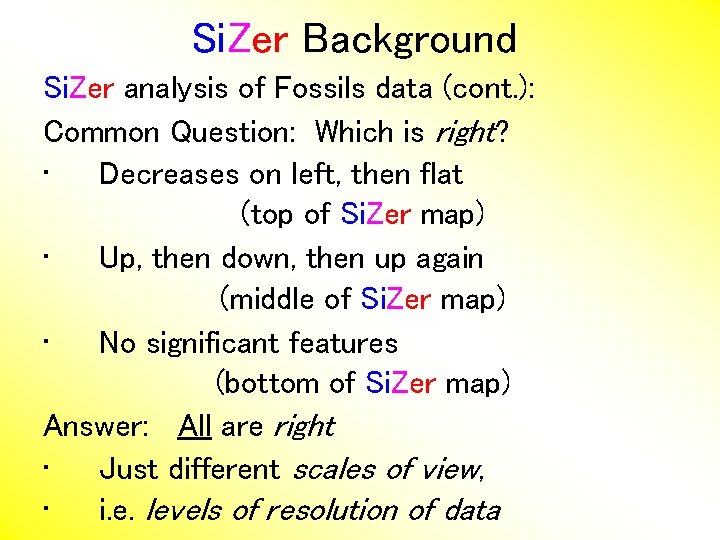
Si. Zer Background Si. Zer analysis of Fossils data (cont. ): Common Question: Which is right? • Decreases on left, then flat (top of Si. Zer map) • Up, then down, then up again (middle of Si. Zer map) • No significant features (bottom of Si. Zer map) Answer: All are right • Just different scales of view, • i. e. levels of resolution of data
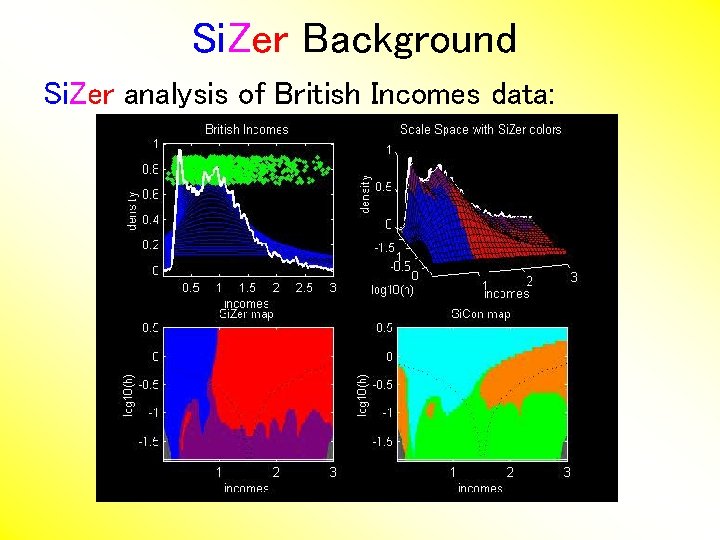
Si. Zer Background Si. Zer analysis of British Incomes data:
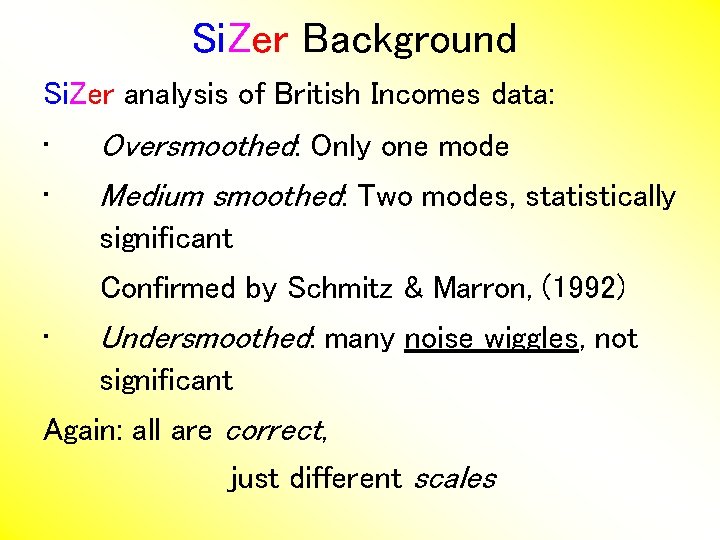
Si. Zer Background Si. Zer analysis of British Incomes data: • • Oversmoothed: Only one mode Medium smoothed: Two modes, statistically significant Confirmed by Schmitz & Marron, (1992) • Undersmoothed: many noise wiggles, not significant Again: all are correct, just different scales
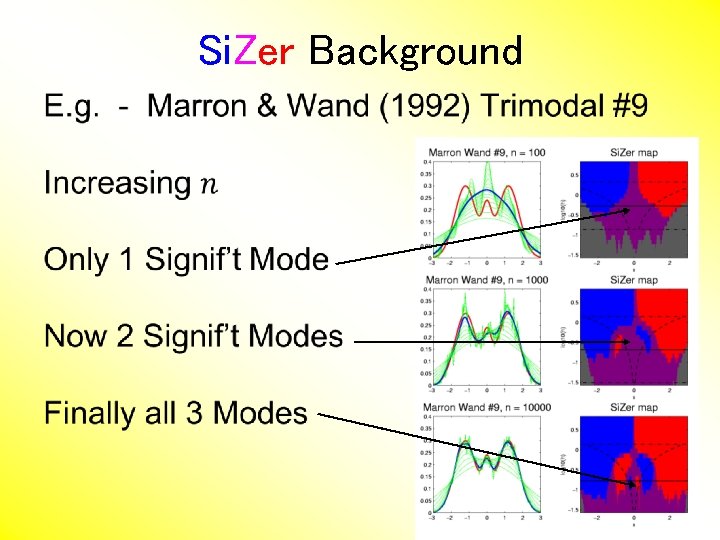
Si. Zer Background •
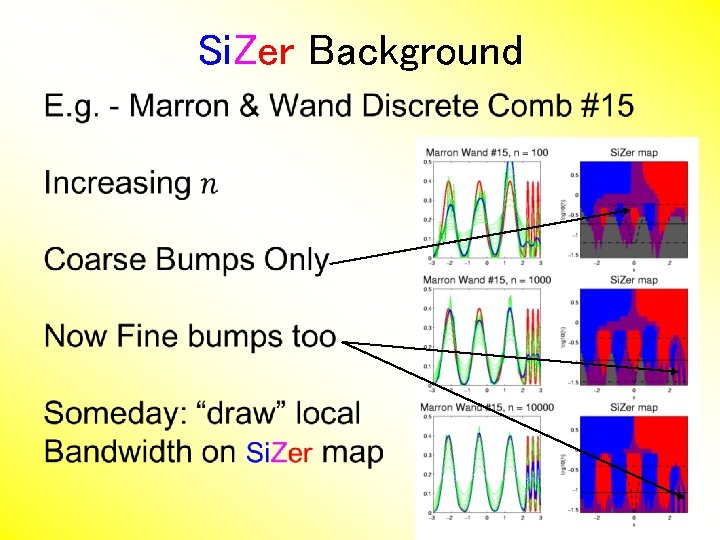
Si. Zer Background •
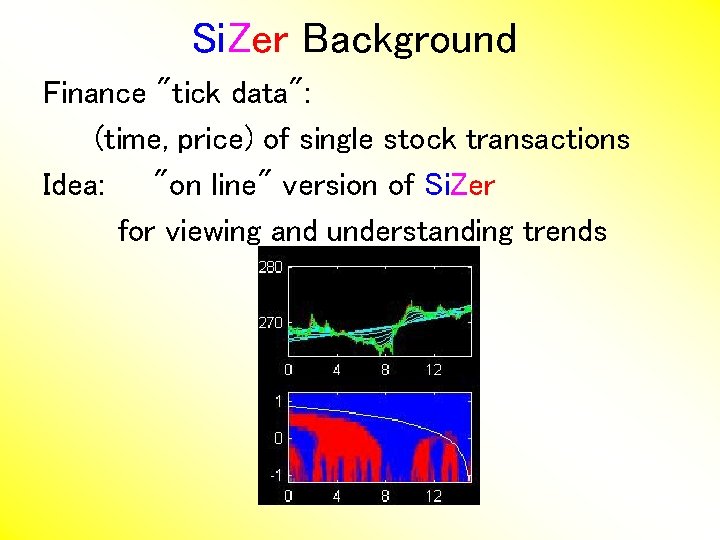
Si. Zer Background Finance "tick data": (time, price) of single stock transactions Idea: "on line" version of Si. Zer for viewing and understanding trends
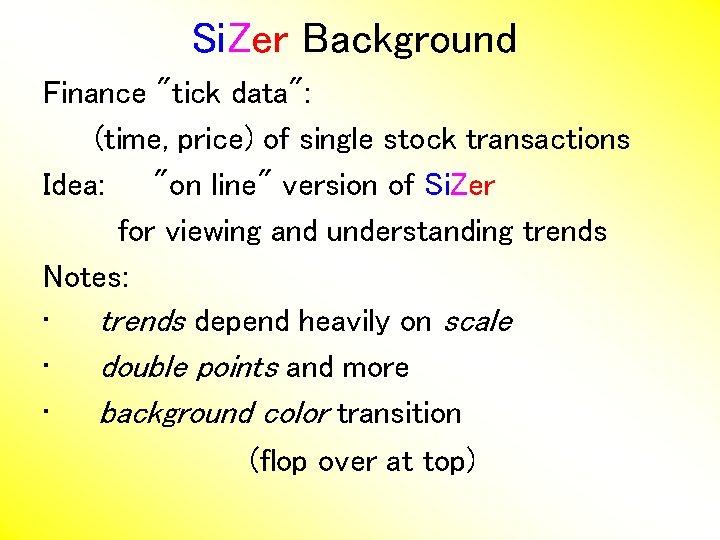
Si. Zer Background Finance "tick data": (time, price) of single stock transactions Idea: "on line" version of Si. Zer for viewing and understanding trends Notes: • trends depend heavily on scale • double points and more • background color transition (flop over at top)
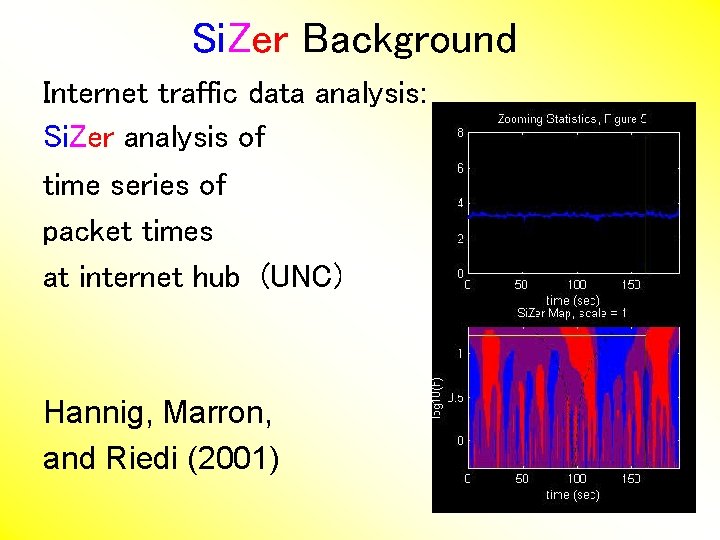
Si. Zer Background Internet traffic data analysis: Si. Zer analysis of time series of packet times at internet hub (UNC) Hannig, Marron, and Riedi (2001)
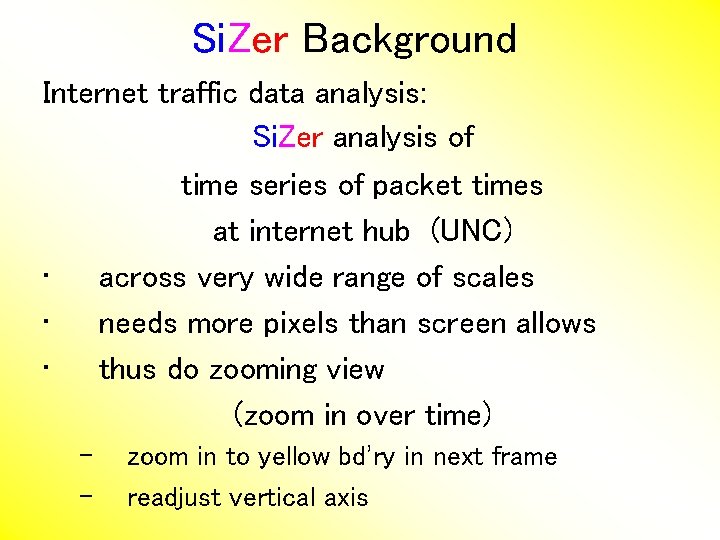
Si. Zer Background Internet traffic data analysis: Si. Zer analysis of time series of packet times at internet hub (UNC) • across very wide range of scales • needs more pixels than screen allows • thus do zooming view (zoom in over time) – – zoom in to yellow bd’ry in next frame readjust vertical axis
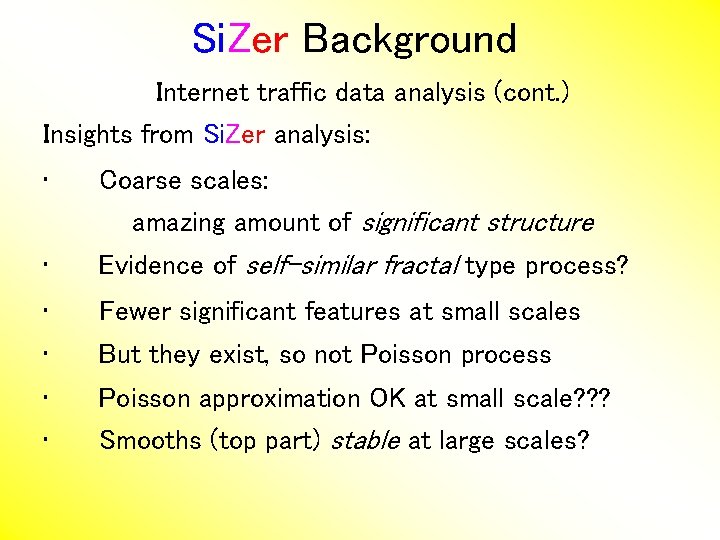
Si. Zer Background Internet traffic data analysis (cont. ) Insights from Si. Zer analysis: • Coarse scales: amazing amount of significant structure • Evidence of self-similar fractal type process? • Fewer significant features at small scales • But they exist, so not Poisson process • • Poisson approximation OK at small scale? ? ? Smooths (top part) stable at large scales?
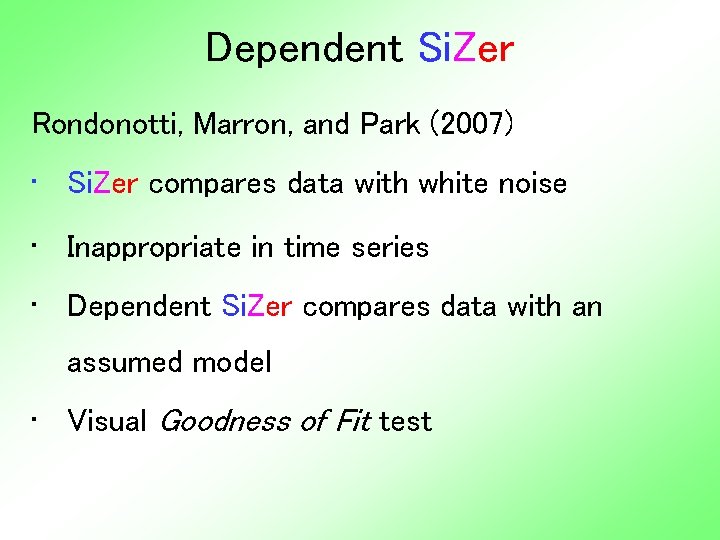
Dependent Si. Zer Rondonotti, Marron, and Park (2007) • Si. Zer compares data with white noise • Inappropriate in time series • Dependent Si. Zer compares data with an assumed model • Visual Goodness of Fit test
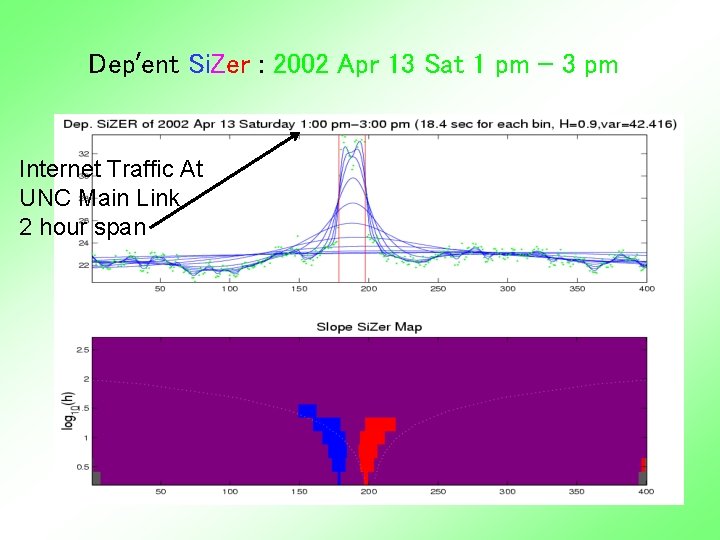
Dep’ent Si. Zer : 2002 Apr 13 Sat 1 pm – 3 pm Internet Traffic At UNC Main Link 2 hour span
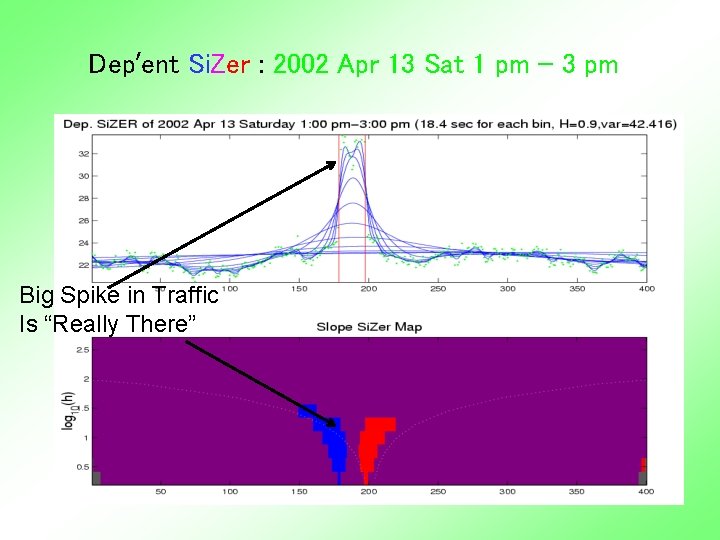
Dep’ent Si. Zer : 2002 Apr 13 Sat 1 pm – 3 pm Big Spike in Traffic Is “Really There”
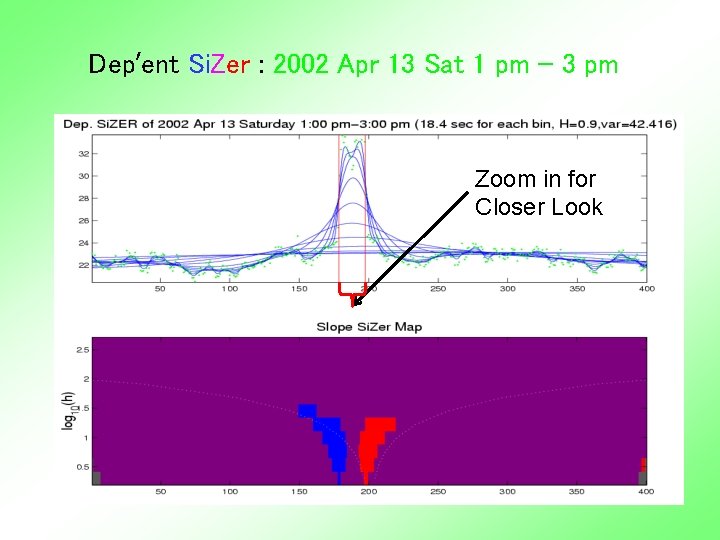
Dep’ent Si. Zer : 2002 Apr 13 Sat 1 pm – 3 pm Zoom in for Closer Look
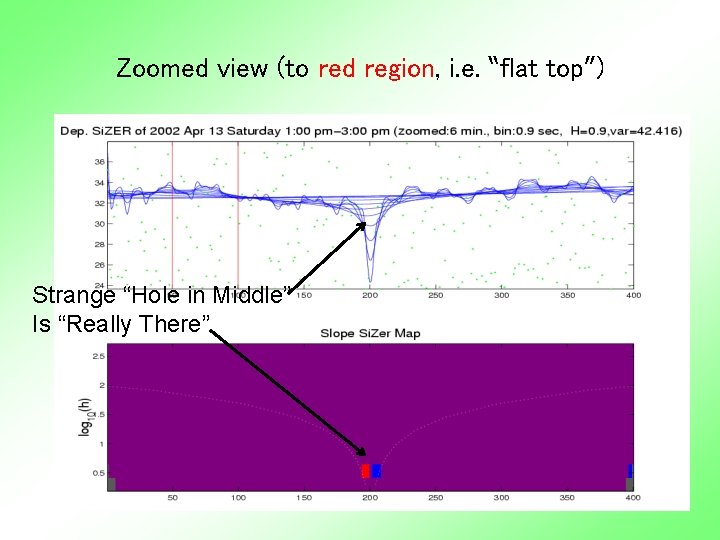
Zoomed view (to red region, i. e. “flat top”) Strange “Hole in Middle” Is “Really There”
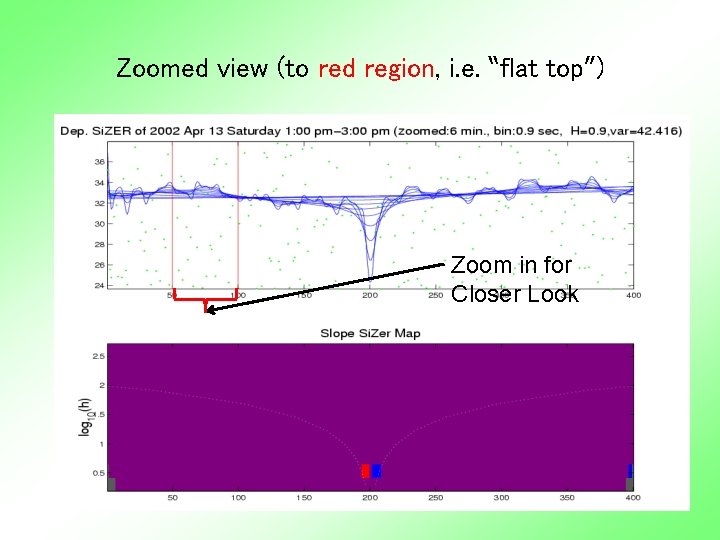
Zoomed view (to red region, i. e. “flat top”) Zoom in for Closer Look
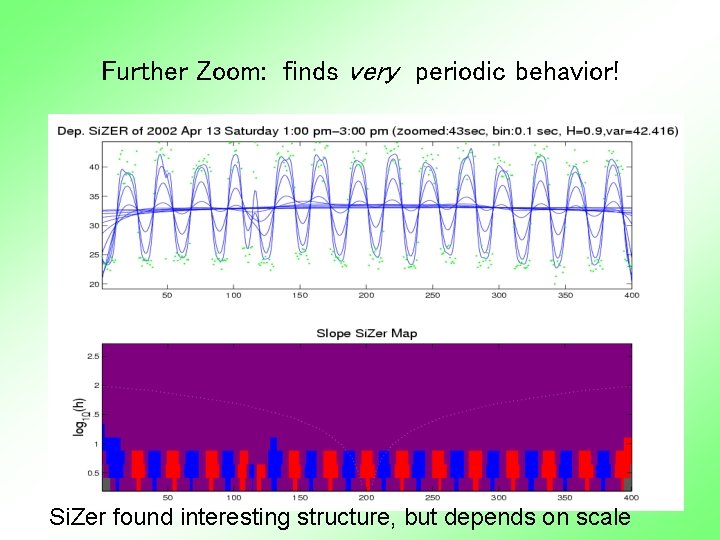
Further Zoom: finds very periodic behavior! Si. Zer found interesting structure, but depends on scale
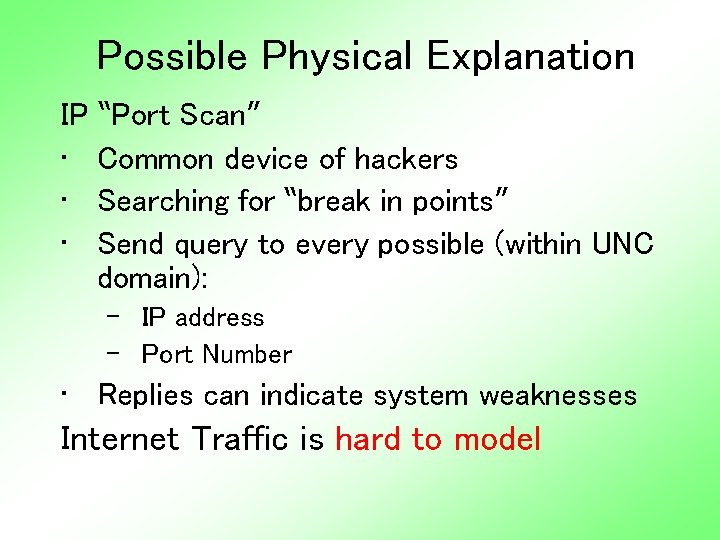
Possible Physical Explanation IP “Port Scan” • Common device of hackers • Searching for “break in points” • Send query to every possible (within UNC domain): – IP address – Port Number • Replies can indicate system weaknesses Internet Traffic is hard to model
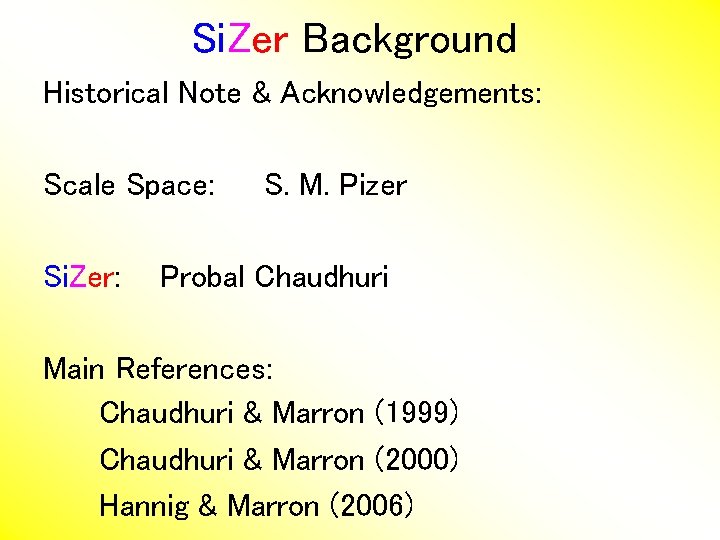
Si. Zer Background Historical Note & Acknowledgements: Scale Space: Si. Zer: S. M. Pizer Probal Chaudhuri Main References: Chaudhuri & Marron (1999) Chaudhuri & Marron (2000) Hannig & Marron (2006)
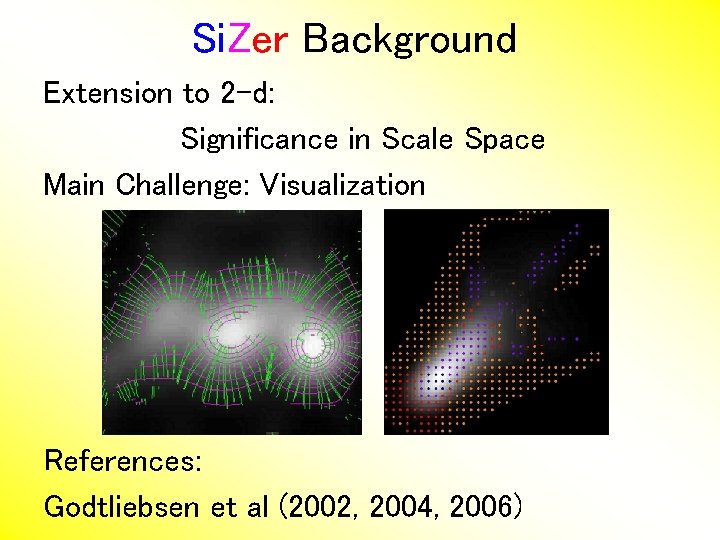
Si. Zer Background Extension to 2 -d: Significance in Scale Space Main Challenge: Visualization References: Godtliebsen et al (2002, 2004, 2006)
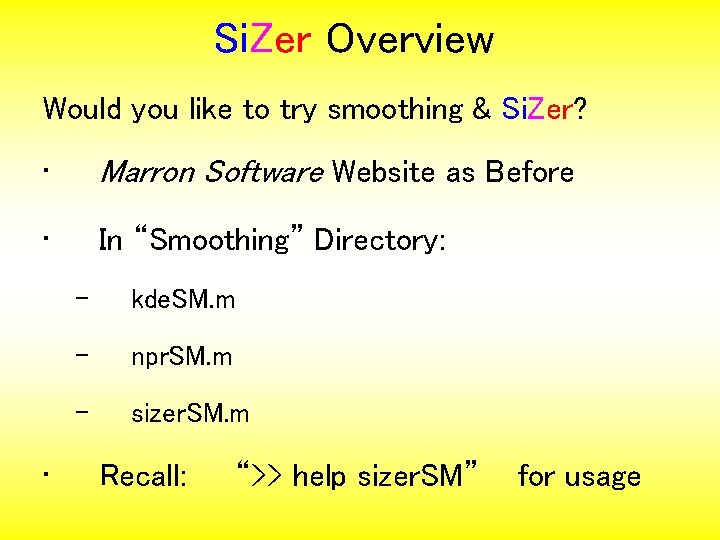
Si. Zer Overview Would you like to try smoothing & Si. Zer? • Marron Software Website as Before • In “Smoothing” Directory: • – kde. SM. m – npr. SM. m – sizer. SM. m Recall: “>> help sizer. SM” for usage
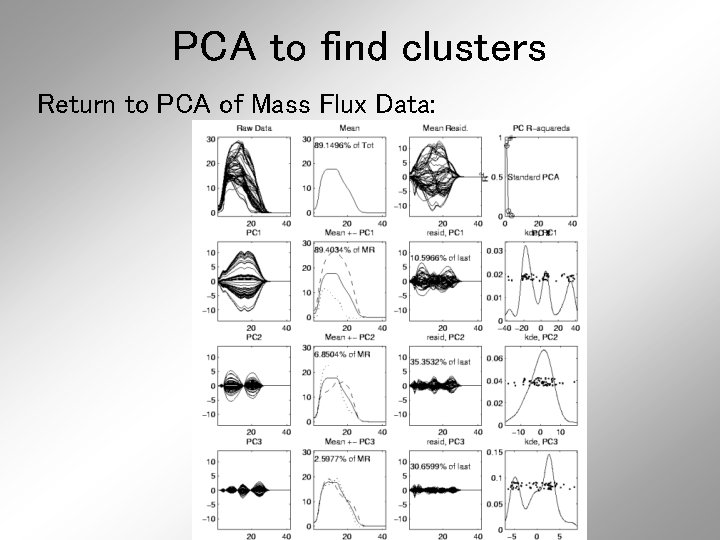
PCA to find clusters Return to PCA of Mass Flux Data:
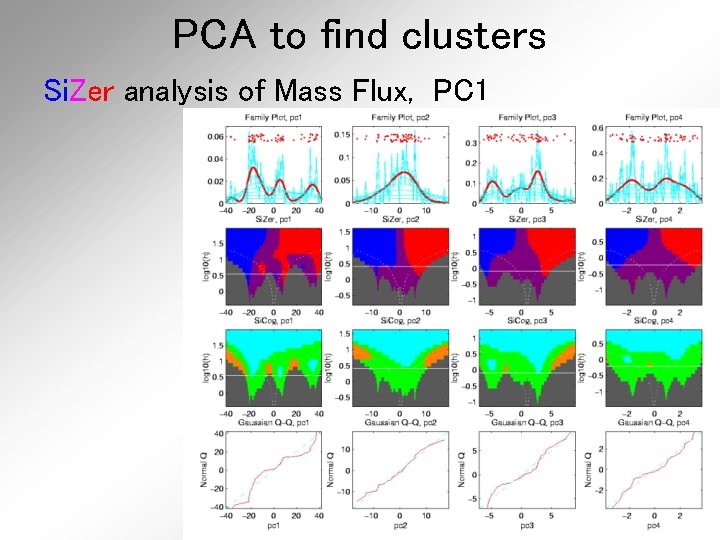
PCA to find clusters Si. Zer analysis of Mass Flux, PC 1
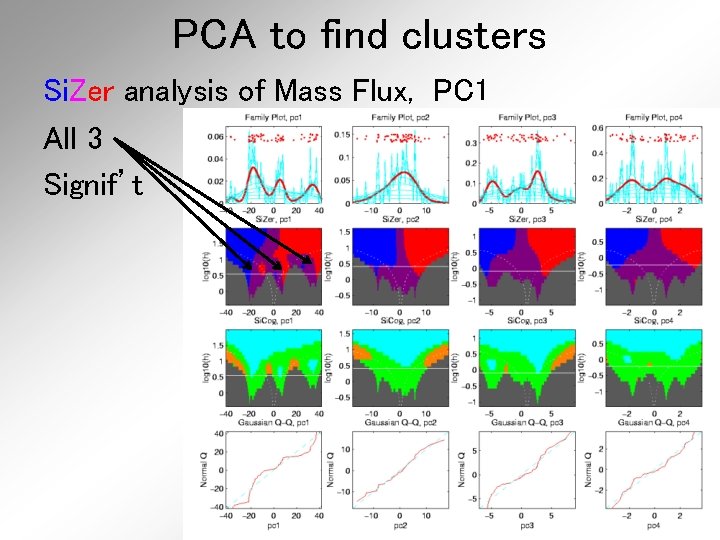
PCA to find clusters Si. Zer analysis of Mass Flux, PC 1 All 3 Signif’t
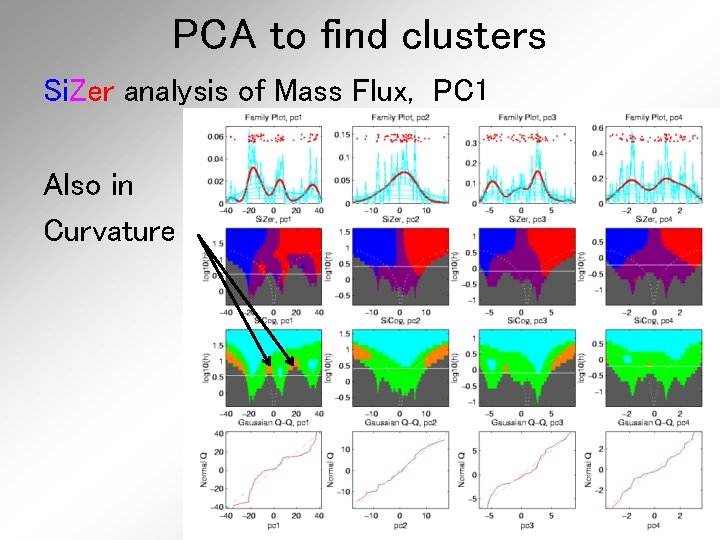
PCA to find clusters Si. Zer analysis of Mass Flux, PC 1 Also in Curvature
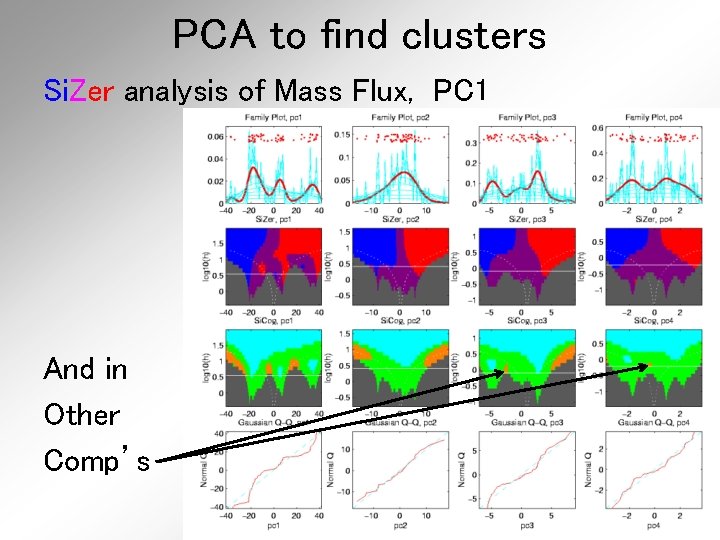
PCA to find clusters Si. Zer analysis of Mass Flux, PC 1 And in Other Comp’s
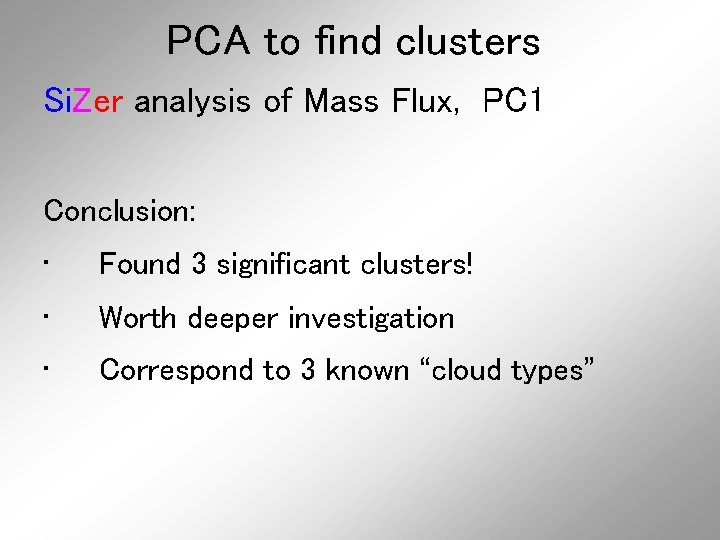
PCA to find clusters Si. Zer analysis of Mass Flux, PC 1 Conclusion: • Found 3 significant clusters! • Worth deeper investigation • Correspond to 3 known “cloud types”
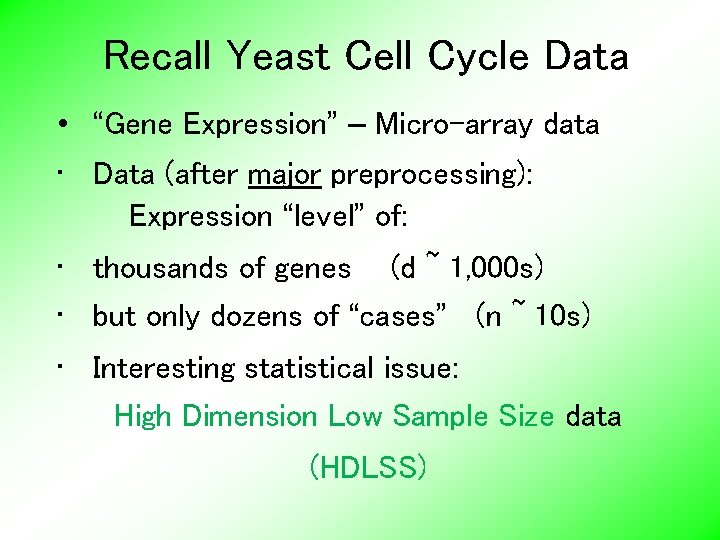
Recall Yeast Cell Cycle Data • “Gene Expression” – Micro-array data • Data (after major preprocessing): Expression “level” of: • thousands of genes (d ~ 1, 000 s) • but only dozens of “cases” (n ~ 10 s) • Interesting statistical issue: High Dimension Low Sample Size data (HDLSS)
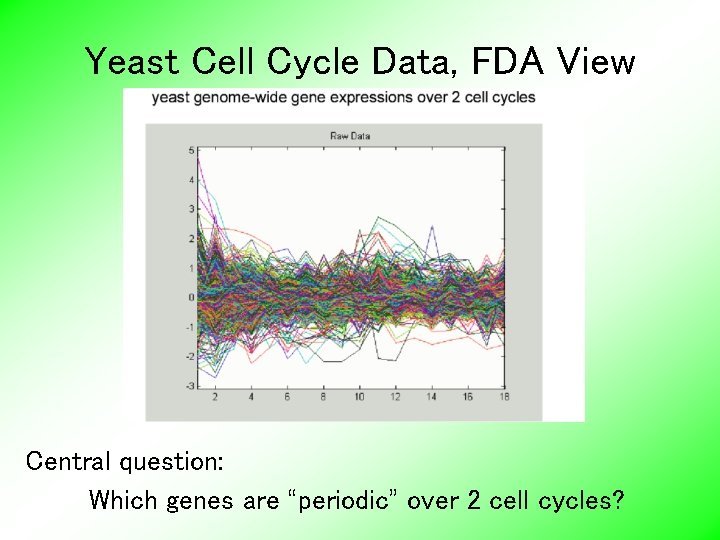
Yeast Cell Cycle Data, FDA View Central question: Which genes are “periodic” over 2 cell cycles?
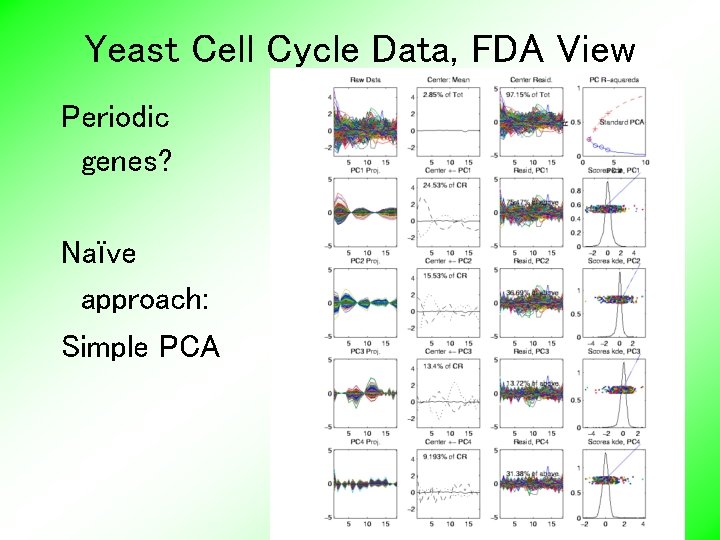
Yeast Cell Cycle Data, FDA View Periodic genes? Naïve approach: Simple PCA
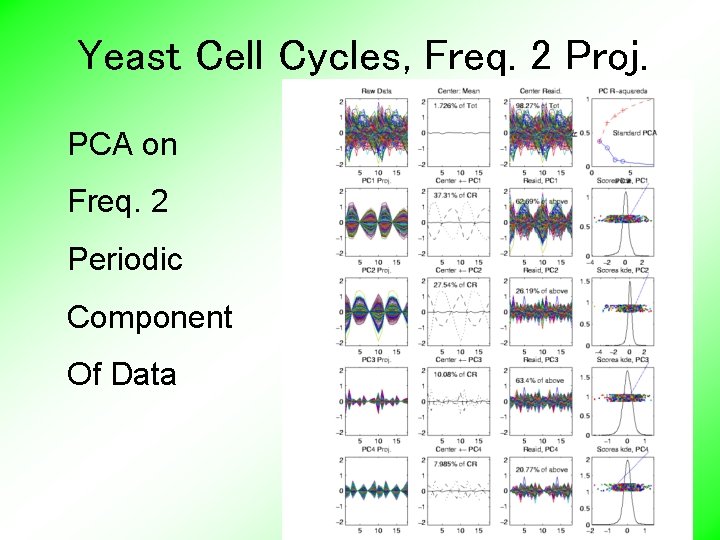
Yeast Cell Cycles, Freq. 2 Proj. PCA on Freq. 2 Periodic Component Of Data
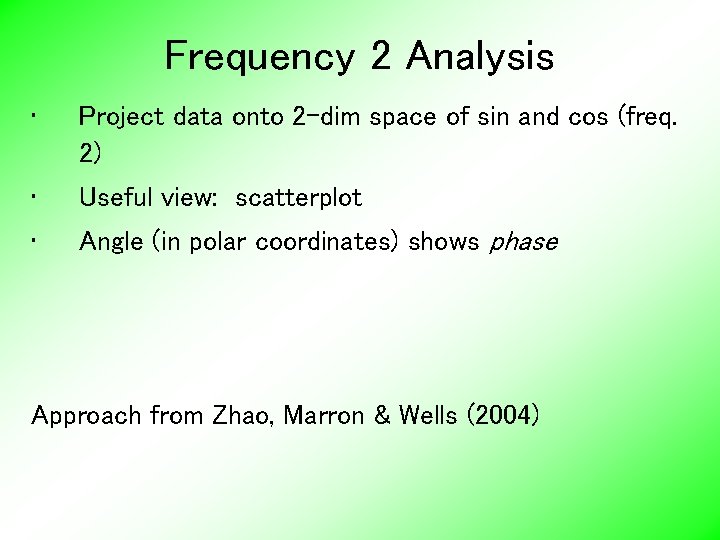
Frequency 2 Analysis • Project data onto 2 -dim space of sin and cos (freq. 2) • Useful view: scatterplot • Angle (in polar coordinates) shows phase Approach from Zhao, Marron & Wells (2004)
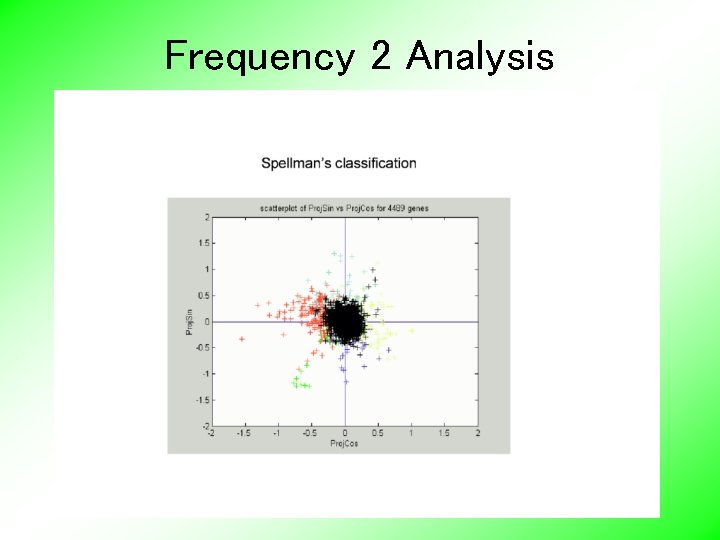
Frequency 2 Analysis
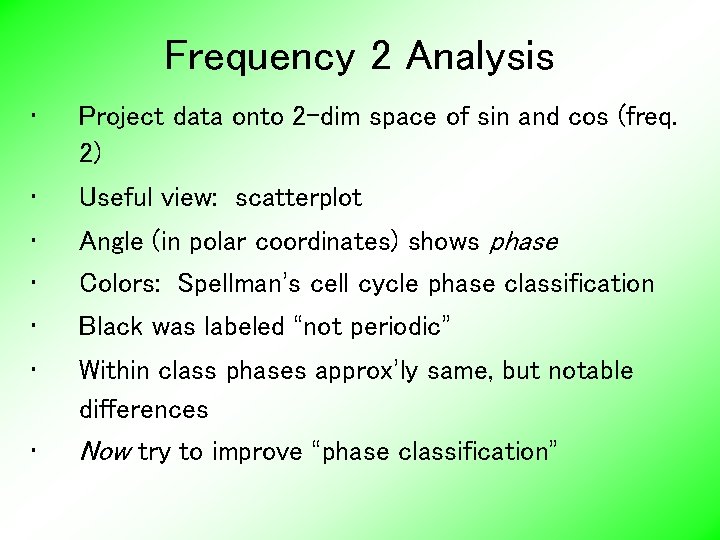
Frequency 2 Analysis • Project data onto 2 -dim space of sin and cos (freq. 2) • Useful view: scatterplot • • Angle (in polar coordinates) shows phase Colors: Spellman’s cell cycle phase classification • Black was labeled “not periodic” • Within class phases approx’ly same, but notable differences Now try to improve “phase classification” •
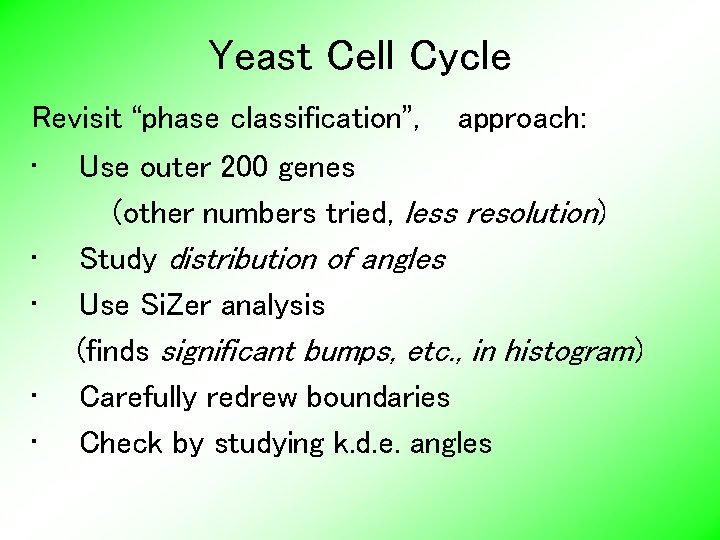
Yeast Cell Cycle Revisit “phase classification”, • • • approach: Use outer 200 genes (other numbers tried, less resolution) Study distribution of angles Use Si. Zer analysis (finds significant bumps, etc. , in histogram) Carefully redrew boundaries Check by studying k. d. e. angles
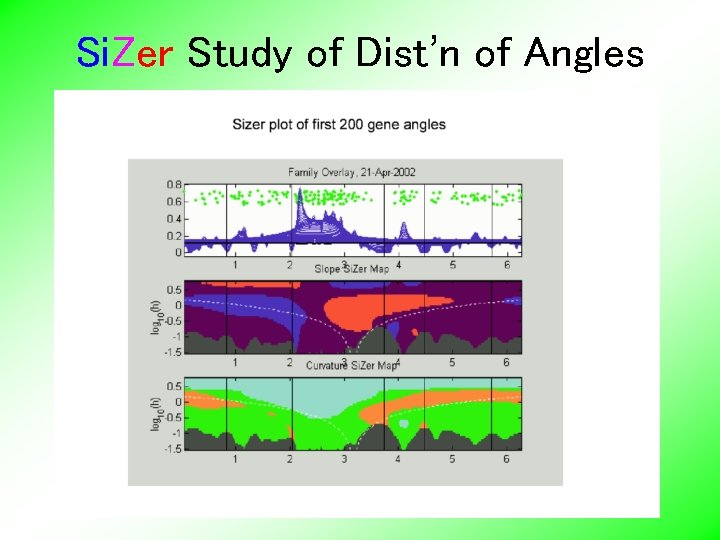
Si. Zer Study of Dist’n of Angles
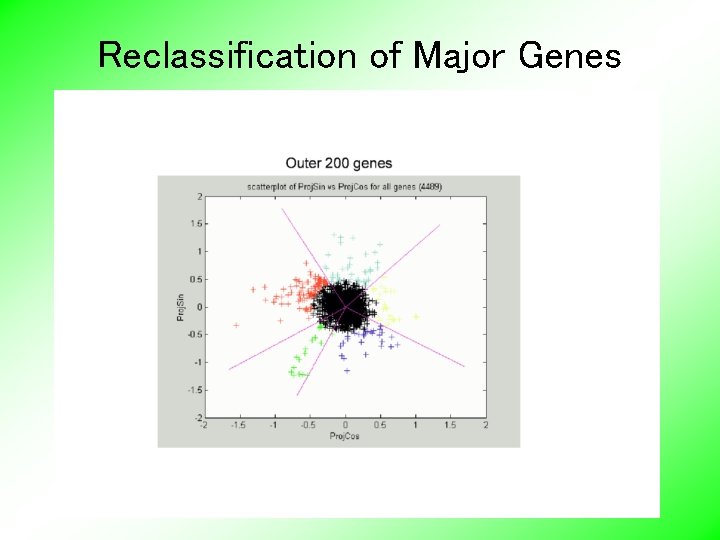
Reclassification of Major Genes
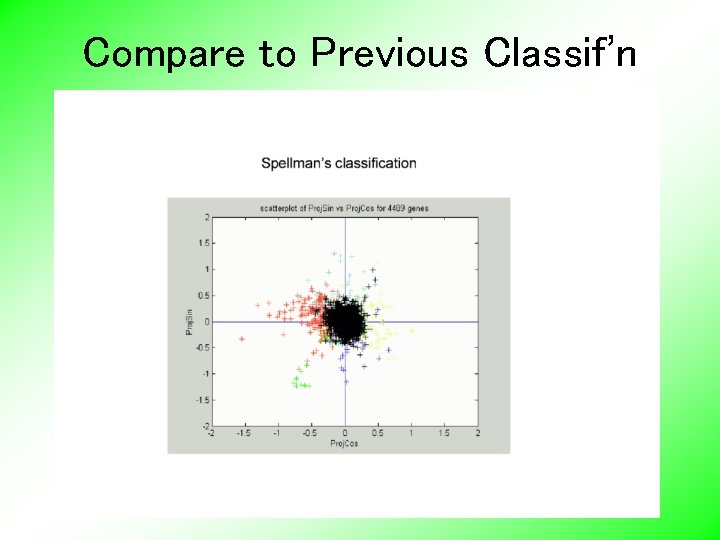
Compare to Previous Classif’n
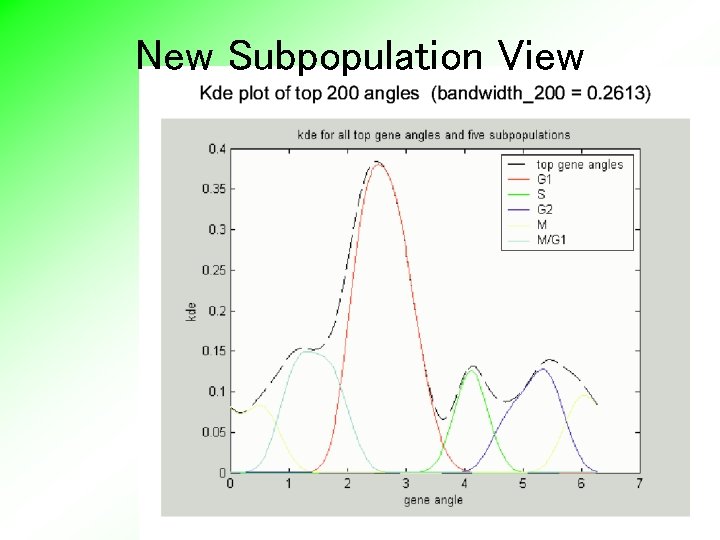
New Subpopulation View
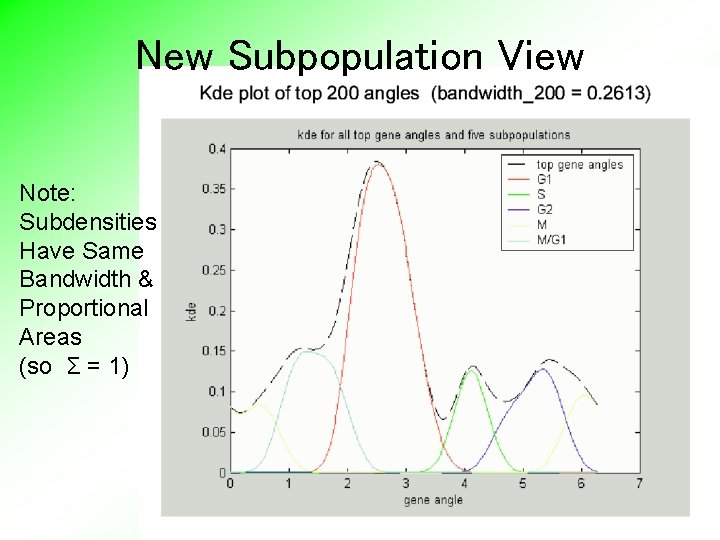
New Subpopulation View Note: Subdensities Have Same Bandwidth & Proportional Areas (so Σ = 1)
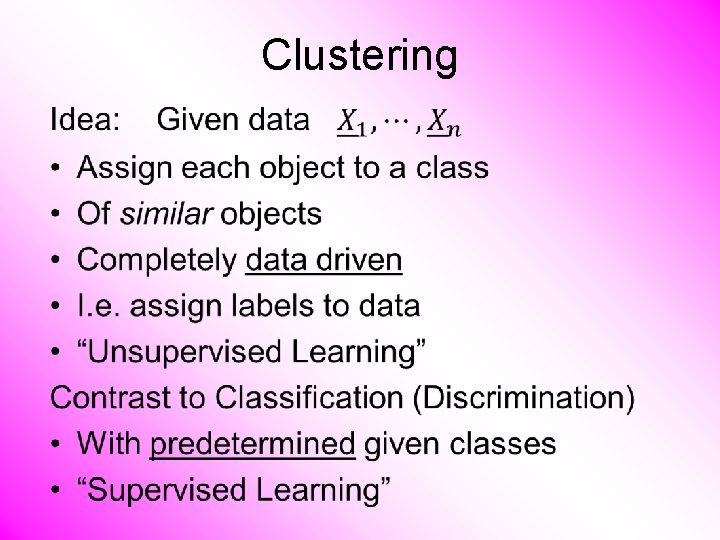
Clustering •
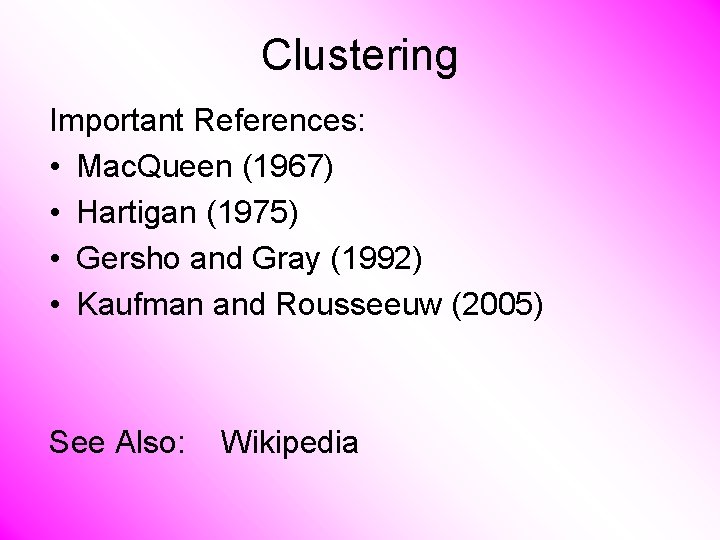
Clustering Important References: • Mac. Queen (1967) • Hartigan (1975) • Gersho and Gray (1992) • Kaufman and Rousseeuw (2005) See Also: Wikipedia
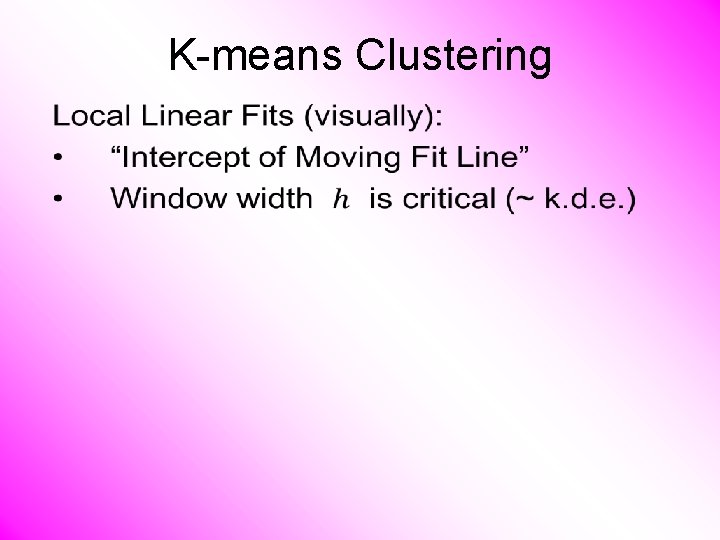
K-means Clustering •
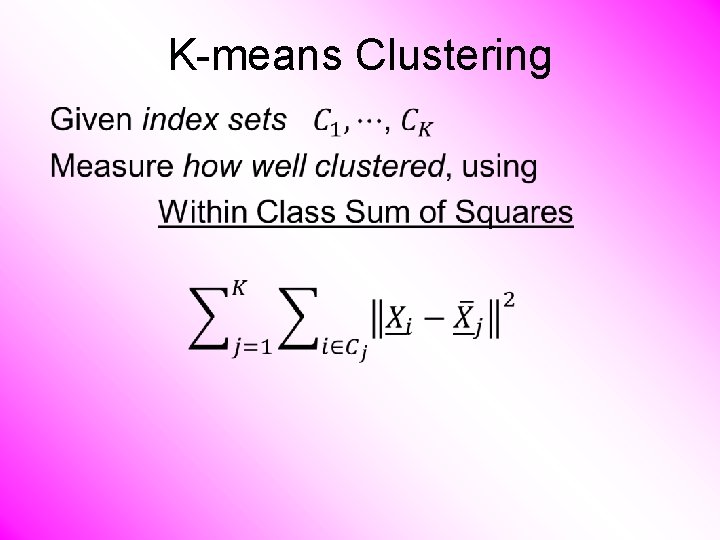
K-means Clustering •
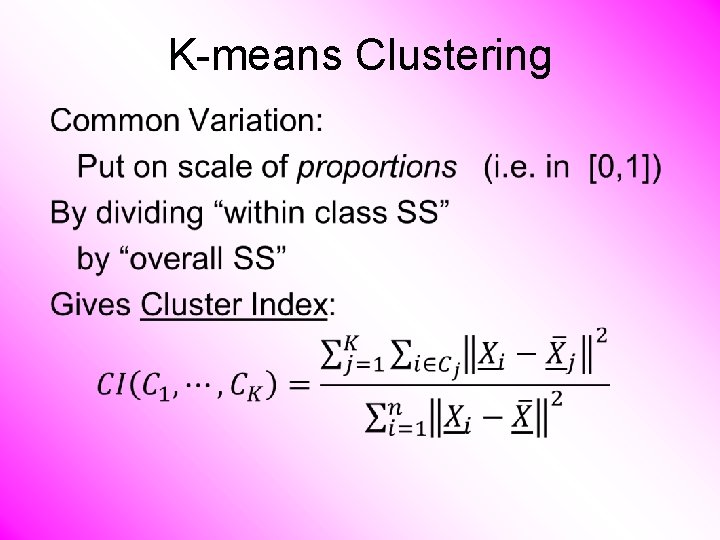
K-means Clustering •
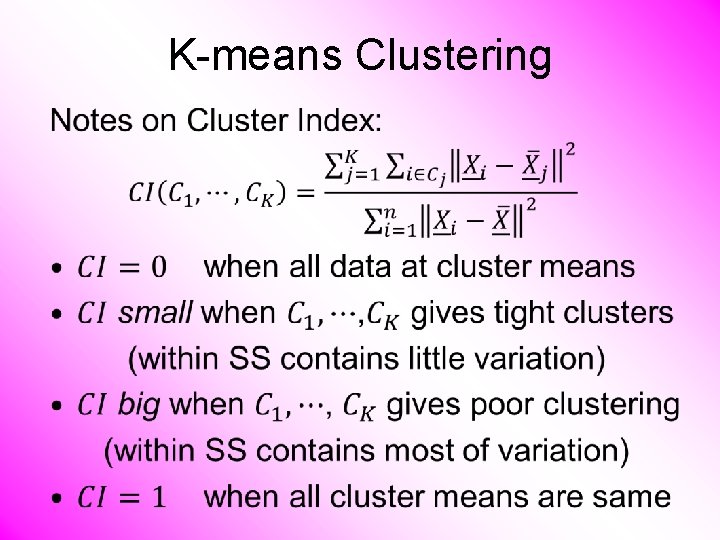
K-means Clustering •
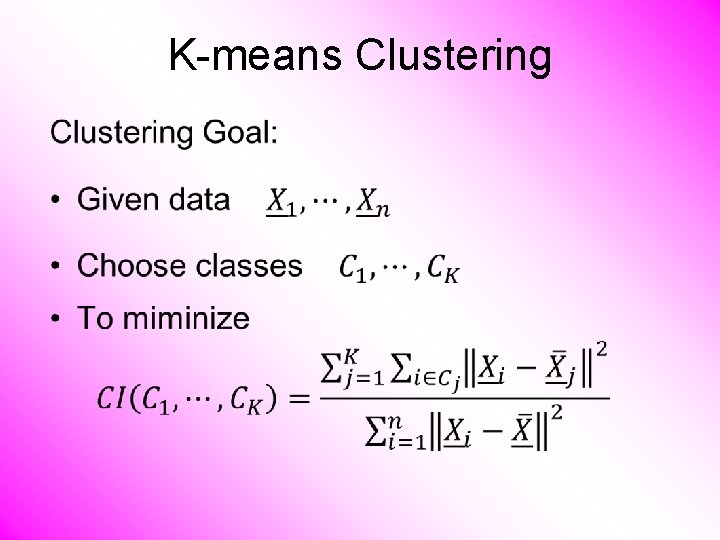
K-means Clustering •
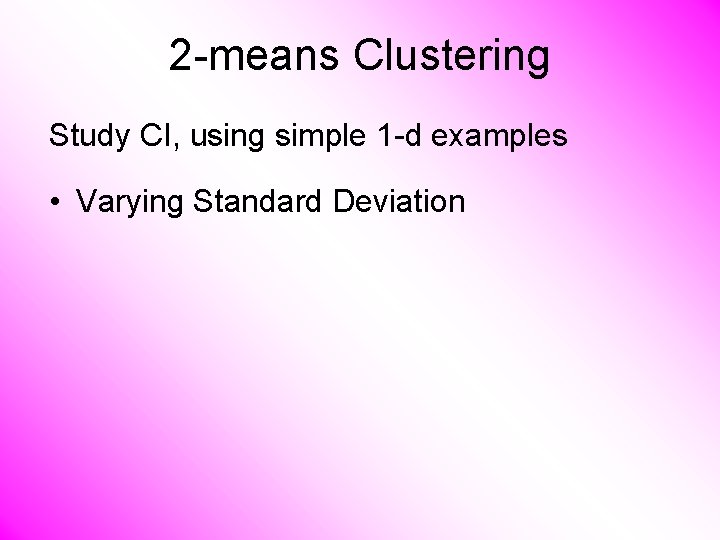
2 -means Clustering Study CI, using simple 1 -d examples • Varying Standard Deviation
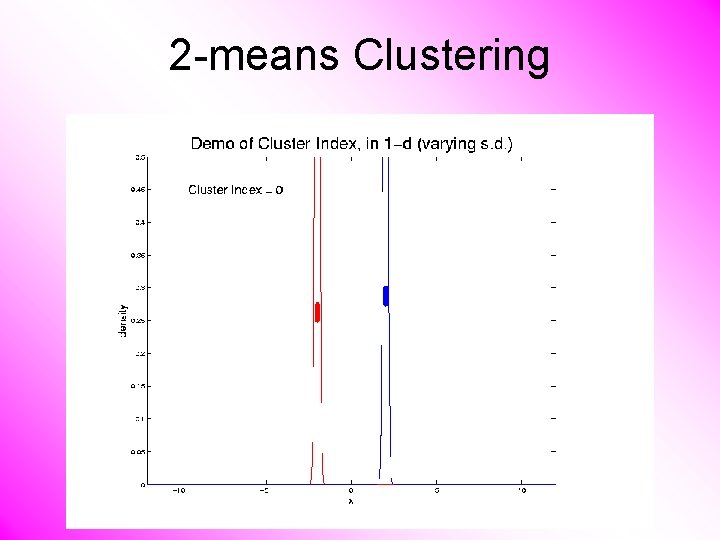
2 -means Clustering
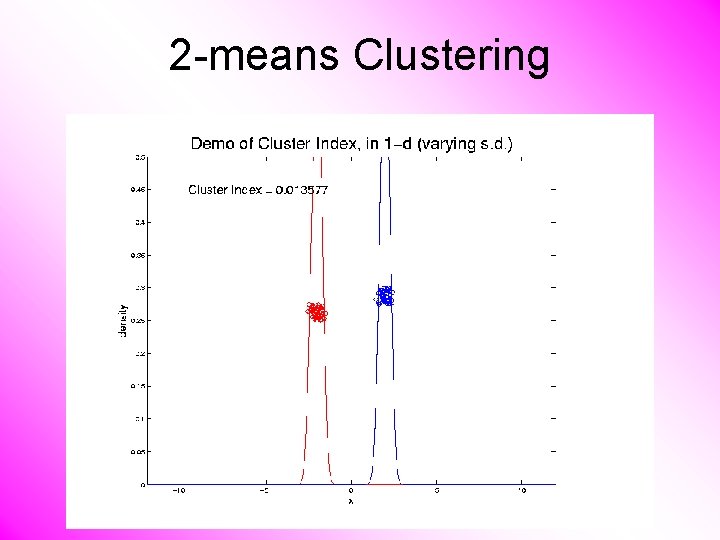
2 -means Clustering
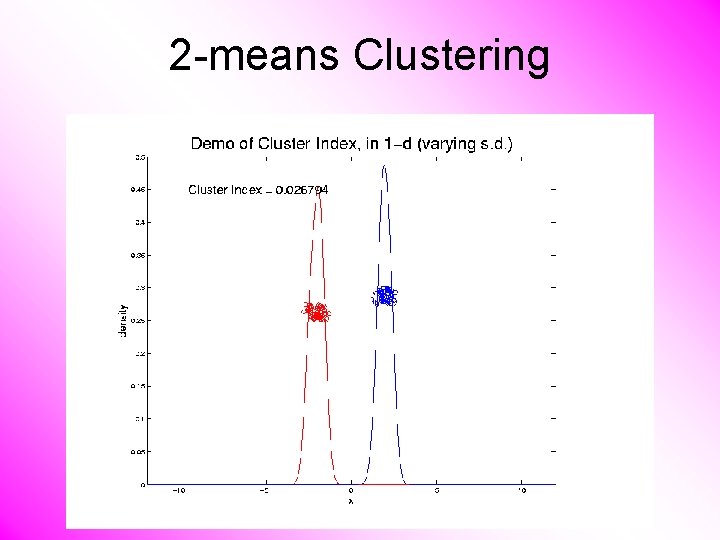
2 -means Clustering
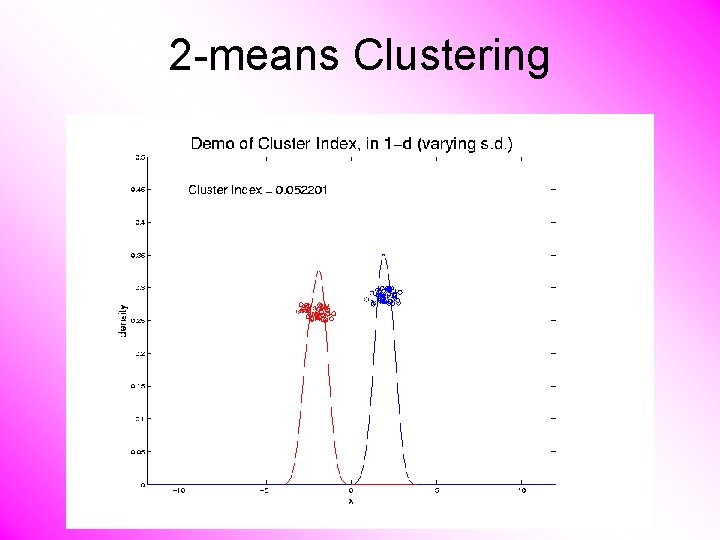
2 -means Clustering
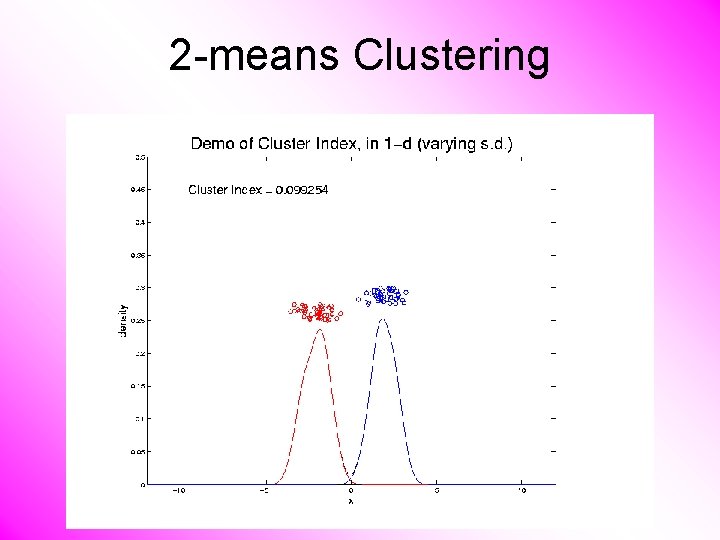
2 -means Clustering
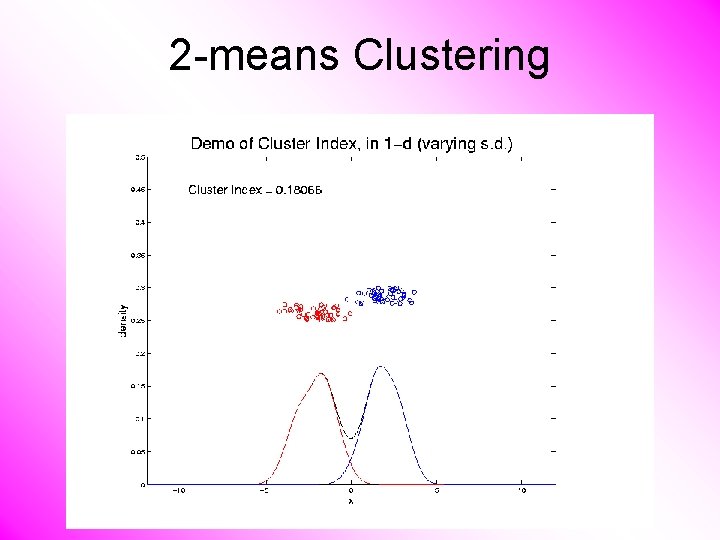
2 -means Clustering
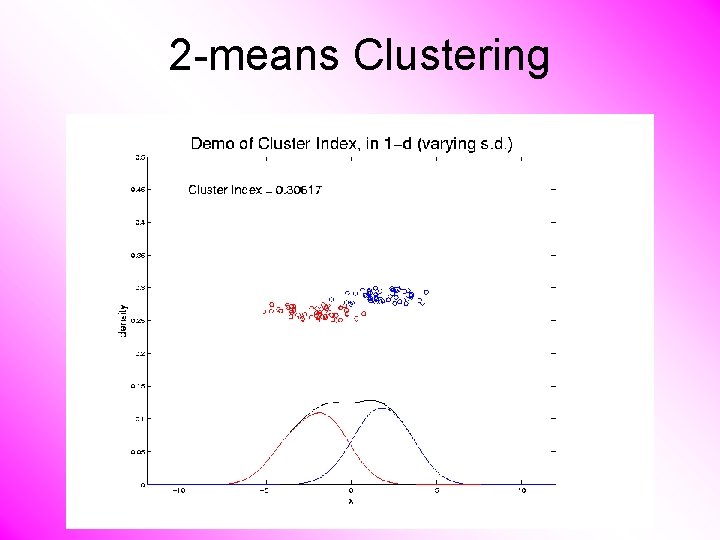
2 -means Clustering
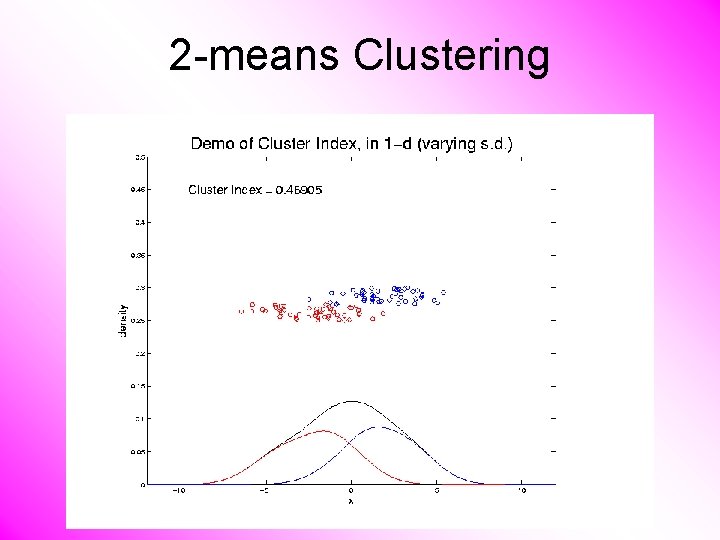
2 -means Clustering
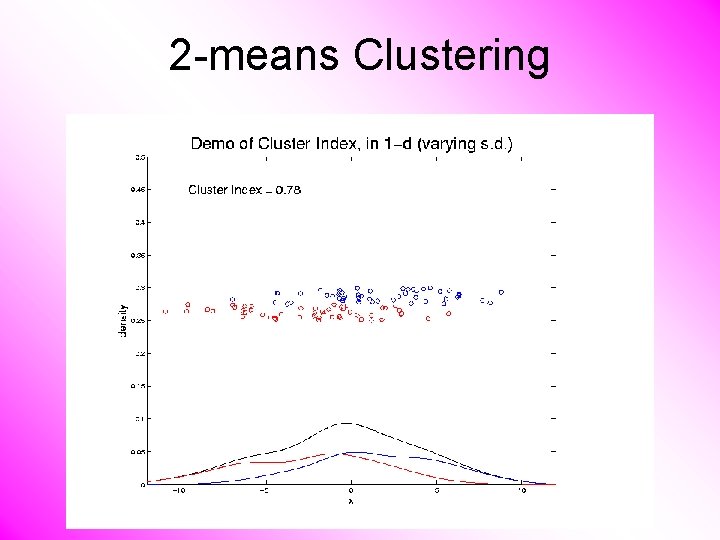
2 -means Clustering
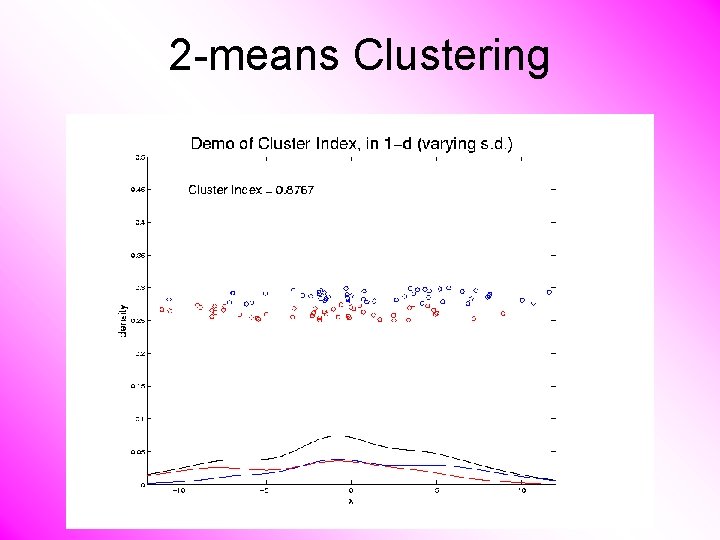
2 -means Clustering
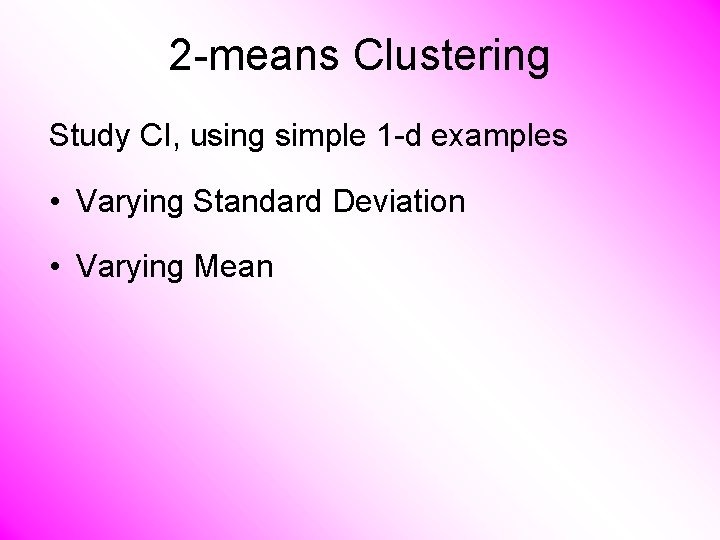
2 -means Clustering Study CI, using simple 1 -d examples • Varying Standard Deviation • Varying Mean
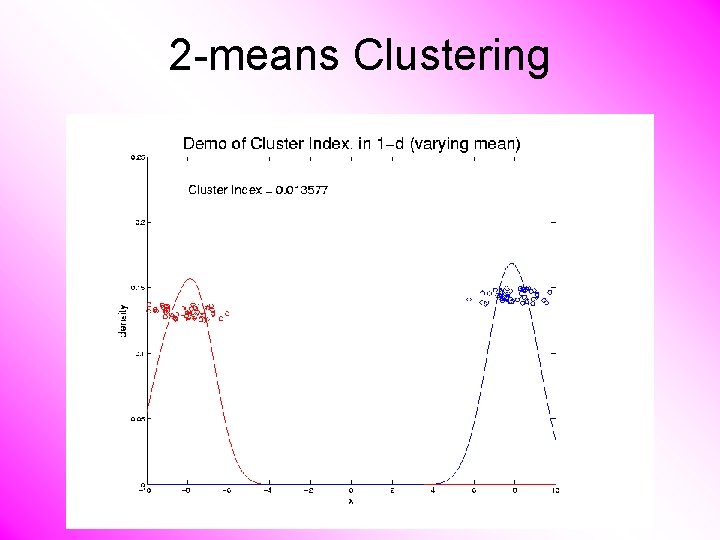
2 -means Clustering
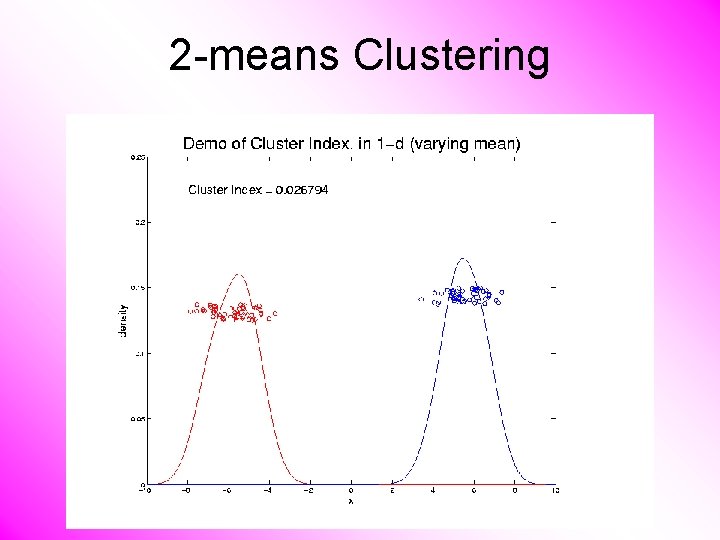
2 -means Clustering
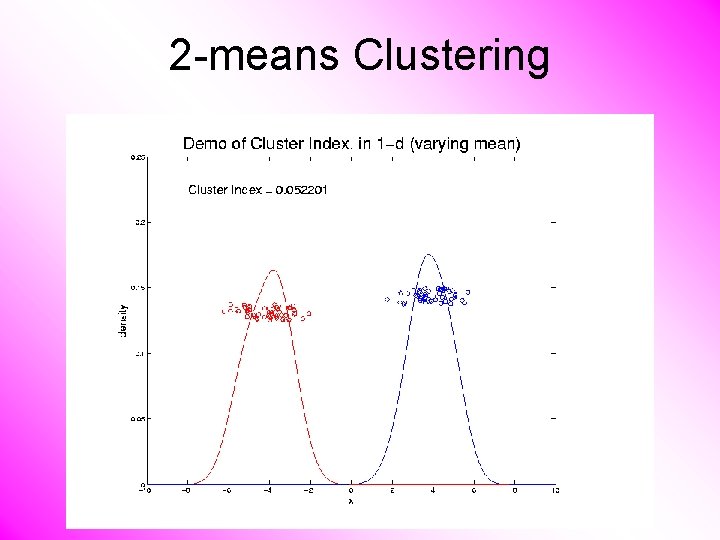
2 -means Clustering
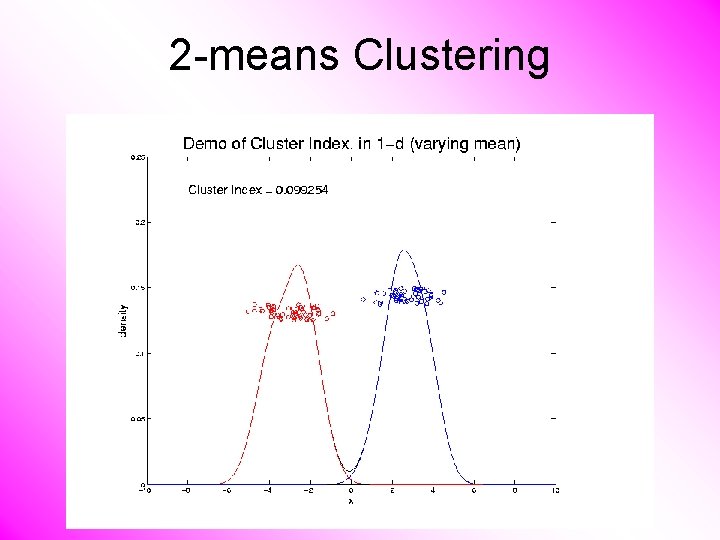
2 -means Clustering
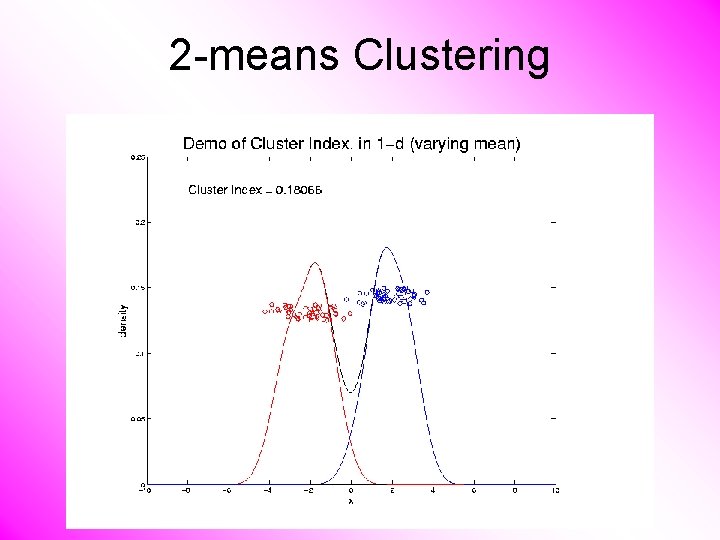
2 -means Clustering
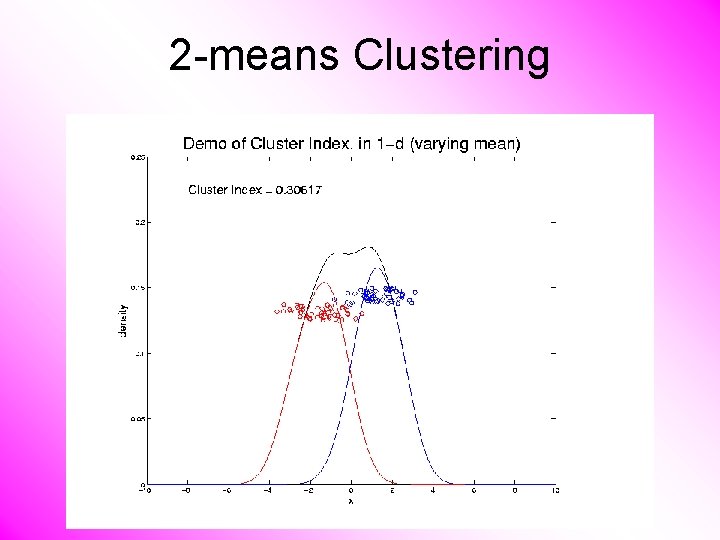
2 -means Clustering
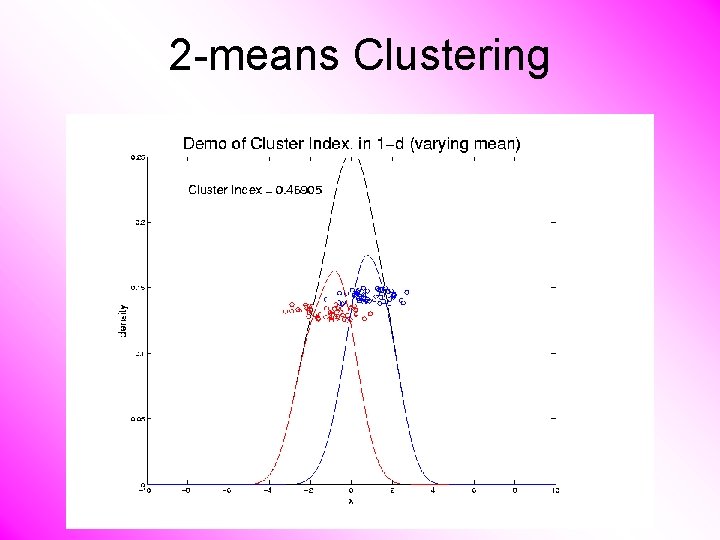
2 -means Clustering
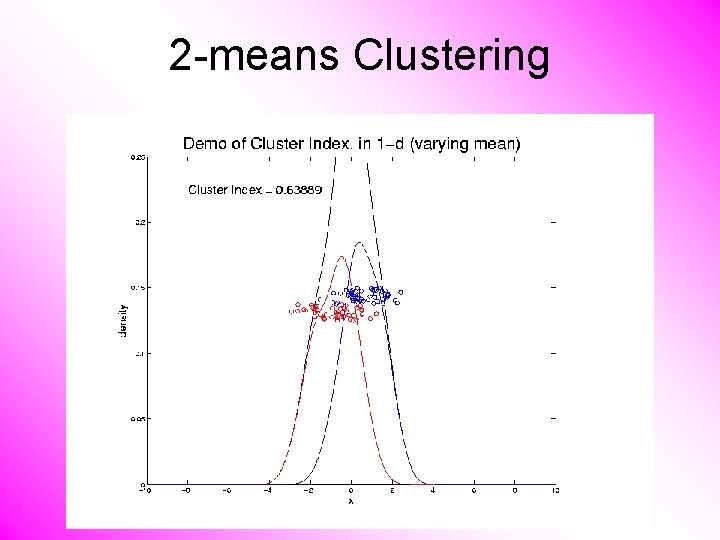
2 -means Clustering
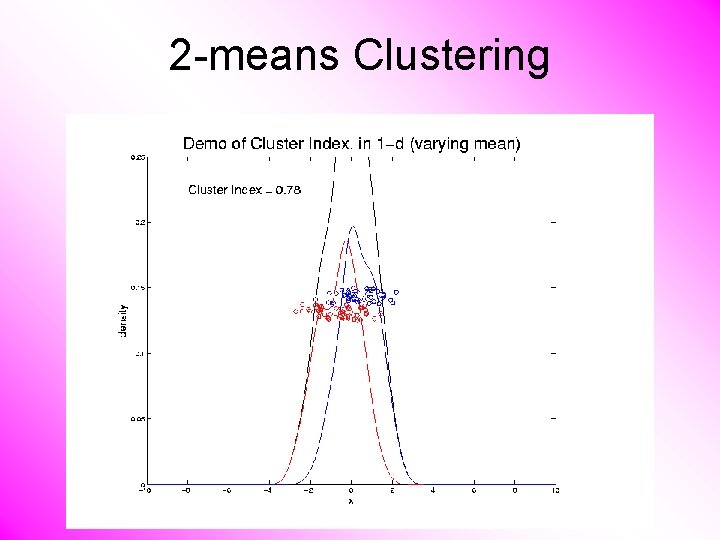
2 -means Clustering
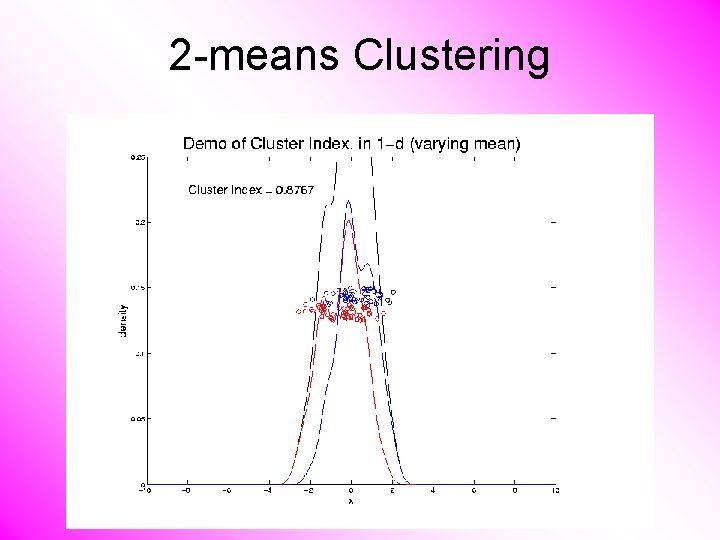
2 -means Clustering
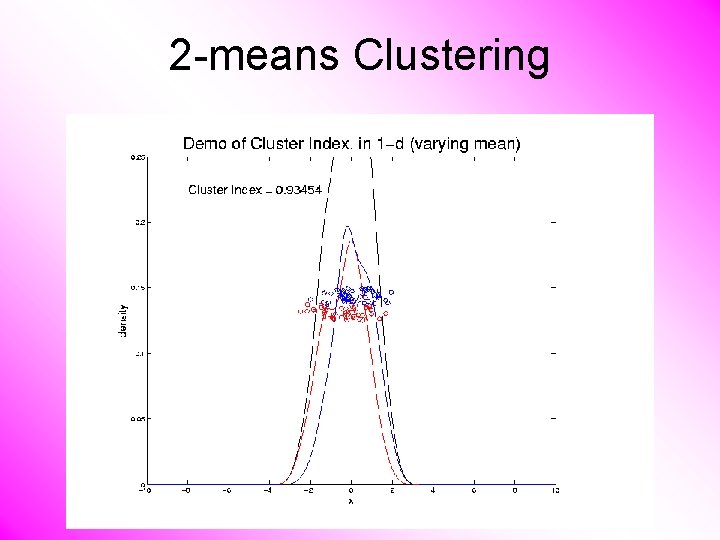
2 -means Clustering
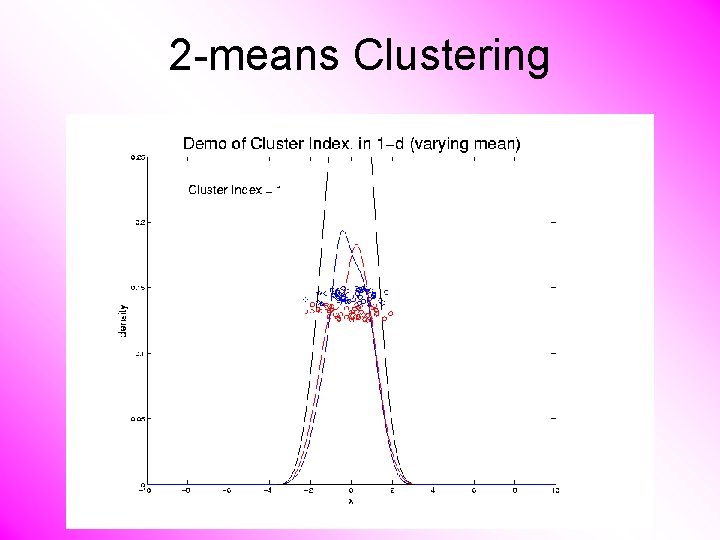
2 -means Clustering
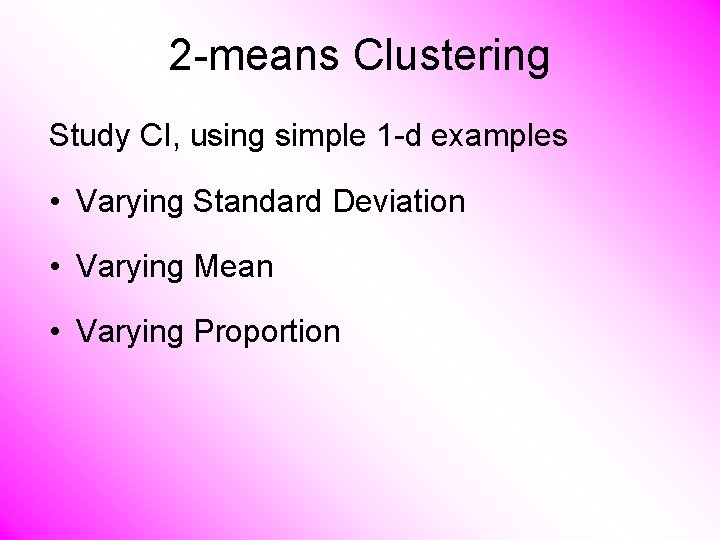
2 -means Clustering Study CI, using simple 1 -d examples • Varying Standard Deviation • Varying Mean • Varying Proportion
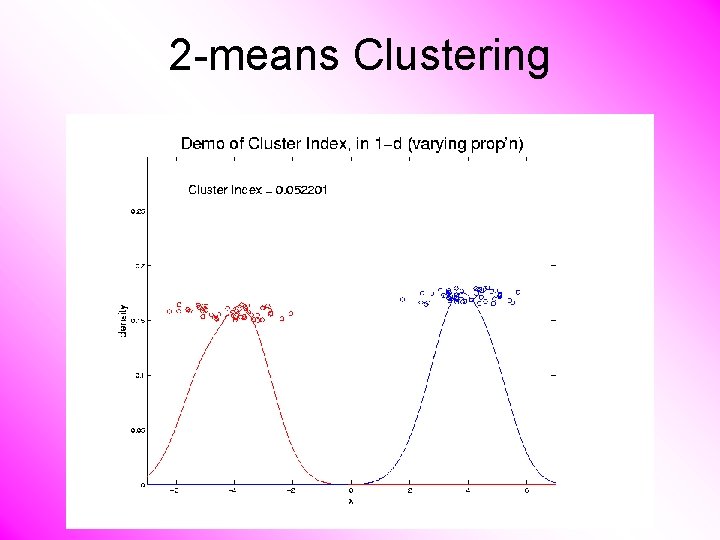
2 -means Clustering
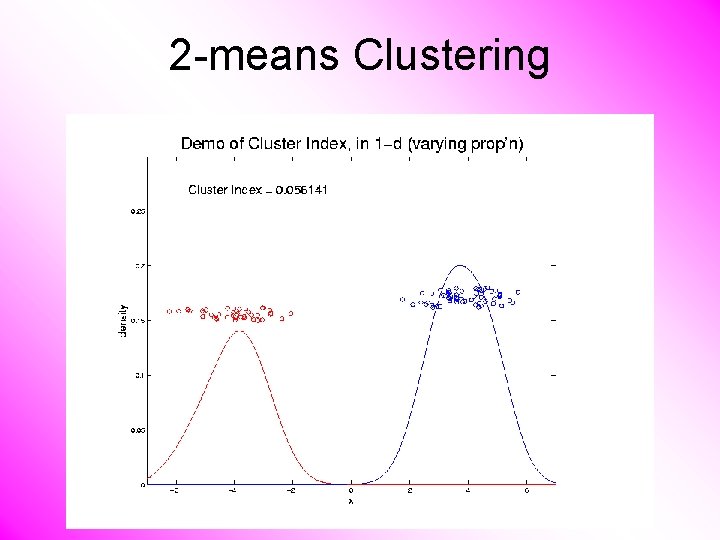
2 -means Clustering
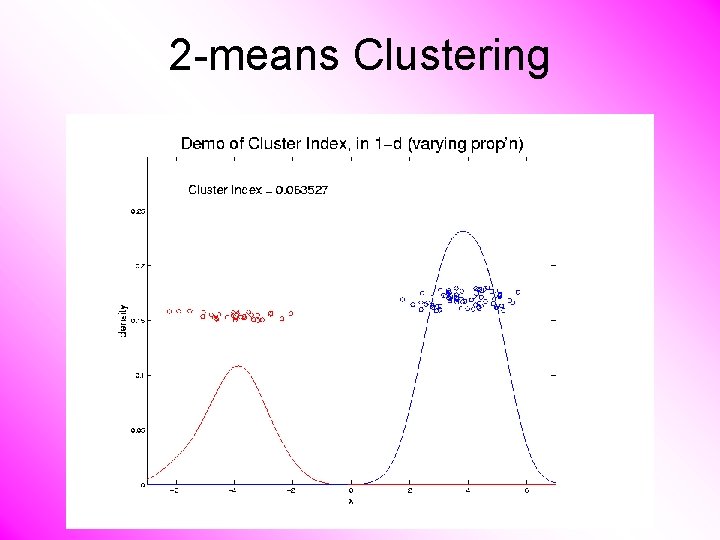
2 -means Clustering
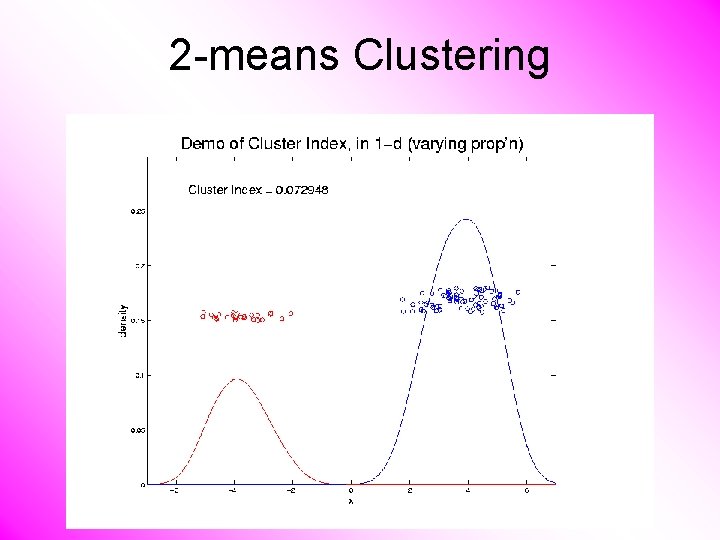
2 -means Clustering
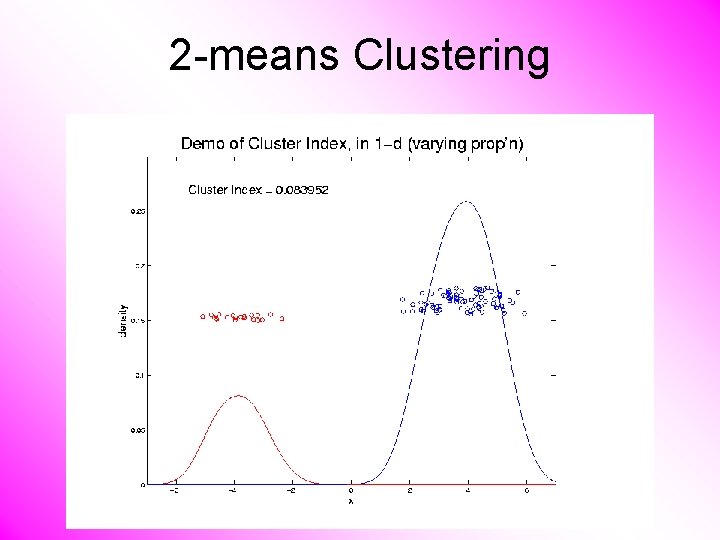
2 -means Clustering
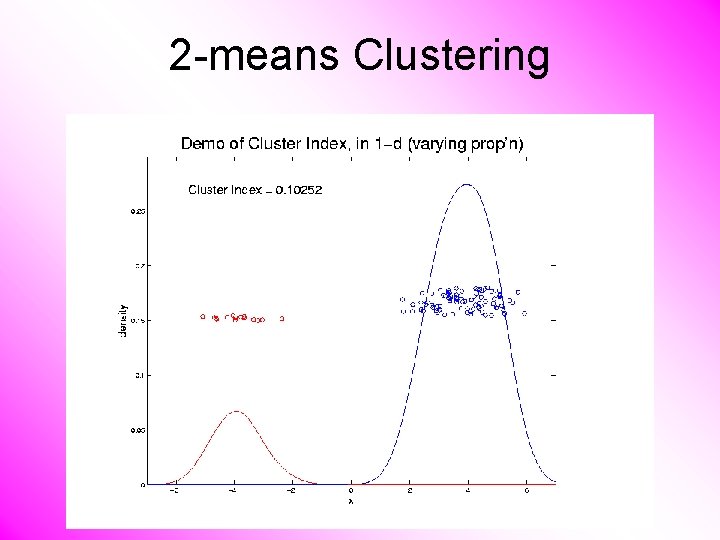
2 -means Clustering
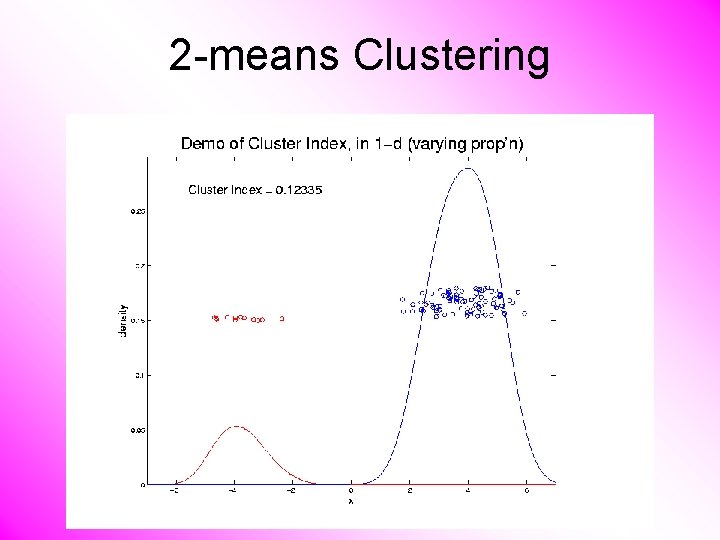
2 -means Clustering
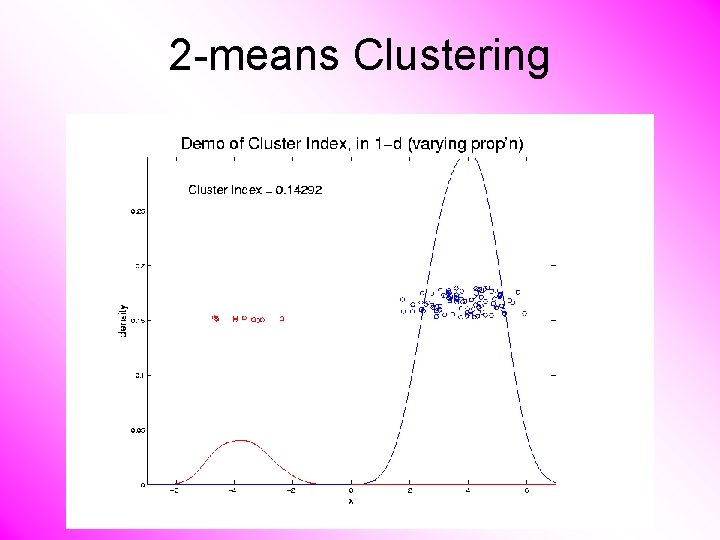
2 -means Clustering
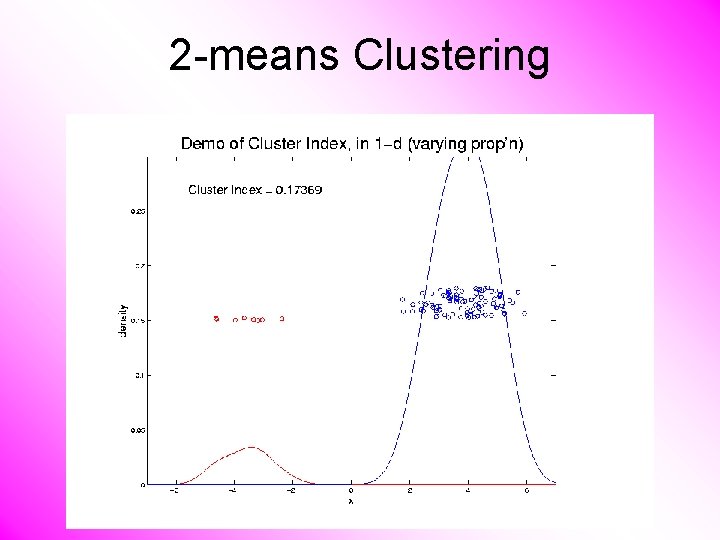
2 -means Clustering
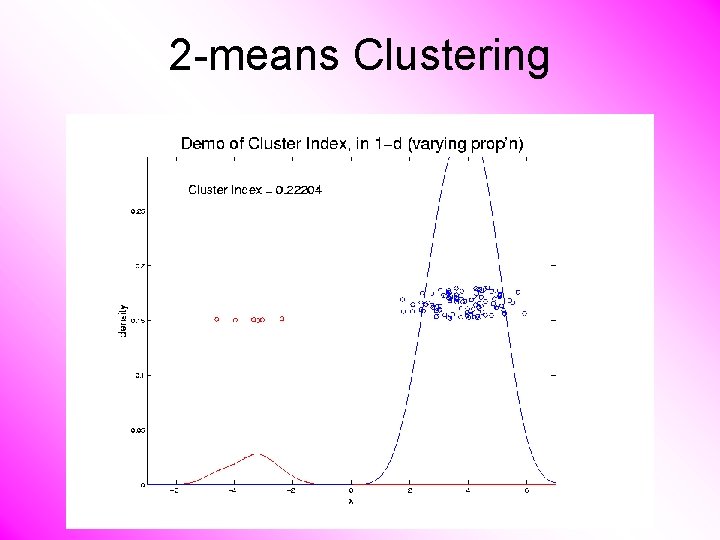
2 -means Clustering
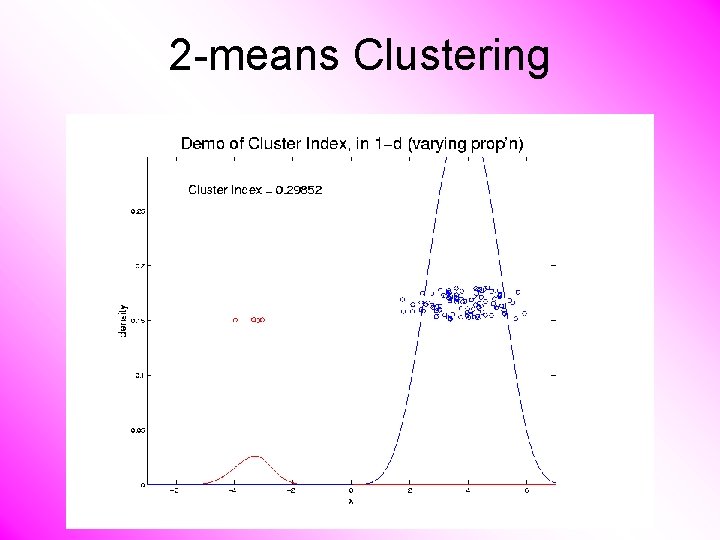
2 -means Clustering
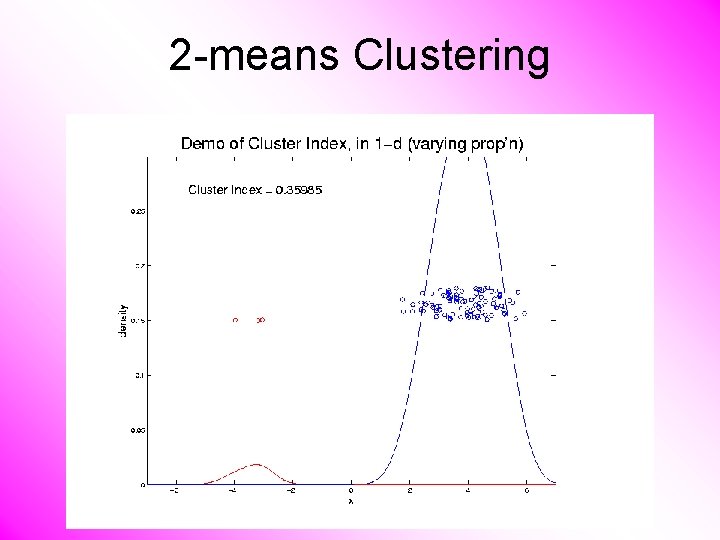
2 -means Clustering
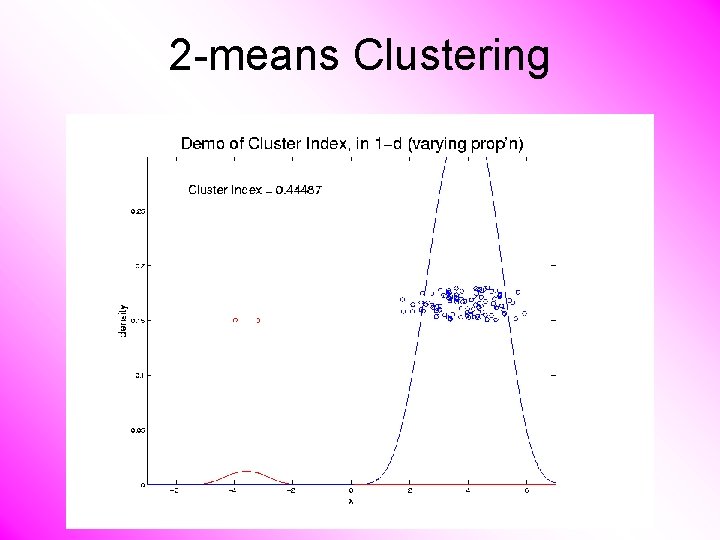
2 -means Clustering
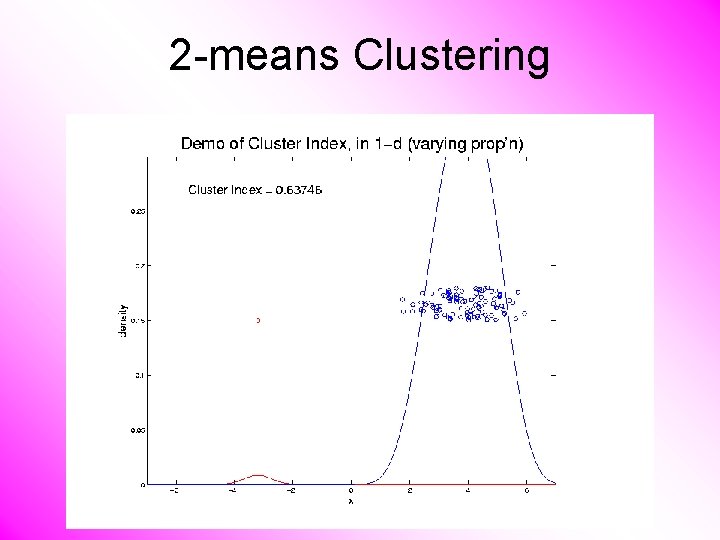
2 -means Clustering
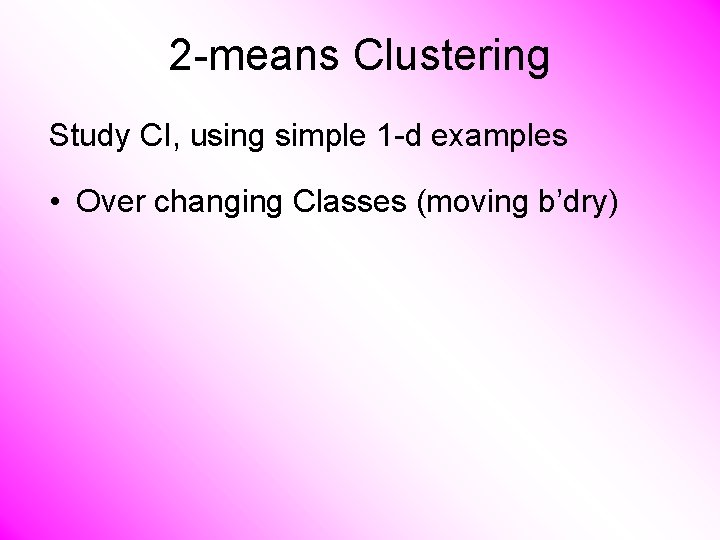
2 -means Clustering Study CI, using simple 1 -d examples • Over changing Classes (moving b’dry)
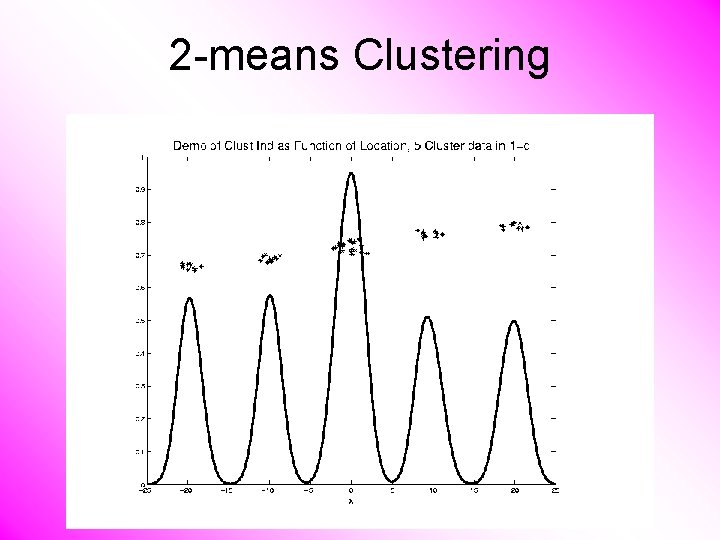
2 -means Clustering
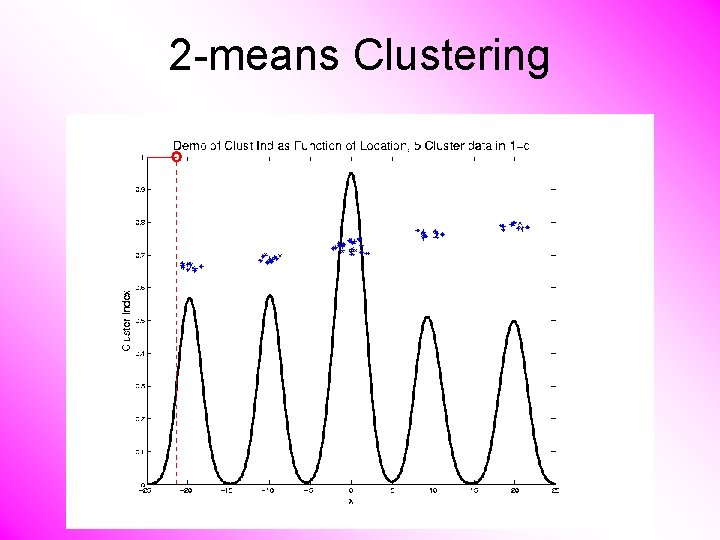
2 -means Clustering
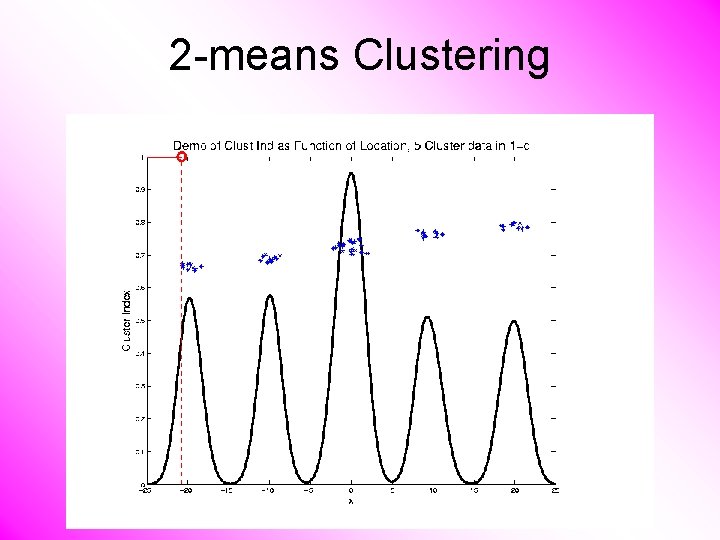
2 -means Clustering
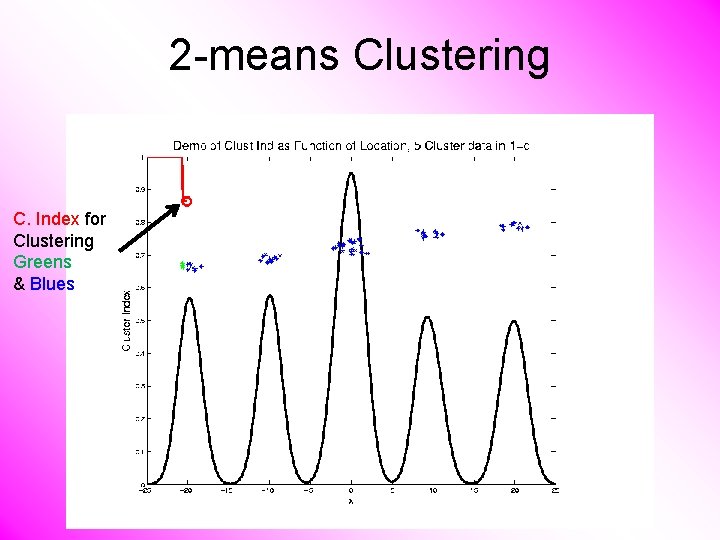
2 -means Clustering C. Index for Clustering Greens & Blues
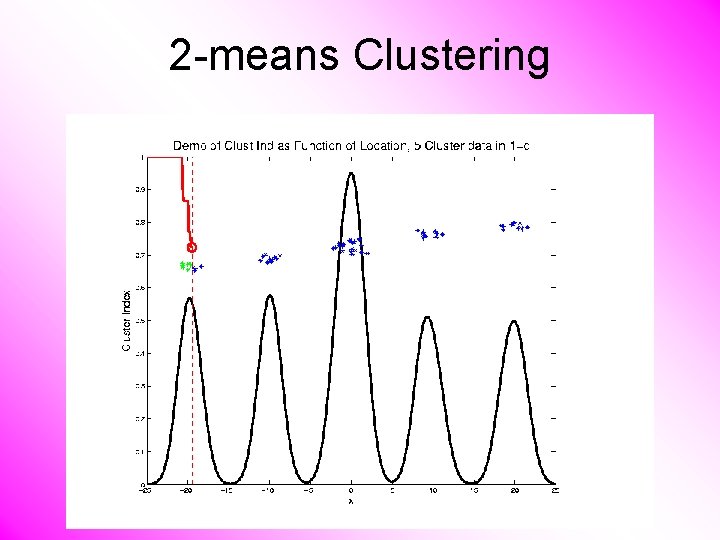
2 -means Clustering
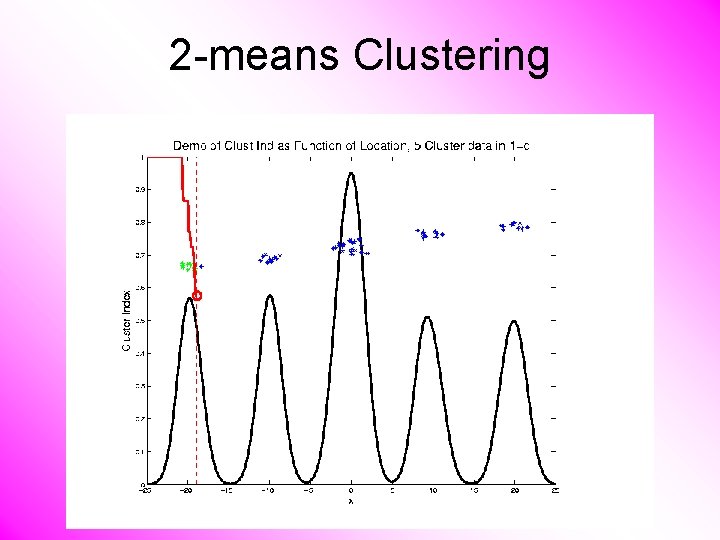
2 -means Clustering
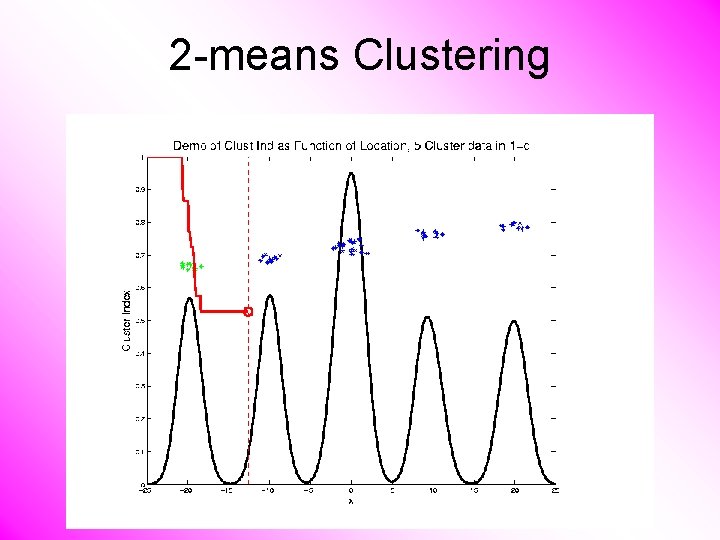
2 -means Clustering
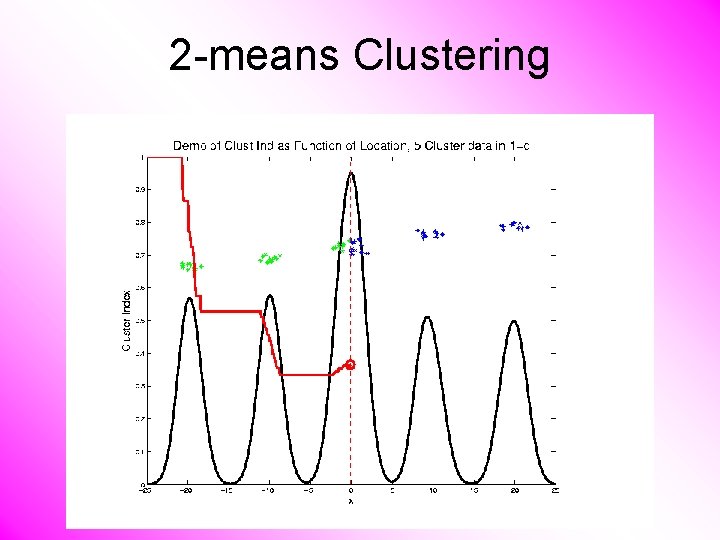
2 -means Clustering
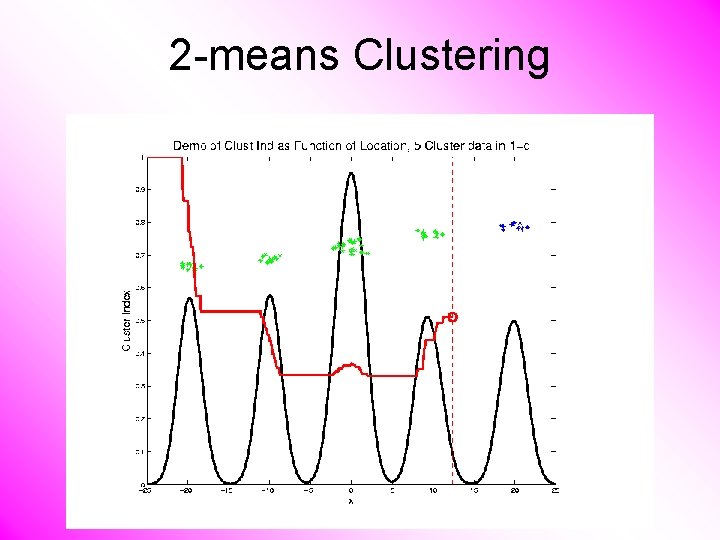
2 -means Clustering
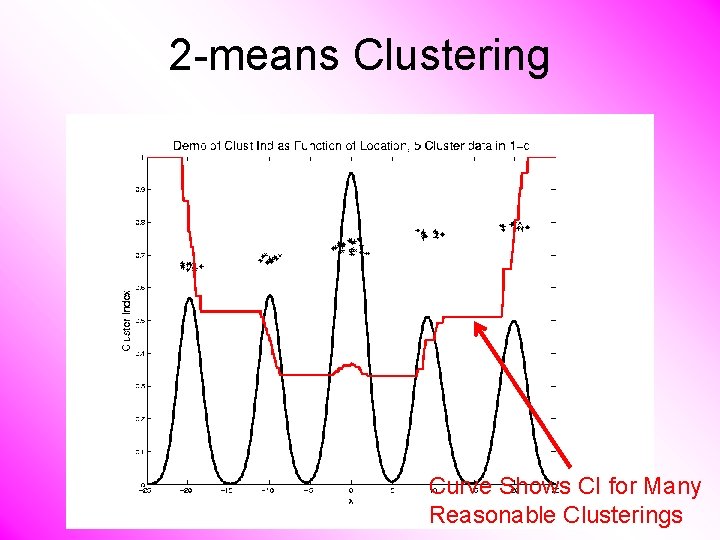
2 -means Clustering Curve Shows CI for Many Reasonable Clusterings
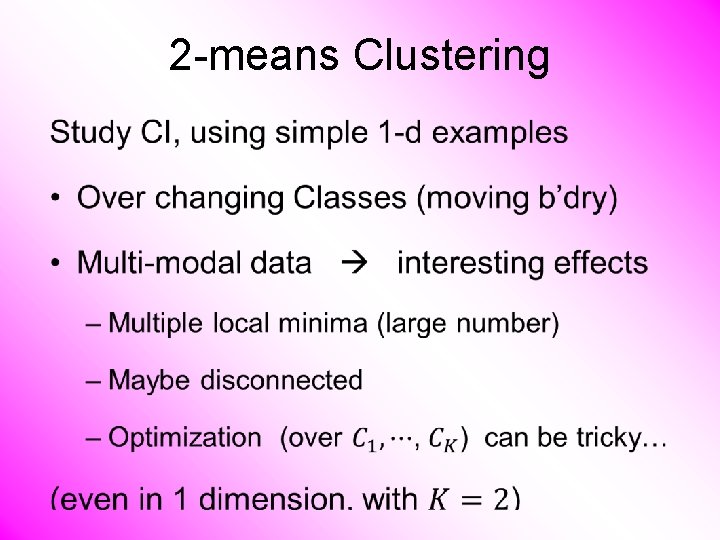
2 -means Clustering •
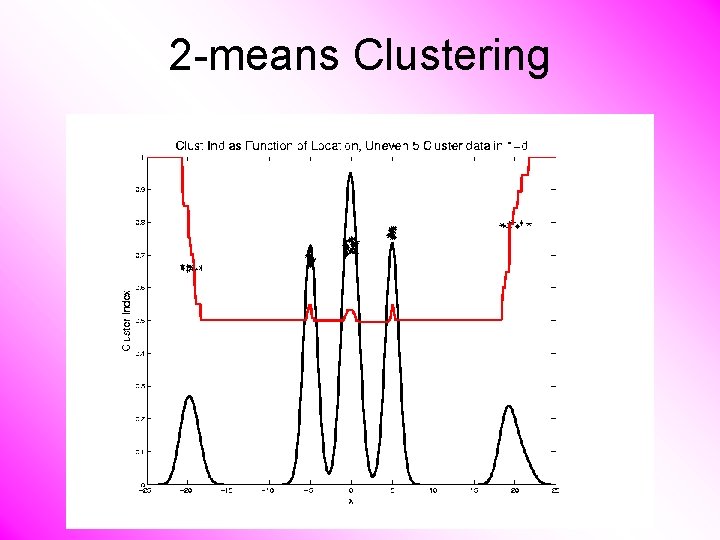
2 -means Clustering
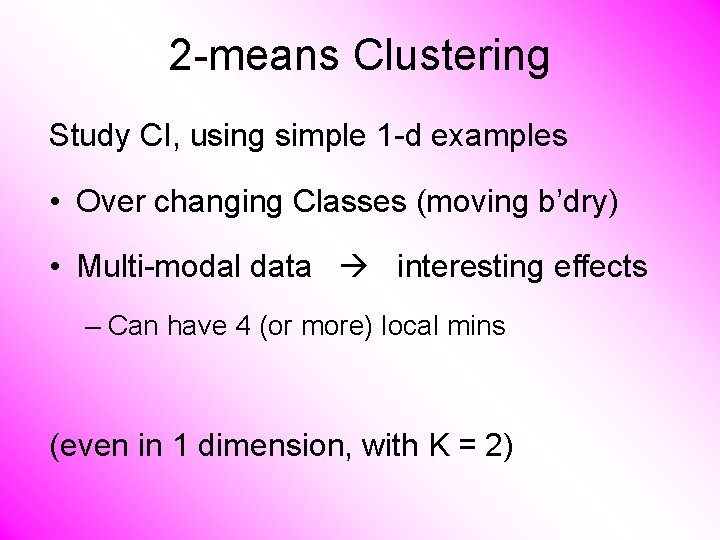
2 -means Clustering Study CI, using simple 1 -d examples • Over changing Classes (moving b’dry) • Multi-modal data interesting effects – Can have 4 (or more) local mins (even in 1 dimension, with K = 2)
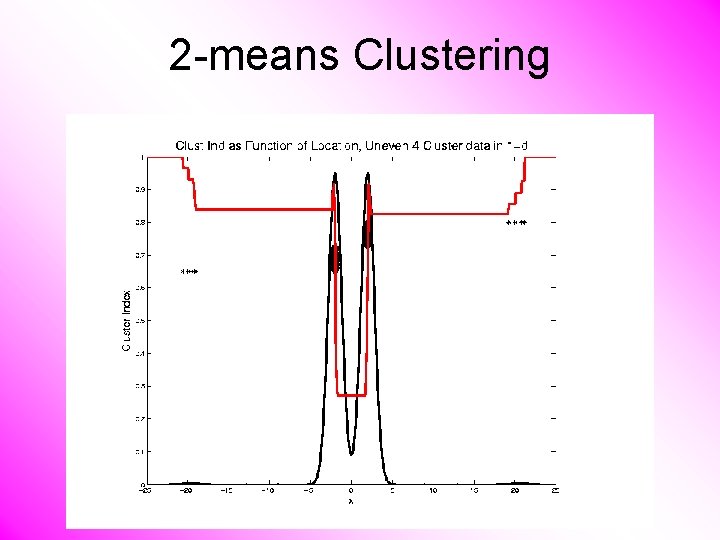
2 -means Clustering
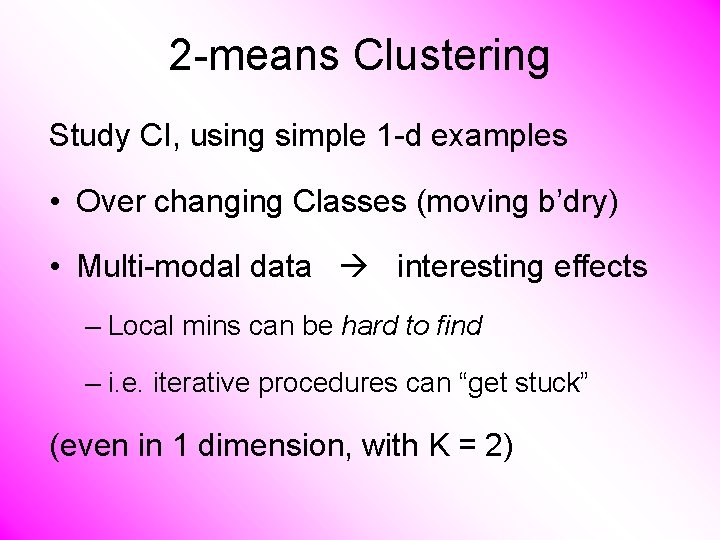
2 -means Clustering Study CI, using simple 1 -d examples • Over changing Classes (moving b’dry) • Multi-modal data interesting effects – Local mins can be hard to find – i. e. iterative procedures can “get stuck” (even in 1 dimension, with K = 2)
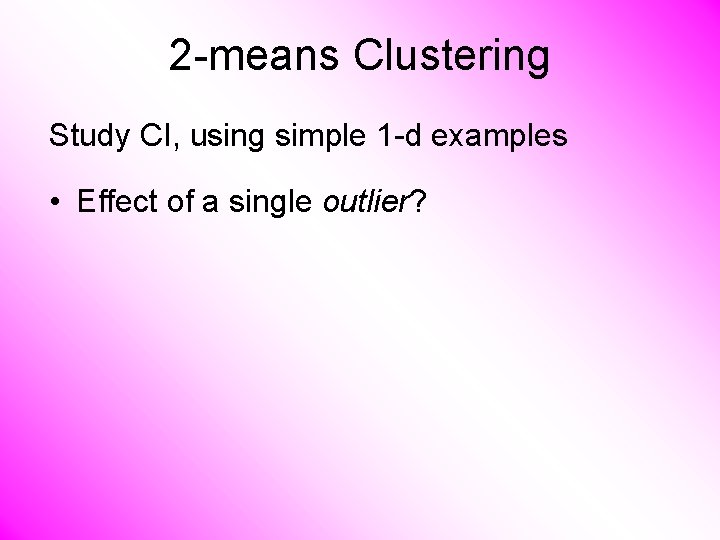
2 -means Clustering Study CI, using simple 1 -d examples • Effect of a single outlier?
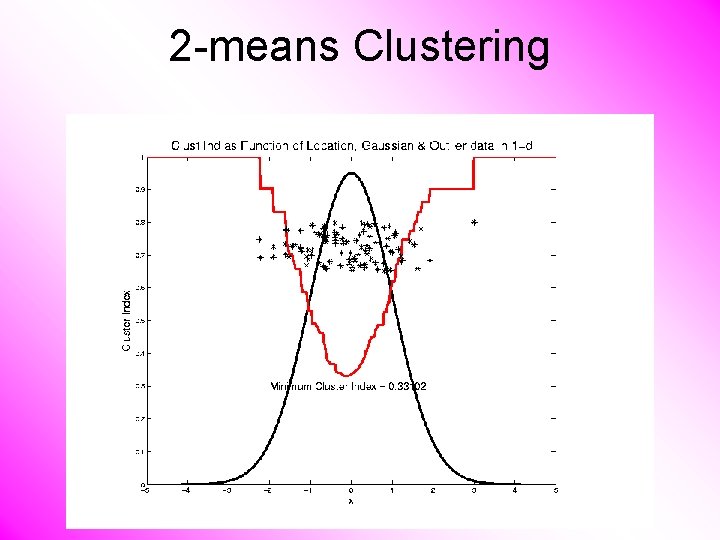
2 -means Clustering
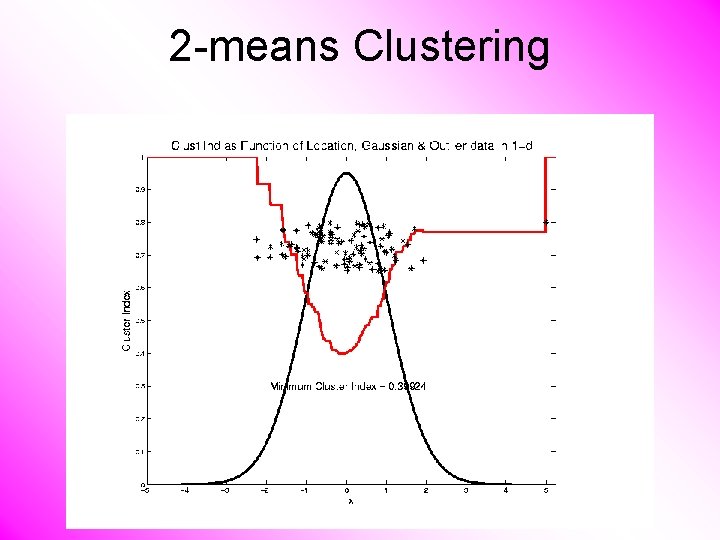
2 -means Clustering
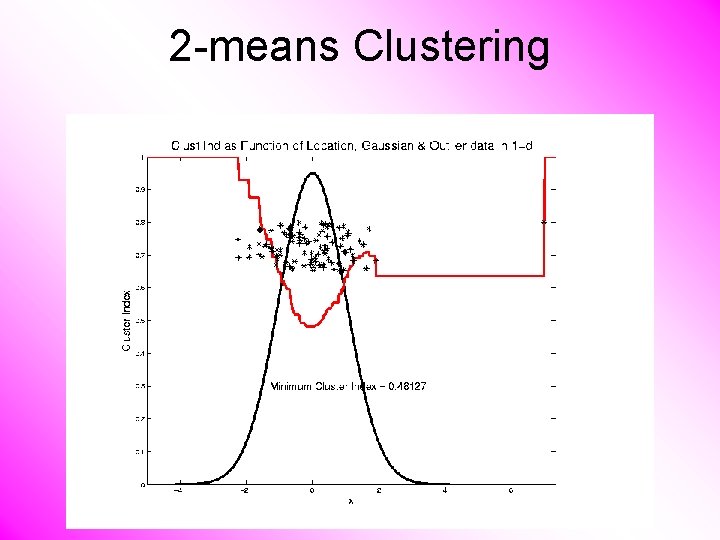
2 -means Clustering
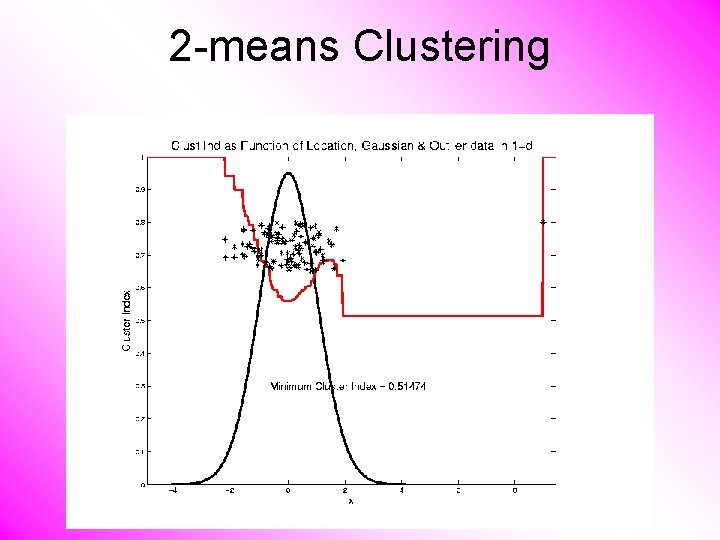
2 -means Clustering
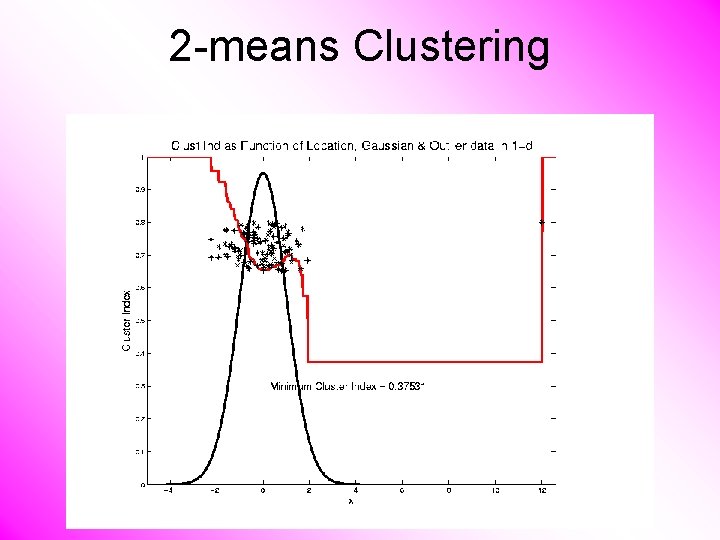
2 -means Clustering
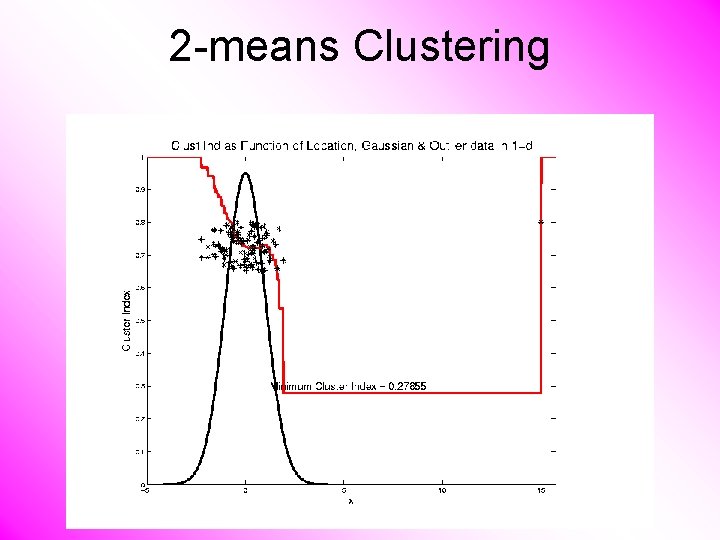
2 -means Clustering
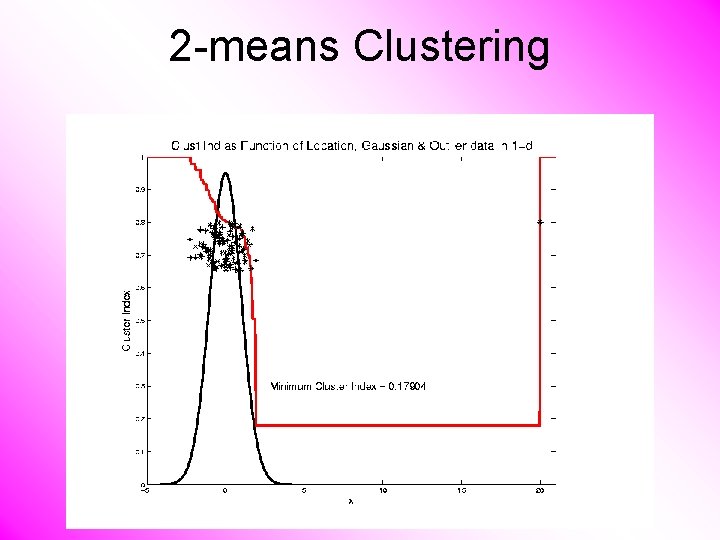
2 -means Clustering
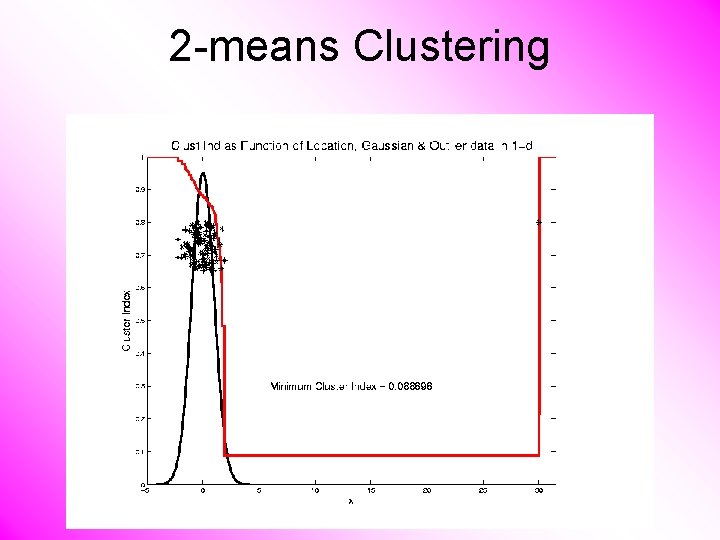
2 -means Clustering
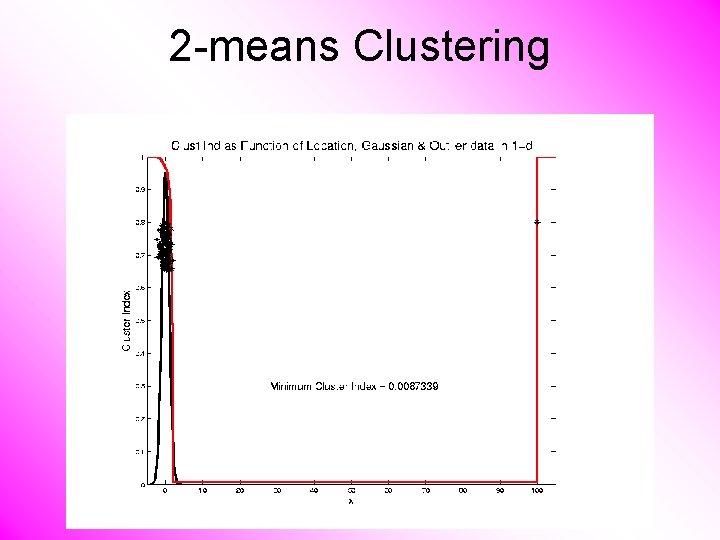
2 -means Clustering
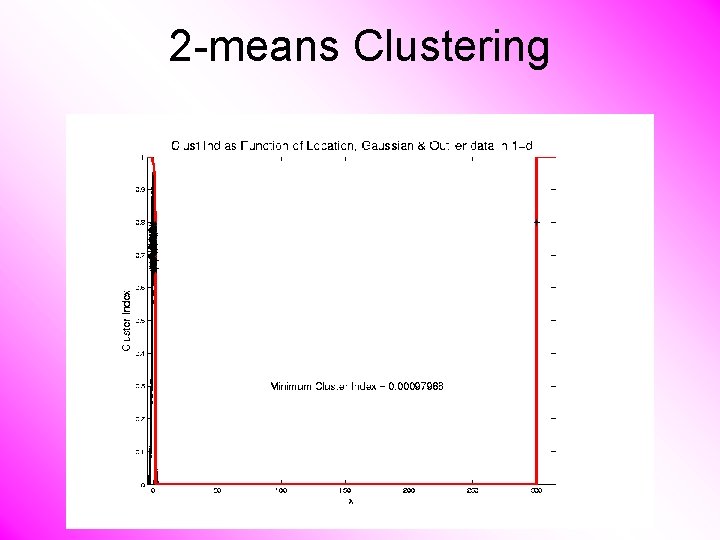
2 -means Clustering
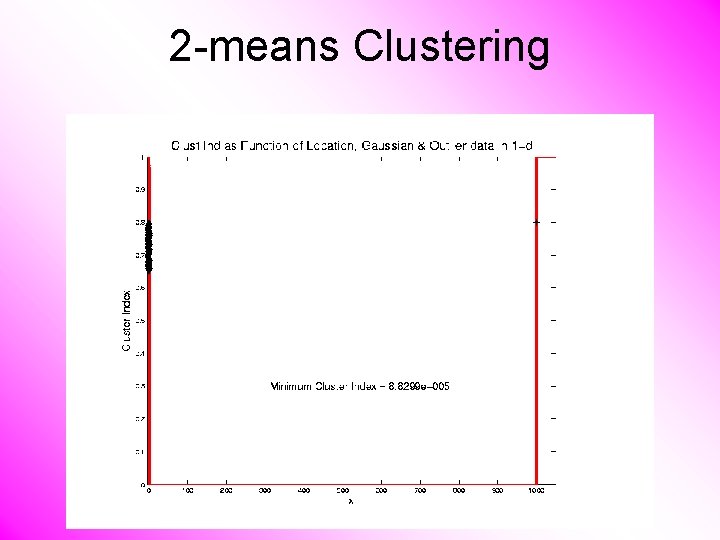
2 -means Clustering
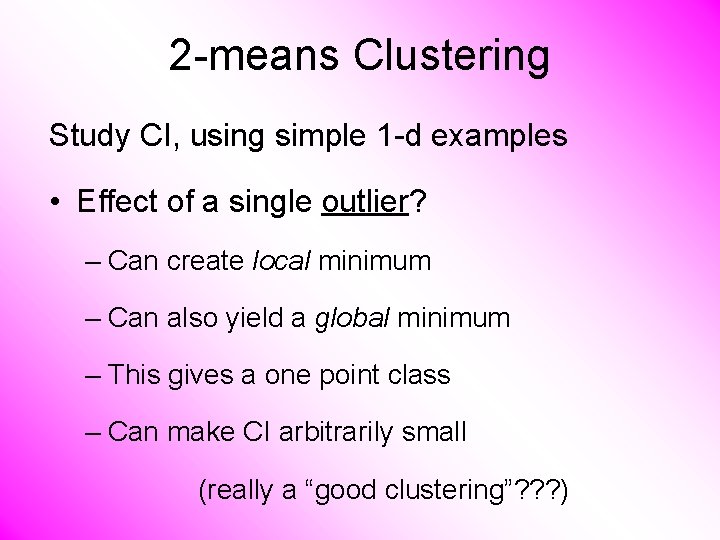
2 -means Clustering Study CI, using simple 1 -d examples • Effect of a single outlier? – Can create local minimum – Can also yield a global minimum – This gives a one point class – Can make CI arbitrarily small (really a “good clustering”? ? ? )
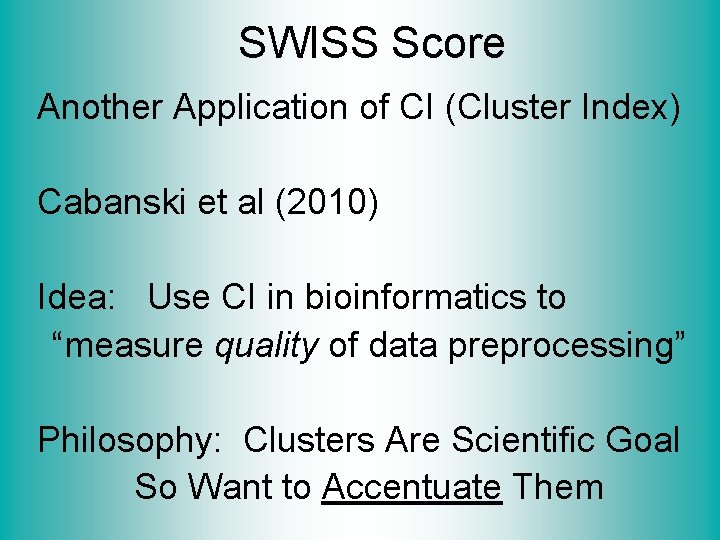
SWISS Score Another Application of CI (Cluster Index) Cabanski et al (2010) Idea: Use CI in bioinformatics to “measure quality of data preprocessing” Philosophy: Clusters Are Scientific Goal So Want to Accentuate Them
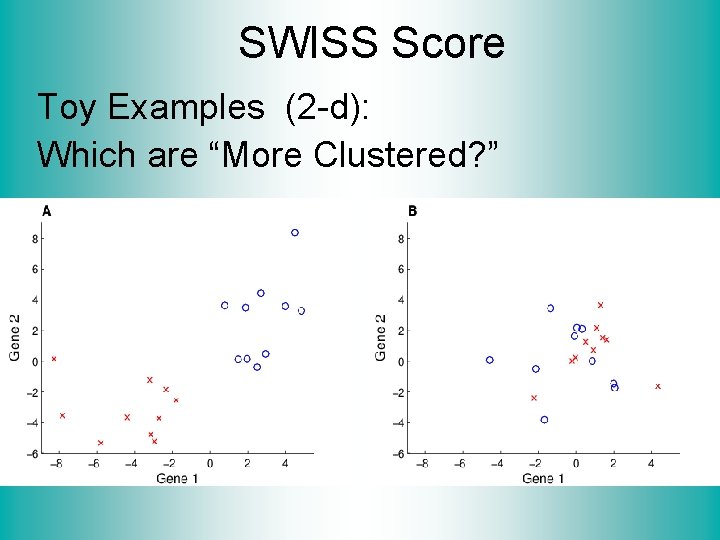
SWISS Score Toy Examples (2 -d): Which are “More Clustered? ”
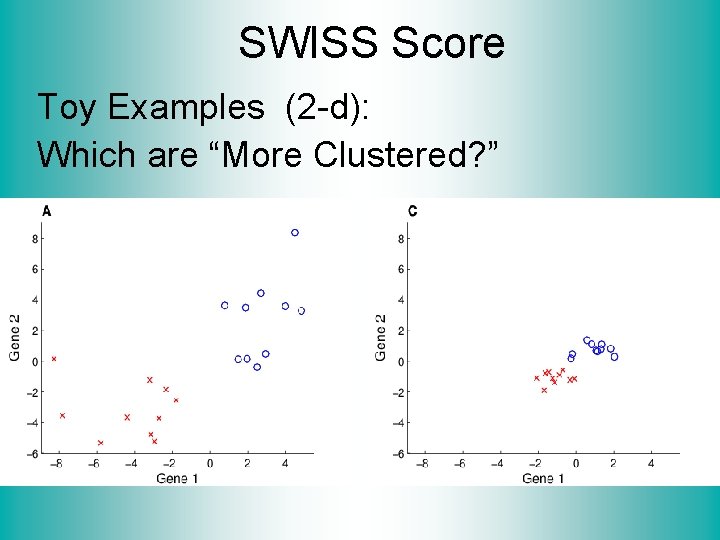
SWISS Score Toy Examples (2 -d): Which are “More Clustered? ”
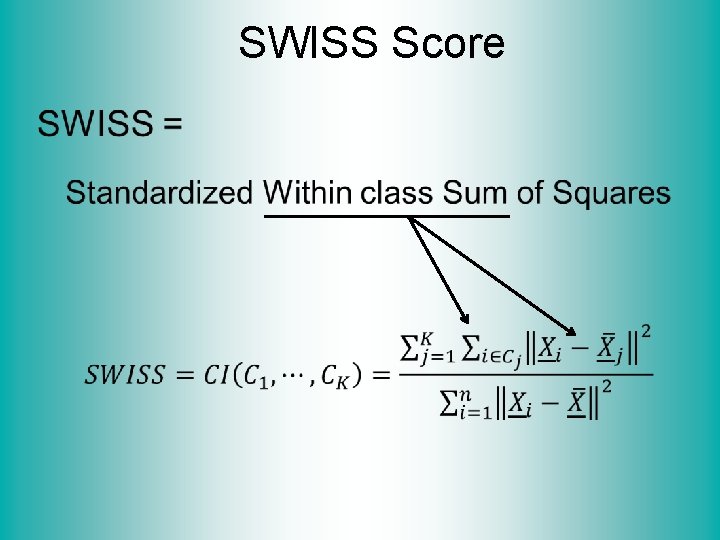
SWISS Score •
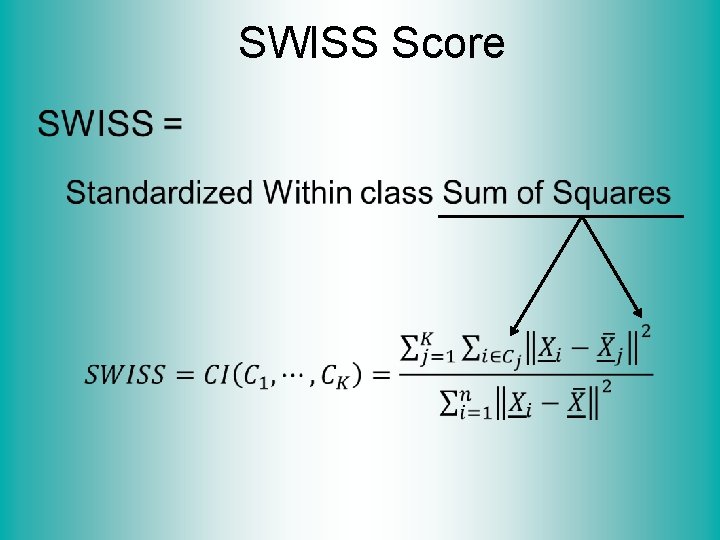
SWISS Score •
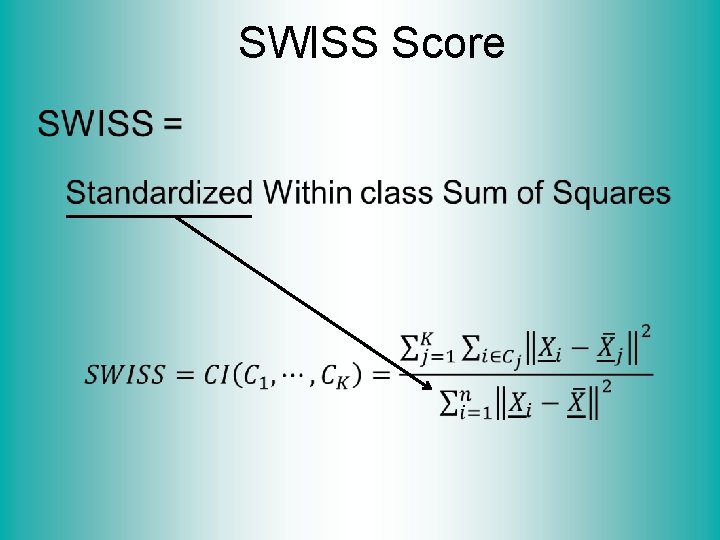
SWISS Score •
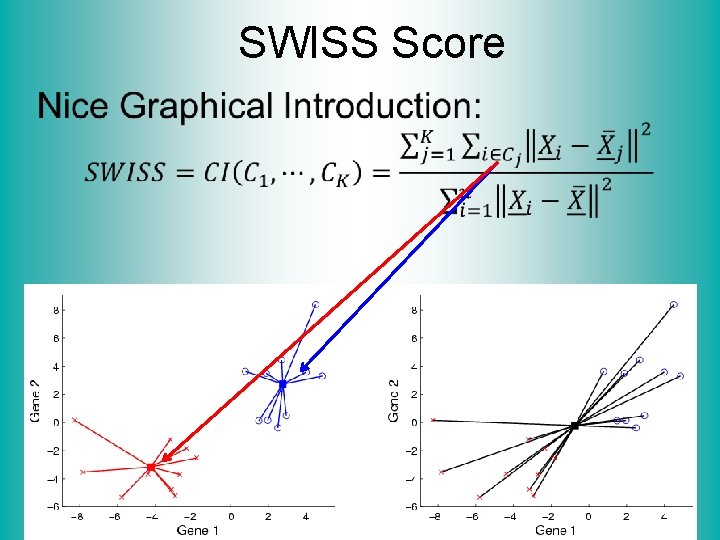
SWISS Score •
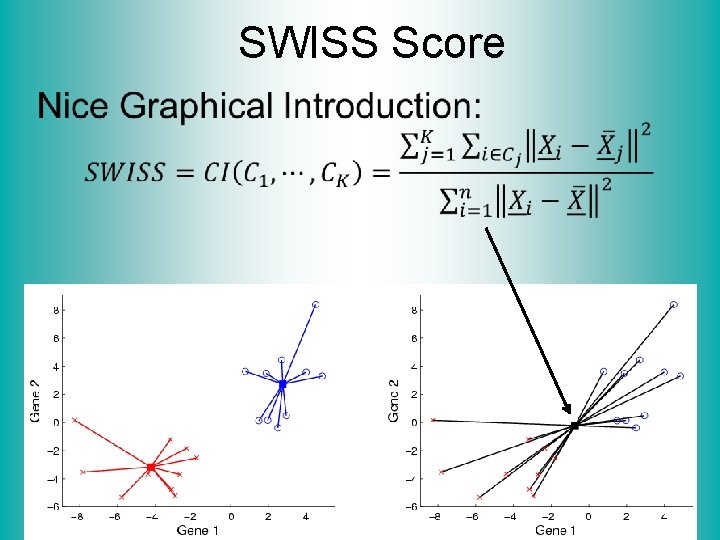
SWISS Score •
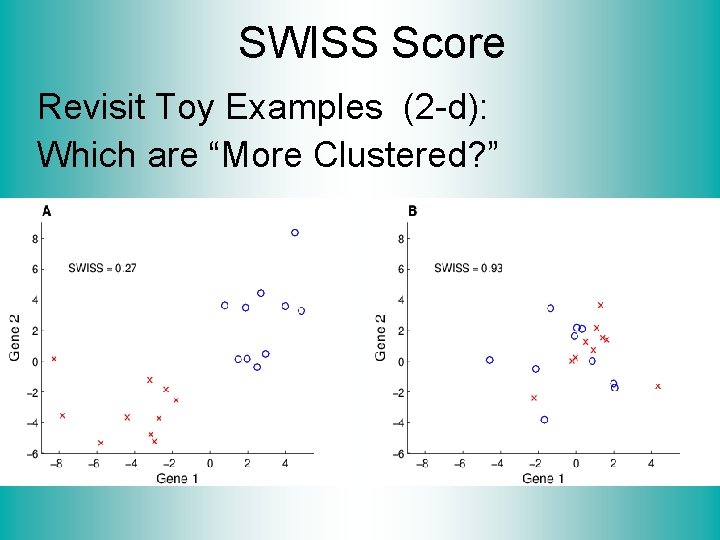
SWISS Score Revisit Toy Examples (2 -d): Which are “More Clustered? ”
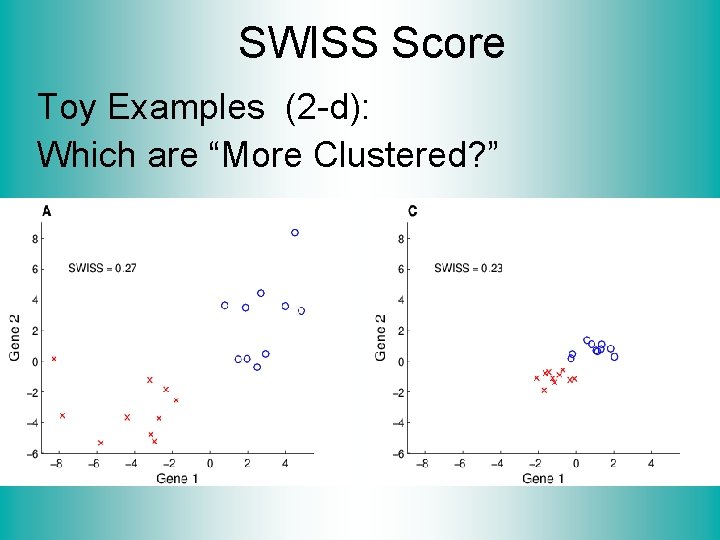
SWISS Score Toy Examples (2 -d): Which are “More Clustered? ”
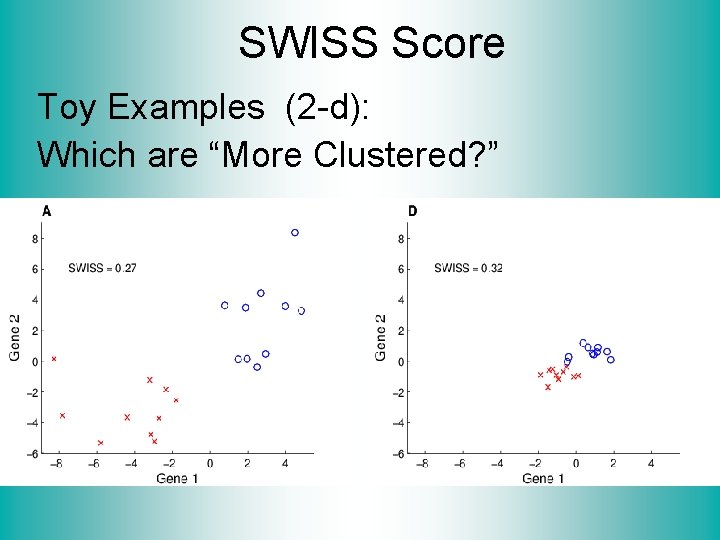
SWISS Score Toy Examples (2 -d): Which are “More Clustered? ”
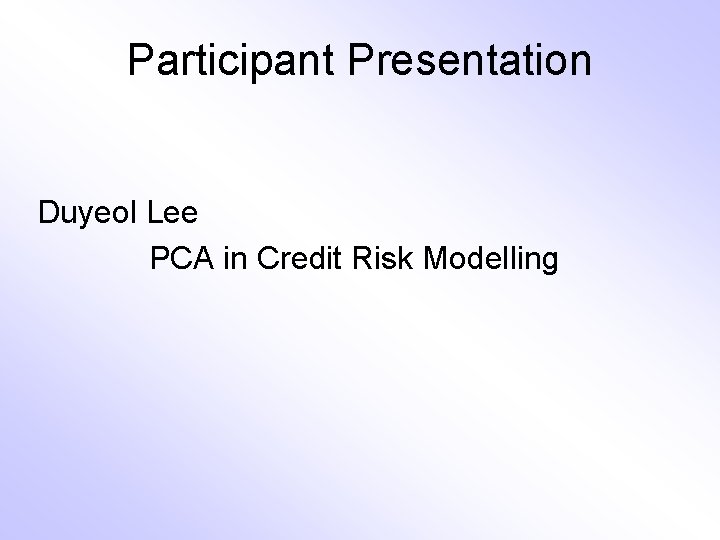
Participant Presentation Duyeol Lee PCA in Credit Risk Modelling
Tiered task bias task
Mapreduce: simplified data processing on large clusters
Guide to computer forensics and investigations
Mapreduce simplified data processing on large clusters
Mapreduce simplified data processing on large clusters
Common assessment task
Statistical treatment of data example
What statistical test for categorical data
Statistical data warehouse
T test ordinal data
Preserving statistical validity in adaptive data analysis
Statistical treatment of data example
Cowan statistical data analysis pdf
State bayes theorem
Statistical analysis of experimental data
Common multiples of 9 and 10
Common anode and common cathode
Factor tree for 56
Lowest common factor
Lcm of 16 24 and 40
Highest common factors and lowest common multiples
Factors of 145
Common factors of 18 and 30
How to find least common multiple
Geometric sequence formulas
Lcm of 10,5,2
Common difference
Gcf of 45 and 18
What are all the factors of 48
Lcd of polynomials
Sum of arithmetic progression formula
Find meaning of the following words
Lowest common multiple of 6 and 8
How to find lcd
Lcm 13 and 3
12 greatest common factor
Highest common factor
Greatest common factor examples
A river proper noun
Task abstraction
List the primitives that specify a data mining task.
Enrichment clusters
Cluster 5 jmu
Missouri connections career clusters
Population distribution
Cocci in grape-like clusters
Model of grand strategy clusters
Paranoid pd
Linux os high performance
Chapter 16 driving on highways
Vowels and consonants difference
Design objectives of computer clusters
Projection ap human geography
Career cluster definition
Danielson clusters
16 national career clusters framework
Hindi transliteration
What career cluster is a chef in
Noun clusters
Types of waves quad clusters answer key
Types of waves quad clusters answer key
Virtualization of clusters in cloud computing
16 career clusters worksheets
Career clusters framework
Rit cs clusters
Mcis career cluster inventory
Why are career clusters important
Career cluster wheel
Lower level brain structures
Short entrance lane
Georgia career clusters
Best dx cluster
Introduction to career clusters - vocabulary
Maryland career clusters
Cluster c
Onet career clusters
Two almond shaped neural clusters
Mn career clusters
Galaxy clusters
Galaxy clusters
Two almond shaped neural clusters
Types of waves quad clusters
Rocks clusters
Galaxy clusters
Mn career clusters
A set of nested clusters organized as a hierarchical tree
Cue clusters in nursing
Rocks cluster tutorial
Regional clusters
Are clusters of measurable ksas
Virginia career clusters
Vawizard.org
Data input and editing in gis
Codap concord
How to use omop
Ohdsi in a box
It provides a common framework to allow data to be shared
Ncdisp
Data conditioning refers to
Colby common data set
Common data elements nih
Ucf spss
Common education data standards
Minimal common oncology data elements
Gss fast stream
Using statistical measures to compare populations
Partition function in statistical mechanics
Statistical mechanics
Classical equipartition
Statistical displays
Types of statistical questions
Statistical time-division multiplexing
Statistical thermodynamics is a study of
What is microcanonical ensemble
Parametric test and non parametric test
In continuous statistical surfaces the z values occur
Statistical significance p value
Statistical power table
Statistical nlp
Define investigator in statistics
Statistical package for the social sciences
Statistical process control ppt
Statistical symbols and meanings
Proof of chebyshev's inequality
Probability and statistical inference 9th solution pdf
National academy of statistical administration
Misleading graphs
Statistical notation
Statistical methods of demand forecasting
Statistical vs deterministic relationship
Partition function in statistical mechanics
Statistical algorithms
Statistical computing environment
Null hypothesis test formula
Statistical test flow chart
Statistical officer civil service
Statistical methods of demand forecasting
Numerical datum crossword puzzle clue
Statistical journal entry example
Types of statistical investigation
Partition function in statistical mechanics
Appropriate statistical test
What is a statistical syllogism?
Sqc in operations management
Distinguish between synchronous and statistical tdm.
Montgomery quality control
What is the sample size in qualitative research?
Statistical intervals based on a single sample
What is a statistical question in math
Hypothetical evidence examples
Statistical physics quiz