An Introduction to Automatic Speech Recognition Author JenWei
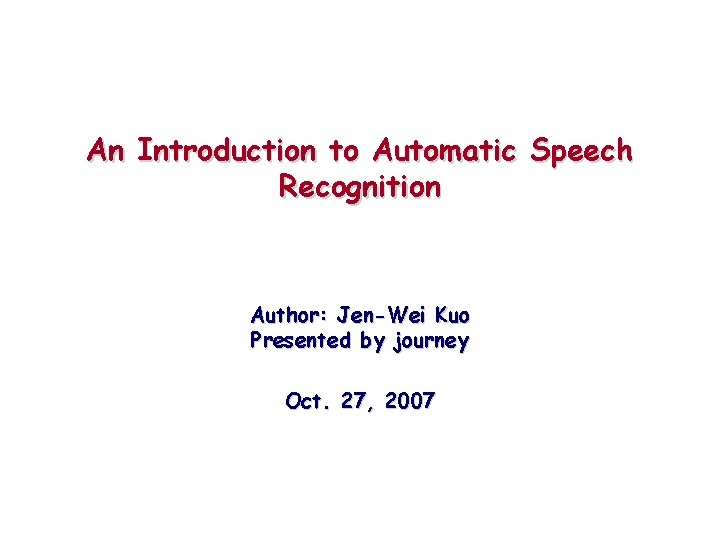
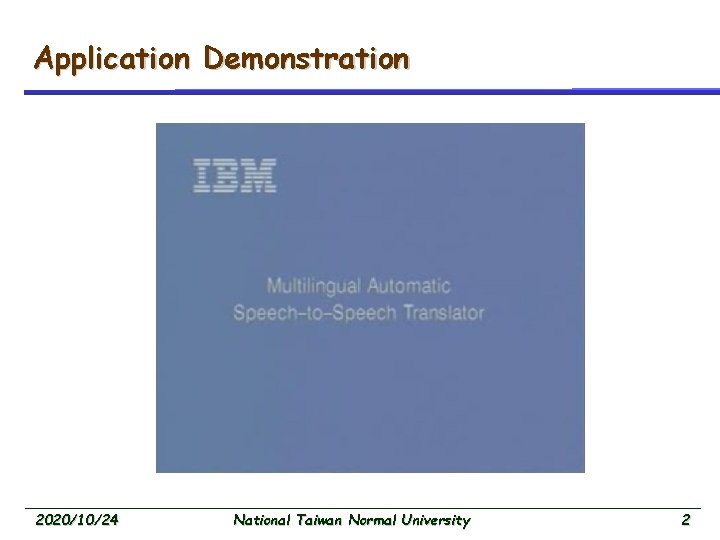
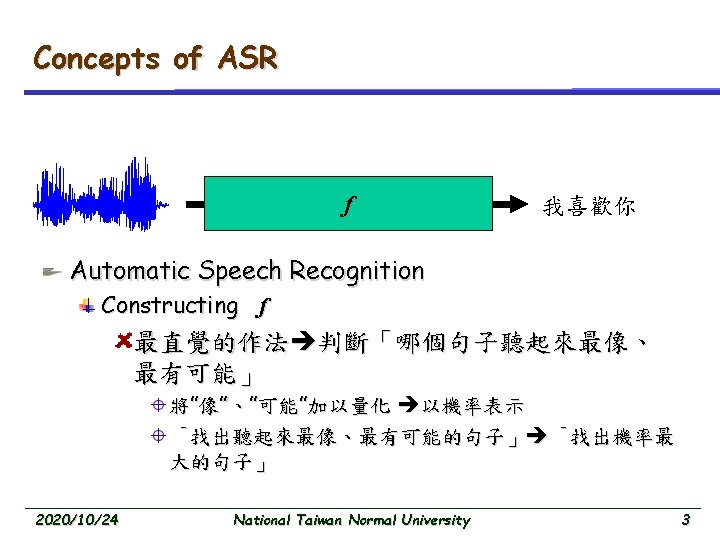
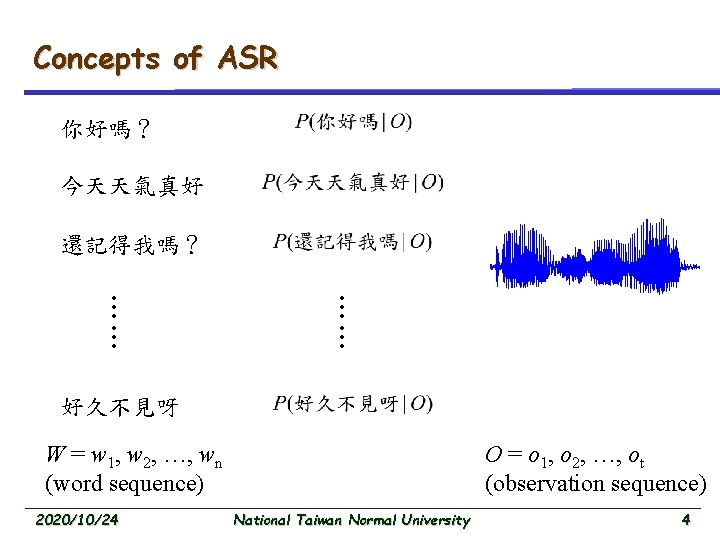
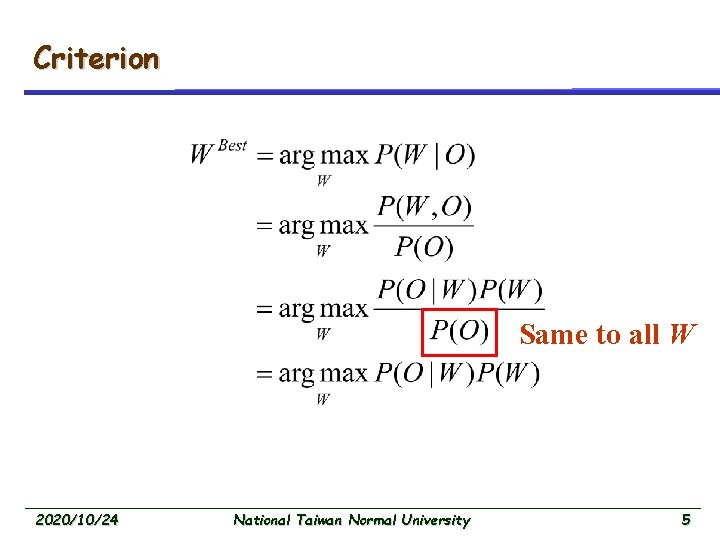
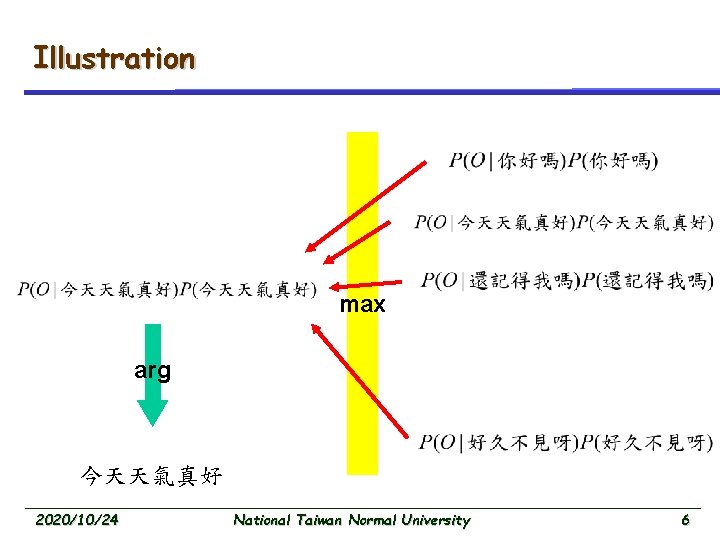
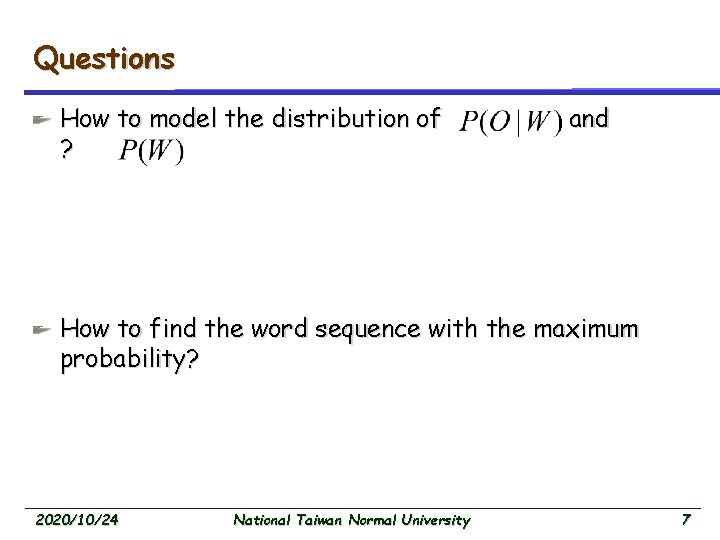
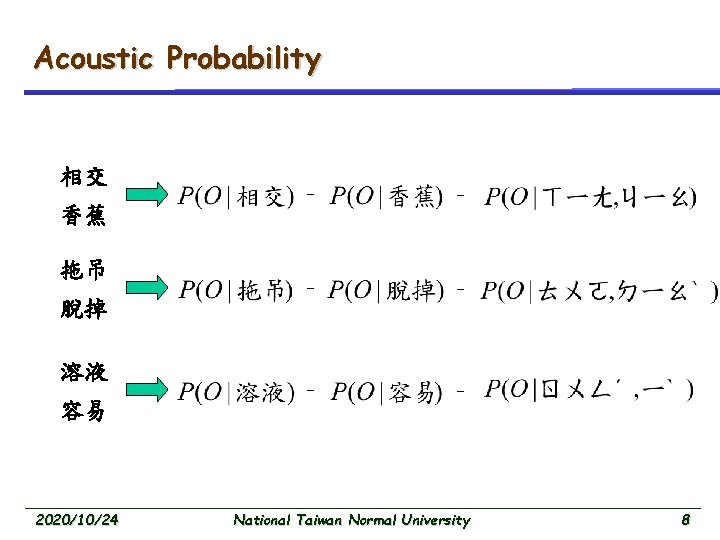
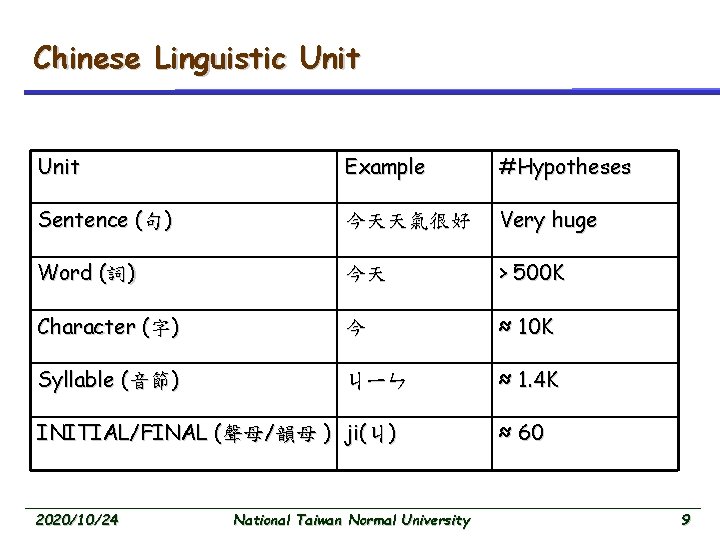
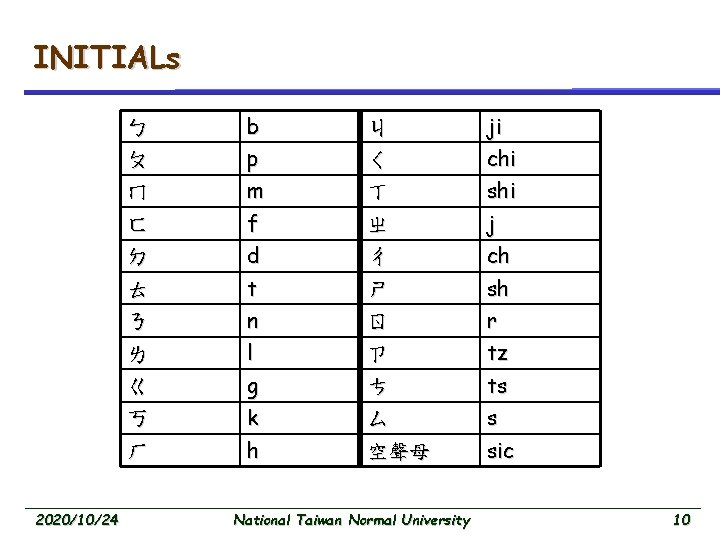
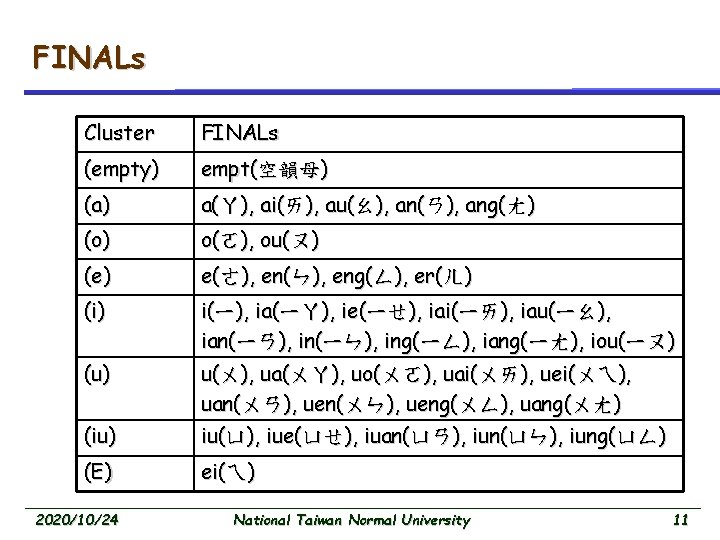
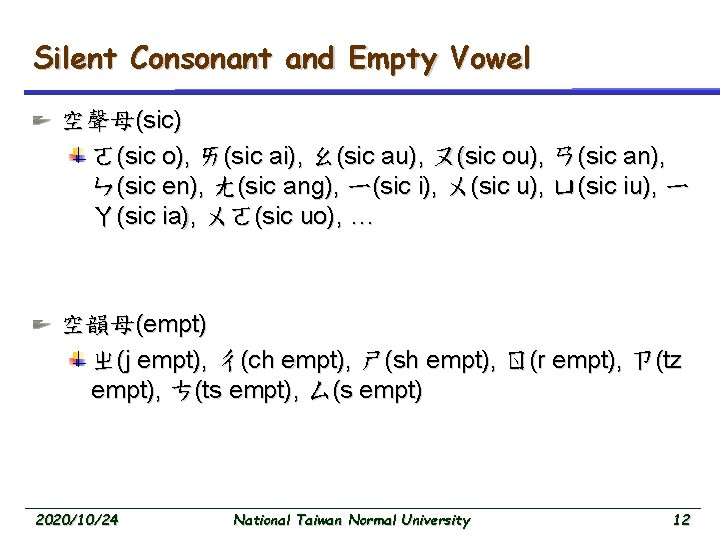
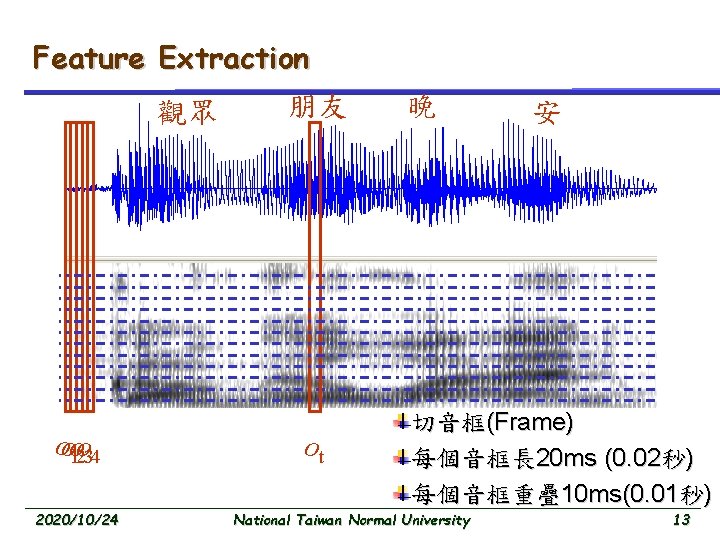
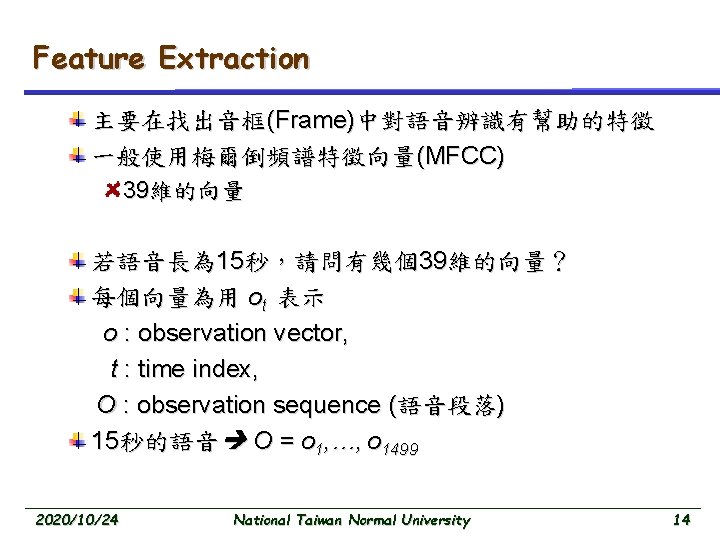
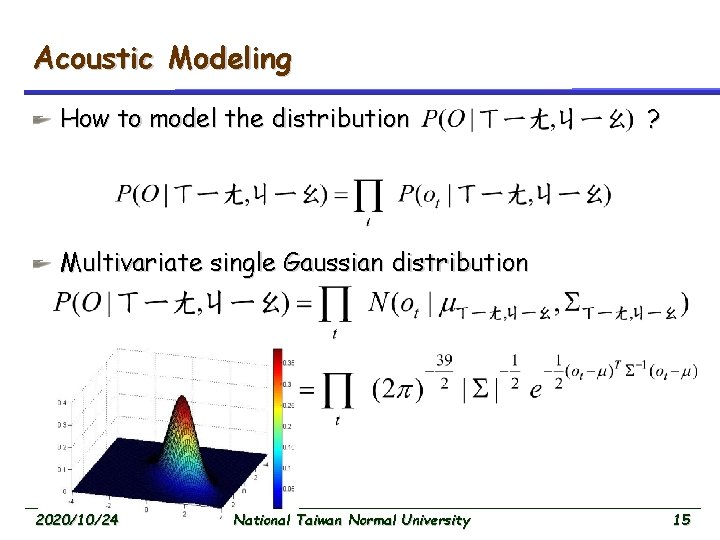
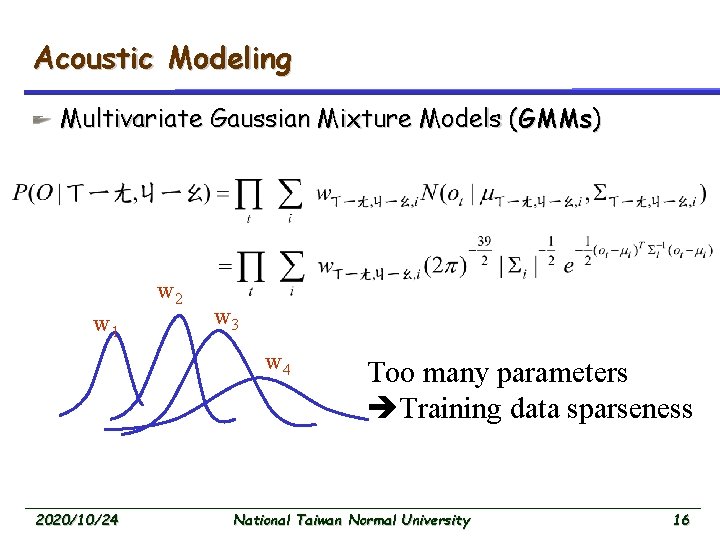
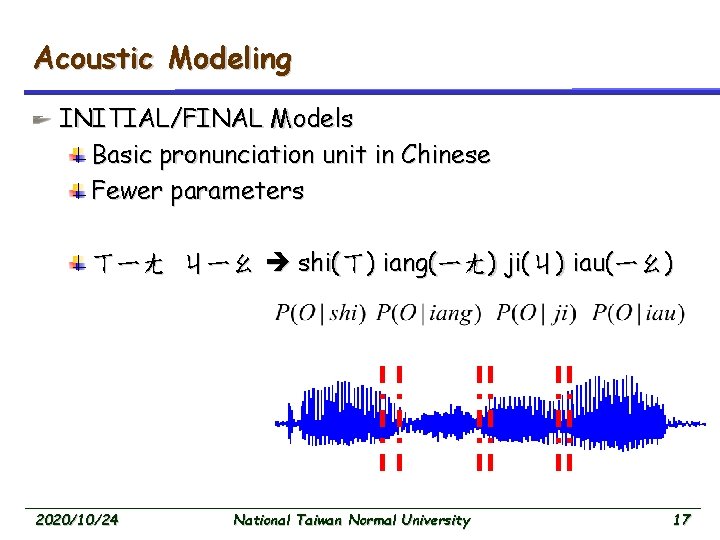
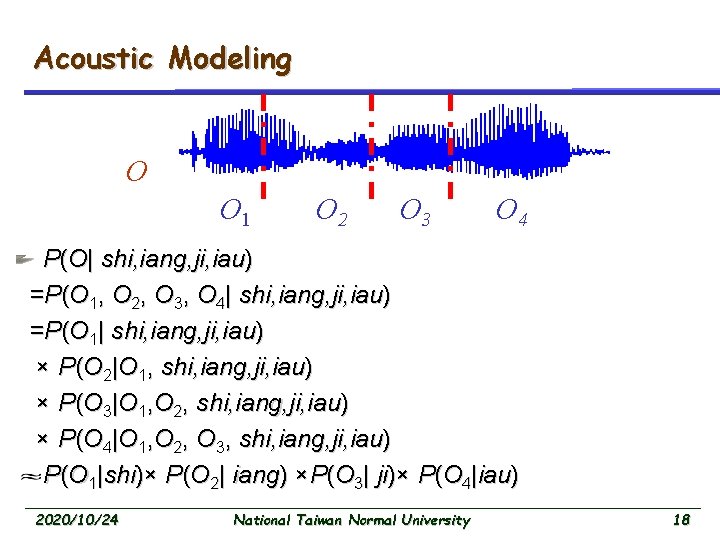
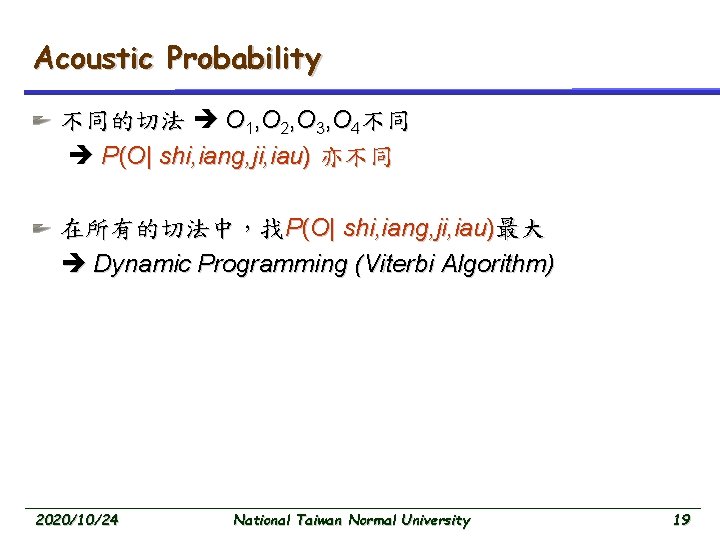
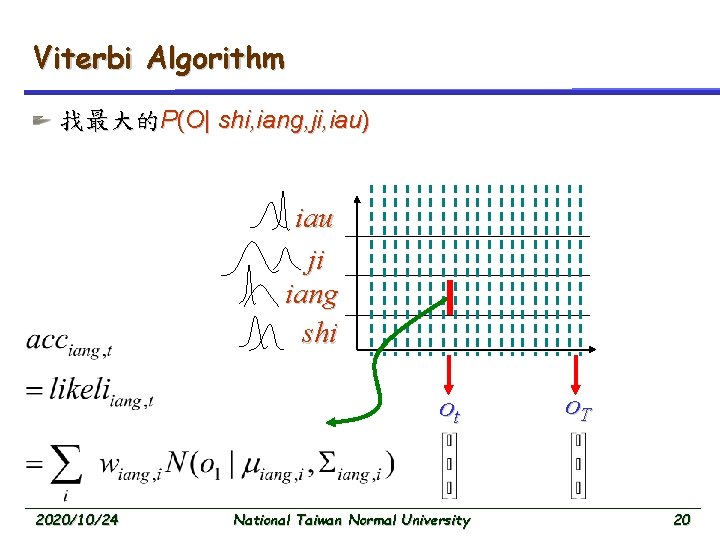
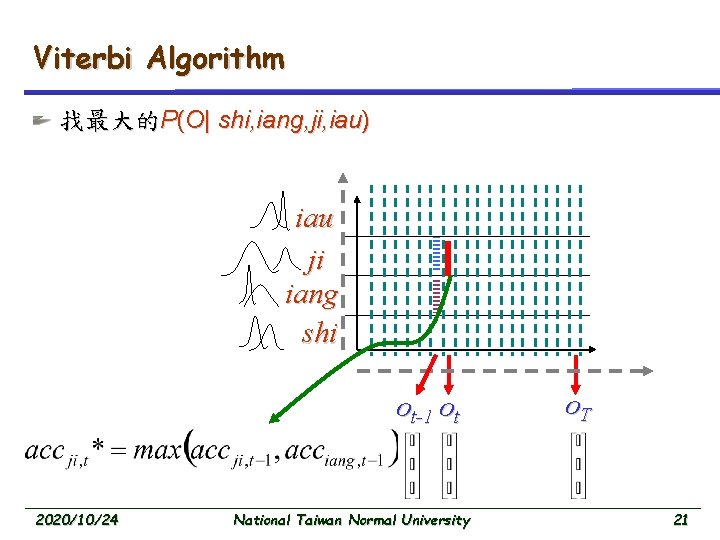
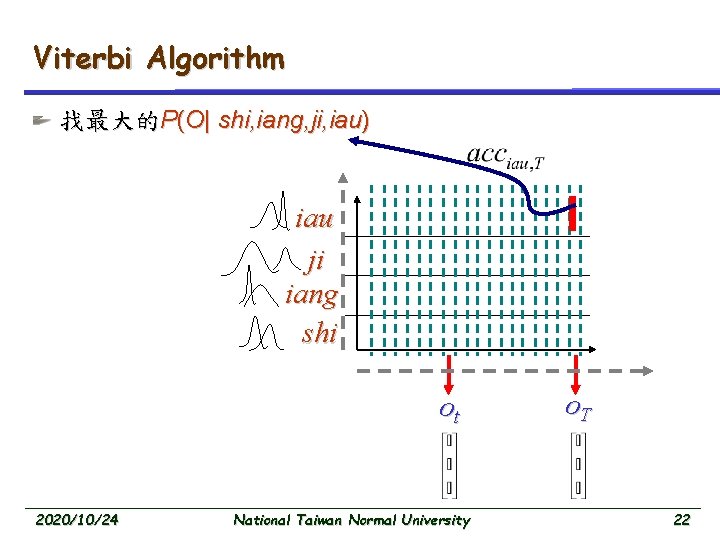
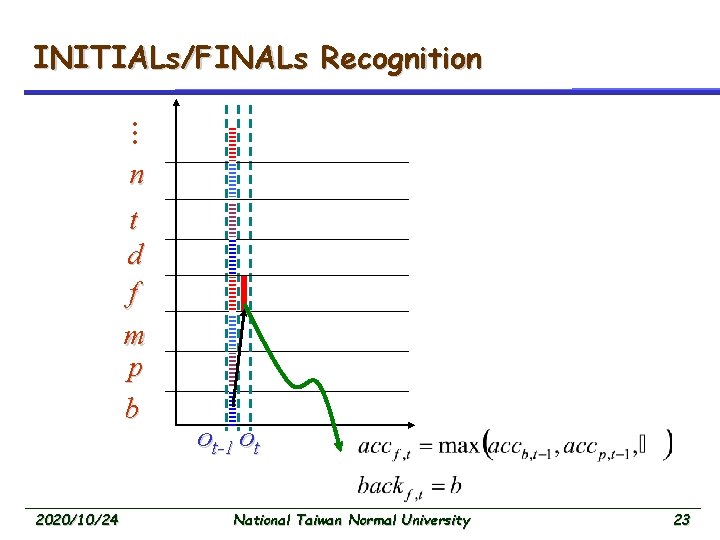
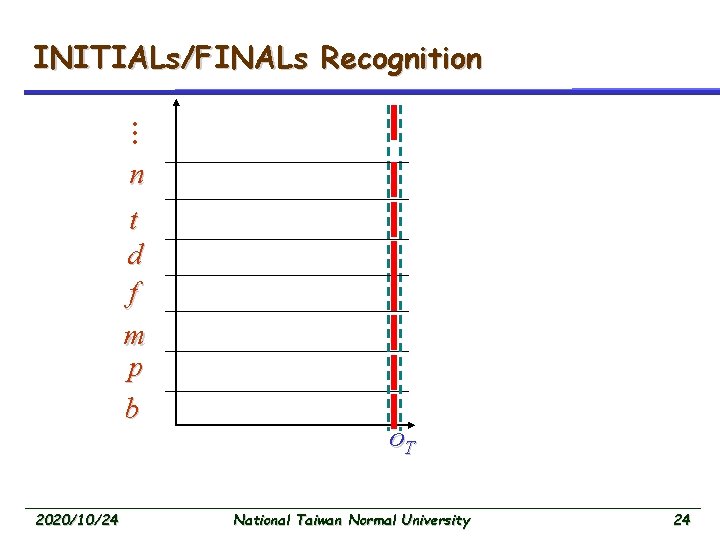
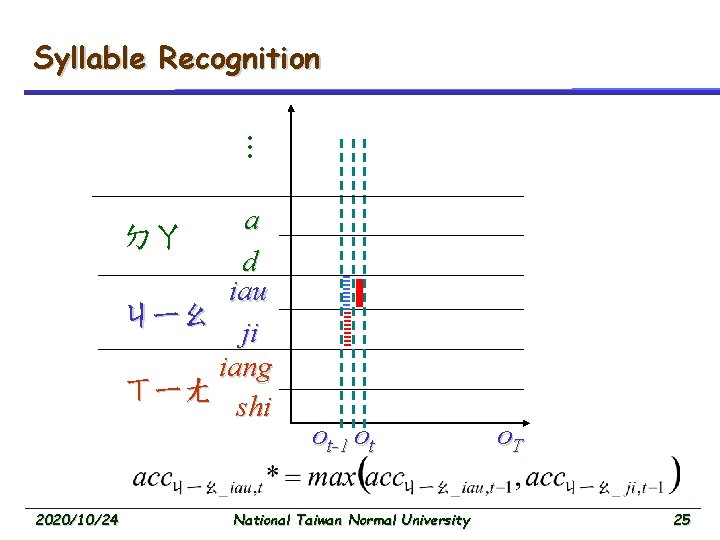
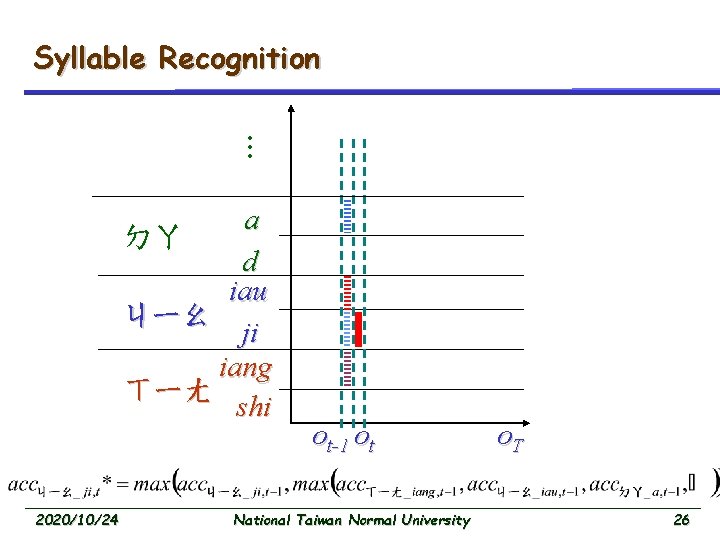
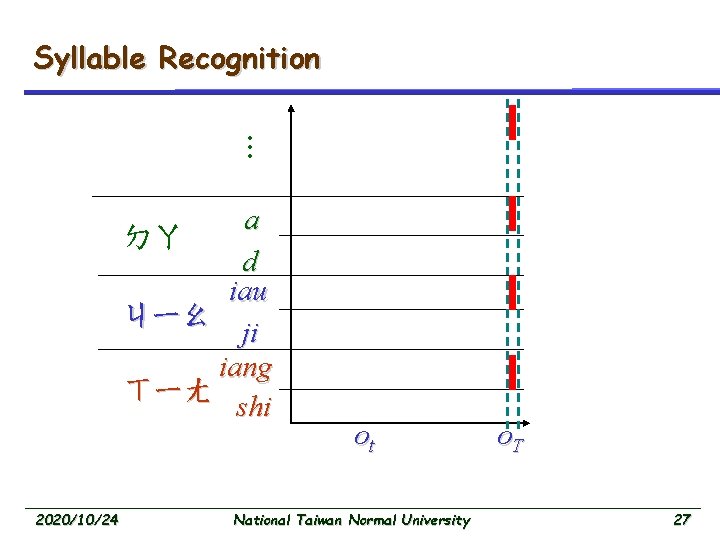
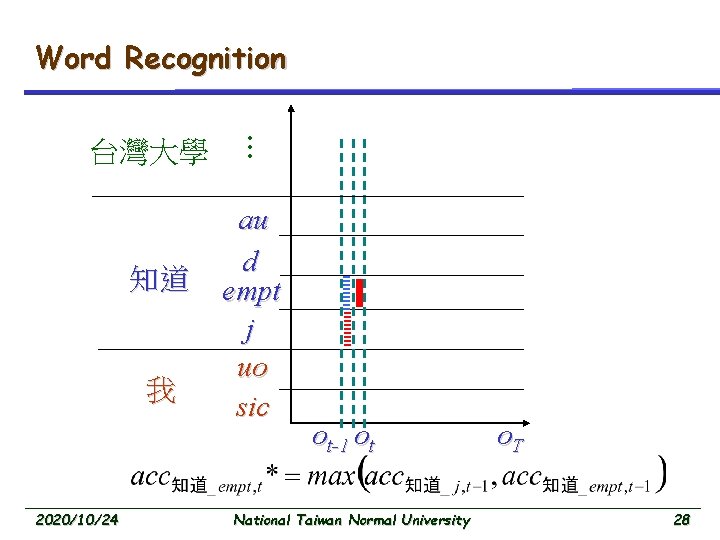
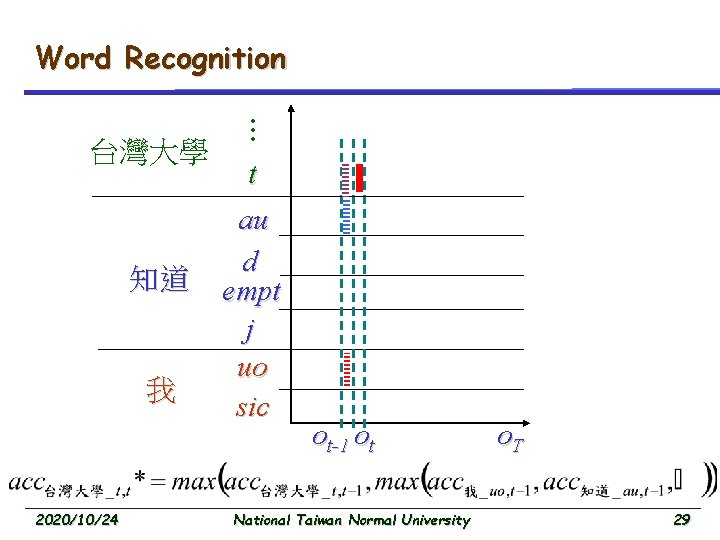
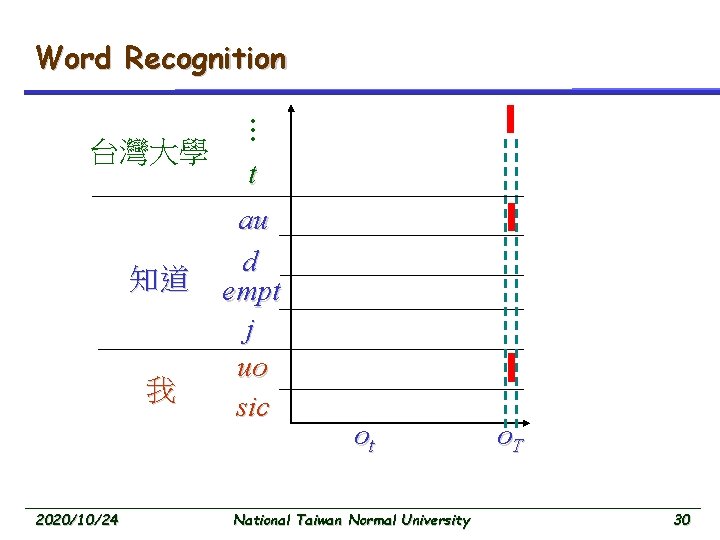
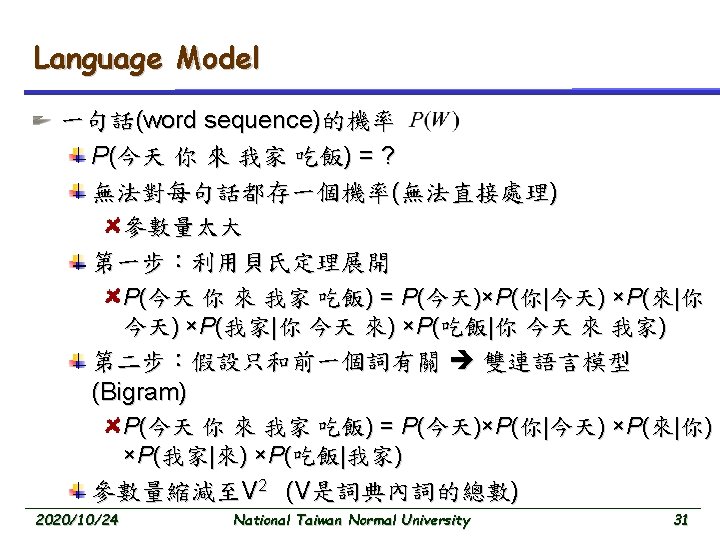
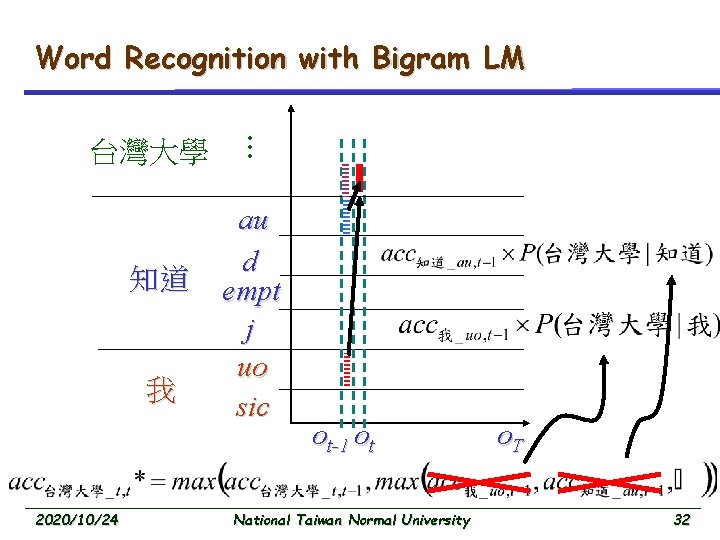
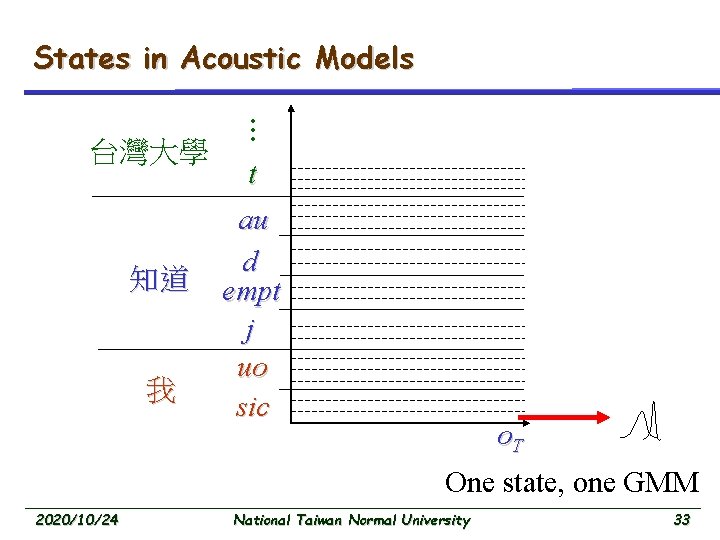
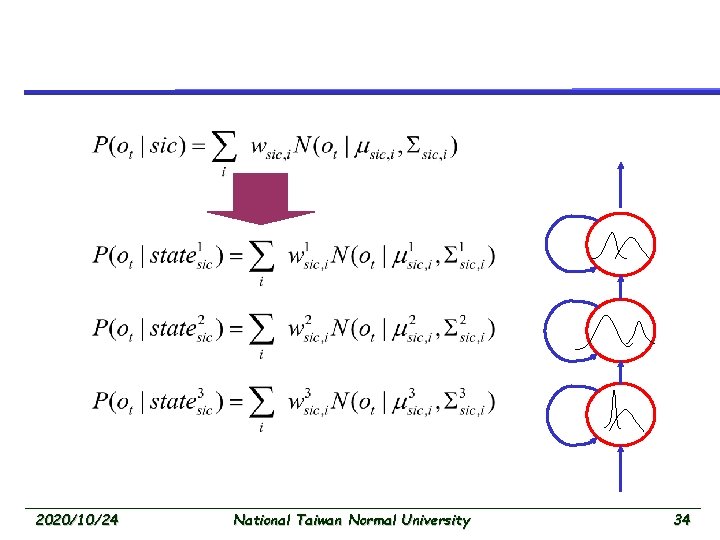
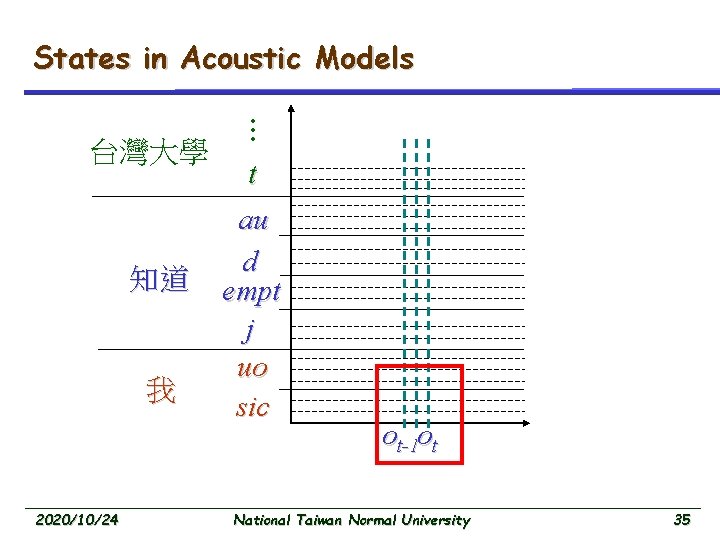
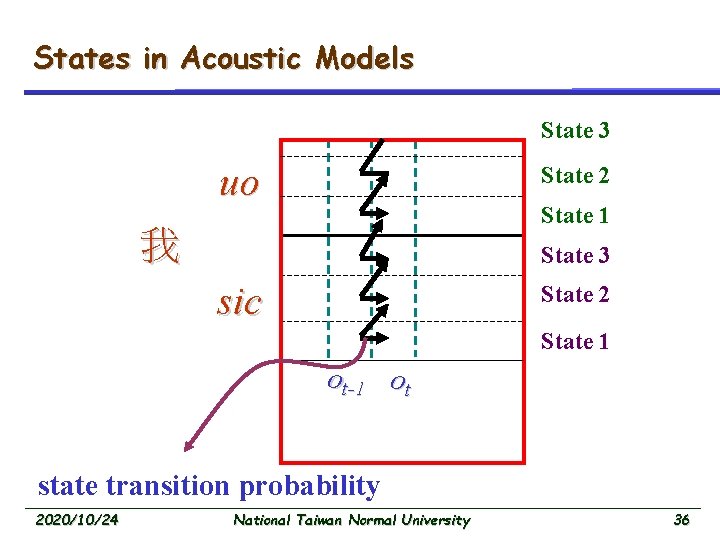
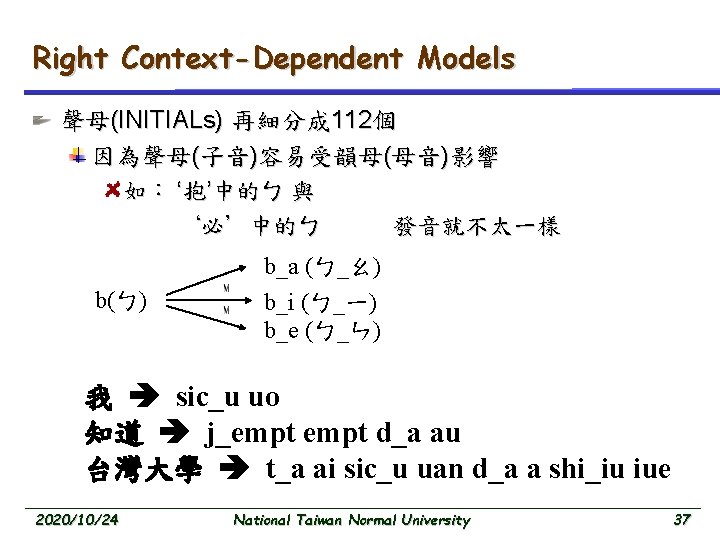
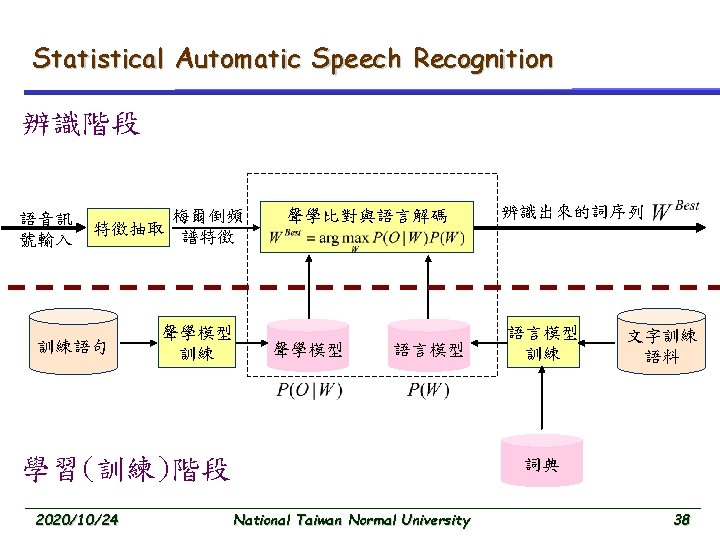
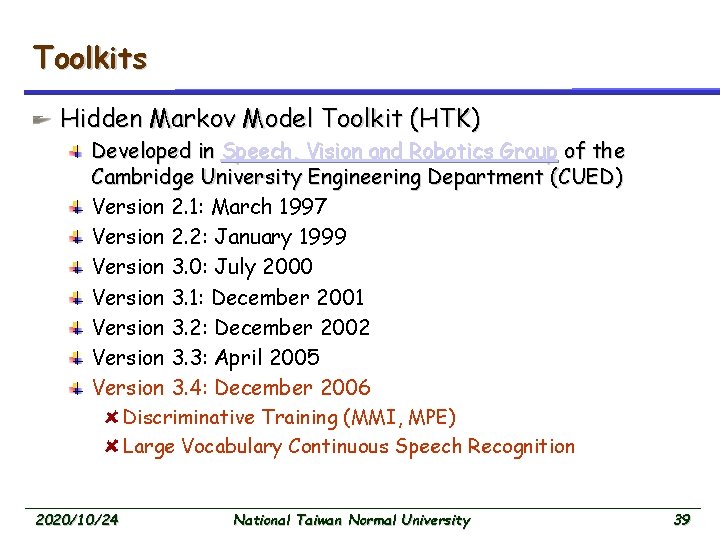
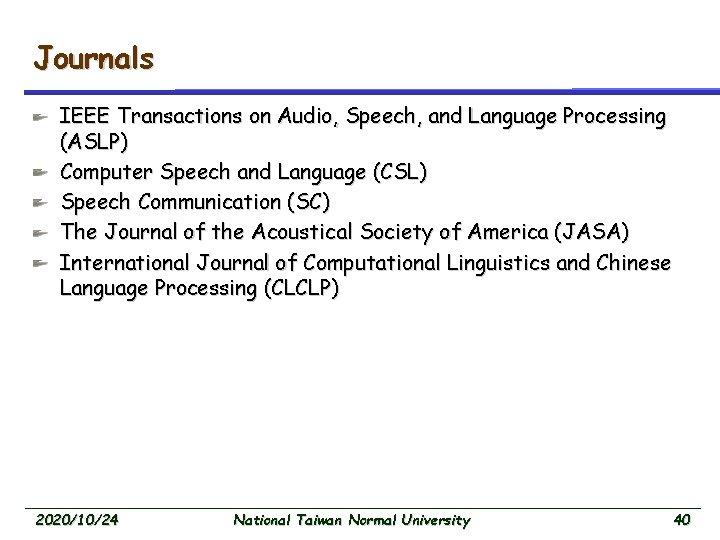
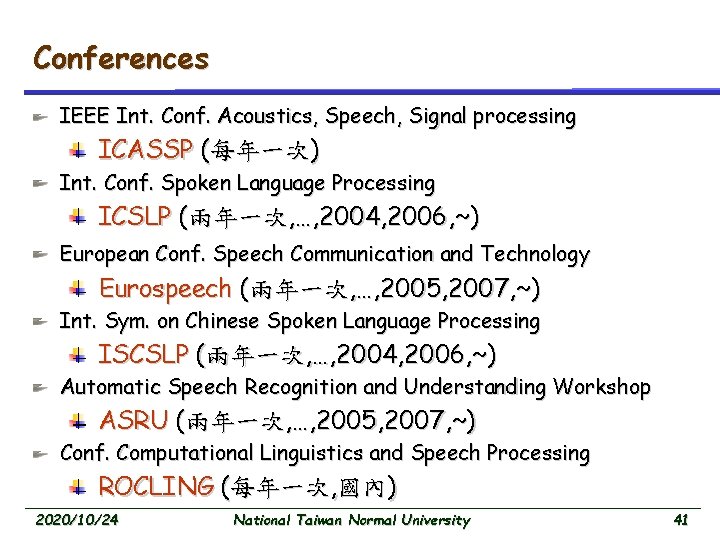
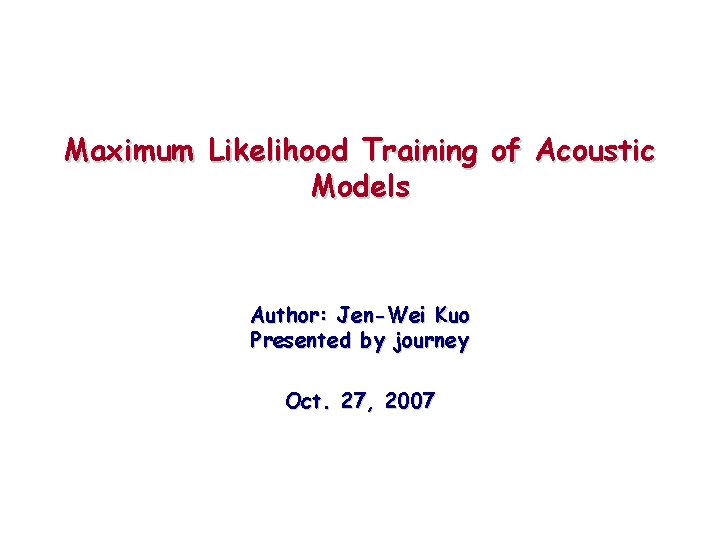
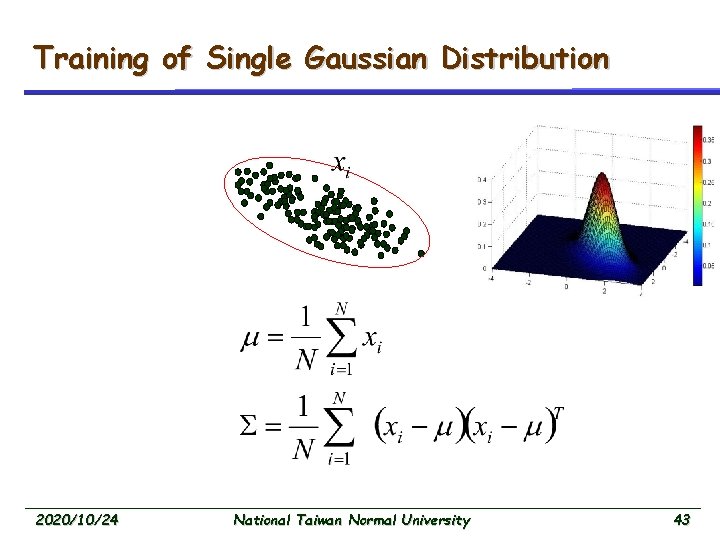
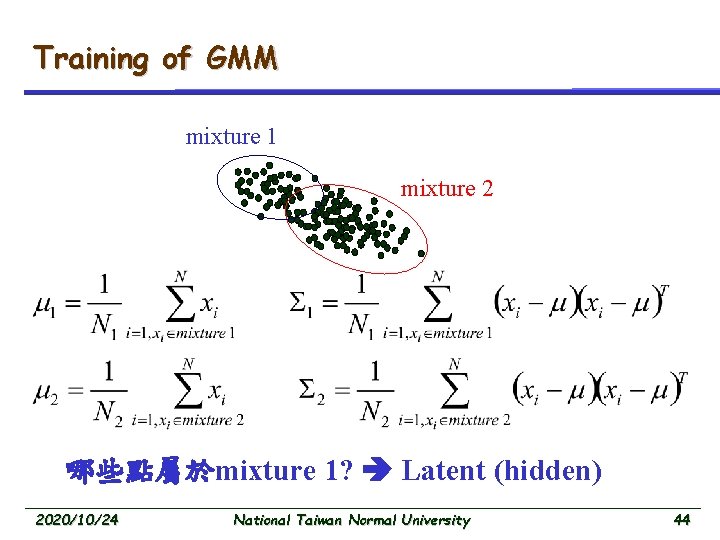
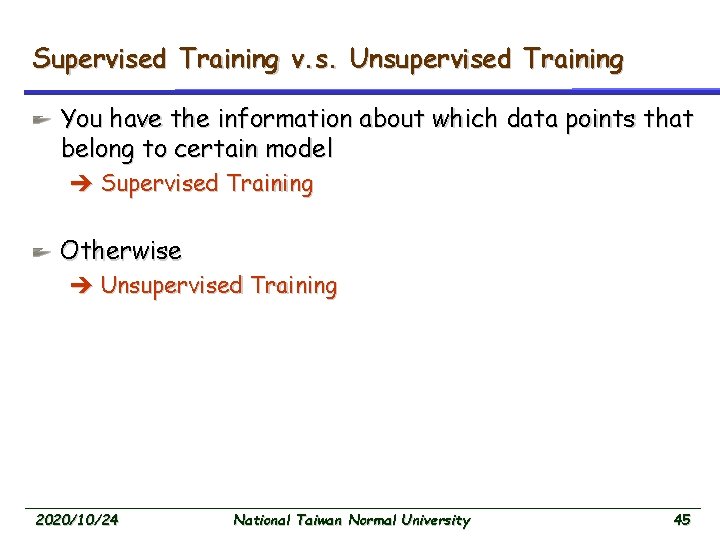
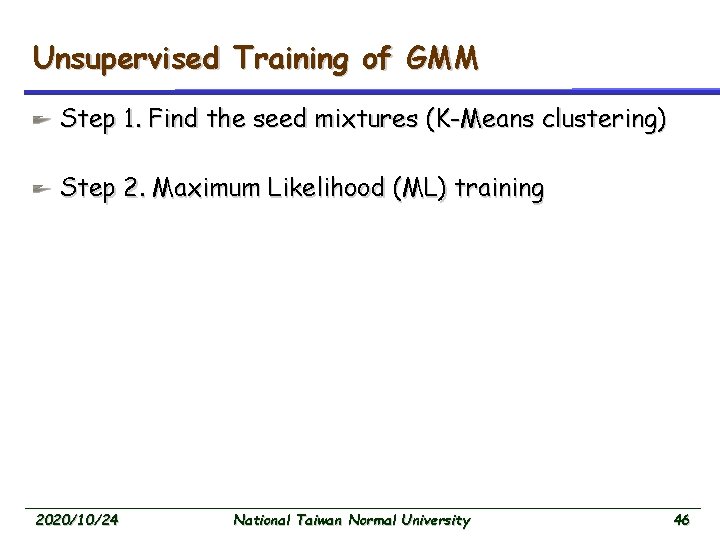
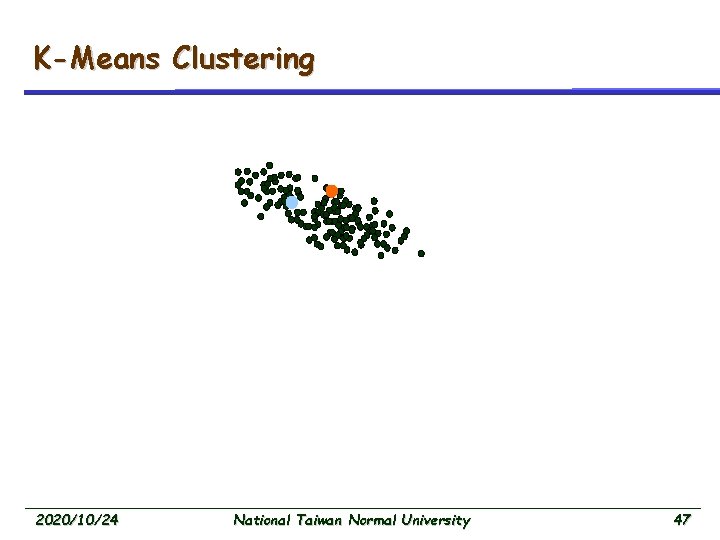
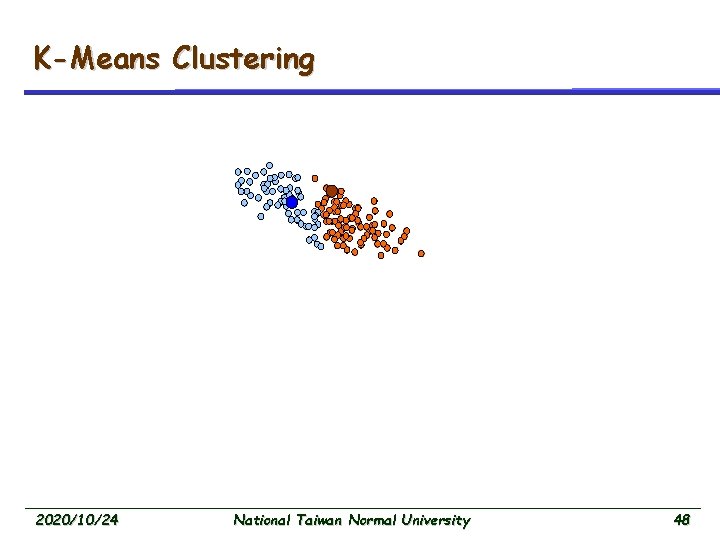
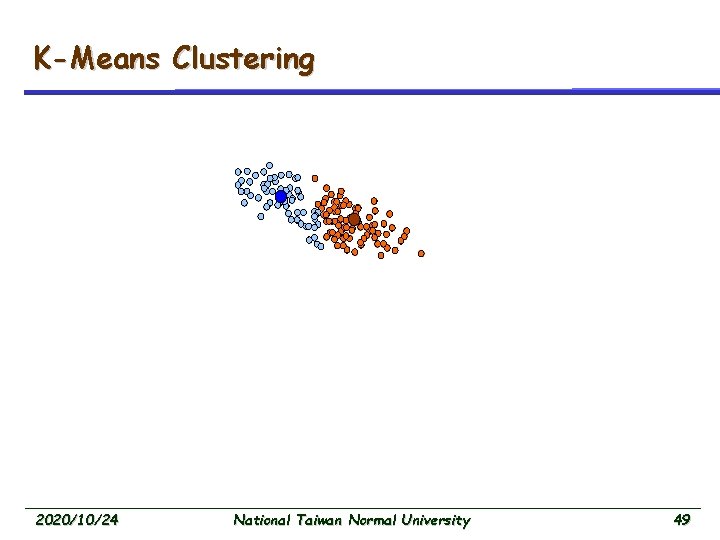
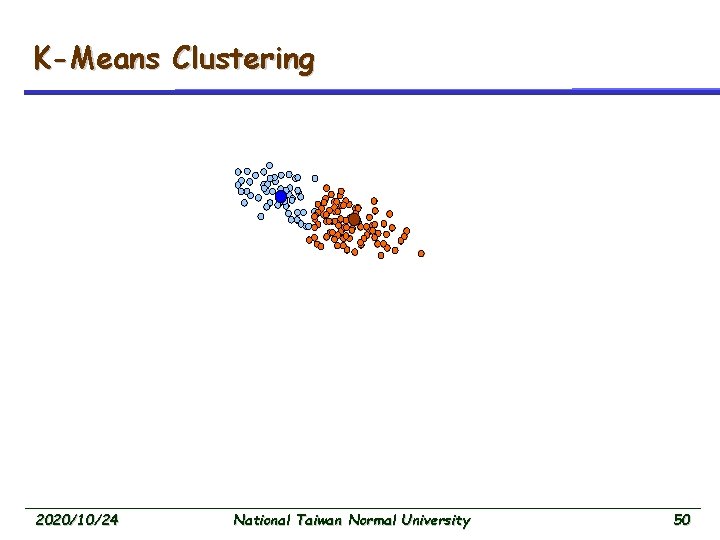
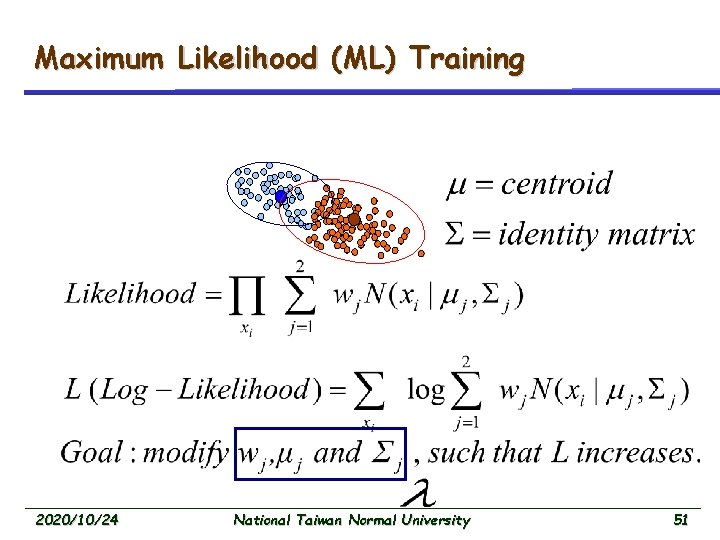
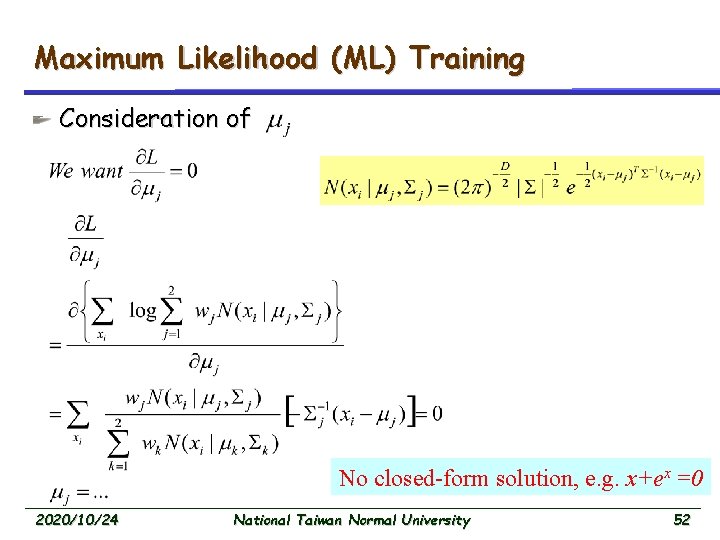
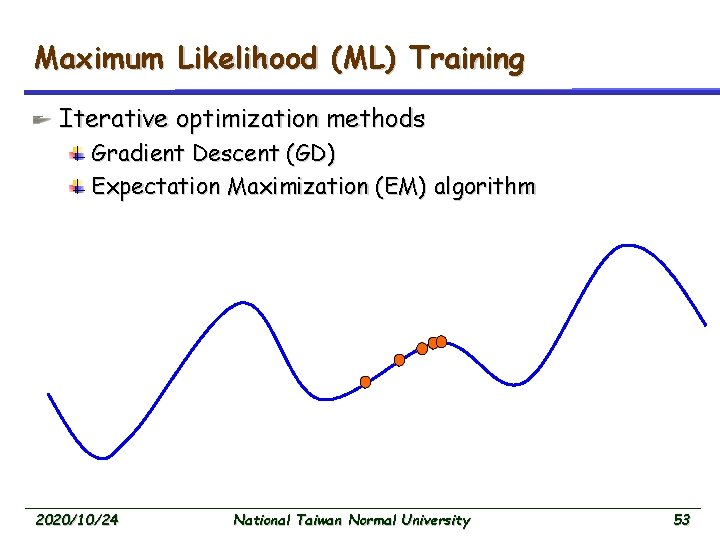
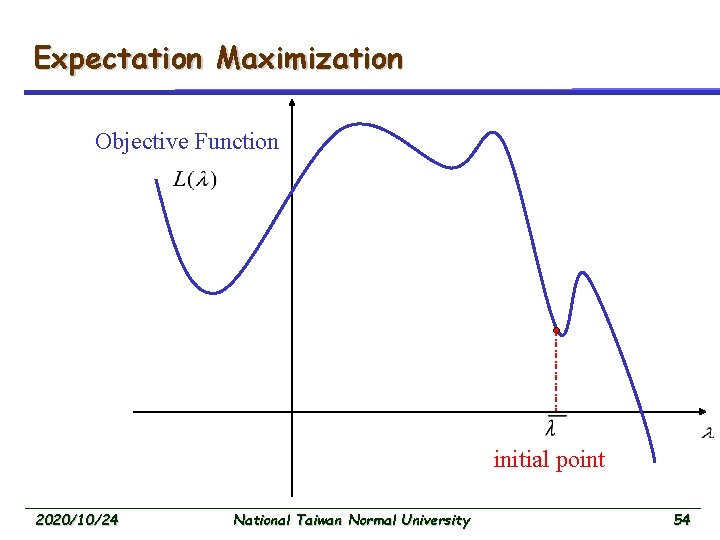
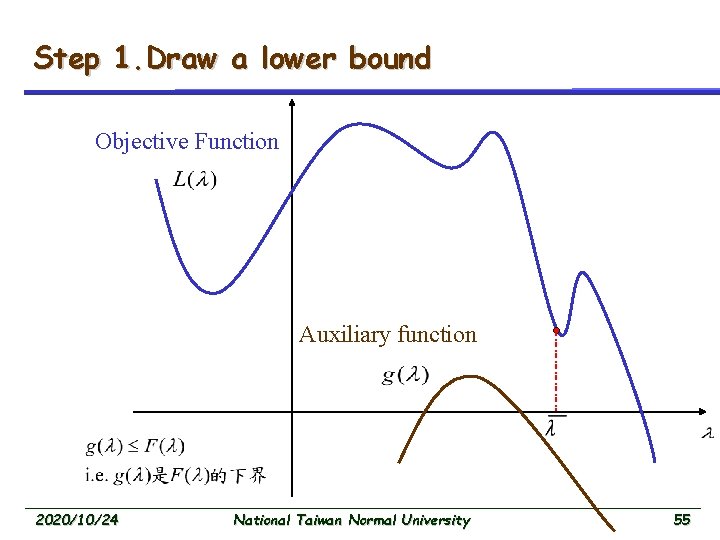
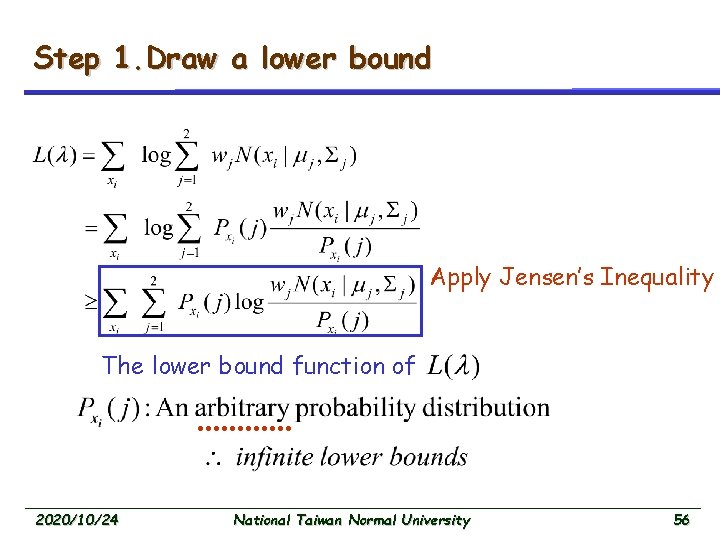
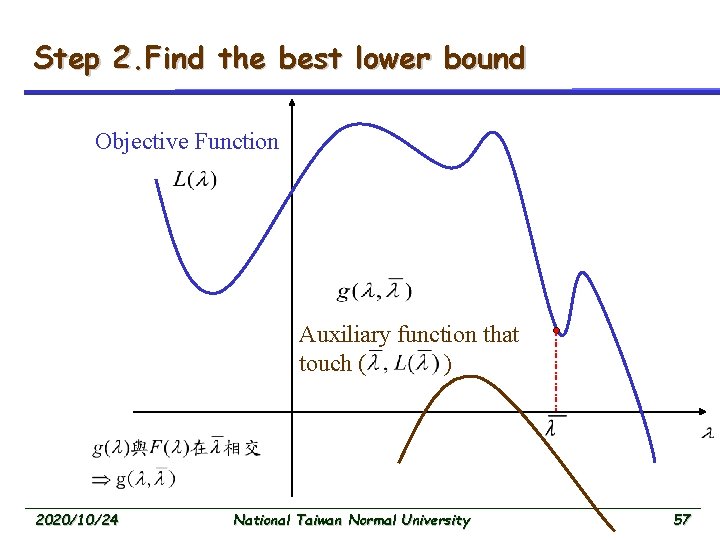
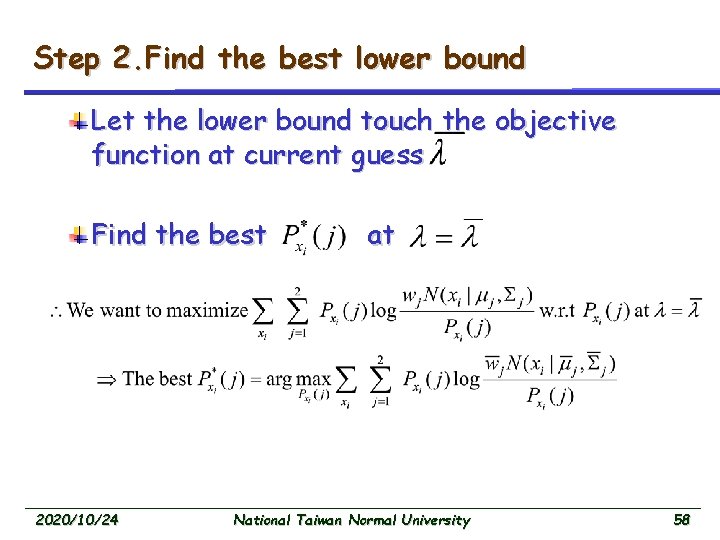
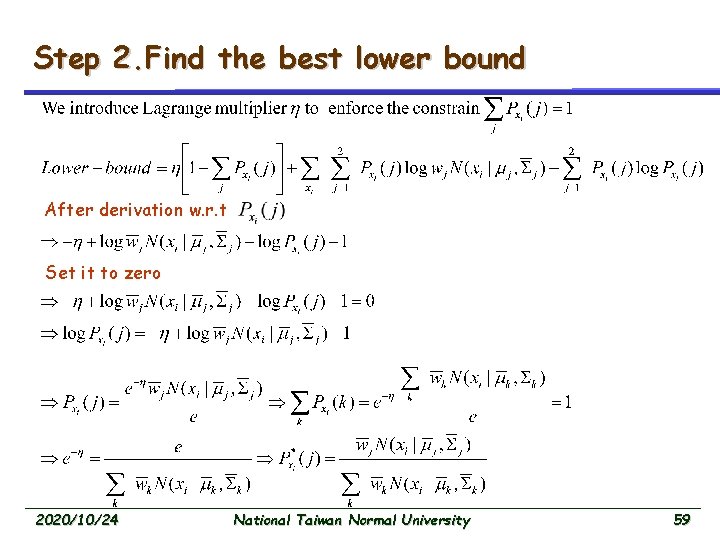
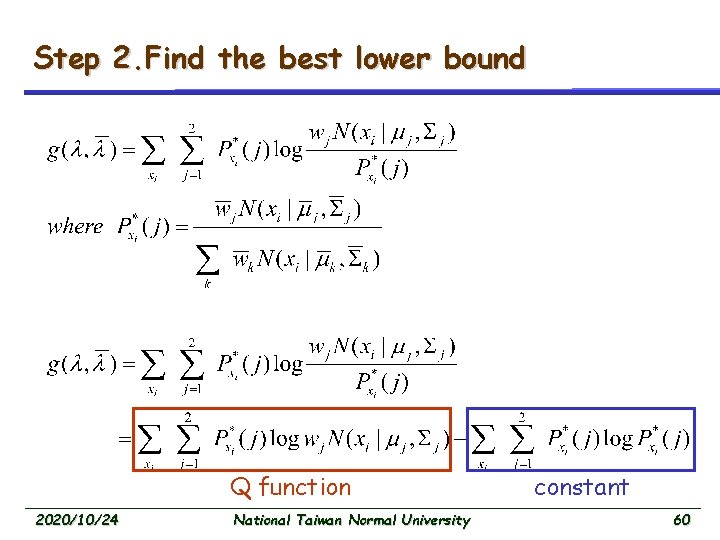
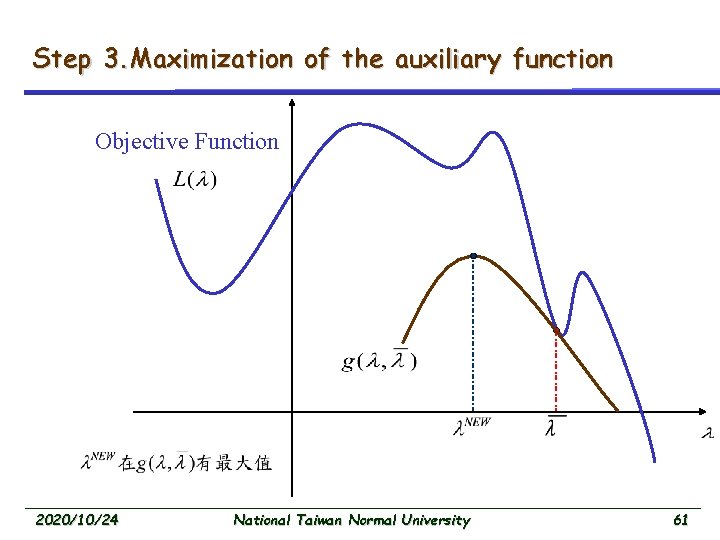
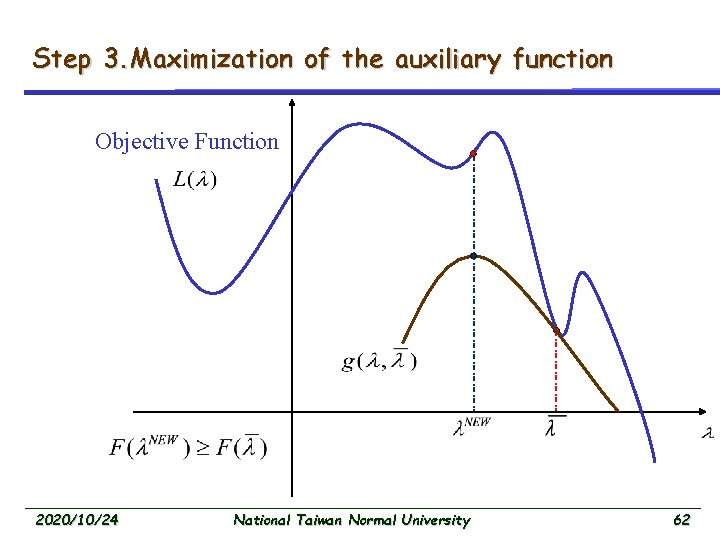
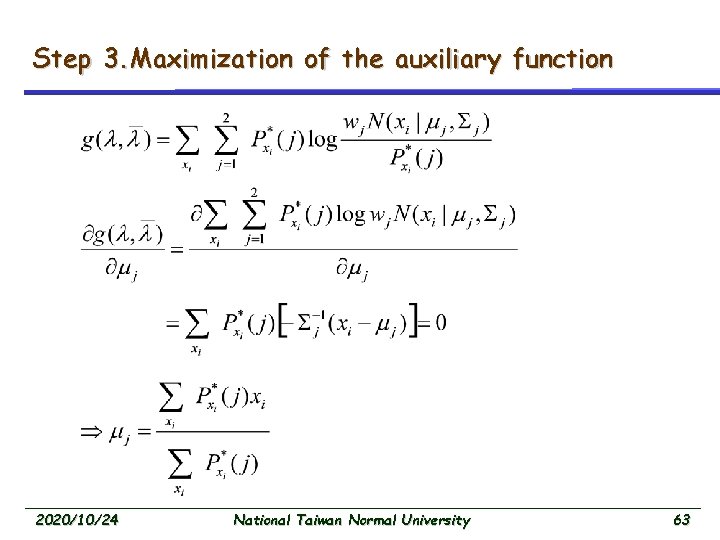
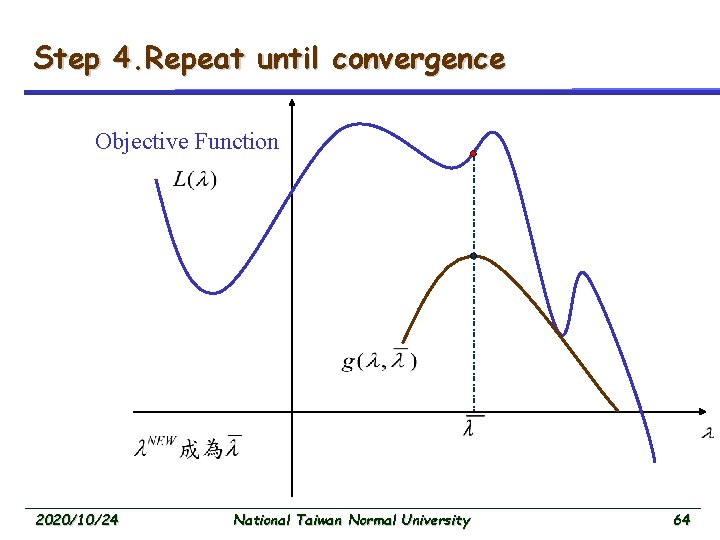
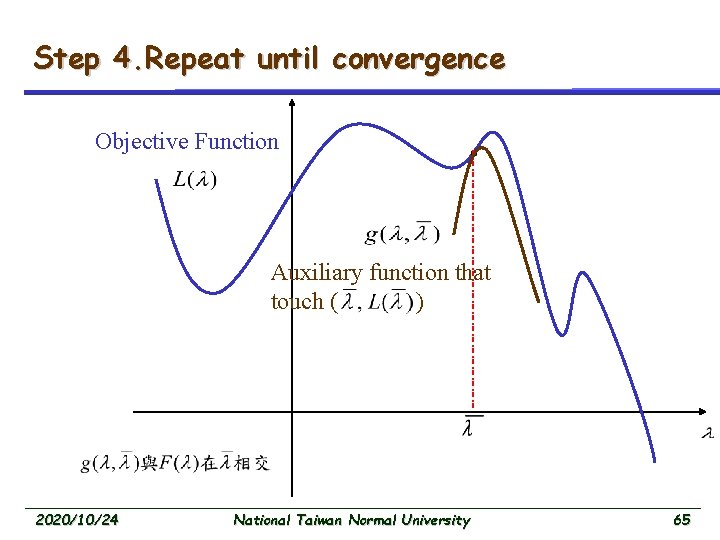
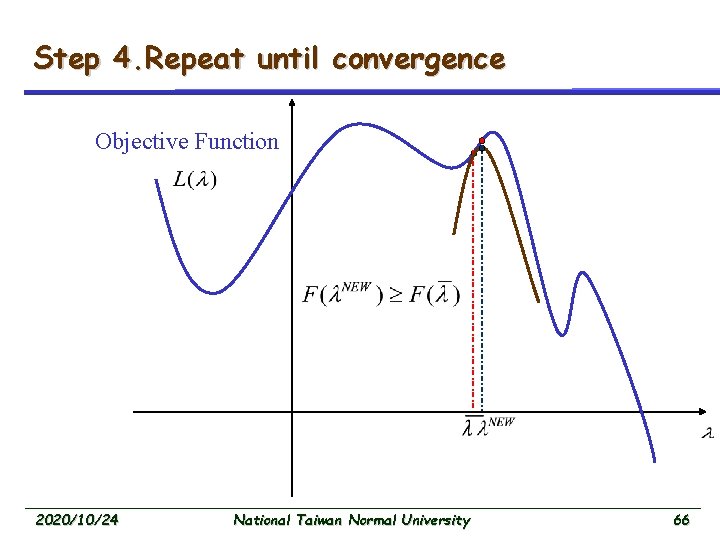
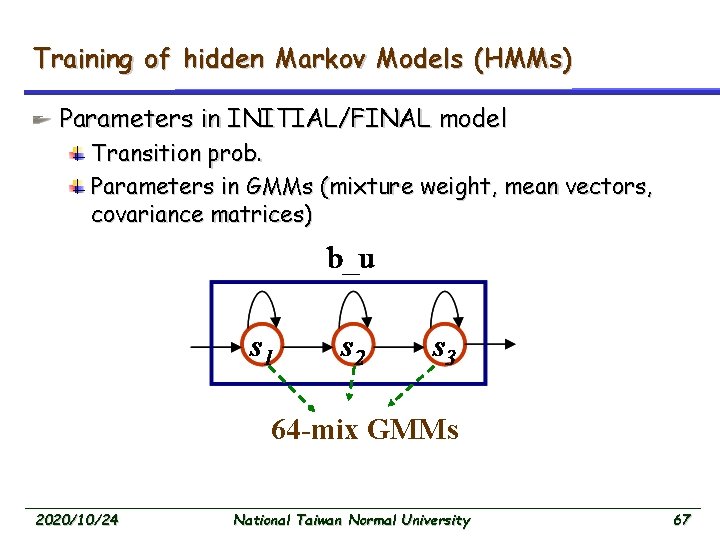
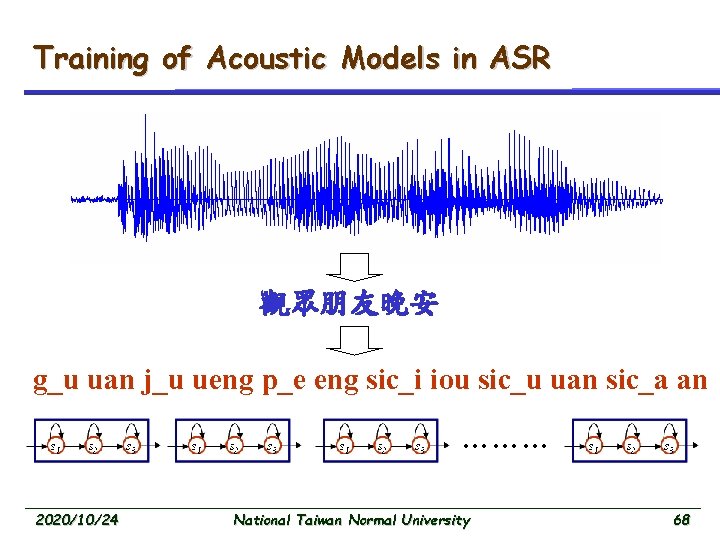
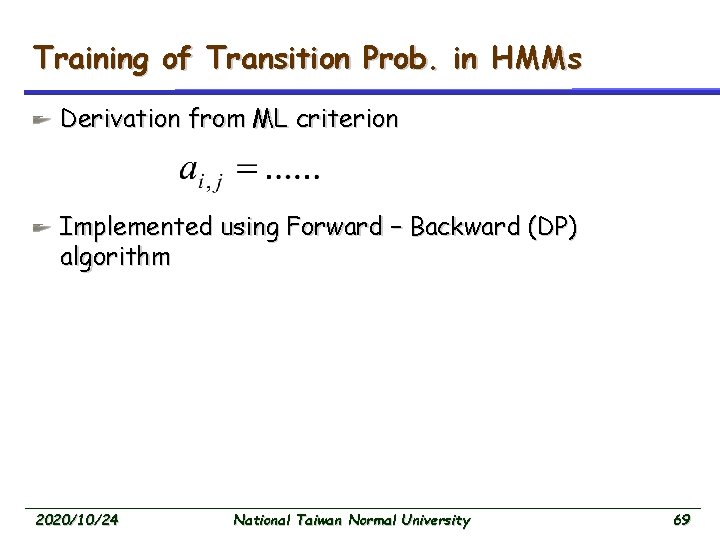
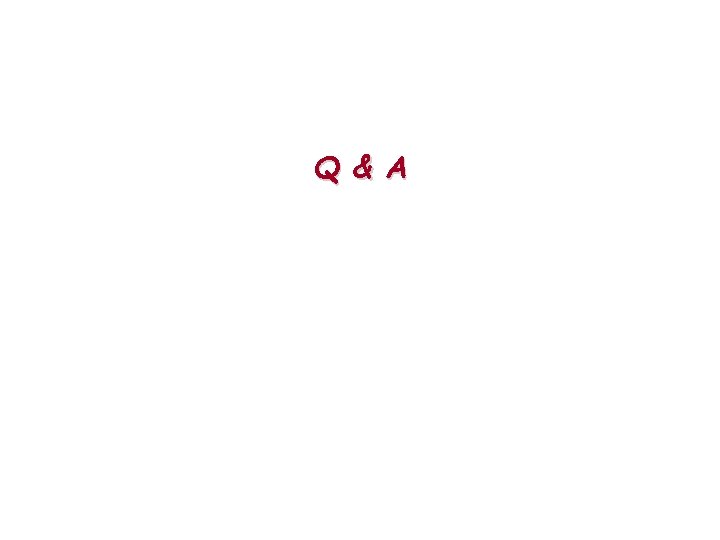
- Slides: 70
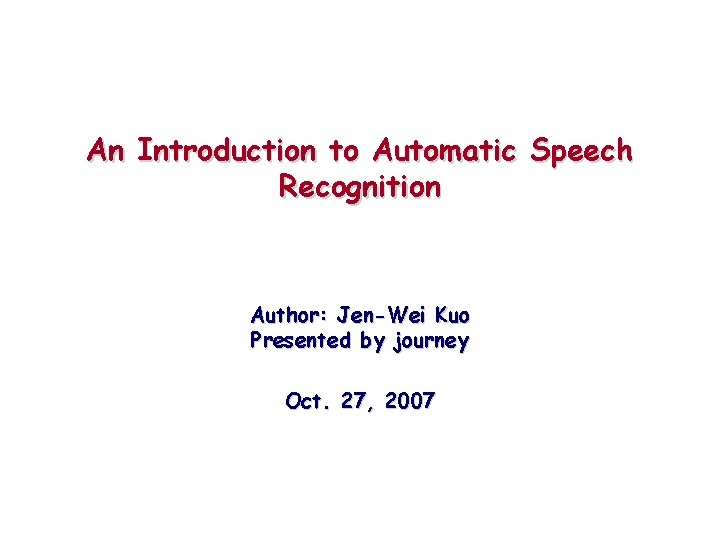
An Introduction to Automatic Speech Recognition Author: Jen-Wei Kuo Presented by journey Oct. 27, 2007
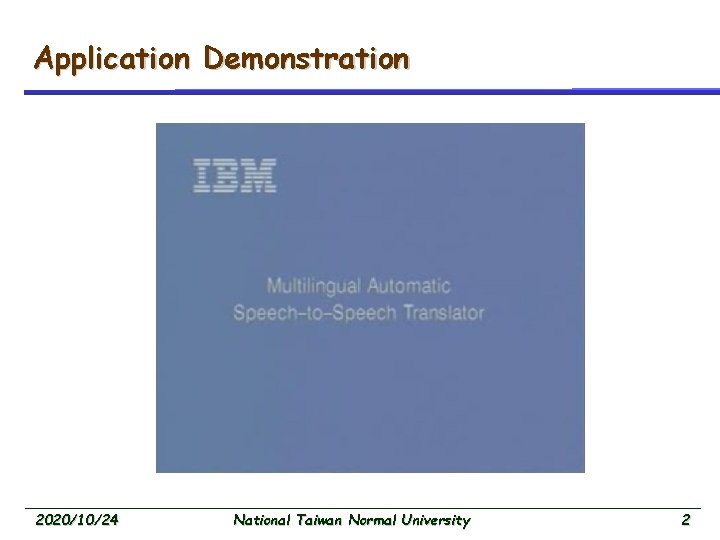
Application Demonstration 2020/10/24 National Taiwan Normal University 2
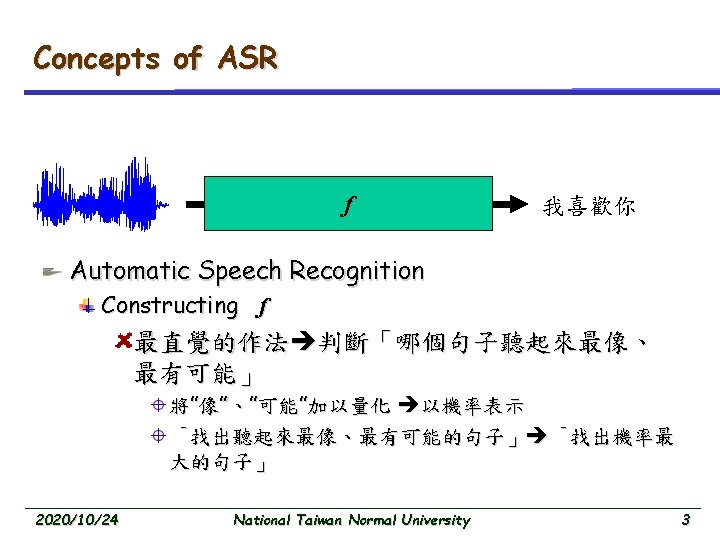
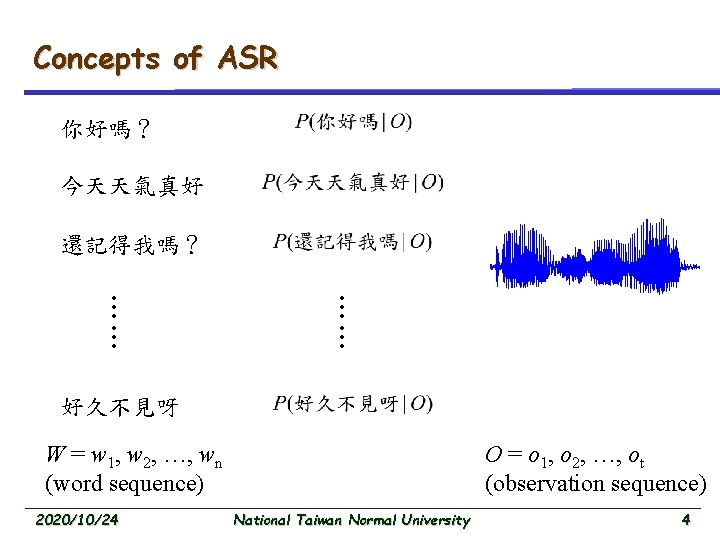
Concepts of ASR 你好嗎? 今天天氣真好 還記得我嗎? …… …… 好久不見呀 W = w 1, w 2, …, wn (word sequence) 2020/10/24 O = o 1, o 2, …, ot (observation sequence) National Taiwan Normal University 4
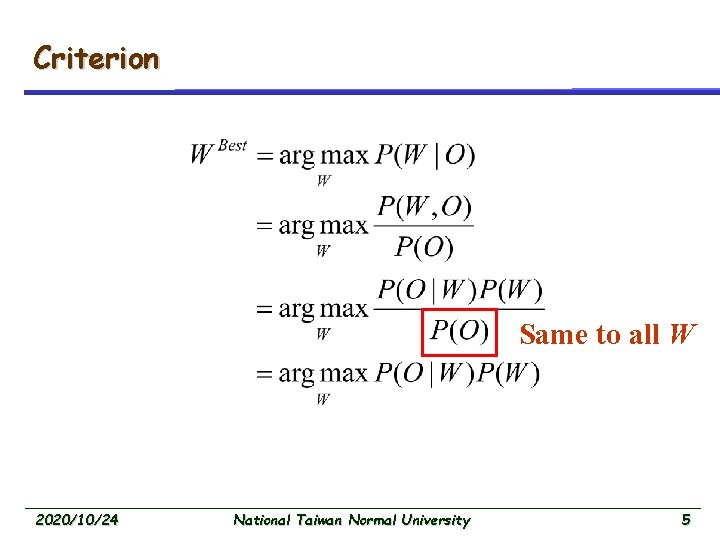
Criterion Same to all W 2020/10/24 National Taiwan Normal University 5
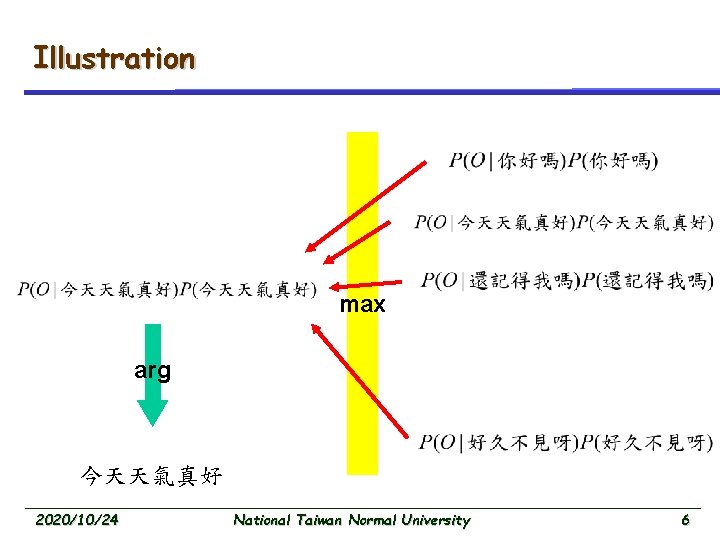
Illustration max arg 今天天氣真好 2020/10/24 National Taiwan Normal University 6
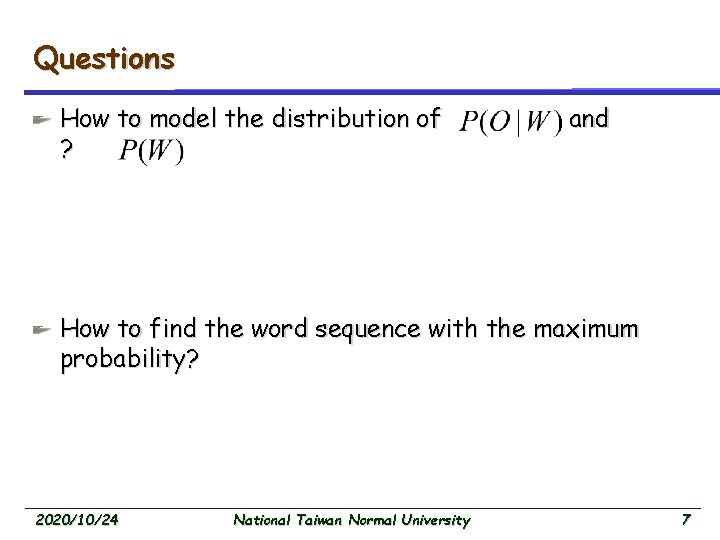
Questions How to model the distribution of ? and How to find the word sequence with the maximum probability? 2020/10/24 National Taiwan Normal University 7
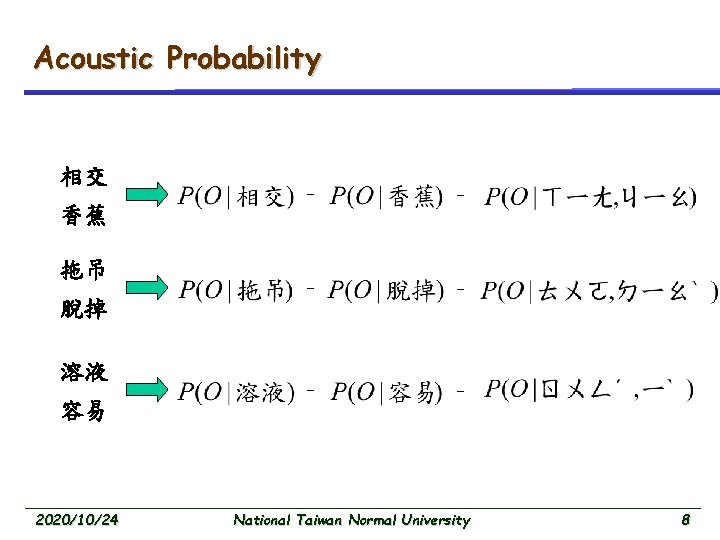
Acoustic Probability 相交 香蕉 拖吊 脫掉 溶液 容易 2020/10/24 = = = National Taiwan Normal University 8
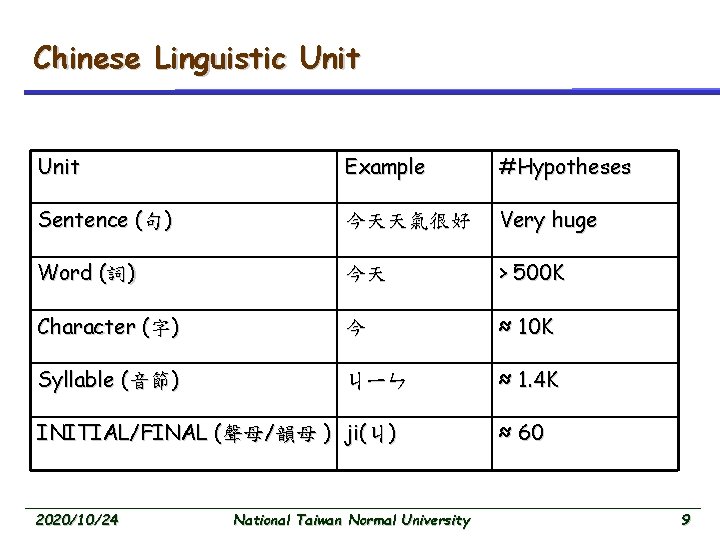
Chinese Linguistic Unit Example #Hypotheses Sentence (句) 今天天氣很好 Very huge Word (詞) 今天 > 500 K Character (字) 今 ≈ 10 K Syllable (音節) ㄐㄧㄣ ≈ 1. 4 K INITIAL/FINAL (聲母/韻母 ) ji(ㄐ) 2020/10/24 National Taiwan Normal University ≈ 60 9
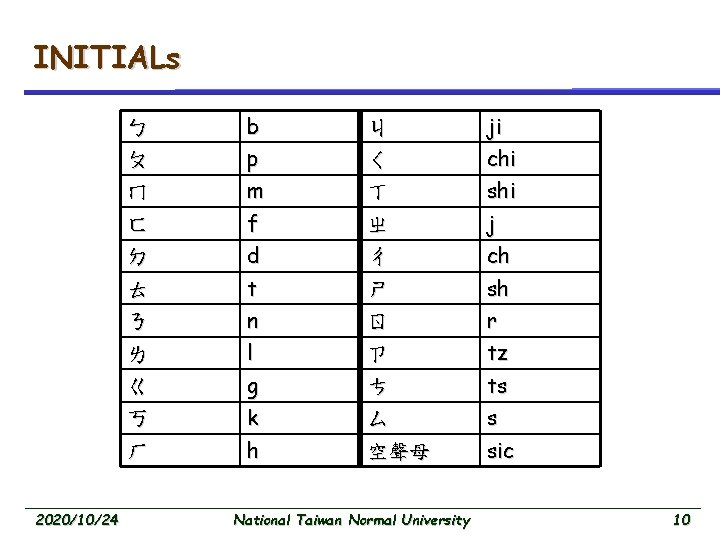
INITIALs b p ㄐ m f ㄒ d t ㄔ n l ㄖ ㄘ ㄎ g k ㄙ ts s ㄏ h 空聲母 sic ㄅ ㄆ ㄇ ㄈ ㄉ ㄊ ㄋ ㄌ ㄍ 2020/10/24 ㄑ ㄓ ㄕ ㄗ National Taiwan Normal University ji chi shi j ch sh r tz 10
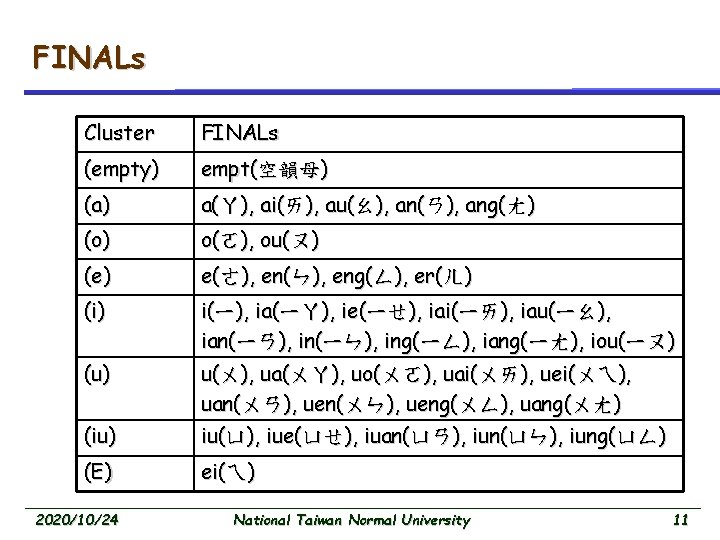
FINALs Cluster FINALs (empty) empt(空韻母) (a) a(ㄚ), ai(ㄞ), au(ㄠ), an(ㄢ), ang(ㄤ) (o) o(ㄛ), ou(ㄡ) (e) e(ㄜ), en(ㄣ), eng(ㄥ), er(ㄦ) (iu) i(ㄧ), ia(ㄧㄚ), ie(ㄧㄝ), iai(ㄧㄞ), iau(ㄧㄠ), ian(ㄧㄢ), in(ㄧㄣ), ing(ㄧㄥ), iang(ㄧㄤ), iou(ㄧㄡ) u(ㄨ), ua(ㄨㄚ), uo(ㄨㄛ), uai(ㄨㄞ), uei(ㄨㄟ), uan(ㄨㄢ), uen(ㄨㄣ), ueng(ㄨㄥ), uang(ㄨㄤ) iu(ㄩ), iue(ㄩㄝ), iuan(ㄩㄢ), iun(ㄩㄣ), iung(ㄩㄥ) (E) ei(ㄟ) (u) 2020/10/24 National Taiwan Normal University 11
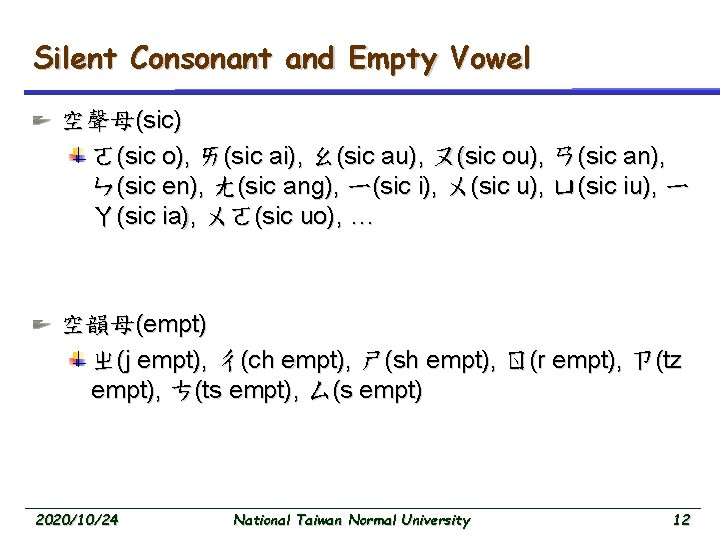
Silent Consonant and Empty Vowel 空聲母(sic) ㄛ(sic o), ㄞ(sic ai), ㄠ(sic au), ㄡ(sic ou), ㄢ(sic an), ㄣ(sic en), ㄤ(sic ang), ㄧ(sic i), ㄨ(sic u), ㄩ(sic iu), ㄧ ㄚ(sic ia), ㄨㄛ(sic uo), … 空韻母(empt) ㄓ(j empt), ㄔ(ch empt), ㄕ(sh empt), ㄖ(r empt), ㄗ(tz empt), ㄘ(ts empt), ㄙ(s empt) 2020/10/24 National Taiwan Normal University 12
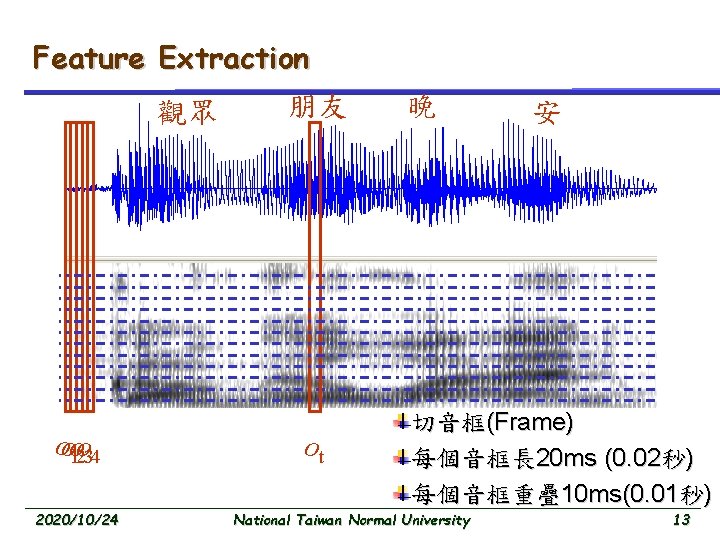
Feature Extraction 觀眾 ooo 1 o 234 2020/10/24 朋友 ot 晚 安 切音框(Frame) 每個音框長 20 ms (0. 02秒) 每個音框重疊10 ms(0. 01秒) National Taiwan Normal University 13
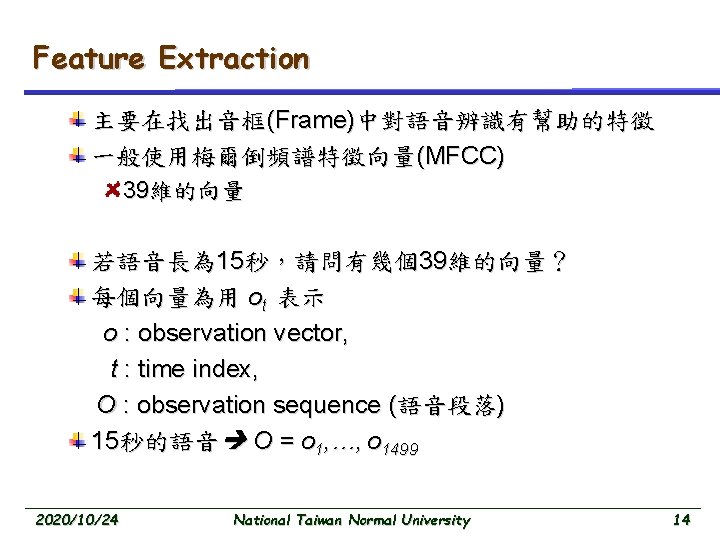
Feature Extraction 主要在找出音框(Frame)中對語音辨識有幫助的特徵 一般使用梅爾倒頻譜特徵向量(MFCC) 39維的向量 若語音長為 15秒,請問有幾個39維的向量? 每個向量為用 ot 表示 o : observation vector, t : time index, O : observation sequence (語音段落) 15秒的語音 O = o 1, …, o 1499 2020/10/24 National Taiwan Normal University 14
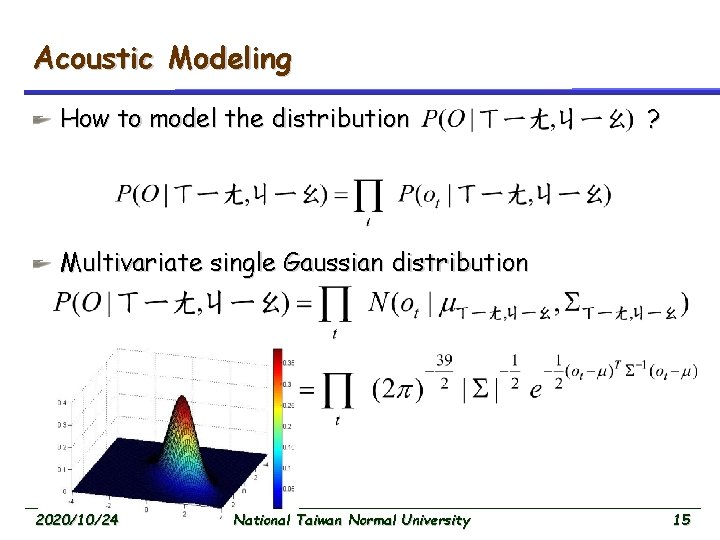
Acoustic Modeling How to model the distribution ? Multivariate single Gaussian distribution 2020/10/24 National Taiwan Normal University 15
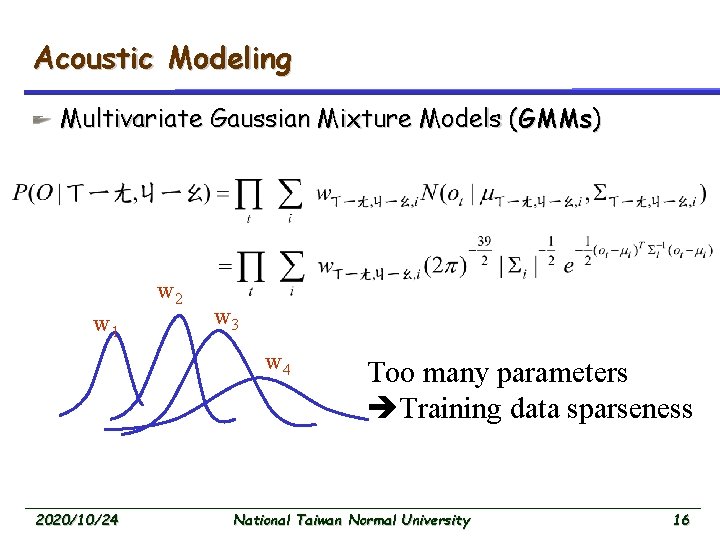
Acoustic Modeling Multivariate Gaussian Mixture Models (GMMs) w 2 w 1 w 3 w 4 2020/10/24 Too many parameters Training data sparseness National Taiwan Normal University 16
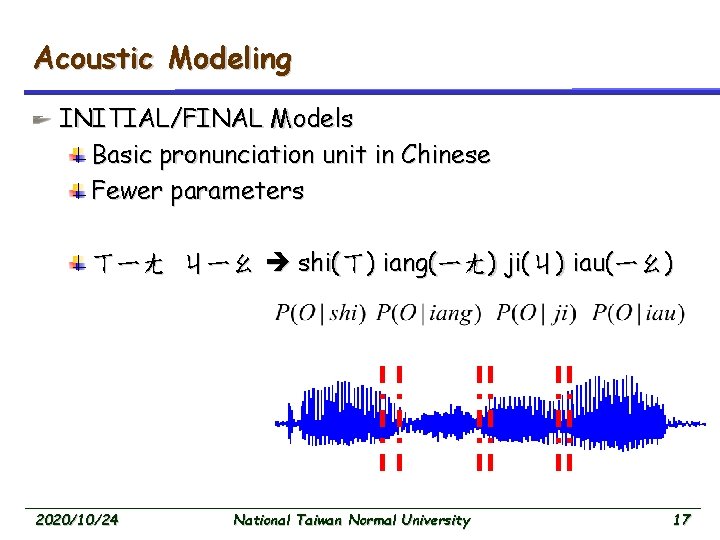
Acoustic Modeling INITIAL/FINAL Models Basic pronunciation unit in Chinese Fewer parameters ㄒㄧㄤ ㄐㄧㄠ shi(ㄒ) iang(ㄧㄤ) ji(ㄐ) iau(ㄧㄠ) 2020/10/24 National Taiwan Normal University 17
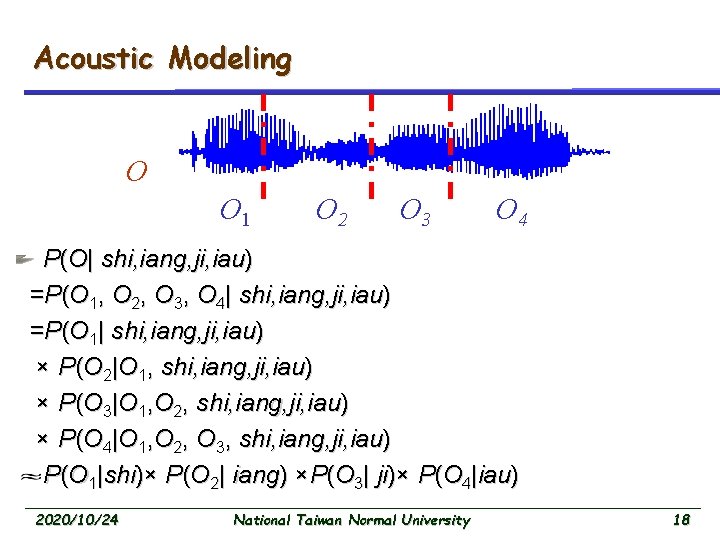
Acoustic Modeling O O 1 O 2 O 3 O 4 P(O| shi, iang, ji, iau) =P(O 1, O 2, O 3, O 4| shi, iang, ji, iau) =P(O 1| shi, iang, ji, iau) × P(O 2|O 1, shi, iang, ji, iau) × P(O 3|O 1, O 2, shi, iang, ji, iau) × P(O 4|O 1, O 2, O 3, shi, iang, ji, iau) P(O 1|shi)× P(O 2| iang) ×P(O 3| ji)× P(O 4|iau) 2020/10/24 National Taiwan Normal University 18
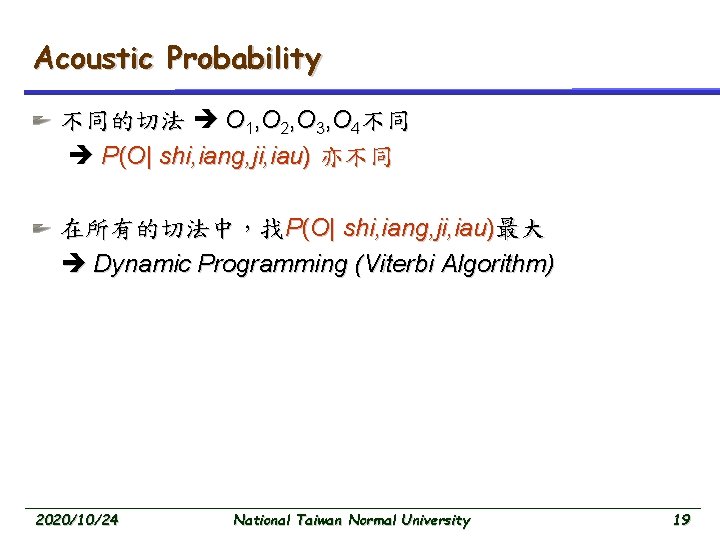
Acoustic Probability 不同的切法 O 1, O 2, O 3, O 4不同 P(O| shi, iang, ji, iau) 亦不同 在所有的切法中,找P(O| shi, iang, ji, iau)最大 Dynamic Programming (Viterbi Algorithm) 2020/10/24 National Taiwan Normal University 19
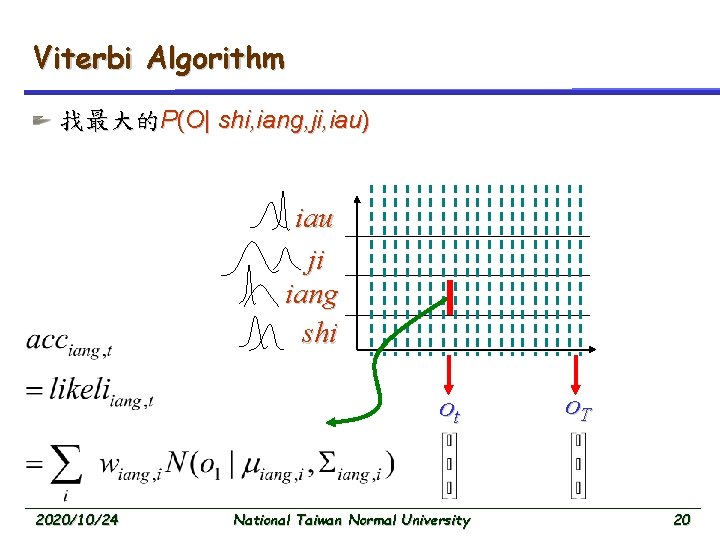
Viterbi Algorithm 找最大的P(O| shi, iang, ji, iau) iau ji iang shi ot 2020/10/24 National Taiwan Normal University o. T 20
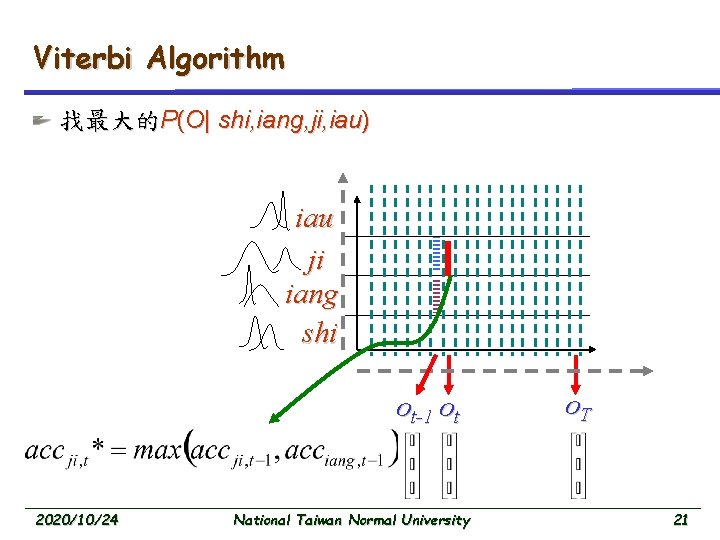
Viterbi Algorithm 找最大的P(O| shi, iang, ji, iau) iau ji iang shi ot-1 ot 2020/10/24 National Taiwan Normal University o. T 21
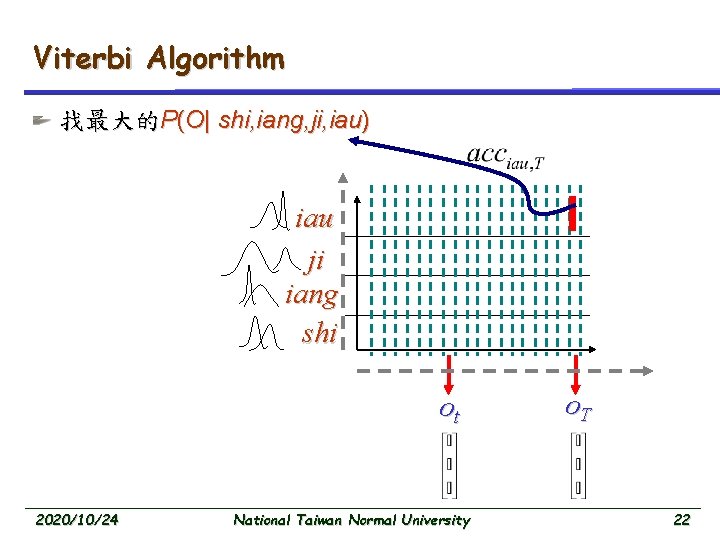
Viterbi Algorithm 找最大的P(O| shi, iang, ji, iau) iau ji iang shi ot 2020/10/24 National Taiwan Normal University o. T 22
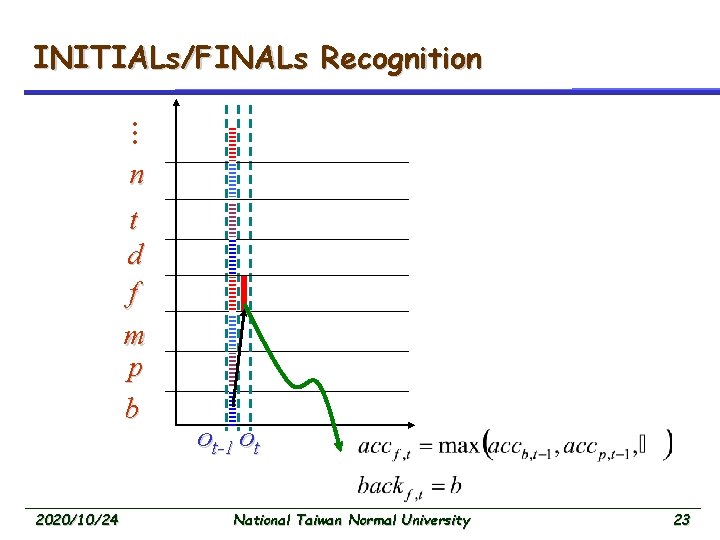
INITIALs/FINALs Recognition … n t d f m p b 2020/10/24 ot-1 ot National Taiwan Normal University 23
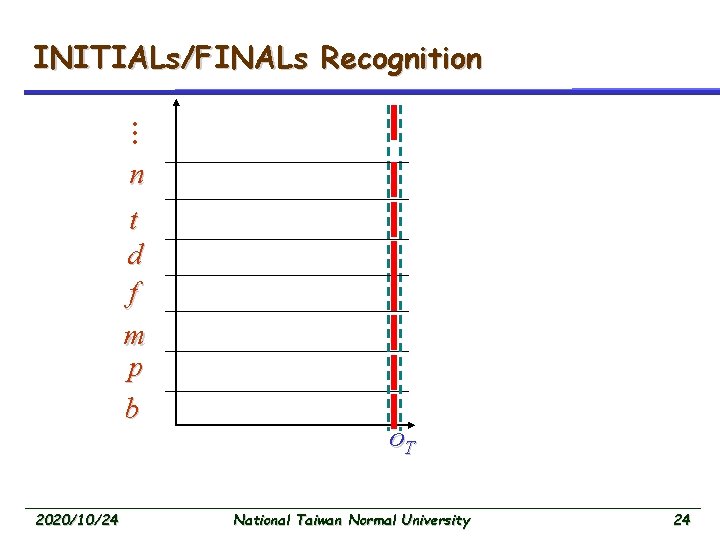
INITIALs/FINALs Recognition … n t d f m p b 2020/10/24 o. T National Taiwan Normal University 24
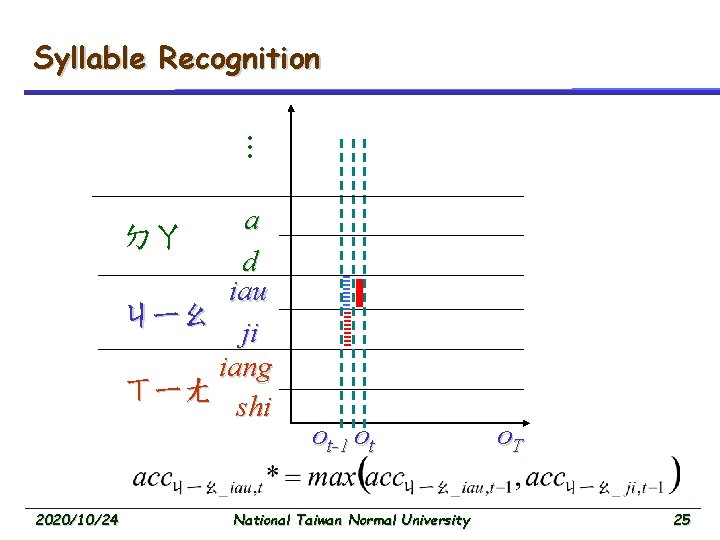
Syllable Recognition … a ㄉㄚ d iau ㄐㄧㄠ ji iang ㄒㄧㄤ shi 2020/10/24 ot-1 ot National Taiwan Normal University o. T 25
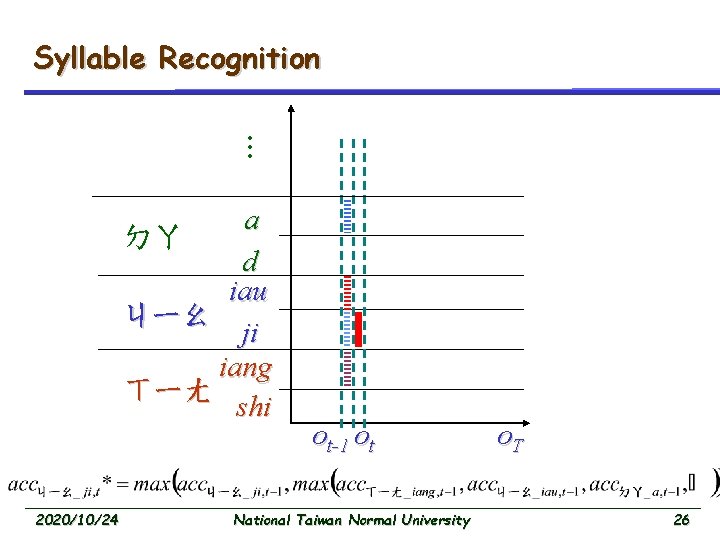
Syllable Recognition … a ㄉㄚ d iau ㄐㄧㄠ ji iang ㄒㄧㄤ shi 2020/10/24 ot-1 ot National Taiwan Normal University o. T 26
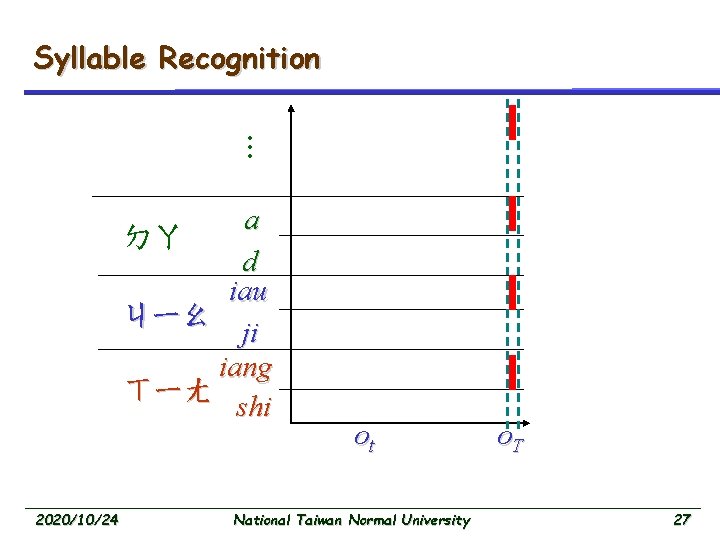
Syllable Recognition … a ㄉㄚ d iau ㄐㄧㄠ ji iang ㄒㄧㄤ shi 2020/10/24 ot National Taiwan Normal University o. T 27
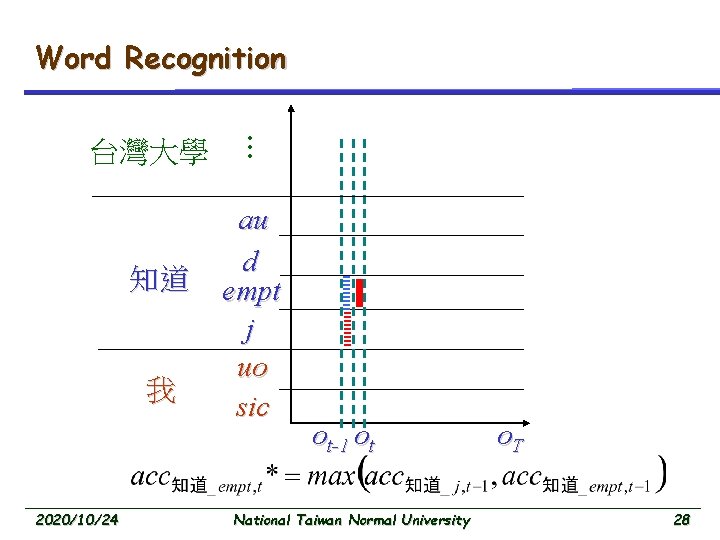
Word Recognition 知道 我 2020/10/24 … 台灣大學 au d empt j uo sic ot-1 ot National Taiwan Normal University o. T 28
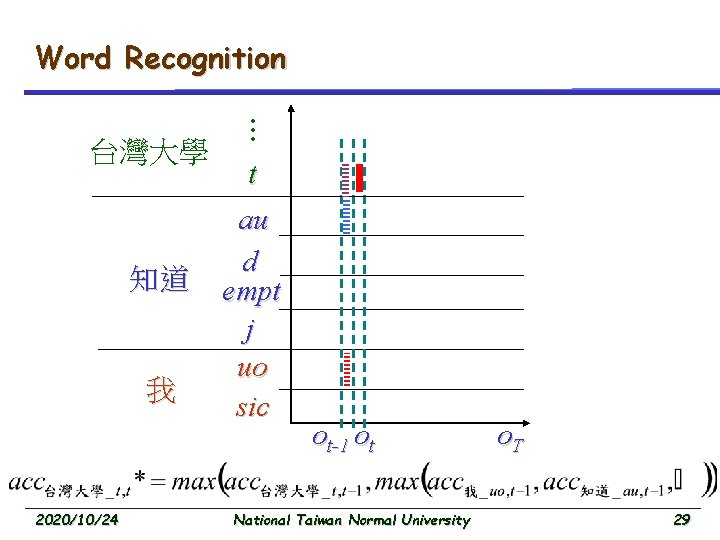
Word Recognition 知道 我 2020/10/24 … 台灣大學 t au d empt j uo sic ot-1 ot National Taiwan Normal University o. T 29
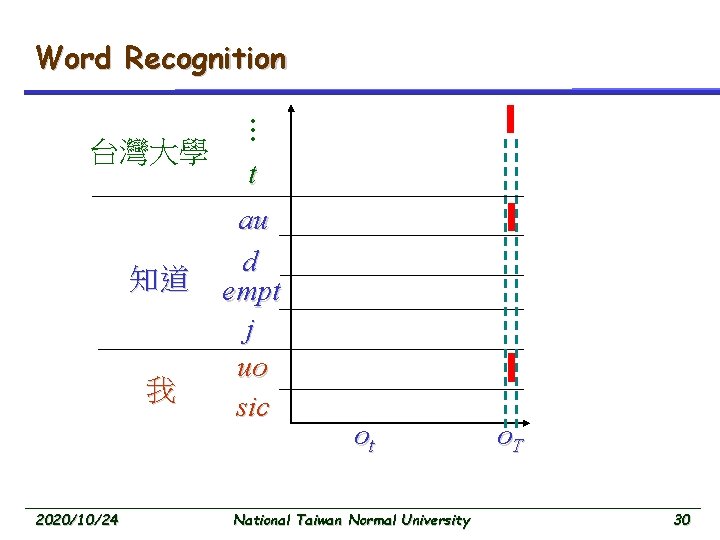
Word Recognition 知道 我 2020/10/24 … 台灣大學 t au d empt j uo sic ot National Taiwan Normal University o. T 30
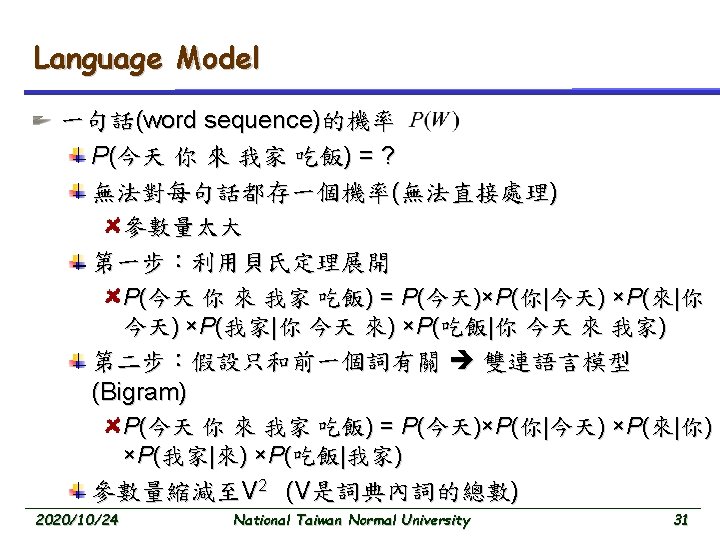
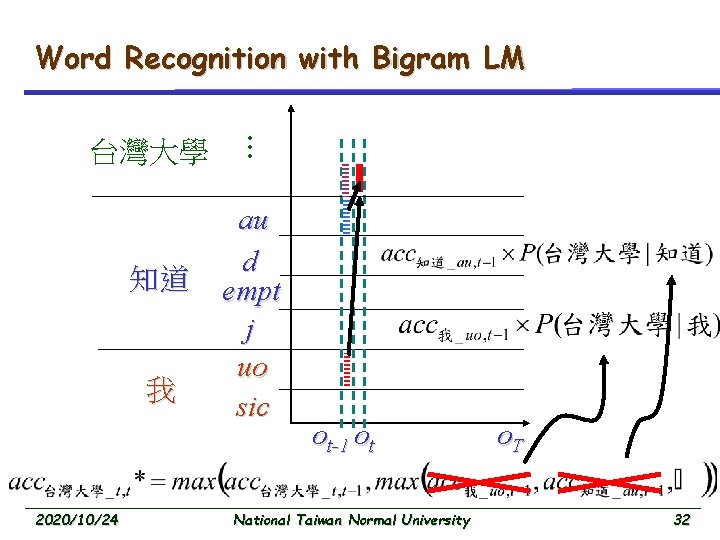
Word Recognition with Bigram LM 知道 我 2020/10/24 … 台灣大學 au d empt j uo sic ot-1 ot National Taiwan Normal University o. T 32
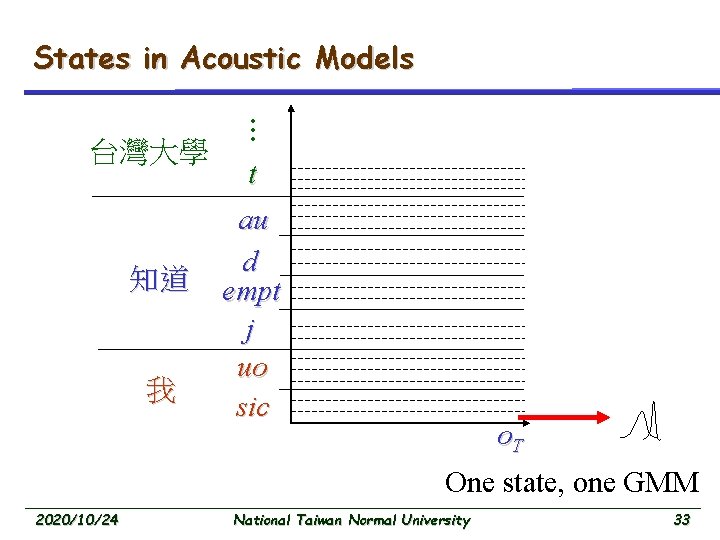
States in Acoustic Models 知道 我 … 台灣大學 t au d empt j uo sic o. T One state, one GMM 2020/10/24 National Taiwan Normal University 33
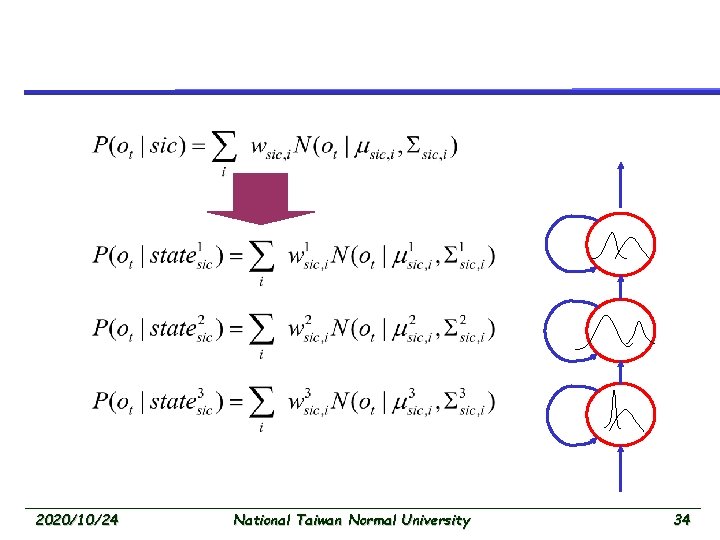
2020/10/24 National Taiwan Normal University 34
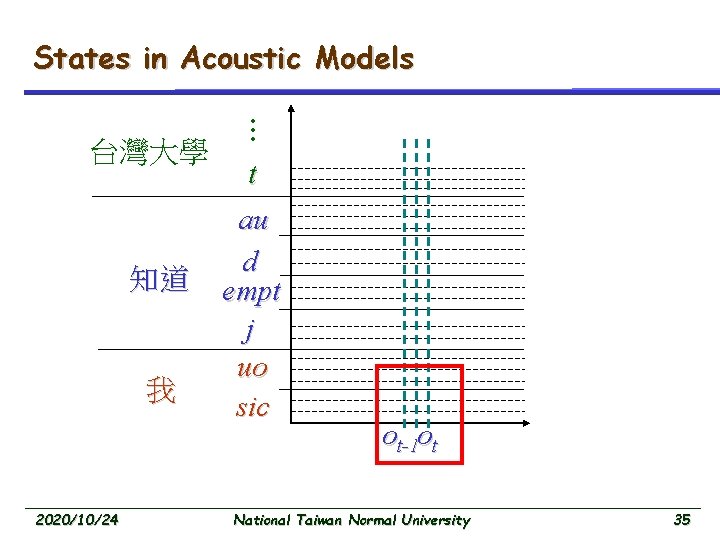
States in Acoustic Models 知道 我 2020/10/24 … 台灣大學 t au d empt j uo sic ot-1 ot National Taiwan Normal University 35
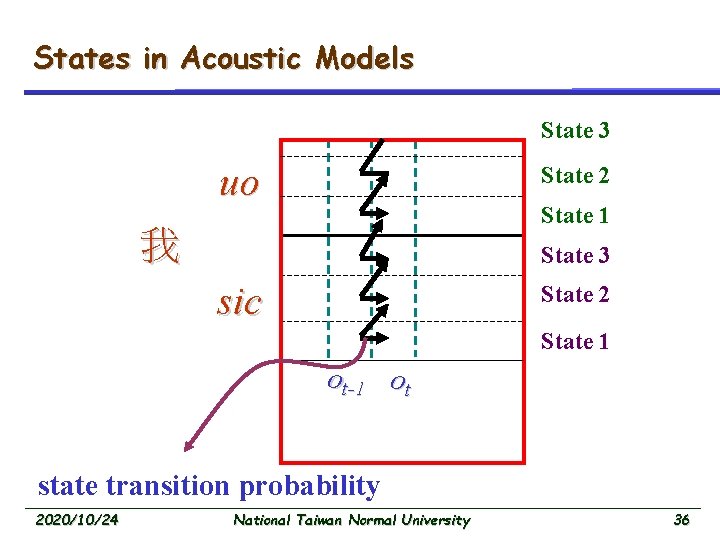
States in Acoustic Models State 3 uo State 2 State 1 我 State 3 sic State 2 State 1 ot-1 ot state transition probability 2020/10/24 National Taiwan Normal University 36
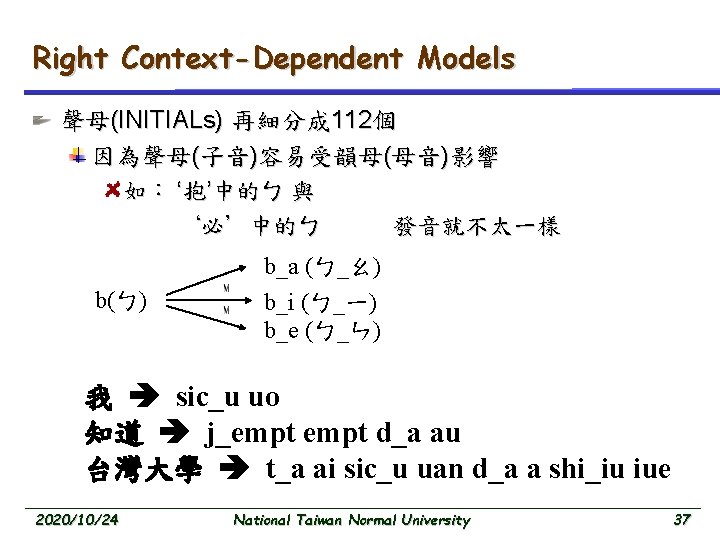
Right Context-Dependent Models 聲母(INITIALs) 再細分成 112個 因為聲母(子音)容易受韻母(母音)影響 如: ‘抱’中的ㄅ 與 ‘必’中的ㄅ 發音就不太一樣 b(ㄅ) b_a (ㄅ_ㄠ) b_i (ㄅ_一) b_e (ㄅ_ㄣ) 我 sic_u uo 知道 j_empt d_a au 台灣大學 t_a ai sic_u uan d_a a shi_iu iue 2020/10/24 National Taiwan Normal University 37
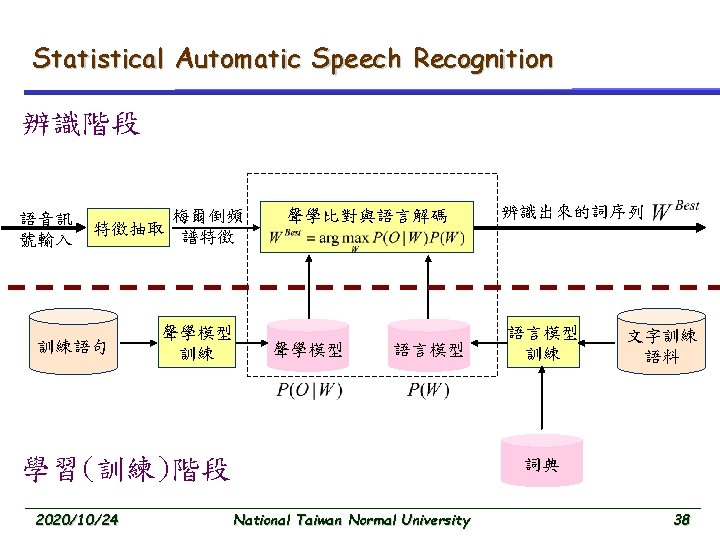
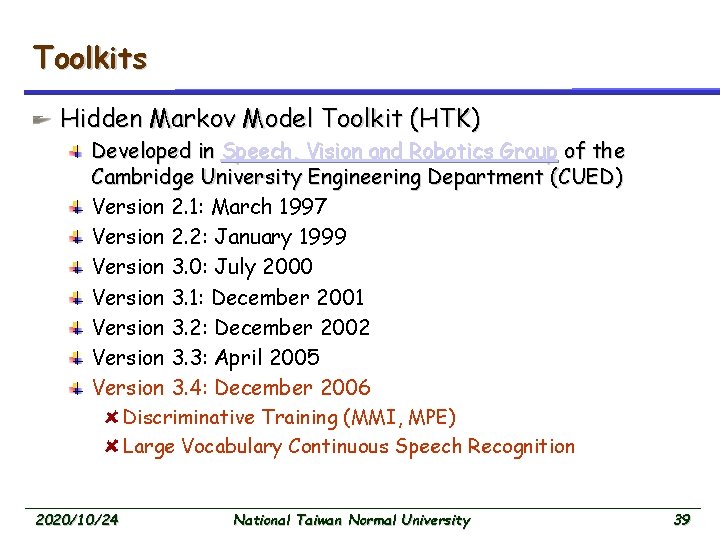
Toolkits Hidden Markov Model Toolkit (HTK) Developed in Speech, Vision and Robotics Group of the Cambridge University Engineering Department (CUED) Version 2. 1: March 1997 Version 2. 2: January 1999 Version 3. 0: July 2000 Version 3. 1: December 2001 Version 3. 2: December 2002 Version 3. 3: April 2005 Version 3. 4: December 2006 Discriminative Training (MMI, MPE) Large Vocabulary Continuous Speech Recognition 2020/10/24 National Taiwan Normal University 39
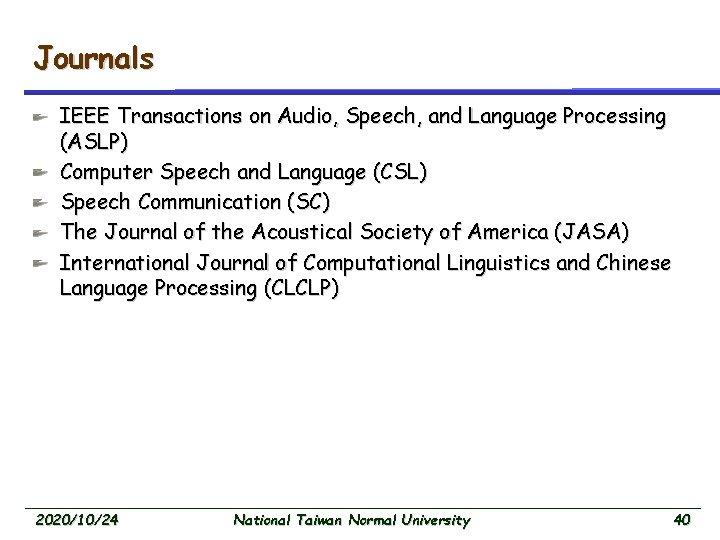
Journals IEEE Transactions on Audio, Speech, and Language Processing (ASLP) Computer Speech and Language (CSL) Speech Communication (SC) The Journal of the Acoustical Society of America (JASA) International Journal of Computational Linguistics and Chinese Language Processing (CLCLP) 2020/10/24 National Taiwan Normal University 40
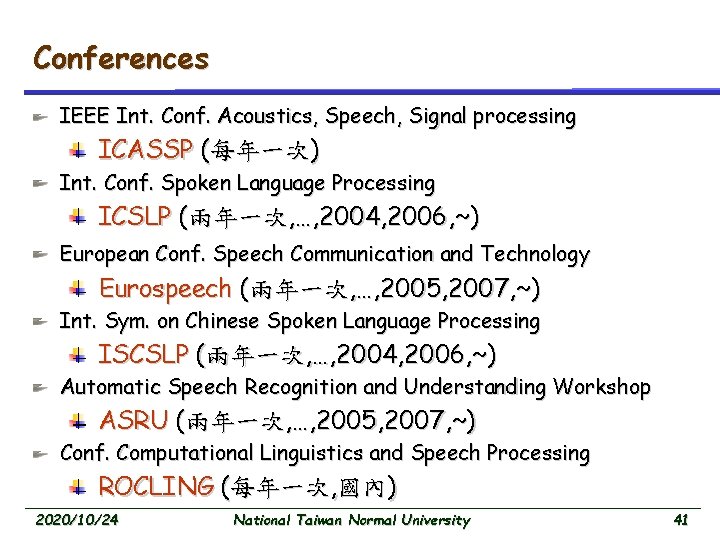
Conferences IEEE Int. Conf. Acoustics, Speech, Signal processing ICASSP (每年一次) Int. Conf. Spoken Language Processing ICSLP (兩年一次, …, 2004, 2006, ~) European Conf. Speech Communication and Technology Eurospeech (兩年一次, …, 2005, 2007, ~) Int. Sym. on Chinese Spoken Language Processing ISCSLP (兩年一次, …, 2004, 2006, ~) Automatic Speech Recognition and Understanding Workshop ASRU (兩年一次, …, 2005, 2007, ~) Conf. Computational Linguistics and Speech Processing ROCLING (每年一次, 國內) 2020/10/24 National Taiwan Normal University 41
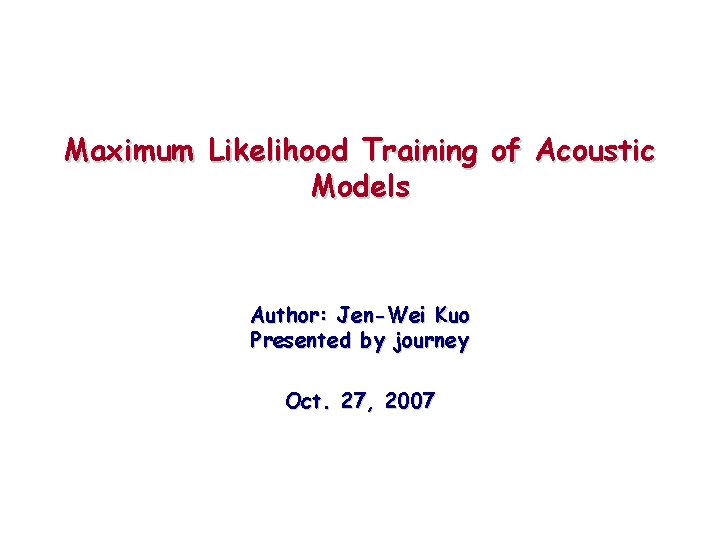
Maximum Likelihood Training of Acoustic Models Author: Jen-Wei Kuo Presented by journey Oct. 27, 2007
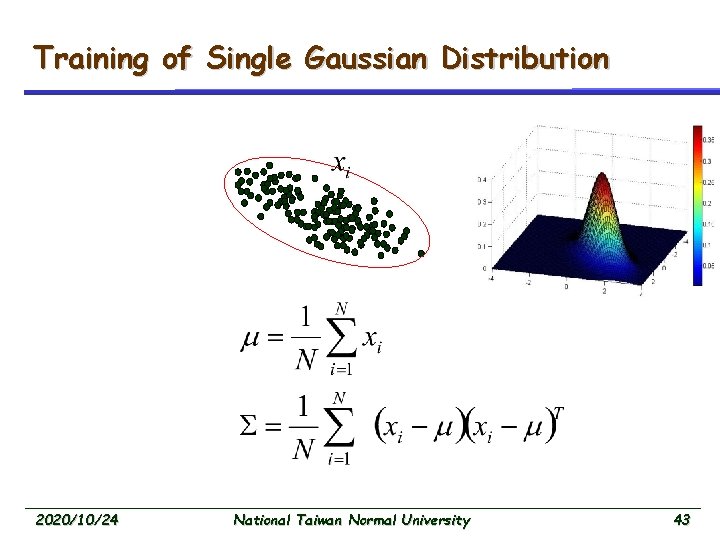
Training of Single Gaussian Distribution 2020/10/24 National Taiwan Normal University 43
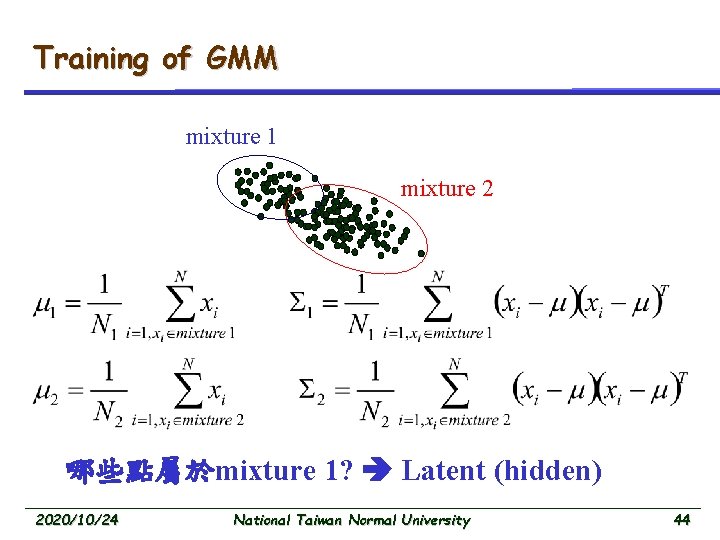
Training of GMM mixture 1 mixture 2 哪些點屬於mixture 1? Latent (hidden) 2020/10/24 National Taiwan Normal University 44
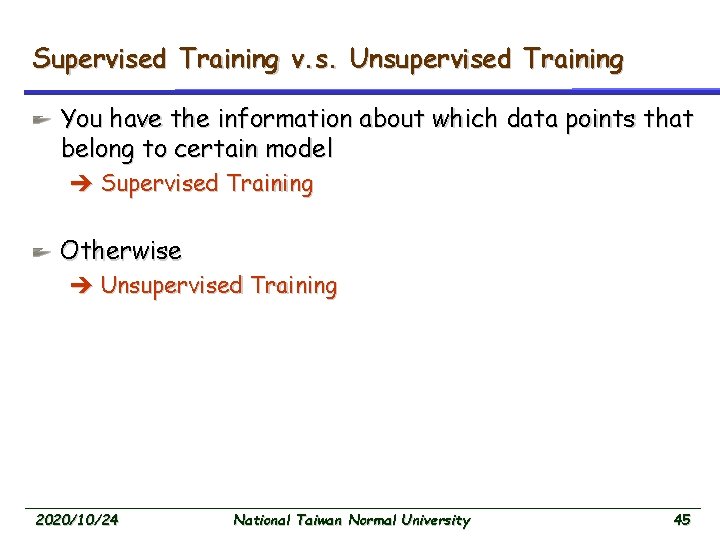
Supervised Training v. s. Unsupervised Training You have the information about which data points that belong to certain model Supervised Training Otherwise Unsupervised Training 2020/10/24 National Taiwan Normal University 45
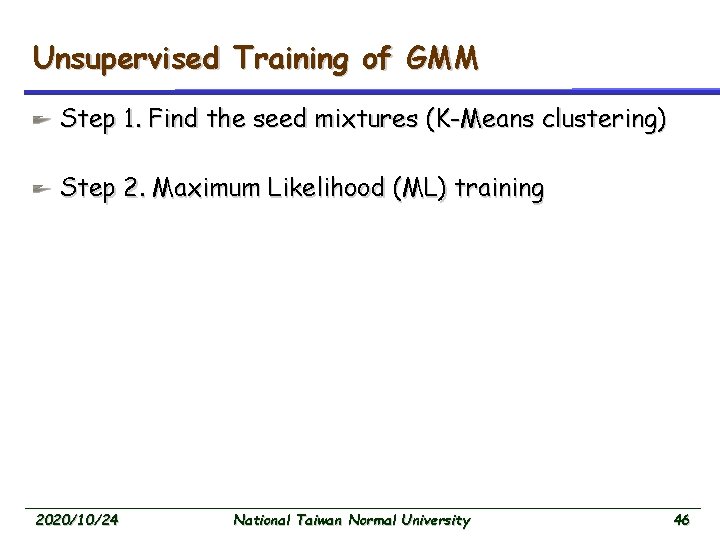
Unsupervised Training of GMM Step 1. Find the seed mixtures (K-Means clustering) Step 2. Maximum Likelihood (ML) training 2020/10/24 National Taiwan Normal University 46
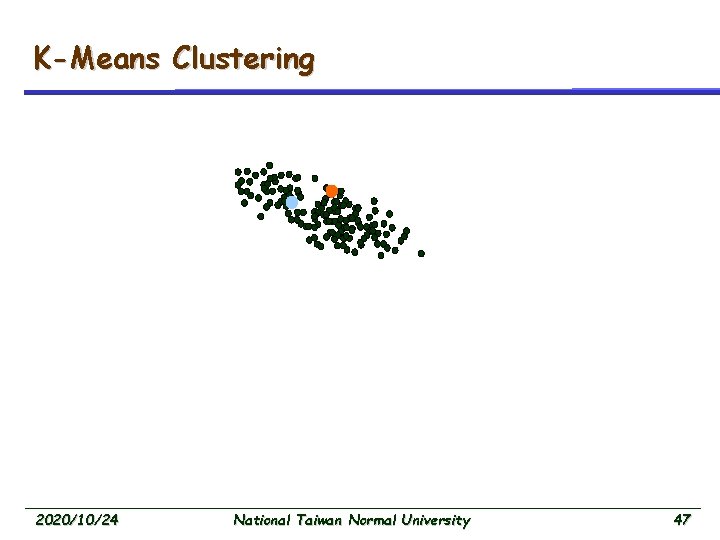
K-Means Clustering 2020/10/24 National Taiwan Normal University 47
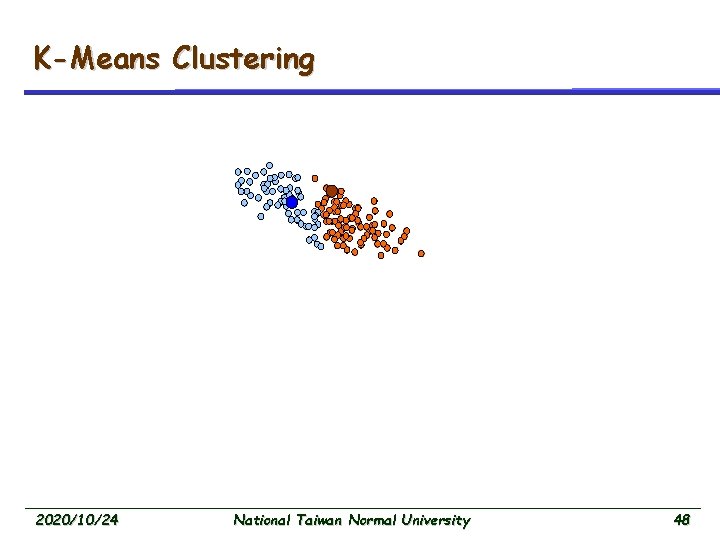
K-Means Clustering 2020/10/24 National Taiwan Normal University 48
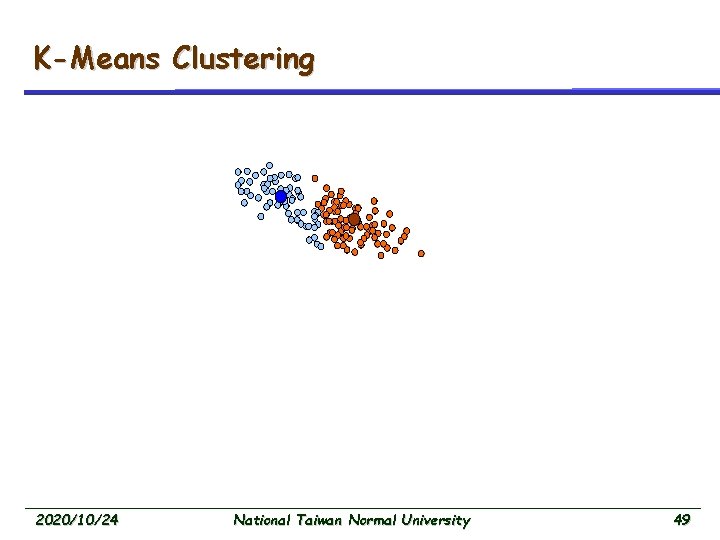
K-Means Clustering 2020/10/24 National Taiwan Normal University 49
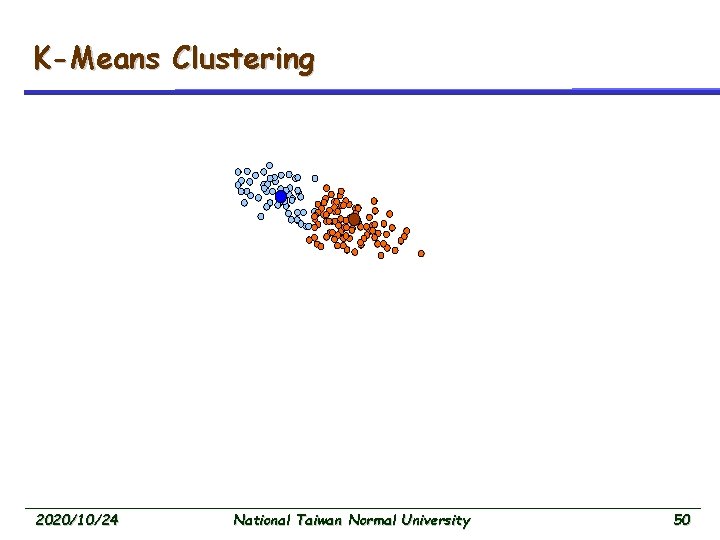
K-Means Clustering 2020/10/24 National Taiwan Normal University 50
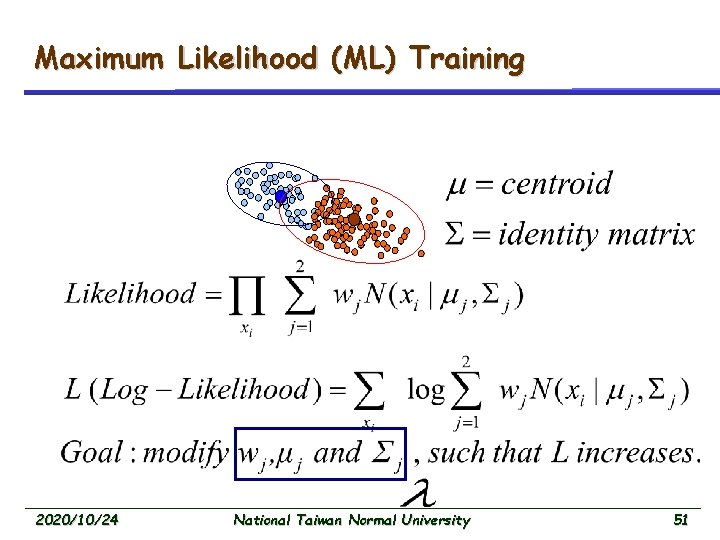
Maximum Likelihood (ML) Training 2020/10/24 National Taiwan Normal University 51
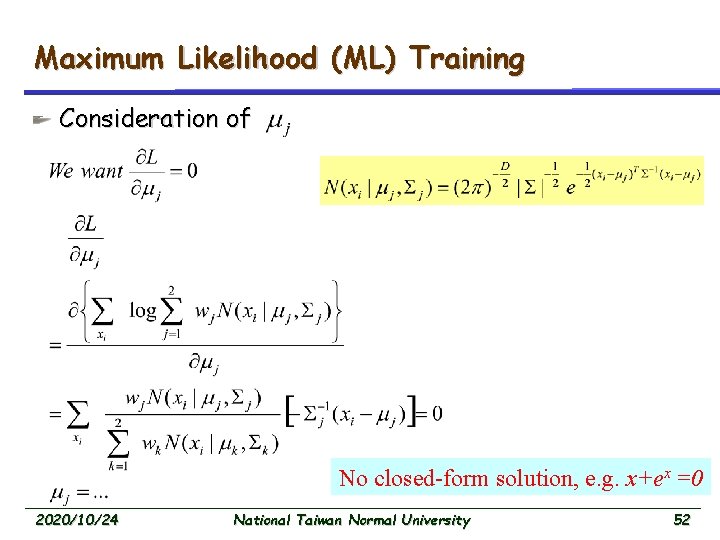
Maximum Likelihood (ML) Training Consideration of No closed-form solution, e. g. x+ex =0 2020/10/24 National Taiwan Normal University 52
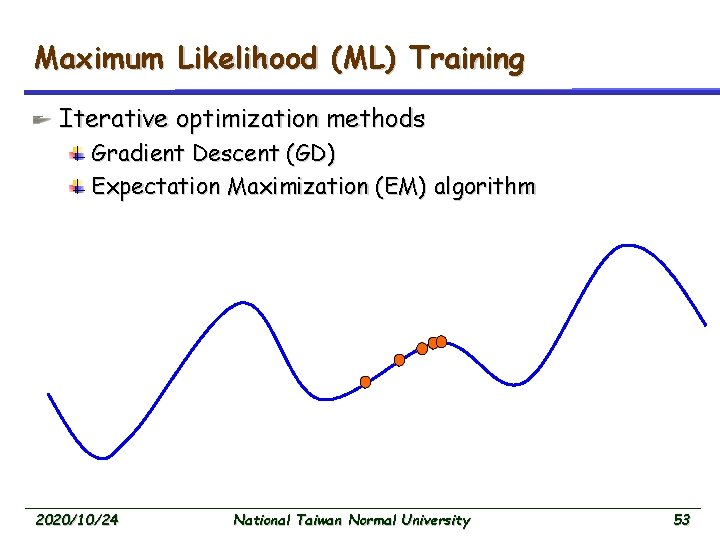
Maximum Likelihood (ML) Training Iterative optimization methods Gradient Descent (GD) Expectation Maximization (EM) algorithm 2020/10/24 National Taiwan Normal University 53
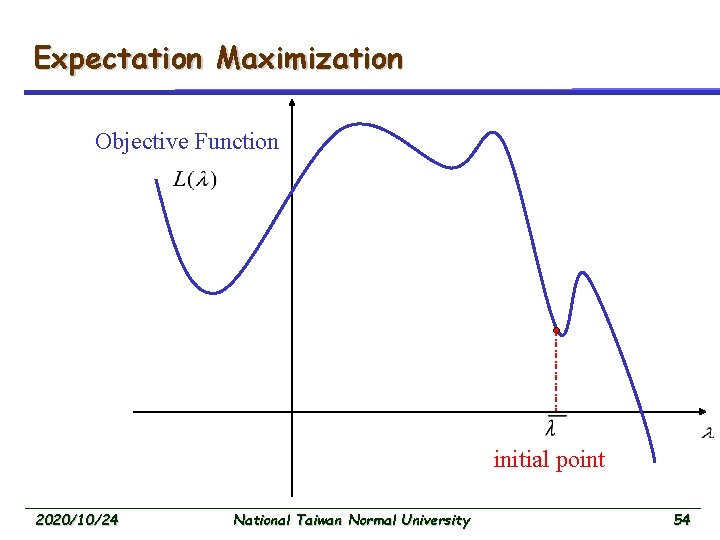
Expectation Maximization Objective Function initial point 2020/10/24 National Taiwan Normal University 54
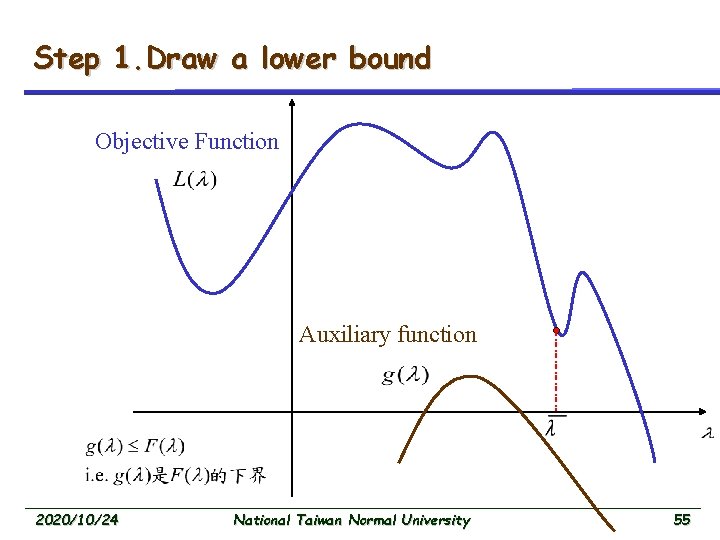
Step 1. Draw a lower bound Objective Function Auxiliary function 2020/10/24 National Taiwan Normal University 55
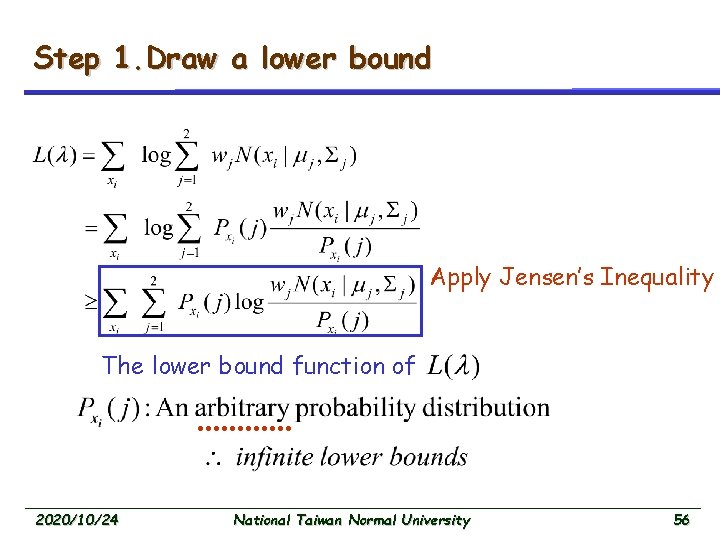
Step 1. Draw a lower bound Apply Jensen’s Inequality The lower bound function of 2020/10/24 National Taiwan Normal University 56
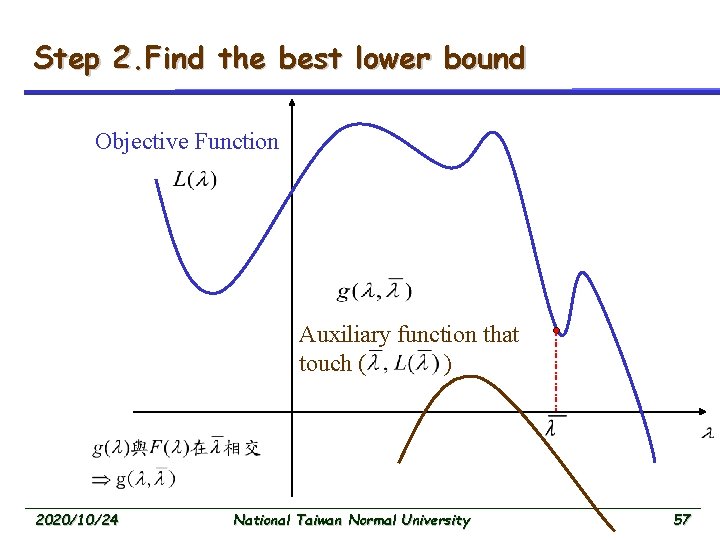
Step 2. Find the best lower bound Objective Function Auxiliary function that touch ( ) 2020/10/24 National Taiwan Normal University 57
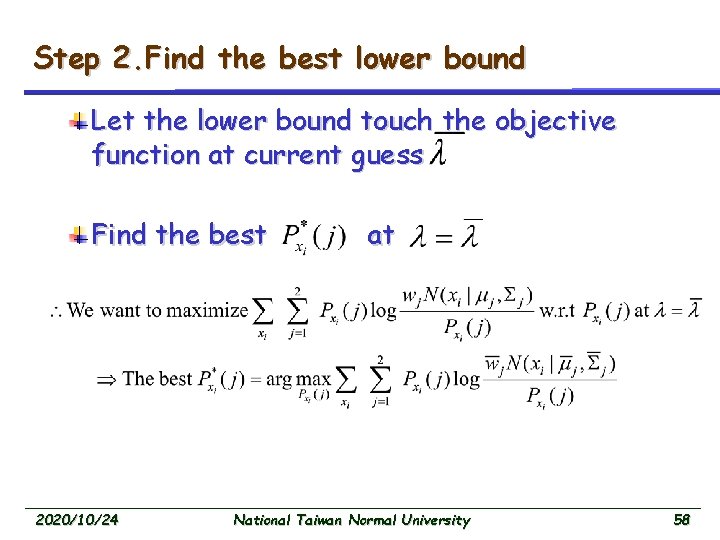
Step 2. Find the best lower bound Let the lower bound touch the objective function at current guess Find the best 2020/10/24 at National Taiwan Normal University 58
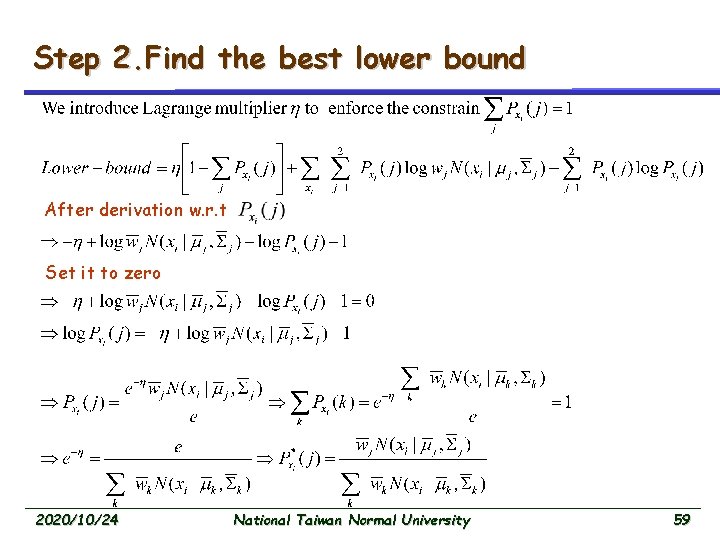
Step 2. Find the best lower bound After derivation w. r. t Set it to zero 2020/10/24 National Taiwan Normal University 59
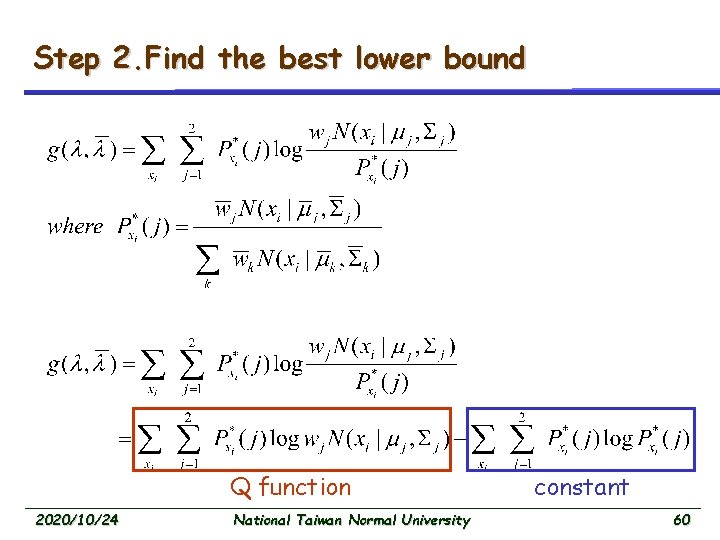
Step 2. Find the best lower bound Q function 2020/10/24 National Taiwan Normal University constant 60
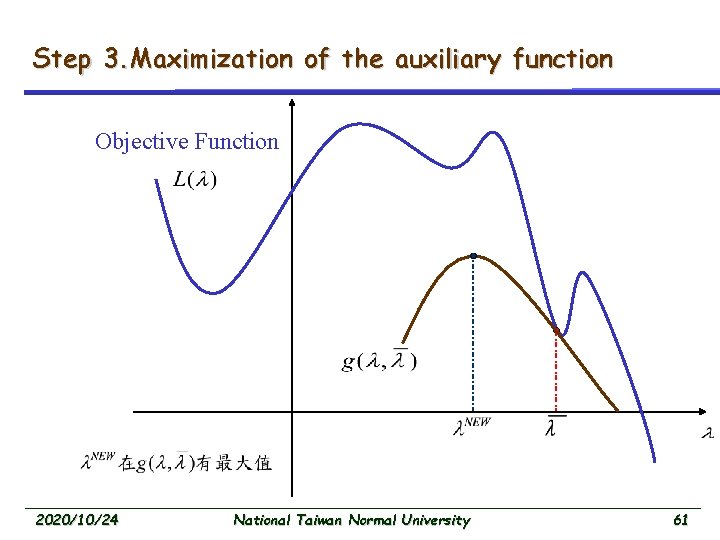
Step 3. Maximization of the auxiliary function Objective Function 2020/10/24 National Taiwan Normal University 61
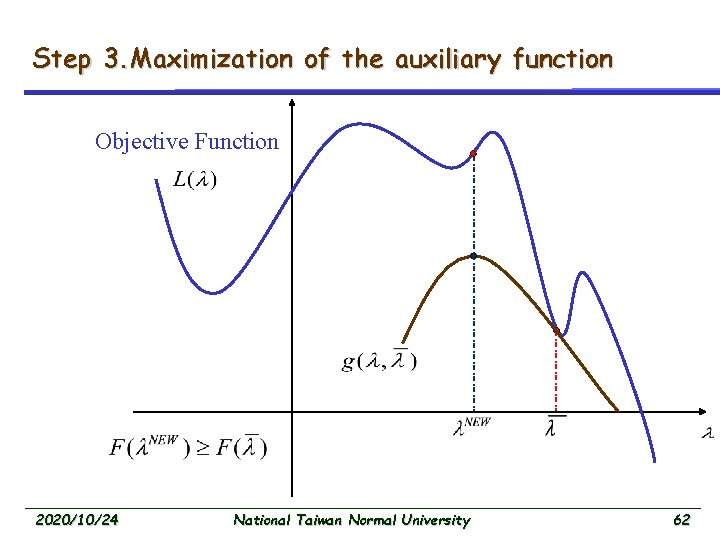
Step 3. Maximization of the auxiliary function Objective Function 2020/10/24 National Taiwan Normal University 62
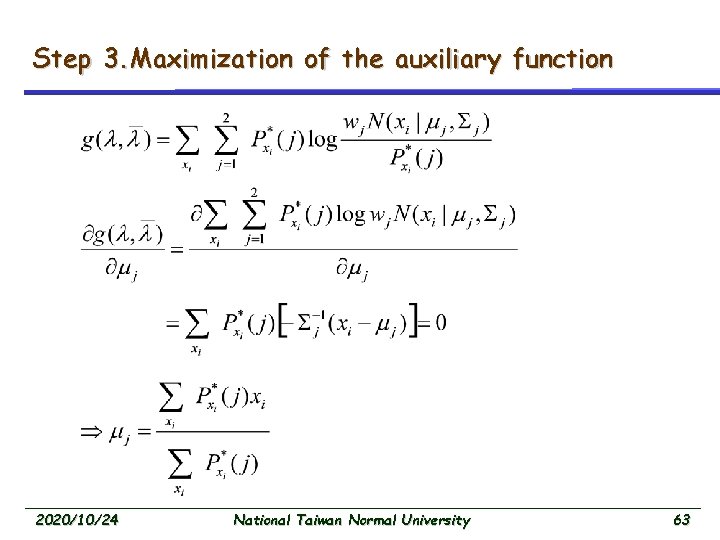
Step 3. Maximization of the auxiliary function 2020/10/24 National Taiwan Normal University 63
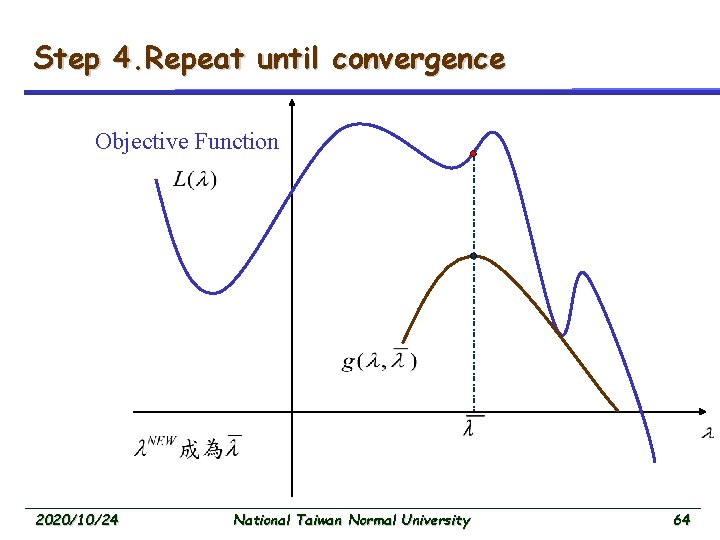
Step 4. Repeat until convergence Objective Function 2020/10/24 National Taiwan Normal University 64
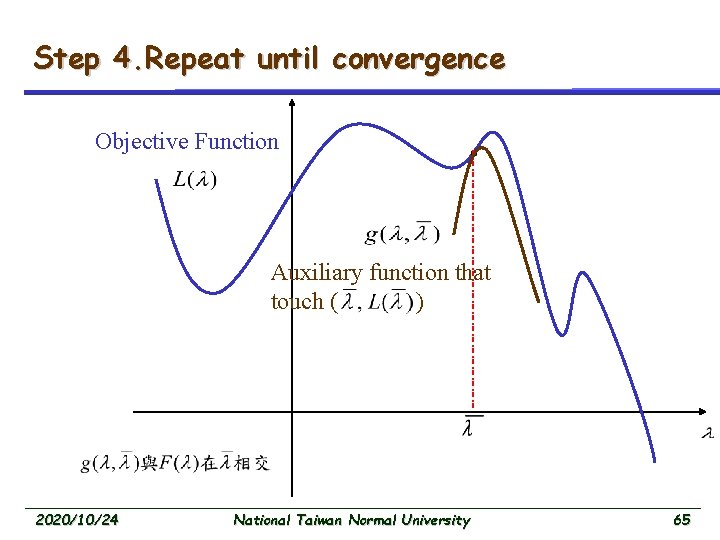
Step 4. Repeat until convergence Objective Function Auxiliary function that touch ( ) 2020/10/24 National Taiwan Normal University 65
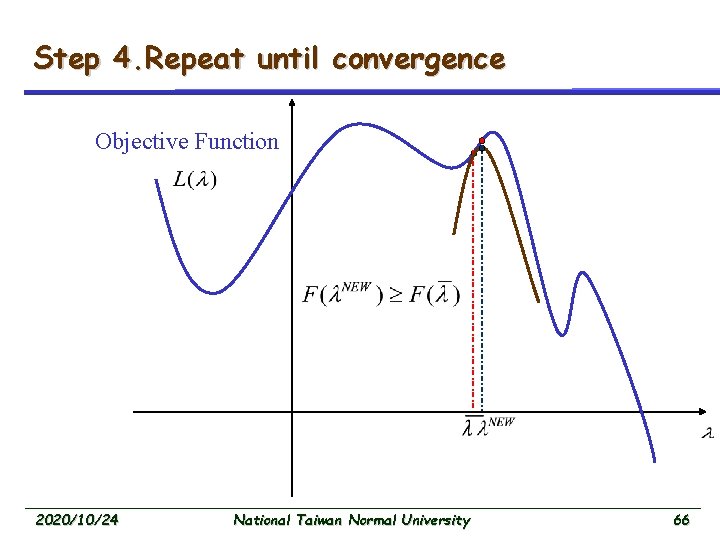
Step 4. Repeat until convergence Objective Function 2020/10/24 National Taiwan Normal University 66
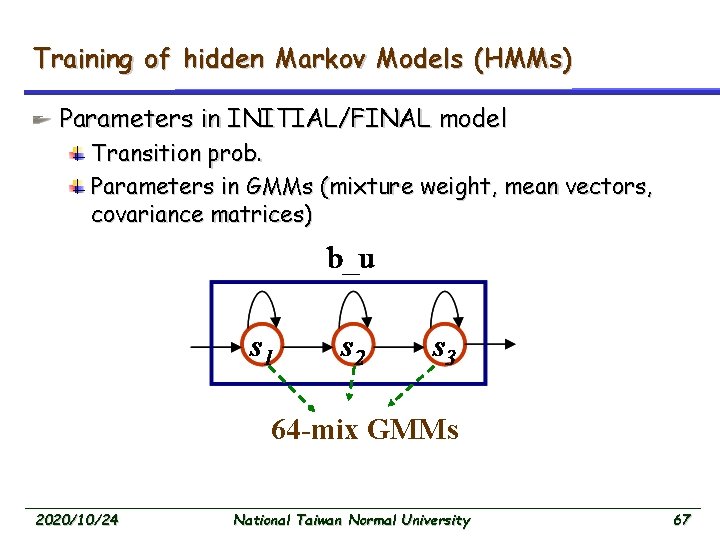
Training of hidden Markov Models (HMMs) Parameters in INITIAL/FINAL model Transition prob. Parameters in GMMs (mixture weight, mean vectors, covariance matrices) 64 -mix GMMs 2020/10/24 National Taiwan Normal University 67
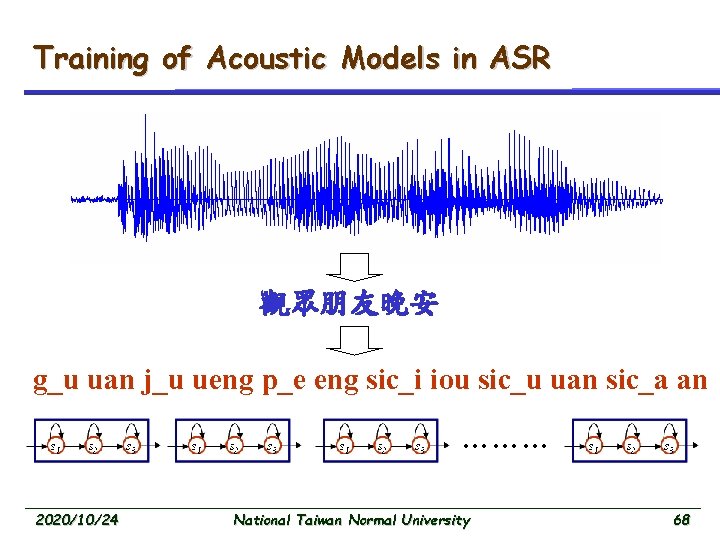
Training of Acoustic Models in ASR 觀眾朋友晚安 g_u uan j_u ueng p_e eng sic_i iou sic_u uan sic_a an ……… 2020/10/24 National Taiwan Normal University 68
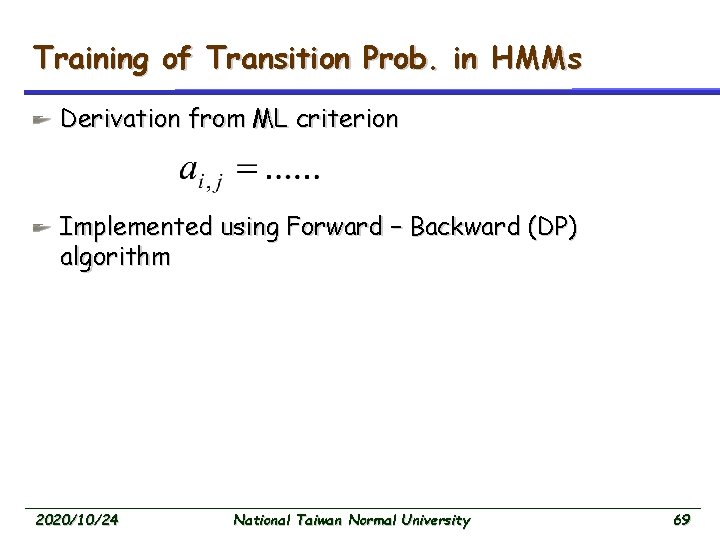
Training of Transition Prob. in HMMs Derivation from ML criterion Implemented using Forward – Backward (DP) algorithm 2020/10/24 National Taiwan Normal University 69
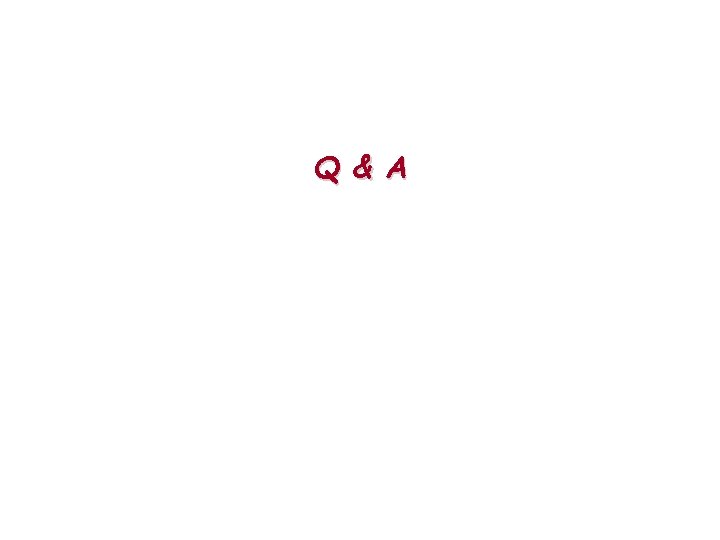
Q & A
First author second author third author
Automatic defect recognition
Automatic target recognition
Kinect for windows speech recognition language pack
Fundamentals of speech recognition
Deep learning speech recognition
Ionic speech recognition
Julia speech recognition
Melspectrum
How a dyslexic person sees text
Cmu speech recognition
Speech recognition
Speech recognition app inventor
Dragon speech recognition
Electron speech recognition
Htk speech recognition tutorial
Vyr737
Night of the scorpion author introduction
Reported speech already
Pure speech vs symbolic speech
Speech to the young gwendolyn brooks
Quoted speech vs reported speech
Informative speech vs persuasive speech
Report present simple
Reported speech suggestions
Direct and indirect speech wh questions
Sentence from direct to indirect speech
Speech to the young poem
How many kinds of sentence
Indirect speech present simple
Reported speech
Direct speech
Informative vs persuasive speech
Before indirect speech
Direct speech into reported speech
Philip said i was playing football in reported speech
Narration exercise
Reported speech and quoted speech
Direct speach
Maintenance de pipette
Octopus deploy demo
Autonomous vs automatic bladder
Automatic pet feeder project report
Automatic input devices
Randoop
Automatic reinforcement aba example
Private automatic branch exchange
Office automatic system
Automatic transmission troubleshooting chart
Transfer pipette definition
Which of the following is machine independent language
Automatic library search
Automatic library search in system software
History of automatic control
A source monitoring error
Disadvantages of input devices
Affordable fleet automatic vehicle tracking
Komponen utama automatic transmission
Portrait matting
Automatic thoughts examples
Automatic thought record
Adf direction finder
Three-term contingency example
Types of autoanalyzer
Automatic wrappers for large scale web extraction
Automatic vehicle locator system
Automatic positive airway pressure
Automatic generation control block diagram
Automatic data capture devices
What is counter and looping
Automatic control