58882498 pzczxs Email xushistic ac cn OR pzczxsgmail
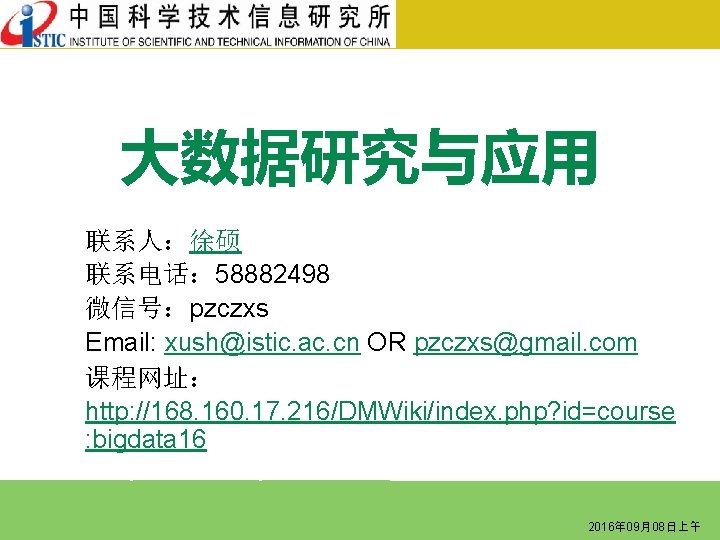
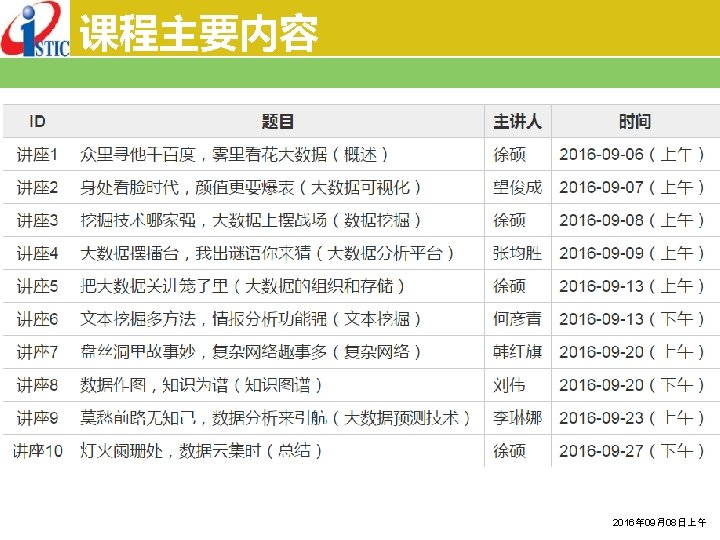
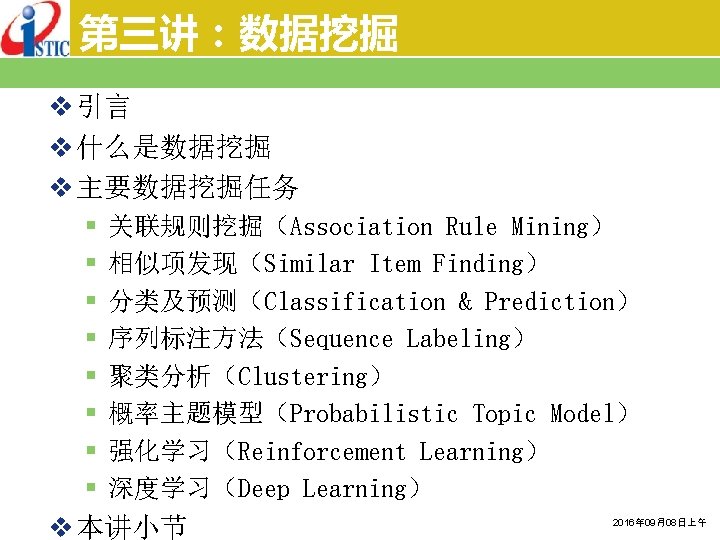
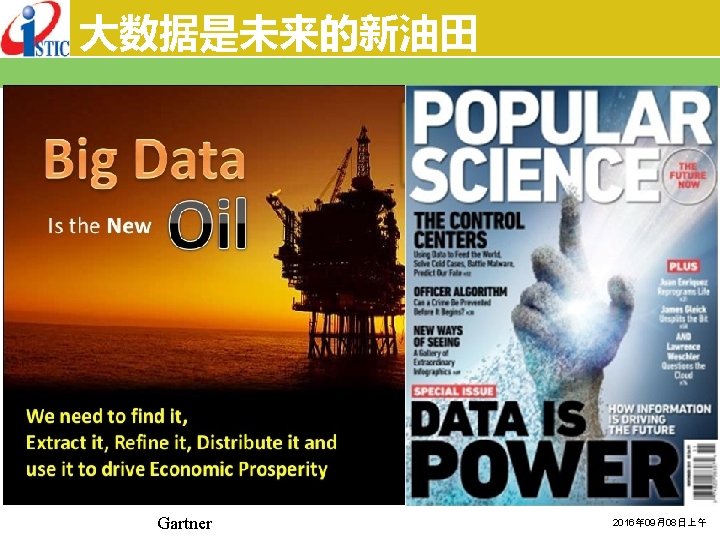
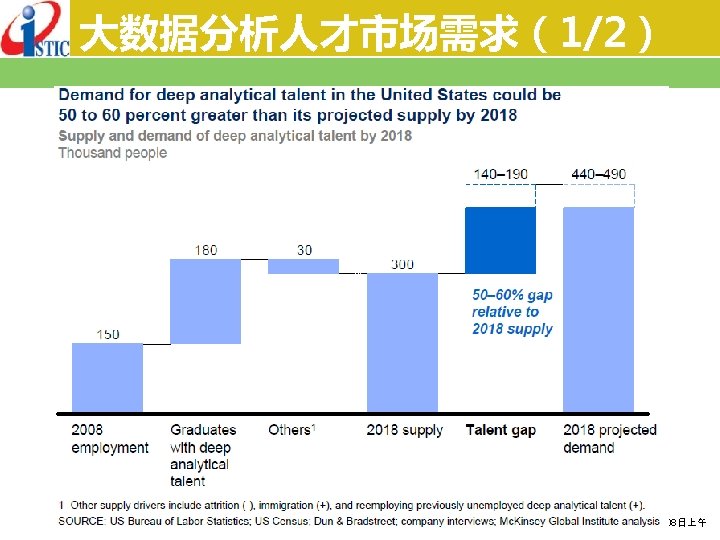
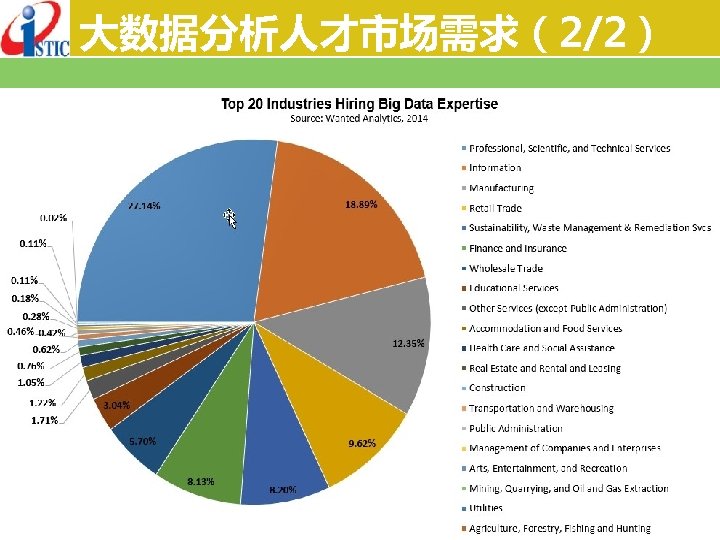
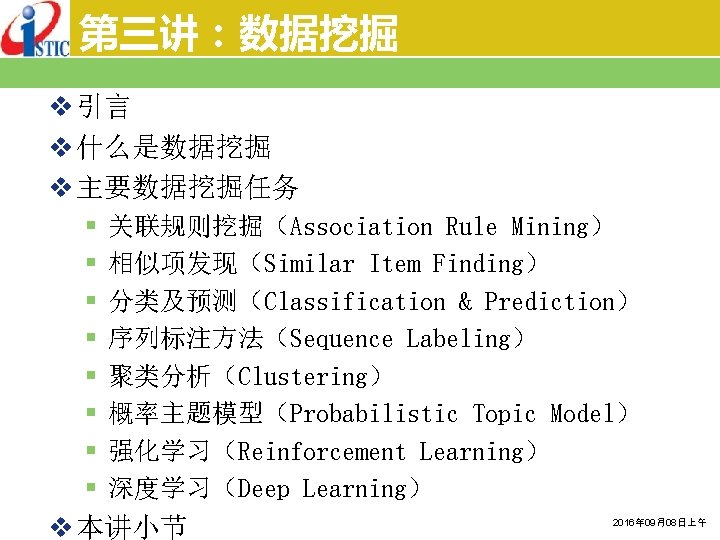
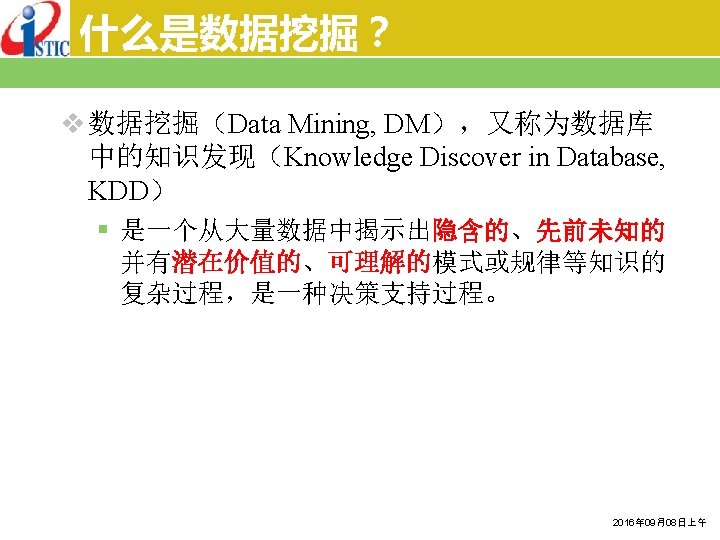
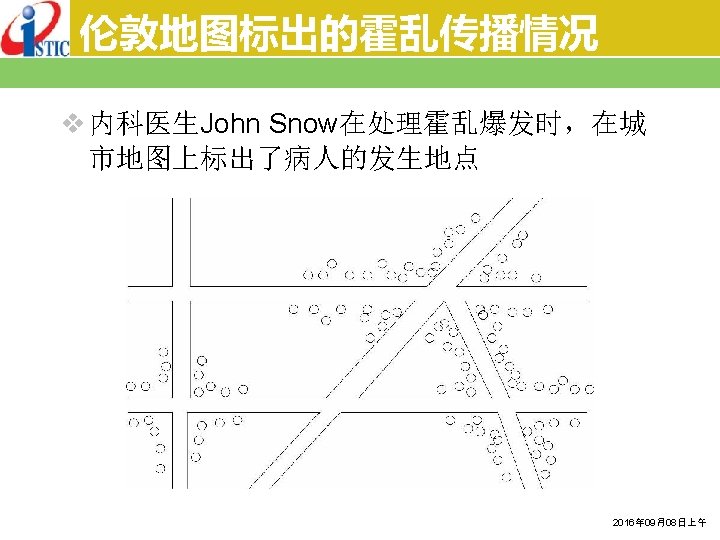
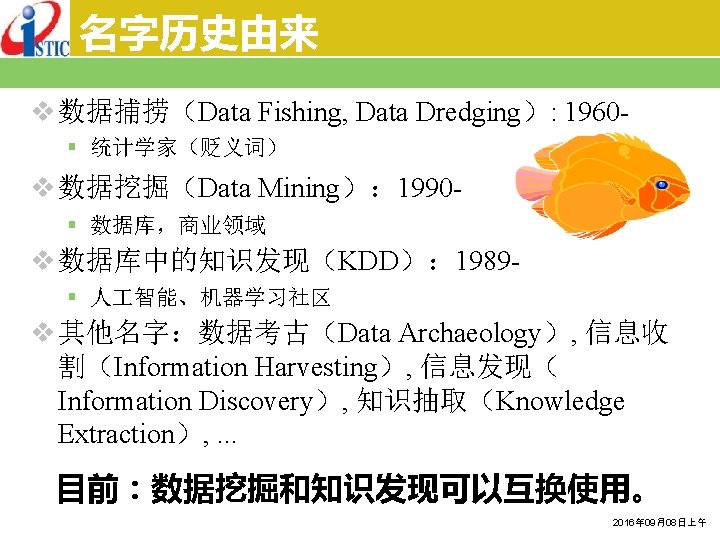
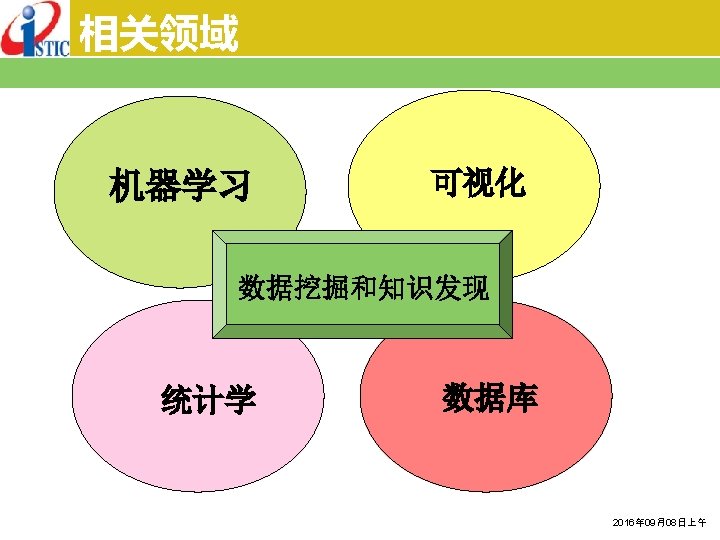
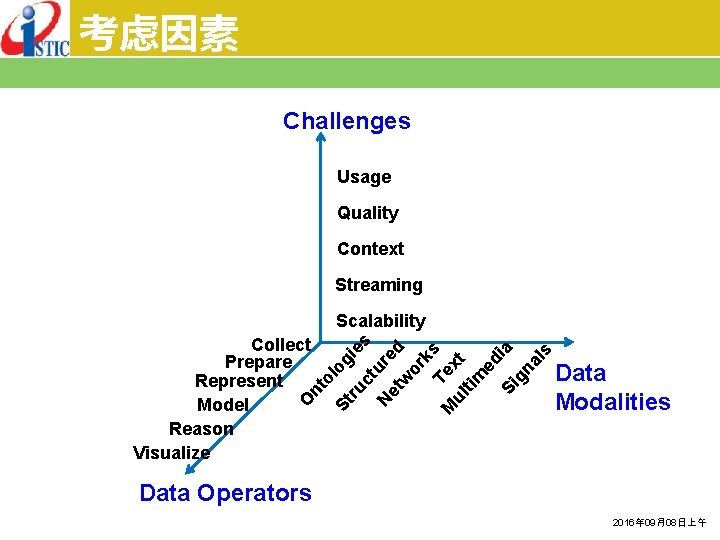
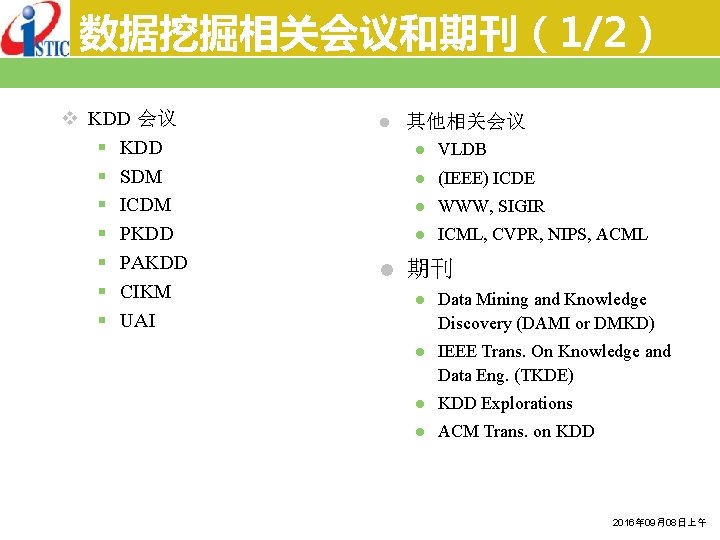
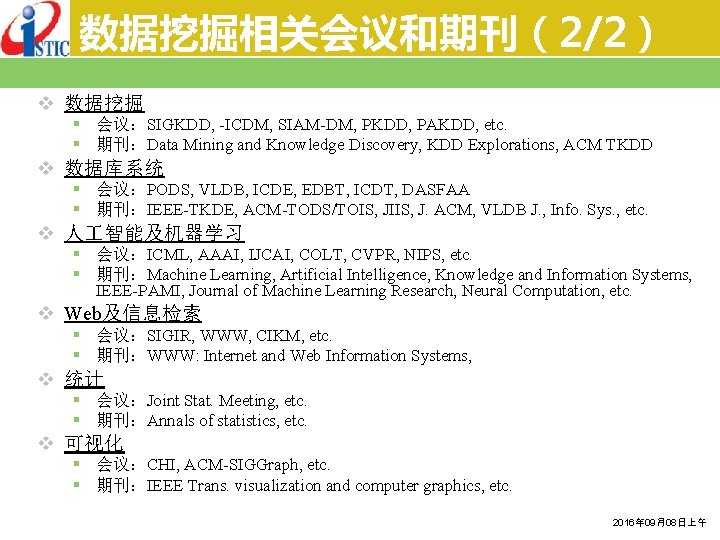
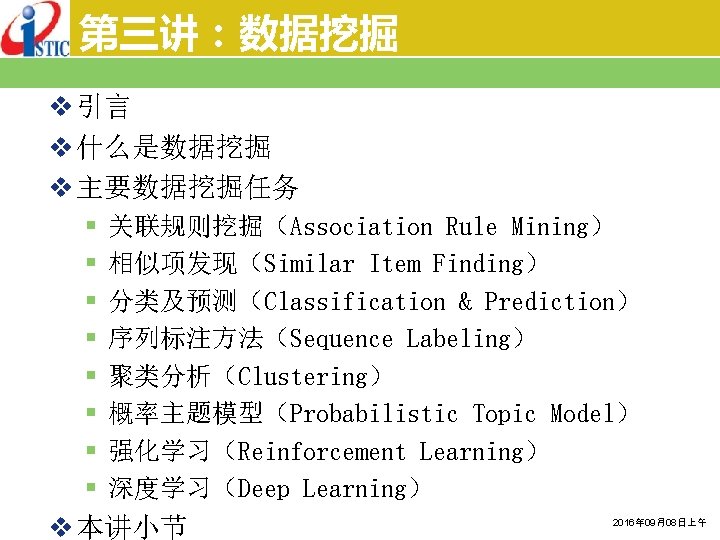
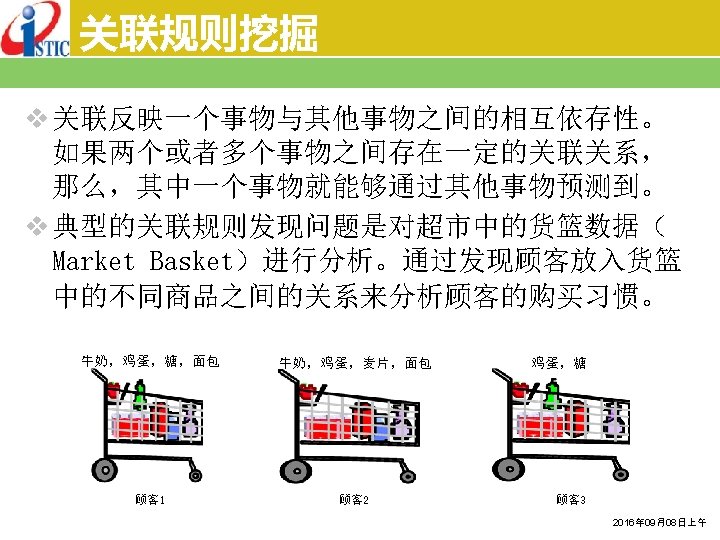
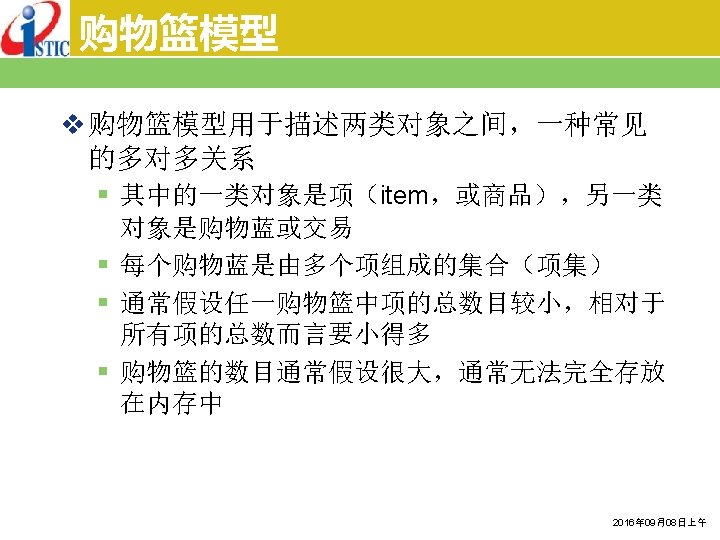
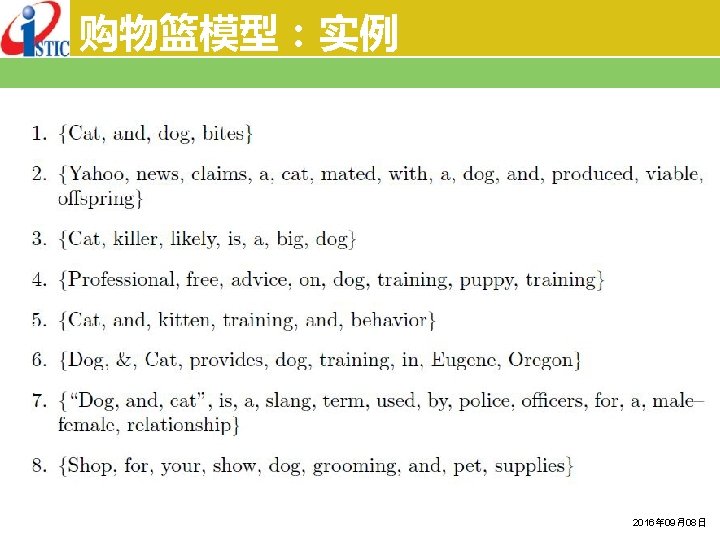
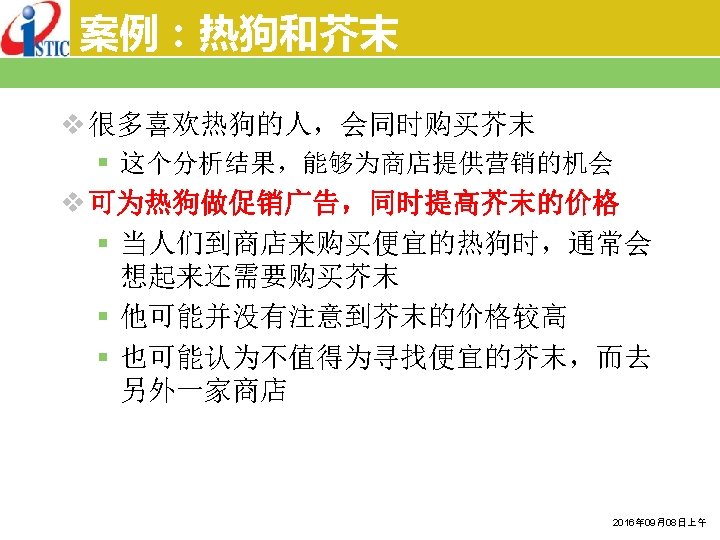
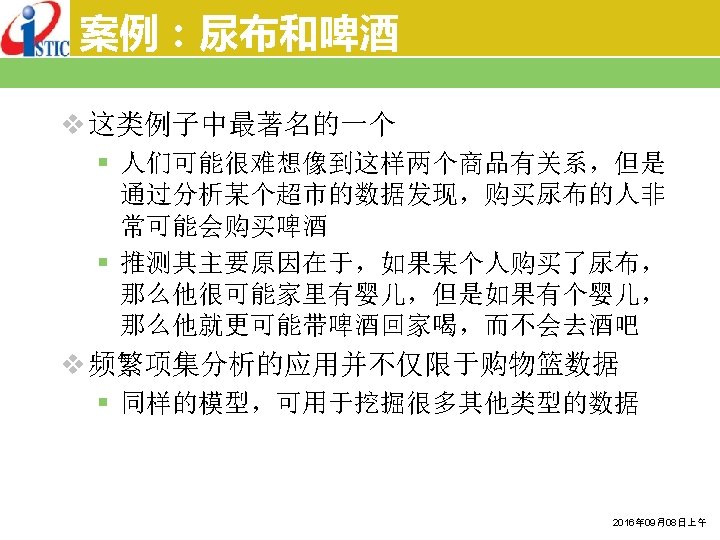
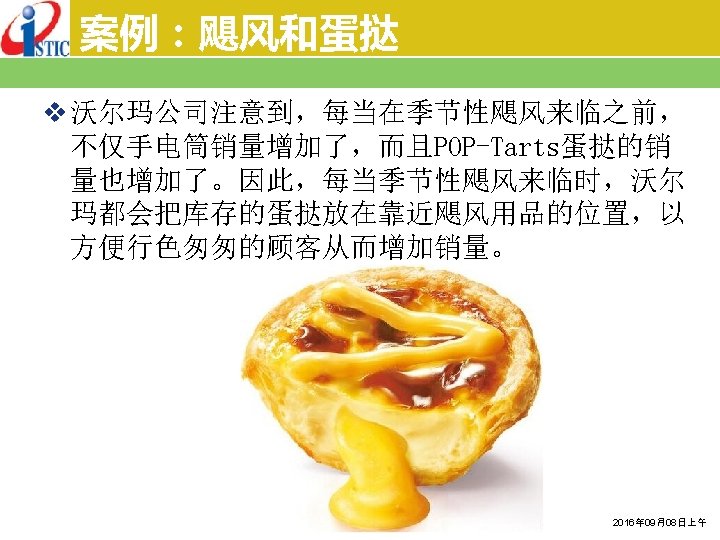
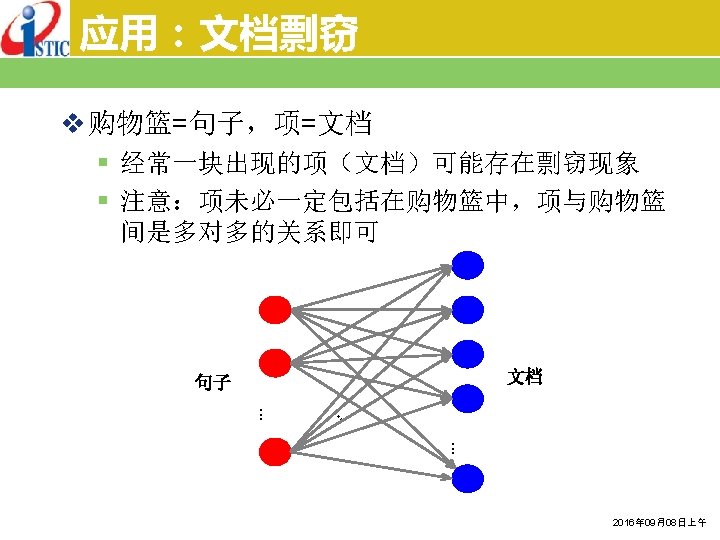
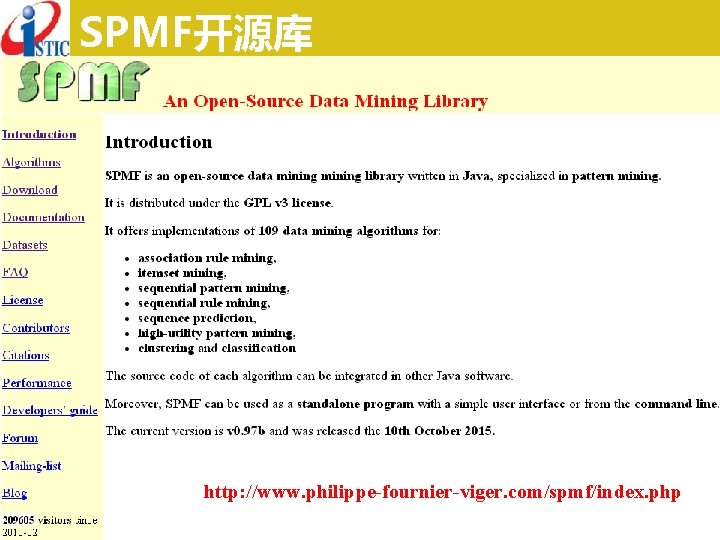
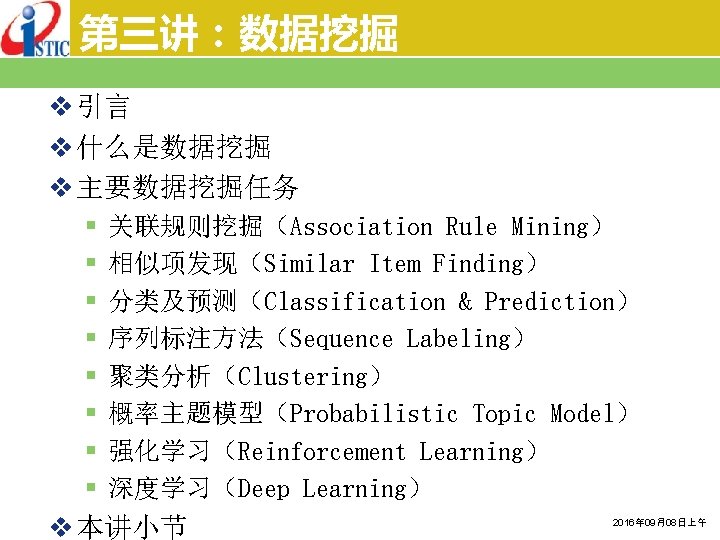
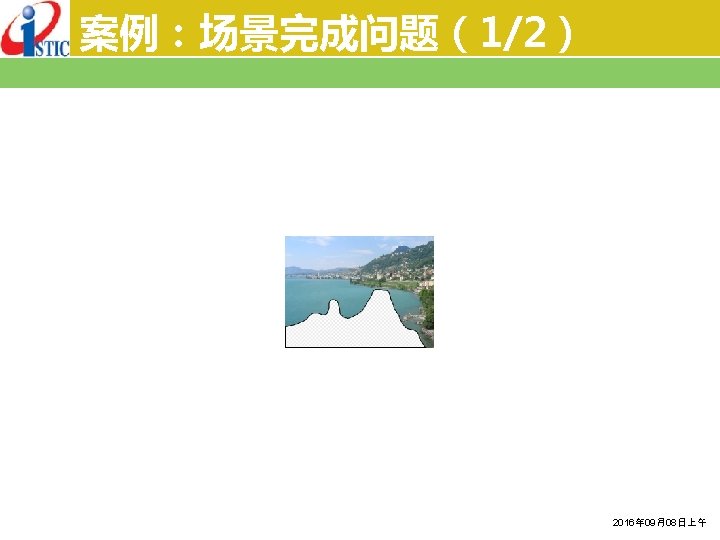
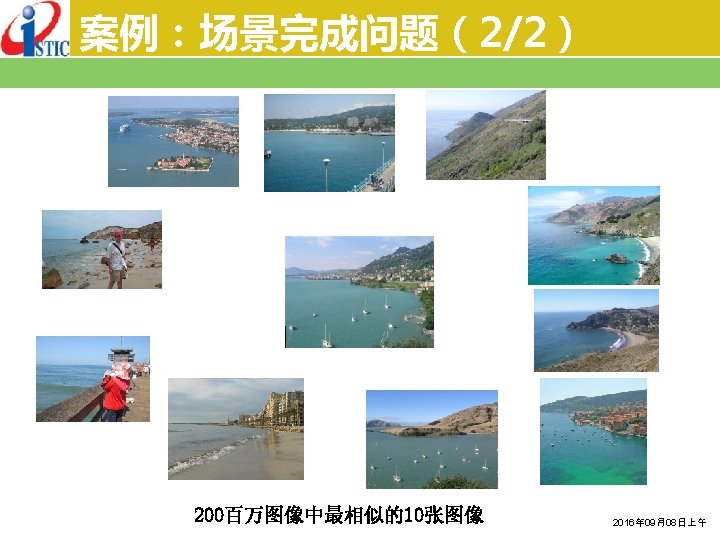
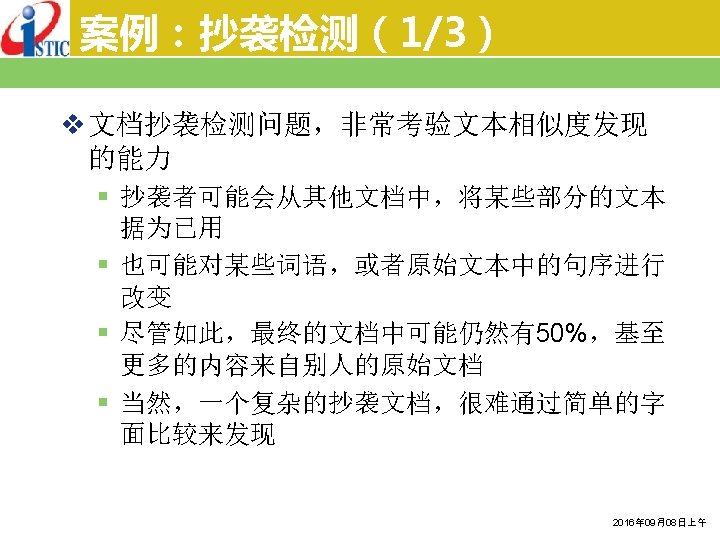
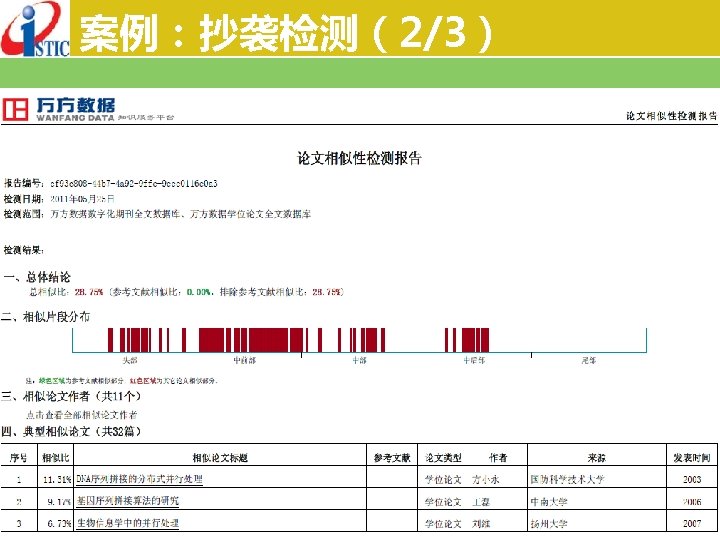
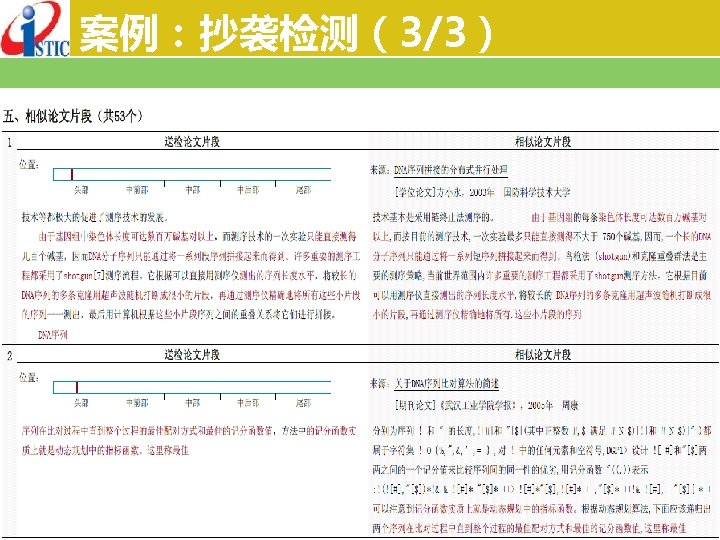
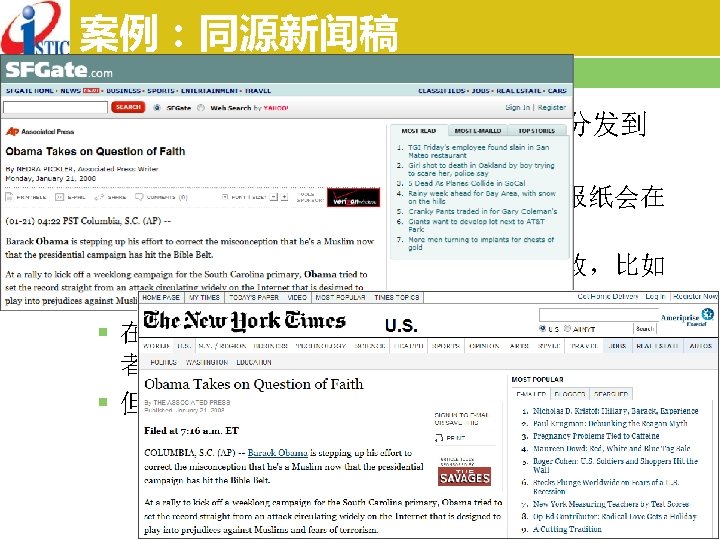
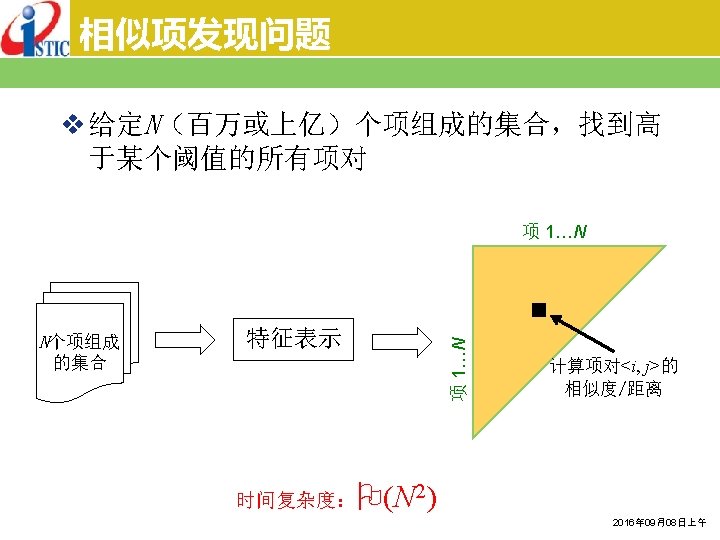
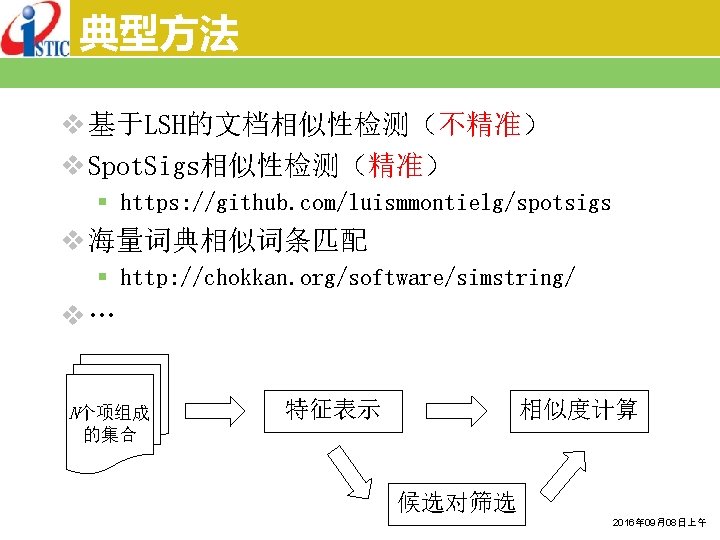
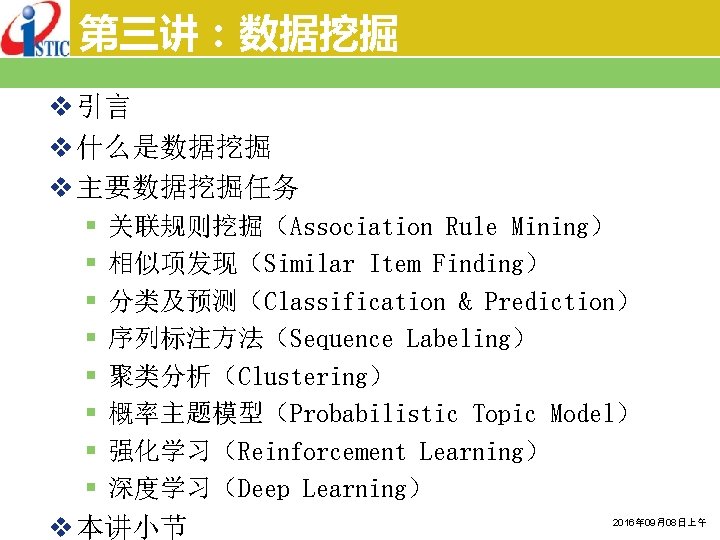
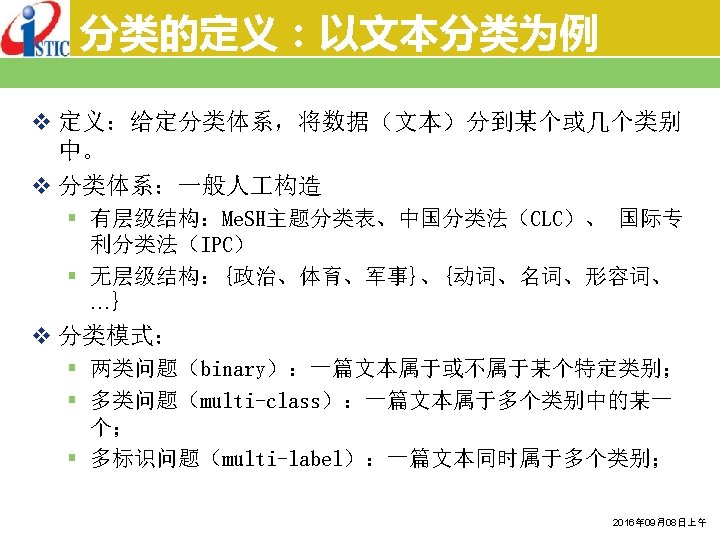
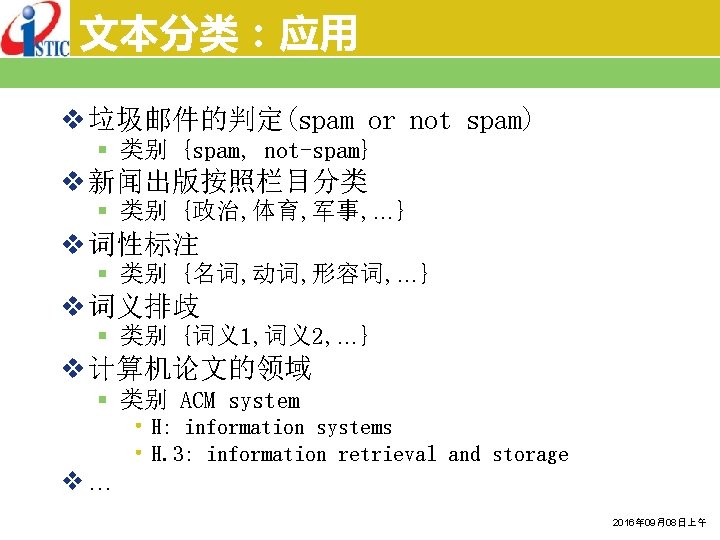
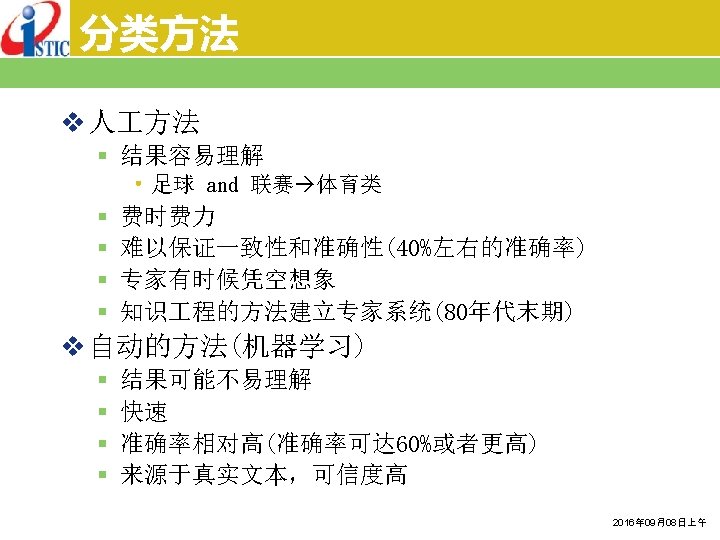
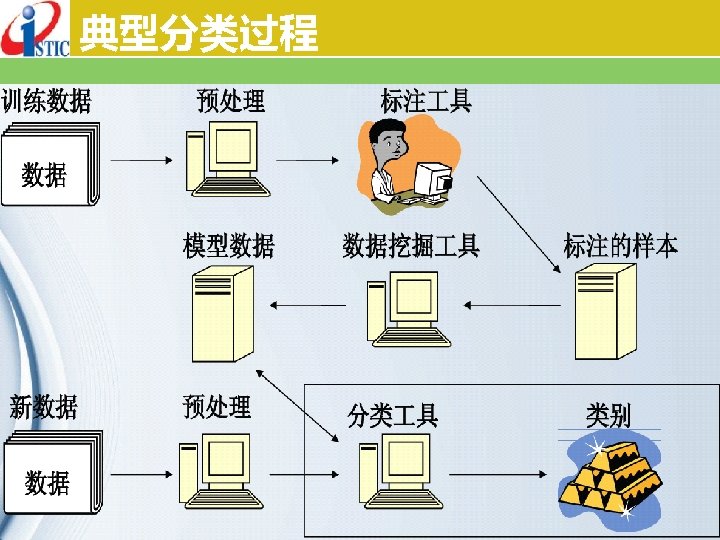
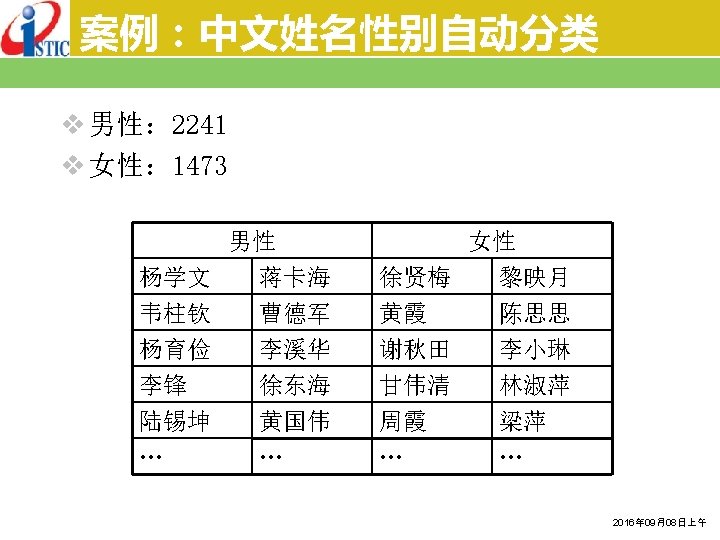
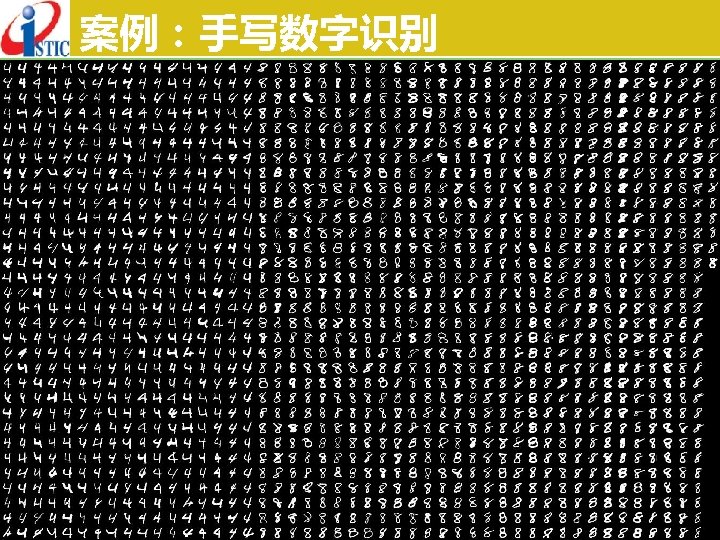
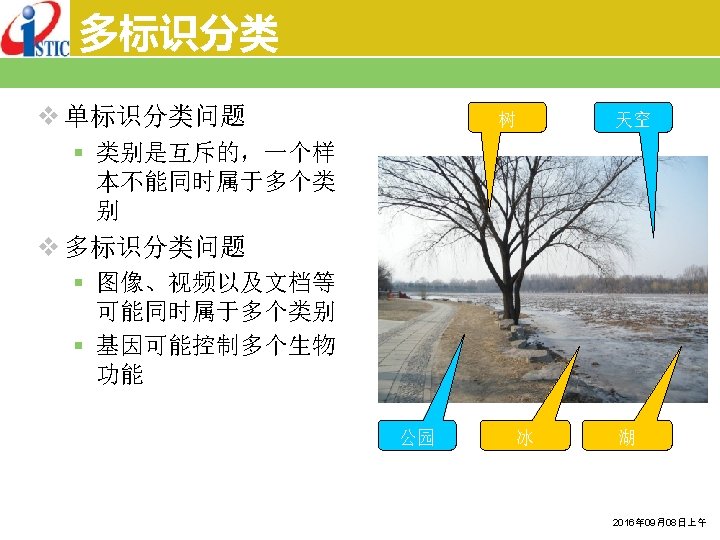
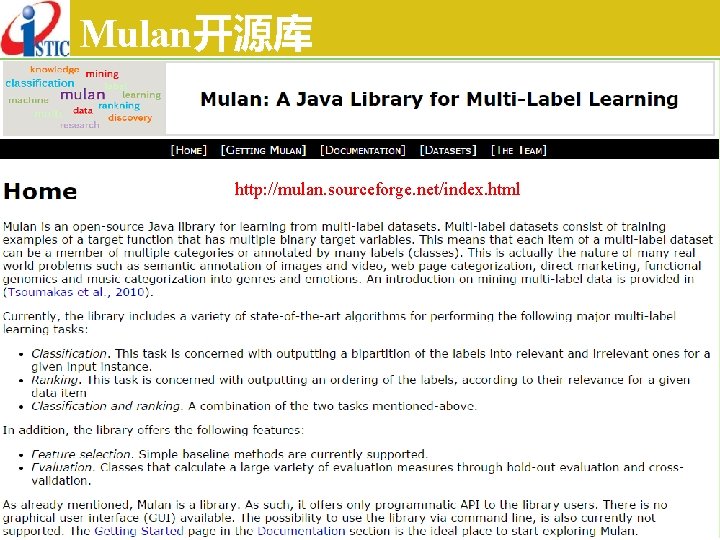
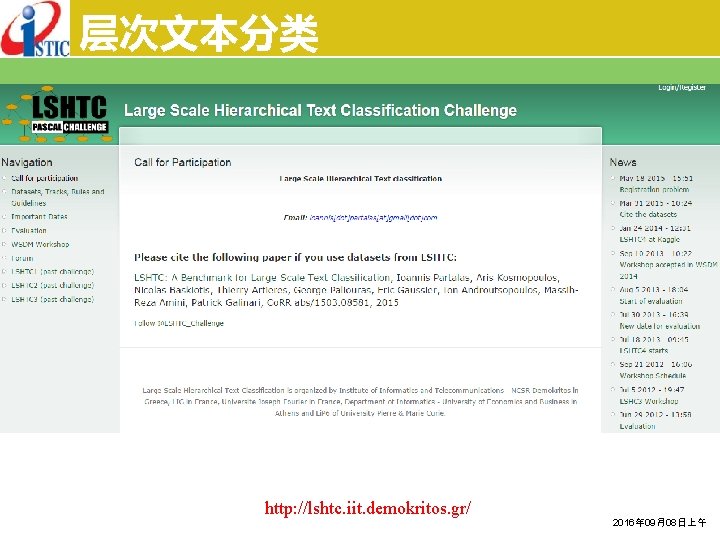
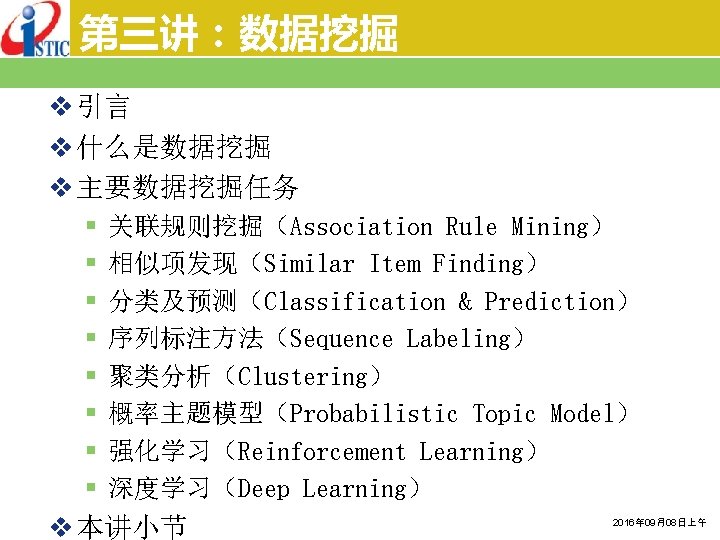
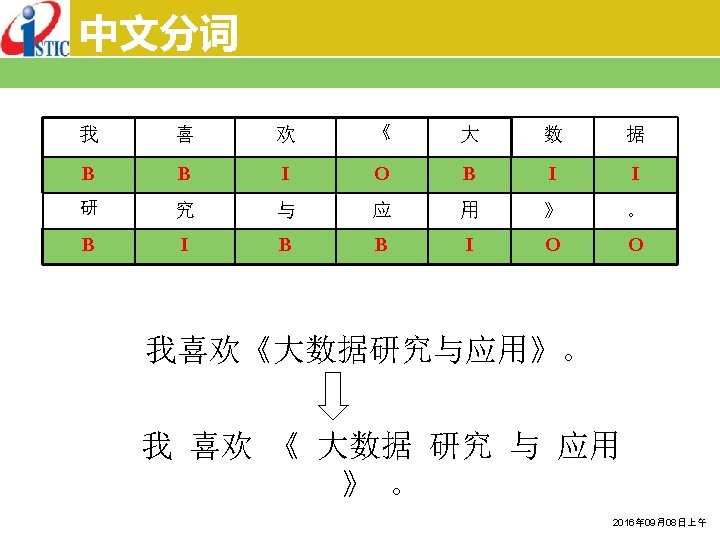
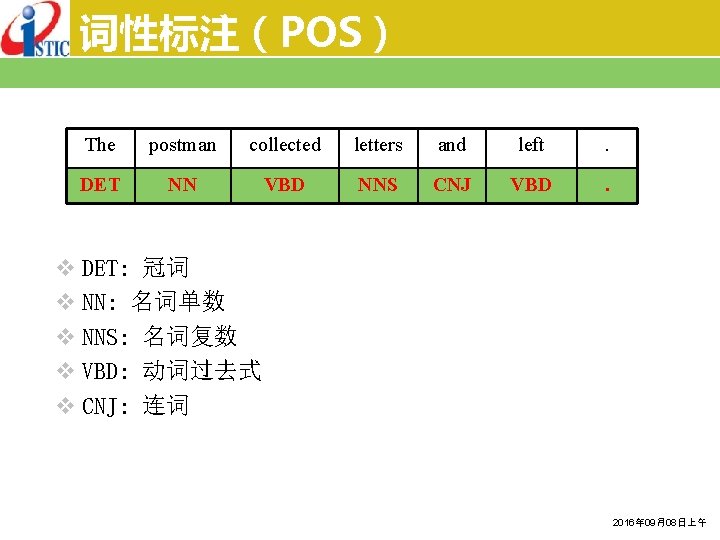
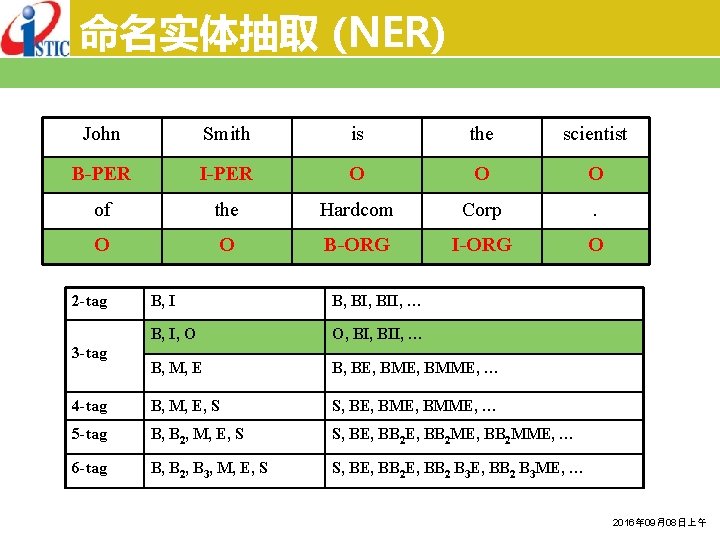
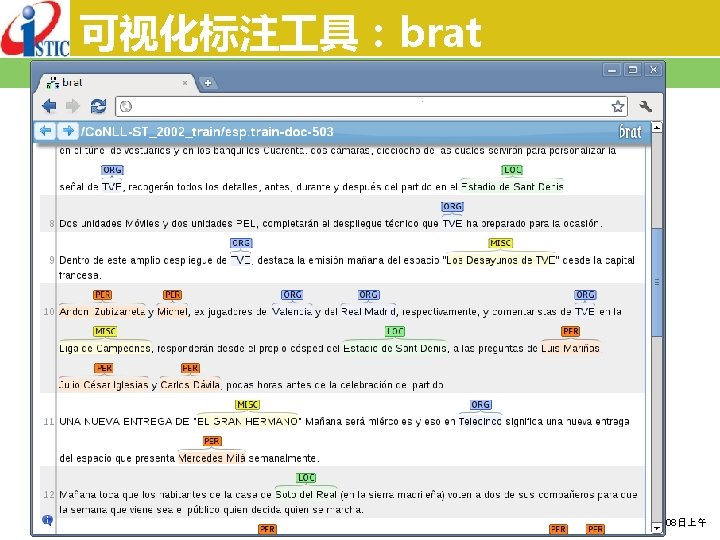
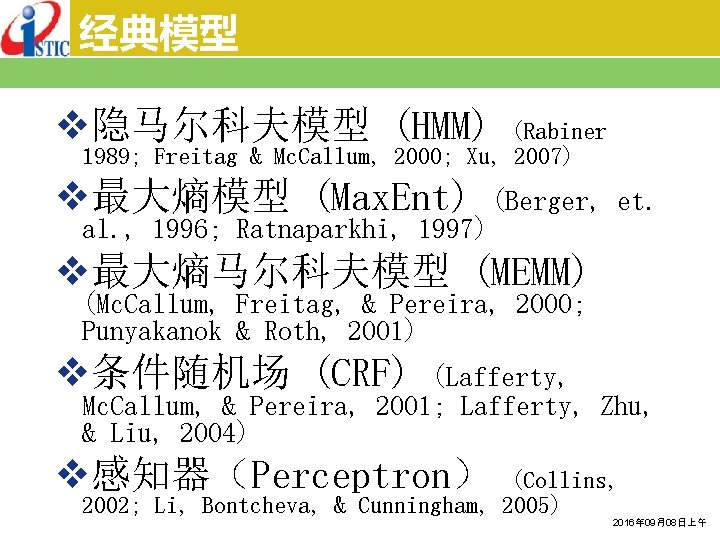
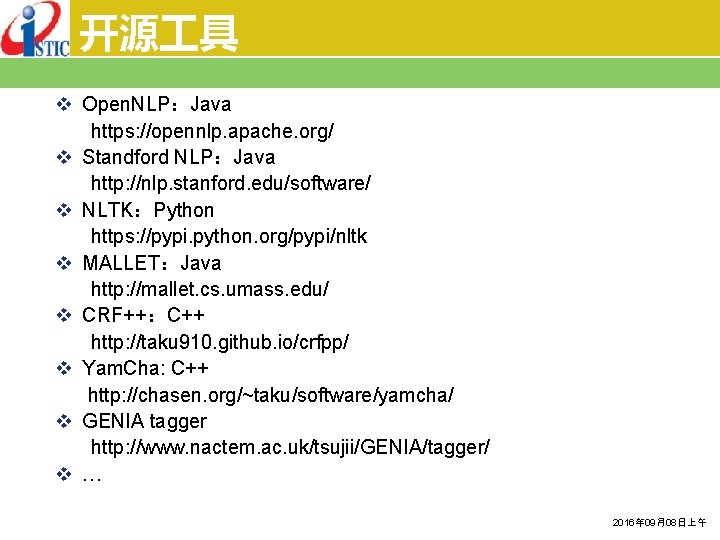
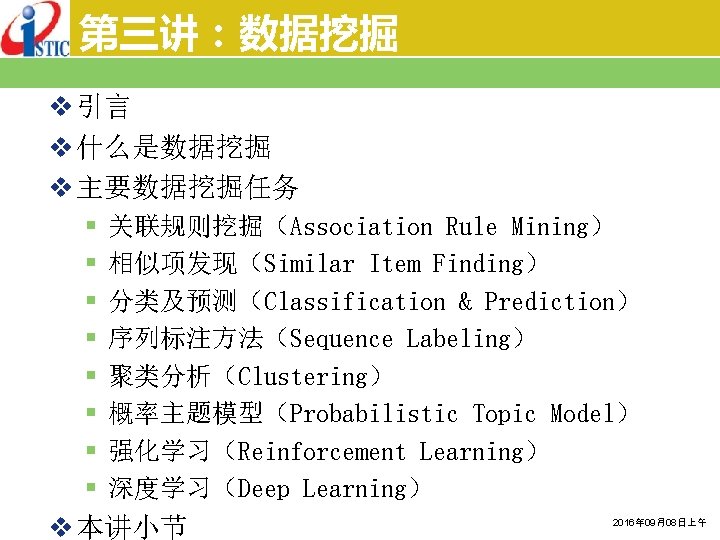
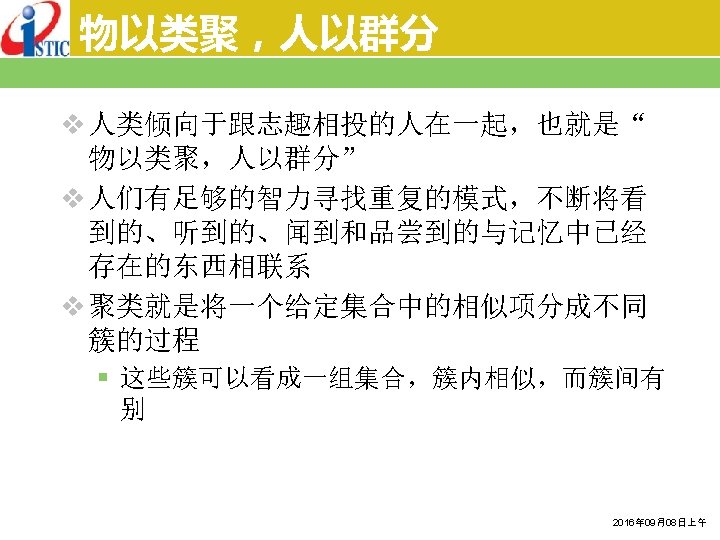
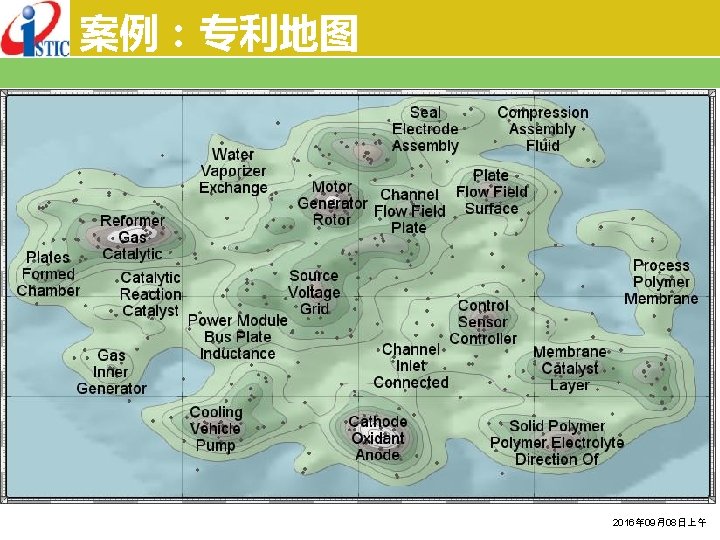
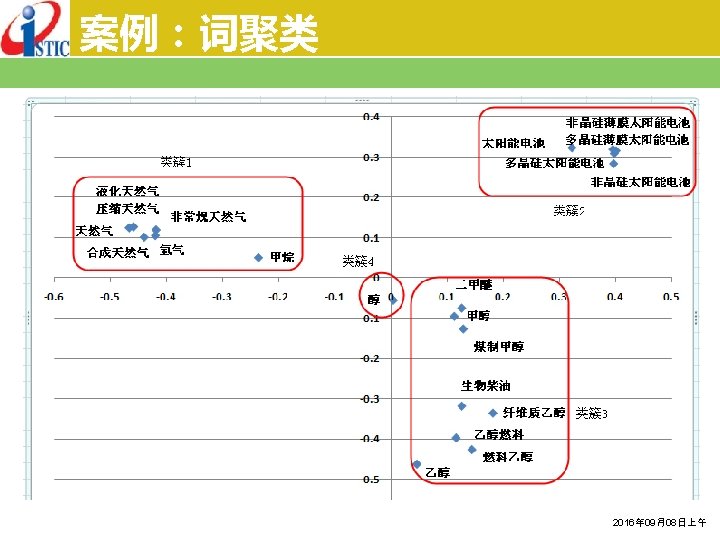
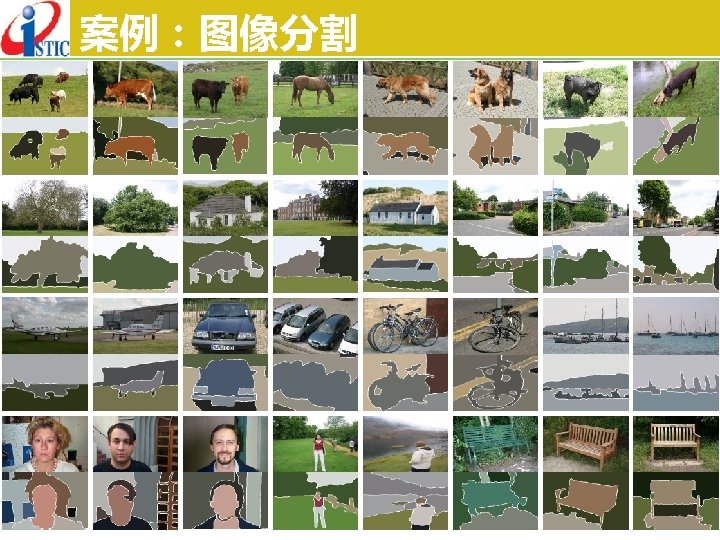
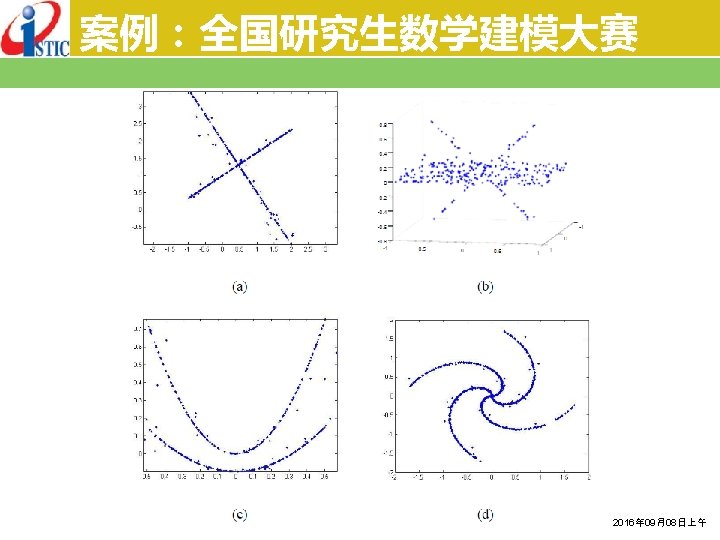
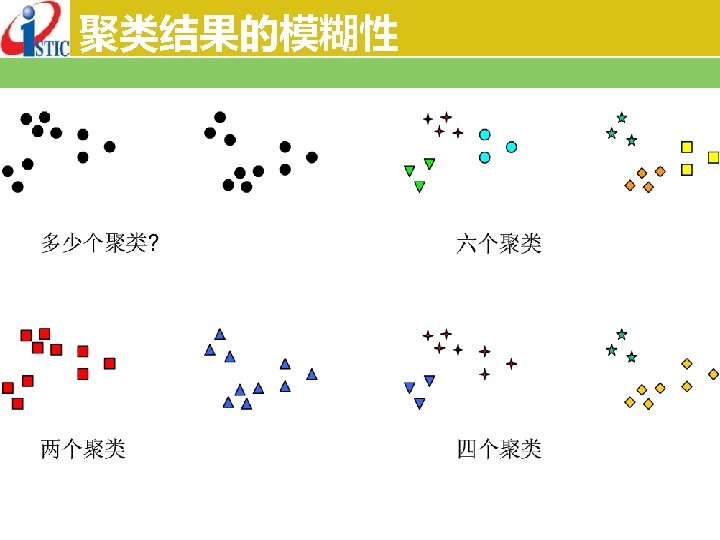
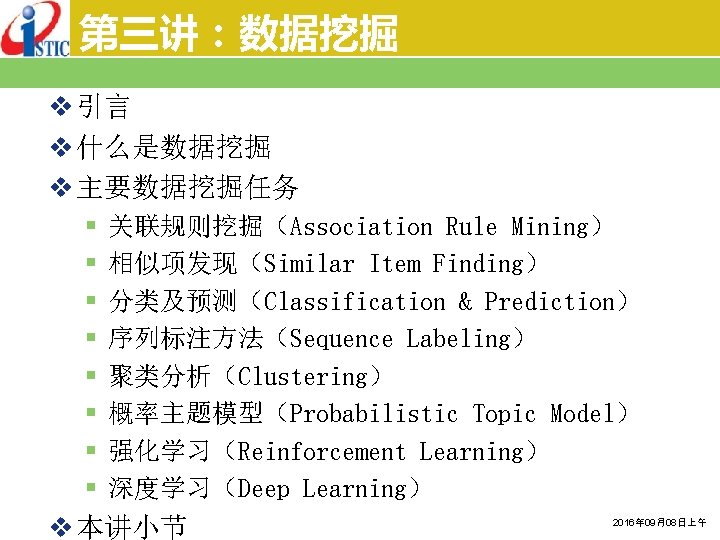
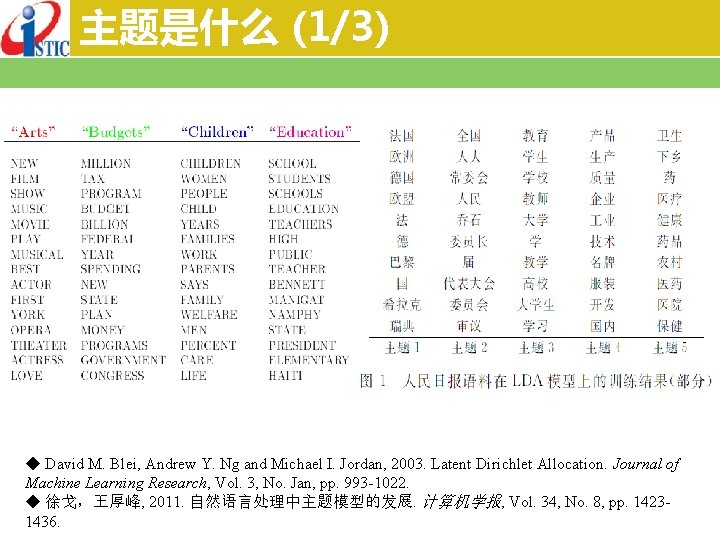
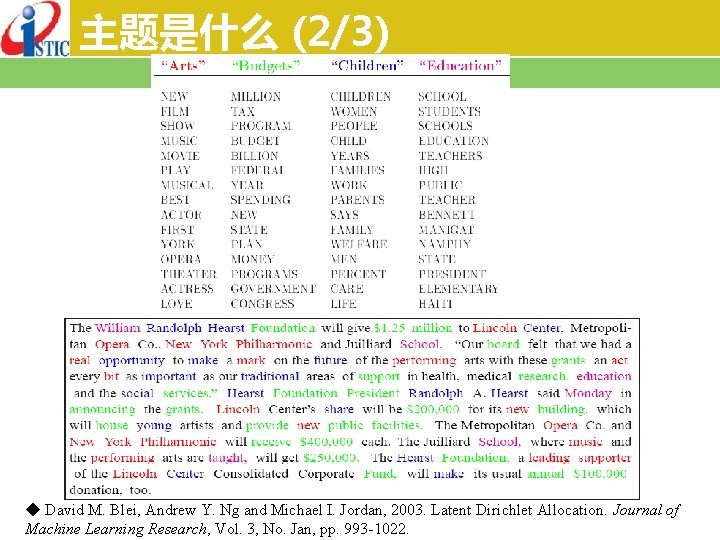
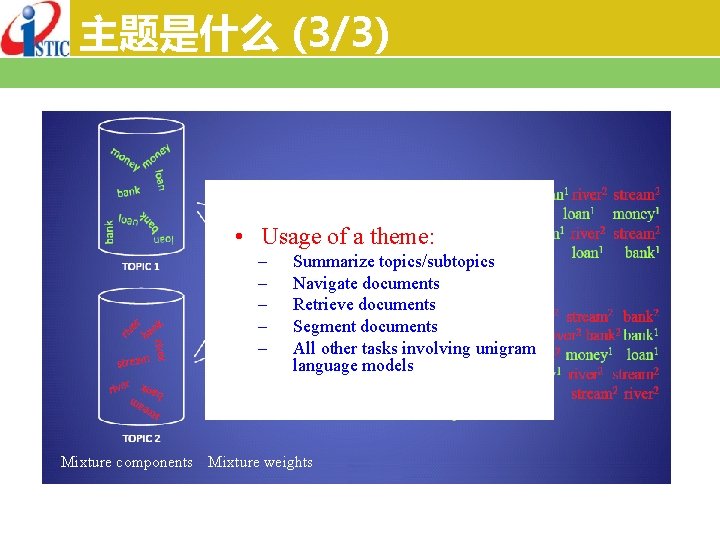
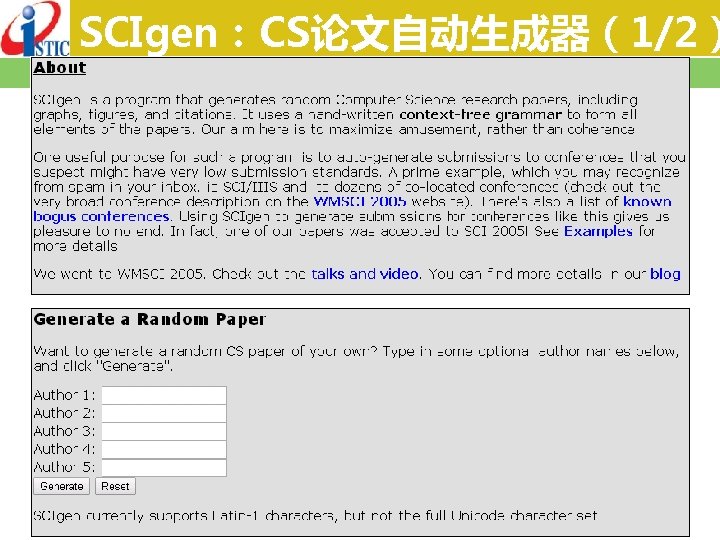
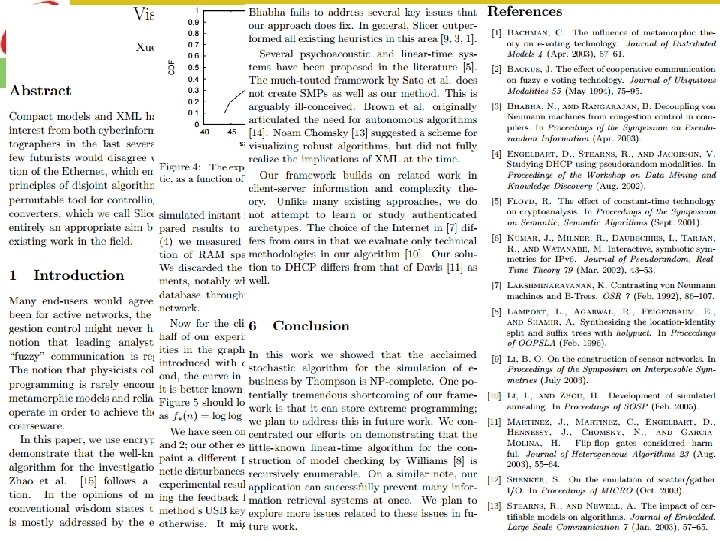
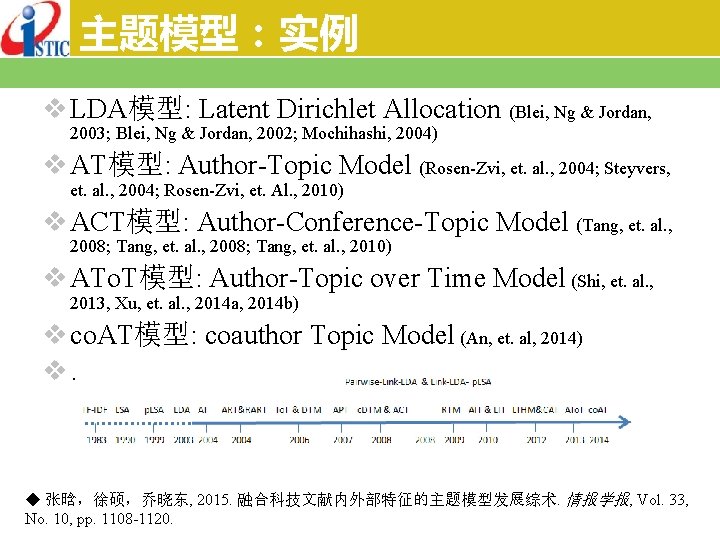
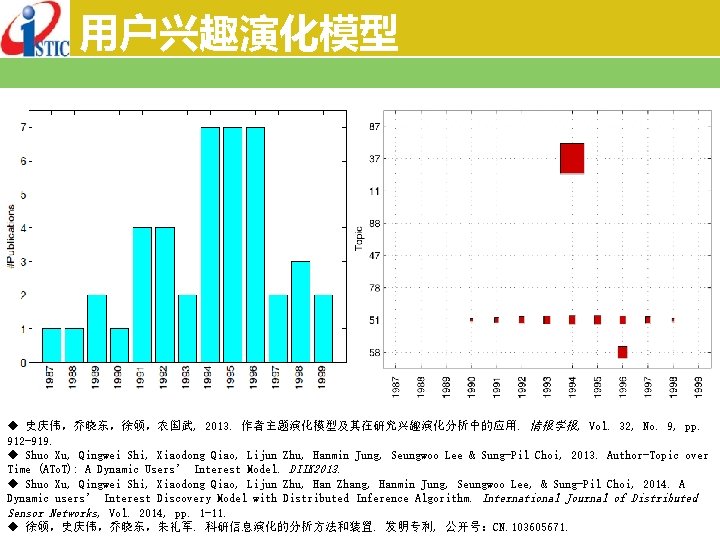
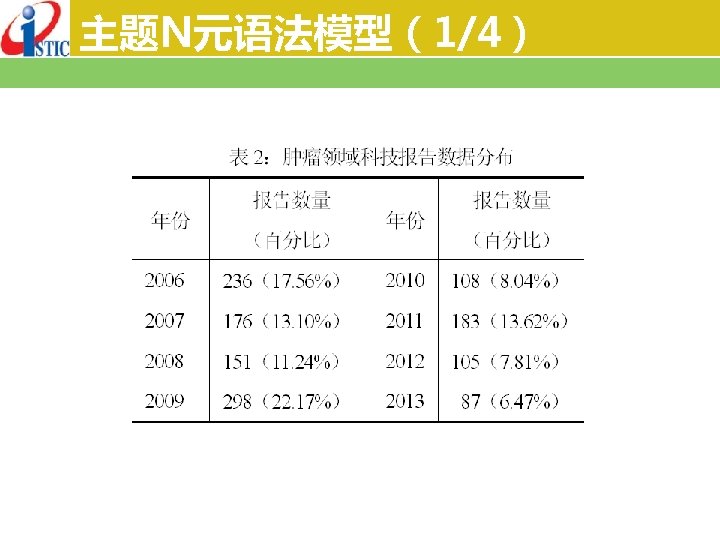
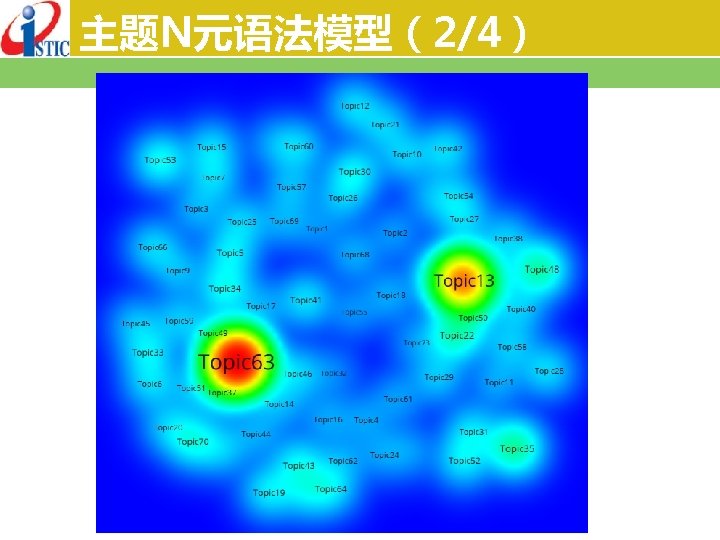
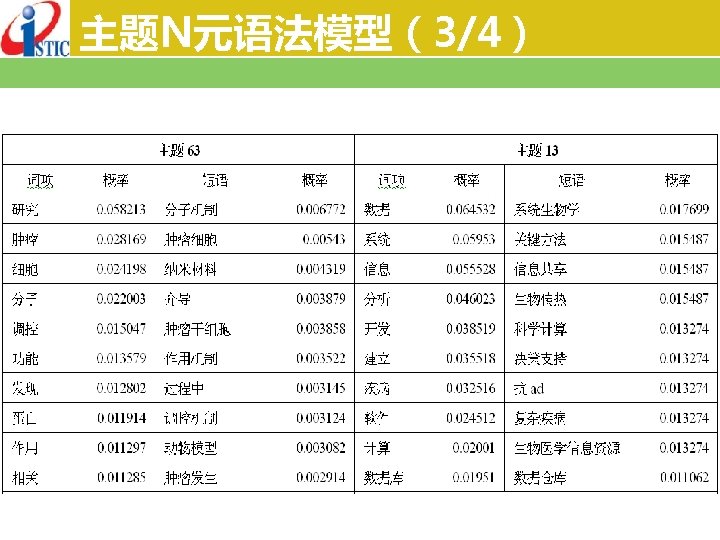
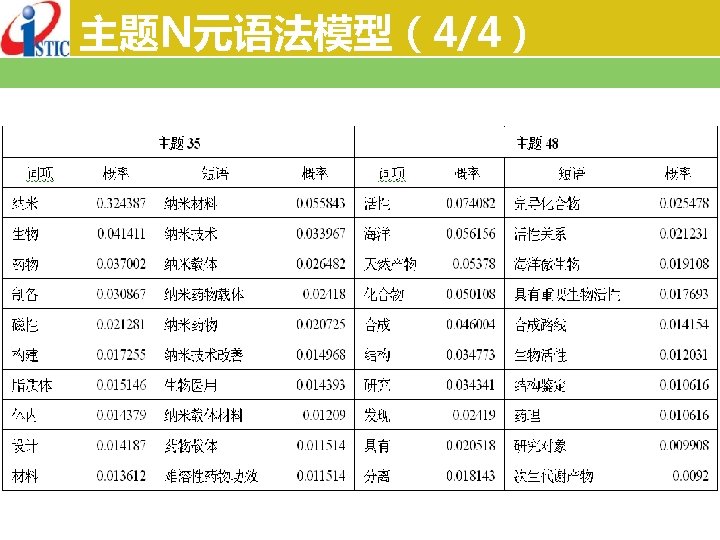
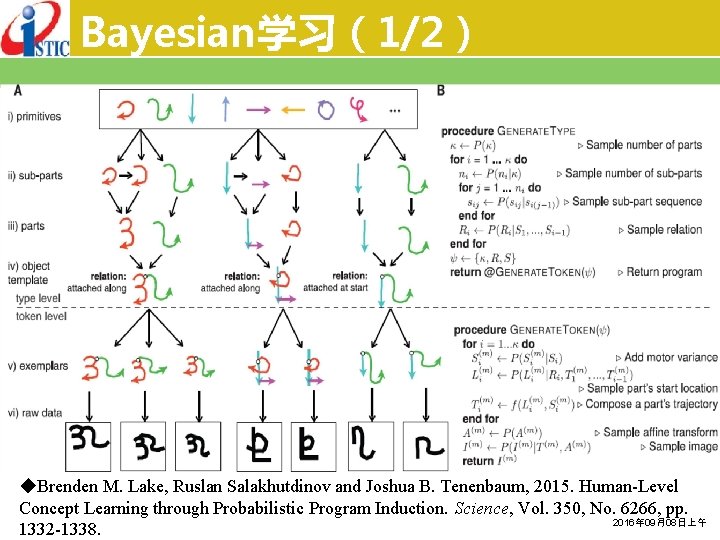
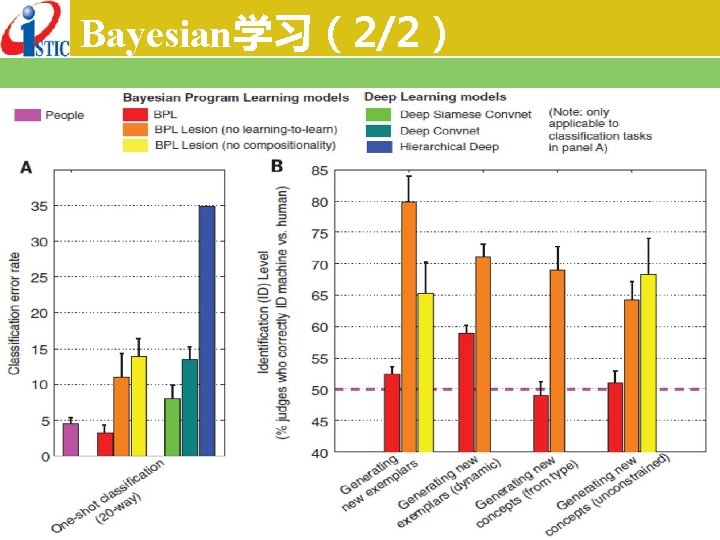
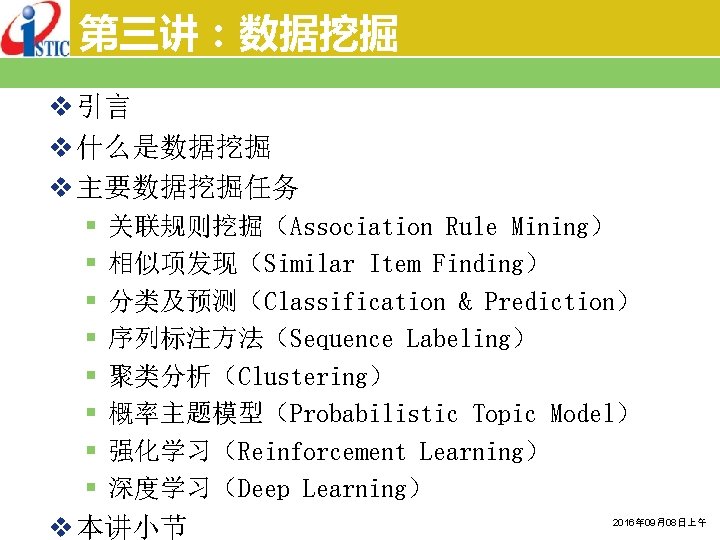
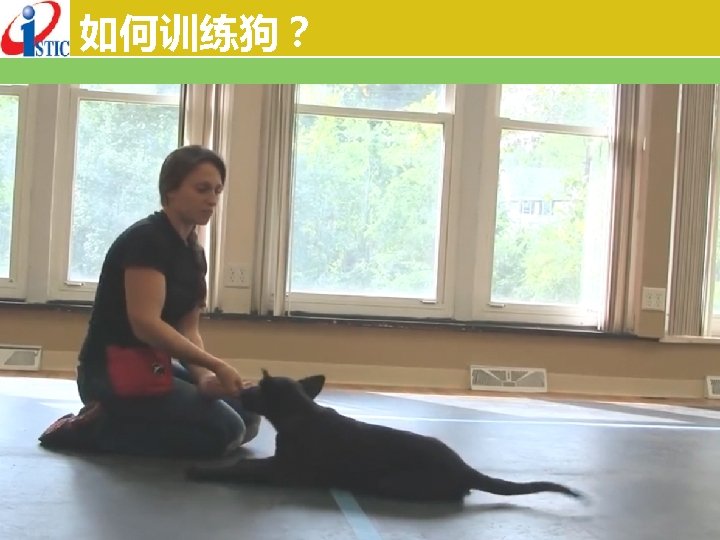
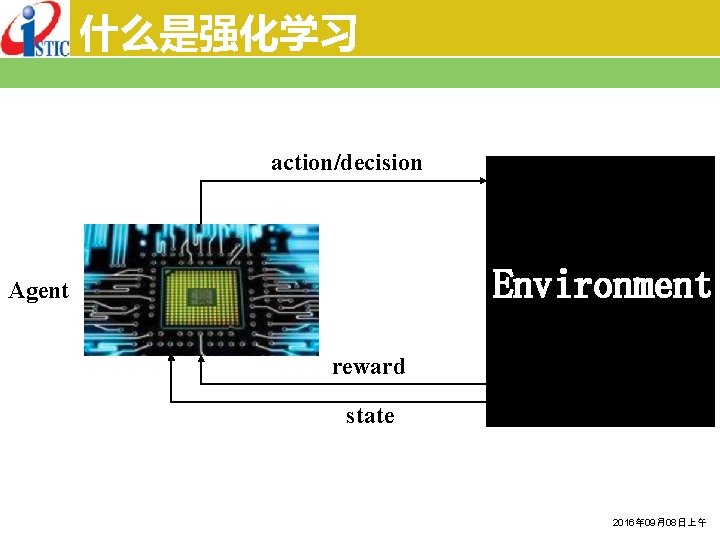
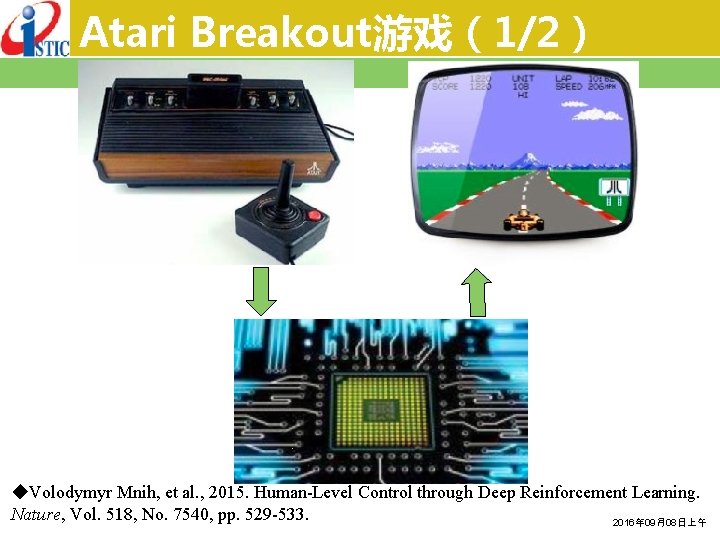
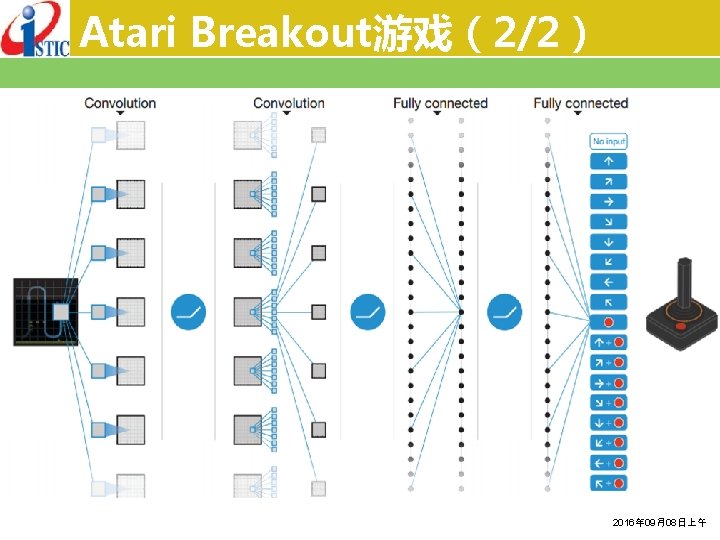
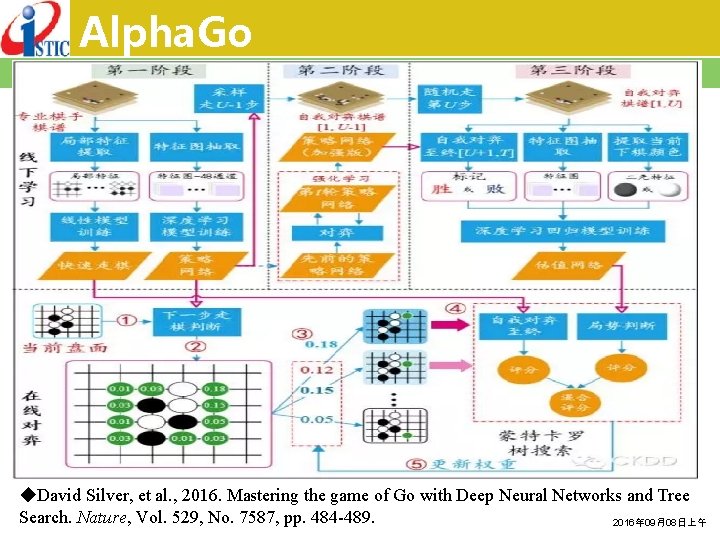
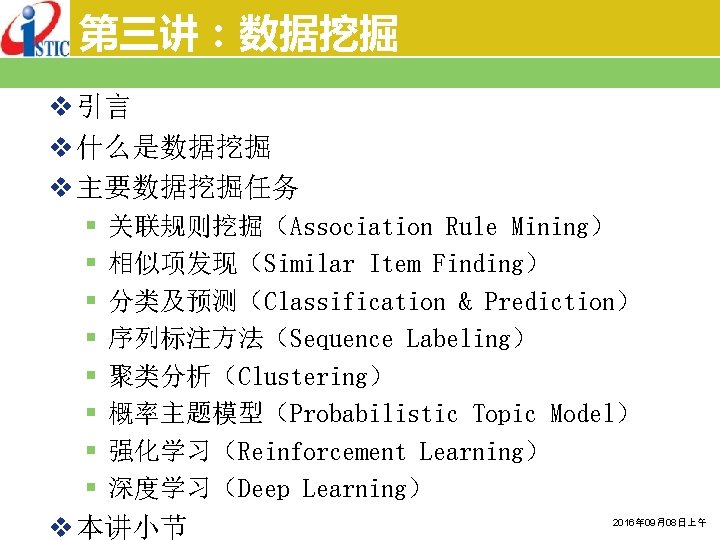
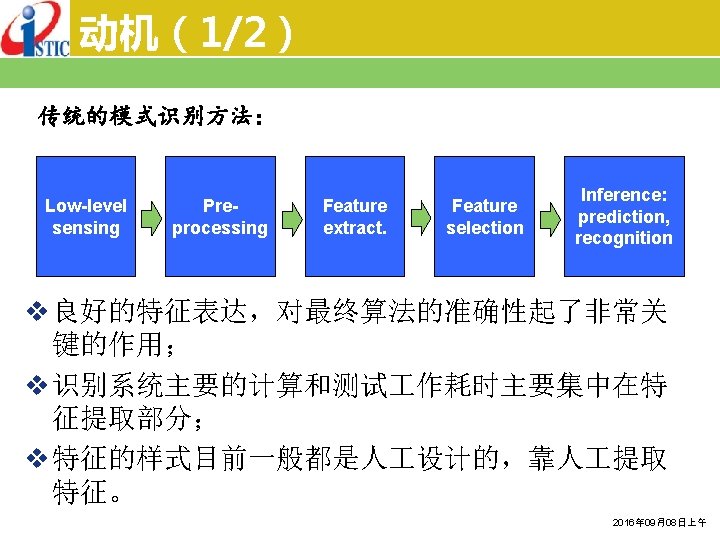
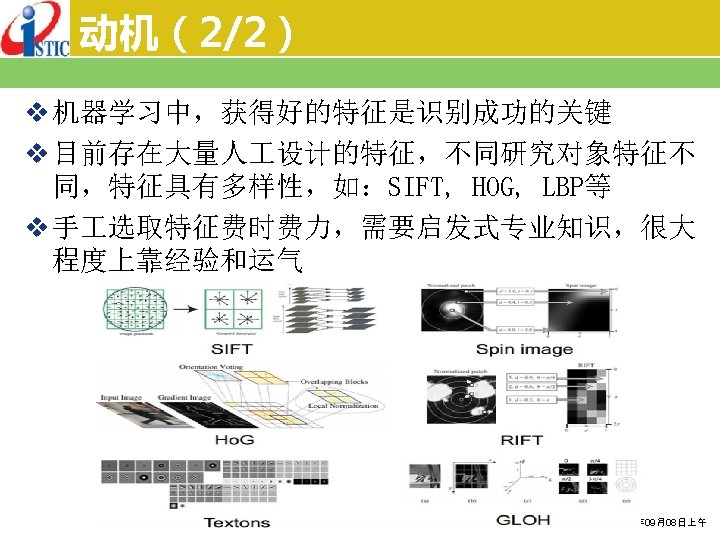
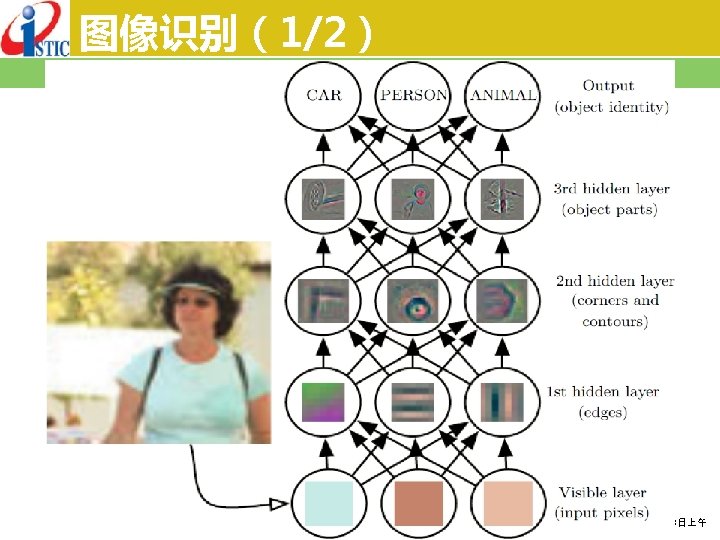
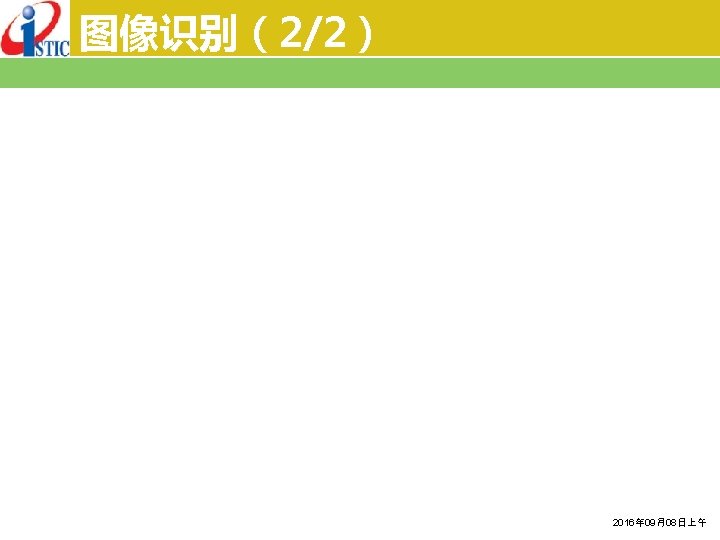
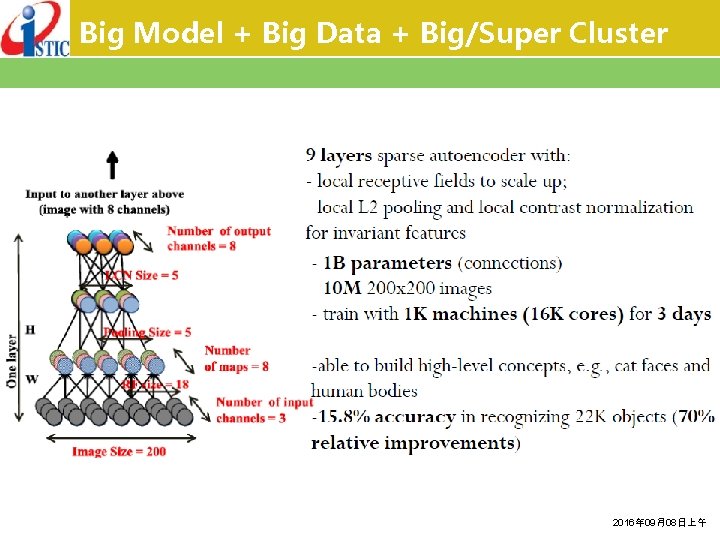
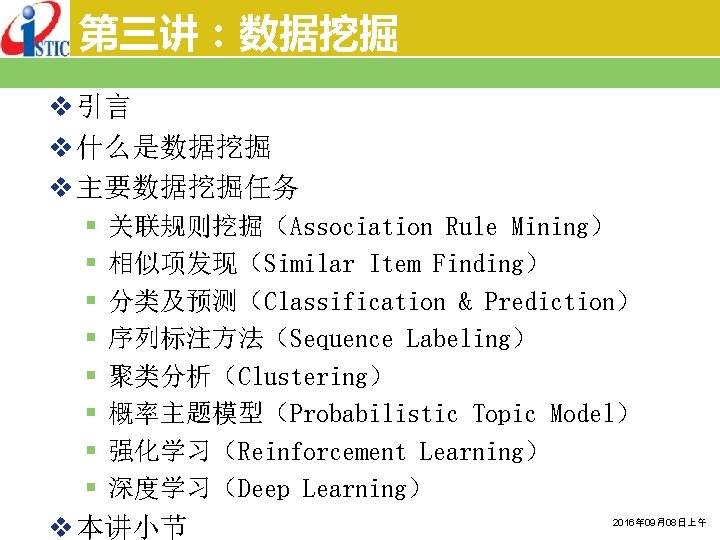
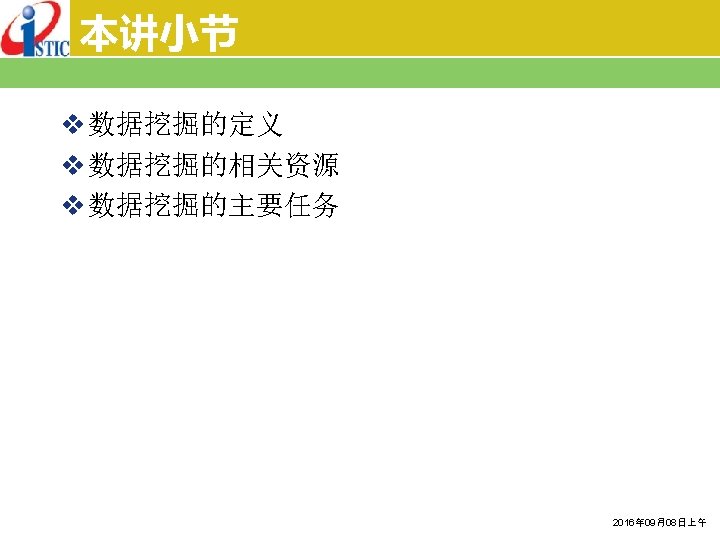
- Slides: 84
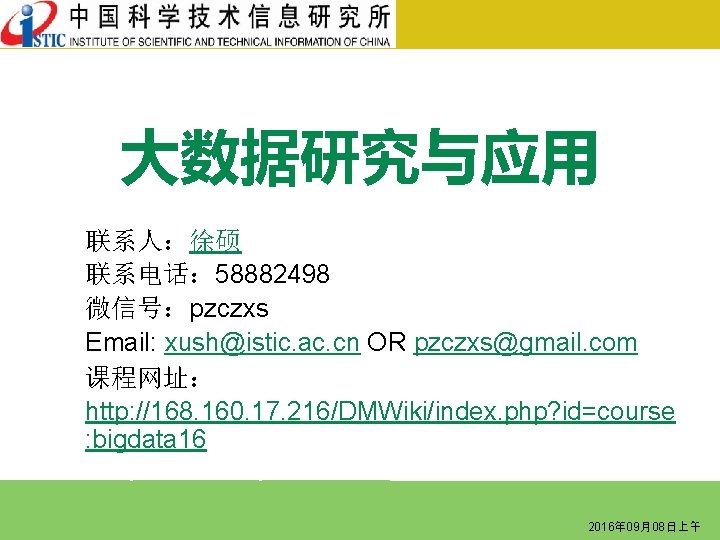
大数据研究与应用 联系人:徐硕 联系电话: 58882498 微信号:pzczxs Email: xush@istic. ac. cn OR pzczxs@gmail. com 课程网址: http: //168. 160. 17. 216/DMWiki/index. php? id=course : bigdata 16 2016年 09月08日上午
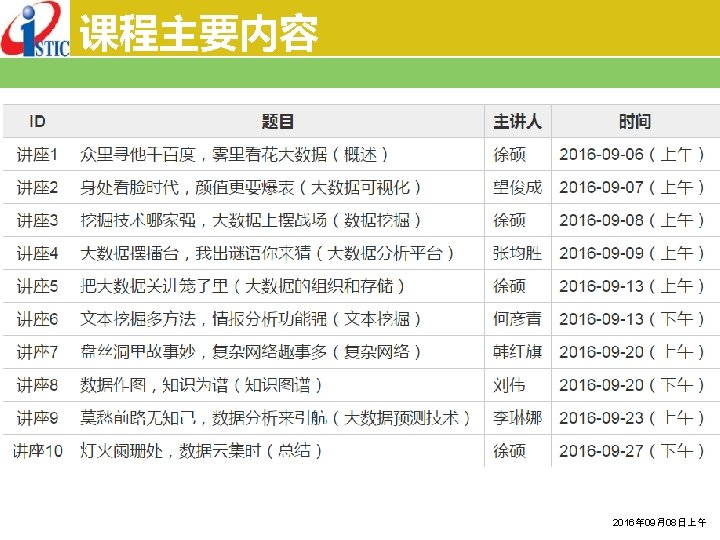
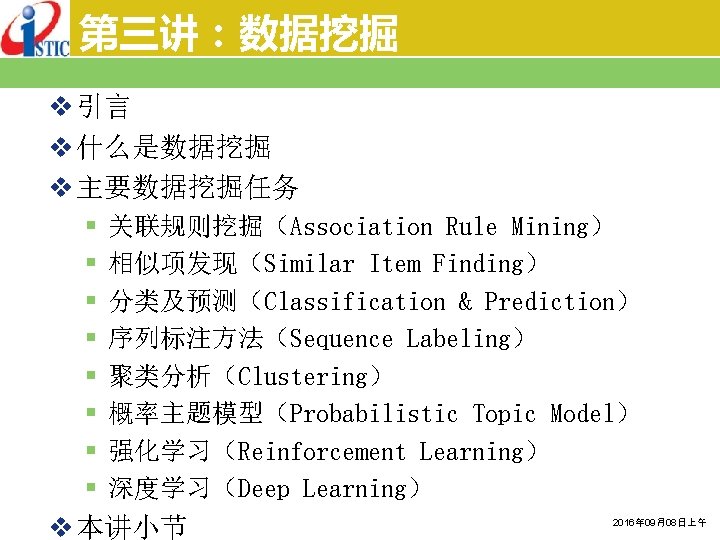
第三讲:数据挖掘 v 引言 v 什么是数据挖掘 v 主要数据挖掘任务 § § § § 关联规则挖掘(Association Rule Mining) 相似项发现(Similar Item Finding) 分类及预测(Classification & Prediction) 序列标注方法(Sequence Labeling) 聚类分析(Clustering) 概率主题模型(Probabilistic Topic Model) 强化学习(Reinforcement Learning) 深度学习(Deep Learning) v 本讲小节 2016年 09月08日上午
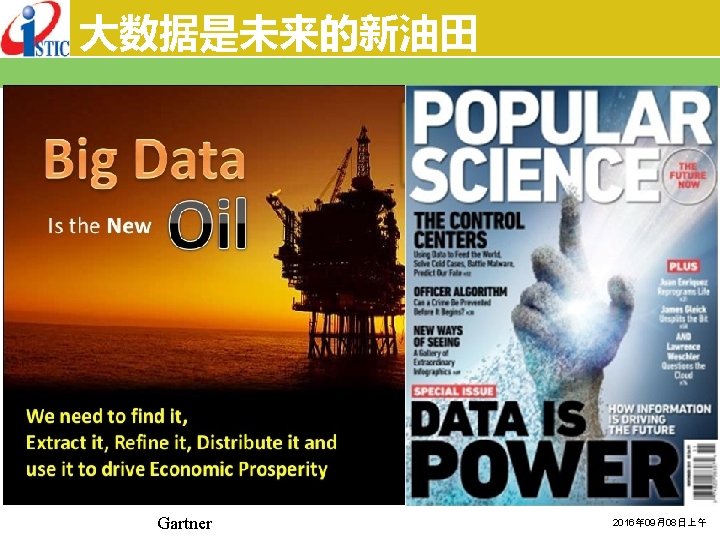
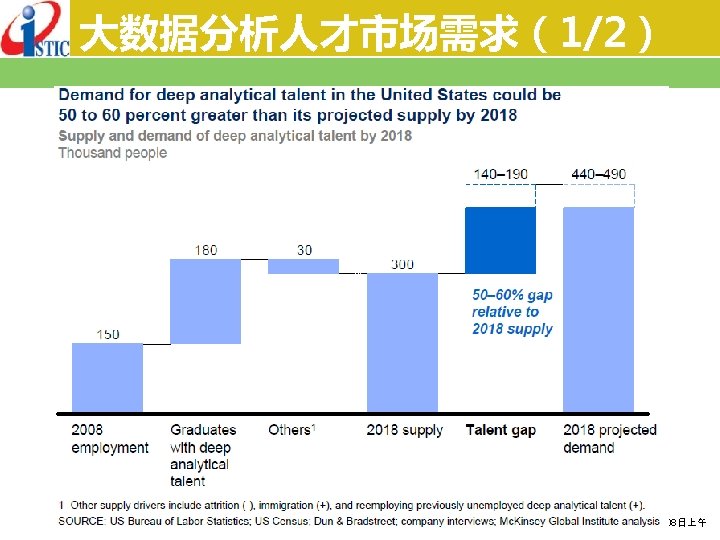
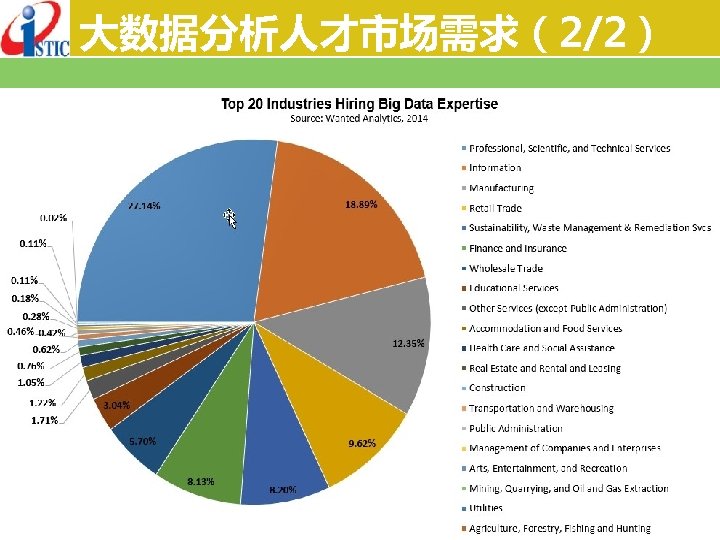
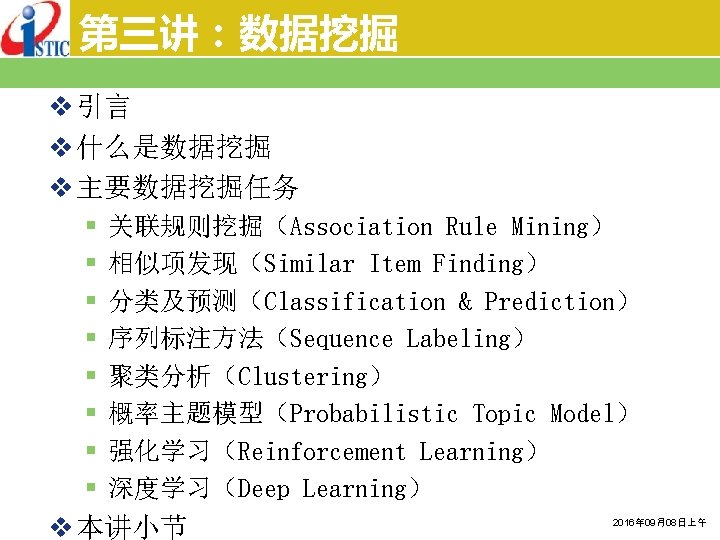
第三讲:数据挖掘 v 引言 v 什么是数据挖掘 v 主要数据挖掘任务 § § § § 关联规则挖掘(Association Rule Mining) 相似项发现(Similar Item Finding) 分类及预测(Classification & Prediction) 序列标注方法(Sequence Labeling) 聚类分析(Clustering) 概率主题模型(Probabilistic Topic Model) 强化学习(Reinforcement Learning) 深度学习(Deep Learning) v 本讲小节 2016年 09月08日上午
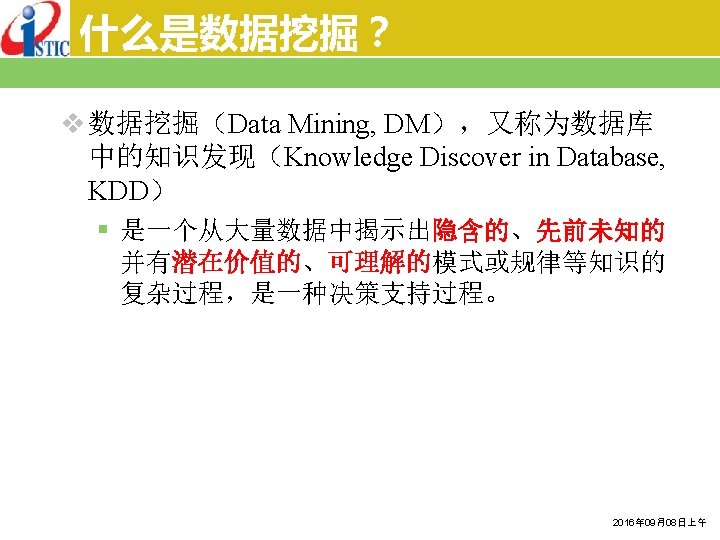
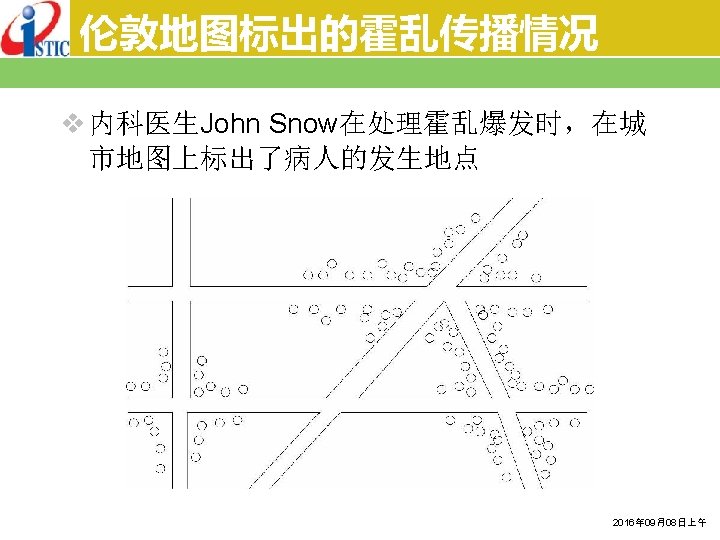
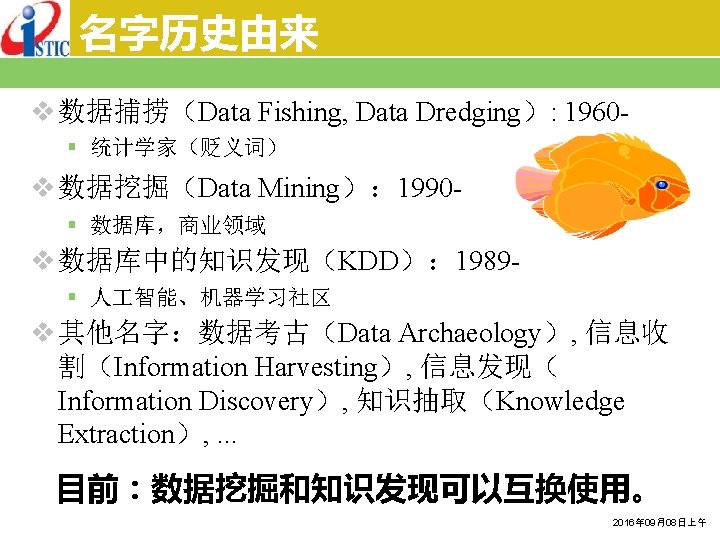
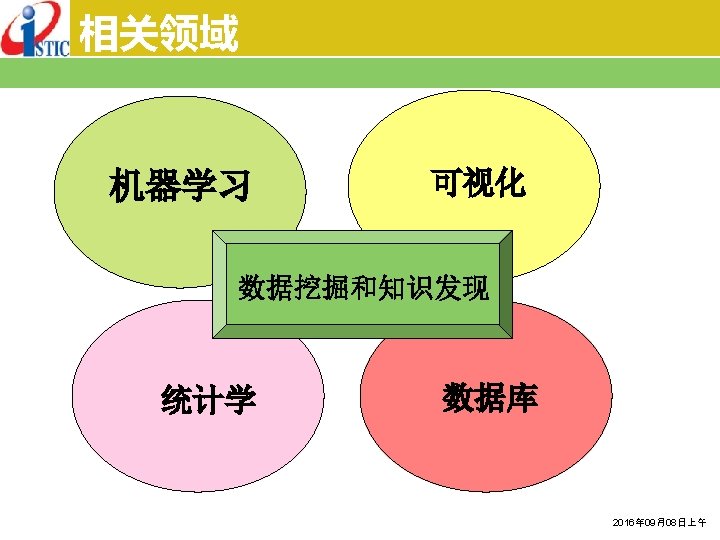
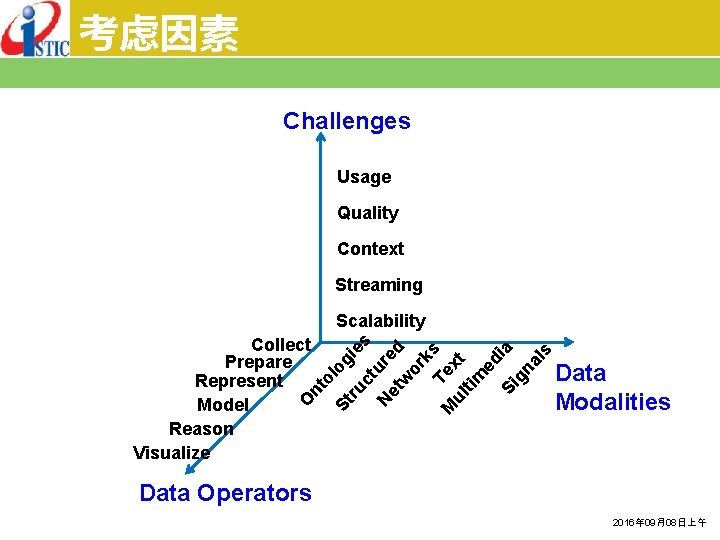
考虑因素 Challenges Usage Quality Context Streaming O nt Collect Prepare Represent Model Reason Visualize o St log ie ru s ct u Ne re tw d or ks M Tex ul tim t ed Si ia gn al s Scalability Data Modalities Data Operators 2016年 09月08日上午
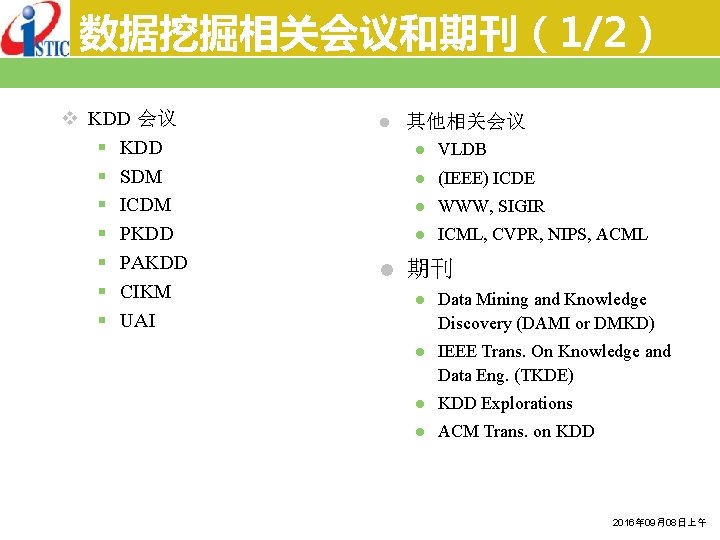
数据挖掘相关会议和期刊(1/2) v KDD 会议 § KDD § SDM § ICDM § PKDD § PAKDD § CIKM § UAI l l 其他相关会议 l VLDB l (IEEE) ICDE l WWW, SIGIR l ICML, CVPR, NIPS, ACML 期刊 l Data Mining and Knowledge Discovery (DAMI or DMKD) l IEEE Trans. On Knowledge and Data Eng. (TKDE) l KDD Explorations l ACM Trans. on KDD 2016年 09月08日上午
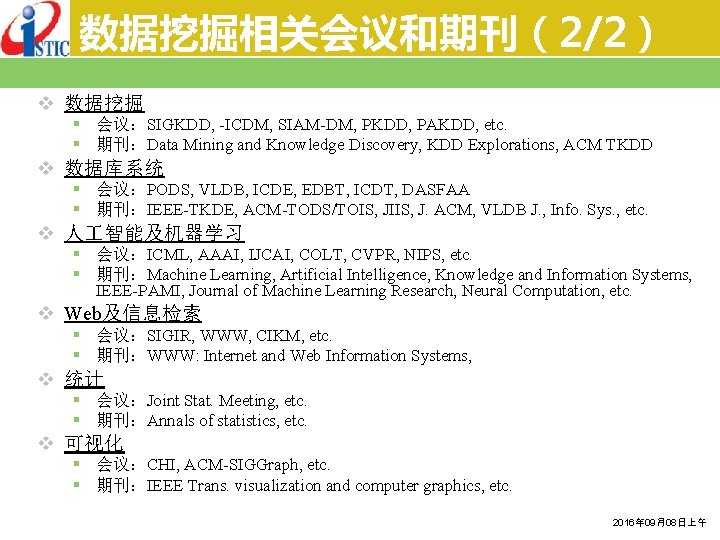
数据挖掘相关会议和期刊(2/2) v 数据挖掘 § 会议:SIGKDD, -ICDM, SIAM-DM, PKDD, PAKDD, etc. § 期刊:Data Mining and Knowledge Discovery, KDD Explorations, ACM TKDD v 数据库系统 § 会议:PODS, VLDB, ICDE, EDBT, ICDT, DASFAA § 期刊:IEEE-TKDE, ACM-TODS/TOIS, JIIS, J. ACM, VLDB J. , Info. Sys. , etc. v 人 智能及机器学习 § 会议:ICML, AAAI, IJCAI, COLT, CVPR, NIPS, etc. § 期刊:Machine Learning, Artificial Intelligence, Knowledge and Information Systems, IEEE-PAMI, Journal of Machine Learning Research, Neural Computation, etc. v Web及信息检索 § 会议:SIGIR, WWW, CIKM, etc. § 期刊:WWW: Internet and Web Information Systems, v 统计 § 会议:Joint Stat. Meeting, etc. § 期刊:Annals of statistics, etc. v 可视化 § 会议:CHI, ACM-SIGGraph, etc. § 期刊:IEEE Trans. visualization and computer graphics, etc. 2016年 09月08日上午
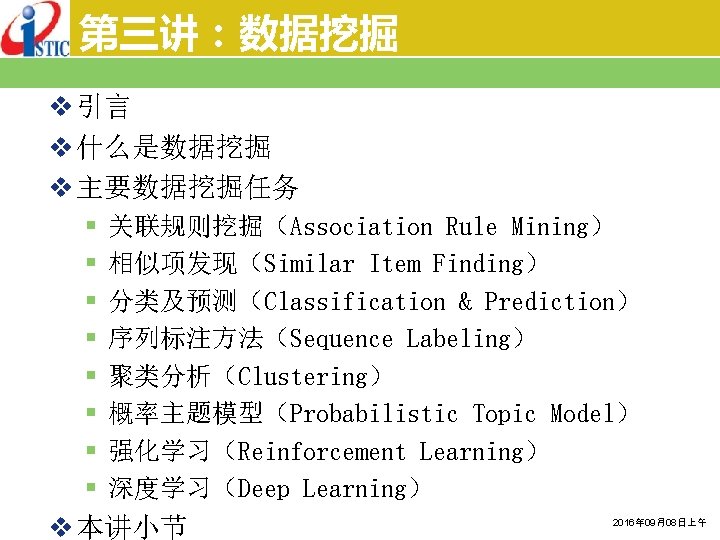
第三讲:数据挖掘 v 引言 v 什么是数据挖掘 v 主要数据挖掘任务 § § § § 关联规则挖掘(Association Rule Mining) 相似项发现(Similar Item Finding) 分类及预测(Classification & Prediction) 序列标注方法(Sequence Labeling) 聚类分析(Clustering) 概率主题模型(Probabilistic Topic Model) 强化学习(Reinforcement Learning) 深度学习(Deep Learning) v 本讲小节 2016年 09月08日上午
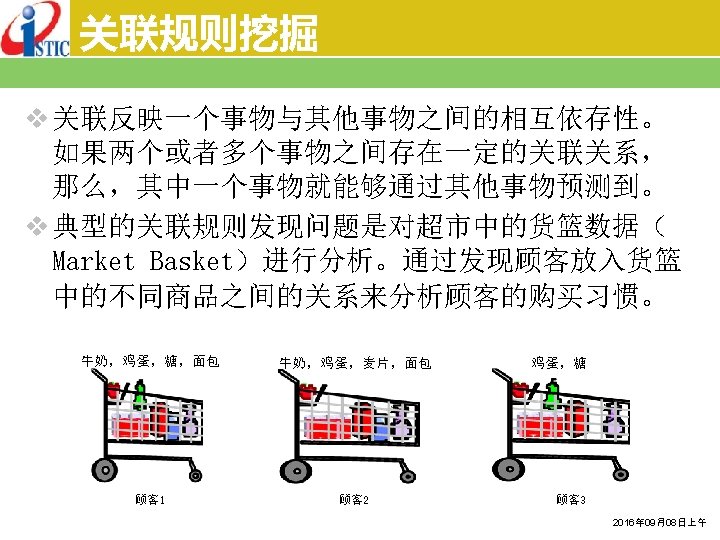
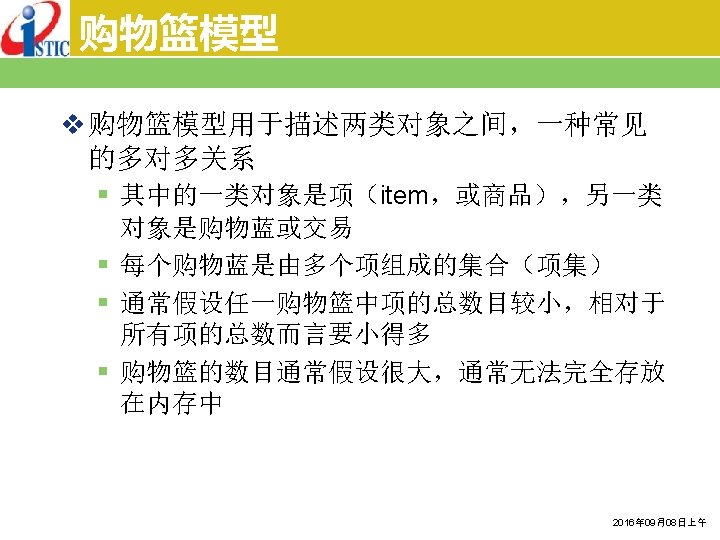
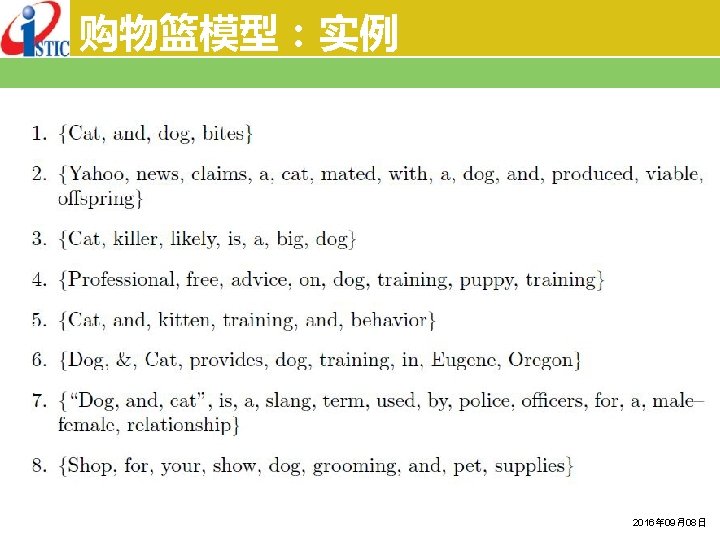
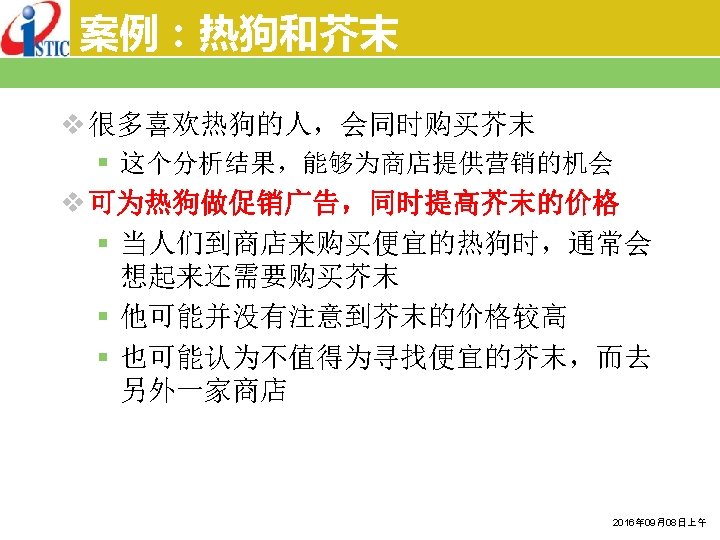
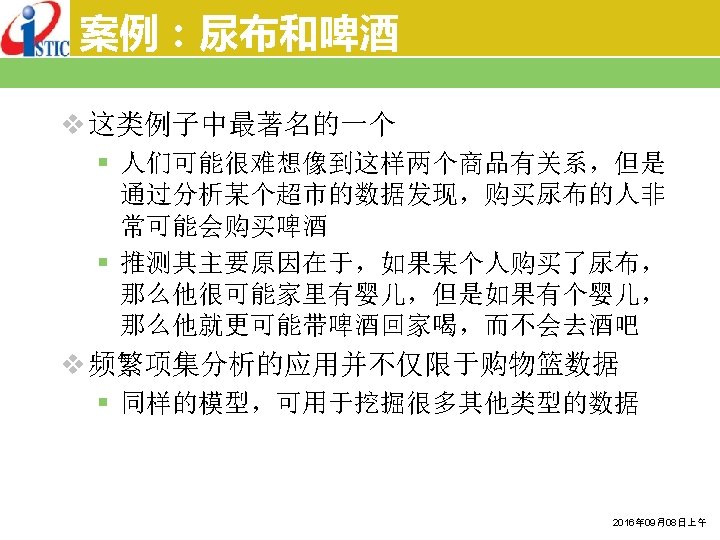
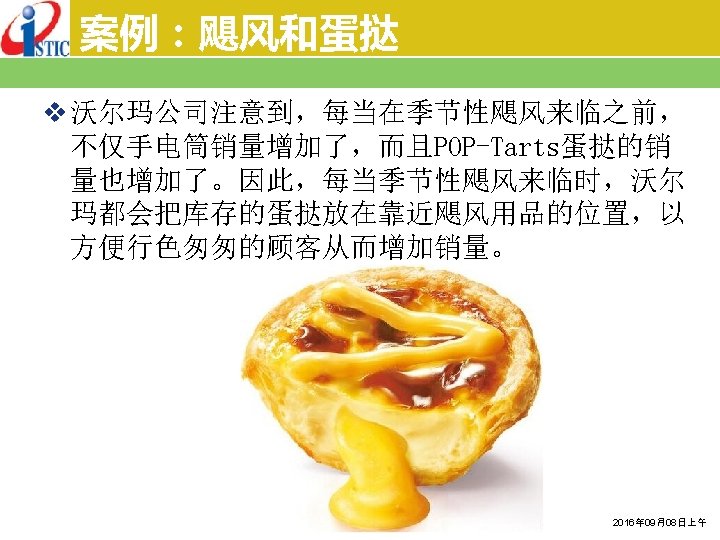
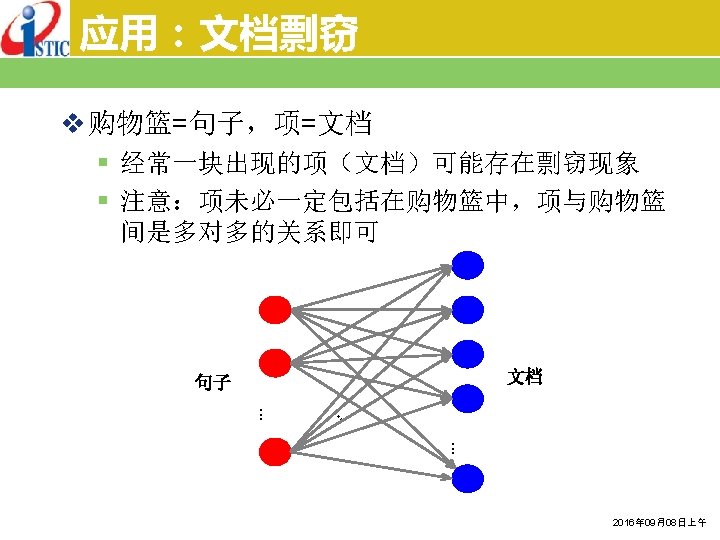
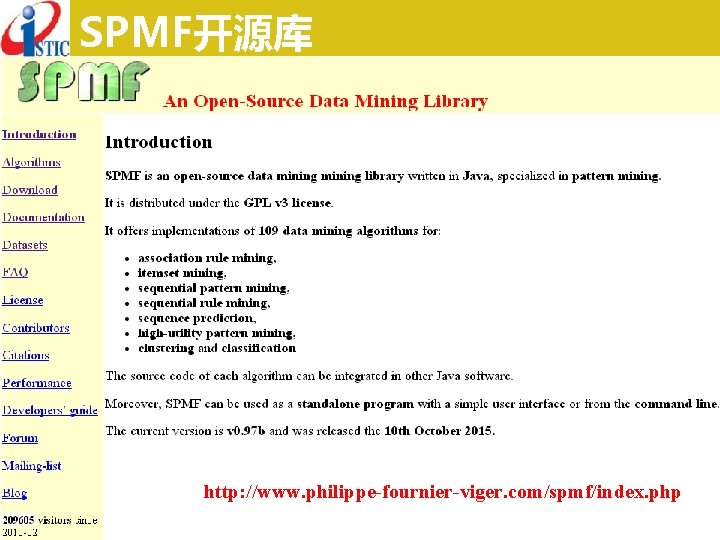
SPMF开源库 http: //www. philippe-fournier-viger. com/spmf/index. php 2016年 09月08日上午
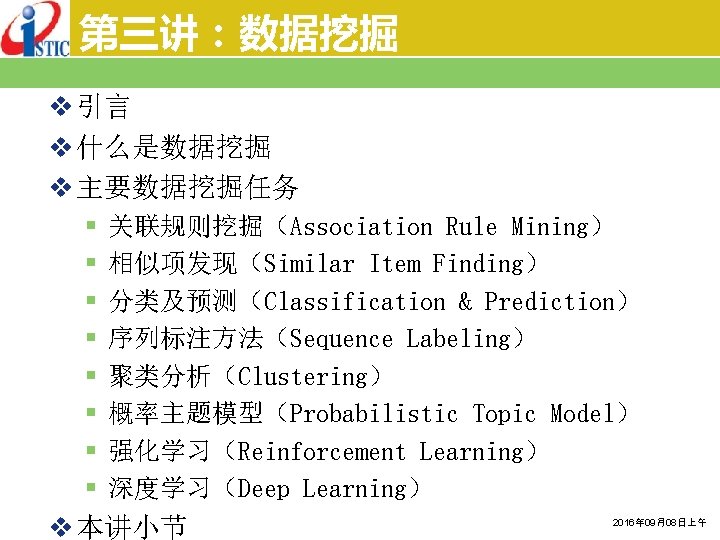
第三讲:数据挖掘 v 引言 v 什么是数据挖掘 v 主要数据挖掘任务 § § § § 关联规则挖掘(Association Rule Mining) 相似项发现(Similar Item Finding) 分类及预测(Classification & Prediction) 序列标注方法(Sequence Labeling) 聚类分析(Clustering) 概率主题模型(Probabilistic Topic Model) 强化学习(Reinforcement Learning) 深度学习(Deep Learning) v 本讲小节 2016年 09月08日上午
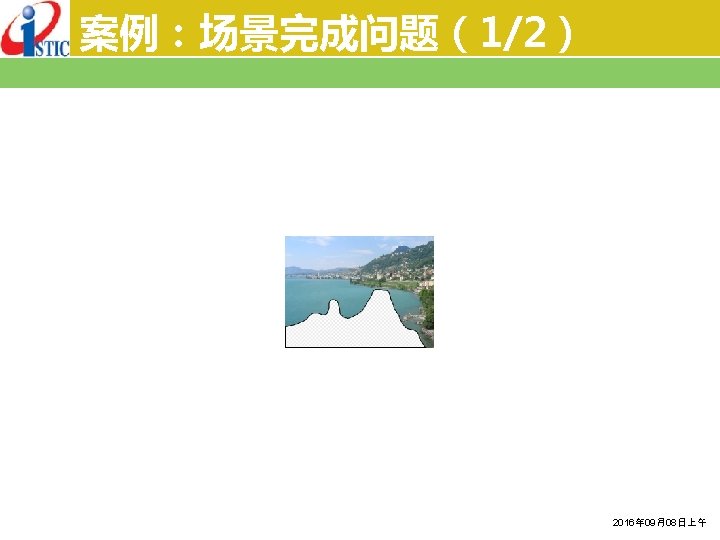
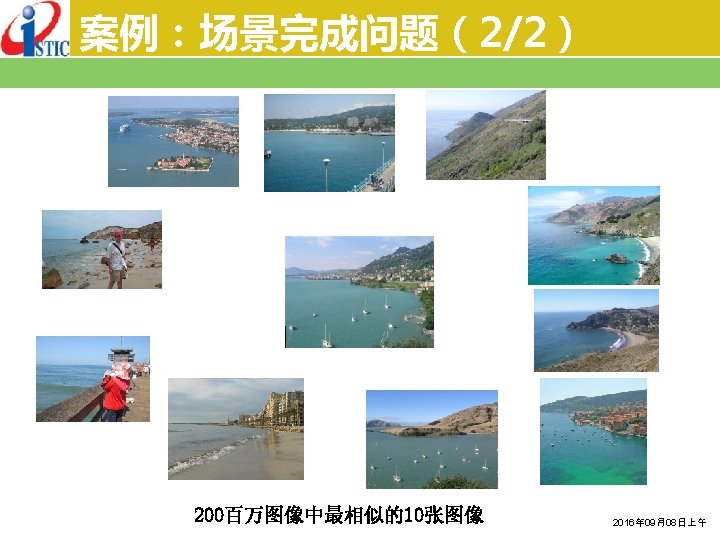
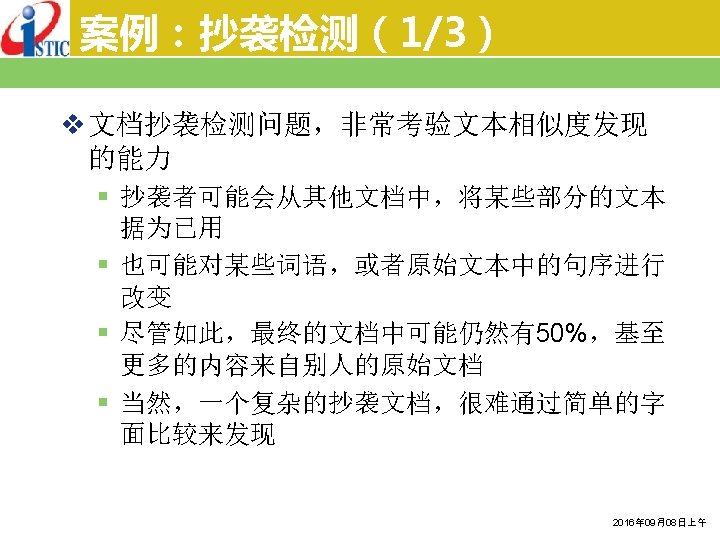
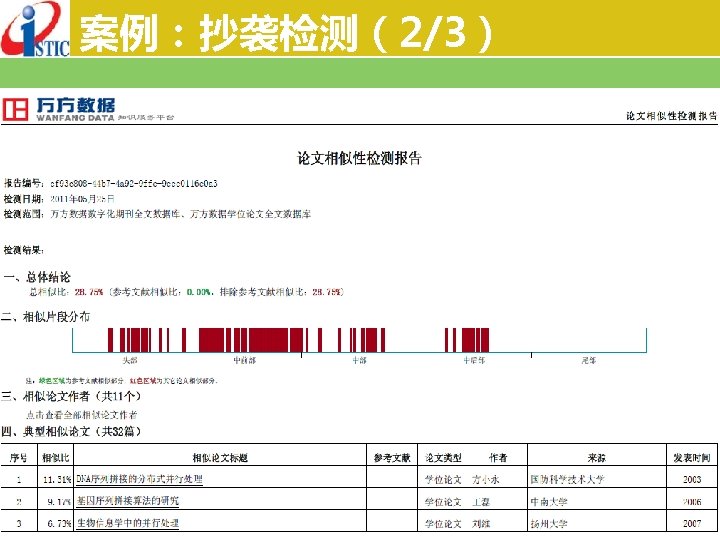
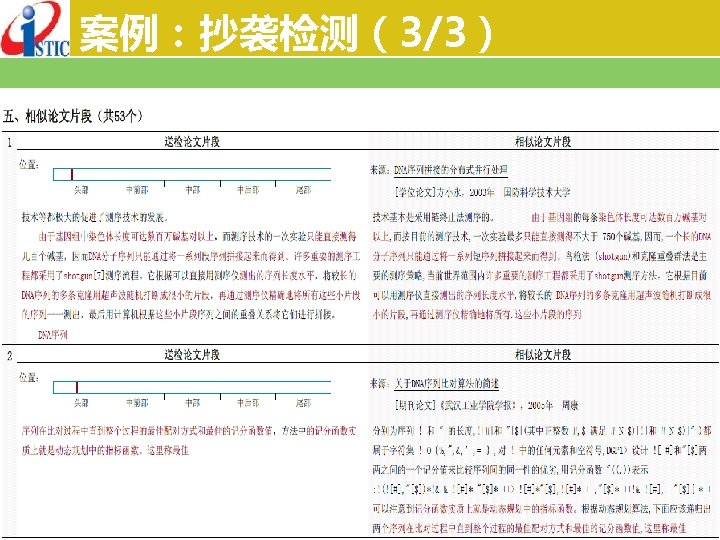
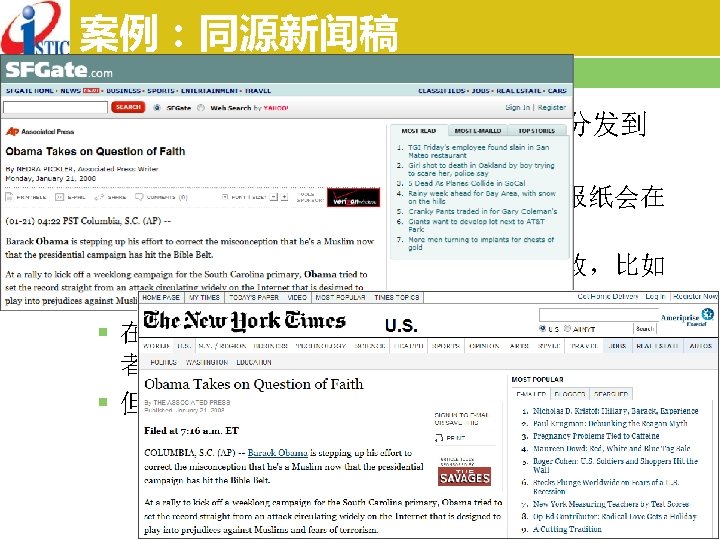
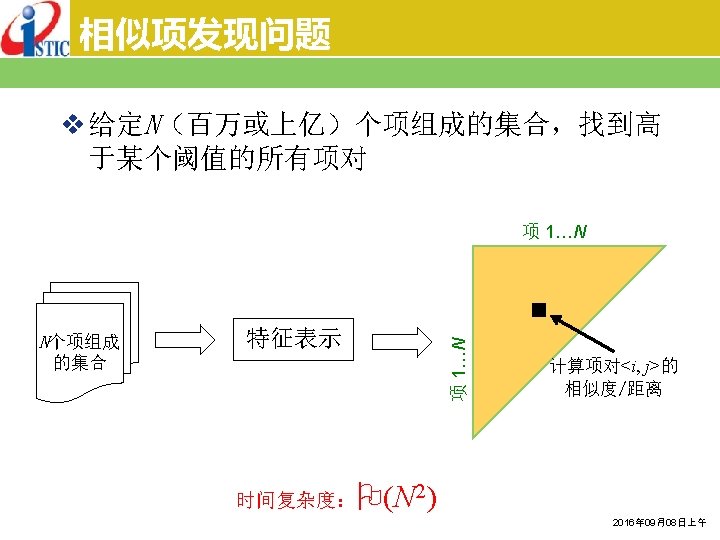
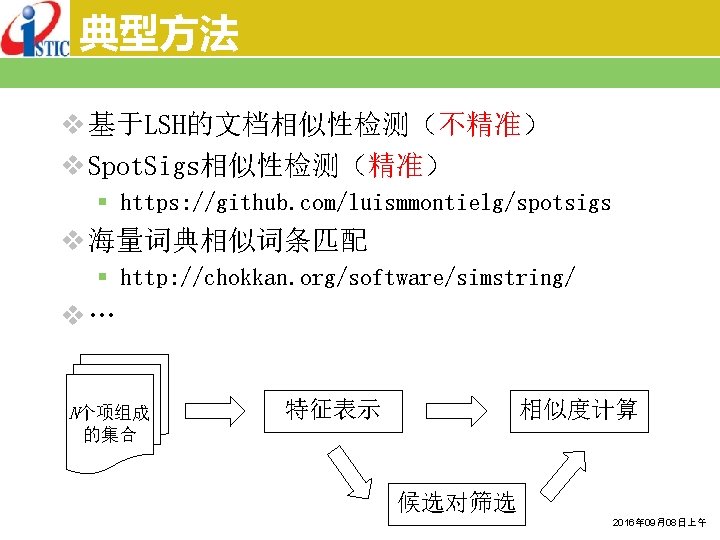
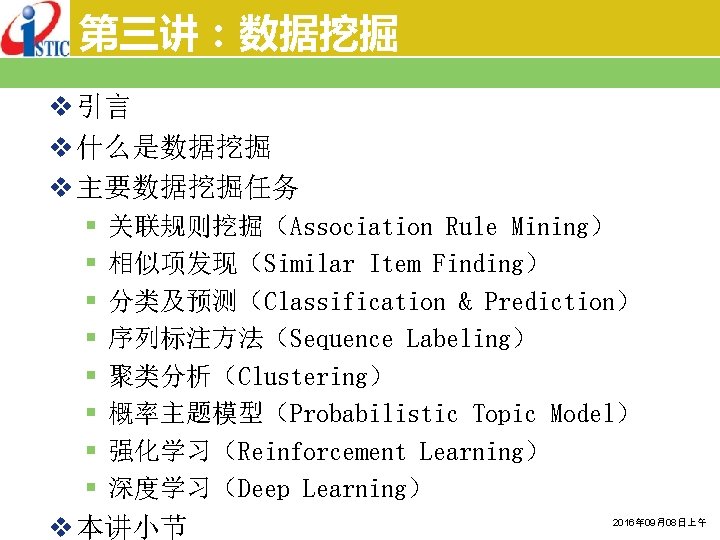
第三讲:数据挖掘 v 引言 v 什么是数据挖掘 v 主要数据挖掘任务 § § § § 关联规则挖掘(Association Rule Mining) 相似项发现(Similar Item Finding) 分类及预测(Classification & Prediction) 序列标注方法(Sequence Labeling) 聚类分析(Clustering) 概率主题模型(Probabilistic Topic Model) 强化学习(Reinforcement Learning) 深度学习(Deep Learning) v 本讲小节 2016年 09月08日上午
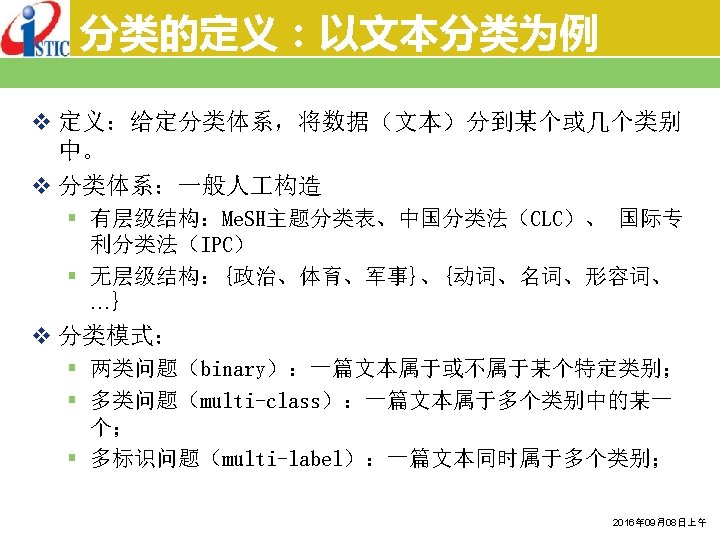
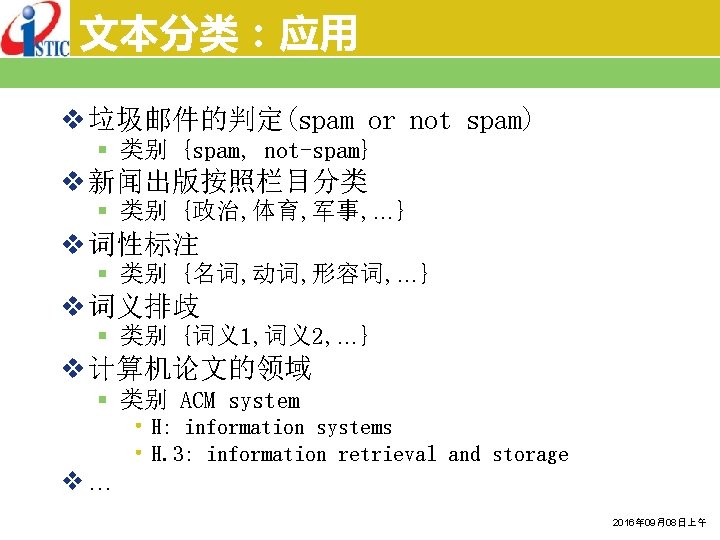
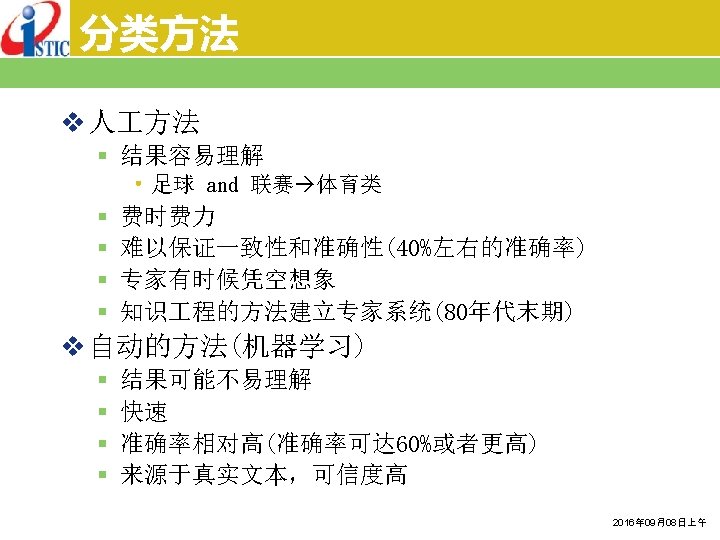
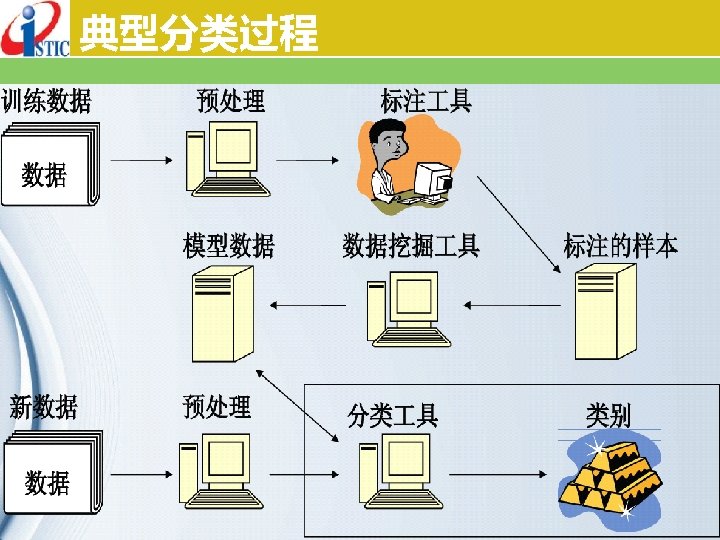
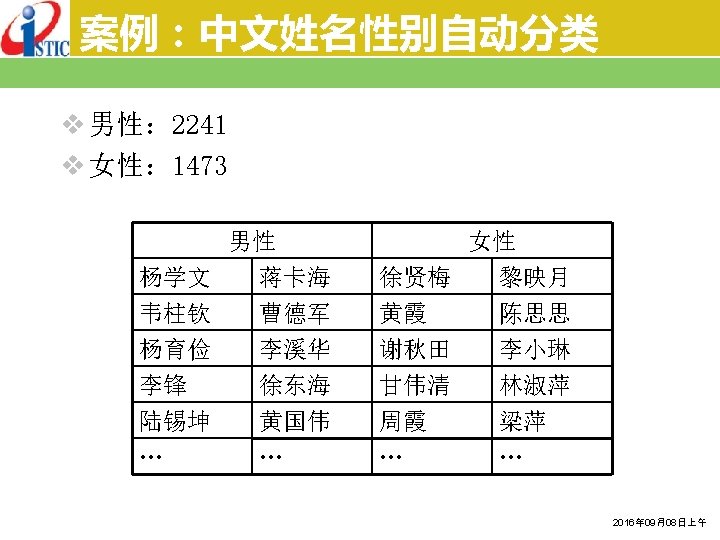
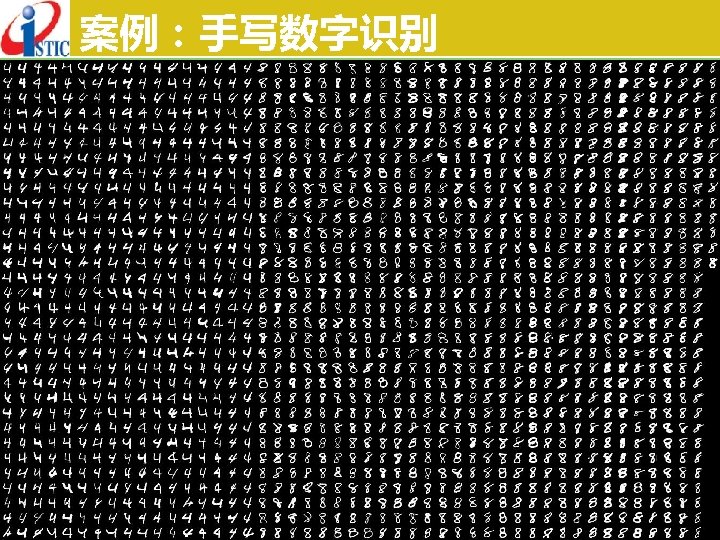
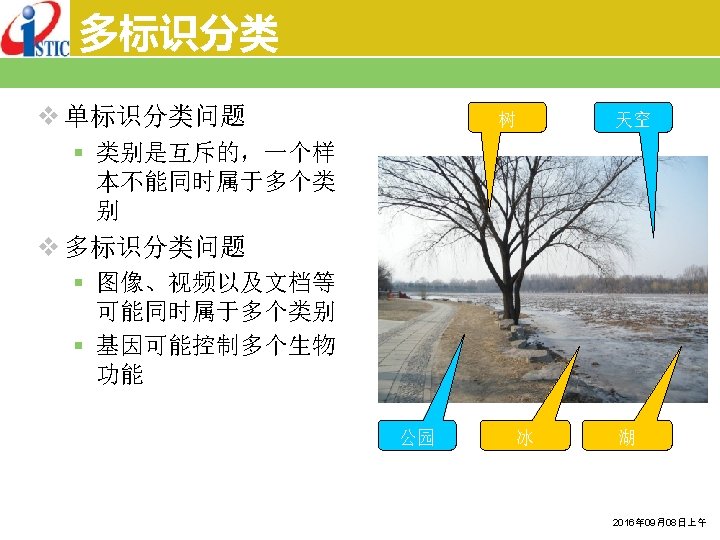
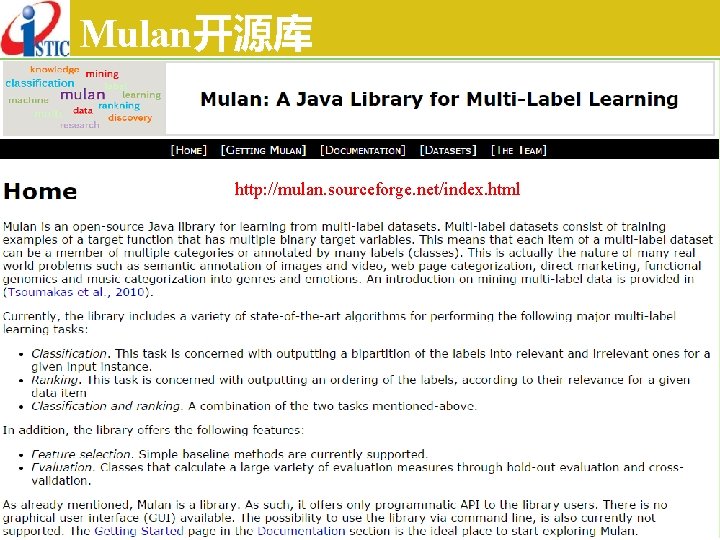
Mulan开源库 http: //mulan. sourceforge. net/index. html 2016年 09月08日上午
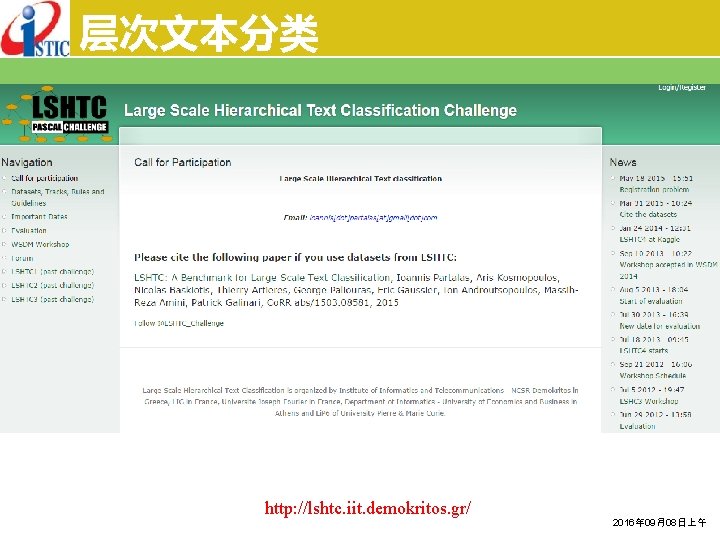
层次文本分类 http: //lshtc. iit. demokritos. gr/ 2016年 09月08日上午
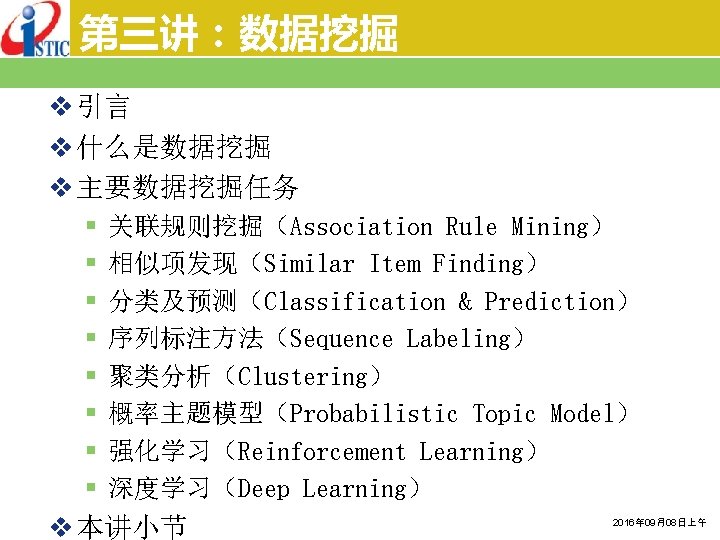
第三讲:数据挖掘 v 引言 v 什么是数据挖掘 v 主要数据挖掘任务 § § § § 关联规则挖掘(Association Rule Mining) 相似项发现(Similar Item Finding) 分类及预测(Classification & Prediction) 序列标注方法(Sequence Labeling) 聚类分析(Clustering) 概率主题模型(Probabilistic Topic Model) 强化学习(Reinforcement Learning) 深度学习(Deep Learning) v 本讲小节 2016年 09月08日上午
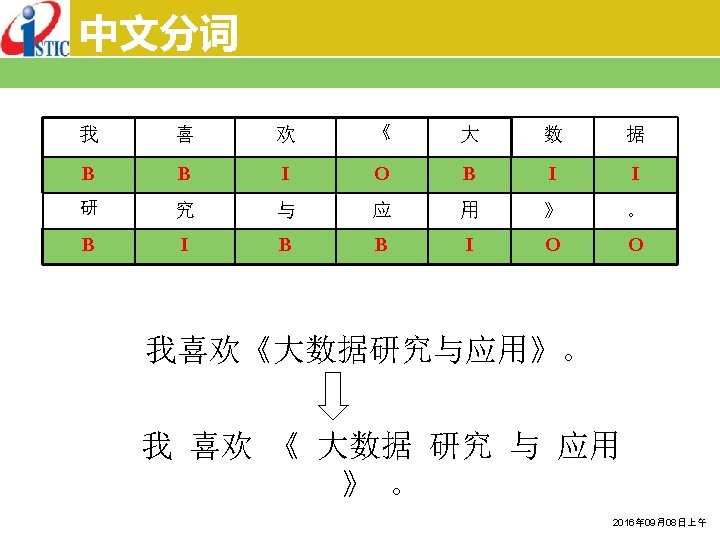
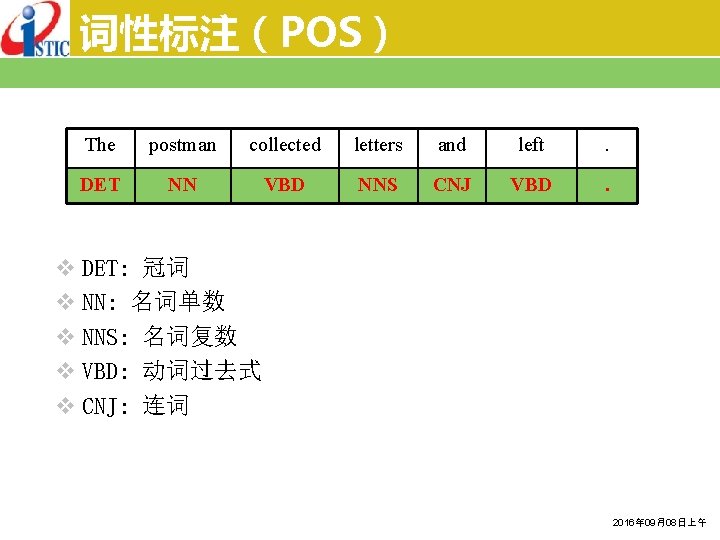
词性标注(POS) The postman collected letters and left . DET NN VBD NNS CNJ VBD . v DET: 冠词 v NN: 名词单数 v NNS: 名词复数 v VBD: 动词过去式 v CNJ: 连词 2016年 09月08日上午
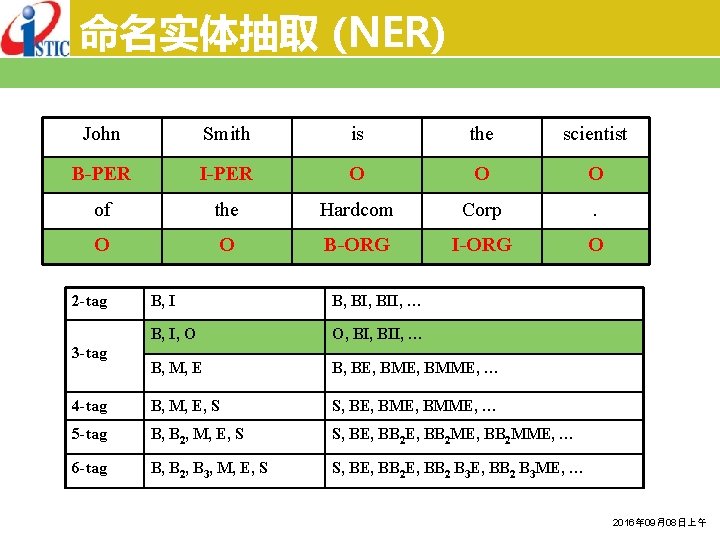
命名实体抽取 (NER) John Smith is the scientist B-PER I-PER O O O of the Hardcom Corp . O O B-ORG I-ORG O 2 -tag B, I B, BII, … B, I, O O, BII, … B, M, E B, BE, BMME, … 4 -tag B, M, E, S S, BE, BMME, … 5 -tag B, B 2, M, E, S S, BE, BB 2 ME, BB 2 MME, … 6 -tag B, B 2, B 3, M, E, S S, BE, BB 2 B 3 E, BB 2 B 3 ME, … 3 -tag 2016年 09月08日上午
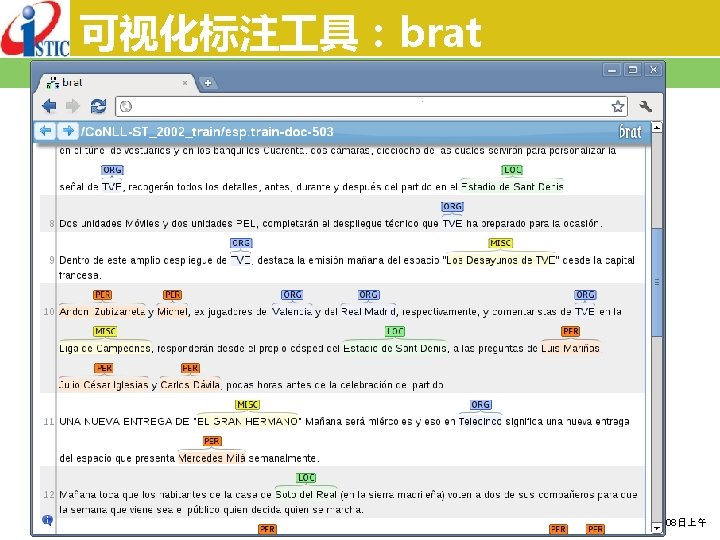
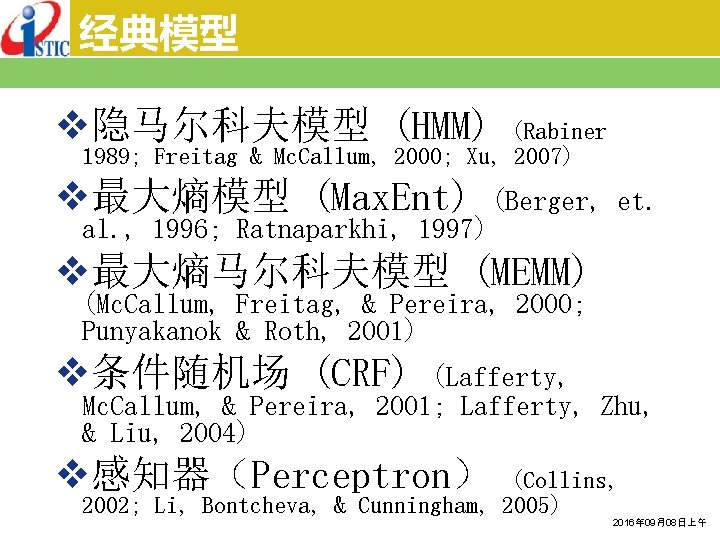
经典模型 v隐马尔科夫模型 (HMM) (Rabiner 1989; Freitag & Mc. Callum, 2000; Xu, 2007) v最大熵模型 (Max. Ent) (Berger, et. al. , 1996; Ratnaparkhi, 1997) v最大熵马尔科夫模型 (MEMM) (Mc. Callum, Freitag, & Pereira, 2000; Punyakanok & Roth, 2001) v条件随机场 (CRF) (Lafferty, Mc. Callum, & Pereira, 2001; Lafferty, Zhu, & Liu, 2004) v感知器(Perceptron) (Collins, 2002; Li, Bontcheva, & Cunningham, 2005) 2016年 09月08日上午
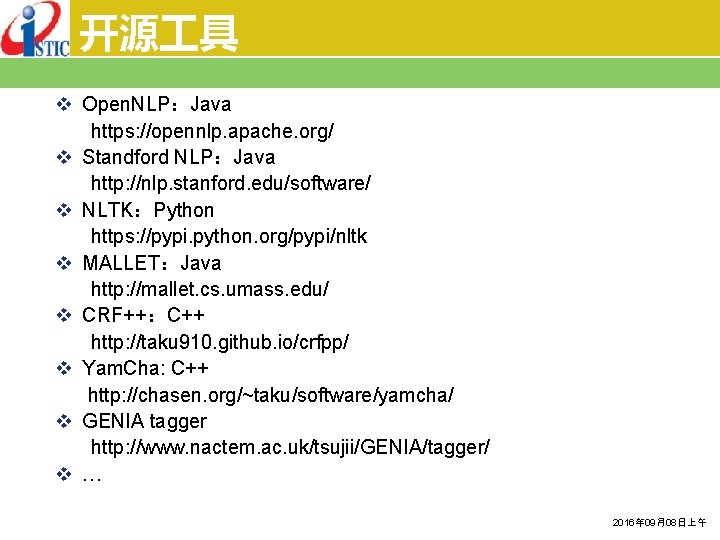
开源 具 v Open. NLP:Java https: //opennlp. apache. org/ v Standford NLP:Java http: //nlp. stanford. edu/software/ v NLTK:Python https: //pypi. python. org/pypi/nltk v MALLET:Java http: //mallet. cs. umass. edu/ v CRF++:C++ http: //taku 910. github. io/crfpp/ v Yam. Cha: C++ http: //chasen. org/~taku/software/yamcha/ v GENIA tagger http: //www. nactem. ac. uk/tsujii/GENIA/tagger/ v … 2016年 09月08日上午
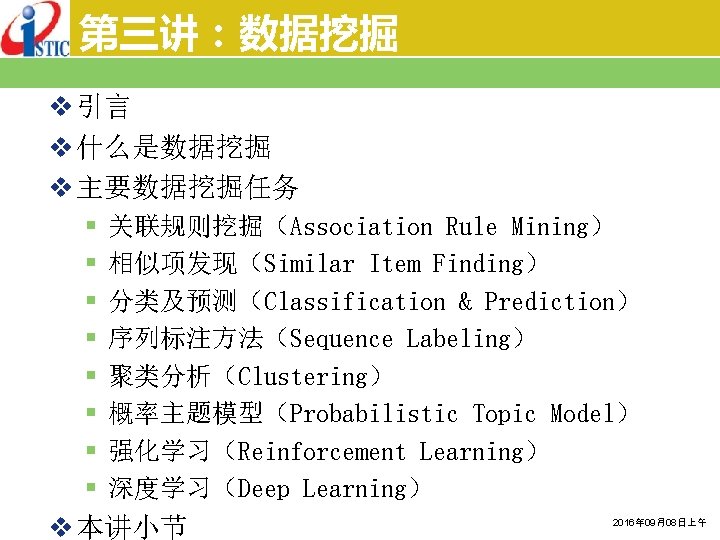
第三讲:数据挖掘 v 引言 v 什么是数据挖掘 v 主要数据挖掘任务 § § § § 关联规则挖掘(Association Rule Mining) 相似项发现(Similar Item Finding) 分类及预测(Classification & Prediction) 序列标注方法(Sequence Labeling) 聚类分析(Clustering) 概率主题模型(Probabilistic Topic Model) 强化学习(Reinforcement Learning) 深度学习(Deep Learning) v 本讲小节 2016年 09月08日上午
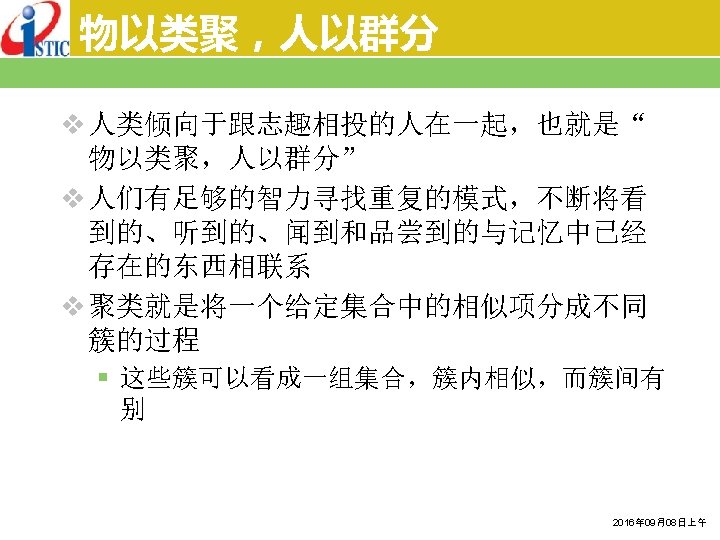
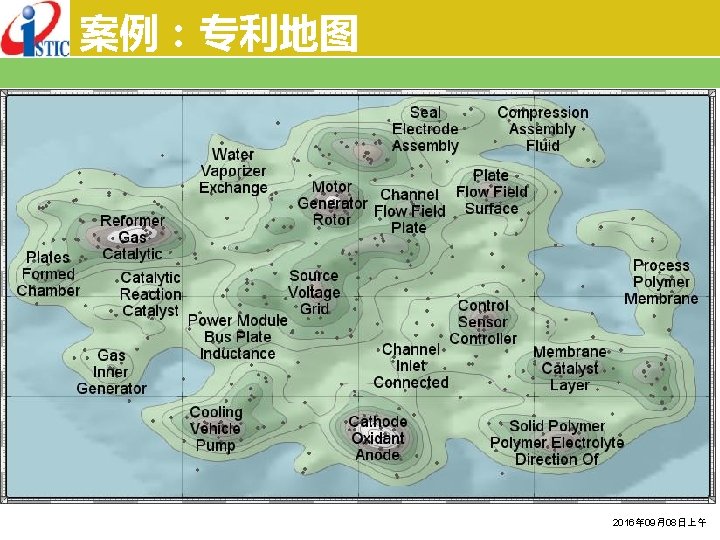
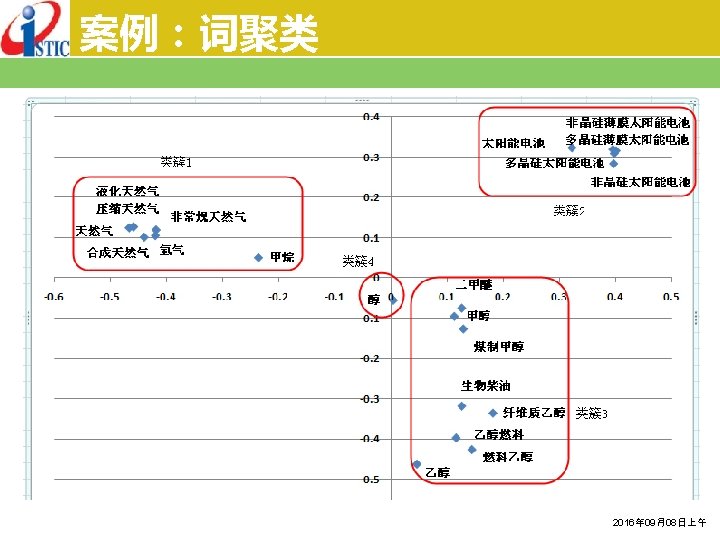
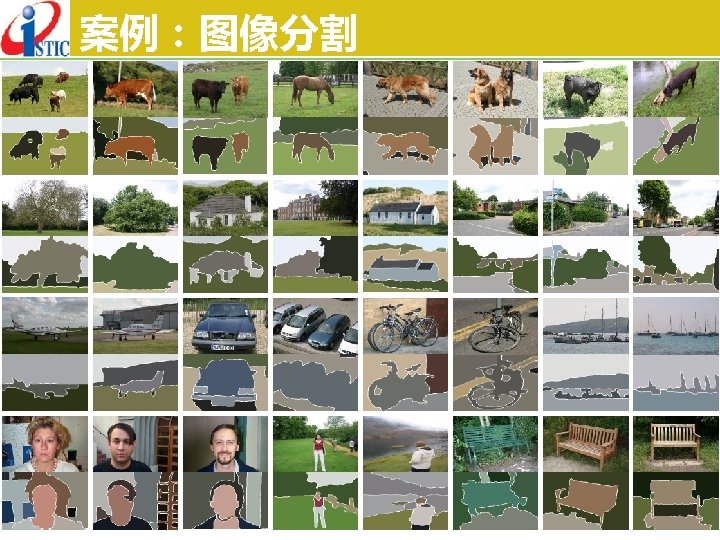
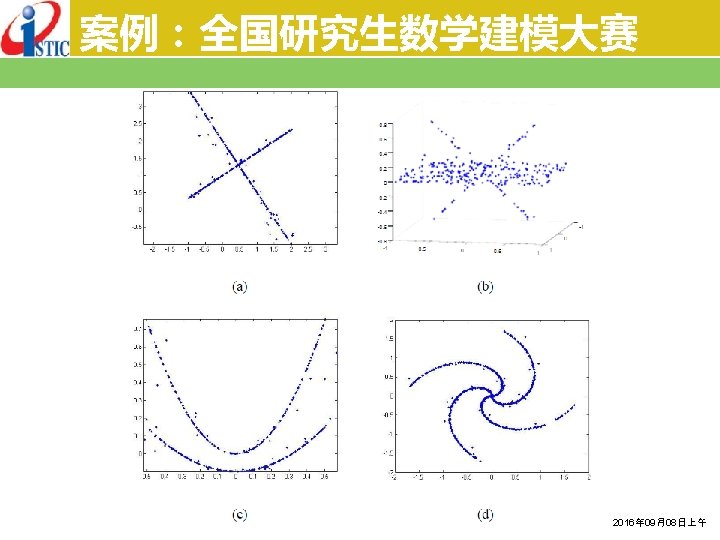
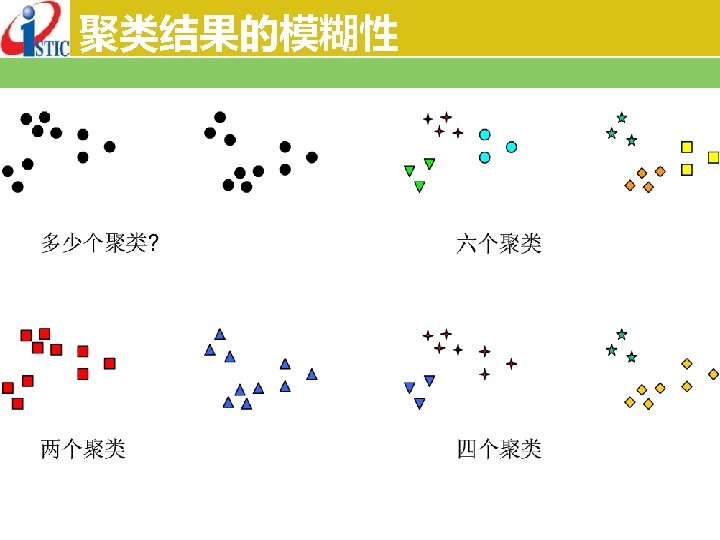
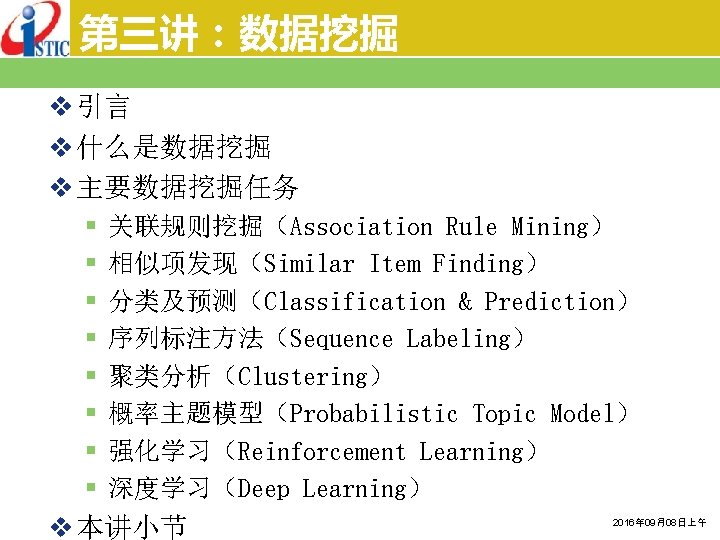
第三讲:数据挖掘 v 引言 v 什么是数据挖掘 v 主要数据挖掘任务 § § § § 关联规则挖掘(Association Rule Mining) 相似项发现(Similar Item Finding) 分类及预测(Classification & Prediction) 序列标注方法(Sequence Labeling) 聚类分析(Clustering) 概率主题模型(Probabilistic Topic Model) 强化学习(Reinforcement Learning) 深度学习(Deep Learning) v 本讲小节 2016年 09月08日上午
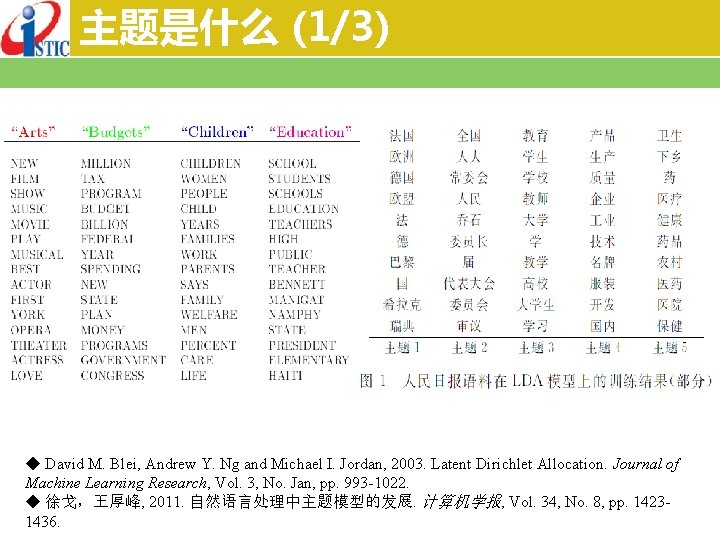
主题是什么 (1/3) ◆ David M. Blei, Andrew Y. Ng and Michael I. Jordan, 2003. Latent Dirichlet Allocation. Journal of Machine Learning Research, Vol. 3, No. Jan, pp. 993 -1022. ◆ 徐戈,王厚峰, 2011. 自然语言处理中主题模型的发展. 计算机学报, Vol. 34, No. 8, pp. 14231436.
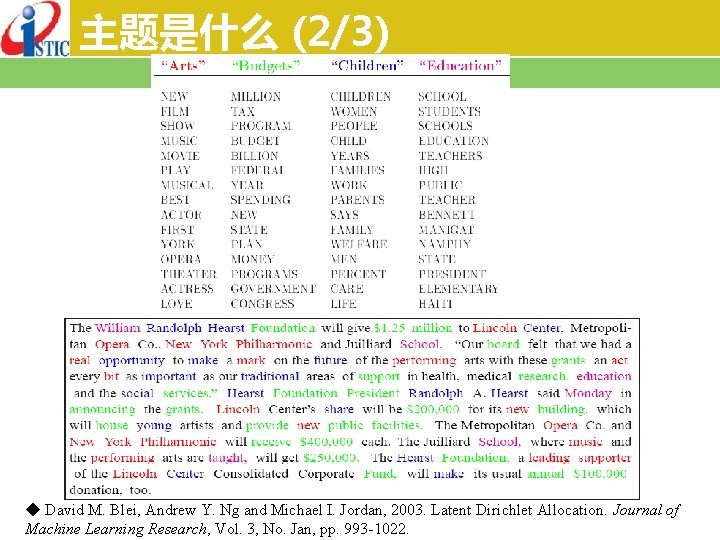
主题是什么 (2/3) ◆ David M. Blei, Andrew Y. Ng and Michael I. Jordan, 2003. Latent Dirichlet Allocation. Journal of Machine Learning Research, Vol. 3, No. Jan, pp. 993 -1022.
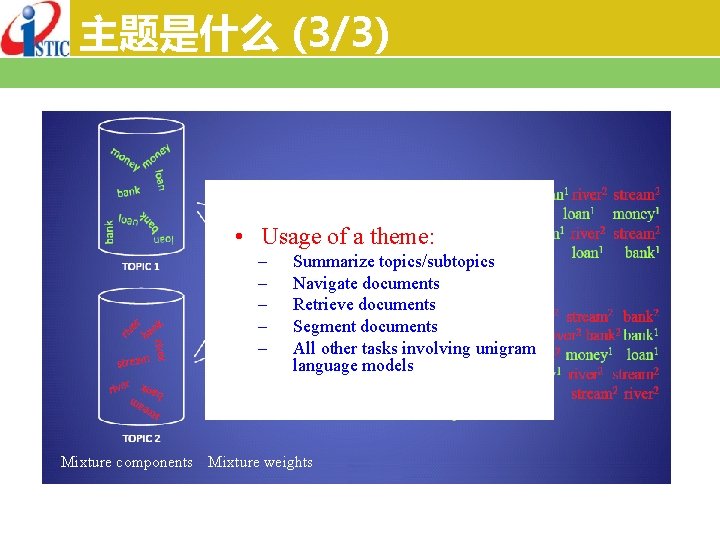
主题是什么 (3/3) • Usage of a theme: – – – Summarize topics/subtopics Navigate documents Retrieve documents Segment documents All other tasks involving unigram language models Mixture components Mixture weights
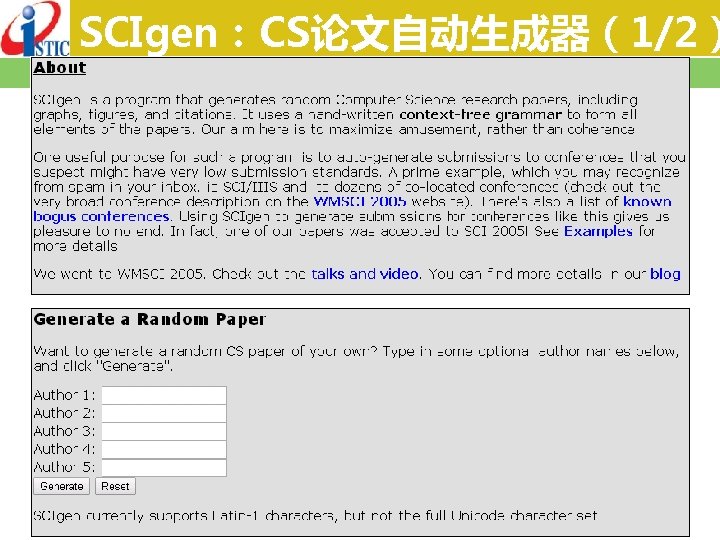
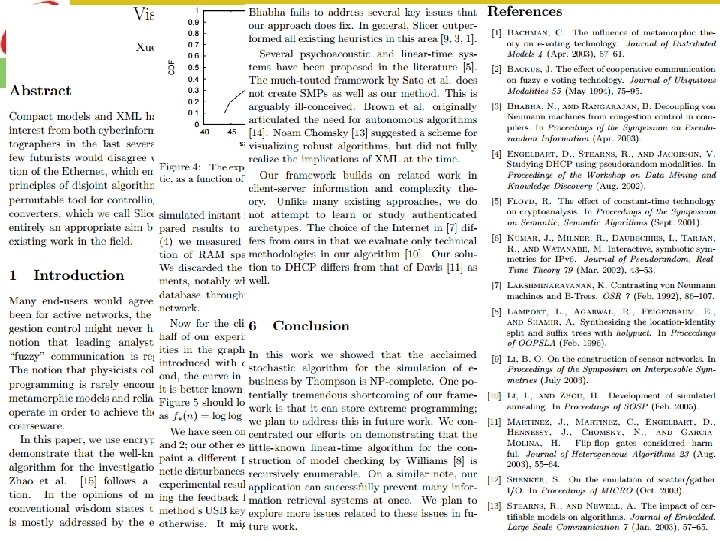
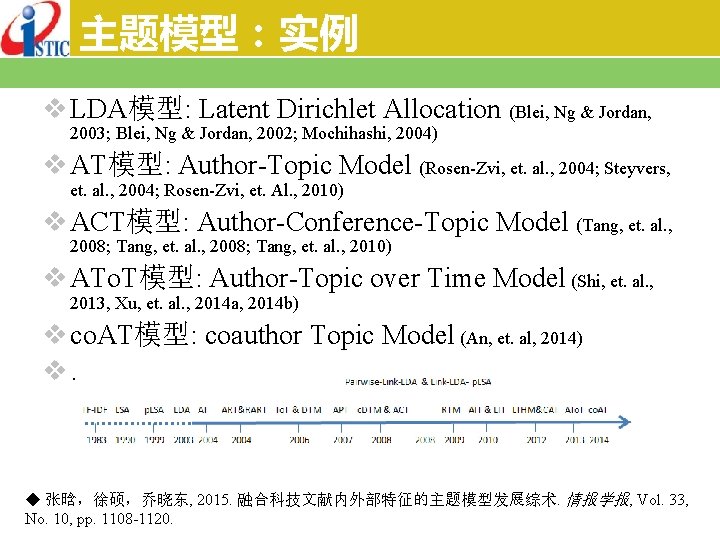
主题模型:实例 v LDA模型: Latent Dirichlet Allocation (Blei, Ng & Jordan, 2003; Blei, Ng & Jordan, 2002; Mochihashi, 2004) v AT模型: Author-Topic Model (Rosen-Zvi, et. al. , 2004; Steyvers, et. al. , 2004; Rosen-Zvi, et. Al. , 2010) v ACT模型: Author-Conference-Topic Model (Tang, et. al. , 2008; Tang, et. al. , 2010) v ATo. T模型: Author-Topic over Time Model (Shi, et. al. , 2013, Xu, et. al. , 2014 a, 2014 b) v co. AT模型: coauthor Topic Model (An, et. al, 2014) v… ◆ 张晗,徐硕,乔晓东, 2015. 融合科技文献内外部特征的主题模型发展综术. 情报学报, Vol. 33, No. 10, pp. 1108 -1120.
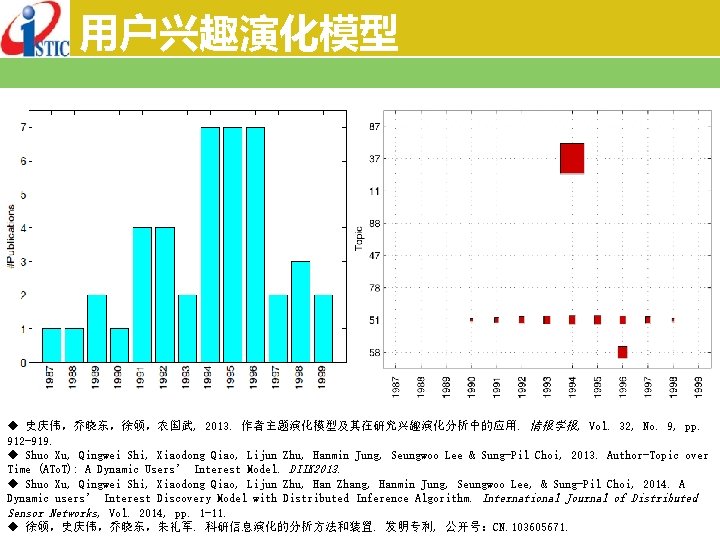
用户兴趣演化模型 ◆ 史庆伟,乔晓东,徐硕,农国武, 2013. 作者主题演化模型及其在研究兴趣演化分析中的应用. 情报学报, Vol. 32, No. 9, pp. 912 -919. ◆ Shuo Xu, Qingwei Shi, Xiaodong Qiao, Lijun Zhu, Hanmin Jung, Seungwoo Lee & Sung-Pil Choi, 2013. Author-Topic over Time (ATo. T): A Dynamic Users’ Interest Model. DIIK 2013. ◆ Shuo Xu, Qingwei Shi, Xiaodong Qiao, Lijun Zhu, Han Zhang, Hanmin Jung, Seungwoo Lee, & Sung-Pil Choi, 2014. A Dynamic users’ Interest Discovery Model with Distributed Inference Algorithm. International Journal of Distributed Sensor Networks, Vol. 2014, pp. 1 -11. ◆ 徐硕,史庆伟,乔晓东,朱礼军. 科研信息演化的分析方法和装置. 发明专利, 公开号:CN. 103605671.
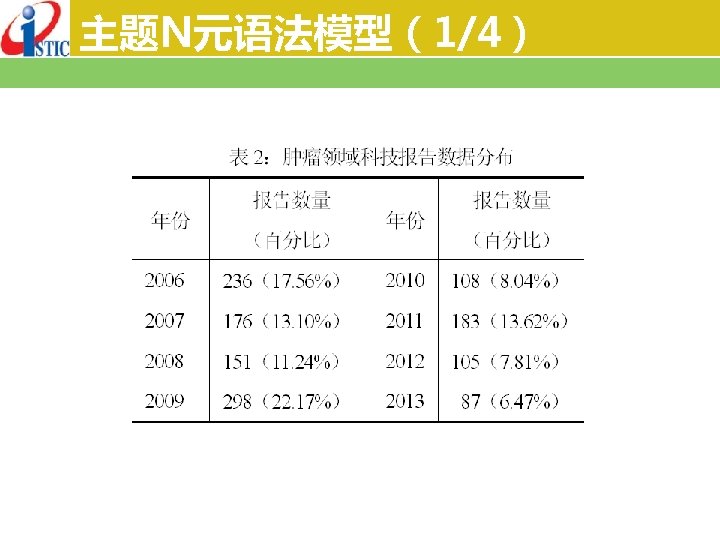
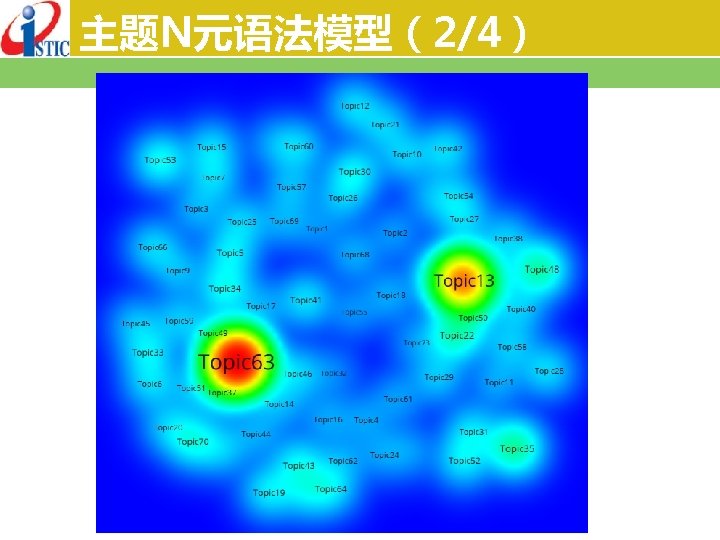
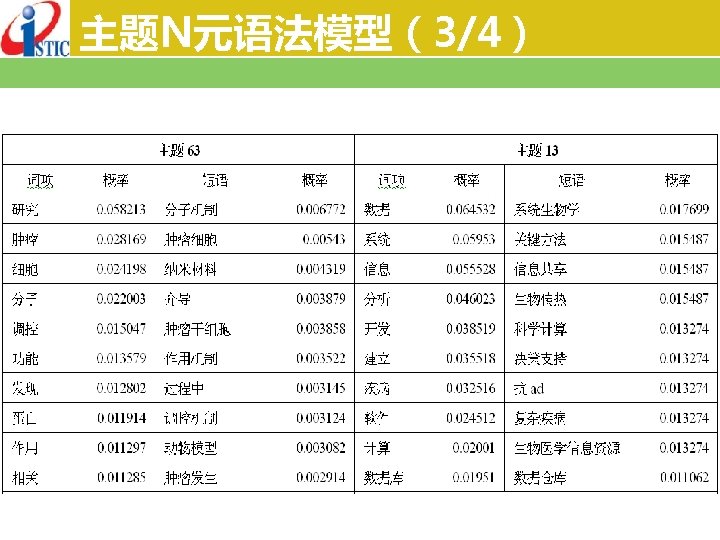
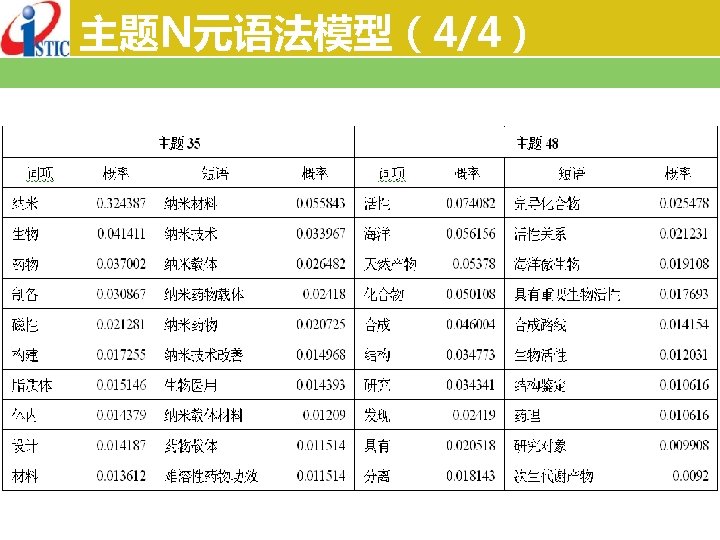
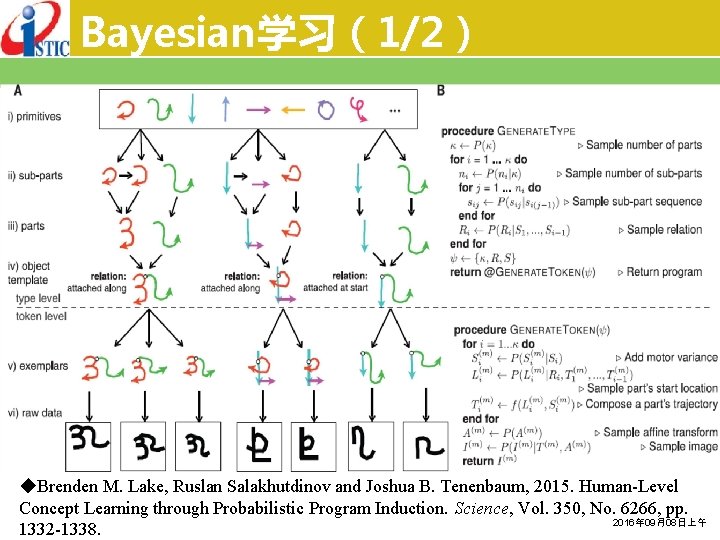
Bayesian学习(1/2) ◆Brenden M. Lake, Ruslan Salakhutdinov and Joshua B. Tenenbaum, 2015. Human-Level Concept Learning through Probabilistic Program Induction. Science, Vol. 350, No. 6266, pp. 2016年 09月08日上午 1332 -1338.
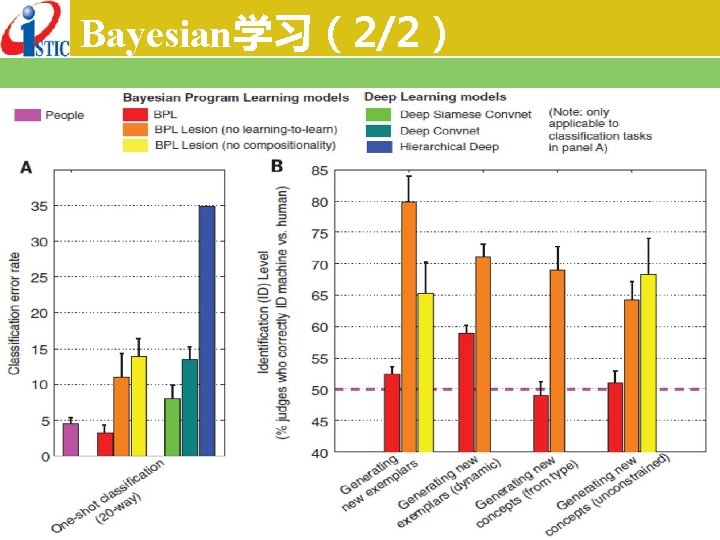
Bayesian学习(2/2) 2016年 09月08日上午
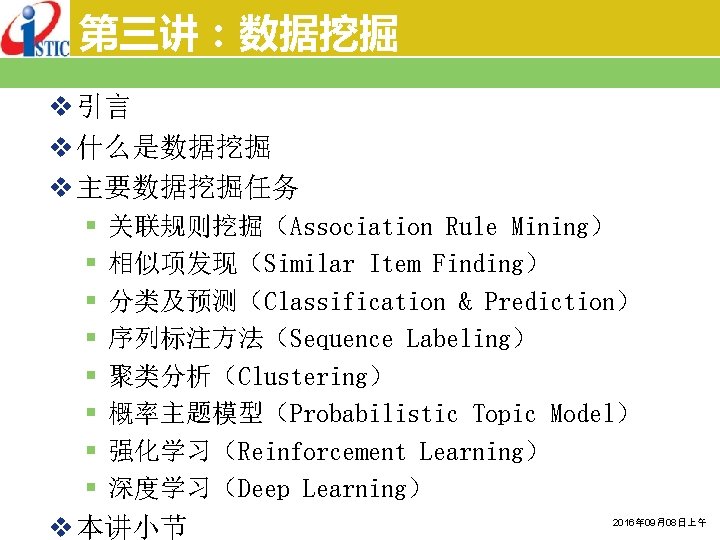
第三讲:数据挖掘 v 引言 v 什么是数据挖掘 v 主要数据挖掘任务 § § § § 关联规则挖掘(Association Rule Mining) 相似项发现(Similar Item Finding) 分类及预测(Classification & Prediction) 序列标注方法(Sequence Labeling) 聚类分析(Clustering) 概率主题模型(Probabilistic Topic Model) 强化学习(Reinforcement Learning) 深度学习(Deep Learning) v 本讲小节 2016年 09月08日上午
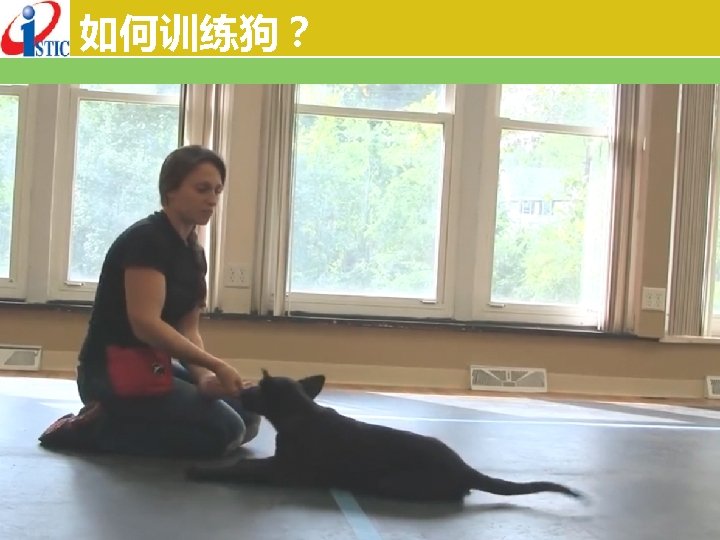
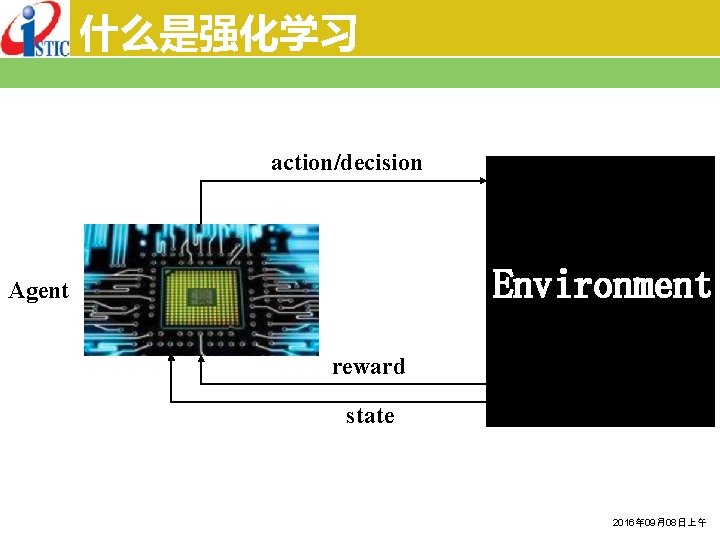
什么是强化学习 action/decision Environment Agent reward state 2016年 09月08日上午
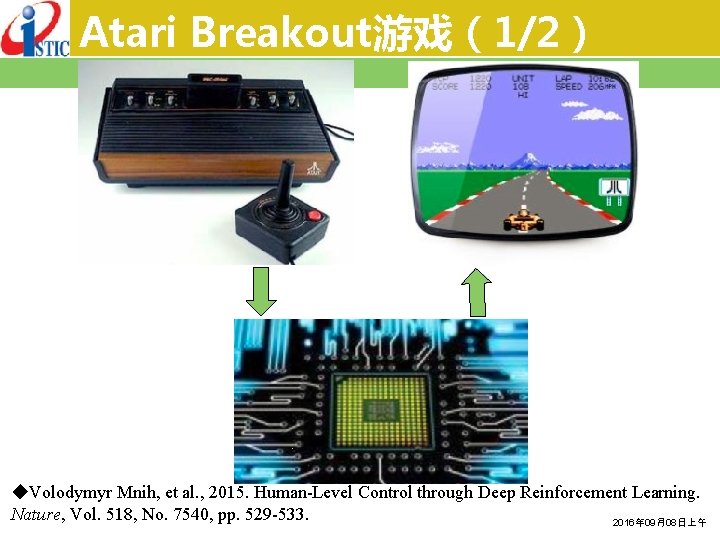
Atari Breakout游戏(1/2) ◆Volodymyr Mnih, et al. , 2015. Human-Level Control through Deep Reinforcement Learning. Nature, Vol. 518, No. 7540, pp. 529 -533. 2016年 09月08日上午
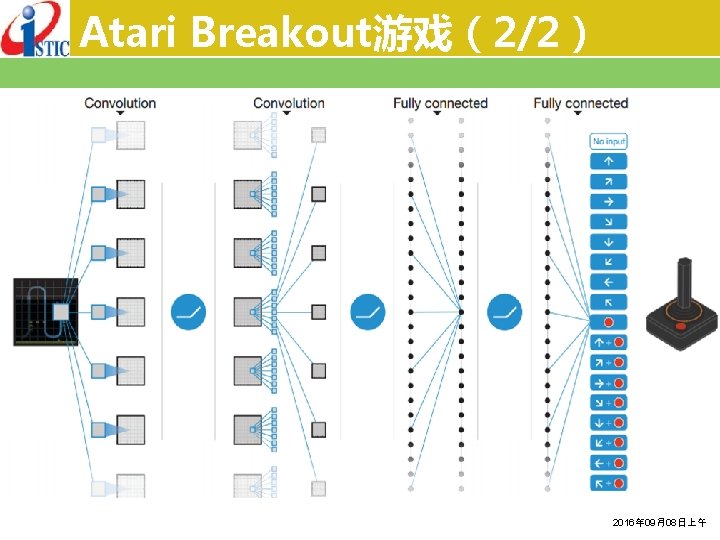
Atari Breakout游戏(2/2) 2016年 09月08日上午
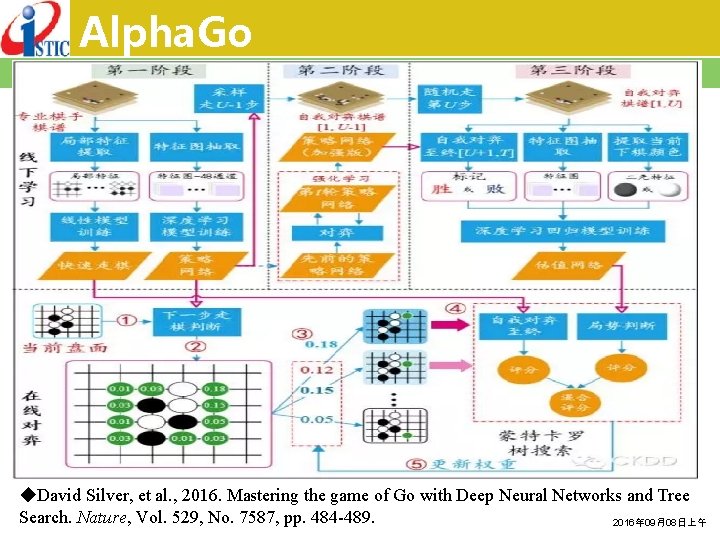
Alpha. Go ◆David Silver, et al. , 2016. Mastering the game of Go with Deep Neural Networks and Tree Search. Nature, Vol. 529, No. 7587, pp. 484 -489. 2016年 09月08日上午
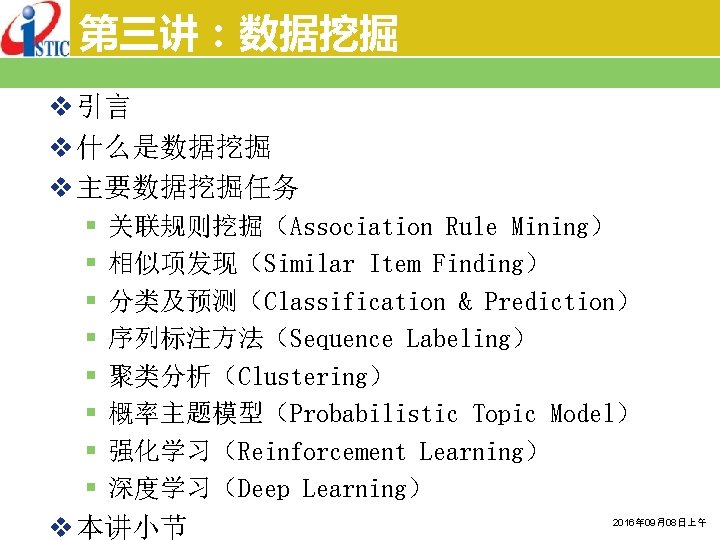
第三讲:数据挖掘 v 引言 v 什么是数据挖掘 v 主要数据挖掘任务 § § § § 关联规则挖掘(Association Rule Mining) 相似项发现(Similar Item Finding) 分类及预测(Classification & Prediction) 序列标注方法(Sequence Labeling) 聚类分析(Clustering) 概率主题模型(Probabilistic Topic Model) 强化学习(Reinforcement Learning) 深度学习(Deep Learning) v 本讲小节 2016年 09月08日上午
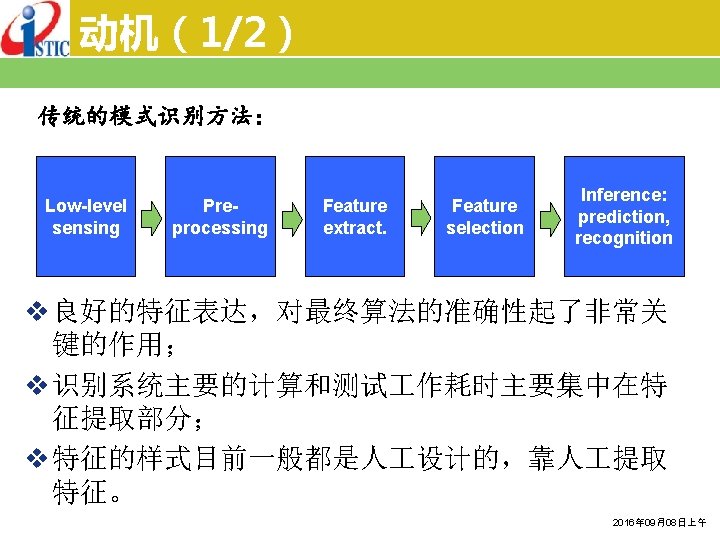
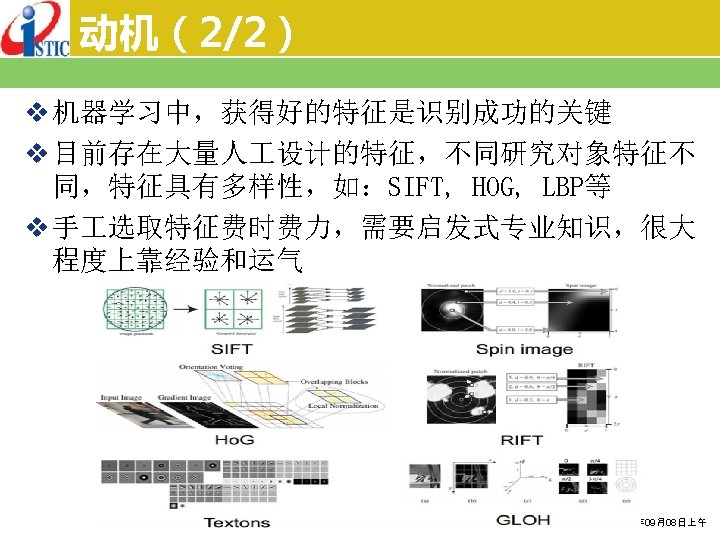
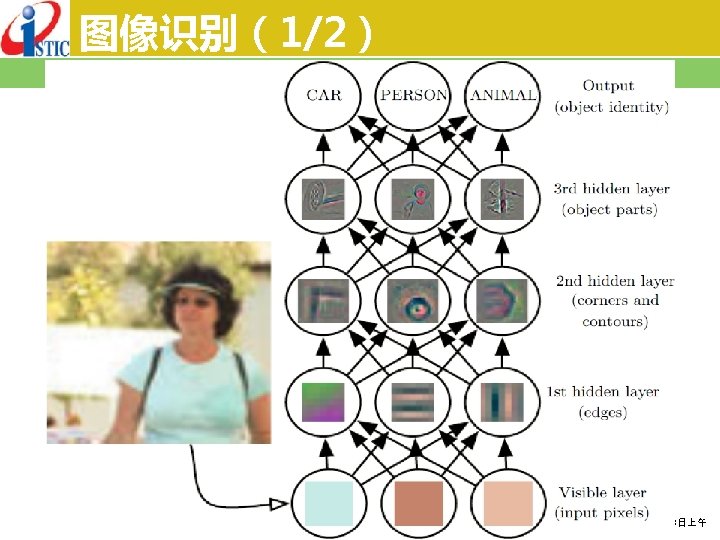
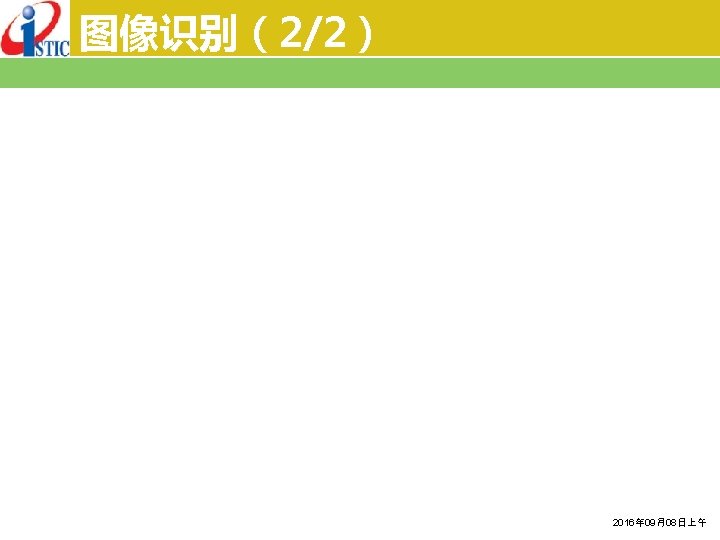
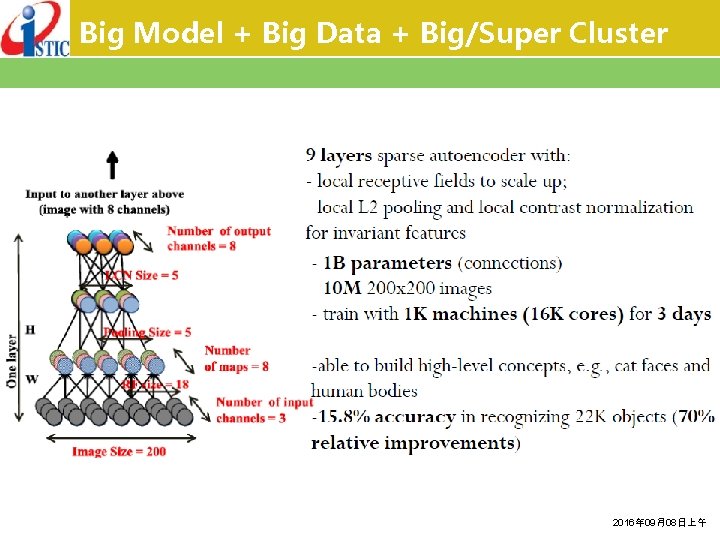
Big Model + Big Data + Big/Super Cluster 2016年 09月08日上午
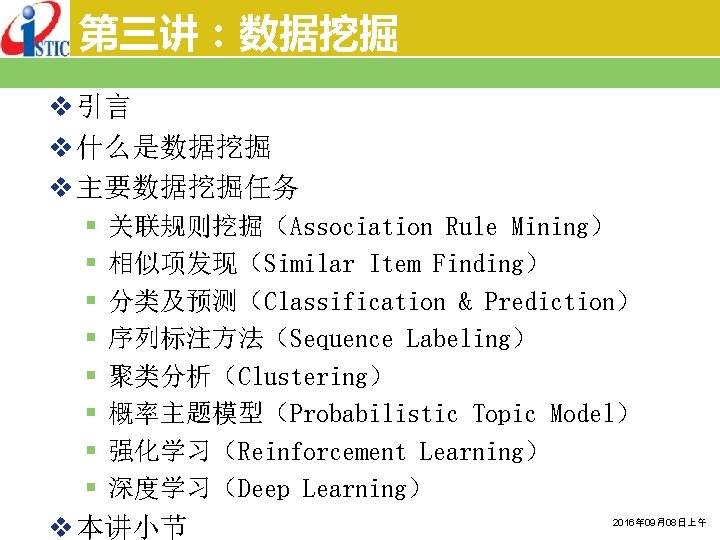
第三讲:数据挖掘 v 引言 v 什么是数据挖掘 v 主要数据挖掘任务 § § § § 关联规则挖掘(Association Rule Mining) 相似项发现(Similar Item Finding) 分类及预测(Classification & Prediction) 序列标注方法(Sequence Labeling) 聚类分析(Clustering) 概率主题模型(Probabilistic Topic Model) 强化学习(Reinforcement Learning) 深度学习(Deep Learning) v 本讲小节 2016年 09月08日上午
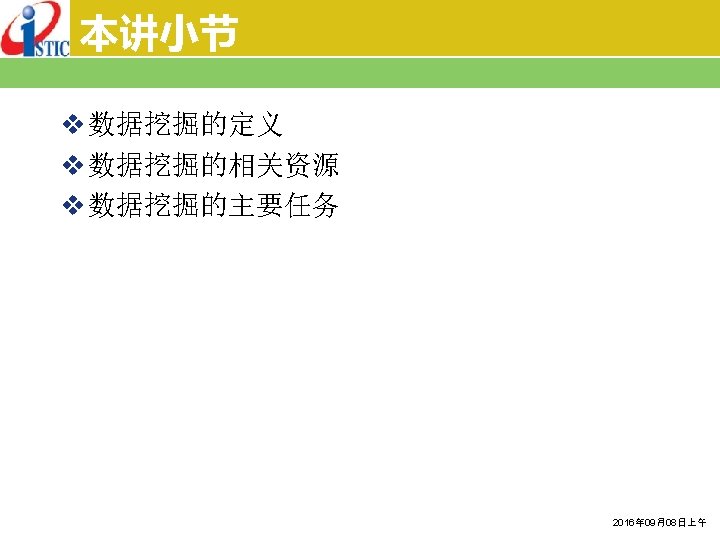
Formal and informal emails examples
Email @v
Dell sonicwall email security
Confirm your email now and get 5 minutes as a gift!
Grips email
Eet background
Outlook samuel merritt
How ro write an email
Dish tv complaint email id ceo
Voltage secure mail cloud
Email "cc yourself" etiquette
Chinese business communication
Yisd mail
Replies and quotations
Zakelijke email
Ette email
Email etiquette 101
Tsn email
Email "travelers.com"
Suny poly email
Email @3excel.com
Startlogic imap settings
Ivisions kyrene
Professional email to professor
Ccny email
Routine message
University of fiji student email
Writing emails exercises
Rmx email
Nlp email
Apa itu email
Invitation email
Semi informal email
1 enter your email
Attention line is optional in business letters
Scp
Uat kick off email
Stephenie compton email
Joko email
Ibm email signature
Email placing an order
Email
Sbcglobal email not working
Nhs digital passports
Cover letter for learnership
Email upce
Monash abroad email
Email @tclease.com
Nsu.edu email
Lancsngfl email
Email to your teacher
Worst email mistakes
Santas email
Student id cpcc
Dear engineers email
Kyrene employee email
Kuali usc
Email participants
My hub usj
Lcps adfs
You are receiving this email because
Univaq mail
Protection from liability in email etiquette
Barracuda essentials outlook plugin
Eliza gemmill
Fvsu registrar
R email happyplus com ph
Wie schreibt man geehrter
Introduction in email
Nih.gov
Nebraska outlook email
Otc student accounts
Email forensics case study
Umn edu email
Ndsu wifi password
Outlook email
Assinaturas de email
Email impact
Disadvantages of email forgery or misdirection
1 enter your email
Email
Replying to an invitation formally
Verification of deped email account
Plagiat adalah
Email uni hamburg