RNASeq Xiaole Shirley Liu STAT 115 STAT 215
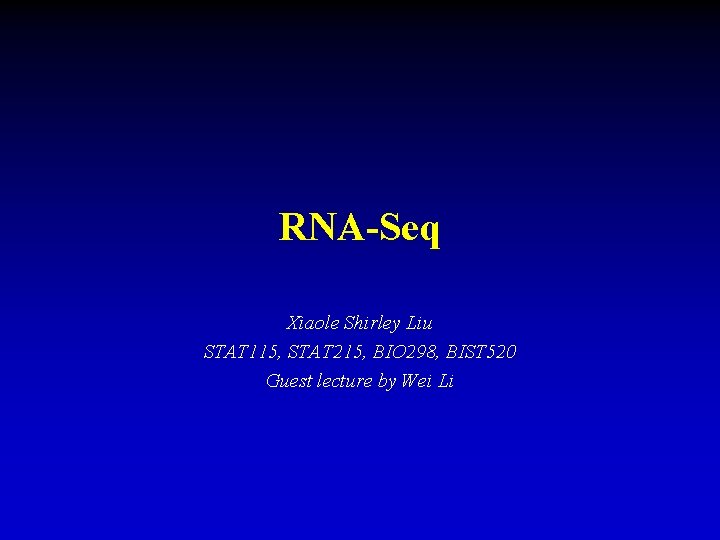
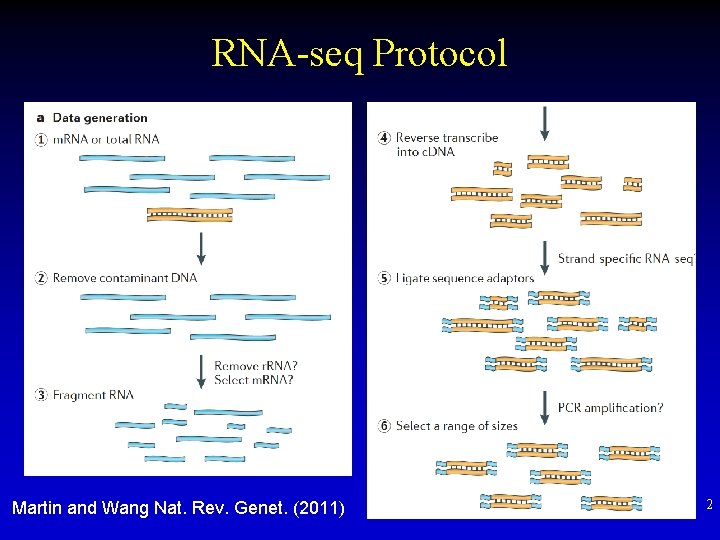
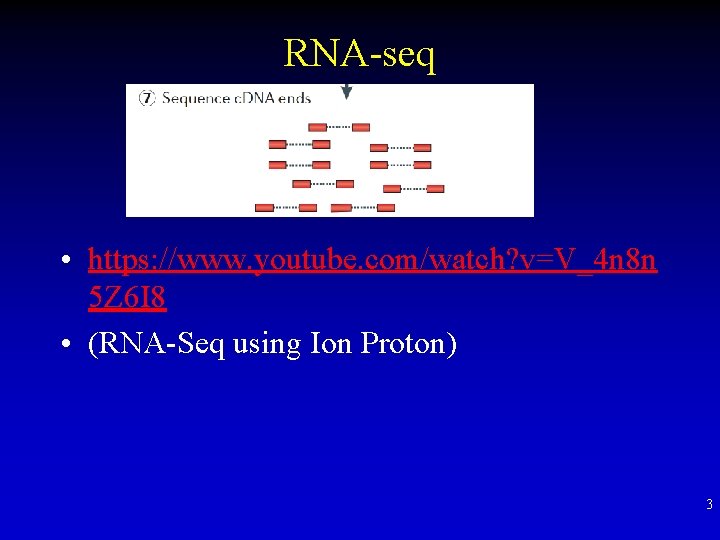
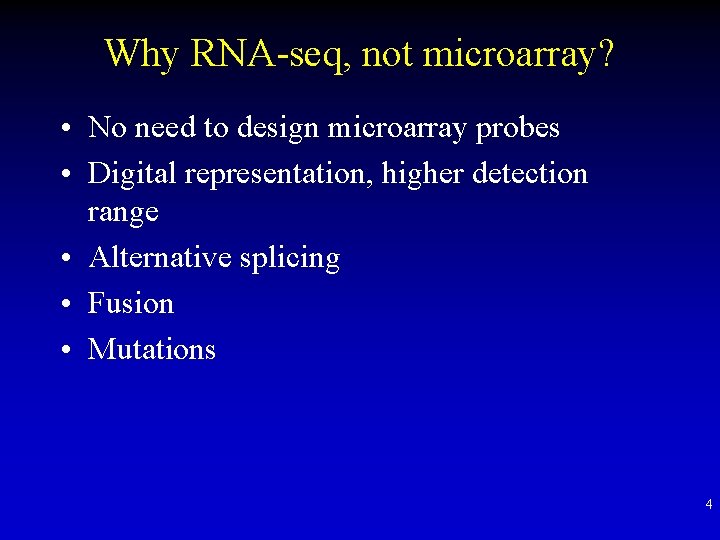
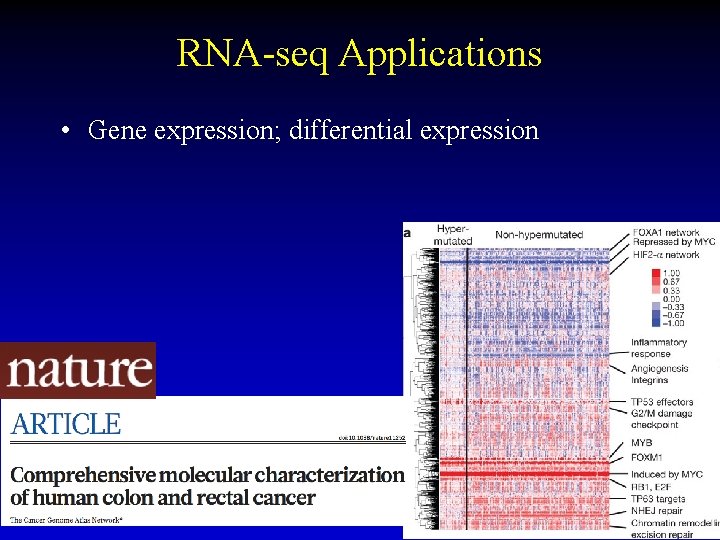
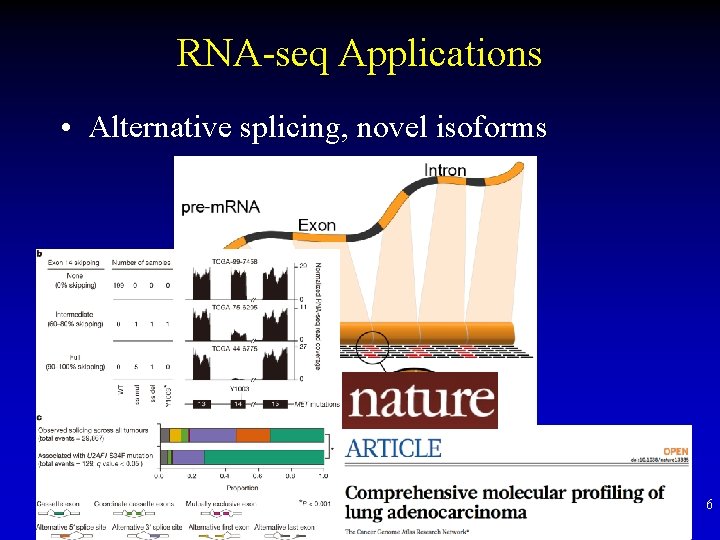
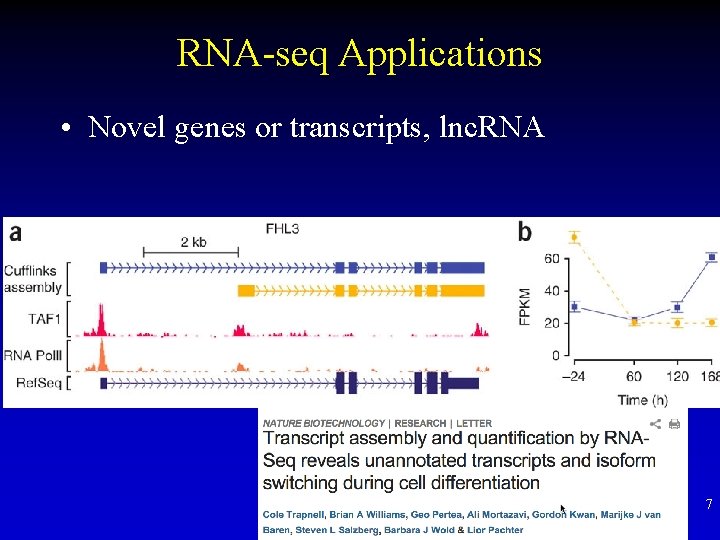
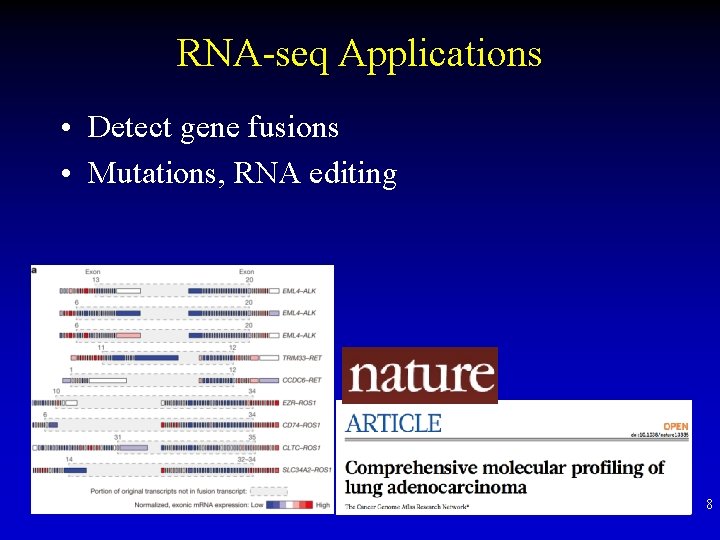
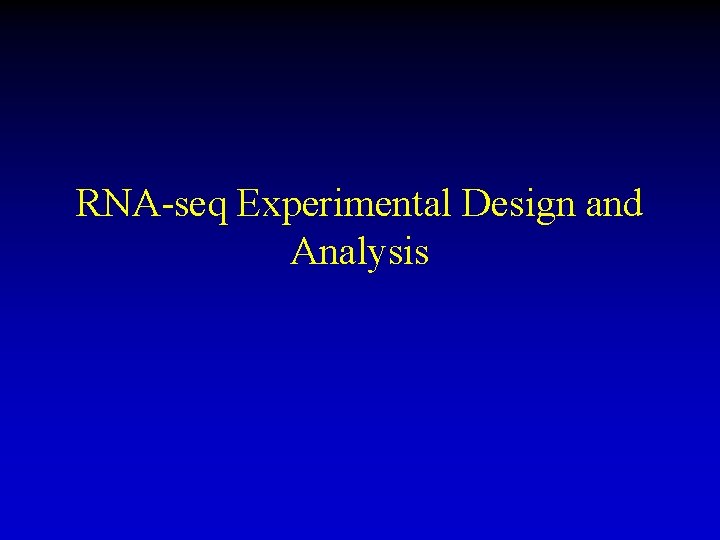
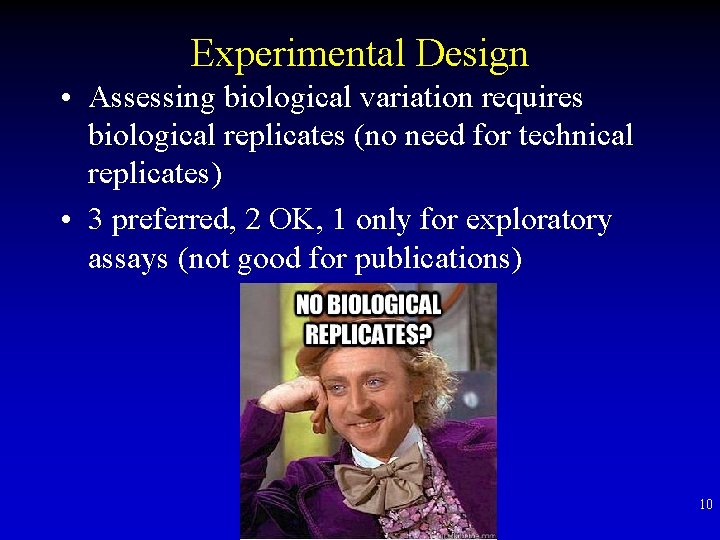
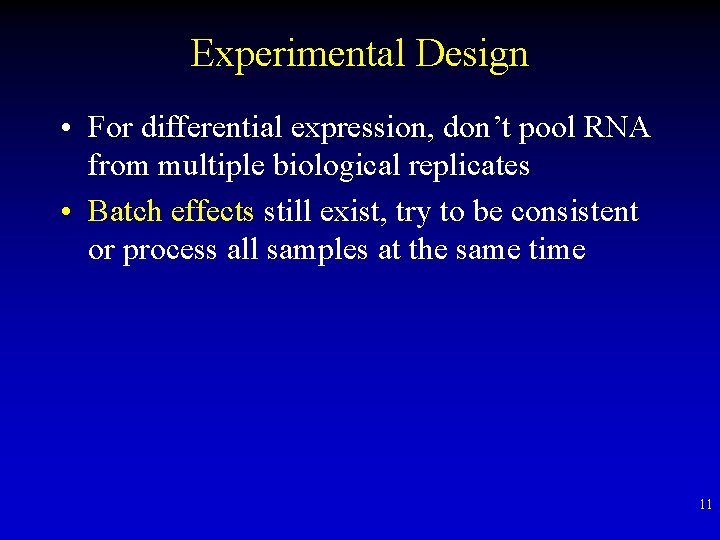
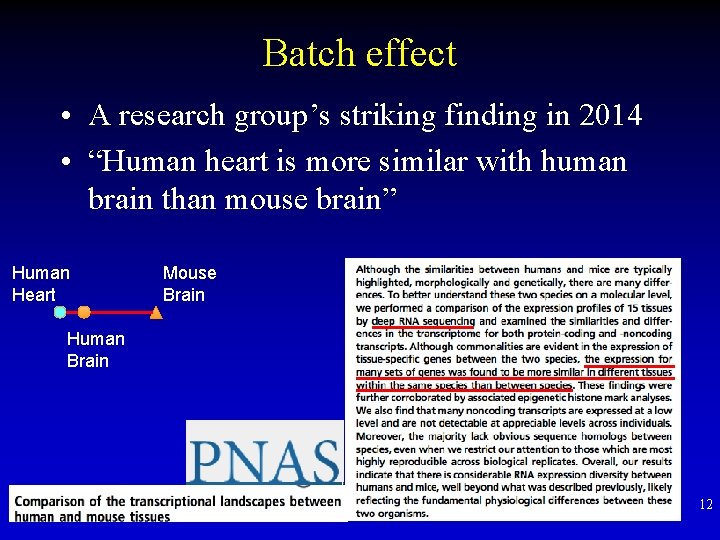
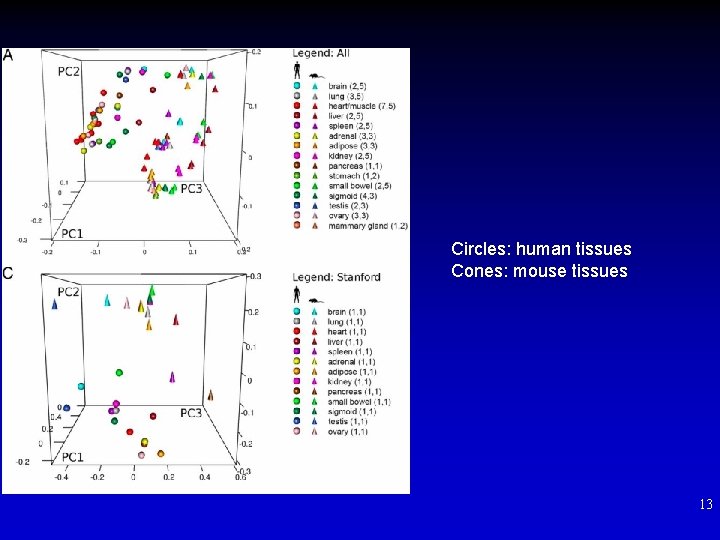
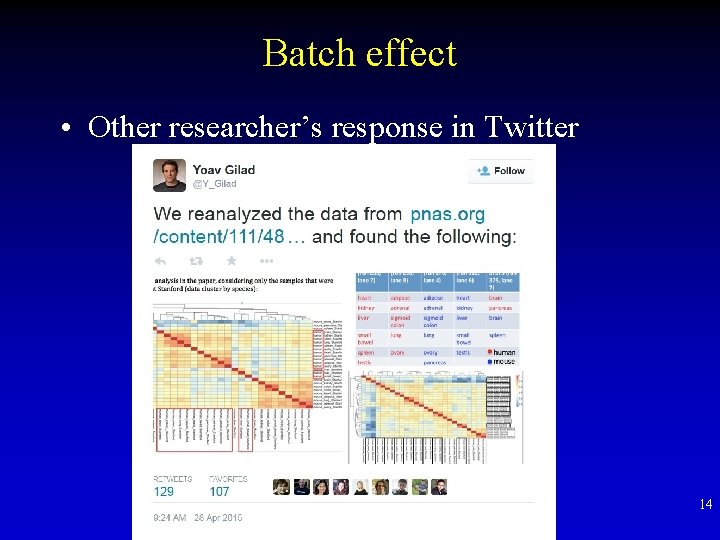
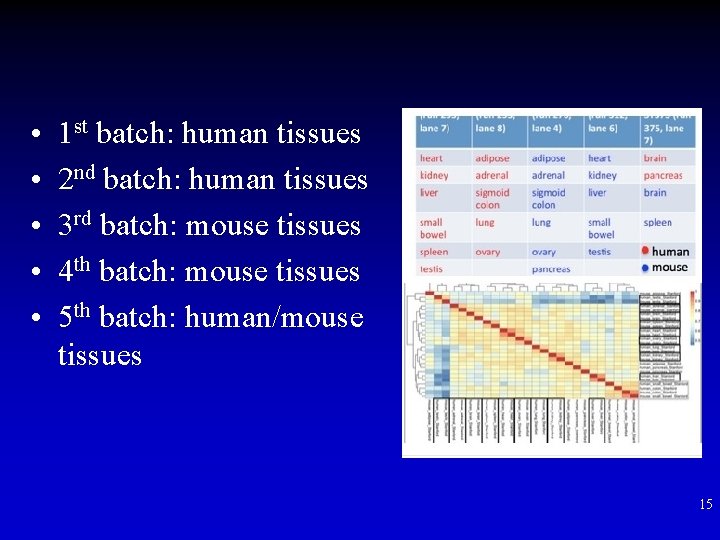
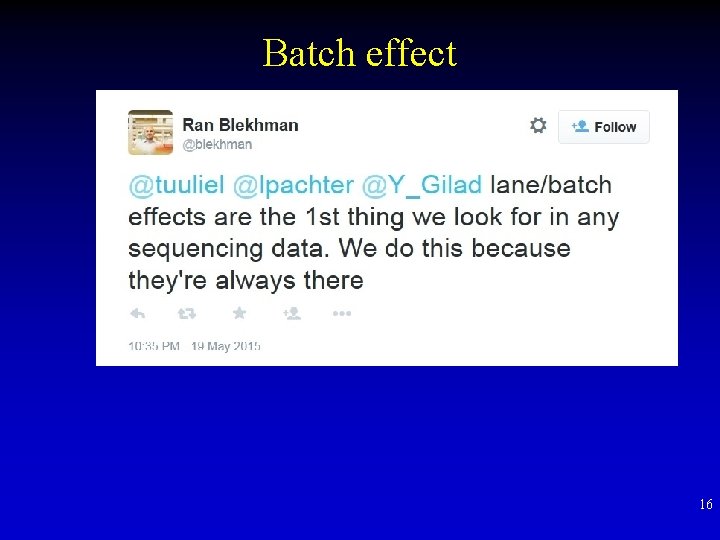
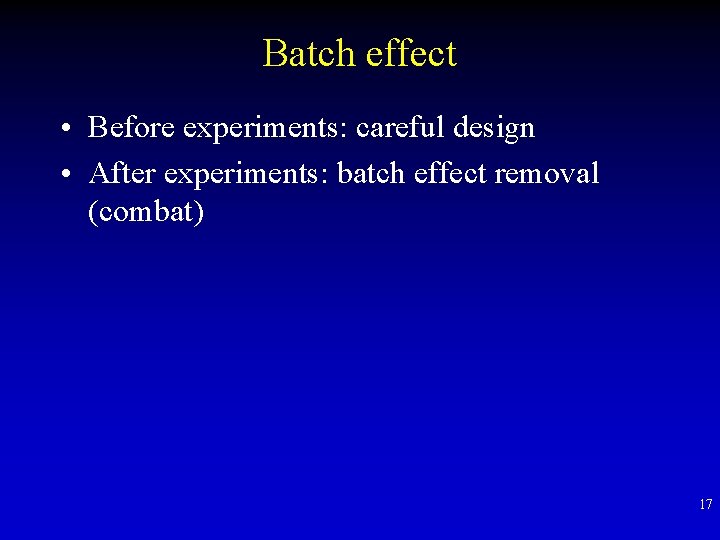
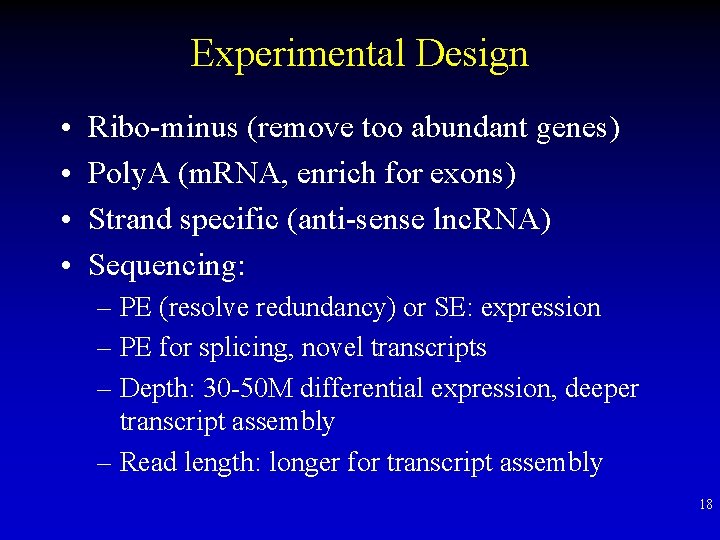
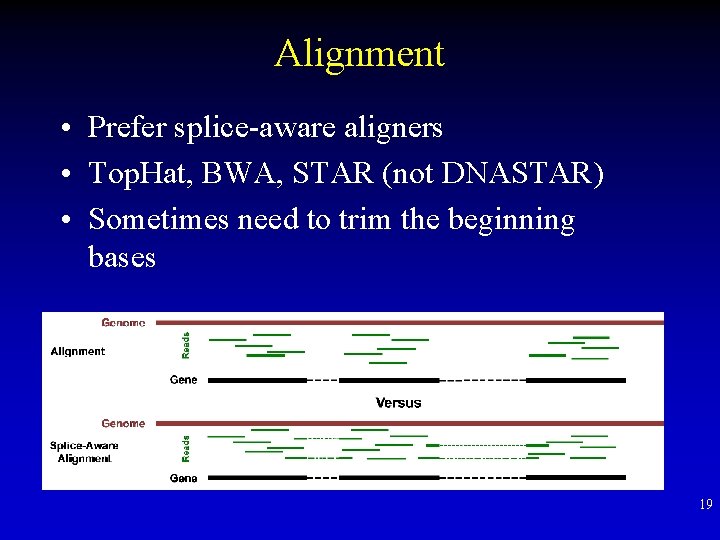
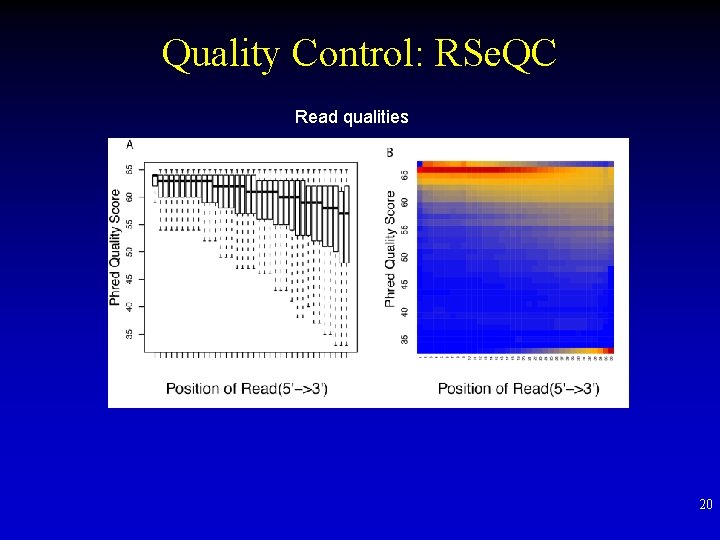
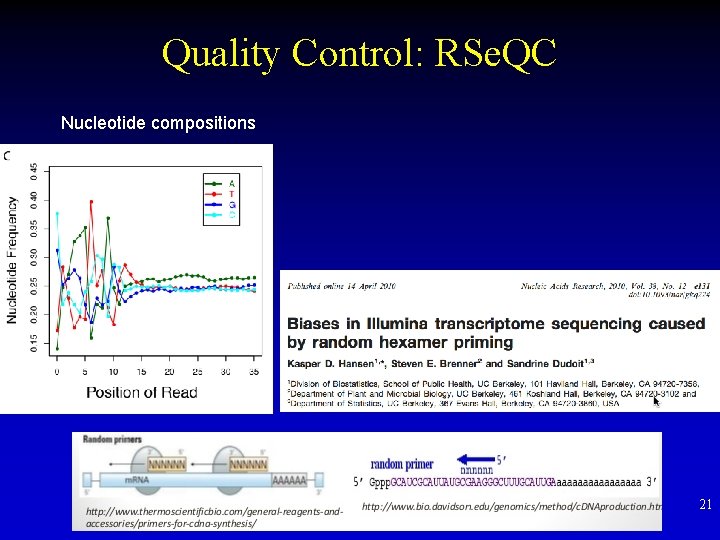
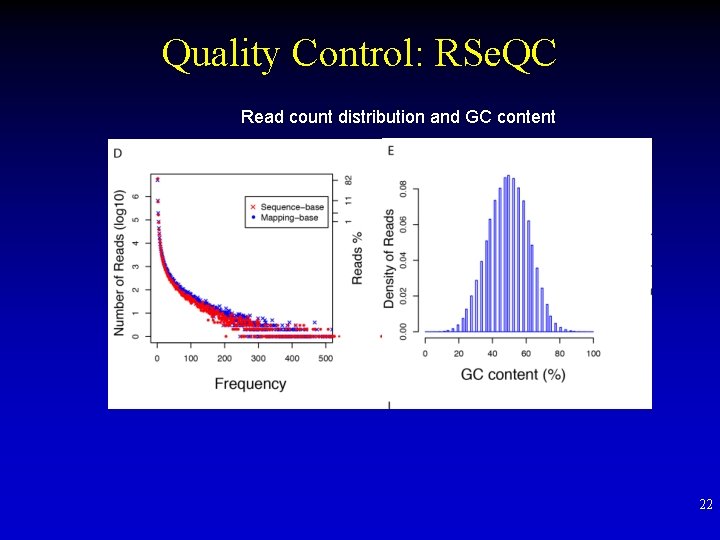
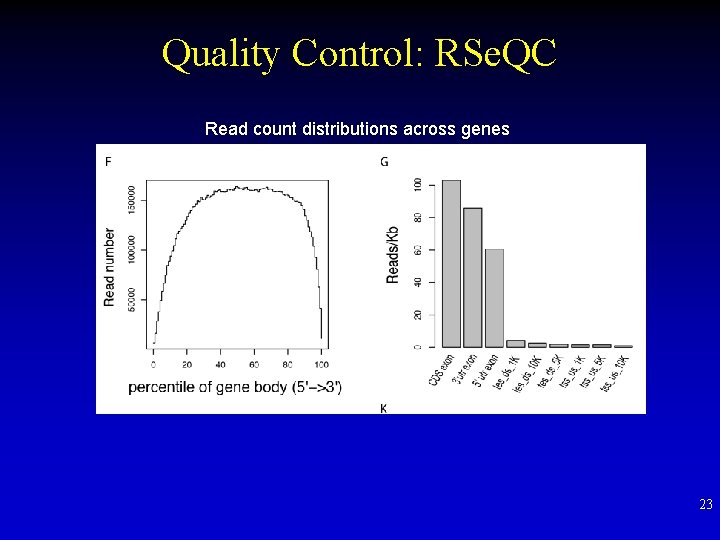
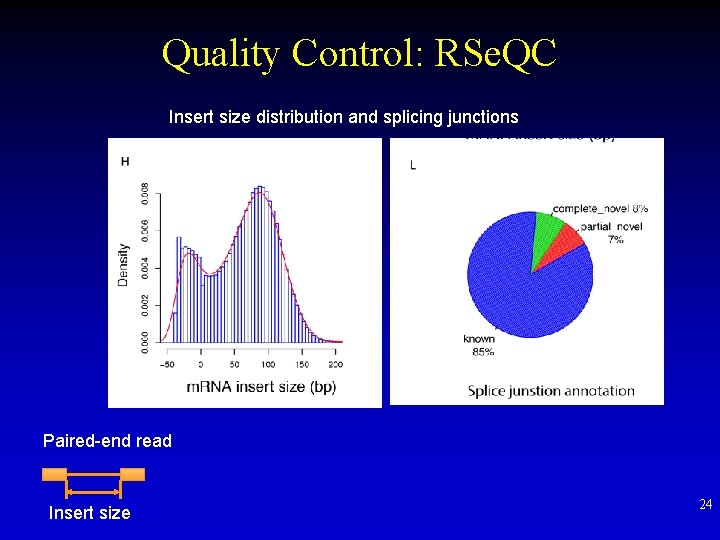
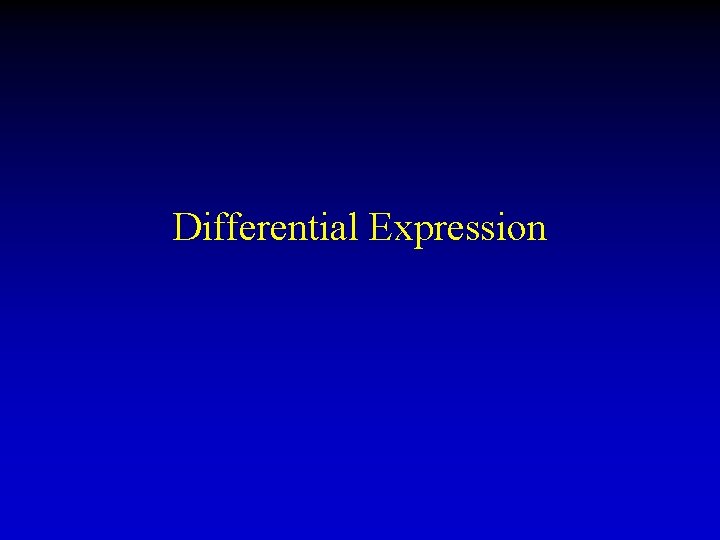
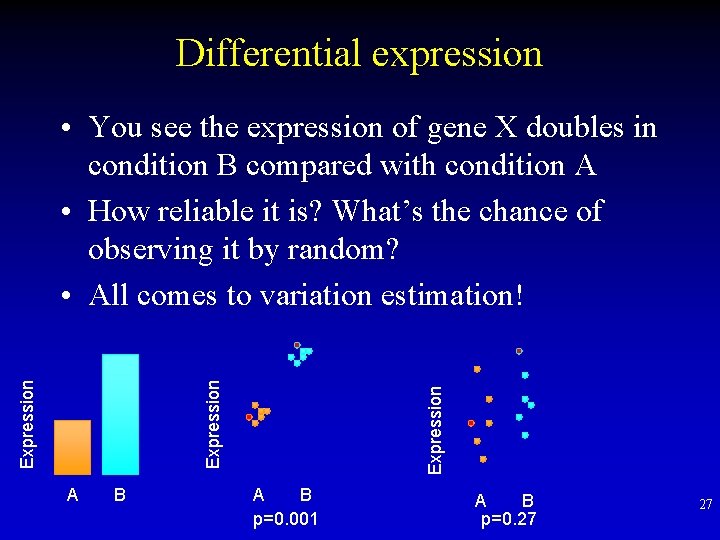
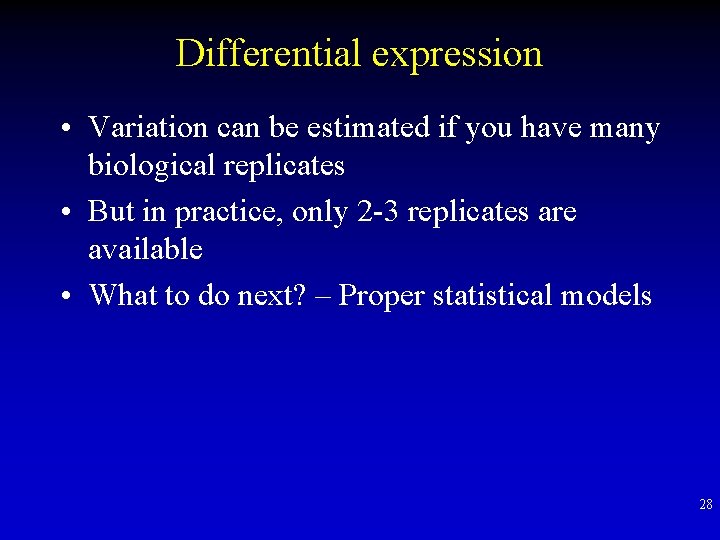
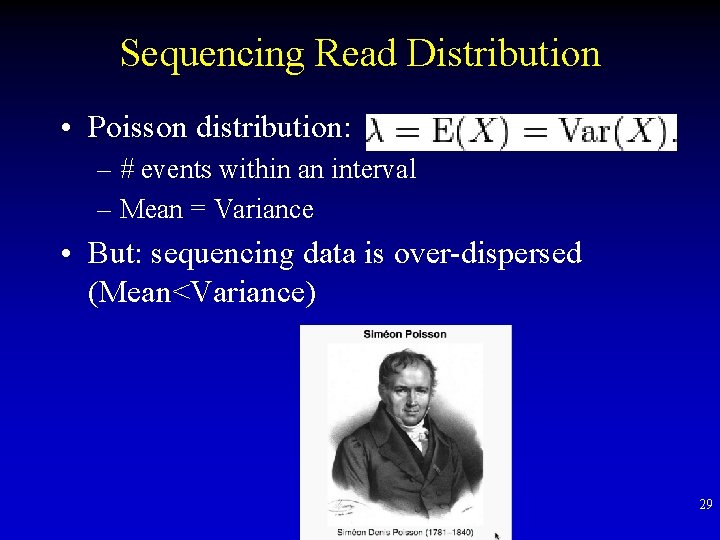
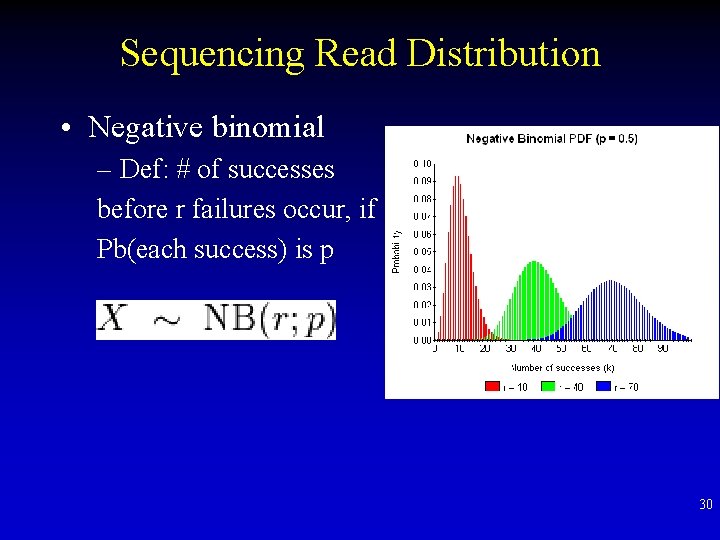
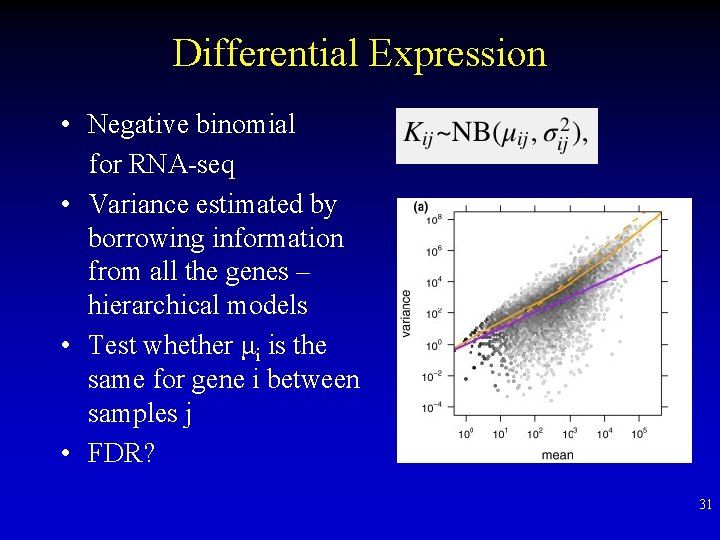
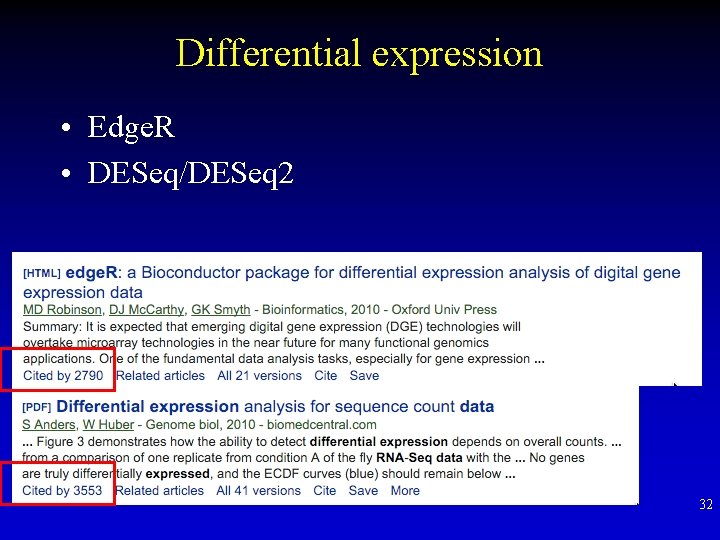
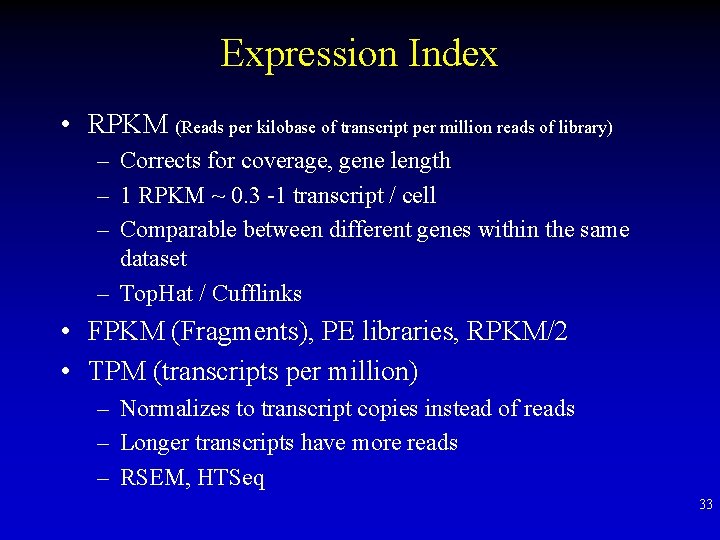
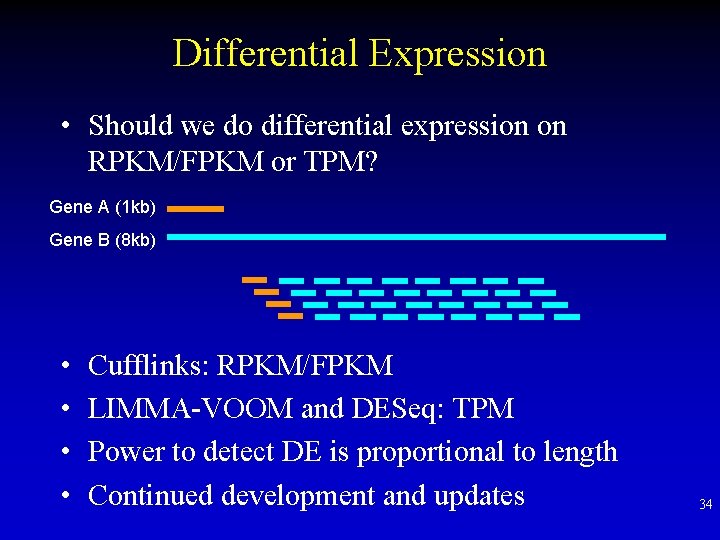
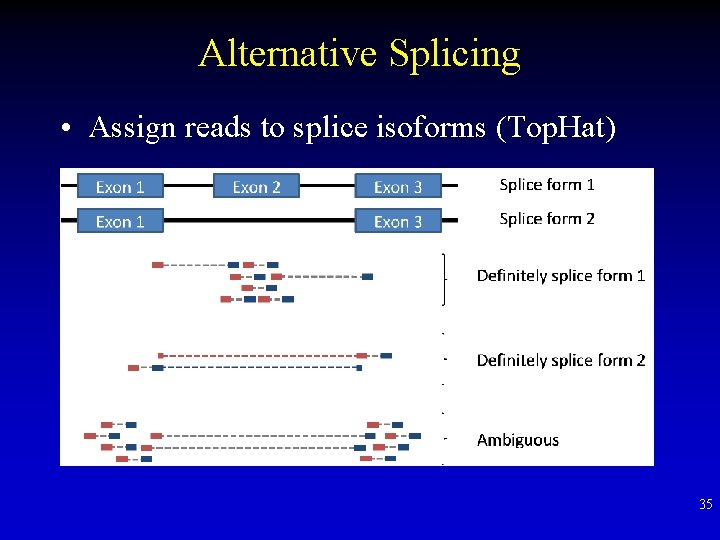
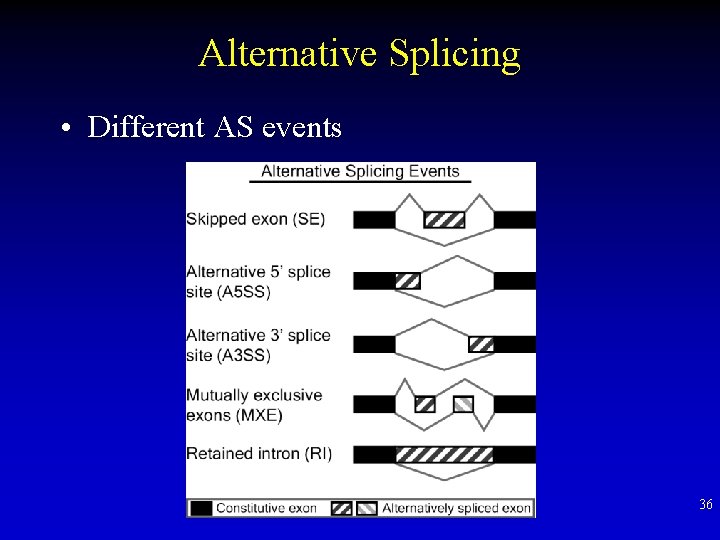
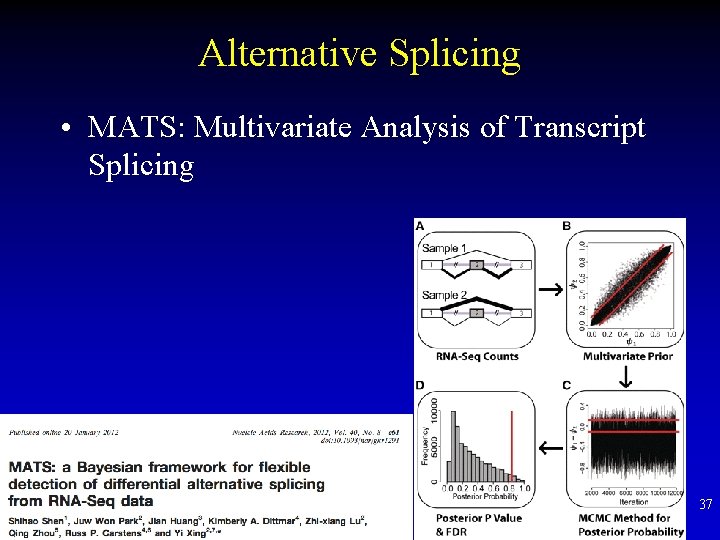
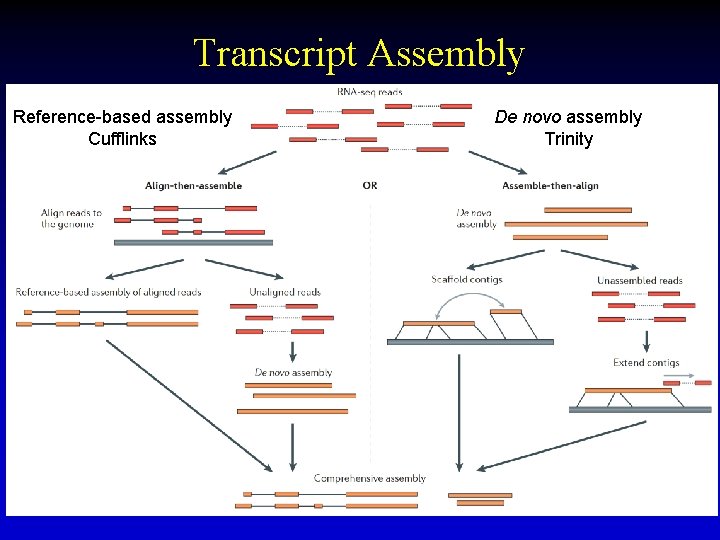
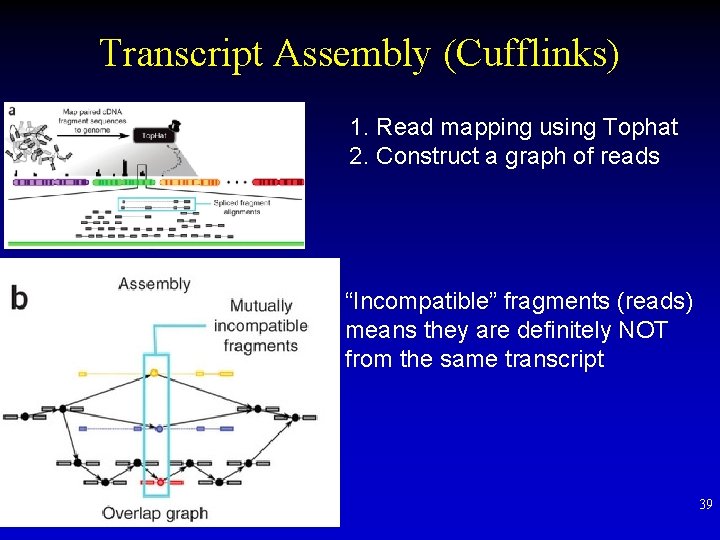
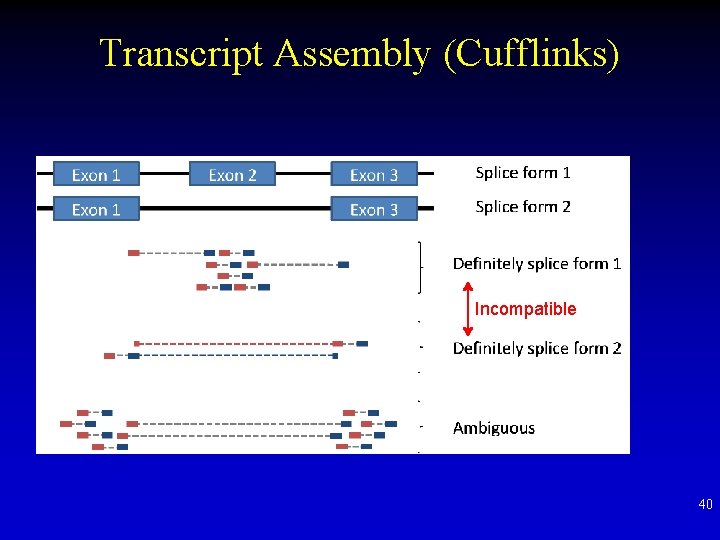
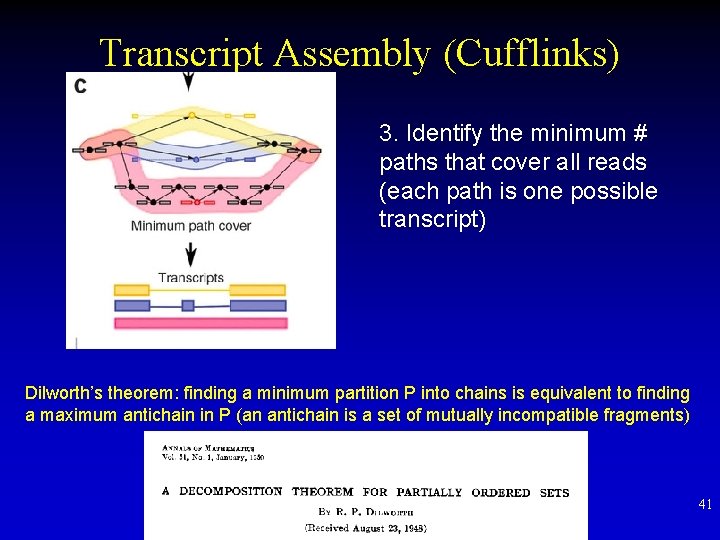
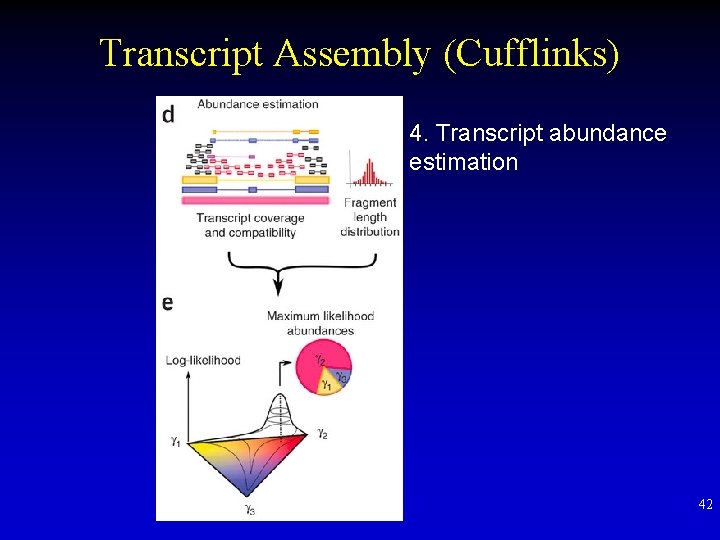
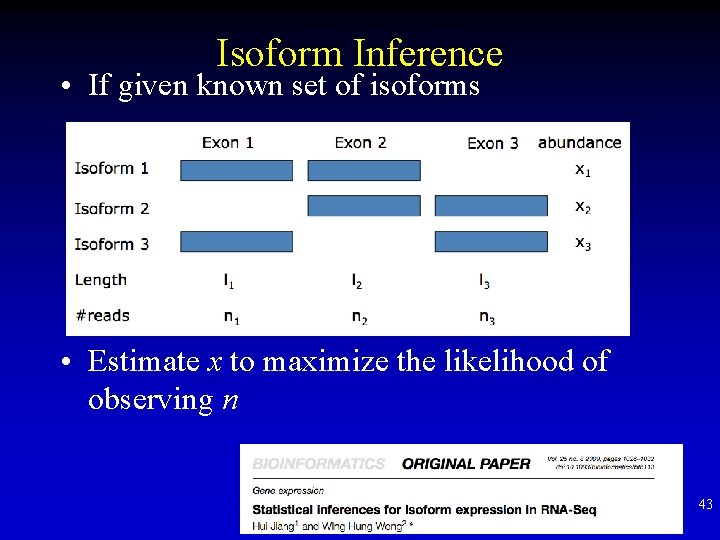
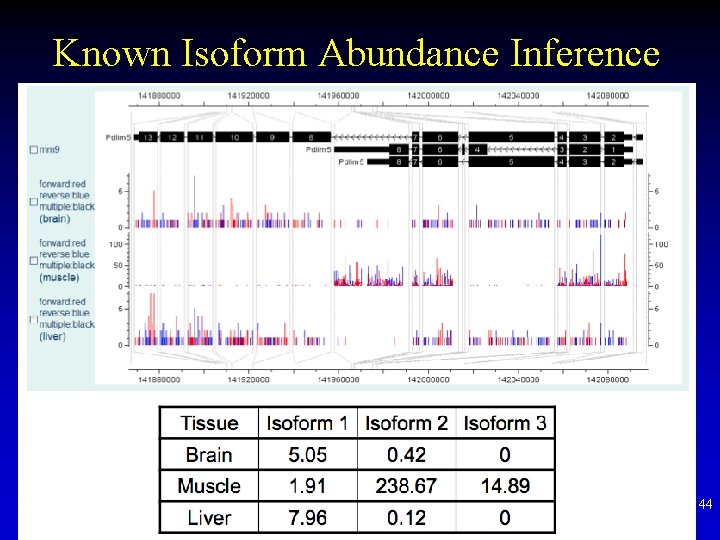
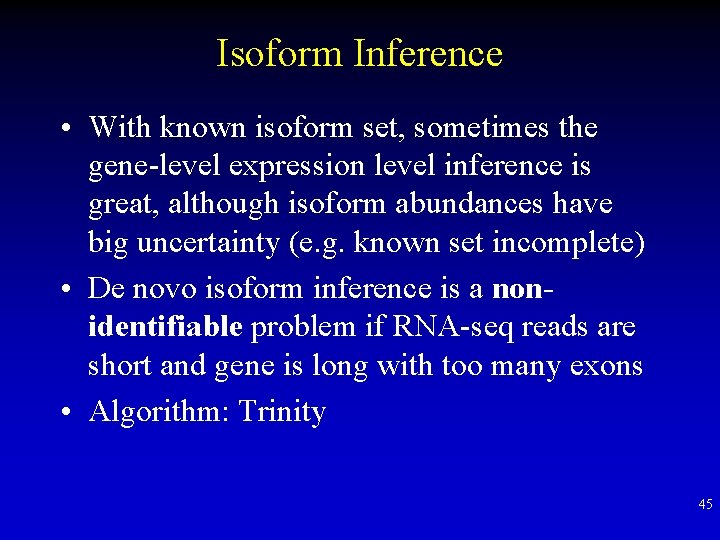
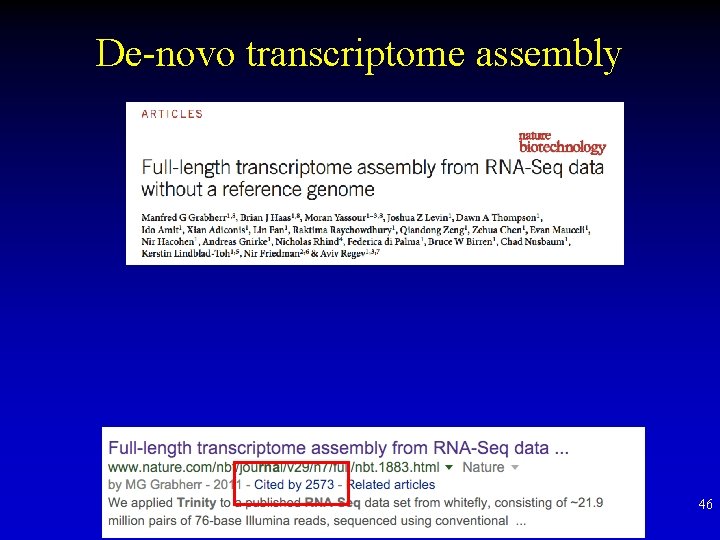
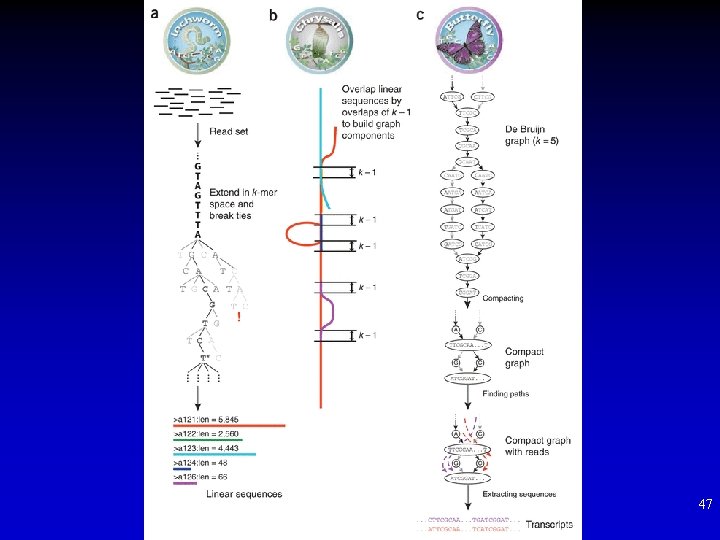
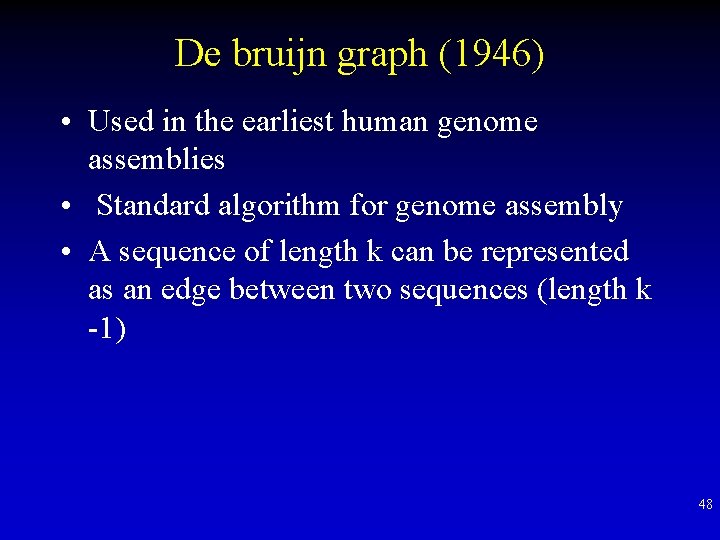
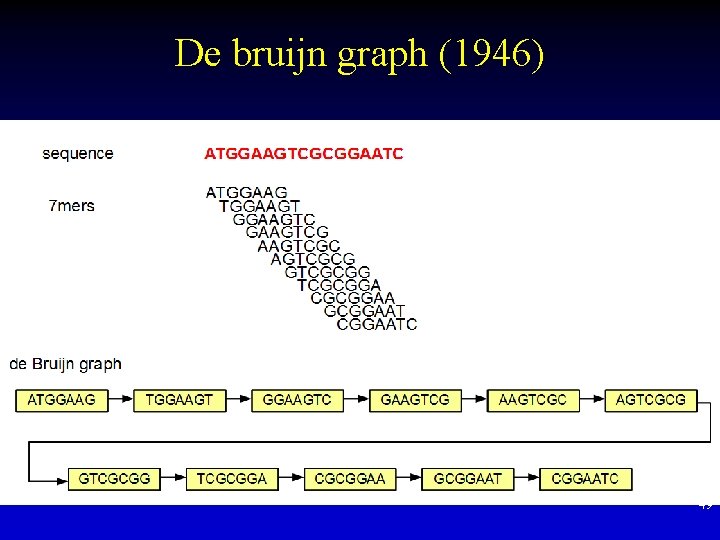
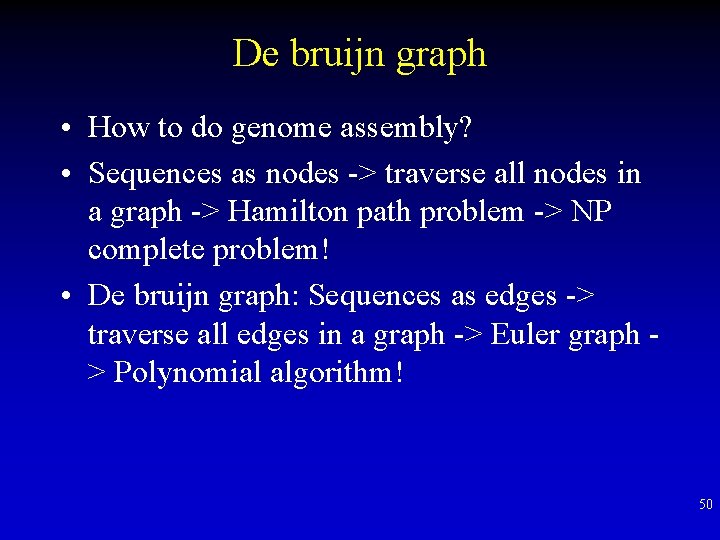
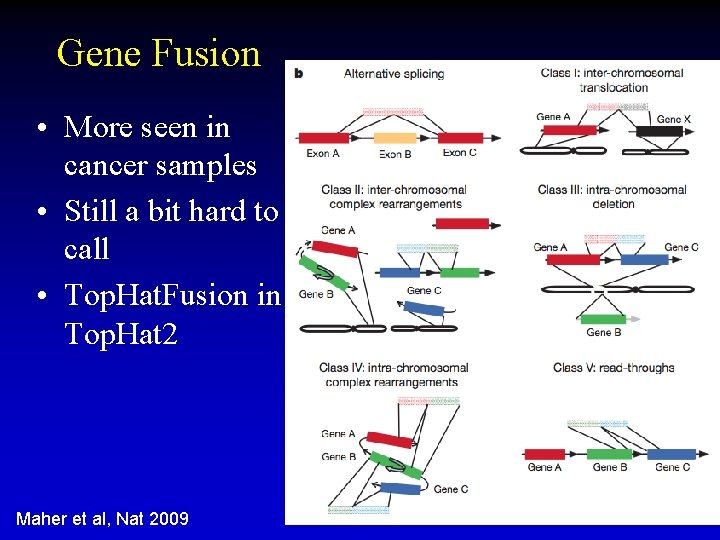
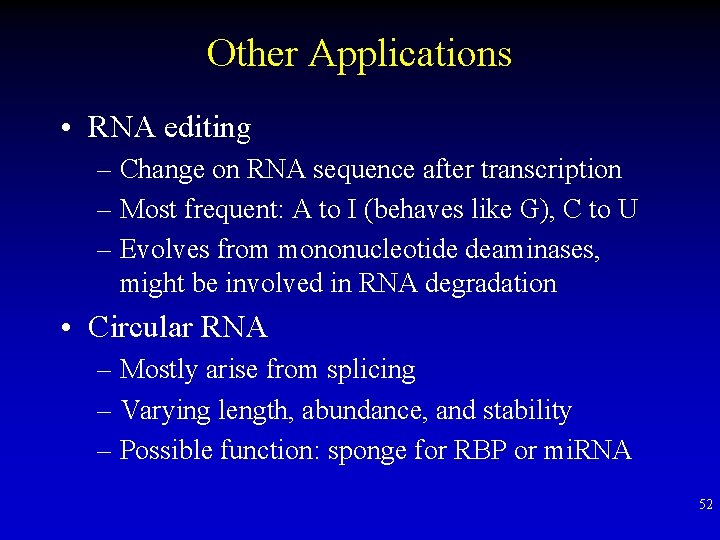
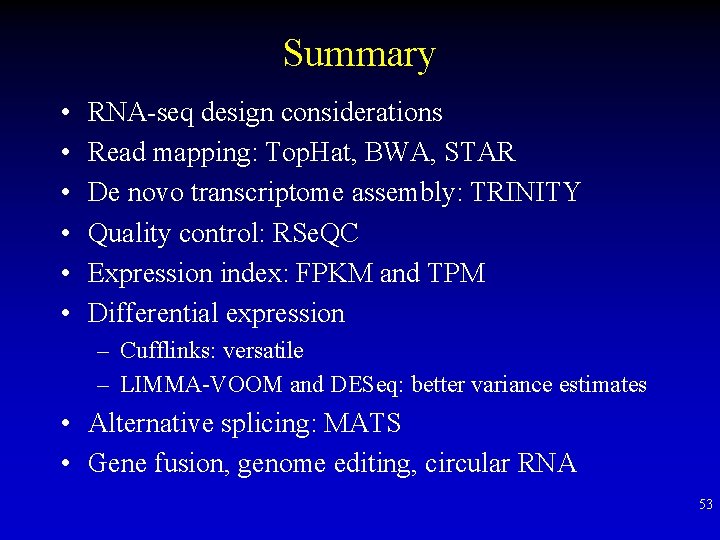
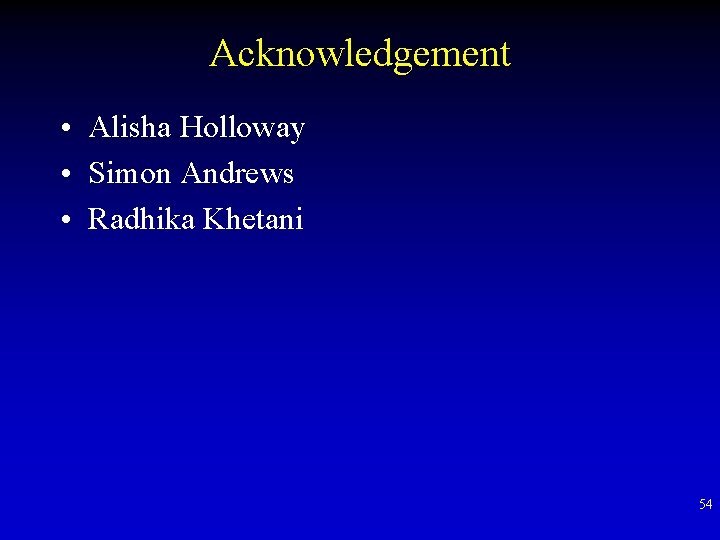
- Slides: 53
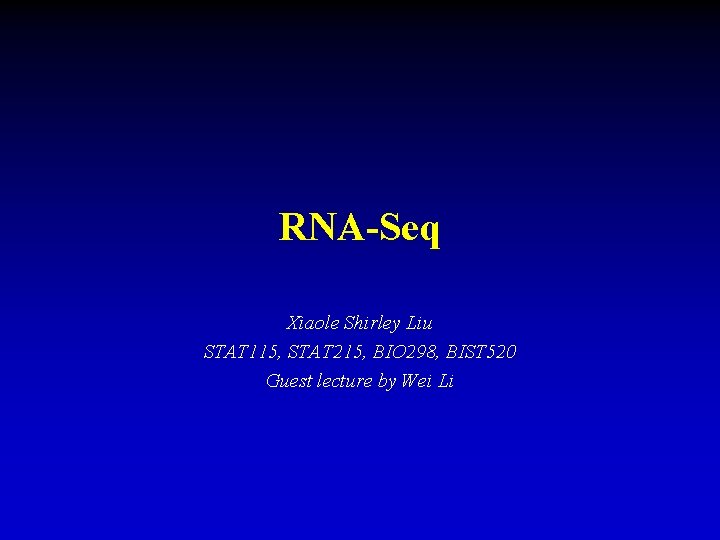
RNA-Seq Xiaole Shirley Liu STAT 115, STAT 215, BIO 298, BIST 520 Guest lecture by Wei Li
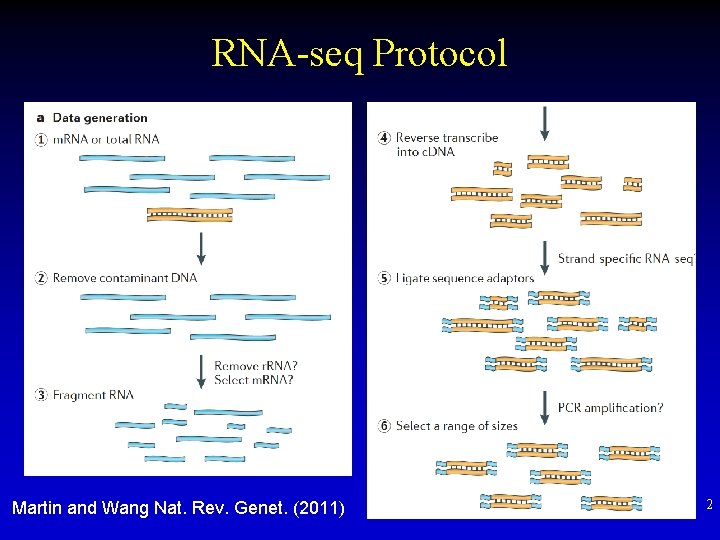
RNA-seq Protocol Martin and Wang Nat. Rev. Genet. (2011) 2
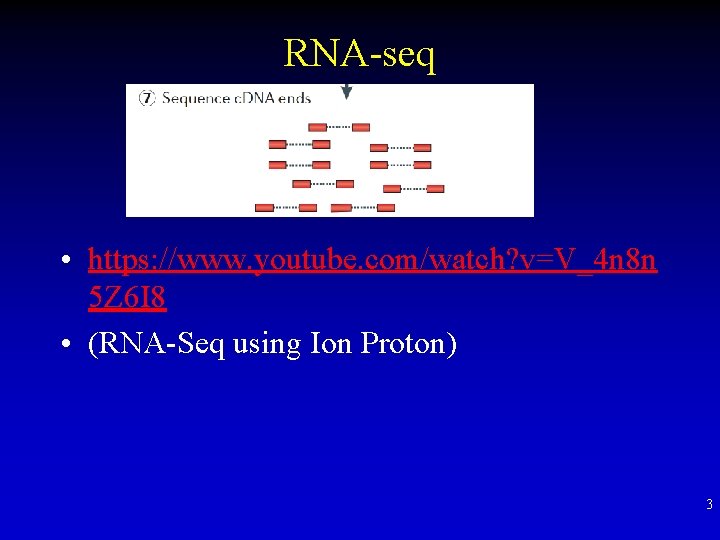
RNA-seq • https: //www. youtube. com/watch? v=V_4 n 8 n 5 Z 6 I 8 • (RNA-Seq using Ion Proton) 3
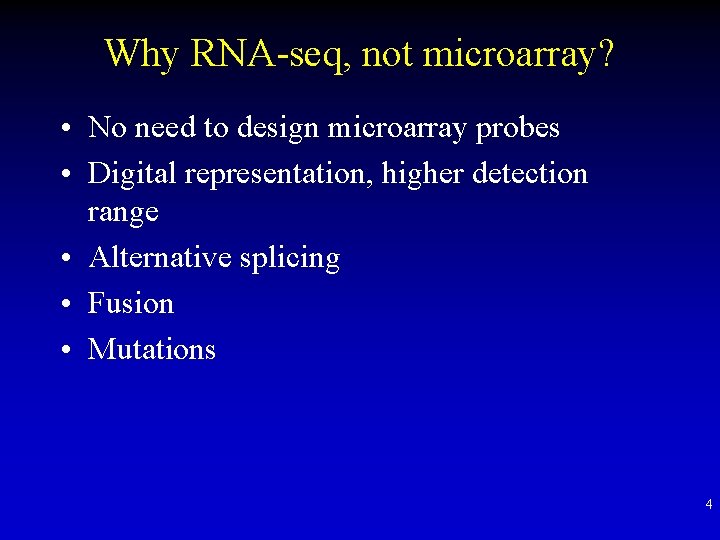
Why RNA-seq, not microarray? • No need to design microarray probes • Digital representation, higher detection range • Alternative splicing • Fusion • Mutations 4
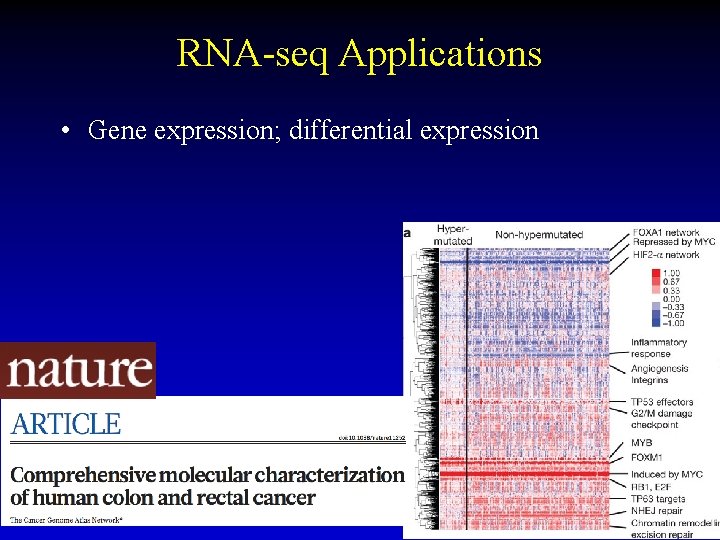
RNA-seq Applications • Gene expression; differential expression 5
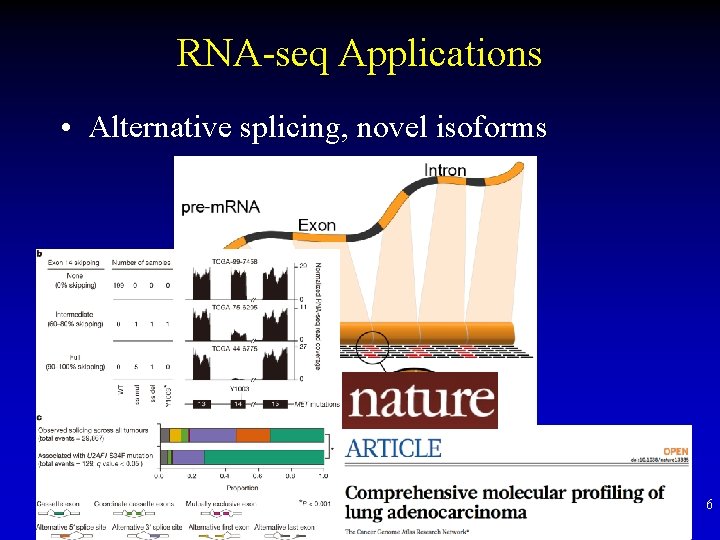
RNA-seq Applications • Alternative splicing, novel isoforms 6
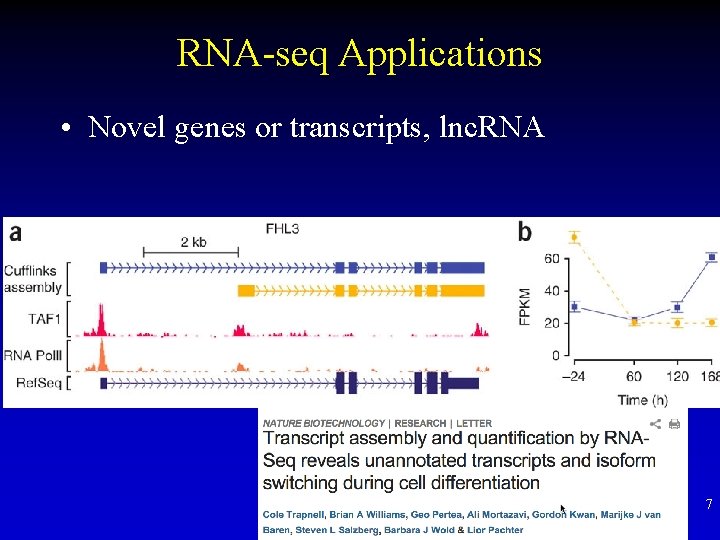
RNA-seq Applications • Novel genes or transcripts, lnc. RNA 7
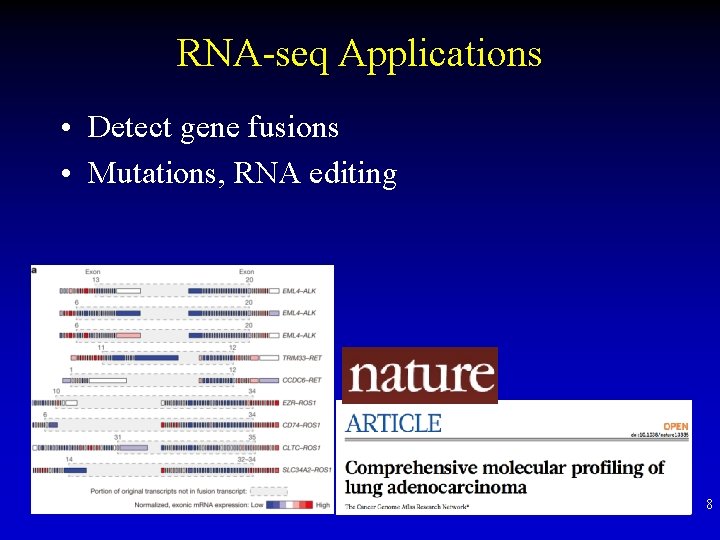
RNA-seq Applications • Detect gene fusions • Mutations, RNA editing 8
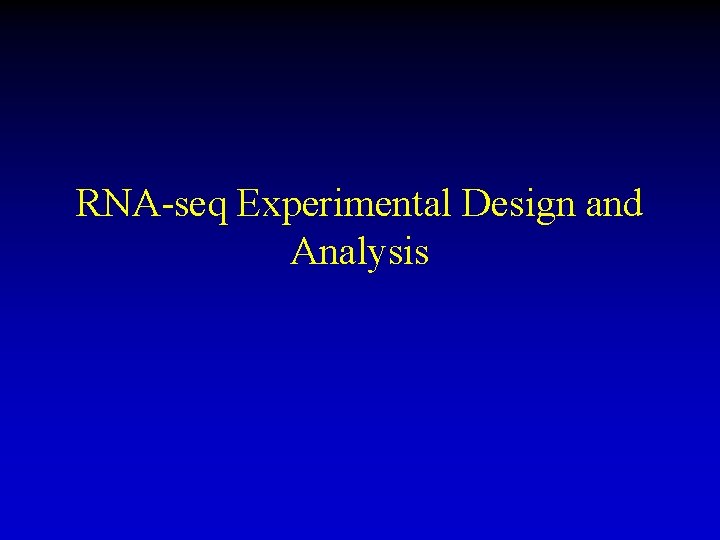
RNA-seq Experimental Design and Analysis
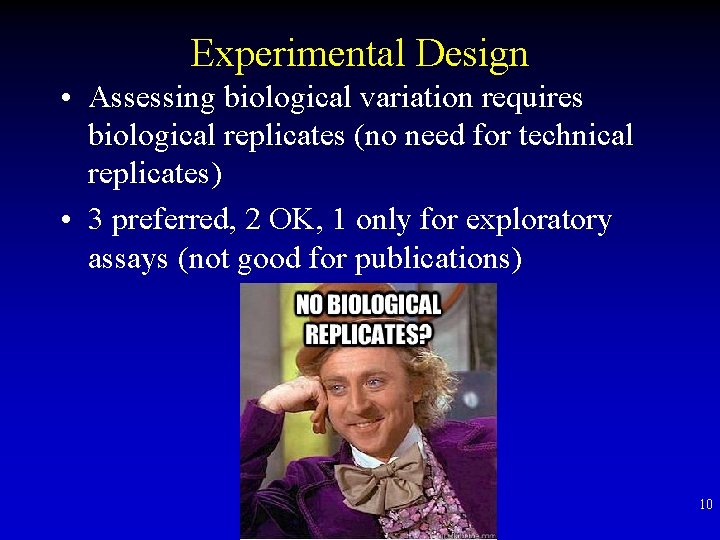
Experimental Design • Assessing biological variation requires biological replicates (no need for technical replicates) • 3 preferred, 2 OK, 1 only for exploratory assays (not good for publications) 10
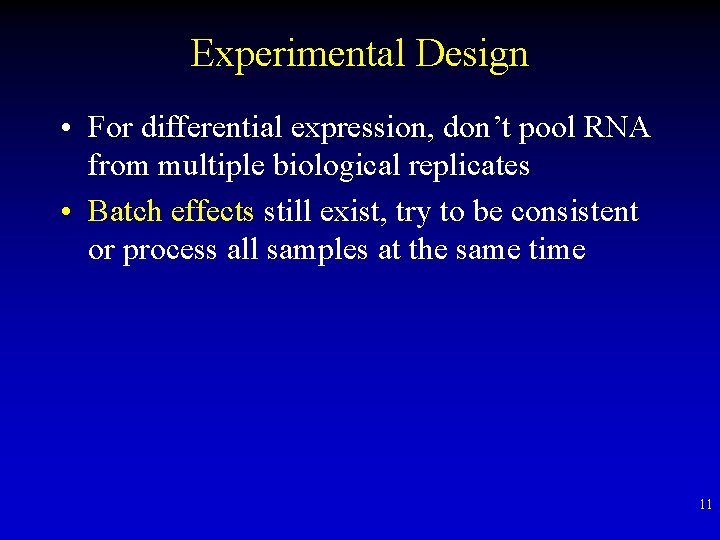
Experimental Design • For differential expression, don’t pool RNA from multiple biological replicates • Batch effects still exist, try to be consistent or process all samples at the same time 11
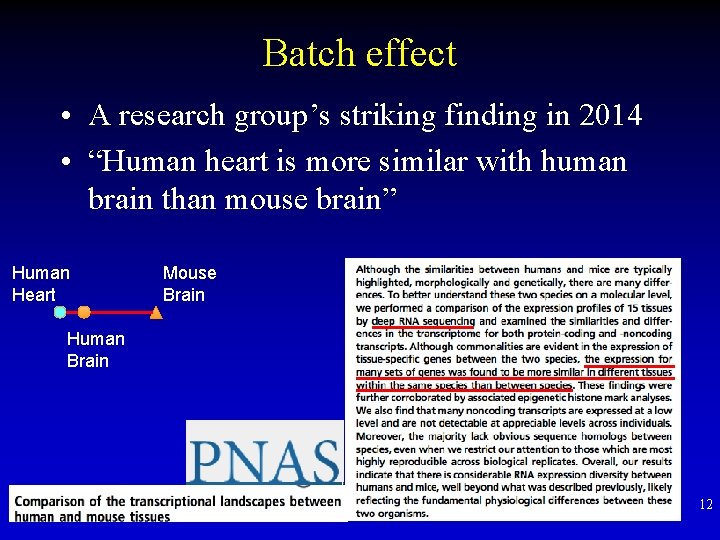
Batch effect • A research group’s striking finding in 2014 • “Human heart is more similar with human brain than mouse brain” Human Heart Mouse Brain Human Brain 12
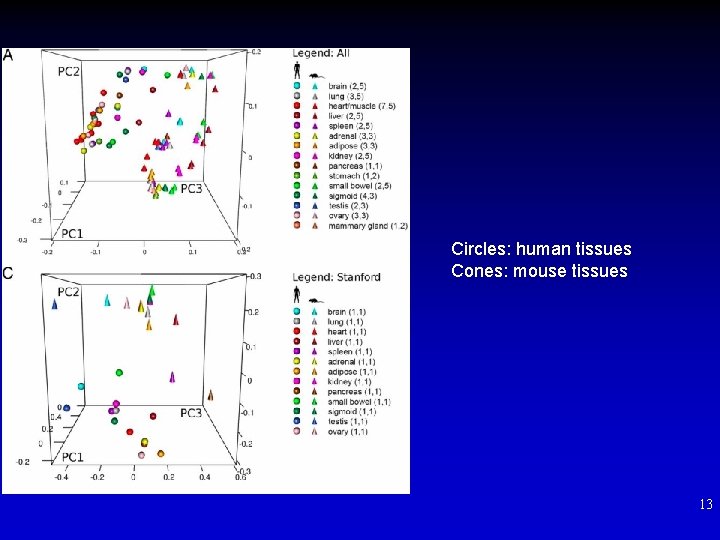
Circles: human tissues Cones: mouse tissues 13
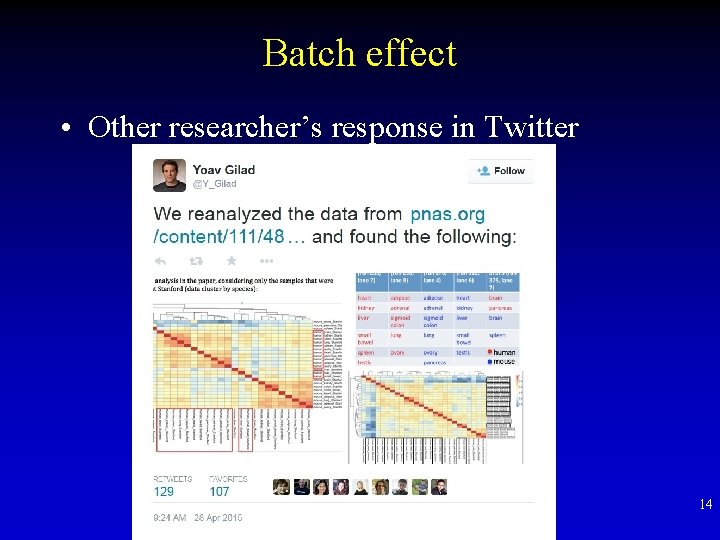
Batch effect • Other researcher’s response in Twitter 14
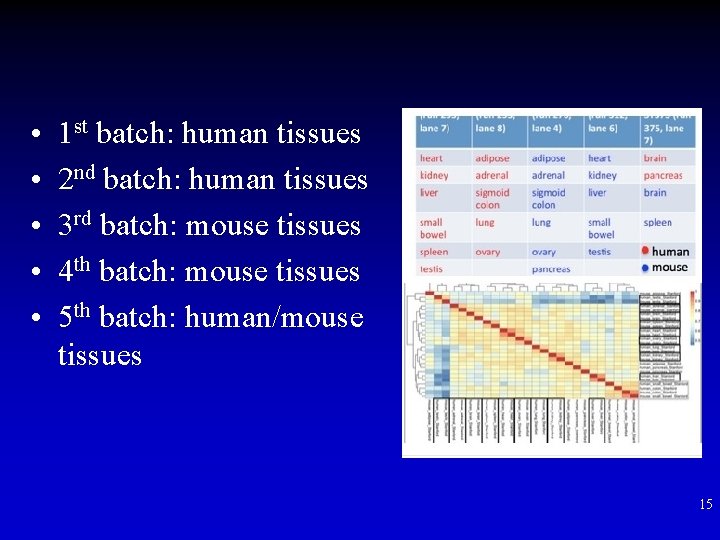
• • • 1 st batch: human tissues 2 nd batch: human tissues 3 rd batch: mouse tissues 4 th batch: mouse tissues 5 th batch: human/mouse tissues 15
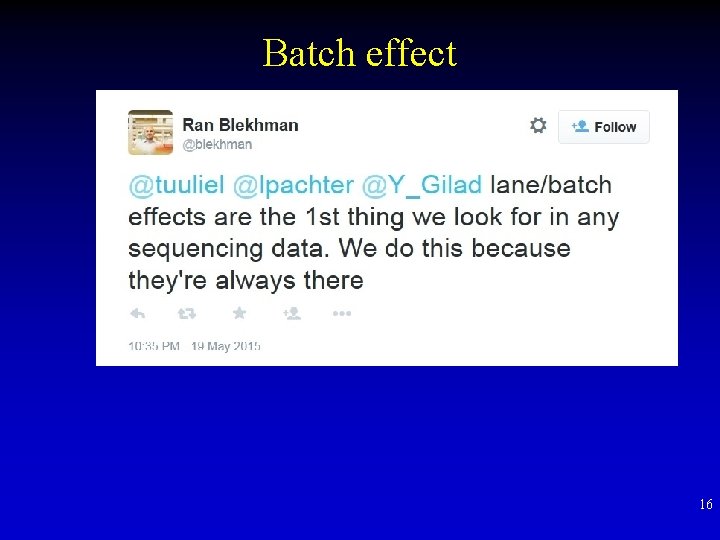
Batch effect 16
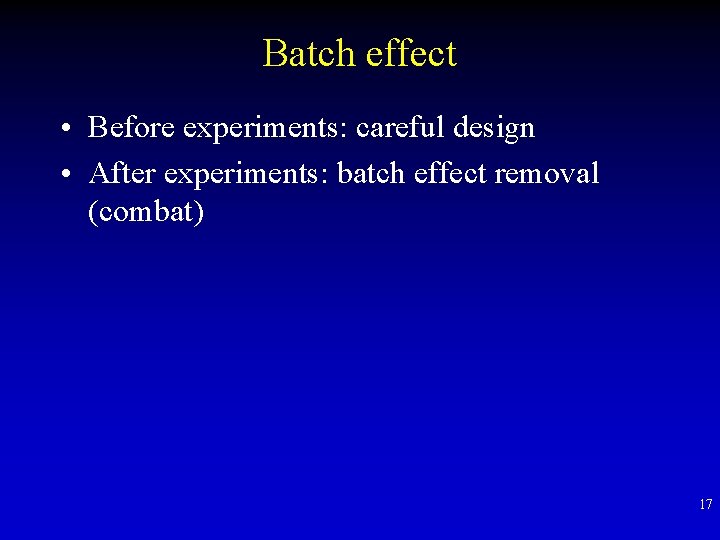
Batch effect • Before experiments: careful design • After experiments: batch effect removal (combat) 17
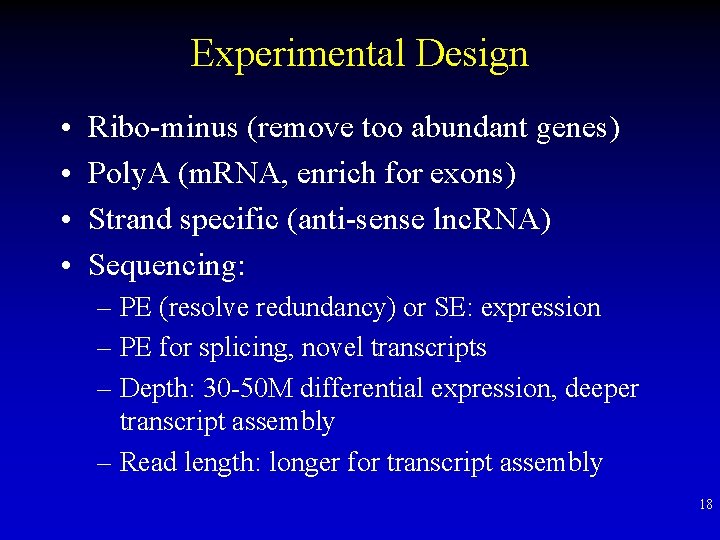
Experimental Design • • Ribo-minus (remove too abundant genes) Poly. A (m. RNA, enrich for exons) Strand specific (anti-sense lnc. RNA) Sequencing: – PE (resolve redundancy) or SE: expression – PE for splicing, novel transcripts – Depth: 30 -50 M differential expression, deeper transcript assembly – Read length: longer for transcript assembly 18
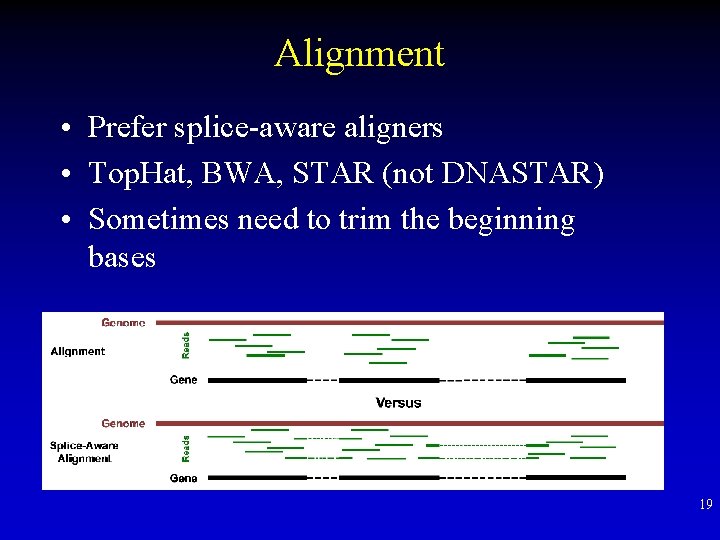
Alignment • Prefer splice-aware aligners • Top. Hat, BWA, STAR (not DNASTAR) • Sometimes need to trim the beginning bases 19
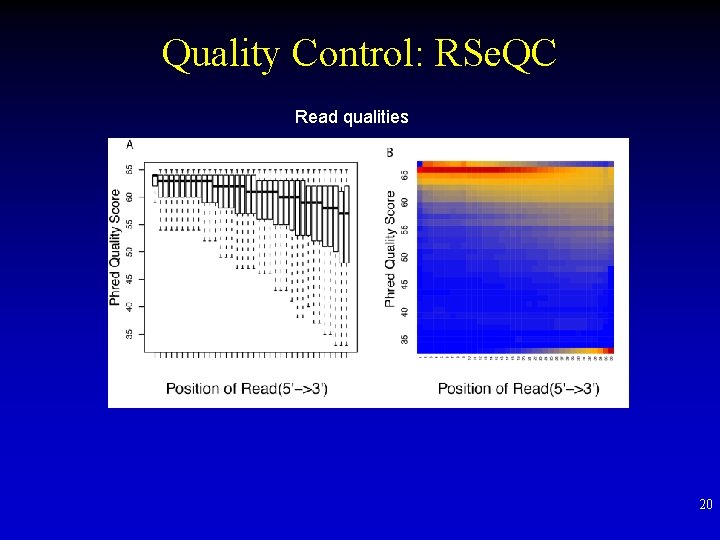
Quality Control: RSe. QC Read qualities 20
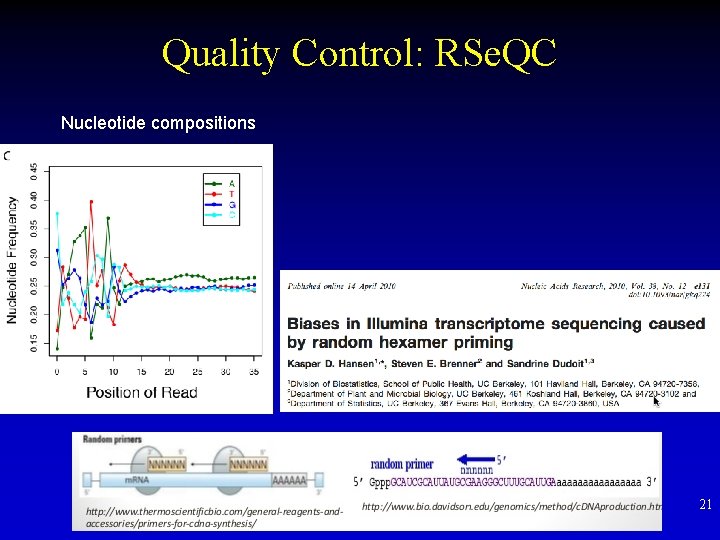
Quality Control: RSe. QC Nucleotide compositions 21
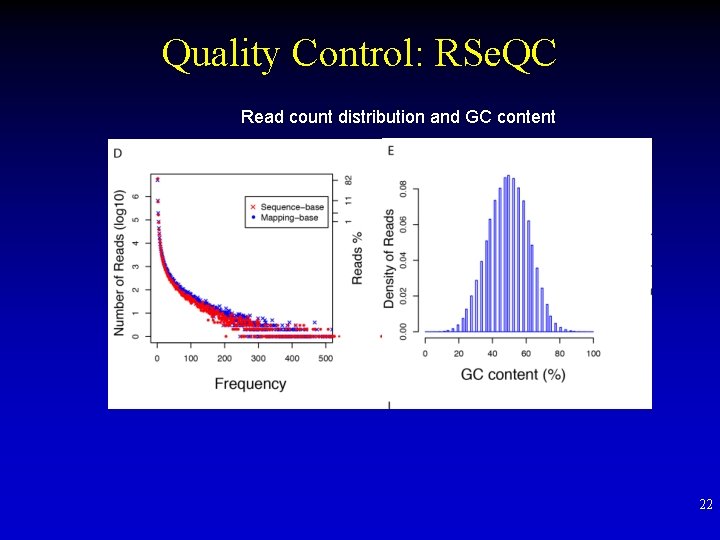
Quality Control: RSe. QC Read count distribution and GC content 22
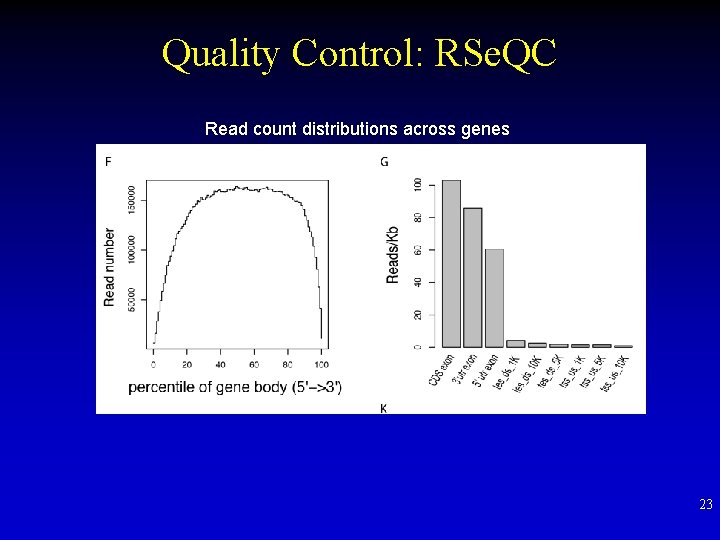
Quality Control: RSe. QC Read count distributions across genes 23
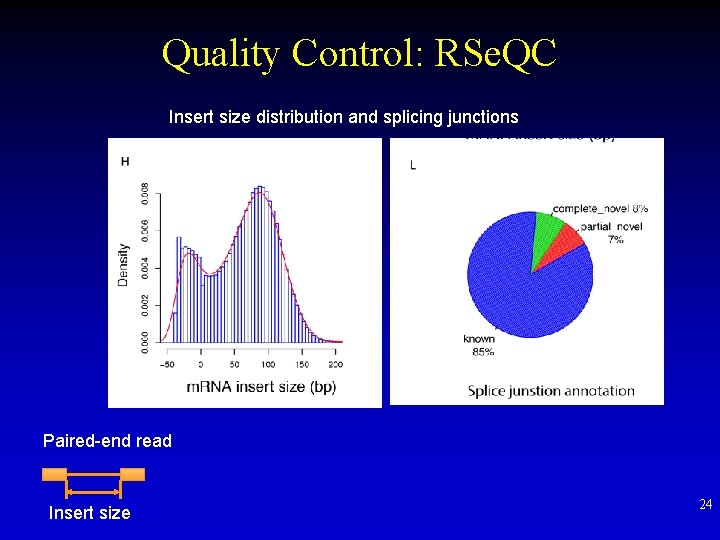
Quality Control: RSe. QC Insert size distribution and splicing junctions Paired-end read Insert size 24
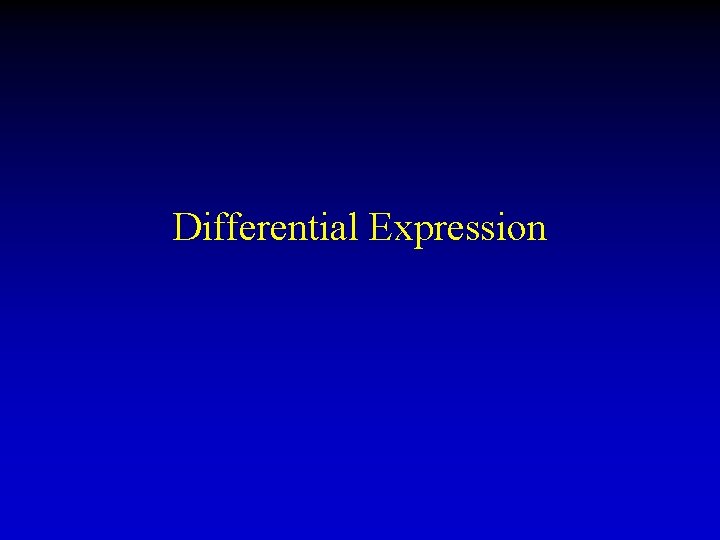
Differential Expression
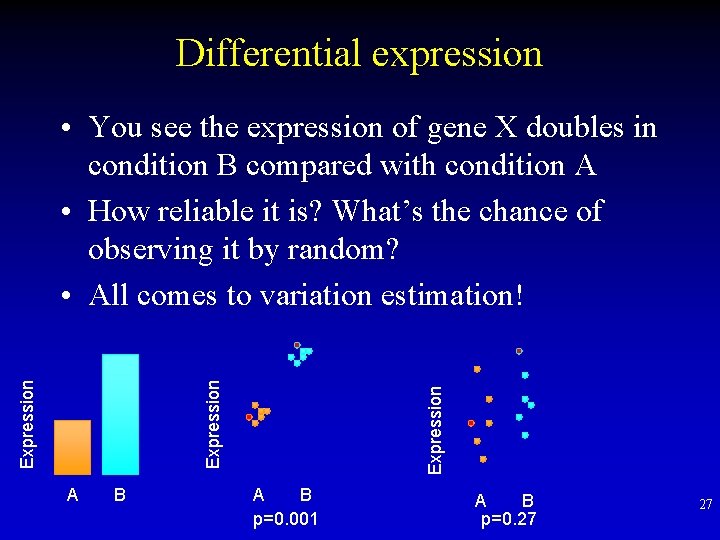
Differential expression A B Expression • You see the expression of gene X doubles in condition B compared with condition A • How reliable it is? What’s the chance of observing it by random? • All comes to variation estimation! A B p=0. 001 A B p=0. 27 27
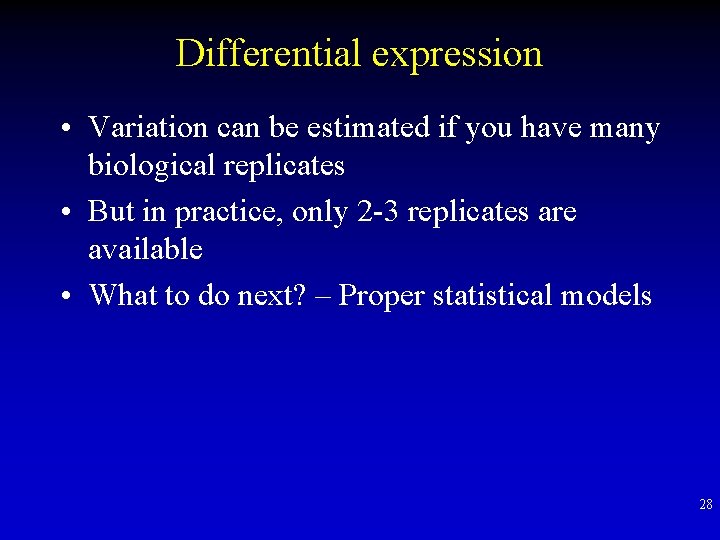
Differential expression • Variation can be estimated if you have many biological replicates • But in practice, only 2 -3 replicates are available • What to do next? – Proper statistical models 28
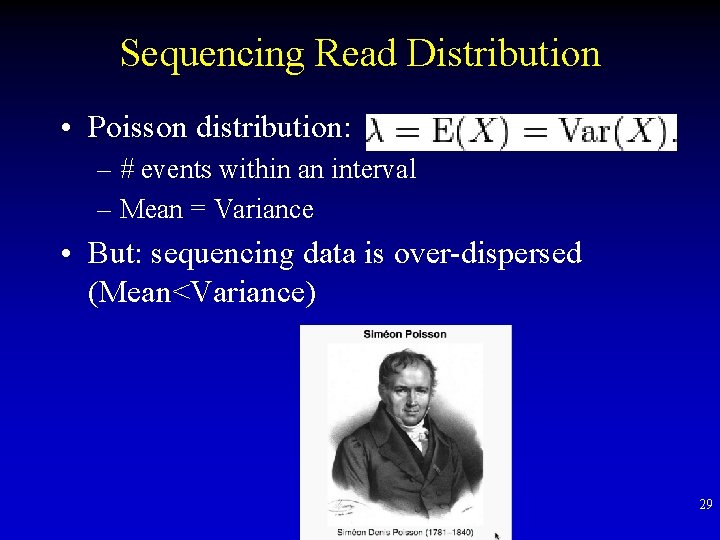
Sequencing Read Distribution • Poisson distribution: – # events within an interval – Mean = Variance • But: sequencing data is over-dispersed (Mean<Variance) 29
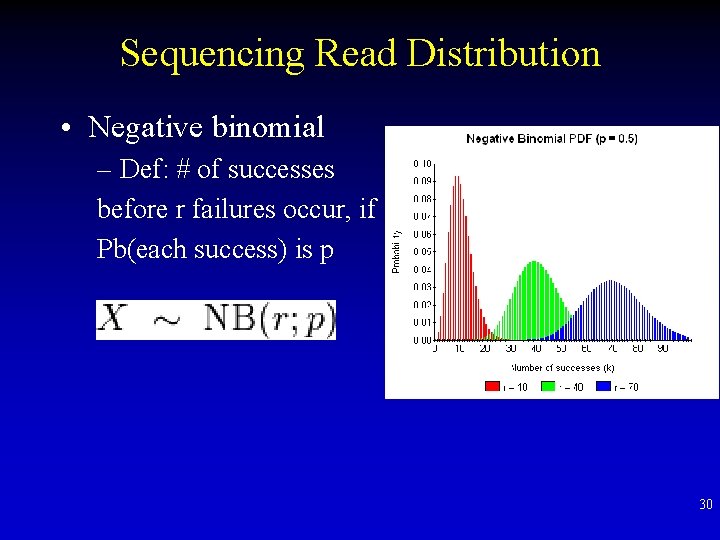
Sequencing Read Distribution • Negative binomial – Def: # of successes before r failures occur, if Pb(each success) is p 30
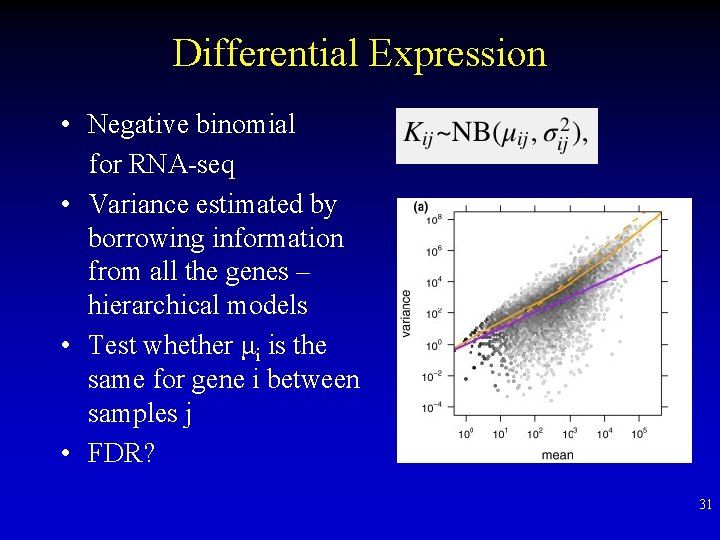
Differential Expression • Negative binomial for RNA-seq • Variance estimated by borrowing information from all the genes – hierarchical models • Test whether μi is the same for gene i between samples j • FDR? 31
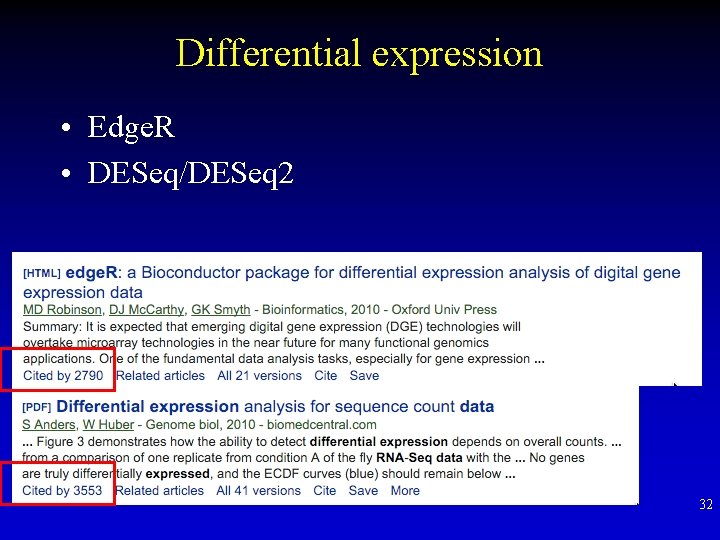
Differential expression • Edge. R • DESeq/DESeq 2 32
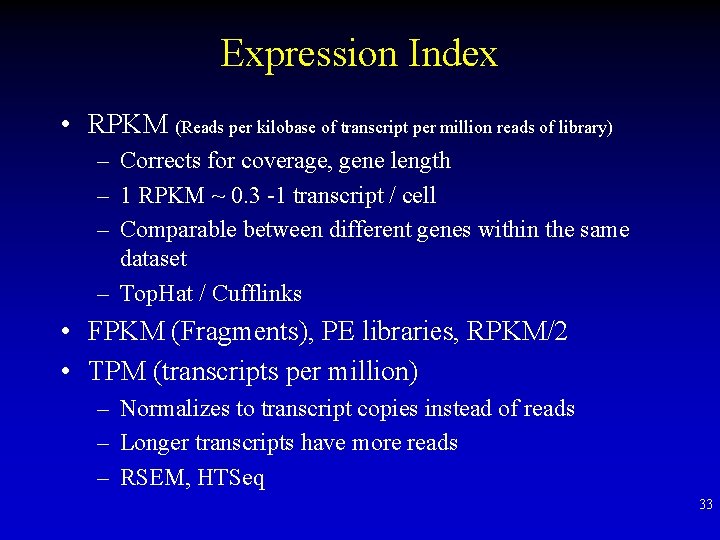
Expression Index • RPKM (Reads per kilobase of transcript per million reads of library) – Corrects for coverage, gene length – 1 RPKM ~ 0. 3 -1 transcript / cell – Comparable between different genes within the same dataset – Top. Hat / Cufflinks • FPKM (Fragments), PE libraries, RPKM/2 • TPM (transcripts per million) – Normalizes to transcript copies instead of reads – Longer transcripts have more reads – RSEM, HTSeq 33
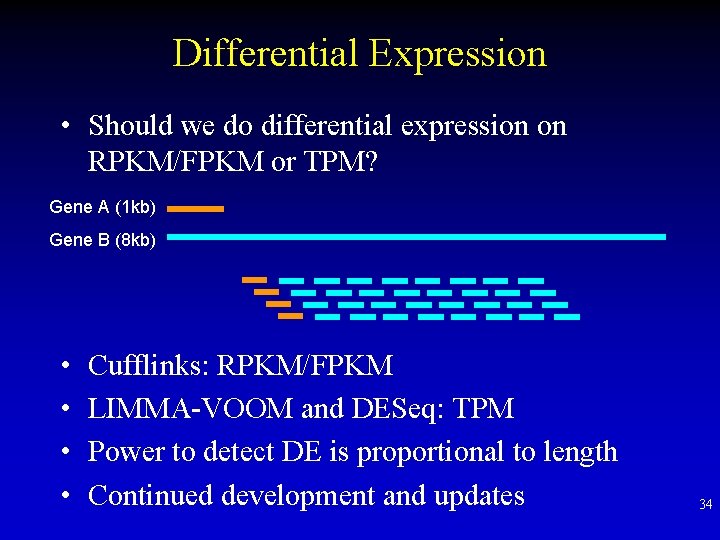
Differential Expression • Should we do differential expression on RPKM/FPKM or TPM? Gene A (1 kb) Gene B (8 kb) • • Cufflinks: RPKM/FPKM LIMMA-VOOM and DESeq: TPM Power to detect DE is proportional to length Continued development and updates 34
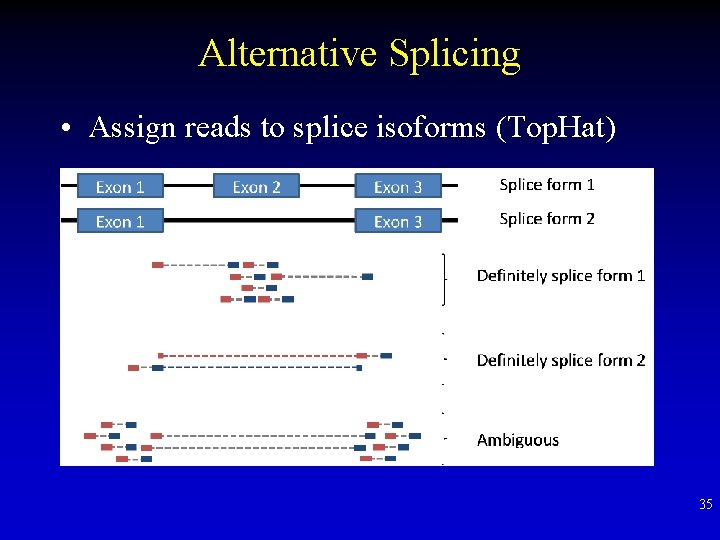
Alternative Splicing • Assign reads to splice isoforms (Top. Hat) 35
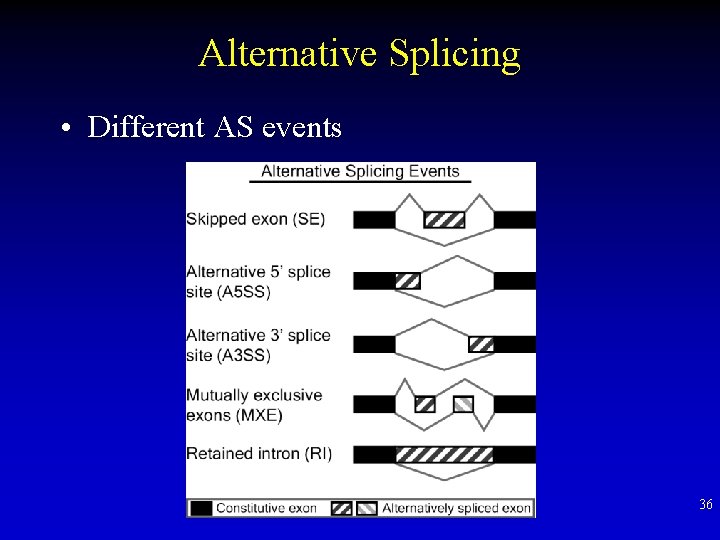
Alternative Splicing • Different AS events 36
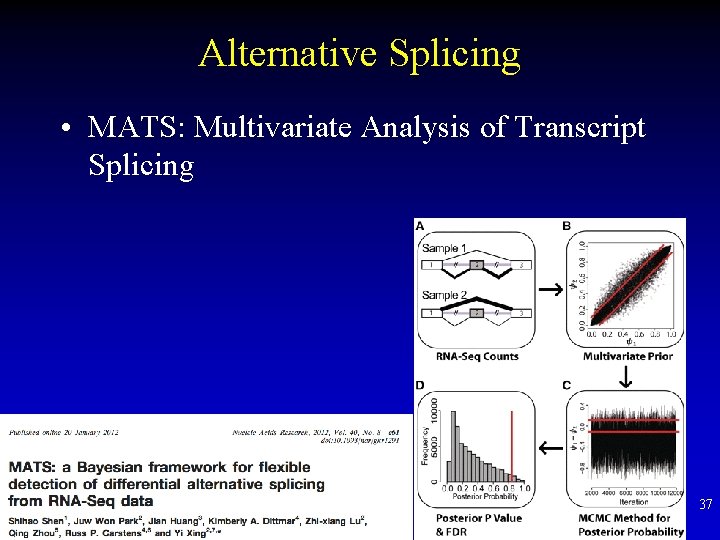
Alternative Splicing • MATS: Multivariate Analysis of Transcript Splicing 37
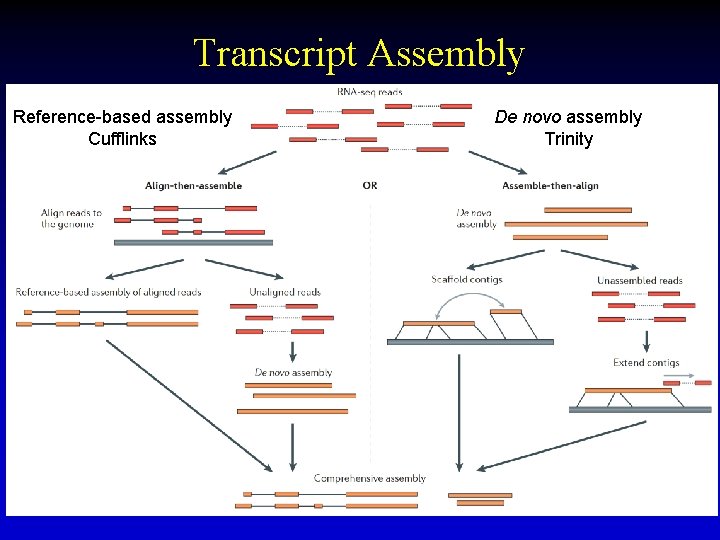
Transcript Assembly Reference-based assembly Cufflinks De novo assembly Trinity 38
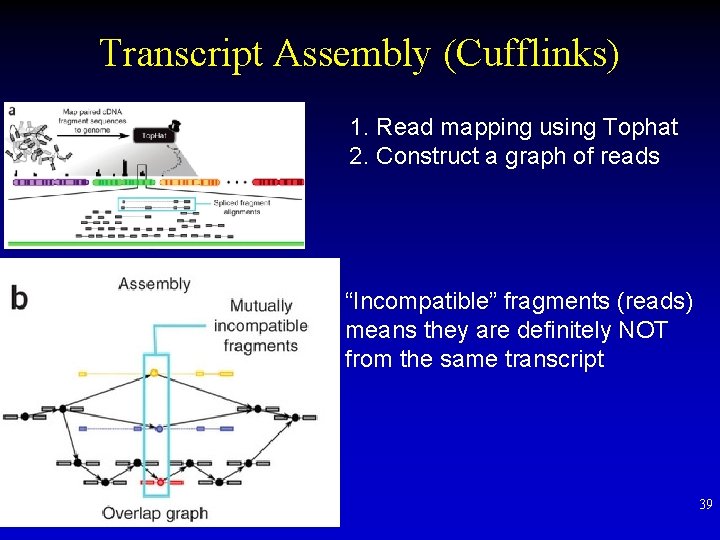
Transcript Assembly (Cufflinks) 1. Read mapping using Tophat 2. Construct a graph of reads “Incompatible” fragments (reads) means they are definitely NOT from the same transcript 39
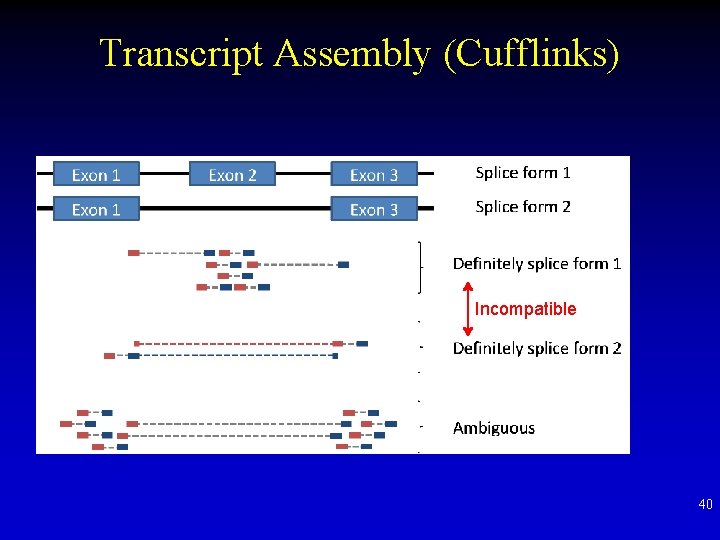
Transcript Assembly (Cufflinks) Incompatible 40
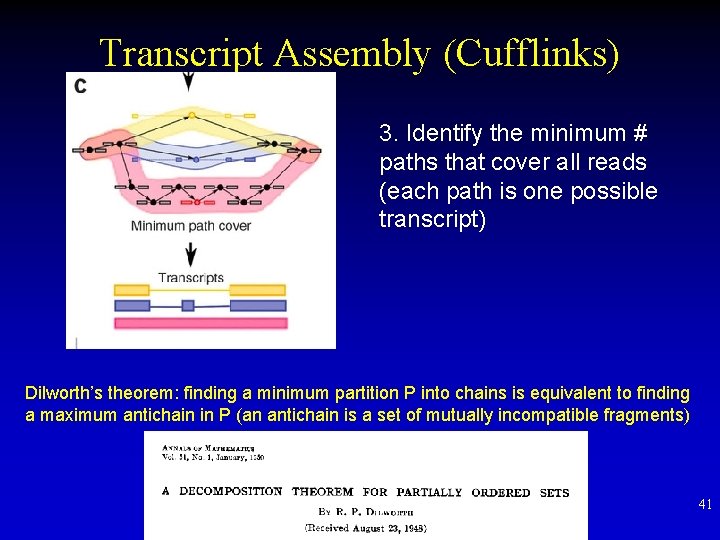
Transcript Assembly (Cufflinks) 3. Identify the minimum # paths that cover all reads (each path is one possible transcript) Dilworth’s theorem: finding a minimum partition P into chains is equivalent to finding a maximum antichain in P (an antichain is a set of mutually incompatible fragments) 41
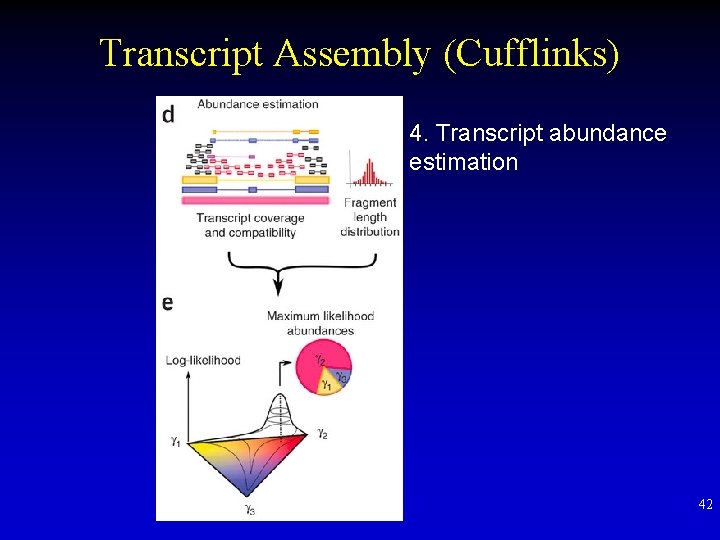
Transcript Assembly (Cufflinks) 4. Transcript abundance estimation 42
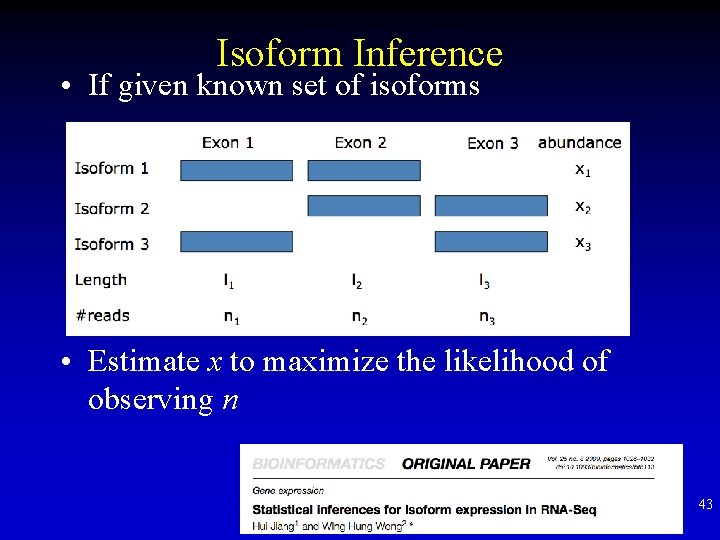
Isoform Inference • If given known set of isoforms • Estimate x to maximize the likelihood of observing n 43
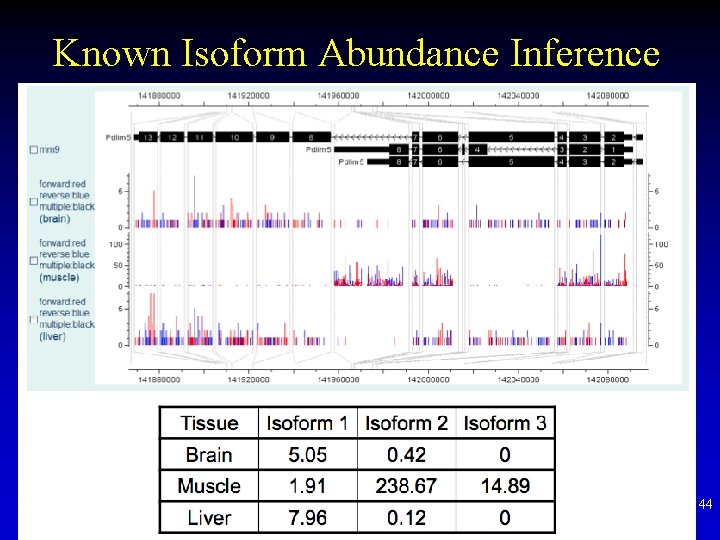
Known Isoform Abundance Inference 44
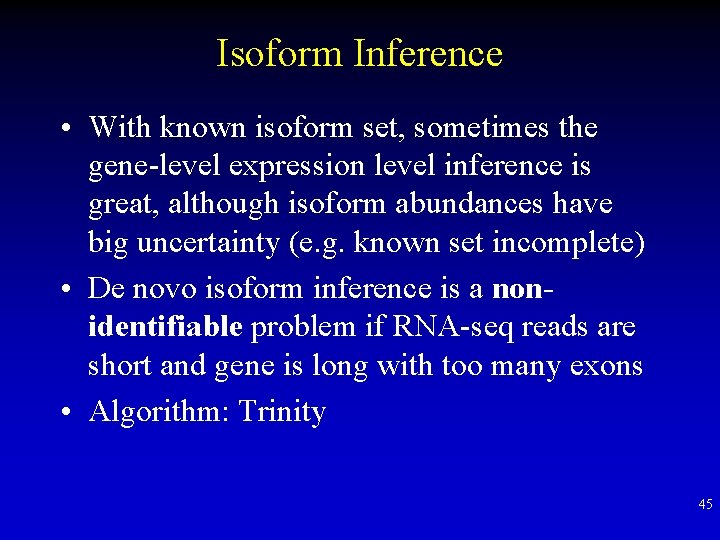
Isoform Inference • With known isoform set, sometimes the gene-level expression level inference is great, although isoform abundances have big uncertainty (e. g. known set incomplete) • De novo isoform inference is a nonidentifiable problem if RNA-seq reads are short and gene is long with too many exons • Algorithm: Trinity 45
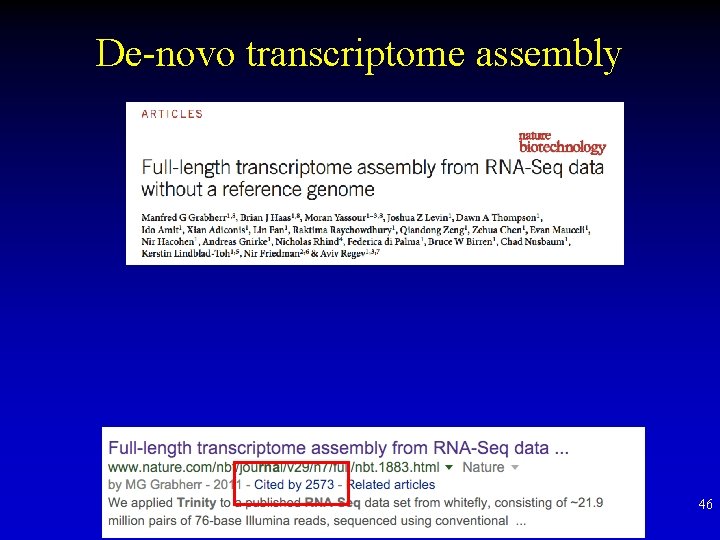
De-novo transcriptome assembly 46
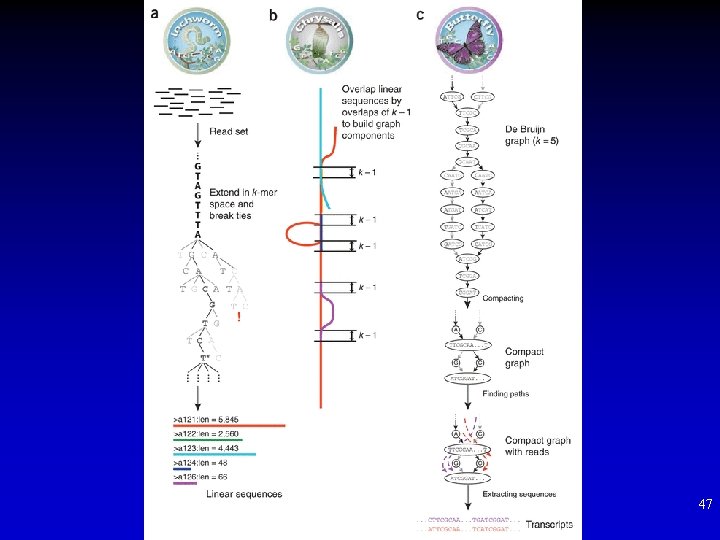
47
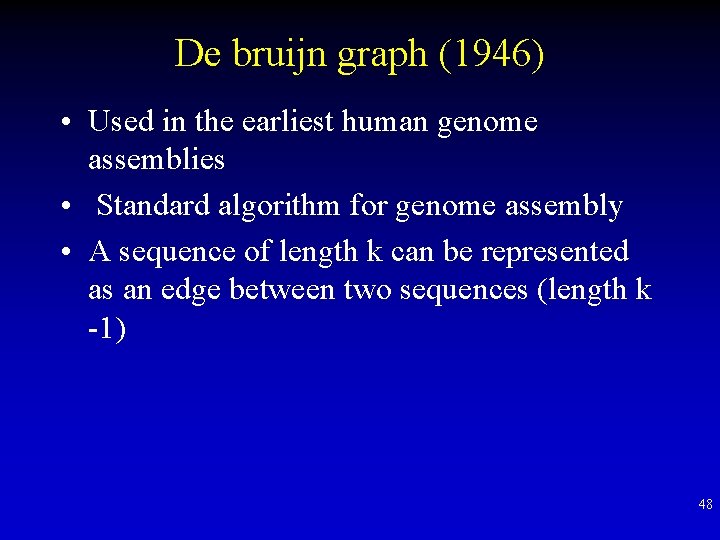
De bruijn graph (1946) • Used in the earliest human genome assemblies • Standard algorithm for genome assembly • A sequence of length k can be represented as an edge between two sequences (length k -1) 48
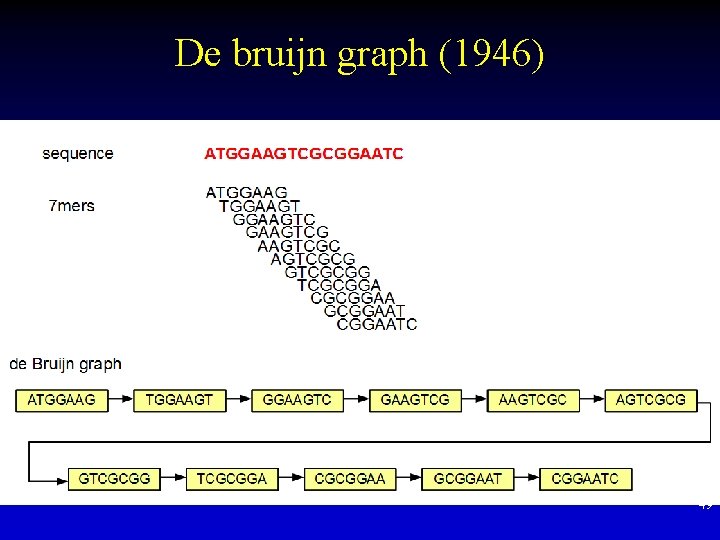
De bruijn graph (1946) 49
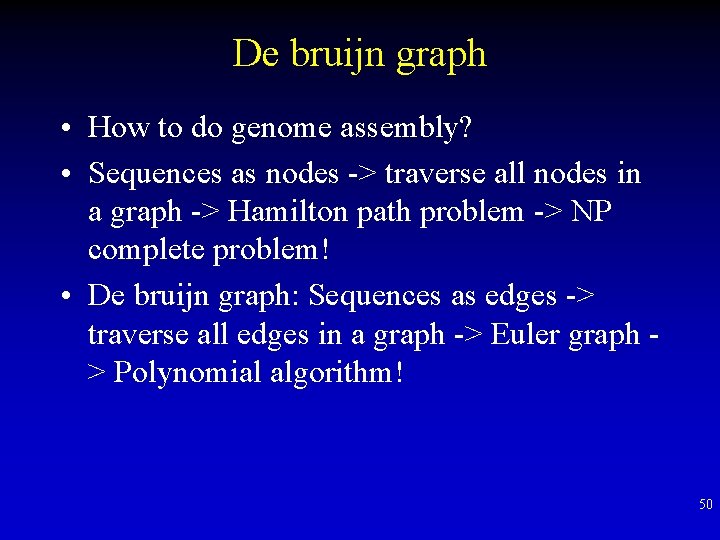
De bruijn graph • How to do genome assembly? • Sequences as nodes -> traverse all nodes in a graph -> Hamilton path problem -> NP complete problem! • De bruijn graph: Sequences as edges -> traverse all edges in a graph -> Euler graph > Polynomial algorithm! 50
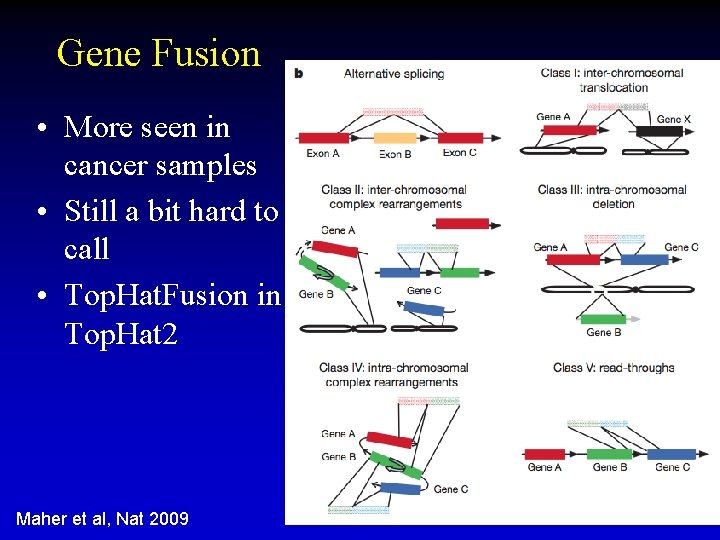
Gene Fusion • More seen in cancer samples • Still a bit hard to call • Top. Hat. Fusion in Top. Hat 2 Maher et al, Nat 2009 51
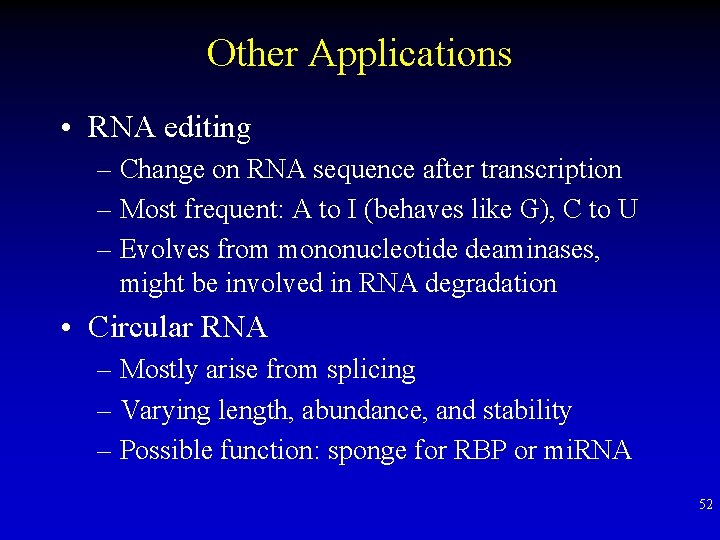
Other Applications • RNA editing – Change on RNA sequence after transcription – Most frequent: A to I (behaves like G), C to U – Evolves from mononucleotide deaminases, might be involved in RNA degradation • Circular RNA – Mostly arise from splicing – Varying length, abundance, and stability – Possible function: sponge for RBP or mi. RNA 52
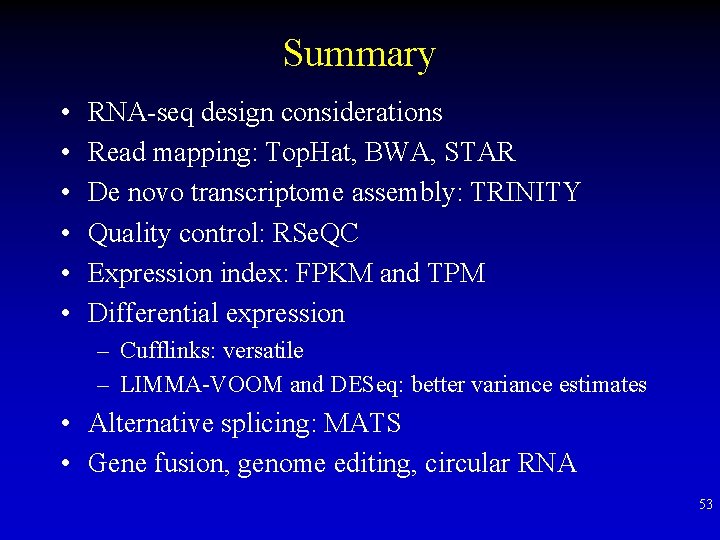
Summary • • • RNA-seq design considerations Read mapping: Top. Hat, BWA, STAR De novo transcriptome assembly: TRINITY Quality control: RSe. QC Expression index: FPKM and TPM Differential expression – Cufflinks: versatile – LIMMA-VOOM and DESeq: better variance estimates • Alternative splicing: MATS • Gene fusion, genome editing, circular RNA 53
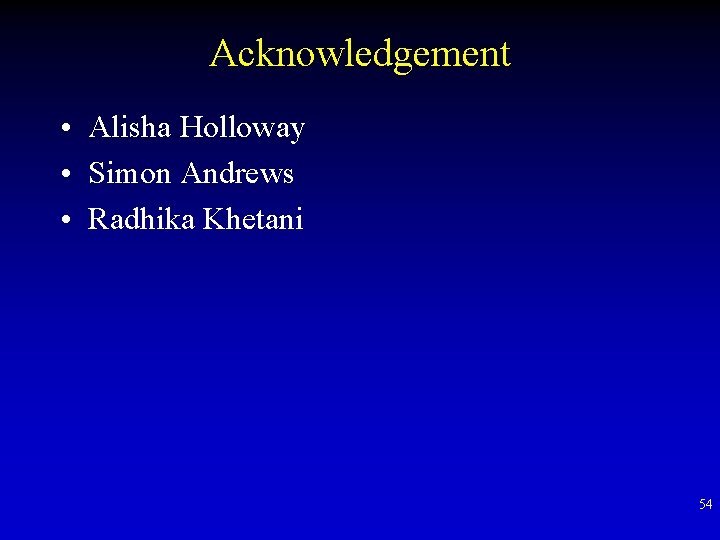
Acknowledgement • Alisha Holloway • Simon Andrews • Radhika Khetani 54
Xiaole liu
Agtcc
Xiaole liu
Rnaseq illumina
Stat 115
Líu líu lo lo ta ca hát say sưa
Alex liu cecilia liu
/servsoc/inicio.aspx
Iso/tc 215
Ley 40/215
Economic 215
Po box 1220 philadelphia pa 19105
Rounding word problems
Work physic
The formula c=5p+215 relates c
Sentenza n. 215/87 della corte costituzionale
Economic 215
Fixed point addition and subtraction flowchart
Dtu-215
Completed ics 215 form
Stc 215/1994
Eecs 427
Ratio for tangent
Ppt-115
How to name an angle in four ways
Psalm 1 gnt
Sonotron pa 2000
Math 115
Next hfa 115
Hbu 131
Read the transcription and write the words
Ieor 115
Ejemplo de un ensayo narrativo
Crónica de d joão i resumo capítulo 115
Harvey vs facey
Gambar
Fas 107
Ieee 115
Canto salmo 115
Cse 115
256 en yakın yüzlüğe yuvarlama
Round 115 to the nearest hundred
Ind as 115 applicability
Bus 115
Edthp 115
Csc 115
Next hfa 115
Cse 115
Elements of contract
Sec 115 jb
Psalm 115 esv
Solicitud de licencia articulo 114 y 115 decreto 688/93
Shirley gaw
Shirley c heim middle school