STAT 497 LECTURE NOTES 3 STATIONARY TIME SERIES
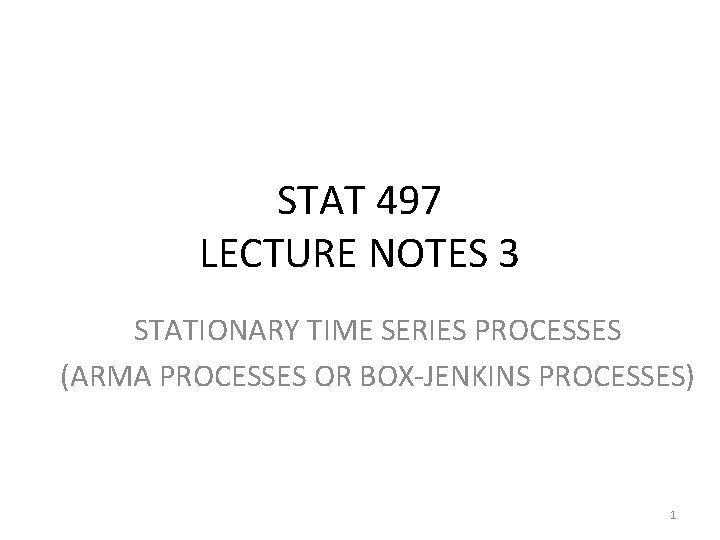
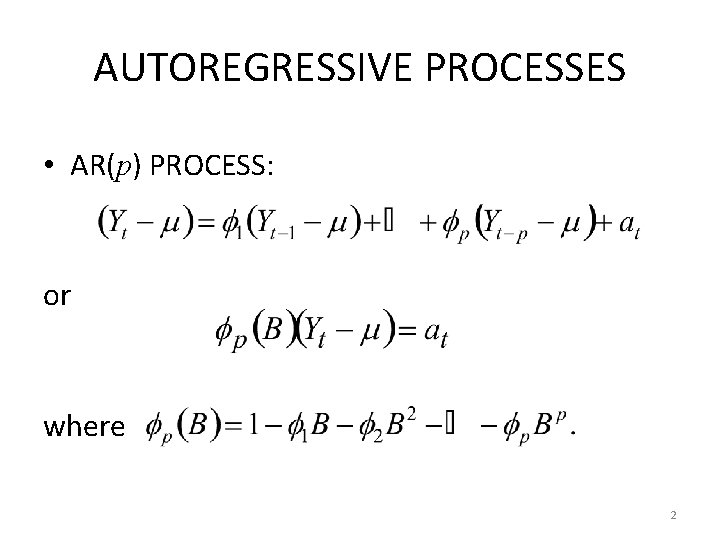
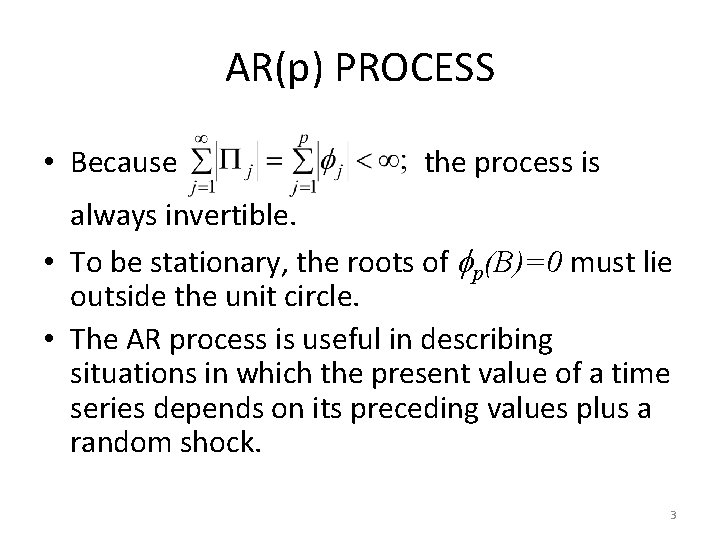
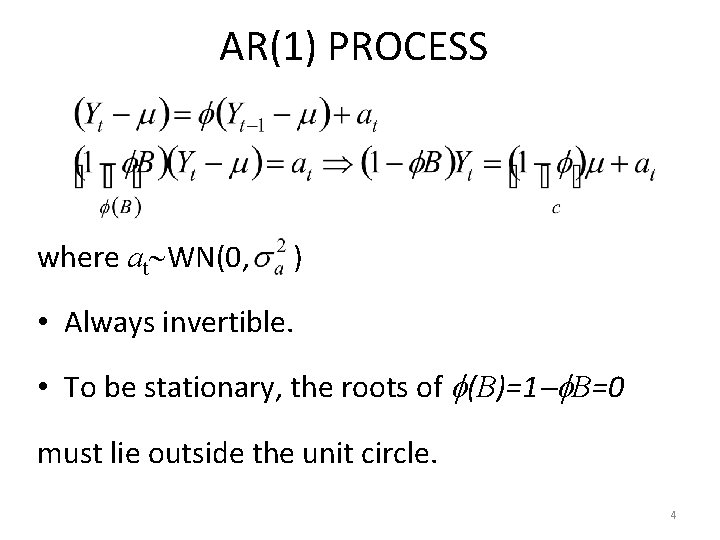
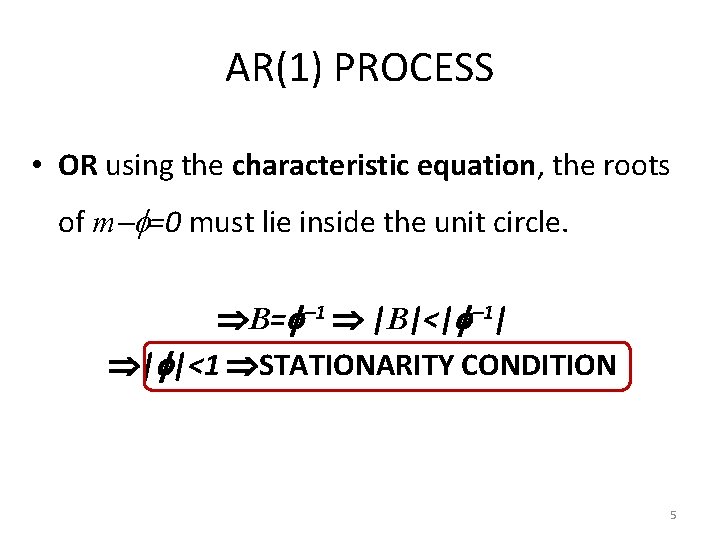
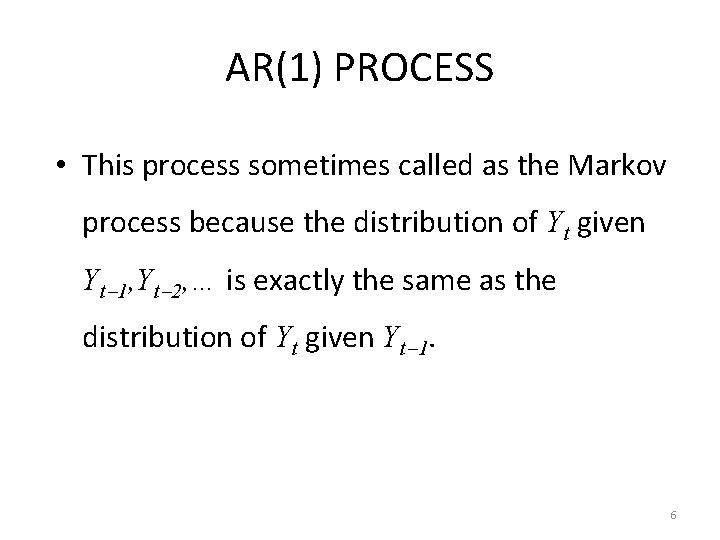
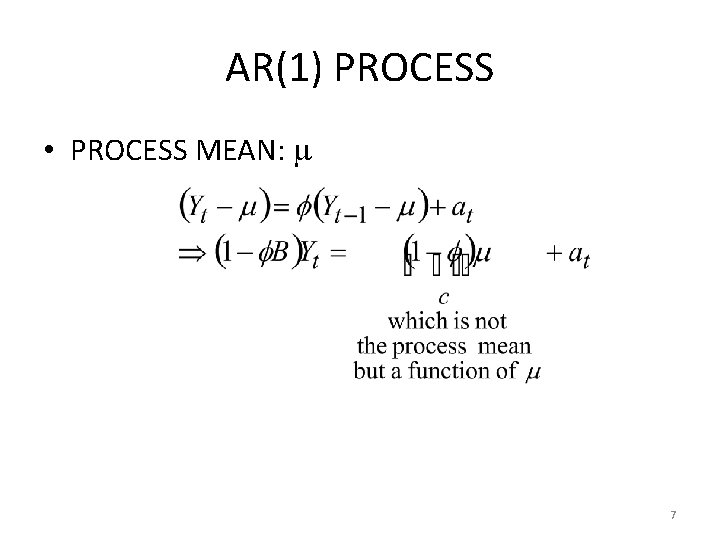
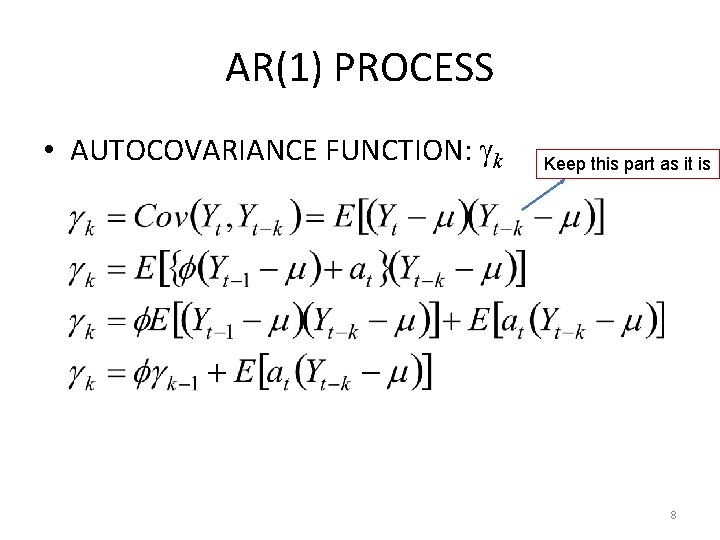
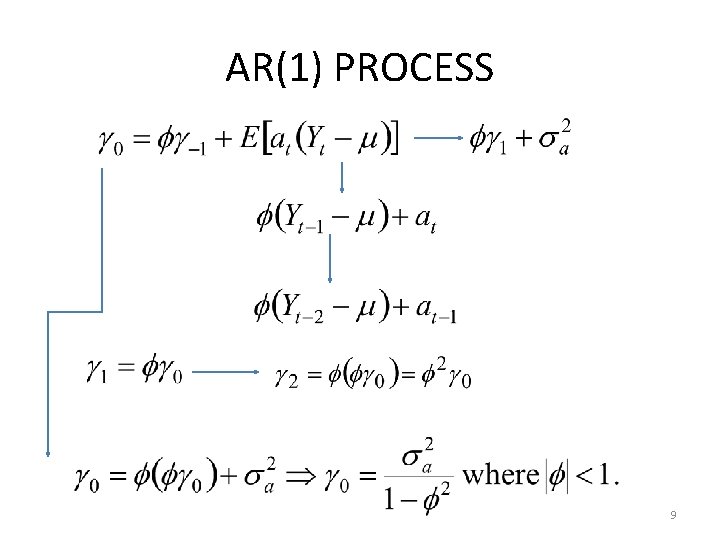
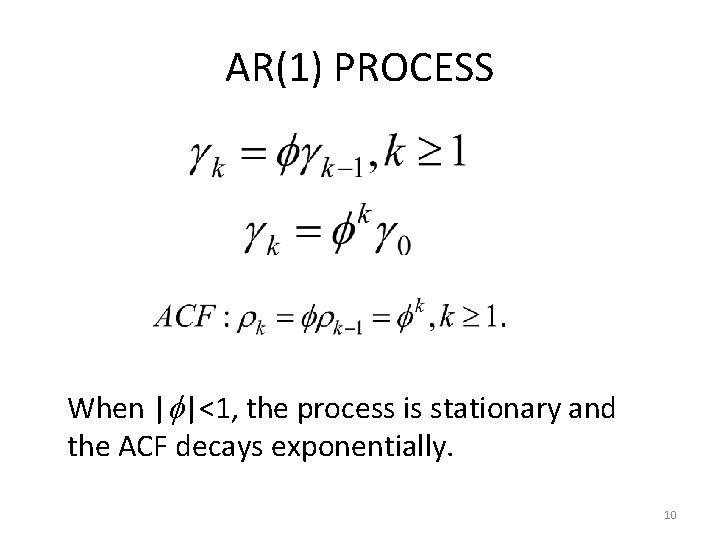
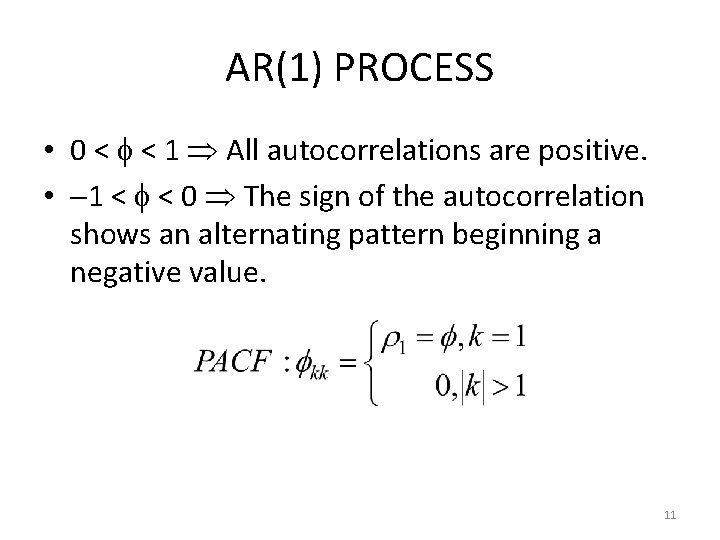
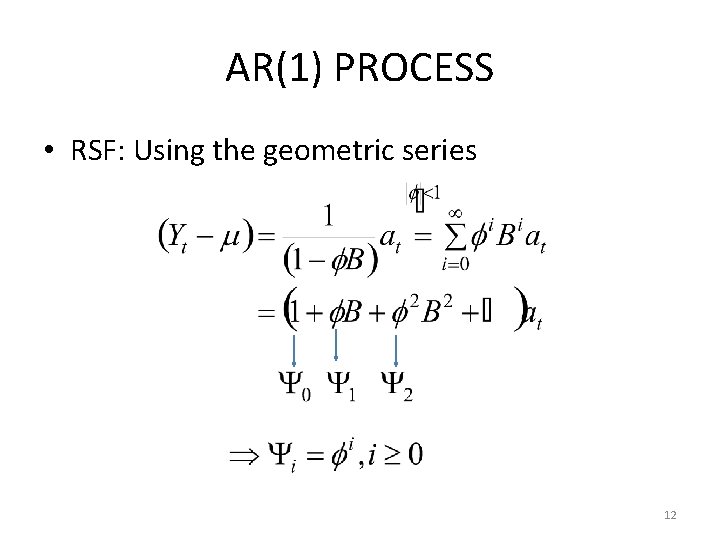
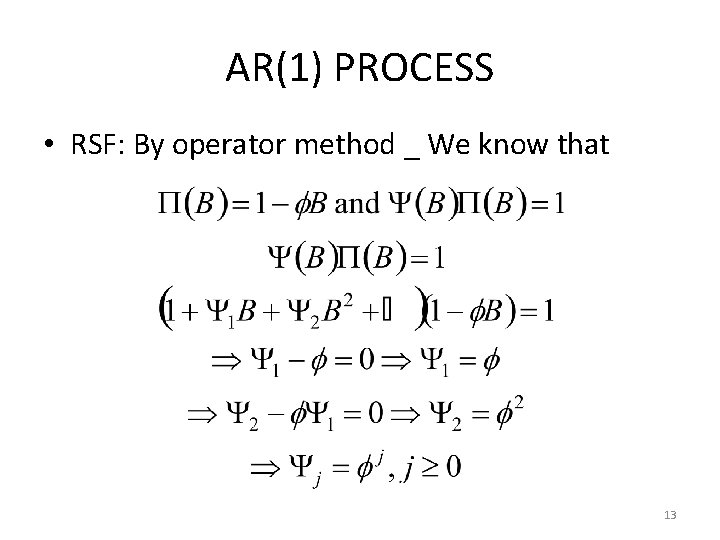
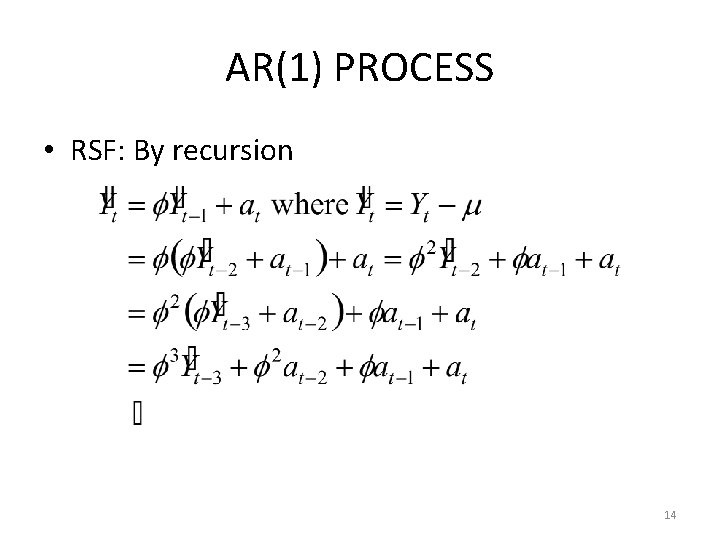
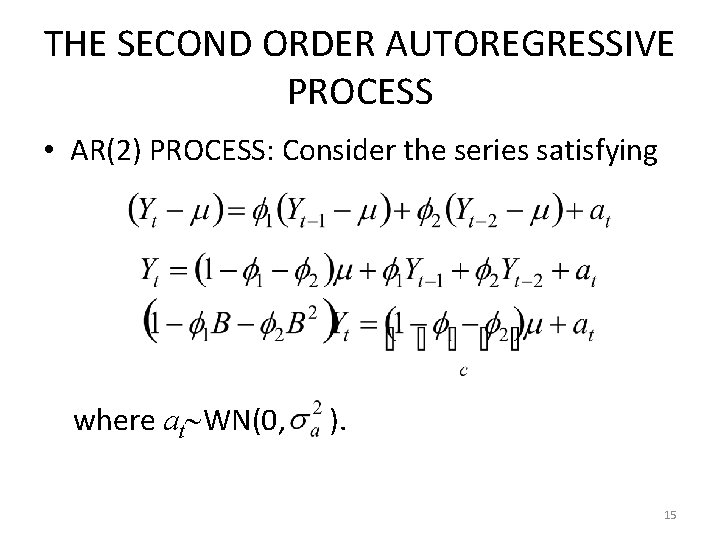
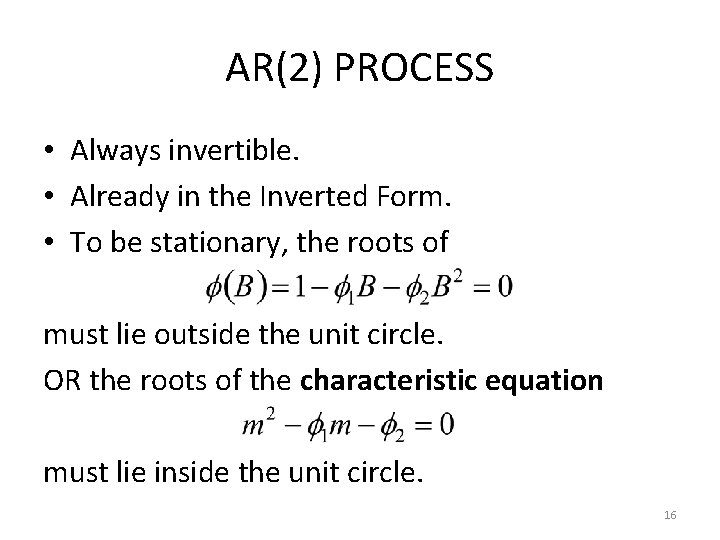
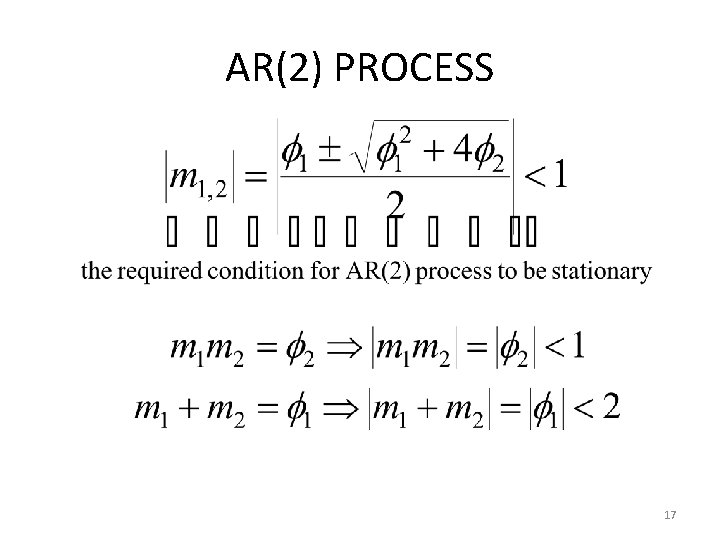
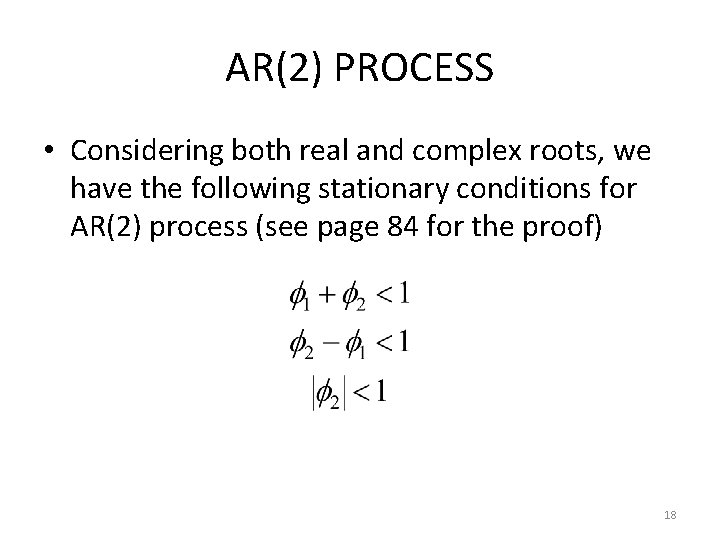
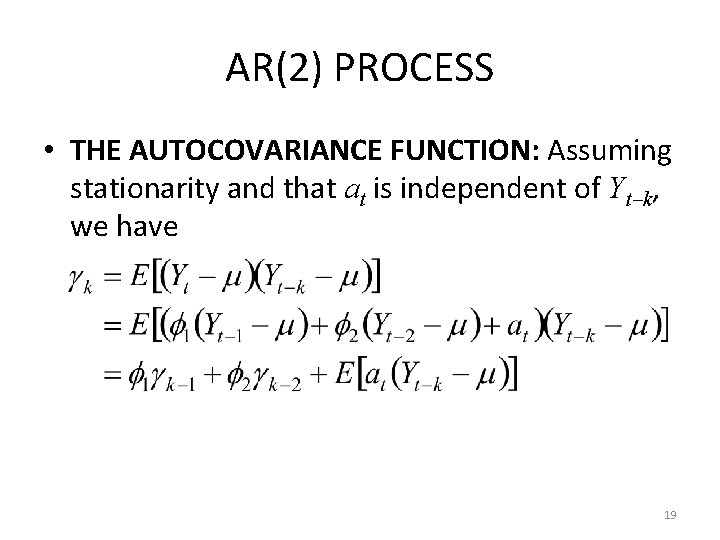
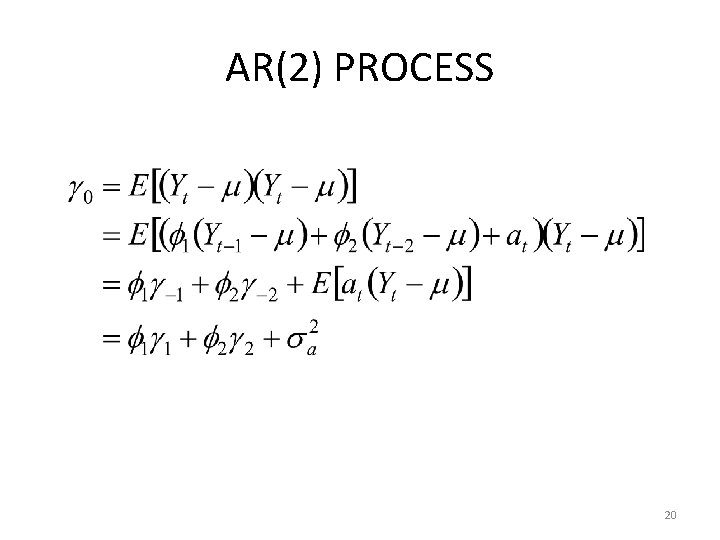
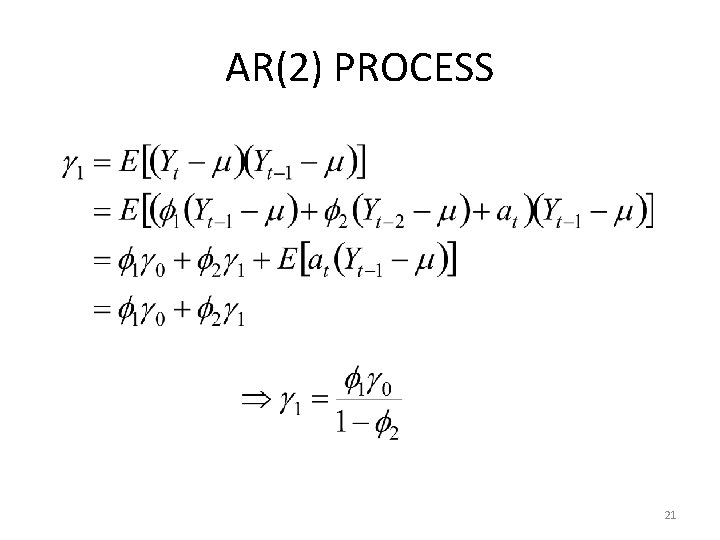
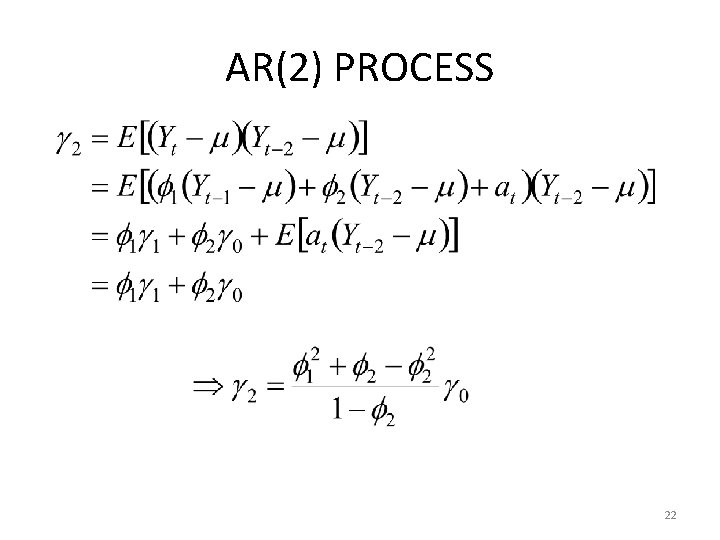
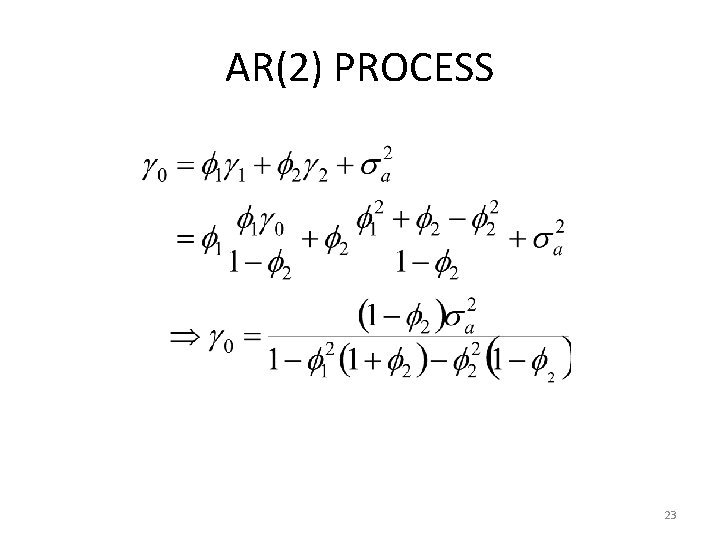
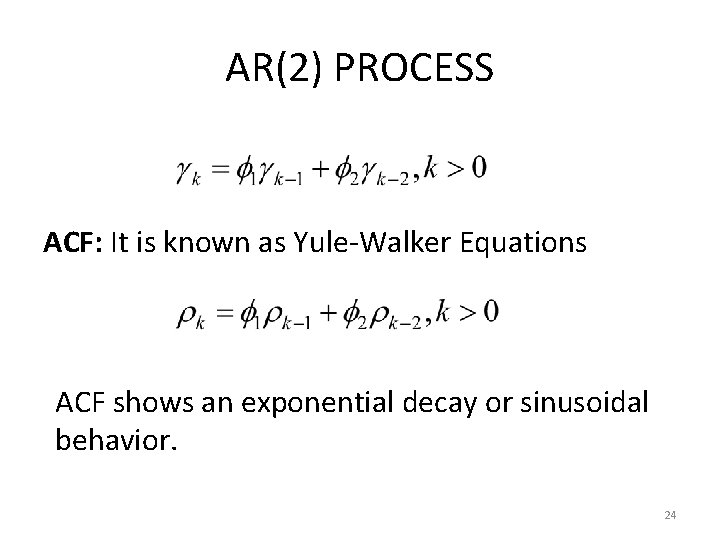
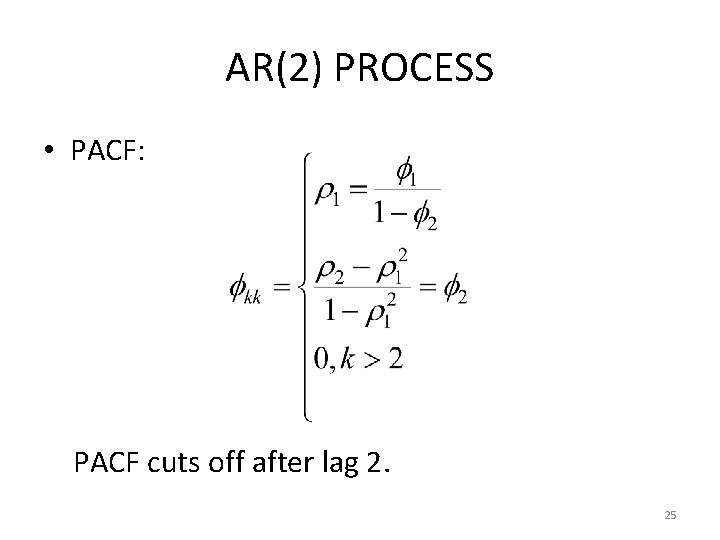
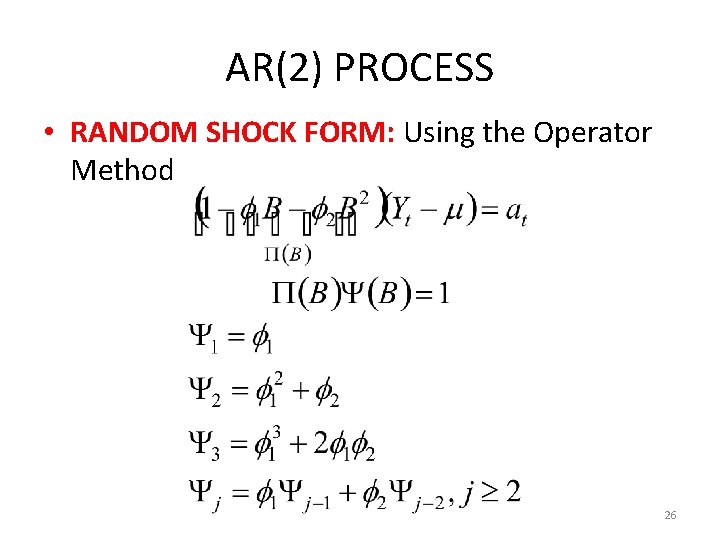
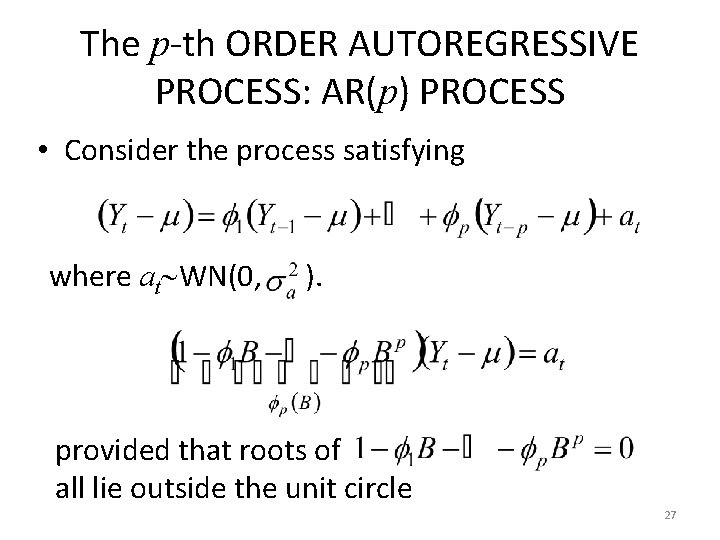
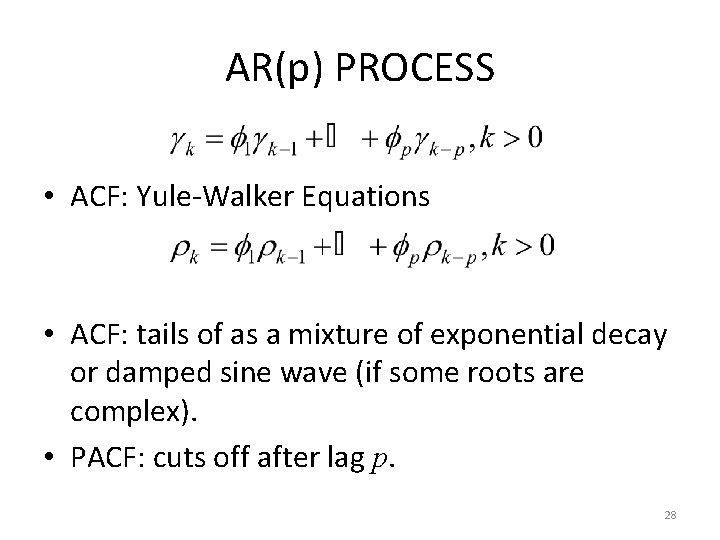
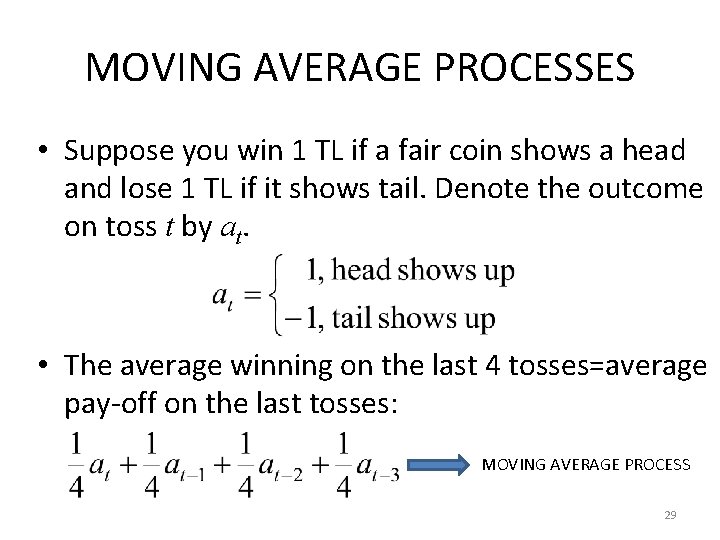
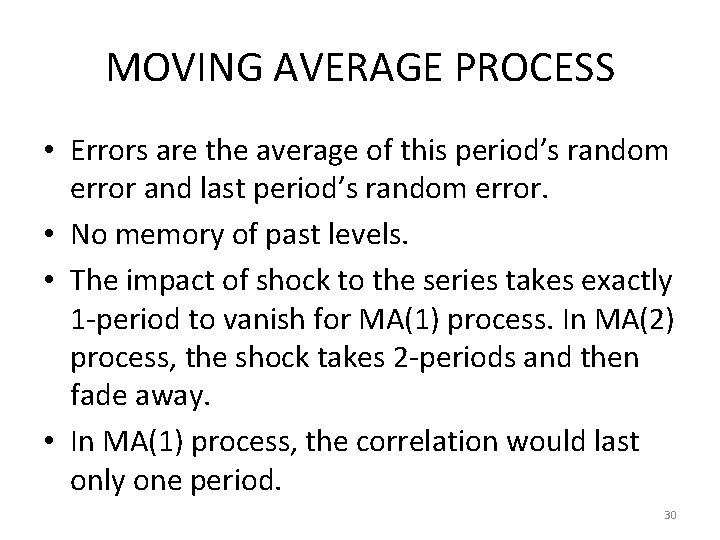
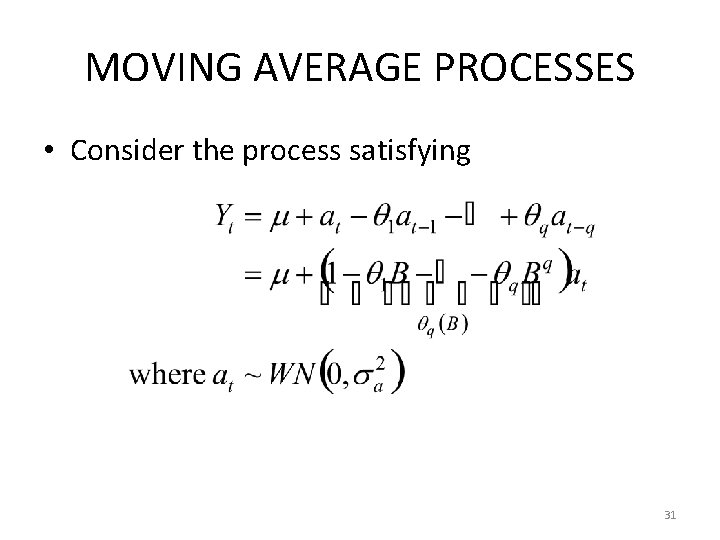
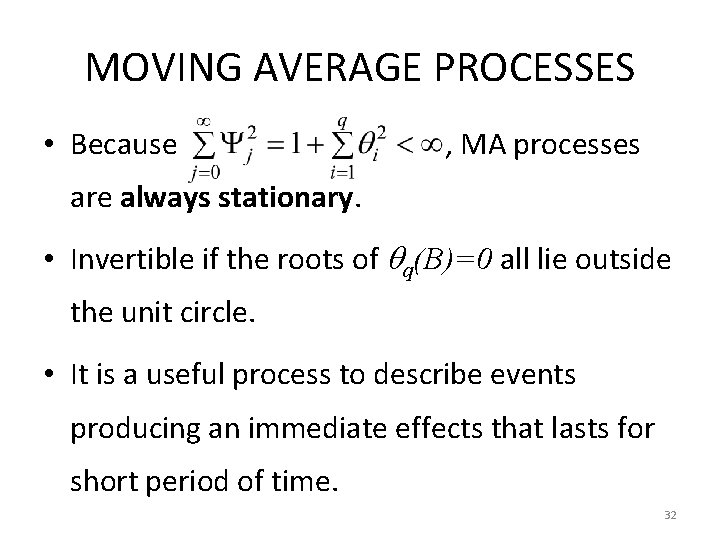
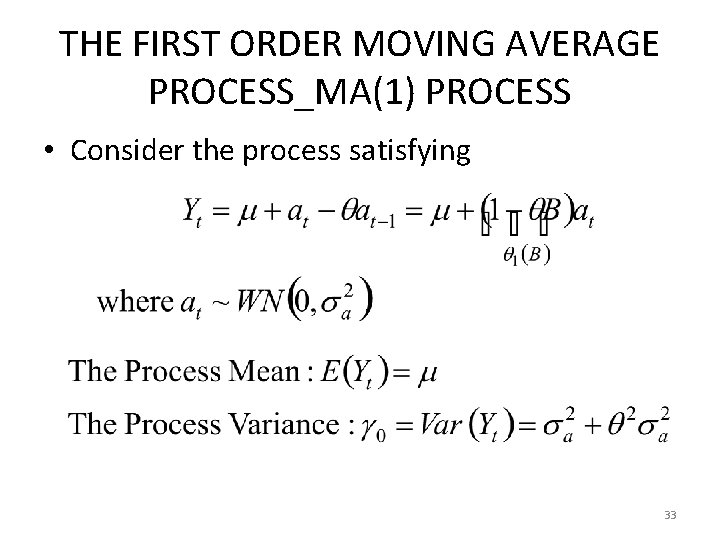
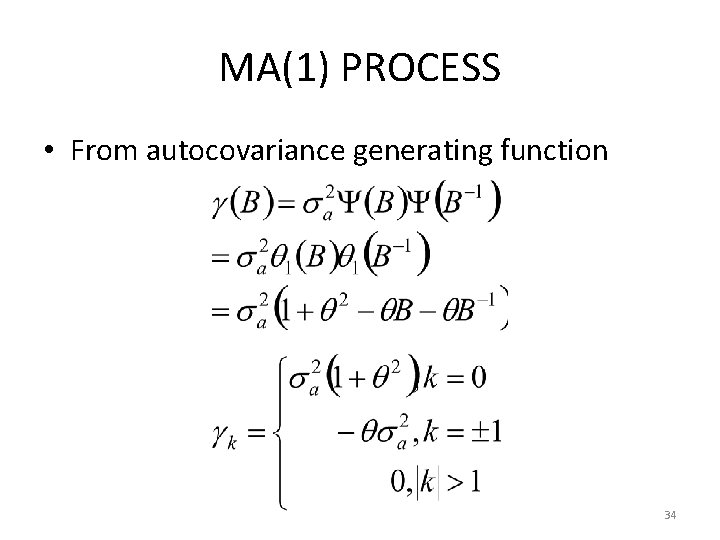
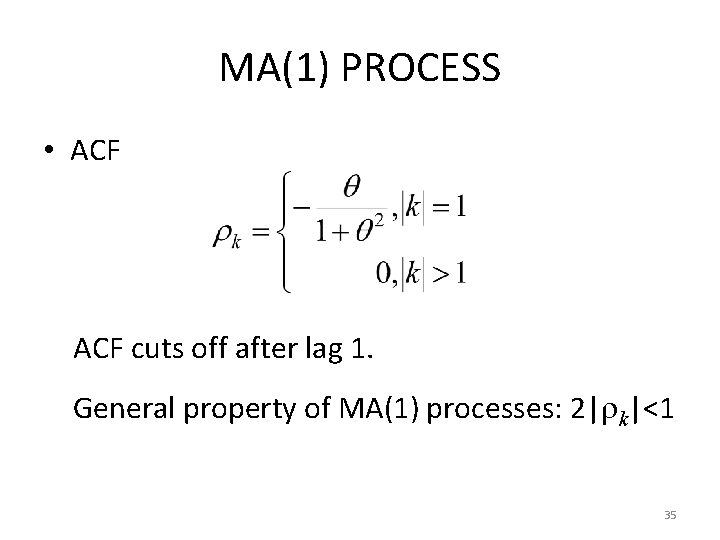
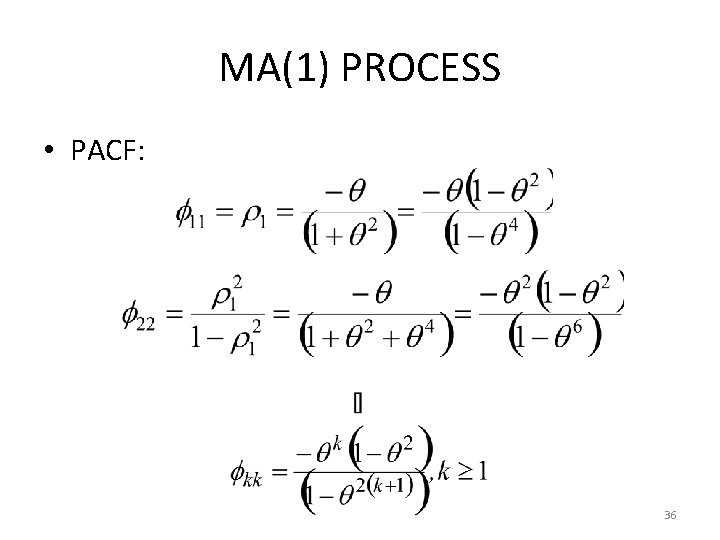
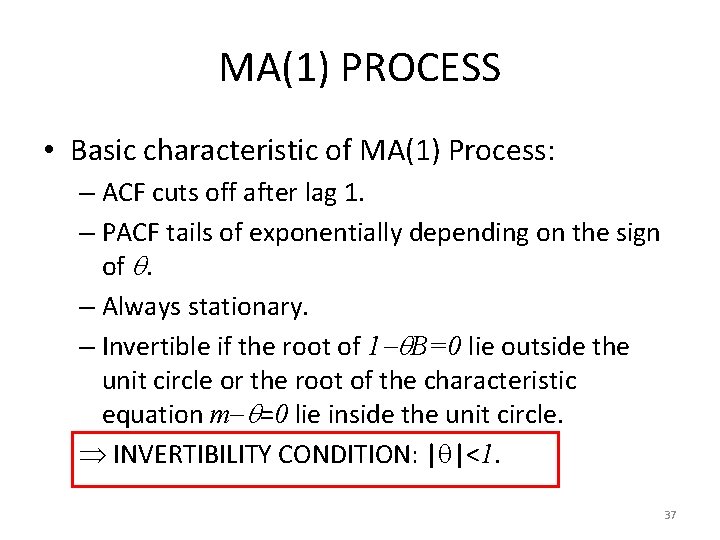
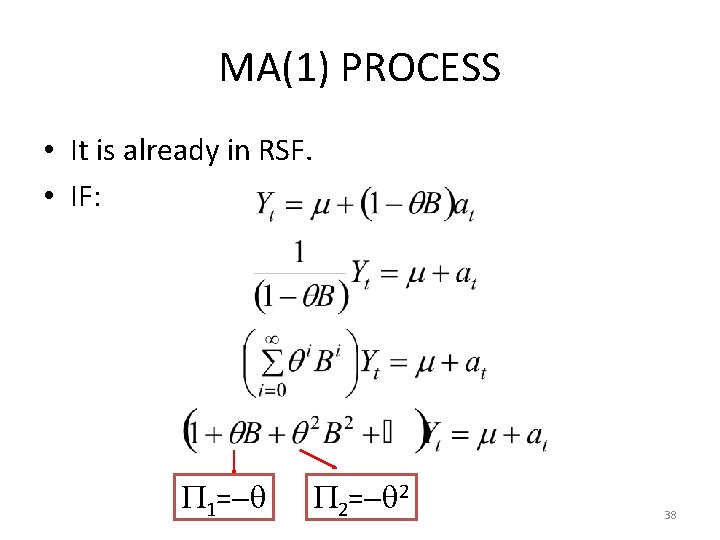
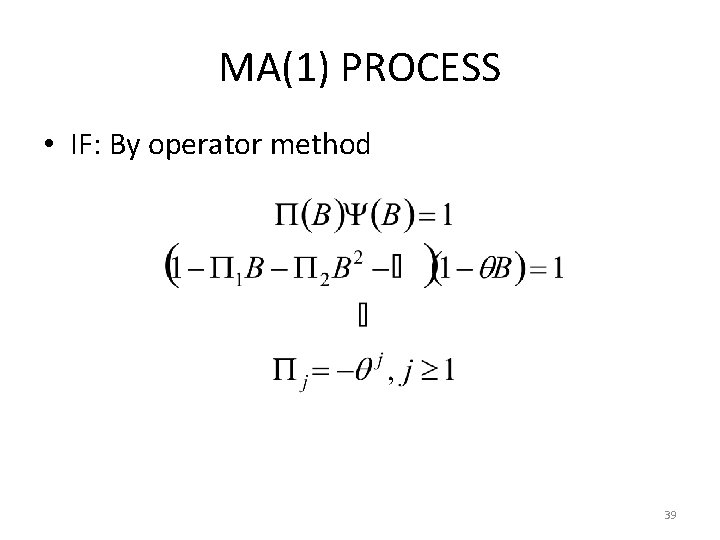
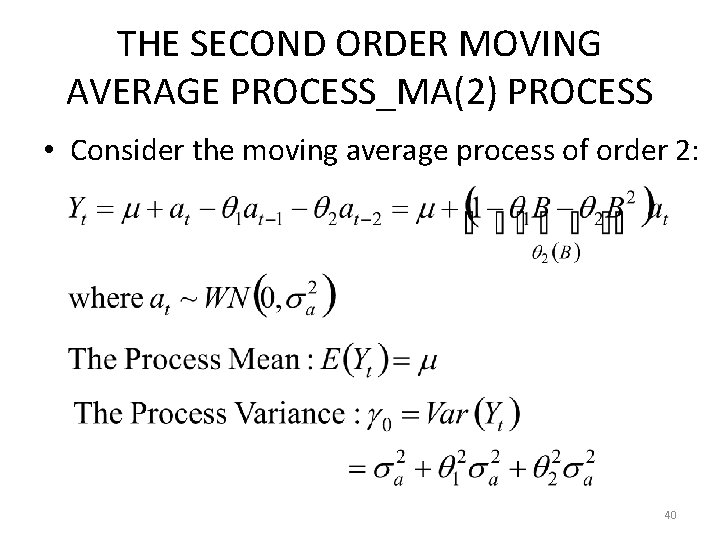
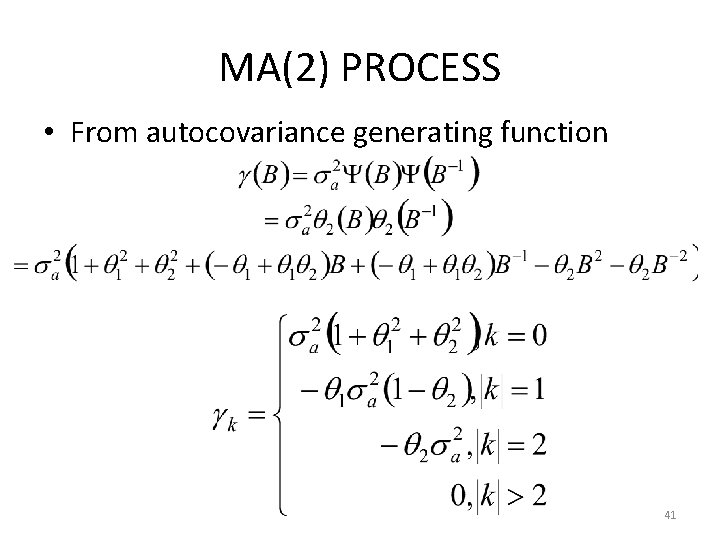
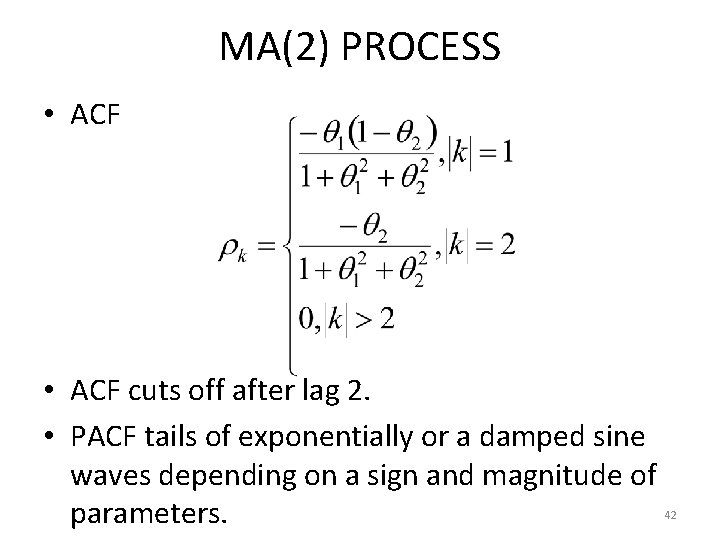
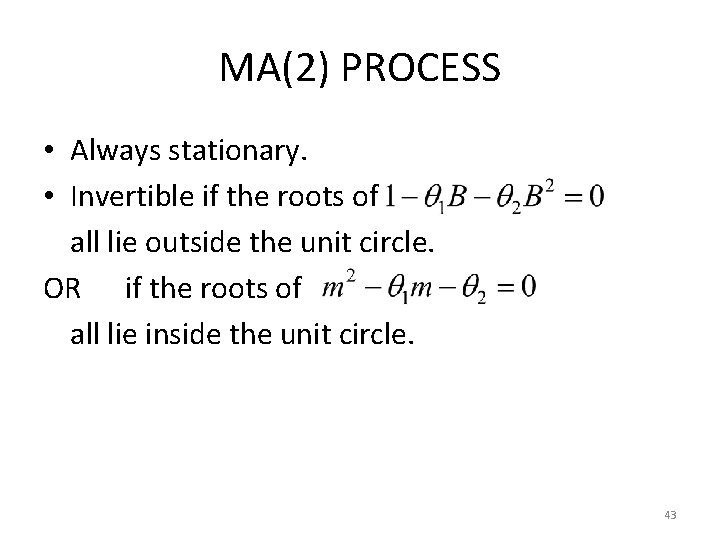
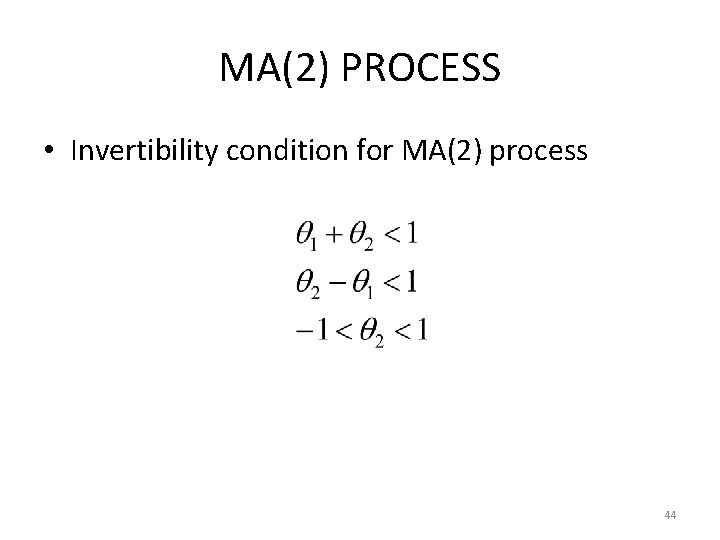
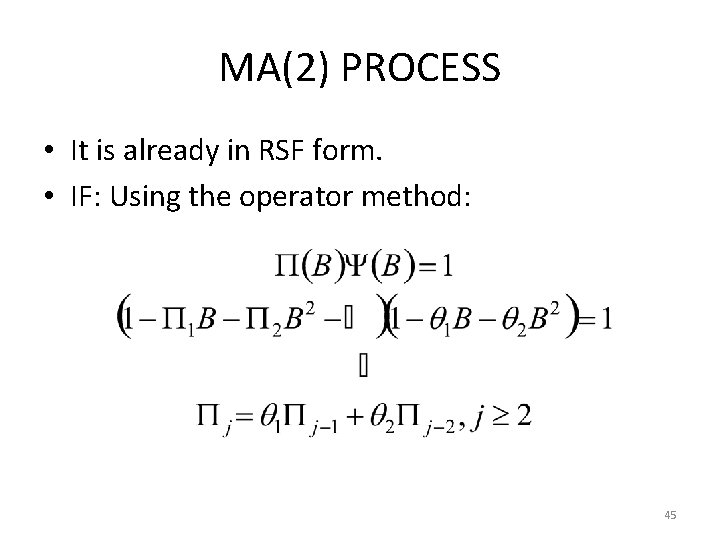
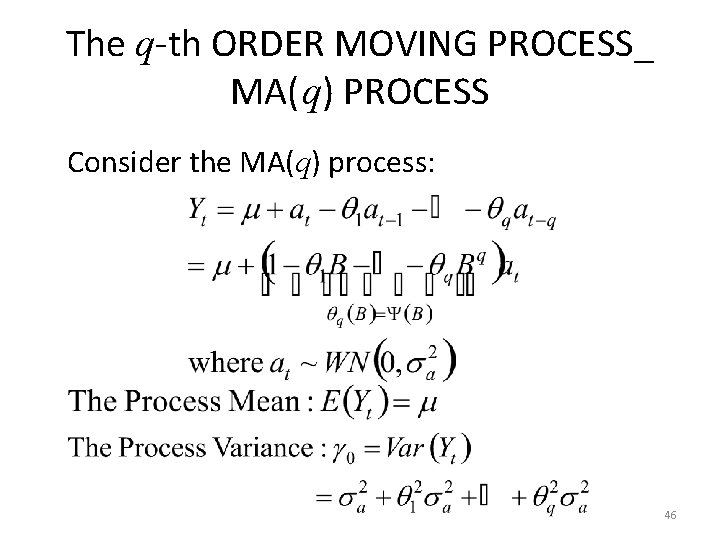
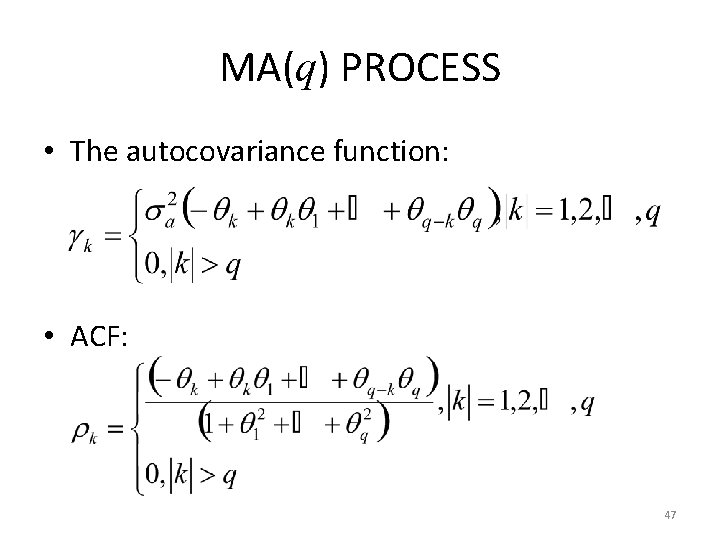
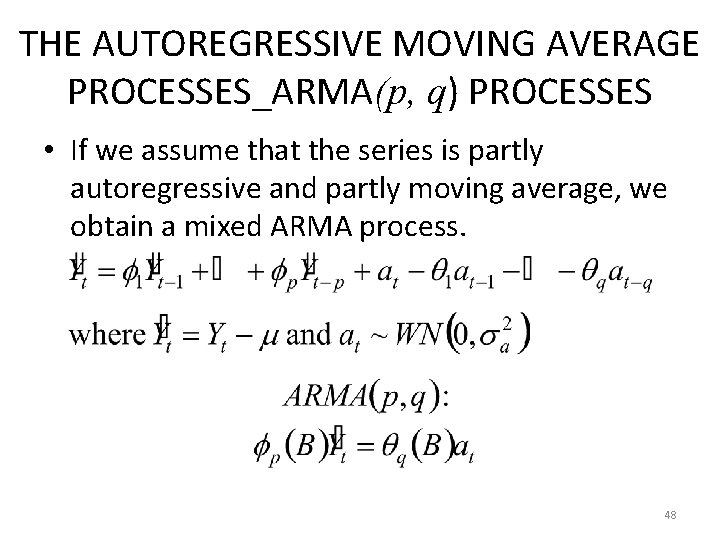
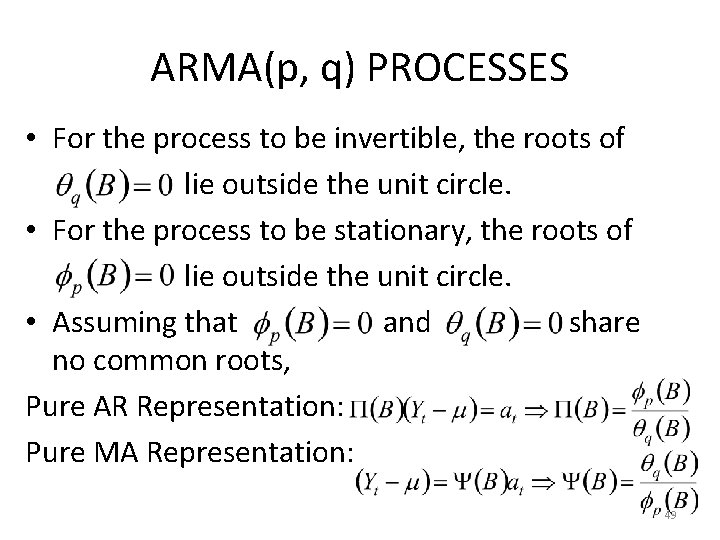
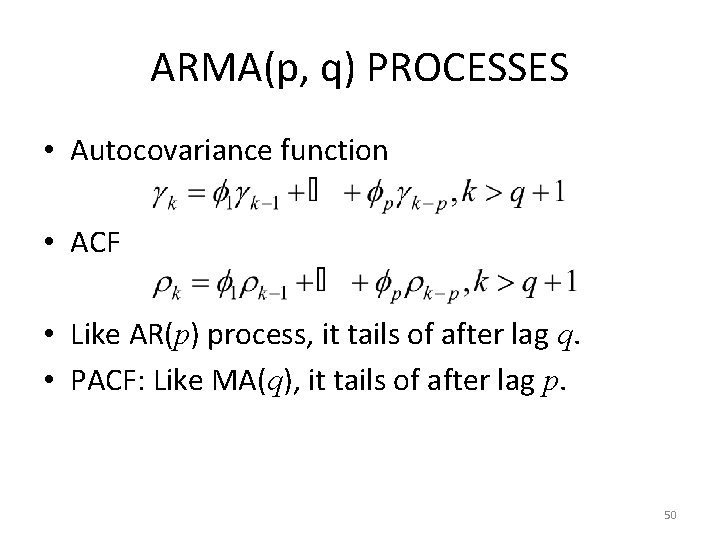
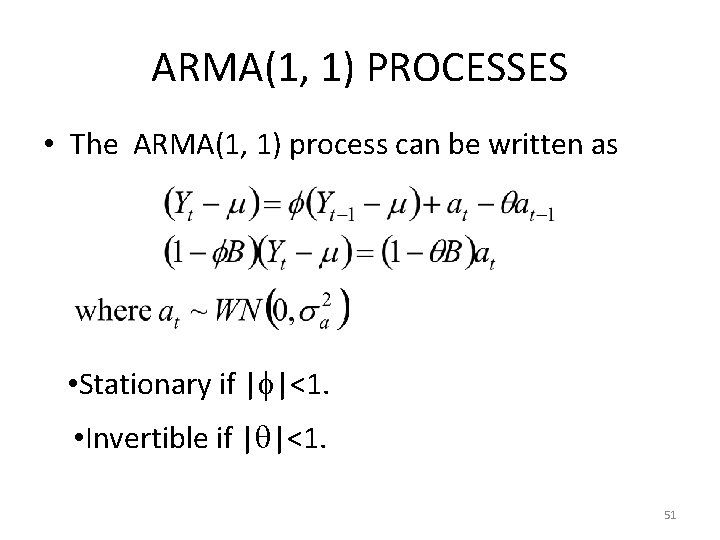
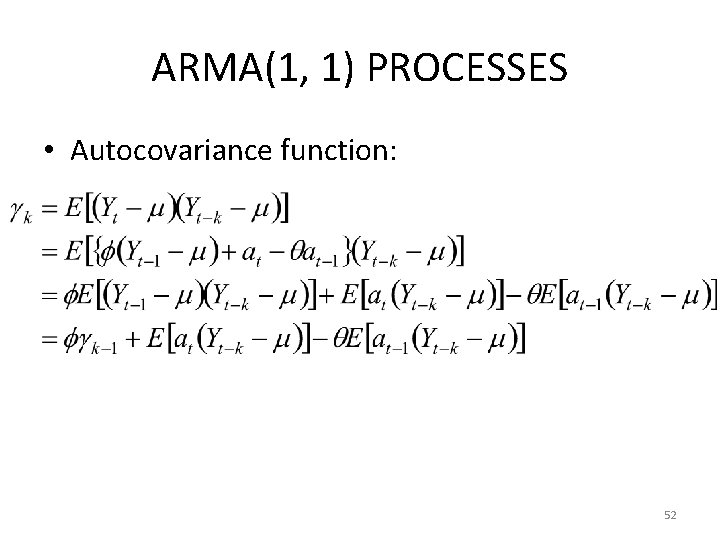
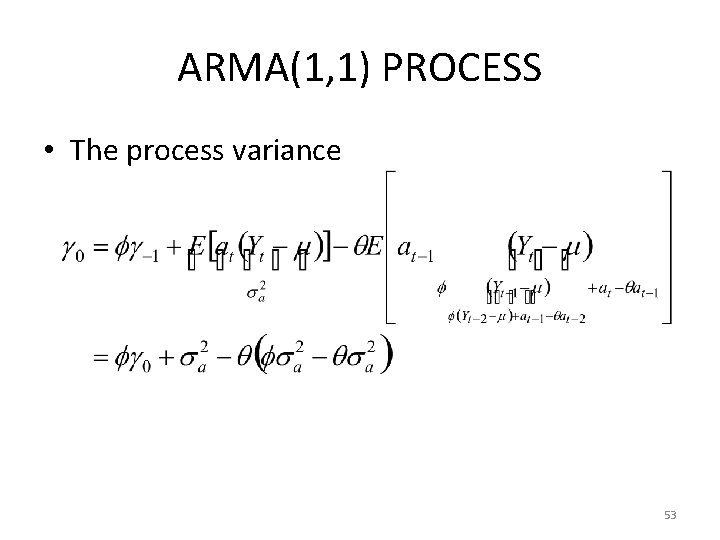
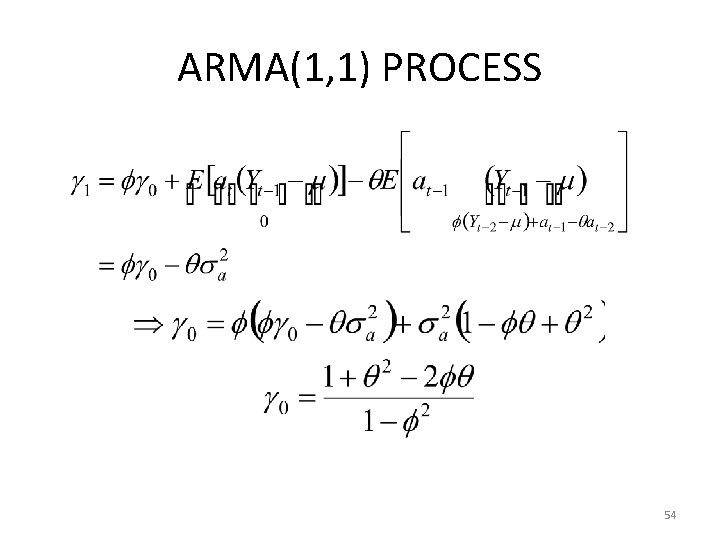
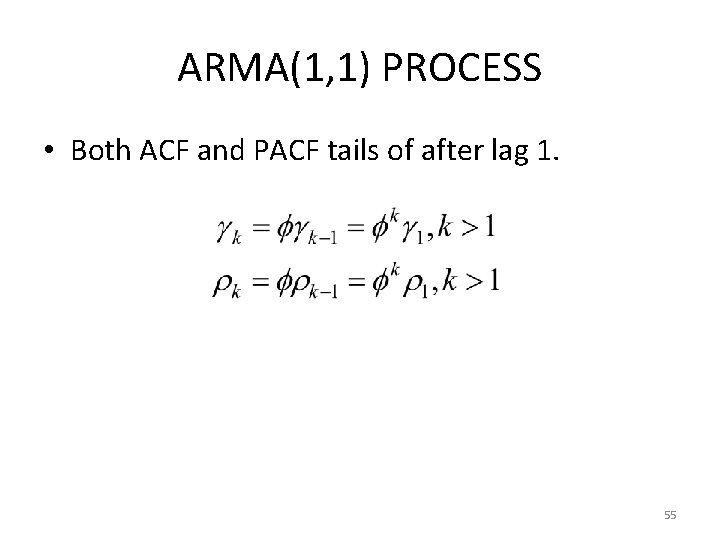
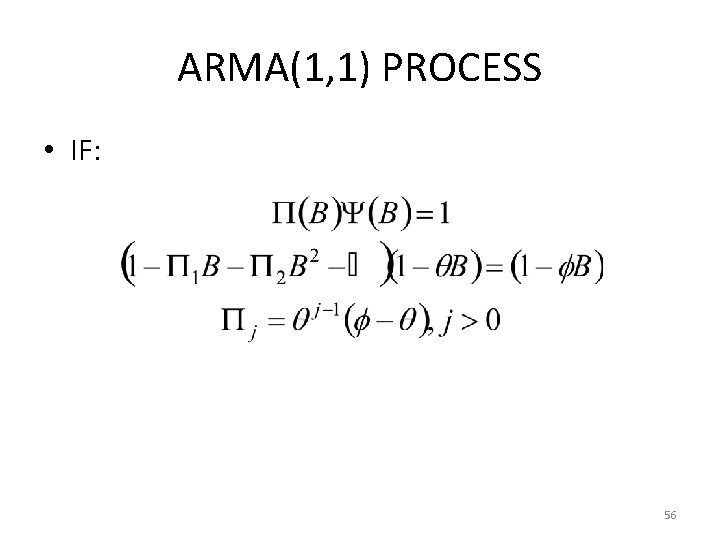
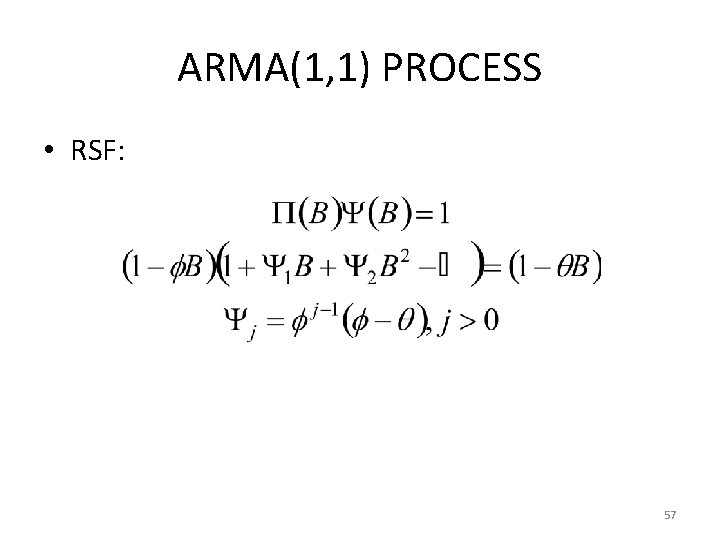
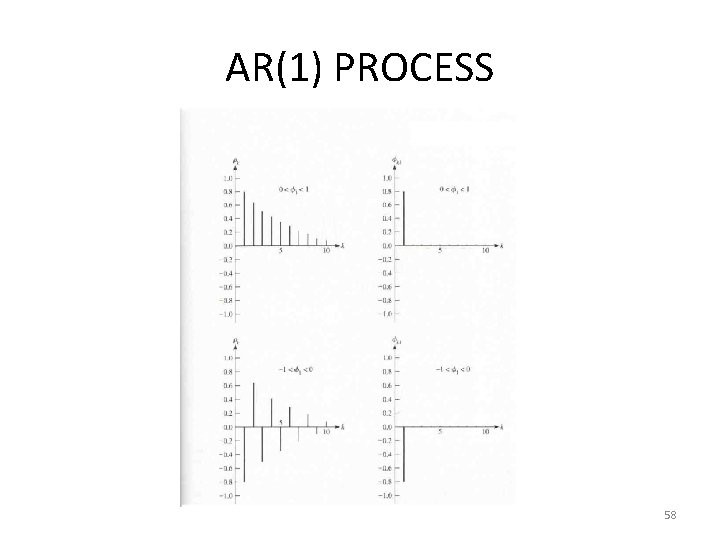
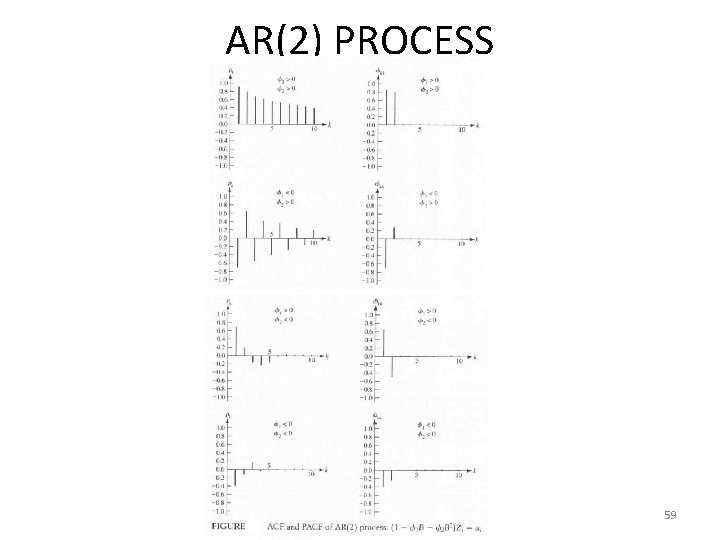
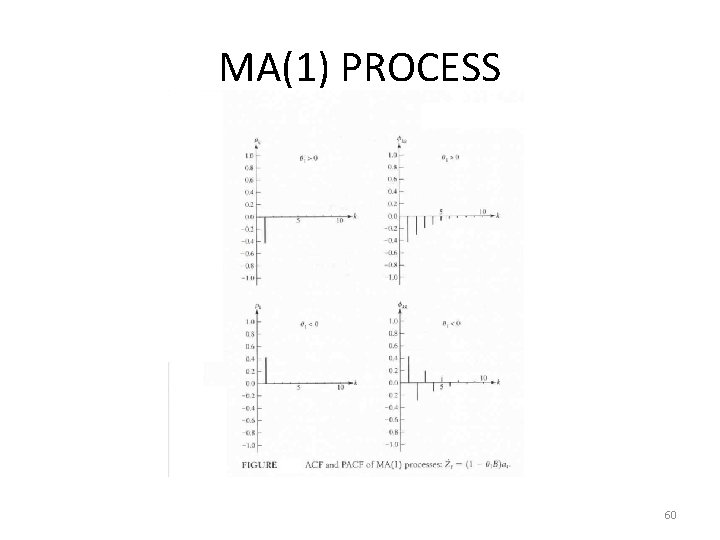
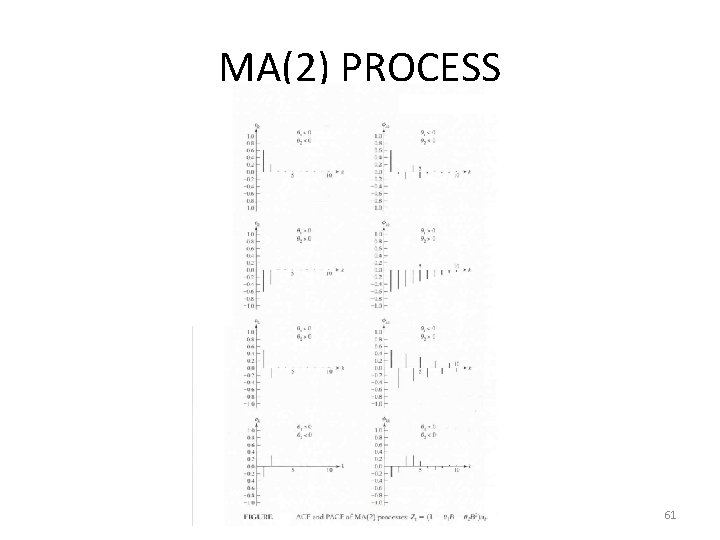
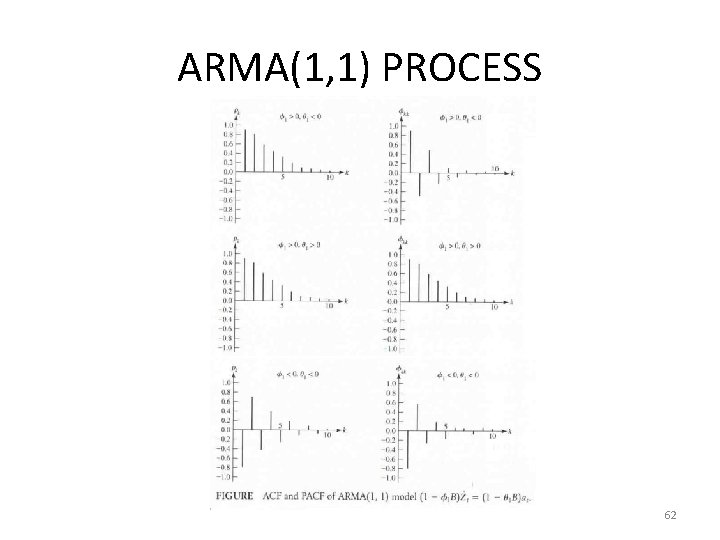
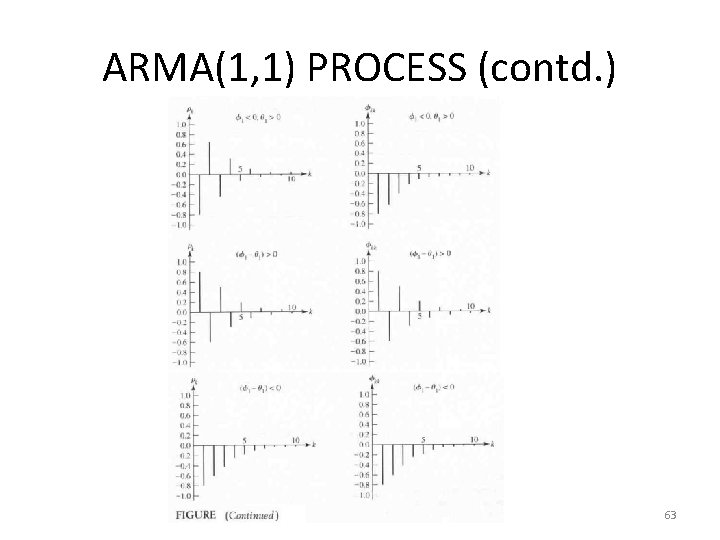
- Slides: 63
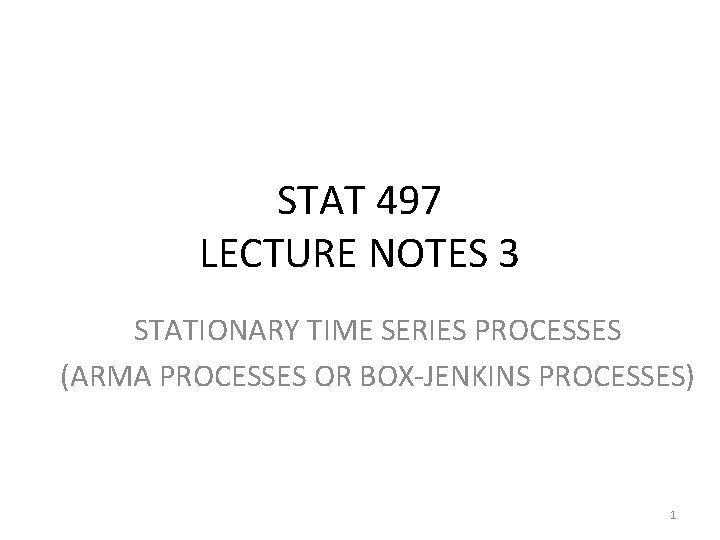
STAT 497 LECTURE NOTES 3 STATIONARY TIME SERIES PROCESSES (ARMA PROCESSES OR BOX-JENKINS PROCESSES) 1
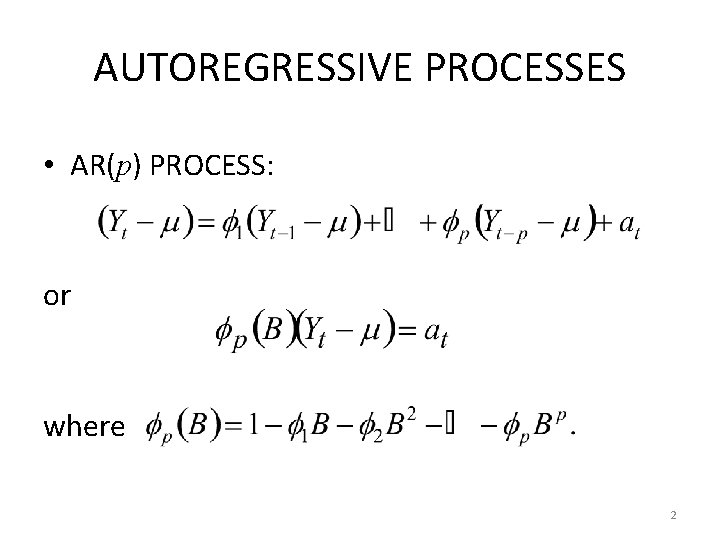
AUTOREGRESSIVE PROCESSES • AR(p) PROCESS: or where 2
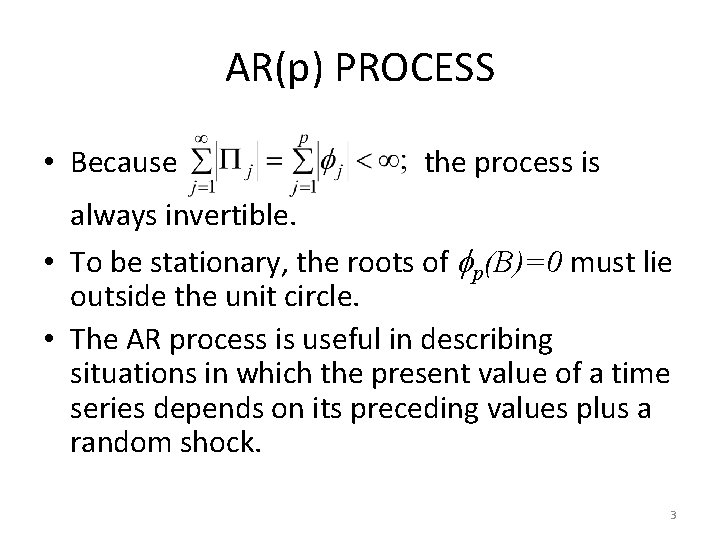
AR(p) PROCESS • Because the process is always invertible. • To be stationary, the roots of p(B)=0 must lie outside the unit circle. • The AR process is useful in describing situations in which the present value of a time series depends on its preceding values plus a random shock. 3
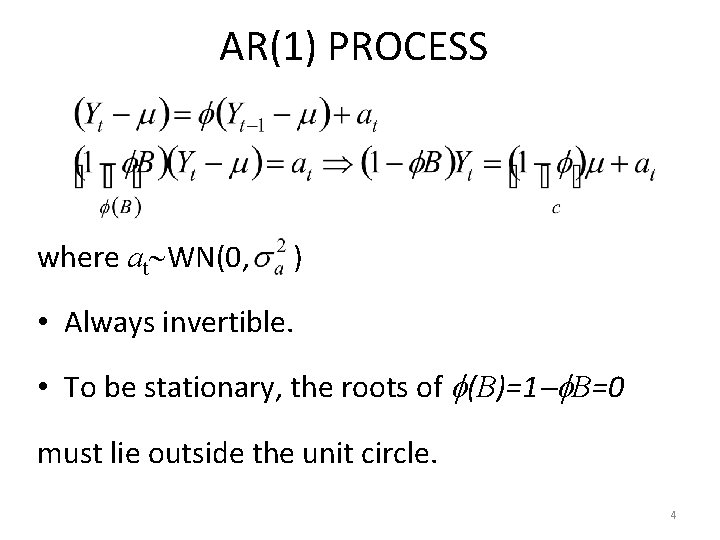
AR(1) PROCESS where at WN(0, ) • Always invertible. • To be stationary, the roots of (B)=1 B=0 must lie outside the unit circle. 4
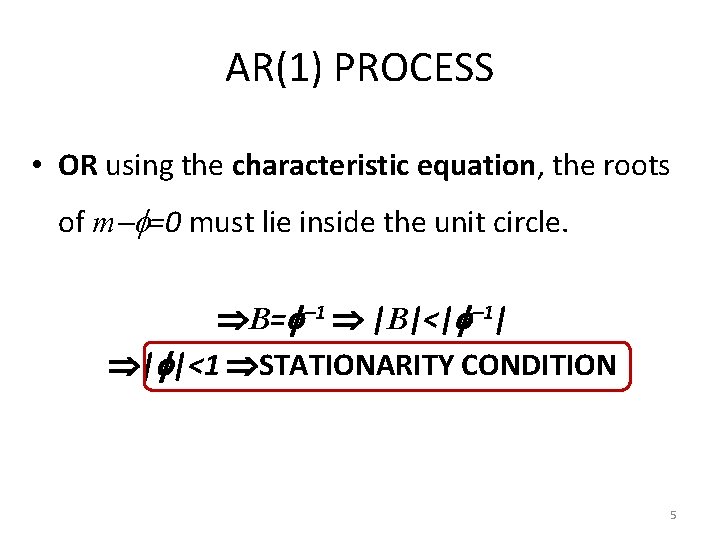
AR(1) PROCESS • OR using the characteristic equation, the roots of m =0 must lie inside the unit circle. B= 1 |B|<| 1| | |<1 STATIONARITY CONDITION 5
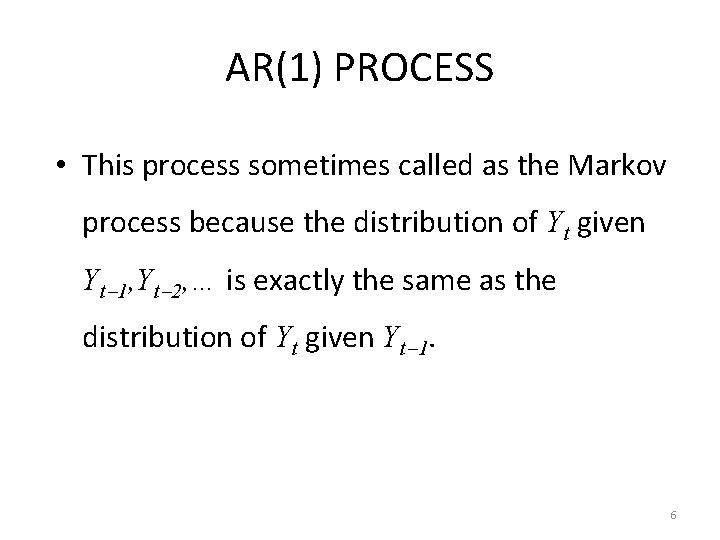
AR(1) PROCESS • This process sometimes called as the Markov process because the distribution of Yt given Yt 1, Yt 2, … is exactly the same as the distribution of Yt given Yt 1. 6
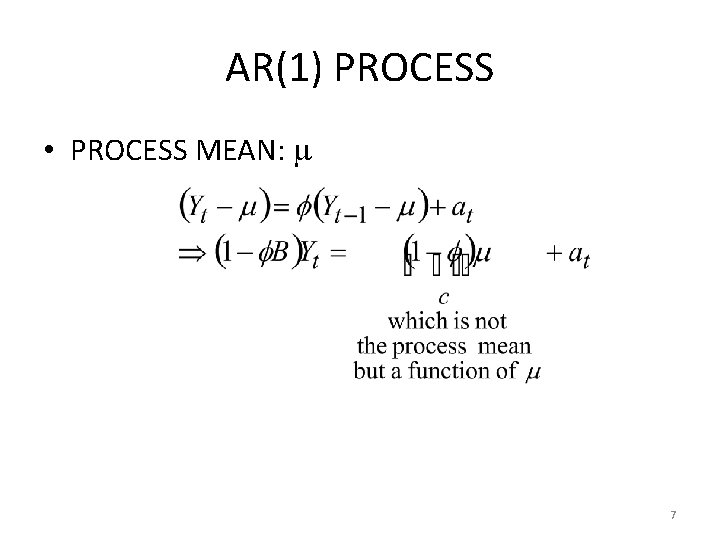
AR(1) PROCESS • PROCESS MEAN: 7
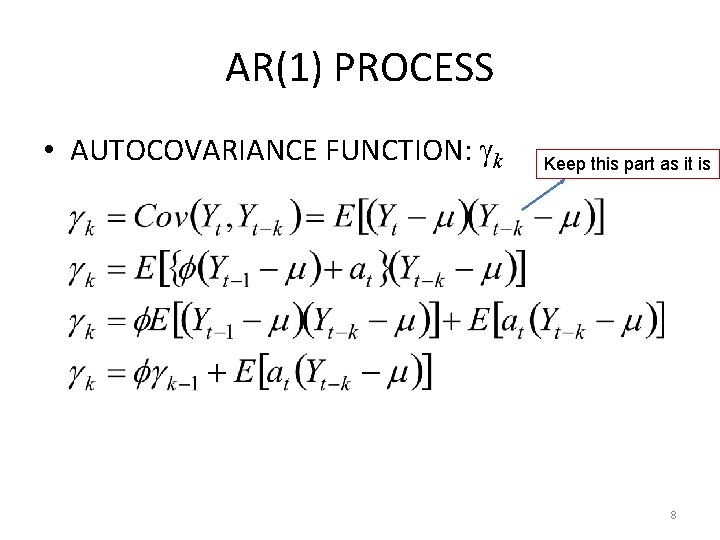
AR(1) PROCESS • AUTOCOVARIANCE FUNCTION: k Keep this part as it is 8
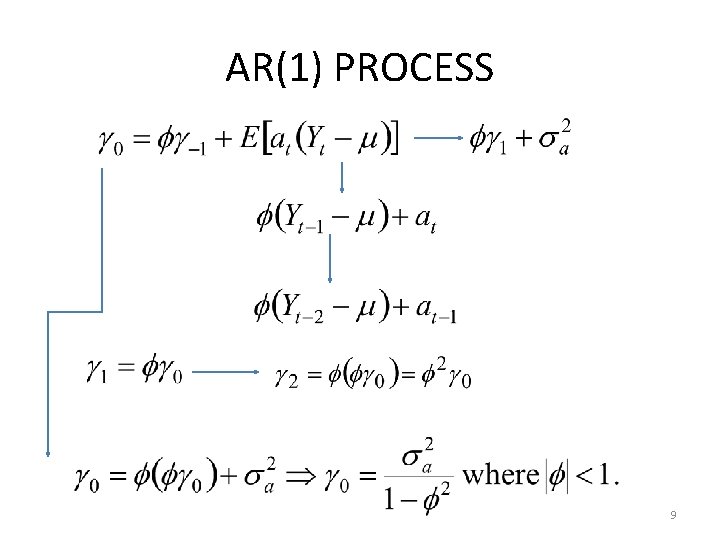
AR(1) PROCESS 9
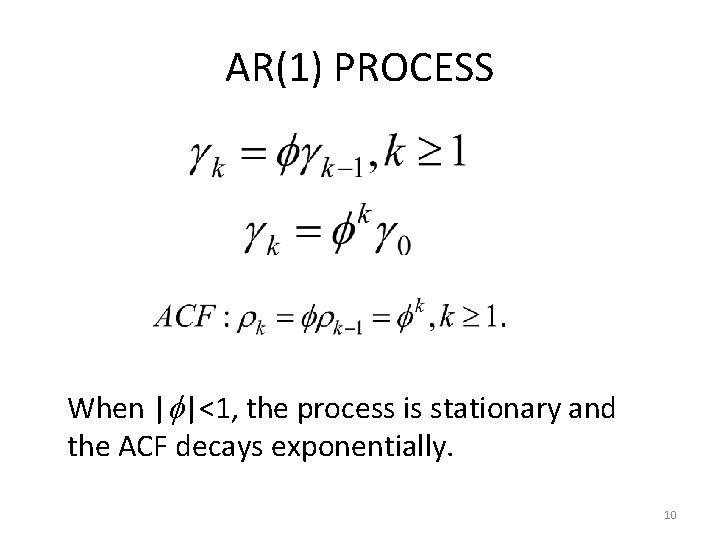
AR(1) PROCESS When | |<1, the process is stationary and the ACF decays exponentially. 10
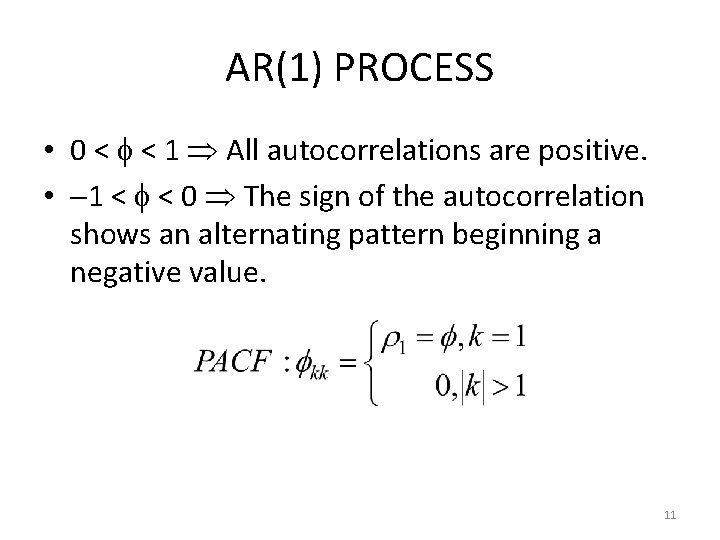
AR(1) PROCESS • 0 < < 1 All autocorrelations are positive. • 1 < < 0 The sign of the autocorrelation shows an alternating pattern beginning a negative value. 11
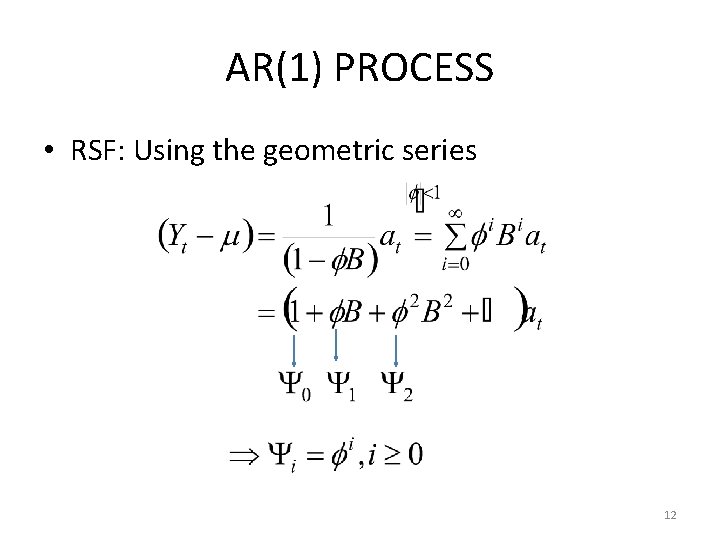
AR(1) PROCESS • RSF: Using the geometric series 12
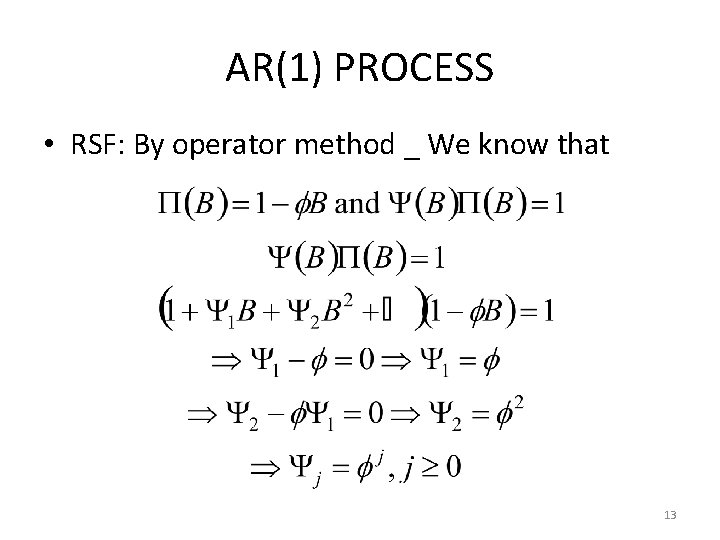
AR(1) PROCESS • RSF: By operator method _ We know that 13
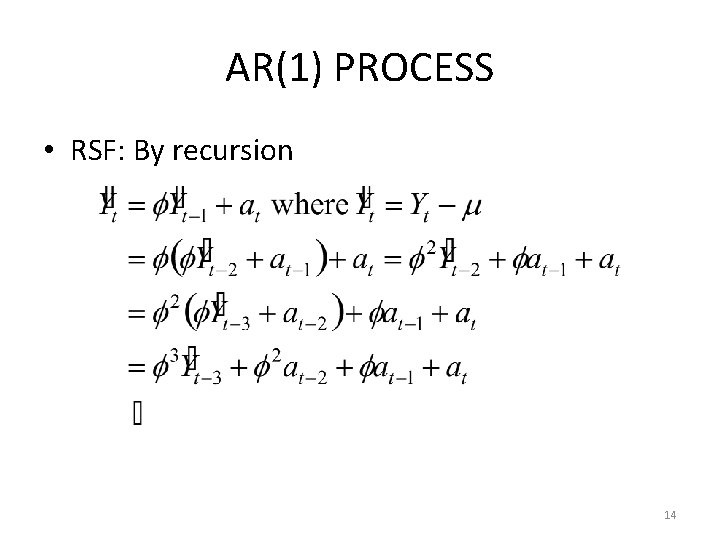
AR(1) PROCESS • RSF: By recursion 14
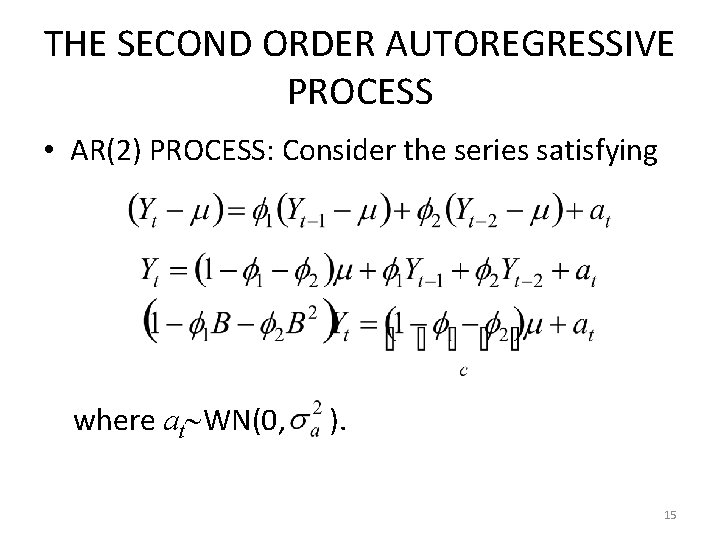
THE SECOND ORDER AUTOREGRESSIVE PROCESS • AR(2) PROCESS: Consider the series satisfying where at WN(0, ). 15
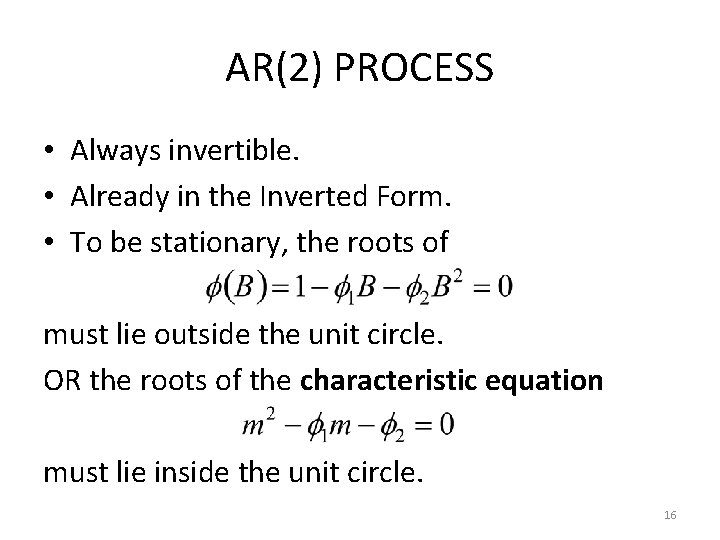
AR(2) PROCESS • Always invertible. • Already in the Inverted Form. • To be stationary, the roots of must lie outside the unit circle. OR the roots of the characteristic equation must lie inside the unit circle. 16
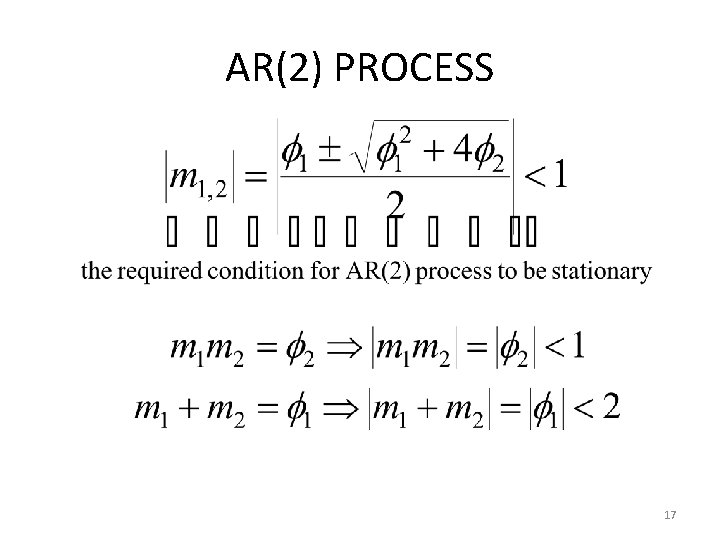
AR(2) PROCESS 17
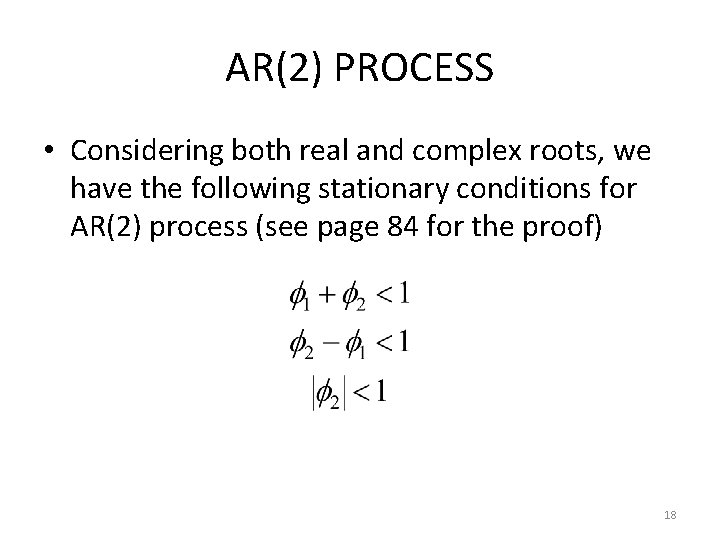
AR(2) PROCESS • Considering both real and complex roots, we have the following stationary conditions for AR(2) process (see page 84 for the proof) 18
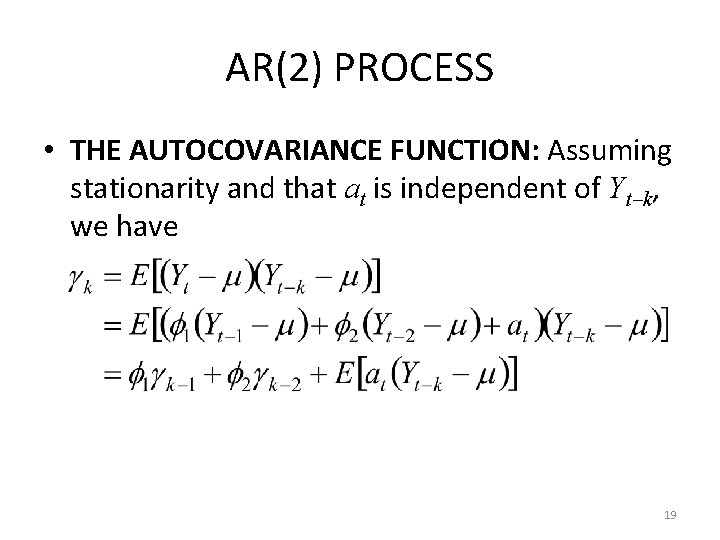
AR(2) PROCESS • THE AUTOCOVARIANCE FUNCTION: Assuming stationarity and that at is independent of Yt k, we have 19
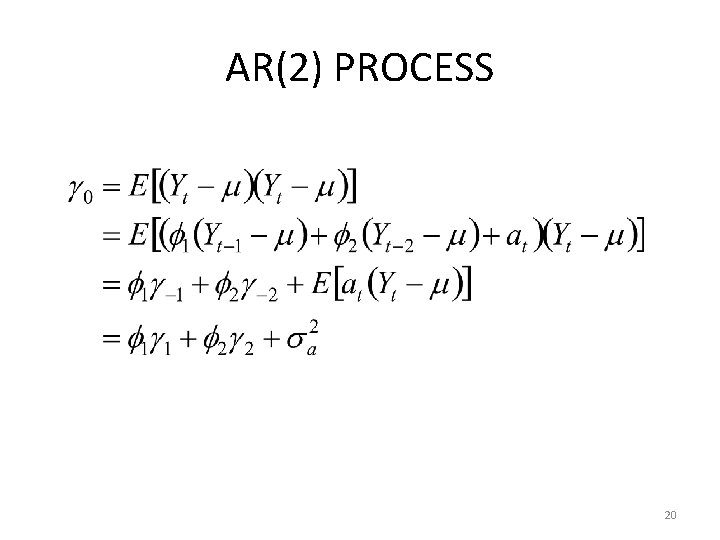
AR(2) PROCESS 20
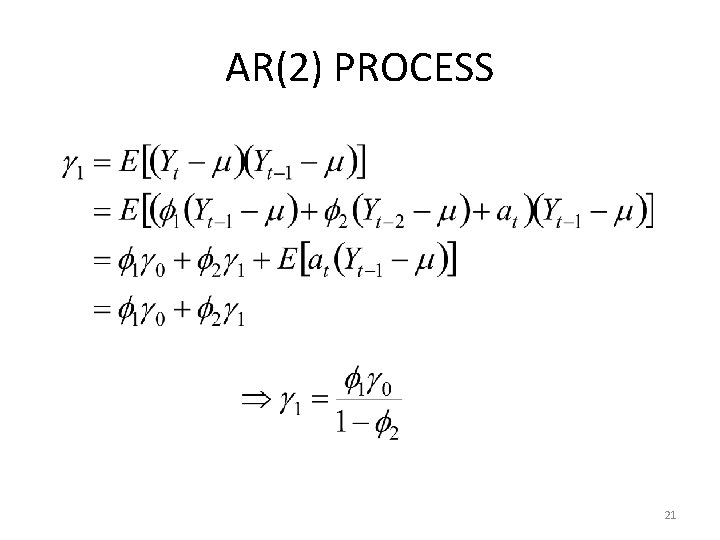
AR(2) PROCESS 21
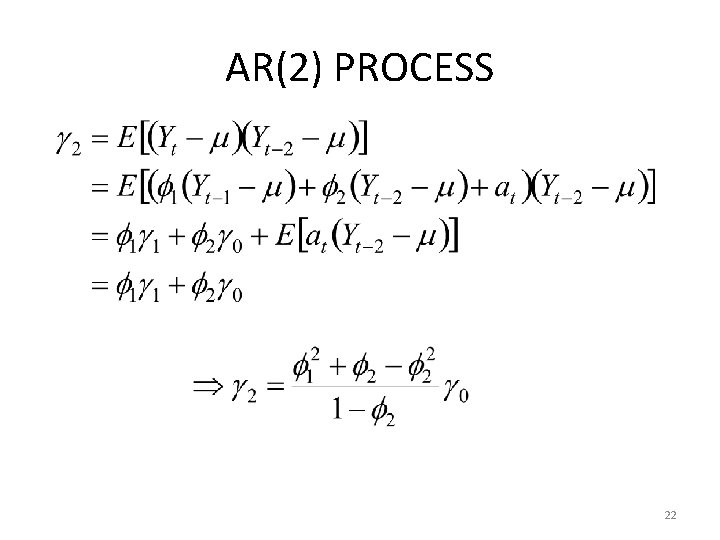
AR(2) PROCESS 22
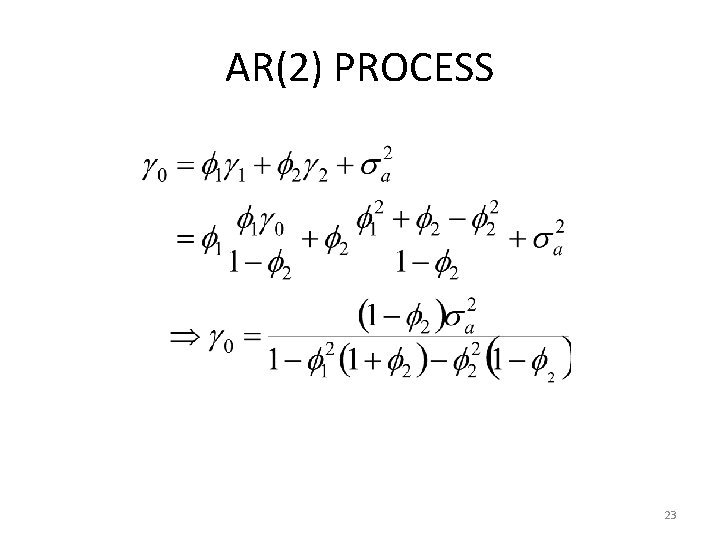
AR(2) PROCESS 23
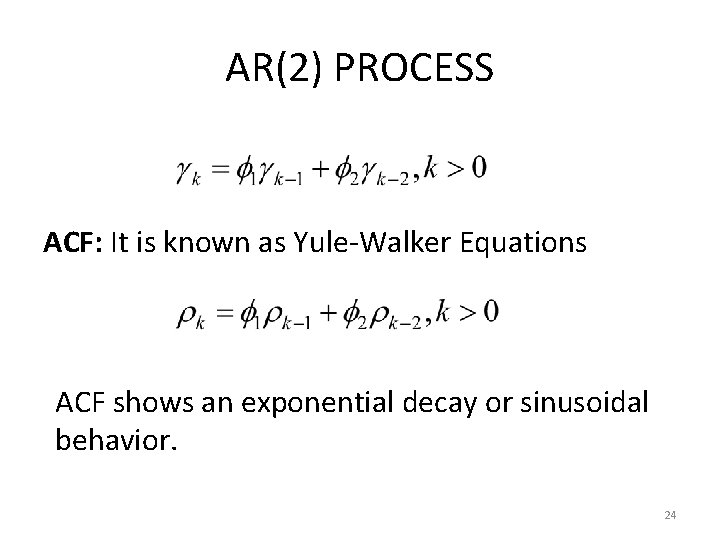
AR(2) PROCESS ACF: It is known as Yule-Walker Equations ACF shows an exponential decay or sinusoidal behavior. 24
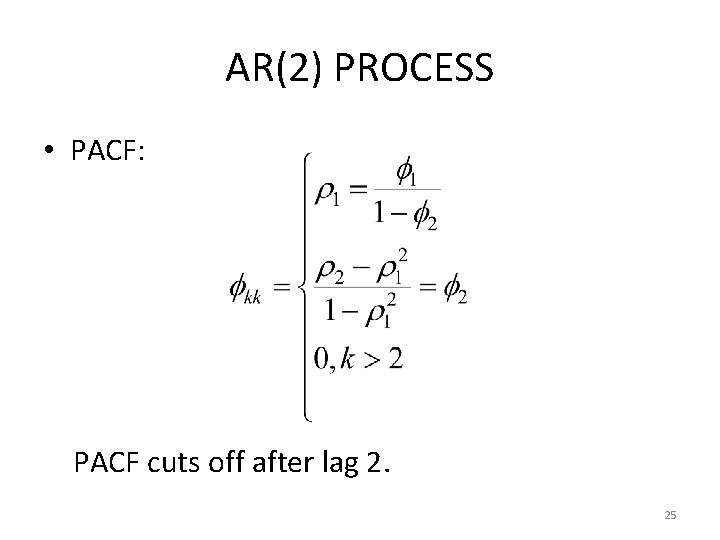
AR(2) PROCESS • PACF: PACF cuts off after lag 2. 25
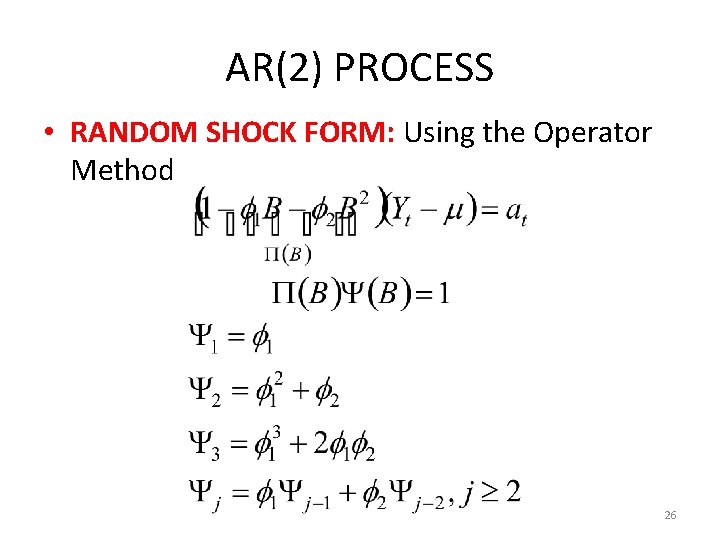
AR(2) PROCESS • RANDOM SHOCK FORM: Using the Operator Method 26
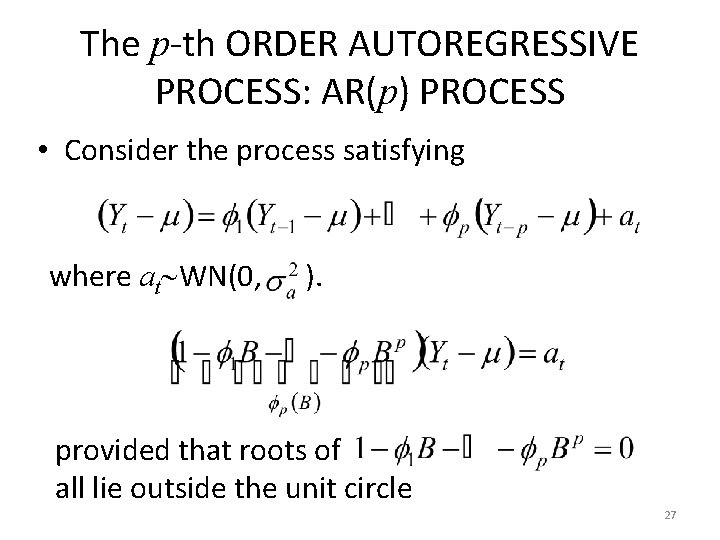
The p-th ORDER AUTOREGRESSIVE PROCESS: AR(p) PROCESS • Consider the process satisfying where at WN(0, ). provided that roots of all lie outside the unit circle 27
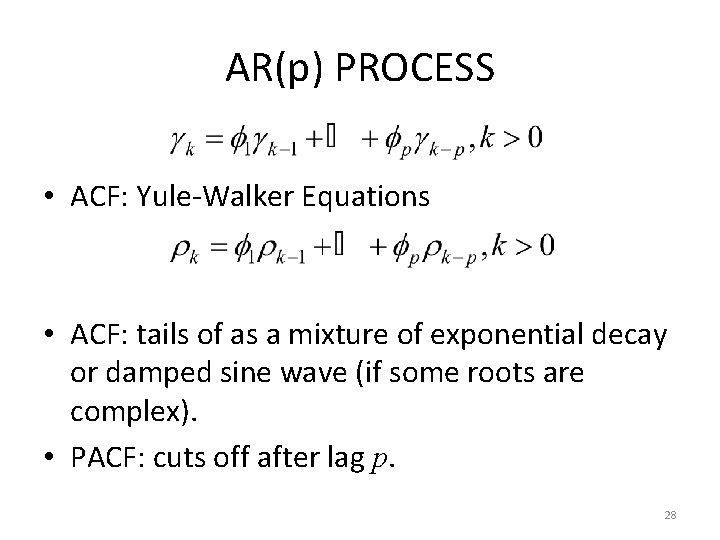
AR(p) PROCESS • ACF: Yule-Walker Equations • ACF: tails of as a mixture of exponential decay or damped sine wave (if some roots are complex). • PACF: cuts off after lag p. 28
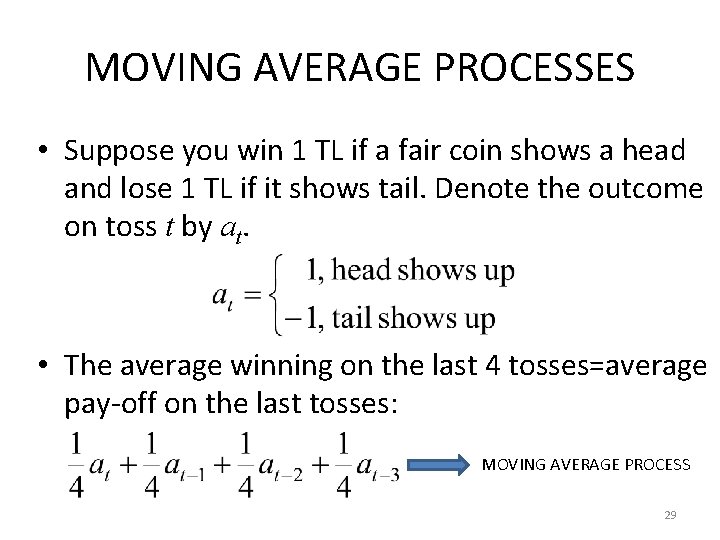
MOVING AVERAGE PROCESSES • Suppose you win 1 TL if a fair coin shows a head and lose 1 TL if it shows tail. Denote the outcome on toss t by at. • The average winning on the last 4 tosses=average pay-off on the last tosses: MOVING AVERAGE PROCESS 29
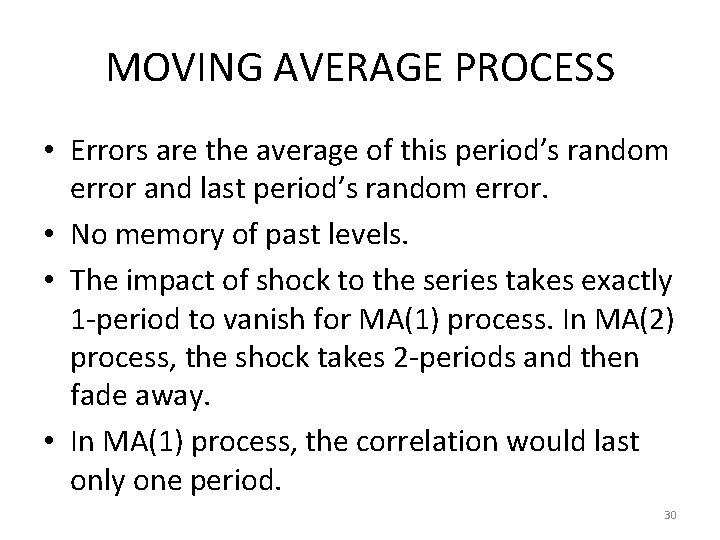
MOVING AVERAGE PROCESS • Errors are the average of this period’s random error and last period’s random error. • No memory of past levels. • The impact of shock to the series takes exactly 1 -period to vanish for MA(1) process. In MA(2) process, the shock takes 2 -periods and then fade away. • In MA(1) process, the correlation would last only one period. 30
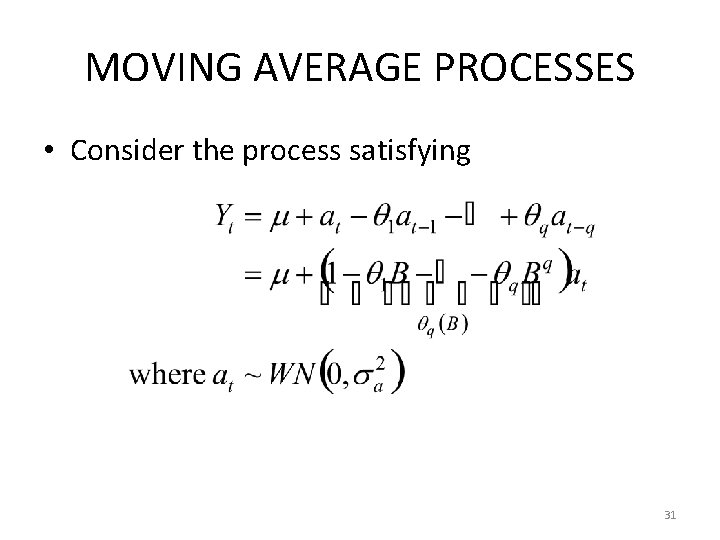
MOVING AVERAGE PROCESSES • Consider the process satisfying 31
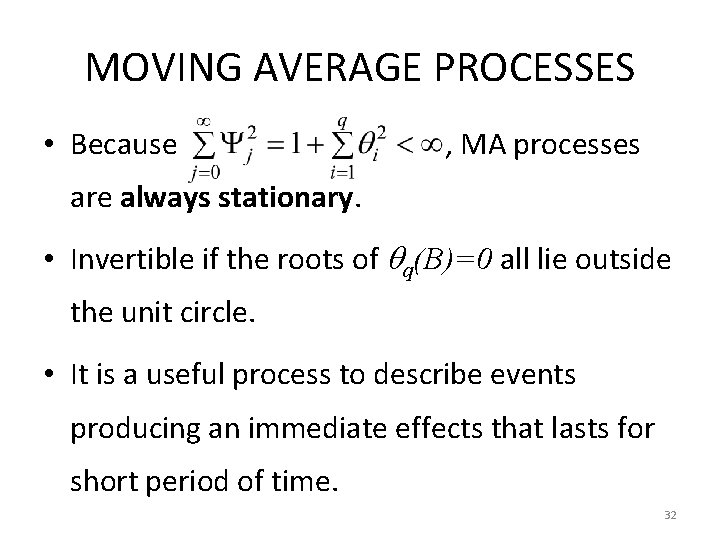
MOVING AVERAGE PROCESSES • Because , MA processes are always stationary. • Invertible if the roots of q(B)=0 all lie outside the unit circle. • It is a useful process to describe events producing an immediate effects that lasts for short period of time. 32
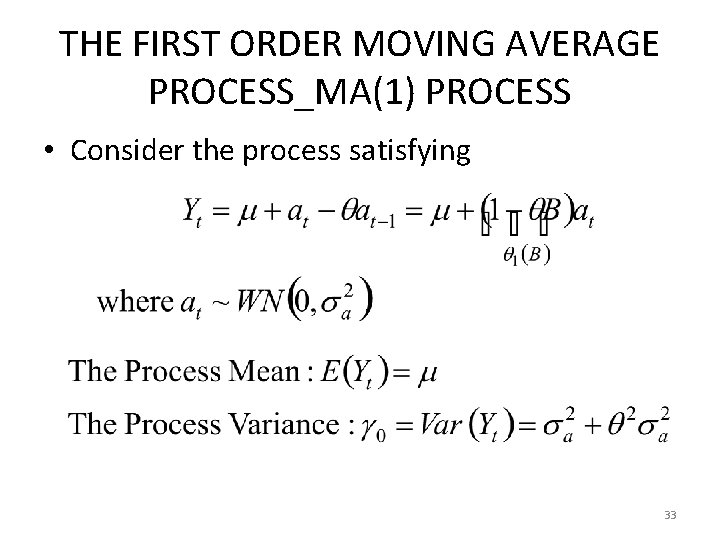
THE FIRST ORDER MOVING AVERAGE PROCESS_MA(1) PROCESS • Consider the process satisfying 33
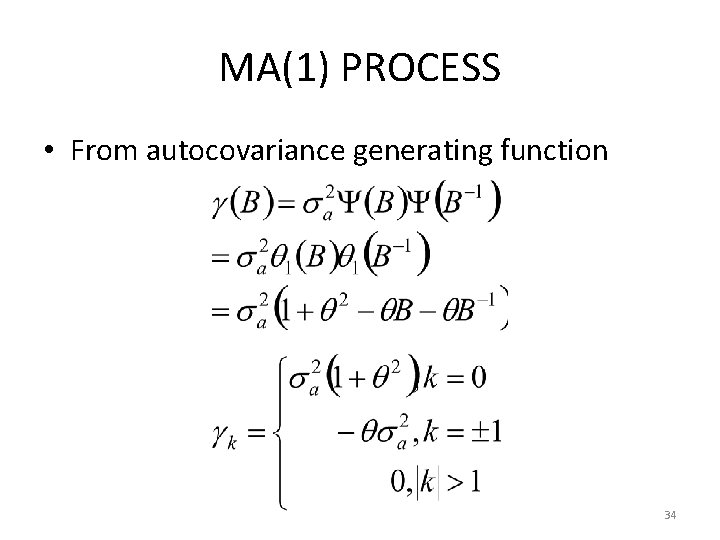
MA(1) PROCESS • From autocovariance generating function 34
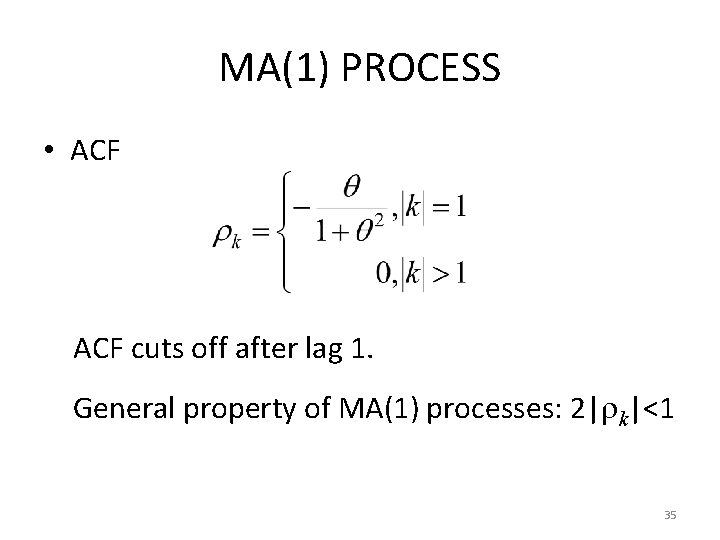
MA(1) PROCESS • ACF cuts off after lag 1. General property of MA(1) processes: 2| k|<1 35
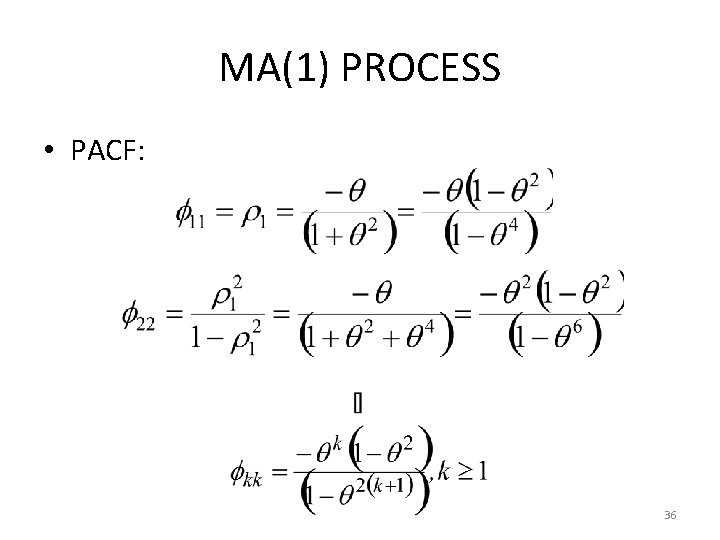
MA(1) PROCESS • PACF: 36
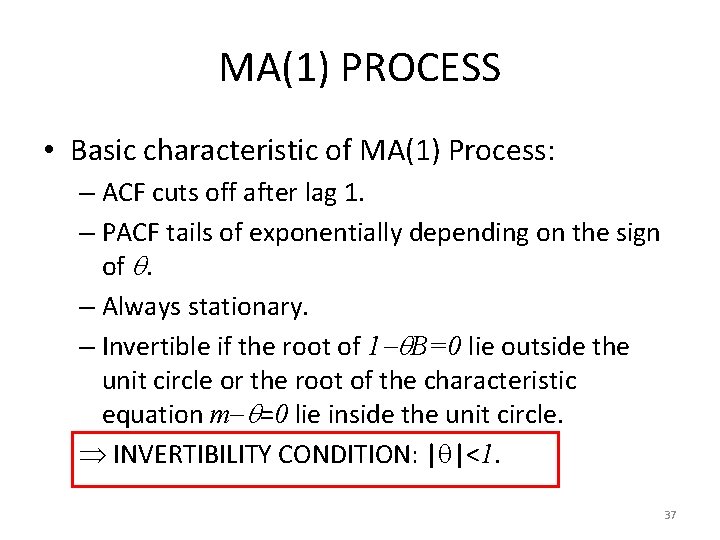
MA(1) PROCESS • Basic characteristic of MA(1) Process: – ACF cuts off after lag 1. – PACF tails of exponentially depending on the sign of . – Always stationary. – Invertible if the root of 1 B=0 lie outside the unit circle or the root of the characteristic equation m =0 lie inside the unit circle. INVERTIBILITY CONDITION: | |<1. 37
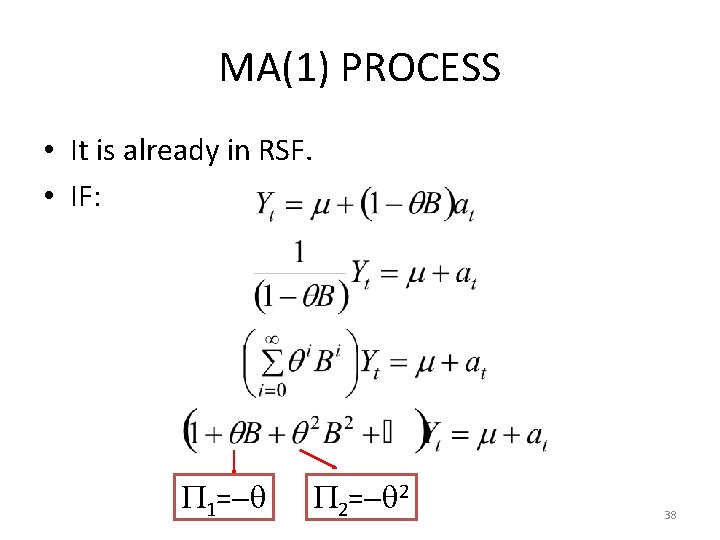
MA(1) PROCESS • It is already in RSF. • IF: 1= 2= 2 38
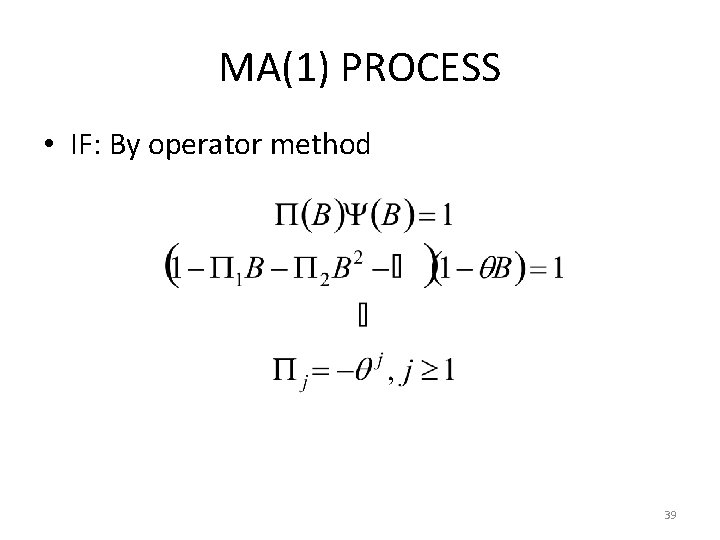
MA(1) PROCESS • IF: By operator method 39
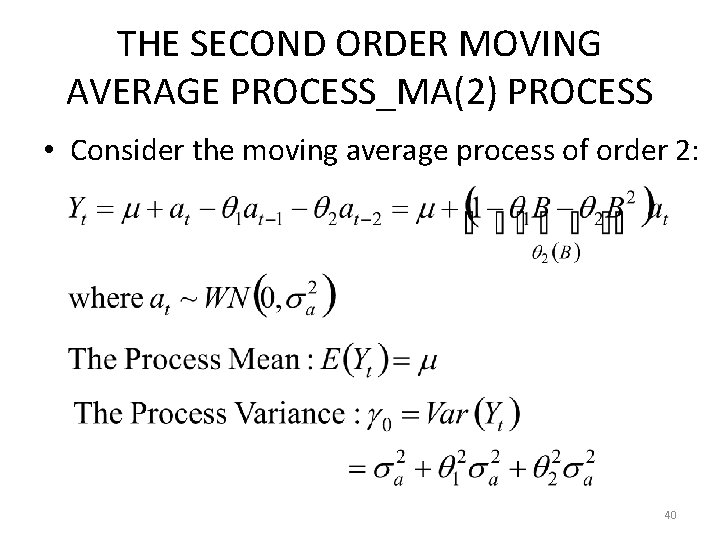
THE SECOND ORDER MOVING AVERAGE PROCESS_MA(2) PROCESS • Consider the moving average process of order 2: 40
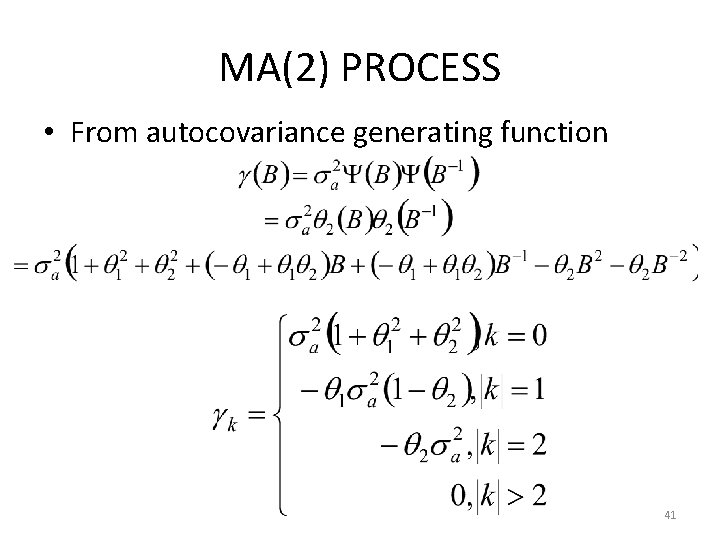
MA(2) PROCESS • From autocovariance generating function 41
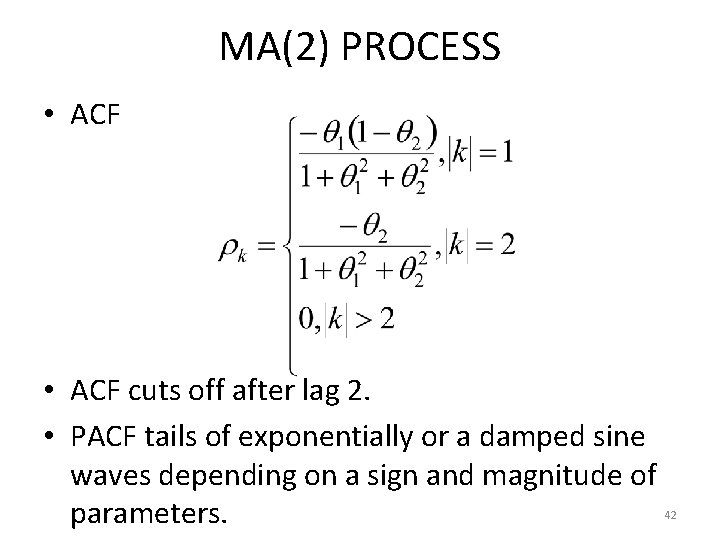
MA(2) PROCESS • ACF cuts off after lag 2. • PACF tails of exponentially or a damped sine waves depending on a sign and magnitude of parameters. 42
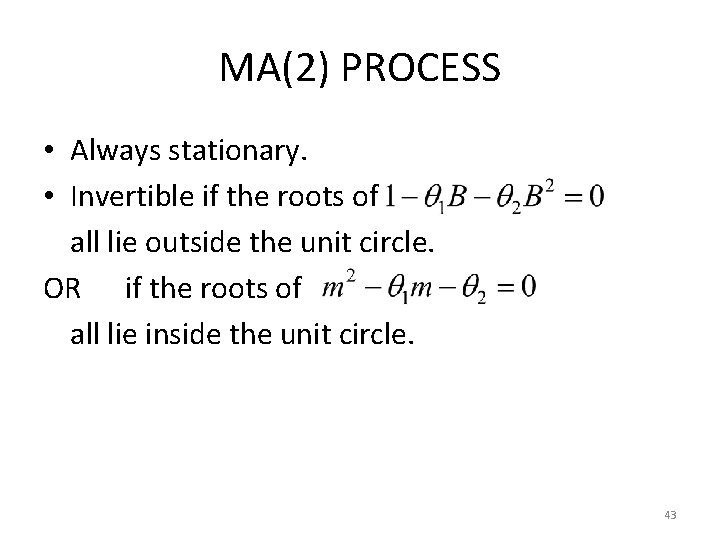
MA(2) PROCESS • Always stationary. • Invertible if the roots of all lie outside the unit circle. OR if the roots of all lie inside the unit circle. 43
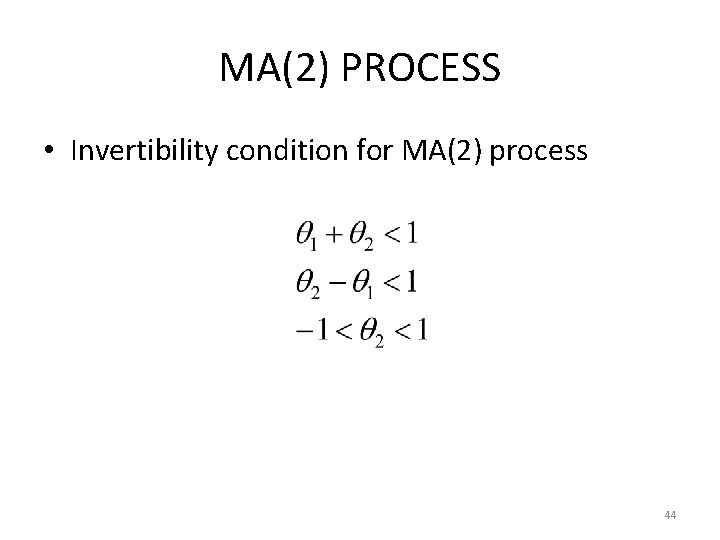
MA(2) PROCESS • Invertibility condition for MA(2) process 44
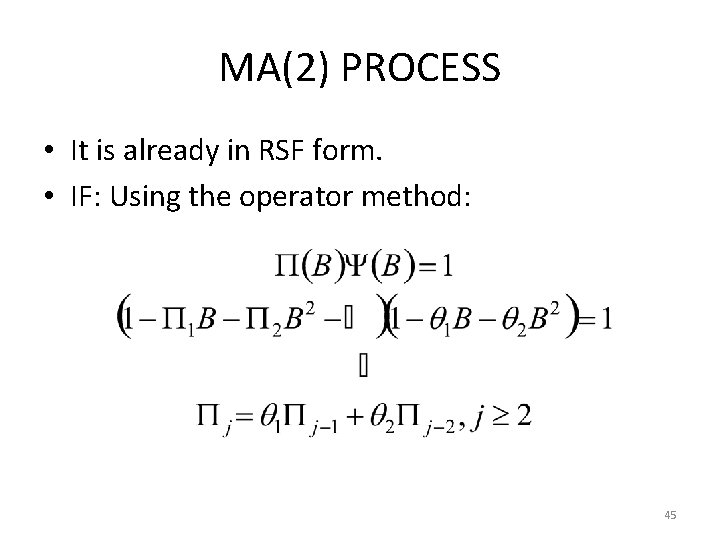
MA(2) PROCESS • It is already in RSF form. • IF: Using the operator method: 45
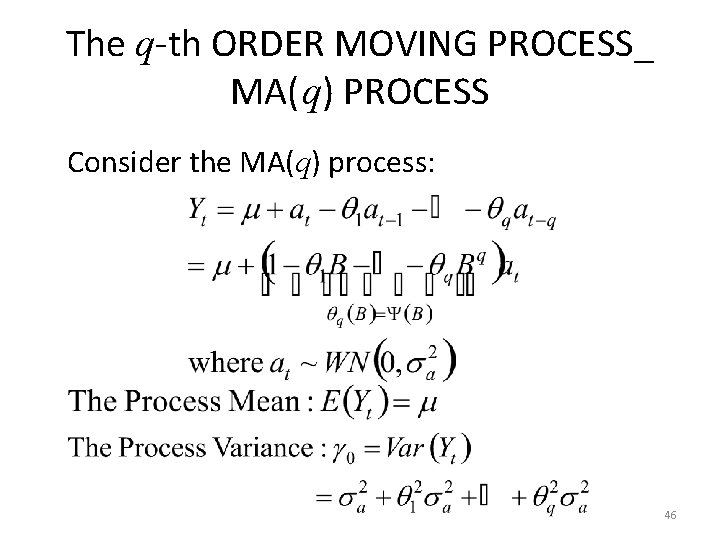
The q-th ORDER MOVING PROCESS_ MA(q) PROCESS Consider the MA(q) process: 46
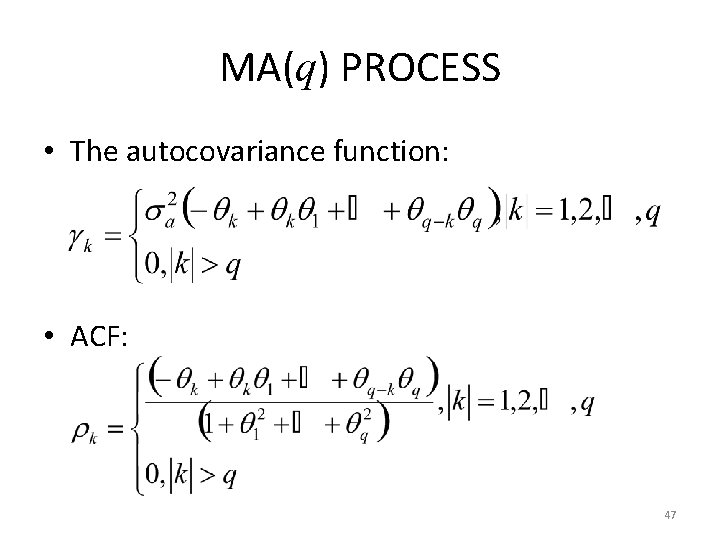
MA(q) PROCESS • The autocovariance function: • ACF: 47
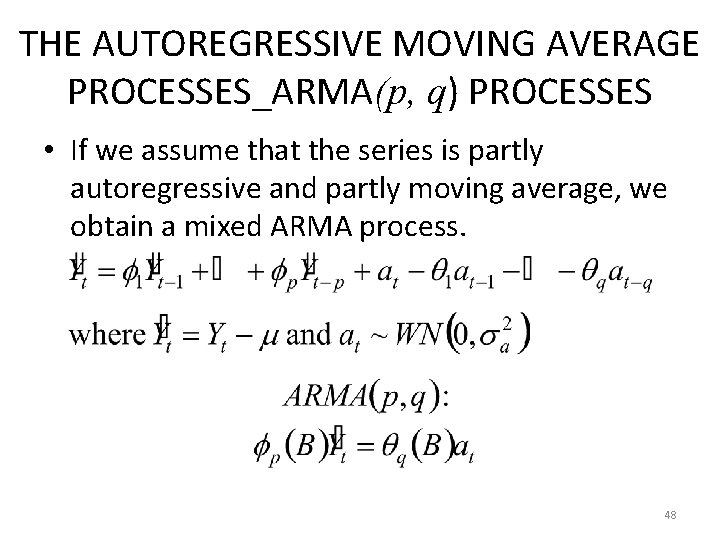
THE AUTOREGRESSIVE MOVING AVERAGE PROCESSES_ARMA(p, q) PROCESSES • If we assume that the series is partly autoregressive and partly moving average, we obtain a mixed ARMA process. 48
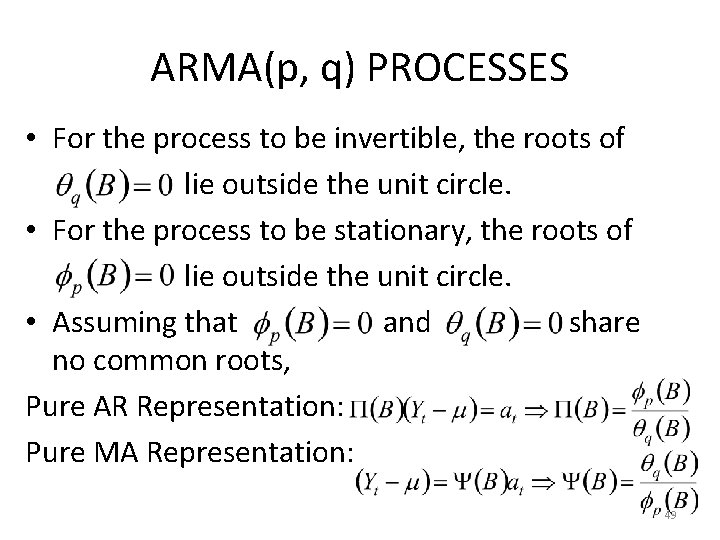
ARMA(p, q) PROCESSES • For the process to be invertible, the roots of lie outside the unit circle. • For the process to be stationary, the roots of lie outside the unit circle. • Assuming that and share no common roots, Pure AR Representation: Pure MA Representation: 49
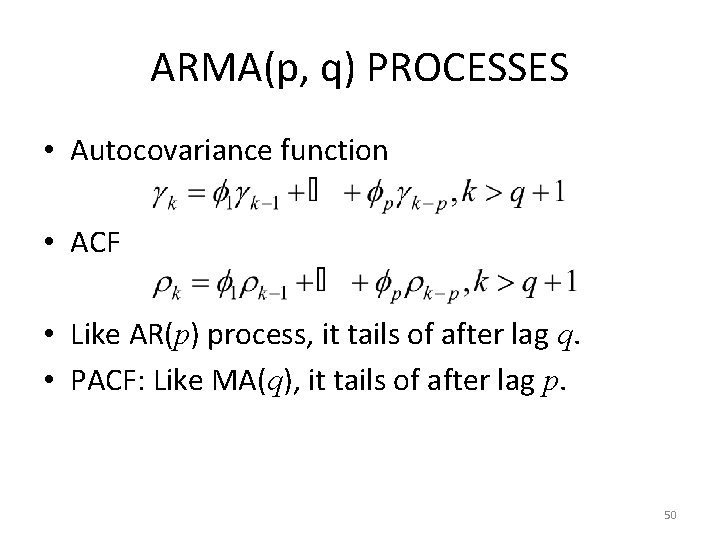
ARMA(p, q) PROCESSES • Autocovariance function • ACF • Like AR(p) process, it tails of after lag q. • PACF: Like MA(q), it tails of after lag p. 50
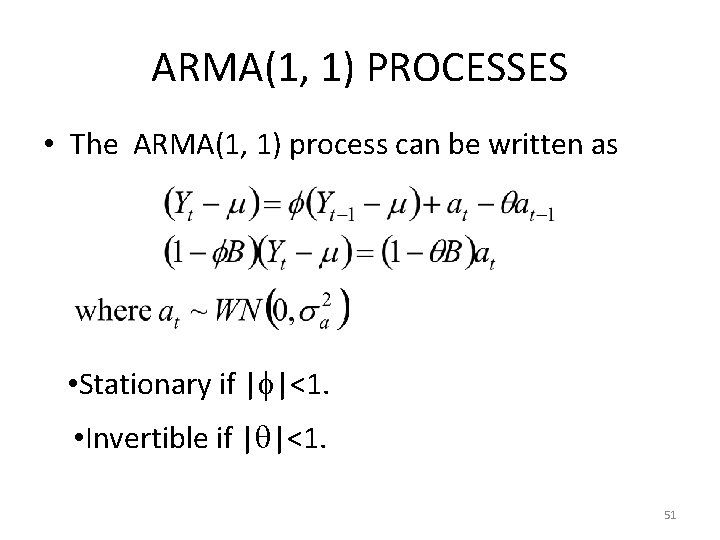
ARMA(1, 1) PROCESSES • The ARMA(1, 1) process can be written as • Stationary if | |<1. • Invertible if | |<1. 51
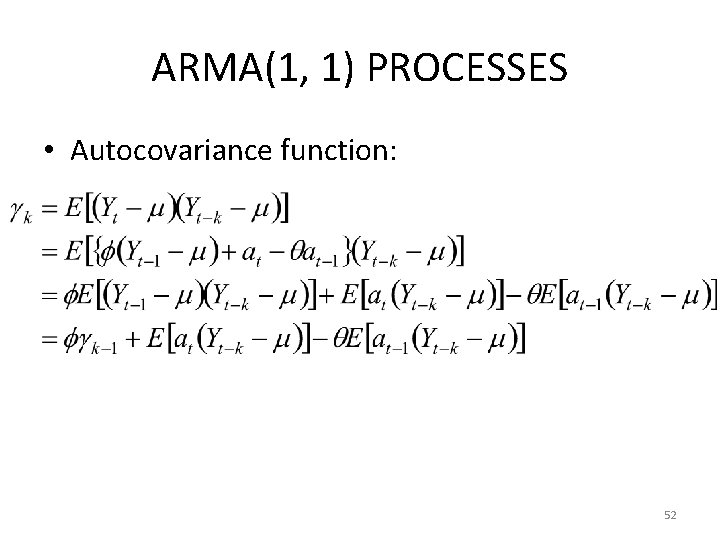
ARMA(1, 1) PROCESSES • Autocovariance function: 52
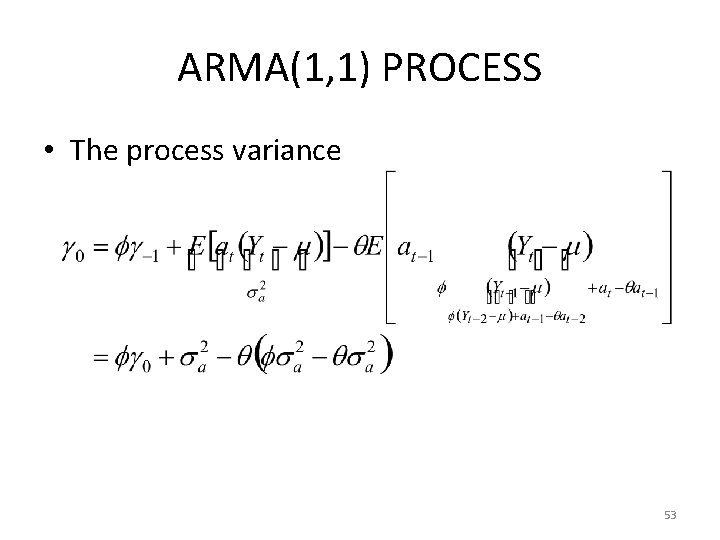
ARMA(1, 1) PROCESS • The process variance 53
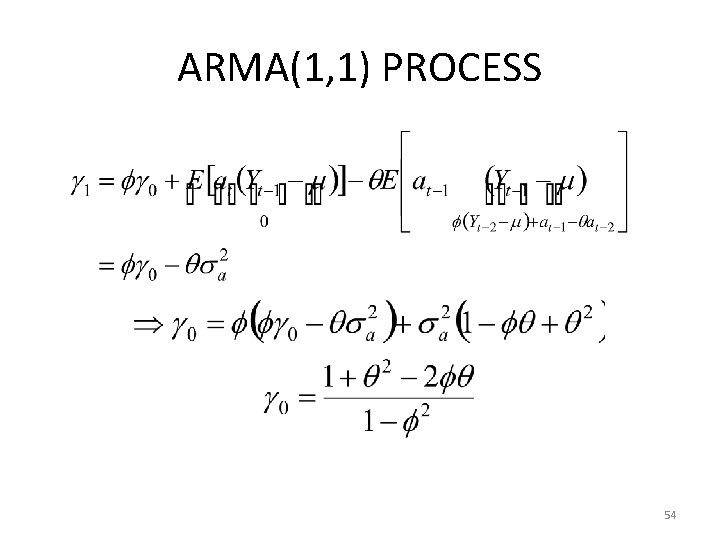
ARMA(1, 1) PROCESS 54
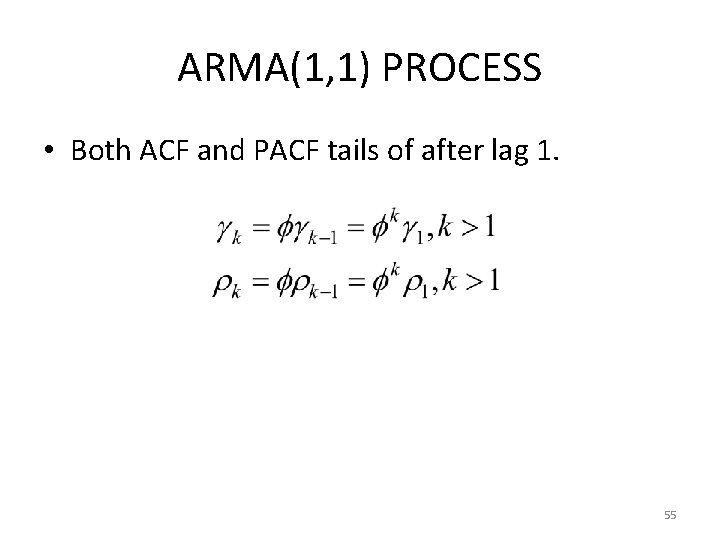
ARMA(1, 1) PROCESS • Both ACF and PACF tails of after lag 1. 55
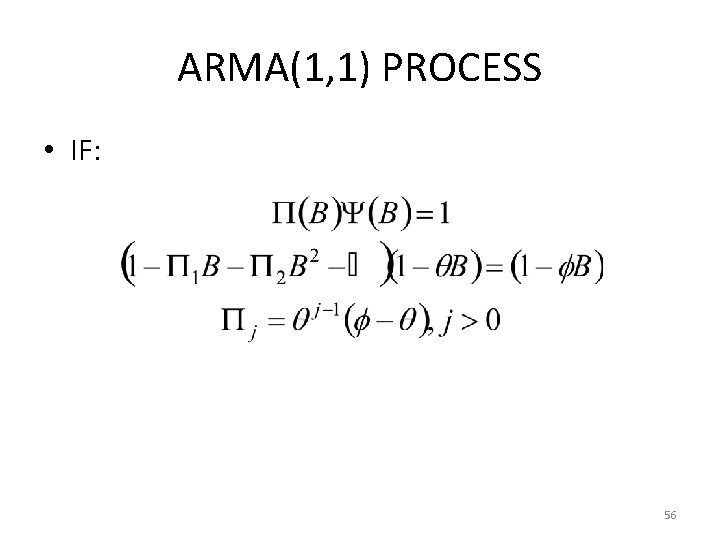
ARMA(1, 1) PROCESS • IF: 56
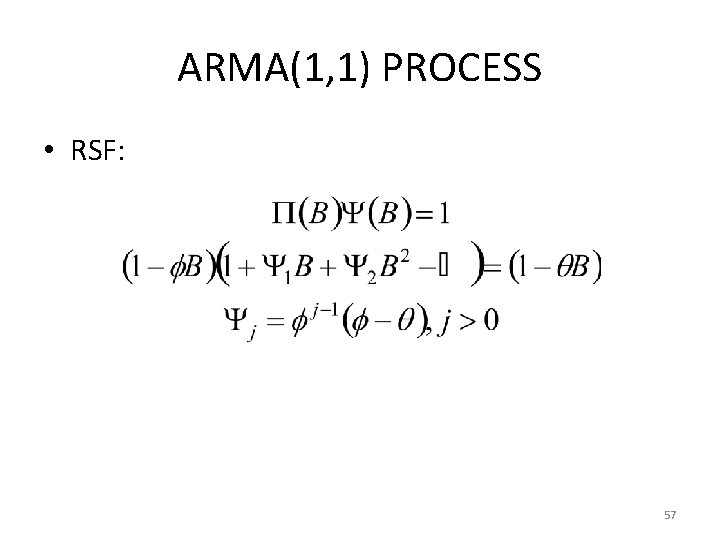
ARMA(1, 1) PROCESS • RSF: 57
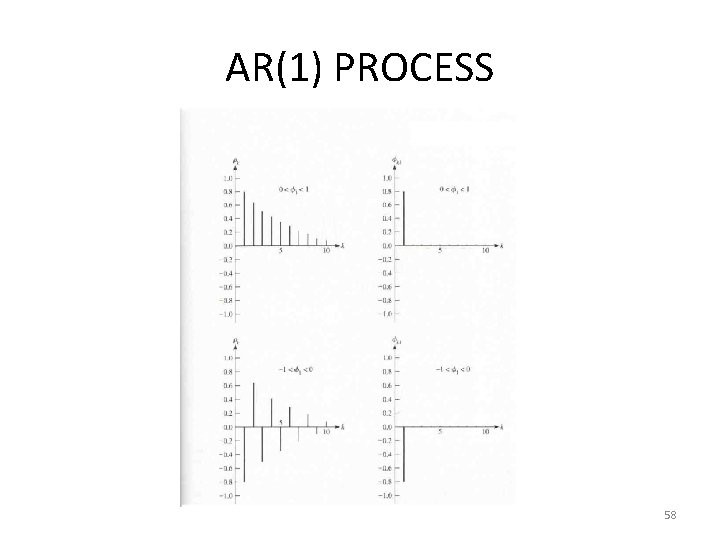
AR(1) PROCESS 58
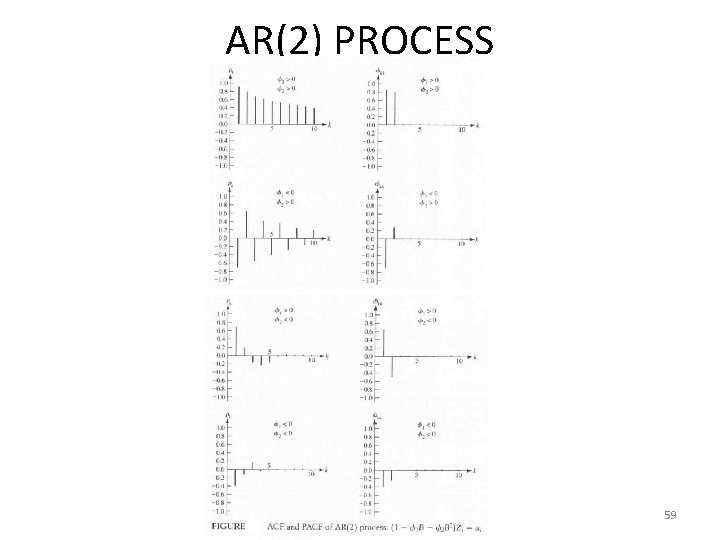
AR(2) PROCESS 59
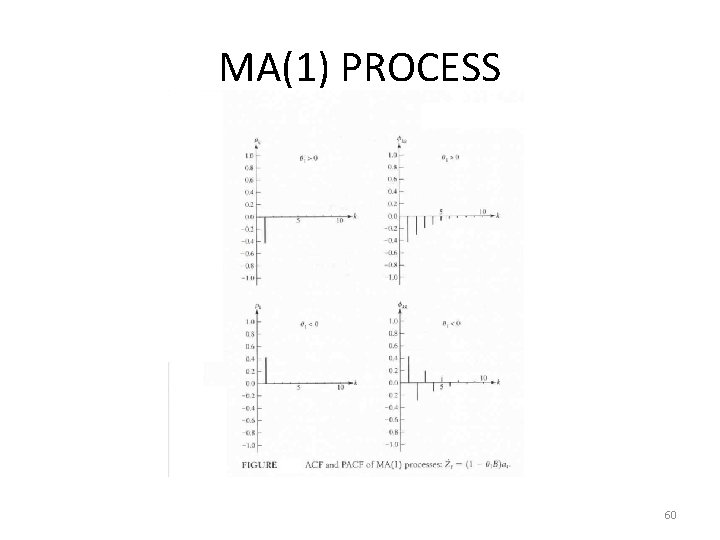
MA(1) PROCESS 60
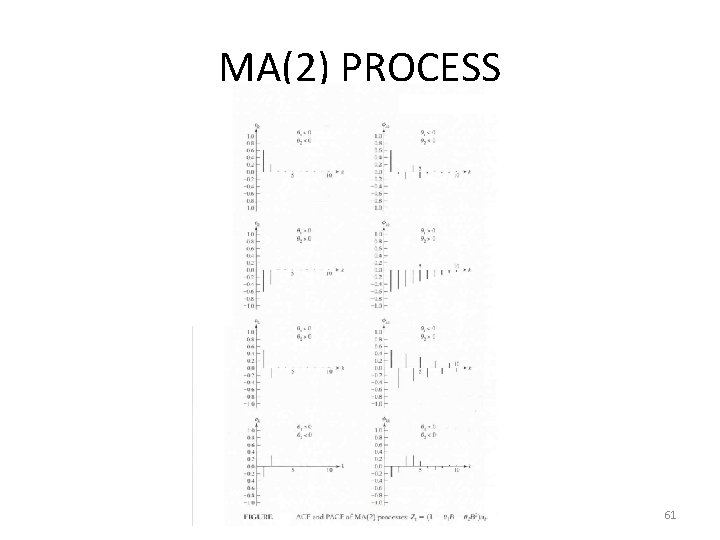
MA(2) PROCESS 61
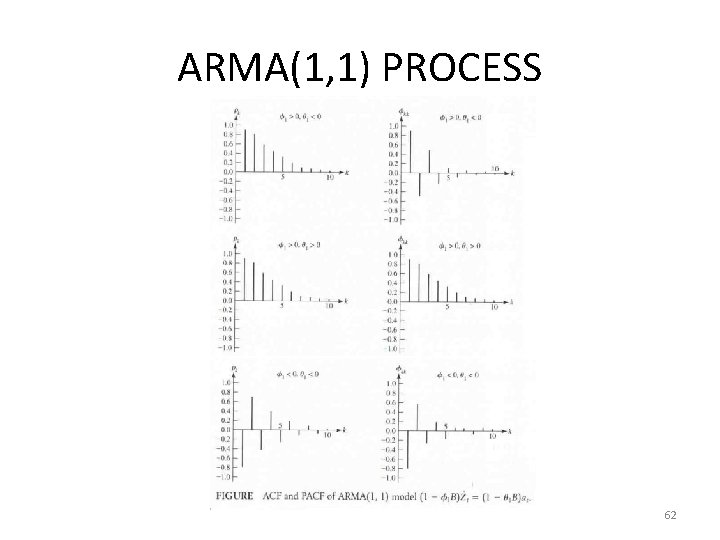
ARMA(1, 1) PROCESS 62
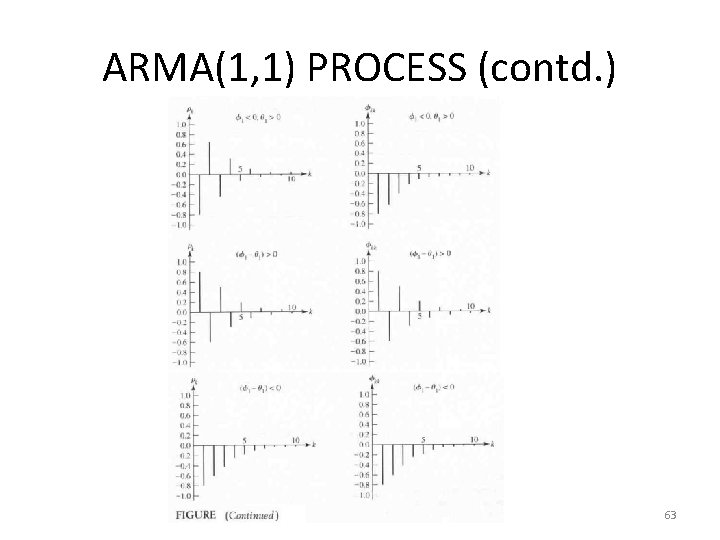
ARMA(1, 1) PROCESS (contd. ) 63
01:640:244 lecture notes - lecture 15: plat, idah, farad
Mech 497
Cs 497
Opwekking 497 tekst
Ansc 497
Mg 497
Ansc 497
Ansc 497
Stationary on a velocity time graph
Geology lecture series
Dcac lecture series
Project procurement management lecture notes
Theology proper lecture notes
Public sector accounting pdf notes
Project management for software development
Magnetism
Classical mechanics
Physical science lecture notes
Power system dynamics and stability lecture notes
Microbial physiology notes
Sensors and actuators lecture notes
Limits fits and tolerances lecture notes
Money-time relationship and equivalence
Bjt lecture notes
Requirement analysis in software engineering notes
Ofdm lecture notes
Land use planning '' lecture notes
Project management lecture notes doc
Lecture notes on homiletics
Foundation engineering lecture notes
Image processing lecture notes
Intermediate microeconomics lecture notes
Parallel and distributed computing lecture notes
Bayesian decision theory lecture notes
Polynomial regression least squares
Advanced inorganic chemistry lecture notes
50h8 tolerance
Global stiffness matrix
Muskingum method
Stat root word
Shape memory alloys lecture notes
Research methods notes kenya
Financial markets and institutions ppt
What is a harmonic wave in physics
Om306
Nlp lecture notes
Linux lecture notes
General parasitology lecture notes
Introduction to biochemistry lecture notes
Stern-gerlach experiment lecture notes
Land use planning '' lecture notes
Exploratory data analysis lecture notes
Slidetodoc
Interior design lecture notes+ppt
Bayesian classification in data mining lecture notes
Data mining lecture notes
Architecture lecture notes
Franck condon principle
Biopotential electrodes lecture notes
Catalysis lecture notes
Bayesian decision theory lecture notes
Gujarati basic econometrics lecture notes ppt
Anderson localization lecture notes
Operating system lecture notes