13 1 The Chisquare GoodnessofFit test Agenda for
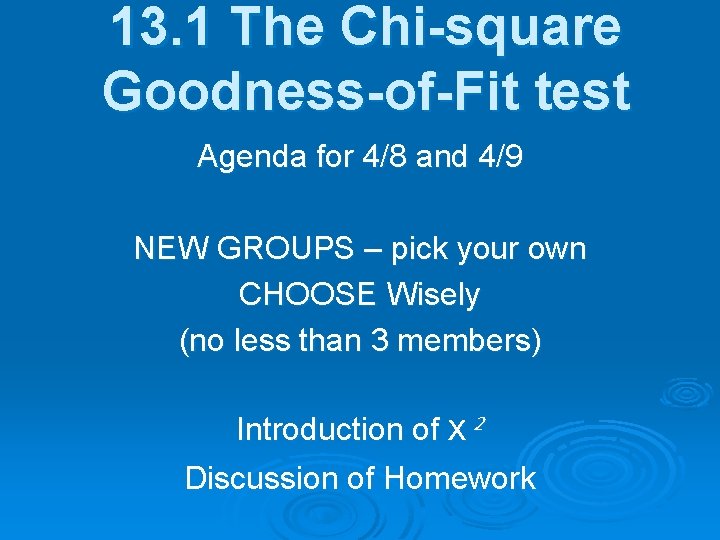
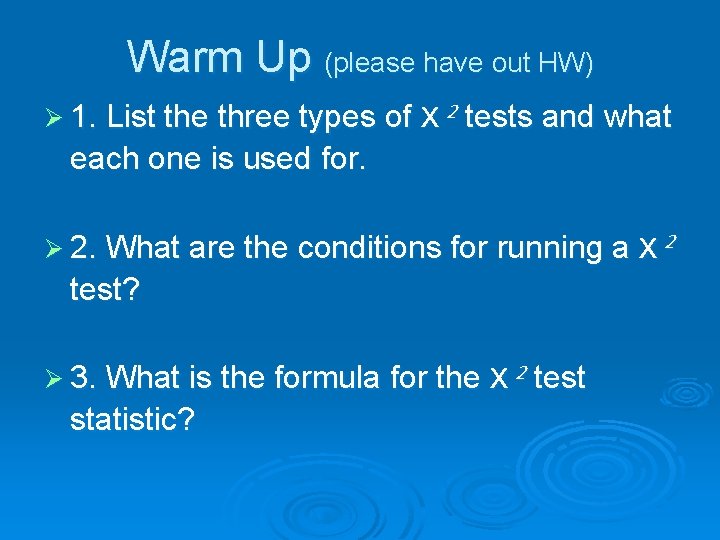
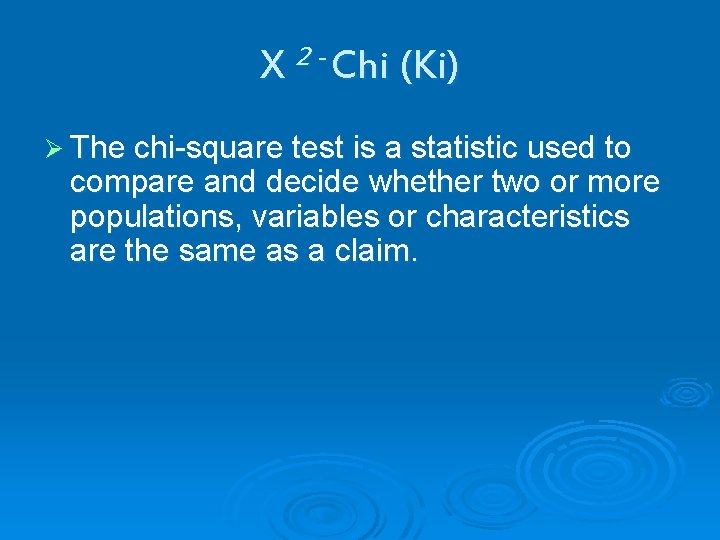
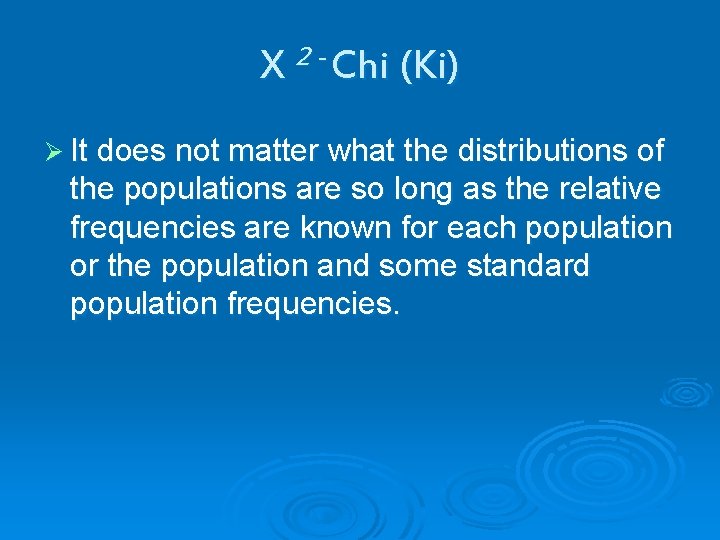
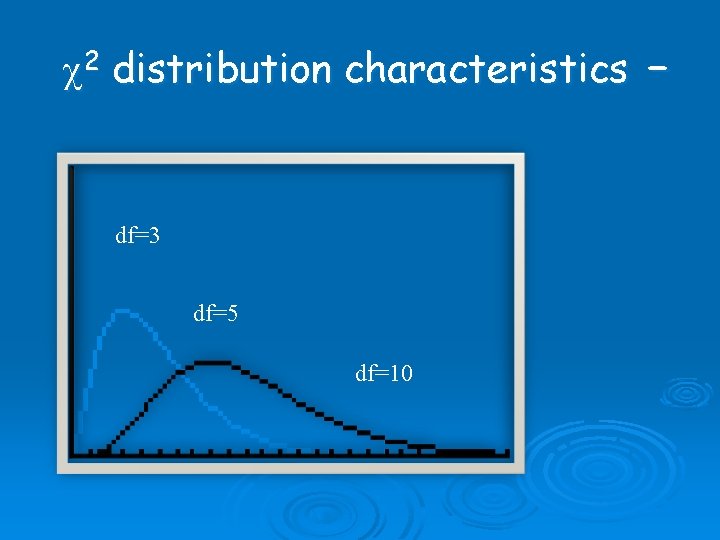
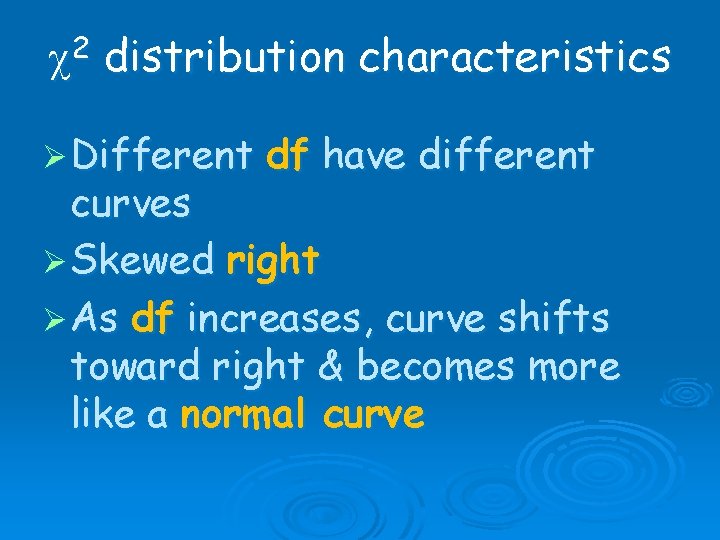
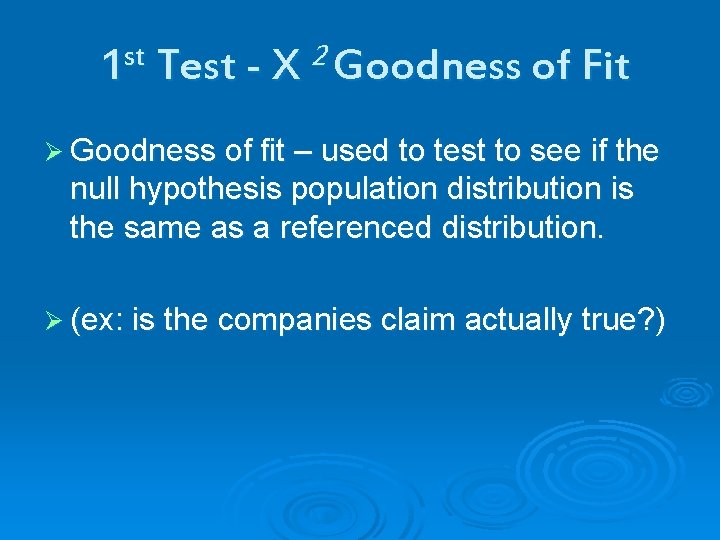
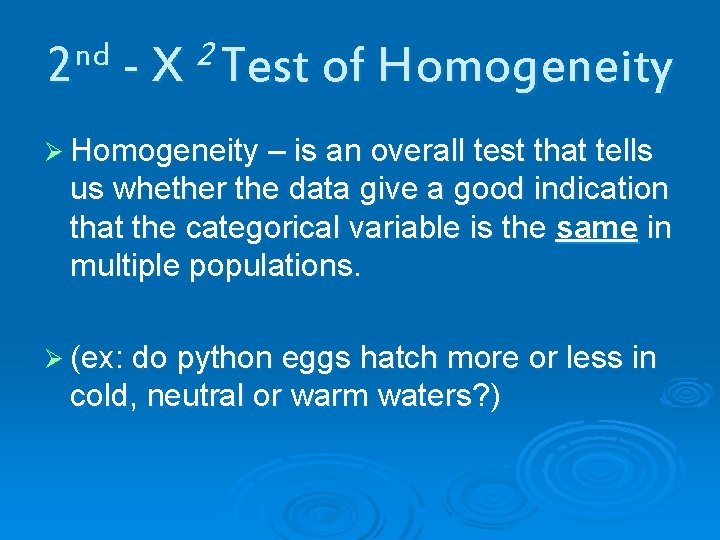
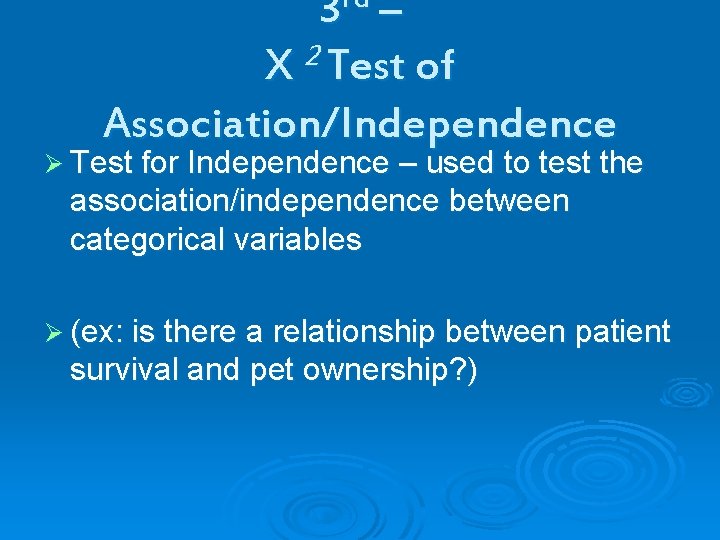
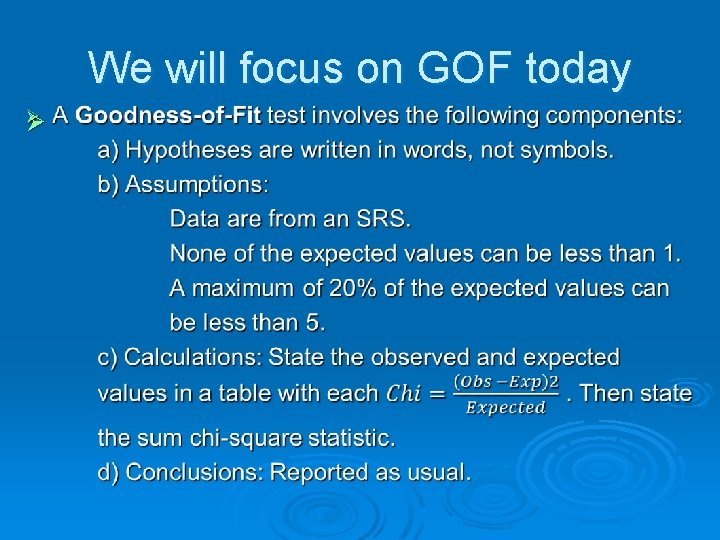
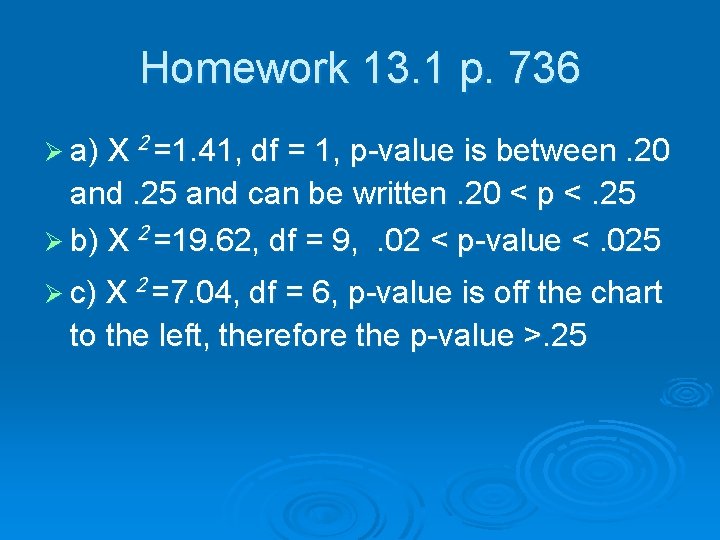
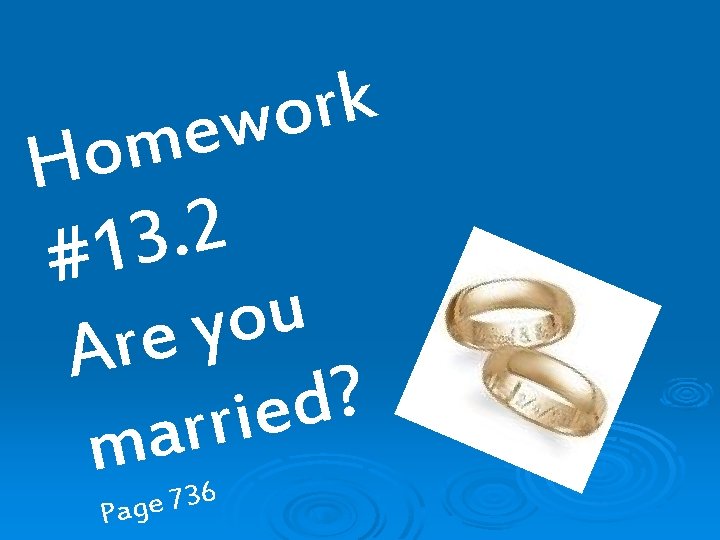
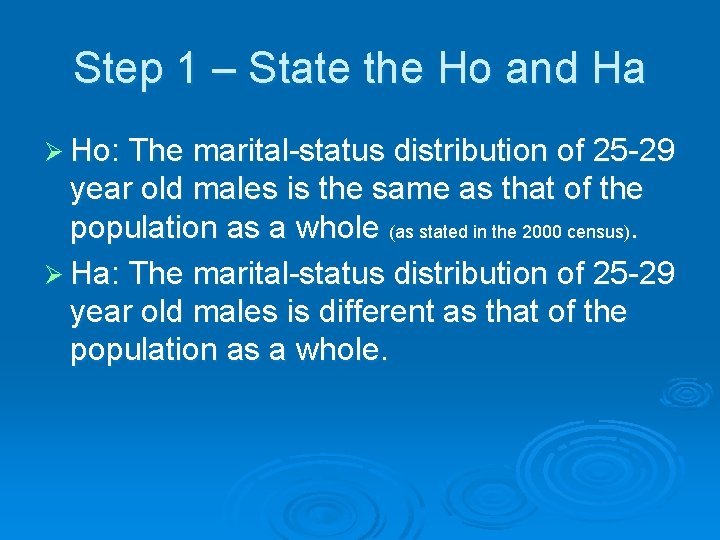
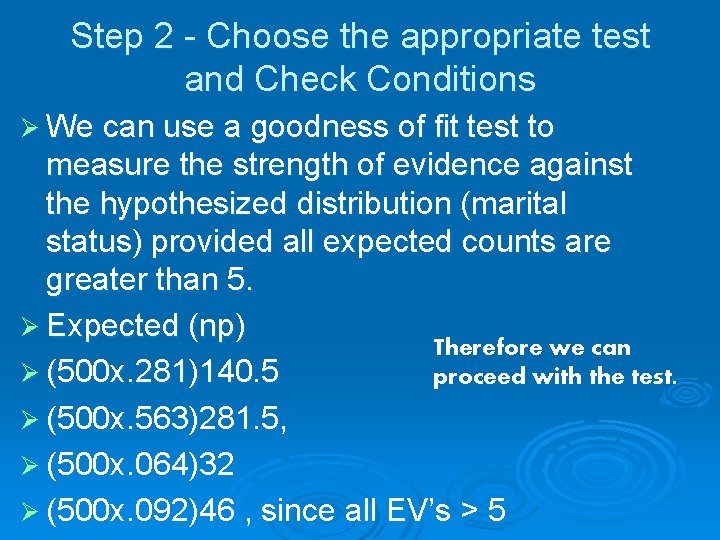
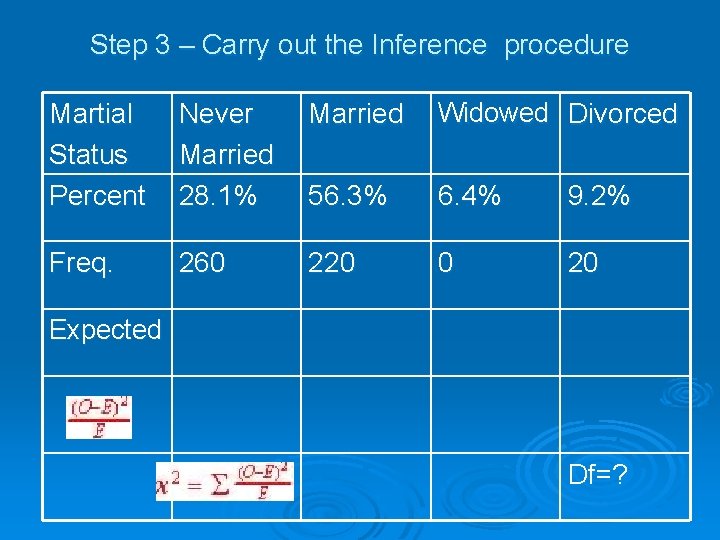
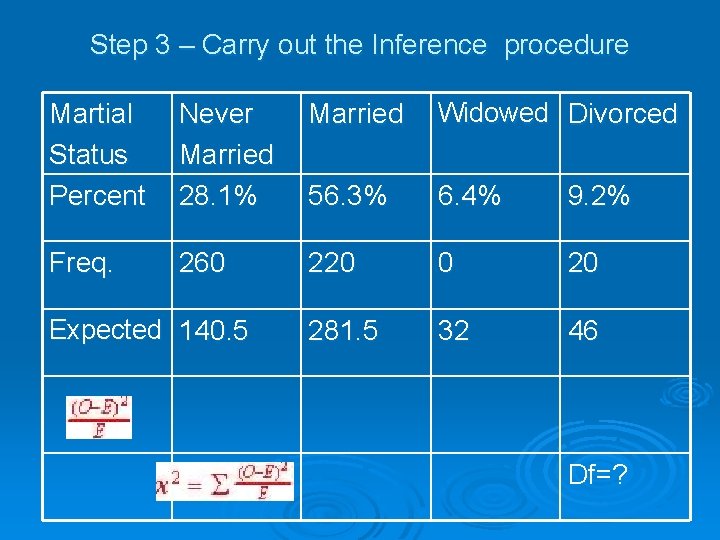
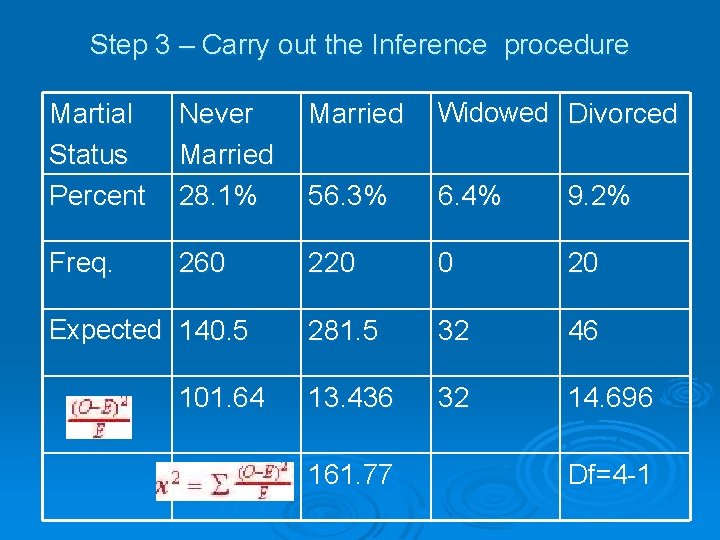
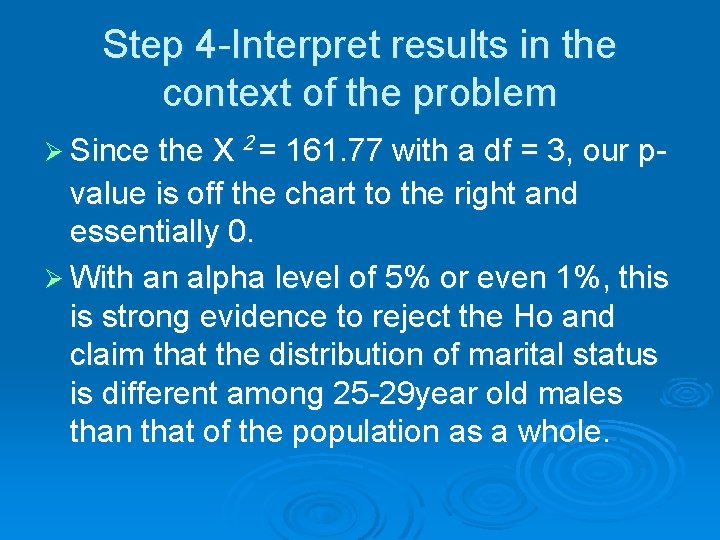
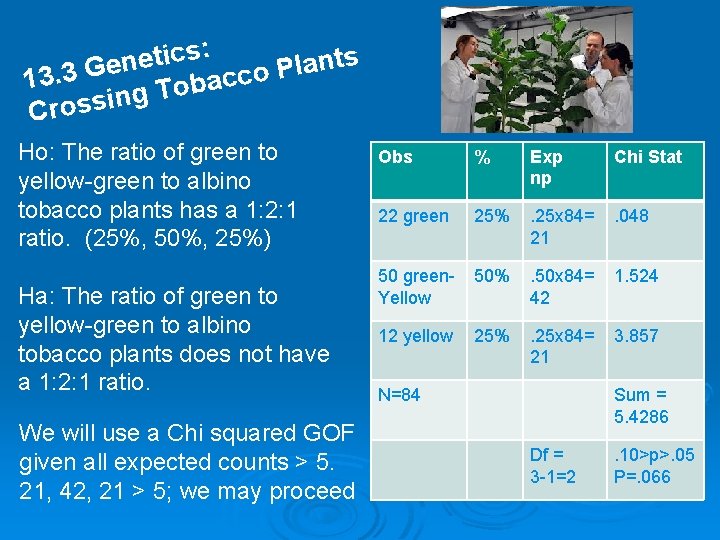
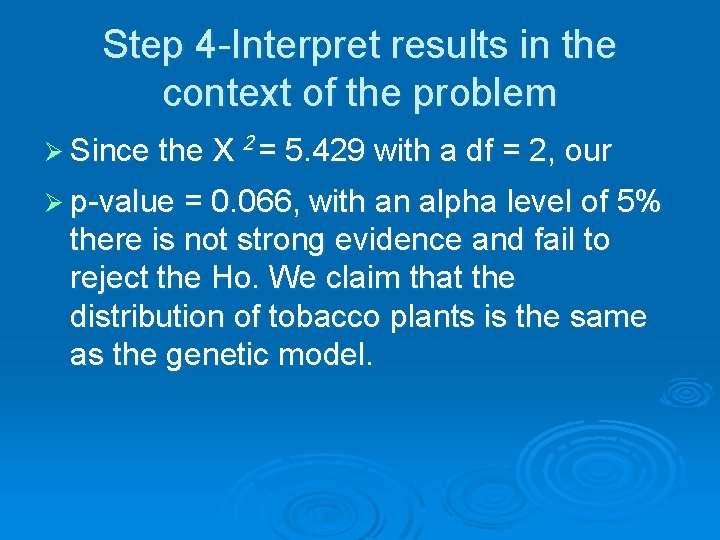
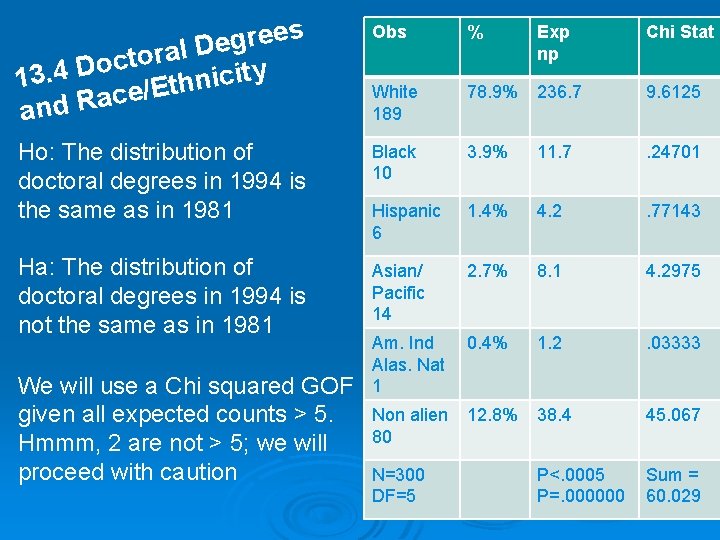
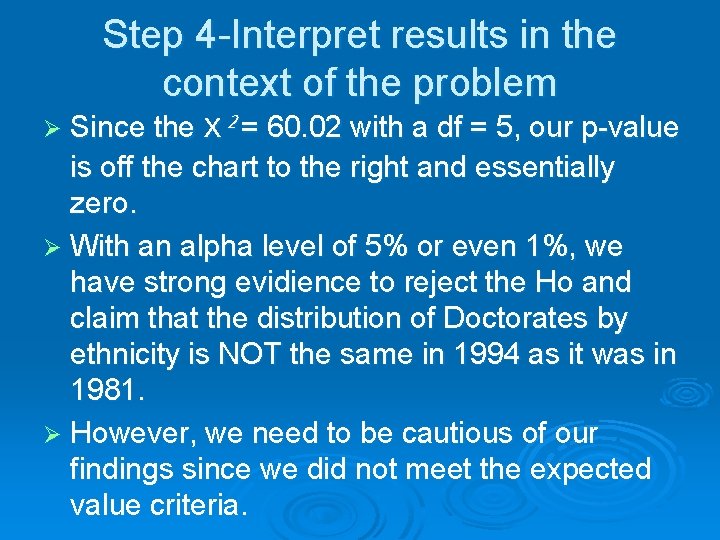
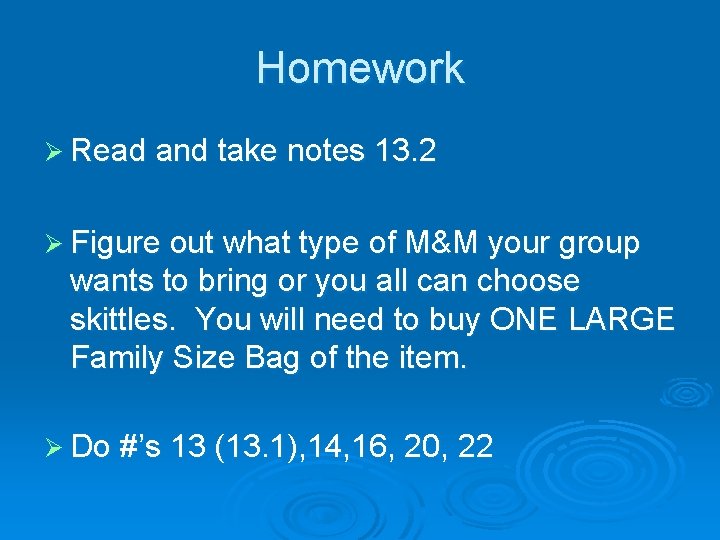
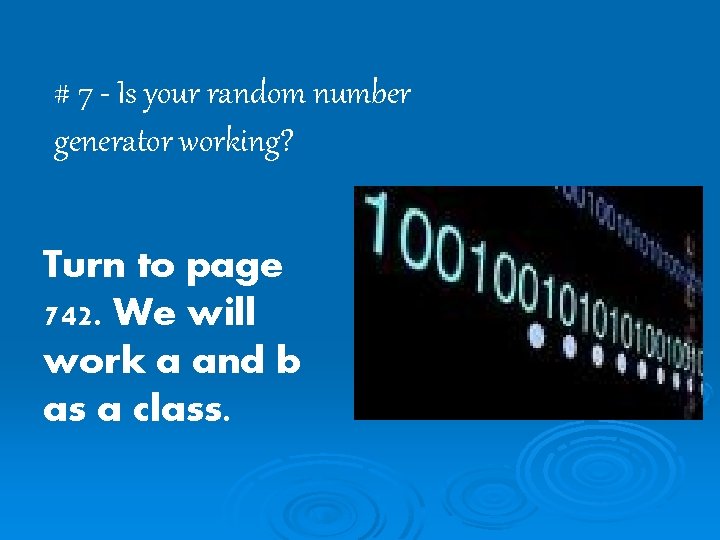
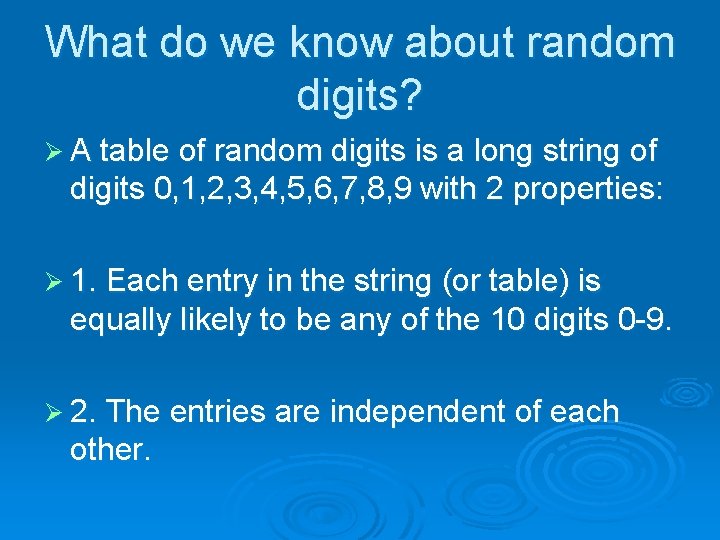
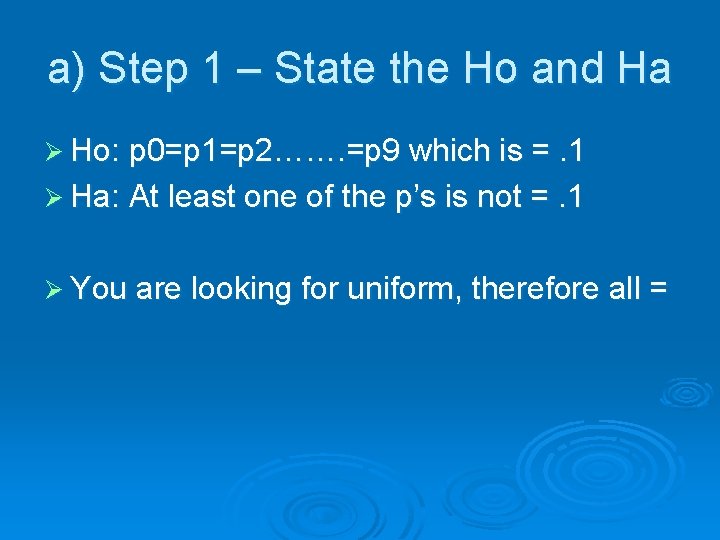
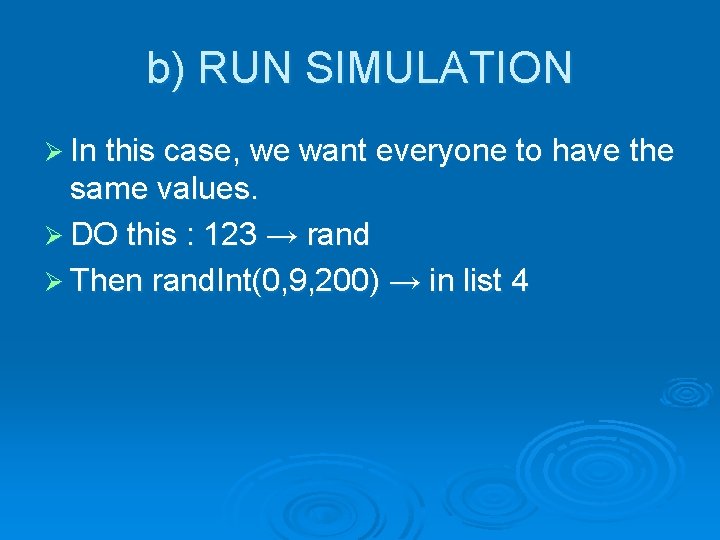
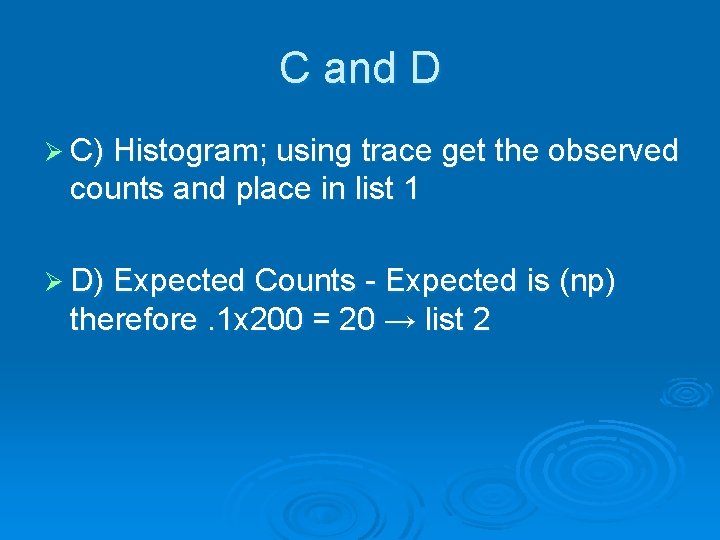
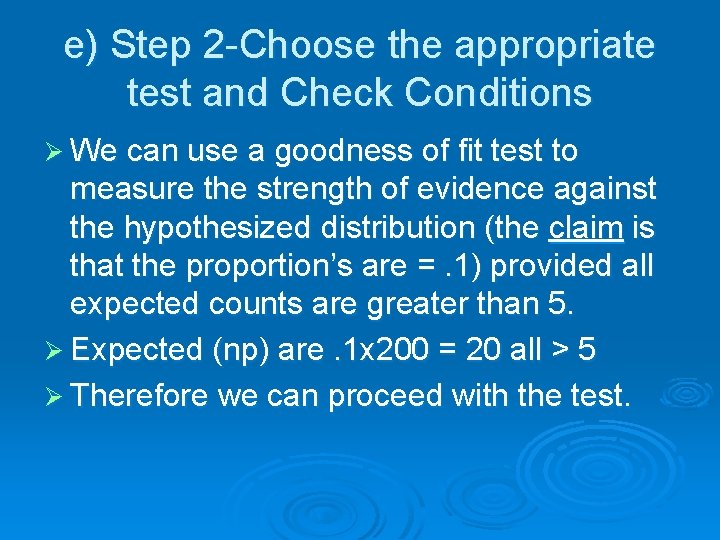
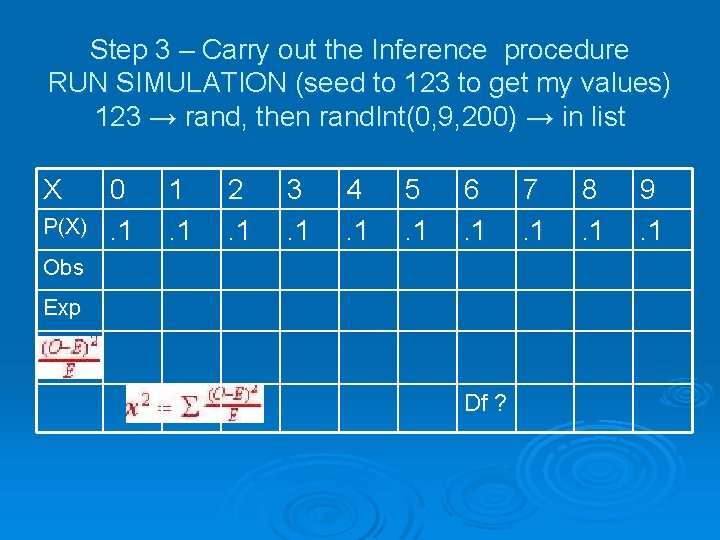
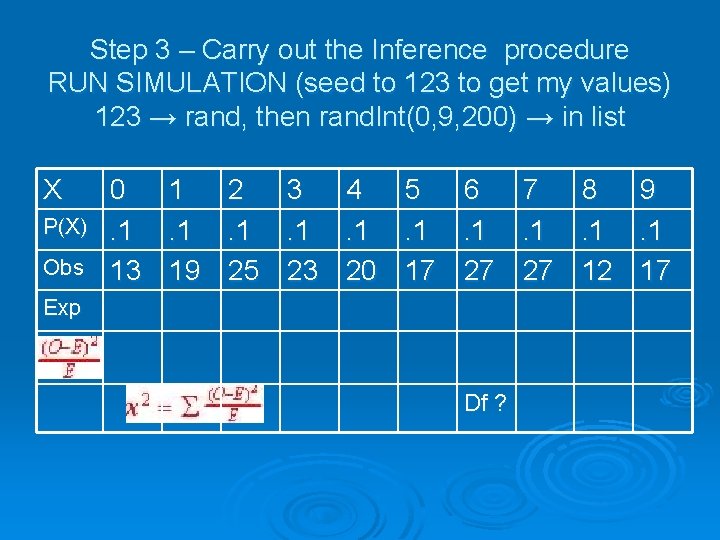
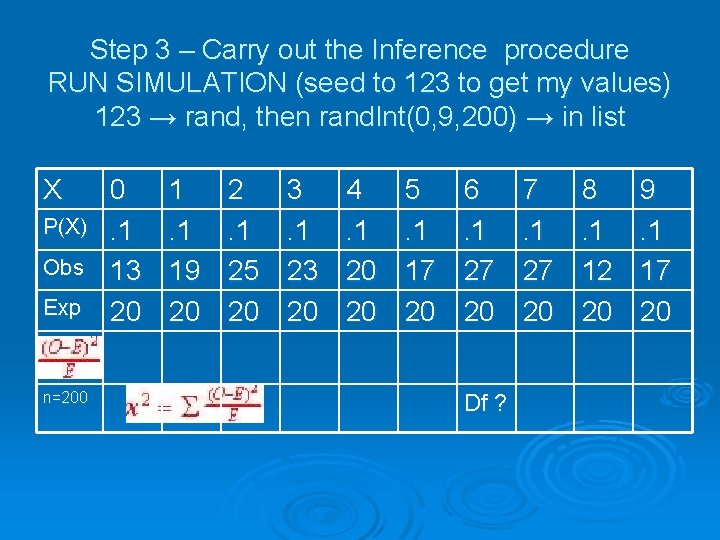
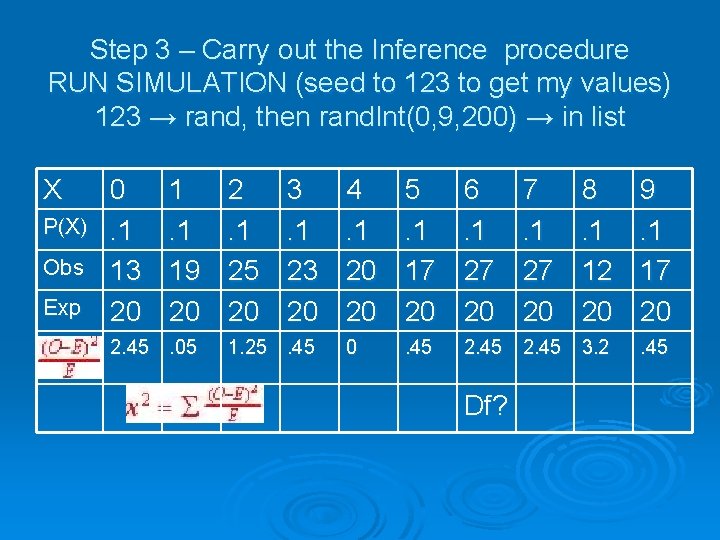
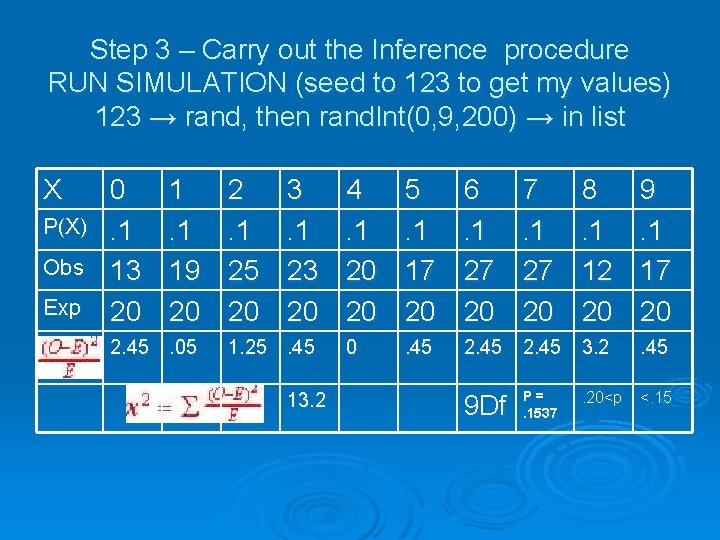
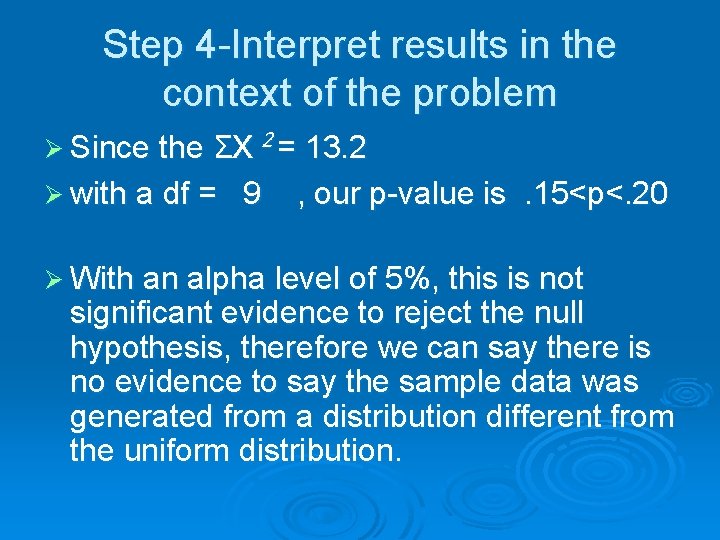
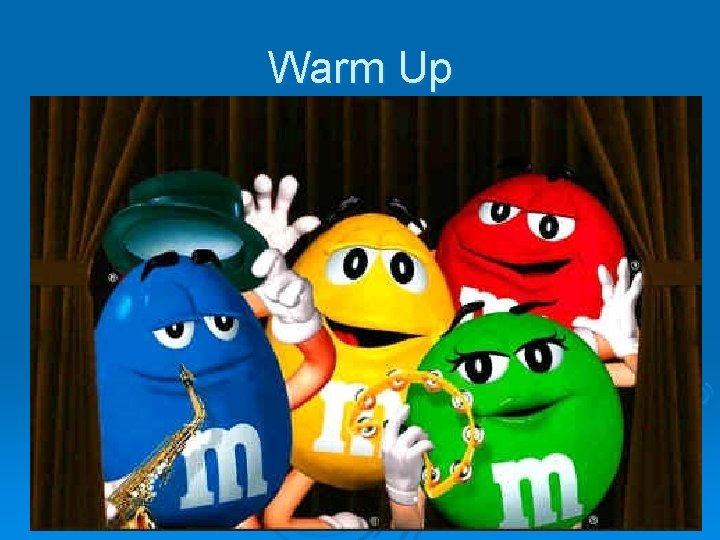
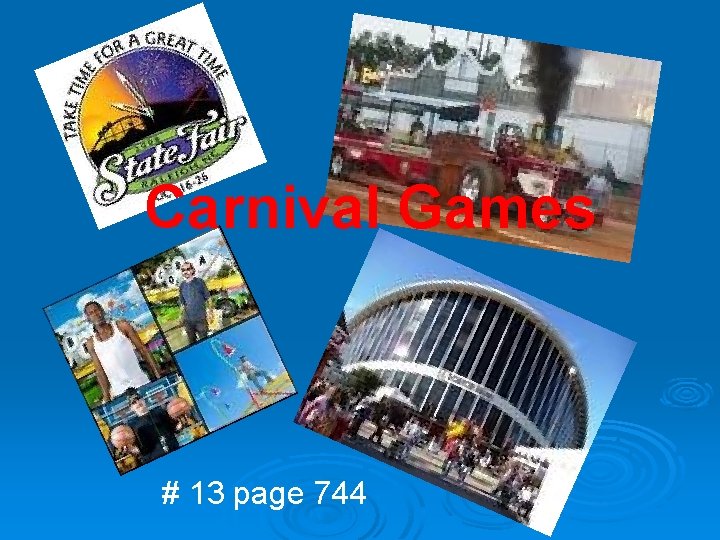
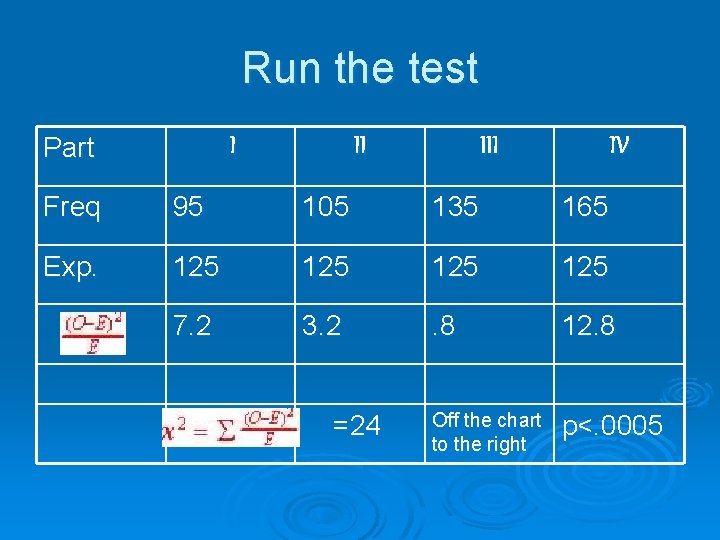
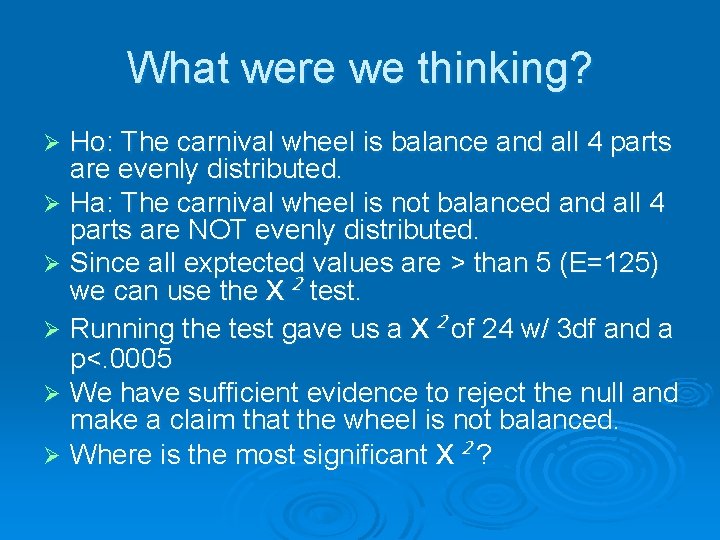
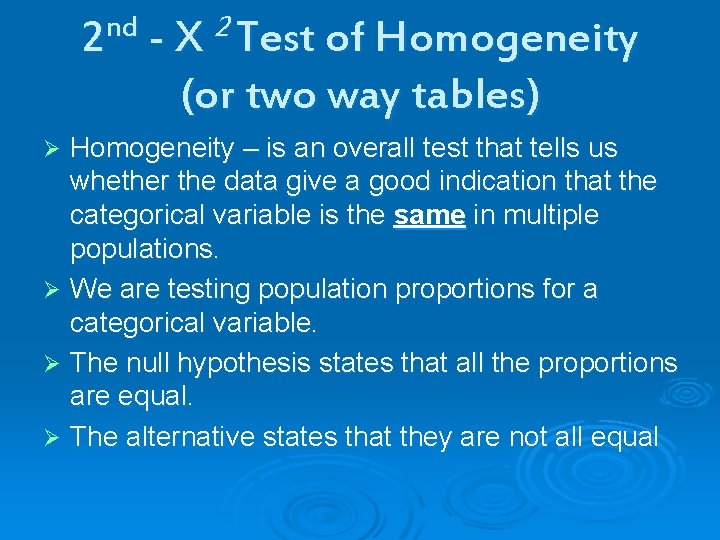
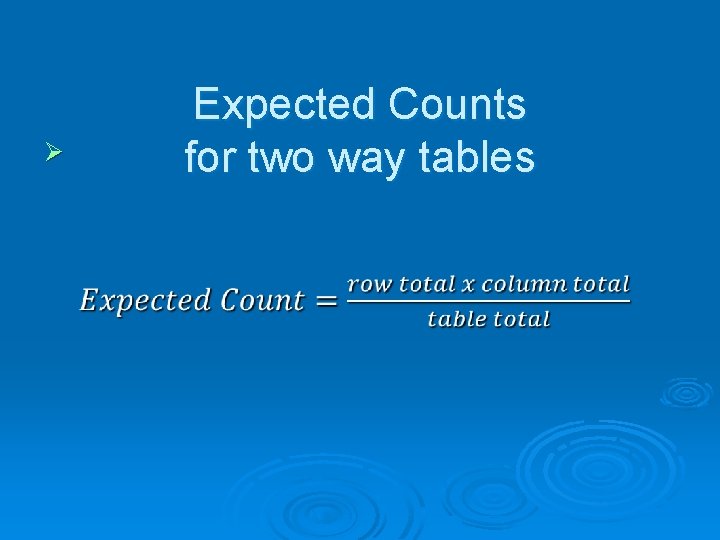
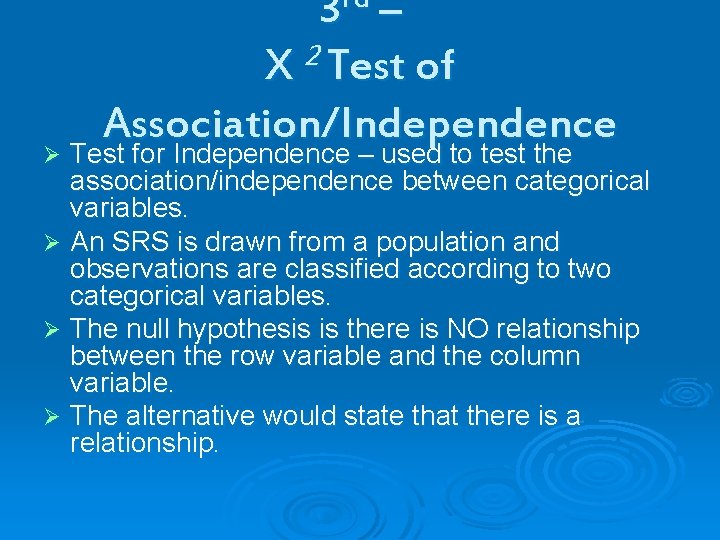
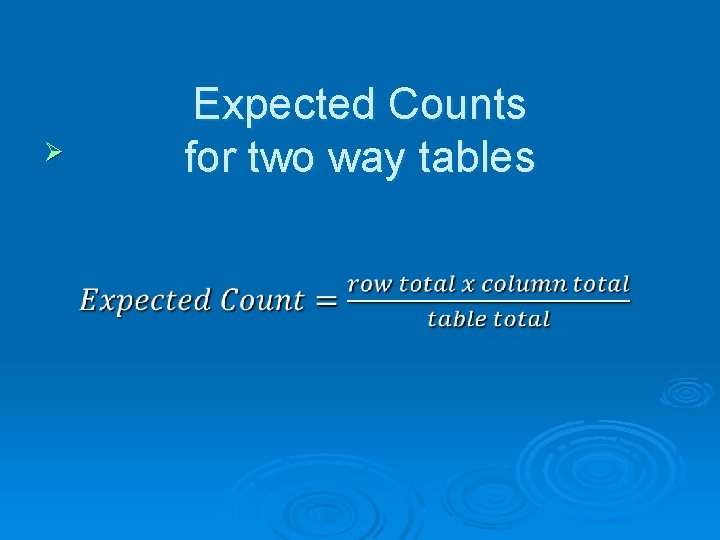
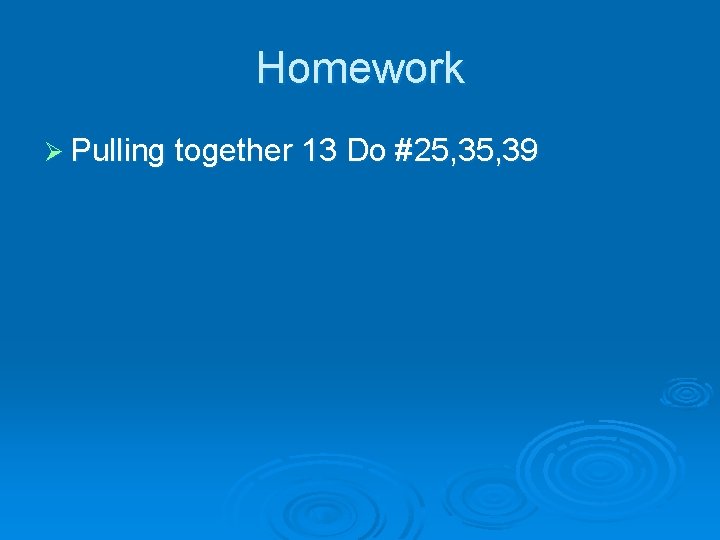
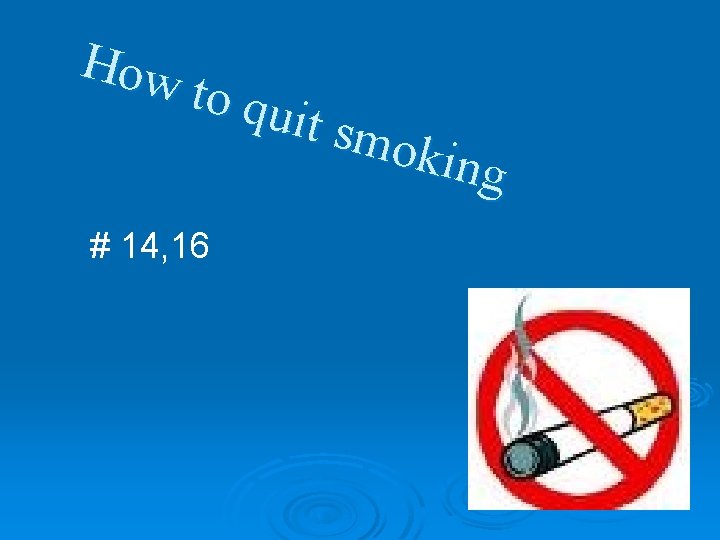
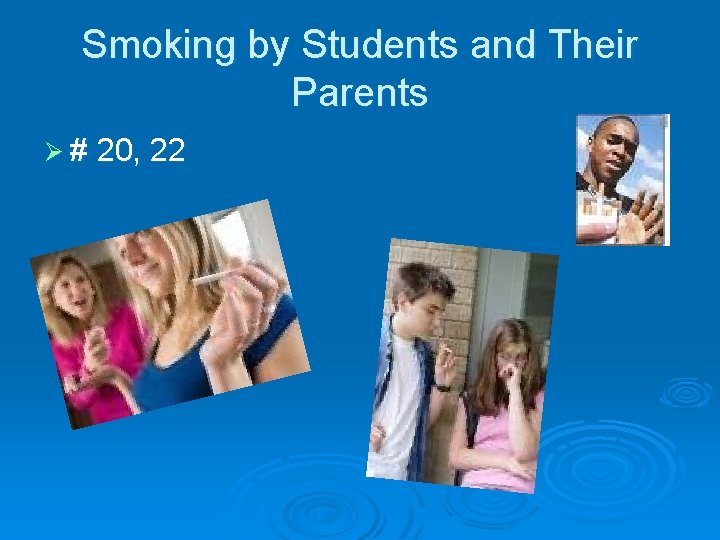
- Slides: 46
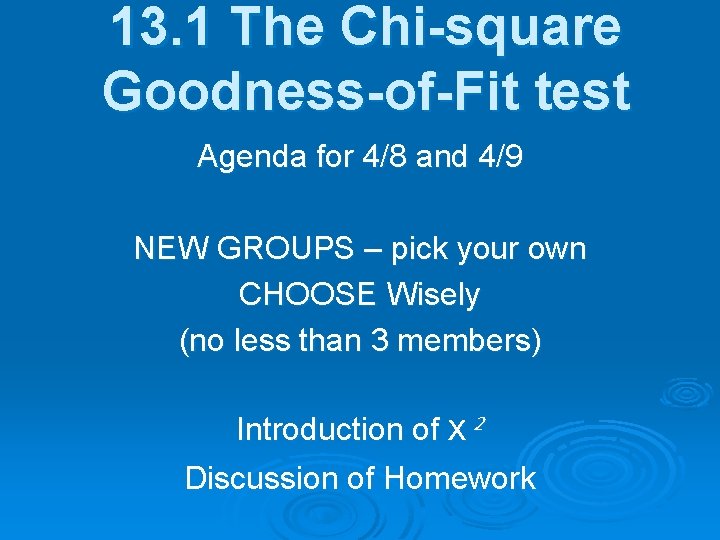
13. 1 The Chi-square Goodness-of-Fit test Agenda for 4/8 and 4/9 NEW GROUPS – pick your own CHOOSE Wisely (no less than 3 members) Introduction of X 2 Discussion of Homework
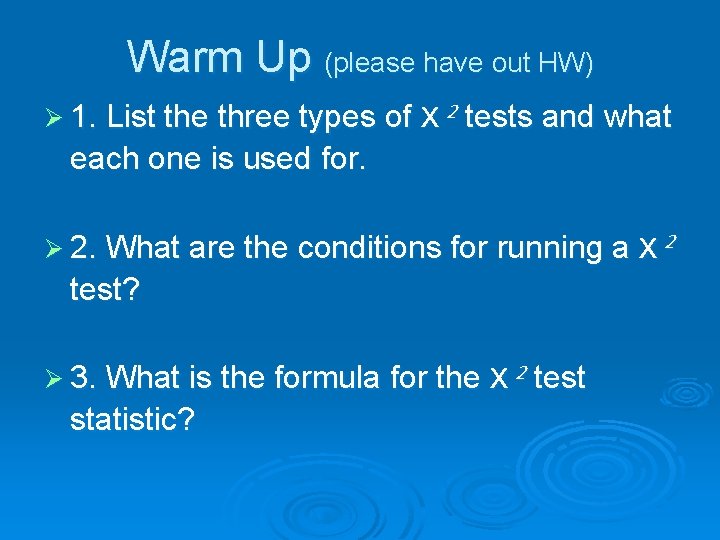
Warm Up (please have out HW) Ø 1. List the three types of X 2 tests and what each one is used for. Ø 2. What are the conditions for running a test? Ø 3. What is the formula for the statistic? X 2 test X 2
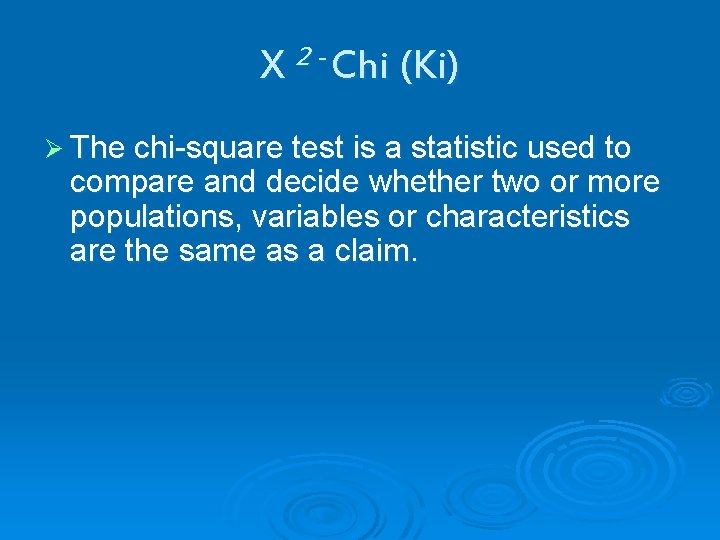
X 2 - Chi (Ki) Ø The chi-square test is a statistic used to compare and decide whether two or more populations, variables or characteristics are the same as a claim.
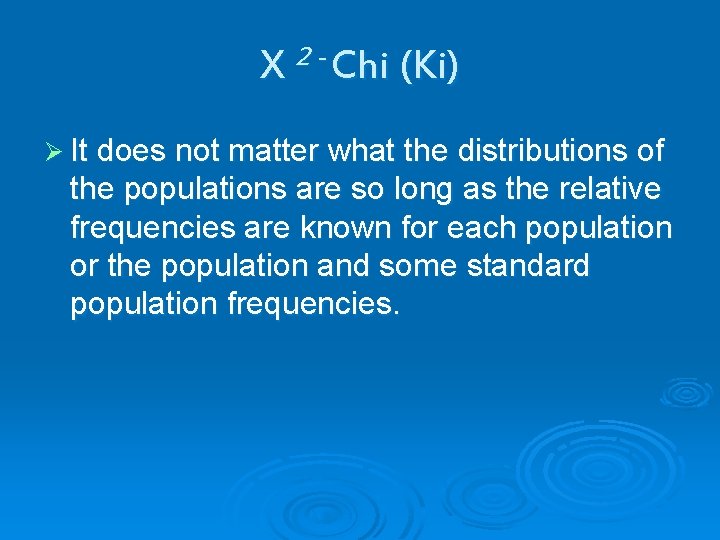
X 2 - Chi (Ki) Ø It does not matter what the distributions of the populations are so long as the relative frequencies are known for each population or the population and some standard population frequencies.
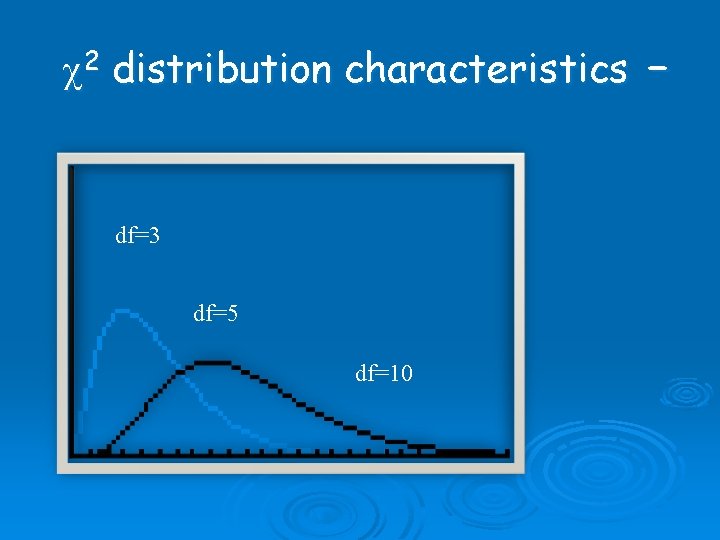
c 2 distribution characteristics – df=3 df=5 df=10
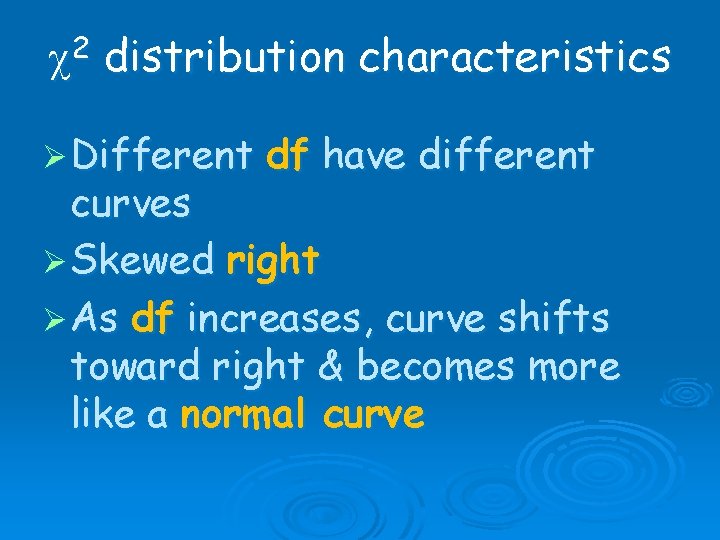
c 2 distribution characteristics Ø Different df have different curves Ø Skewed right Ø As df increases, curve shifts toward right & becomes more like a normal curve
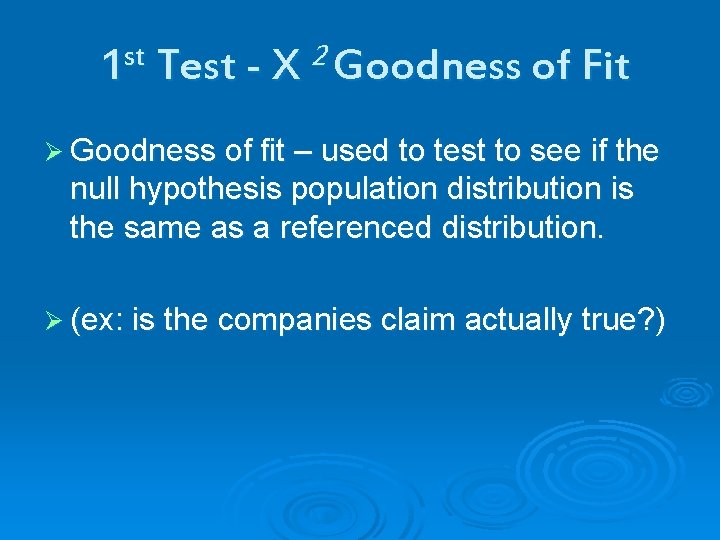
1 st Test - X 2 Goodness of Fit Ø Goodness of fit – used to test to see if the null hypothesis population distribution is the same as a referenced distribution. Ø (ex: is the companies claim actually true? )
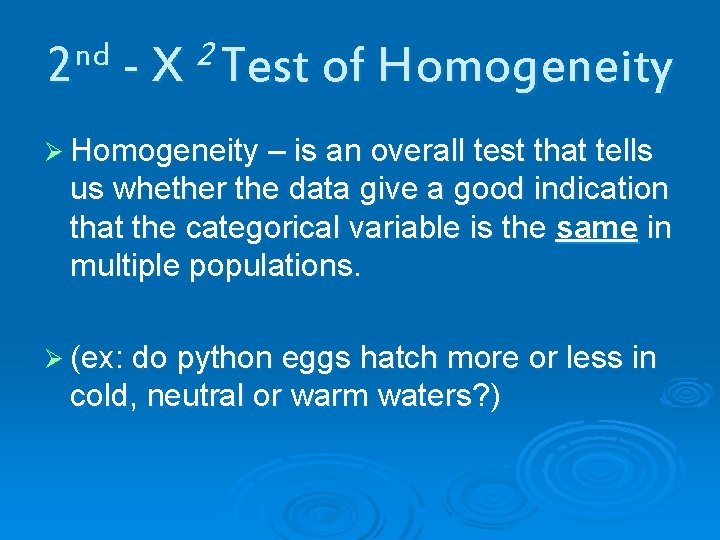
nd 2 2 - X Test of Homogeneity Ø Homogeneity – is an overall test that tells us whether the data give a good indication that the categorical variable is the same in multiple populations. Ø (ex: do python eggs hatch more or less in cold, neutral or warm waters? )
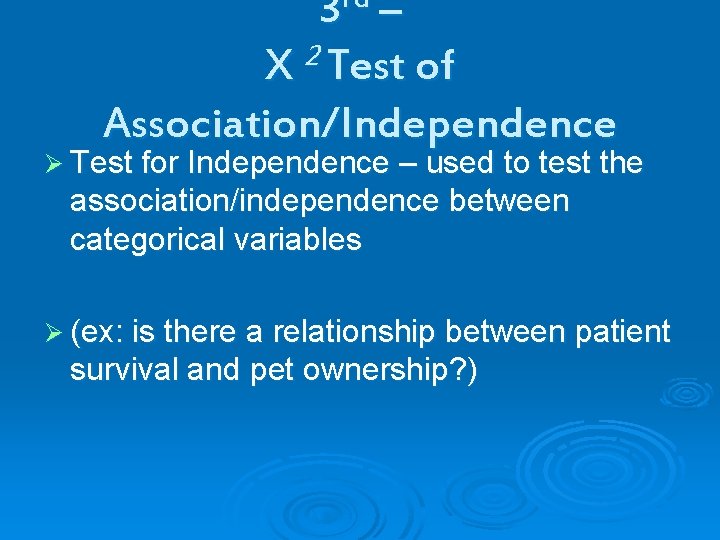
rd 3 – X 2 Test of Association/Independence Ø Test for Independence – used to test the association/independence between categorical variables Ø (ex: is there a relationship between patient survival and pet ownership? )
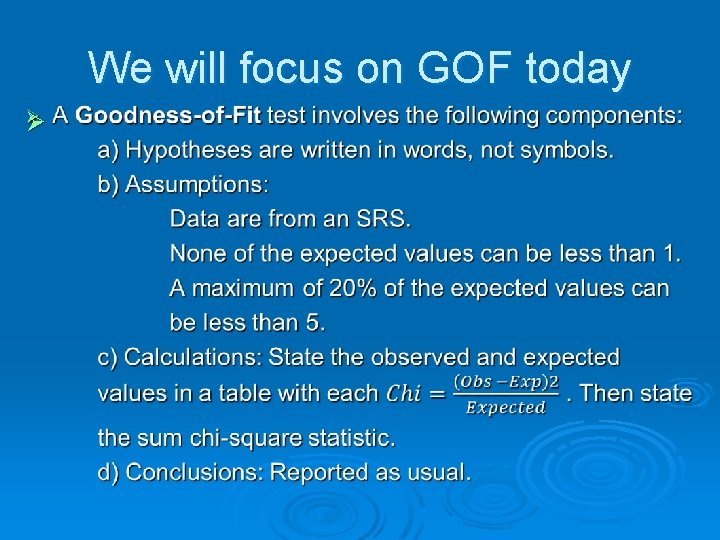
We will focus on GOF today Ø
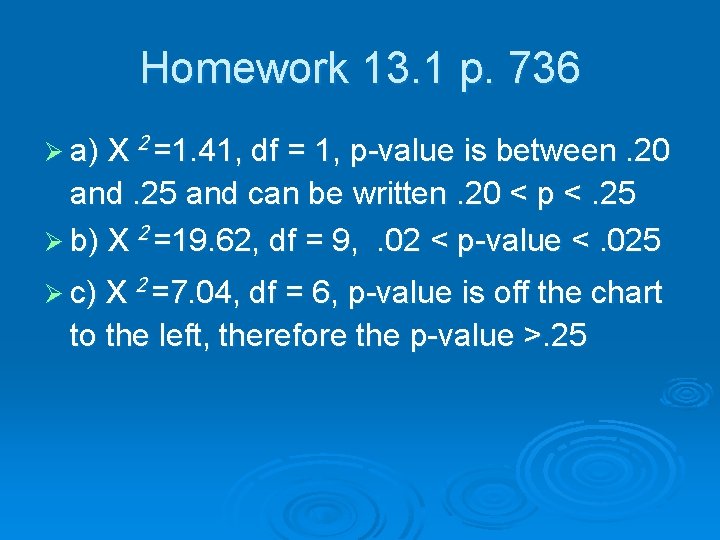
Homework 13. 1 p. 736 Ø a) X 2 =1. 41, df = 1, p-value is between. 20 and. 25 and can be written. 20 < p <. 25 Ø b) X 2 =19. 62, df = 9, . 02 < p-value <. 025 Ø c) X 2 =7. 04, df = 6, p-value is off the chart to the left, therefore the p-value >. 25
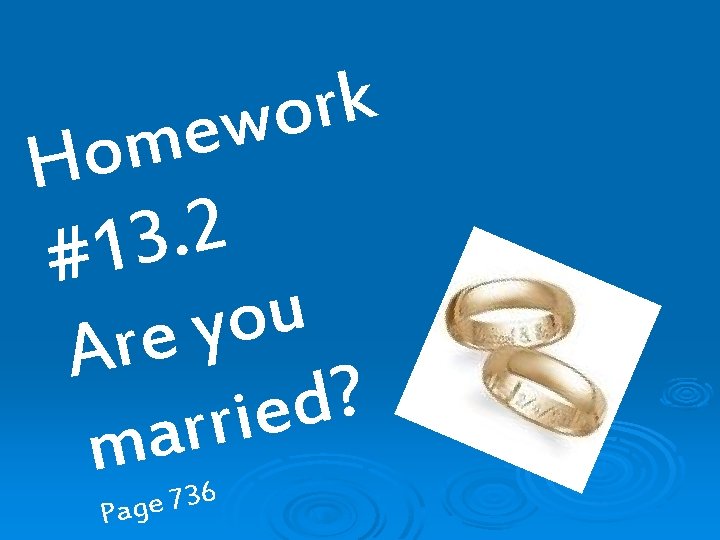
k r o w e m o H 2. 3 #1 u o y e Ar ? d e i r r a m 6 3 7 e Pag
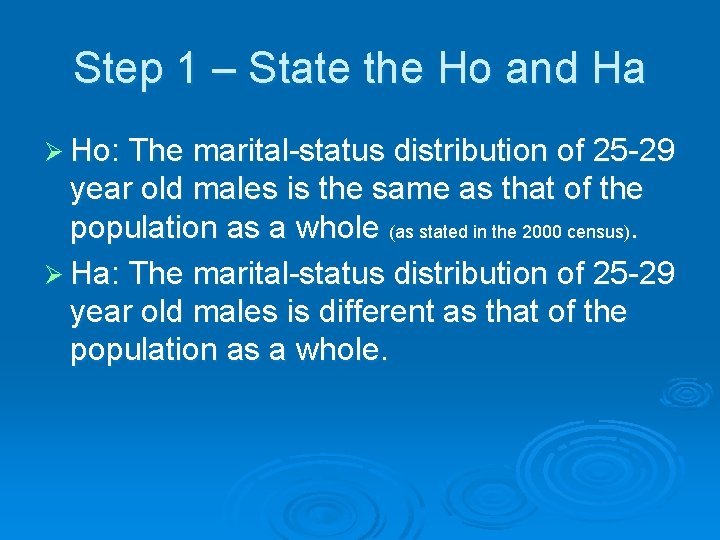
Step 1 – State the Ho and Ha Ø Ho: The marital-status distribution of 25 -29 year old males is the same as that of the population as a whole (as stated in the 2000 census). Ø Ha: The marital-status distribution of 25 -29 year old males is different as that of the population as a whole.
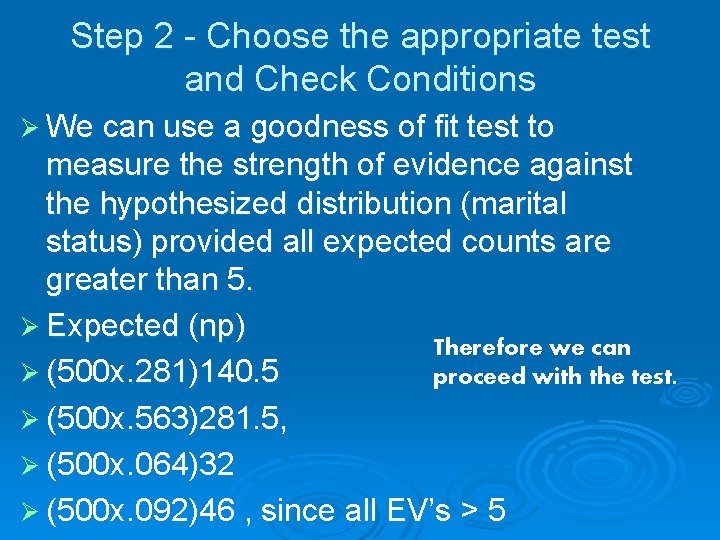
Step 2 - Choose the appropriate test and Check Conditions Ø We can use a goodness of fit test to measure the strength of evidence against the hypothesized distribution (marital status) provided all expected counts are greater than 5. Ø Expected (np) Therefore we can Ø (500 x. 281)140. 5 proceed with the test. Ø (500 x. 563)281. 5, Ø (500 x. 064)32 Ø (500 x. 092)46 , since all EV’s > 5
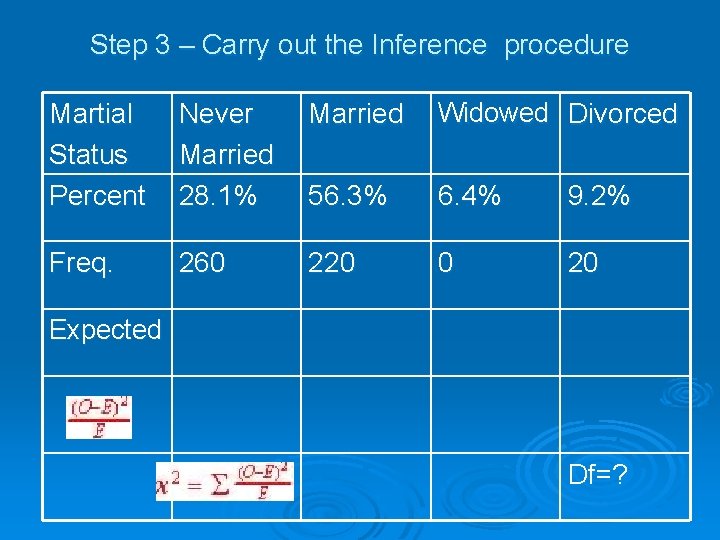
Step 3 – Carry out the Inference procedure Martial Status Percent Never Married 28. 1% Married Widowed Divorced 56. 3% 6. 4% 9. 2% Freq. 260 220 0 20 Expected Df=?
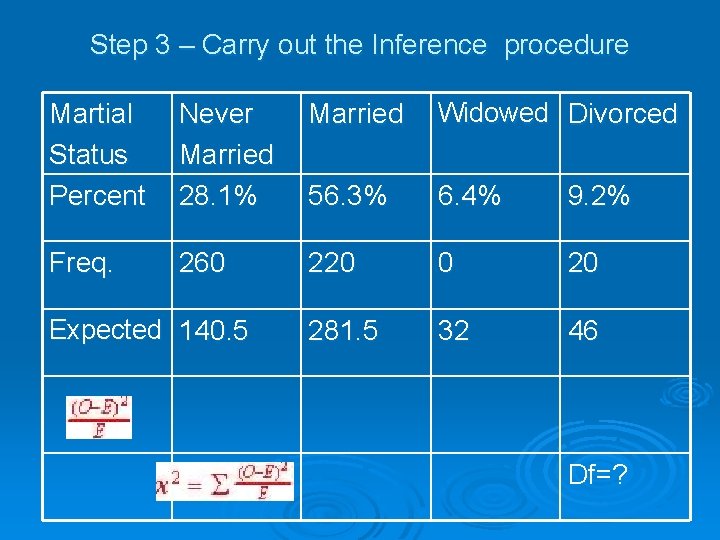
Step 3 – Carry out the Inference procedure Martial Status Percent Never Married 28. 1% Married Widowed Divorced 56. 3% 6. 4% 9. 2% Freq. 260 220 0 20 281. 5 32 46 Expected 140. 5 Df=?
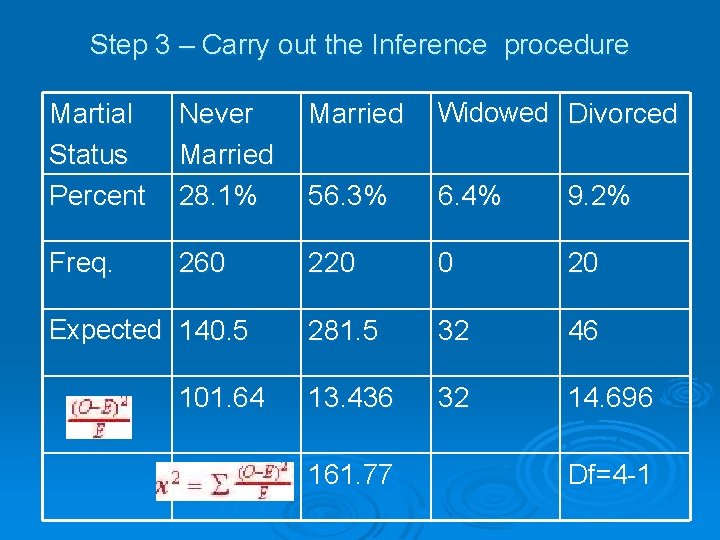
Step 3 – Carry out the Inference procedure Martial Status Percent Never Married 28. 1% Married Widowed Divorced 56. 3% 6. 4% 9. 2% Freq. 260 220 0 20 281. 5 32 46 13. 436 32 14. 696 Expected 140. 5 101. 64 161. 77 Df=4 -1
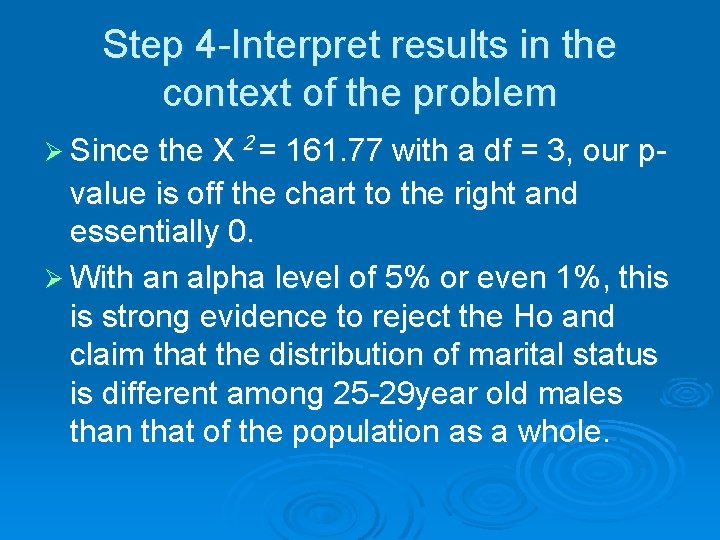
Step 4 -Interpret results in the context of the problem Ø Since the X 2 = 161. 77 with a df = 3, our p- value is off the chart to the right and essentially 0. Ø With an alpha level of 5% or even 1%, this is strong evidence to reject the Ho and claim that the distribution of marital status is different among 25 -29 year old males than that of the population as a whole.
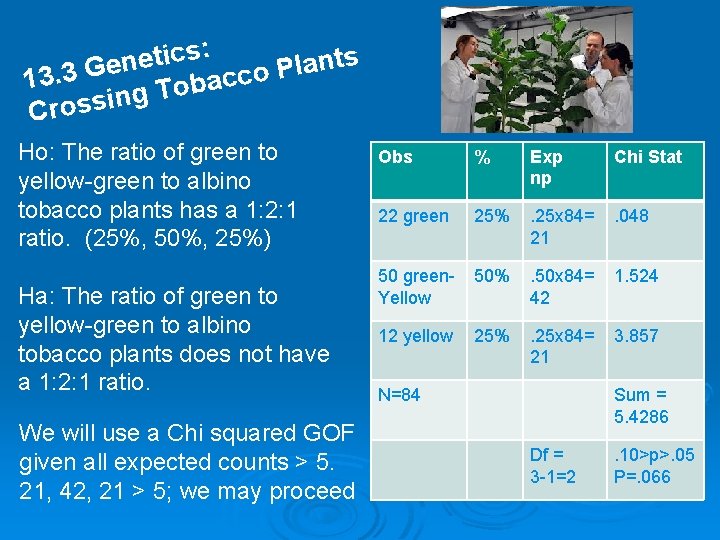
s: c i t ts e n n a e l G P o c c 13. 3 a b To g n i s Cros Ho: The ratio of green to yellow-green to albino tobacco plants has a 1: 2: 1 ratio. (25%, 50%, 25%) Ha: The ratio of green to yellow-green to albino tobacco plants does not have a 1: 2: 1 ratio. We will use a Chi squared GOF given all expected counts > 5. 21, 42, 21 > 5; we may proceed Obs % Exp np Chi Stat 22 green 25% . 25 x 84= 21 . 048 50 green. Yellow 50% . 50 x 84= 42 1. 524 12 yellow 25% . 25 x 84= 21 3. 857 N=84 Sum = 5. 4286 Df = 3 -1=2 . 10>p>. 05 P=. 066
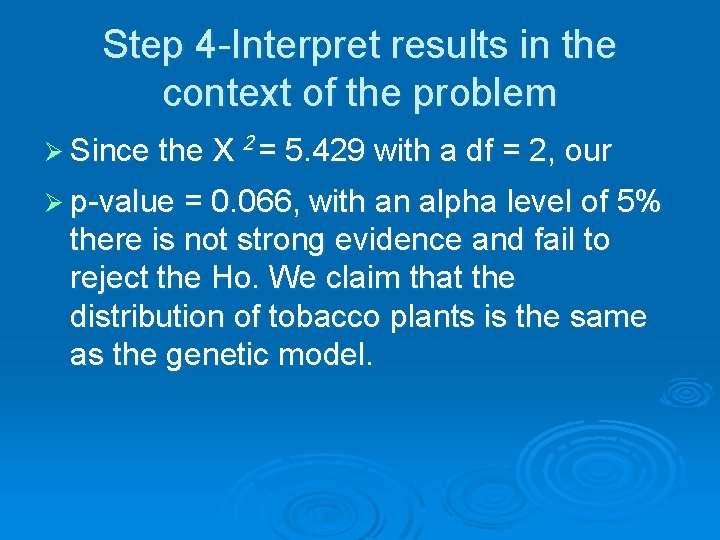
Step 4 -Interpret results in the context of the problem Ø Since the X 2 = 5. 429 with a df = 2, our Ø p-value = 0. 066, with an alpha level of 5% there is not strong evidence and fail to reject the Ho. We claim that the distribution of tobacco plants is the same as the genetic model.
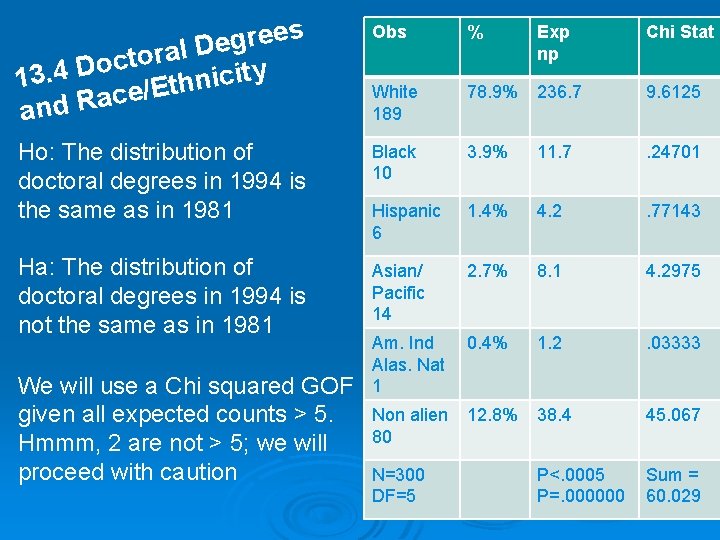
s e e r g e D l a r o ct o D 4. ity c i 13 n h t /E e c a R and Obs % Exp np Chi Stat White 189 78. 9% 236. 7 9. 6125 Ho: The distribution of doctoral degrees in 1994 is the same as in 1981 Black 10 3. 9% 11. 7 . 24701 Hispanic 6 1. 4% 4. 2 . 77143 Ha: The distribution of doctoral degrees in 1994 is not the same as in 1981 Asian/ Pacific 14 2. 7% 8. 1 4. 2975 0. 4% 1. 2 . 03333 We will use a Chi squared GOF given all expected counts > 5. Hmmm, 2 are not > 5; we will proceed with caution Am. Ind Alas. Nat 1 Non alien 80 12. 8% 38. 4 45. 067 P<. 0005 P=. 000000 Sum = 60. 029 N=300 DF=5
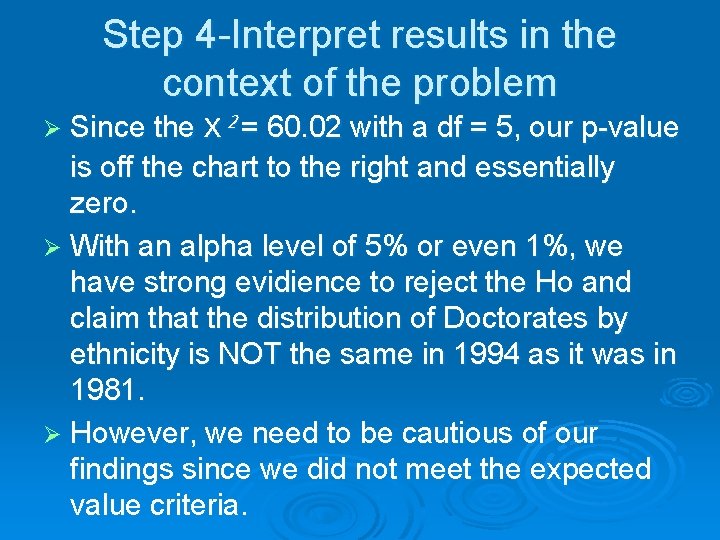
Step 4 -Interpret results in the context of the problem Ø Since the X 2 = 60. 02 with a df = 5, our p-value is off the chart to the right and essentially zero. Ø With an alpha level of 5% or even 1%, we have strong evidience to reject the Ho and claim that the distribution of Doctorates by ethnicity is NOT the same in 1994 as it was in 1981. Ø However, we need to be cautious of our findings since we did not meet the expected value criteria.
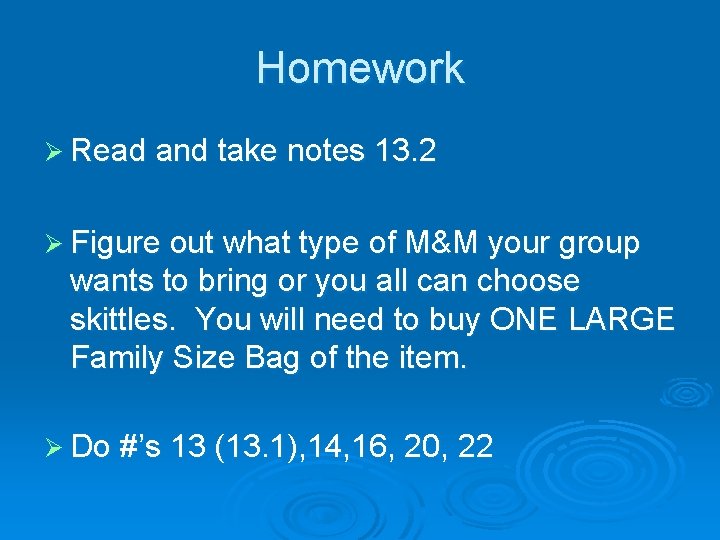
Homework Ø Read and take notes 13. 2 Ø Figure out what type of M&M your group wants to bring or you all can choose skittles. You will need to buy ONE LARGE Family Size Bag of the item. Ø Do #’s 13 (13. 1), 14, 16, 20, 22
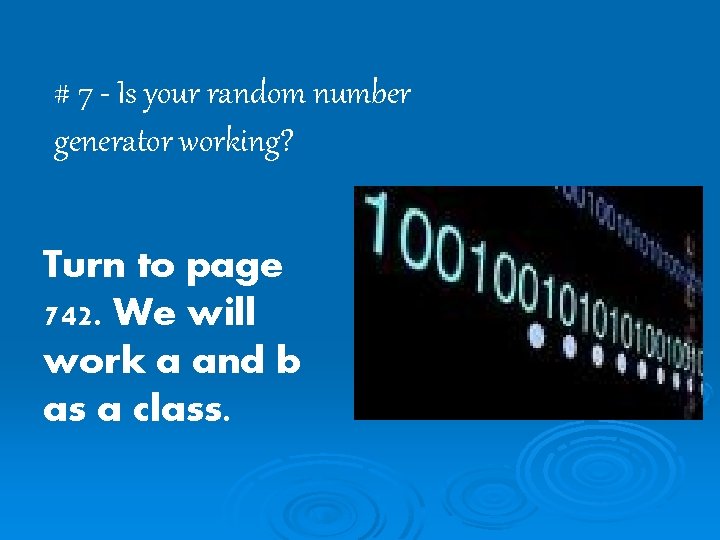
# 7 - Is your random number generator working? Turn to page 742. We will work a and b as a class.
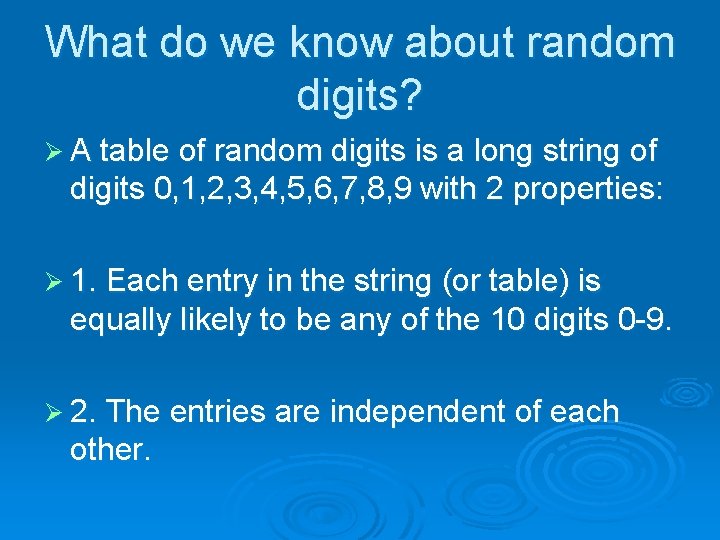
What do we know about random digits? Ø A table of random digits is a long string of digits 0, 1, 2, 3, 4, 5, 6, 7, 8, 9 with 2 properties: Ø 1. Each entry in the string (or table) is equally likely to be any of the 10 digits 0 -9. Ø 2. The entries are independent of each other.
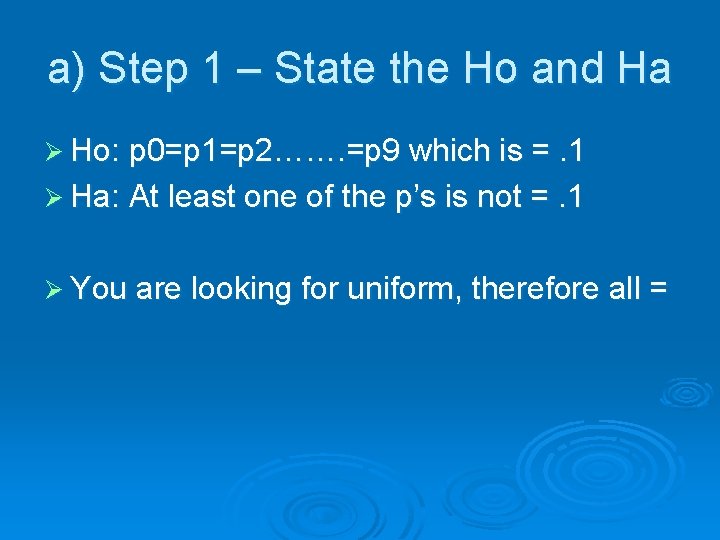
a) Step 1 – State the Ho and Ha Ø Ho: p 0=p 1=p 2……. =p 9 which is =. 1 Ø Ha: At least one of the p’s is not =. 1 Ø You are looking for uniform, therefore all =
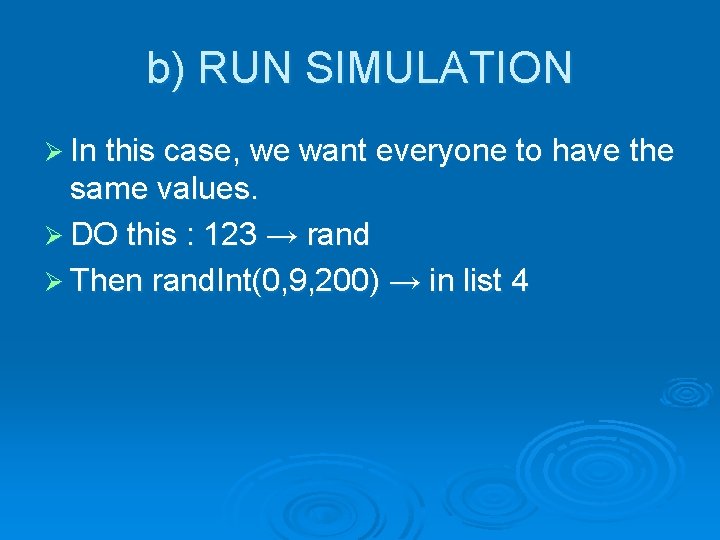
b) RUN SIMULATION Ø In this case, we want everyone to have the same values. Ø DO this : 123 → rand Ø Then rand. Int(0, 9, 200) → in list 4
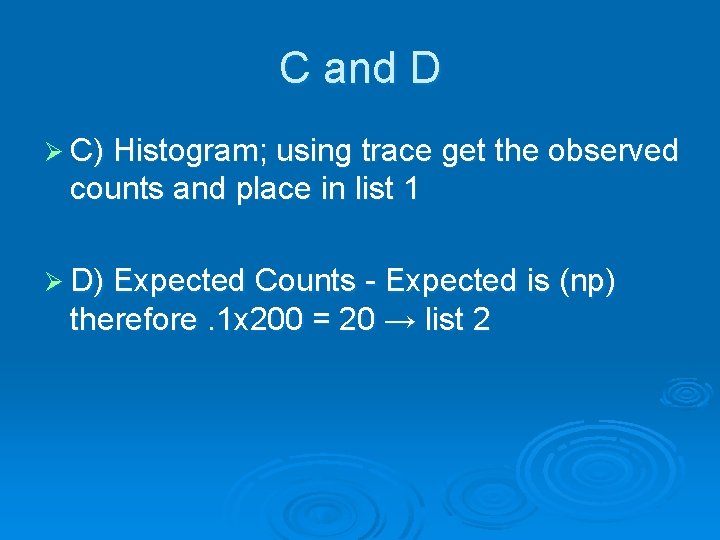
C and D Ø C) Histogram; using trace get the observed counts and place in list 1 Ø D) Expected Counts - Expected is (np) therefore. 1 x 200 = 20 → list 2
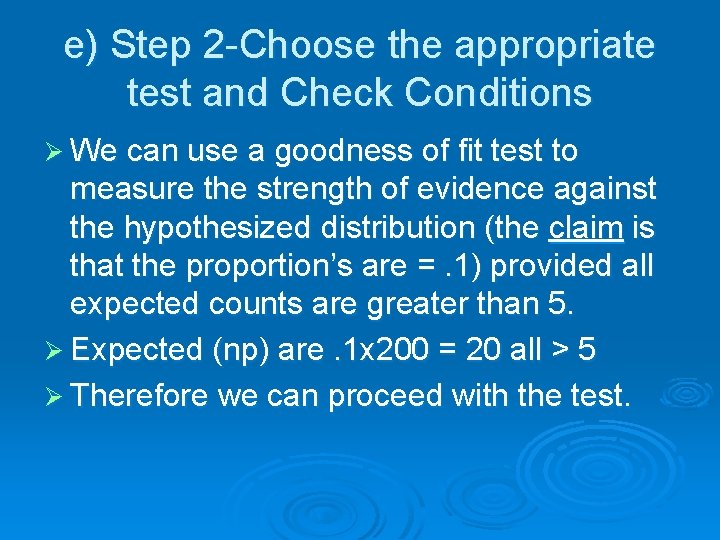
e) Step 2 -Choose the appropriate test and Check Conditions Ø We can use a goodness of fit test to measure the strength of evidence against the hypothesized distribution (the claim is that the proportion’s are =. 1) provided all expected counts are greater than 5. Ø Expected (np) are. 1 x 200 = 20 all > 5 Ø Therefore we can proceed with the test.
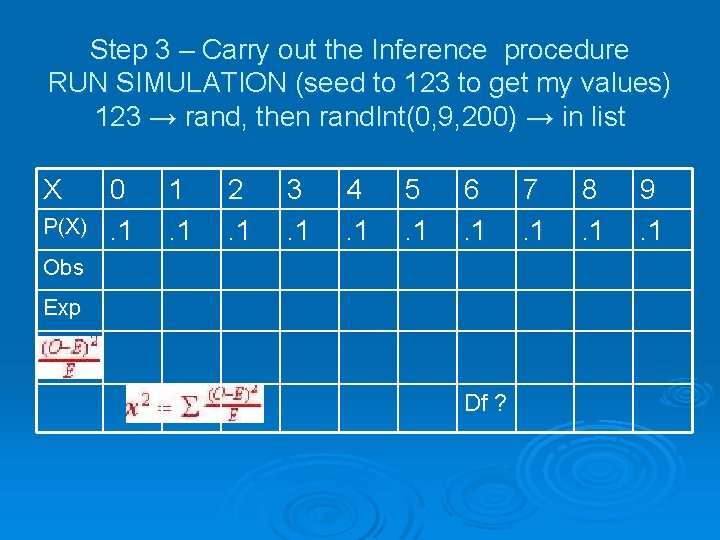
Step 3 – Carry out the Inference procedure RUN SIMULATION (seed to 123 to get my values) 123 → rand, then rand. Int(0, 9, 200) → in list X P(X) 0. 1 1. 1 2. 1 3. 1 4. 1 5. 1 6. 1 Obs Exp Df ? 7. 1 8. 1 9. 1
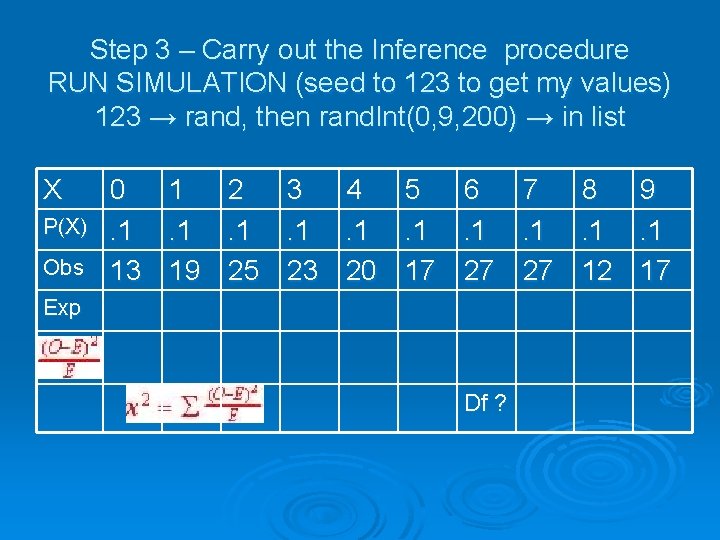
Step 3 – Carry out the Inference procedure RUN SIMULATION (seed to 123 to get my values) 123 → rand, then rand. Int(0, 9, 200) → in list X P(X) Obs 0. 1 13 1. 1 19 2. 1 25 3. 1 23 4. 1 20 5. 1 17 6. 1 27 Exp Df ? 7. 1 27 8. 1 12 9. 1 17
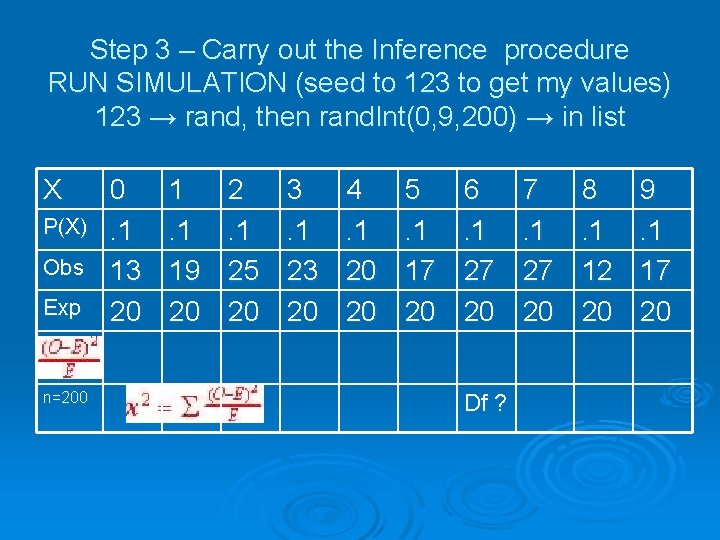
Step 3 – Carry out the Inference procedure RUN SIMULATION (seed to 123 to get my values) 123 → rand, then rand. Int(0, 9, 200) → in list X P(X) Obs Exp n=200 0. 1 13 20 1. 1 19 20 2. 1 25 20 3. 1 23 20 4. 1 20 20 5. 1 17 20 6. 1 27 20 Df ? 7. 1 27 20 8. 1 12 20 9. 1 17 20
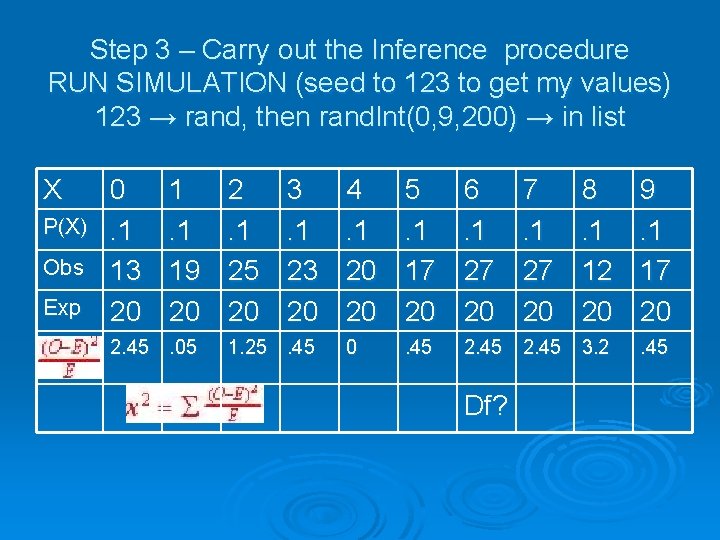
Step 3 – Carry out the Inference procedure RUN SIMULATION (seed to 123 to get my values) 123 → rand, then rand. Int(0, 9, 200) → in list X P(X) Obs Exp 0. 1 13 20 1. 1 19 20 2. 45. 05 2. 1 25 20 3. 1 23 20 1. 25. 45 4. 1 20 20 5. 1 17 20 6. 1 27 20 8. 1 12 20 9. 1 17 20 0 . 45 2. 45 3. 2 . 45 Df? 7. 1 27 20
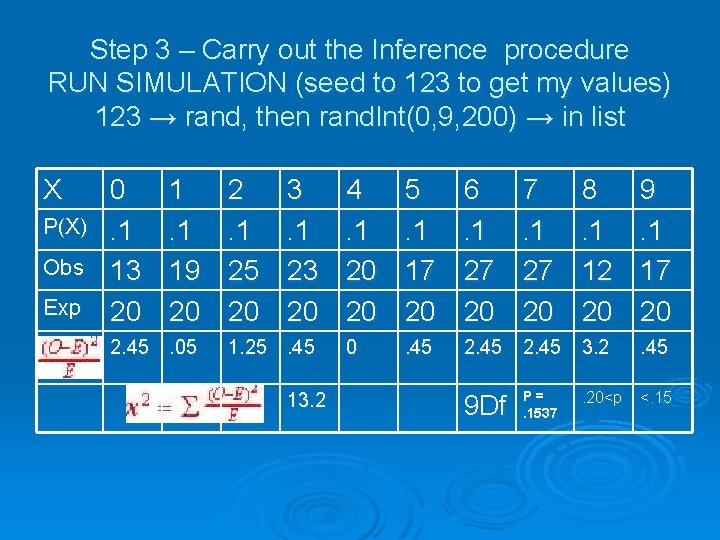
Step 3 – Carry out the Inference procedure RUN SIMULATION (seed to 123 to get my values) 123 → rand, then rand. Int(0, 9, 200) → in list X P(X) Obs Exp 0. 1 13 20 1. 1 19 20 2. 45. 05 2. 1 25 20 3. 1 23 20 1. 25. 45 13. 2 4. 1 20 20 5. 1 17 20 6. 1 27 20 8. 1 12 20 9. 1 17 20 0 . 45 2. 45 3. 2 . 45 P=. 1537 <. 15 9 Df 7. 1 27 20 . 20<p
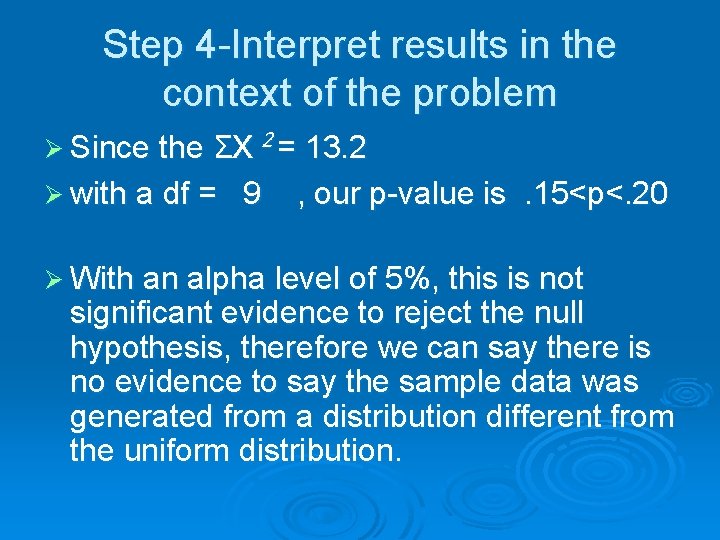
Step 4 -Interpret results in the context of the problem Ø Since the ΣX 2 = 13. 2 Ø with a df = 9 , our p-value is. 15<p<. 20 Ø With an alpha level of 5%, this is not significant evidence to reject the null hypothesis, therefore we can say there is no evidence to say the sample data was generated from a distribution different from the uniform distribution.
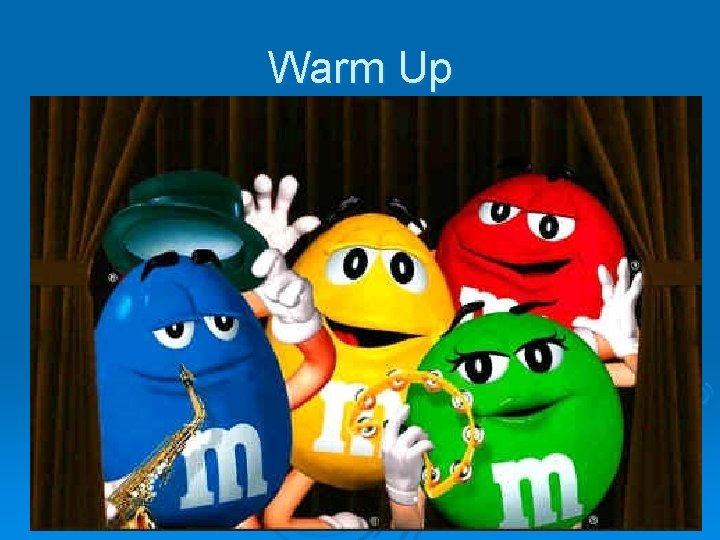
Warm Up
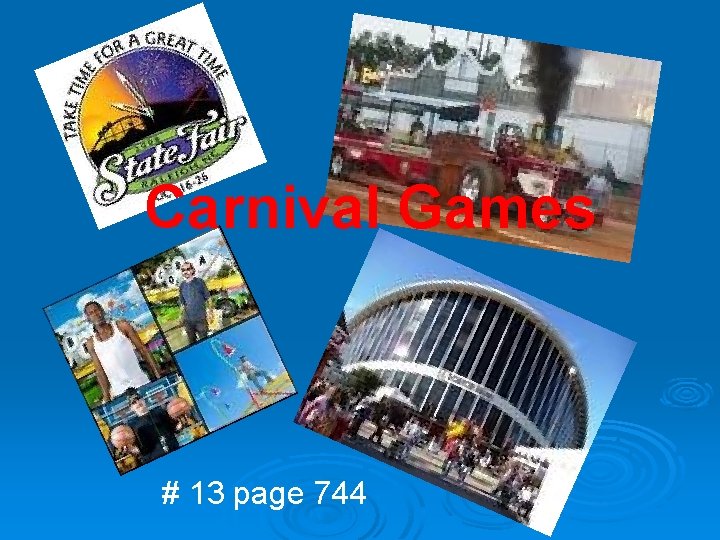
Carnival Games # 13 page 744
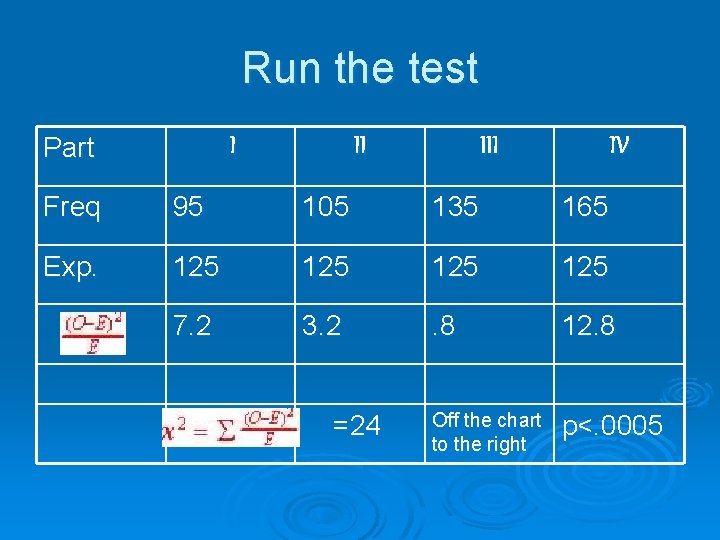
Run the test Part I II IV Freq 95 105 135 165 Exp. 125 125 7. 2 3. 2 . 8 12. 8 Off the chart to the right p<. 0005 =24
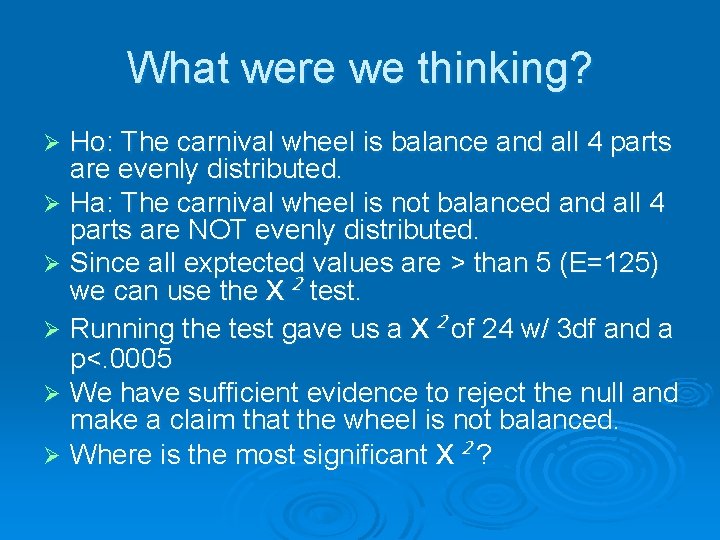
What were we thinking? Ho: The carnival wheel is balance and all 4 parts are evenly distributed. Ø Ha: The carnival wheel is not balanced and all 4 parts are NOT evenly distributed. Ø Since all exptected values are > than 5 (E=125) we can use the X 2 test. Ø Running the test gave us a X 2 of 24 w/ 3 df and a p<. 0005 Ø We have sufficient evidence to reject the null and make a claim that the wheel is not balanced. Ø Where is the most significant X 2 ? Ø
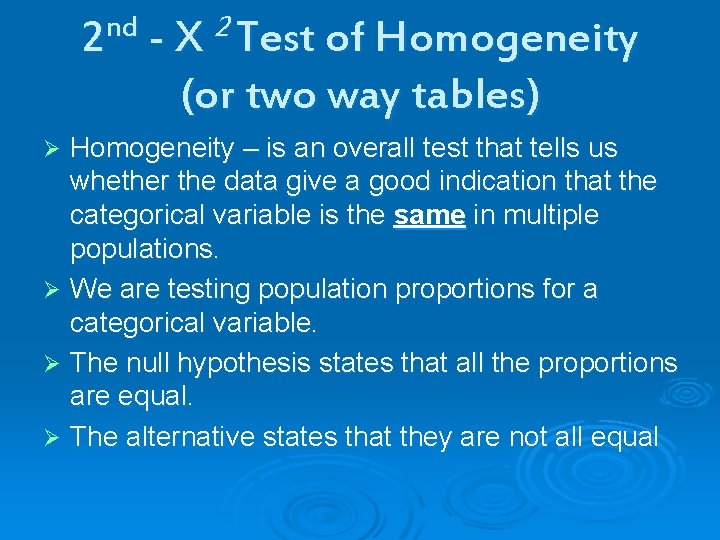
2 nd - X 2 Test of Homogeneity (or two way tables) Homogeneity – is an overall test that tells us whether the data give a good indication that the categorical variable is the same in multiple populations. Ø We are testing population proportions for a categorical variable. Ø The null hypothesis states that all the proportions are equal. Ø The alternative states that they are not all equal Ø
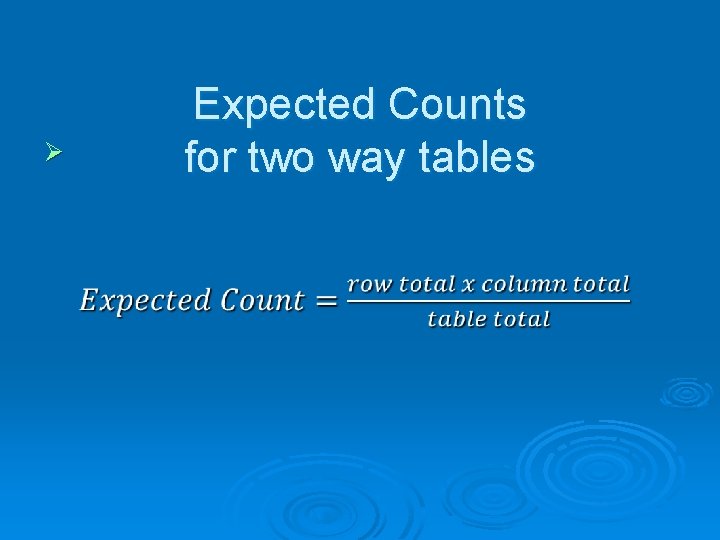
Ø Expected Counts for two way tables
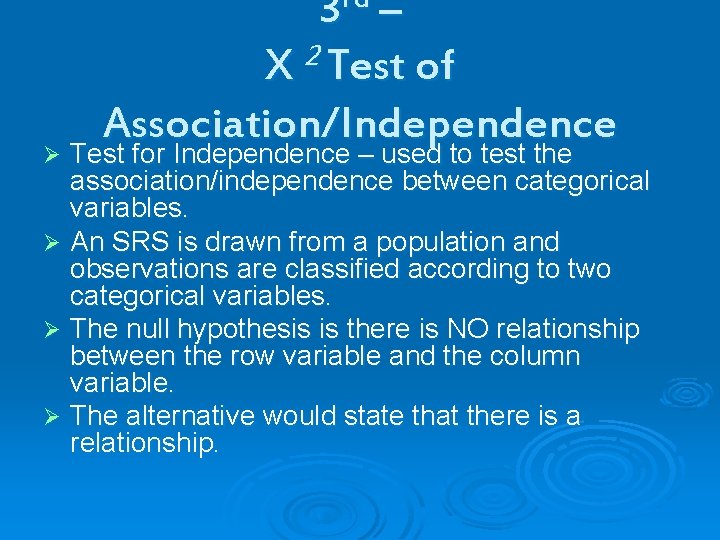
rd 3 – X 2 Test of Association/Independence Test for Independence – used to test the association/independence between categorical variables. Ø An SRS is drawn from a population and observations are classified according to two categorical variables. Ø The null hypothesis is there is NO relationship between the row variable and the column variable. Ø The alternative would state that there is a relationship. Ø
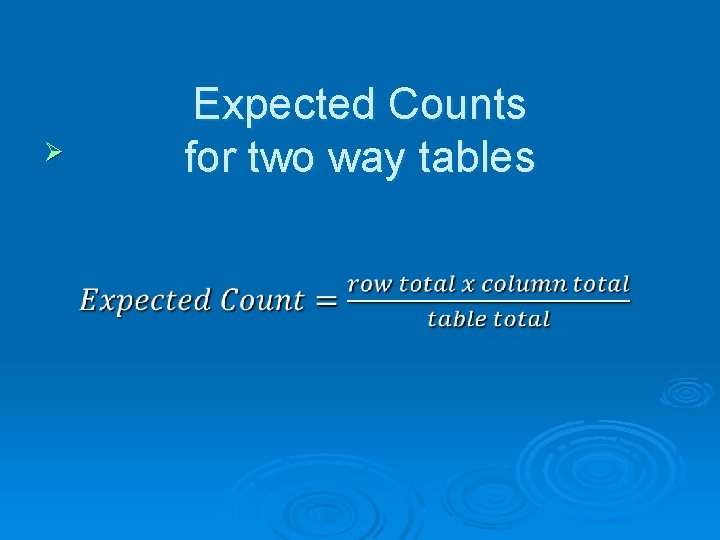
Ø Expected Counts for two way tables
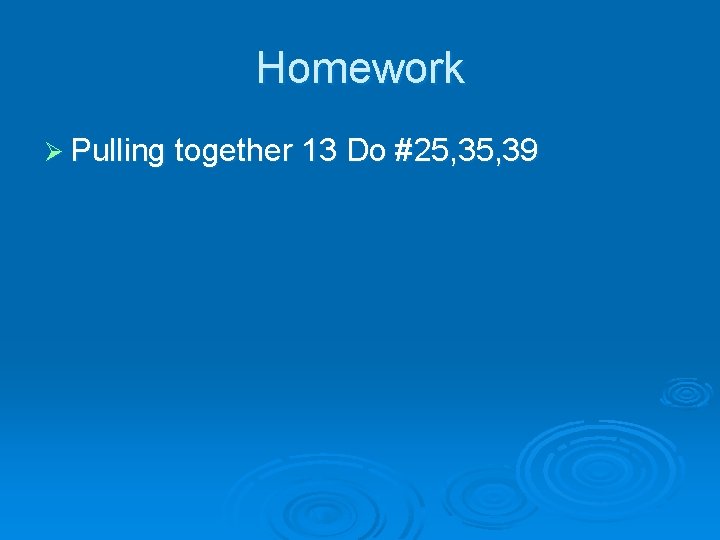
Homework Ø Pulling together 13 Do #25, 39
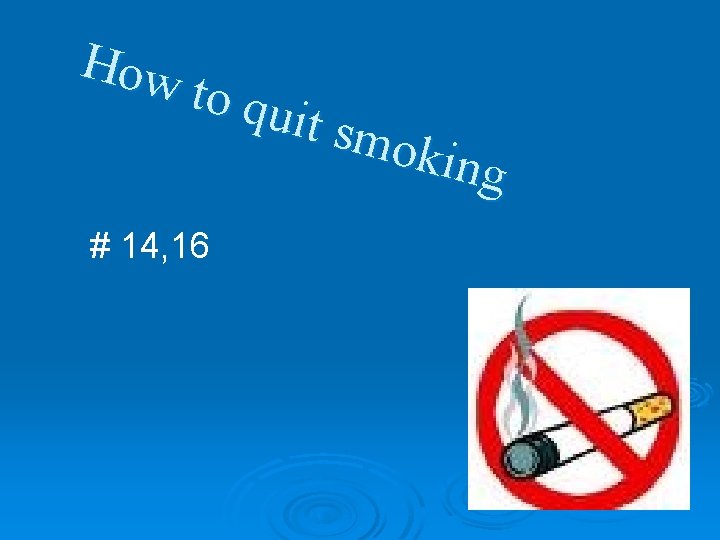
How to qu it sm oking # 14, 16
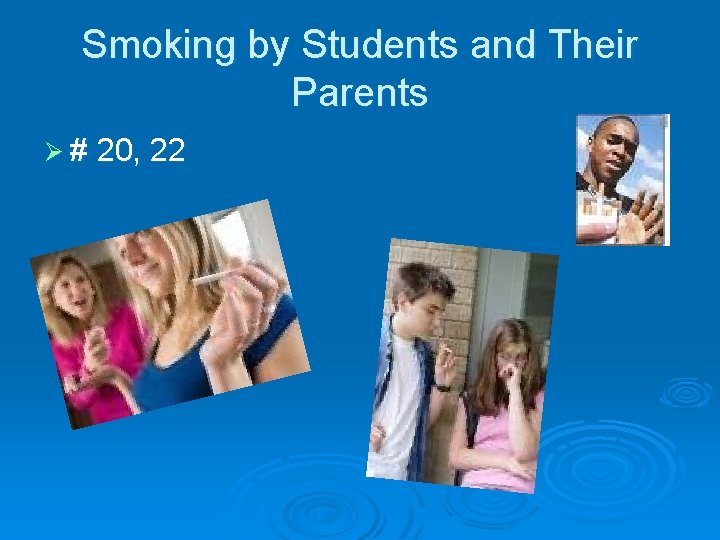
Smoking by Students and Their Parents Ø # 20, 22
Expected frequency symbol
Agenda sistemica y agenda institucional
Fspos vägledning för kontinuitetshantering
Novell typiska drag
Tack för att ni lyssnade bild
Vad står k.r.å.k.a.n för
Varför kallas perioden 1918-1939 för mellankrigstiden
En lathund för arbete med kontinuitetshantering
Särskild löneskatt för pensionskostnader
Personlig tidbok fylla i
A gastrica
Vad är densitet
Datorkunskap för nybörjare
Stig kerman
Mall debattartikel
Delegerande ledarstil
Nyckelkompetenser för livslångt lärande
Påbyggnader för flakfordon
Arkimedes princip formel
Offentlig förvaltning
Bo bergman jag fryser om dina händer
Presentera för publik crossboss
Teckenspråk minoritetsspråk argument
Plats för toran ark
Treserva lathund
Mjälthilus
Bästa kameran för astrofoto
Cks
Verifikationsplan
Mat för unga idrottare
Verktyg för automatisering av utbetalningar
Rutin för avvikelsehantering
Smärtskolan kunskap för livet
Ministerstyre för och nackdelar
Tack för att ni har lyssnat
Hur ser ett referat ut
Redogör för vad psykologi är
Borstål, egenskaper
Tack för att ni har lyssnat
Borra hål för knoppar
Orubbliga rättigheter
Formel för standardavvikelse
Tack för att ni har lyssnat
Rita perspektiv
Vad är verksamhetsanalys
Tobinskatten för och nackdelar
Toppslätskivling effekt