Text Retrieval and Mining Software Engineering Cheng Xiang
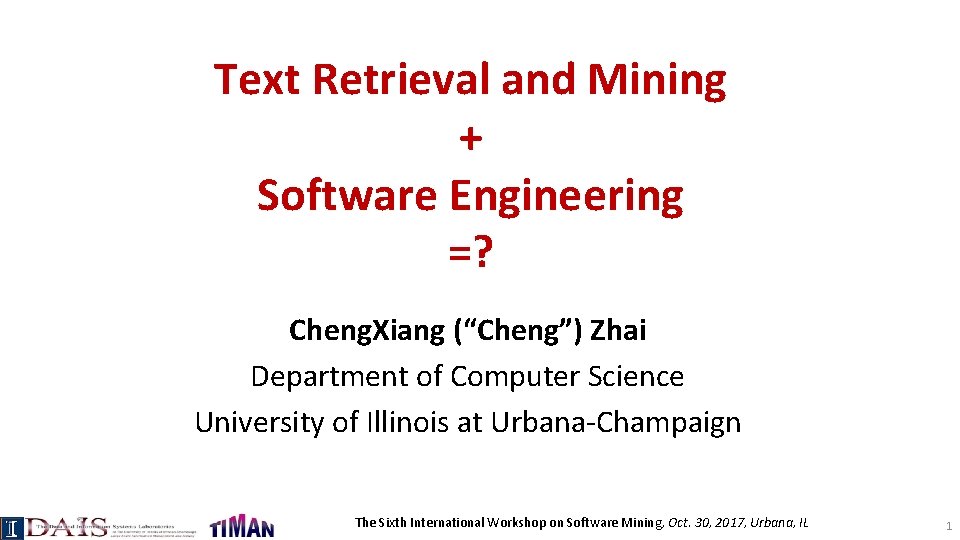
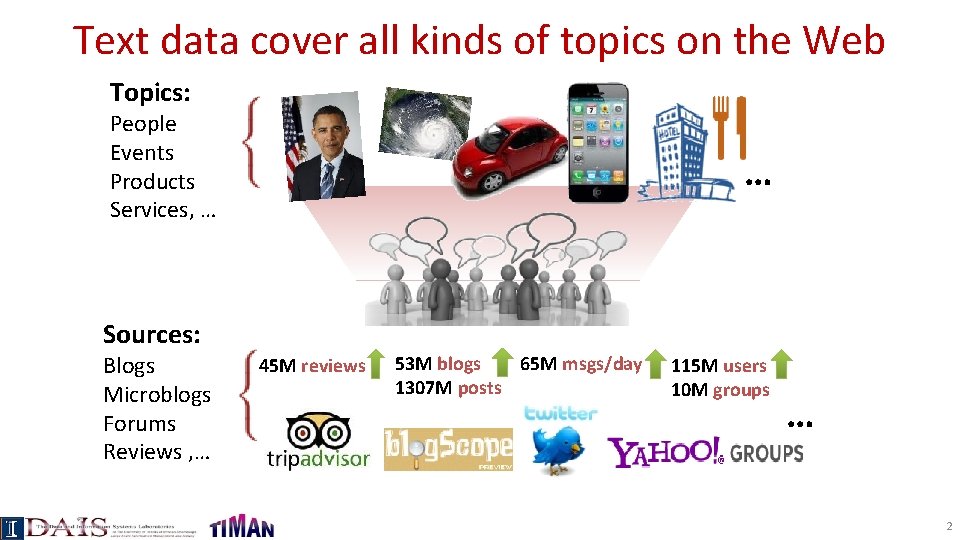
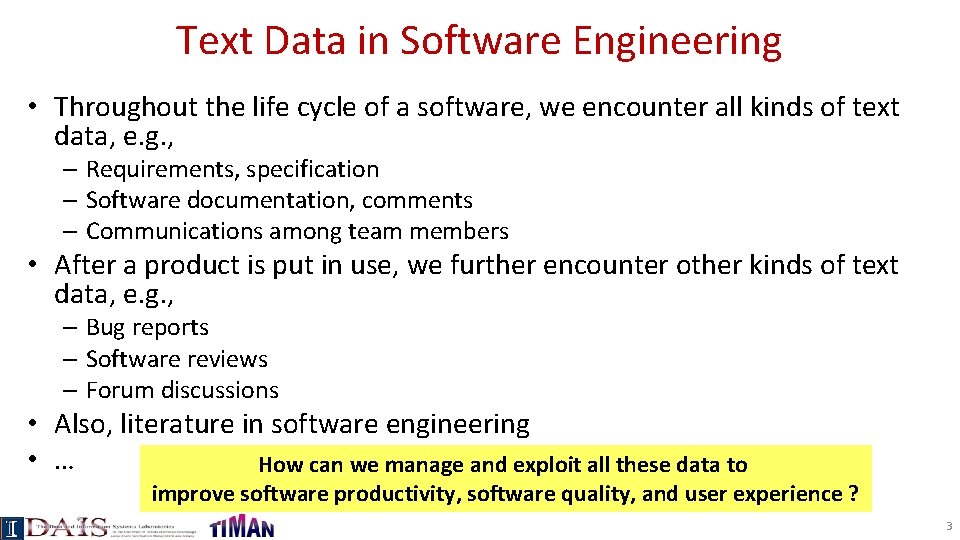
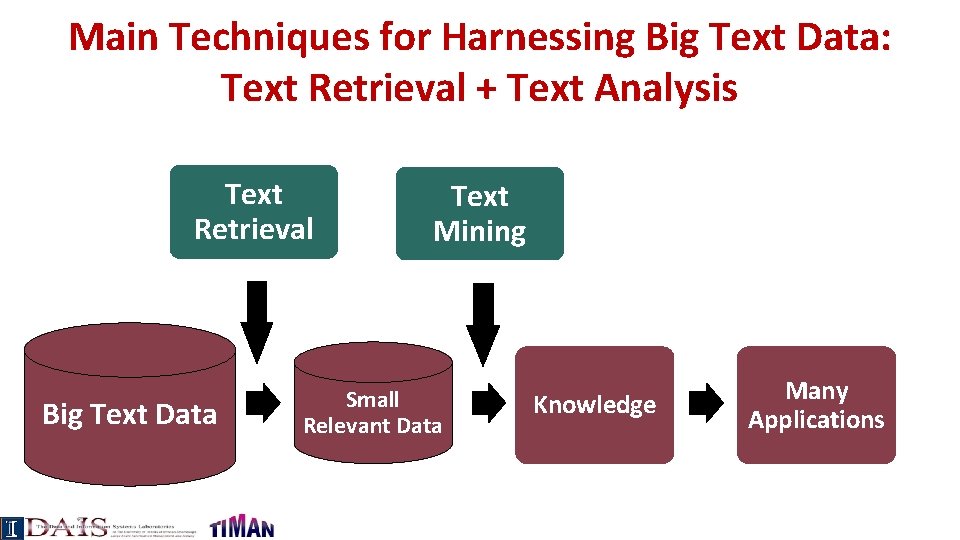
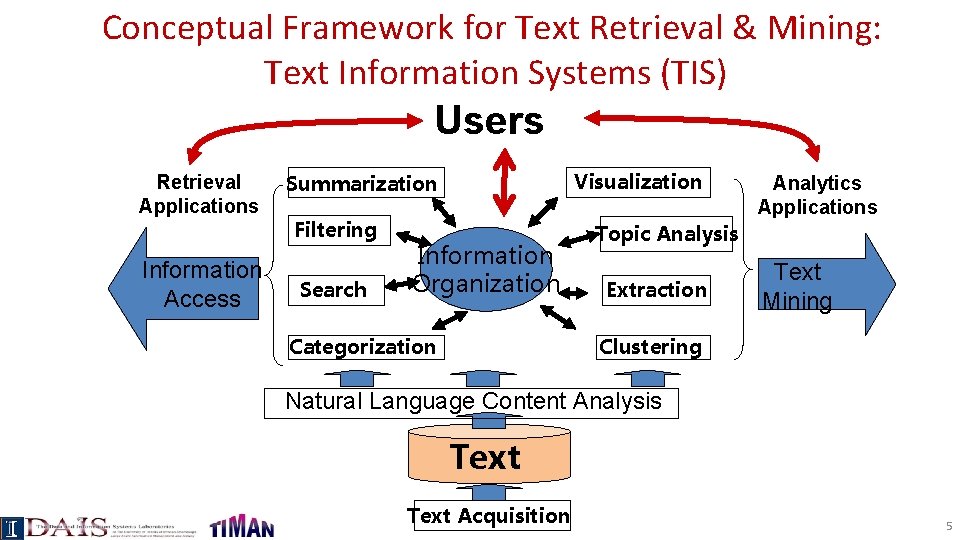
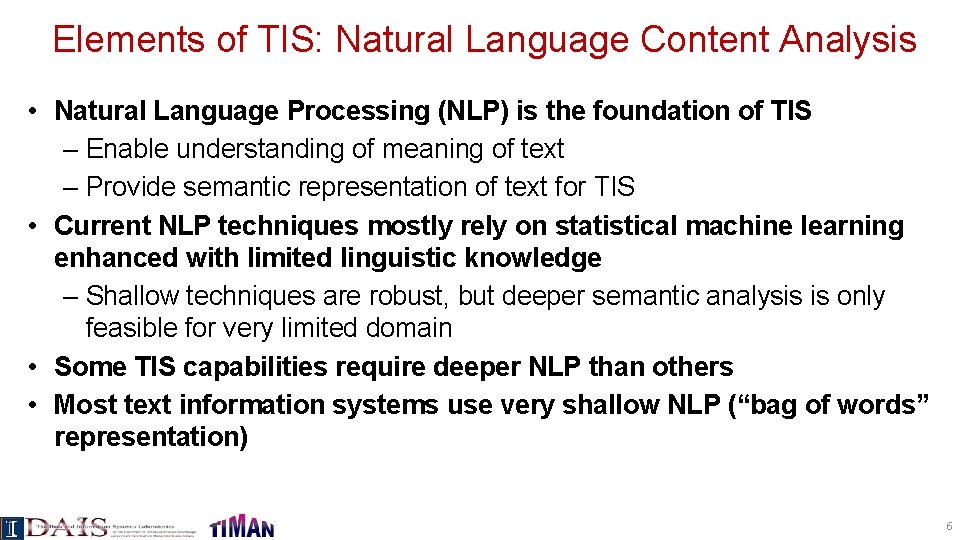
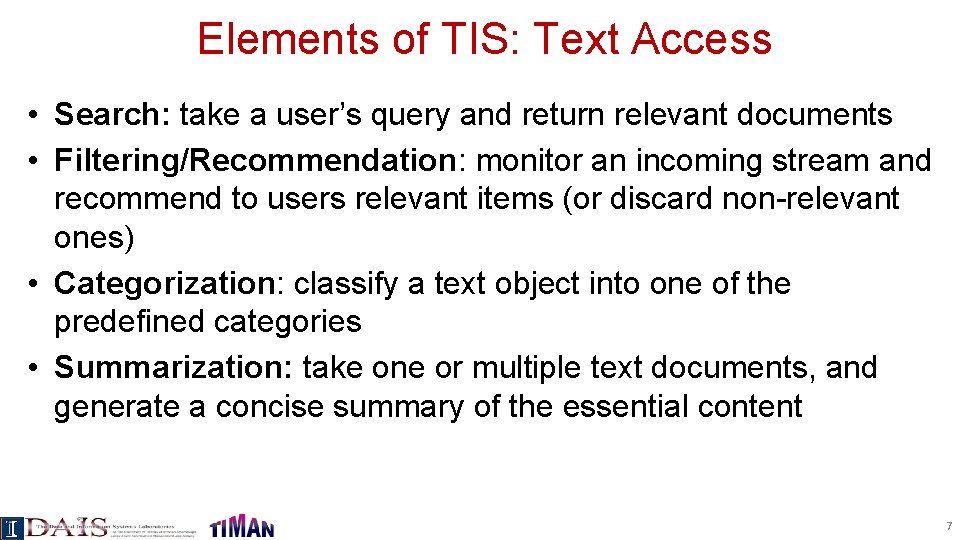
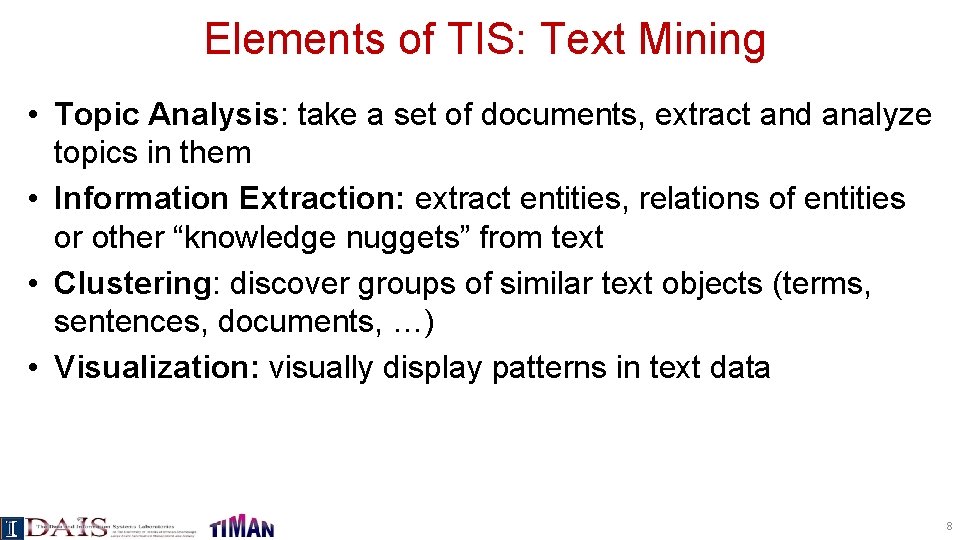
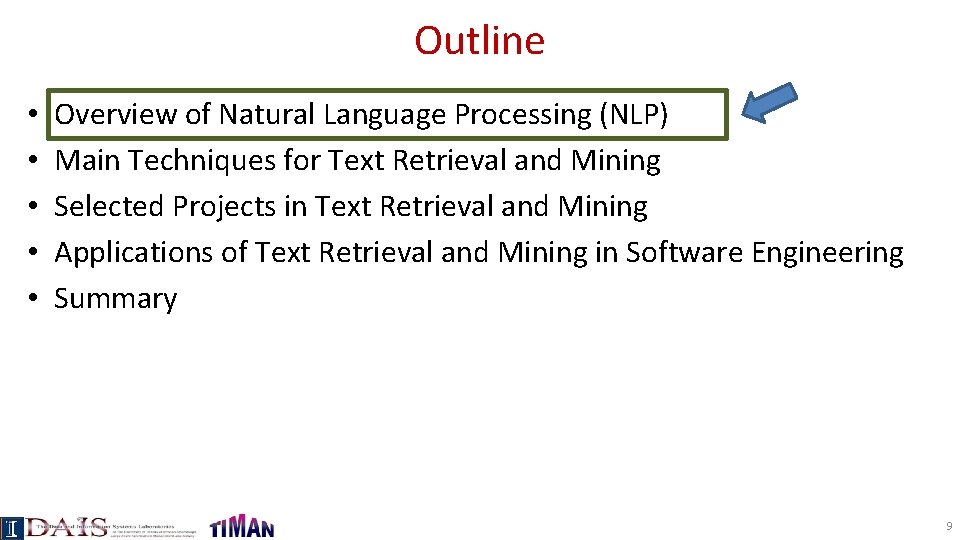
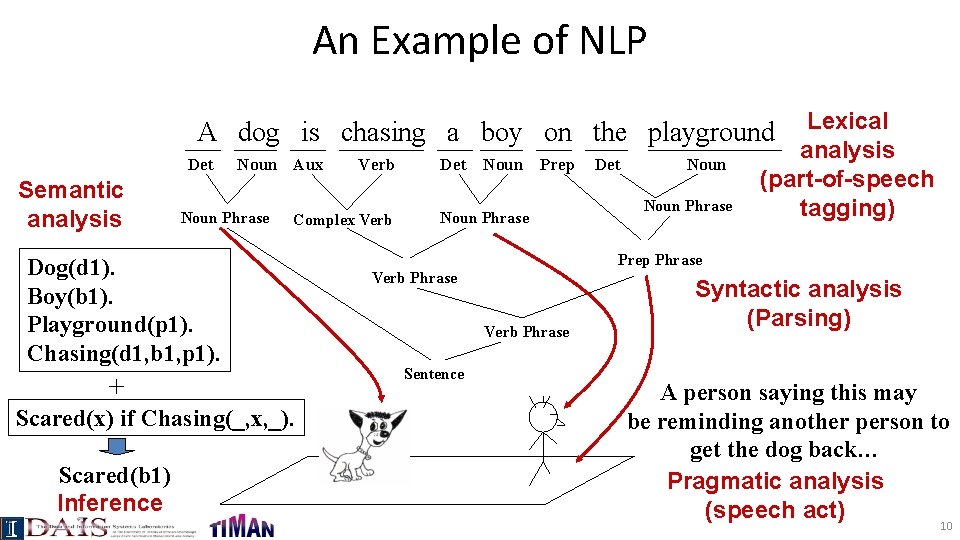
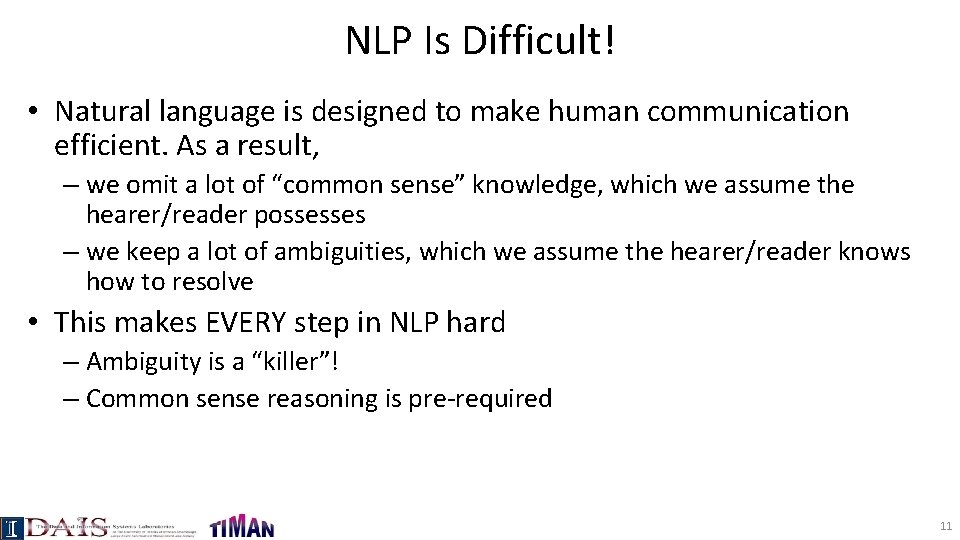
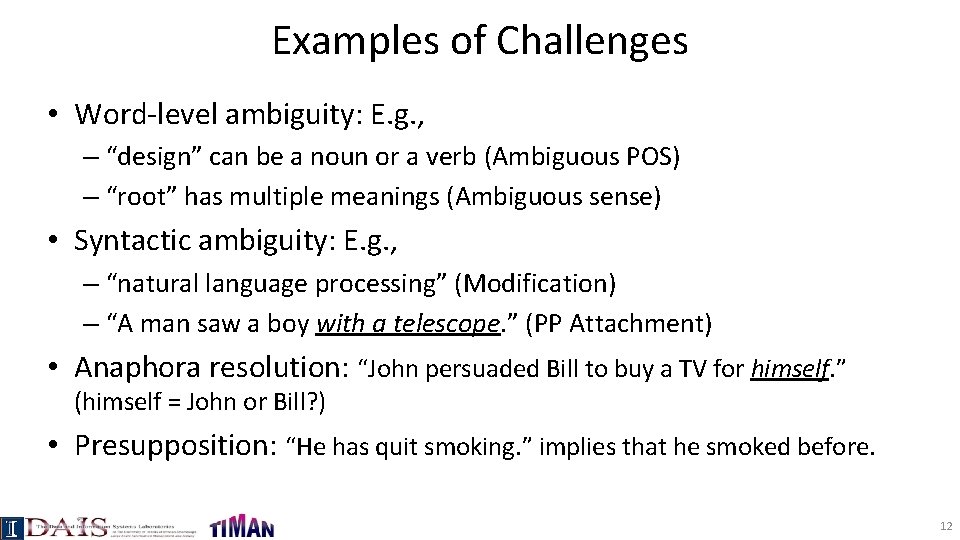
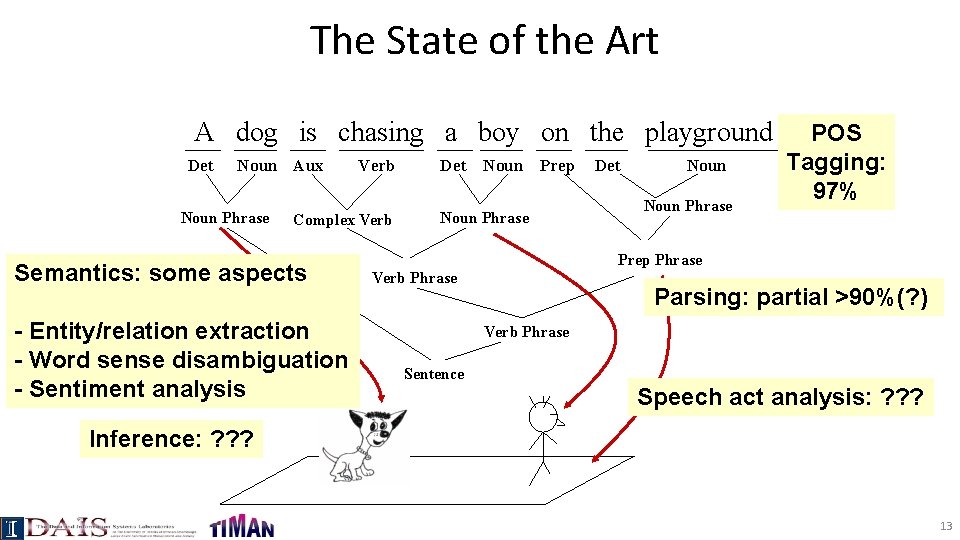
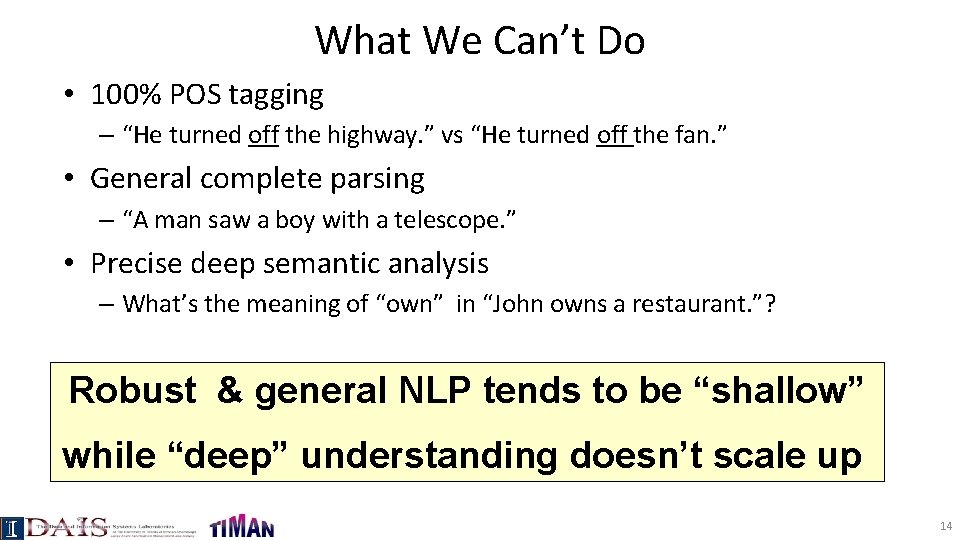
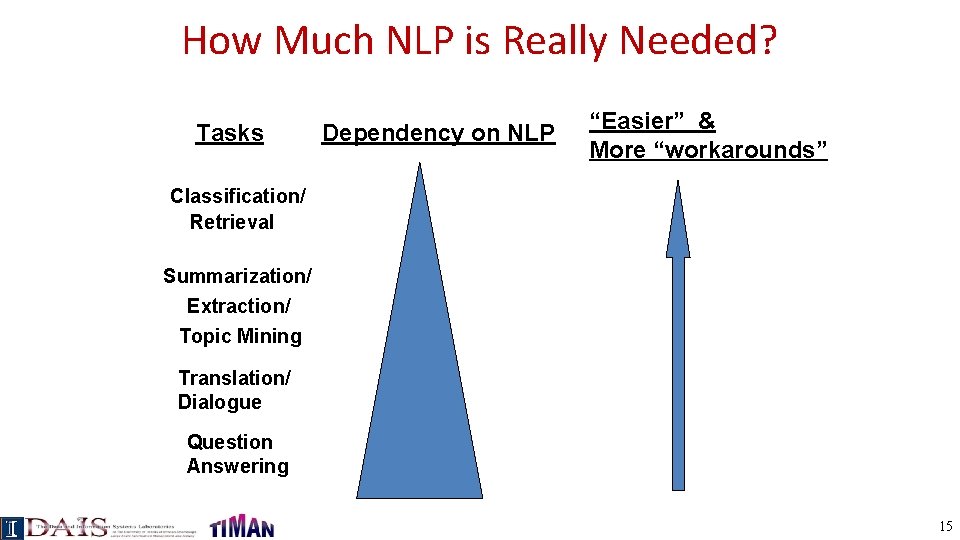
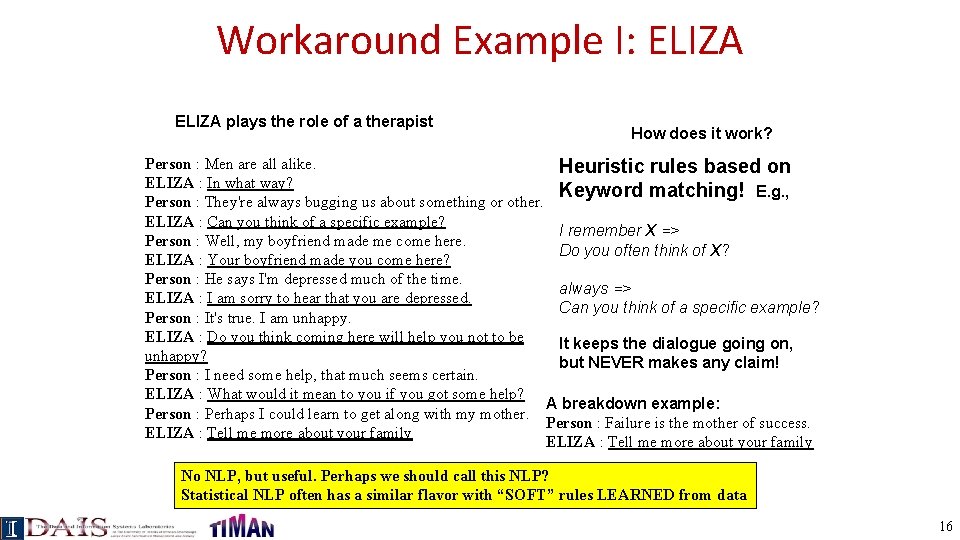
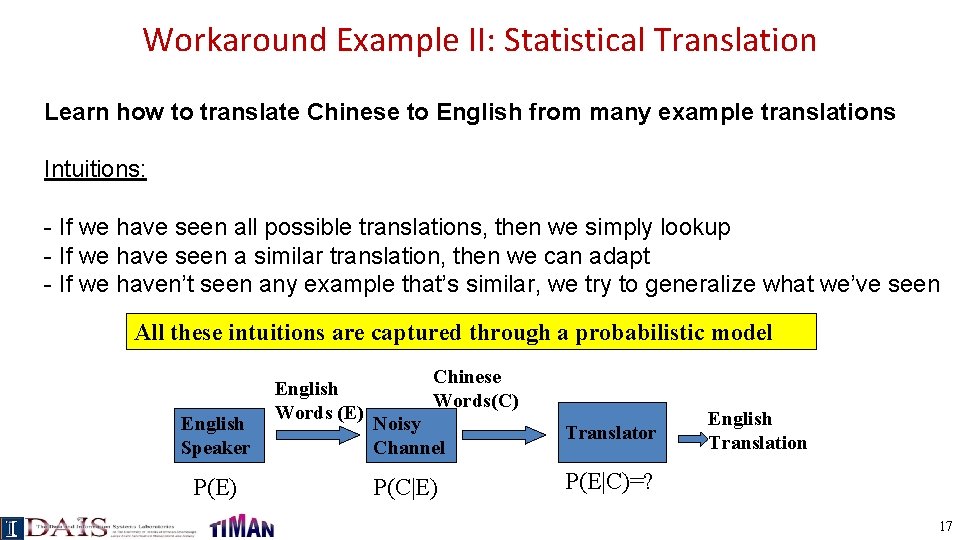
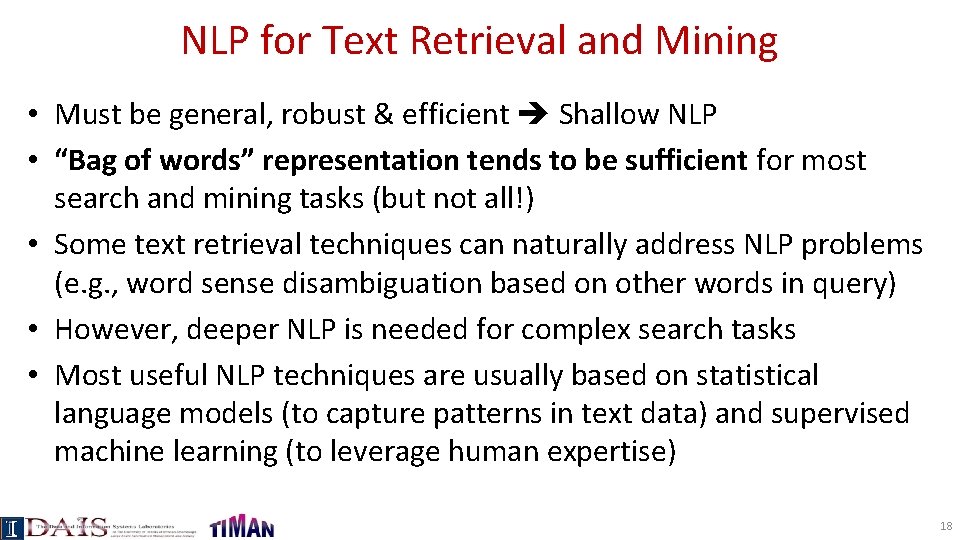
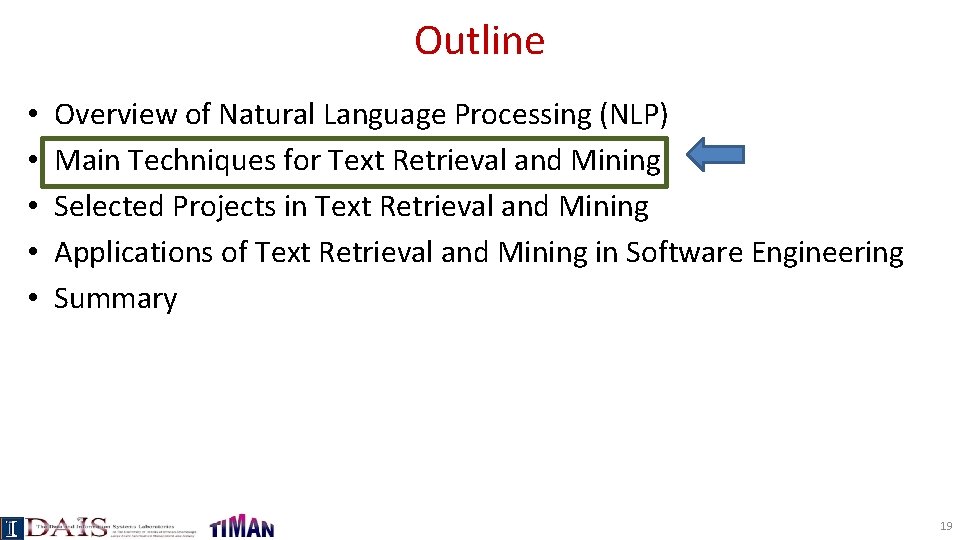
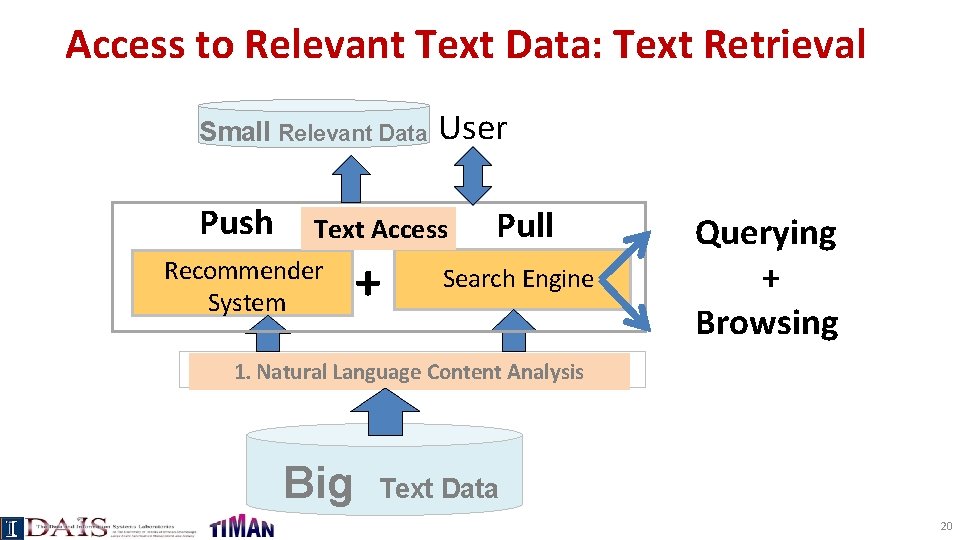
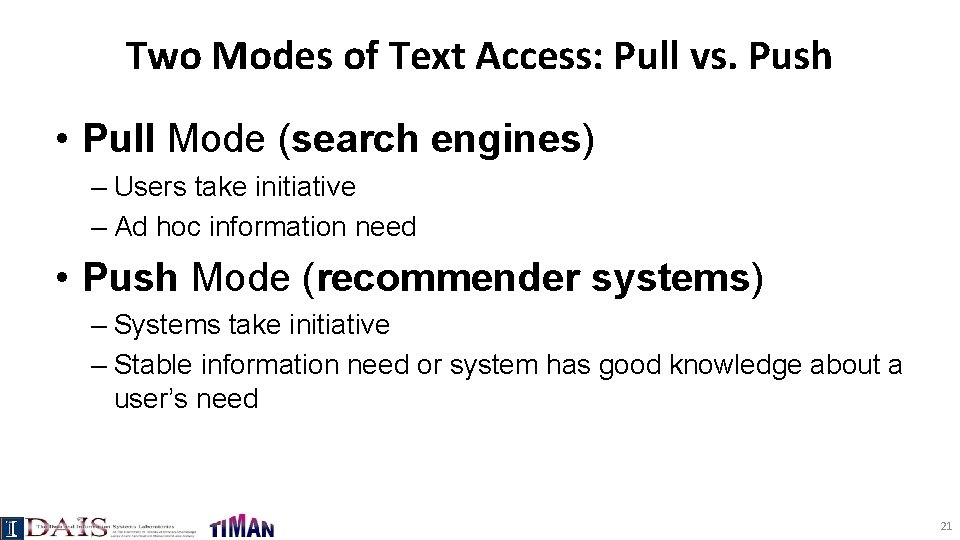
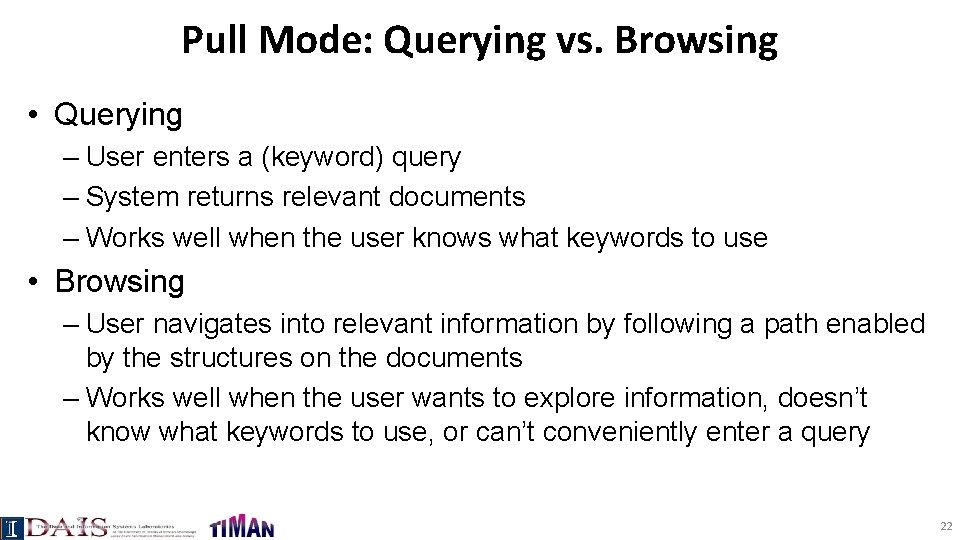
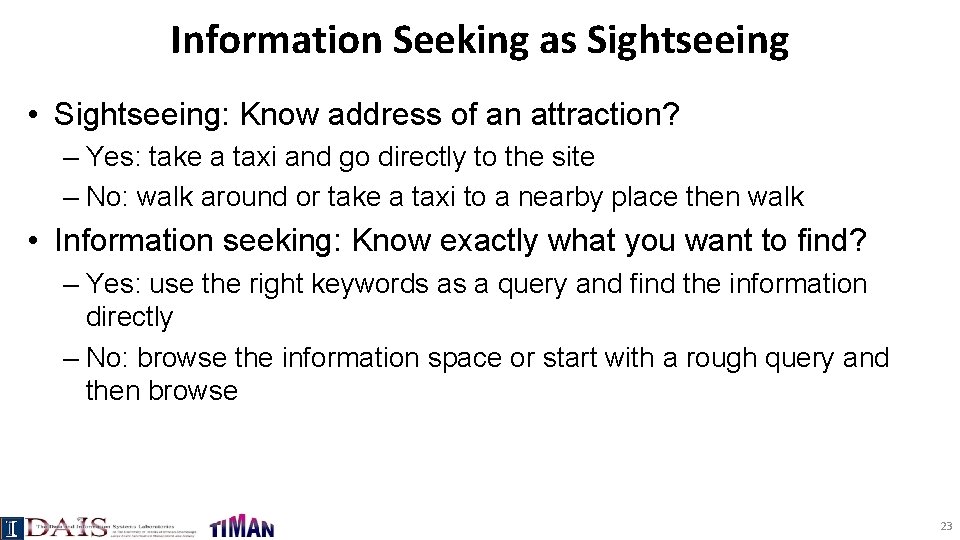
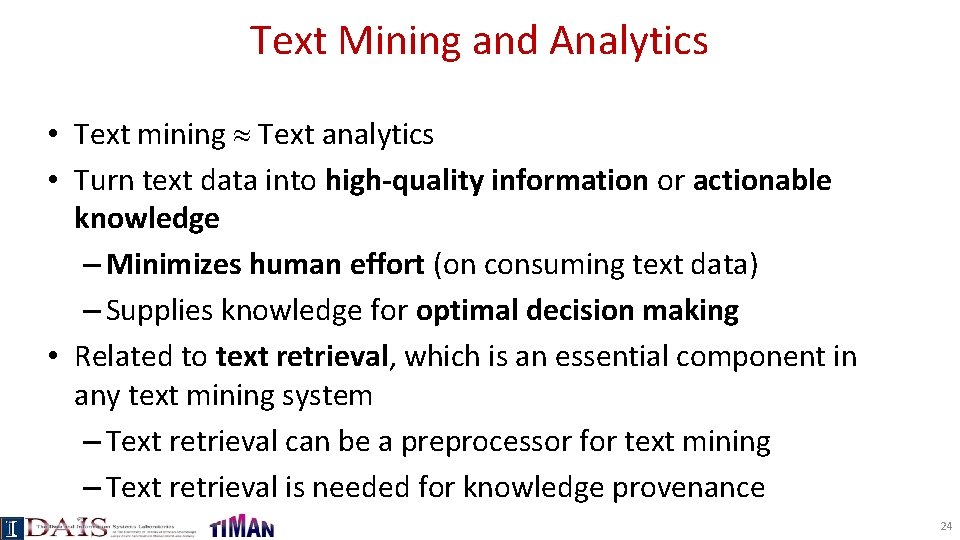
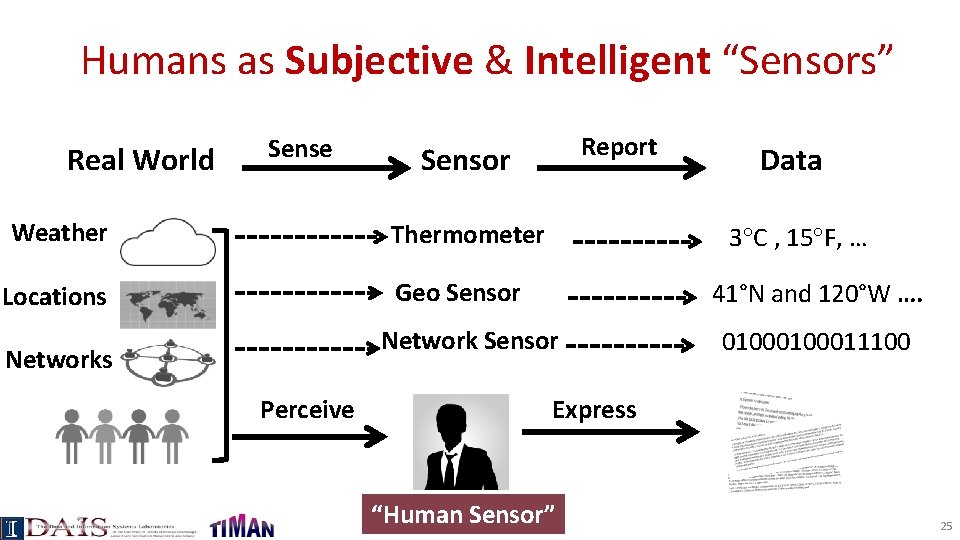
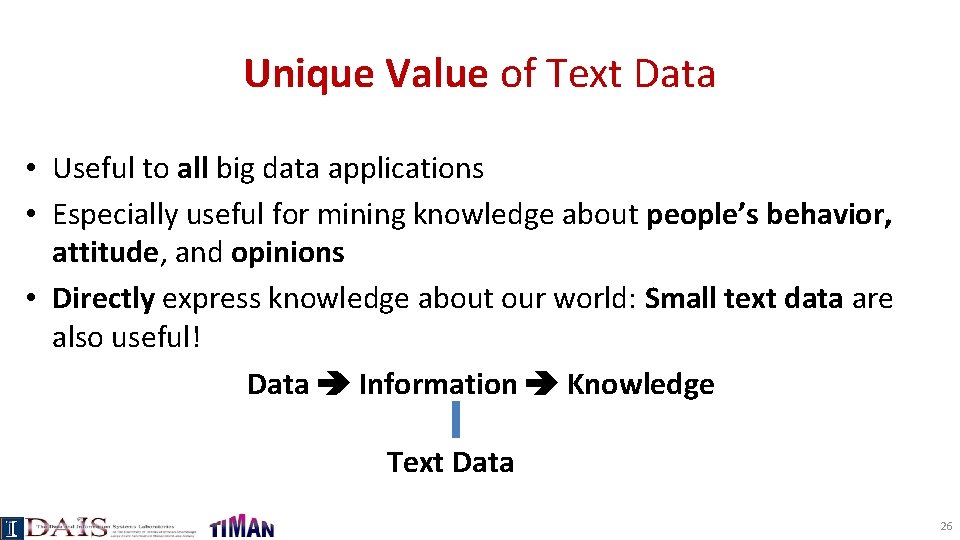
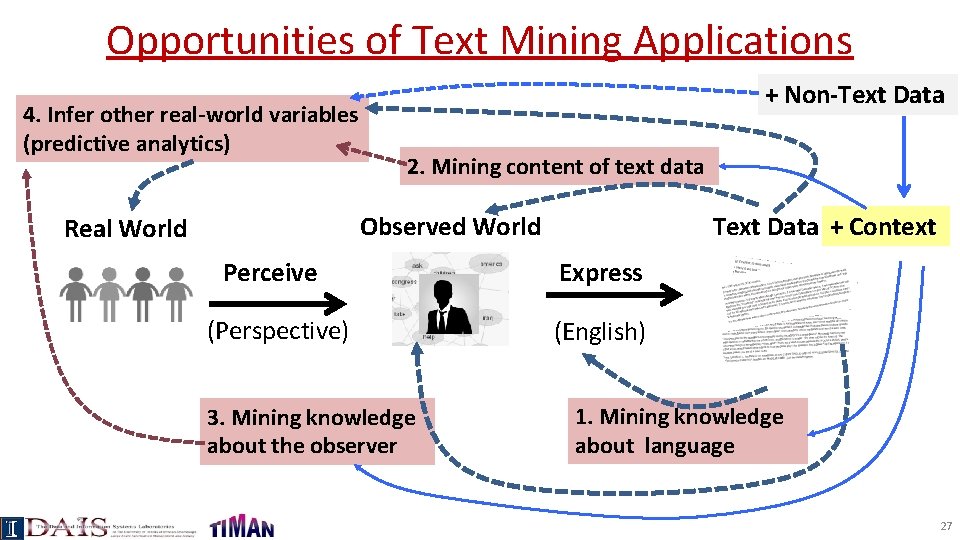
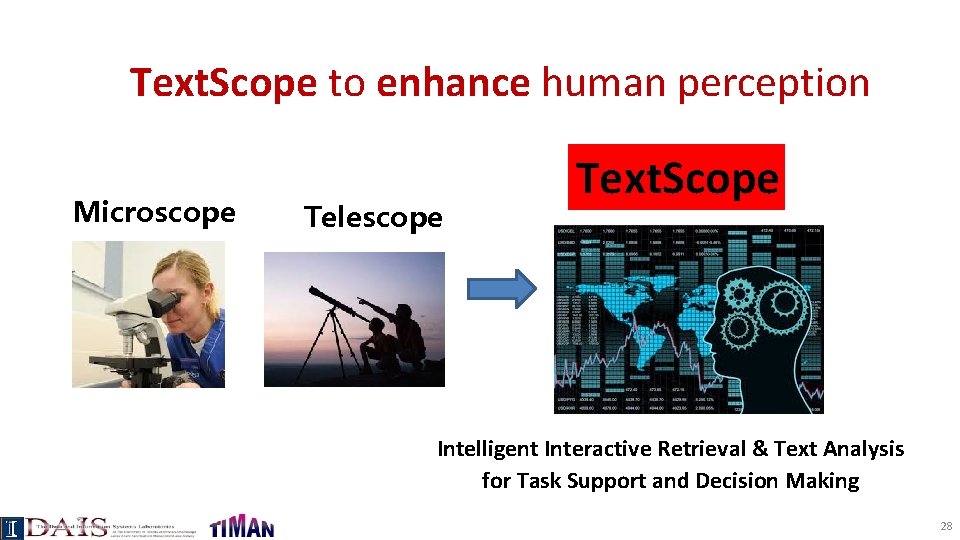
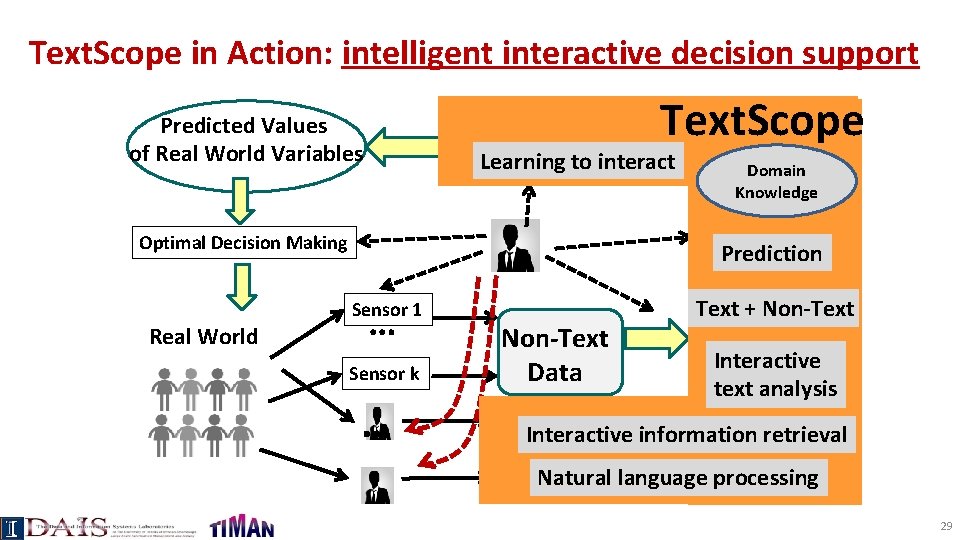
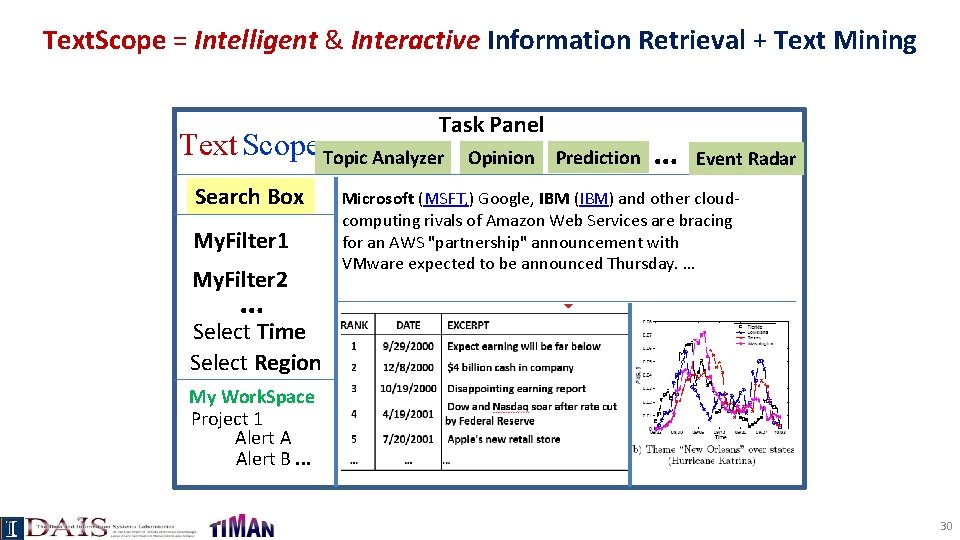
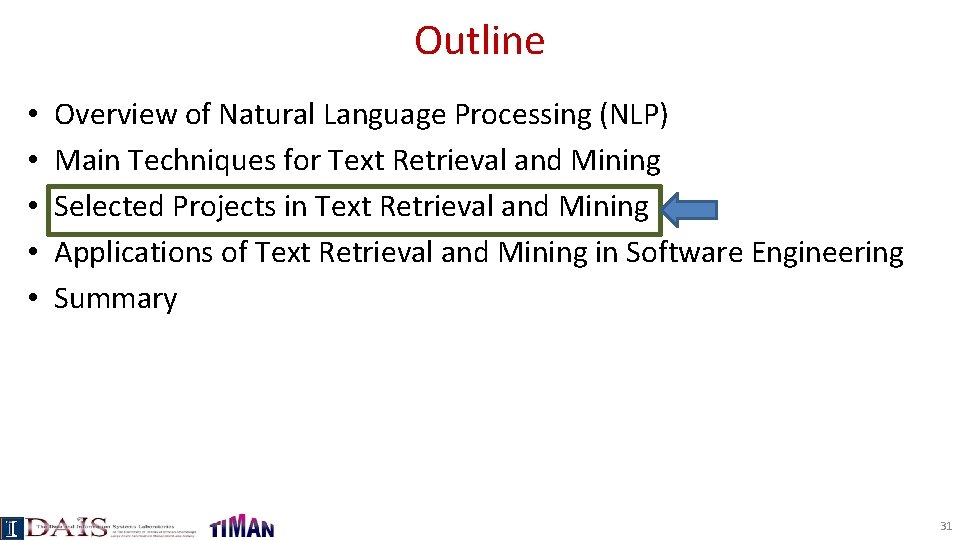
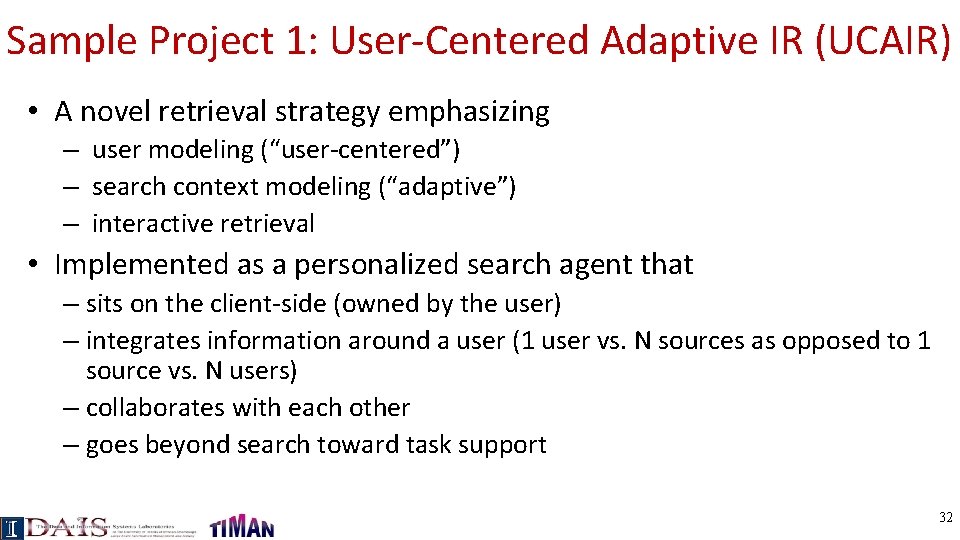
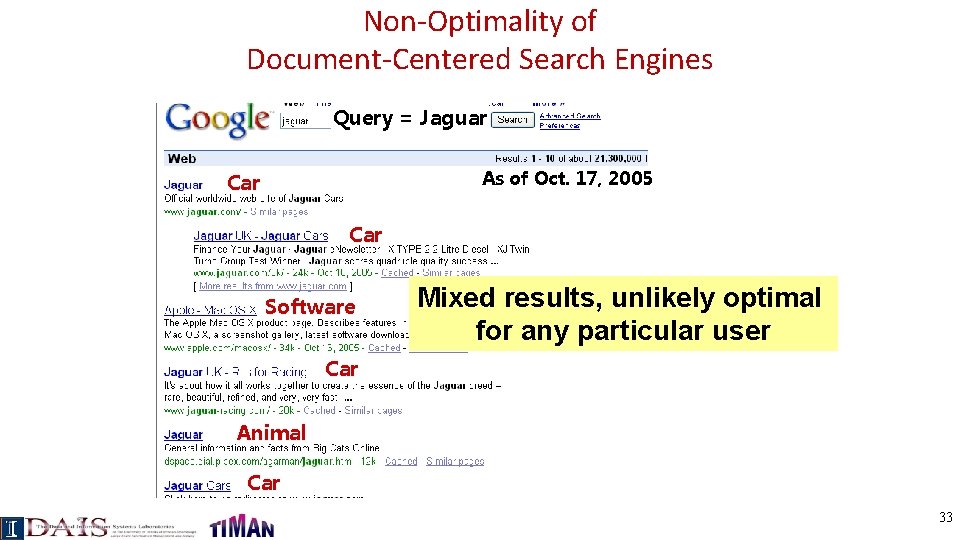
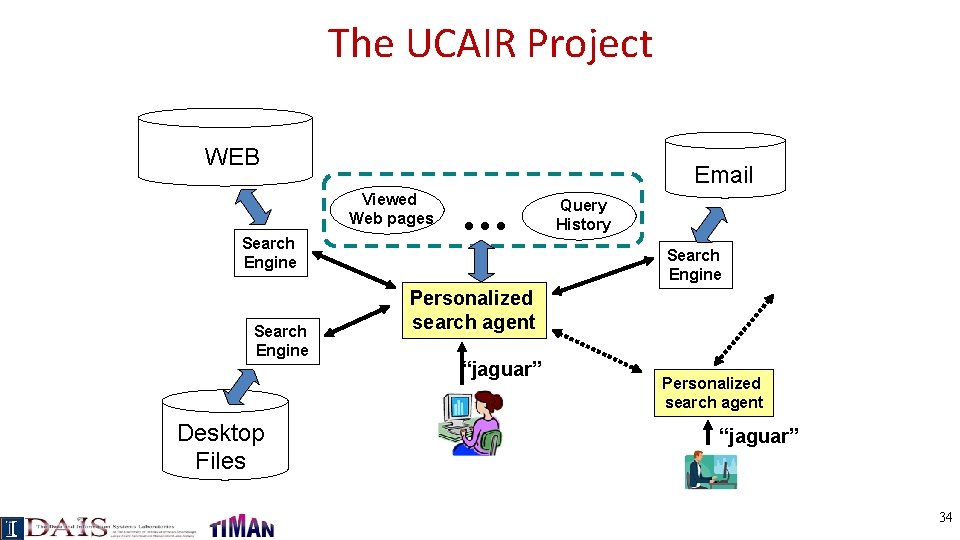
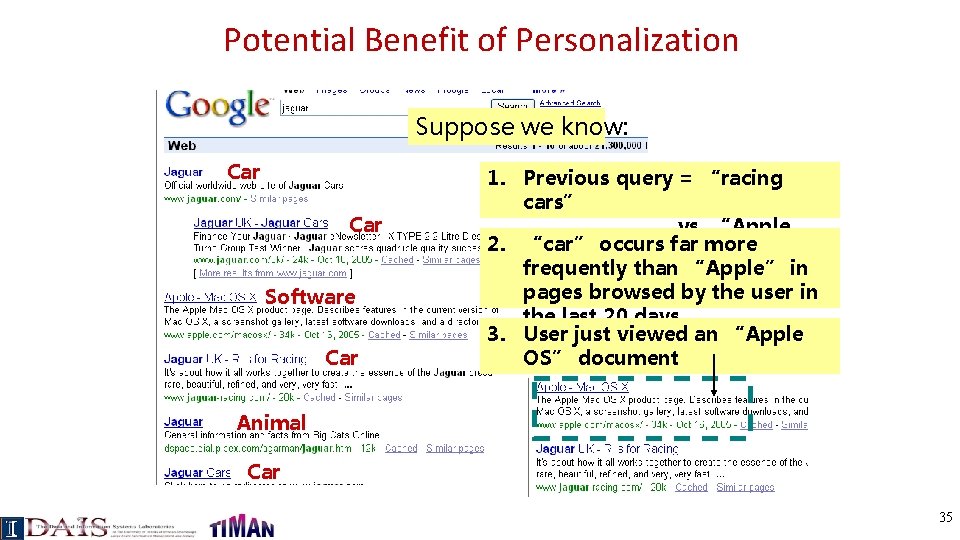
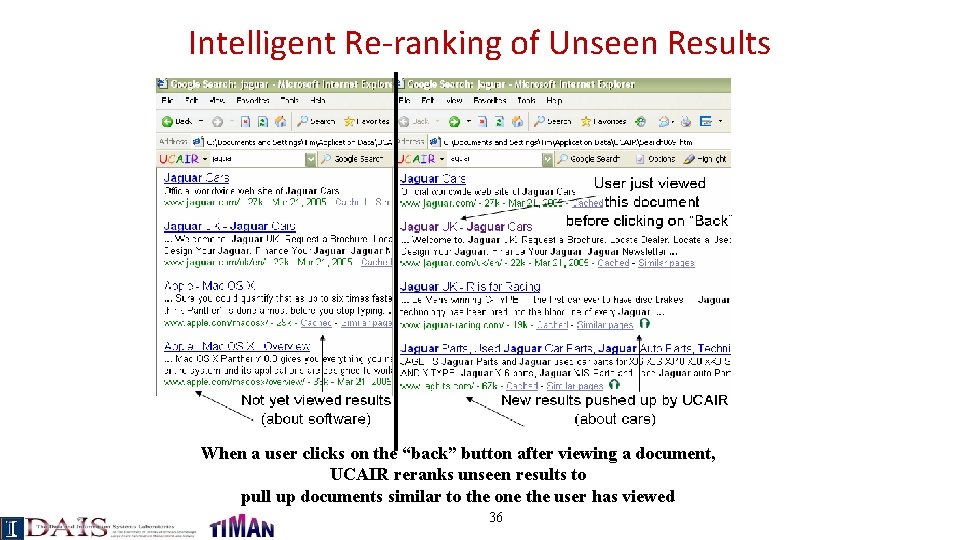
![UCAIR Outperforms Google [Shen et al. 05] Precision at N documents Ranking Method prec@5 UCAIR Outperforms Google [Shen et al. 05] Precision at N documents Ranking Method prec@5](https://slidetodoc.com/presentation_image_h/d5a0aa61765e85e4b4d6978a82a63873/image-37.jpg)
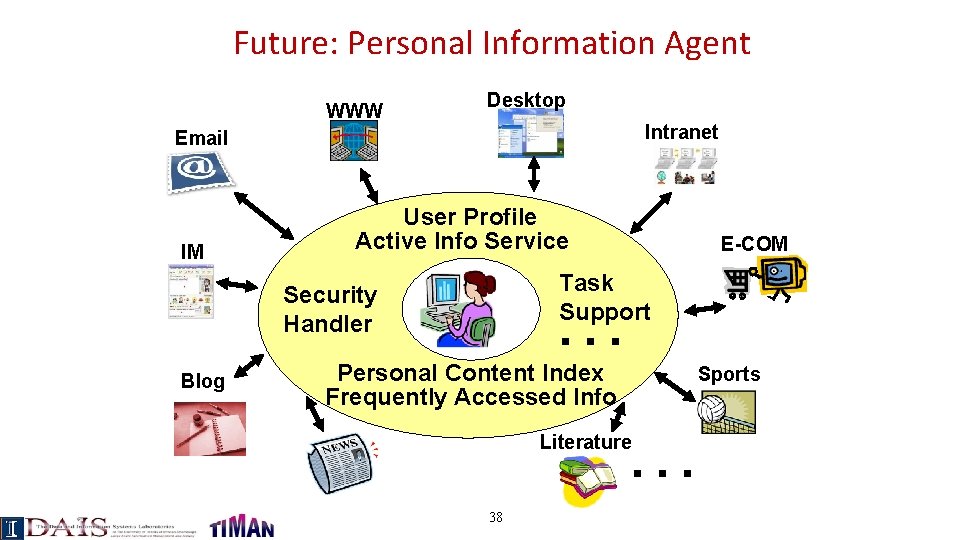
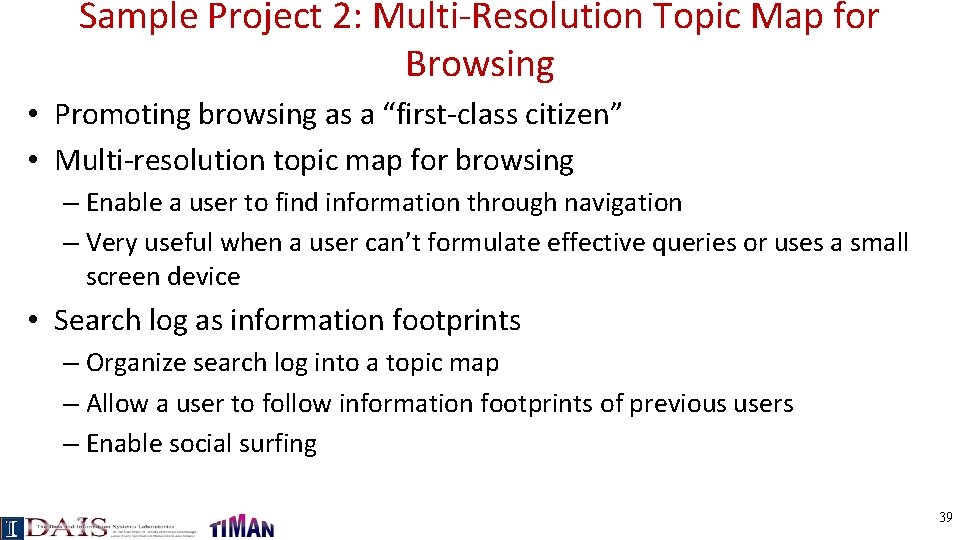
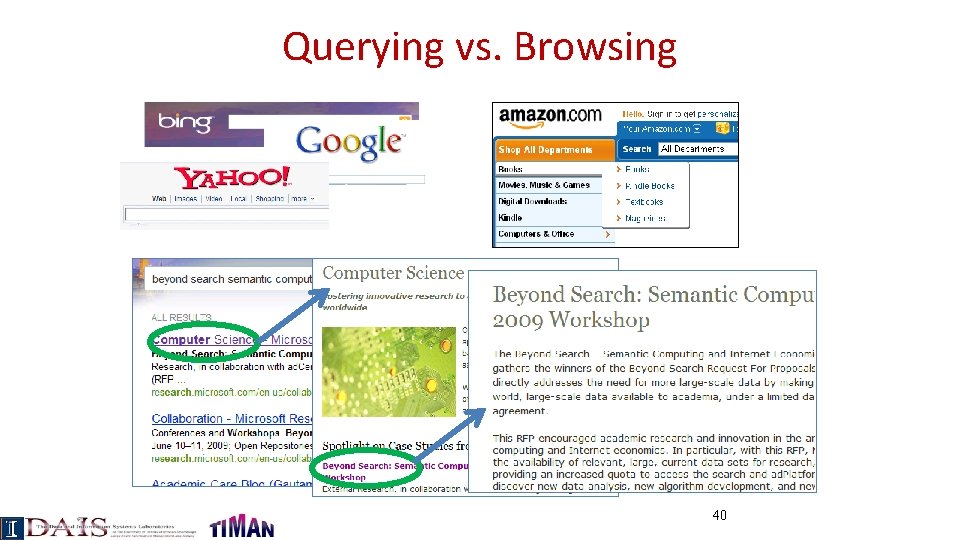
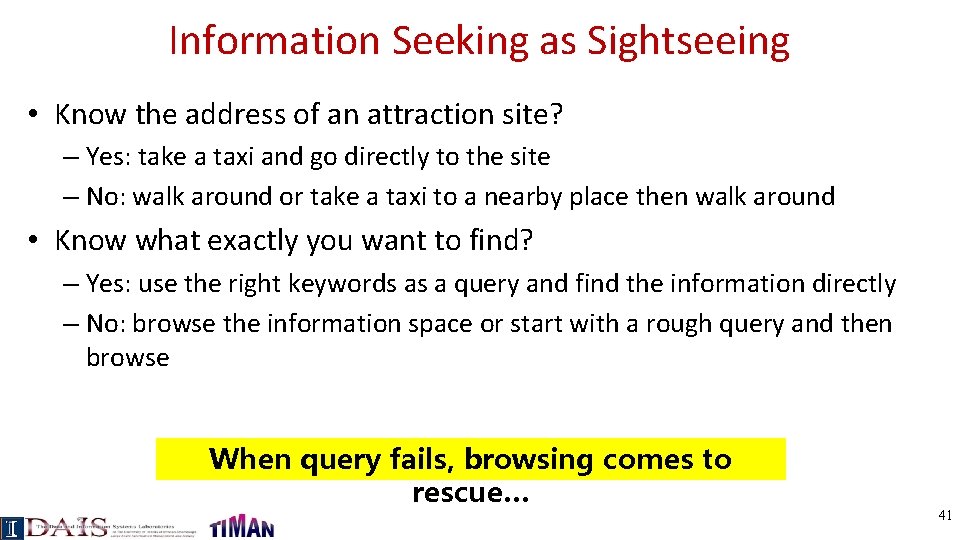
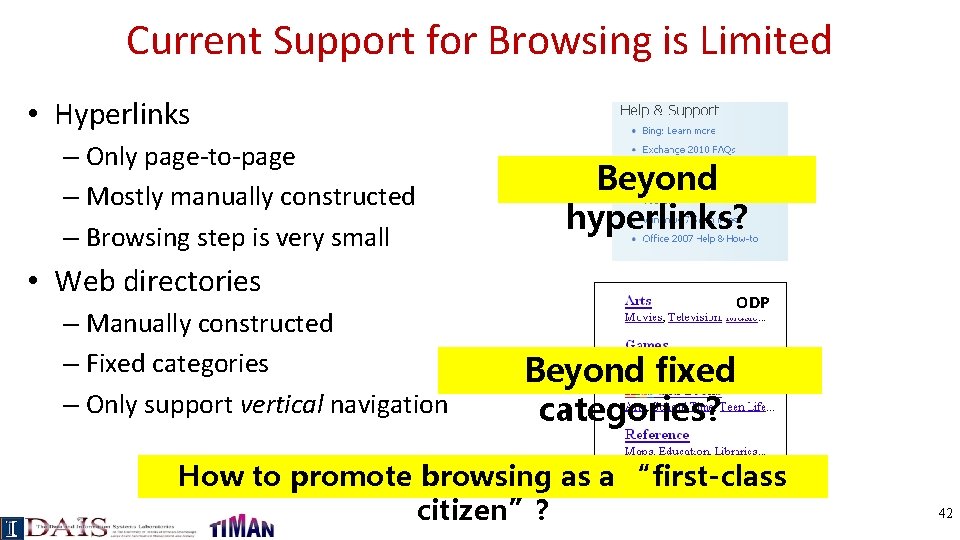
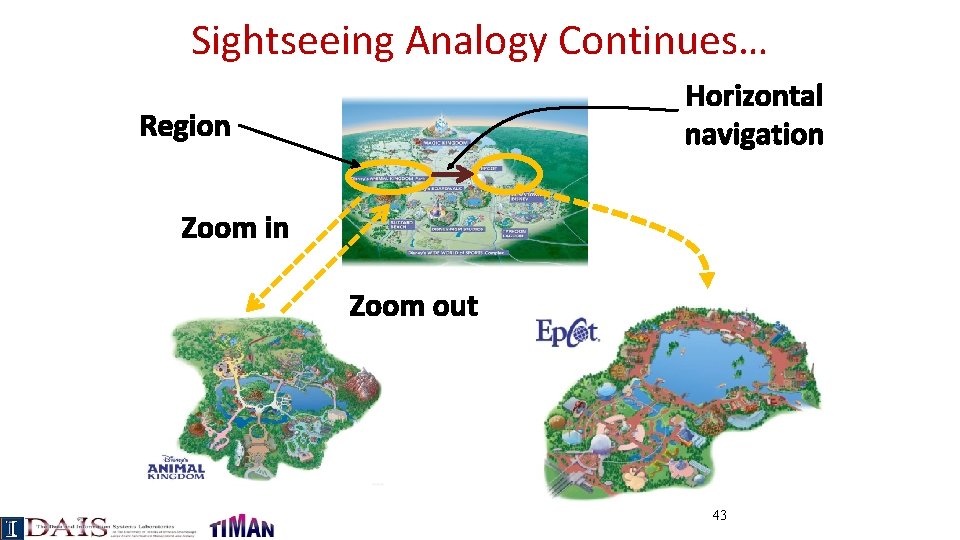
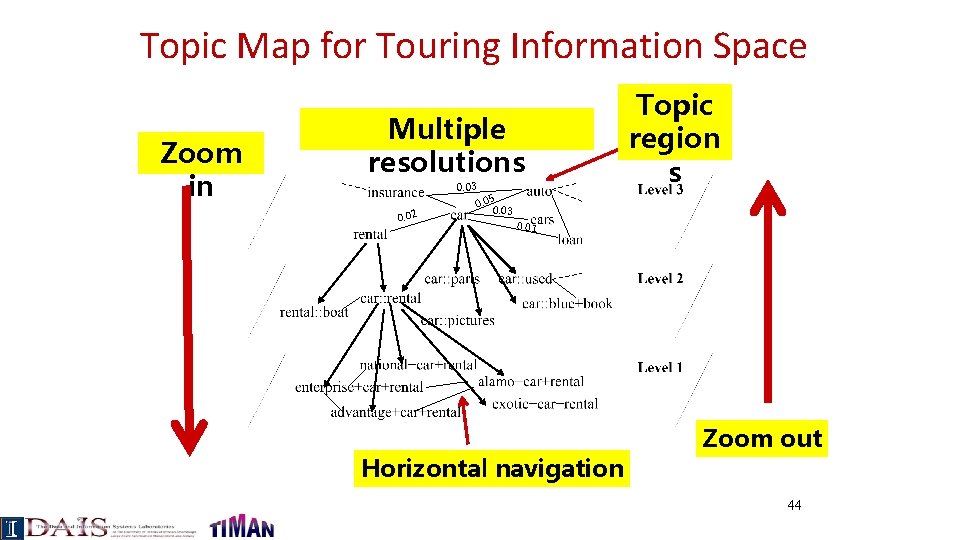
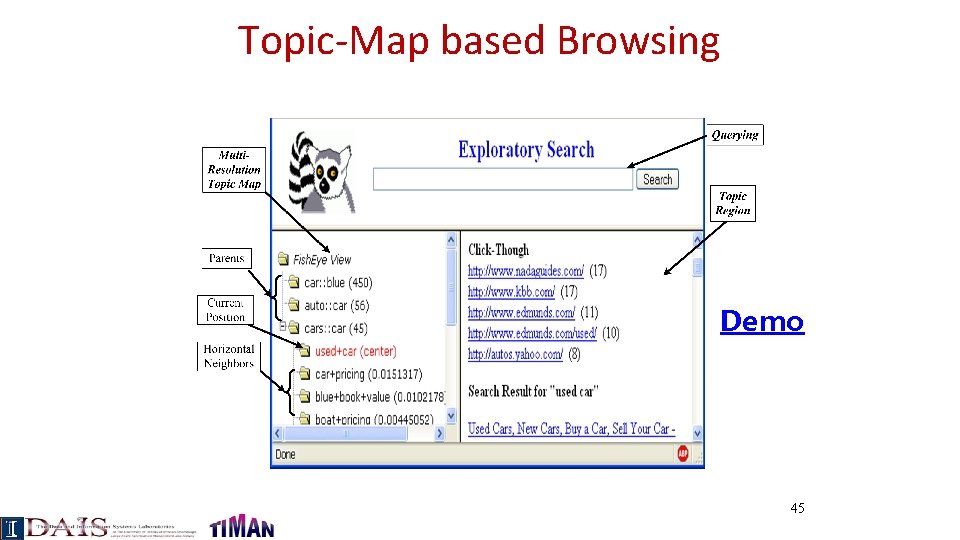
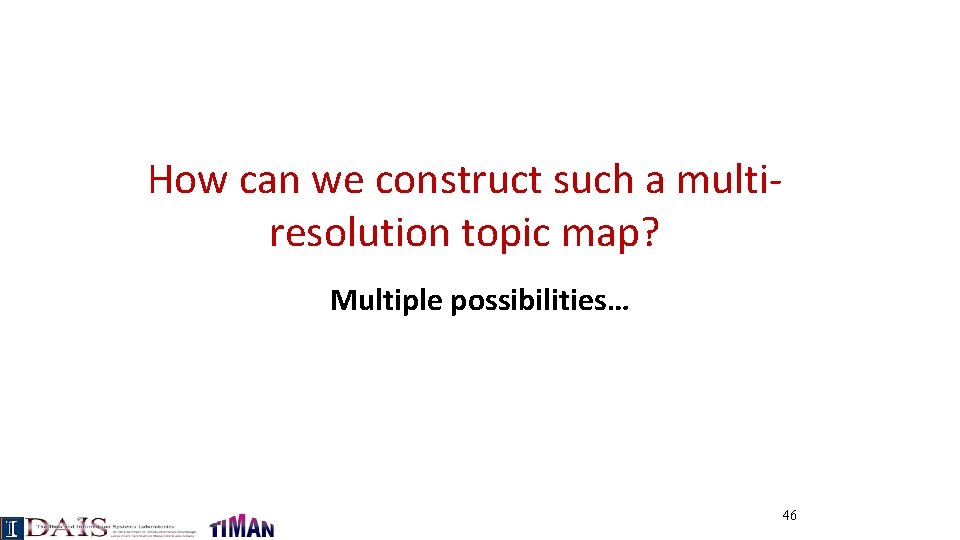
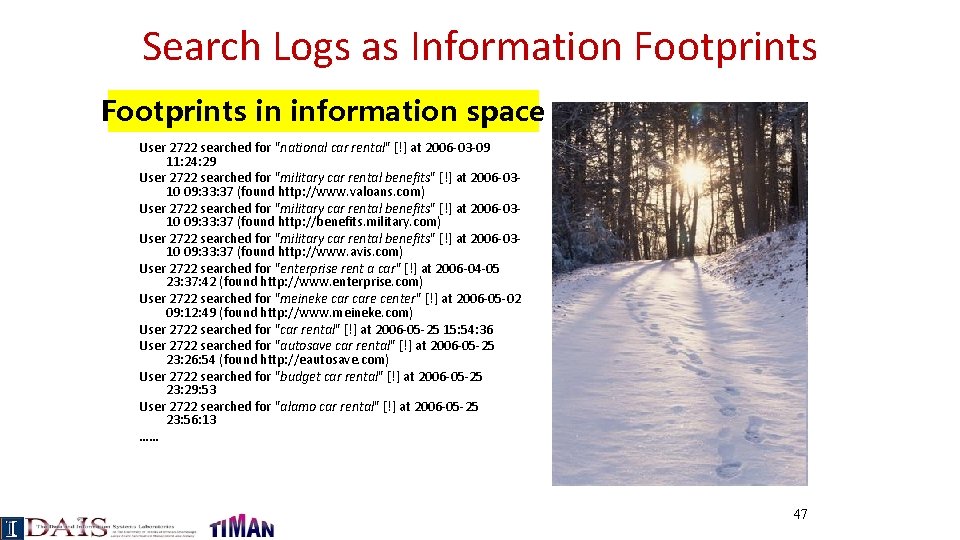
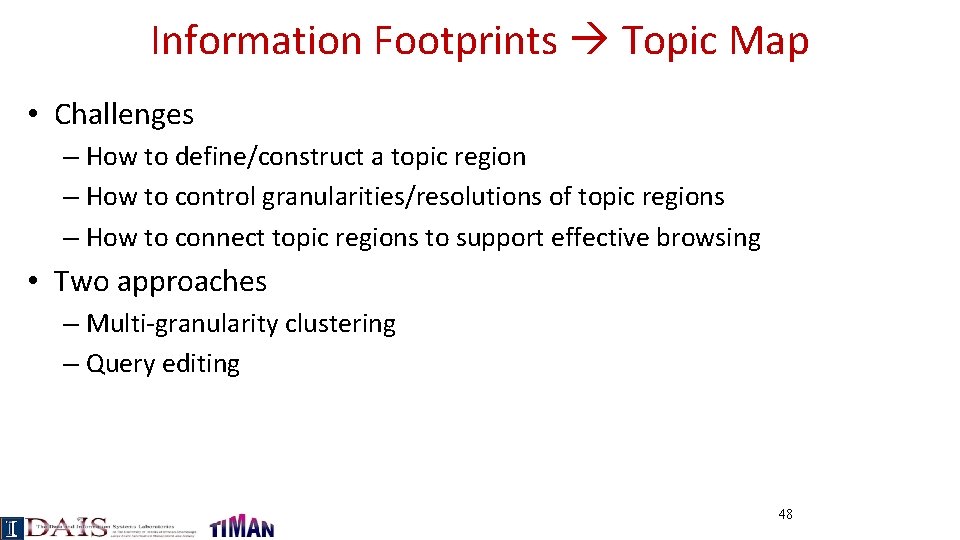
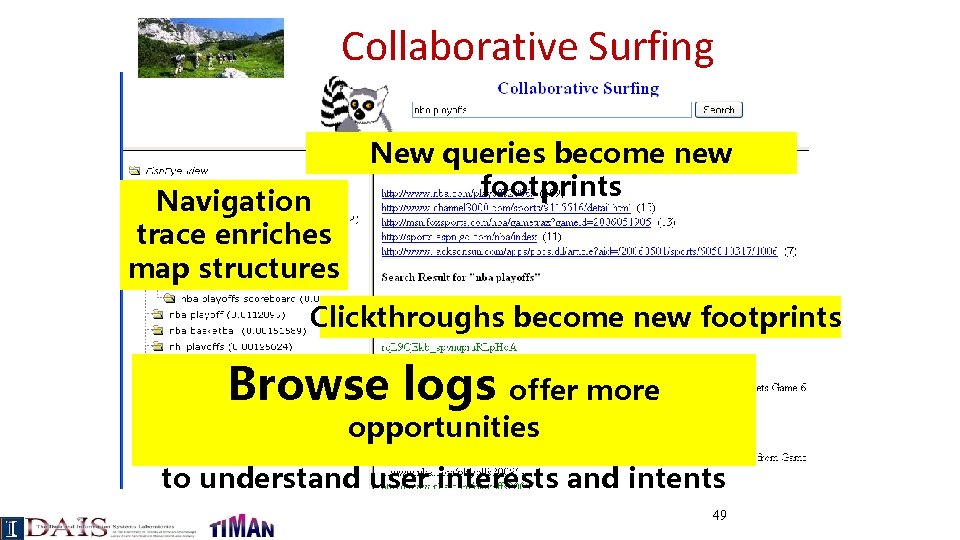
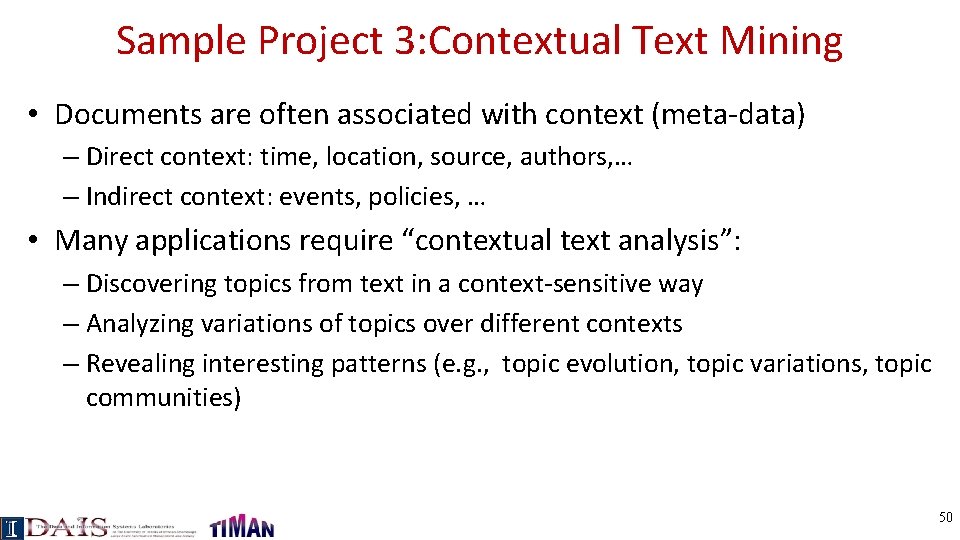
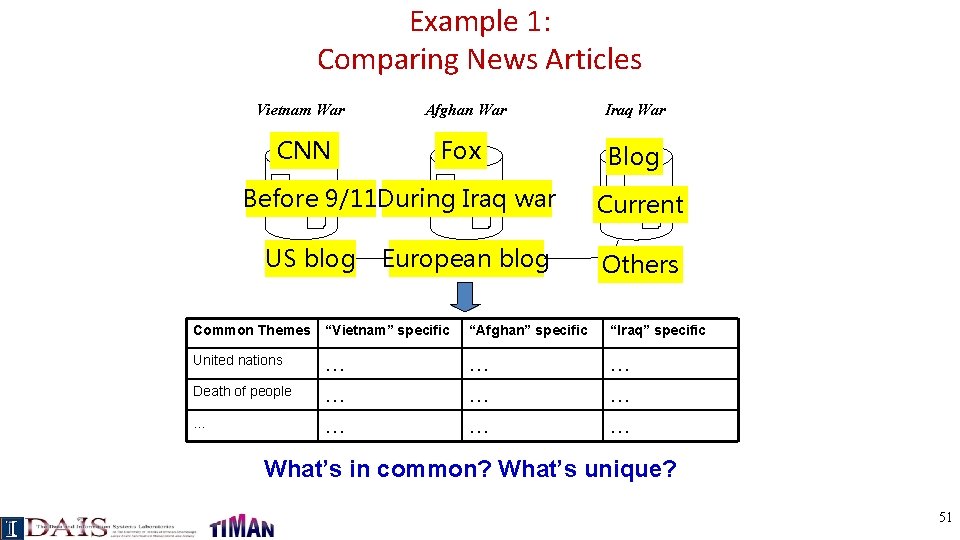
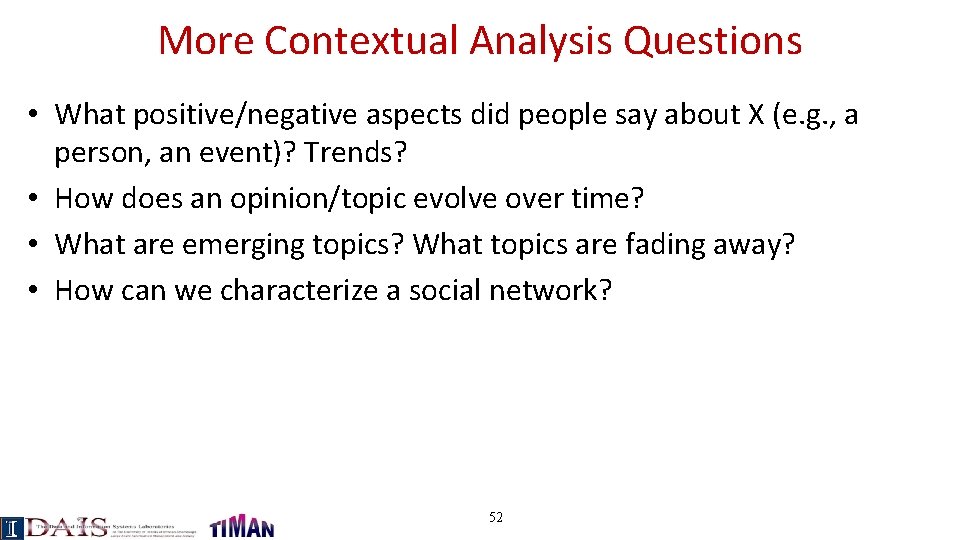
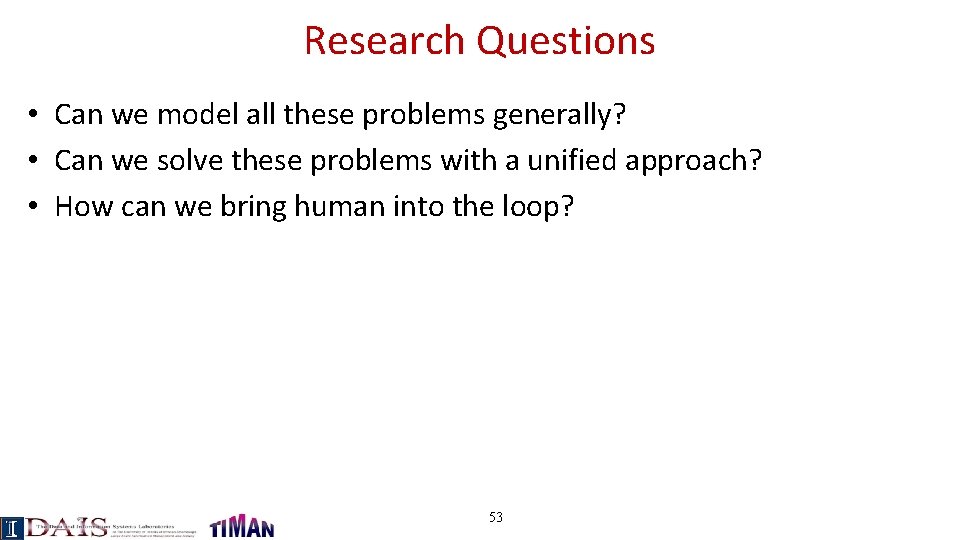
![Contextual Probabilistic Latent Semantics Analysis ([KDD 2006]…) Themes View 1 View 2 View 3 Contextual Probabilistic Latent Semantics Analysis ([KDD 2006]…) Themes View 1 View 2 View 3](https://slidetodoc.com/presentation_image_h/d5a0aa61765e85e4b4d6978a82a63873/image-54.jpg)
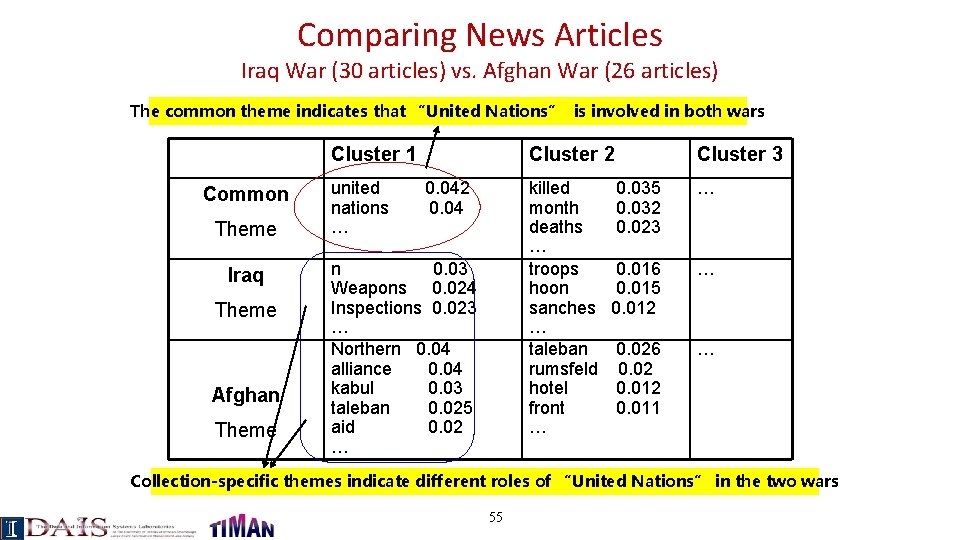
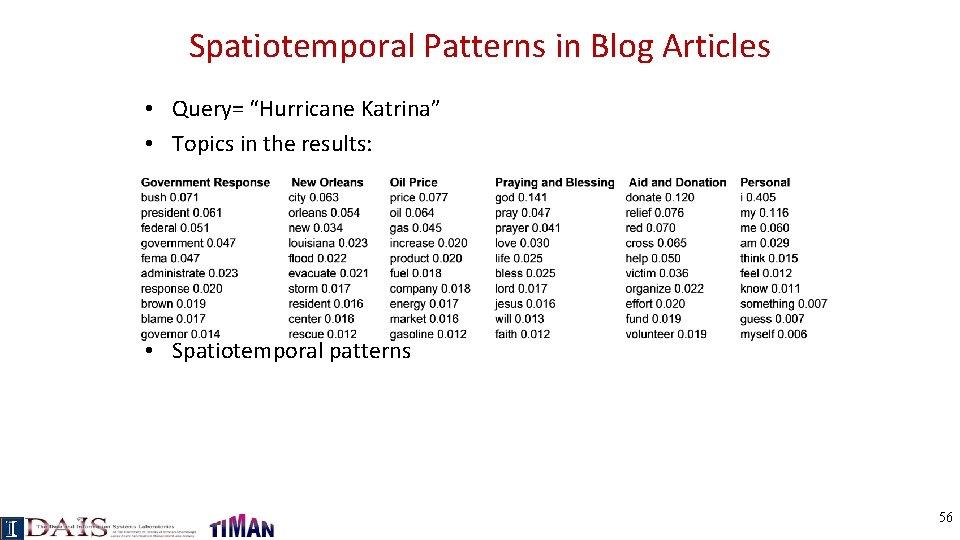
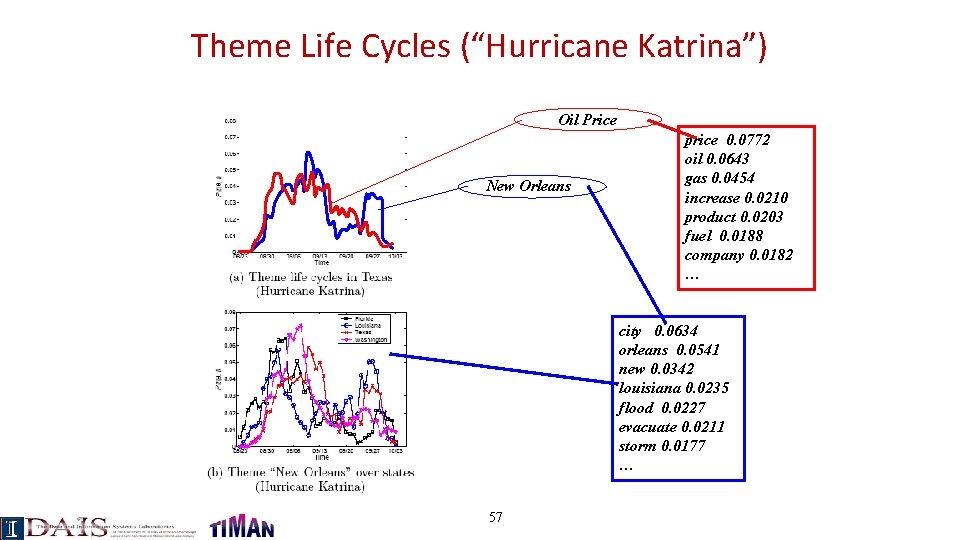
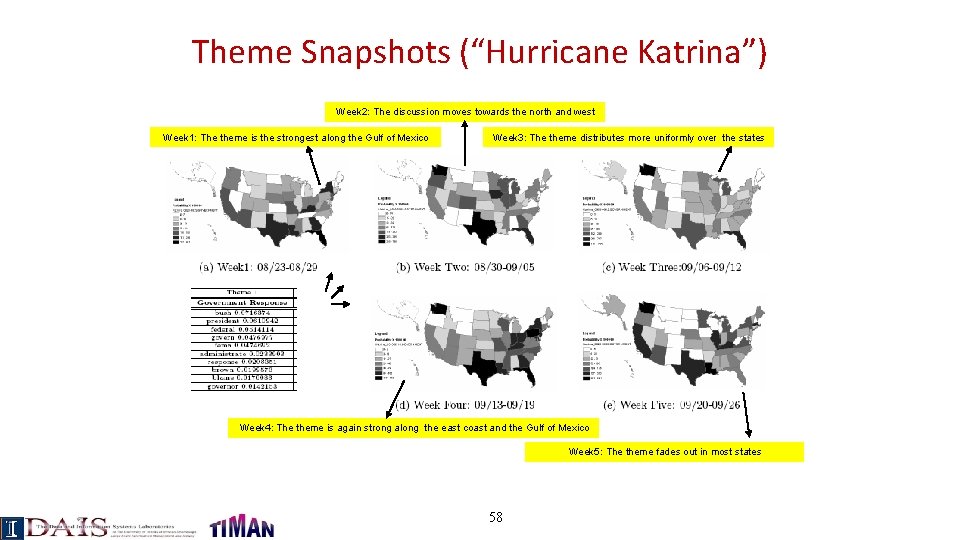
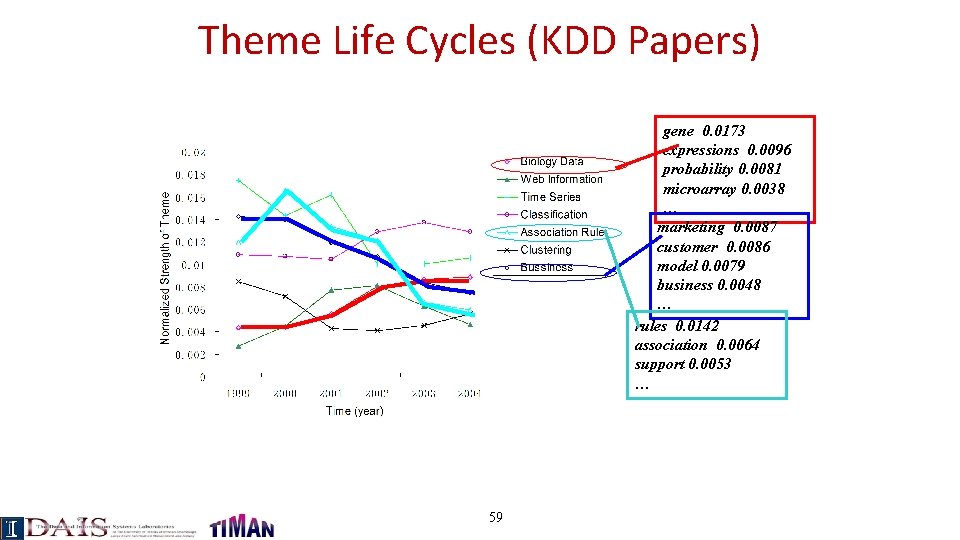
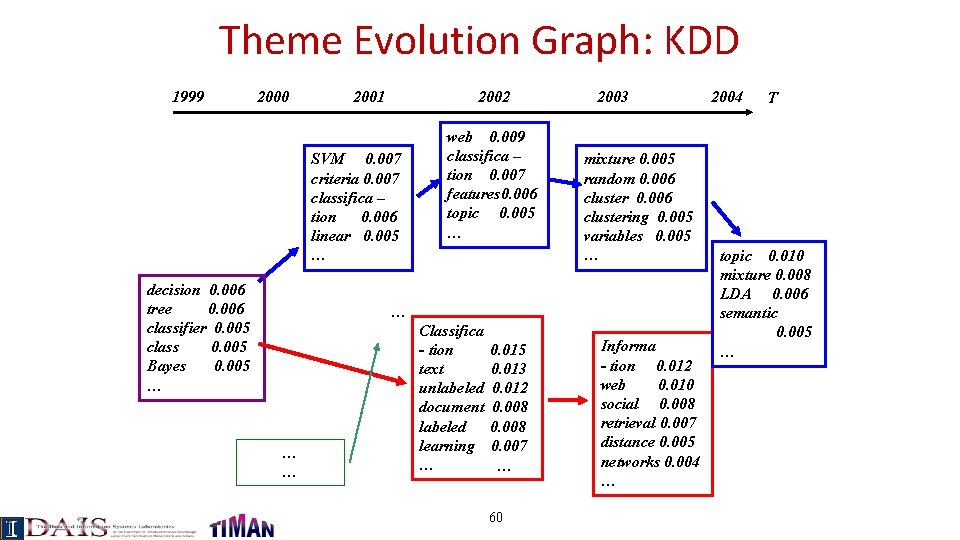
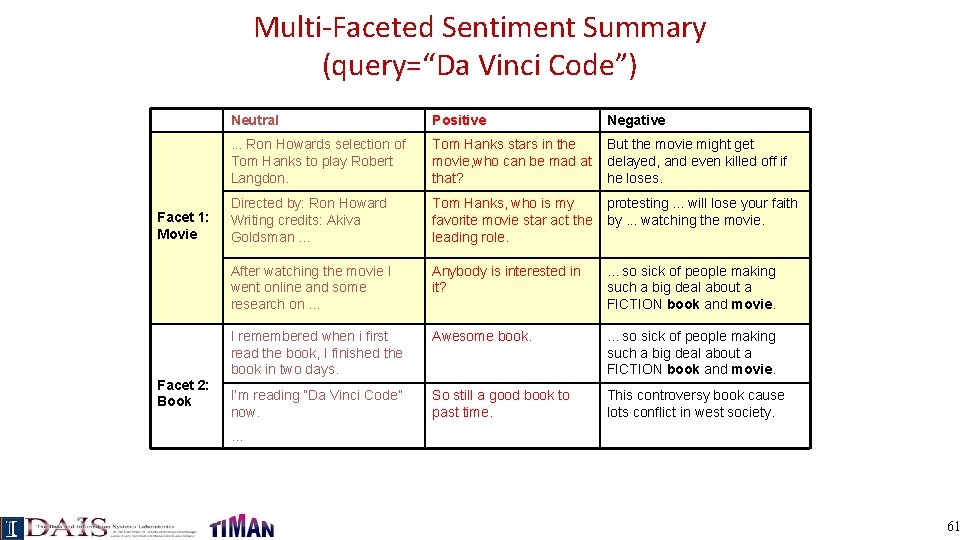
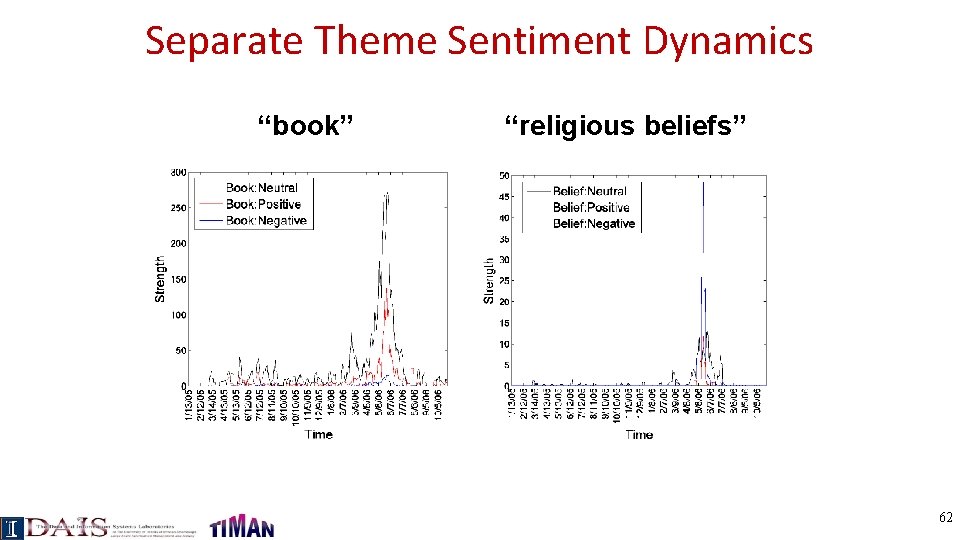
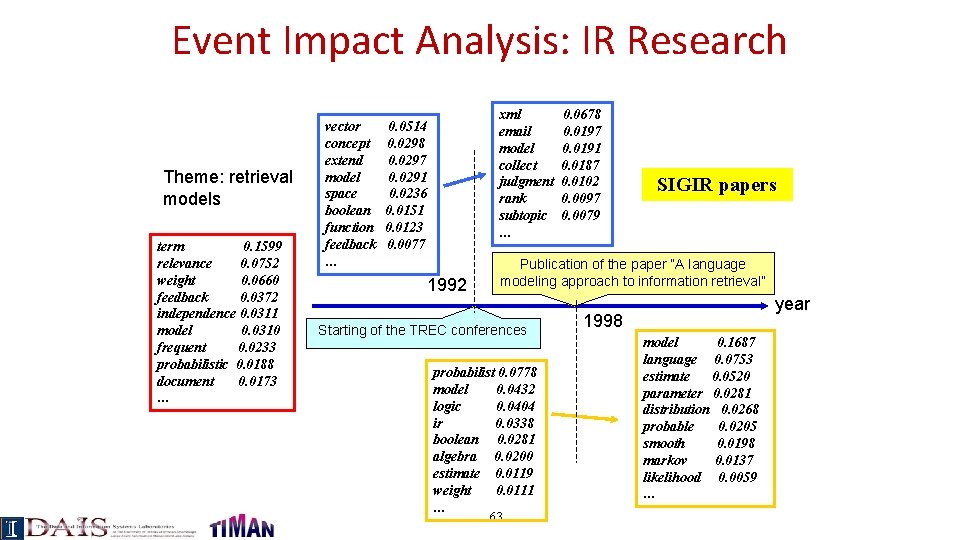
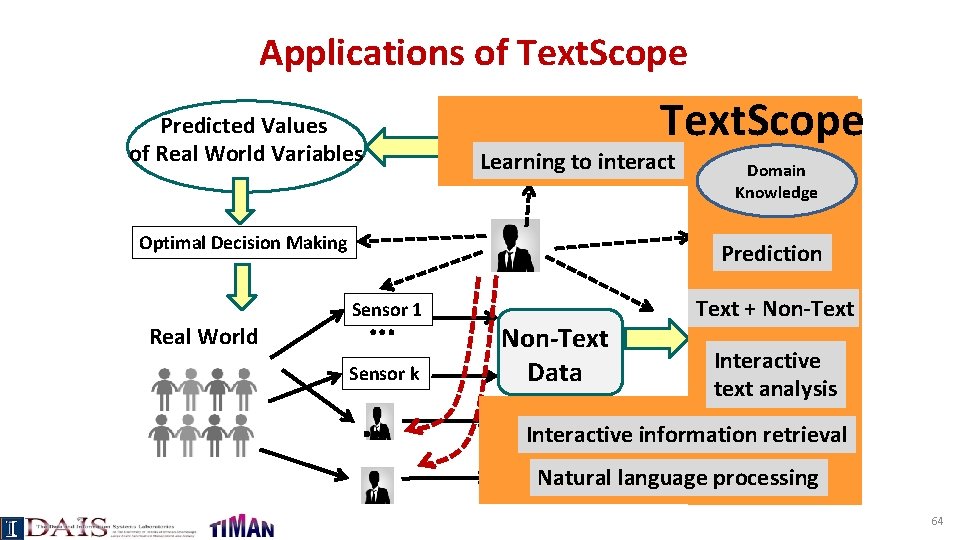
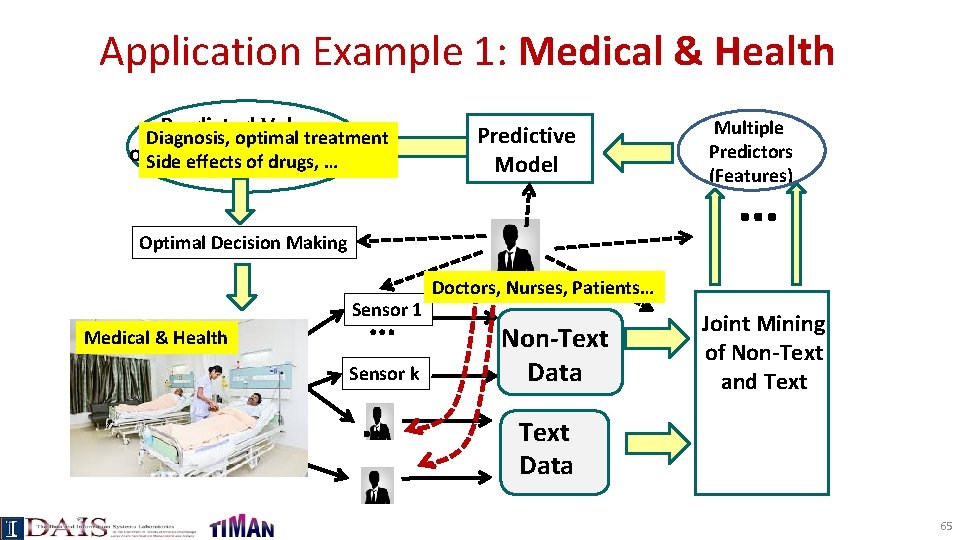
![Discovery of Adverse Drug Reactions from Forums [Wang et al. 14] Green: Disease symptoms Discovery of Adverse Drug Reactions from Forums [Wang et al. 14] Green: Disease symptoms](https://slidetodoc.com/presentation_image_h/d5a0aa61765e85e4b4d6978a82a63873/image-66.jpg)
![Sample ADRs Discovered [Wang et al. 14] Drug(Freq) Drug Use Symptoms in Descending Order Sample ADRs Discovered [Wang et al. 14] Drug(Freq) Drug Use Symptoms in Descending Order](https://slidetodoc.com/presentation_image_h/d5a0aa61765e85e4b4d6978a82a63873/image-67.jpg)
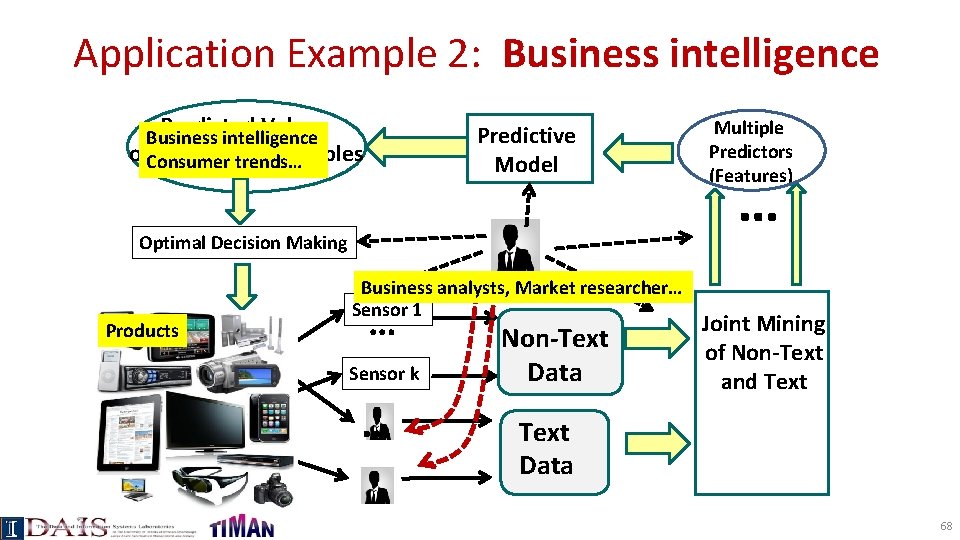
![Latent Aspect Rating Analysis (LARA) [Wang et al. 10] Text. Scope How to infer Latent Aspect Rating Analysis (LARA) [Wang et al. 10] Text. Scope How to infer](https://slidetodoc.com/presentation_image_h/d5a0aa61765e85e4b4d6978a82a63873/image-69.jpg)
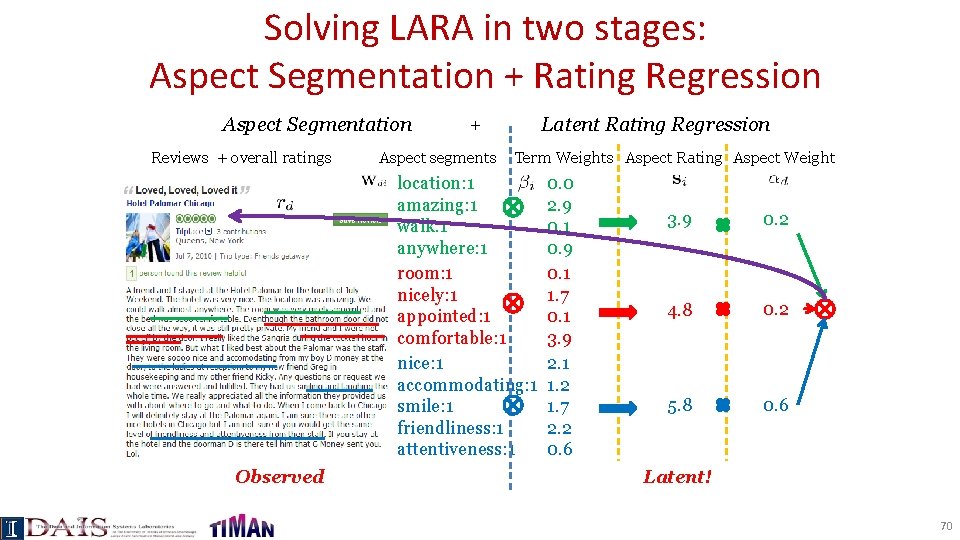
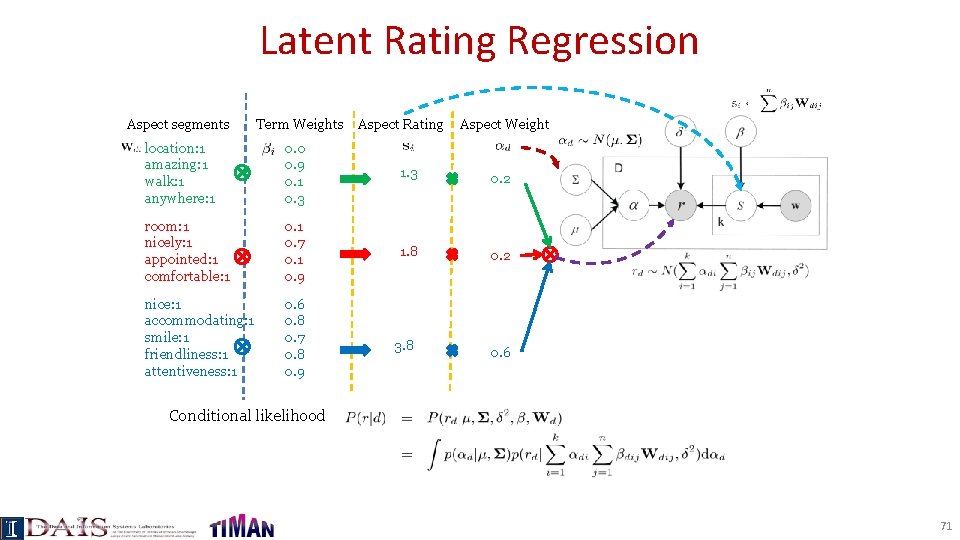
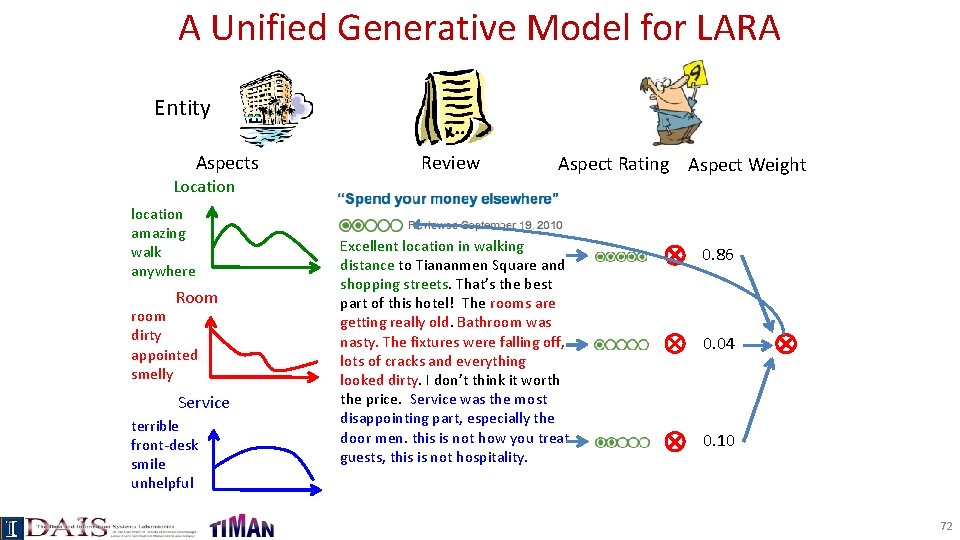
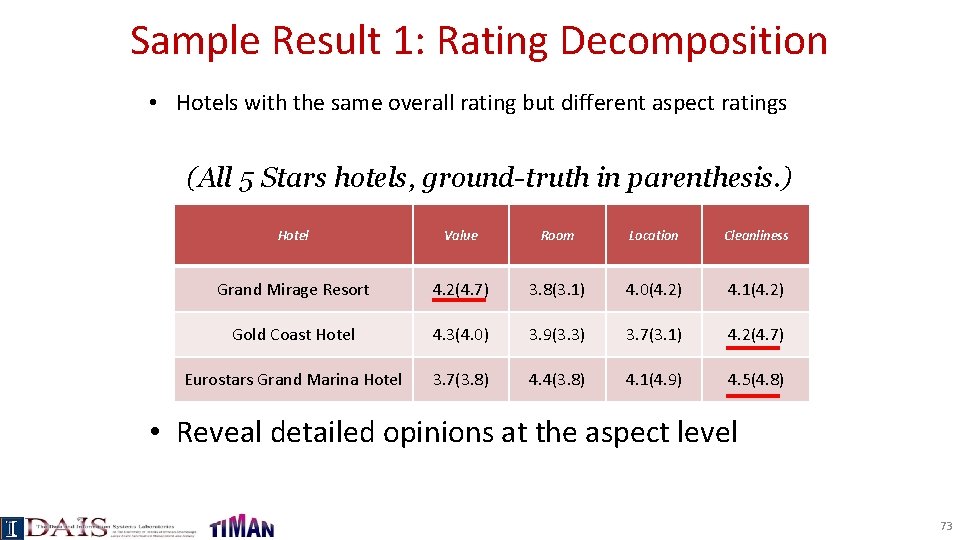
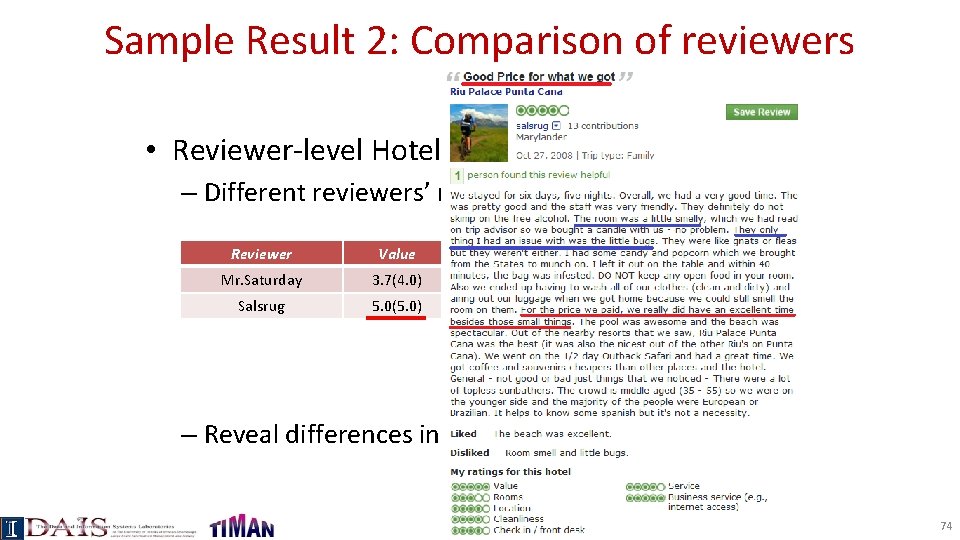
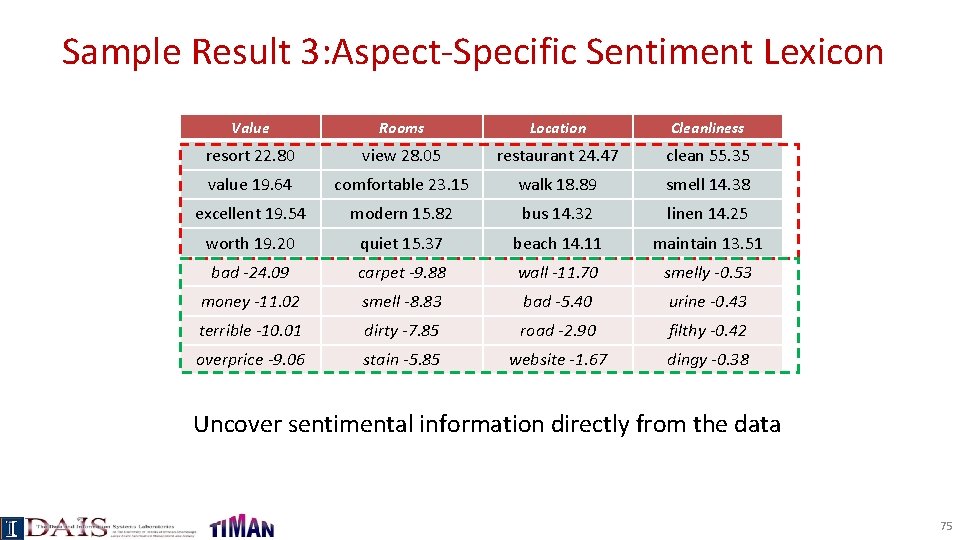
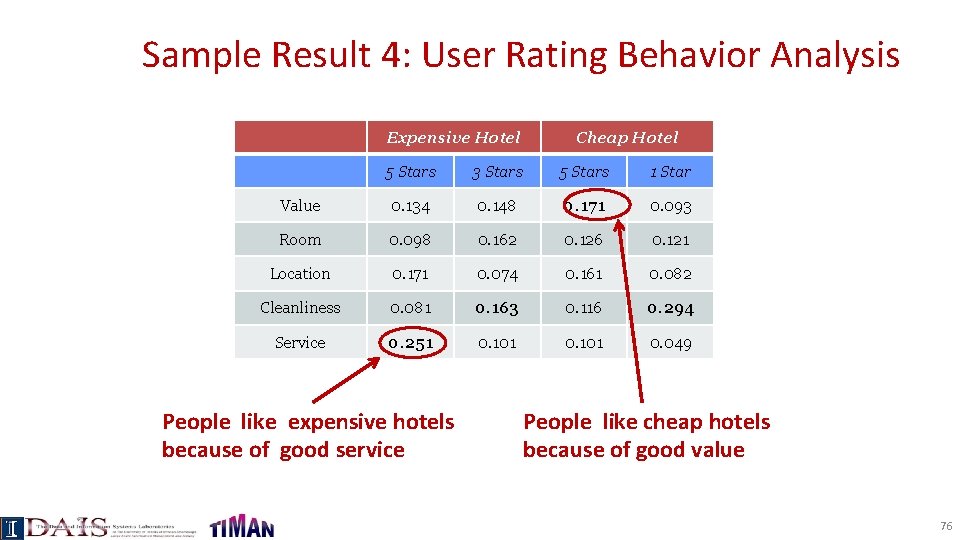
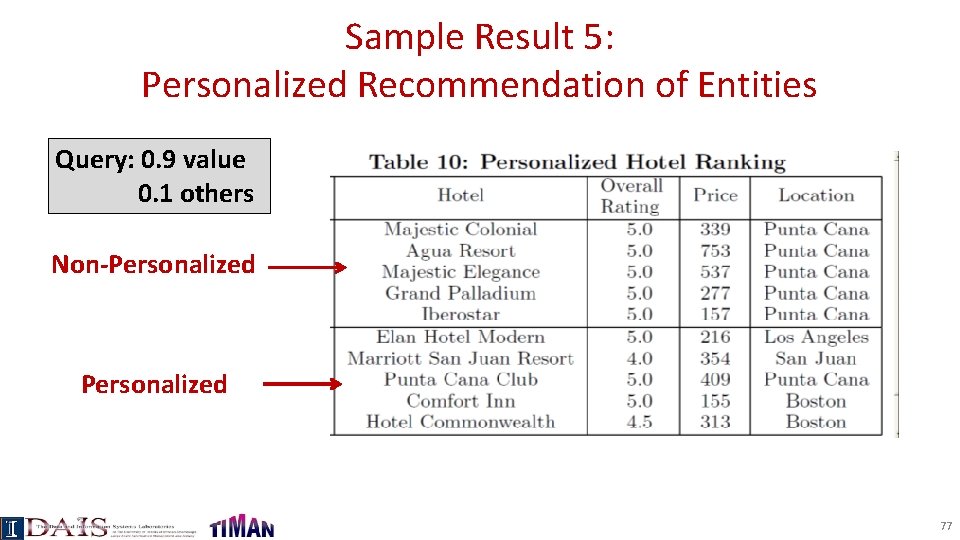
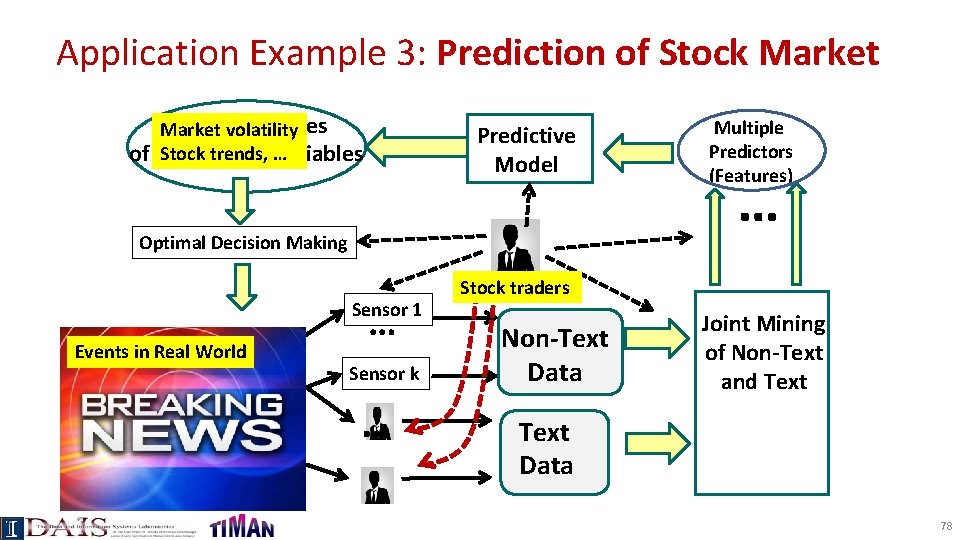
![Text Mining for Understanding Time Series [Kim et al. CIKM’ 13] What might Text Mining for Understanding Time Series [Kim et al. CIKM’ 13] What might](https://slidetodoc.com/presentation_image_h/d5a0aa61765e85e4b4d6978a82a63873/image-79.jpg)
![A General Framework for Causal Topic Modeling [Kim et al. CIKM’ 13] Text Stream A General Framework for Causal Topic Modeling [Kim et al. CIKM’ 13] Text Stream](https://slidetodoc.com/presentation_image_h/d5a0aa61765e85e4b4d6978a82a63873/image-80.jpg)
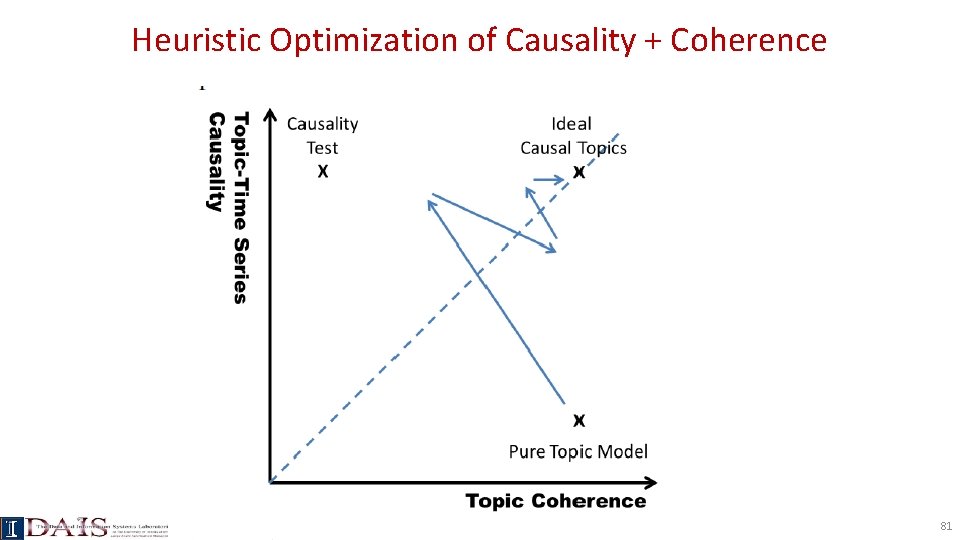
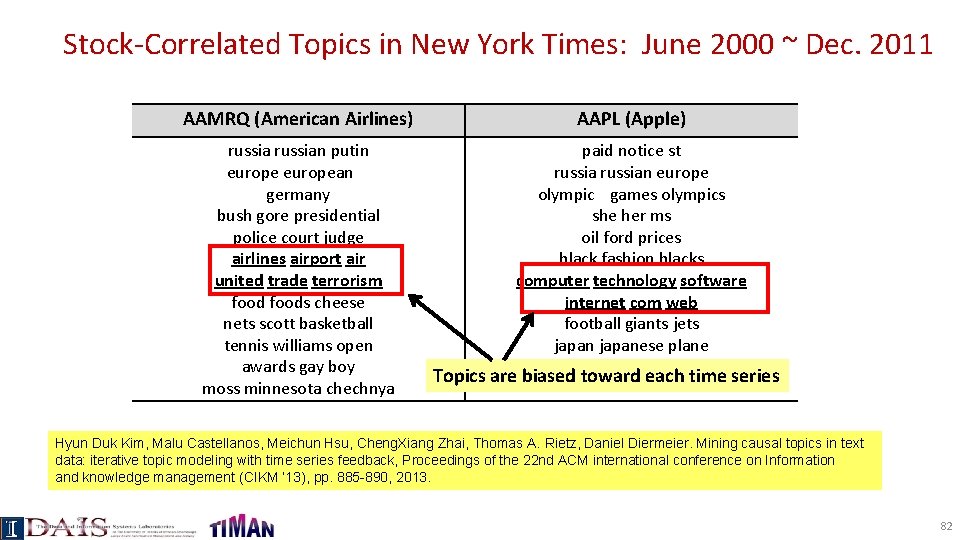
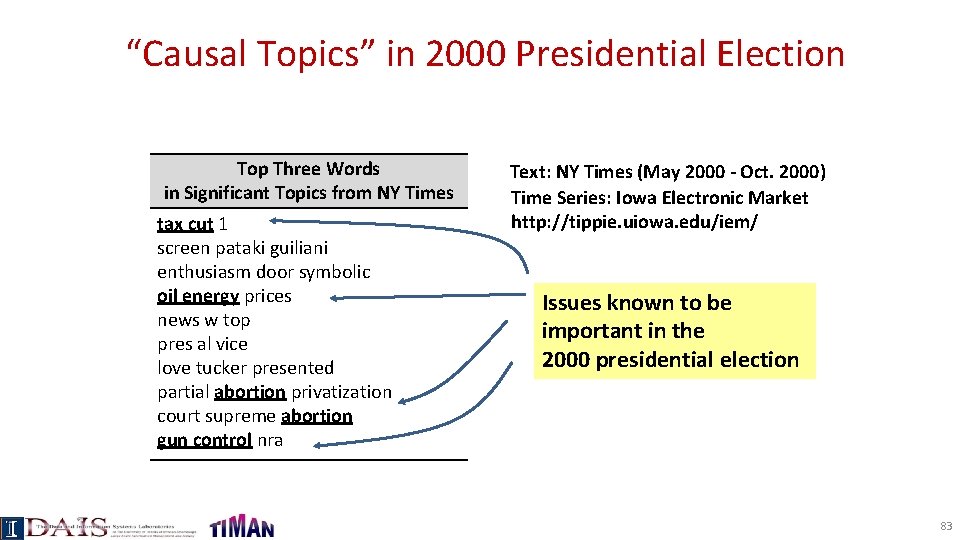
![Retrieval with Time Series Query [Kim et al. ICTIR’ 13] News 70 60 50 Retrieval with Time Series Query [Kim et al. ICTIR’ 13] News 70 60 50](https://slidetodoc.com/presentation_image_h/d5a0aa61765e85e4b4d6978a82a63873/image-84.jpg)
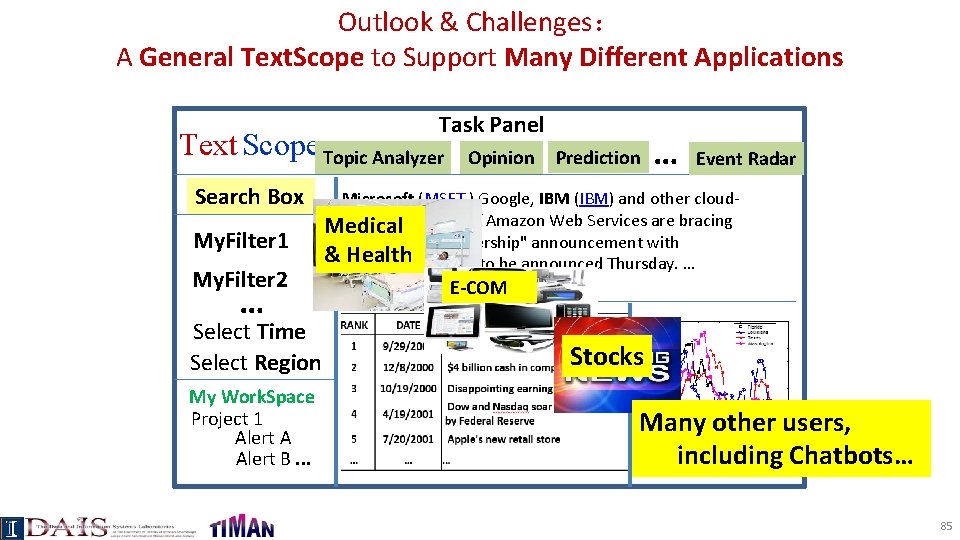
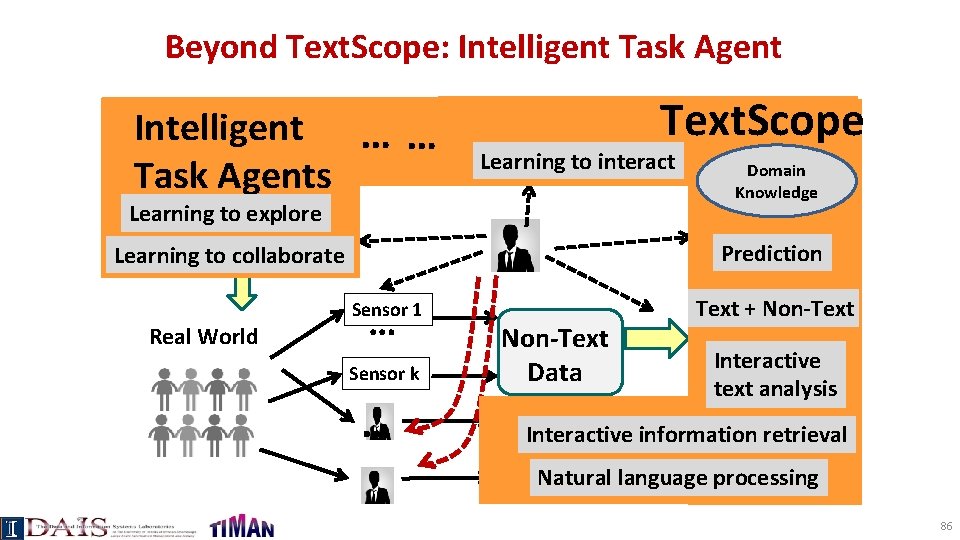
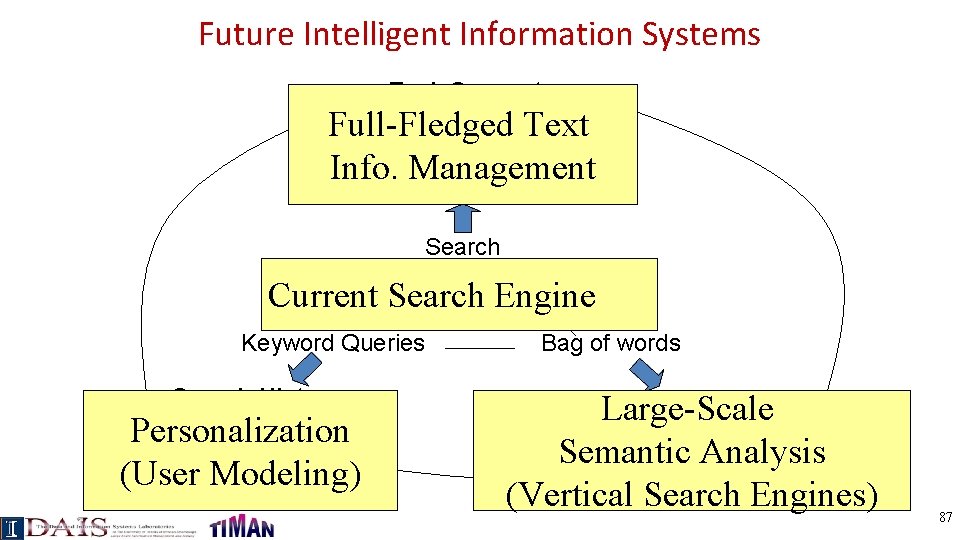
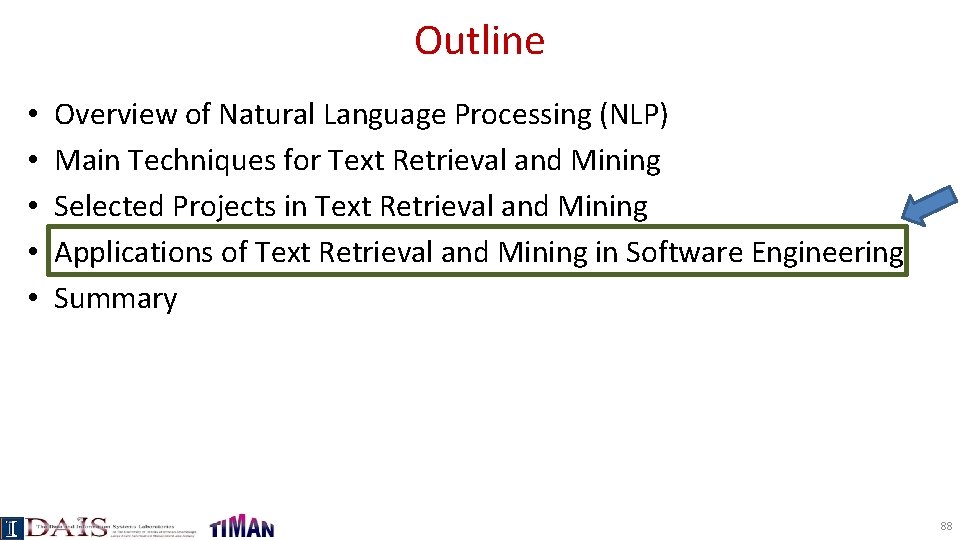
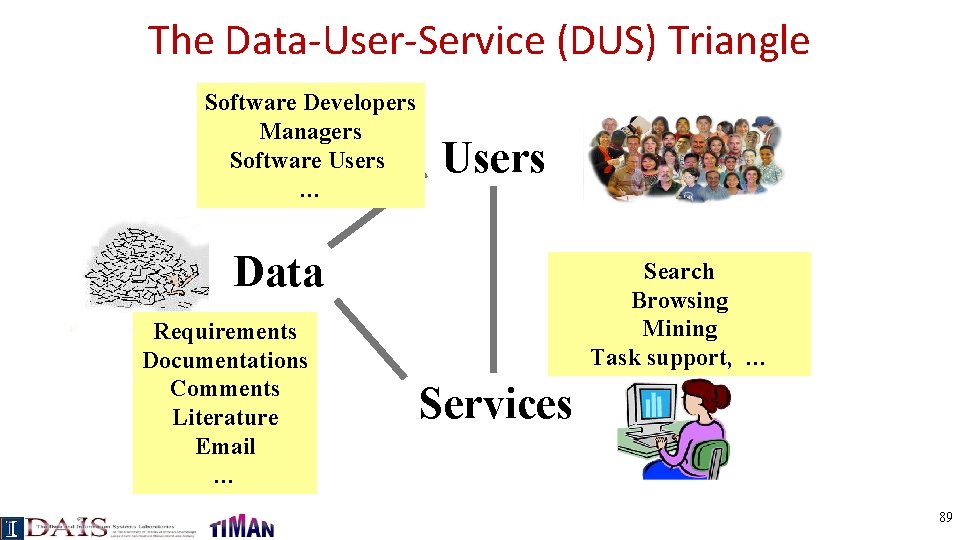
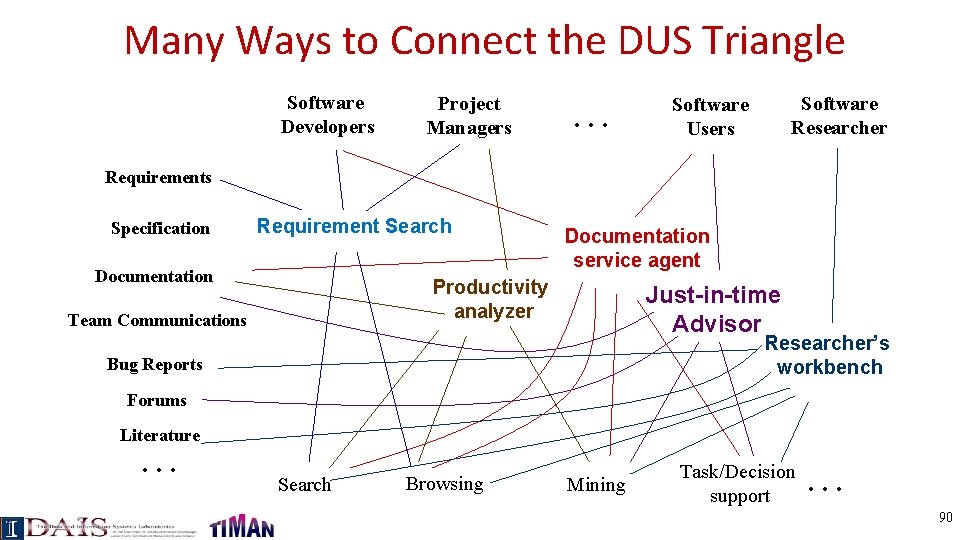
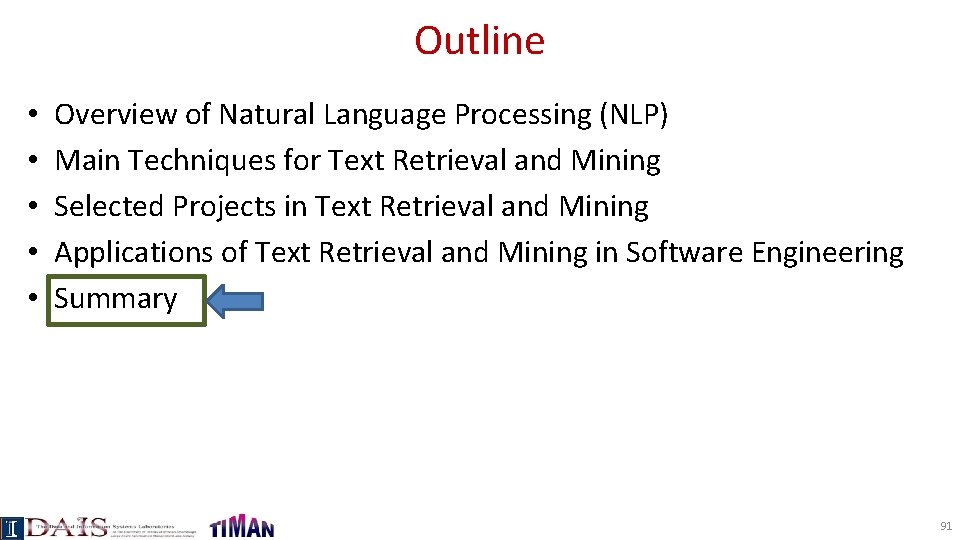
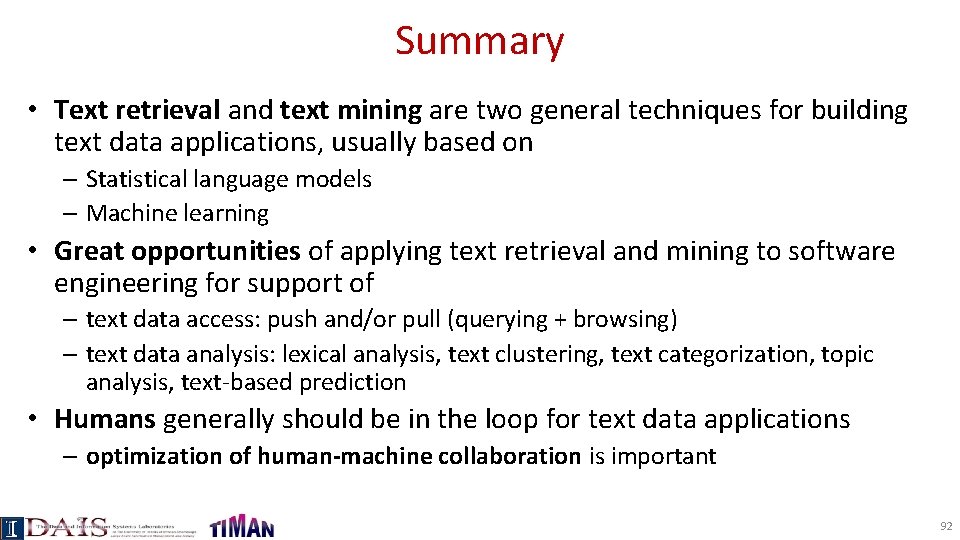
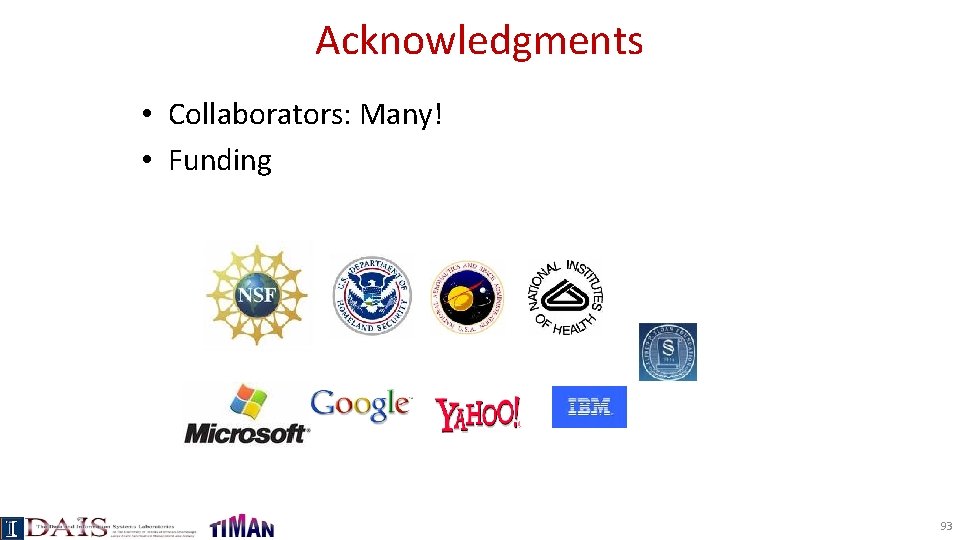
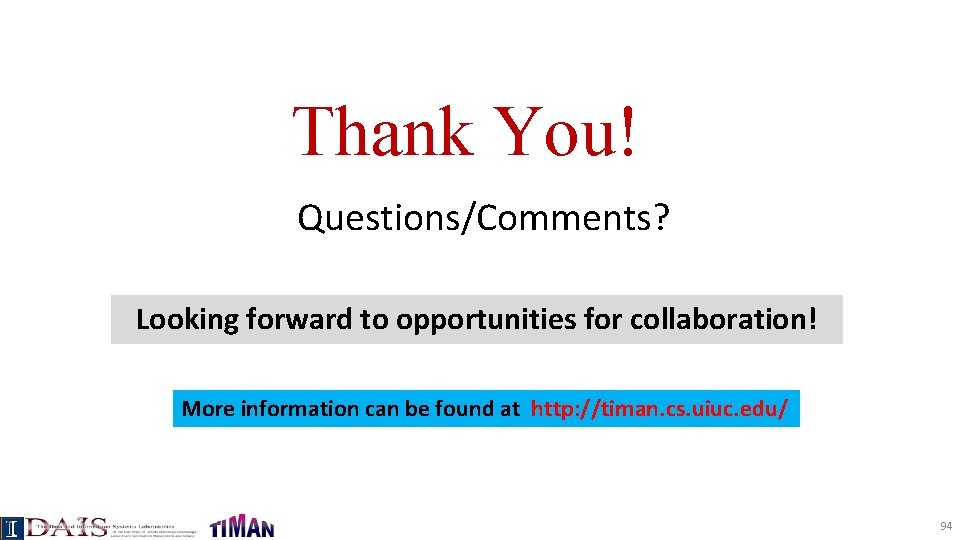
- Slides: 94
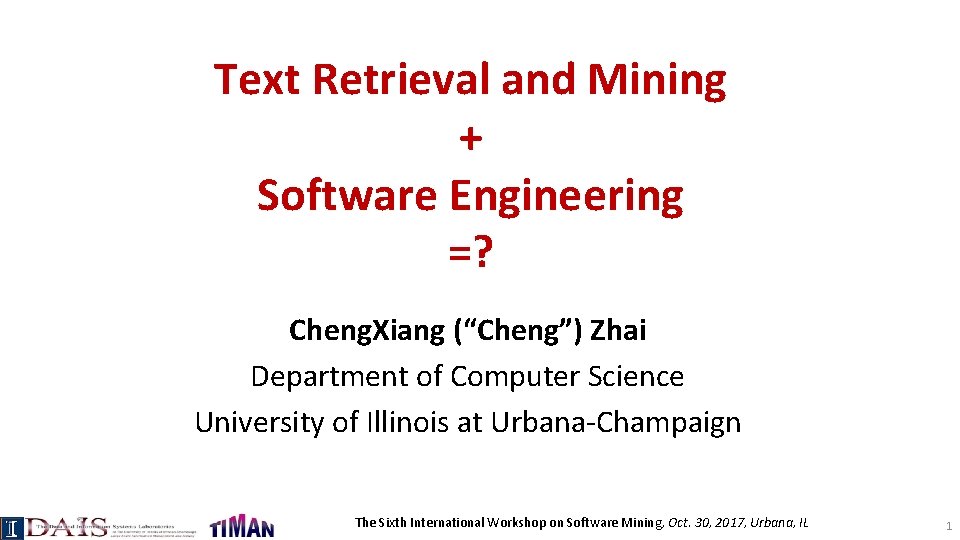
Text Retrieval and Mining + Software Engineering =? Cheng. Xiang (“Cheng”) Zhai Department of Computer Science University of Illinois at Urbana-Champaign The Sixth International Workshop on Software Mining, Oct. 30, 2017, Urbana, IL 1
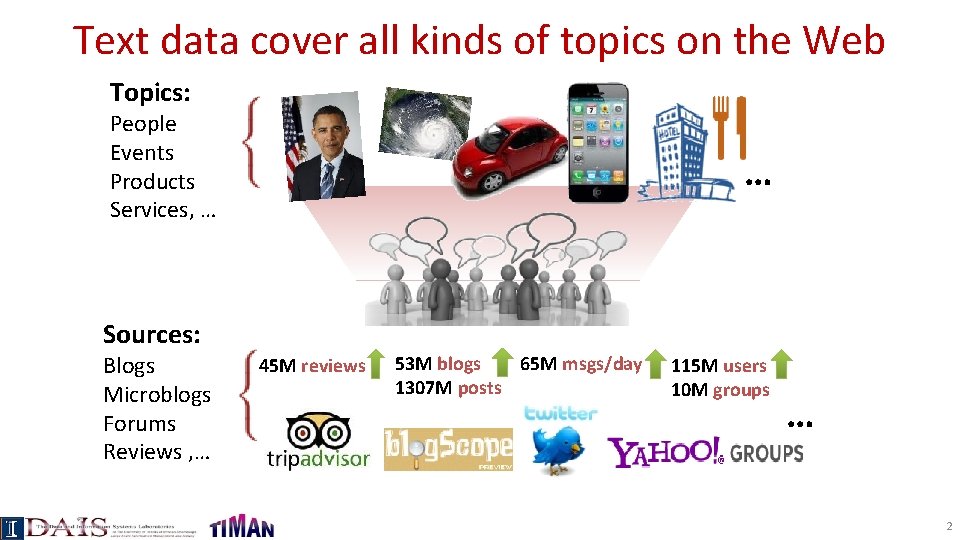
Text data cover all kinds of topics on the Web Topics: People Events Products Services, … … Sources: Blogs Microblogs Forums Reviews , … 45 M reviews 65 M msgs/day 53 M blogs 1307 M posts 115 M users 10 M groups … 2
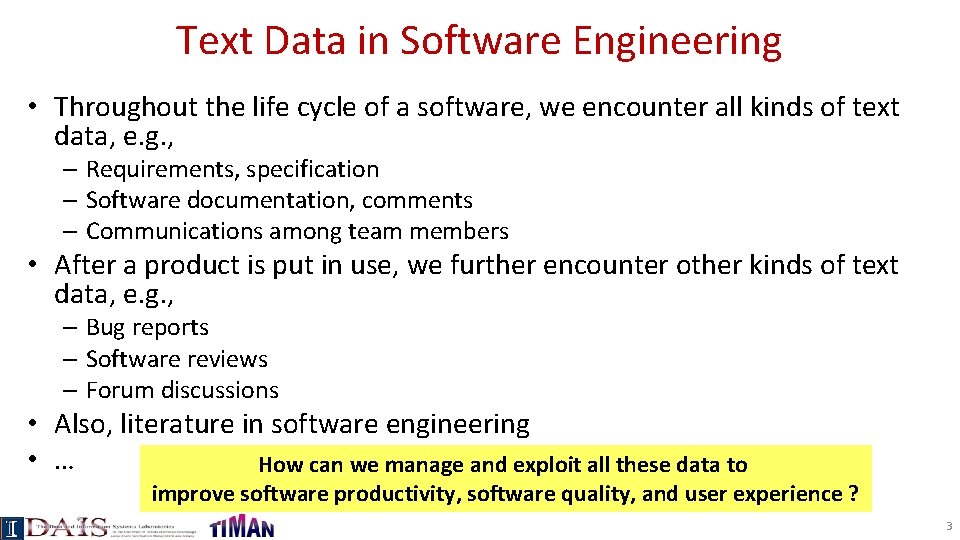
Text Data in Software Engineering • Throughout the life cycle of a software, we encounter all kinds of text data, e. g. , – Requirements, specification – Software documentation, comments – Communications among team members • After a product is put in use, we further encounter other kinds of text data, e. g. , – Bug reports – Software reviews – Forum discussions • Also, literature in software engineering • … How can we manage and exploit all these data to improve software productivity, software quality, and user experience ? 3
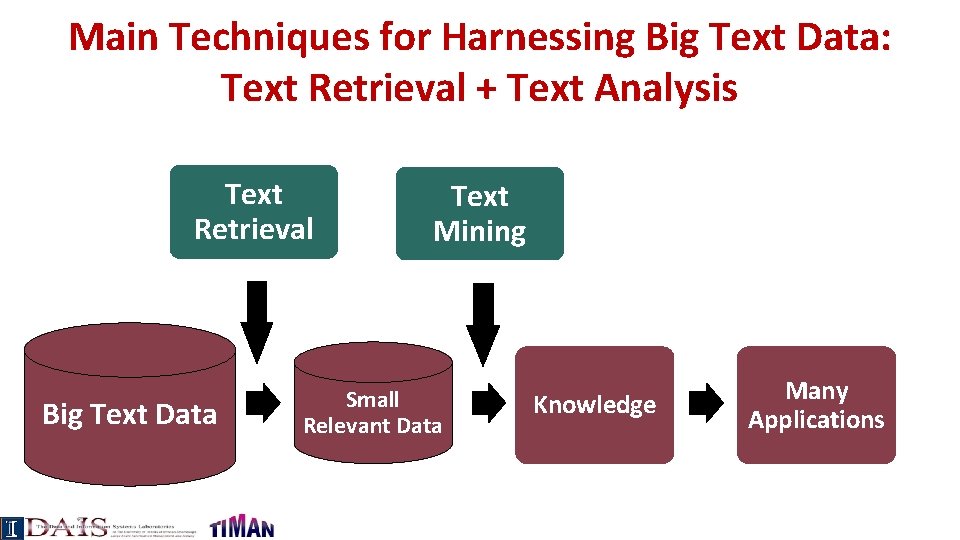
Main Techniques for Harnessing Big Text Data: Text Retrieval + Text Analysis Text Retrieval Big Text Data Text Mining Small Relevant Small Data Relevant Data Knowledge Many Applications
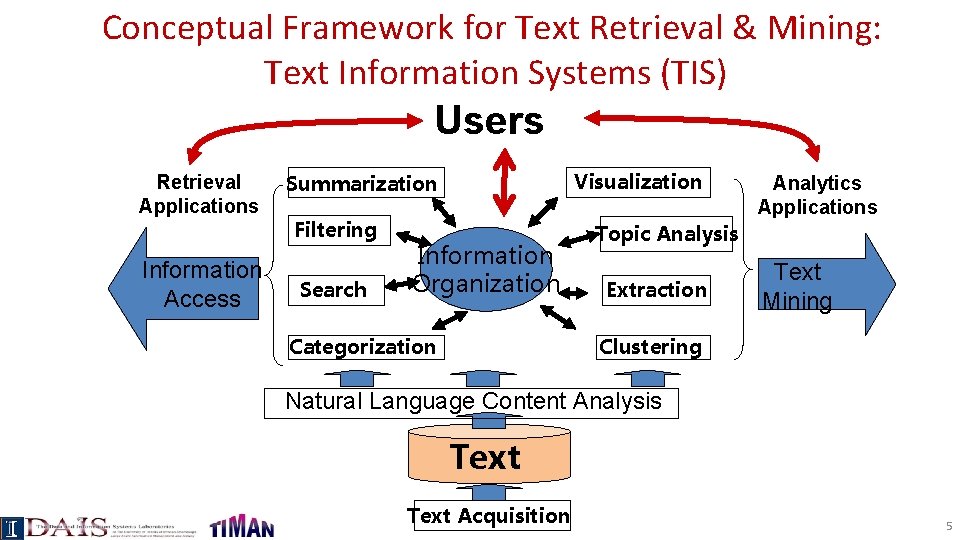
Conceptual Framework for Text Retrieval & Mining: Text Information Systems (TIS) Users Retrieval Applications Information Access Visualization Summarization Filtering Search Information Organization Categorization Analytics Applications Topic Analysis Extraction Text Mining Clustering Natural Language Content Analysis Text Acquisition 5
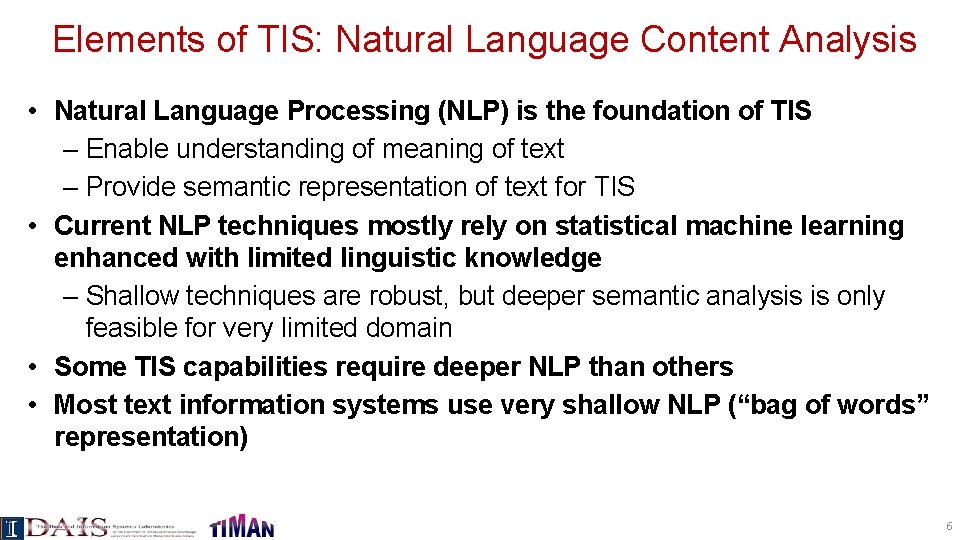
Elements of TIS: Natural Language Content Analysis • Natural Language Processing (NLP) is the foundation of TIS – Enable understanding of meaning of text – Provide semantic representation of text for TIS • Current NLP techniques mostly rely on statistical machine learning enhanced with limited linguistic knowledge – Shallow techniques are robust, but deeper semantic analysis is only feasible for very limited domain • Some TIS capabilities require deeper NLP than others • Most text information systems use very shallow NLP (“bag of words” representation) 6
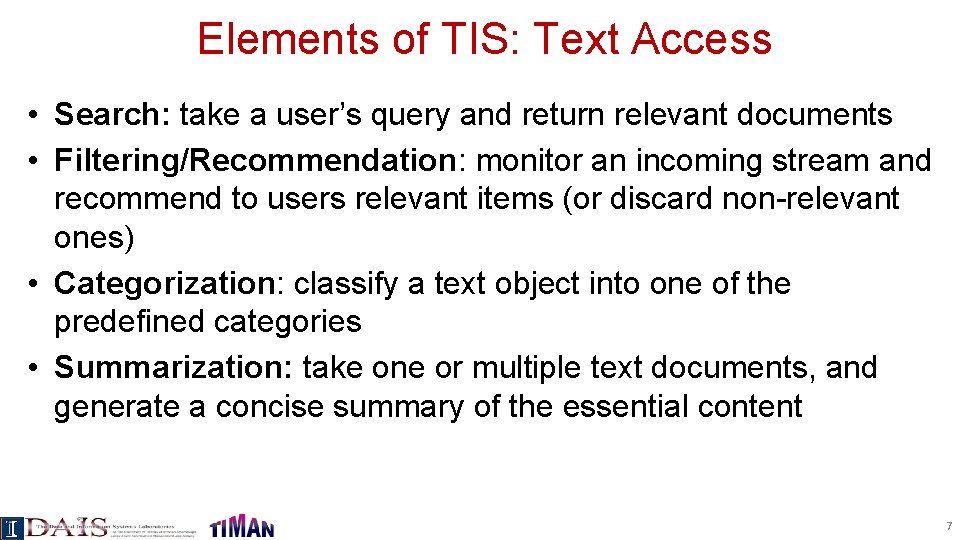
Elements of TIS: Text Access • Search: take a user’s query and return relevant documents • Filtering/Recommendation: monitor an incoming stream and recommend to users relevant items (or discard non-relevant ones) • Categorization: classify a text object into one of the predefined categories • Summarization: take one or multiple text documents, and generate a concise summary of the essential content 7
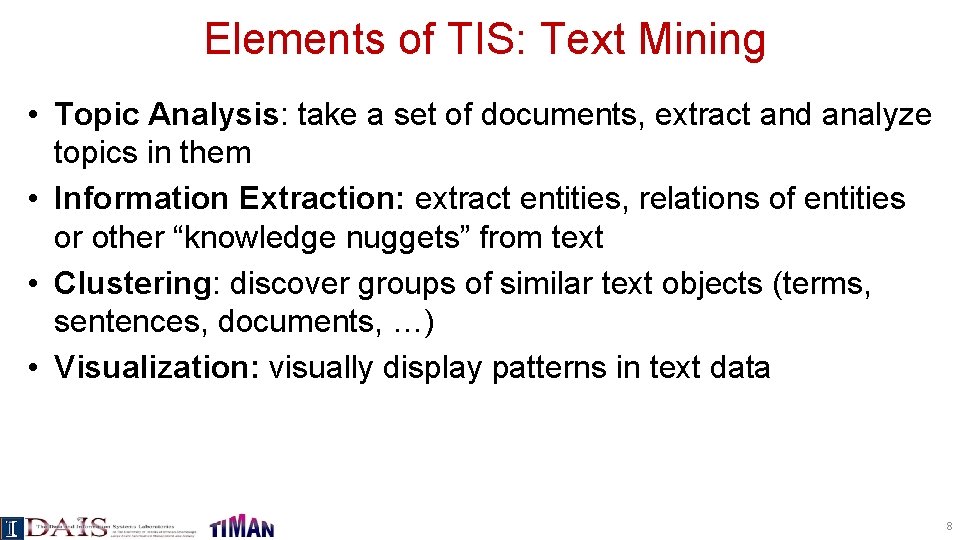
Elements of TIS: Text Mining • Topic Analysis: take a set of documents, extract and analyze topics in them • Information Extraction: extract entities, relations of entities or other “knowledge nuggets” from text • Clustering: discover groups of similar text objects (terms, sentences, documents, …) • Visualization: visually display patterns in text data 8
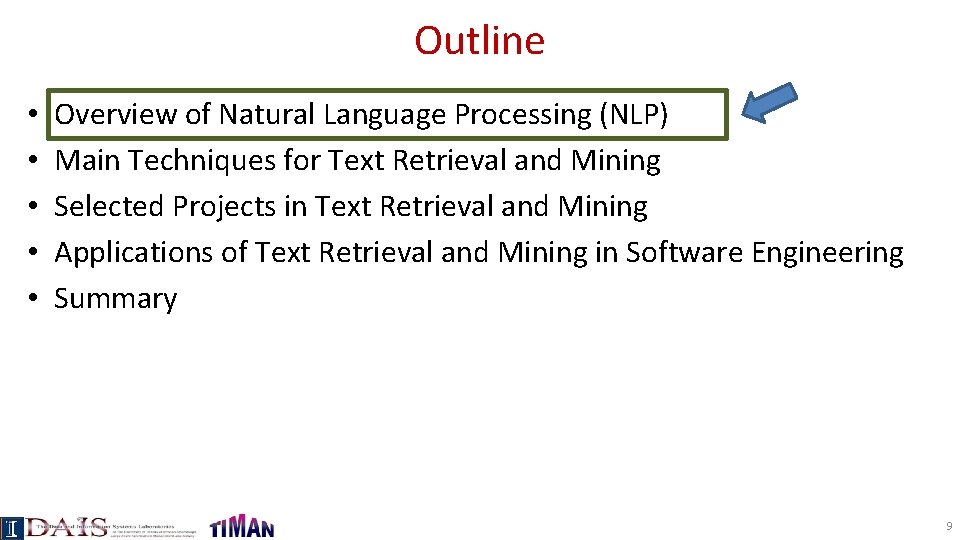
Outline • • • Overview of Natural Language Processing (NLP) Main Techniques for Text Retrieval and Mining Selected Projects in Text Retrieval and Mining Applications of Text Retrieval and Mining in Software Engineering Summary 9
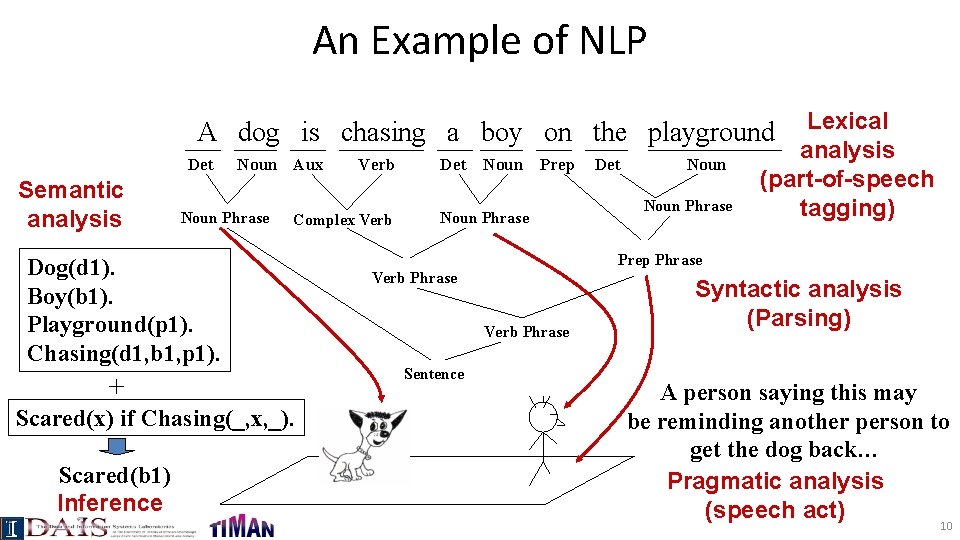
An Example of NLP Lexical analysis (part-of-speech tagging) A dog is chasing a boy on the playground Det Semantic analysis Noun Aux Noun Phrase Complex Verb Dog(d 1). Boy(b 1). Playground(p 1). Chasing(d 1, b 1, p 1). + Scared(x) if Chasing(_, x, _). Scared(b 1) Inference Verb Det Noun Prep Noun Phrase Det Noun Phrase Prep Phrase Verb Phrase Sentence Syntactic analysis (Parsing) A person saying this may be reminding another person to get the dog back… Pragmatic analysis (speech act) 10
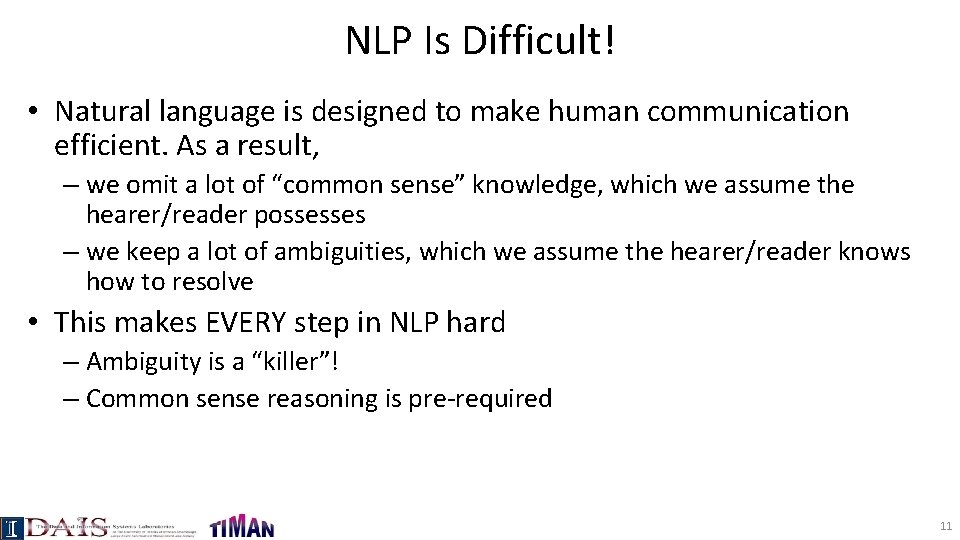
NLP Is Difficult! • Natural language is designed to make human communication efficient. As a result, – we omit a lot of “common sense” knowledge, which we assume the hearer/reader possesses – we keep a lot of ambiguities, which we assume the hearer/reader knows how to resolve • This makes EVERY step in NLP hard – Ambiguity is a “killer”! – Common sense reasoning is pre-required 11
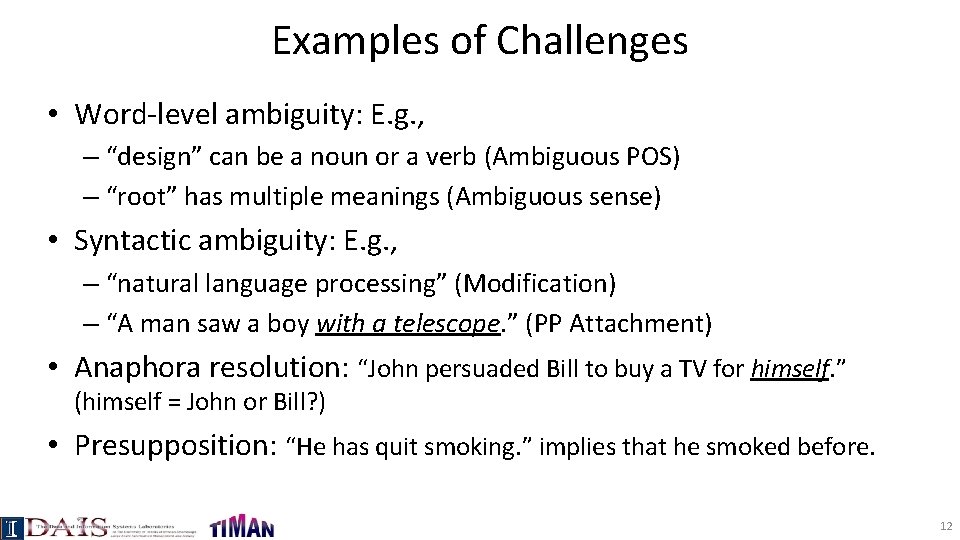
Examples of Challenges • Word-level ambiguity: E. g. , – “design” can be a noun or a verb (Ambiguous POS) – “root” has multiple meanings (Ambiguous sense) • Syntactic ambiguity: E. g. , – “natural language processing” (Modification) – “A man saw a boy with a telescope. ” (PP Attachment) • Anaphora resolution: “John persuaded Bill to buy a TV for himself. ” (himself = John or Bill? ) • Presupposition: “He has quit smoking. ” implies that he smoked before. 12
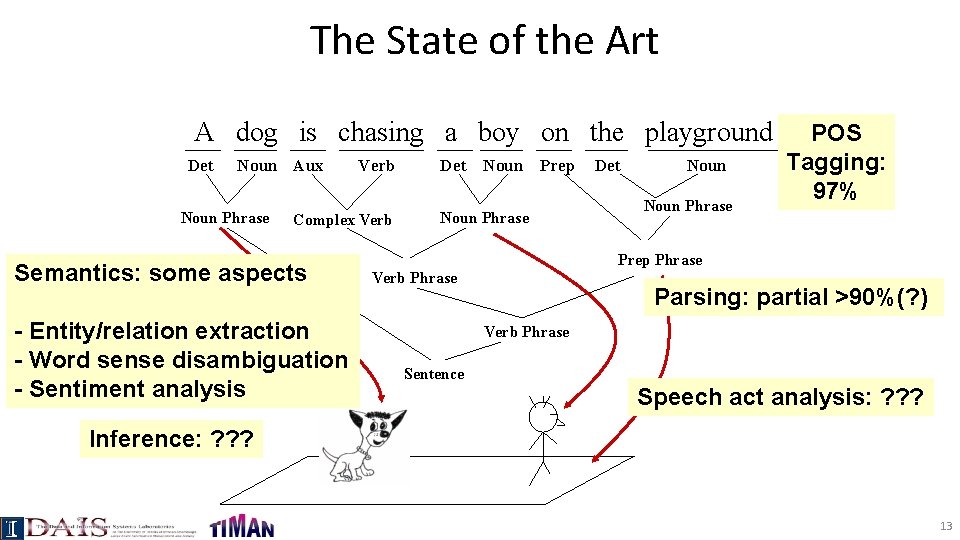
The State of the Art A dog is chasing a boy on the playground Det Noun Aux Noun Phrase Verb Complex Verb Semantics: some aspects - Entity/relation extraction - Word sense disambiguation - Sentiment analysis Det Noun Prep Noun Phrase Det Noun Phrase POS Tagging: 97% Prep Phrase Verb Phrase Parsing: partial >90%(? ) Verb Phrase Sentence Speech act analysis: ? ? ? Inference: ? ? ? 13
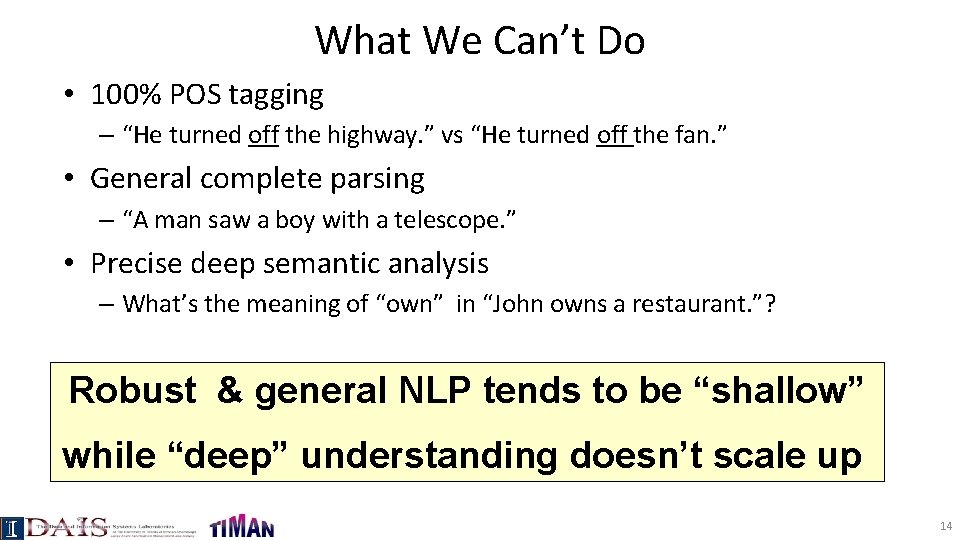
What We Can’t Do • 100% POS tagging – “He turned off the highway. ” vs “He turned off the fan. ” • General complete parsing – “A man saw a boy with a telescope. ” • Precise deep semantic analysis – What’s the meaning of “own” in “John owns a restaurant. ”? Robust & general NLP tends to be “shallow” while “deep” understanding doesn’t scale up 14
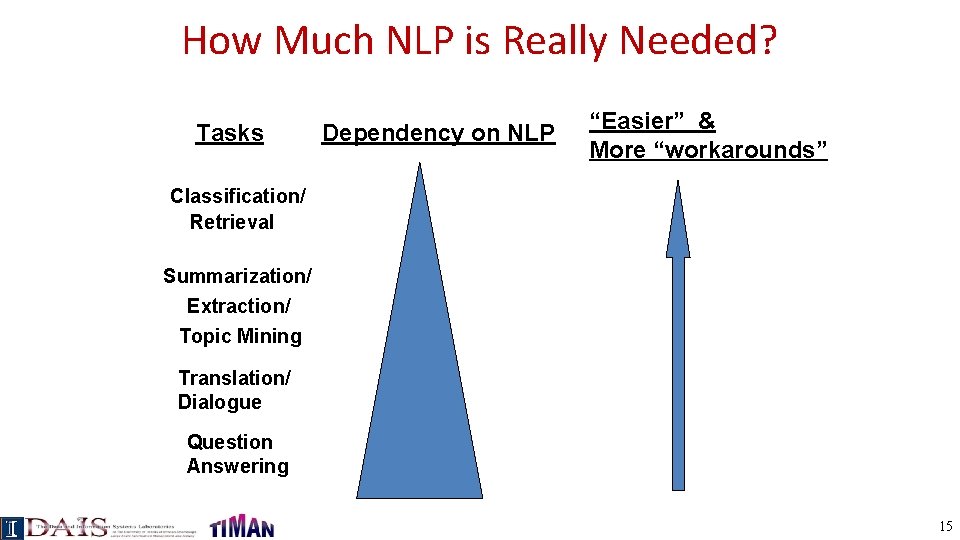
How Much NLP is Really Needed? Tasks Dependency on NLP “Easier” & More “workarounds” Classification/ Retrieval Summarization/ Extraction/ Topic Mining Translation/ Dialogue Question Answering 15
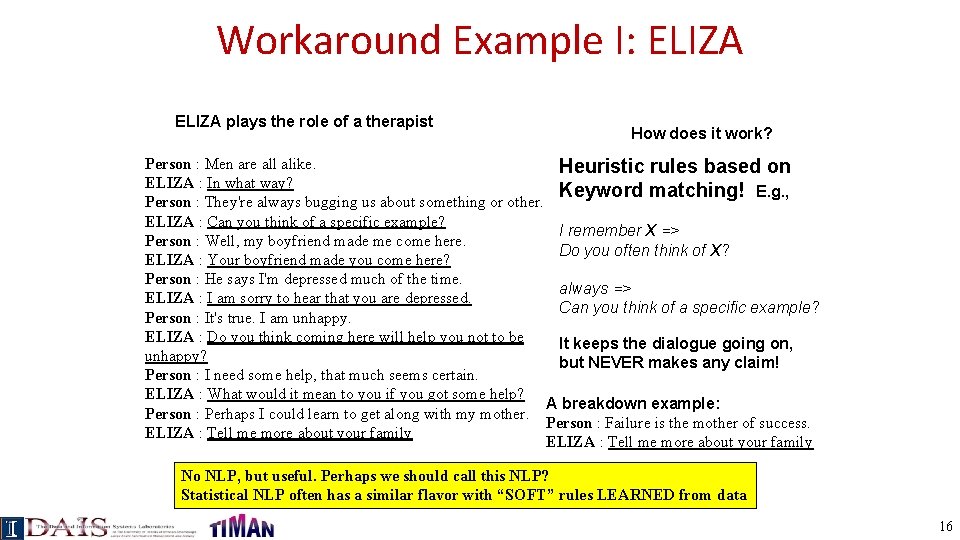
Workaround Example I: ELIZA plays the role of a therapist How does it work? Person : Men are all alike. Heuristic rules based on ELIZA : In what way? Keyword matching! E. g. , Person : They're always bugging us about something or other. ELIZA : Can you think of a specific example? I remember X => Person : Well, my boyfriend made me come here. Do you often think of X? ELIZA : Your boyfriend made you come here? Person : He says I'm depressed much of the time. always => ELIZA : I am sorry to hear that you are depressed. Can you think of a specific example? Person : It's true. I am unhappy. ELIZA : Do you think coming here will help you not to be It keeps the dialogue going on, unhappy? but NEVER makes any claim! Person : I need some help, that much seems certain. ELIZA : What would it mean to you if you got some help? A breakdown example: Person : Perhaps I could learn to get along with my mother. Person : Failure is the mother of success. ELIZA : Tell me more about your family No NLP, but useful. Perhaps we should call this NLP? Statistical NLP often has a similar flavor with “SOFT” rules LEARNED from data 16
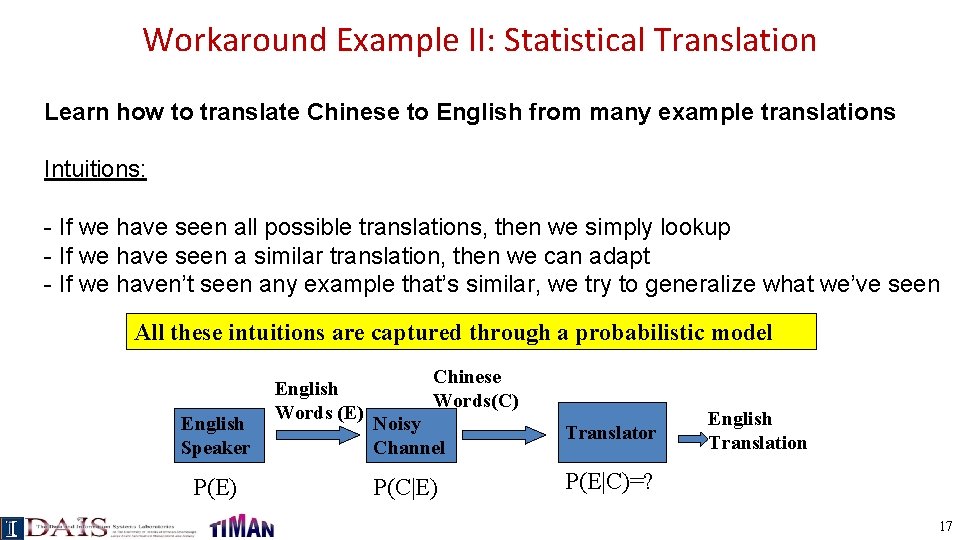
Workaround Example II: Statistical Translation Learn how to translate Chinese to English from many example translations Intuitions: - If we have seen all possible translations, then we simply lookup - If we have seen a similar translation, then we can adapt - If we haven’t seen any example that’s similar, we try to generalize what we’ve seen All these intuitions are captured through a probabilistic model English Speaker P(E) English Words (E) Chinese Words(C) Noisy Channel Translator P(C|E) P(E|C)=? English Translation 17
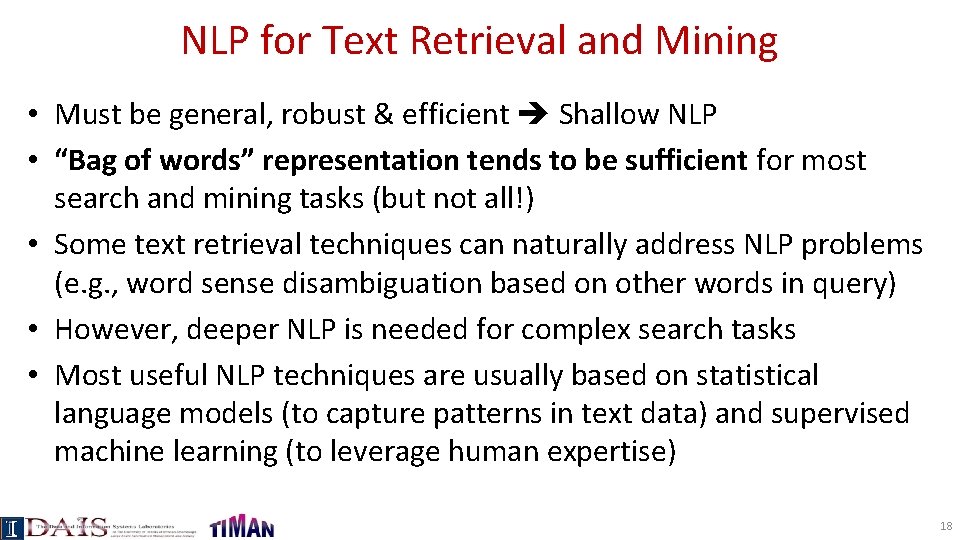
NLP for Text Retrieval and Mining • Must be general, robust & efficient Shallow NLP • “Bag of words” representation tends to be sufficient for most search and mining tasks (but not all!) • Some text retrieval techniques can naturally address NLP problems (e. g. , word sense disambiguation based on other words in query) • However, deeper NLP is needed for complex search tasks • Most useful NLP techniques are usually based on statistical language models (to capture patterns in text data) and supervised machine learning (to leverage human expertise) 18
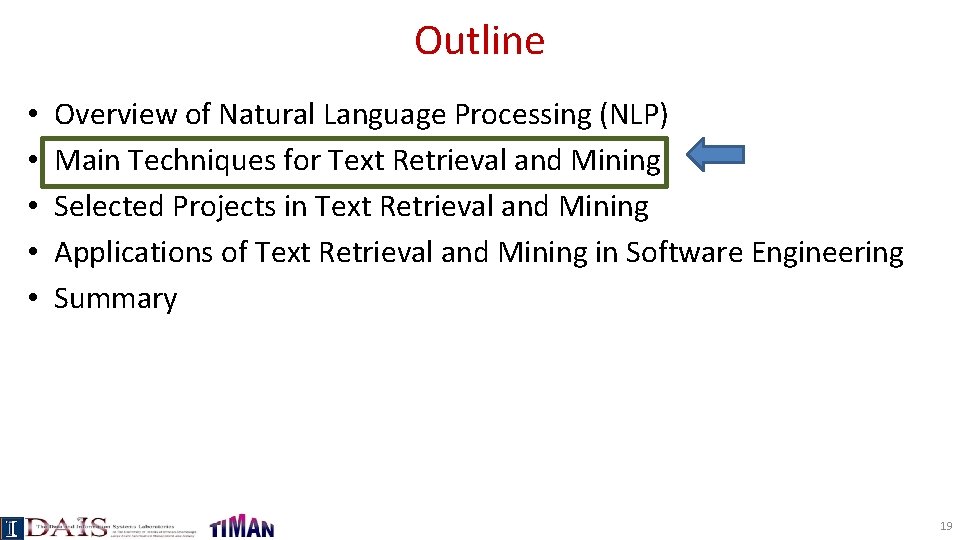
Outline • • • Overview of Natural Language Processing (NLP) Main Techniques for Text Retrieval and Mining Selected Projects in Text Retrieval and Mining Applications of Text Retrieval and Mining in Software Engineering Summary 19
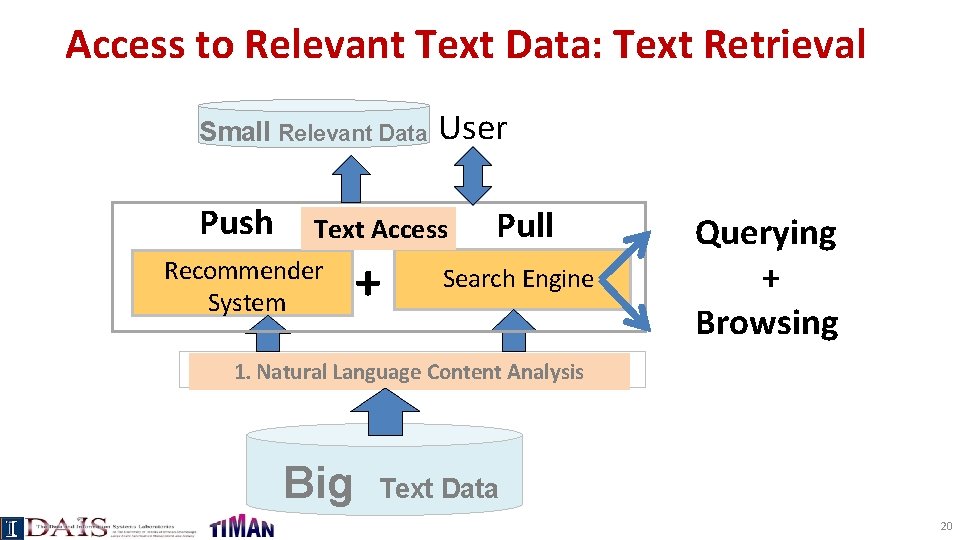
Access to Relevant Text Data: Text Retrieval Small Relevant Data Push User Text Access Recommender System + Pull Search Engine Querying + Browsing 1. Natural Language Content Analysis Big Text Data 20
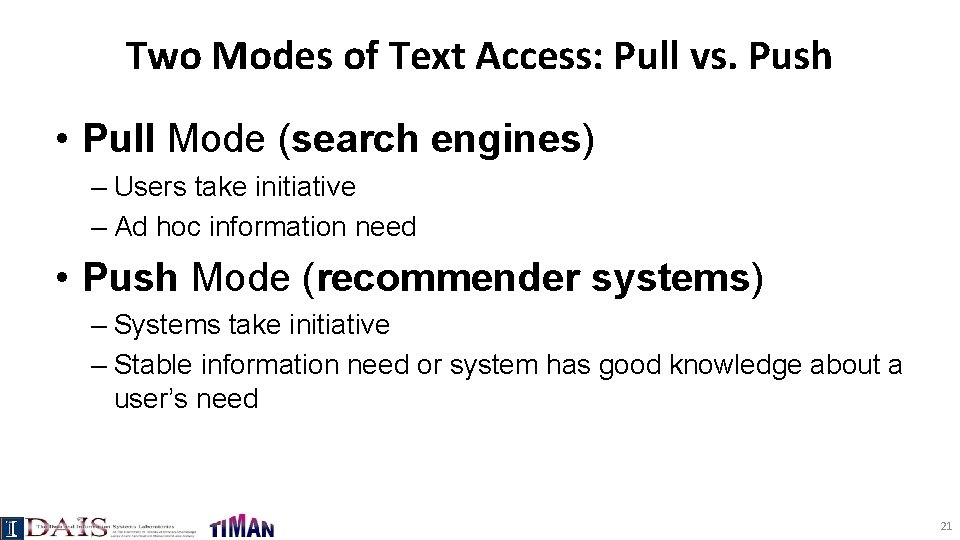
Two Modes of Text Access: Pull vs. Push • Pull Mode (search engines) – Users take initiative – Ad hoc information need • Push Mode (recommender systems) – Systems take initiative – Stable information need or system has good knowledge about a user’s need 21
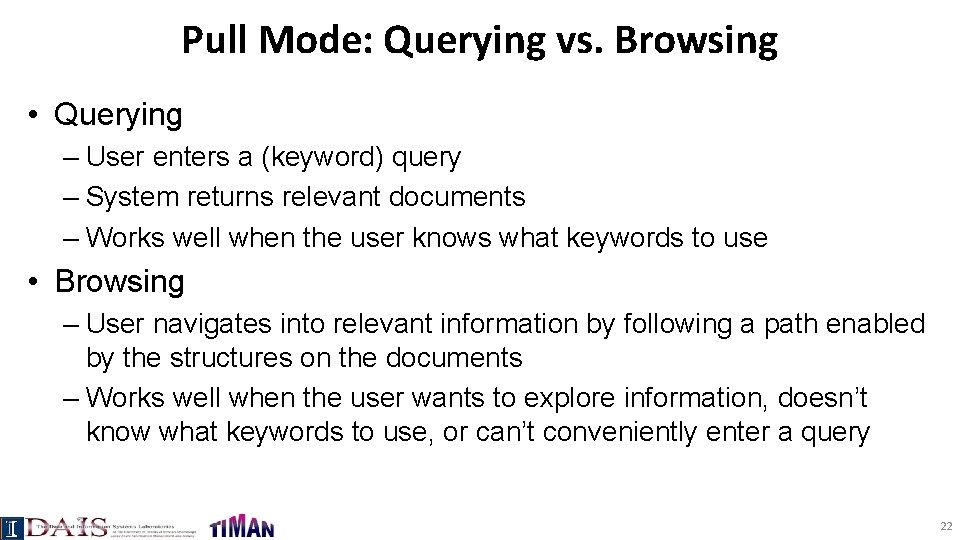
Pull Mode: Querying vs. Browsing • Querying – User enters a (keyword) query – System returns relevant documents – Works well when the user knows what keywords to use • Browsing – User navigates into relevant information by following a path enabled by the structures on the documents – Works well when the user wants to explore information, doesn’t know what keywords to use, or can’t conveniently enter a query 22
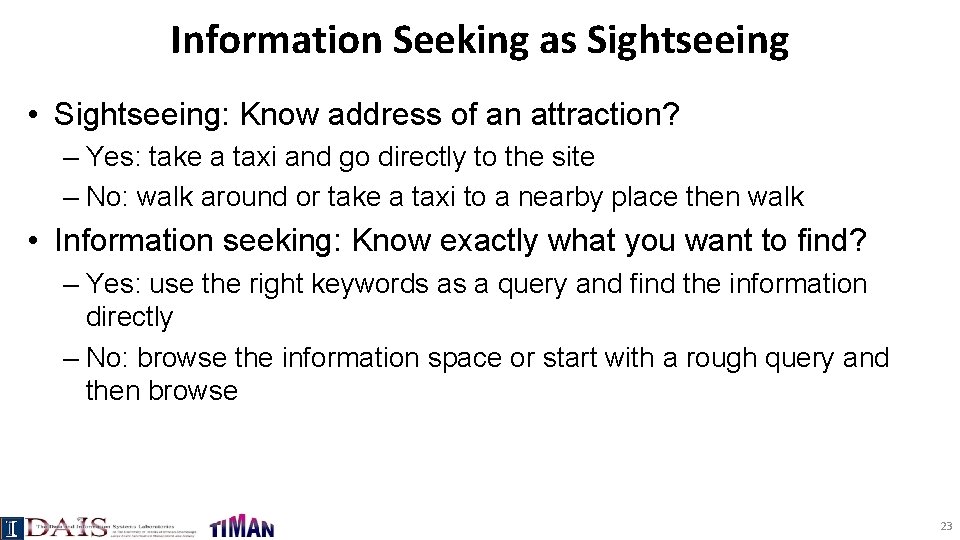
Information Seeking as Sightseeing • Sightseeing: Know address of an attraction? – Yes: take a taxi and go directly to the site – No: walk around or take a taxi to a nearby place then walk • Information seeking: Know exactly what you want to find? – Yes: use the right keywords as a query and find the information directly – No: browse the information space or start with a rough query and then browse 23
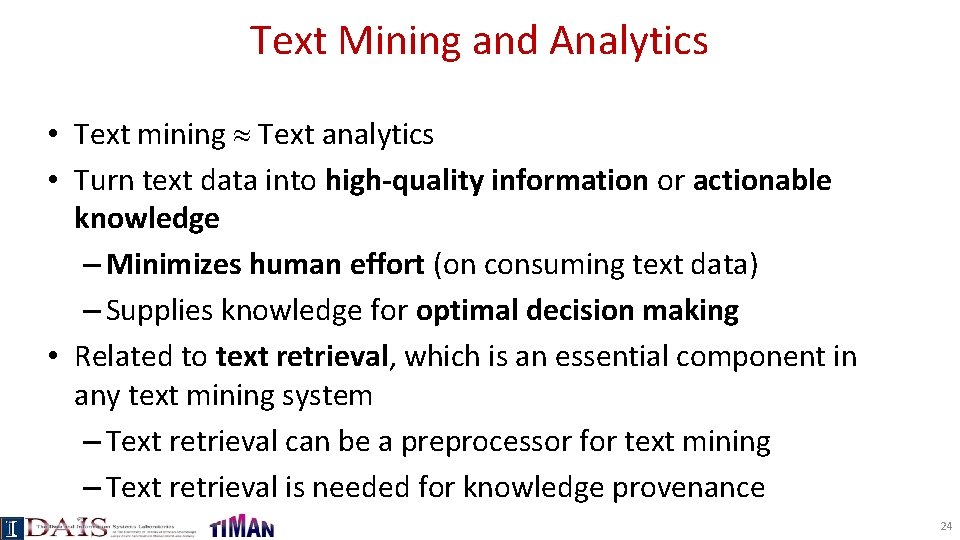
Text Mining and Analytics • Text mining Text analytics • Turn text data into high-quality information or actionable knowledge – Minimizes human effort (on consuming text data) – Supplies knowledge for optimal decision making • Related to text retrieval, which is an essential component in any text mining system – Text retrieval can be a preprocessor for text mining – Text retrieval is needed for knowledge provenance 24
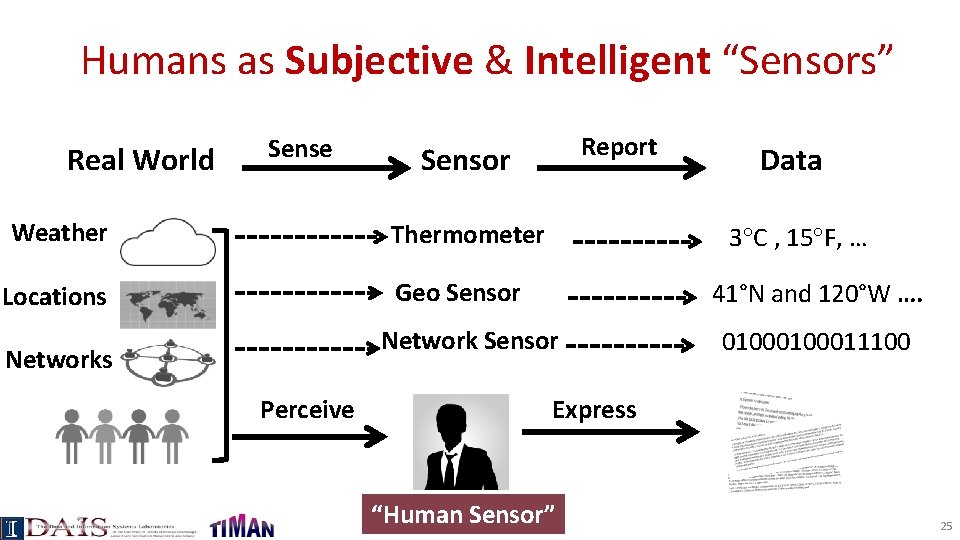
Humans as Subjective & Intelligent “Sensors” Real World Sense Weather Report Sensor Thermometer 3 C , 15 F, … Geo Sensor Locations 41°N and 120°W …. Network Sensor Networks Perceive Data 0100011100 Express “Human Sensor” 25
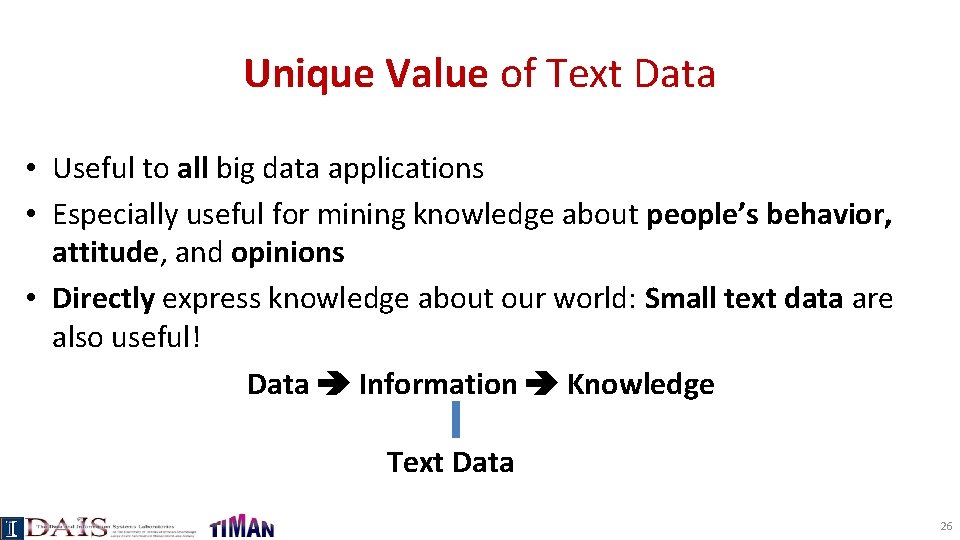
Unique Value of Text Data • Useful to all big data applications • Especially useful for mining knowledge about people’s behavior, attitude, and opinions • Directly express knowledge about our world: Small text data are also useful! Data Information Knowledge Text Data 26
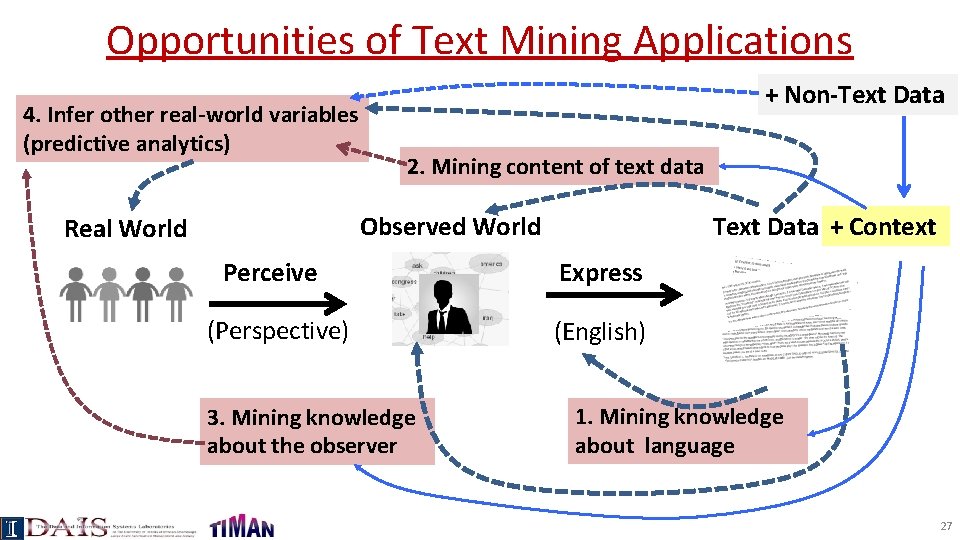
Opportunities of Text Mining Applications 4. Infer other real-world variables (predictive analytics) + Non-Text Data 2. Mining content of text data Observed World Real World Text Data + Context Perceive Express (Perspective) (English) 3. Mining knowledge about the observer 1. Mining knowledge about language 27
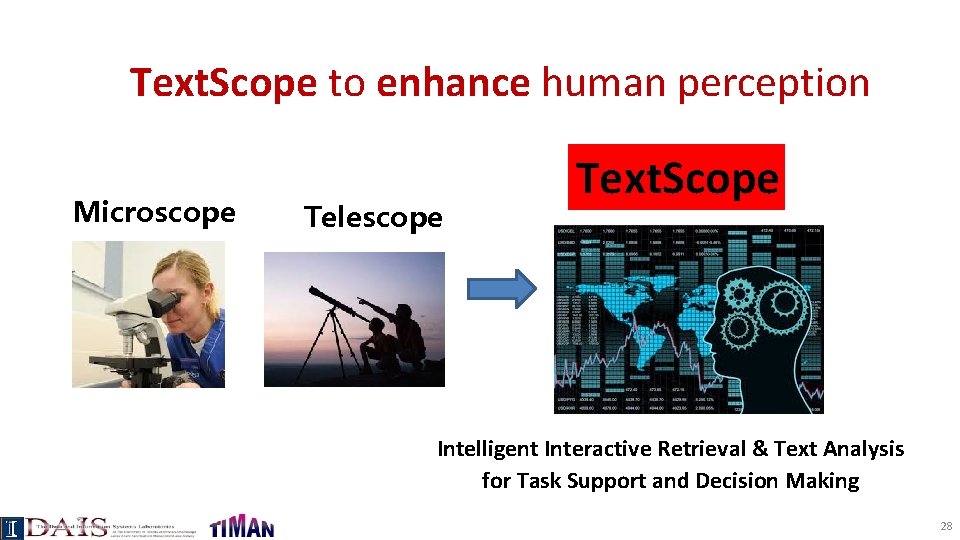
Text. Scope to enhance human perception Microscope Telescope Text. Scope Intelligent Interactive Retrieval & Text Analysis for Task Support and Decision Making 28
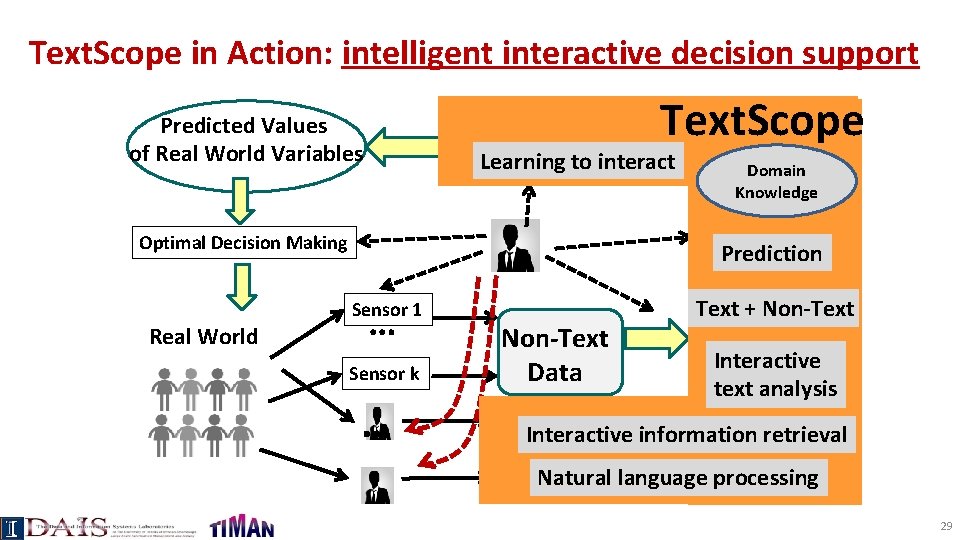
Text. Scope in Action: intelligent interactive decision support Multiple Text. Scope Predictors Predicted Values of Real World Variables Predictive Learning Model to interact Domain (Features) … Knowledge Optimal Decision Making Real World Prediction … Sensor 1 Sensor k … Non-Text Data Text + Non-Text Joint Mining of. Interactive Non-Text andanalysis Text text Text Interactive information retrieval Data Natural language processing 29
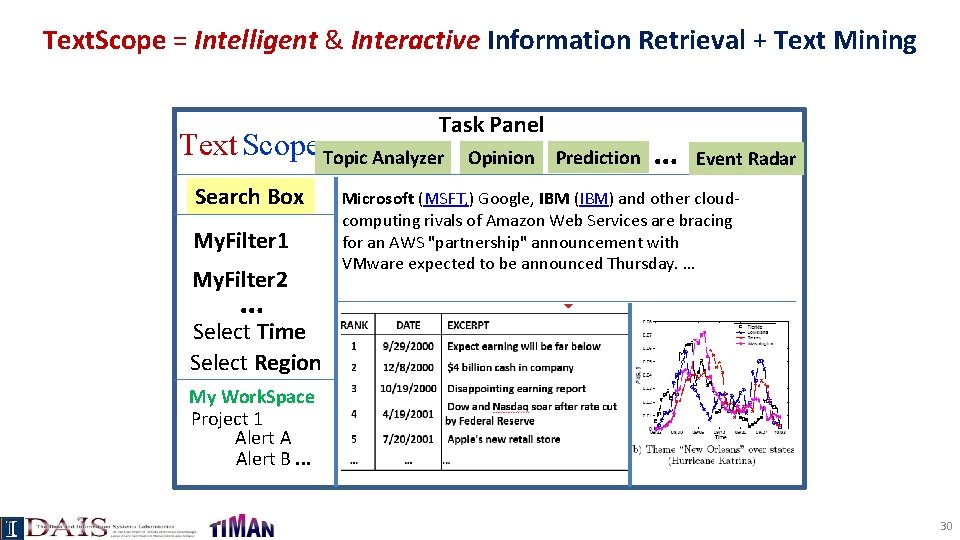
Text. Scope = Intelligent & Interactive Information Retrieval + Text Mining Task Panel Text Scope Topic Analyzer Search Box My. Filter 1 My. Filter 2 Opinion Prediction … Event Radar Microsoft (MSFT, ) Google, IBM (IBM) and other cloudcomputing rivals of Amazon Web Services are bracing for an AWS "partnership" announcement with VMware expected to be announced Thursday. … … Select Time Select Region My Work. Space Project 1 Alert A Alert B. . . 30
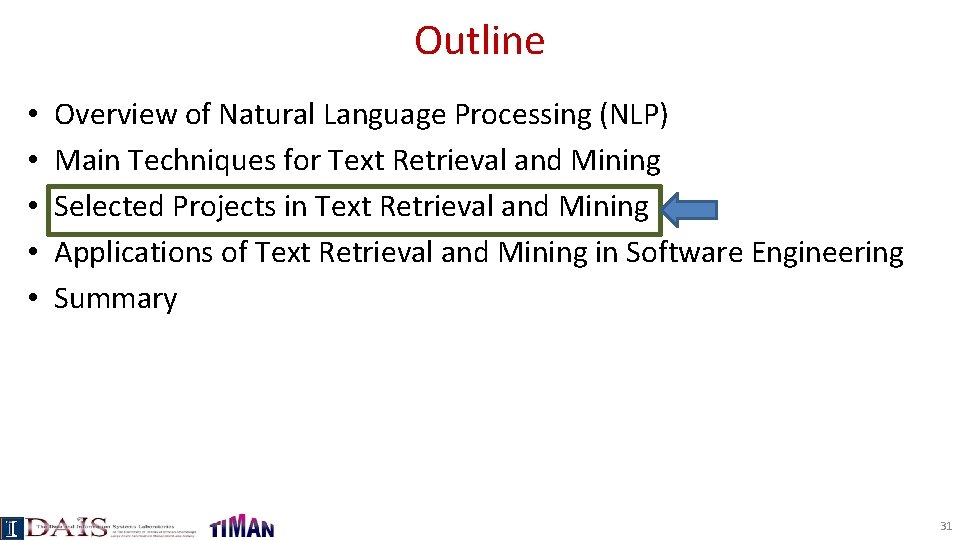
Outline • • • Overview of Natural Language Processing (NLP) Main Techniques for Text Retrieval and Mining Selected Projects in Text Retrieval and Mining Applications of Text Retrieval and Mining in Software Engineering Summary 31
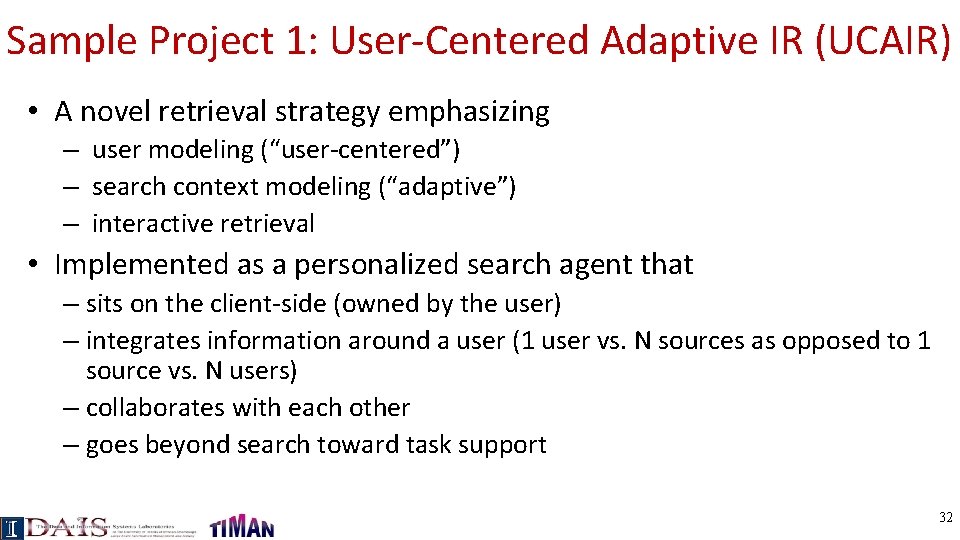
Sample Project 1: User-Centered Adaptive IR (UCAIR) • A novel retrieval strategy emphasizing – user modeling (“user-centered”) – search context modeling (“adaptive”) – interactive retrieval • Implemented as a personalized search agent that – sits on the client-side (owned by the user) – integrates information around a user (1 user vs. N sources as opposed to 1 source vs. N users) – collaborates with each other – goes beyond search toward task support 32
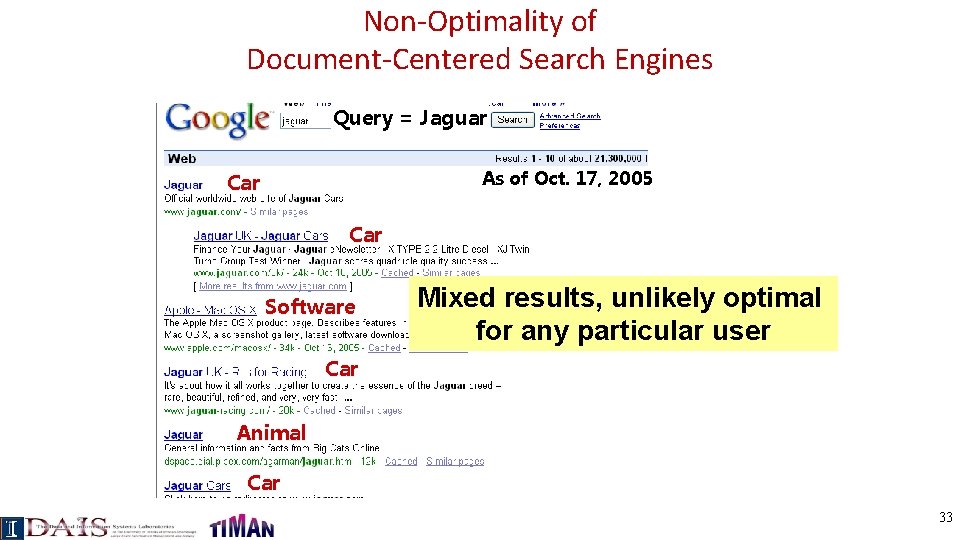
Non-Optimality of Document-Centered Search Engines Query = Jaguar As of Oct. 17, 2005 Car Software Mixed results, unlikely optimal for any particular user Car Animal Car 33
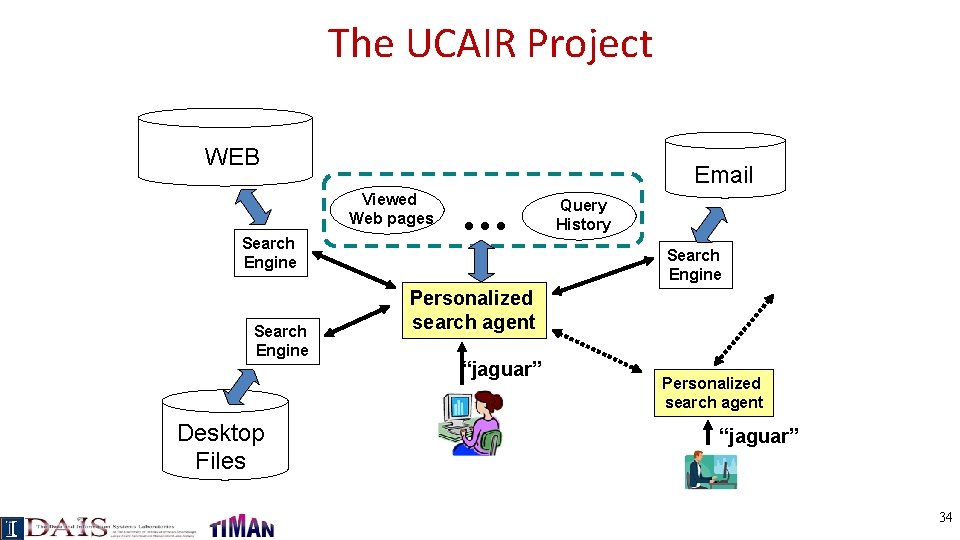
The UCAIR Project WEB Viewed Web pages Search Engine Desktop Files . . . Email Query History Search Engine Personalized search agent “jaguar” 34
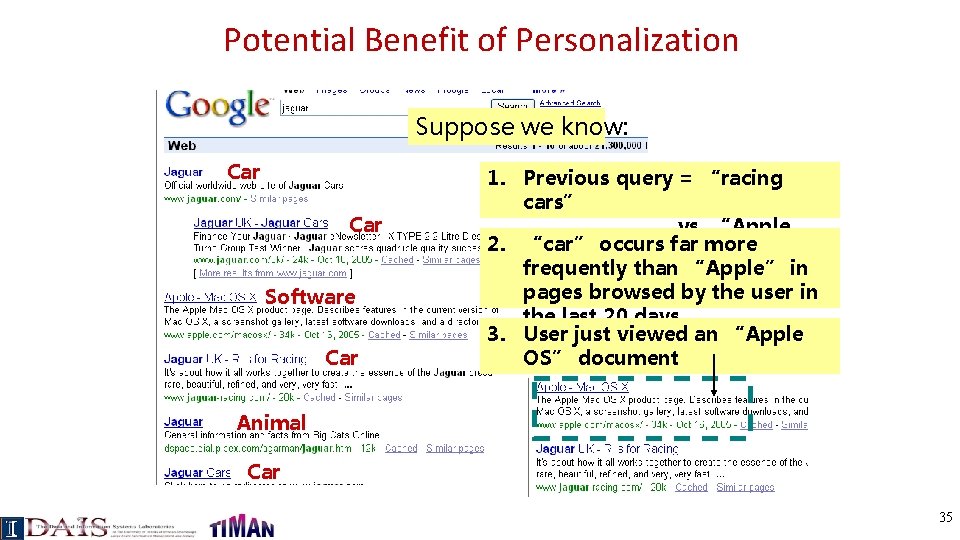
Potential Benefit of Personalization Suppose we know: Car Software Car 1. Previous query = “racing cars” vs. “Apple 2. “car” OS” occurs far more frequently than “Apple” in pages browsed by the user in the last 20 days 3. User just viewed an “Apple OS” document Animal Car 35
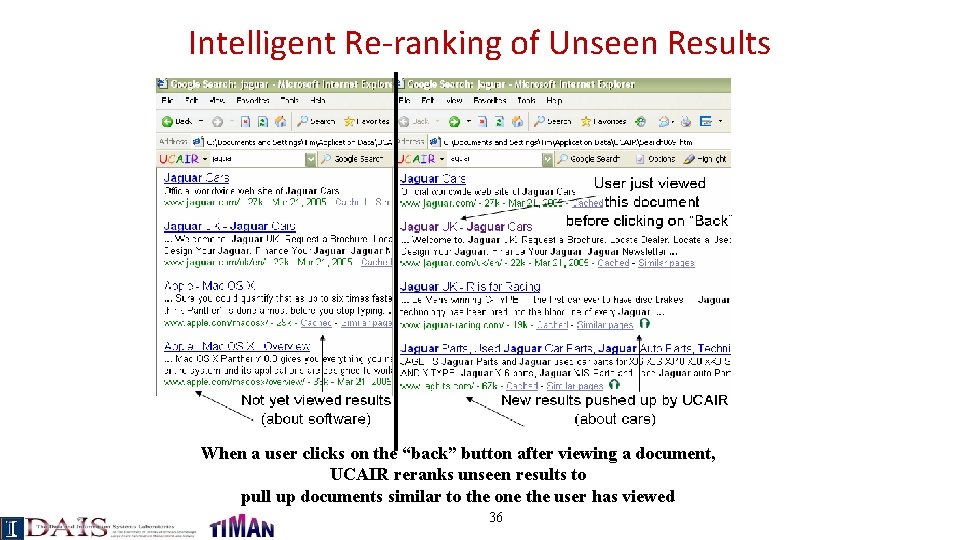
Intelligent Re-ranking of Unseen Results When a user clicks on the “back” button after viewing a document, UCAIR reranks unseen results to pull up documents similar to the one the user has viewed 36
![UCAIR Outperforms Google Shen et al 05 Precision at N documents Ranking Method prec5 UCAIR Outperforms Google [Shen et al. 05] Precision at N documents Ranking Method prec@5](https://slidetodoc.com/presentation_image_h/d5a0aa61765e85e4b4d6978a82a63873/image-37.jpg)
UCAIR Outperforms Google [Shen et al. 05] Precision at N documents Ranking Method prec@5 prec@10 prec@20 prec@30 Google UCAIR 0. 538 0. 581 0. 472 0. 556 0. 377 0. 453 0. 308 0. 375 17. 8% 20. 2% 21. 8% Improvement 8. 0% UCAIR toolbar available at http: //sifaka. cs. uiuc. edu/ir/ucair/ 37
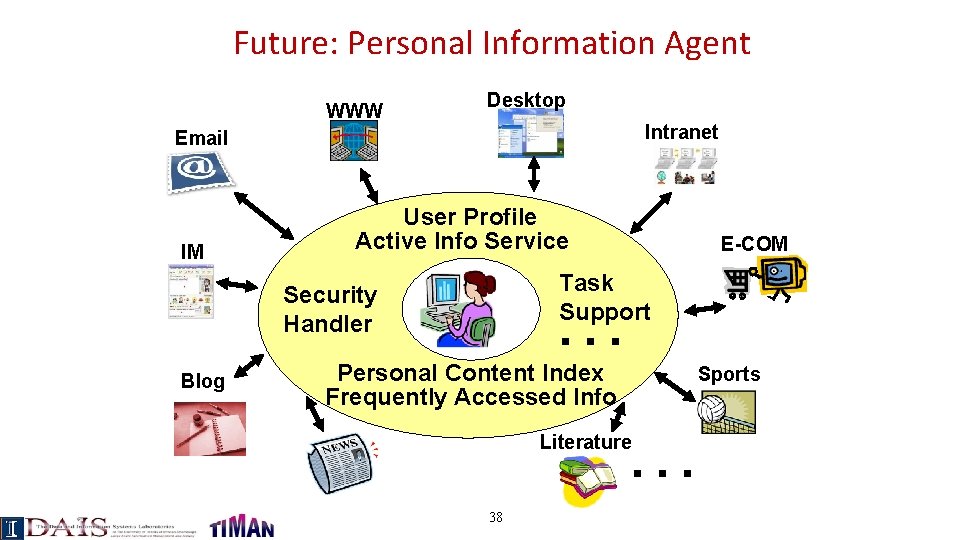
Future: Personal Information Agent WWW Desktop Intranet Email IM User Profile Active Info Service Task Support … Security Handler Blog E-COM Personal Content Index Frequently Accessed Info Sports … Literature 38
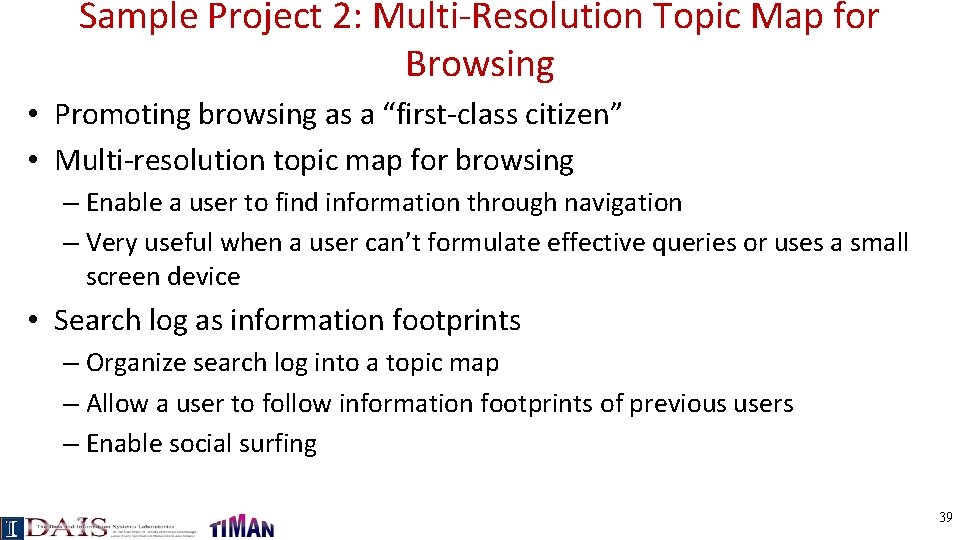
Sample Project 2: Multi-Resolution Topic Map for Browsing • Promoting browsing as a “first-class citizen” • Multi-resolution topic map for browsing – Enable a user to find information through navigation – Very useful when a user can’t formulate effective queries or uses a small screen device • Search log as information footprints – Organize search log into a topic map – Allow a user to follow information footprints of previous users – Enable social surfing 39
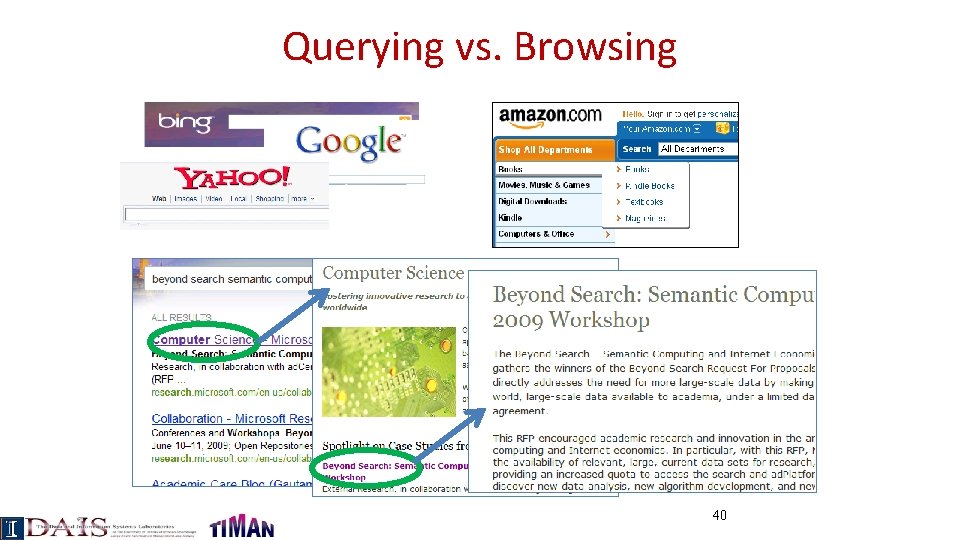
Querying vs. Browsing 40
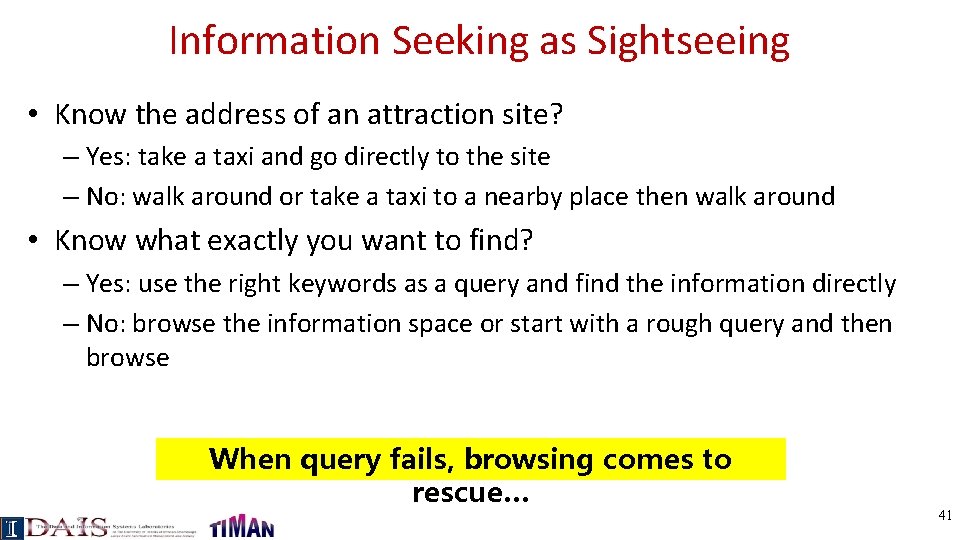
Information Seeking as Sightseeing • Know the address of an attraction site? – Yes: take a taxi and go directly to the site – No: walk around or take a taxi to a nearby place then walk around • Know what exactly you want to find? – Yes: use the right keywords as a query and find the information directly – No: browse the information space or start with a rough query and then browse When query fails, browsing comes to rescue… 41
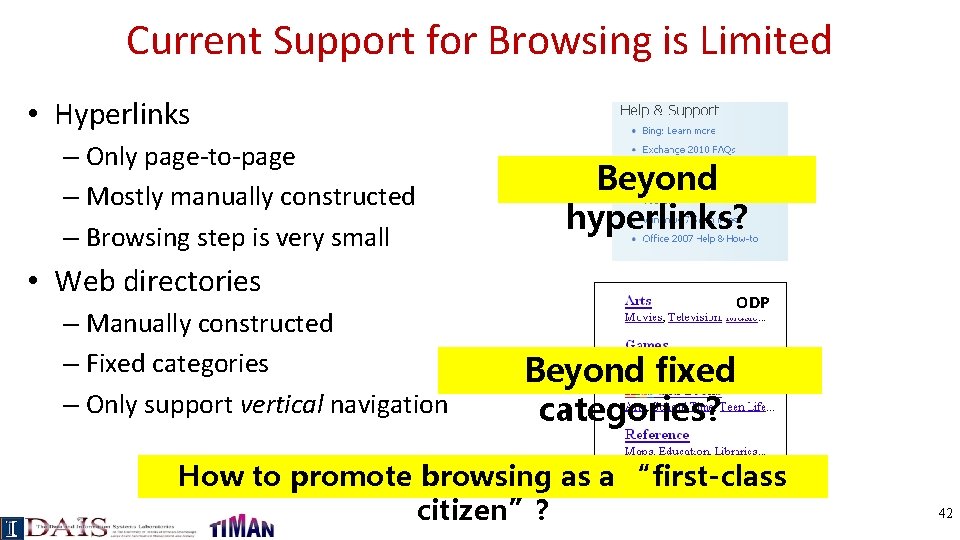
Current Support for Browsing is Limited • Hyperlinks – Only page-to-page – Mostly manually constructed – Browsing step is very small Beyond hyperlinks? • Web directories – Manually constructed – Fixed categories – Only support vertical navigation ODP Beyond fixed categories? How to promote browsing as a “first-class citizen”? 42
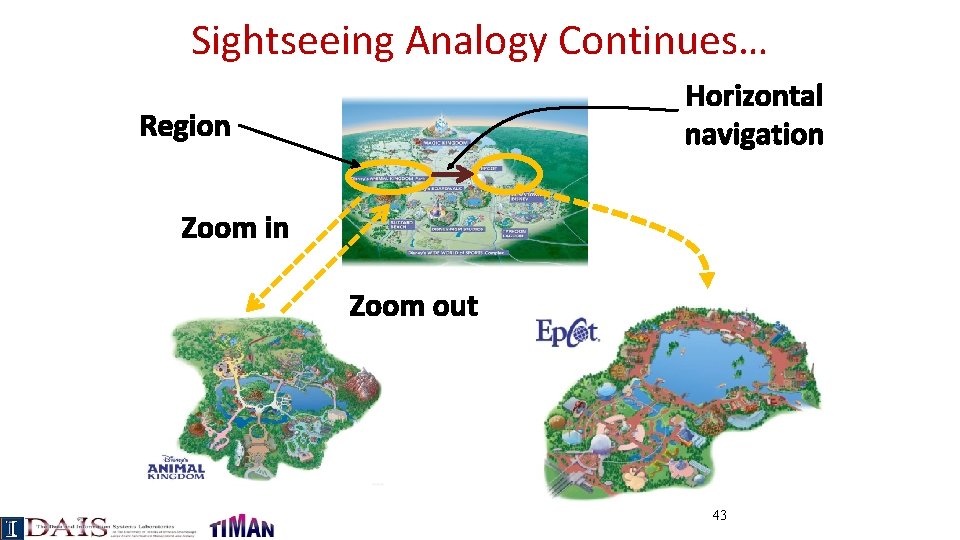
Sightseeing Analogy Continues… Horizontal navigation Region Zoom in Zoom out 43
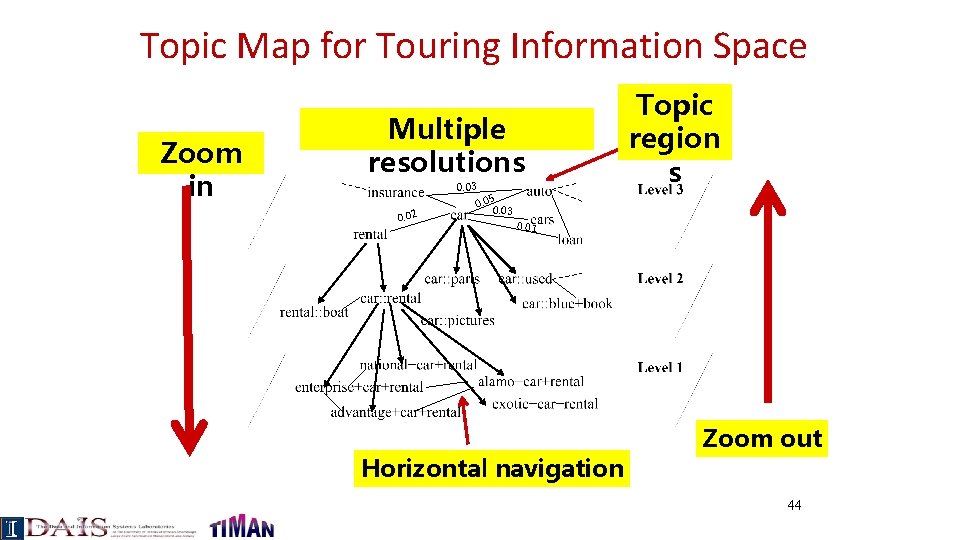
Topic Map for Touring Information Space Zoom in Multiple resolutions 0. 03 0. 02 Topic region s 0. 05 0. 03 0. 01 Horizontal navigation Zoom out 44
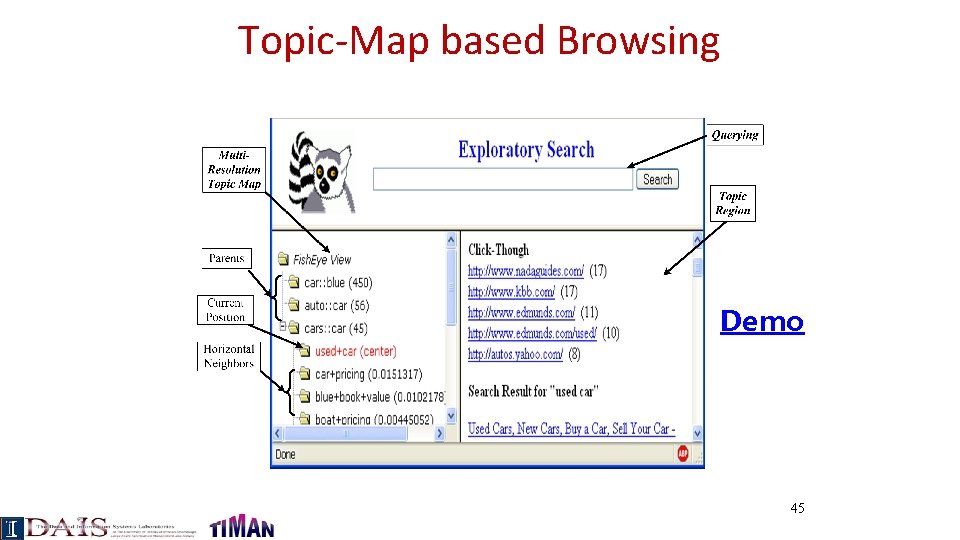
Topic-Map based Browsing Demo 45
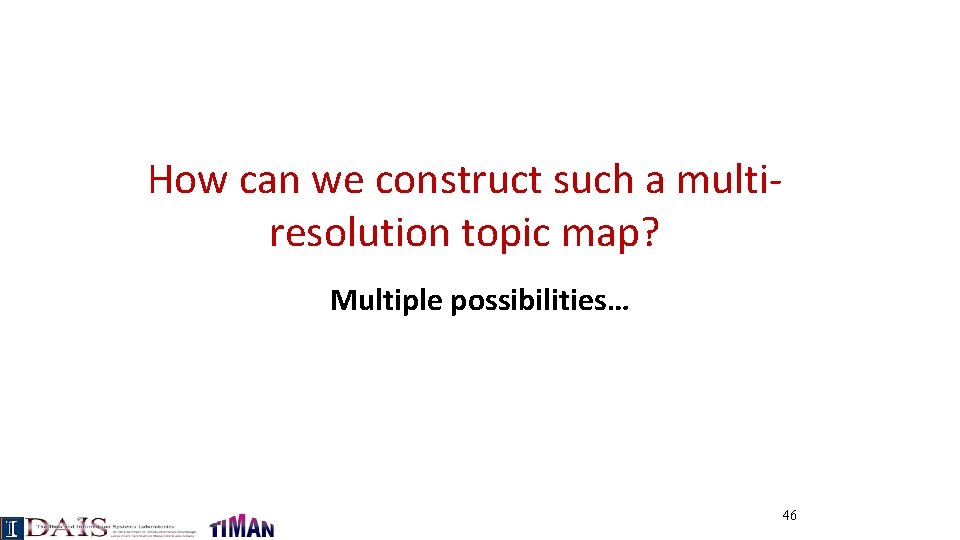
How can we construct such a multiresolution topic map? Multiple possibilities… 46
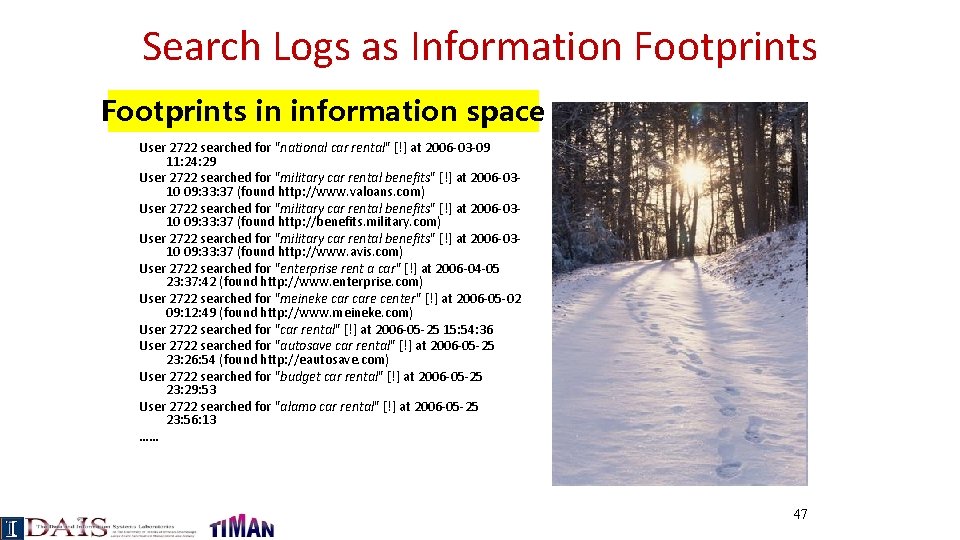
Search Logs as Information Footprints in information space User 2722 searched for "national car rental" [!] at 2006 -03 -09 11: 24: 29 User 2722 searched for "military car rental benefits" [!] at 2006 -0310 09: 33: 37 (found http: //www. valoans. com) User 2722 searched for "military car rental benefits" [!] at 2006 -0310 09: 33: 37 (found http: //benefits. military. com) User 2722 searched for "military car rental benefits" [!] at 2006 -0310 09: 33: 37 (found http: //www. avis. com) User 2722 searched for "enterprise rent a car" [!] at 2006 -04 -05 23: 37: 42 (found http: //www. enterprise. com) User 2722 searched for "meineke care center" [!] at 2006 -05 -02 09: 12: 49 (found http: //www. meineke. com) User 2722 searched for "car rental" [!] at 2006 -05 -25 15: 54: 36 User 2722 searched for "autosave car rental" [!] at 2006 -05 -25 23: 26: 54 (found http: //eautosave. com) User 2722 searched for "budget car rental" [!] at 2006 -05 -25 23: 29: 53 User 2722 searched for "alamo car rental" [!] at 2006 -05 -25 23: 56: 13 …… 47
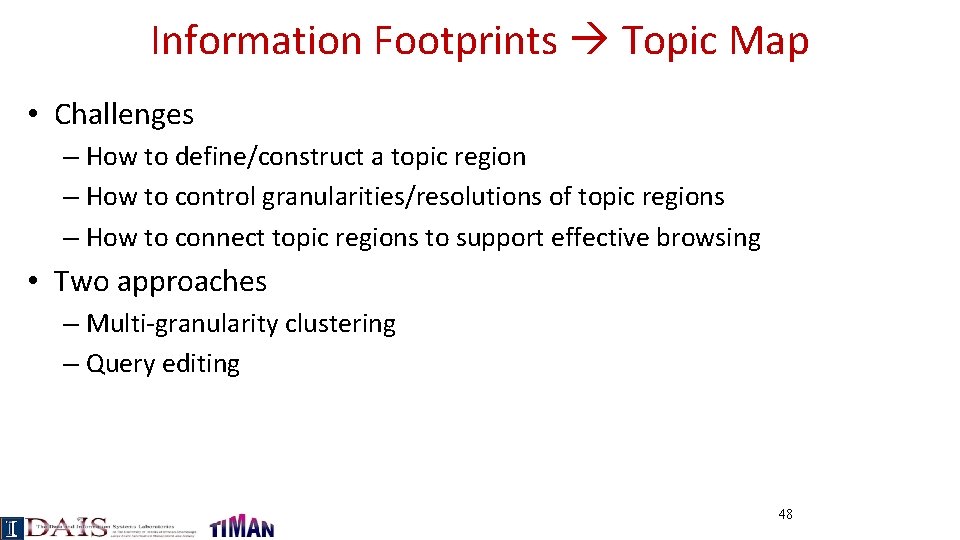
Information Footprints Topic Map • Challenges – How to define/construct a topic region – How to control granularities/resolutions of topic regions – How to connect topic regions to support effective browsing • Two approaches – Multi-granularity clustering – Query editing 48
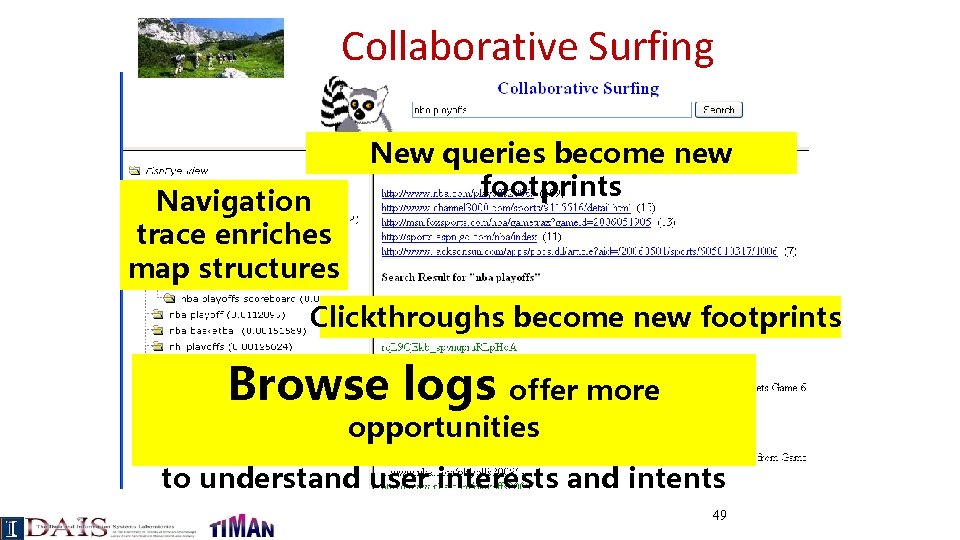
Collaborative Surfing Navigation trace enriches map structures New queries become new footprints Clickthroughs become new footprints Browse logs offer more opportunities to understand user interests and intents 49
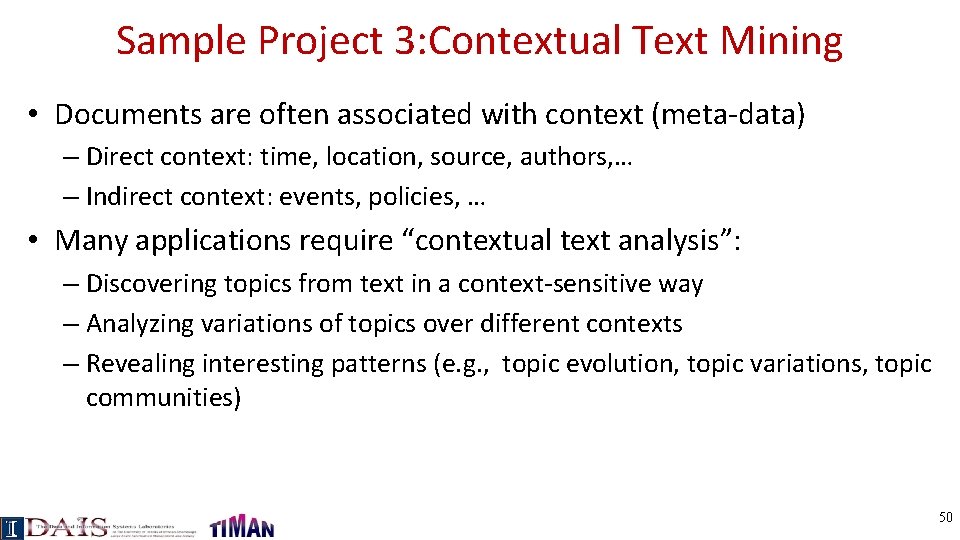
Sample Project 3: Contextual Text Mining • Documents are often associated with context (meta-data) – Direct context: time, location, source, authors, … – Indirect context: events, policies, … • Many applications require “contextual text analysis”: – Discovering topics from text in a context-sensitive way – Analyzing variations of topics over different contexts – Revealing interesting patterns (e. g. , topic evolution, topic variations, topic communities) 50
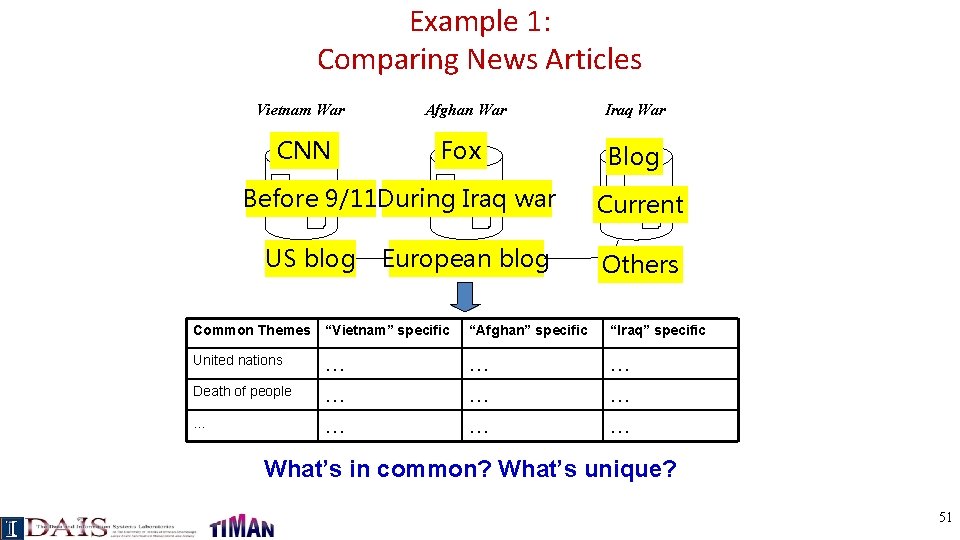
Example 1: Comparing News Articles Vietnam War CNN Afghan War Fox Before 9/11 During Iraq war US blog European blog Iraq War Blog Current Others Common Themes “Vietnam” specific “Afghan” specific “Iraq” specific United nations … … … Death of people … … … … What’s in common? What’s unique? 51
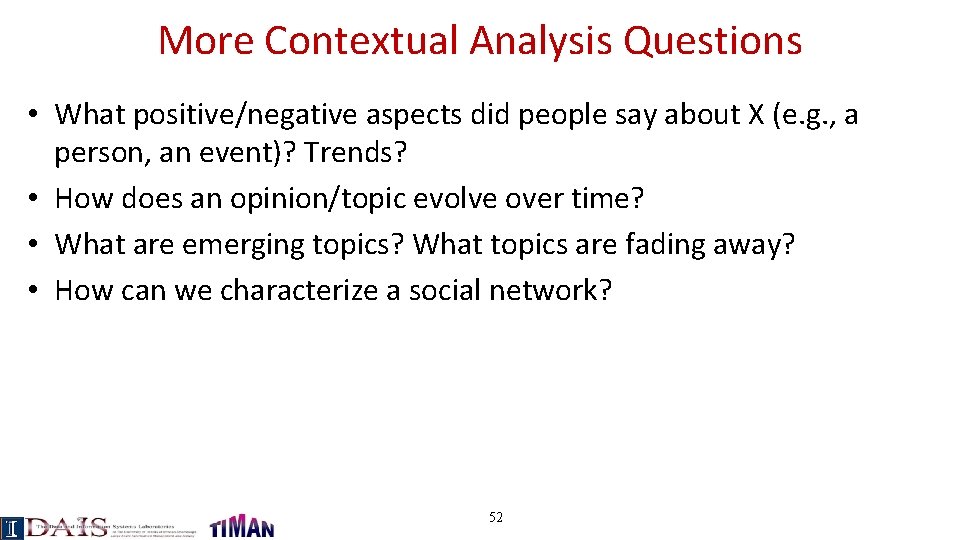
More Contextual Analysis Questions • What positive/negative aspects did people say about X (e. g. , a person, an event)? Trends? • How does an opinion/topic evolve over time? • What are emerging topics? What topics are fading away? • How can we characterize a social network? 52
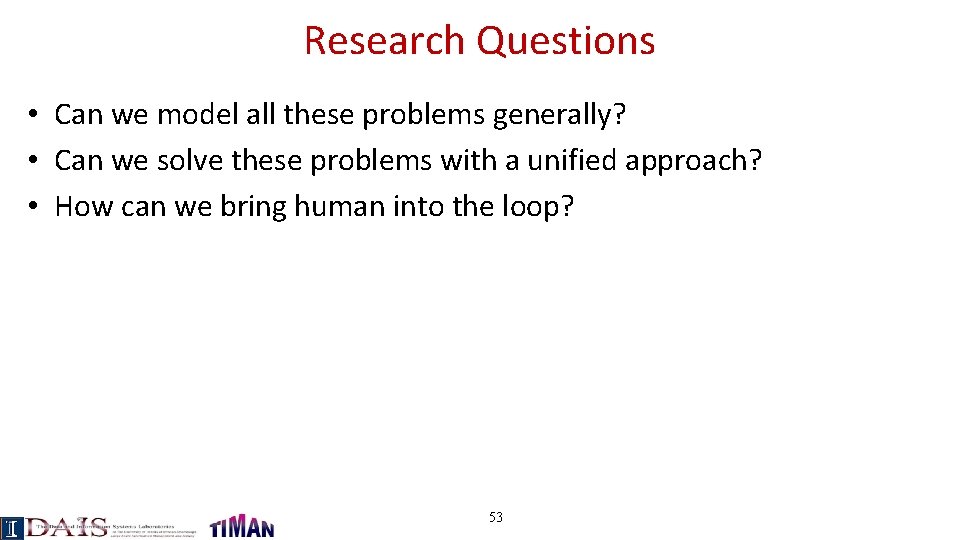
Research Questions • Can we model all these problems generally? • Can we solve these problems with a unified approach? • How can we bring human into the loop? 53
![Contextual Probabilistic Latent Semantics Analysis KDD 2006 Themes View 1 View 2 View 3 Contextual Probabilistic Latent Semantics Analysis ([KDD 2006]…) Themes View 1 View 2 View 3](https://slidetodoc.com/presentation_image_h/d5a0aa61765e85e4b4d6978a82a63873/image-54.jpg)
Contextual Probabilistic Latent Semantics Analysis ([KDD 2006]…) Themes View 1 View 2 View 3 government Choose a theme Criticism of government Draw a word from i response togovernment the hurricane government 0. 3 primarily consisted of response 0. 2. . Document response criticism of its response context: to … The total shut-in oil Time = from July the 2005 production Gulf Location = Texas of Mexico …donate approximately = xxx 24% of. Author the annual help aid production the shut. Occup. = and Sociologist in gas Over Ageproduction Group = … 45+ seventy countries pledged …Orleans monetary donations or new other assistance. … donate 0. 1 relief 0. 05 help 0. 02. . donation city 0. 2 new 0. 1 orleans 0. 05. . New Orleans Texas July 2005 sociolo gist Choose a view Theme coverages : …… Texas July 2005 document Choose a Coverage 54
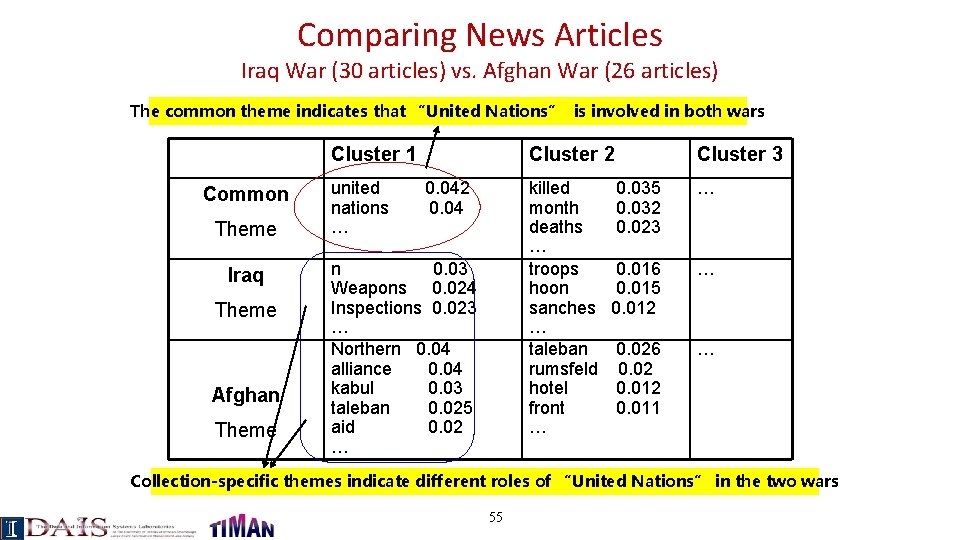
Comparing News Articles Iraq War (30 articles) vs. Afghan War (26 articles) The common theme indicates that “United Nations” is involved in both wars Cluster 1 Common Theme Iraq Theme Afghan Theme united nations … Cluster 2 0. 04 killed month deaths … troops hoon sanches … taleban rumsfeld hotel front … n 0. 03 Weapons 0. 024 Inspections 0. 023 … Northern 0. 04 alliance 0. 04 kabul 0. 03 taleban 0. 025 aid 0. 02 … Cluster 3 0. 035 0. 032 0. 023 … 0. 016 0. 015 0. 012 … 0. 026 0. 02 0. 011 … Collection-specific themes indicate different roles of “United Nations” in the two wars 55
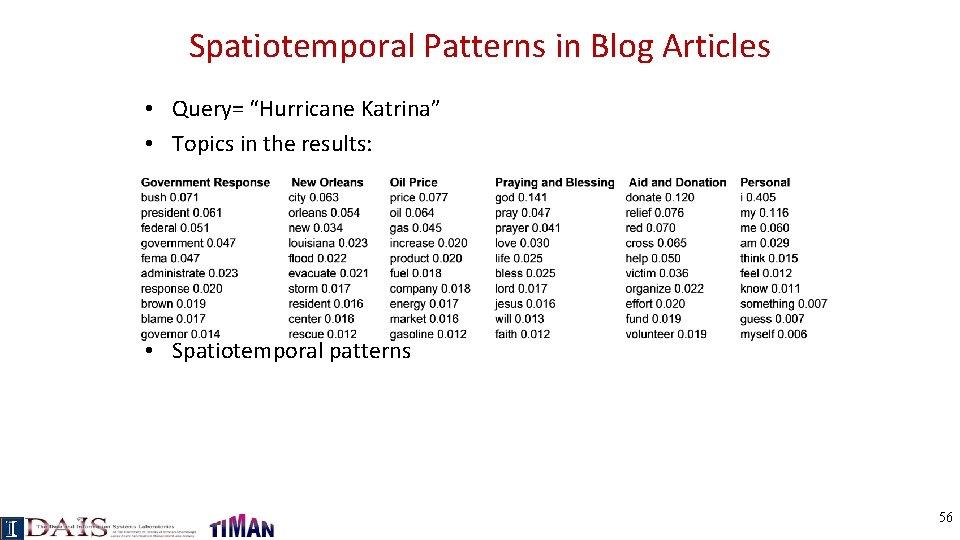
Spatiotemporal Patterns in Blog Articles • Query= “Hurricane Katrina” • Topics in the results: • Spatiotemporal patterns 56
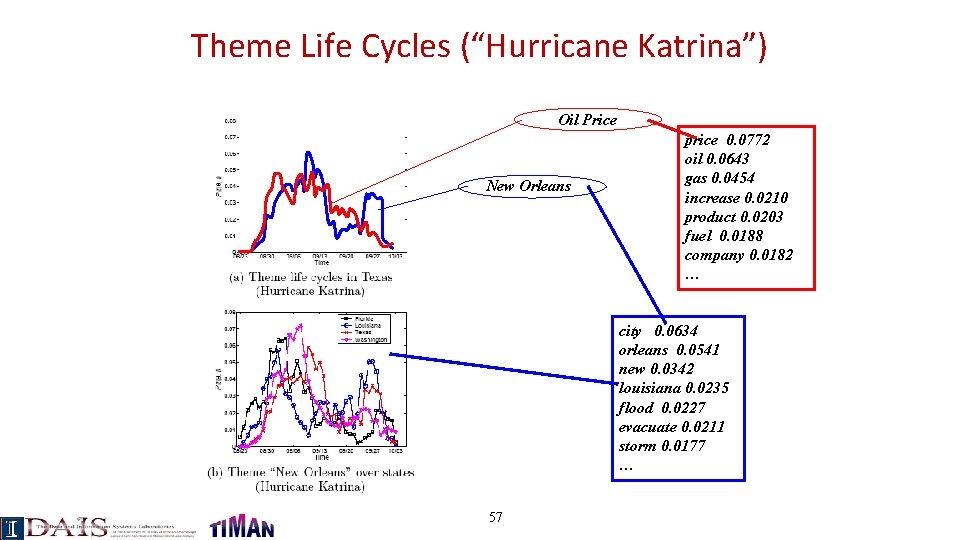
Theme Life Cycles (“Hurricane Katrina”) Oil Price New Orleans price 0. 0772 oil 0. 0643 gas 0. 0454 increase 0. 0210 product 0. 0203 fuel 0. 0188 company 0. 0182 … city 0. 0634 orleans 0. 0541 new 0. 0342 louisiana 0. 0235 flood 0. 0227 evacuate 0. 0211 storm 0. 0177 … 57
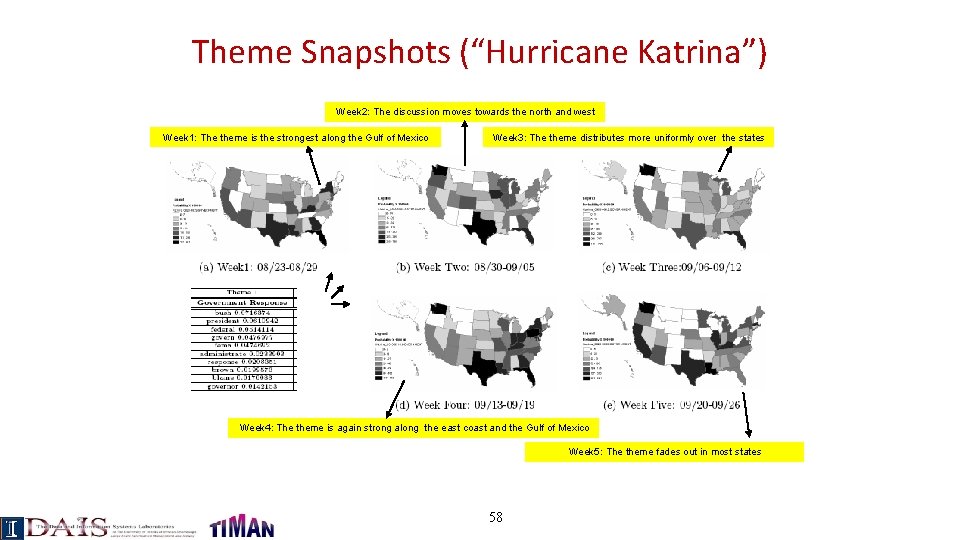
Theme Snapshots (“Hurricane Katrina”) Week 2: The discussion moves towards the north and west Week 1: The theme is the strongest along the Gulf of Mexico Week 3: The theme distributes more uniformly over the states Week 4: The theme is again strong along the east coast and the Gulf of Mexico Week 5: The theme fades out in most states 58
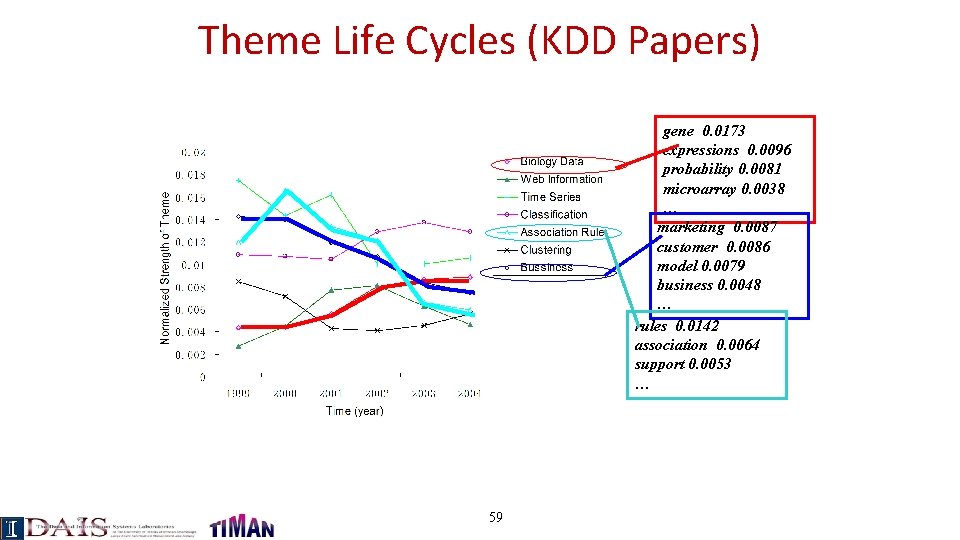
Theme Life Cycles (KDD Papers) gene 0. 0173 expressions 0. 0096 probability 0. 0081 microarray 0. 0038 … marketing 0. 0087 customer 0. 0086 model 0. 0079 business 0. 0048 … rules 0. 0142 association 0. 0064 support 0. 0053 … 59
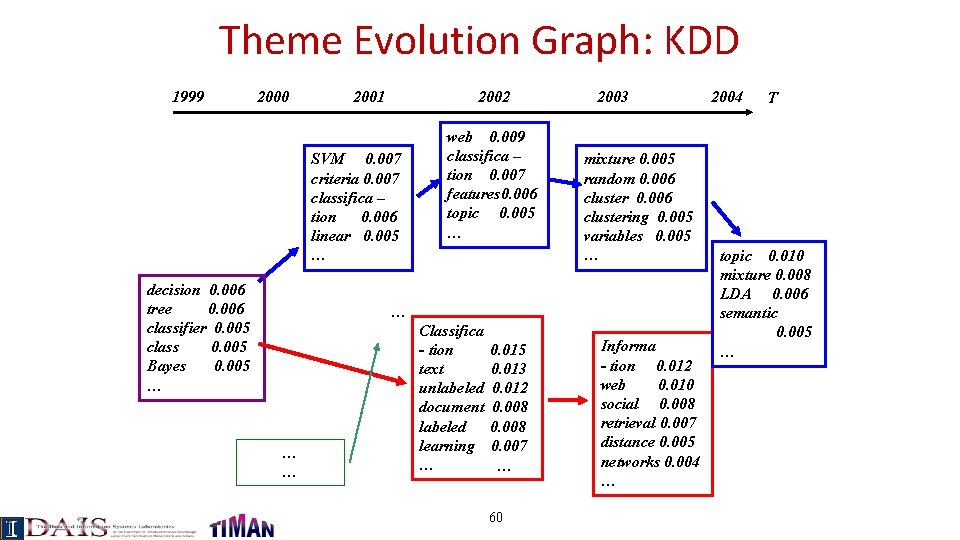
Theme Evolution Graph: KDD 1999 2000 2001 2002 SVM 0. 007 criteria 0. 007 classifica – tion 0. 006 linear 0. 005 … decision 0. 006 tree 0. 006 classifier 0. 005 class 0. 005 Bayes 0. 005 … web 0. 009 classifica – tion 0. 007 features 0. 006 topic 0. 005 … 2003 mixture 0. 005 random 0. 006 clustering 0. 005 variables 0. 005 … … Classifica - tion text unlabeled document labeled learning … 0. 015 0. 013 0. 012 0. 008 0. 007 … 60 Informa - tion 0. 012 web 0. 010 social 0. 008 retrieval 0. 007 distance 0. 005 networks 0. 004 … 2004 T topic 0. 010 mixture 0. 008 LDA 0. 006 semantic 0. 005 …
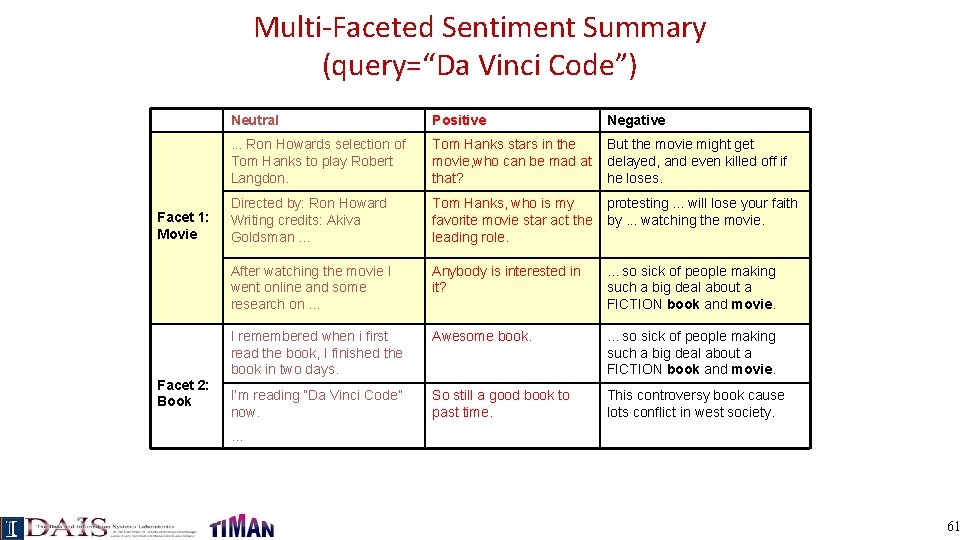
Multi-Faceted Sentiment Summary (query=“Da Vinci Code”) Facet 1: Movie Facet 2: Book Neutral Positive Negative . . . Ron Howards selection of Tom Hanks to play Robert Langdon. Tom Hanks stars in the movie, who can be mad at that? But the movie might get delayed, and even killed off if he loses. Directed by: Ron Howard Writing credits: Akiva Goldsman. . . Tom Hanks, who is my favorite movie star act the leading role. protesting. . . will lose your faith by. . . watching the movie. After watching the movie I went online and some research on. . . Anybody is interested in it? . . . so sick of people making such a big deal about a FICTION book and movie. I remembered when i first read the book, I finished the book in two days. Awesome book. . so sick of people making such a big deal about a FICTION book and movie. I’m reading “Da Vinci Code” now. So still a good book to past time. This controversy book cause lots conflict in west society. … 61
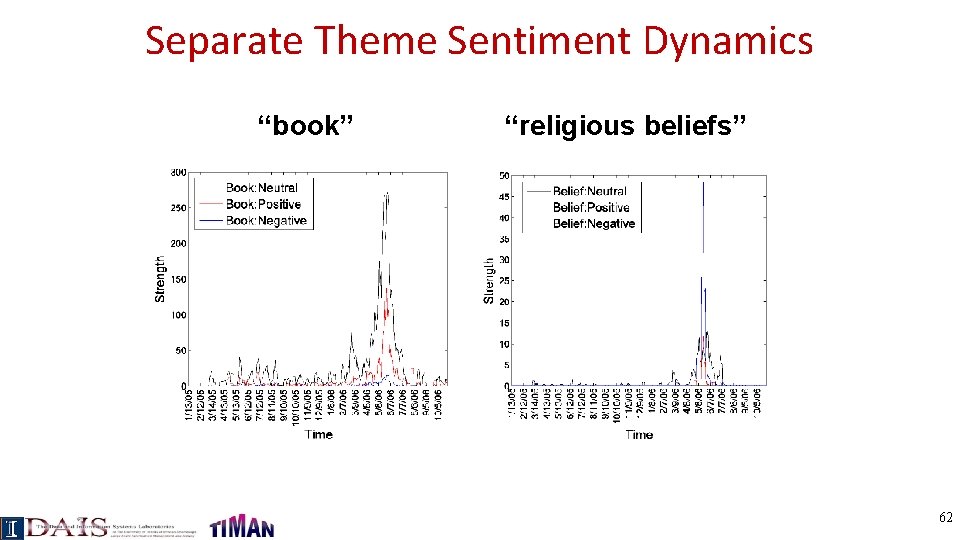
Separate Theme Sentiment Dynamics “book” “religious beliefs” 62
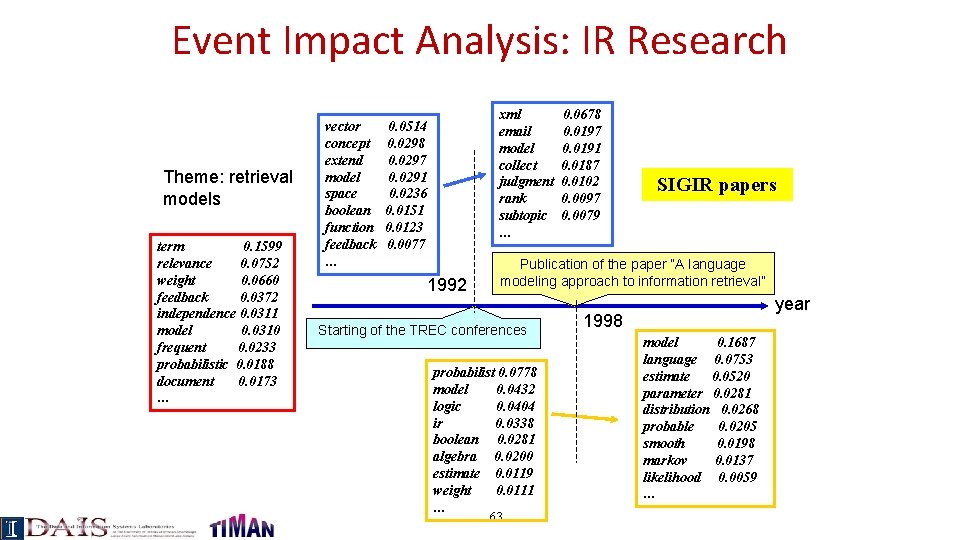
Event Impact Analysis: IR Research Theme: retrieval models term 0. 1599 relevance 0. 0752 weight 0. 0660 feedback 0. 0372 independence 0. 0311 model 0. 0310 frequent 0. 0233 probabilistic 0. 0188 document 0. 0173 … vector concept extend model space boolean function feedback … xml email model collect judgment rank subtopic … 0. 0514 0. 0298 0. 0297 0. 0291 0. 0236 0. 0151 0. 0123 0. 0077 1992 0. 0678 0. 0197 0. 0191 0. 0187 0. 0102 0. 0097 0. 0079 SIGIR papers Publication of the paper “A language modeling approach to information retrieval” Starting of the TREC conferences probabilist 0. 0778 model 0. 0432 logic 0. 0404 ir 0. 0338 boolean 0. 0281 algebra 0. 0200 estimate 0. 0119 weight 0. 0111 … 63 year 1998 model 0. 1687 language 0. 0753 estimate 0. 0520 parameter 0. 0281 distribution 0. 0268 probable 0. 0205 smooth 0. 0198 markov 0. 0137 likelihood 0. 0059 …
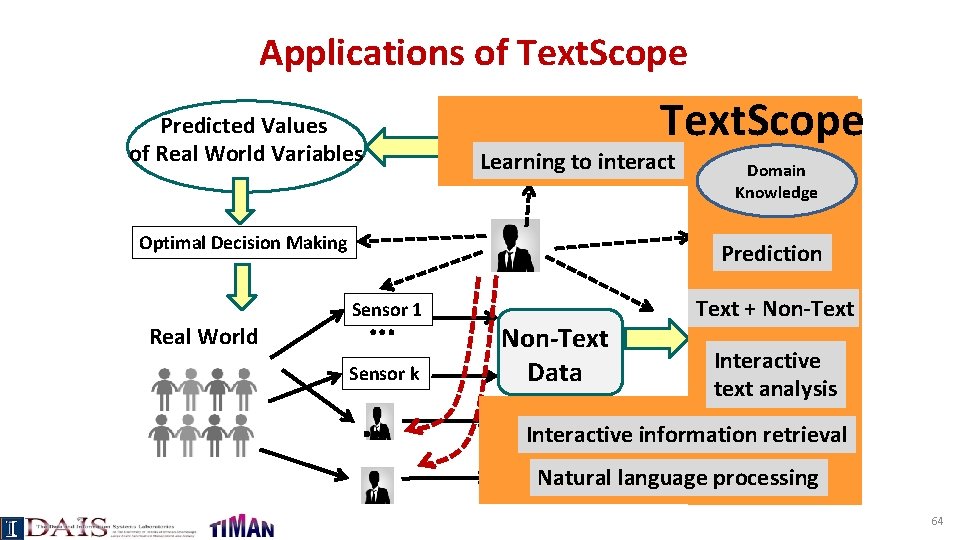
Applications of Text. Scope Multiple Text. Scope Predictors Predicted Values of Real World Variables Predictive Learning Model to interact Domain (Features) … Knowledge Optimal Decision Making Real World Prediction … Sensor 1 Sensor k … Non-Text Data Text + Non-Text Joint Mining of. Interactive Non-Text andanalysis Text text Text Interactive information retrieval Data Natural language processing 64
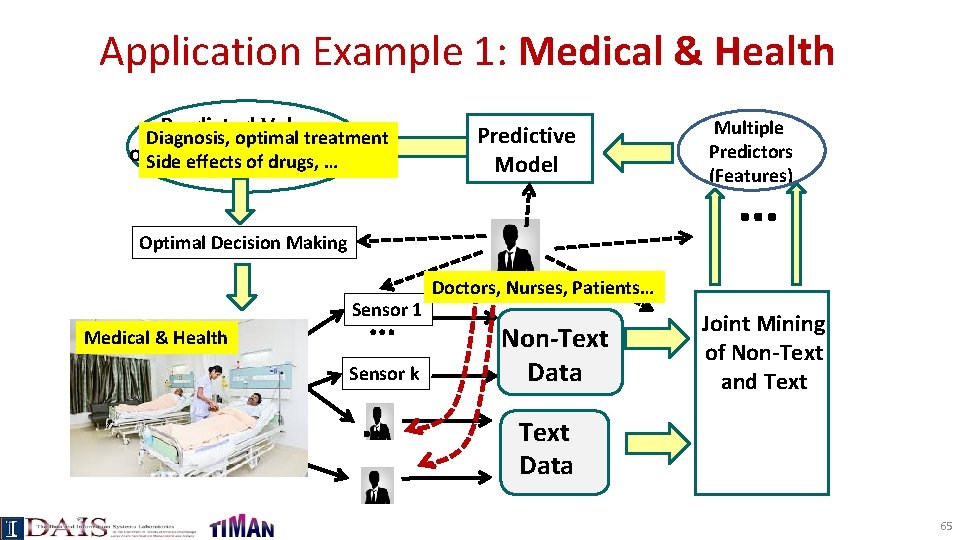
Application Example 1: Medical & Health Predicted Values Diagnosis, optimal treatment of. Side Real World Variables effects of drugs, … Predictive Model Optimal Decision Making Medical. Real & Health World … Sensor 1 Sensor k … Multiple Predictors (Features) … Doctors, Nurses, Patients… Non-Text Data Joint Mining of Non-Text and Text Data 65
![Discovery of Adverse Drug Reactions from Forums Wang et al 14 Green Disease symptoms Discovery of Adverse Drug Reactions from Forums [Wang et al. 14] Green: Disease symptoms](https://slidetodoc.com/presentation_image_h/d5a0aa61765e85e4b4d6978a82a63873/image-66.jpg)
Discovery of Adverse Drug Reactions from Forums [Wang et al. 14] Green: Disease symptoms Blue: Side effect symptoms Red: Drug Text. Scope Drug: Cefalexin ADR: panic attack faint …. Sheng Wang et al. 2014. Side. Effect. PTM: an unsupervised topic model to mine adverse drug reactions from health forums. In ACM BCB 2014. 66
![Sample ADRs Discovered Wang et al 14 DrugFreq Drug Use Symptoms in Descending Order Sample ADRs Discovered [Wang et al. 14] Drug(Freq) Drug Use Symptoms in Descending Order](https://slidetodoc.com/presentation_image_h/d5a0aa61765e85e4b4d6978a82a63873/image-67.jpg)
Sample ADRs Discovered [Wang et al. 14] Drug(Freq) Drug Use Symptoms in Descending Order Zoloft (84) antidepressant weigh gain, weight, depression, side effects, mgs, gain weight, anxiety, nausea, head, brain, pregnancy, pregnant, headaches, depressed, tired Ativan (33) anxiety disorders Ativan, sleep, Seroquel, doc prescribed seroqual, raising blood sugar levels, anti-psychotic drug, diabetic, constipation, diabetes, 10 mg, benzo, addicted Unreported to FDA Topamax (20) anticonvulsant Topmax, liver, side effects, migraines, headaches, weight, Topamax, pdoc, neurologist, supplement, sleep, fatigue, seizures, liver problems, kidney stones Ephedrine (2) stimulant dizziness, stomach, Benadryl, dizzy, tired, lethargic, tapering, tremors, panic attach, head Sheng Wang et al. 2014. Side. Effect. PTM: an unsupervised topic model to mine adverse drug reactions from health forums. In ACM BCB 2014. 67
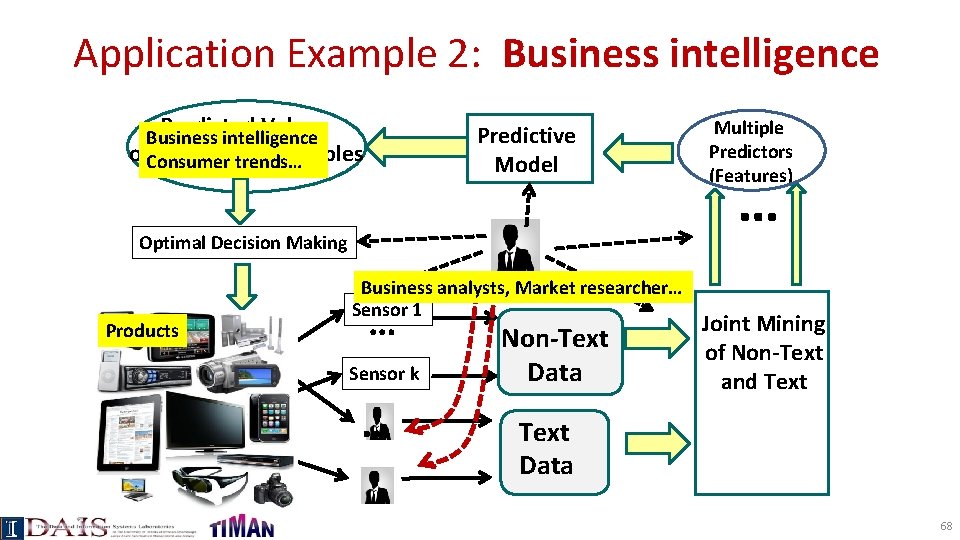
Application Example 2: Business intelligence Predicted Values Predictive Model Business intelligence of. Consumer Real World Variables trends… Optimal Decision Making Products Real World Business analysts, Market researcher… Sensor 1 … Sensor k … Non-Text Data Multiple Predictors (Features) … Joint Mining of Non-Text and Text Data 68
![Latent Aspect Rating Analysis LARA Wang et al 10 Text Scope How to infer Latent Aspect Rating Analysis (LARA) [Wang et al. 10] Text. Scope How to infer](https://slidetodoc.com/presentation_image_h/d5a0aa61765e85e4b4d6978a82a63873/image-69.jpg)
Latent Aspect Rating Analysis (LARA) [Wang et al. 10] Text. Scope How to infer aspect ratings? How to infer aspect weights? Value Location Service … Hongning Wang, Yue Lu, Cheng. Xiang Zhai. Latent Aspect Rating Analysis on Review Text Data: A Rating Regression Approach, Proceedings of the 17 th ACM SIGKDD International Conference on Knowledge Discovery and Data Mining (KDD'10), pages 115 -124, 2010. 69
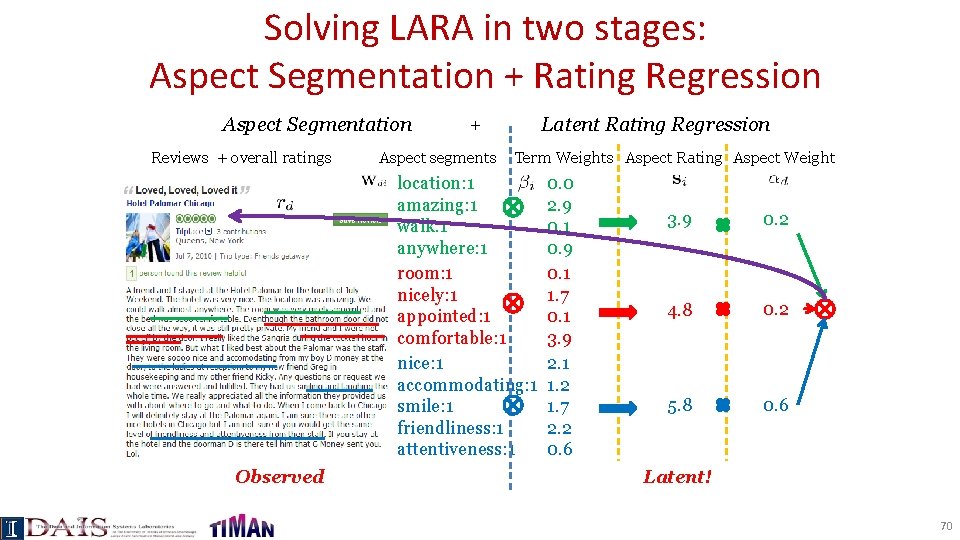
Solving LARA in two stages: Aspect Segmentation + Rating Regression Aspect Segmentation Reviews + overall ratings + Aspect segments Latent Rating Regression Term Weights Aspect Rating Aspect Weight location: 1 amazing: 1 walk: 1 anywhere: 1 room: 1 nicely: 1 appointed: 1 comfortable: 1 nice: 1 accommodating: 1 smile: 1 friendliness: 1 attentiveness: 1 Observed 0. 0 2. 9 0. 1 0. 9 0. 1 1. 7 0. 1 3. 9 2. 1 1. 2 1. 7 2. 2 0. 6 3. 9 0. 2 4. 8 0. 2 5. 8 0. 6 Latent! 70
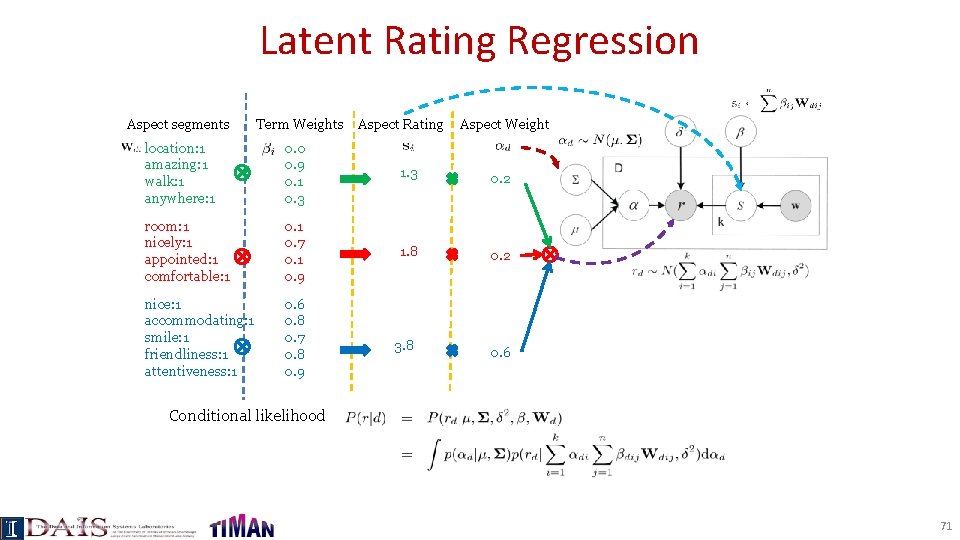
Latent Rating Regression Aspect segments Term Weights Aspect Rating Aspect Weight location: 1 amazing: 1 walk: 1 anywhere: 1 0. 0 0. 9 0. 1 0. 3 1. 3 0. 2 room: 1 nicely: 1 appointed: 1 comfortable: 1 0. 7 0. 1 0. 9 1. 8 0. 2 nice: 1 accommodating: 1 smile: 1 friendliness: 1 attentiveness: 1 0. 6 0. 8 0. 7 0. 8 0. 9 3. 8 0. 6 Conditional likelihood 71
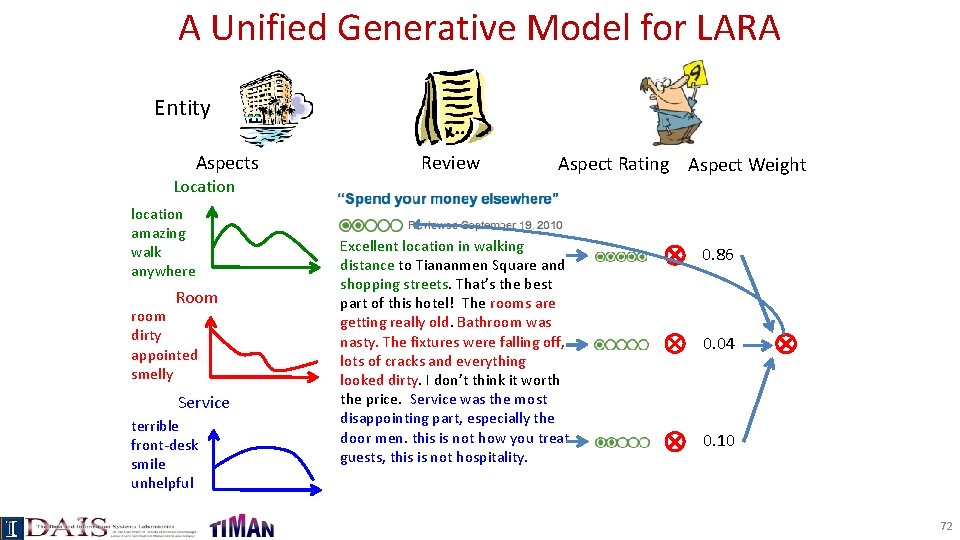
A Unified Generative Model for LARA Entity Aspects Location location amazing walk anywhere Room room dirty appointed smelly Service terrible front-desk smile unhelpful Review Aspect Rating Aspect Weight Excellent location in walking distance to Tiananmen Square and shopping streets. That’s the best part of this hotel! The rooms are getting really old. Bathroom was nasty. The fixtures were falling off, lots of cracks and everything looked dirty. I don’t think it worth the price. Service was the most disappointing part, especially the door men. this is not how you treat guests, this is not hospitality. 0. 86 0. 04 0. 10 72
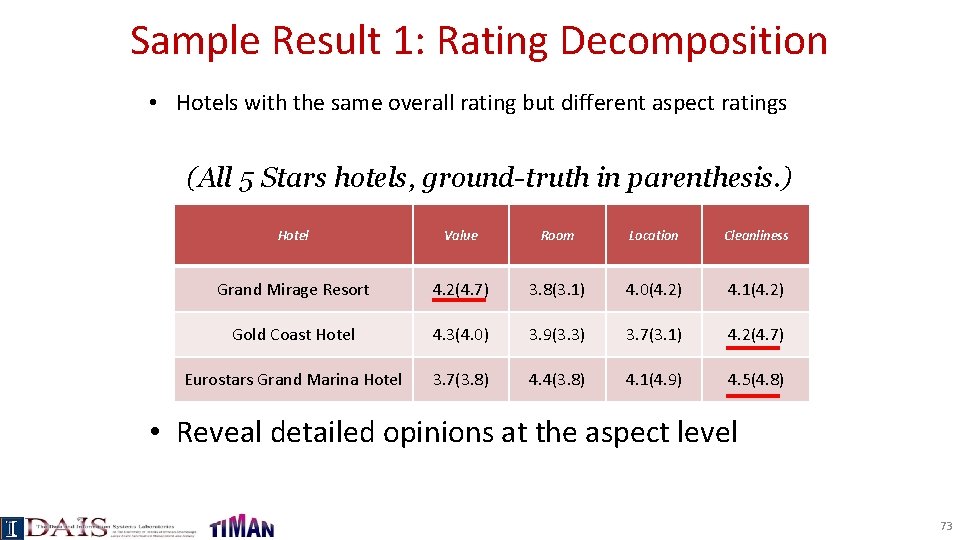
Sample Result 1: Rating Decomposition • Hotels with the same overall rating but different aspect ratings (All 5 Stars hotels, ground-truth in parenthesis. ) Hotel Value Room Location Cleanliness Grand Mirage Resort 4. 2(4. 7) 3. 8(3. 1) 4. 0(4. 2) 4. 1(4. 2) Gold Coast Hotel 4. 3(4. 0) 3. 9(3. 3) 3. 7(3. 1) 4. 2(4. 7) Eurostars Grand Marina Hotel 3. 7(3. 8) 4. 4(3. 8) 4. 1(4. 9) 4. 5(4. 8) • Reveal detailed opinions at the aspect level 73
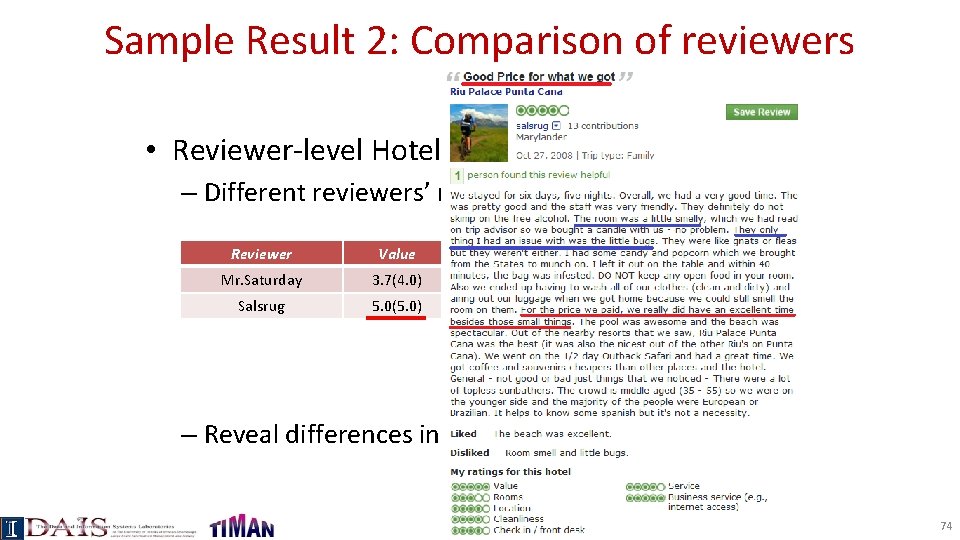
Sample Result 2: Comparison of reviewers • Reviewer-level Hotel Analysis – Different reviewers’ ratings on the same hotel Reviewer Value Room Location Cleanliness Mr. Saturday 3. 7(4. 0) 3. 5(4. 0) 3. 7(4. 0) 5. 8(5. 0) Salsrug 5. 0(5. 0) 3. 0(3. 0) 5. 0(4. 0) 3. 5(4. 0) (Hotel Riu Palace Punta Cana) – Reveal differences in opinions of different reviewers 74
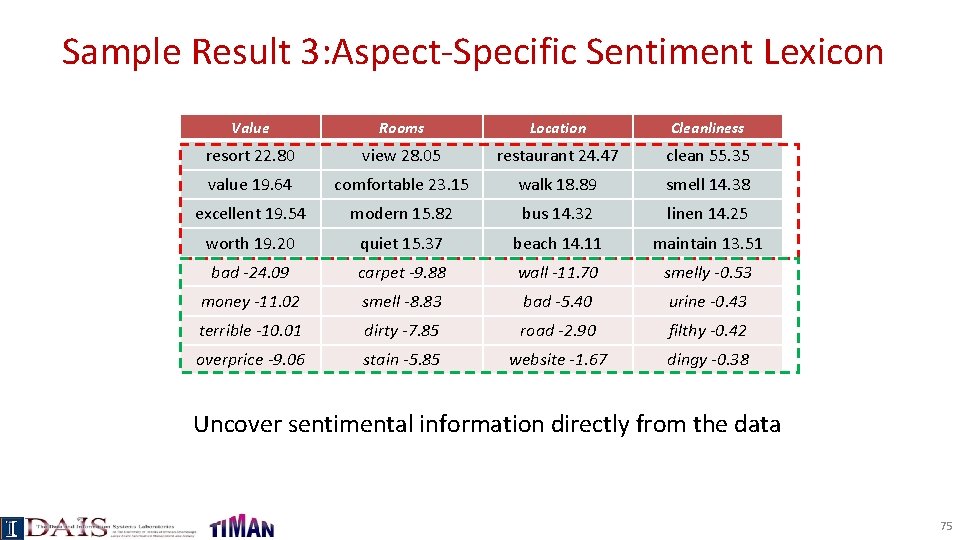
Sample Result 3: Aspect-Specific Sentiment Lexicon Value Rooms Location Cleanliness resort 22. 80 view 28. 05 restaurant 24. 47 clean 55. 35 value 19. 64 comfortable 23. 15 walk 18. 89 smell 14. 38 excellent 19. 54 modern 15. 82 bus 14. 32 linen 14. 25 worth 19. 20 quiet 15. 37 beach 14. 11 maintain 13. 51 bad -24. 09 carpet -9. 88 wall -11. 70 smelly -0. 53 money -11. 02 smell -8. 83 bad -5. 40 urine -0. 43 terrible -10. 01 dirty -7. 85 road -2. 90 filthy -0. 42 overprice -9. 06 stain -5. 85 website -1. 67 dingy -0. 38 Uncover sentimental information directly from the data 75
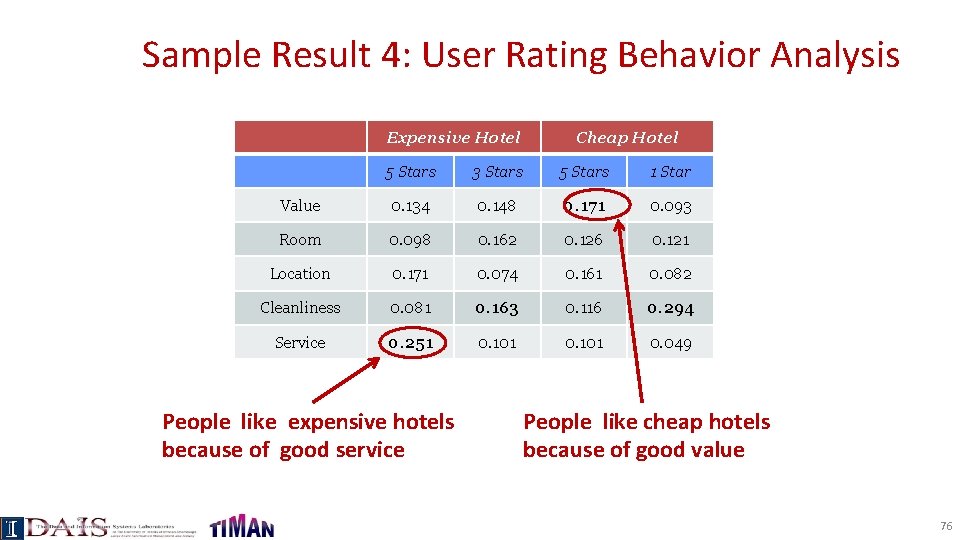
Sample Result 4: User Rating Behavior Analysis Expensive Hotel Cheap Hotel 5 Stars 3 Stars 5 Stars 1 Star Value 0. 134 0. 148 0. 171 0. 093 Room 0. 098 0. 162 0. 126 0. 121 Location 0. 171 0. 074 0. 161 0. 082 Cleanliness 0. 081 0. 163 0. 116 0. 294 Service 0. 251 0. 101 0. 049 People like expensive hotels because of good service People like cheap hotels because of good value 76
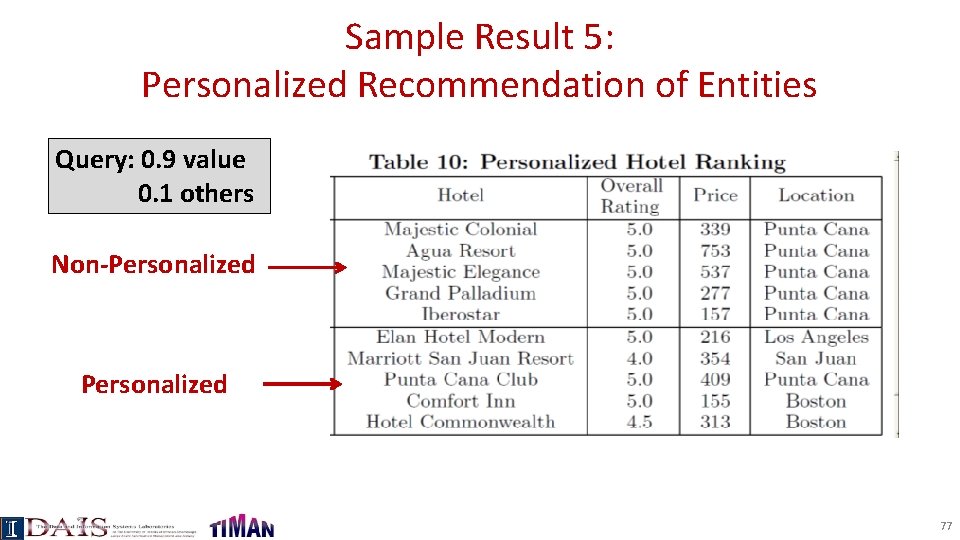
Sample Result 5: Personalized Recommendation of Entities Query: 0. 9 value 0. 1 others Non-Personalized 77
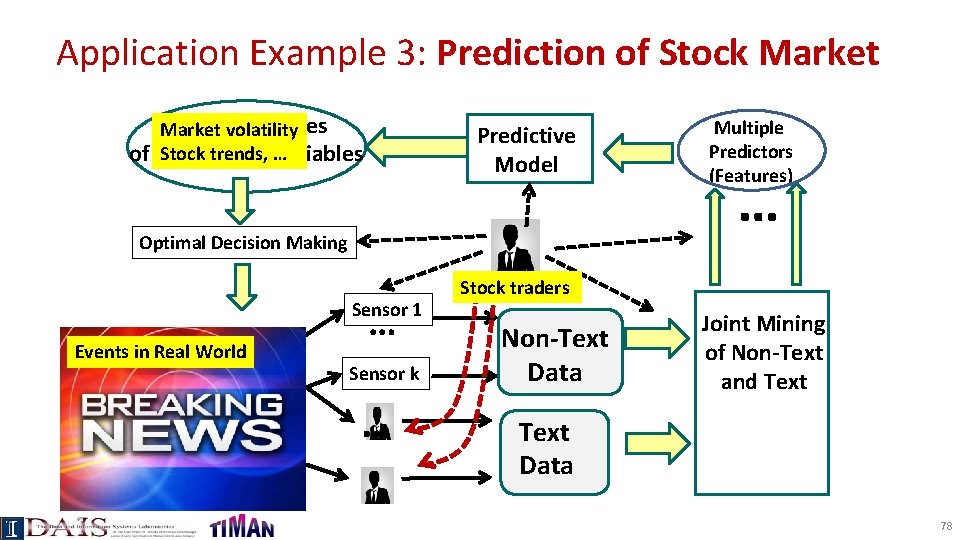
Application Example 3: Prediction of Stock Market Predicted Values Market volatility Stock. World trends, Variables … of Real Predictive Model Optimal Decision Making Real World Events in Real World … Sensor 1 Sensor k … Multiple Predictors (Features) … Stock traders Non-Text Data Joint Mining of Non-Text and Text Data 78
![Text Mining for Understanding Time Series Kim et al CIKM 13 What might Text Mining for Understanding Time Series [Kim et al. CIKM’ 13] What might](https://slidetodoc.com/presentation_image_h/d5a0aa61765e85e4b4d6978a82a63873/image-79.jpg)
Text Mining for Understanding Time Series [Kim et al. CIKM’ 13] What might have caused the stock market crash? Sept 11 attack! Text. Scope Dow Jones Industrial Average [Source: Yahoo Finance] … Time Any clues in the companion news stream? H. Kim, M. Castellanos, M. Hsu, C. Zhai, T. A. Rietz, D. Diermeier. Mining causal topics in text data: iterative topic modeling with time series feedback, Proceedings of ACM CIKM 2013, pp. 885 -890, 2013. 79
![A General Framework for Causal Topic Modeling Kim et al CIKM 13 Text Stream A General Framework for Causal Topic Modeling [Kim et al. CIKM’ 13] Text Stream](https://slidetodoc.com/presentation_image_h/d5a0aa61765e85e4b4d6978a82a63873/image-80.jpg)
A General Framework for Causal Topic Modeling [Kim et al. CIKM’ 13] Text Stream Sep 2001 Oct … 2001 Topic Modeling Causal Topics Topic 1 Topic 2 Topic 3 Topic 4 Non-text Time Series Feedback as Prior Split Words Topic 1 -1 W 3 + + Topic 1 -2 W 4 --- Zoom into Word Level Topic 1 W 2 W 3 W 4 W 5 … + -+ -- Causal Words H. Kim, M. Castellanos, M. Hsu, C. Zhai, T. A. Rietz, D. Diermeier. Mining causal topics in text data: iterative topic modeling with time series feedback, Proceedings of ACM CIKM 2013, pp. 885 -890, 2013. 80
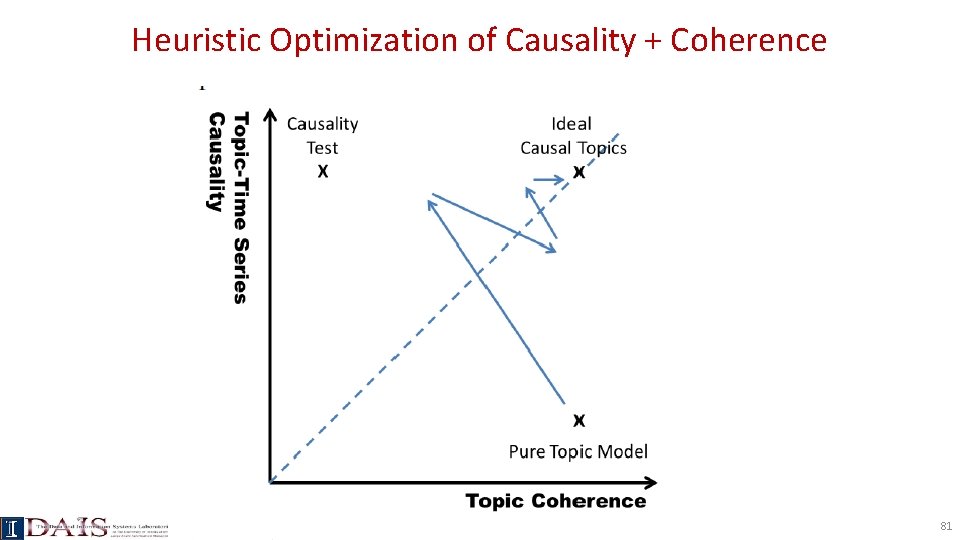
Heuristic Optimization of Causality + Coherence 81
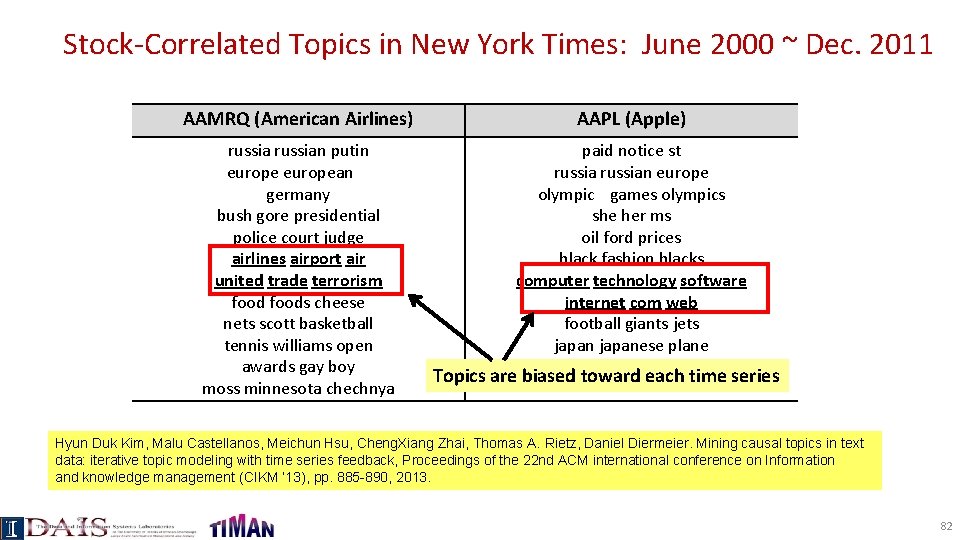
Stock-Correlated Topics in New York Times: June 2000 ~ Dec. 2011 AAMRQ (American Airlines) AAPL (Apple) russian putin european germany bush gore presidential police court judge airlines airport air united trade terrorism foods cheese nets scott basketball tennis williams open awards gay boy moss minnesota chechnya paid notice st russian europe olympic games olympics she her ms oil ford prices black fashion blacks computer technology software internet com web football giants jets japanese plane … Topics are biased toward each time series Hyun Duk Kim, Malu Castellanos, Meichun Hsu, Cheng. Xiang Zhai, Thomas A. Rietz, Daniel Diermeier. Mining causal topics in text data: iterative topic modeling with time series feedback, Proceedings of the 22 nd ACM international conference on Information and knowledge management (CIKM ’ 13), pp. 885 -890, 2013. 82
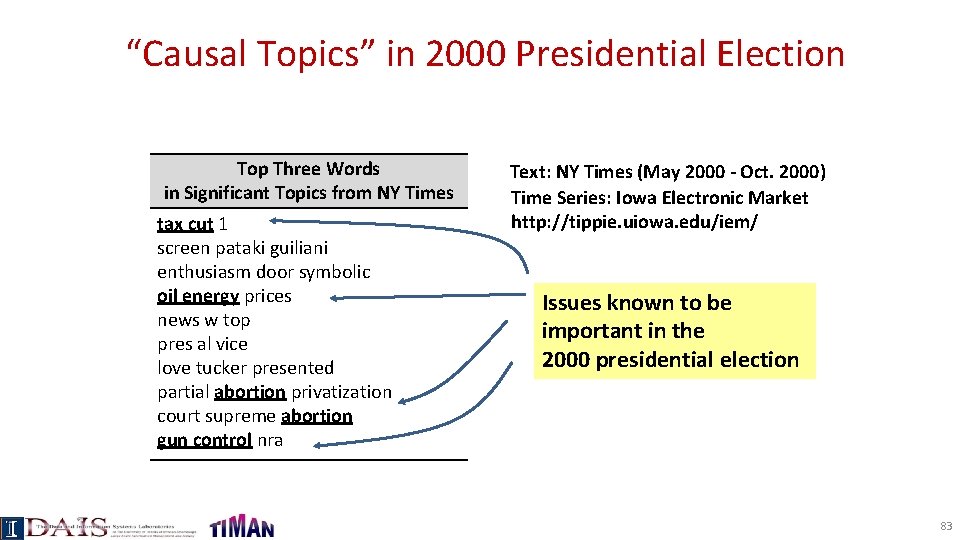
“Causal Topics” in 2000 Presidential Election Top Three Words in Significant Topics from NY Times tax cut 1 screen pataki guiliani enthusiasm door symbolic oil energy prices news w top pres al vice love tucker presented partial abortion privatization court supreme abortion gun control nra Text: NY Times (May 2000 - Oct. 2000) Time Series: Iowa Electronic Market http: //tippie. uiowa. edu/iem/ Issues known to be important in the 2000 presidential election 83
![Retrieval with Time Series Query Kim et al ICTIR 13 News 70 60 50 Retrieval with Time Series Query [Kim et al. ICTIR’ 13] News 70 60 50](https://slidetodoc.com/presentation_image_h/d5a0aa61765e85e4b4d6978a82a63873/image-84.jpg)
Retrieval with Time Series Query [Kim et al. ICTIR’ 13] News 70 60 50 40 30 20 10 0 2001 … 12/3/2001 11/3/2001 9/3/2001 8/3/2001 7/3/2001 6/3/2001 10/3/2001 Date 5/3/2001 4/3/2001 3/3/2001 2/3/2001 12/3/2000 11/3/2000 10/3/2000 9/3/2000 8/3/2000 7/3/2000 Price ($) Apple Stock Price RANK DATE EXCERPT 1 9/29/2000 Expect earning will be far below 2 12/8/2000 $4 billion cash in company 3 10/19/2000 Disappointing earning report 4 4/19/2001 Dow and Nasdaq soar after rate cut by Federal Reserve 5 7/20/2001 Apple's new retail store … … … Hyun Duk Kim, Danila Nikitin, Cheng. Xiang Zhai, Malu Castellanos, and Meichun Hsu. 2013. Information Retrieval with Time Series Query. In Proceedings of the 2013 Conference on the Theory of Information Retrieval (ICTIR '13), 84
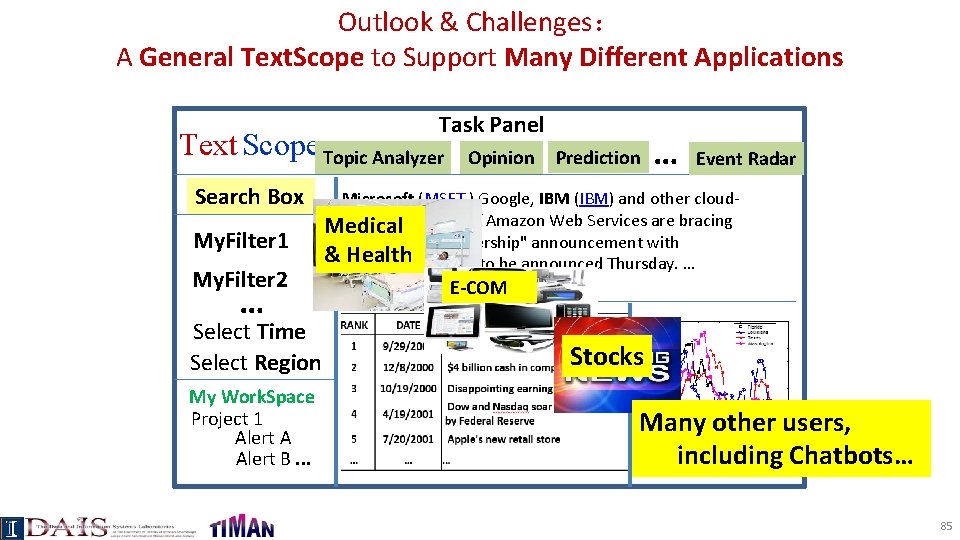
Outlook & Challenges: A General Text. Scope to Support Many Different Applications Task Panel Text Scope Topic Analyzer Search Box My. Filter 1 My. Filter 2 … Select Time Select Region My Work. Space Project 1 Alert A Alert B. . . Opinion Prediction … Event Radar Microsoft (MSFT, ) Google, IBM (IBM) and other cloudcomputing rivals of Amazon Web Services are bracing Medical for an AWS "partnership" announcement with &VMware expected to be announced Thursday. … Health E-COM Stocks Many other users, including Chatbots… 85
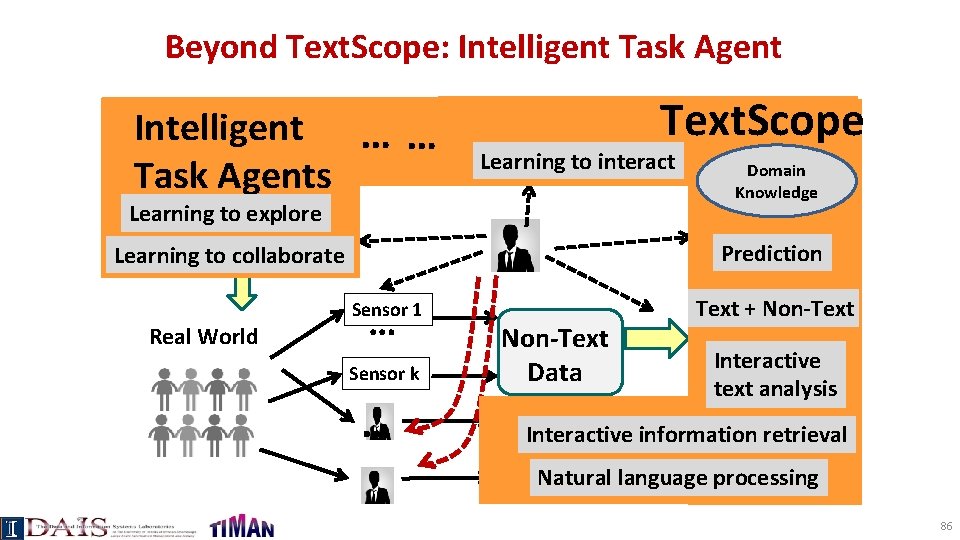
Beyond Text. Scope: Intelligent Task Agent Predicted Values Intelligent. . . …… of Real World Variables Task Agents Multiple Text. Scope Predictors Predictive Learning Model to interact … Knowledge Learning to explore Optimal Decision Making Prediction Learning to collaborate Real World Domain (Features) … Sensor 1 Sensor k … Non-Text Data Text + Non-Text Joint Mining of. Interactive Non-Text andanalysis Text text Text Interactive information retrieval Data Natural language processing 86
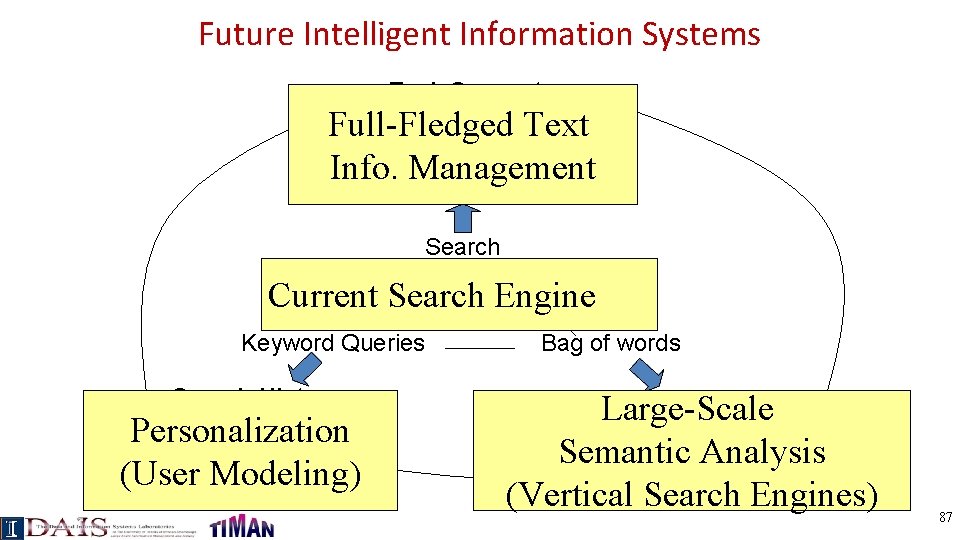
Future Intelligent Information Systems Task Support Full-Fledged Mining Text Info. Management Access Search Current Search Engine Keyword Queries Search History Personalization Complete User Model (User Modeling) Bag of words Entities-Relations Large-Scale Semantic Analysis Knowledge Representation (Vertical Search Engines) 87
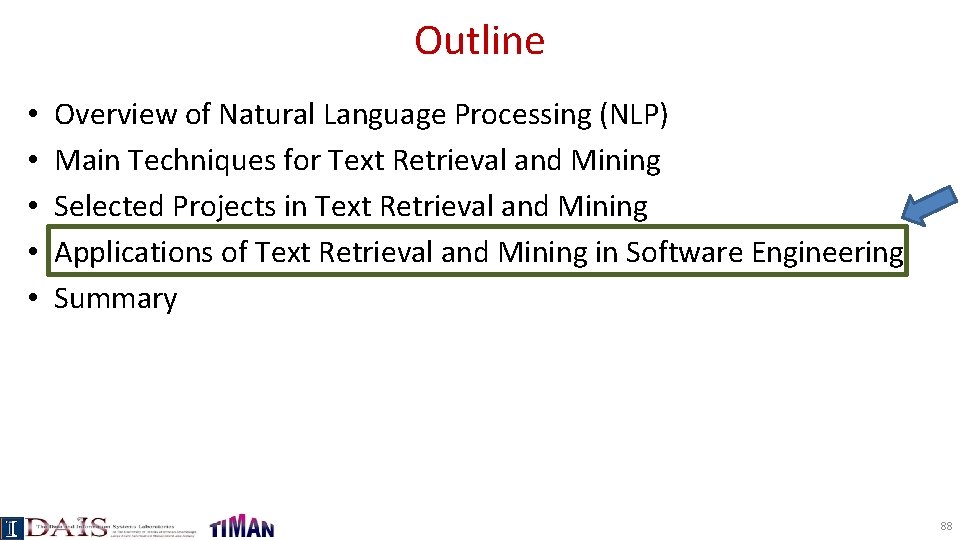
Outline • • • Overview of Natural Language Processing (NLP) Main Techniques for Text Retrieval and Mining Selected Projects in Text Retrieval and Mining Applications of Text Retrieval and Mining in Software Engineering Summary 88
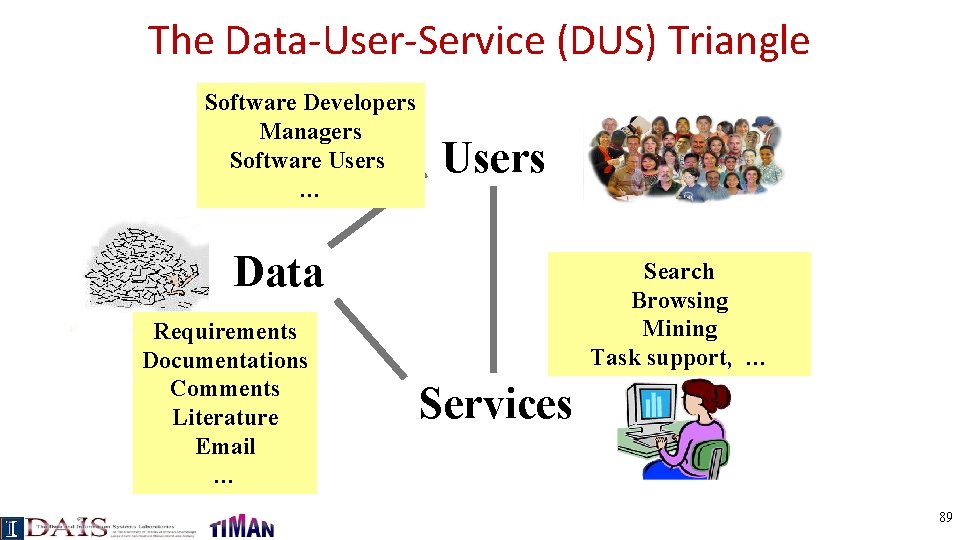
The Data-User-Service (DUS) Triangle Software Developers Managers Software Users … Users Data Requirements Documentations Comments Literature Email … Search Browsing Mining Task support, … Services 89
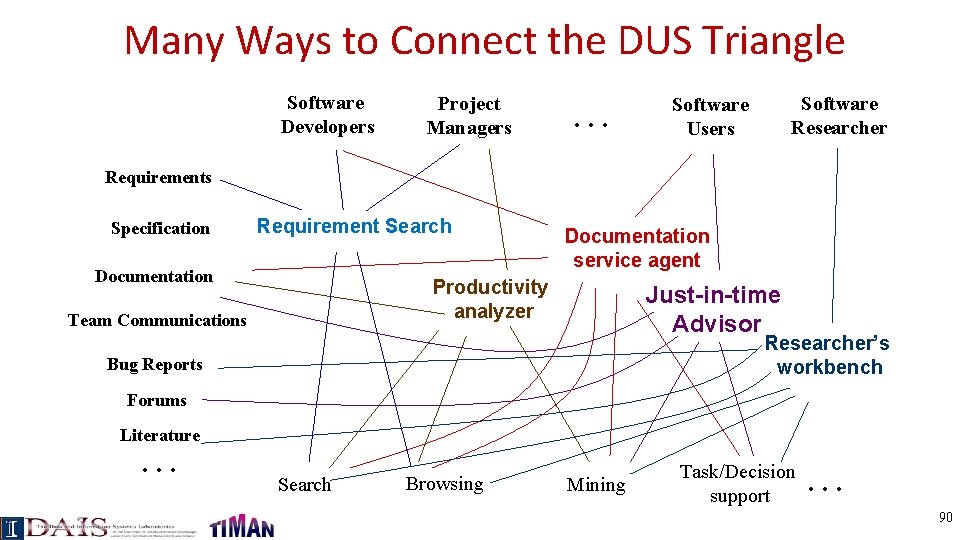
Many Ways to Connect the DUS Triangle Software Developers Project Managers … Software Researcher Software Users Requirements Specification Requirement Search Documentation service agent Productivity analyzer Team Communications Just-in-time Advisor Researcher’s workbench Bug Reports Forums Literature … Search Browsing Mining Task/Decision support … 90
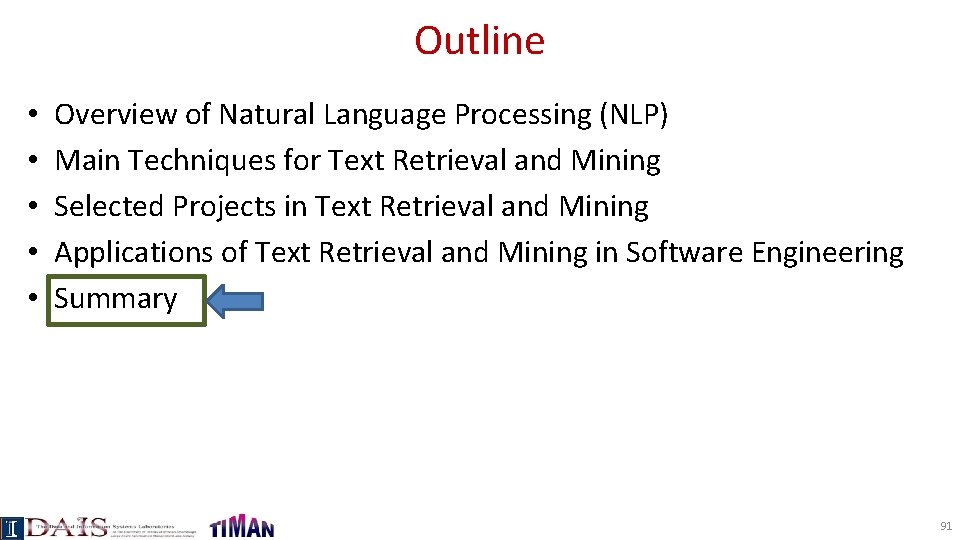
Outline • • • Overview of Natural Language Processing (NLP) Main Techniques for Text Retrieval and Mining Selected Projects in Text Retrieval and Mining Applications of Text Retrieval and Mining in Software Engineering Summary 91
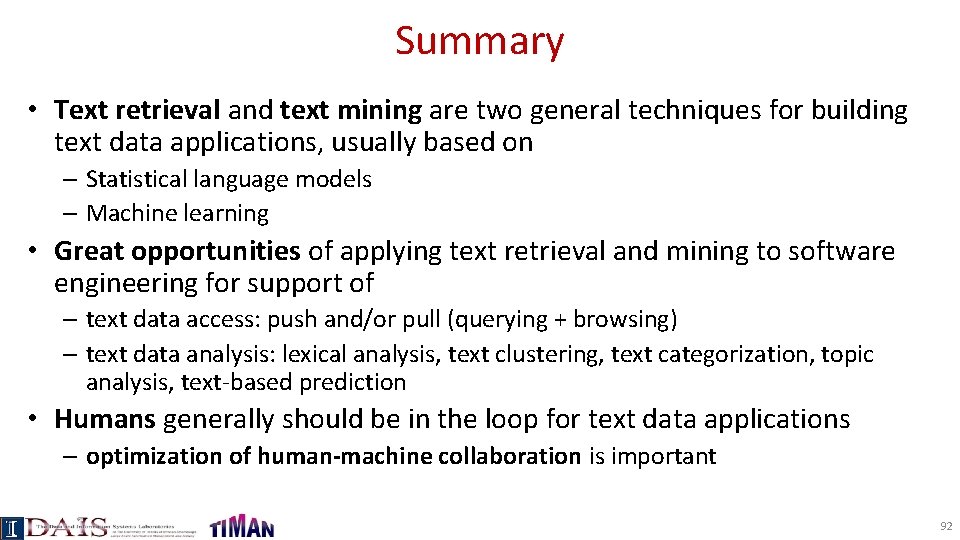
Summary • Text retrieval and text mining are two general techniques for building text data applications, usually based on – Statistical language models – Machine learning • Great opportunities of applying text retrieval and mining to software engineering for support of – text data access: push and/or pull (querying + browsing) – text data analysis: lexical analysis, text clustering, text categorization, topic analysis, text-based prediction • Humans generally should be in the loop for text data applications – optimization of human-machine collaboration is important 92
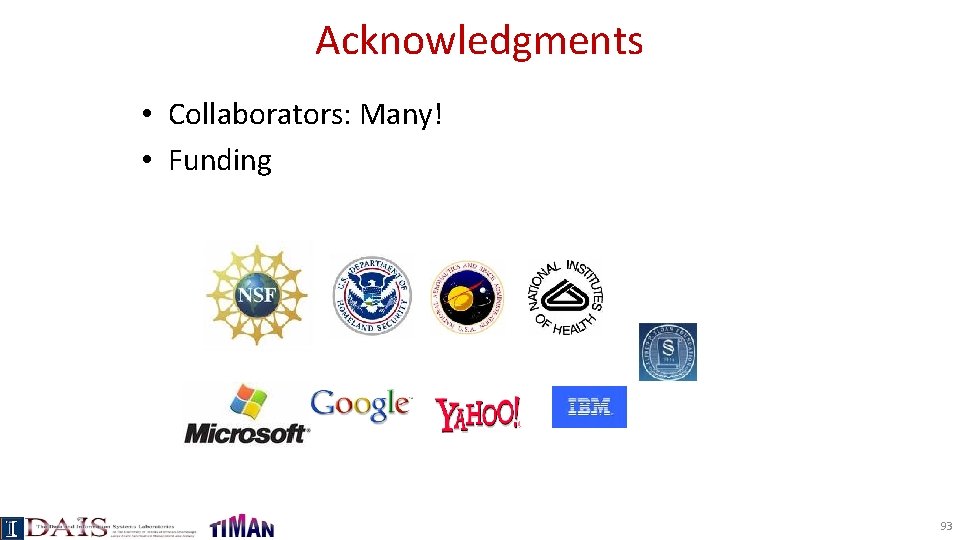
Acknowledgments • Collaborators: Many! • Funding 93
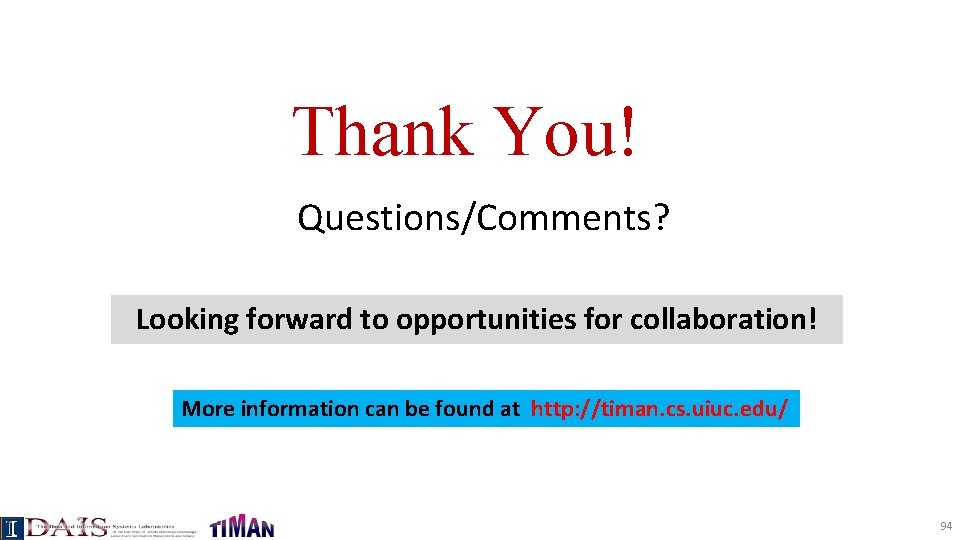
Thank You! Questions/Comments? Looking forward to opportunities for collaboration! More information can be found at http: //timan. cs. uiuc. edu/ 94
E e e poem
Cheng xiang zhai
Cheng xiang zhai
Cheng xiang zhai
Cheng xiang zhai
Cheng xiang zhai
Routing table
Cheng xiang zhai
Morrp
Difference between text mining and web mining
Text retrieval software
Text-to-media connection
Text analytics and text mining
Text analytics and text mining
Difference between strip mining and open pit mining
The planners by boey kim cheng
Strip mining vs open pit mining
Chapter 13 mineral resources and mining
Mining multimedia databases
Mining complex types of data
Software measurement and metrics
Vex xiang
Liu xiang weightlifter
Liu xiang
Xiang yu liu bang
Its rosewhatt
Xiang yang liu
Miscosoft forms
Record producer job description
Xiang jiao ping guo
Perfume xia xiang
Vex xiang
Yongqing xiang
Modern information retrieval
Text based image retrieval
Computer based system engineering in software engineering
Forward engineering in software engineering
W62vr
Catbeller
Software maintenance in software engineering ppt
Frank maurer
Metrics computer science
Types of software crisis
Real time software design in software engineering
Design principles in software engineering
Cheng field and wave electromagnetics
Svd text mining
Piotr gawrysiak
Text mining
Text mining
Txttool stata
Logiciel de text mining
Text mining social media
Text mining
Text mining application programming
Mining estimating software
Disappeared boey kim cheng
Bier block
Peter chen diagram
Antony cheng
King cheng
Avery cheng
Cheng
Ck cheng ucsd
Wei cheng lee
Mktg 303
Cervical radiculopathy differential diagnosis
Yizong cheng
Cheng ruize
Lou cheng
Boey kim cheng
Kung fu panda tai chi
Chung-kuan cheng
Chia liang cheng
Sophia cheng accident
Cheng-few lee
Kiddonet games
Judy cheng hopkins
Luke daemen
Ismael herrera
Cộc cách tùng cheng
Pauline cheng
Wayne cheng loma linda
Sega cheng
Precision recall information retrieval
Storage and retrieval technologies in multimedia
Memory encoding techniques
Long term storage and retrieval
Memory encoding
Information retrieval and web search
Information retrieval data structures and algorithms
Westlaw boolean
Examples of information retrieval tools
Signature file structure in information retrieval system
Defect amplification and removal in software engineering