RANDOMISATION TECHNIQUES A P Dr Hayati Kadir Shahar
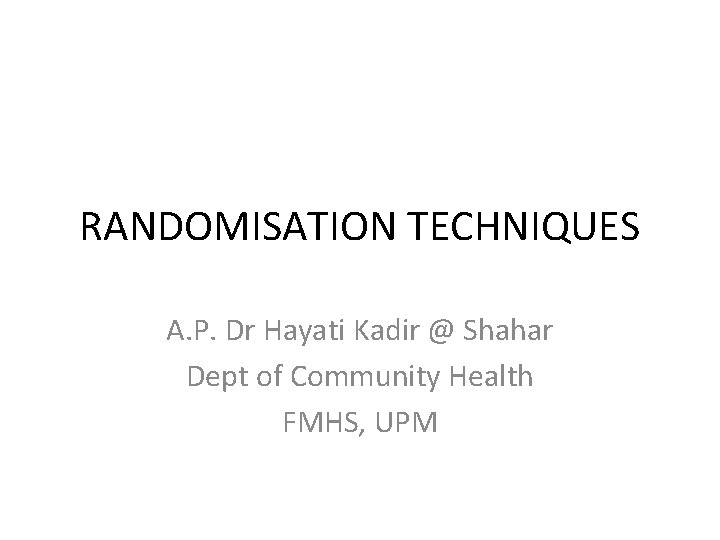
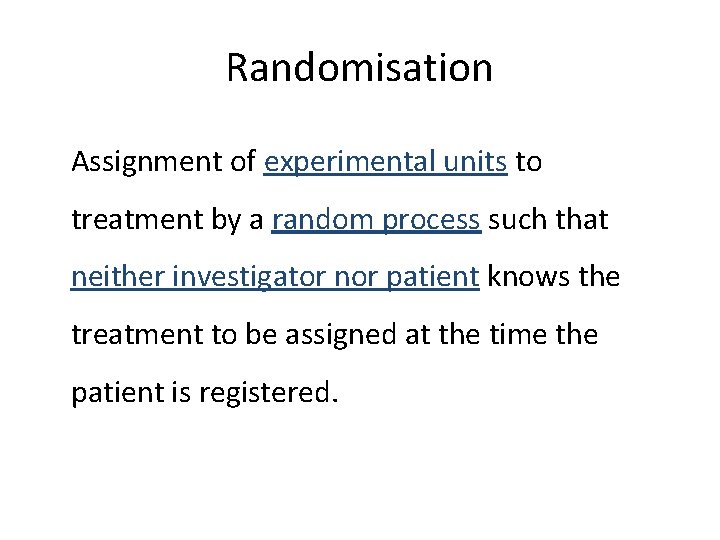
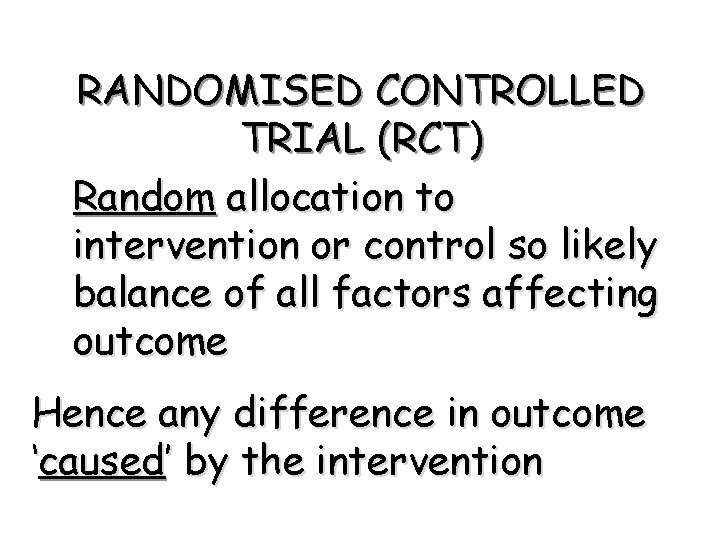
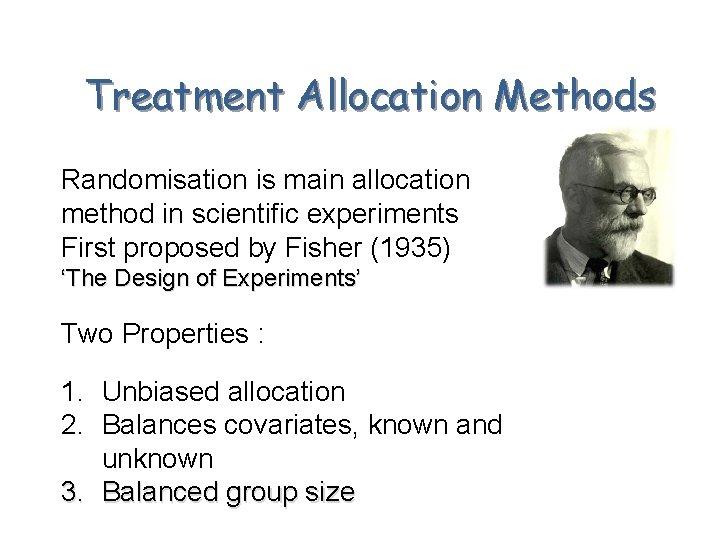
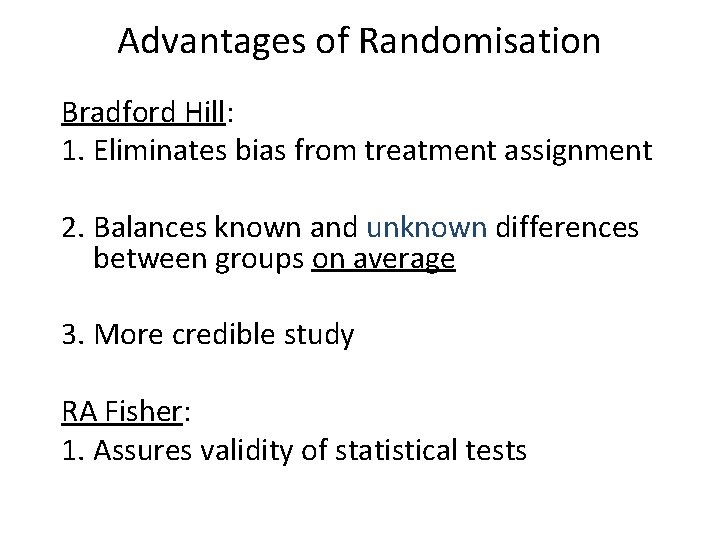
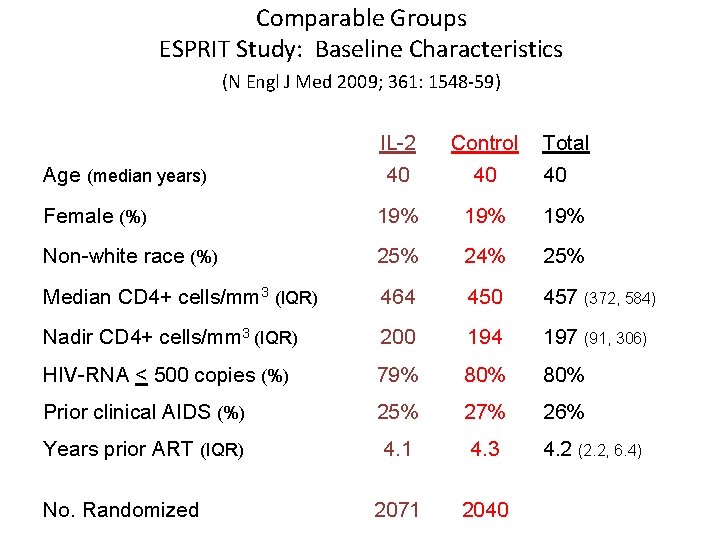
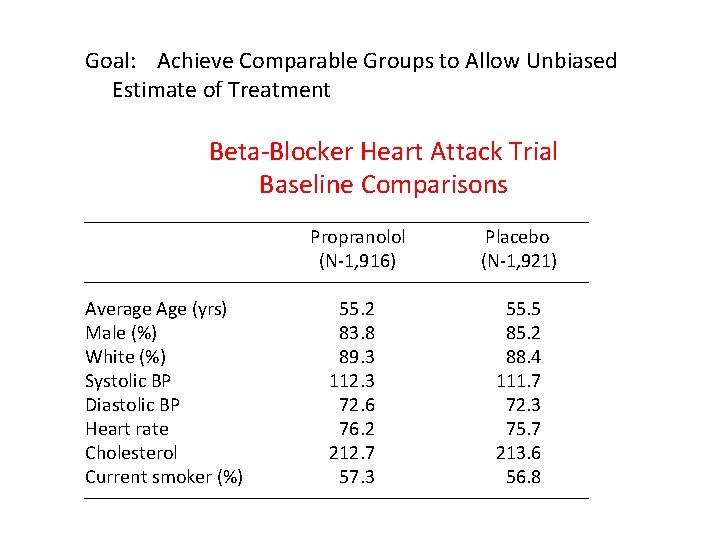
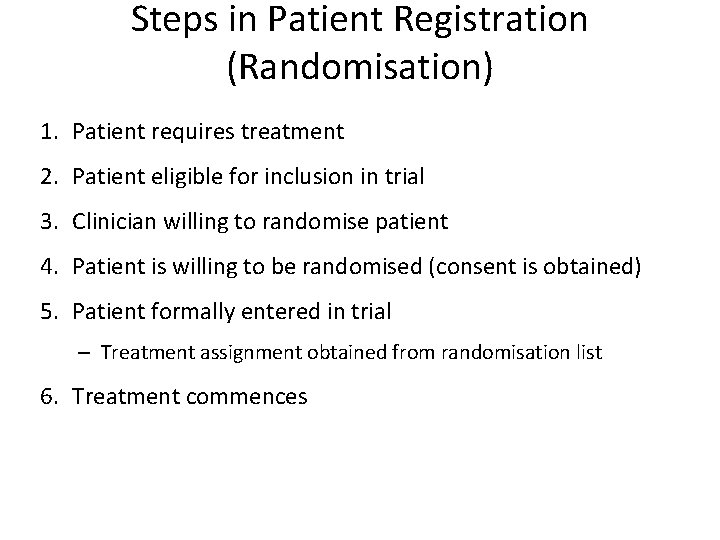
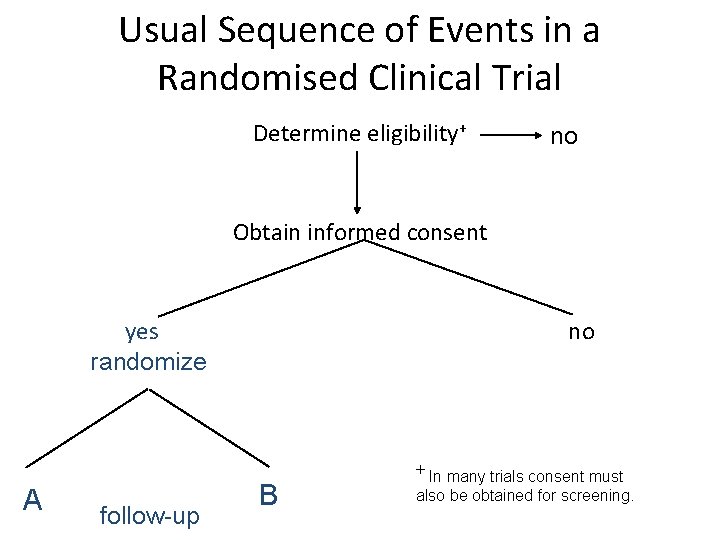
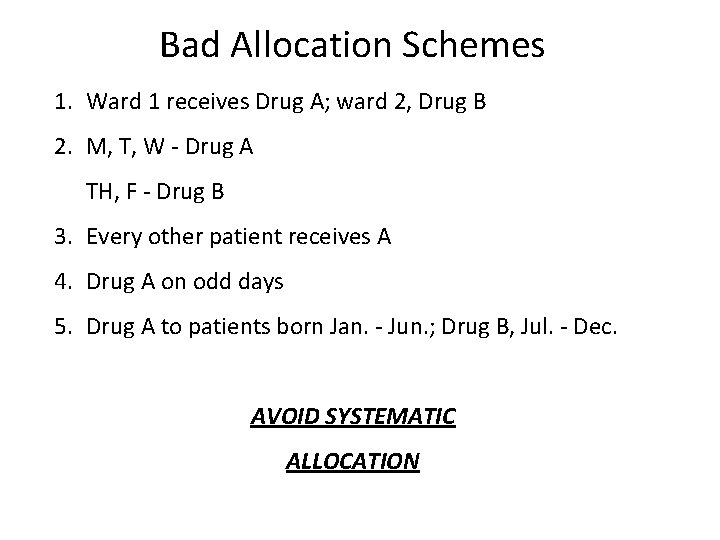
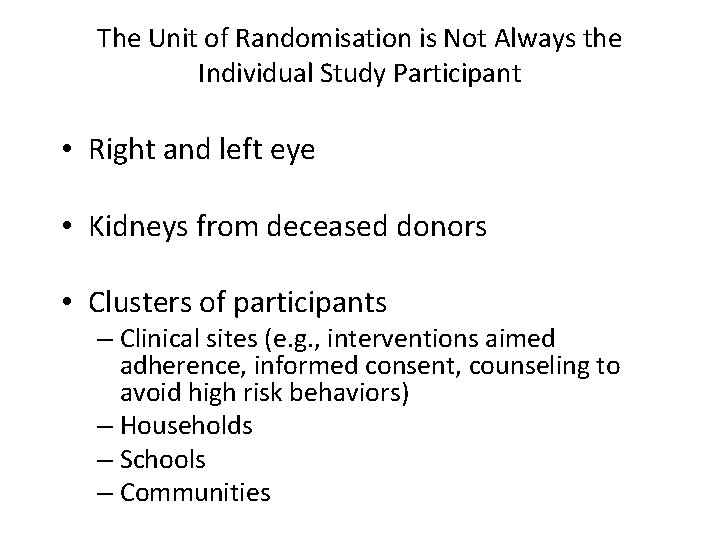
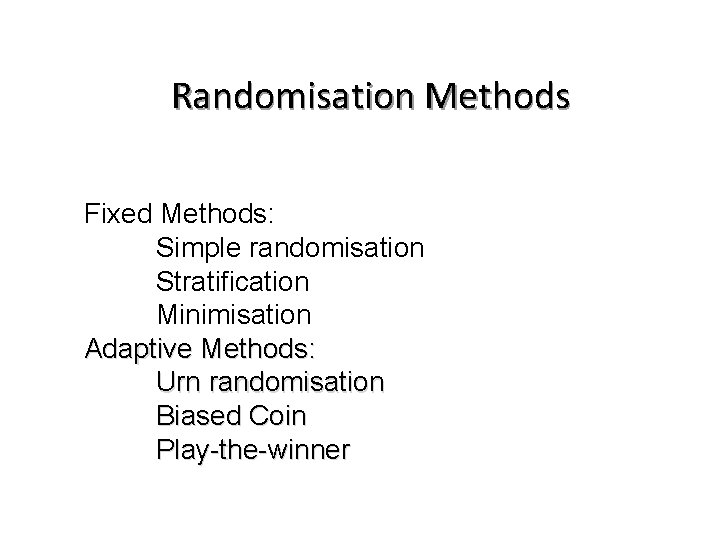
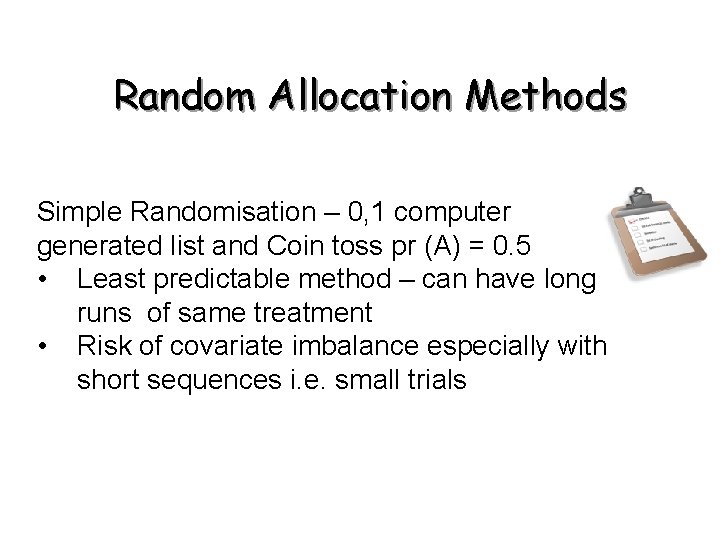
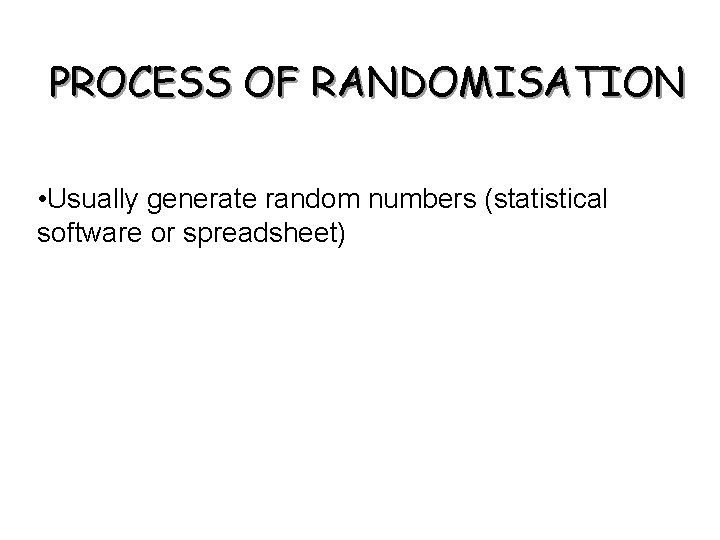
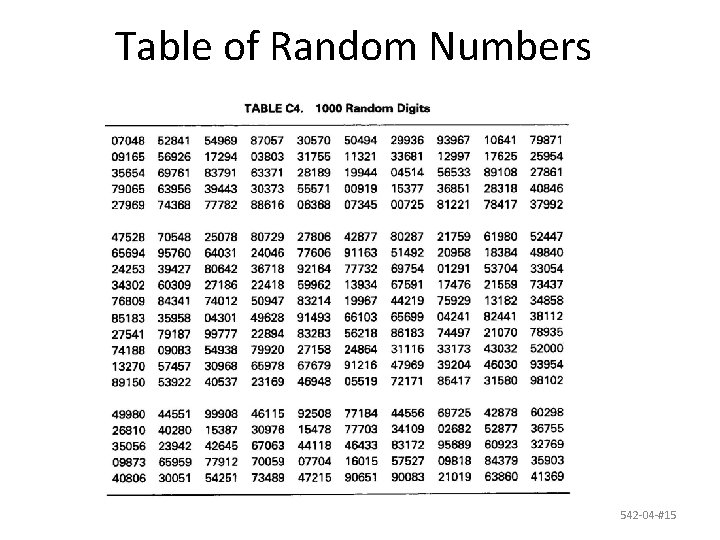
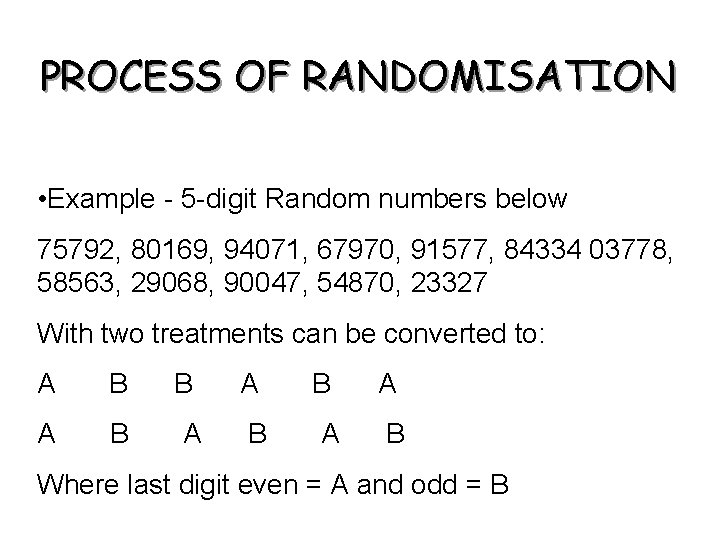
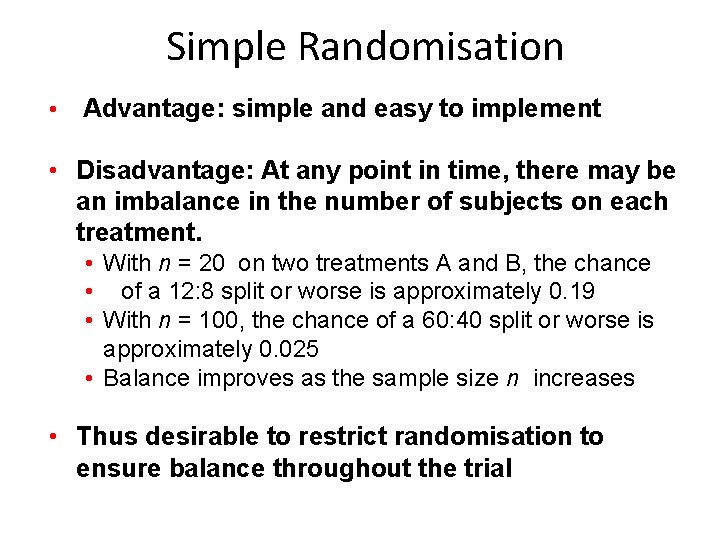
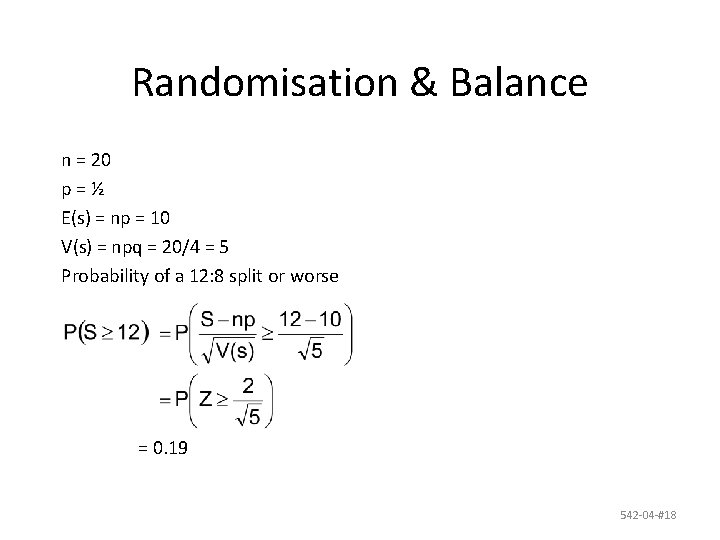
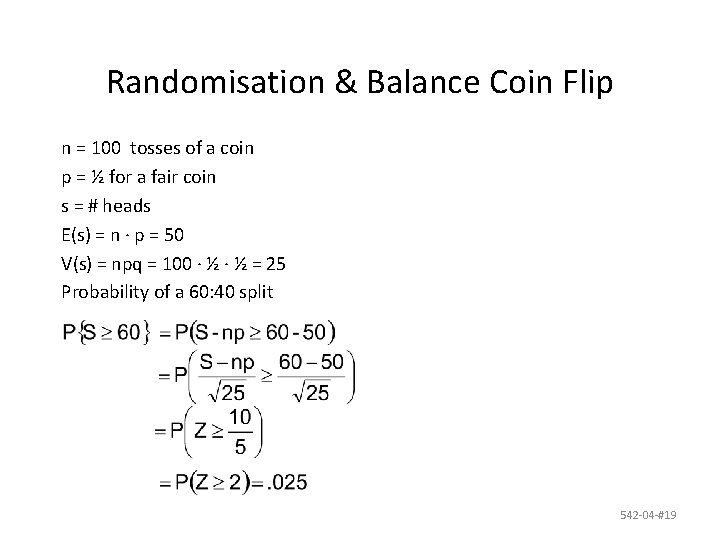
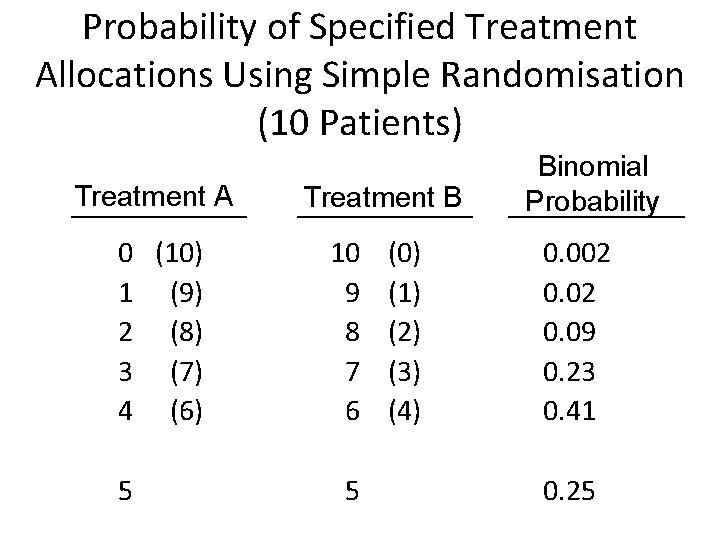
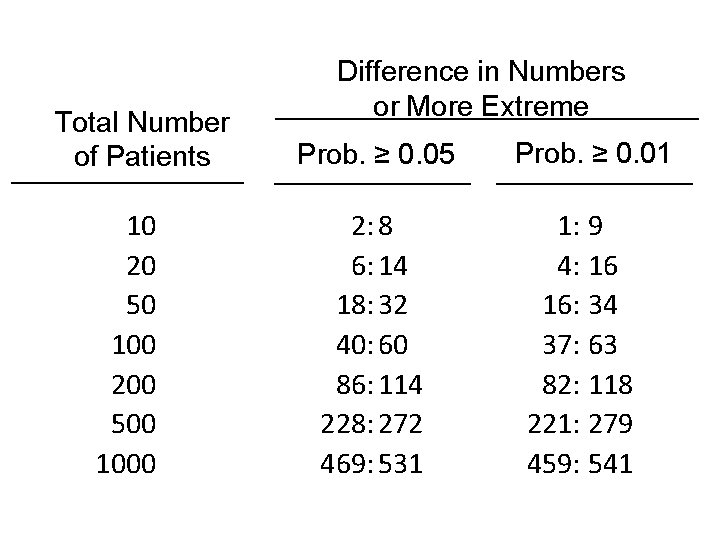
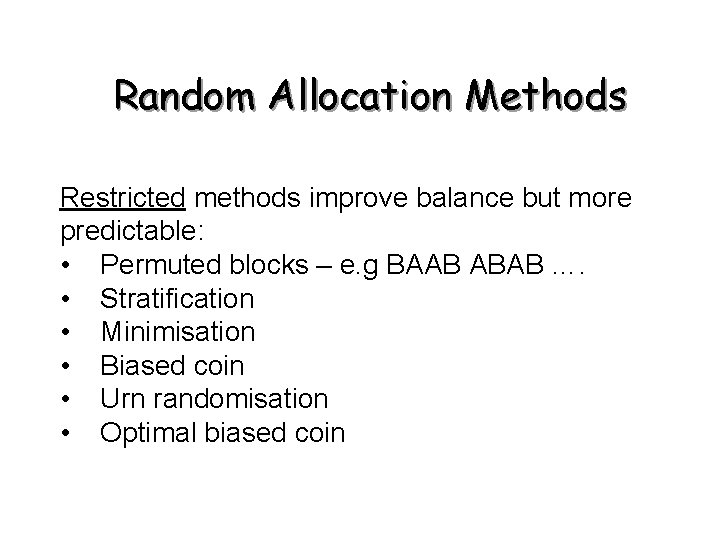
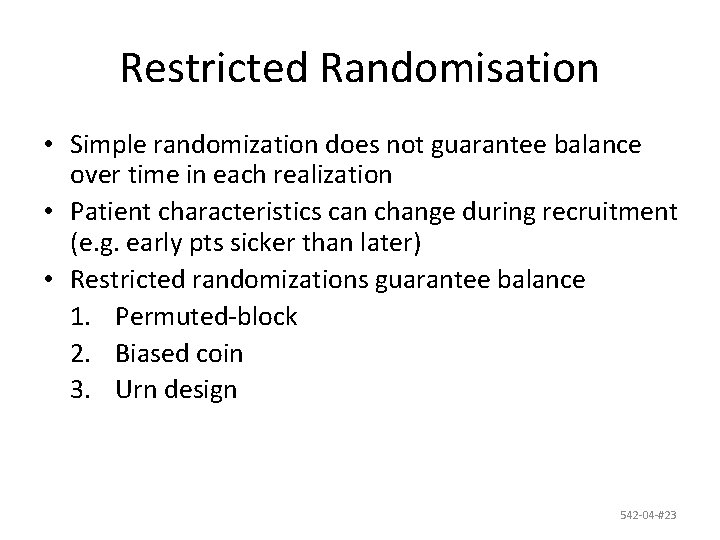
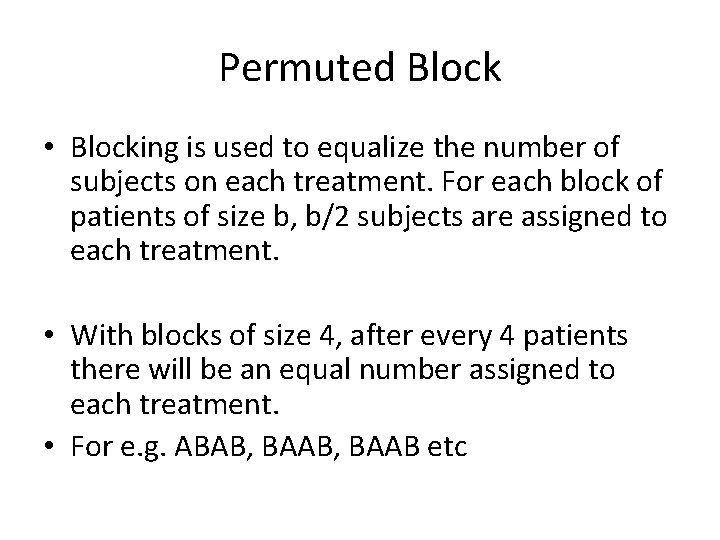
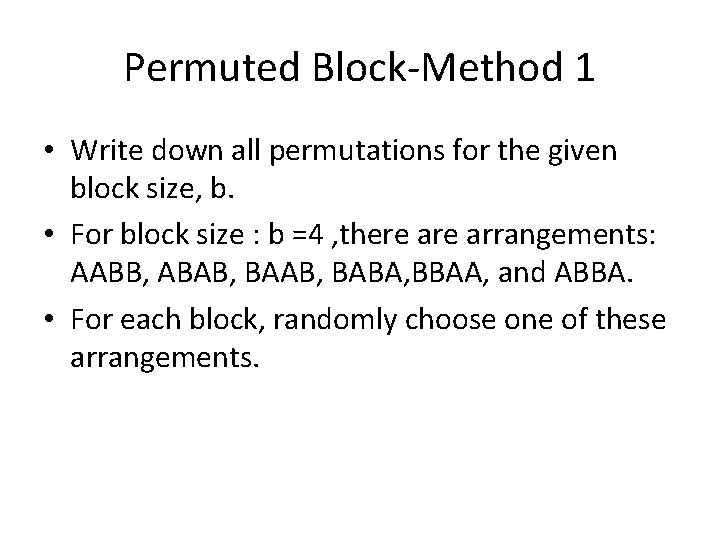
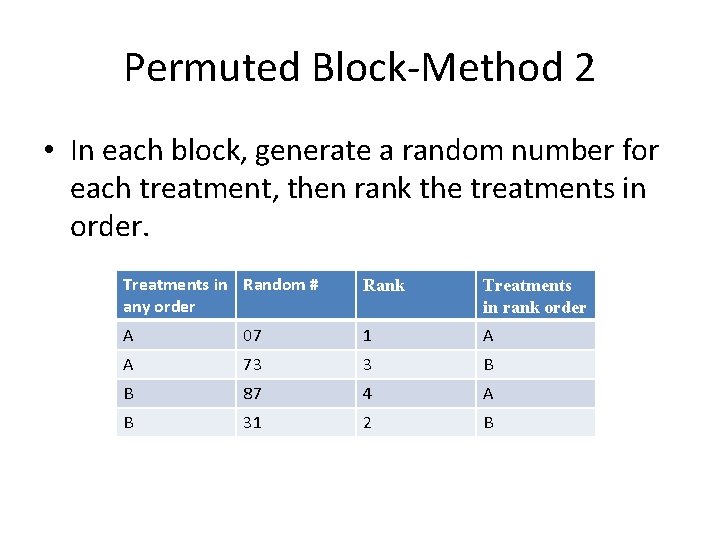
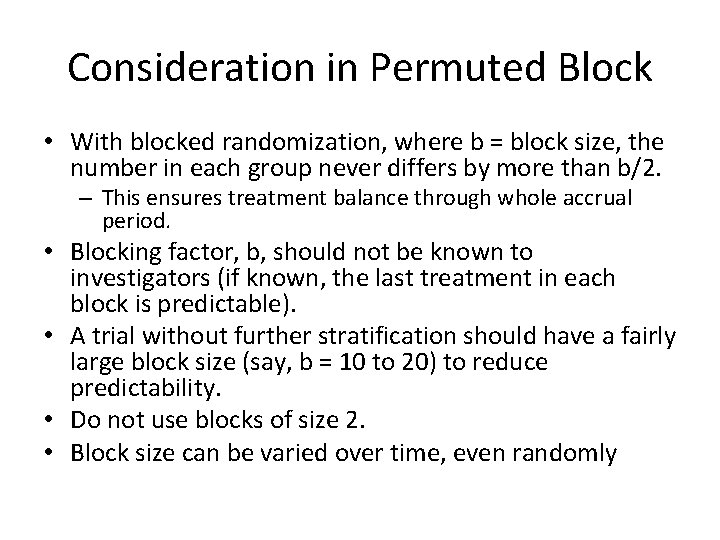
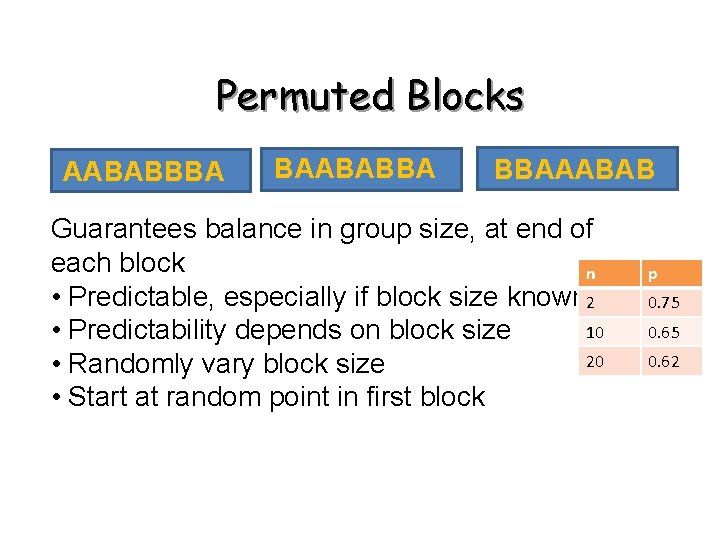
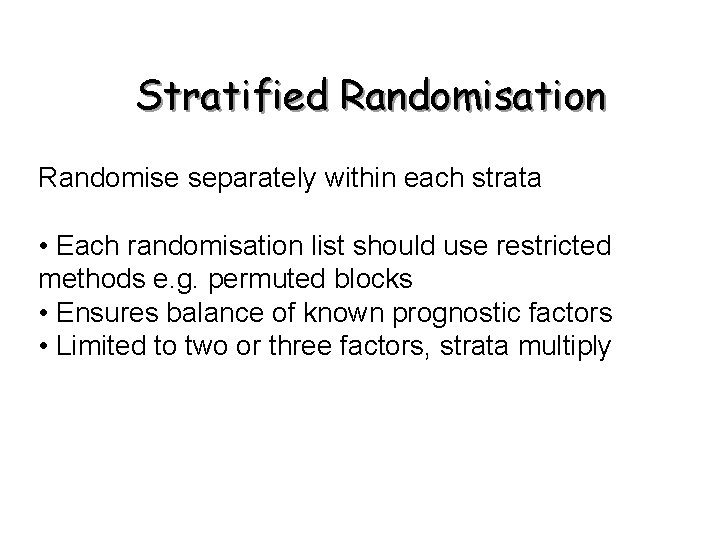
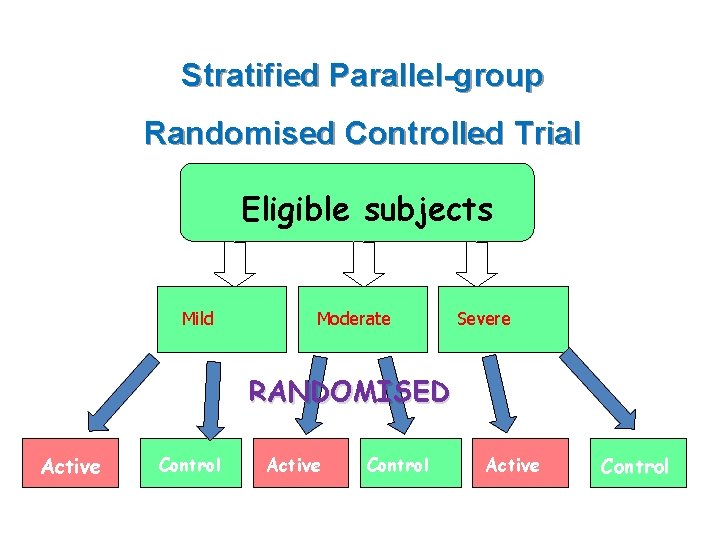
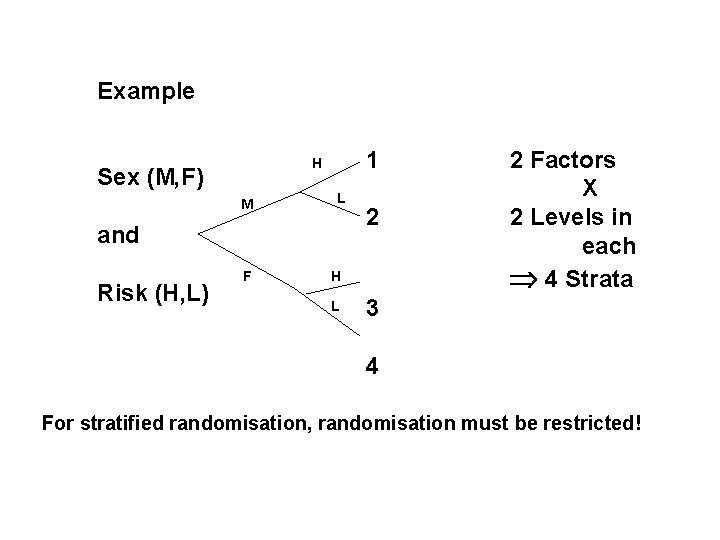
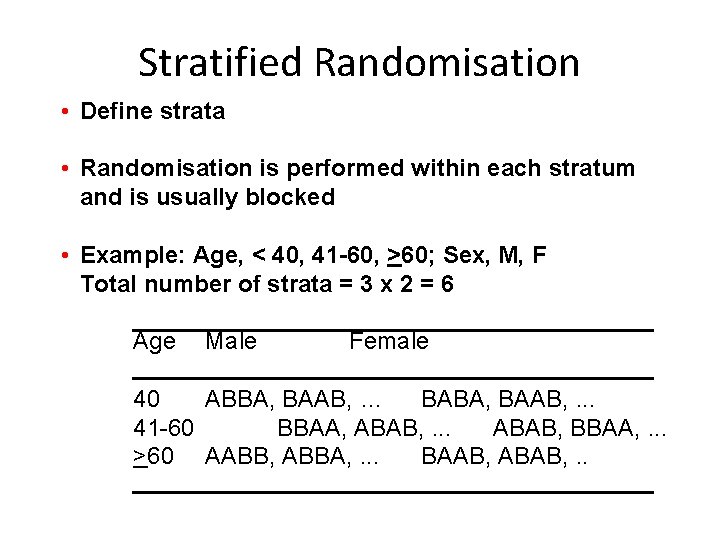
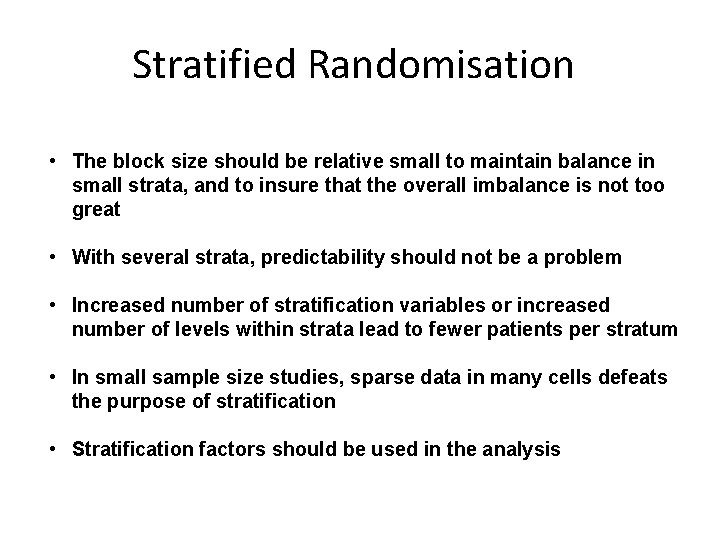
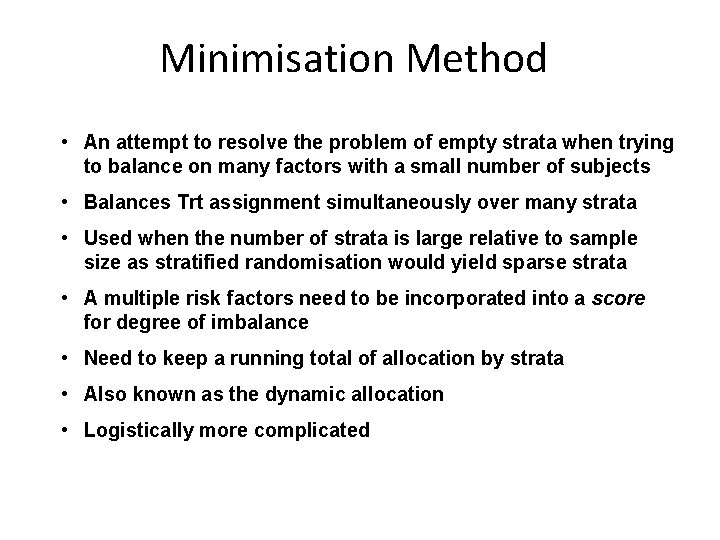
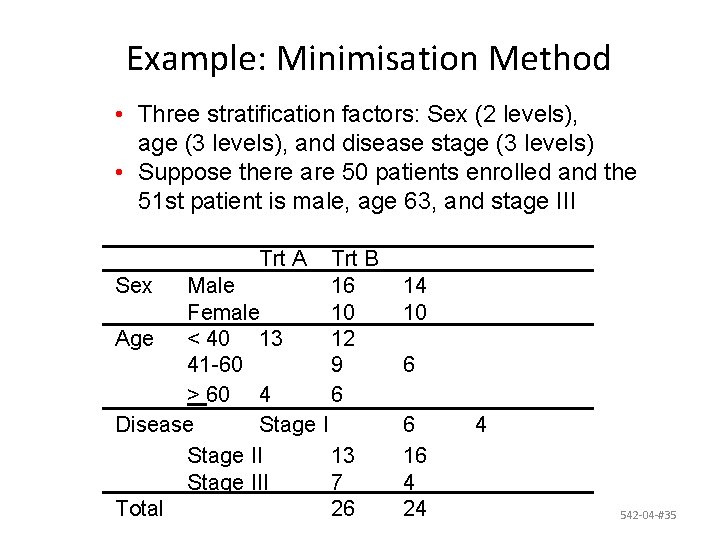
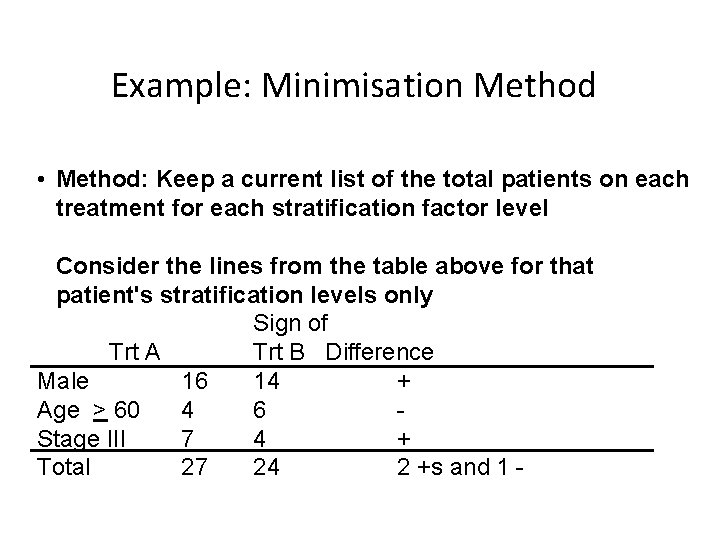
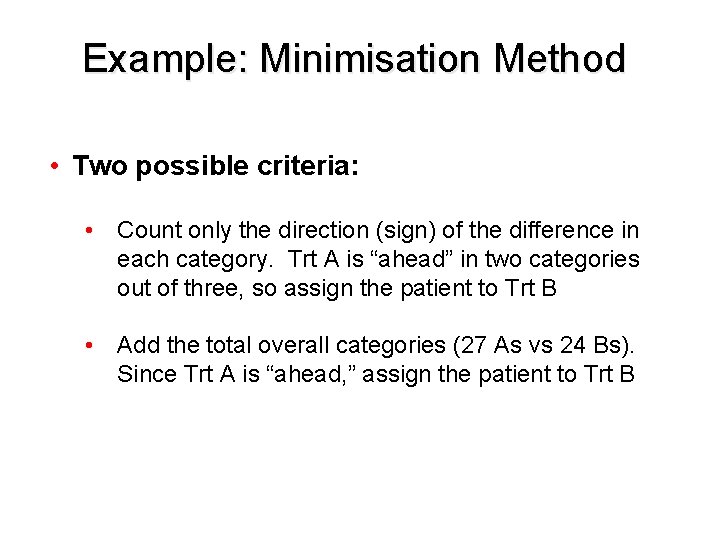
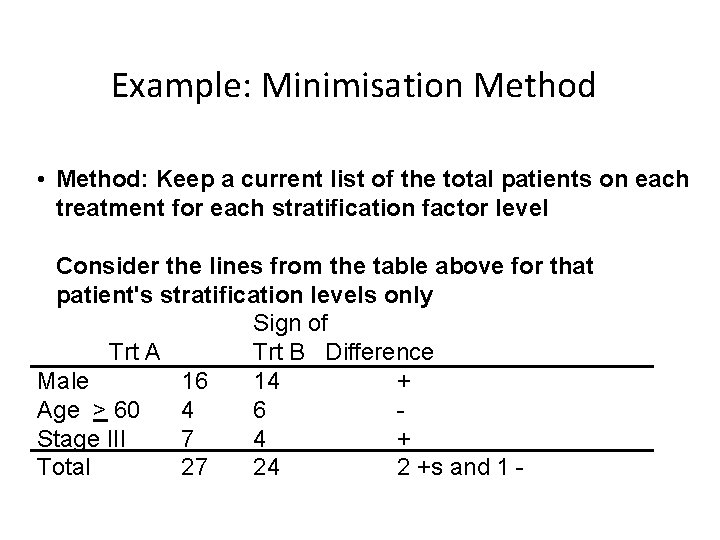
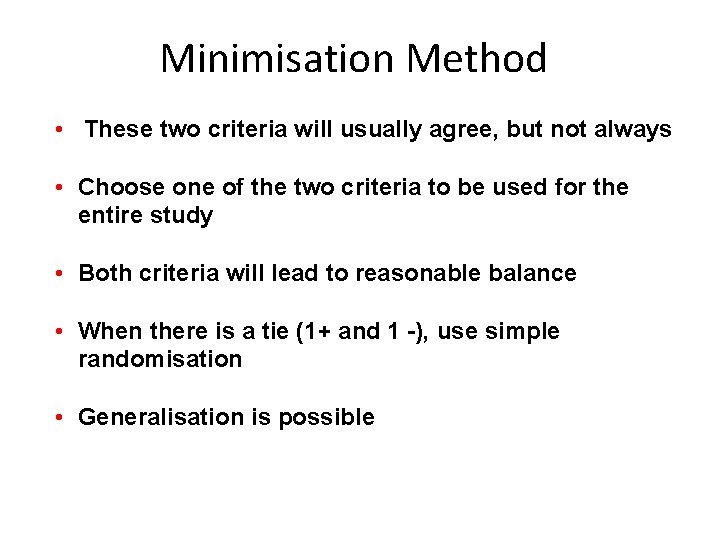
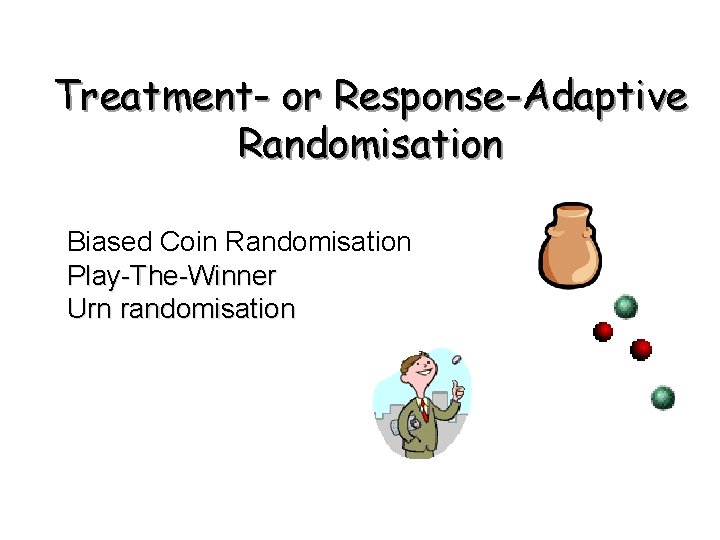
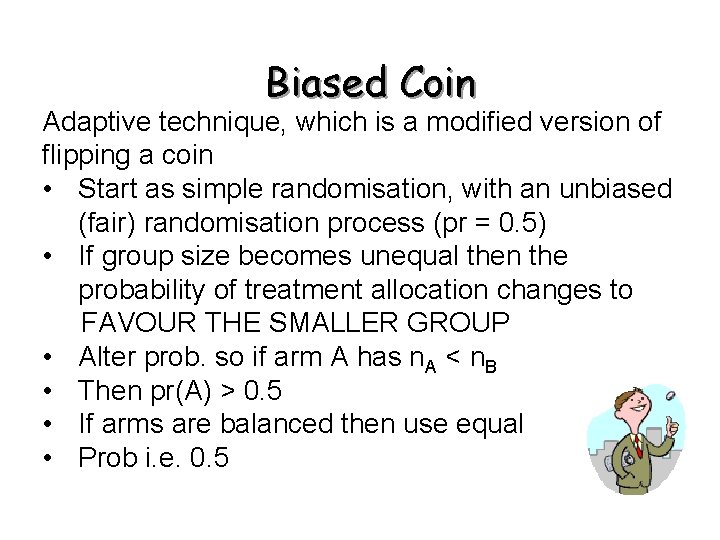
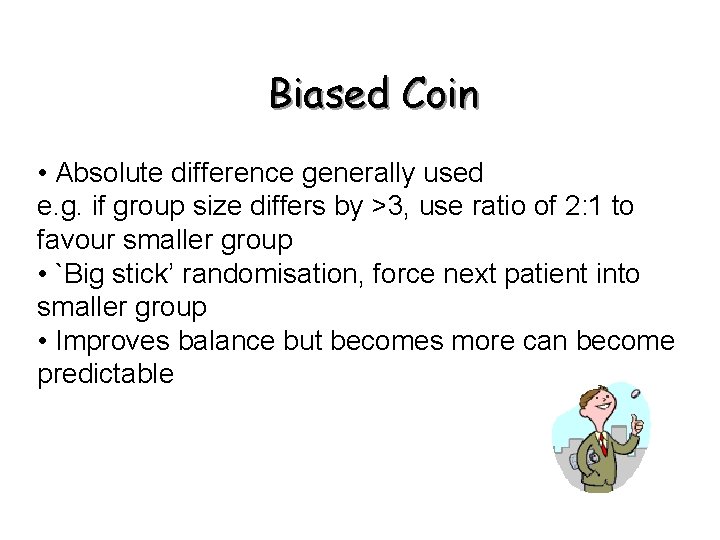
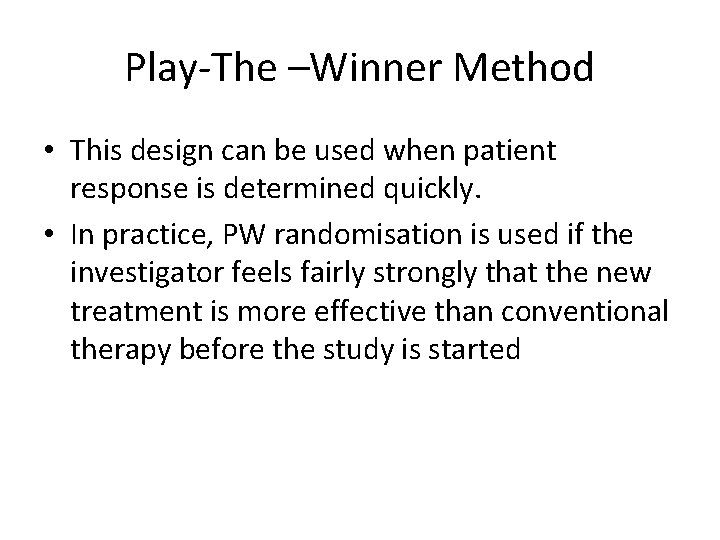
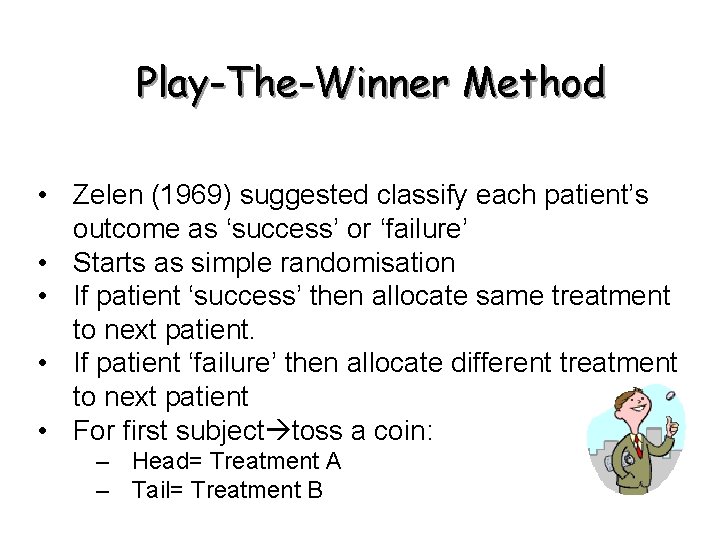
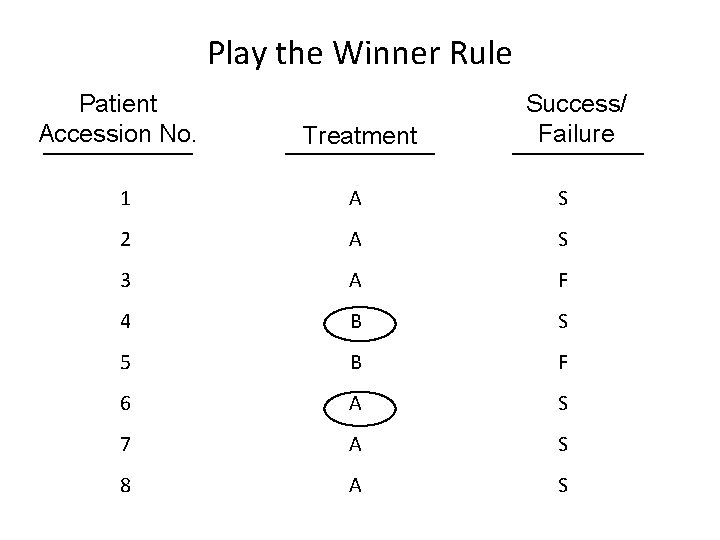
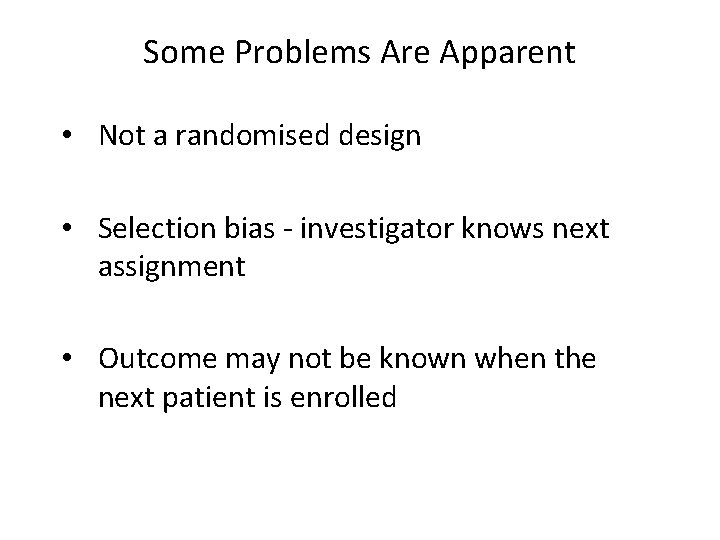
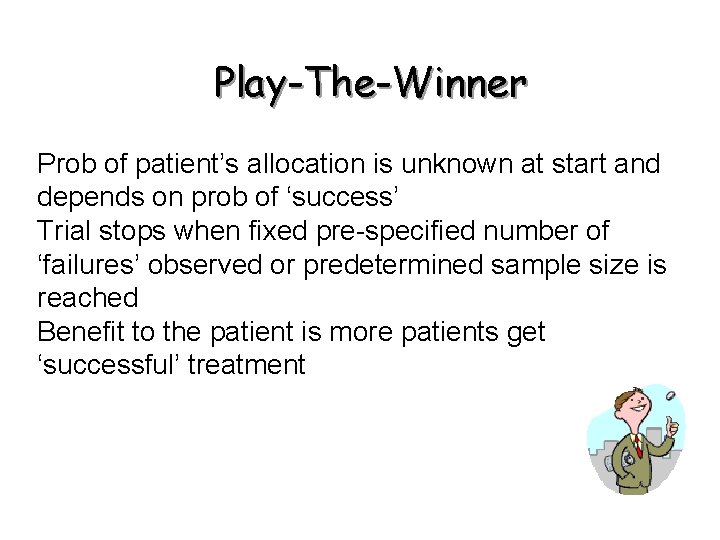
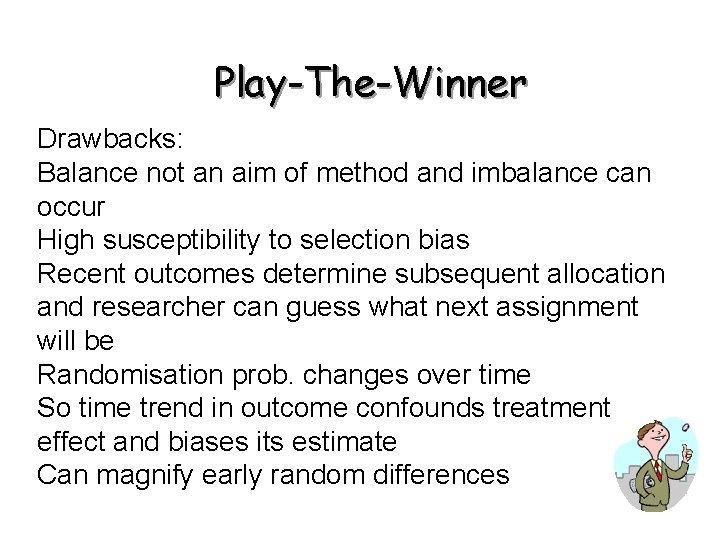
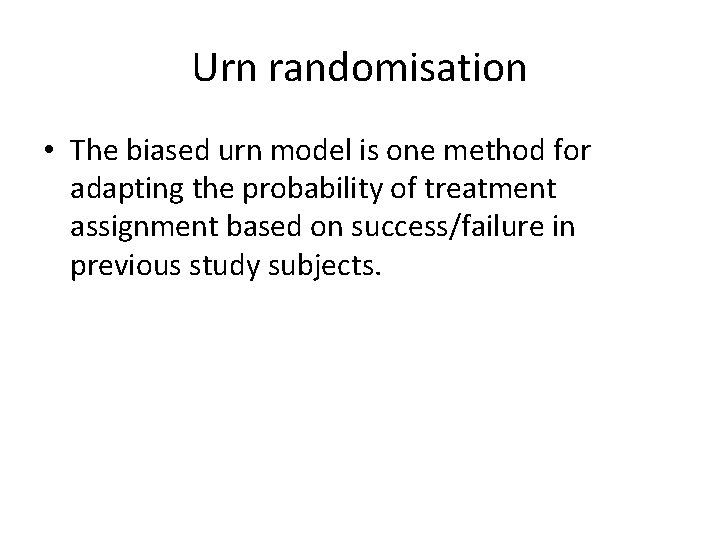
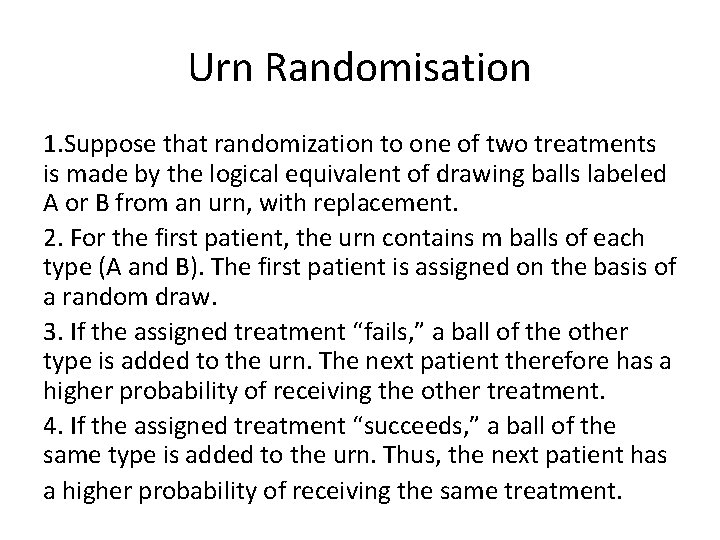
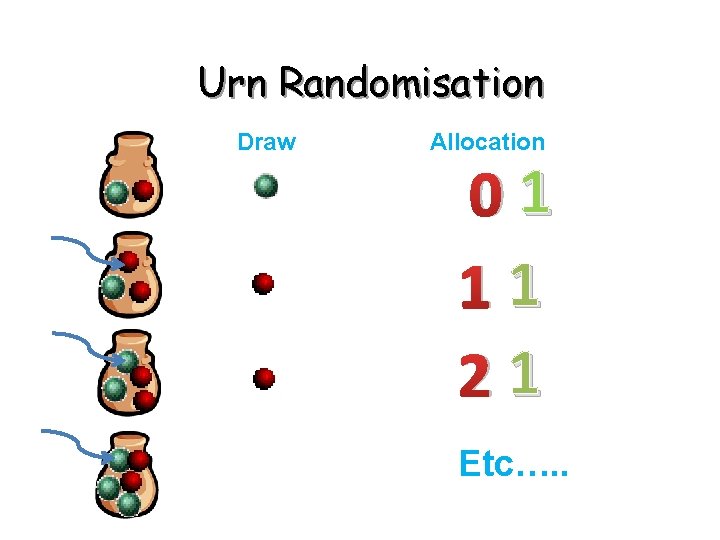
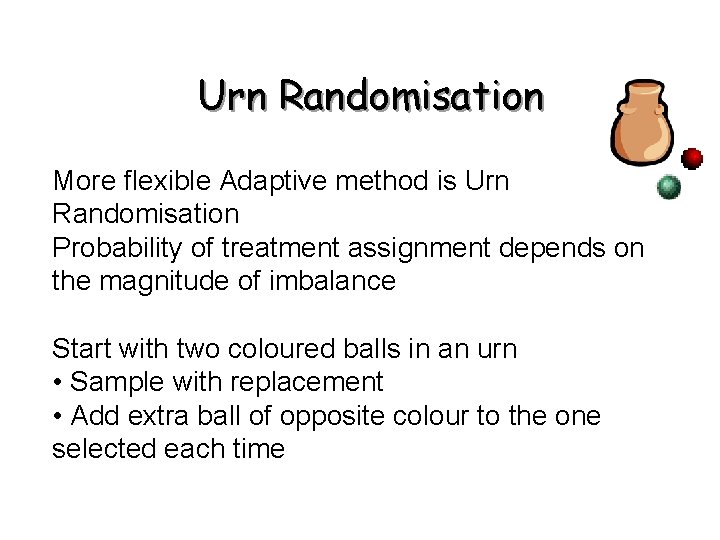
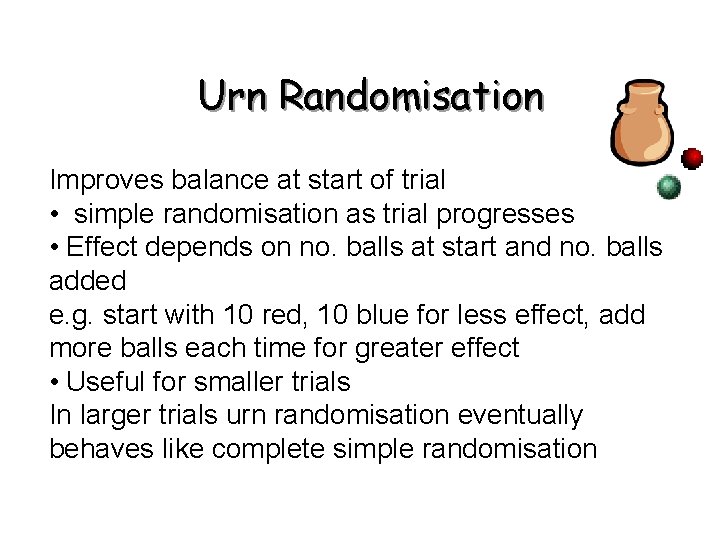
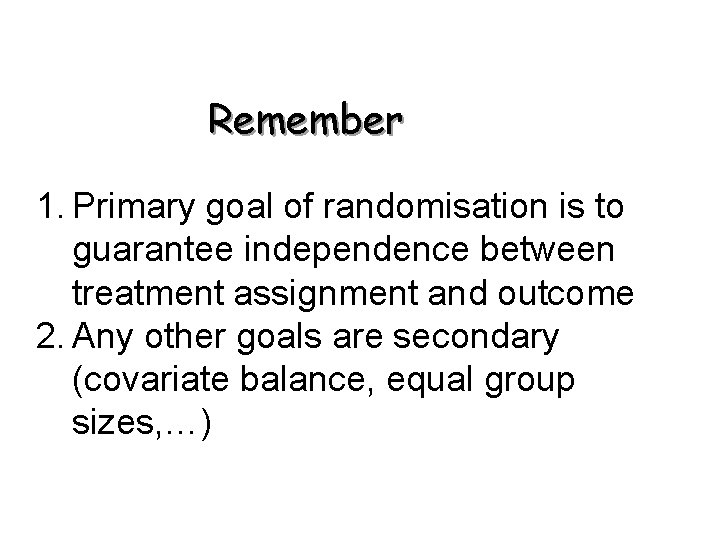
- Slides: 54
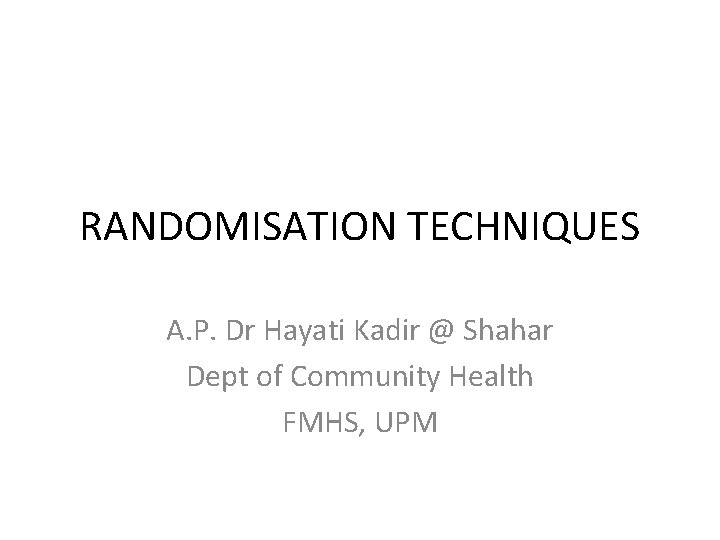
RANDOMISATION TECHNIQUES A. P. Dr Hayati Kadir @ Shahar Dept of Community Health FMHS, UPM
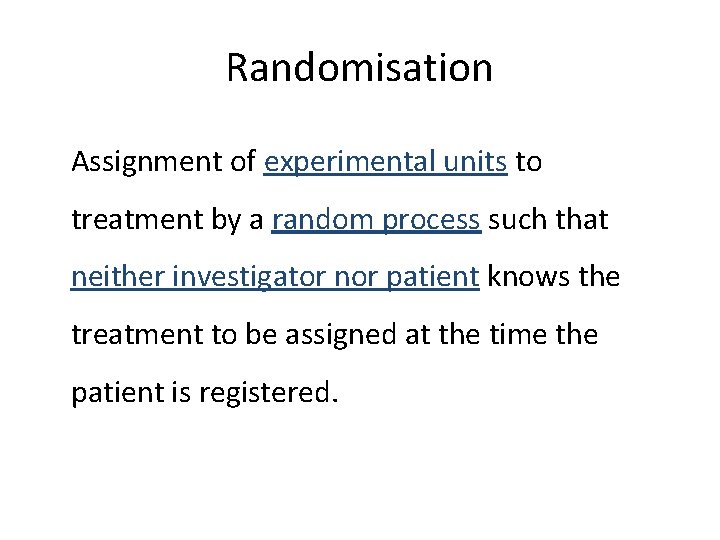
Randomisation Assignment of experimental units to treatment by a random process such that neither investigator nor patient knows the treatment to be assigned at the time the patient is registered.
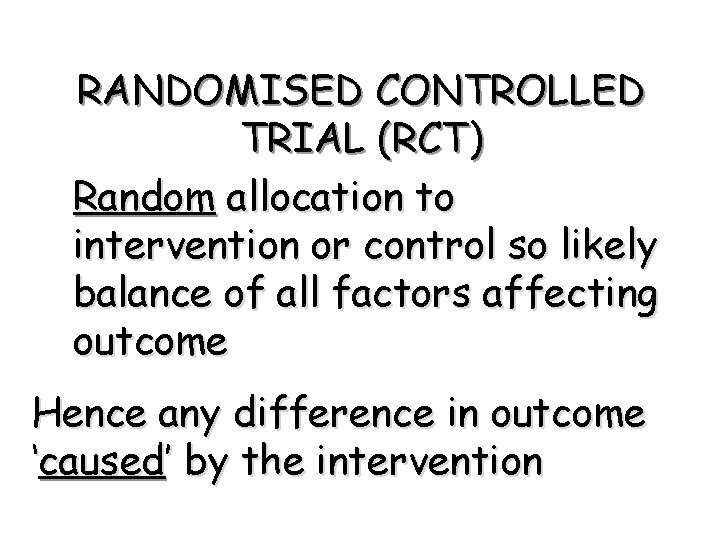
RANDOMISED CONTROLLED TRIAL (RCT) Random allocation to intervention or control so likely balance of all factors affecting outcome Hence any difference in outcome ‘caused’ by the intervention
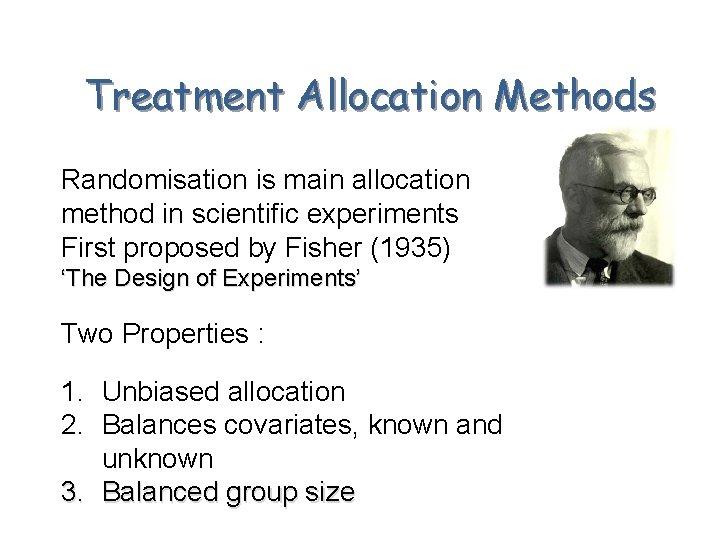
Treatment Allocation Methods Randomisation is main allocation method in scientific experiments First proposed by Fisher (1935) ‘The Design of Experiments’ Two Properties : 1. Unbiased allocation 2. Balances covariates, known and unknown 3. Balanced group size
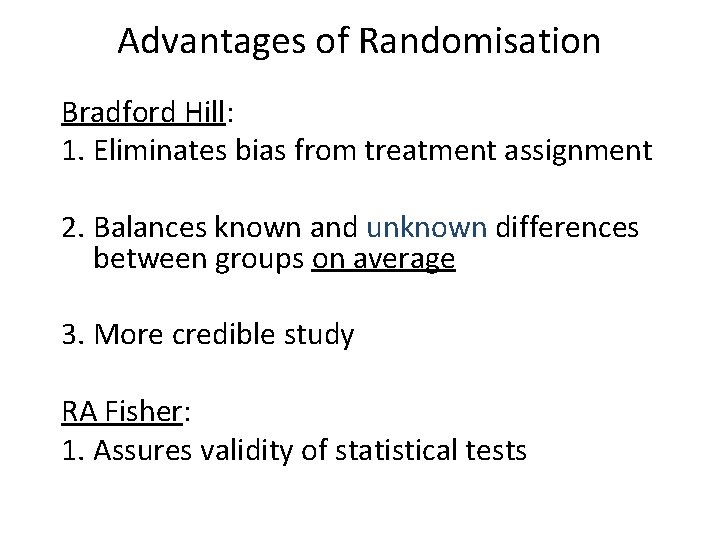
Advantages of Randomisation Bradford Hill: 1. Eliminates bias from treatment assignment 2. Balances known and unknown differences between groups on average 3. More credible study RA Fisher: 1. Assures validity of statistical tests
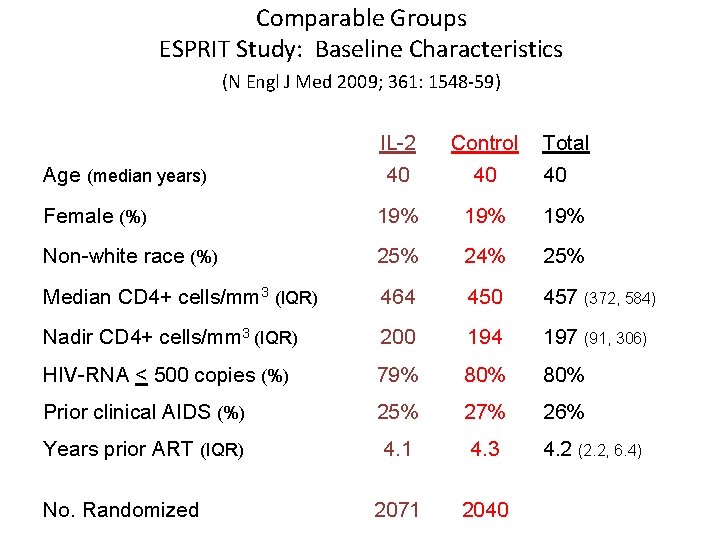
Comparable Groups ESPRIT Study: Baseline Characteristics (N Engl J Med 2009; 361: 1548 -59) IL-2 Control 40 40 Female (%) 19% 19% Non-white race (%) 25% 24% 25% Median CD 4+ cells/mm 3 (IQR) 464 450 457 (372, 584) Nadir CD 4+ cells/mm 3 (IQR) 200 194 197 (91, 306) HIV-RNA < 500 copies (%) 79% 80% Prior clinical AIDS (%) 25% 27% 26% Years prior ART (IQR) 4. 1 4. 3 2071 2040 Age (median years) No. Randomized Total 40 4. 2 (2. 2, 6. 4)
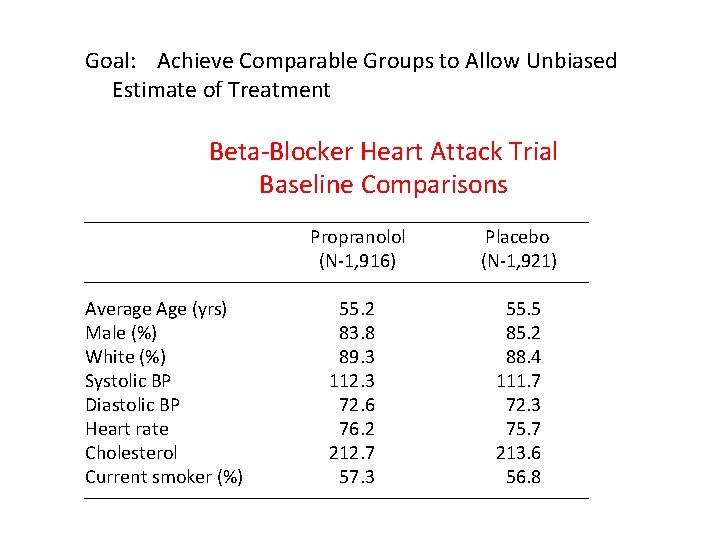
Goal: Achieve Comparable Groups to Allow Unbiased Estimate of Treatment Beta-Blocker Heart Attack Trial Baseline Comparisons Propranolol (N-1, 916) Average Age (yrs) Male (%) White (%) Systolic BP Diastolic BP Heart rate Cholesterol Current smoker (%) 55. 2 83. 8 89. 3 112. 3 72. 6 76. 2 212. 7 57. 3 Placebo (N-1, 921) 55. 5 85. 2 88. 4 111. 7 72. 3 75. 7 213. 6 56. 8
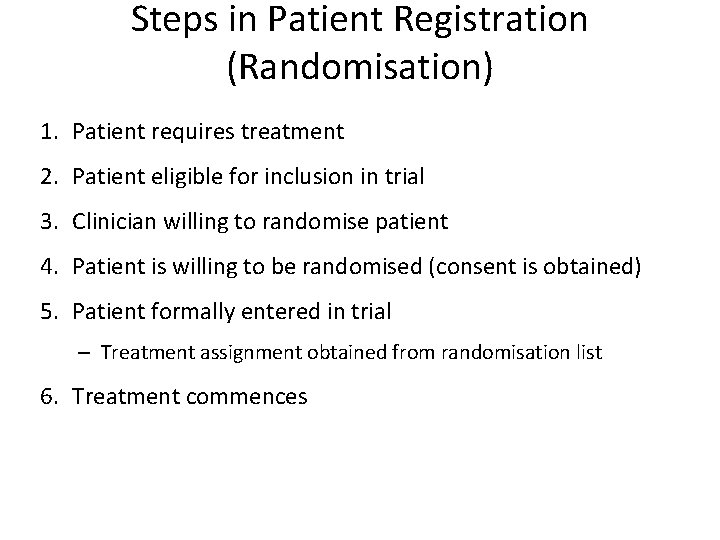
Steps in Patient Registration (Randomisation) 1. Patient requires treatment 2. Patient eligible for inclusion in trial 3. Clinician willing to randomise patient 4. Patient is willing to be randomised (consent is obtained) 5. Patient formally entered in trial – Treatment assignment obtained from randomisation list 6. Treatment commences
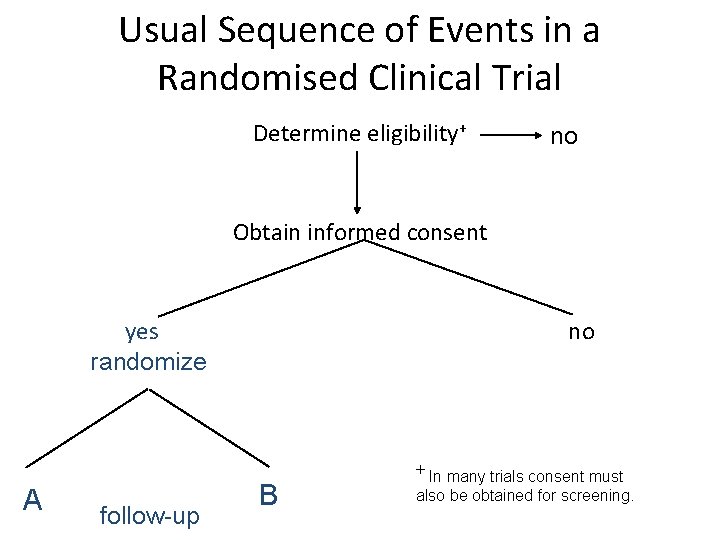
Usual Sequence of Events in a Randomised Clinical Trial Determine eligibility+ no Obtain informed consent yes randomize A follow-up no B + In many trials consent must also be obtained for screening.
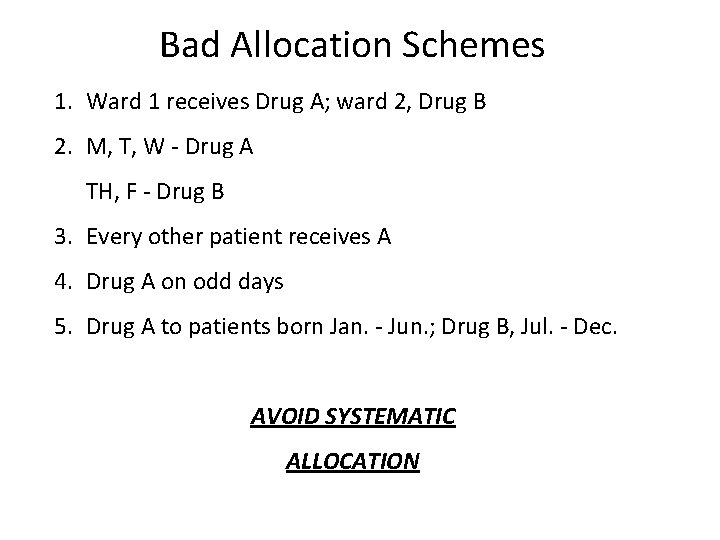
Bad Allocation Schemes 1. Ward 1 receives Drug A; ward 2, Drug B 2. M, T, W - Drug A TH, F - Drug B 3. Every other patient receives A 4. Drug A on odd days 5. Drug A to patients born Jan. - Jun. ; Drug B, Jul. - Dec. AVOID SYSTEMATIC ALLOCATION
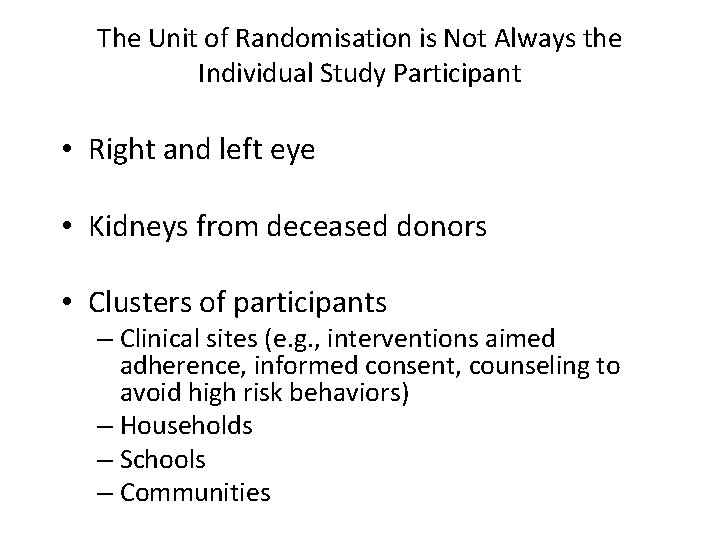
The Unit of Randomisation is Not Always the Individual Study Participant • Right and left eye • Kidneys from deceased donors • Clusters of participants – Clinical sites (e. g. , interventions aimed adherence, informed consent, counseling to avoid high risk behaviors) – Households – Schools – Communities
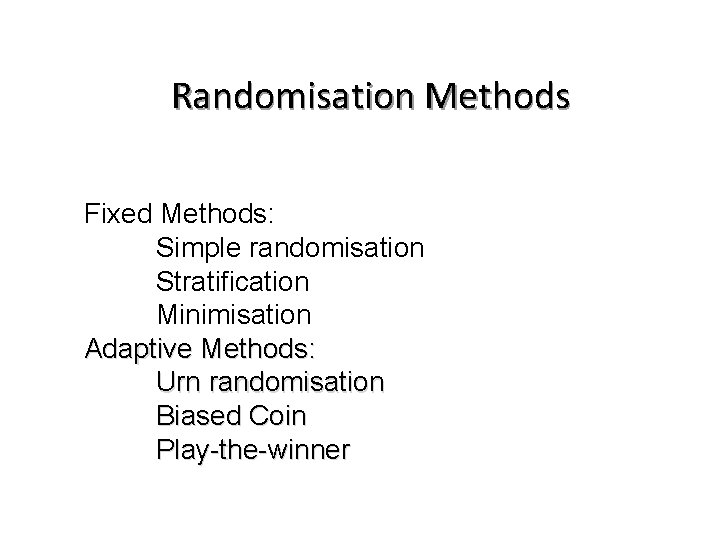
Randomisation Methods Fixed Methods: Simple randomisation Stratification Minimisation Adaptive Methods: Urn randomisation Biased Coin Play-the-winner
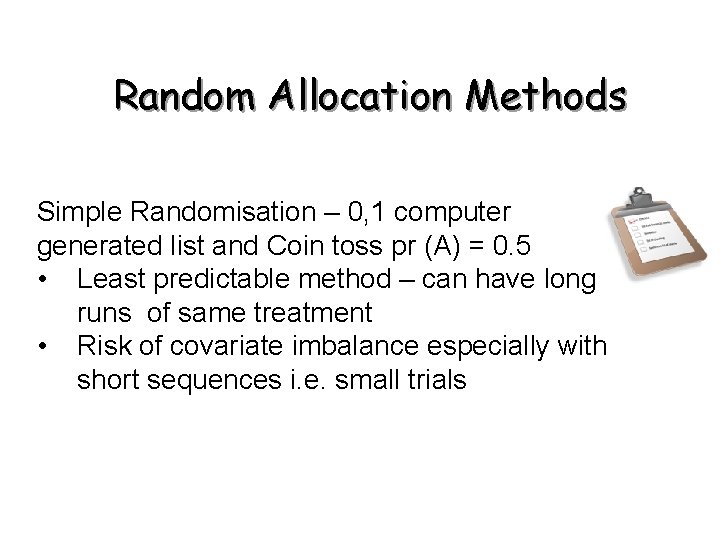
Random Allocation Methods Simple Randomisation – 0, 1 computer generated list and Coin toss pr (A) = 0. 5 • Least predictable method – can have long runs of same treatment • Risk of covariate imbalance especially with short sequences i. e. small trials
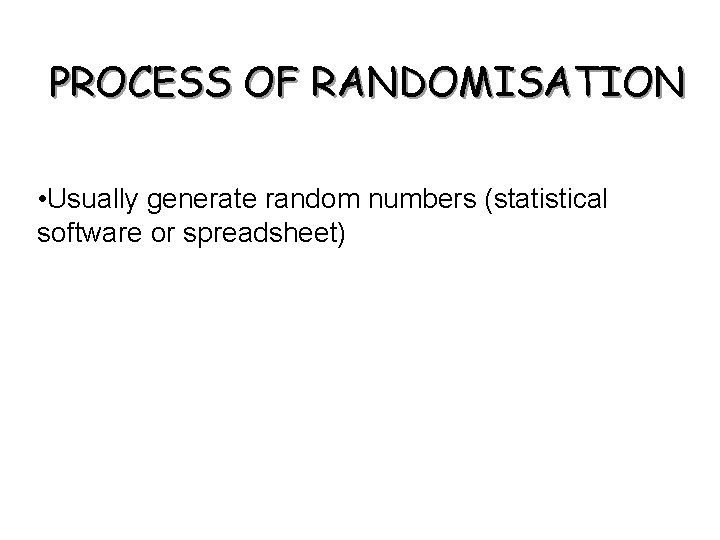
PROCESS OF RANDOMISATION • Usually generate random numbers (statistical software or spreadsheet)
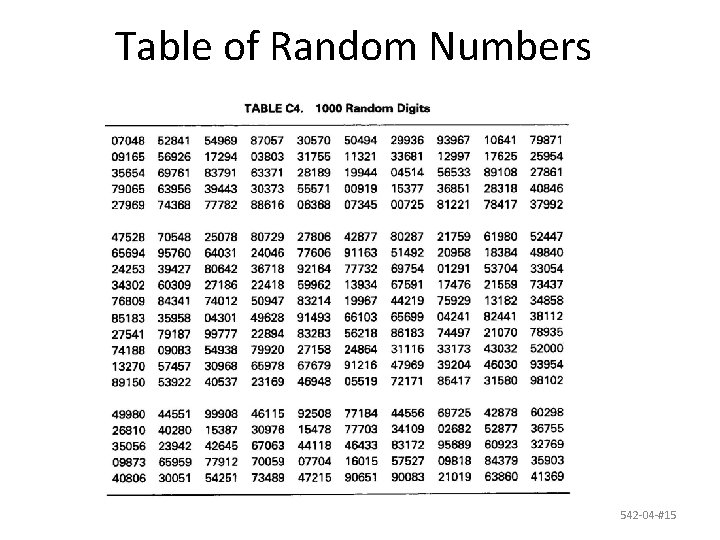
Table of Random Numbers 542 -04 -#15
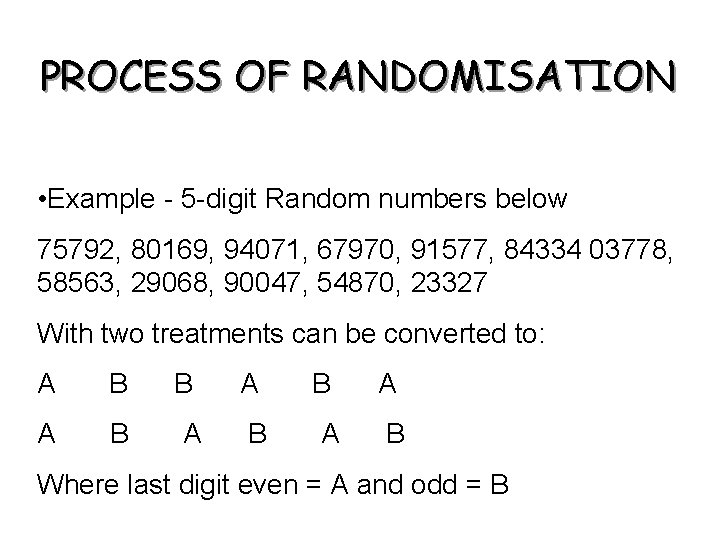
PROCESS OF RANDOMISATION • Example - 5 -digit Random numbers below 75792, 80169, 94071, 67970, 91577, 84334 03778, 58563, 29068, 90047, 54870, 23327 With two treatments can be converted to: A B B A A B Where last digit even = A and odd = B
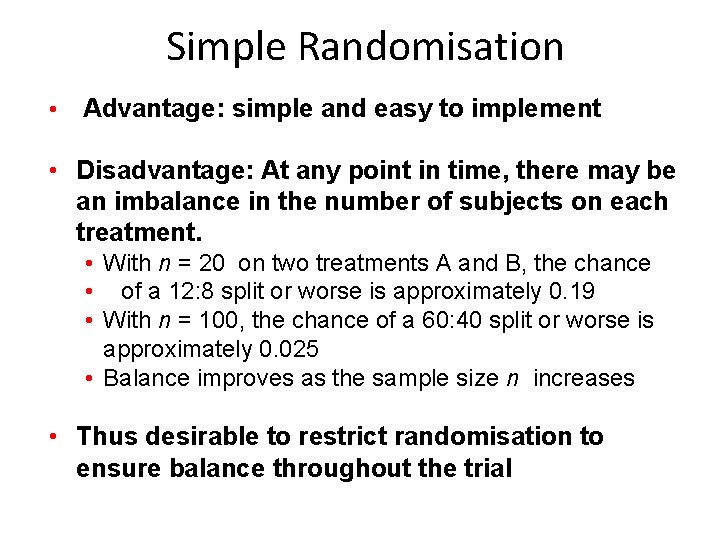
Simple Randomisation • Advantage: simple and easy to implement • Disadvantage: At any point in time, there may be an imbalance in the number of subjects on each treatment. • With n = 20 on two treatments A and B, the chance • of a 12: 8 split or worse is approximately 0. 19 • With n = 100, the chance of a 60: 40 split or worse is approximately 0. 025 • Balance improves as the sample size n increases • Thus desirable to restrict randomisation to ensure balance throughout the trial
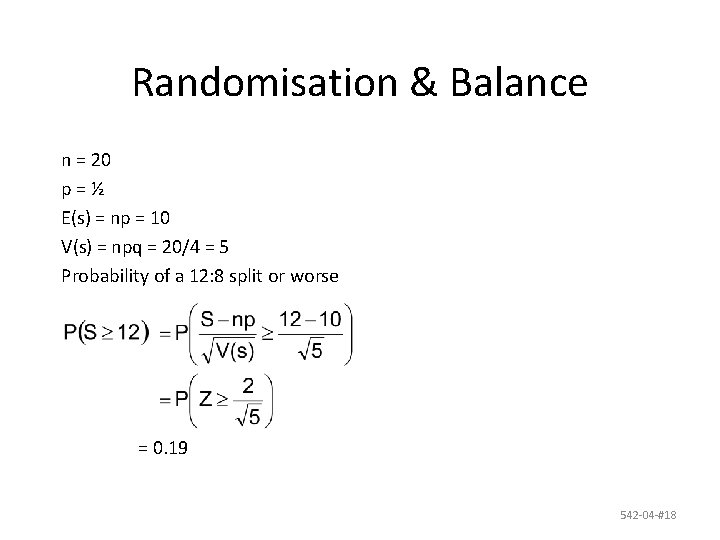
Randomisation & Balance n = 20 p=½ E(s) = np = 10 V(s) = npq = 20/4 = 5 Probability of a 12: 8 split or worse = 0. 19 542 -04 -#18
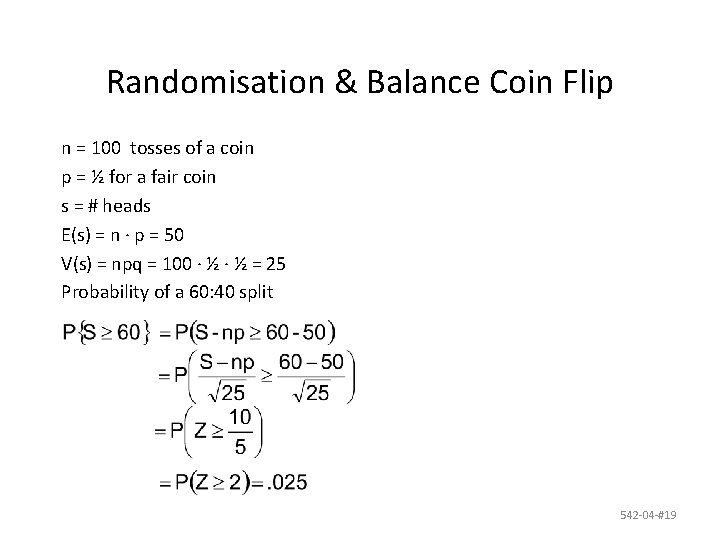
Randomisation & Balance Coin Flip n = 100 tosses of a coin p = ½ for a fair coin s = # heads E(s) = n · p = 50 V(s) = npq = 100 · ½ = 25 Probability of a 60: 40 split 542 -04 -#19
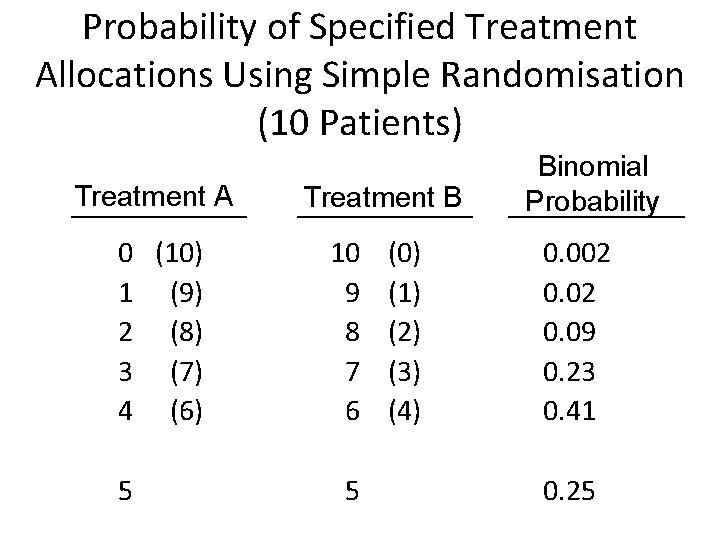
Probability of Specified Treatment Allocations Using Simple Randomisation (10 Patients) Treatment A 0 (10) 1 (9) 2 (8) 3 (7) 4 (6) 5 Treatment B 10 9 8 7 6 5 (0) (1) (2) (3) (4) Binomial Probability 0. 002 0. 09 0. 23 0. 41 0. 25
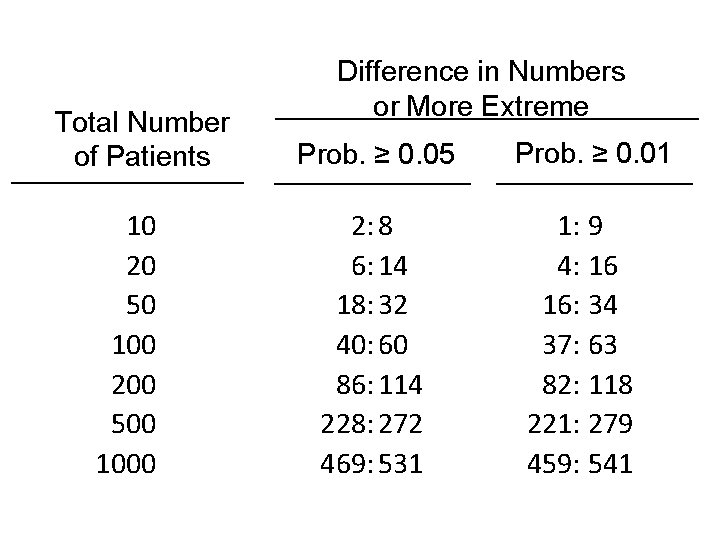
Total Number of Patients 10 20 50 100 200 500 1000 Difference in Numbers or More Extreme Prob. ≥ 0. 05 2: 8 6: 14 18: 32 40: 60 86: 114 228: 272 469: 531 Prob. ≥ 0. 01 1: 9 4: 16 16: 34 37: 63 82: 118 221: 279 459: 541
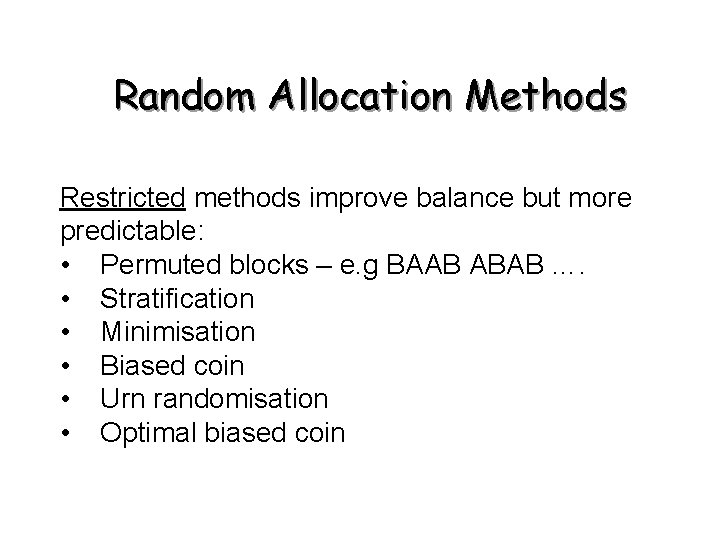
Random Allocation Methods Restricted methods improve balance but more predictable: • Permuted blocks – e. g BAAB ABAB …. • Stratification • Minimisation • Biased coin • Urn randomisation • Optimal biased coin
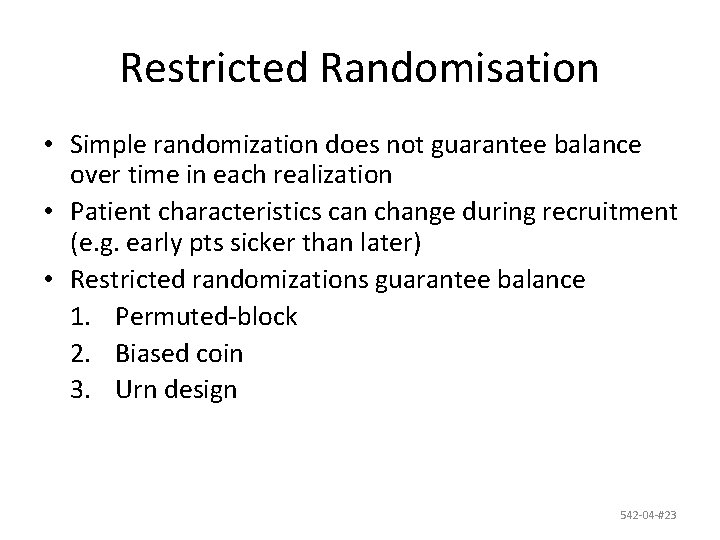
Restricted Randomisation • Simple randomization does not guarantee balance over time in each realization • Patient characteristics can change during recruitment (e. g. early pts sicker than later) • Restricted randomizations guarantee balance 1. Permuted-block 2. Biased coin 3. Urn design 542 -04 -#23
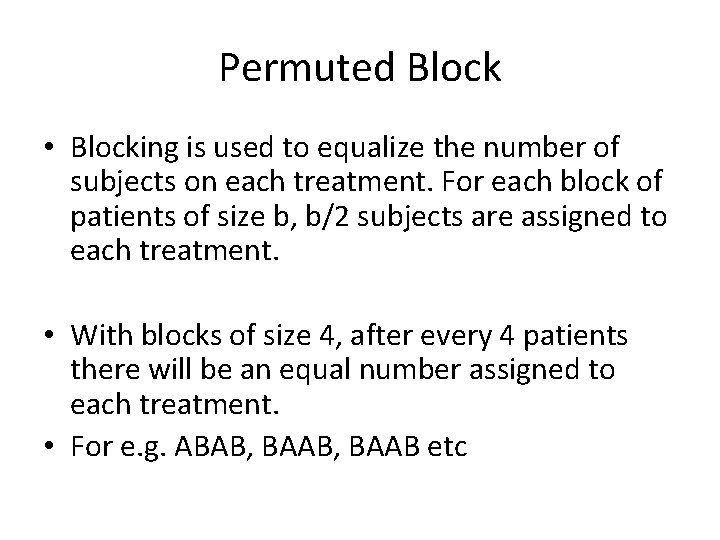
Permuted Block • Blocking is used to equalize the number of subjects on each treatment. For each block of patients of size b, b/2 subjects are assigned to each treatment. • With blocks of size 4, after every 4 patients there will be an equal number assigned to each treatment. • For e. g. ABAB, BAAB etc
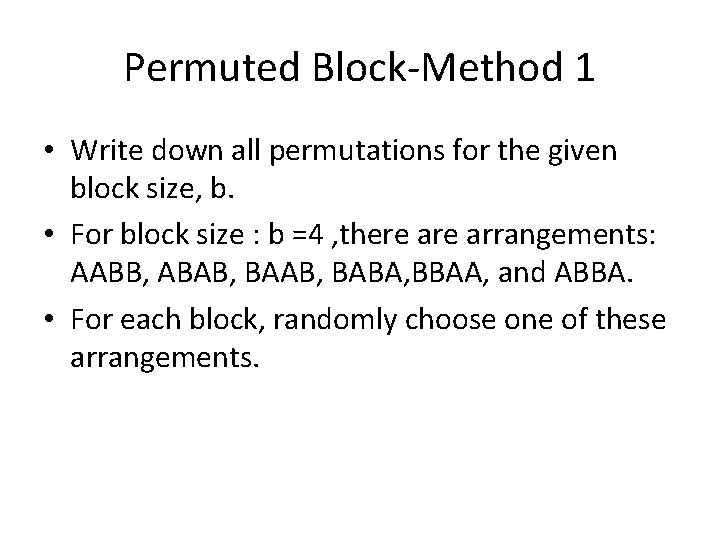
Permuted Block-Method 1 • Write down all permutations for the given block size, b. • For block size : b =4 , there arrangements: AABB, ABAB, BABA, BBAA, and ABBA. • For each block, randomly choose one of these arrangements.
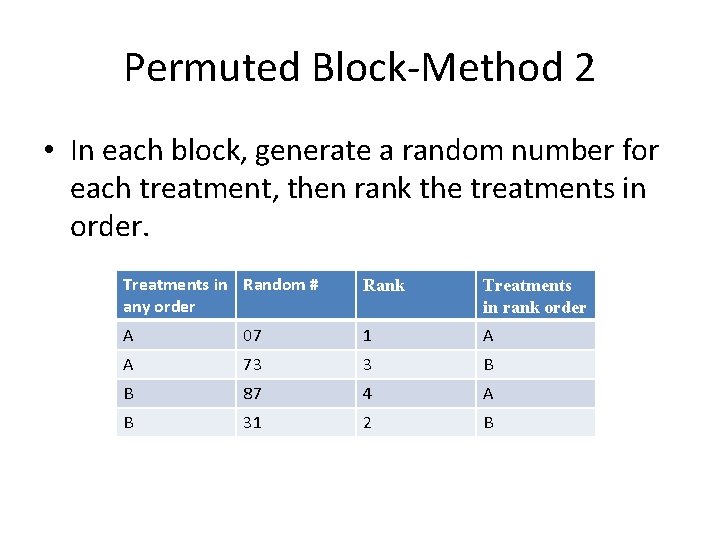
Permuted Block-Method 2 • In each block, generate a random number for each treatment, then rank the treatments in order. Treatments in Random # any order Rank Treatments in rank order A 07 1 A A 73 3 B B 87 4 A B 31 2 B
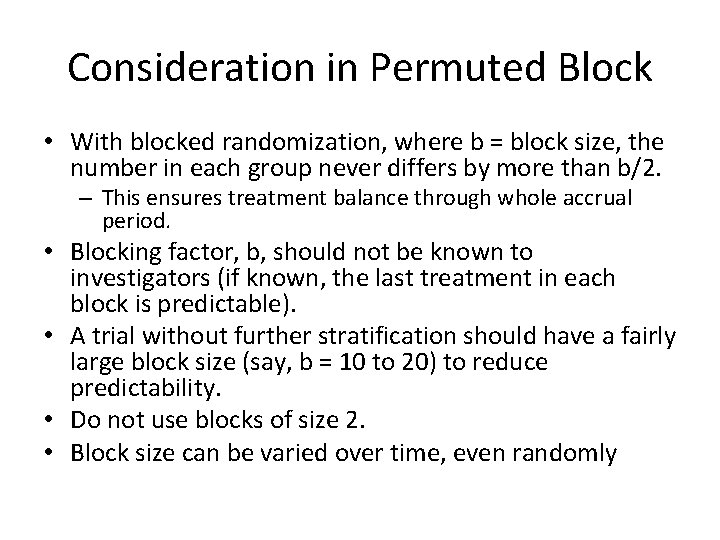
Consideration in Permuted Block • With blocked randomization, where b = block size, the number in each group never differs by more than b/2. – This ensures treatment balance through whole accrual period. • Blocking factor, b, should not be known to investigators (if known, the last treatment in each block is predictable). • A trial without further stratification should have a fairly large block size (say, b = 10 to 20) to reduce predictability. • Do not use blocks of size 2. • Block size can be varied over time, even randomly
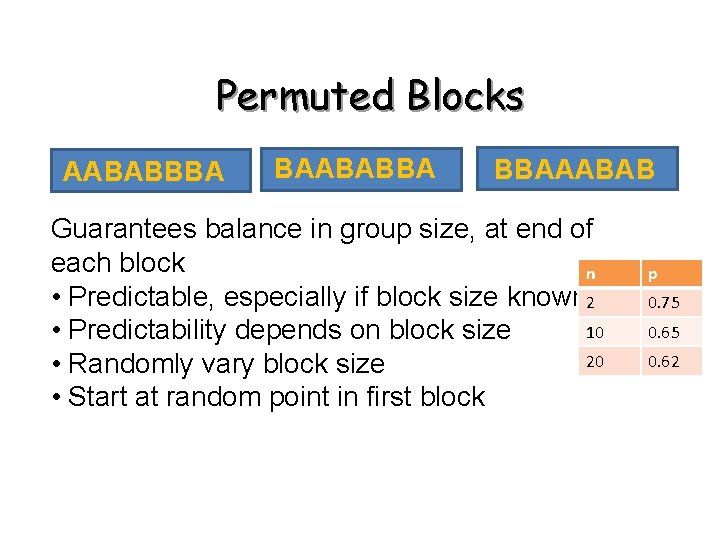
Permuted Blocks AABABBBA BAABABBA BBAAABAB Guarantees balance in group size, at end of each block n • Predictable, especially if block size known 2 10 • Predictability depends on block size 20 • Randomly vary block size • Start at random point in first block p 0. 75 0. 62
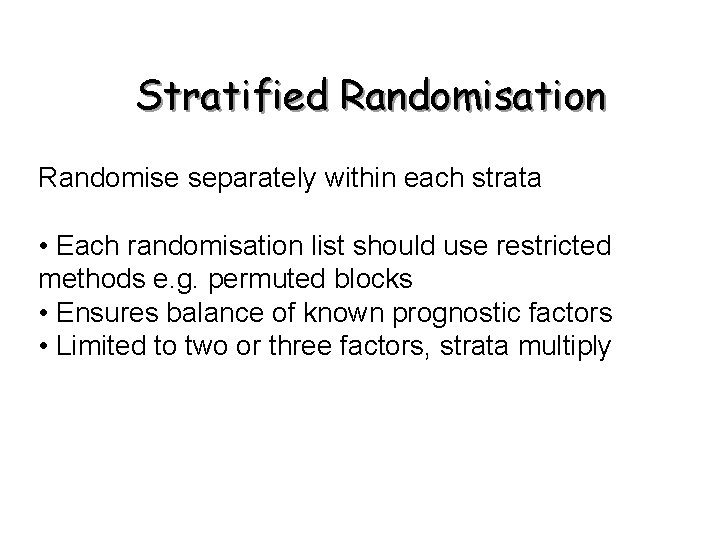
Stratified Randomisation Randomise separately within each strata • Each randomisation list should use restricted methods e. g. permuted blocks • Ensures balance of known prognostic factors • Limited to two or three factors, strata multiply
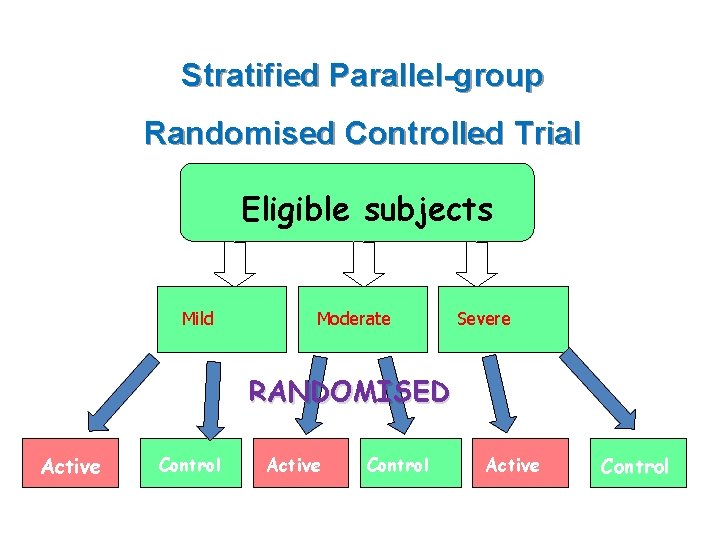
Stratified Parallel-group Randomised Controlled Trial Eligible subjects Stratum Mild Moderate Severe RANDOMISED Active Control
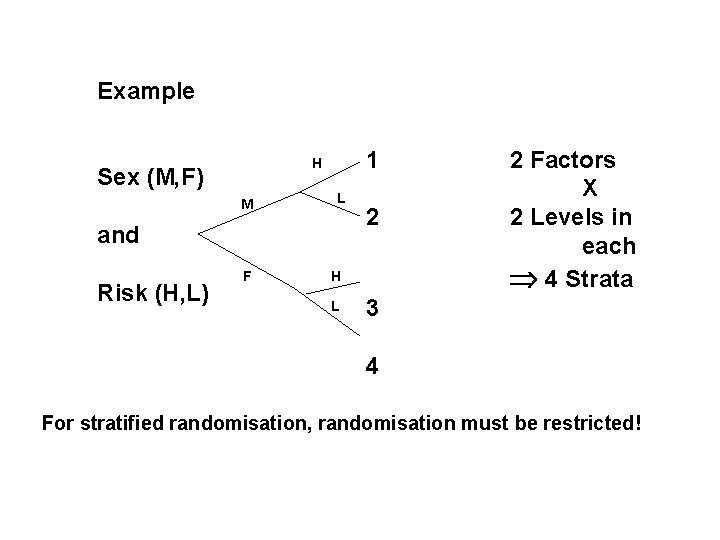
Example 1 H Sex (M, F) M L F H and Risk (H, L) L 2 2 Factors X 2 Levels in each 4 Strata 3 4 For stratified randomisation, randomisation must be restricted!
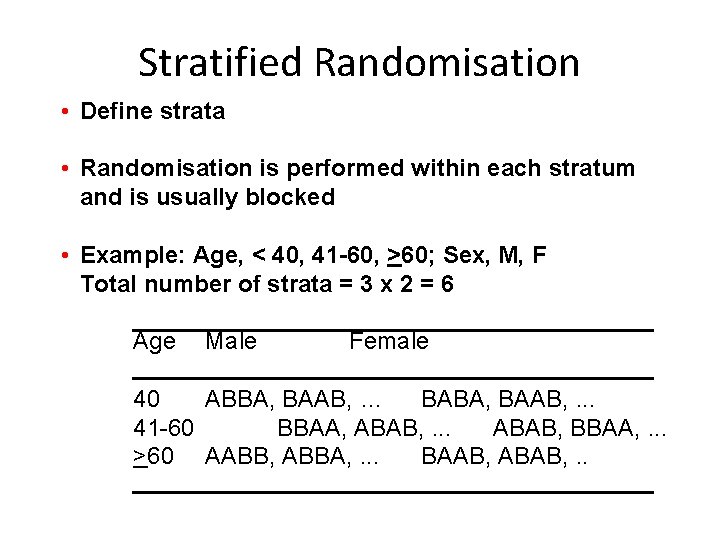
Stratified Randomisation • Define strata • Randomisation is performed within each stratum and is usually blocked • Example: Age, < 40, 41 -60, >60; Sex, M, F Total number of strata = 3 x 2 = 6 Age Male Female 40 ABBA, BAAB, … BABA, BAAB, . . . 41 -60 BBAA, ABAB, . . . ABAB, BBAA, . . . >60 AABB, ABBA, . . . BAAB, ABAB, . .
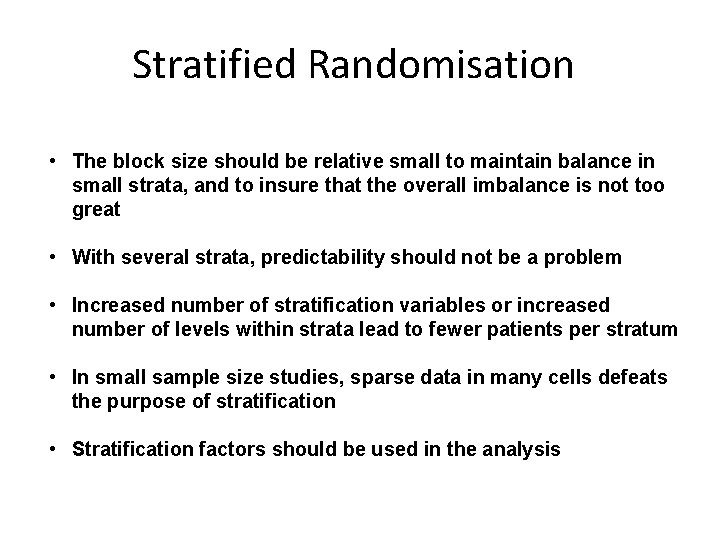
Stratified Randomisation • The block size should be relative small to maintain balance in small strata, and to insure that the overall imbalance is not too great • With several strata, predictability should not be a problem • Increased number of stratification variables or increased number of levels within strata lead to fewer patients per stratum • In small sample size studies, sparse data in many cells defeats the purpose of stratification • Stratification factors should be used in the analysis
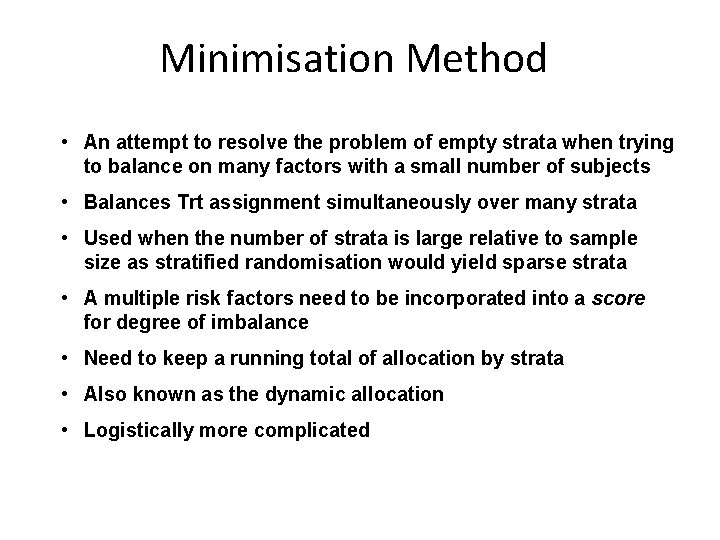
Minimisation Method • An attempt to resolve the problem of empty strata when trying to balance on many factors with a small number of subjects • Balances Trt assignment simultaneously over many strata • Used when the number of strata is large relative to sample size as stratified randomisation would yield sparse strata • A multiple risk factors need to be incorporated into a score for degree of imbalance • Need to keep a running total of allocation by strata • Also known as the dynamic allocation • Logistically more complicated
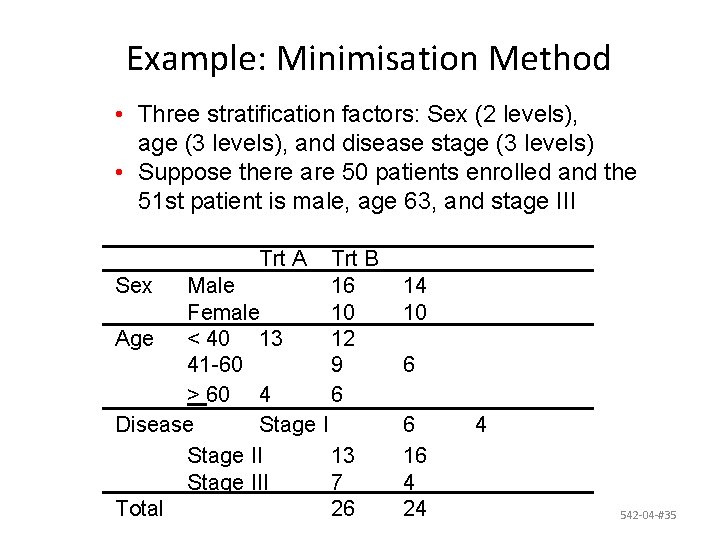
Example: Minimisation Method • Three stratification factors: Sex (2 levels), age (3 levels), and disease stage (3 levels) • Suppose there are 50 patients enrolled and the 51 st patient is male, age 63, and stage III Trt A Sex Trt B 16 10 12 9 6 Male Female Age < 40 13 41 -60 > 60 4 Disease Stage II 13 Stage III 7 Total 26 14 10 6 6 16 4 24 4 542 -04 -#35
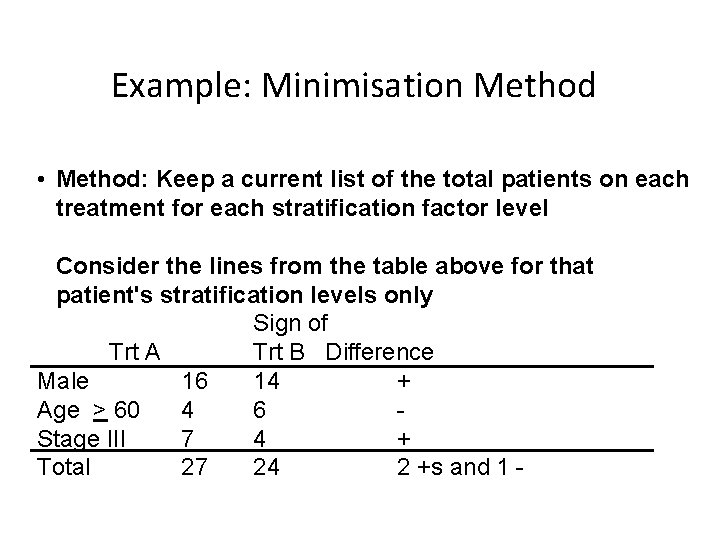
Example: Minimisation Method • Method: Keep a current list of the total patients on each treatment for each stratification factor level Consider the lines from the table above for that patient's stratification levels only Sign of Trt A Trt B Difference Male 16 14 + Age > 60 4 6 Stage III 7 4 + Total 27 24 2 +s and 1 -
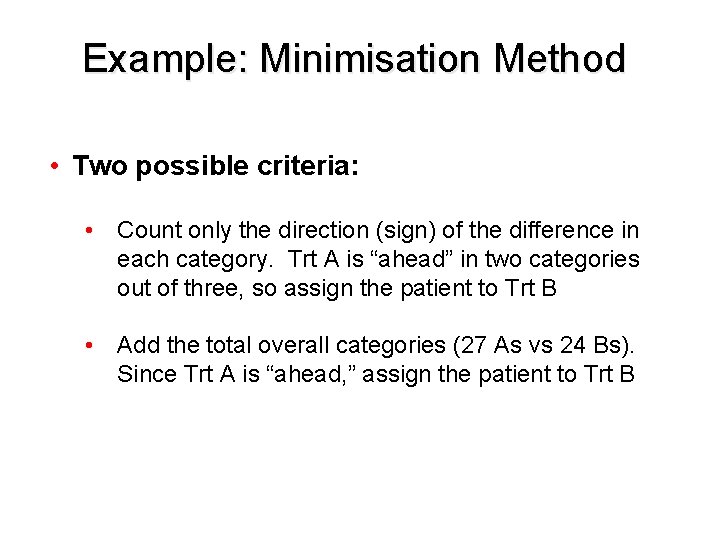
Example: Minimisation Method • Two possible criteria: • Count only the direction (sign) of the difference in each category. Trt A is “ahead” in two categories out of three, so assign the patient to Trt B • Add the total overall categories (27 As vs 24 Bs). Since Trt A is “ahead, ” assign the patient to Trt B
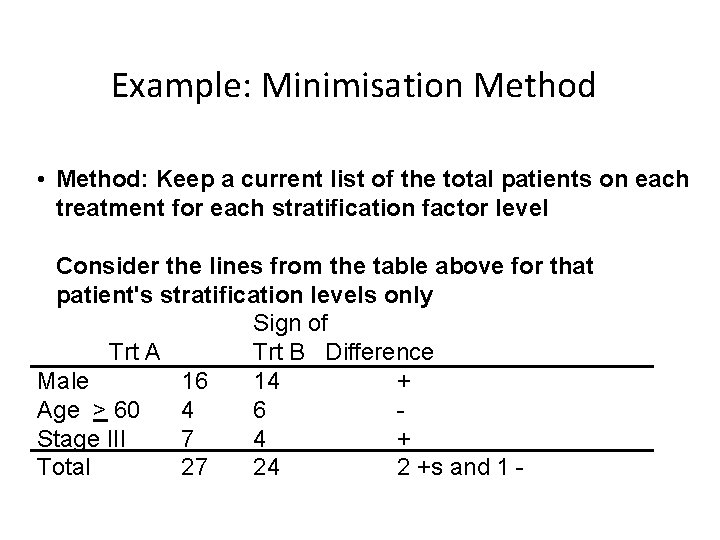
Example: Minimisation Method • Method: Keep a current list of the total patients on each treatment for each stratification factor level Consider the lines from the table above for that patient's stratification levels only Sign of Trt A Trt B Difference Male 16 14 + Age > 60 4 6 Stage III 7 4 + Total 27 24 2 +s and 1 -
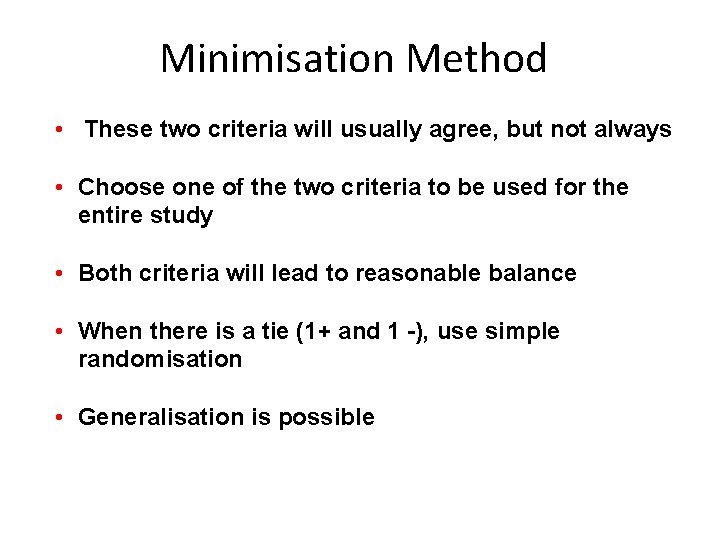
Minimisation Method • These two criteria will usually agree, but not always • Choose one of the two criteria to be used for the entire study • Both criteria will lead to reasonable balance • When there is a tie (1+ and 1 -), use simple randomisation • Generalisation is possible
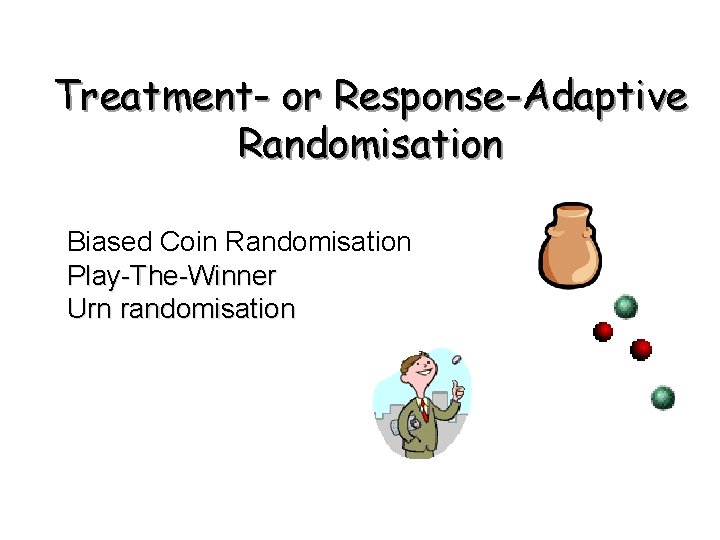
Treatment- or Response-Adaptive Randomisation Biased Coin Randomisation Play-The-Winner Urn randomisation
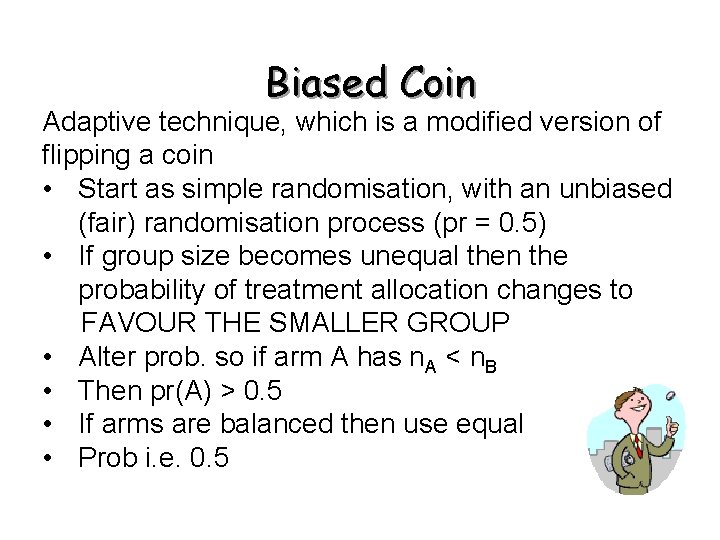
Biased Coin Adaptive technique, which is a modified version of flipping a coin • Start as simple randomisation, with an unbiased (fair) randomisation process (pr = 0. 5) • If group size becomes unequal then the probability of treatment allocation changes to FAVOUR THE SMALLER GROUP • Alter prob. so if arm A has n. A < n. B • Then pr(A) > 0. 5 • If arms are balanced then use equal • Prob i. e. 0. 5
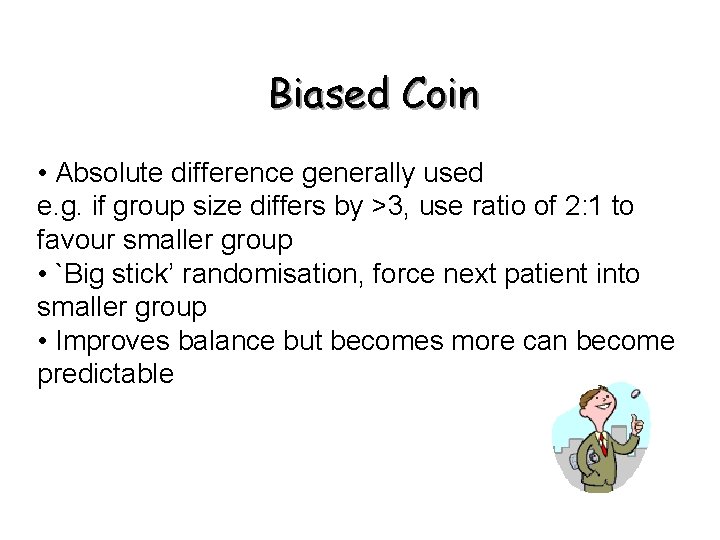
Biased Coin • Absolute difference generally used e. g. if group size differs by >3, use ratio of 2: 1 to favour smaller group • `Big stick’ randomisation, force next patient into smaller group • Improves balance but becomes more can become predictable
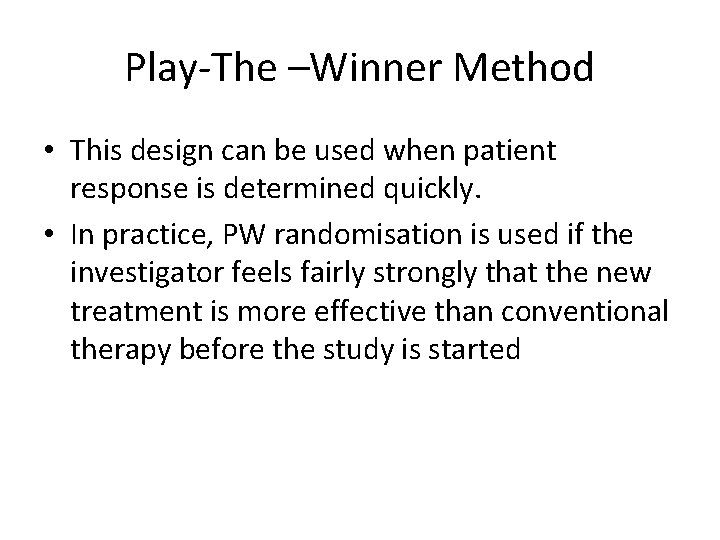
Play-The –Winner Method • This design can be used when patient response is determined quickly. • In practice, PW randomisation is used if the investigator feels fairly strongly that the new treatment is more effective than conventional therapy before the study is started
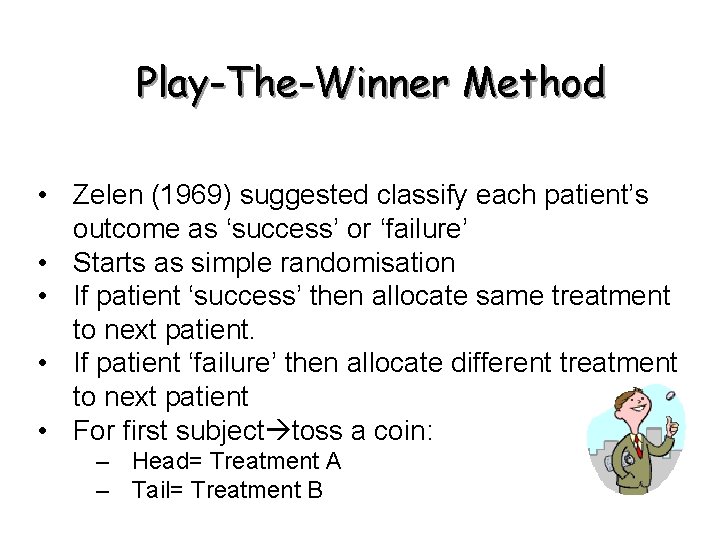
Play-The-Winner Method • Zelen (1969) suggested classify each patient’s outcome as ‘success’ or ‘failure’ • Starts as simple randomisation • If patient ‘success’ then allocate same treatment to next patient. • If patient ‘failure’ then allocate different treatment to next patient • For first subject toss a coin: – Head= Treatment A – Tail= Treatment B
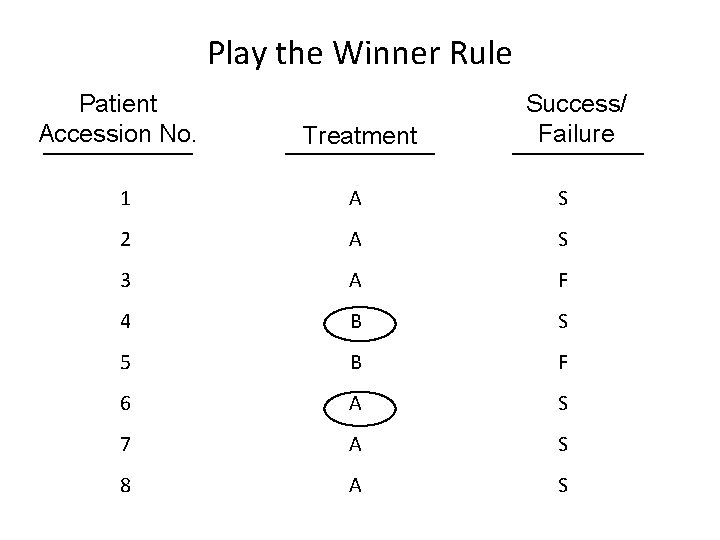
Play the Winner Rule Patient Accession No. Treatment Success/ Failure 1 A S 2 A S 3 A F 4 B S 5 B F 6 A S 7 A S 8 A S
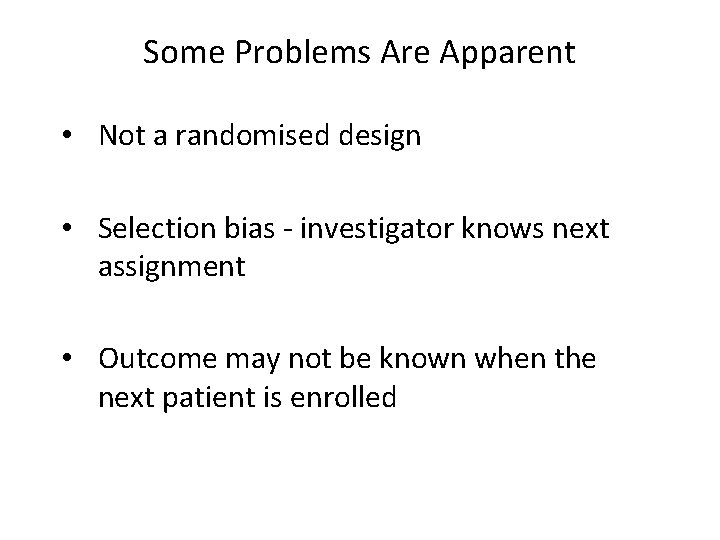
Some Problems Are Apparent • Not a randomised design • Selection bias - investigator knows next assignment • Outcome may not be known when the next patient is enrolled
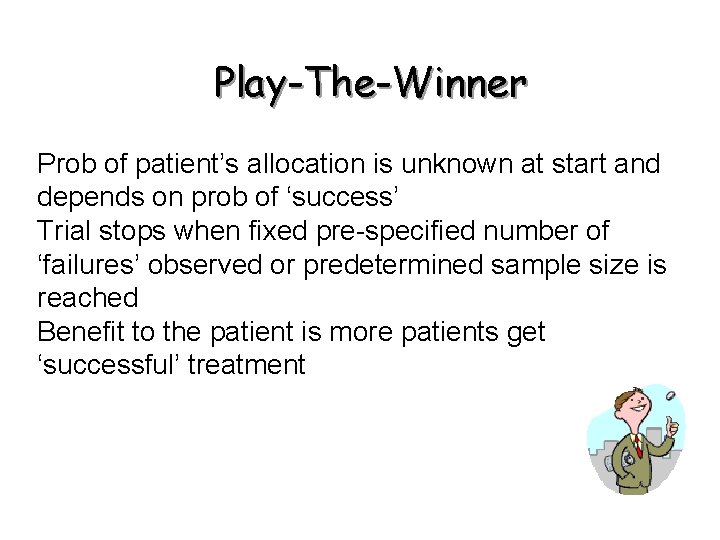
Play-The-Winner Prob of patient’s allocation is unknown at start and depends on prob of ‘success’ Trial stops when fixed pre-specified number of ‘failures’ observed or predetermined sample size is reached Benefit to the patient is more patients get ‘successful’ treatment
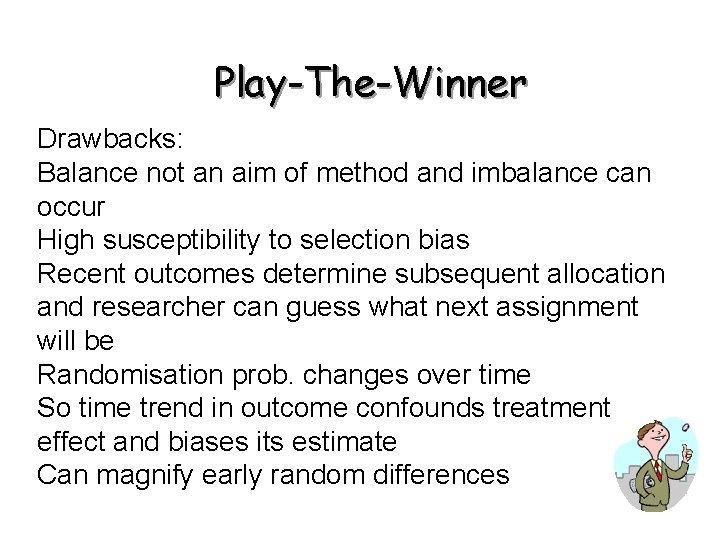
Play-The-Winner Drawbacks: Balance not an aim of method and imbalance can occur High susceptibility to selection bias Recent outcomes determine subsequent allocation and researcher can guess what next assignment will be Randomisation prob. changes over time So time trend in outcome confounds treatment effect and biases its estimate Can magnify early random differences
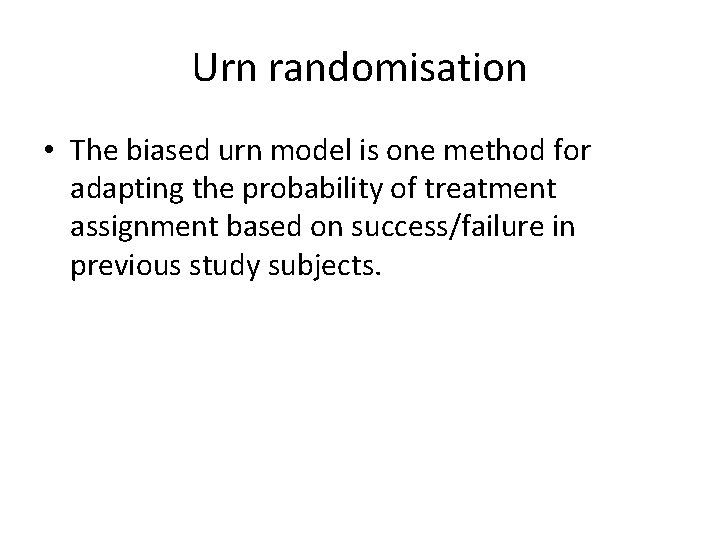
Urn randomisation • The biased urn model is one method for adapting the probability of treatment assignment based on success/failure in previous study subjects.
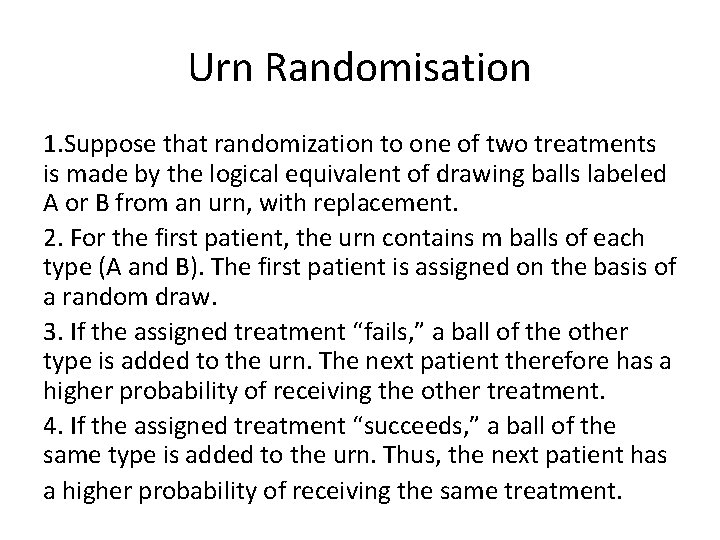
Urn Randomisation 1. Suppose that randomization to one of two treatments is made by the logical equivalent of drawing balls labeled A or B from an urn, with replacement. 2. For the first patient, the urn contains m balls of each type (A and B). The first patient is assigned on the basis of a random draw. 3. If the assigned treatment “fails, ” a ball of the other type is added to the urn. The next patient therefore has a higher probability of receiving the other treatment. 4. If the assigned treatment “succeeds, ” a ball of the same type is added to the urn. Thus, the next patient has a higher probability of receiving the same treatment.
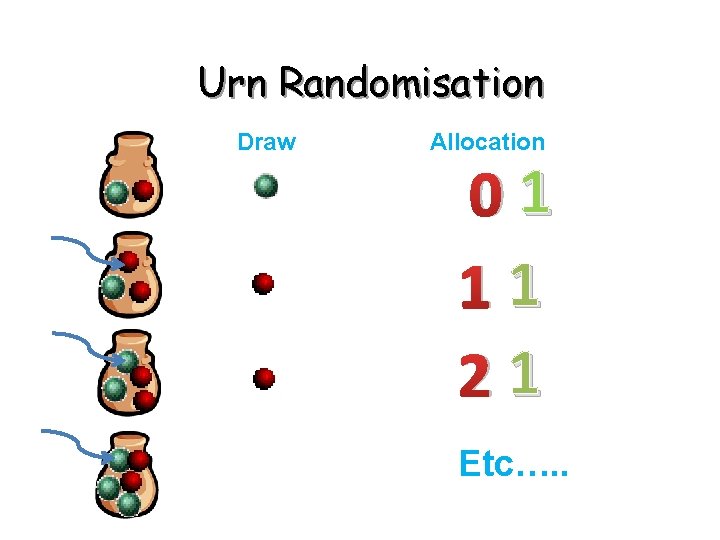
Urn Randomisation Draw Allocation 01 11 21 Etc…. .
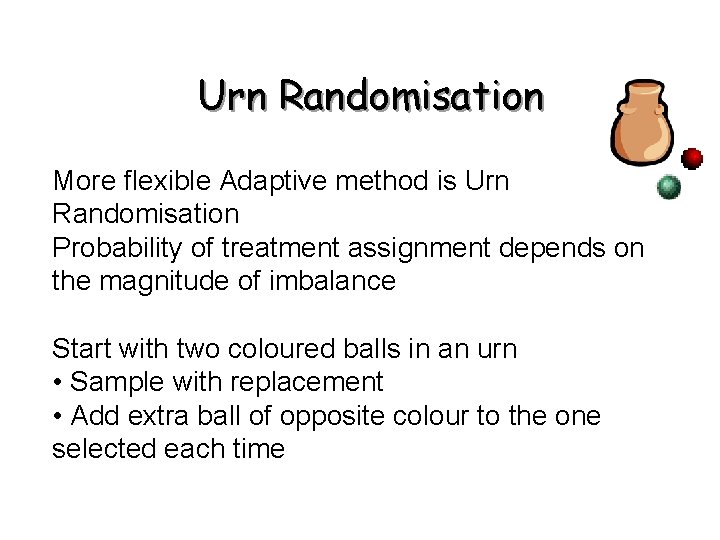
Urn Randomisation More flexible Adaptive method is Urn Randomisation Probability of treatment assignment depends on the magnitude of imbalance Start with two coloured balls in an urn • Sample with replacement • Add extra ball of opposite colour to the one selected each time
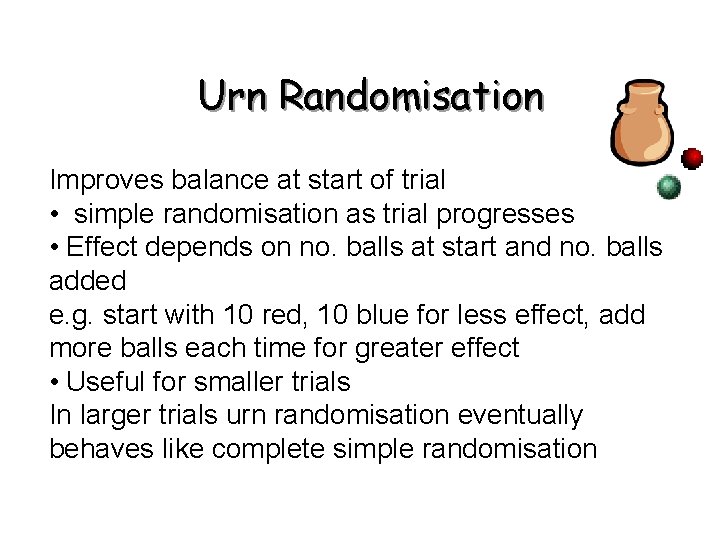
Urn Randomisation Improves balance at start of trial • simple randomisation as trial progresses • Effect depends on no. balls at start and no. balls added e. g. start with 10 red, 10 blue for less effect, add more balls each time for greater effect • Useful for smaller trials In larger trials urn randomisation eventually behaves like complete simple randomisation
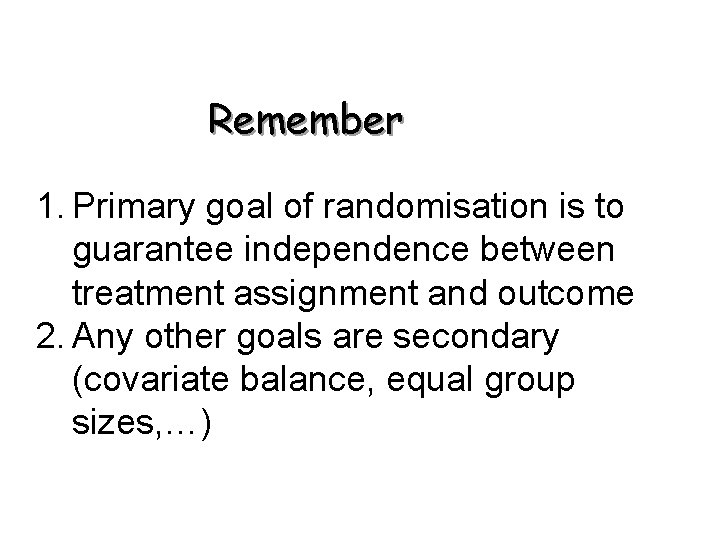
Remember 1. Primary goal of randomisation is to guarantee independence between treatment assignment and outcome 2. Any other goals are secondary (covariate balance, equal group sizes, …)
Randomisation en cluster
Stratified randomisation
Drug
Böritigin i. nasir han
Kadir ersin temizel
Dr kadir ceylan
Kadir erkan
Halkman
Hz ali kadir gecesi
Pengertian dari array/larik adalah?
Causal diagrams for epidemiologic research
Qisqa ko‘paytirish formulalari
Shahar kovalsky
Sparta davlati
Momaqaymoq sheri
Abdulla qahhor sarob romani
Anat shahar
Navoiy shahar 10-maktab
Kend heyati biography
Apa pengertian dari keberagaman
Metin ingeç hayatı
6. sınıf hz yahya hayatı
Pupuk feng shou untuk ternak
Taylor teoremi
Ahiret hayatı nedir
Template ppt keanekaragaman hayati
Afermat
Jelaskan nilai estetika keanekaragaman hayati
özel hukuk dalları
Pengertian keanekaragaman hayati
Kend hayati
Gambar keanekaragaman hayati tingkat jenis
Thomas edison hayatı slayt
Anders seneca
Hz yahya 6. sınıf
Hz isa nın hayatı 7. sınıf
Klasifikasi keanekaragaman hayati
Menelaus matematiğe katkıları
Hz ibrahim hayatı 5.sınıf
Mangal sefası karagöz hacivat
5 upaya pelestarian keanekaragaman hayati
Hz lokman oğluna verdiği öğütler
Yunus emrenin hayatı slayt
Samad hayati
Soyadı sayın olan sanatçılar
Seyreyledim eşkal-i hayatı ben havz-ı hayalin sularında
Kend hayati
Kend hayati
Masatoşi gündüz ikeda eserleri
Mustafa kemalin çocukluk dönemi ve öğrenim hayatı
Leonardo fibonacci kimdir
Kend hayati
Kend hayati
Indikator keanekaragaman hayati
Piri reisin hayatı