confidence measures for speech recognition in Euro Speech
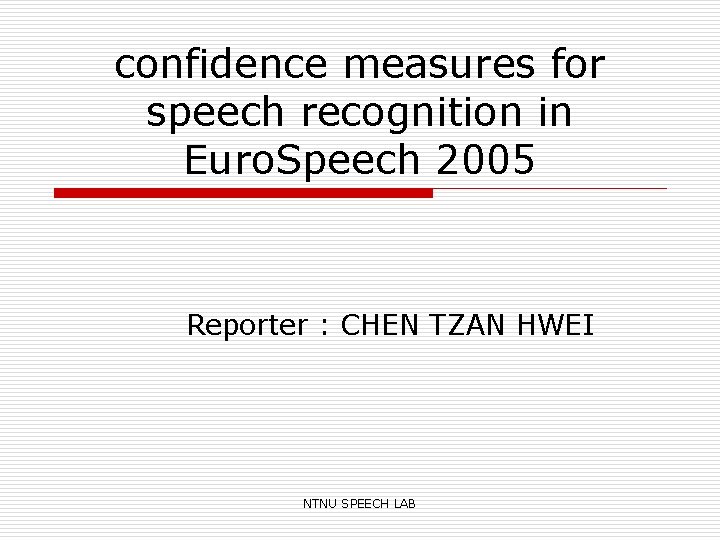
![Reference o o o [1] B. Dong, Q. Zhao and Y. Yan, “A fast Reference o o o [1] B. Dong, Q. Zhao and Y. Yan, “A fast](https://slidetodoc.com/presentation_image/d56784347b19e83bbe834b5dcfe341b5/image-2.jpg)
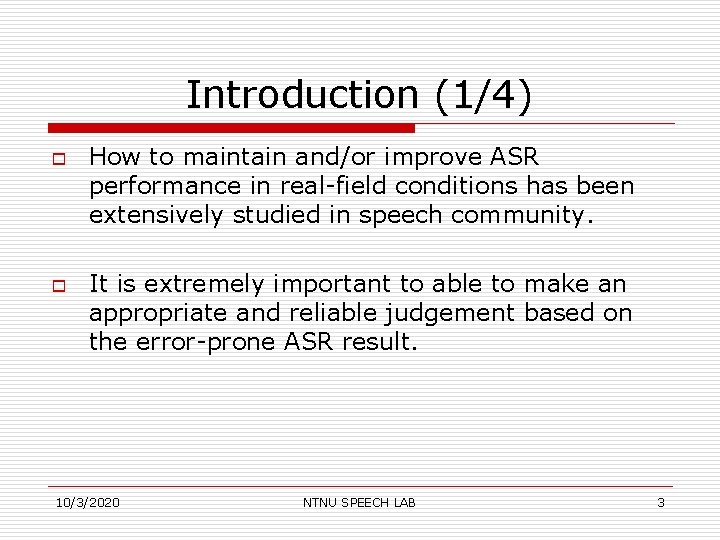
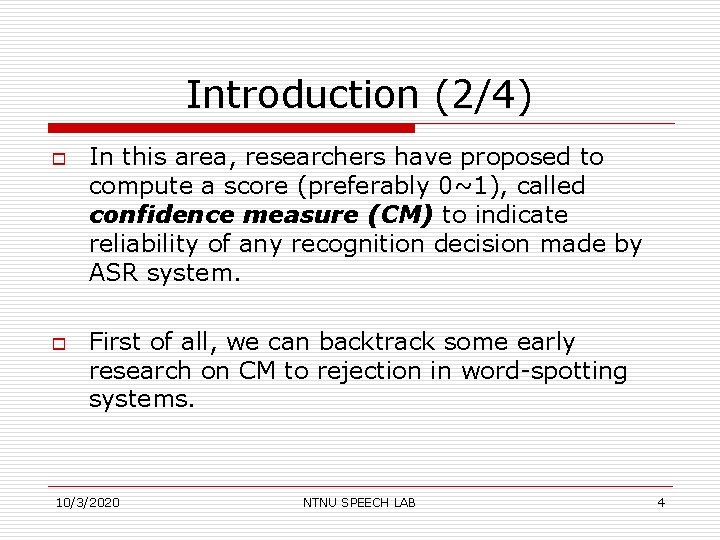
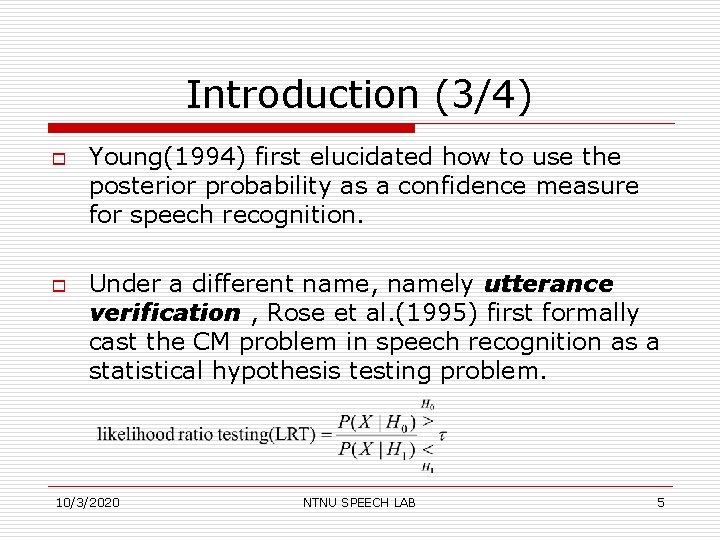
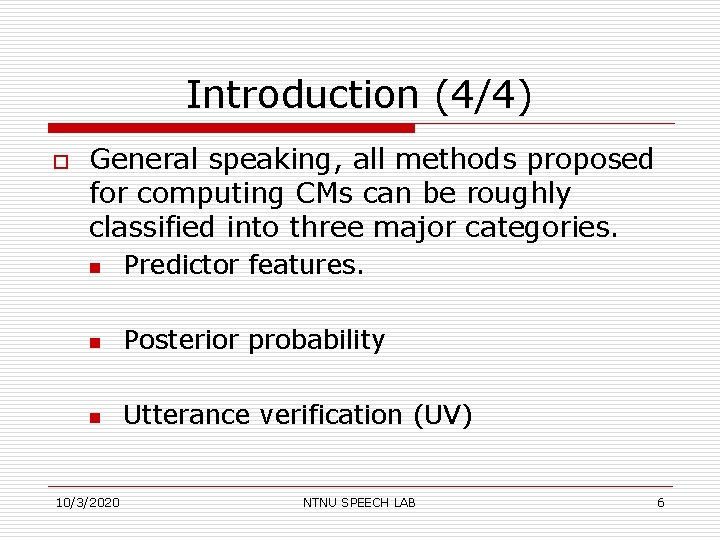
![[1] method (1/4) o o in this paper, posterior probability of each state is [1] method (1/4) o o in this paper, posterior probability of each state is](https://slidetodoc.com/presentation_image/d56784347b19e83bbe834b5dcfe341b5/image-7.jpg)
![[1] method (2/4) o o The posterior probability of each phoneme : Traditional two-pass [1] method (2/4) o o The posterior probability of each phoneme : Traditional two-pass](https://slidetodoc.com/presentation_image/d56784347b19e83bbe834b5dcfe341b5/image-8.jpg)
![[1] method (3/4) o One-pass synchronous calculating algorithm for CM 10/3/2020 NTNU SPEECH LAB [1] method (3/4) o One-pass synchronous calculating algorithm for CM 10/3/2020 NTNU SPEECH LAB](https://slidetodoc.com/presentation_image/d56784347b19e83bbe834b5dcfe341b5/image-9.jpg)
![[1] method (4/4) 10/3/2020 NTNU SPEECH LAB 10 [1] method (4/4) 10/3/2020 NTNU SPEECH LAB 10](https://slidetodoc.com/presentation_image/d56784347b19e83bbe834b5dcfe341b5/image-10.jpg)
![[1] experiments (1/2) o o The task is to evaluate name recognition accuracy with [1] experiments (1/2) o o The task is to evaluate name recognition accuracy with](https://slidetodoc.com/presentation_image/d56784347b19e83bbe834b5dcfe341b5/image-11.jpg)
![[1] experiments (2/2) 10/3/2020 NTNU SPEECH LAB 12 [1] experiments (2/2) 10/3/2020 NTNU SPEECH LAB 12](https://slidetodoc.com/presentation_image/d56784347b19e83bbe834b5dcfe341b5/image-12.jpg)
![[2] method (1/5) o Word graph sparseness in CFG based ASR 10/3/2020 NTNU SPEECH [2] method (1/5) o Word graph sparseness in CFG based ASR 10/3/2020 NTNU SPEECH](https://slidetodoc.com/presentation_image/d56784347b19e83bbe834b5dcfe341b5/image-13.jpg)
![[2] method (2/5) o However, in some CFG constrained ASR application, the lexical and [2] method (2/5) o However, in some CFG constrained ASR application, the lexical and](https://slidetodoc.com/presentation_image/d56784347b19e83bbe834b5dcfe341b5/image-14.jpg)
![[2] method (3/5) o o To alleviate this graph sparseness problem Based on background [2] method (3/5) o o To alleviate this graph sparseness problem Based on background](https://slidetodoc.com/presentation_image/d56784347b19e83bbe834b5dcfe341b5/image-15.jpg)
![[2] method (4/5) o o For each arc in the background model graph Finally, [2] method (4/5) o o For each arc in the background model graph Finally,](https://slidetodoc.com/presentation_image/d56784347b19e83bbe834b5dcfe341b5/image-16.jpg)
![[2] method (5/5) o Background model selection n Phoneme based background models o o [2] method (5/5) o Background model selection n Phoneme based background models o o](https://slidetodoc.com/presentation_image/d56784347b19e83bbe834b5dcfe341b5/image-17.jpg)
![[2] experiment (1/4) o The CFG are built with all legal phrases arranged in [2] experiment (1/4) o The CFG are built with all legal phrases arranged in](https://slidetodoc.com/presentation_image/d56784347b19e83bbe834b5dcfe341b5/image-18.jpg)
![[2] experiment (2/4) o The rejection performance 10/3/2020 NTNU SPEECH LAB 19 [2] experiment (2/4) o The rejection performance 10/3/2020 NTNU SPEECH LAB 19](https://slidetodoc.com/presentation_image/d56784347b19e83bbe834b5dcfe341b5/image-19.jpg)
![[2] experiment (3/4) o Recognition tests o Syllable set selection in English 10/3/2020 NTNU [2] experiment (3/4) o Recognition tests o Syllable set selection in English 10/3/2020 NTNU](https://slidetodoc.com/presentation_image/d56784347b19e83bbe834b5dcfe341b5/image-20.jpg)
![[2] experiment (4/4) o Rejection tests : in MPP tests, the background model graphs [2] experiment (4/4) o Rejection tests : in MPP tests, the background model graphs](https://slidetodoc.com/presentation_image/d56784347b19e83bbe834b5dcfe341b5/image-21.jpg)
![[3] Method (1/4) o Typical spoken language systems consist : n n 10/3/2020 An [3] Method (1/4) o Typical spoken language systems consist : n n 10/3/2020 An](https://slidetodoc.com/presentation_image/d56784347b19e83bbe834b5dcfe341b5/image-22.jpg)
![[3] Method (2/4) o In-domain confidence 10/3/2020 NTNU SPEECH LAB 23 [3] Method (2/4) o In-domain confidence 10/3/2020 NTNU SPEECH LAB 23](https://slidetodoc.com/presentation_image/d56784347b19e83bbe834b5dcfe341b5/image-23.jpg)
![[3] Method (3/4) o o Discourse coherence : based on topic consistency across consecutive [3] Method (3/4) o o Discourse coherence : based on topic consistency across consecutive](https://slidetodoc.com/presentation_image/d56784347b19e83bbe834b5dcfe341b5/image-24.jpg)
![[3] Method (4/4) o Joint confidence by combining multiple measures 10/3/2020 NTNU SPEECH LAB [3] Method (4/4) o Joint confidence by combining multiple measures 10/3/2020 NTNU SPEECH LAB](https://slidetodoc.com/presentation_image/d56784347b19e83bbe834b5dcfe341b5/image-25.jpg)
![[3] Experiments (1/2) o The performance was evaluated on spontaneous dialogue via the ATR [3] Experiments (1/2) o The performance was evaluated on spontaneous dialogue via the ATR](https://slidetodoc.com/presentation_image/d56784347b19e83bbe834b5dcfe341b5/image-26.jpg)
![[3] Experiments (2/2) 10/3/2020 NTNU SPEECH LAB 27 [3] Experiments (2/2) 10/3/2020 NTNU SPEECH LAB 27](https://slidetodoc.com/presentation_image/d56784347b19e83bbe834b5dcfe341b5/image-27.jpg)
- Slides: 27
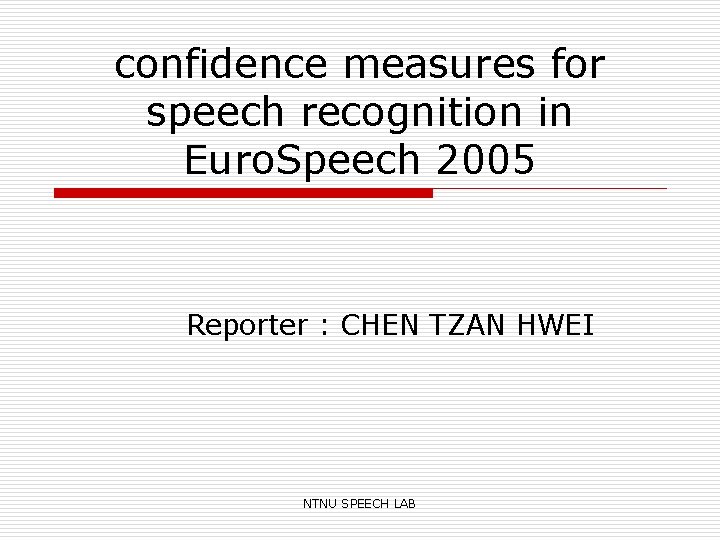
confidence measures for speech recognition in Euro. Speech 2005 Reporter : CHEN TZAN HWEI NTNU SPEECH LAB
![Reference o o o 1 B Dong Q Zhao and Y Yan A fast Reference o o o [1] B. Dong, Q. Zhao and Y. Yan, “A fast](https://slidetodoc.com/presentation_image/d56784347b19e83bbe834b5dcfe341b5/image-2.jpg)
Reference o o o [1] B. Dong, Q. Zhao and Y. Yan, “A fast confidence measure algorithm for continuous speech recognition” [2]P Liu, Y. Tian, J. -L. Zhou and F. K. Soong, “Background model based posterior probability for measuring confidence” [3]I. R Lane and T. Kawahara, “Utterance Verification Incorporating In-Domain Confidence and Discourse Measures. ” 10/3/2020 NTNU SPEECH LAB 2
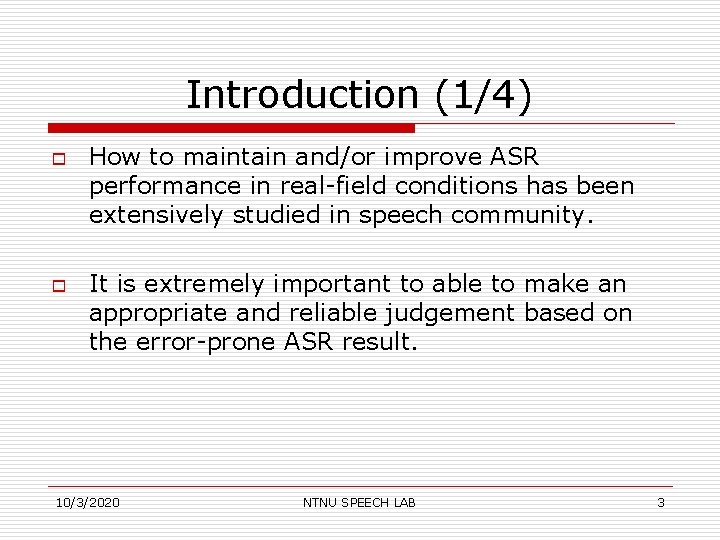
Introduction (1/4) o o How to maintain and/or improve ASR performance in real-field conditions has been extensively studied in speech community. It is extremely important to able to make an appropriate and reliable judgement based on the error-prone ASR result. 10/3/2020 NTNU SPEECH LAB 3
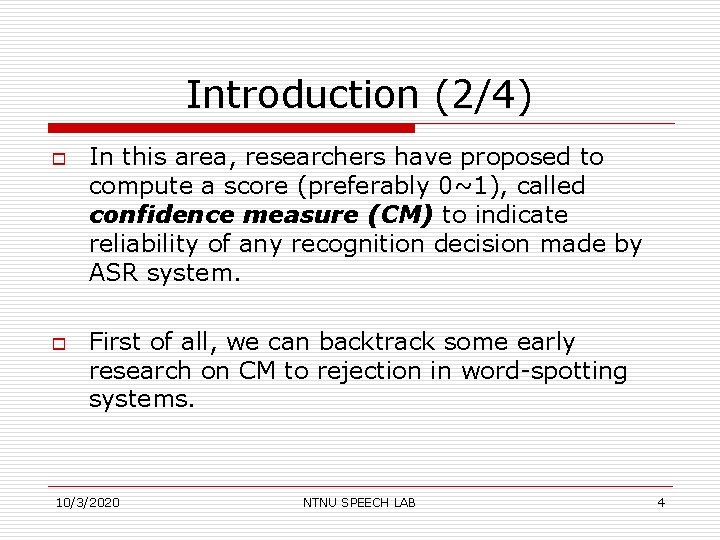
Introduction (2/4) o o In this area, researchers have proposed to compute a score (preferably 0~1), called confidence measure (CM) to indicate reliability of any recognition decision made by ASR system. First of all, we can backtrack some early research on CM to rejection in word-spotting systems. 10/3/2020 NTNU SPEECH LAB 4
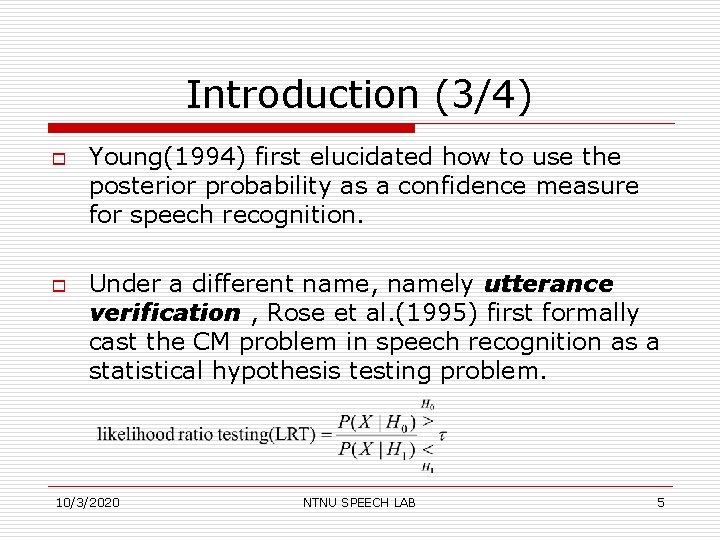
Introduction (3/4) o o Young(1994) first elucidated how to use the posterior probability as a confidence measure for speech recognition. Under a different name, namely utterance verification , Rose et al. (1995) first formally cast the CM problem in speech recognition as a statistical hypothesis testing problem. 10/3/2020 NTNU SPEECH LAB 5
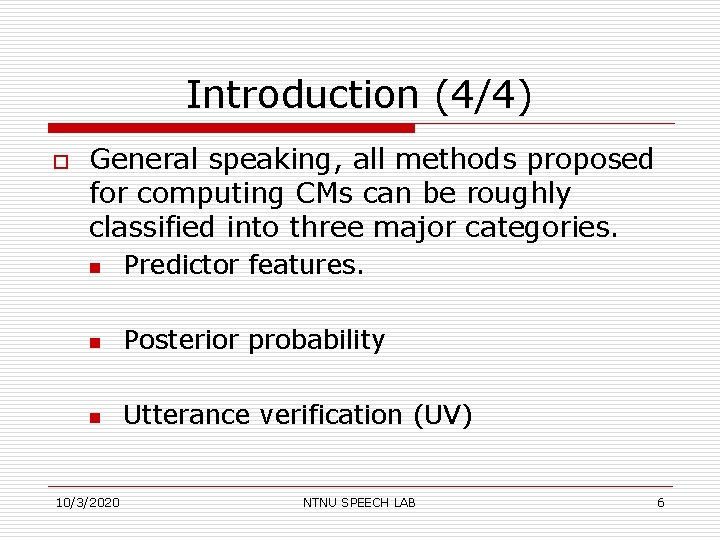
Introduction (4/4) o General speaking, all methods proposed for computing CMs can be roughly classified into three major categories. n Predictor features. n Posterior probability n Utterance verification (UV) 10/3/2020 NTNU SPEECH LAB 6
![1 method 14 o o in this paper posterior probability of each state is [1] method (1/4) o o in this paper, posterior probability of each state is](https://slidetodoc.com/presentation_image/d56784347b19e83bbe834b5dcfe341b5/image-7.jpg)
[1] method (1/4) o o in this paper, posterior probability of each state is used as the feature for the confidence measure During decoding, the time information at each state can be retrieve, the posterior probability is normalized 10/3/2020 NTNU SPEECH LAB 7
![1 method 24 o o The posterior probability of each phoneme Traditional twopass [1] method (2/4) o o The posterior probability of each phoneme : Traditional two-pass](https://slidetodoc.com/presentation_image/d56784347b19e83bbe834b5dcfe341b5/image-8.jpg)
[1] method (2/4) o o The posterior probability of each phoneme : Traditional two-pass method for calculating confidence 10/3/2020 NTNU SPEECH LAB 8
![1 method 34 o Onepass synchronous calculating algorithm for CM 1032020 NTNU SPEECH LAB [1] method (3/4) o One-pass synchronous calculating algorithm for CM 10/3/2020 NTNU SPEECH LAB](https://slidetodoc.com/presentation_image/d56784347b19e83bbe834b5dcfe341b5/image-9.jpg)
[1] method (3/4) o One-pass synchronous calculating algorithm for CM 10/3/2020 NTNU SPEECH LAB 9
![1 method 44 1032020 NTNU SPEECH LAB 10 [1] method (4/4) 10/3/2020 NTNU SPEECH LAB 10](https://slidetodoc.com/presentation_image/d56784347b19e83bbe834b5dcfe341b5/image-10.jpg)
[1] method (4/4) 10/3/2020 NTNU SPEECH LAB 10
![1 experiments 12 o o The task is to evaluate name recognition accuracy with [1] experiments (1/2) o o The task is to evaluate name recognition accuracy with](https://slidetodoc.com/presentation_image/d56784347b19e83bbe834b5dcfe341b5/image-11.jpg)
[1] experiments (1/2) o o The task is to evaluate name recognition accuracy with a test set size of 1278 names. In the test set, 180 names are out-ofdomain utterances. 10/3/2020 NTNU SPEECH LAB 11
![1 experiments 22 1032020 NTNU SPEECH LAB 12 [1] experiments (2/2) 10/3/2020 NTNU SPEECH LAB 12](https://slidetodoc.com/presentation_image/d56784347b19e83bbe834b5dcfe341b5/image-12.jpg)
[1] experiments (2/2) 10/3/2020 NTNU SPEECH LAB 12
![2 method 15 o Word graph sparseness in CFG based ASR 1032020 NTNU SPEECH [2] method (1/5) o Word graph sparseness in CFG based ASR 10/3/2020 NTNU SPEECH](https://slidetodoc.com/presentation_image/d56784347b19e83bbe834b5dcfe341b5/image-13.jpg)
[2] method (1/5) o Word graph sparseness in CFG based ASR 10/3/2020 NTNU SPEECH LAB 13
![2 method 25 o However in some CFG constrained ASR application the lexical and [2] method (2/5) o However, in some CFG constrained ASR application, the lexical and](https://slidetodoc.com/presentation_image/d56784347b19e83bbe834b5dcfe341b5/image-14.jpg)
[2] method (2/5) o However, in some CFG constrained ASR application, the lexical and language model constraints can limit the number of hypotheses. 10/3/2020 NTNU SPEECH LAB 14
![2 method 35 o o To alleviate this graph sparseness problem Based on background [2] method (3/5) o o To alleviate this graph sparseness problem Based on background](https://slidetodoc.com/presentation_image/d56784347b19e83bbe834b5dcfe341b5/image-15.jpg)
[2] method (3/5) o o To alleviate this graph sparseness problem Based on background model graph, Model based Posterior Probability (MPP) can be calculated. 10/3/2020 NTNU SPEECH LAB 15
![2 method 45 o o For each arc in the background model graph Finally [2] method (4/5) o o For each arc in the background model graph Finally,](https://slidetodoc.com/presentation_image/d56784347b19e83bbe834b5dcfe341b5/image-16.jpg)
[2] method (4/5) o o For each arc in the background model graph Finally, MPP is normalized by the total number of 10/3/2020 NTNU SPEECH LAB 16
![2 method 55 o Background model selection n Phoneme based background models o o [2] method (5/5) o Background model selection n Phoneme based background models o o](https://slidetodoc.com/presentation_image/d56784347b19e83bbe834b5dcfe341b5/image-17.jpg)
[2] method (5/5) o Background model selection n Phoneme based background models o o n Syllable based background models o o 10/3/2020 40 phonemes in English There around 70 toneless initials and finals in Chinese In Chinese, there are only about 400 syllables. For English, the number of syllables exceeds 15, 000 NTNU SPEECH LAB 17
![2 experiment 14 o The CFG are built with all legal phrases arranged in [2] experiment (1/4) o The CFG are built with all legal phrases arranged in](https://slidetodoc.com/presentation_image/d56784347b19e83bbe834b5dcfe341b5/image-18.jpg)
[2] experiment (1/4) o The CFG are built with all legal phrases arranged in parallel 10/3/2020 NTNU SPEECH LAB 18
![2 experiment 24 o The rejection performance 1032020 NTNU SPEECH LAB 19 [2] experiment (2/4) o The rejection performance 10/3/2020 NTNU SPEECH LAB 19](https://slidetodoc.com/presentation_image/d56784347b19e83bbe834b5dcfe341b5/image-19.jpg)
[2] experiment (2/4) o The rejection performance 10/3/2020 NTNU SPEECH LAB 19
![2 experiment 34 o Recognition tests o Syllable set selection in English 1032020 NTNU [2] experiment (3/4) o Recognition tests o Syllable set selection in English 10/3/2020 NTNU](https://slidetodoc.com/presentation_image/d56784347b19e83bbe834b5dcfe341b5/image-20.jpg)
[2] experiment (3/4) o Recognition tests o Syllable set selection in English 10/3/2020 NTNU SPEECH LAB 20
![2 experiment 44 o Rejection tests in MPP tests the background model graphs [2] experiment (4/4) o Rejection tests : in MPP tests, the background model graphs](https://slidetodoc.com/presentation_image/d56784347b19e83bbe834b5dcfe341b5/image-21.jpg)
[2] experiment (4/4) o Rejection tests : in MPP tests, the background model graphs were generated by a decoder with 4 tokes. 10/3/2020 NTNU SPEECH LAB 21
![3 Method 14 o Typical spoken language systems consist n n 1032020 An [3] Method (1/4) o Typical spoken language systems consist : n n 10/3/2020 An](https://slidetodoc.com/presentation_image/d56784347b19e83bbe834b5dcfe341b5/image-22.jpg)
[3] Method (1/4) o Typical spoken language systems consist : n n 10/3/2020 An ASR front-end A NLP back-end NTNU SPEECH LAB 22
![3 Method 24 o Indomain confidence 1032020 NTNU SPEECH LAB 23 [3] Method (2/4) o In-domain confidence 10/3/2020 NTNU SPEECH LAB 23](https://slidetodoc.com/presentation_image/d56784347b19e83bbe834b5dcfe341b5/image-23.jpg)
[3] Method (2/4) o In-domain confidence 10/3/2020 NTNU SPEECH LAB 23
![3 Method 34 o o Discourse coherence based on topic consistency across consecutive [3] Method (3/4) o o Discourse coherence : based on topic consistency across consecutive](https://slidetodoc.com/presentation_image/d56784347b19e83bbe834b5dcfe341b5/image-24.jpg)
[3] Method (3/4) o o Discourse coherence : based on topic consistency across consecutive utterances We adopt an inter-utterance distance based on the topic consistency between two utterance 10/3/2020 NTNU SPEECH LAB 24
![3 Method 44 o Joint confidence by combining multiple measures 1032020 NTNU SPEECH LAB [3] Method (4/4) o Joint confidence by combining multiple measures 10/3/2020 NTNU SPEECH LAB](https://slidetodoc.com/presentation_image/d56784347b19e83bbe834b5dcfe341b5/image-25.jpg)
[3] Method (4/4) o Joint confidence by combining multiple measures 10/3/2020 NTNU SPEECH LAB 25
![3 Experiments 12 o The performance was evaluated on spontaneous dialogue via the ATR [3] Experiments (1/2) o The performance was evaluated on spontaneous dialogue via the ATR](https://slidetodoc.com/presentation_image/d56784347b19e83bbe834b5dcfe341b5/image-26.jpg)
[3] Experiments (1/2) o The performance was evaluated on spontaneous dialogue via the ATR speechto-speech translation system. 10/3/2020 NTNU SPEECH LAB 26
![3 Experiments 22 1032020 NTNU SPEECH LAB 27 [3] Experiments (2/2) 10/3/2020 NTNU SPEECH LAB 27](https://slidetodoc.com/presentation_image/d56784347b19e83bbe834b5dcfe341b5/image-27.jpg)
[3] Experiments (2/2) 10/3/2020 NTNU SPEECH LAB 27
Confidence interval vs confidence level
Confidence interval z value
Repeated measures design vs independent measures design
Htk speech recognition tutorial
Electron speech recognition
Julia speech recognition
Speech recognition
Fundamentals of speech recognition
Speech recognition presentation
Speech recognition app inventor
Deep learning speech recognition
Speech recognition software
Dragon speech recognition
Ionic speech recognition
Cmu speech recognition
Kinect for windows speech recognition language pack
Confidence part of speech
Atmosfr
Programskede byggprocessen
Rutin för avvikelsehantering
Vanlig celldelning
Presentera för publik crossboss
Rådet för byggkompetens
Myndigheten för delaktighet
Kung dog 1611
Densitet vatten
Tobinskatten för och nackdelar
Tack för att ni lyssnade bild