Computation of LargeScale Genomic Evaluations Paul Van Raden
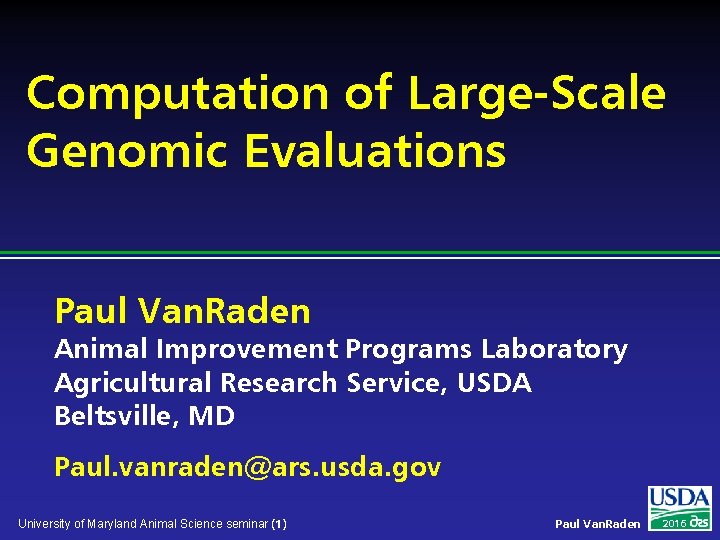
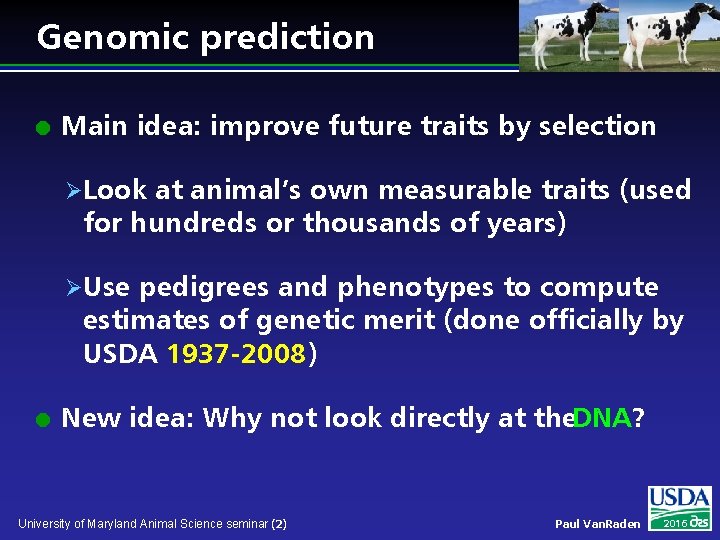
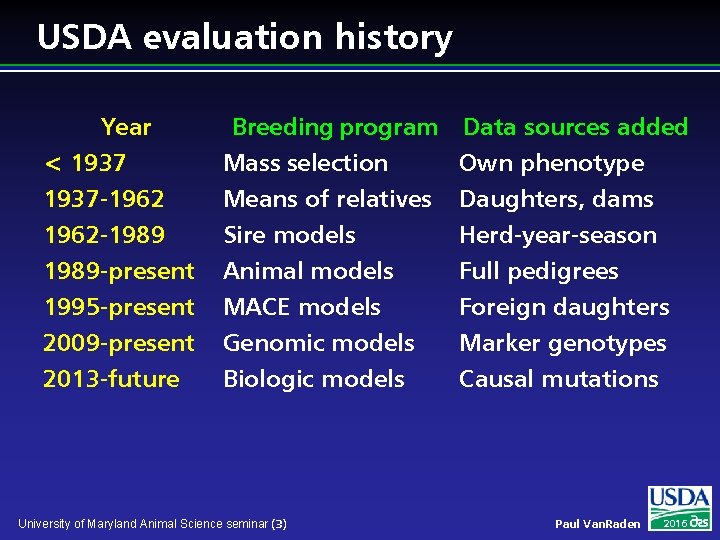
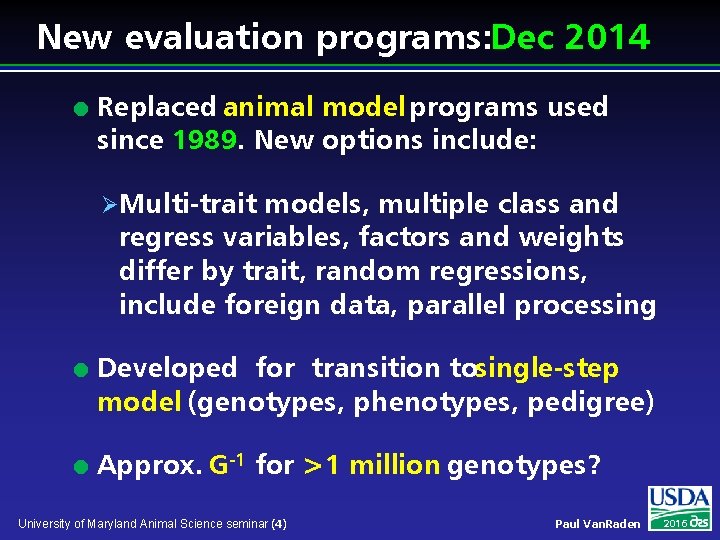
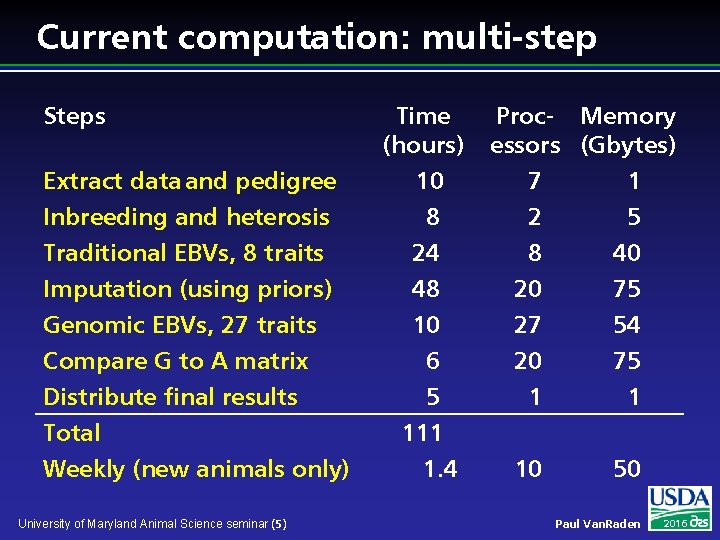
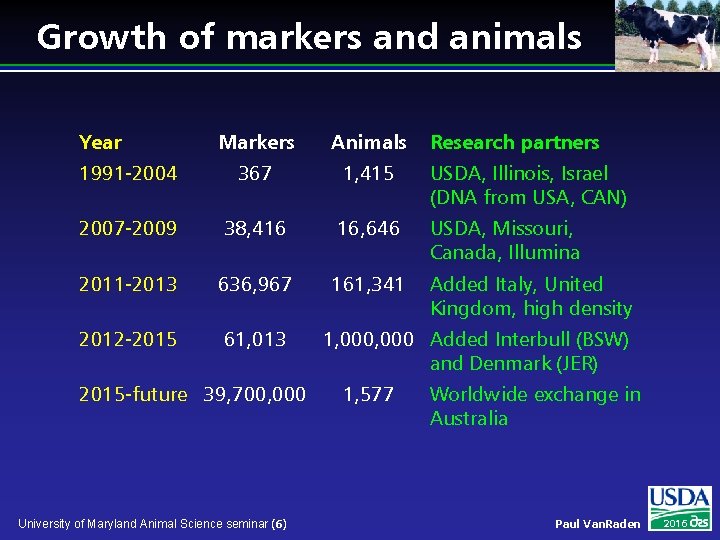
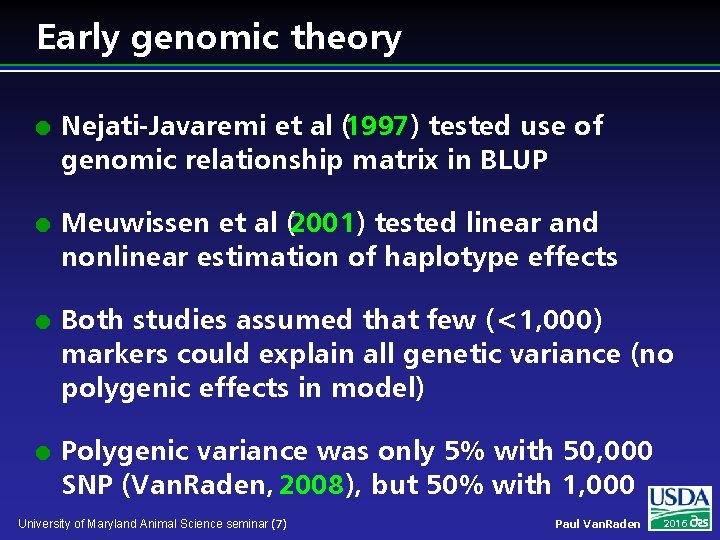
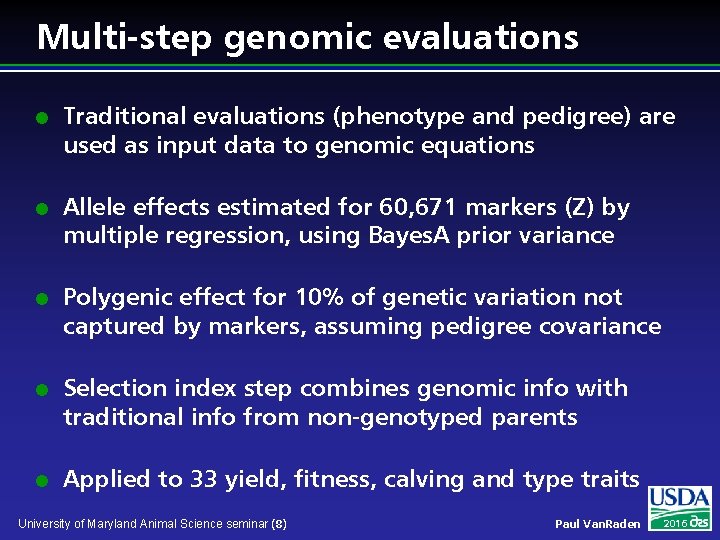
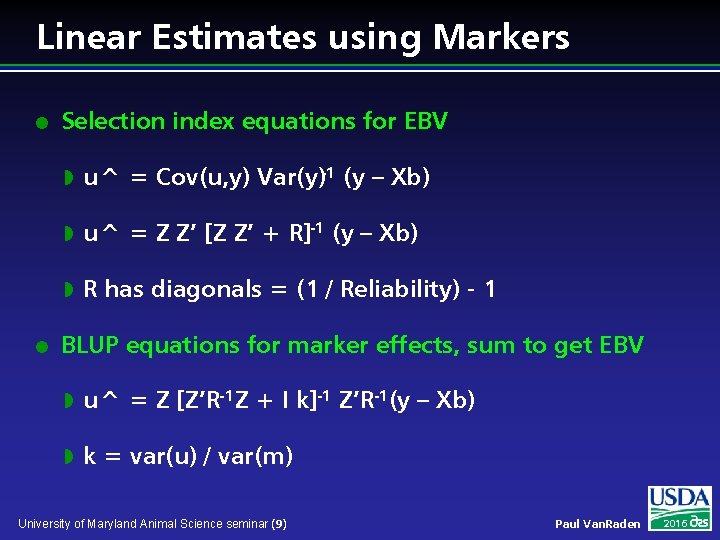
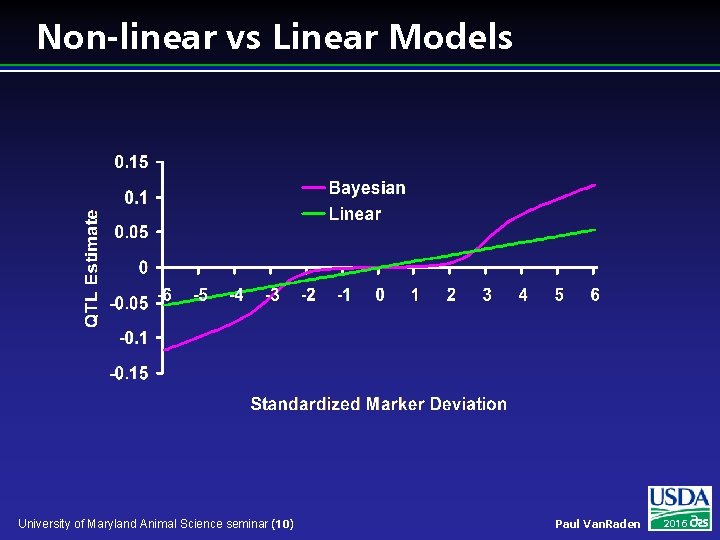
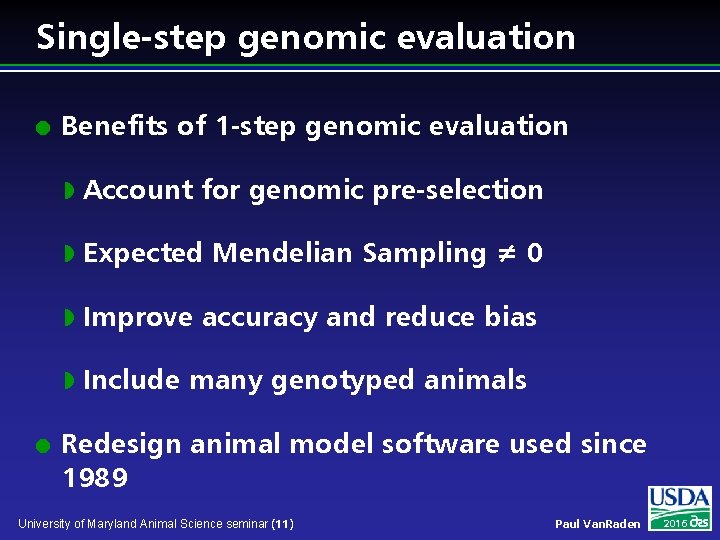
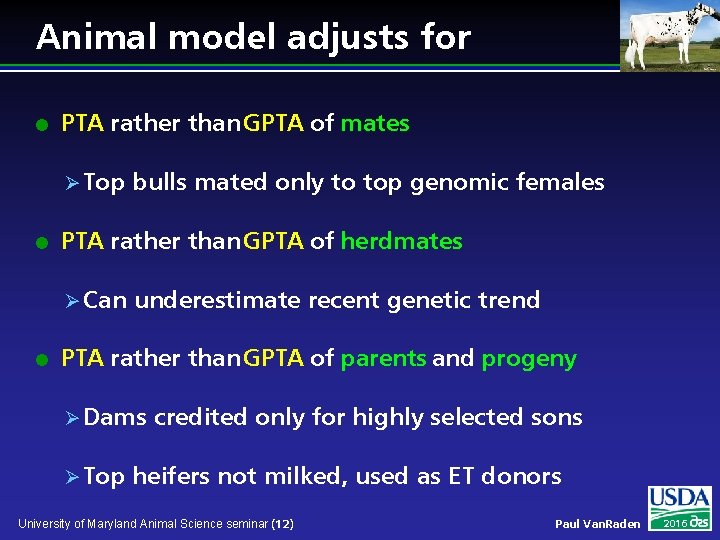
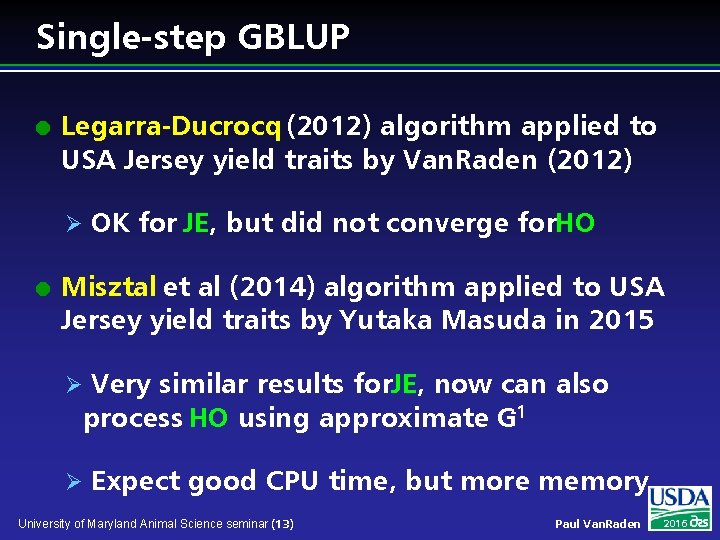
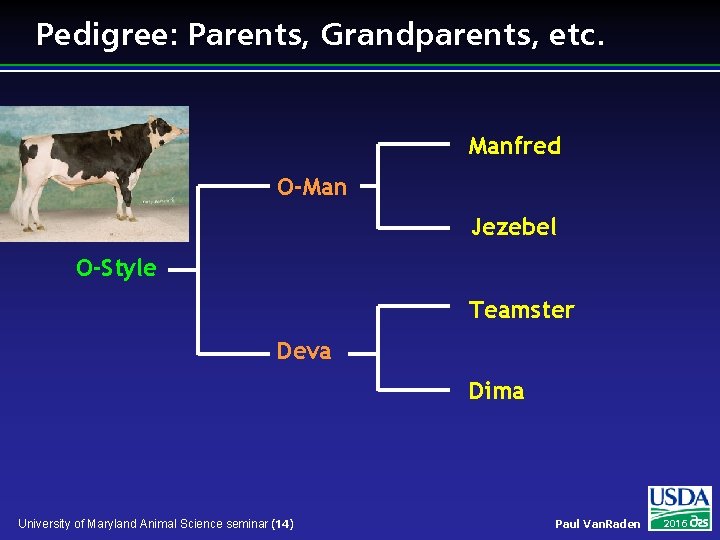
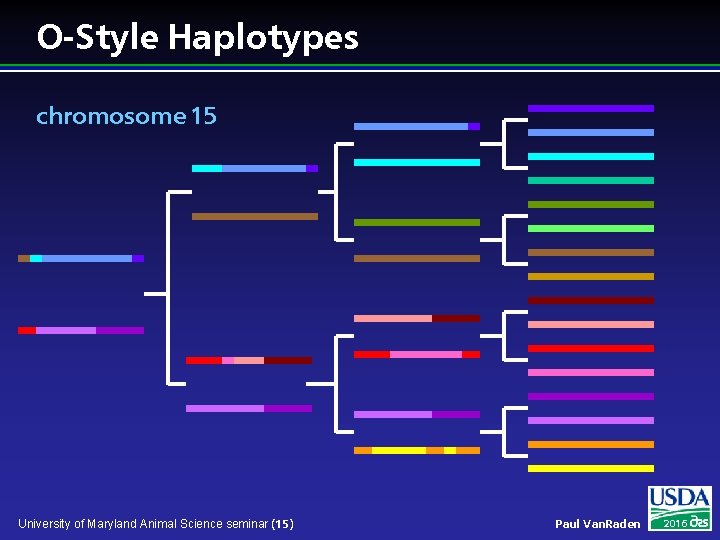
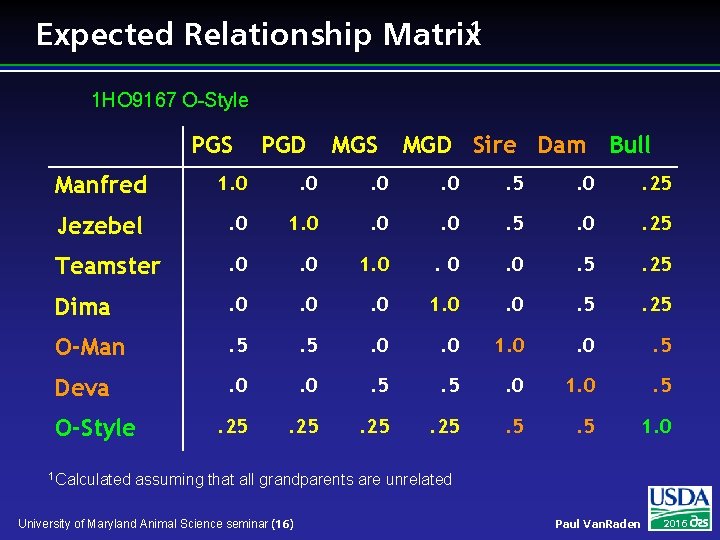
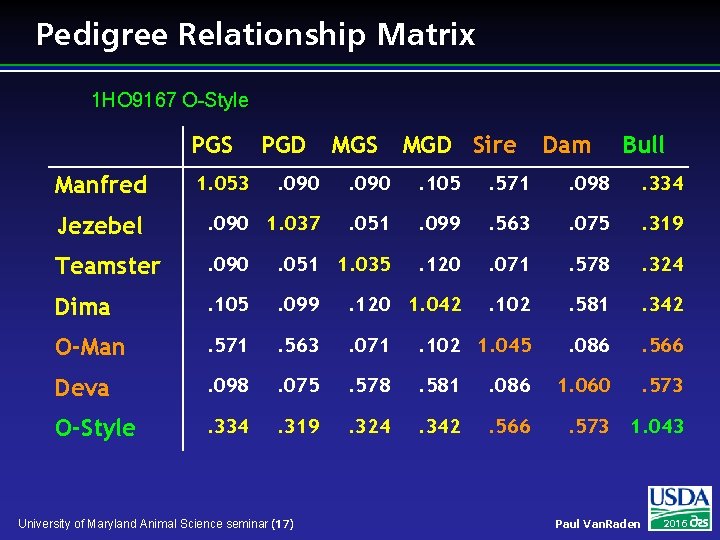
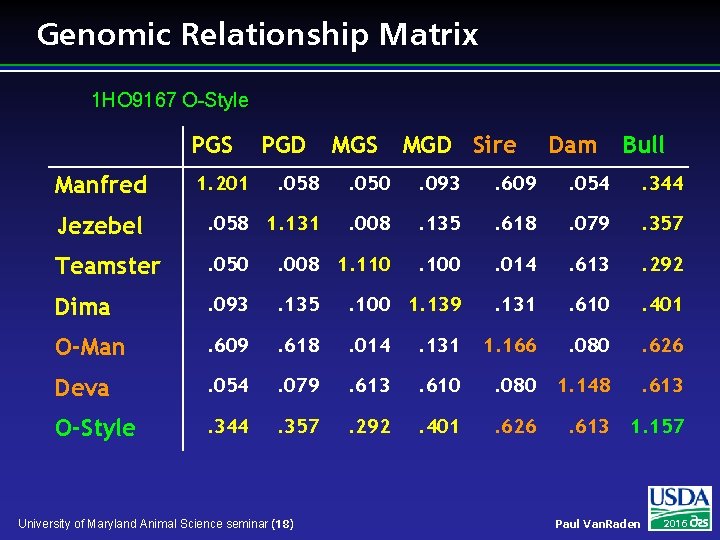
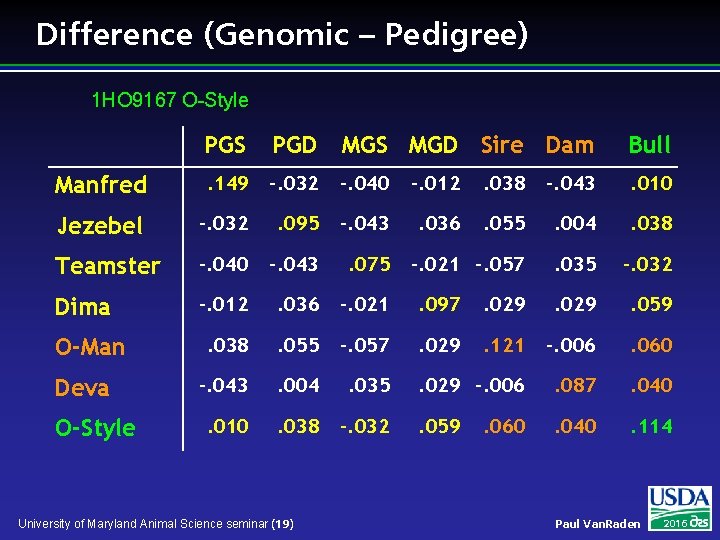
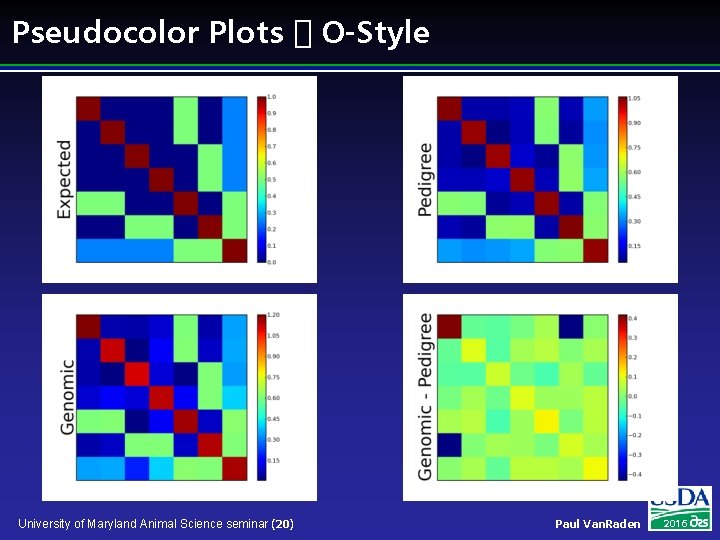
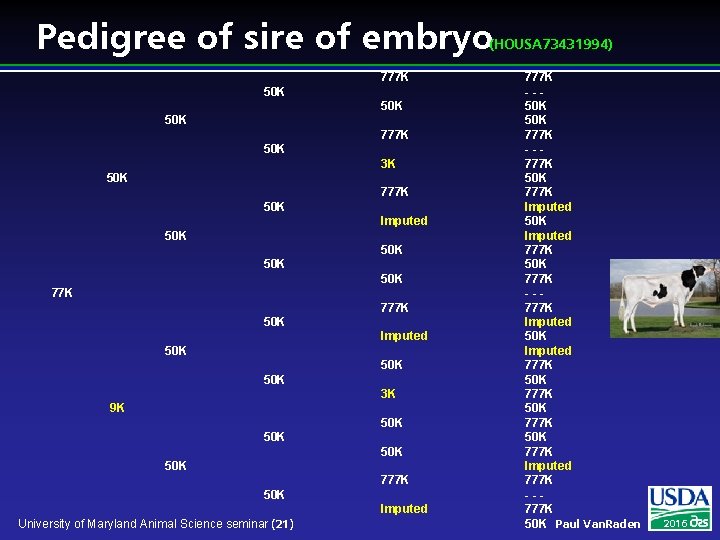
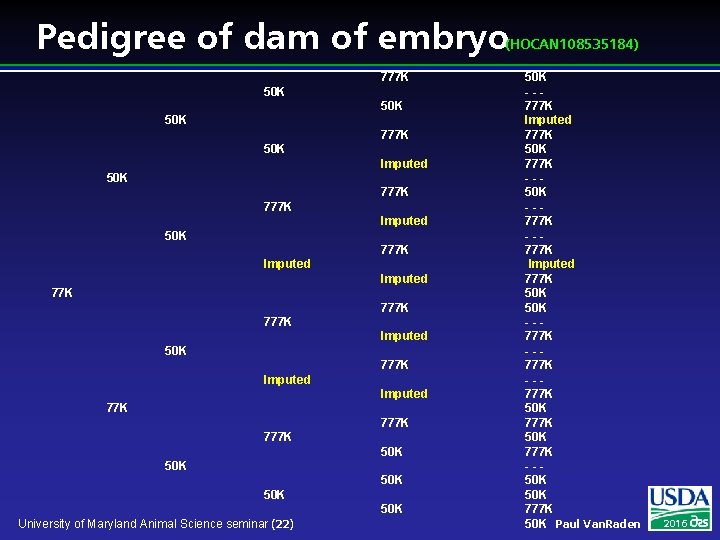
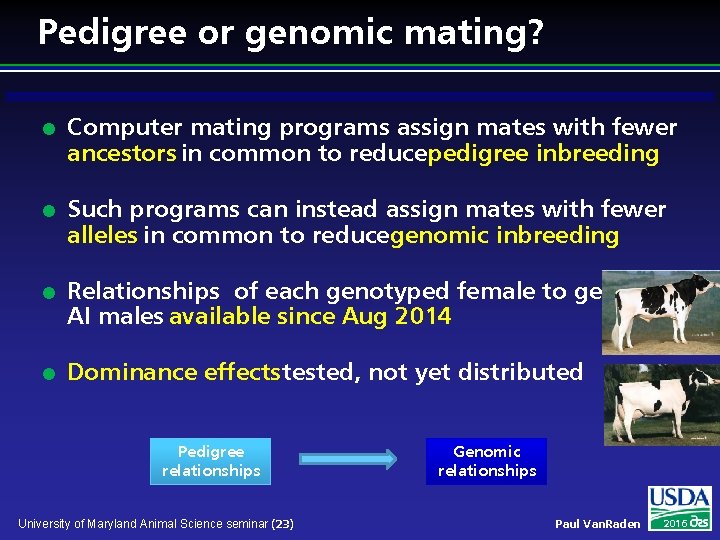
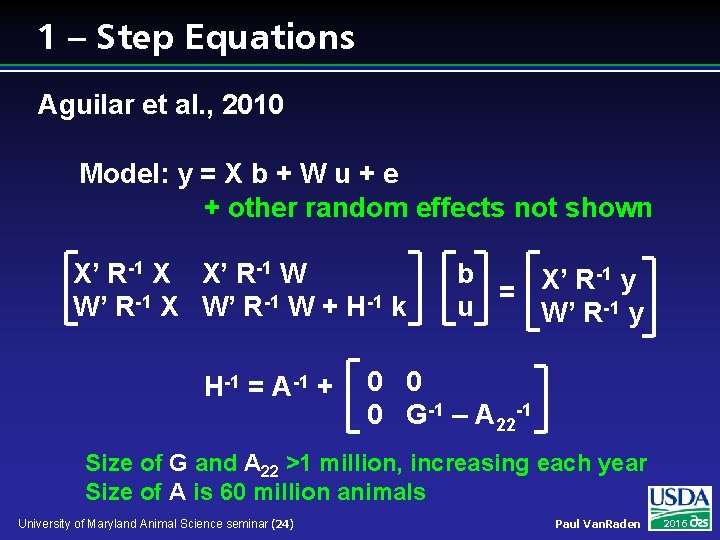
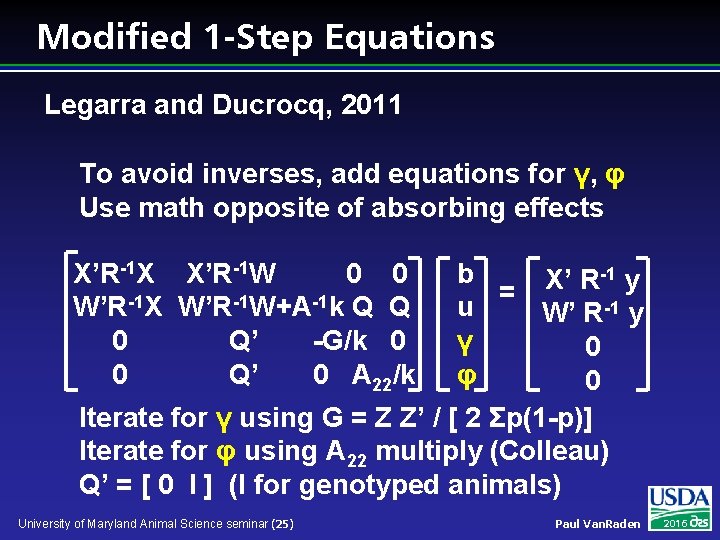
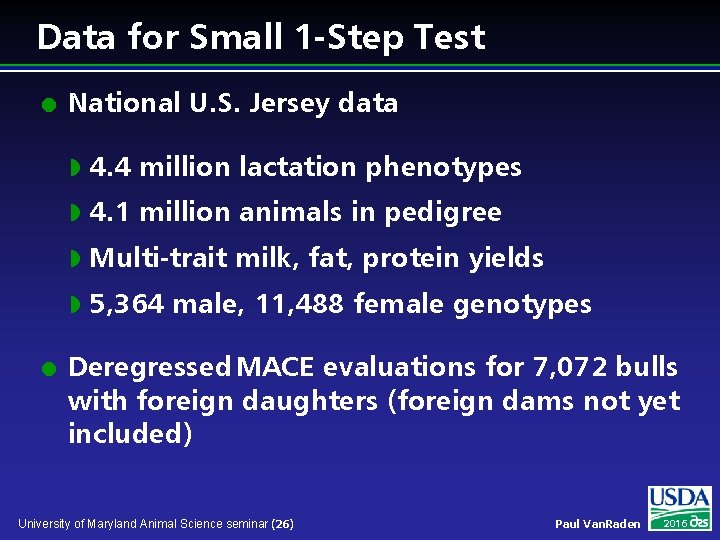
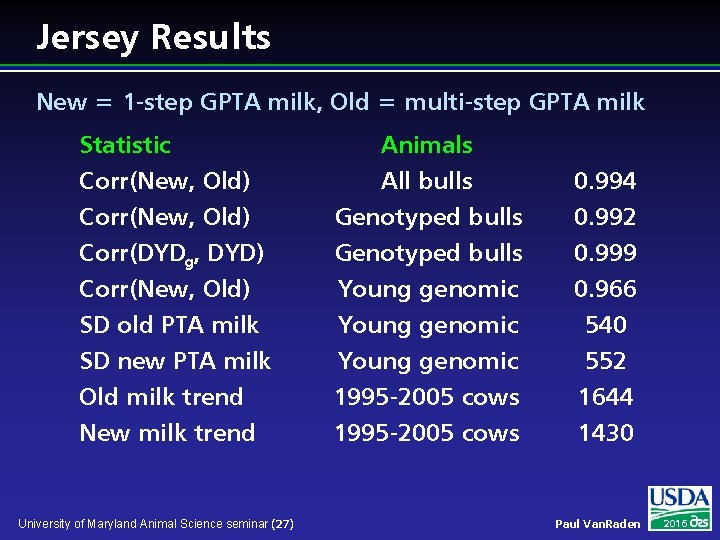
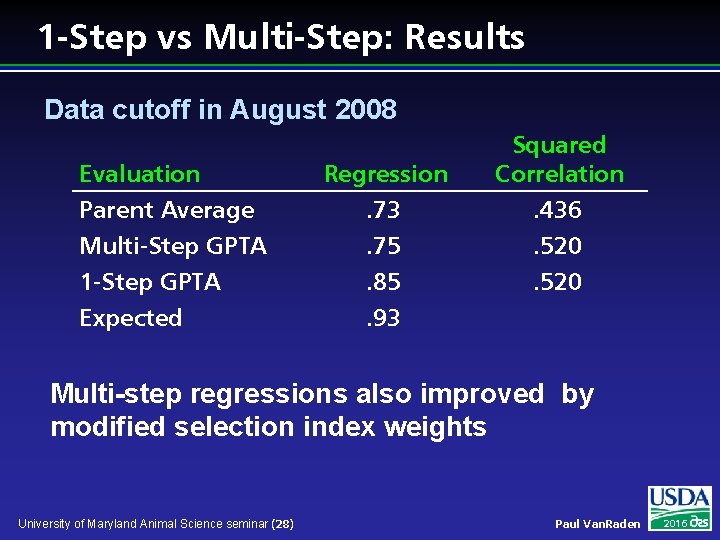
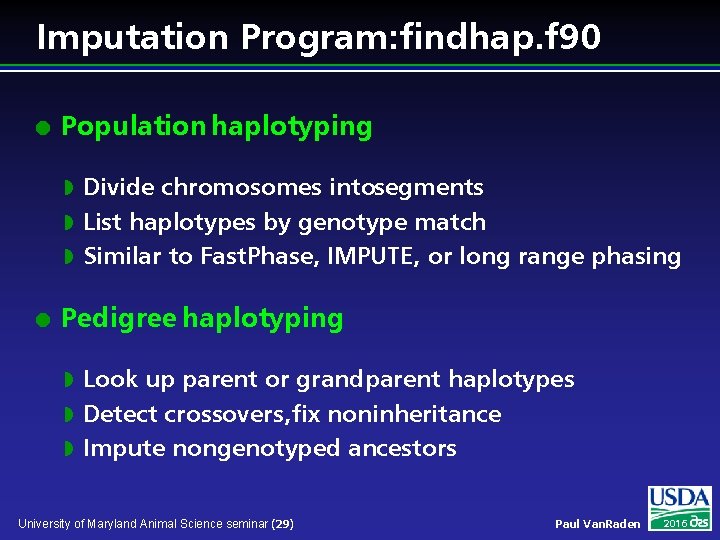
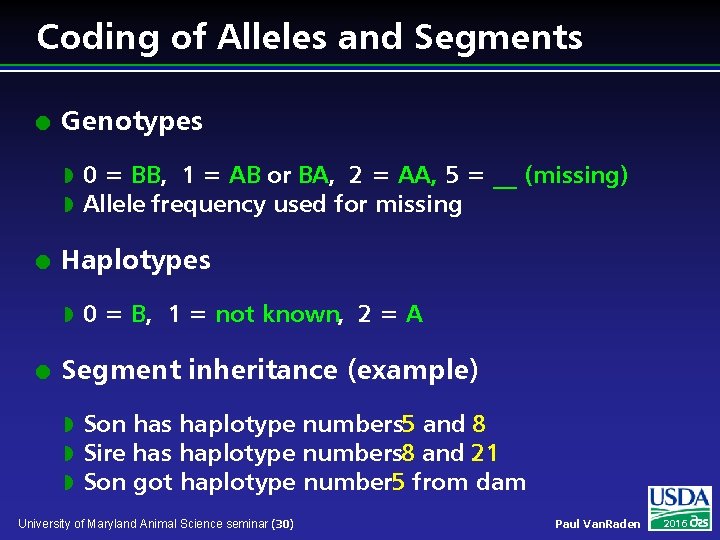
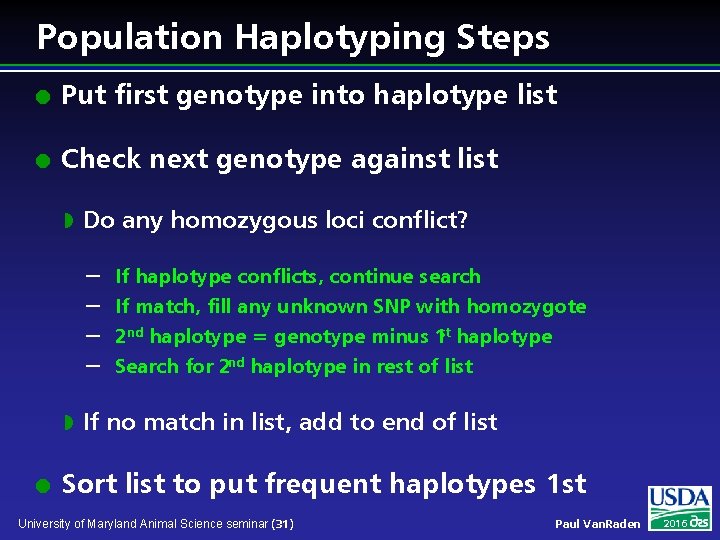
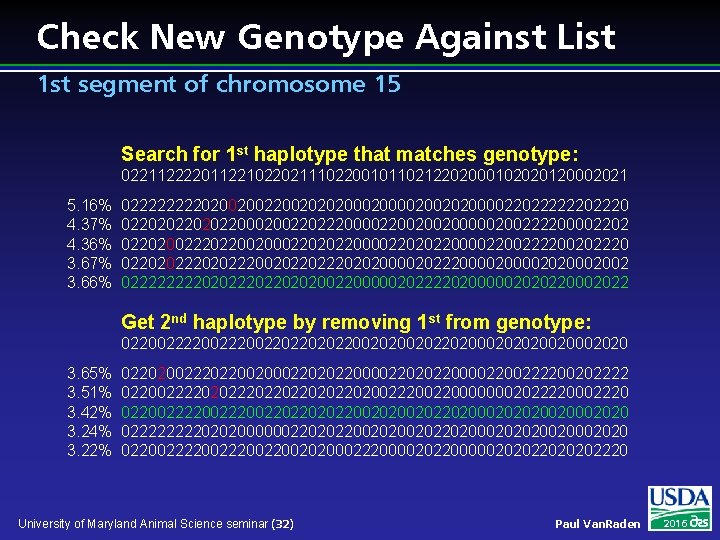
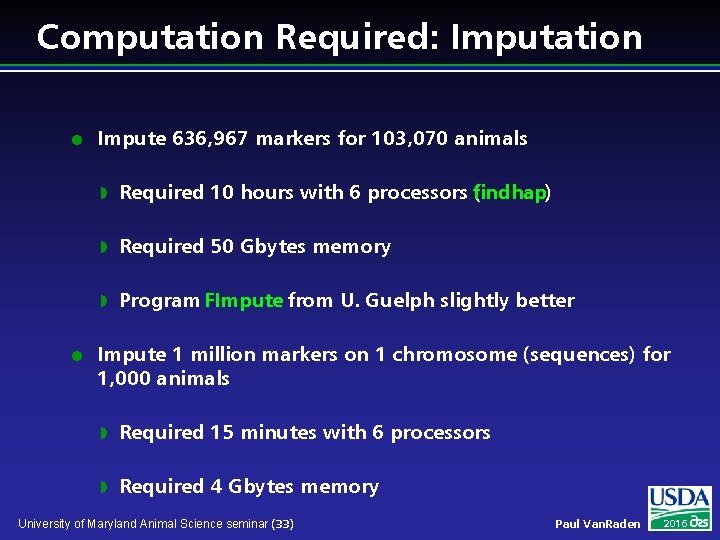
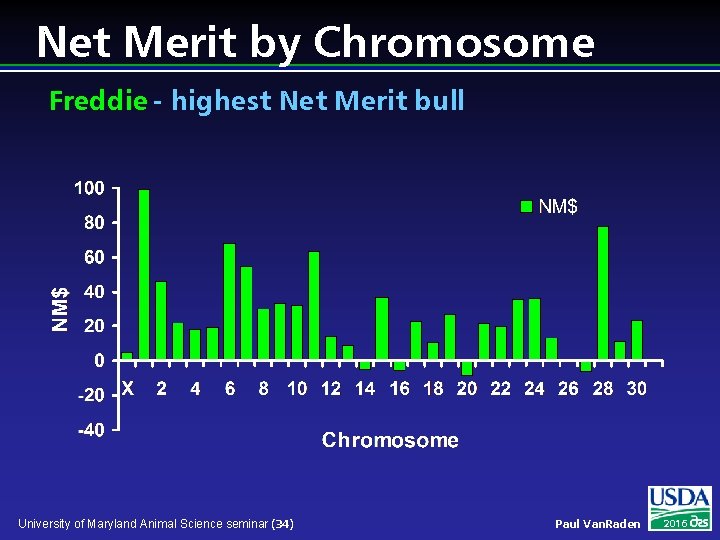
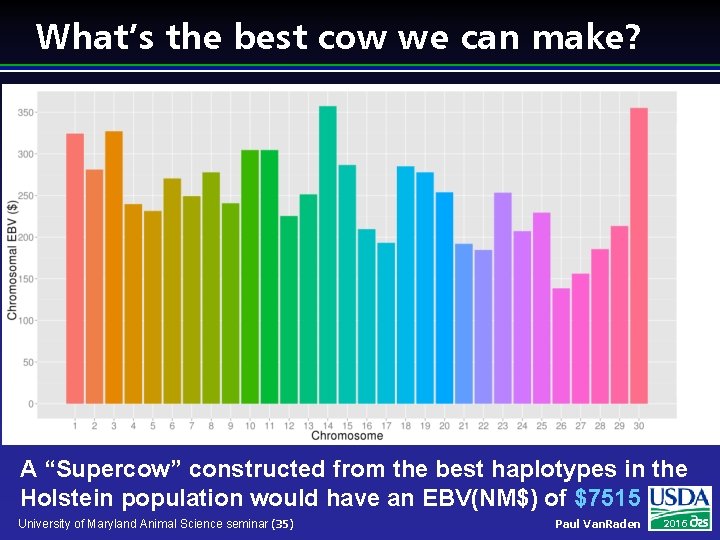
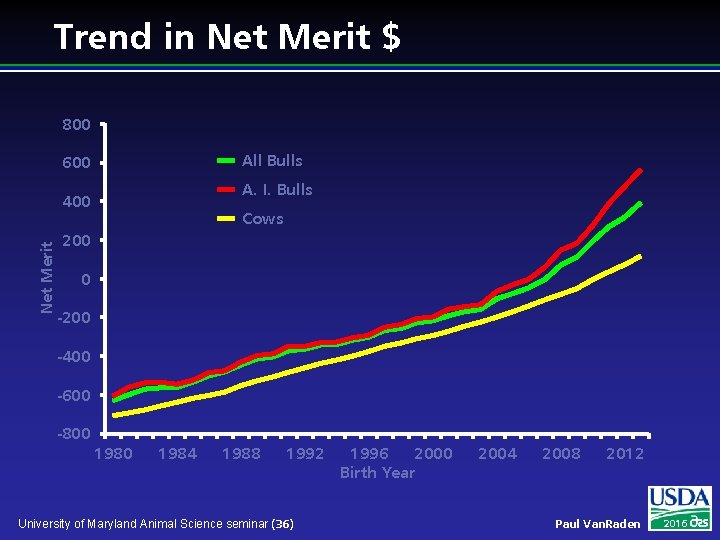
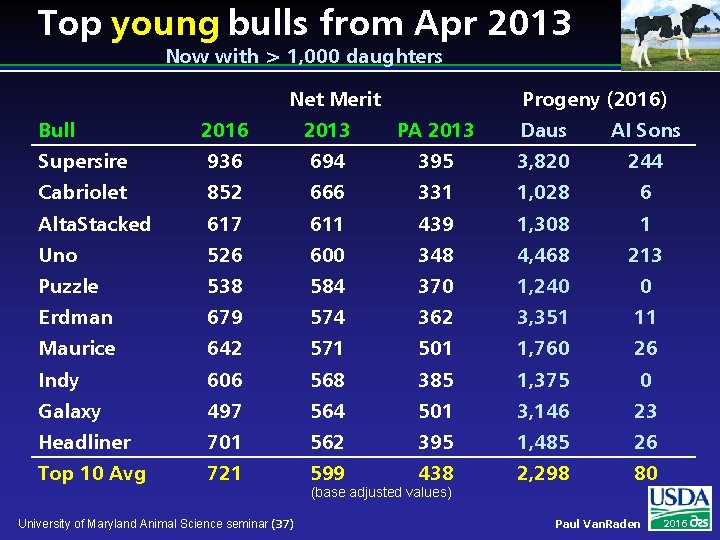
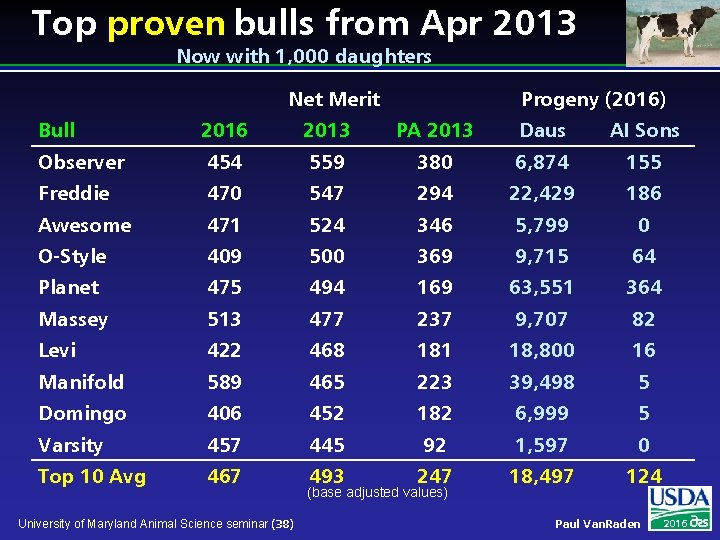
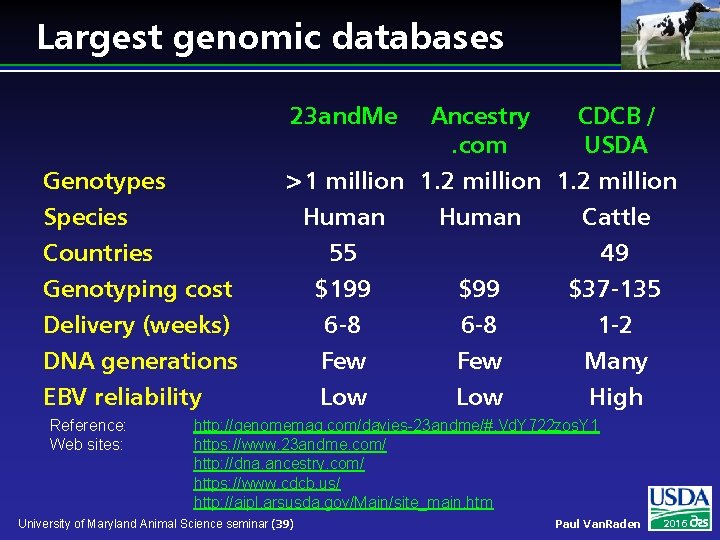
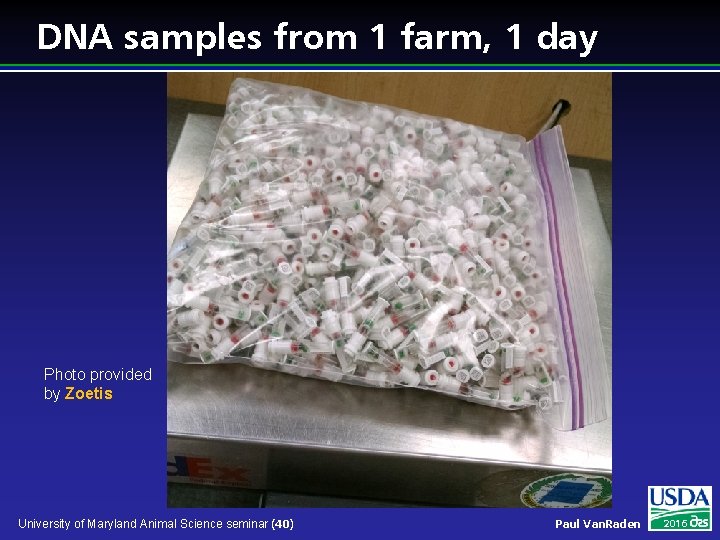
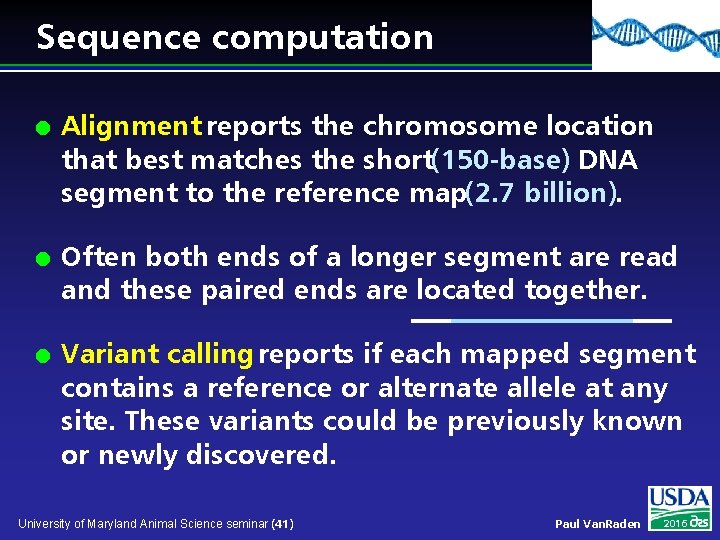
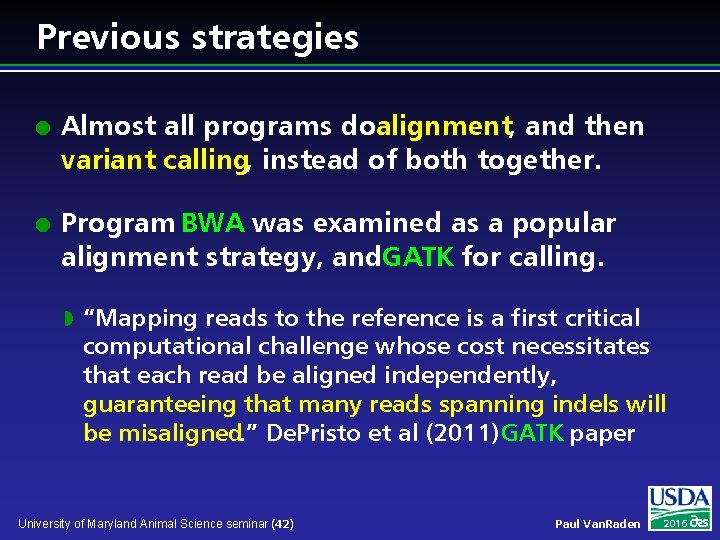
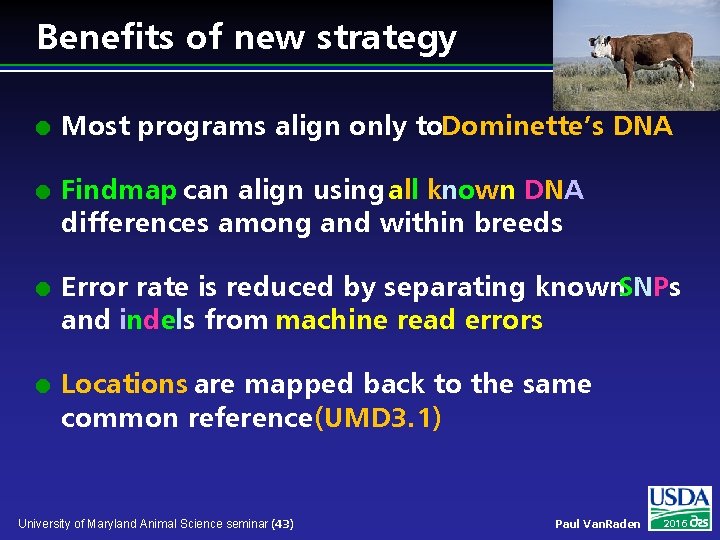
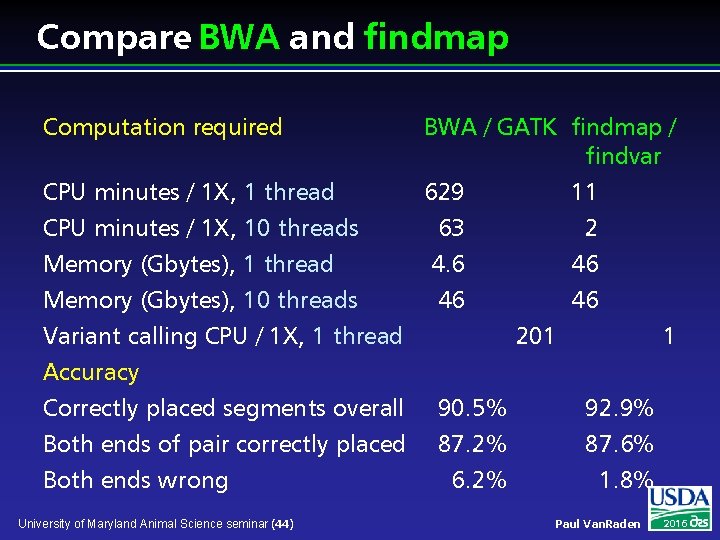
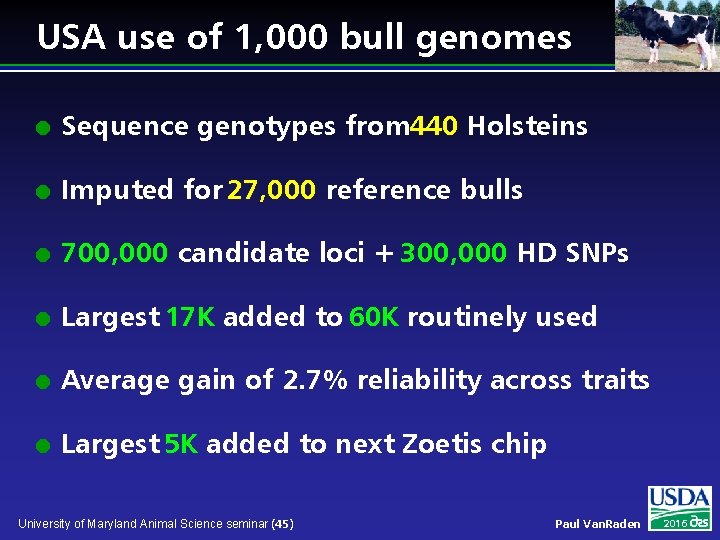
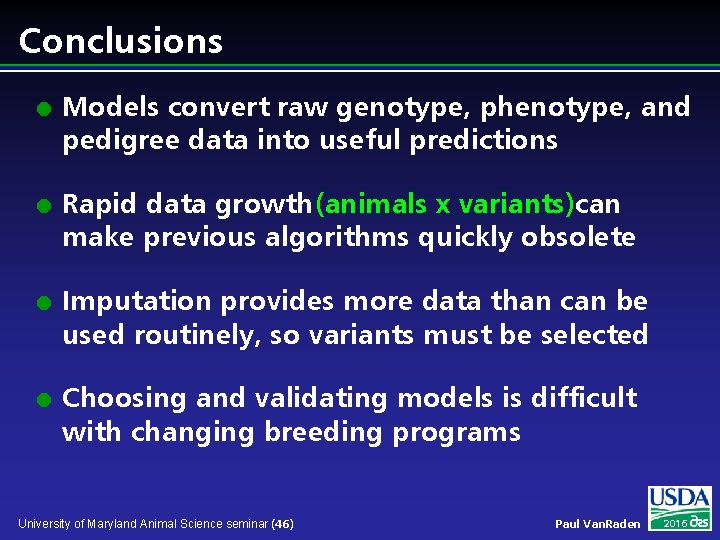
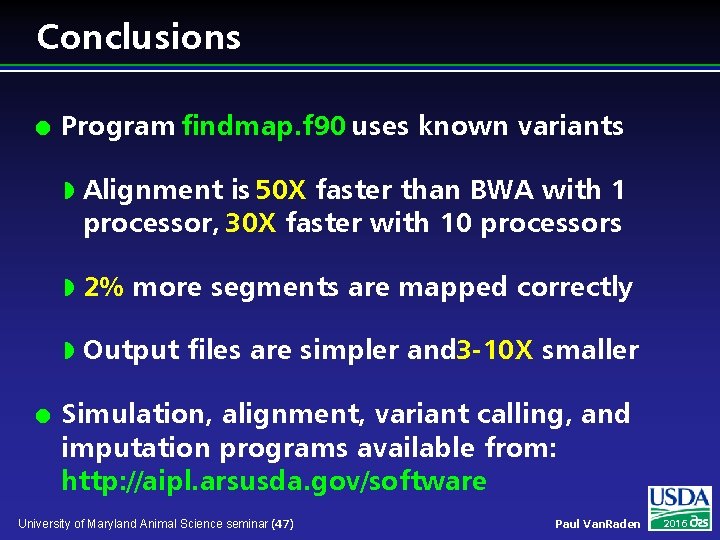
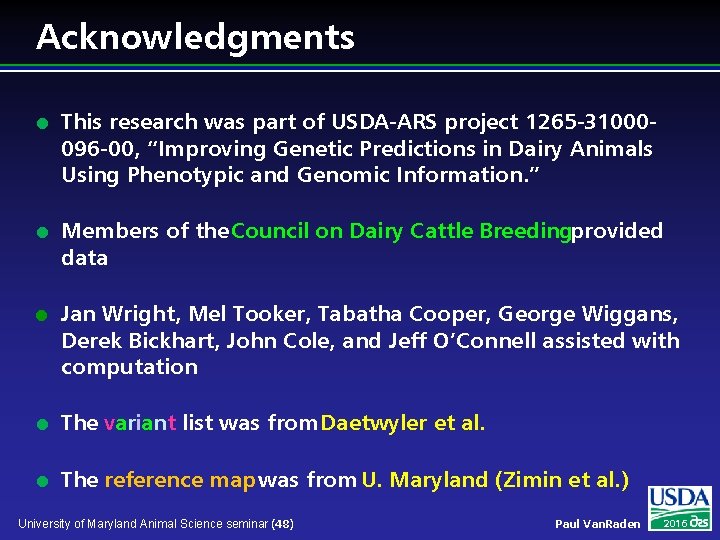
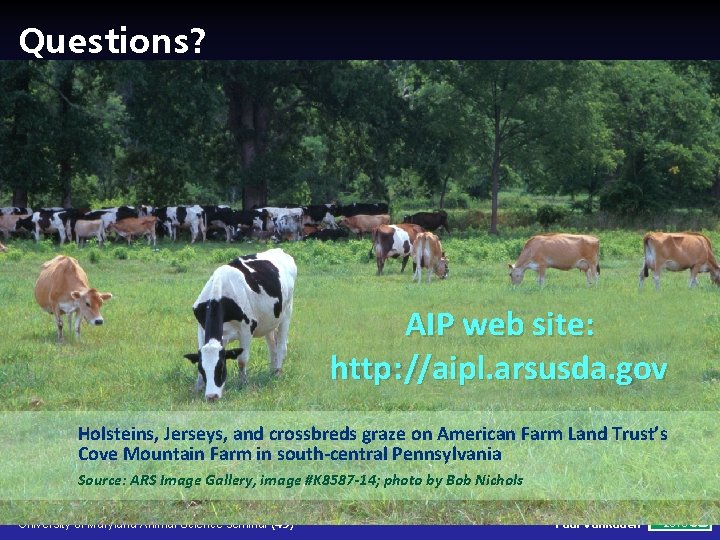
- Slides: 49
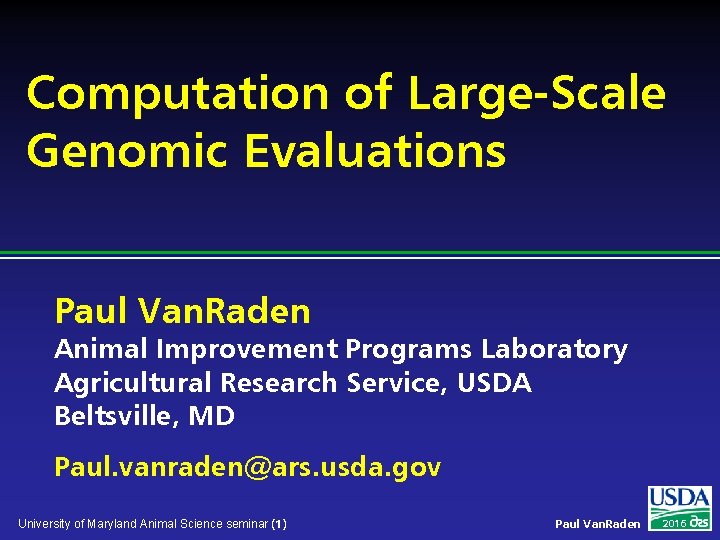
Computation of Large-Scale Genomic Evaluations Paul Van. Raden Animal Improvement Programs Laboratory Agricultural Research Service, USDA Beltsville, MD Paul. vanraden@ars. usda. gov University of Maryland Animal Science seminar (1) Paul Van. Raden 2016
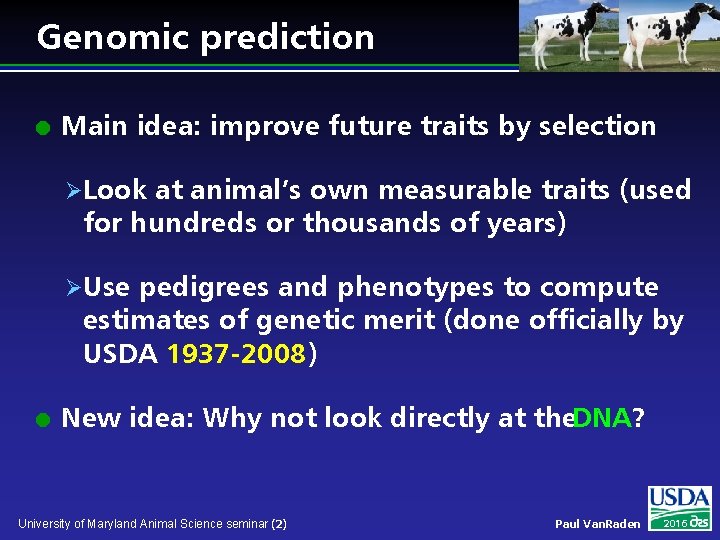
Genomic prediction l Main idea: improve future traits by selection ØLook at animal’s own measurable traits (used for hundreds or thousands of years) ØUse pedigrees and phenotypes to compute estimates of genetic merit (done officially by USDA 1937 -2008) l New idea: Why not look directly at the. DNA? University of Maryland Animal Science seminar (2) Paul Van. Raden 2016
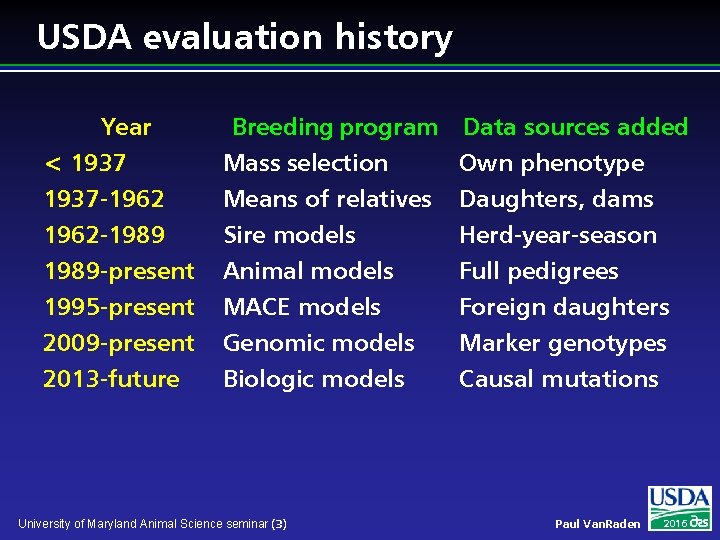
USDA evaluation history Year < 1937 -1962 -1989 Breeding program Data sources added Mass selection Own phenotype Means of relatives Daughters, dams Sire models Herd-year-season 1989 -present 1995 -present 2009 -present 2013 -future Animal models MACE models Genomic models Biologic models University of Maryland Animal Science seminar (3) Full pedigrees Foreign daughters Marker genotypes Causal mutations Paul Van. Raden 2016
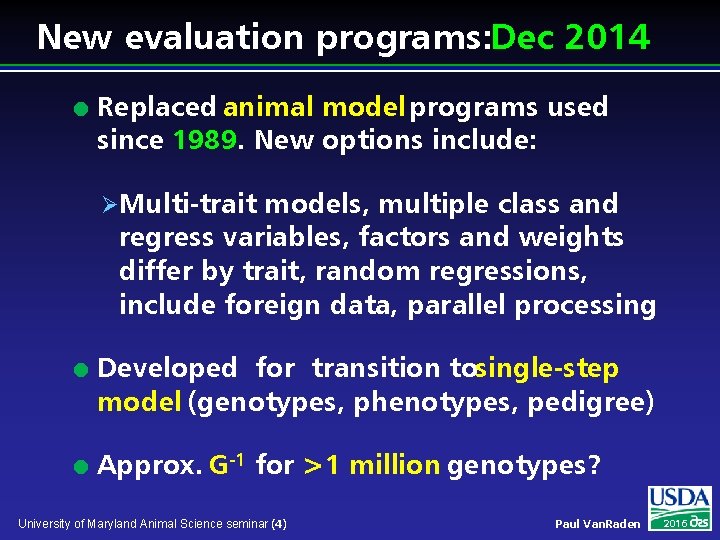
New evaluation programs: Dec 2014 Replaced animal model programs used since 1989. New options include: ØMulti-trait models, multiple class and regress variables, factors and weights differ by trait, random regressions, include foreign data, parallel processing Developed for transition tosingle-step model (genotypes, phenotypes, pedigree) Approx. G-1 for >1 million genotypes? University of Maryland Animal Science seminar (4) Paul Van. Raden 2016
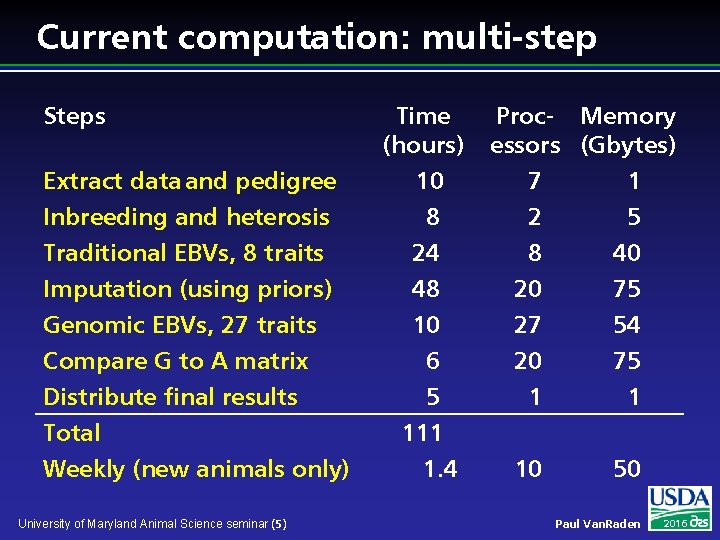
Current computation: multi-step Steps Extract data and pedigree Inbreeding and heterosis Time (hours) 10 8 Traditional EBVs, 8 traits Imputation (using priors) Genomic EBVs, 27 traits Compare G to A matrix Distribute final results Total Weekly (new animals only) 24 48 10 6 5 111 1. 4 University of Maryland Animal Science seminar (5) Proc- Memory essors (Gbytes) 7 1 2 5 8 20 27 20 1 40 75 54 75 1 10 50 Paul Van. Raden 2016
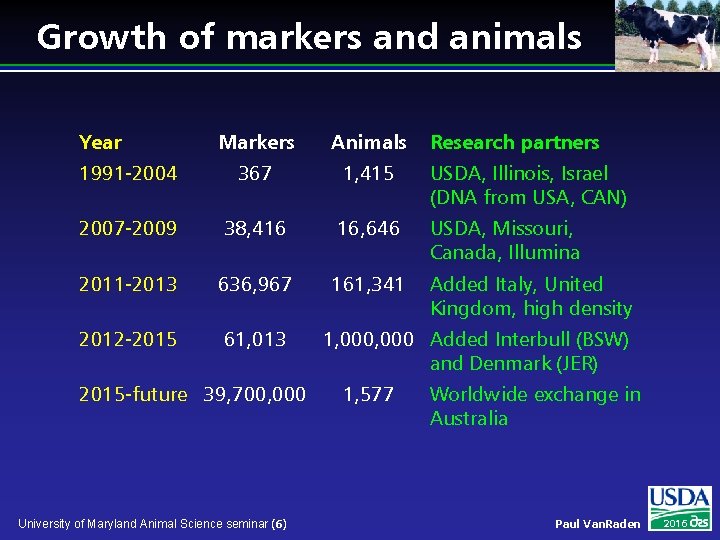
Growth of markers and animals Year Markers Animals 1991 -2004 367 1, 415 USDA, Illinois, Israel (DNA from USA, CAN) 2007 -2009 38, 416 16, 646 USDA, Missouri, Canada, Illumina 2011 -2013 636, 967 161, 341 Added Italy, United Kingdom, high density 2012 -2015 61, 013 2015 -future 39, 700, 000 University of Maryland Animal Science seminar (6) Research partners 1, 000 Added Interbull (BSW) and Denmark (JER) 1, 577 Worldwide exchange in Australia Paul Van. Raden 2016
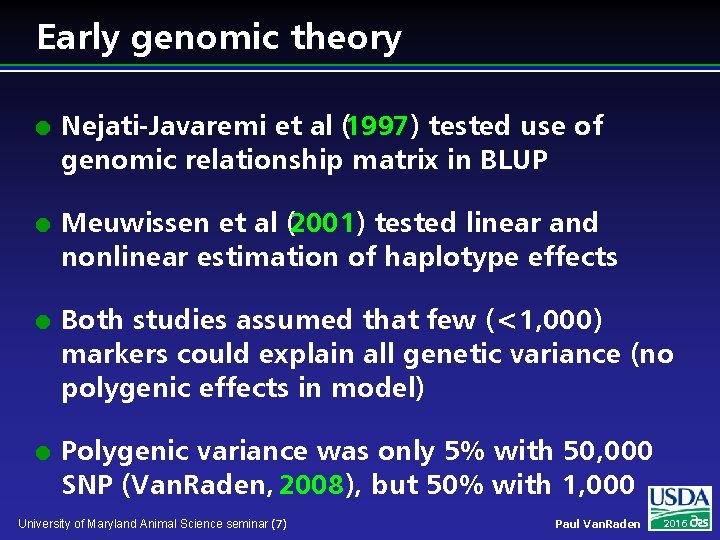
Early genomic theory l l Nejati-Javaremi et al (1997) tested use of genomic relationship matrix in BLUP Meuwissen et al (2001) tested linear and nonlinear estimation of haplotype effects Both studies assumed that few (<1, 000) markers could explain all genetic variance (no polygenic effects in model) Polygenic variance was only 5% with 50, 000 SNP (Van. Raden, 2008), but 50% with 1, 000 University of Maryland Animal Science seminar (7) Paul Van. Raden 2016
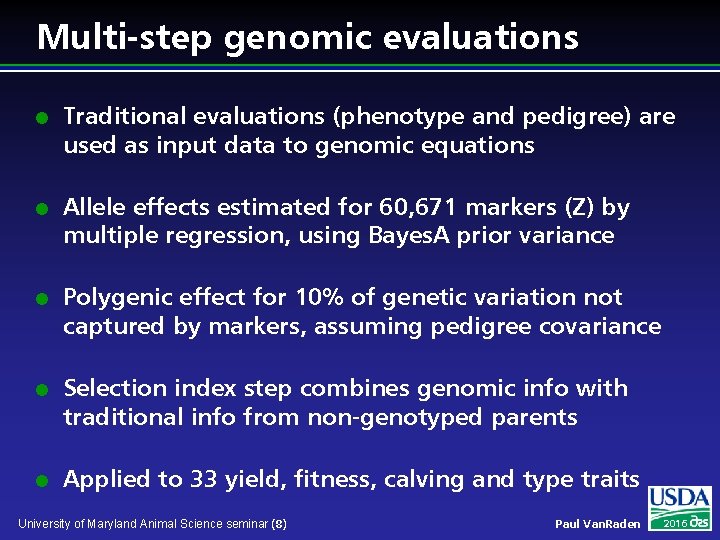
Multi-step genomic evaluations l l l Traditional evaluations (phenotype and pedigree) are used as input data to genomic equations Allele effects estimated for 60, 671 markers (Z) by multiple regression, using Bayes. A prior variance Polygenic effect for 10% of genetic variation not captured by markers, assuming pedigree covariance Selection index step combines genomic info with traditional info from non-genotyped parents Applied to 33 yield, fitness, calving and type traits University of Maryland Animal Science seminar (8) Paul Van. Raden 2016
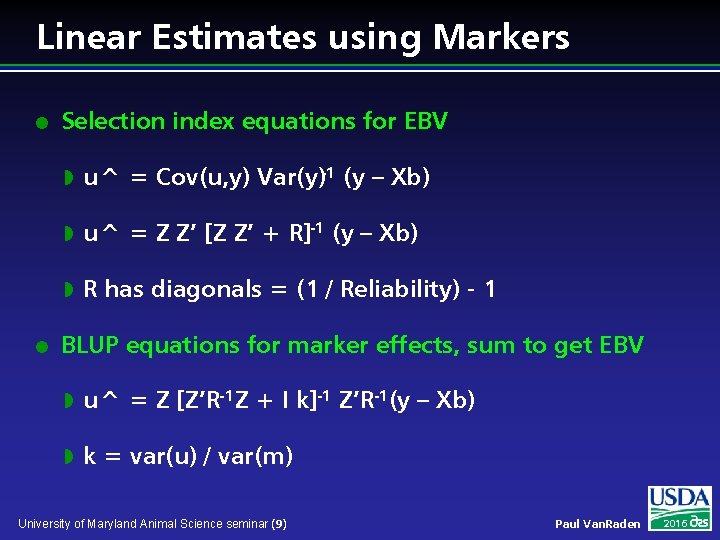
Linear Estimates using Markers l l Selection index equations for EBV w u^ = Cov(u, y) Var(y)-1 (y – Xb) w u^ = Z Z’ [Z Z’ + R]-1 (y – Xb) w R has diagonals = (1 / Reliability) - 1 BLUP equations for marker effects, sum to get EBV w u^ = Z [Z’R-1 Z + I k]-1 Z’R-1(y – Xb) w k = var(u) / var(m) University of Maryland Animal Science seminar (9) Paul Van. Raden 2016
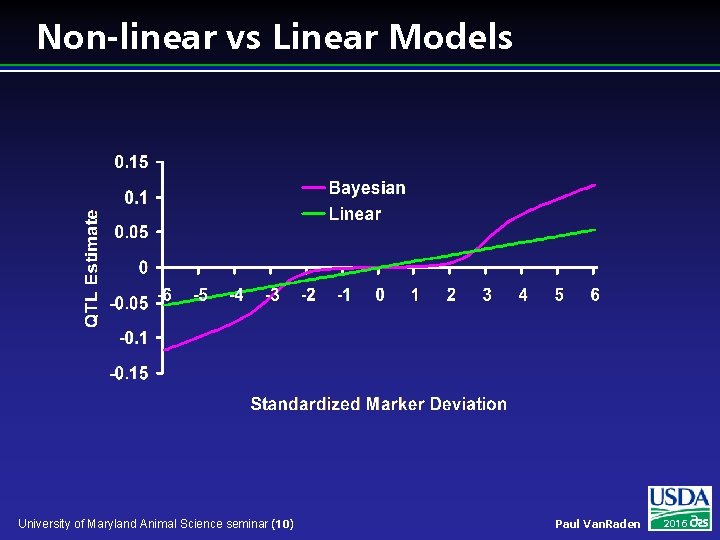
Non-linear vs Linear Models University of Maryland Animal Science seminar (10) Paul Van. Raden 2016
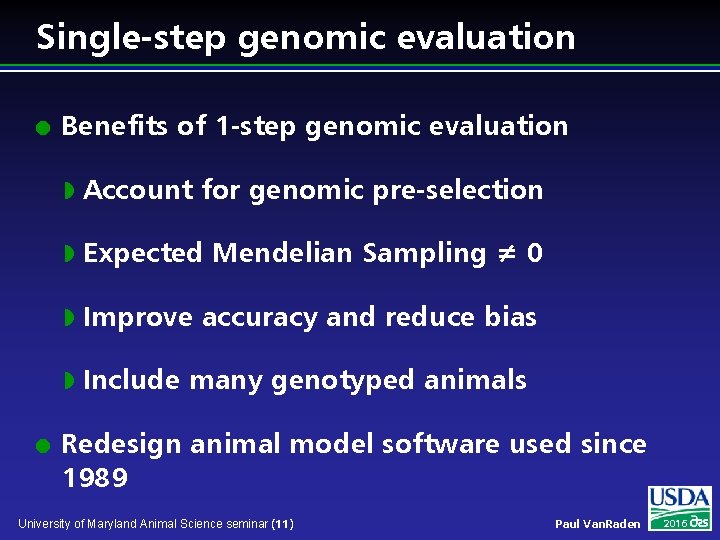
Single-step genomic evaluation l l Benefits of 1 -step genomic evaluation w Account for genomic pre-selection w Expected Mendelian Sampling ≠ 0 w Improve accuracy and reduce bias w Include many genotyped animals Redesign animal model software used since 1989 University of Maryland Animal Science seminar (11) Paul Van. Raden 2016
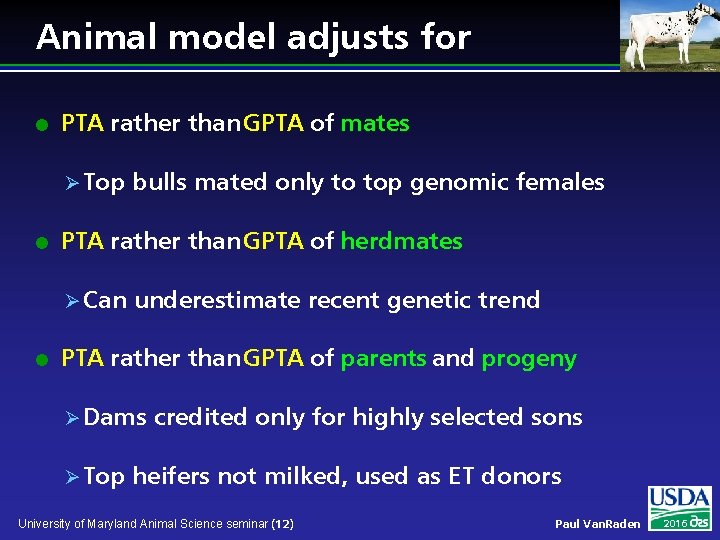
Animal model adjusts for l PTA rather than GPTA of mates Ø Top l PTA rather than GPTA of herdmates Ø Can l bulls mated only to top genomic females underestimate recent genetic trend PTA rather than GPTA of parents and progeny Ø Dams Ø Top credited only for highly selected sons heifers not milked, used as ET donors University of Maryland Animal Science seminar (12) Paul Van. Raden 2016
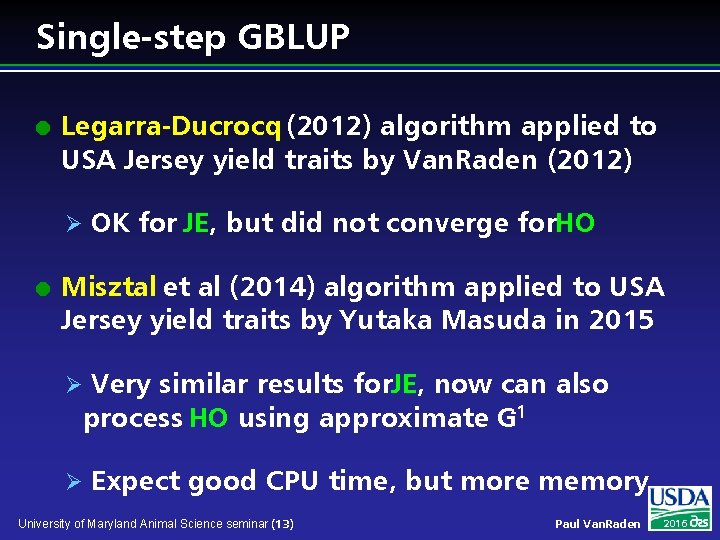
Single-step GBLUP l Legarra-Ducrocq (2012) algorithm applied to USA Jersey yield traits by Van. Raden (2012) Ø l OK for JE, but did not converge for. HO Misztal et al (2014) algorithm applied to USA Jersey yield traits by Yutaka Masuda in 2015 Very similar results for. JE, now can also process HO using approximate G-1 Ø Ø Expect good CPU time, but more memory University of Maryland Animal Science seminar (13) Paul Van. Raden 2016
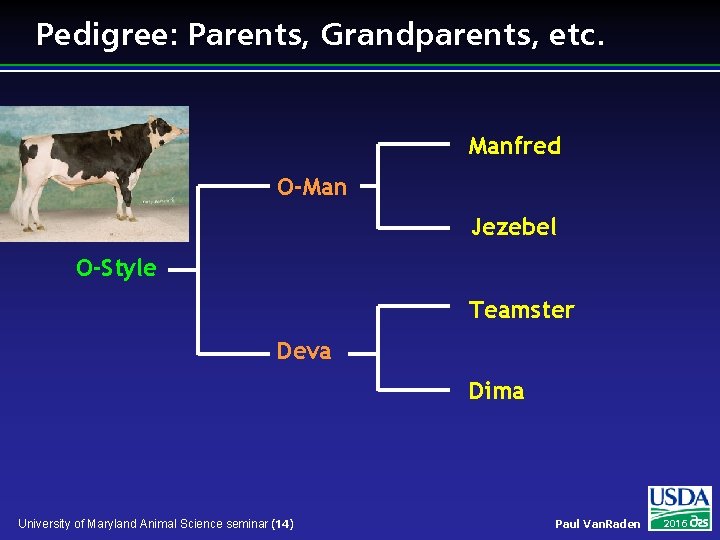
Pedigree: Parents, Grandparents, etc. Manfred O-Man Jezebel O-Style Teamster Deva Dima University of Maryland Animal Science seminar (14) Paul Van. Raden 2016
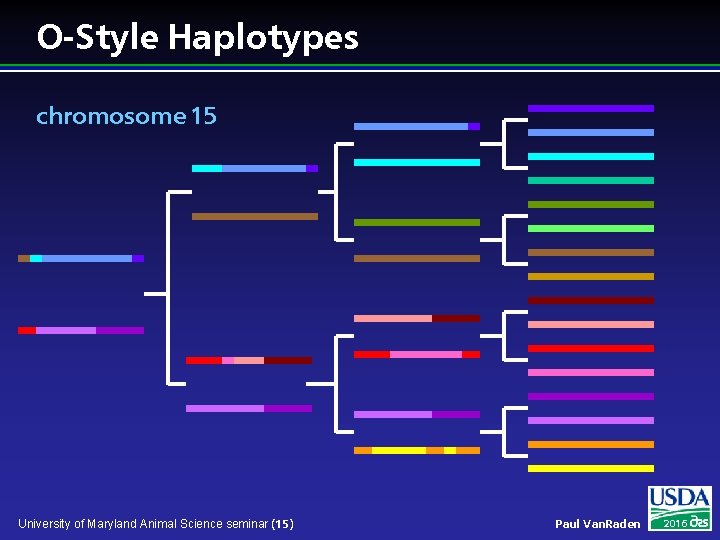
O-Style Haplotypes chromosome 15 University of Maryland Animal Science seminar (15) Paul Van. Raden 2016
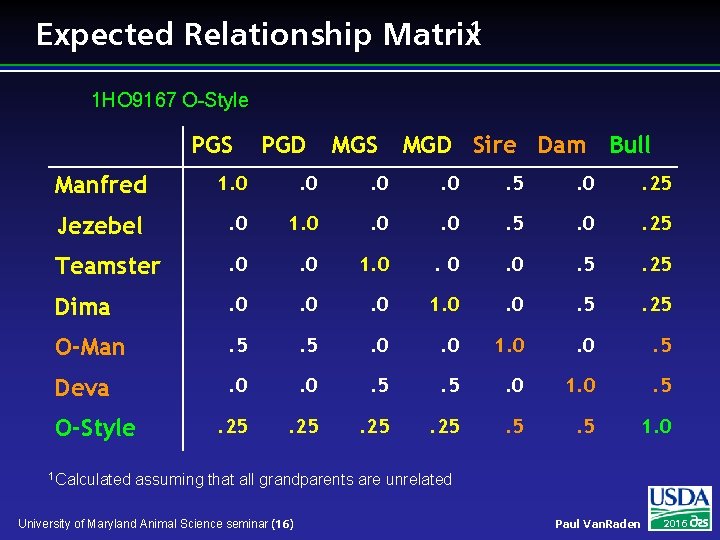
Expected Relationship Matrix 1 1 HO 9167 O-Style PGS PGD MGS MGD Sire Dam Bull Manfred 1. 0 . 0 . 5 . 0 . 25 Jezebel . 0 1. 0 . 0 . 5 . 0 . 25 Teamster . 0 1. 0 . 0 . 5 . 25 Dima . 0 . 0 1. 0 . 5 . 25 O-Man . 5 . 0 1. 0 . 5 Deva . 0 . 5 . 0 1. 0 . 5 . 25 . 5 1. 0 O-Style 1 Calculated assuming that all grandparents are unrelated University of Maryland Animal Science seminar (16) Paul Van. Raden 2016
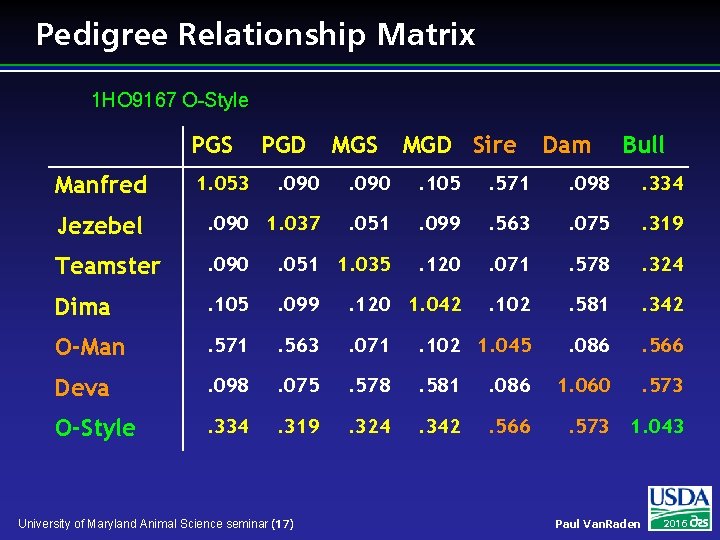
Pedigree Relationship Matrix 1 HO 9167 O-Style PGS MGD Sire Dam Bull . 090 . 105 . 571 . 098 . 334 Jezebel . 090 1. 037 . 051 . 099 . 563 . 075 . 319 Teamster . 090 . 051 1. 035 . 120 . 071 . 578 . 324 Dima . 105 . 099 . 120 1. 042 . 102 . 581 . 342 O-Man . 571 . 563 . 071 . 102 1. 045 . 086 . 566 Deva . 098 . 075 . 578 . 581 . 086 1. 060 . 573 O-Style . 334 . 319 . 324 . 342 . 566 . 573 1. 043 Manfred 1. 053 PGD University of Maryland Animal Science seminar (17) Paul Van. Raden 2016
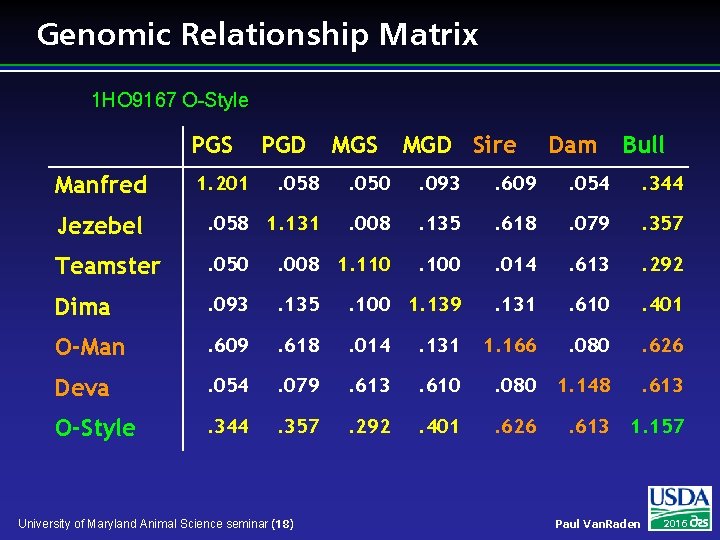
Genomic Relationship Matrix 1 HO 9167 O-Style PGS MGD Sire Dam Bull . 058 . 050 . 093 . 609 . 054 . 344 Jezebel . 058 1. 131 . 008 . 135 . 618 . 079 . 357 Teamster . 050 . 008 1. 110 . 100 . 014 . 613 . 292 Dima . 093 . 135 . 100 1. 139 . 131 . 610 . 401 O-Man . 609 . 618 . 014 . 131 1. 166 . 080 . 626 Deva . 054 . 079 . 613 . 610 . 080 1. 148 . 613 O-Style . 344 . 357 . 292 . 401 . 626 . 613 1. 157 Manfred 1. 201 PGD University of Maryland Animal Science seminar (18) Paul Van. Raden 2016
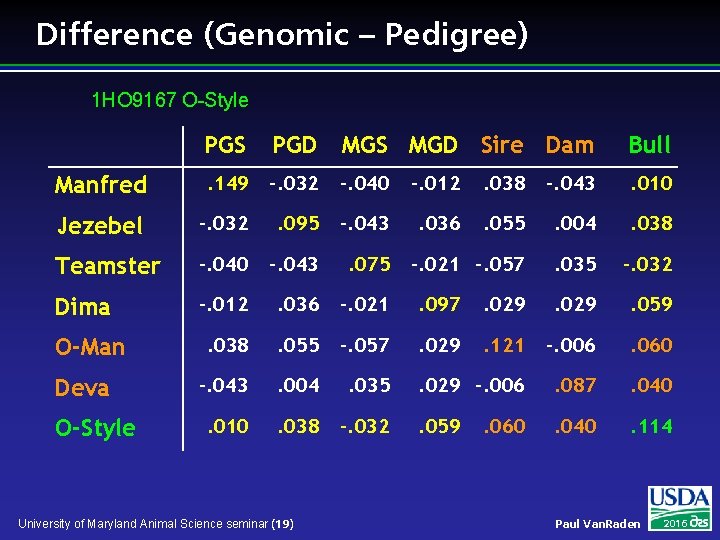
Difference (Genomic – Pedigree) 1 HO 9167 O-Style PGS PGD MGS MGD Sire Dam Bull Manfred . 149 -. 032 -. 040 -. 012 . 038 -. 043 . 010 Jezebel -. 032 . 095 -. 043 . 036 . 055 . 004 . 038 Teamster -. 040 -. 043 . 075 -. 021 -. 057 . 035 -. 032 Dima -. 012 . 036 -. 021 . 097 . 029 . 059 . 038 . 055 -. 057 . 029 . 121 -. 006 . 060 -. 043 . 004 . 035 . 029 -. 006 . 087 . 040 . 010 . 038 -. 032 . 059 . 040 . 114 O-Man Deva O-Style University of Maryland Animal Science seminar (19) . 060 Paul Van. Raden 2016
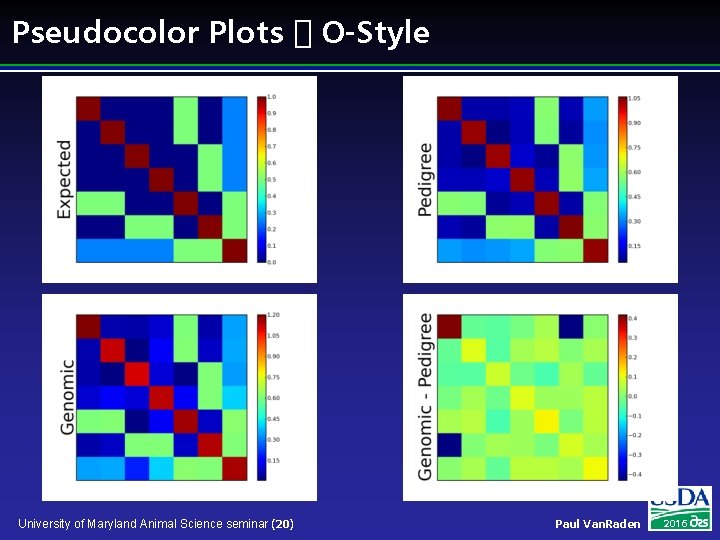
Pseudocolor Plots � O-Style University of Maryland Animal Science seminar (20) Paul Van. Raden 2016
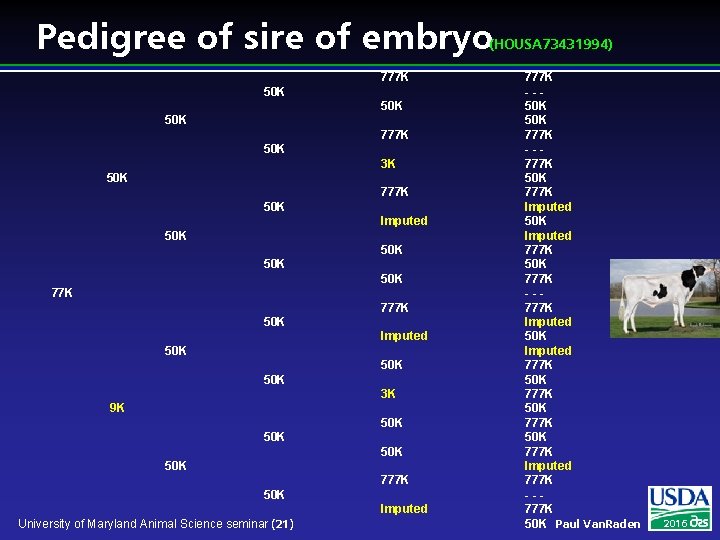
Pedigree of sire of embryo (HOUSA 73431994) 777 K 50 K 50 K 777 K 50 K 3 K 50 K 777 K 50 K Imputed 50 K 50 K 77 K 777 K 50 K Imputed 50 K 50 K 3 K 9 K 50 K 50 K 777 K 50 K Imputed University of Maryland Animal Science seminar (21) 777 K --50 K 777 K --777 K 50 K 777 K Imputed 50 K Imputed 777 K 50 K 777 K --777 K Imputed 50 K Imputed 777 K 50 K 777 K Imputed 777 K --777 K 50 K Paul Van. Raden 2016
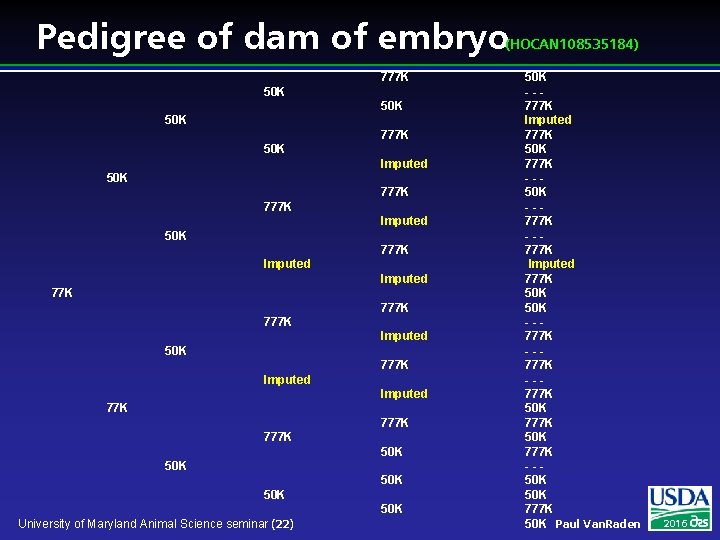
Pedigree of dam of embryo (HOCAN 108535184) 777 K 50 K 50 K 777 K 50 K Imputed 50 K 777 K Imputed 50 K 777 K Imputed 77 K 777 K 50 K 50 K 50 K University of Maryland Animal Science seminar (22) 50 K --777 K Imputed 777 K 50 K 777 K --50 K --777 K Imputed 777 K 50 K --777 K 50 K 777 K --50 K 777 K 50 K Paul Van. Raden 2016
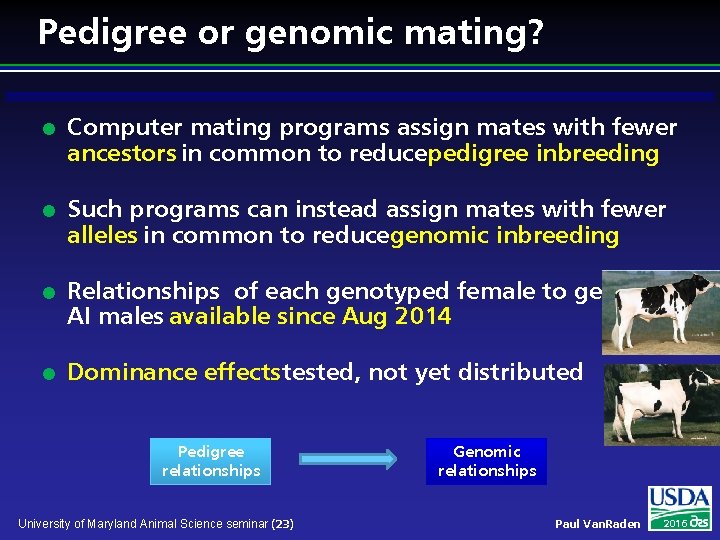
Pedigree or genomic mating? Computer mating programs assign mates with fewer ancestors in common to reducepedigree inbreeding Such programs can instead assign mates with fewer alleles in common to reducegenomic inbreeding Relationships of each genotyped female to genotyped AI males available since Aug 2014 Dominance effects tested, not yet distributed Pedigree relationships University of Maryland Animal Science seminar (23) Genomic relationships Paul Van. Raden 2016
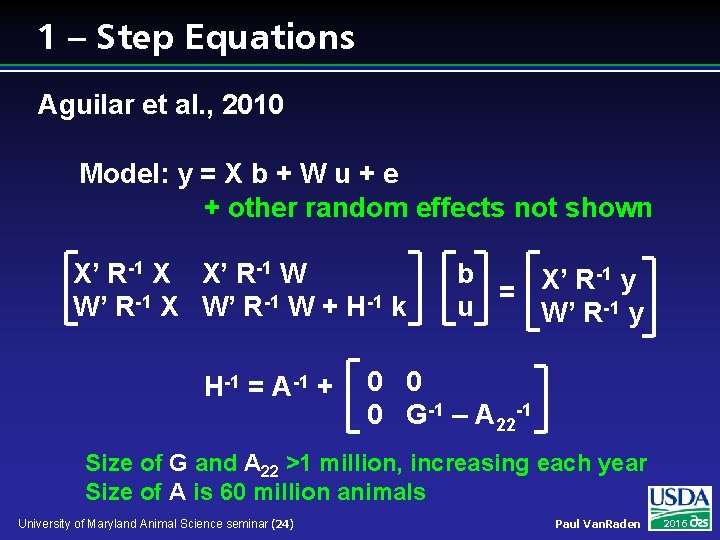
1 – Step Equations Aguilar et al. , 2010 Model: y = X b + W u + e + other random effects not shown X’ R-1 X X’ R-1 W W’ R-1 X W’ R-1 W + H-1 k H-1 = A-1 + -1 y b X’ R = u W’ R-1 y 0 0 0 G-1 – A 22 -1 Size of G and A 22 >1 million, increasing each year Size of A is 60 million animals University of Maryland Animal Science seminar (24) Paul Van. Raden 2016
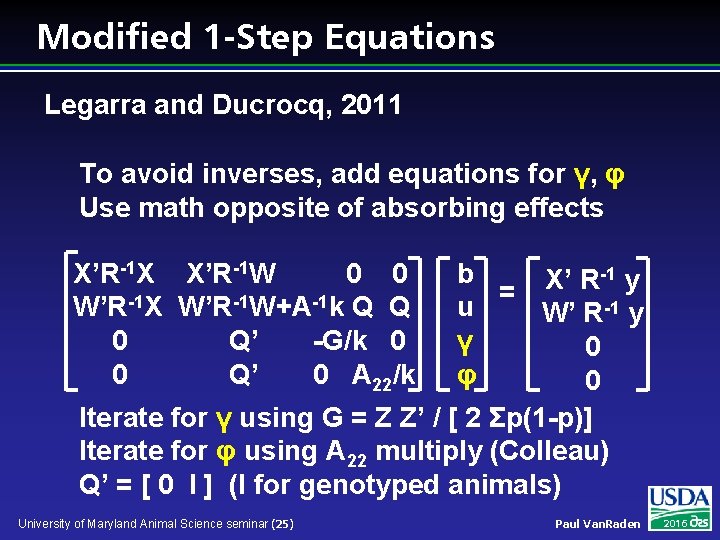
Modified 1 -Step Equations Legarra and Ducrocq, 2011 To avoid inverses, add equations for γ, φ Use math opposite of absorbing effects X’R-1 X X’R-1 W 0 0 W’R-1 X W’R-1 W+A-1 k Q Q 0 Q’ -G/k 0 0 Q’ 0 A 22/k -1 y b X’ R = u W’ R-1 y γ 0 φ 0 Iterate for γ using G = Z Z’ / [ 2 Σp(1 -p)] Iterate for φ using A 22 multiply (Colleau) Q’ = [ 0 I ] (I for genotyped animals) University of Maryland Animal Science seminar (25) Paul Van. Raden 2016
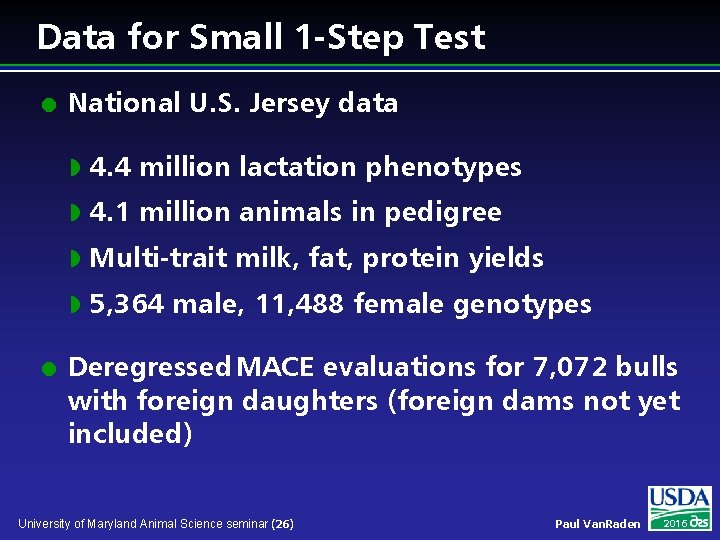
Data for Small 1 -Step Test l l National U. S. Jersey data w 4. 4 million lactation phenotypes w 4. 1 million animals in pedigree w Multi-trait milk, fat, protein yields w 5, 364 male, 11, 488 female genotypes Deregressed MACE evaluations for 7, 072 bulls with foreign daughters (foreign dams not yet included) University of Maryland Animal Science seminar (26) Paul Van. Raden 2016
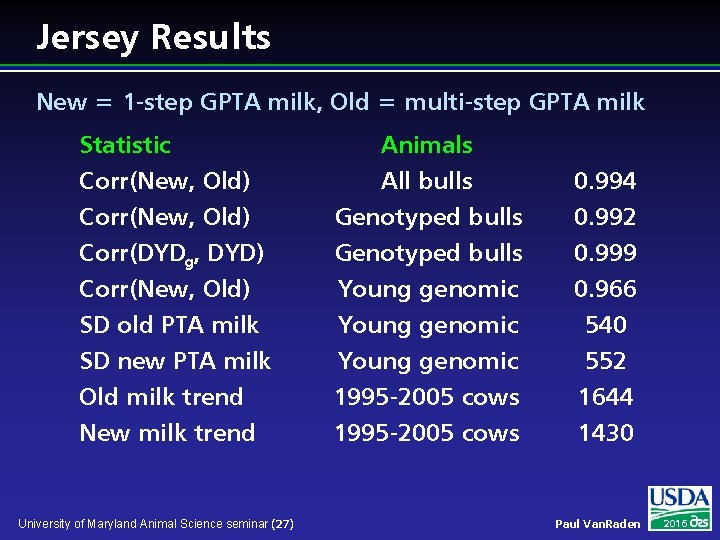
Jersey Results New = 1 -step GPTA milk, Old = multi-step GPTA milk Statistic Corr(New, Old) Corr(DYDg, DYD) Animals All bulls Genotyped bulls 0. 994 0. 992 0. 999 Corr(New, Old) SD old PTA milk SD new PTA milk Old milk trend New milk trend Young genomic 1995 -2005 cows 0. 966 540 552 1644 1430 University of Maryland Animal Science seminar (27) Paul Van. Raden 2016
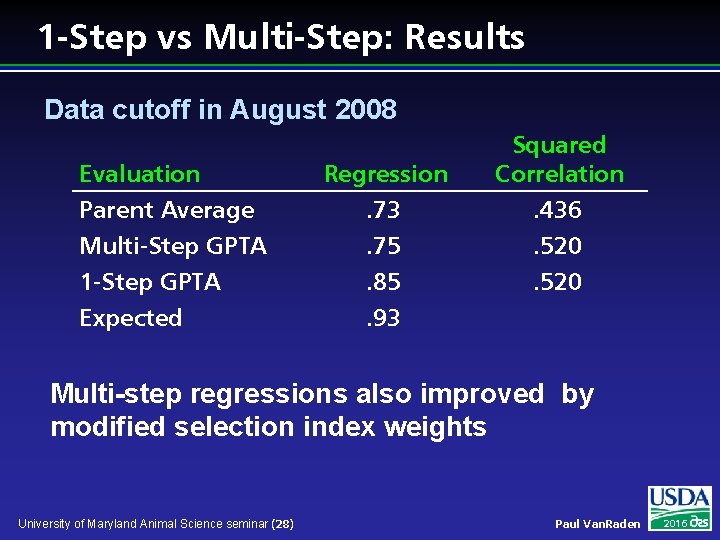
1 -Step vs Multi-Step: Results Data cutoff in August 2008 Evaluation Parent Average Multi-Step GPTA 1 -Step GPTA Expected Regression. 73. 75. 85. 93 Squared Correlation. 436. 520 Multi-step regressions also improved by modified selection index weights University of Maryland Animal Science seminar (28) Paul Van. Raden 2016
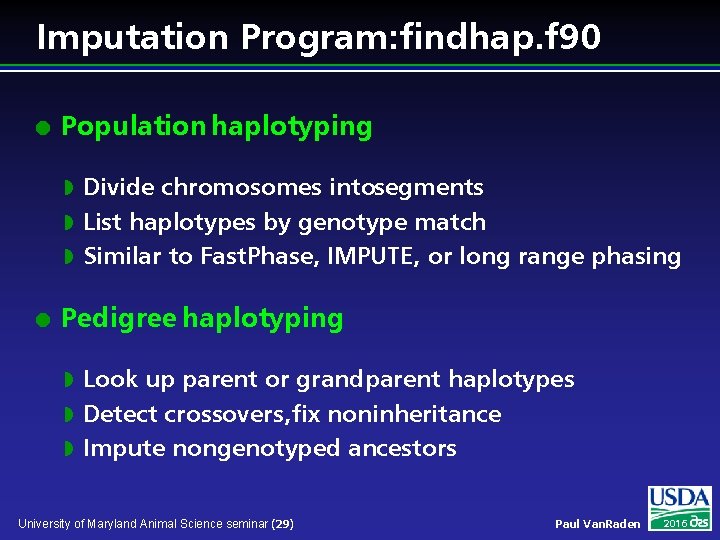
Imputation Program: findhap. f 90 l Population haplotyping Divide chromosomes intosegments w List haplotypes by genotype match w Similar to Fast. Phase, IMPUTE, or long range phasing w l Pedigree haplotyping Look up parent or grandparent haplotypes w Detect crossovers, fix noninheritance w Impute nongenotyped ancestors w University of Maryland Animal Science seminar (29) Paul Van. Raden 2016
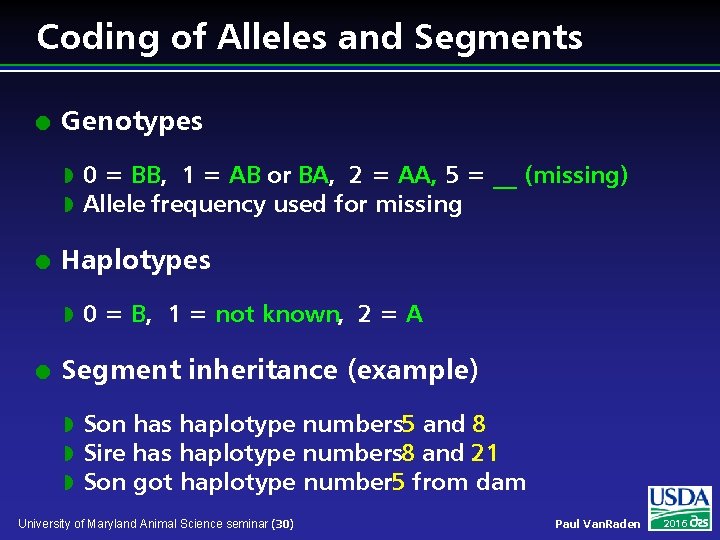
Coding of Alleles and Segments l Genotypes w w l Haplotypes w l 0 = BB, 1 = AB or BA, 2 = AA, 5 = __ (missing) Allele frequency used for missing 0 = B, 1 = not known, 2 = A Segment inheritance (example) w w w Son has haplotype numbers 5 and 8 Sire has haplotype numbers 8 and 21 Son got haplotype number 5 from dam University of Maryland Animal Science seminar (30) Paul Van. Raden 2016
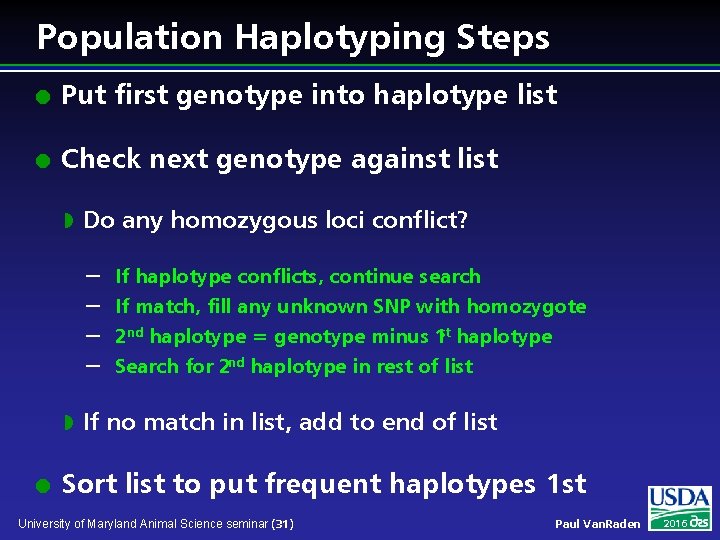
Population Haplotyping Steps l Put first genotype into haplotype list l Check next genotype against list w Do any homozygous loci conflict? − − w l If haplotype conflicts, continue search If match, fill any unknown SNP with homozygote 2 nd haplotype = genotype minus 1 st haplotype Search for 2 nd haplotype in rest of list If no match in list, add to end of list Sort list to put frequent haplotypes 1 st University of Maryland Animal Science seminar (31) Paul Van. Raden 2016
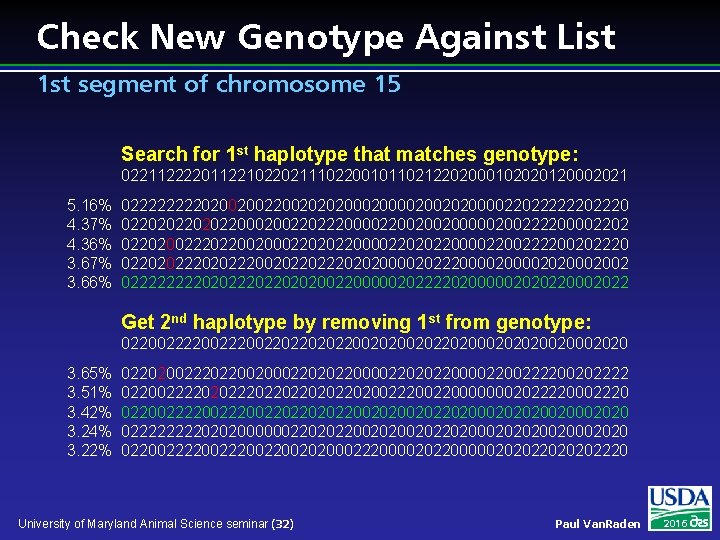
Check New Genotype Against List 1 st segment of chromosome 15 Search for 1 st haplotype that matches genotype: 022112222011221022021110220010110212202000102020120002021 5. 16% 4. 37% 4. 36% 3. 67% 3. 66% 02222020020020202000020020200002202222220 02202022000200220222000022002002000002002222000022020022202200200022020220000222200202220 02202022200202202020000202220000202002 0222202022020200220000020222202000002020220002022 Get 2 nd haplotype by removing 1 st from genotype: 022002220022022002020020220200020202002020 3. 65% 3. 51% 3. 42% 3. 24% 3. 22% 022020022202200200022020220000222200202222 02200222202022022020022200000002022220002220 022002220022022002020020220200020202002020 02222020200000022020220020220200020202002020 0220022200202000222000020220000020202220 University of Maryland Animal Science seminar (32) Paul Van. Raden 2016
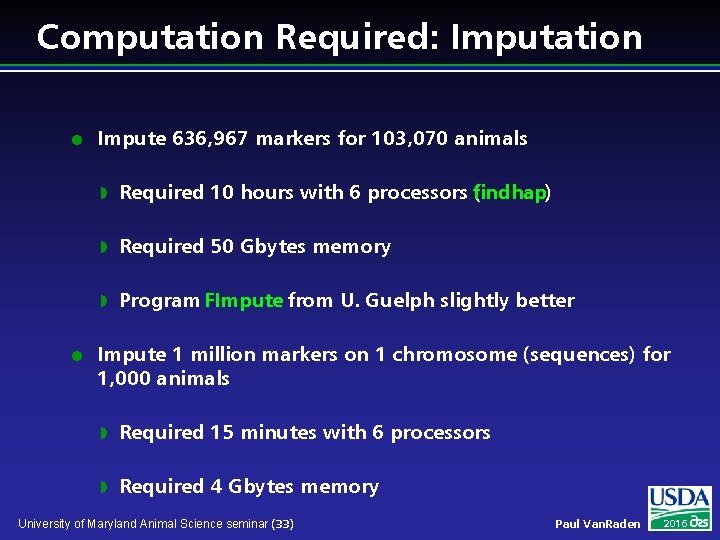
Computation Required: Imputation l l Impute 636, 967 markers for 103, 070 animals w Required 10 hours with 6 processors findhap) ( w Required 50 Gbytes memory w Program FImpute from U. Guelph slightly better Impute 1 million markers on 1 chromosome (sequences) for 1, 000 animals w Required 15 minutes with 6 processors w Required 4 Gbytes memory University of Maryland Animal Science seminar (33) Paul Van. Raden 2016
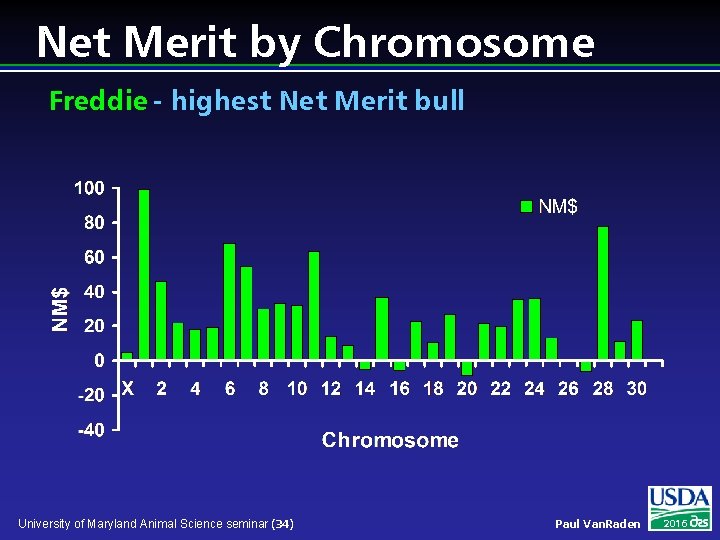
Net Merit by Chromosome Freddie - highest Net Merit bull University of Maryland Animal Science seminar (34) Paul Van. Raden 2016
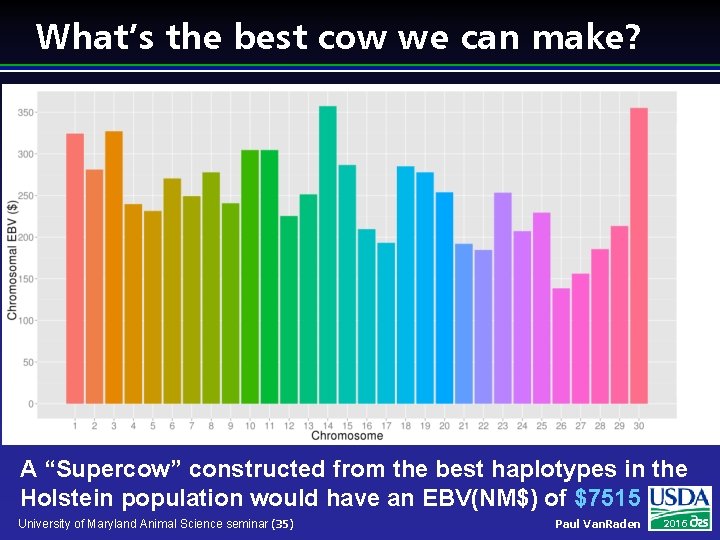
What’s the best cow we can make? A “Supercow” constructed from the best haplotypes in the Holstein population would have an EBV(NM$) of $7515 University of Maryland Animal Science seminar (35) Paul Van. Raden 2016
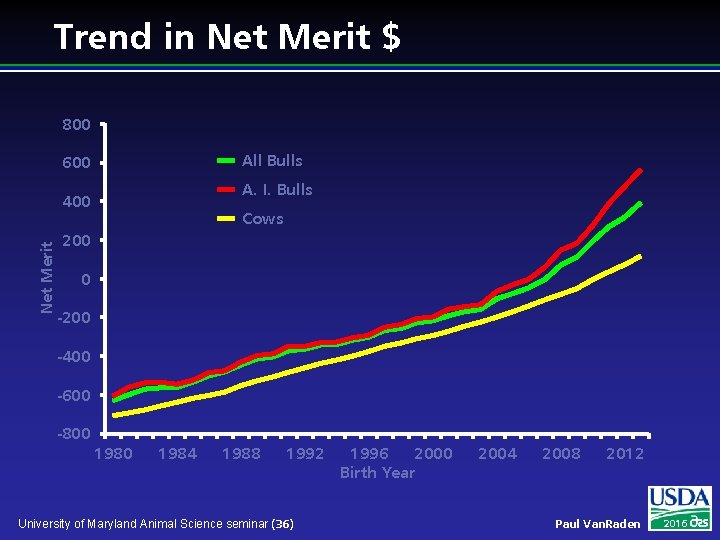
Trend in Net Merit $ 800 All Bulls 600 A. I. Bulls Net Merit 400 Cows 200 0 -200 -400 -600 -800 1984 1988 1992 University of Maryland Animal Science seminar (36) 1996 2000 Birth Year 2004 2008 2012 Paul Van. Raden 2016
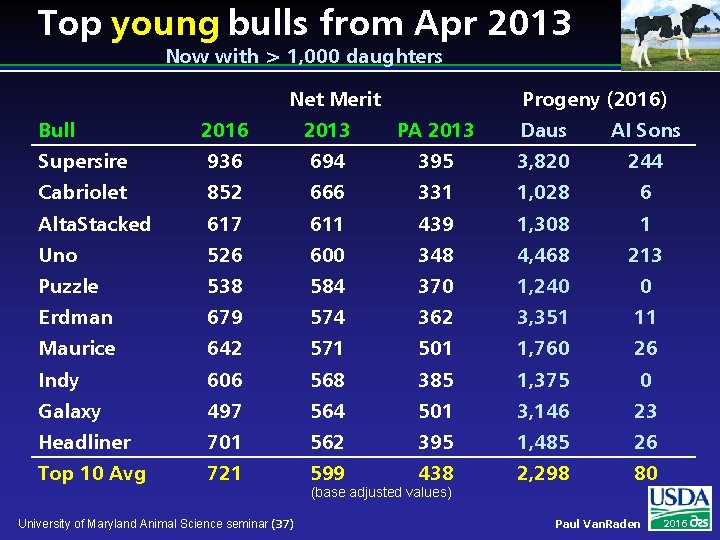
Top young bulls from Apr 2013 Now with > 1, 000 daughters Net Merit Progeny (2016) Bull 2016 2013 PA 2013 Daus AI Sons Supersire 936 694 395 3, 820 244 Cabriolet 852 666 331 1, 028 6 Alta. Stacked 617 611 439 1, 308 1 Uno 526 600 348 4, 468 213 Puzzle 538 584 370 1, 240 0 Erdman 679 574 362 3, 351 11 Maurice 642 571 501 1, 760 26 Indy 606 568 385 1, 375 0 Galaxy 497 564 501 3, 146 23 Headliner 701 562 395 1, 485 26 Top 10 Avg 721 599 438 2, 298 80 University of Maryland Animal Science seminar (37) (base adjusted values) Paul Van. Raden 2016
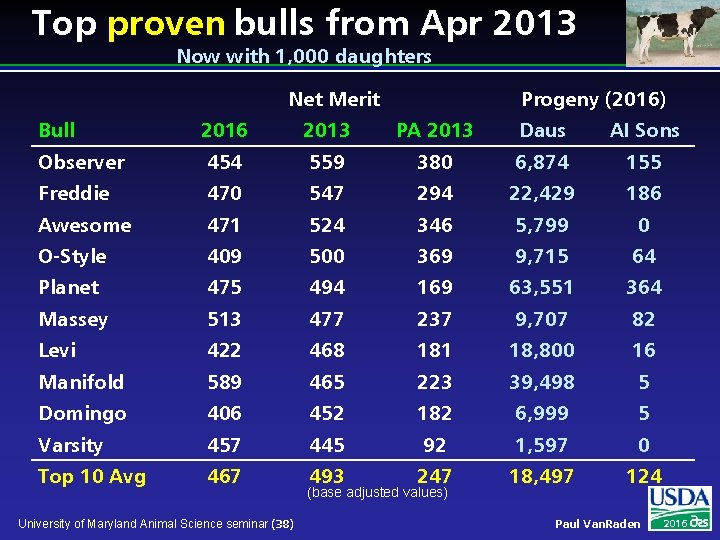
Top proven bulls from Apr 2013 Now with 1, 000 daughters Net Merit Progeny (2016) Bull 2016 2013 PA 2013 Daus AI Sons Observer 454 559 380 6, 874 155 Freddie 470 547 294 22, 429 186 Awesome 471 524 346 5, 799 0 O-Style 409 500 369 9, 715 64 Planet 475 494 169 63, 551 364 Massey 513 477 237 9, 707 82 Levi 422 468 181 18, 800 16 Manifold 589 465 223 39, 498 5 Domingo 406 452 182 6, 999 5 Varsity 457 445 92 1, 597 0 Top 10 Avg 467 493 247 18, 497 124 University of Maryland Animal Science seminar (38) (base adjusted values) Paul Van. Raden 2016
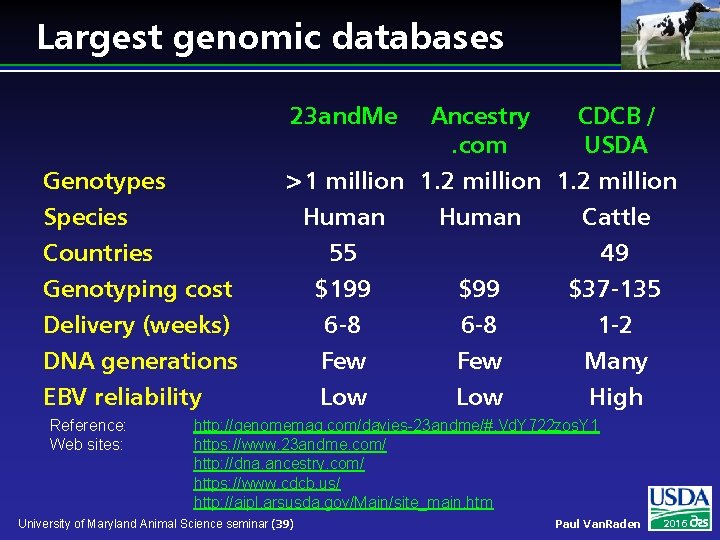
Largest genomic databases 23 and. Me Ancestry CDCB /. com USDA >1 million 1. 2 million Human Cattle Genotypes Species Countries Genotyping cost Delivery (weeks) DNA generations 55 $199 6 -8 Few $99 6 -8 Few 49 $37 -135 1 -2 Many EBV reliability Low High Reference: Web sites: http: //genomemag. com/davies-23 andme/#. Vd. Y 722 zos. Y 1 https: //www. 23 andme. com/ http: //dna. ancestry. com/ https: //www. cdcb. us/ http: //aipl. arsusda. gov/Main/site_main. htm University of Maryland Animal Science seminar (39) Paul Van. Raden 2016
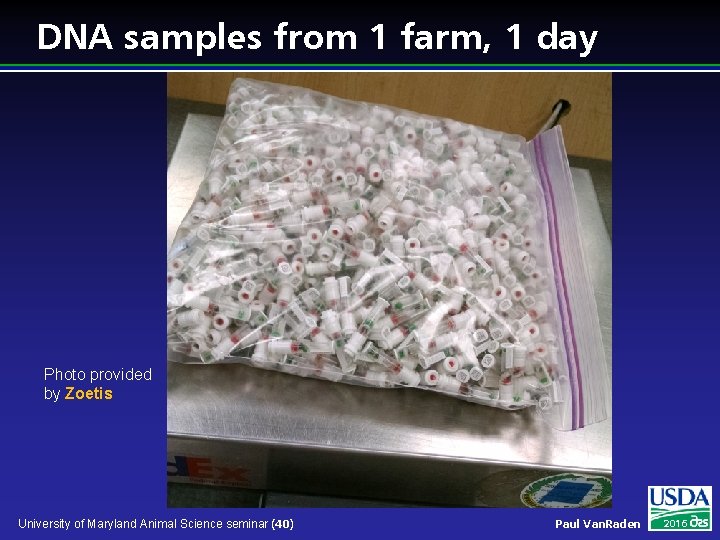
DNA samples from 1 farm, 1 day Photo provided by Zoetis University of Maryland Animal Science seminar (40) Paul Van. Raden 2016
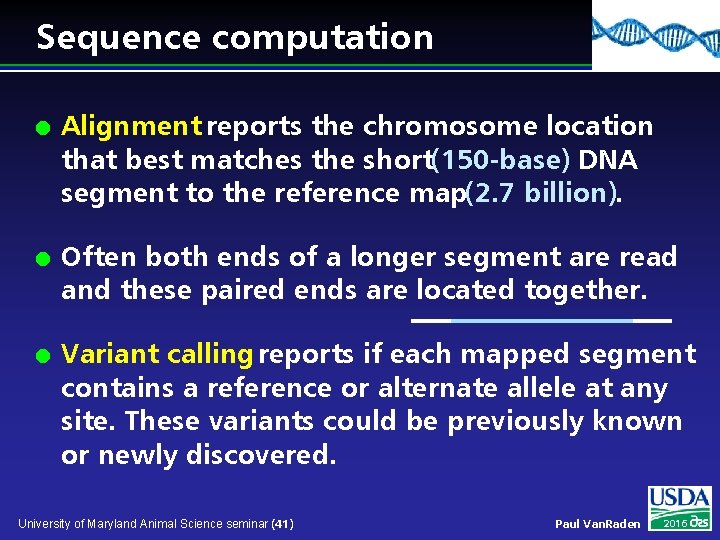
Sequence computation l l l Alignment reports the chromosome location that best matches the short(150 -base) DNA segment to the reference map(2. 7 billion). Often both ends of a longer segment are read and these paired ends are located together. Variant calling reports if each mapped segment contains a reference or alternate allele at any site. These variants could be previously known or newly discovered. University of Maryland Animal Science seminar (41) Paul Van. Raden 2016
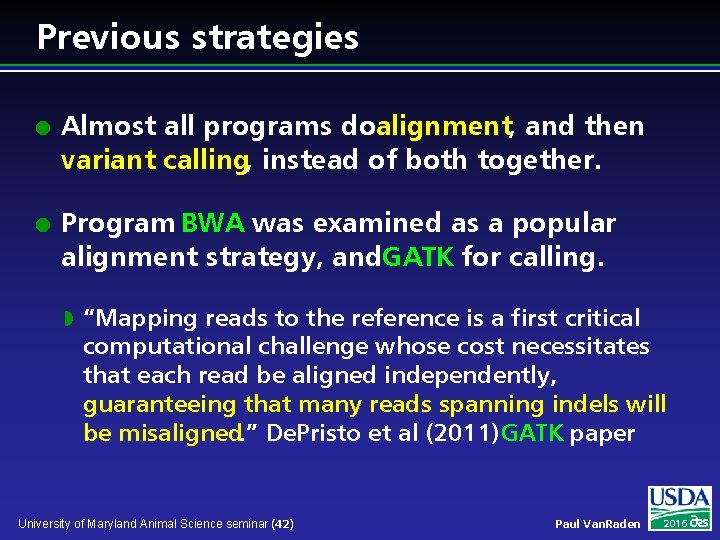
Previous strategies l l Almost all programs doalignment, and then variant calling, instead of both together. Program BWA was examined as a popular alignment strategy, and. GATK for calling. w “Mapping reads to the reference is a first critical computational challenge whose cost necessitates that each read be aligned independently, guaranteeing that many reads spanning indels will be misaligned. ” De. Pristo et al (2011) GATK paper University of Maryland Animal Science seminar (42) Paul Van. Raden 2016
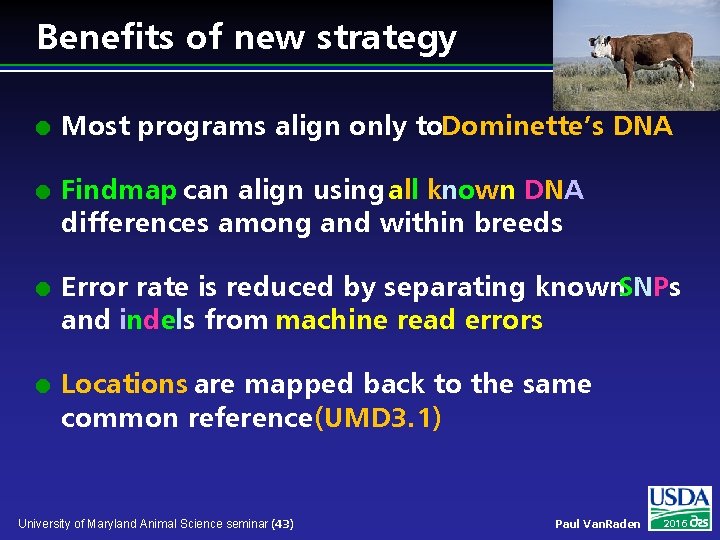
Benefits of new strategy l l Most programs align only to. Dominette’s DNA Findmap can align using all known DNA differences among and within breeds Error rate is reduced by separating known. SNPs and indels from machine read errors Locations are mapped back to the same common reference (UMD 3. 1) University of Maryland Animal Science seminar (43) Paul Van. Raden 2016
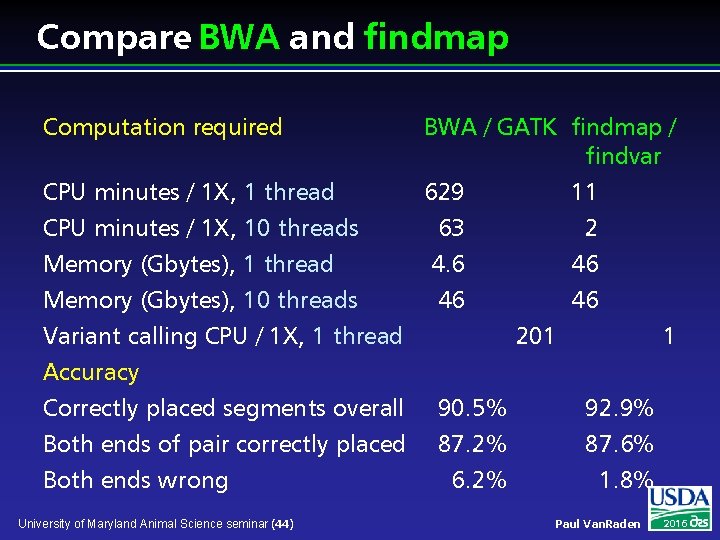
Compare BWA and findmap Computation required CPU minutes / 1 X, 1 thread CPU minutes / 1 X, 10 threads Memory (Gbytes), 1 thread Memory (Gbytes), 10 threads Variant calling CPU / 1 X, 1 thread Accuracy Correctly placed segments overall Both ends of pair correctly placed Both ends wrong University of Maryland Animal Science seminar (44) BWA / GATK findmap / findvar 629 11 63 2 4. 6 46 46 46 201 90. 5% 87. 2% 6. 2% 1 92. 9% 87. 6% 1. 8% Paul Van. Raden 2016
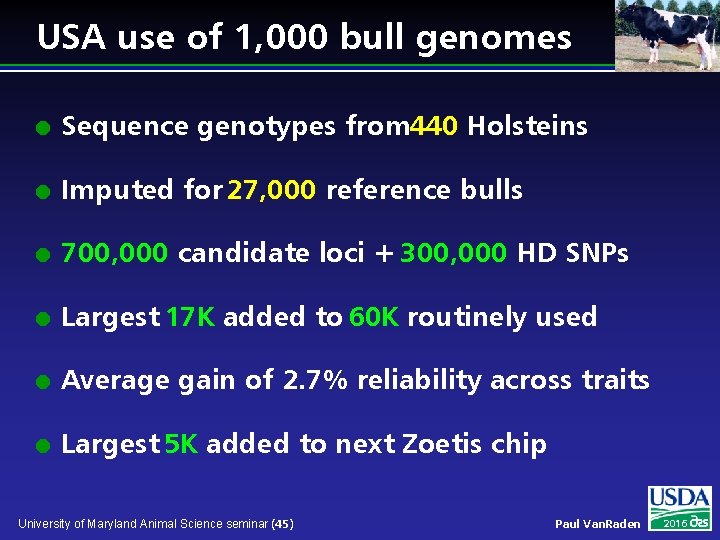
USA use of 1, 000 bull genomes l Sequence genotypes from 440 Holsteins l Imputed for 27, 000 reference bulls l 700, 000 candidate loci + 300, 000 HD SNPs l Largest 17 K added to 60 K routinely used l Average gain of 2. 7% reliability across traits l Largest 5 K added to next Zoetis chip University of Maryland Animal Science seminar (45) Paul Van. Raden 2016
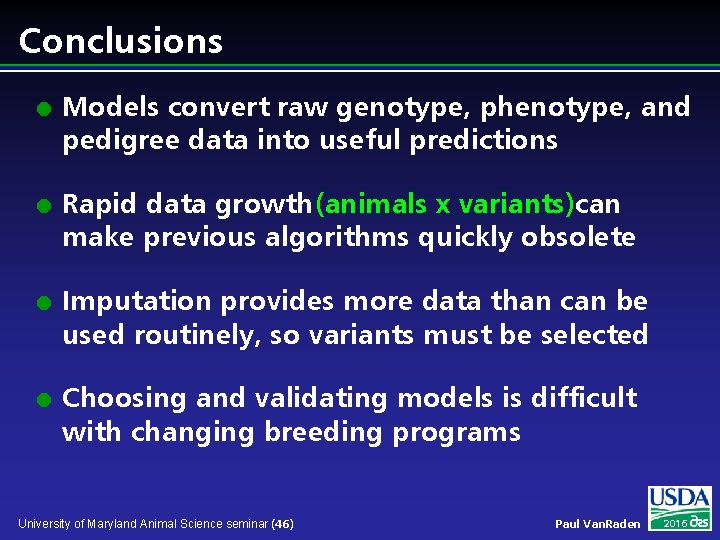
Conclusions l l Models convert raw genotype, phenotype, and pedigree data into useful predictions Rapid data growth(animals x variants)can make previous algorithms quickly obsolete Imputation provides more data than can be used routinely, so variants must be selected Choosing and validating models is difficult with changing breeding programs University of Maryland Animal Science seminar (46) Paul Van. Raden 2016
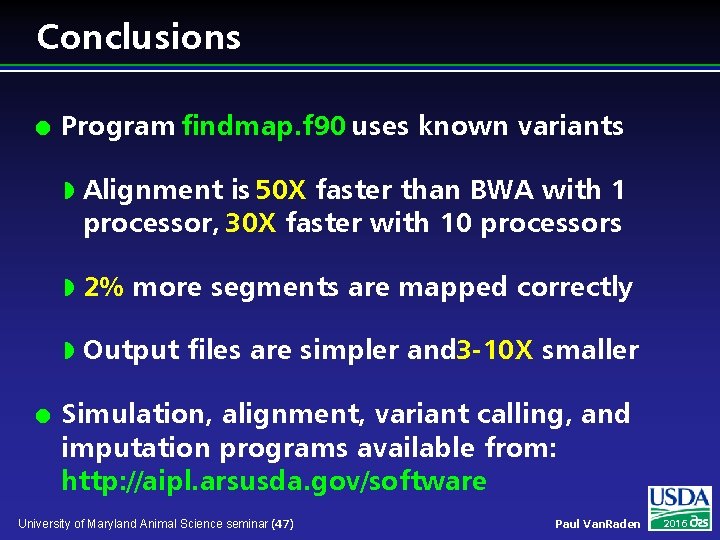
Conclusions l l Program findmap. f 90 uses known variants w Alignment is 50 X faster than BWA with 1 processor, 30 X faster with 10 processors w 2% more segments are mapped correctly w Output files are simpler and 3 -10 X smaller Simulation, alignment, variant calling, and imputation programs available from: http: //aipl. arsusda. gov/software University of Maryland Animal Science seminar (47) Paul Van. Raden 2016
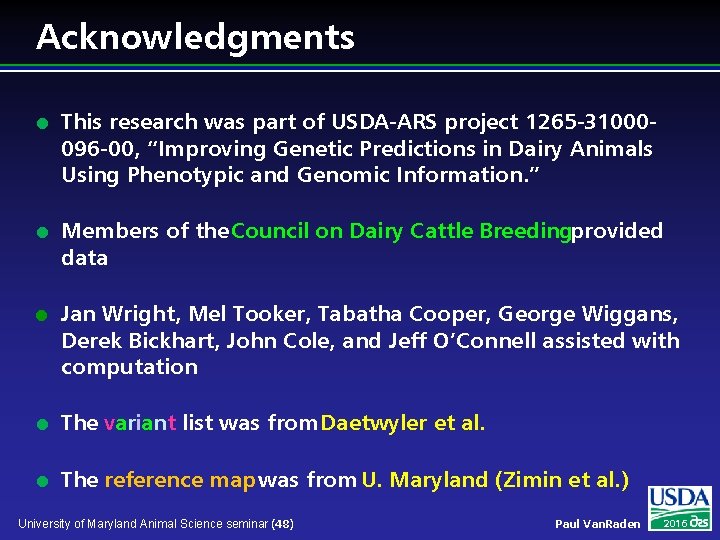
Acknowledgments l This research was part of USDA-ARS project 1265 -31000096 -00, “Improving Genetic Predictions in Dairy Animals Using Phenotypic and Genomic Information. ” Members of the Council on Dairy Cattle Breedingprovided data Jan Wright, Mel Tooker, Tabatha Cooper, George Wiggans, Derek Bickhart, John Cole, and Jeff O’Connell assisted with computation The variant list was from Daetwyler et al. The reference map was from U. Maryland (Zimin et al. ) University of Maryland Animal Science seminar (48) Paul Van. Raden 2016
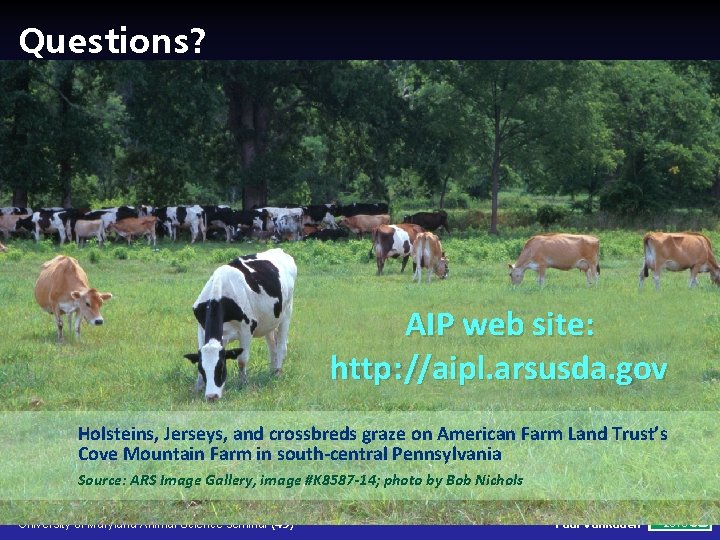
Questions? AIP web site: http : //aipl. arsusda. gov Holsteins, Jerseys, and crossbreds graze on American Farm Land Trust’s Cove Mountain Farm in south-central Pennsylvania Source: ARS Image Gallery, image #K 8587 -14; photo by Bob Nichols University of Maryland Animal Science seminar (49) Paul Van. Raden 2016
Paul raden
University of maryland
Principle of genomic equivalence
Genomic england
Genomic england
Genomic england
Genomic instability
Genomic
Genomic imprinting definition
Genomic signal processing
Comparative genomic hybridization animation
Genomic equivalence definition
Boston university pemba
Restitution évaluations nationales cp
Nau student evaluations
Pharmacoeconomic evaluations amcp
What is non discriminatory evaluation
Uri idea evaluation
Shsu idea evaluations
Nama-nama keturunan prabu siliwangi
Lkp uin jkt
Elearning radenintan.ac.id
Nggrantes basa kramane
Wtax compensation table
Expanded withholding tax computation
Expanded withholding tax computation
Form 2306
Fertilizer computation examples
Eecs 1019
Sipser, m: introduction to the theory of computation
How to compute mcit
Income tax computation format
Iv fluid computation
Income tax computation format
Data cube computation methods
Drug computation
Data cube computation
Index de computation
How to calculate iv fluid
Cuts of a distributed computation
2160704
5 withholding tax on rent
Computation of wealth tax
Board feet calculator
Generalized transition graph
Types of languages in theory of computation
Pagerank computation
The pagerank citation ranking: bringing order to the web
The pagerank citation ranking: bringing order to the web
Binary search in secure computation