Verifying Satellite Precipitation Estimates for Weather and Hydrological
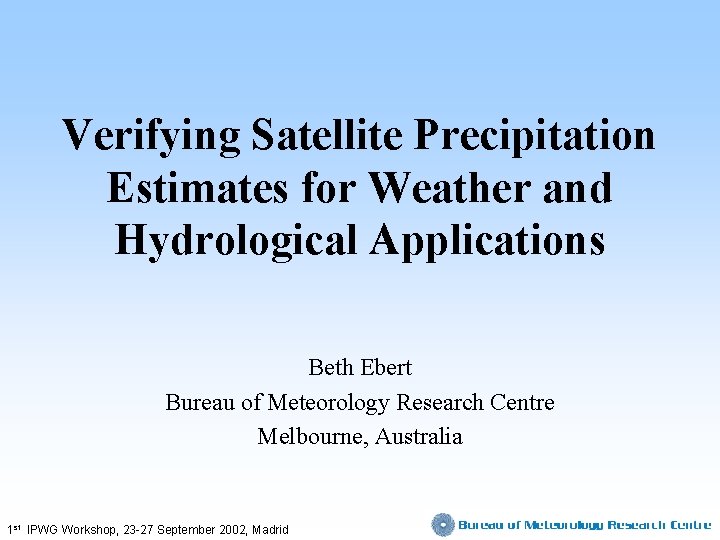
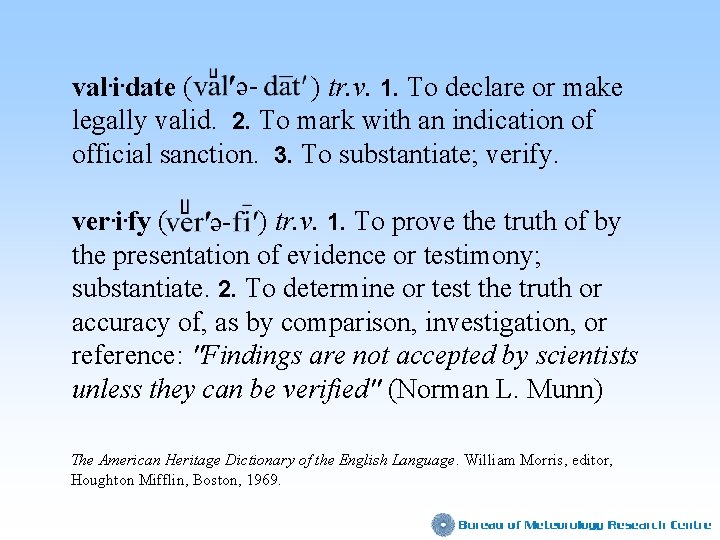
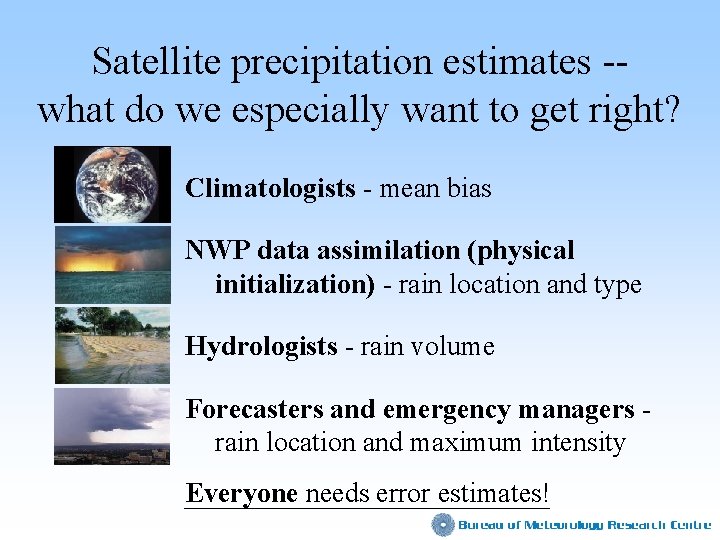
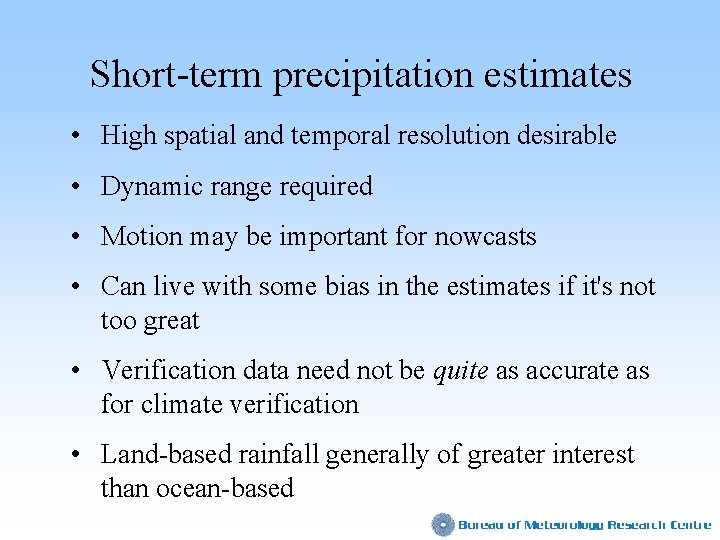
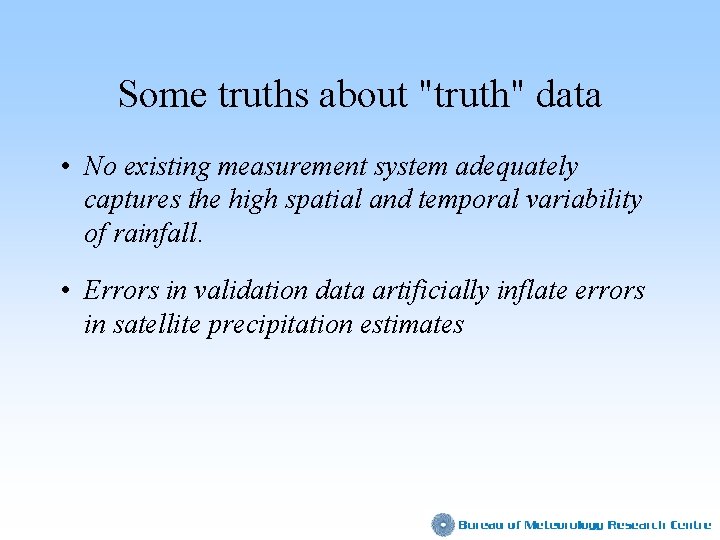
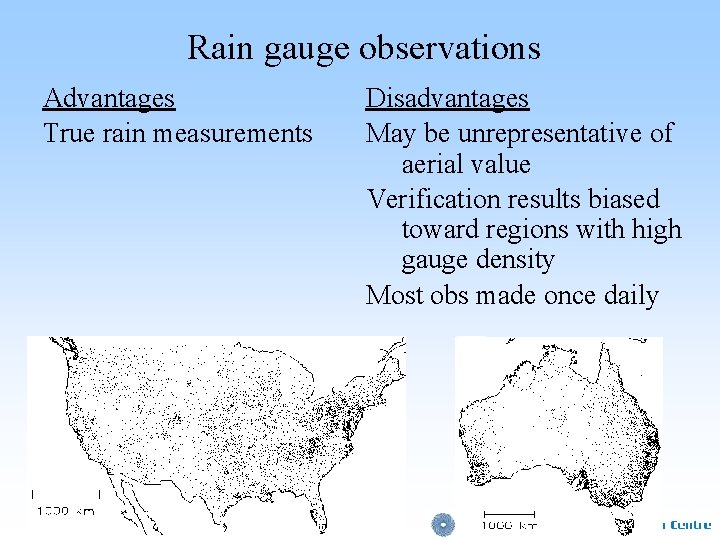
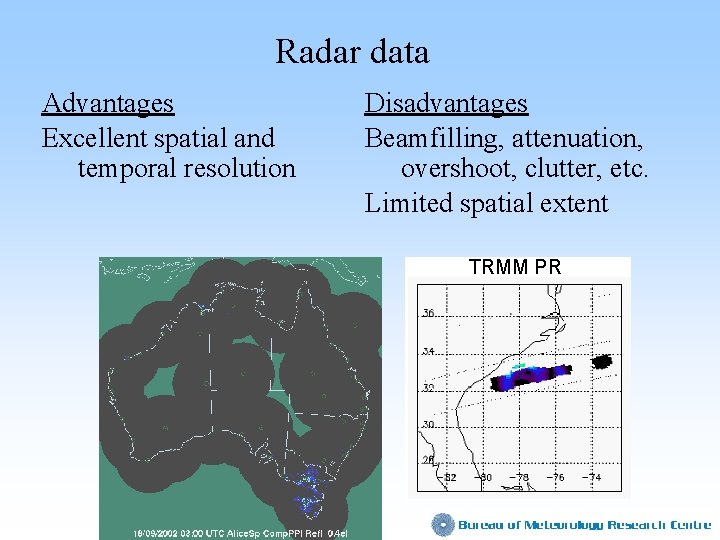
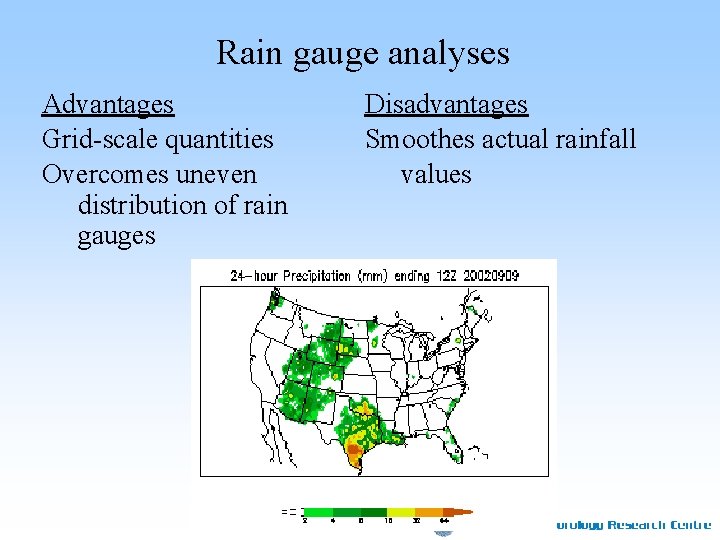
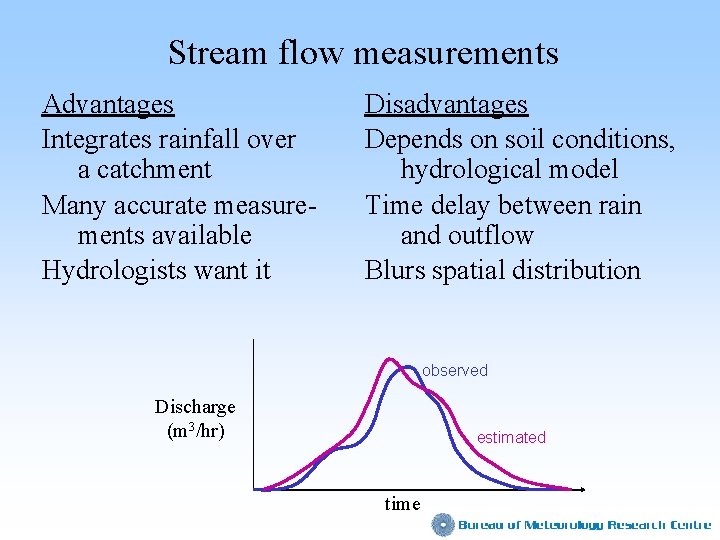
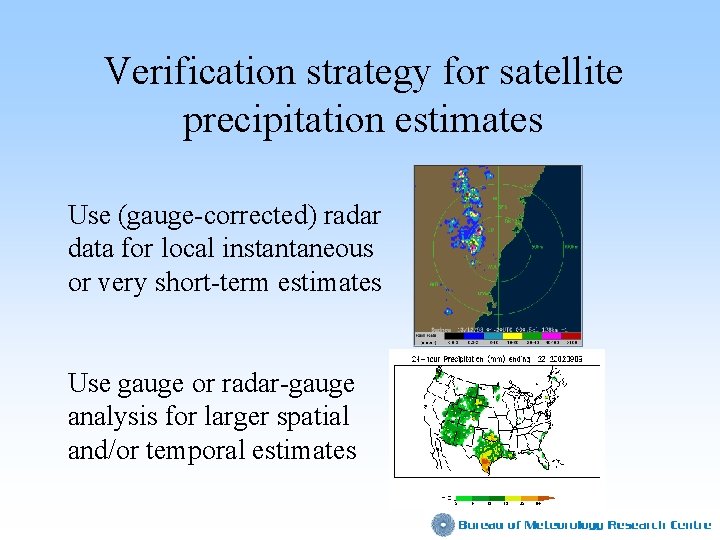
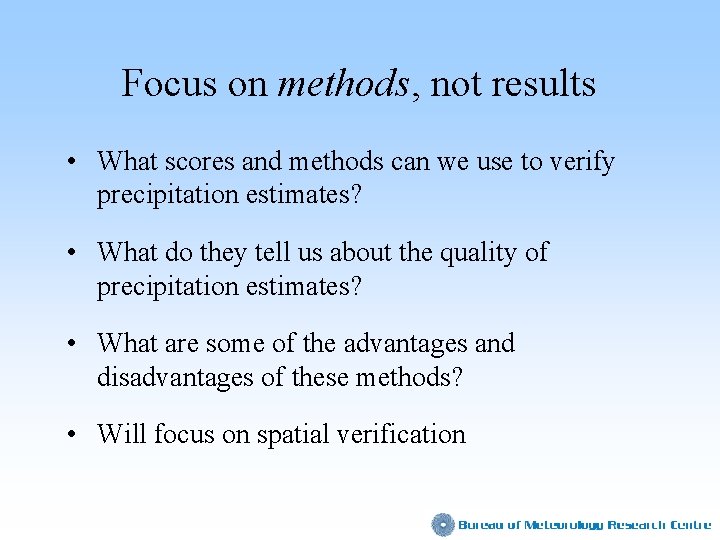
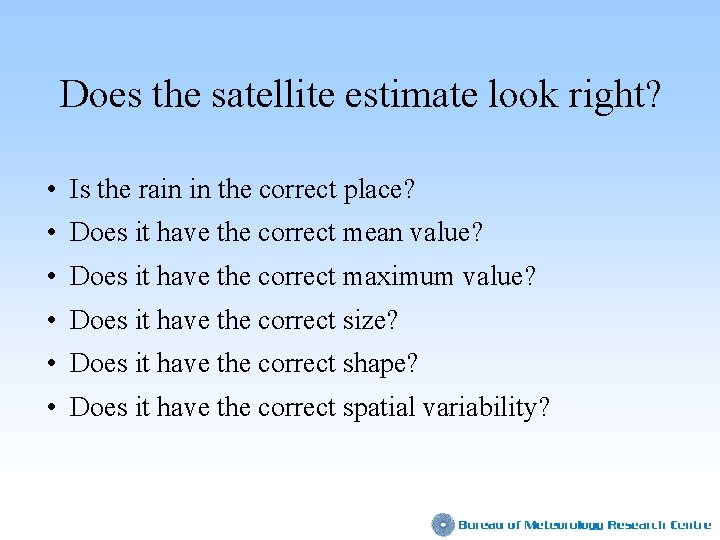
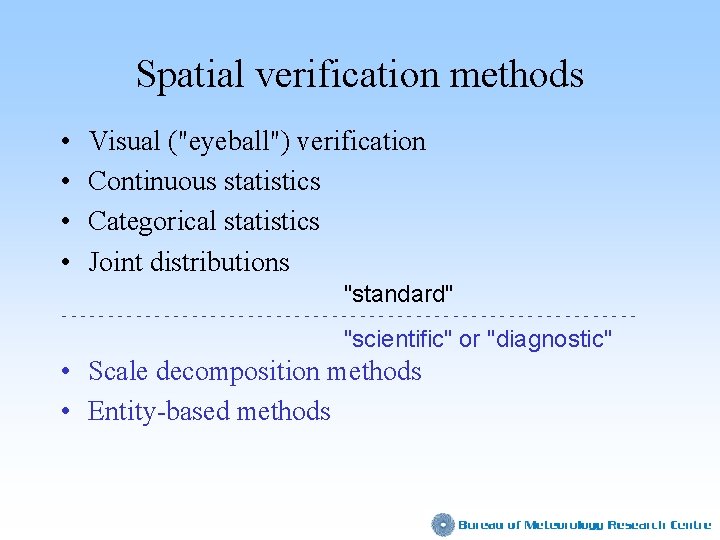
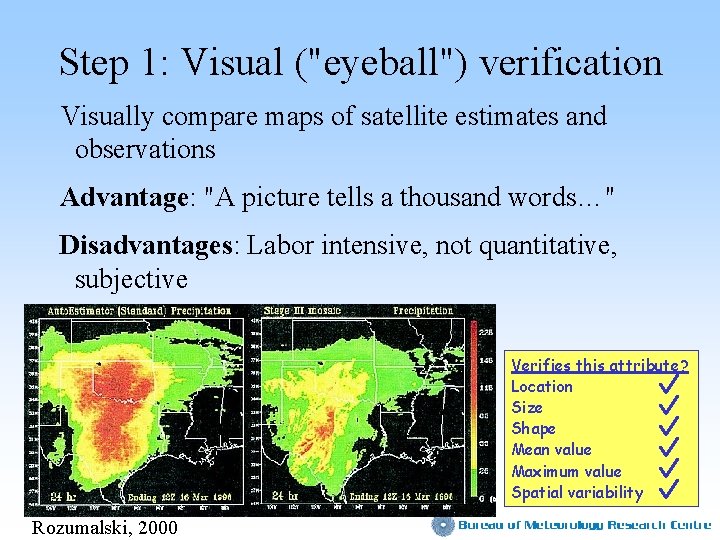
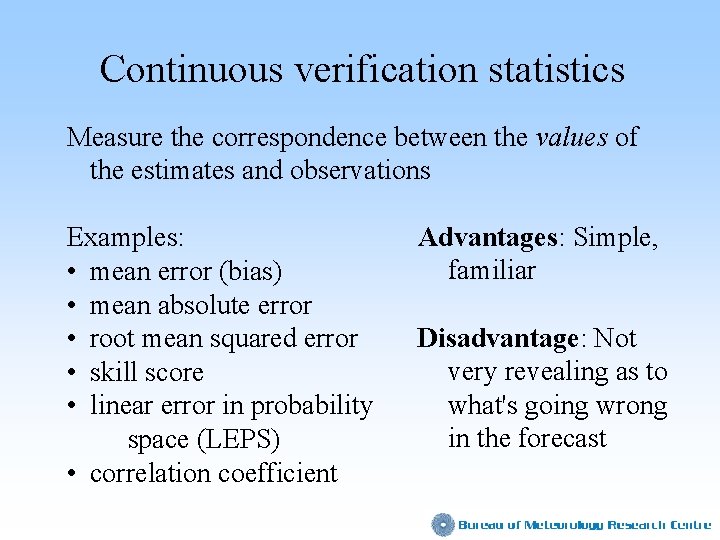
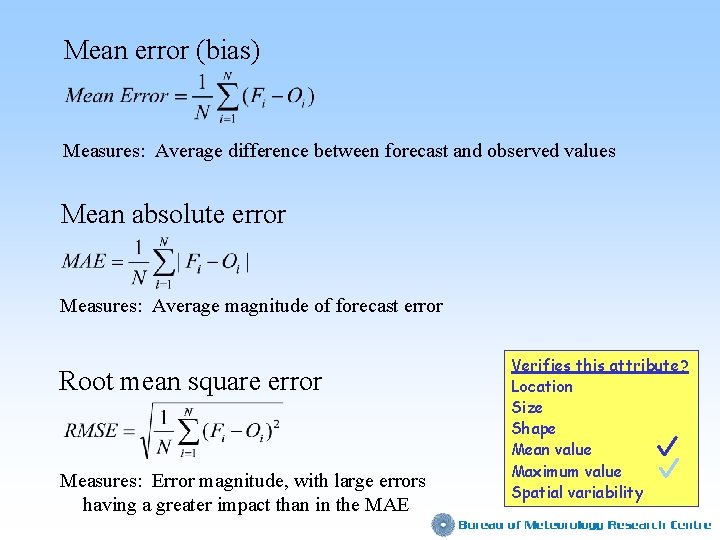
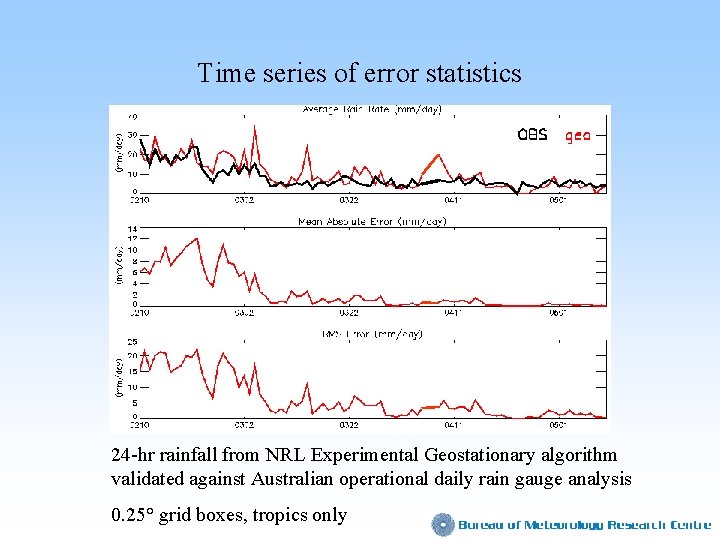
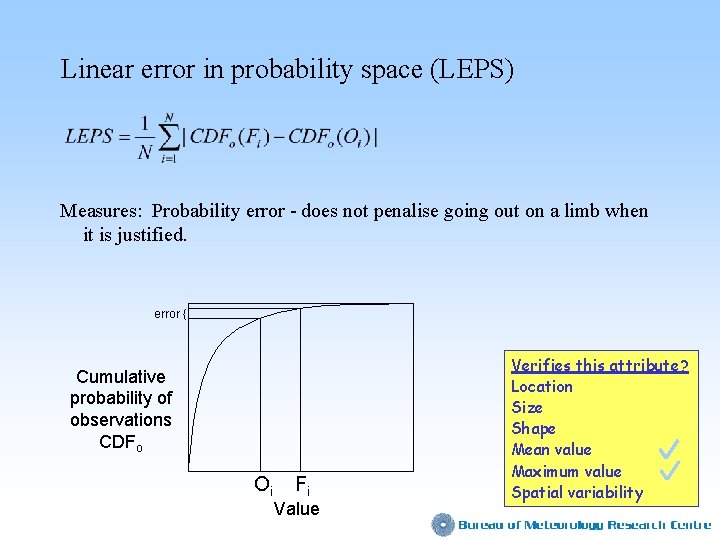
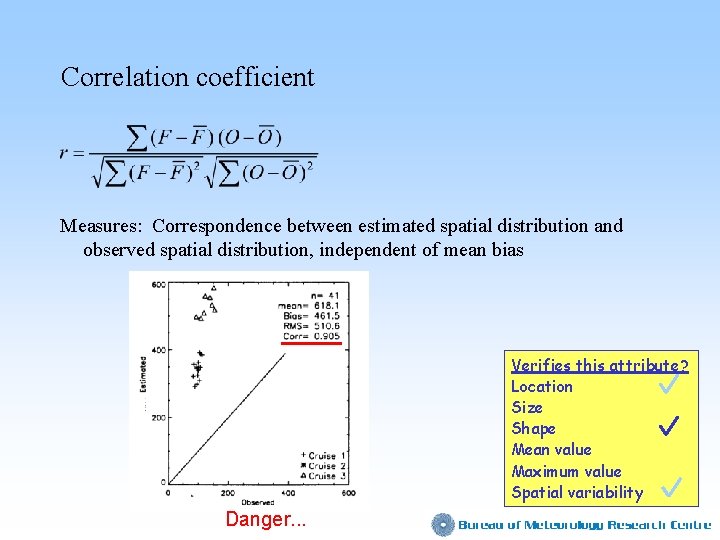
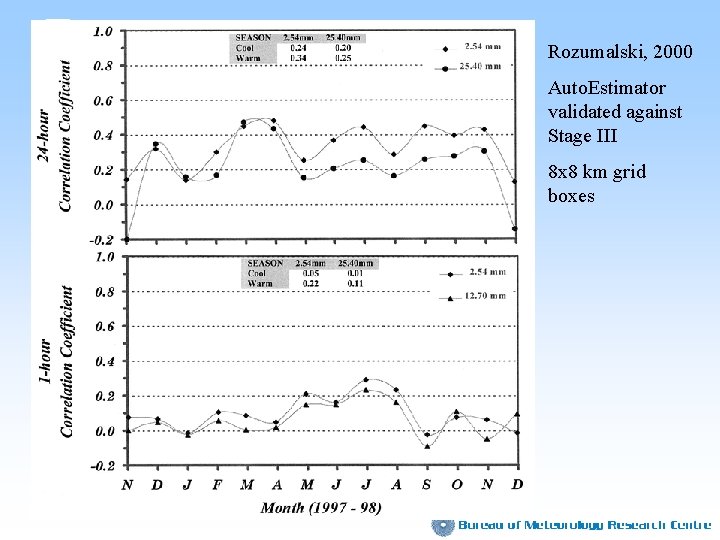
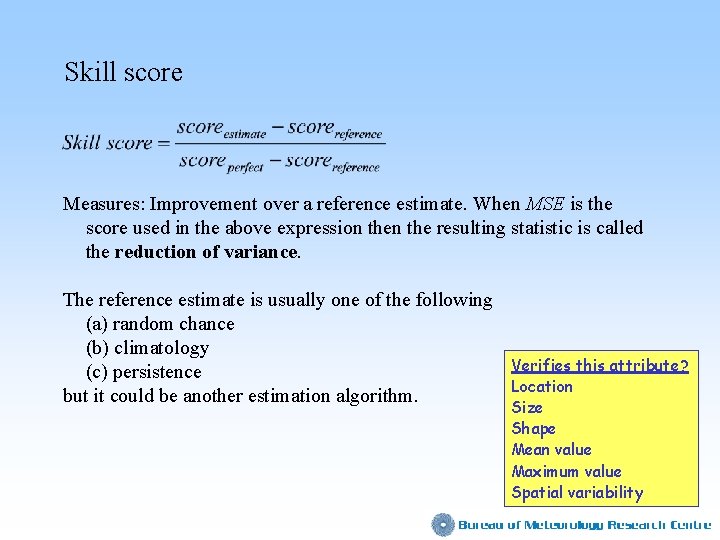
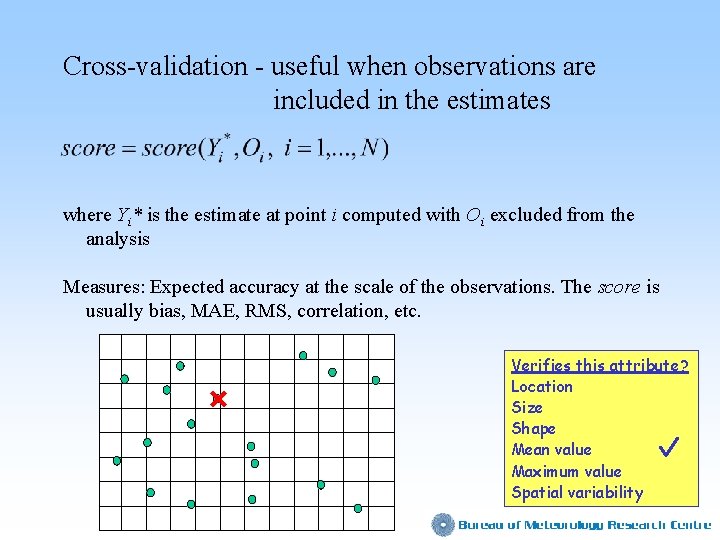
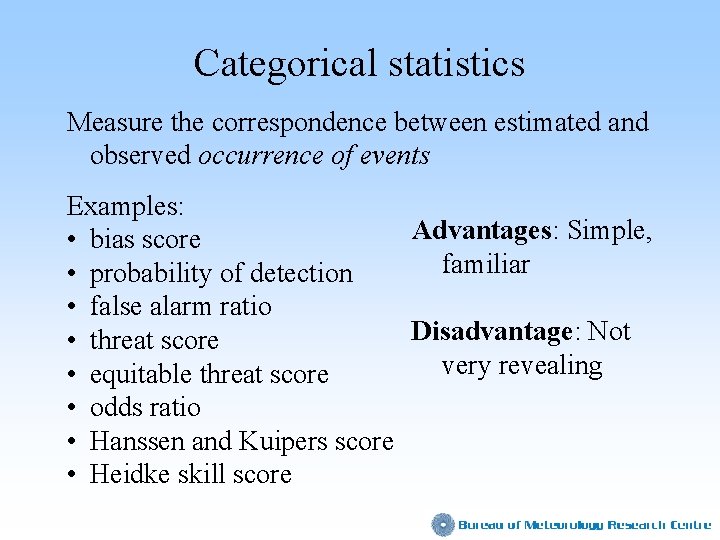
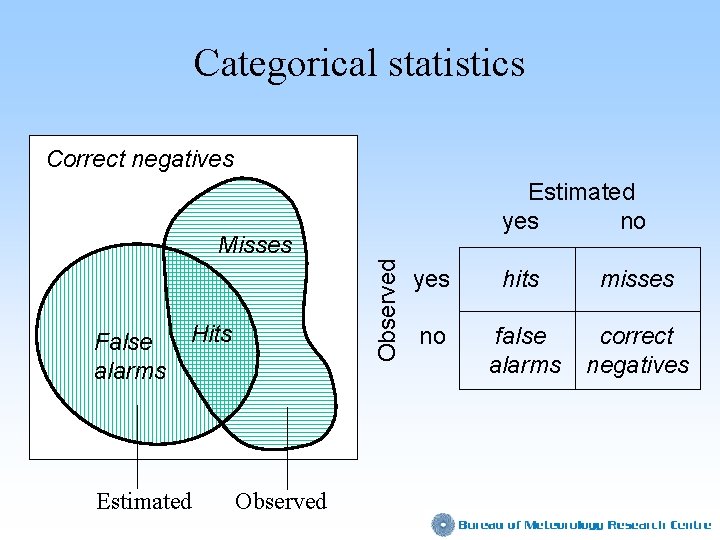
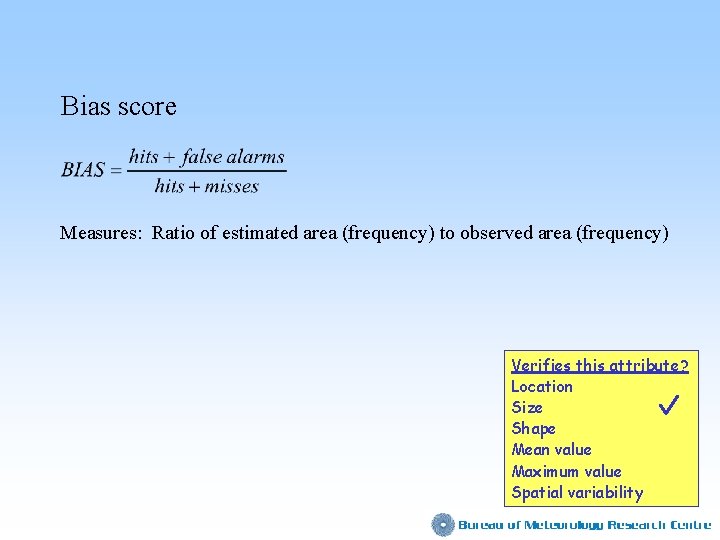
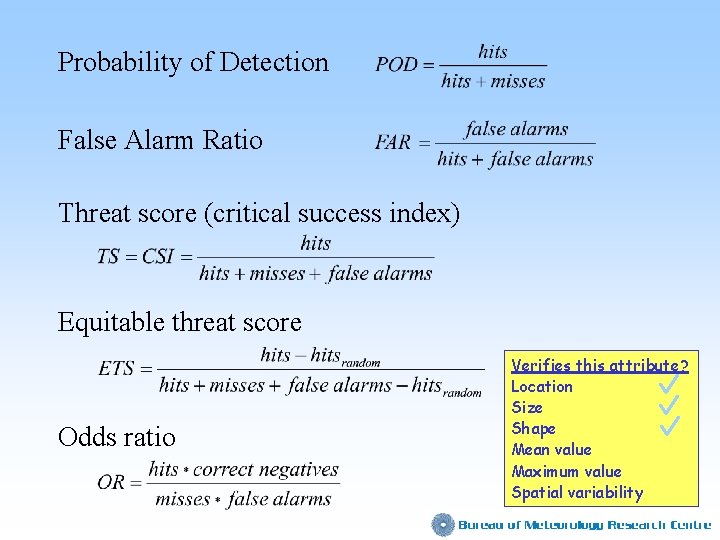
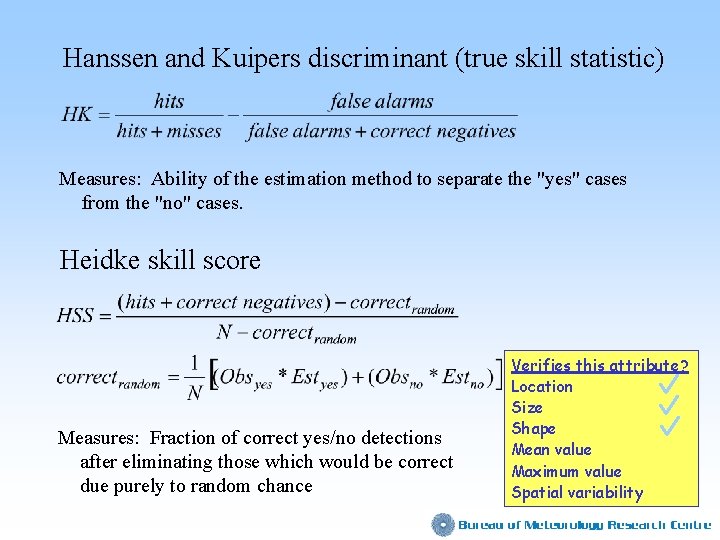
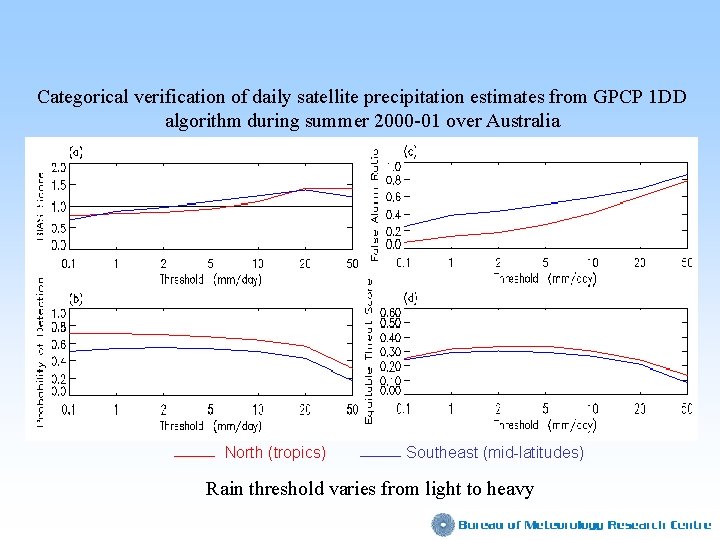
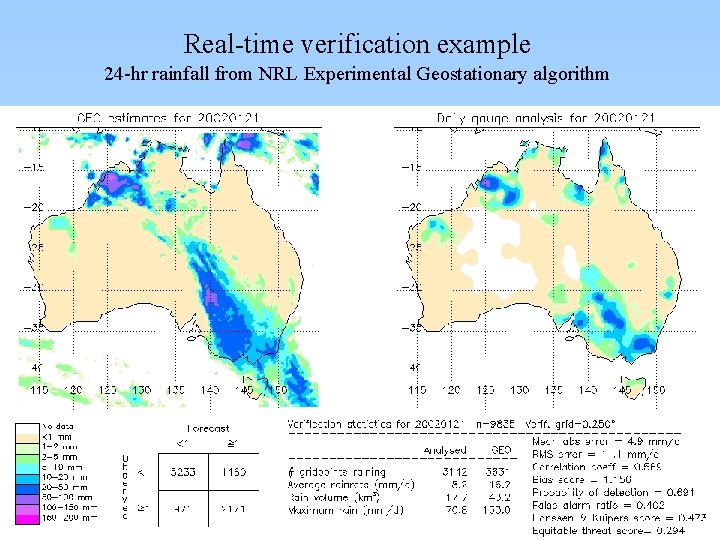
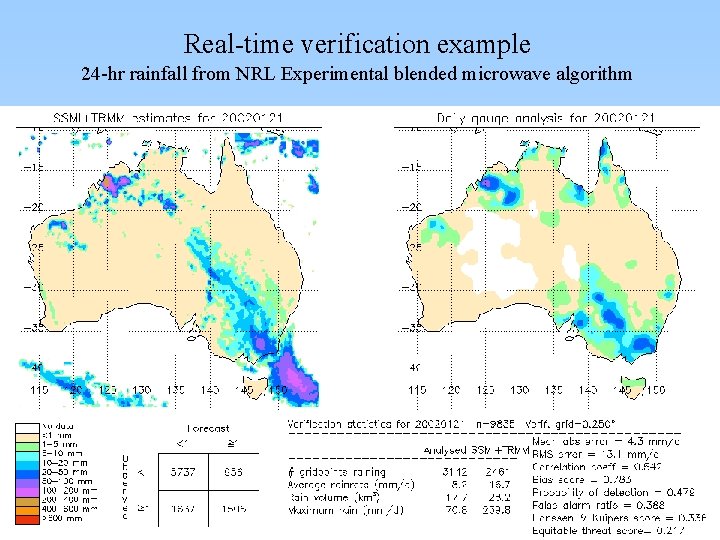
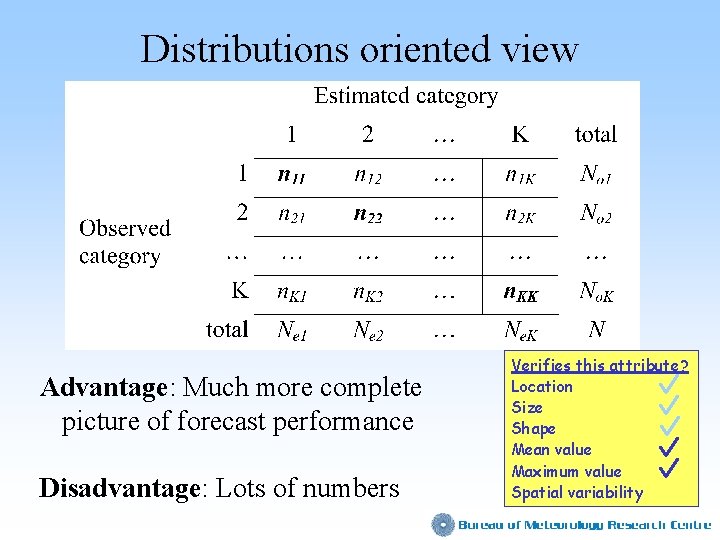
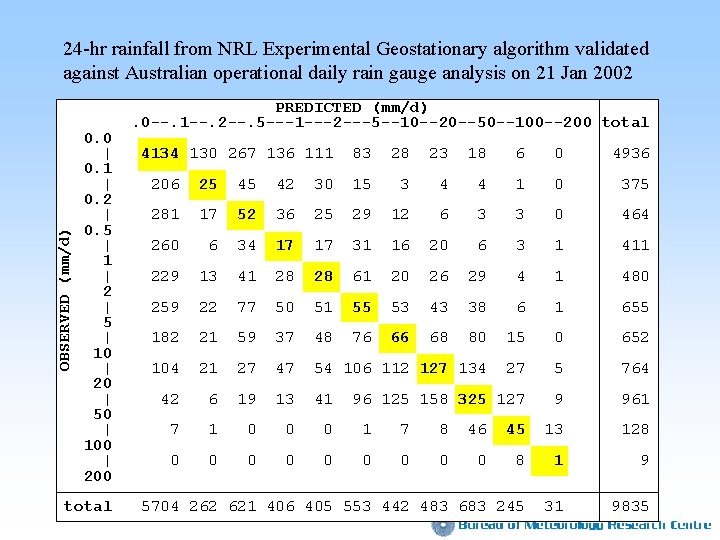
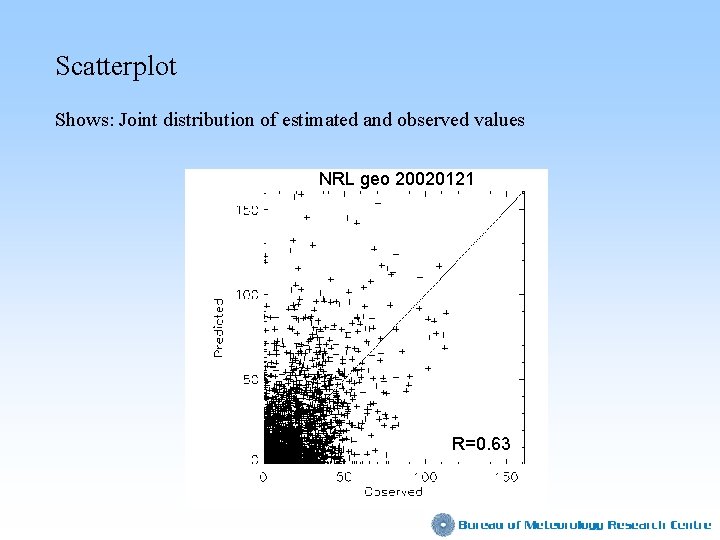
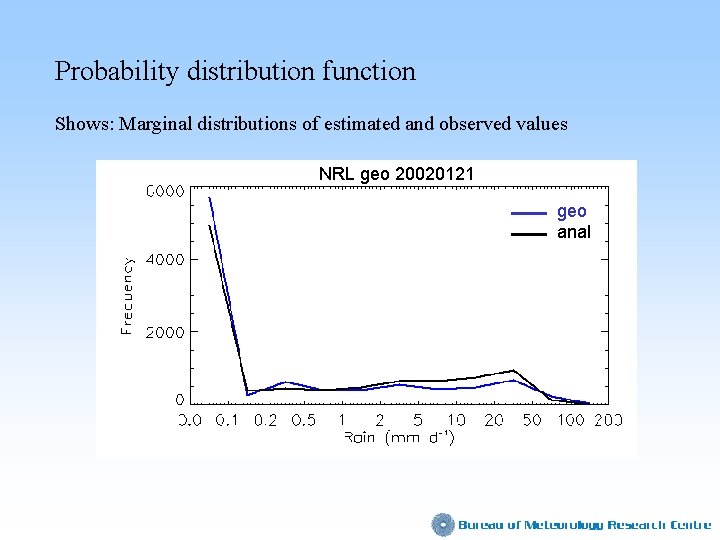
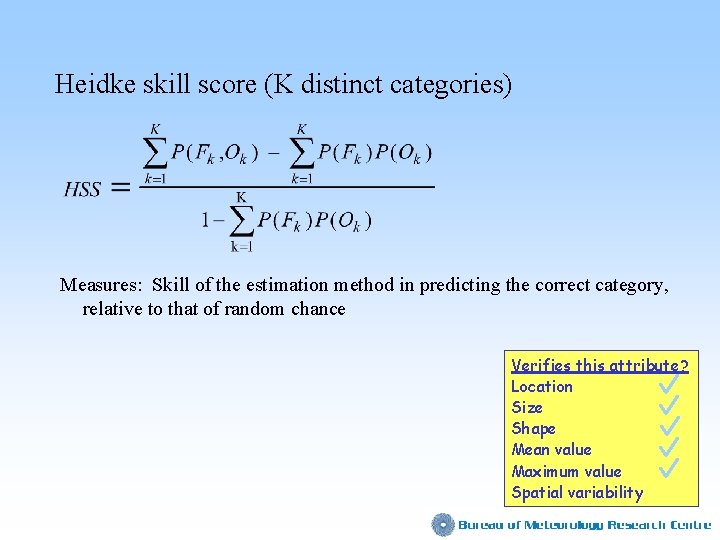
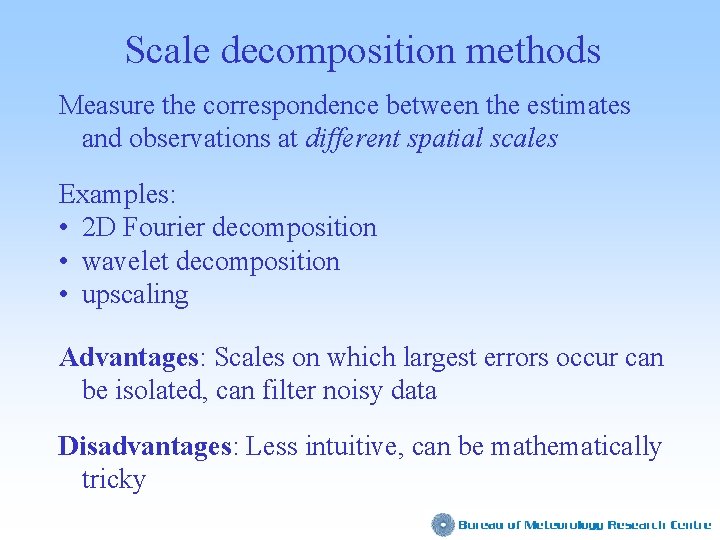
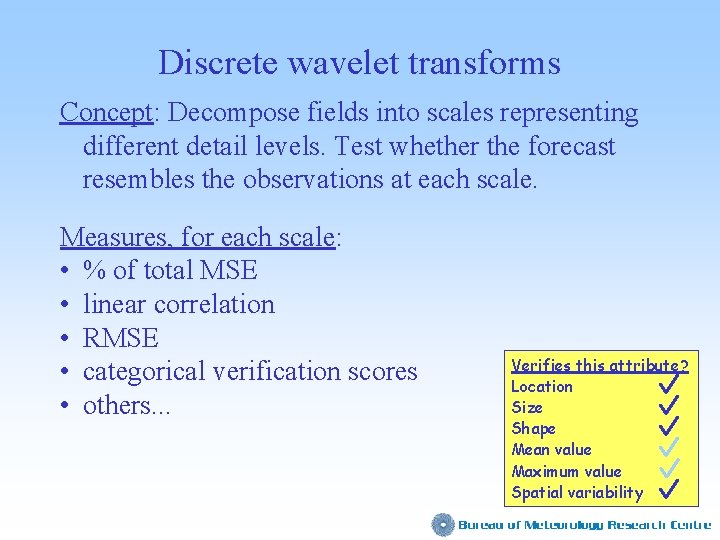
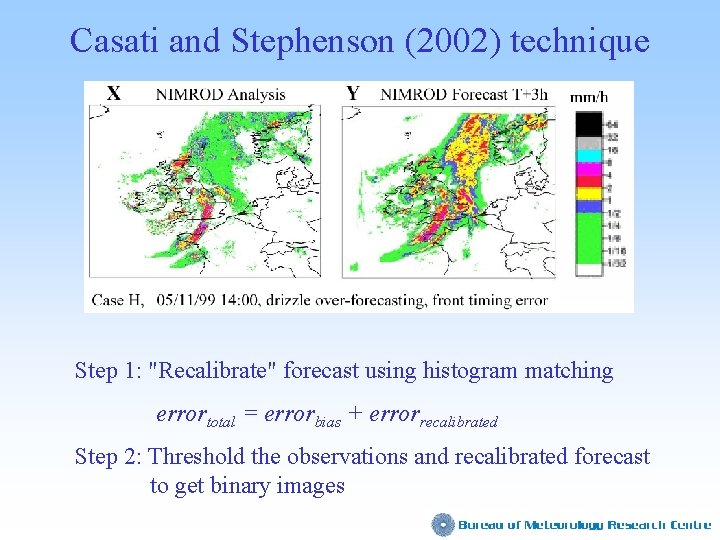
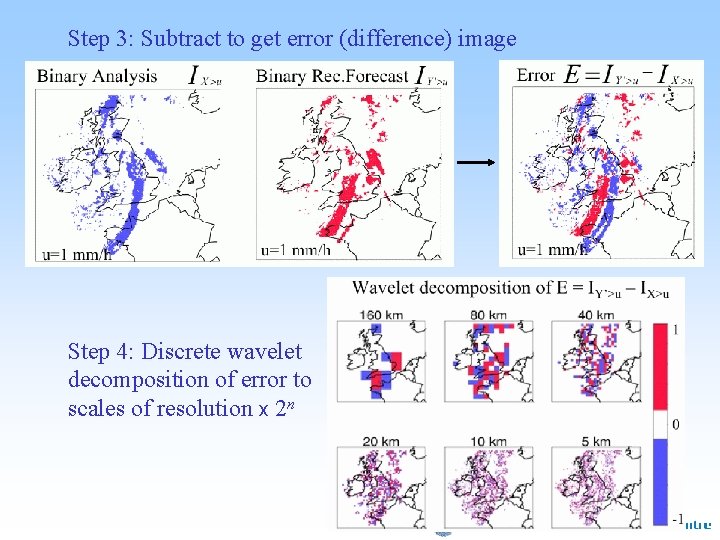
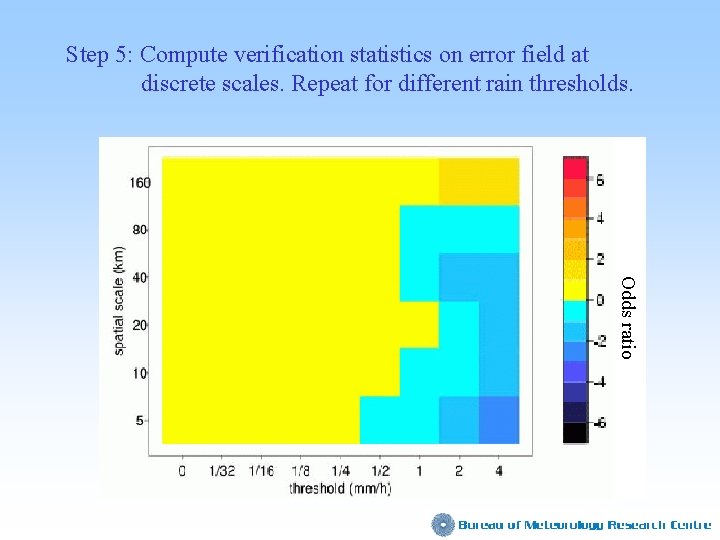
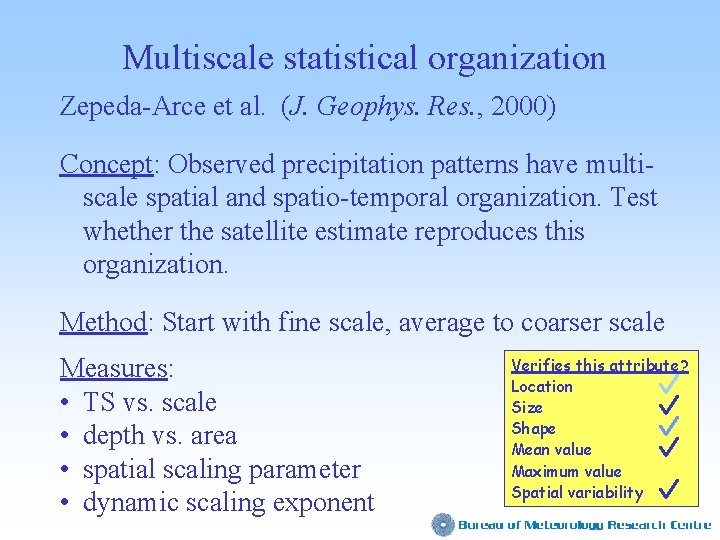
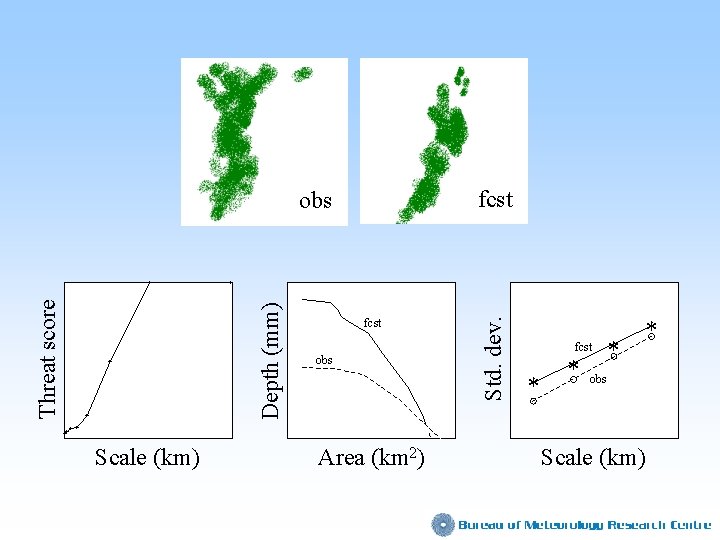
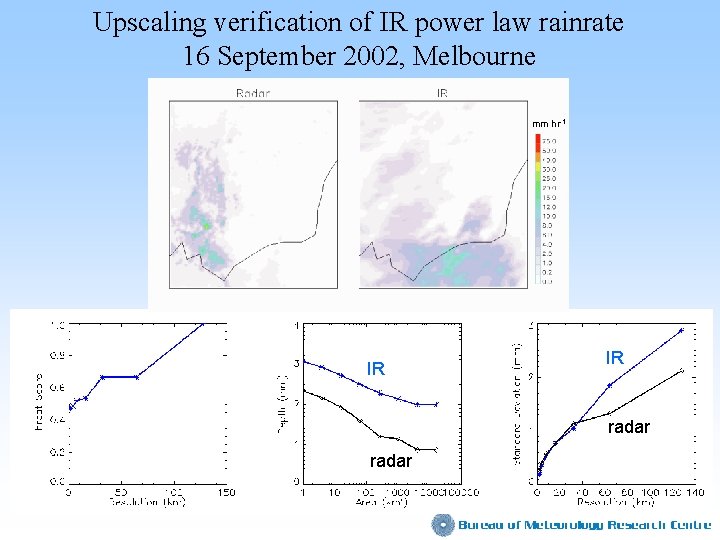
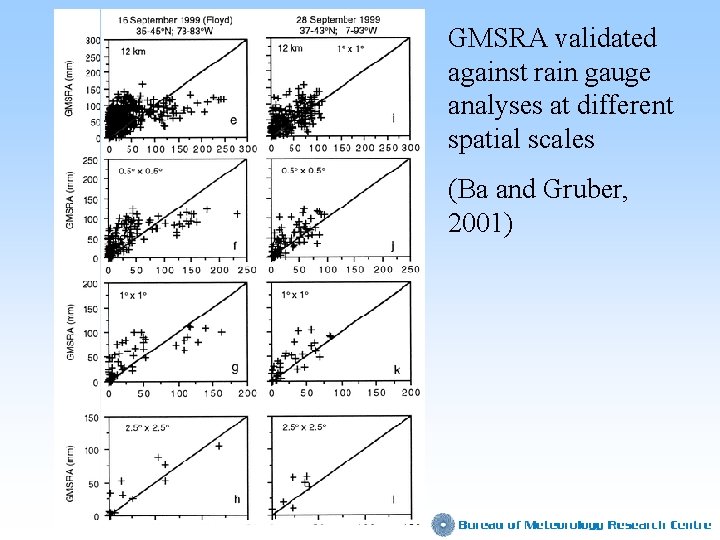
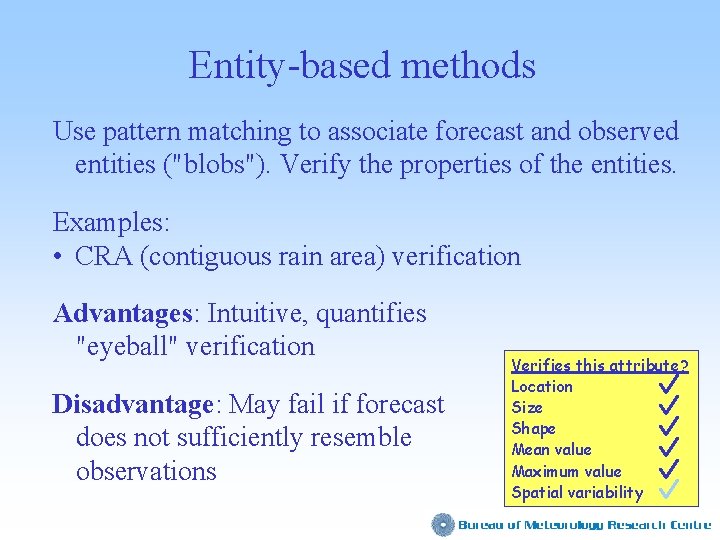
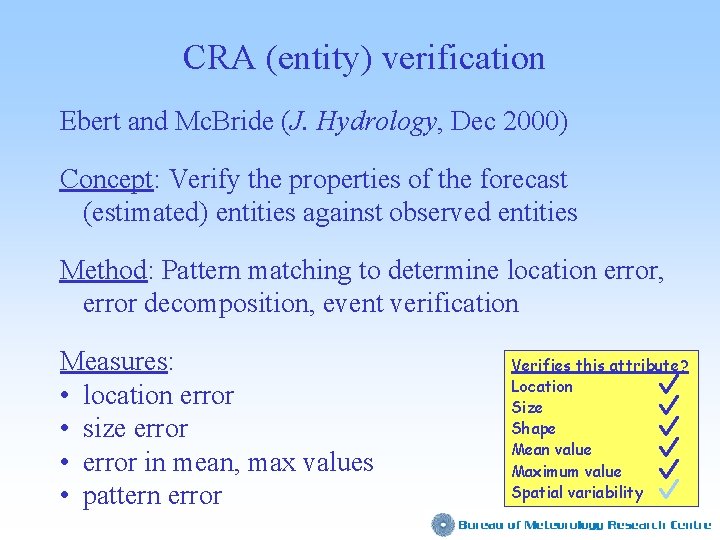
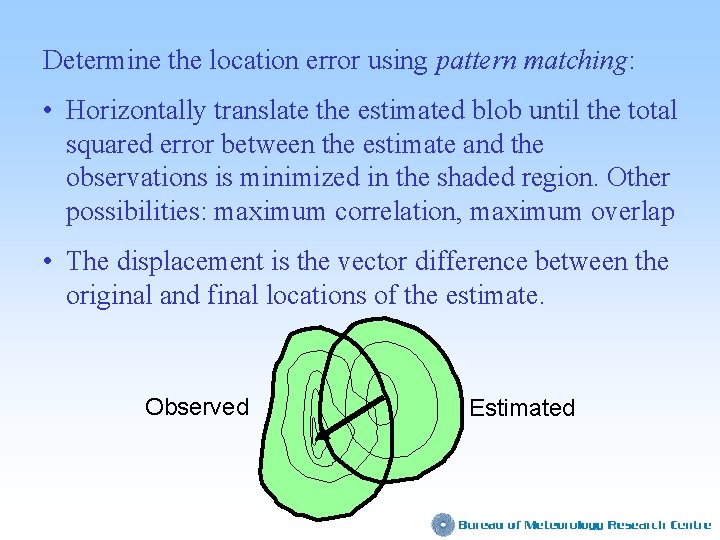
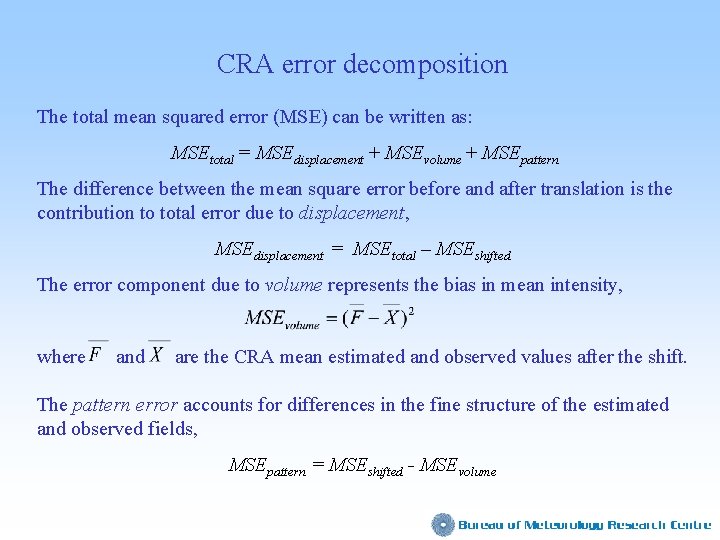
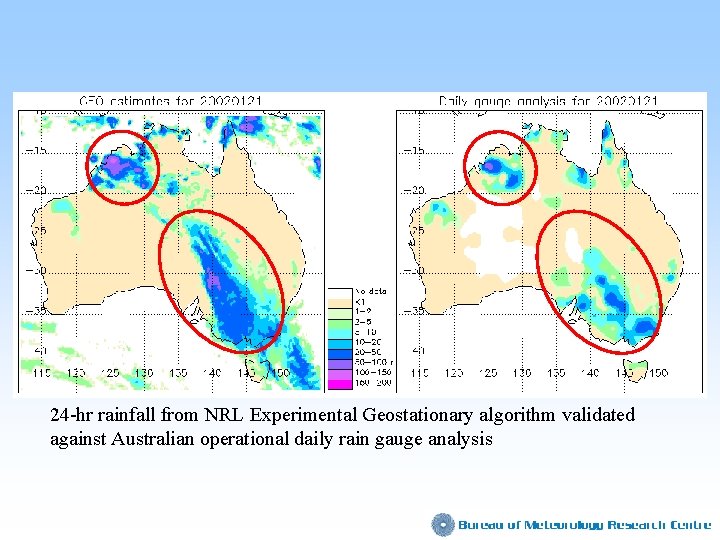
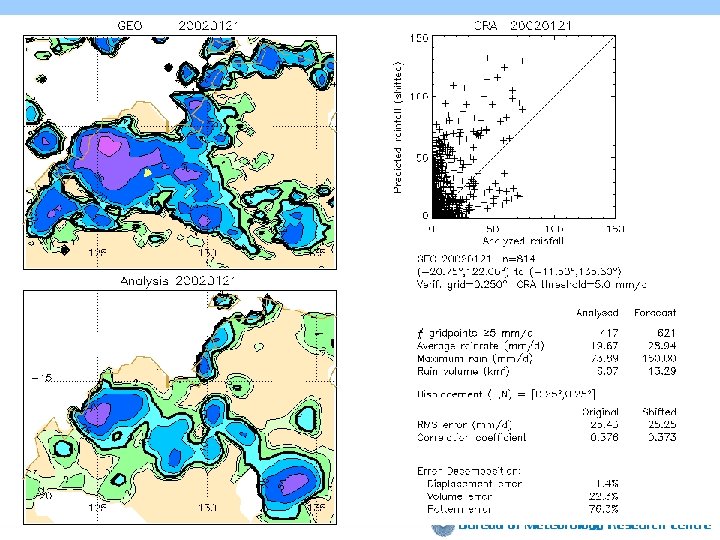
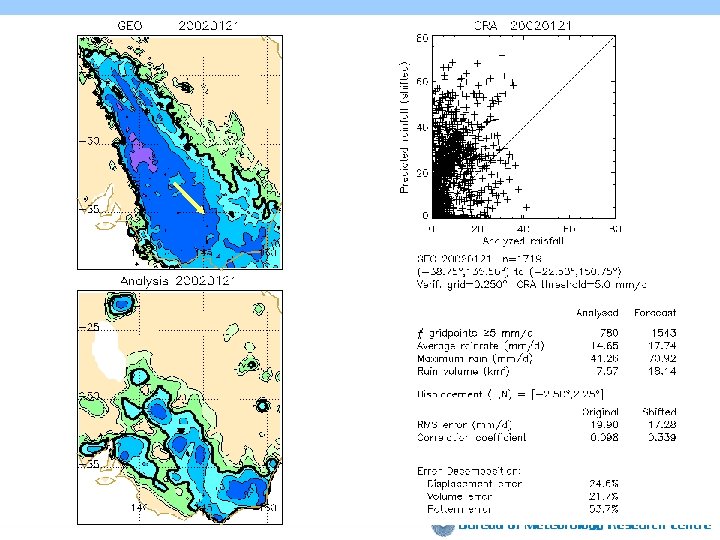
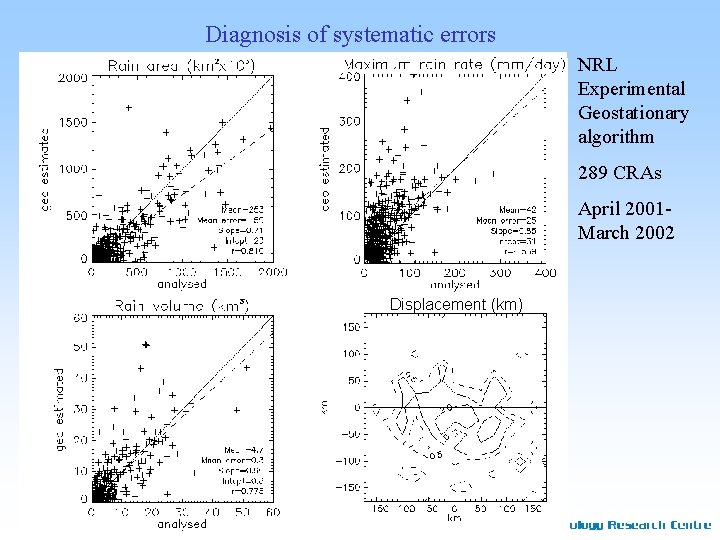
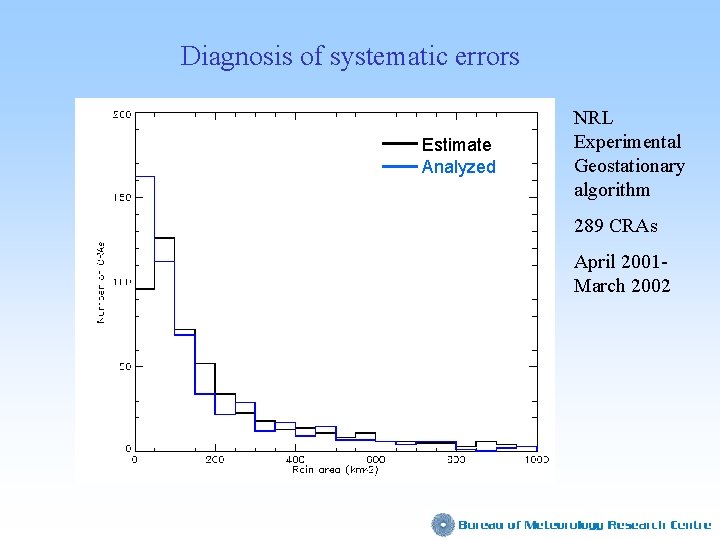
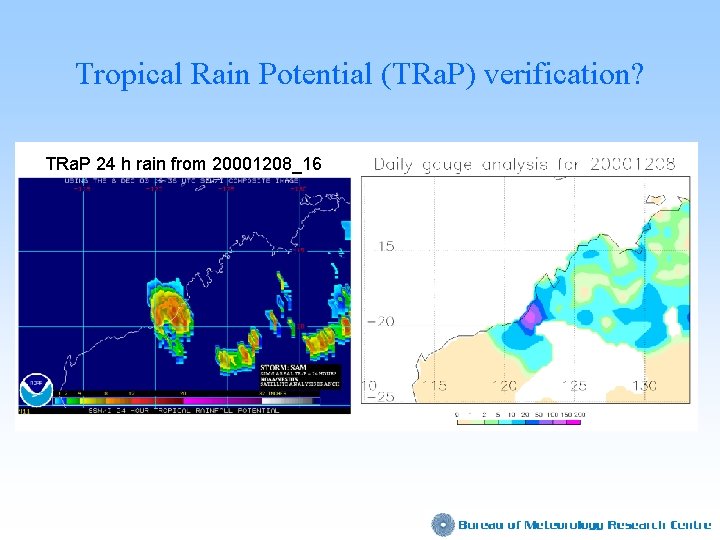
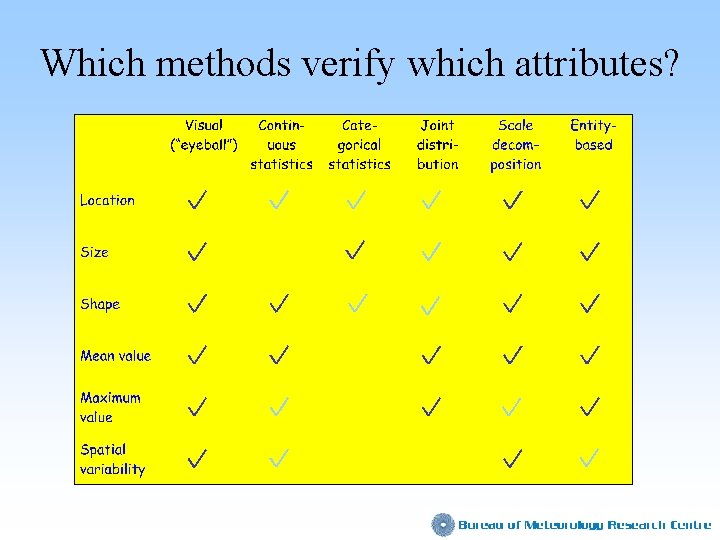
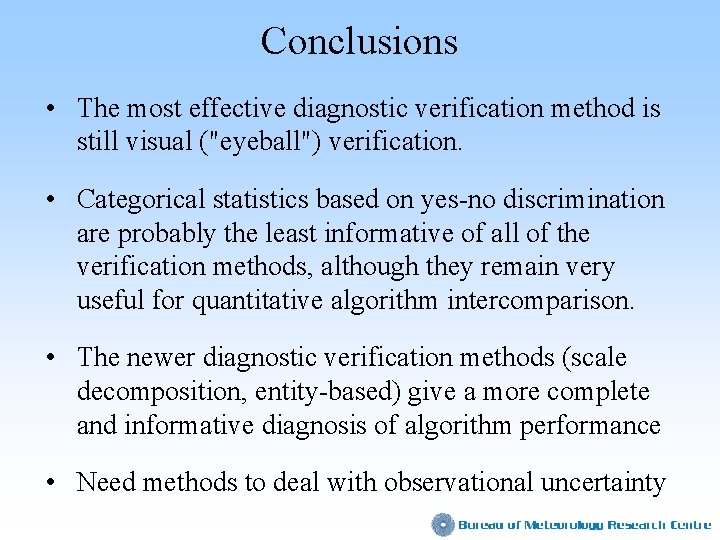
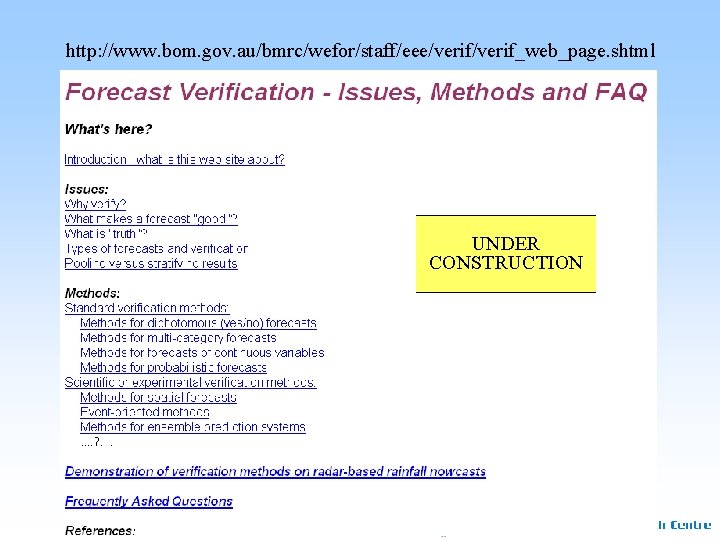
- Slides: 57
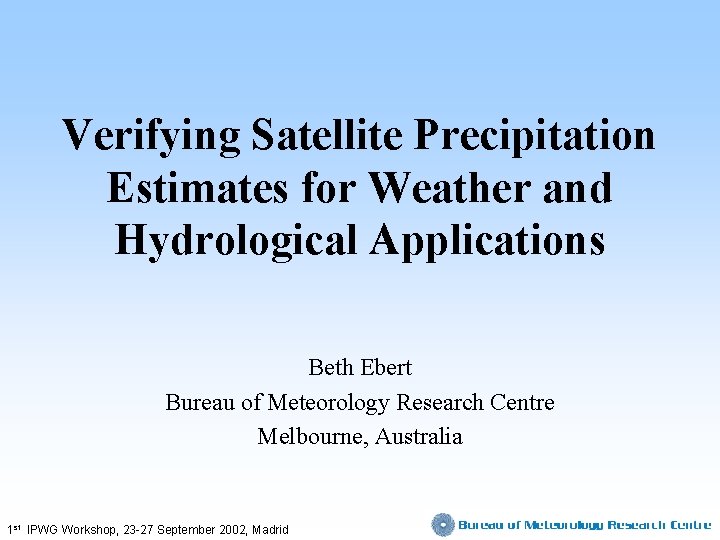
Verifying Satellite Precipitation Estimates for Weather and Hydrological Applications Beth Ebert Bureau of Meteorology Research Centre Melbourne, Australia 1 st IPWG Workshop, 23 -27 September 2002, Madrid
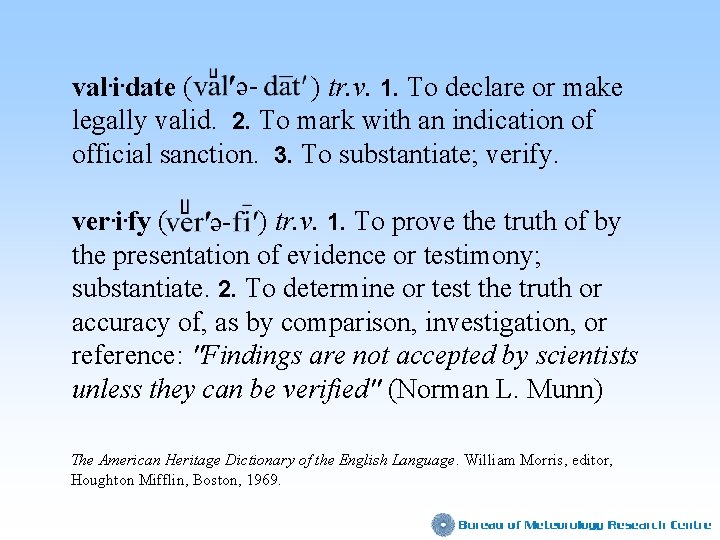
val. i. date ( ) tr. v. 1. To declare or make legally valid. 2. To mark with an indication of official sanction. 3. To substantiate; verify. -e ver. i. fy ( ) tr. v. 1. To prove the truth of by the presentation of evidence or testimony; substantiate. 2. To determine or test the truth or accuracy of, as by comparison, investigation, or reference: "Findings are not accepted by scientists unless they can be verified" (Norman L. Munn) -e The American Heritage Dictionary of the English Language. William Morris, editor, Houghton Mifflin, Boston, 1969.
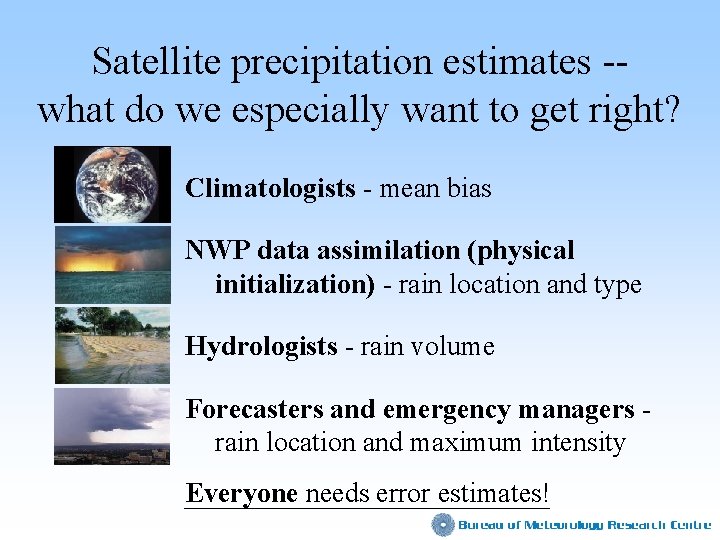
Satellite precipitation estimates -what do we especially want to get right? Climatologists - mean bias NWP data assimilation (physical initialization) - rain location and type Hydrologists - rain volume Forecasters and emergency managers rain location and maximum intensity Everyone needs error estimates!
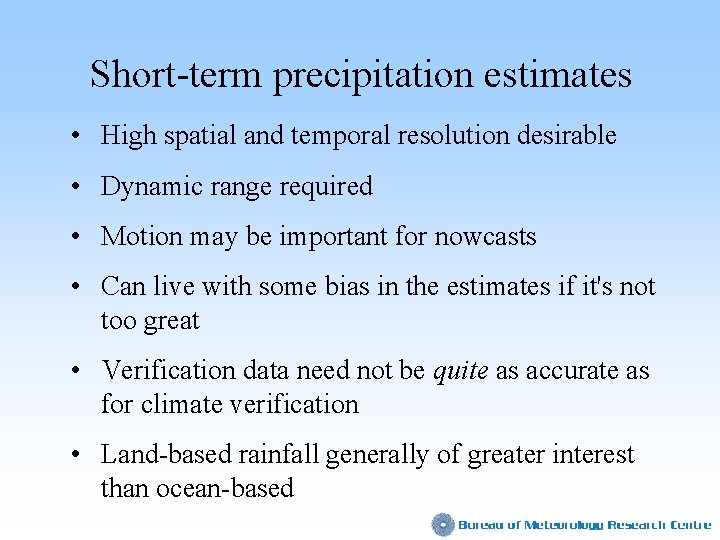
Short-term precipitation estimates • High spatial and temporal resolution desirable • Dynamic range required • Motion may be important for nowcasts • Can live with some bias in the estimates if it's not too great • Verification data need not be quite as accurate as for climate verification • Land-based rainfall generally of greater interest than ocean-based
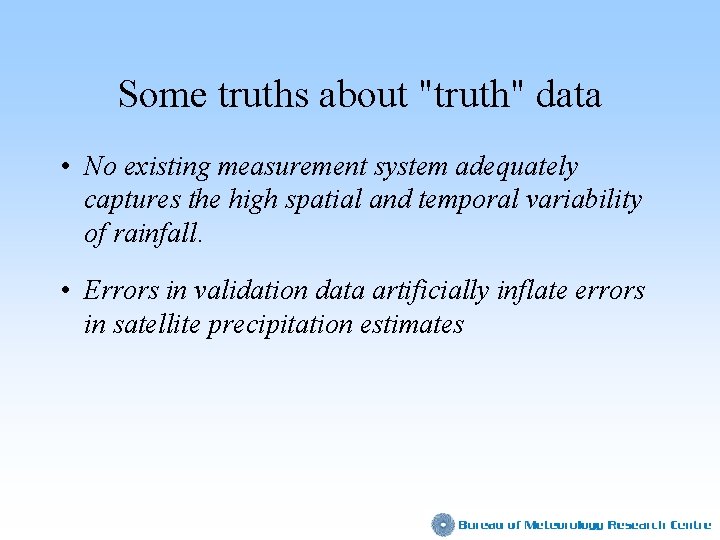
Some truths about "truth" data • No existing measurement system adequately captures the high spatial and temporal variability of rainfall. • Errors in validation data artificially inflate errors in satellite precipitation estimates
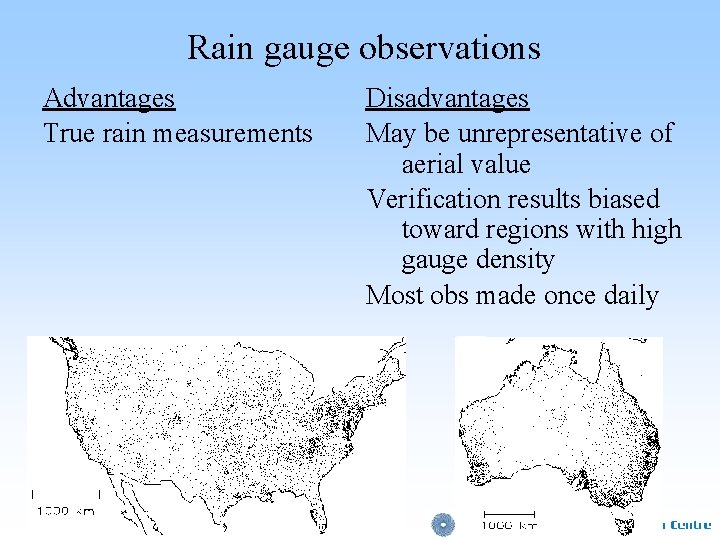
Rain gauge observations Advantages True rain measurements Disadvantages May be unrepresentative of aerial value Verification results biased toward regions with high gauge density Most obs made once daily
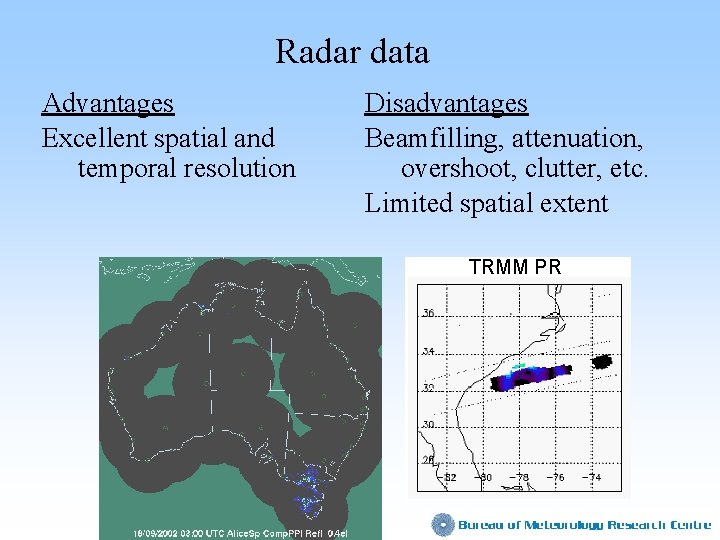
Radar data Advantages Excellent spatial and temporal resolution Disadvantages Beamfilling, attenuation, overshoot, clutter, etc. Limited spatial extent TRMM PR
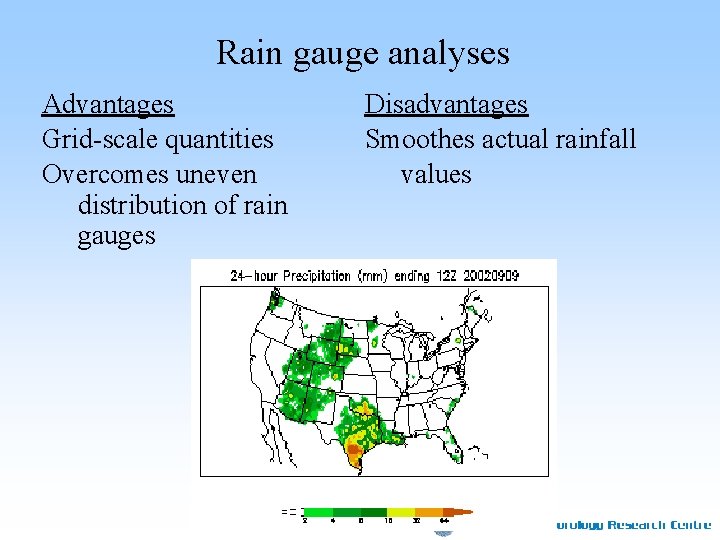
Rain gauge analyses Advantages Grid-scale quantities Overcomes uneven distribution of rain gauges Disadvantages Smoothes actual rainfall values
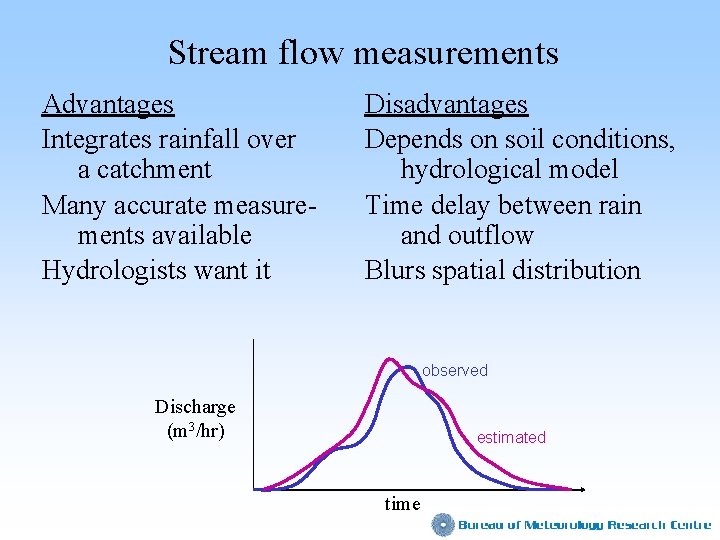
Stream flow measurements Advantages Integrates rainfall over a catchment Many accurate measurements available Hydrologists want it Disadvantages Depends on soil conditions, hydrological model Time delay between rain and outflow Blurs spatial distribution observed Discharge (m 3/hr) estimated time
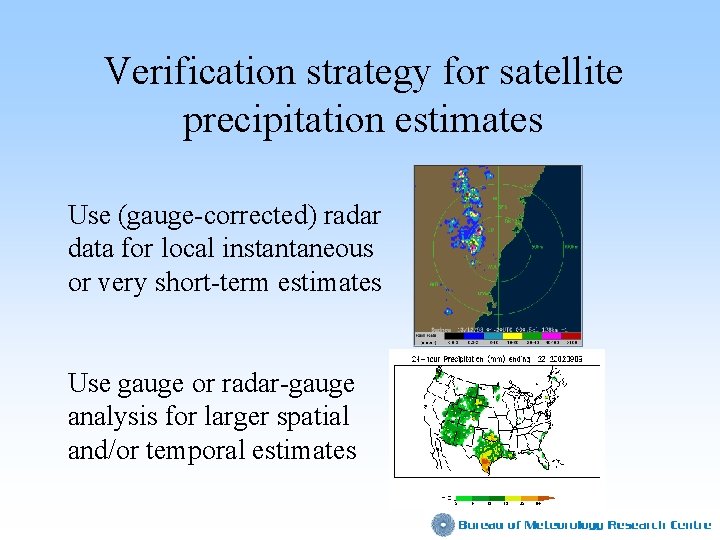
Verification strategy for satellite precipitation estimates Use (gauge-corrected) radar data for local instantaneous or very short-term estimates Use gauge or radar-gauge analysis for larger spatial and/or temporal estimates
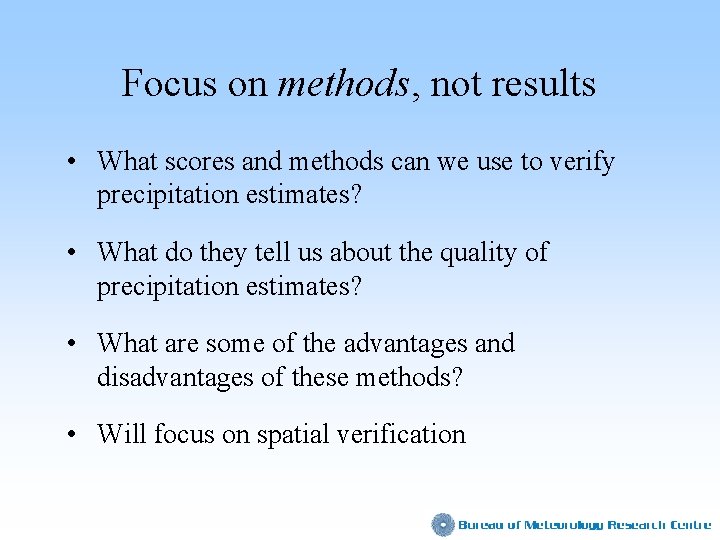
Focus on methods, not results • What scores and methods can we use to verify precipitation estimates? • What do they tell us about the quality of precipitation estimates? • What are some of the advantages and disadvantages of these methods? • Will focus on spatial verification
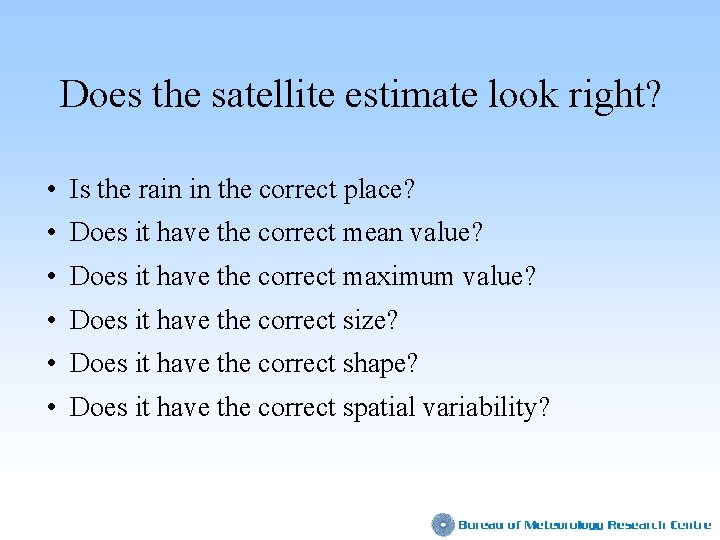
Does the satellite estimate look right? • Is the rain in the correct place? • Does it have the correct mean value? • Does it have the correct maximum value? • Does it have the correct size? • Does it have the correct shape? • Does it have the correct spatial variability?
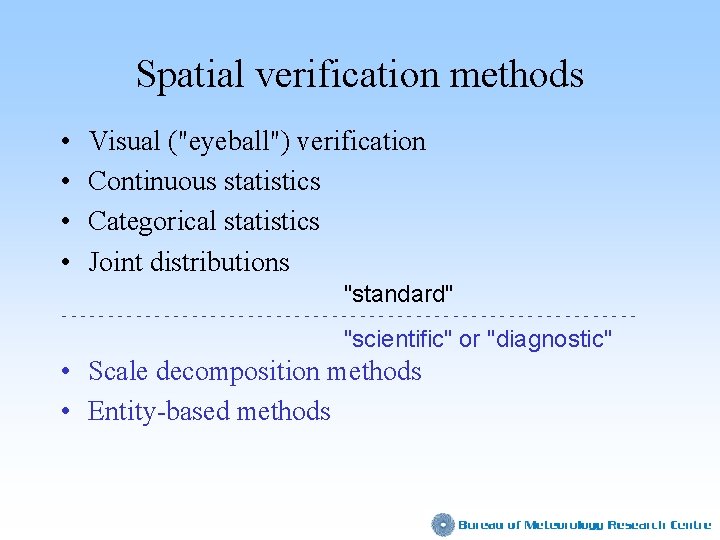
Spatial verification methods • • Visual ("eyeball") verification Continuous statistics Categorical statistics Joint distributions "standard" ------------------------------- "scientific" or "diagnostic" • Scale decomposition methods • Entity-based methods
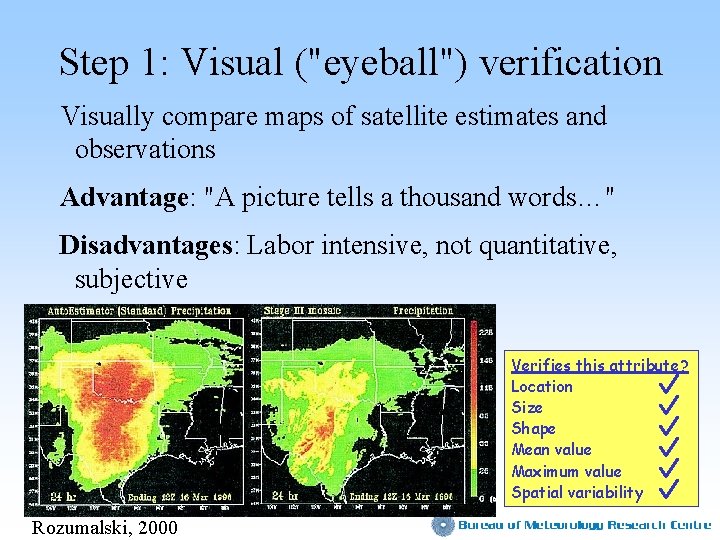
Step 1: Visual ("eyeball") verification Visually compare maps of satellite estimates and observations Advantage: "A picture tells a thousand words…" Disadvantages: Labor intensive, not quantitative, subjective Verifies this attribute? Location Size Shape Mean value Maximum value Spatial variability Rozumalski, 2000
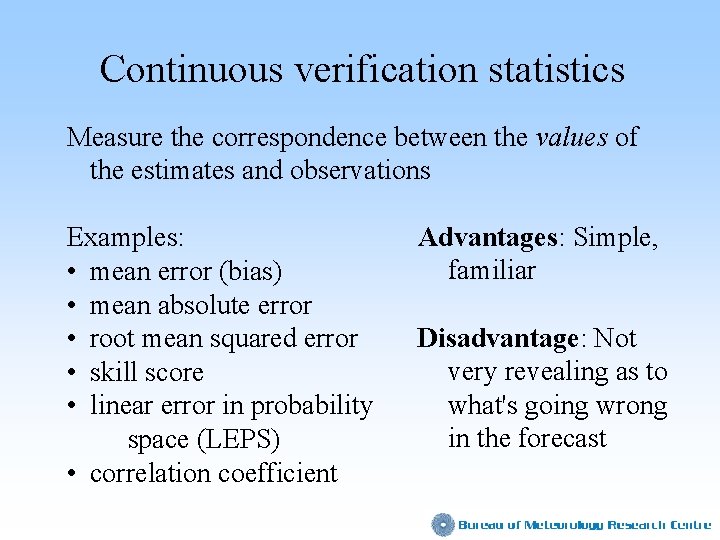
Continuous verification statistics Measure the correspondence between the values of the estimates and observations Examples: • mean error (bias) • mean absolute error • root mean squared error • skill score • linear error in probability space (LEPS) • correlation coefficient Advantages: Simple, familiar Disadvantage: Not very revealing as to what's going wrong in the forecast
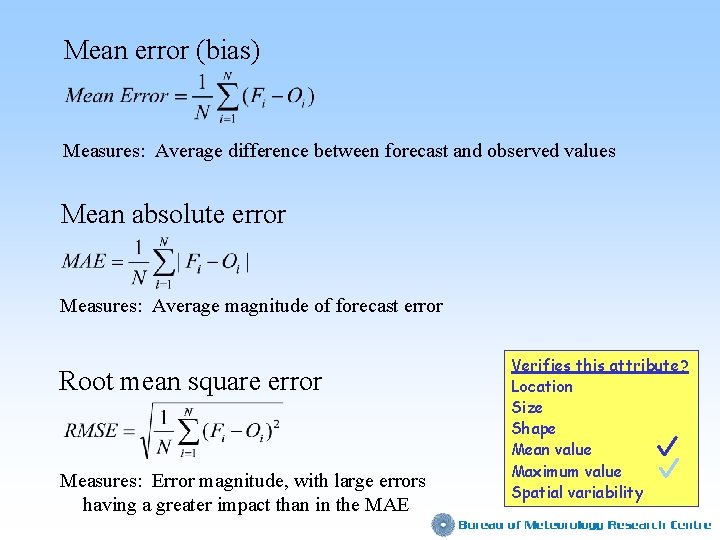
Mean error (bias) Measures: Average difference between forecast and observed values Mean absolute error Measures: Average magnitude of forecast error Root mean square error Measures: Error magnitude, with large errors having a greater impact than in the MAE Verifies this attribute? Location Size Shape Mean value Maximum value Spatial variability
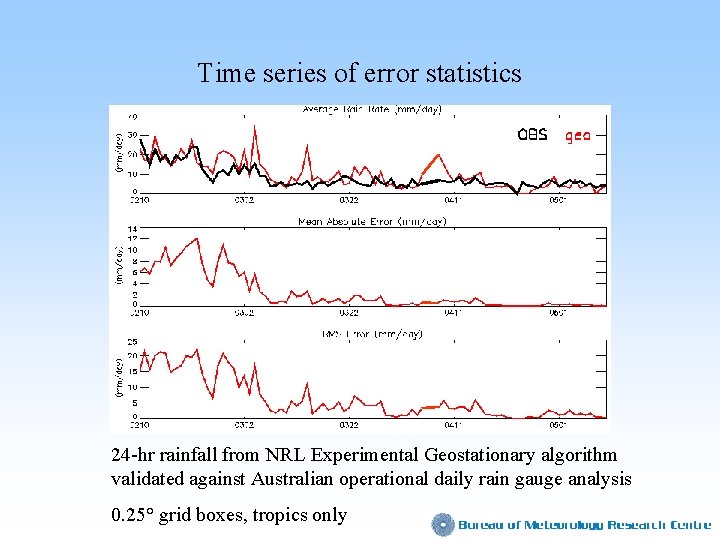
Time series of error statistics 24 -hr rainfall from NRL Experimental Geostationary algorithm validated against Australian operational daily rain gauge analysis 0. 25° grid boxes, tropics only
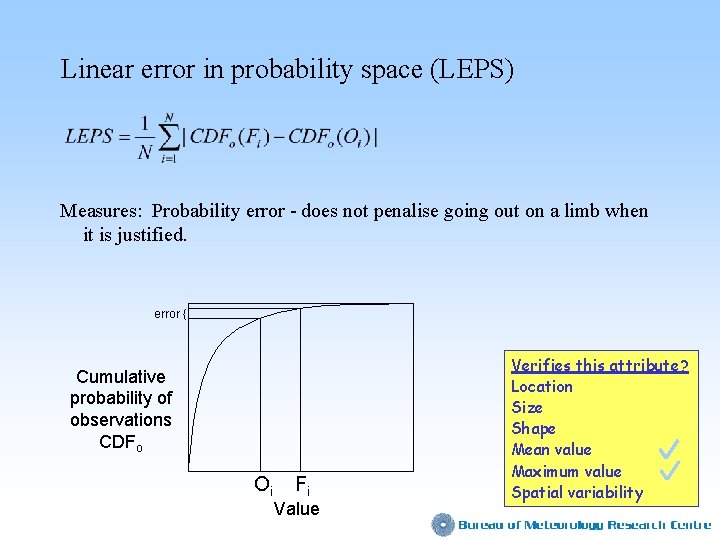
Linear error in probability space (LEPS) Measures: Probability error - does not penalise going out on a limb when it is justified. error { Cumulative probability of observations CDFo Oi Fi Value Verifies this attribute? Location Size Shape Mean value Maximum value Spatial variability
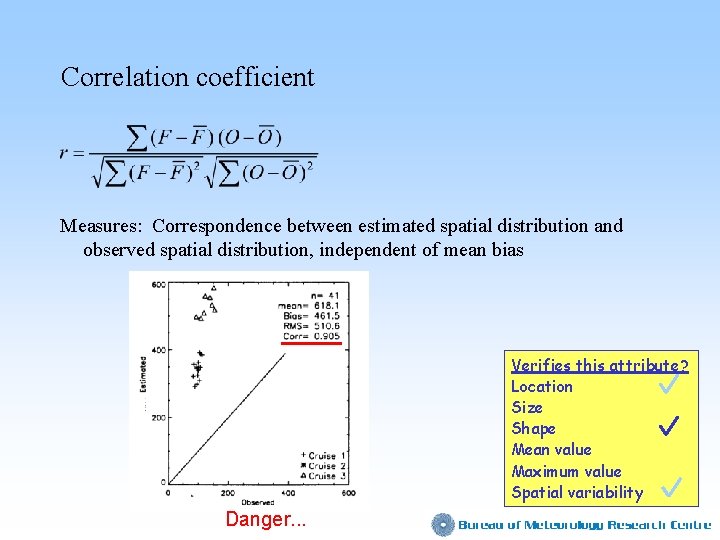
Correlation coefficient Measures: Correspondence between estimated spatial distribution and observed spatial distribution, independent of mean bias Verifies this attribute? Location Size Shape Mean value Maximum value Spatial variability Danger. . .
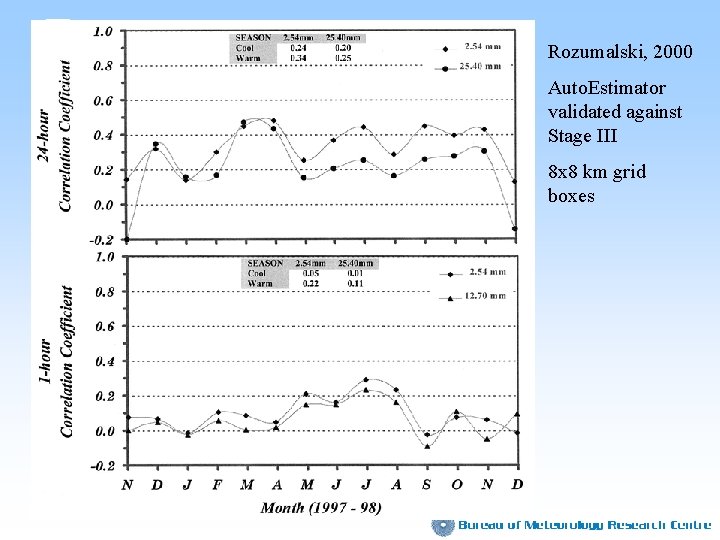
Rozumalski, 2000 Auto. Estimator validated against Stage III 8 x 8 km grid boxes
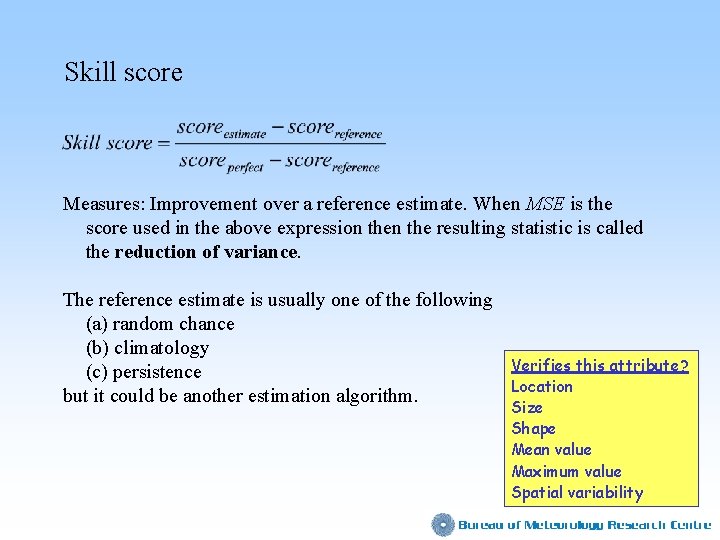
Skill score Measures: Improvement over a reference estimate. When MSE is the score used in the above expression the resulting statistic is called the reduction of variance. The reference estimate is usually one of the following (a) random chance (b) climatology (c) persistence but it could be another estimation algorithm. Verifies this attribute? Location Size Shape Mean value Maximum value Spatial variability
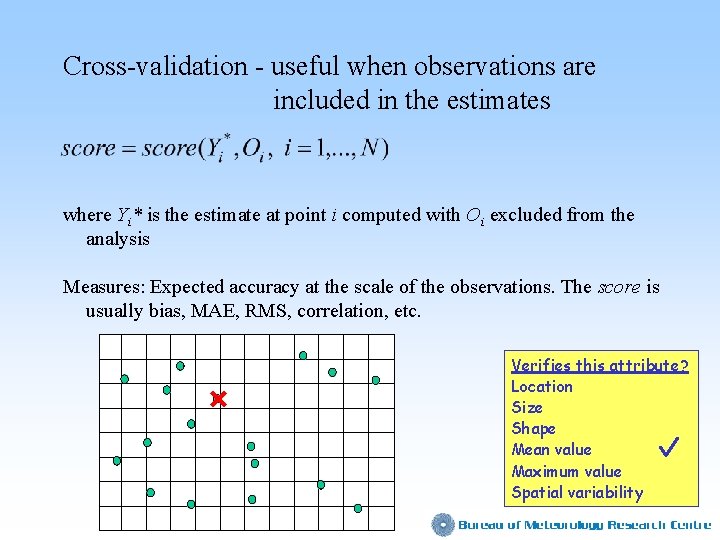
Cross-validation - useful when observations are included in the estimates where Yi* is the estimate at point i computed with Oi excluded from the analysis Measures: Expected accuracy at the scale of the observations. The score is usually bias, MAE, RMS, correlation, etc. Verifies this attribute? Location Size Shape Mean value Maximum value Spatial variability
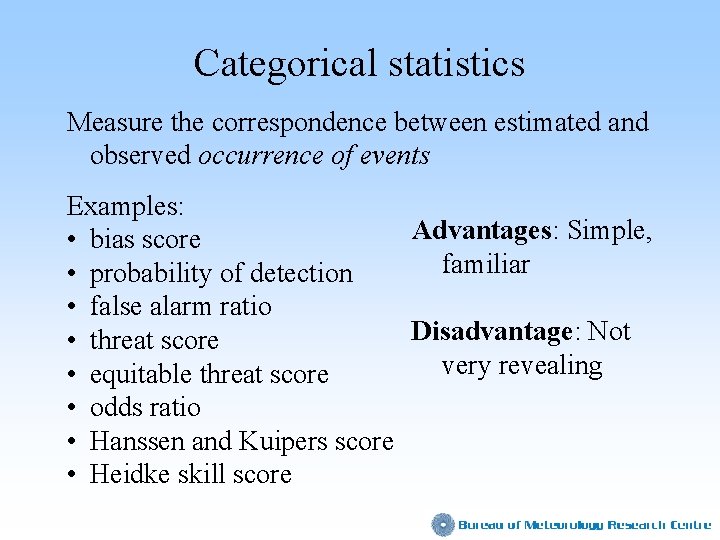
Categorical statistics Measure the correspondence between estimated and observed occurrence of events Examples: Advantages: Simple, • bias score familiar • probability of detection • false alarm ratio Disadvantage: Not • threat score very revealing • equitable threat score • odds ratio • Hanssen and Kuipers score • Heidke skill score
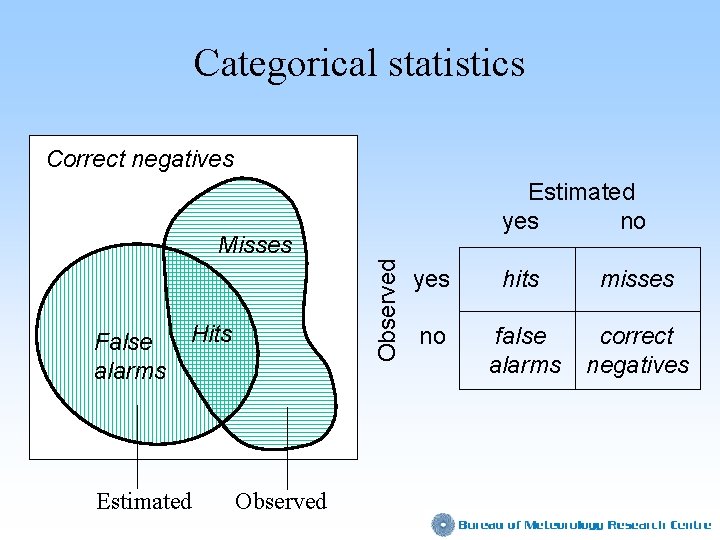
Categorical statistics Correct negatives Estimated yes no False alarms Observed Misses Hits Estimated Observed yes hits misses no false alarms correct negatives
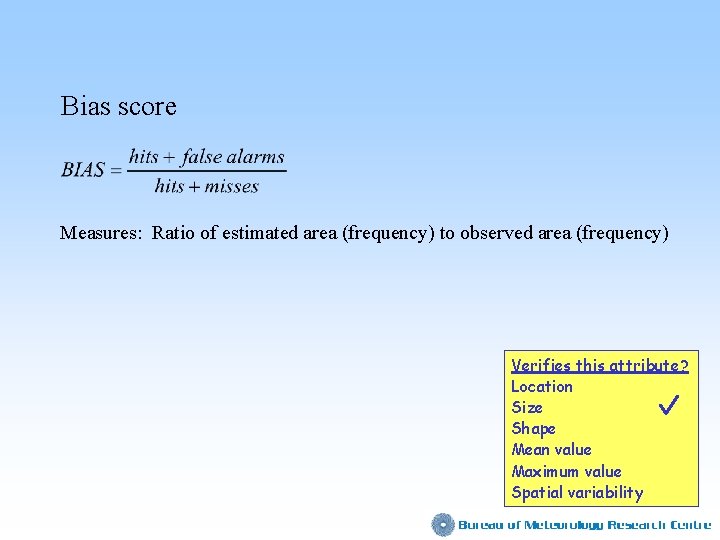
Bias score Measures: Ratio of estimated area (frequency) to observed area (frequency) Verifies this attribute? Location Size Shape Mean value Maximum value Spatial variability
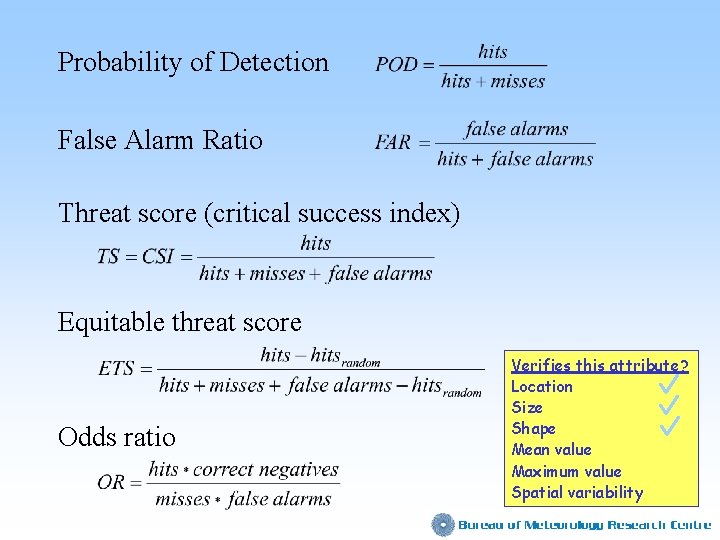
Probability of Detection False Alarm Ratio Threat score (critical success index) Equitable threat score Odds ratio Verifies this attribute? Location Size Shape Mean value Maximum value Spatial variability
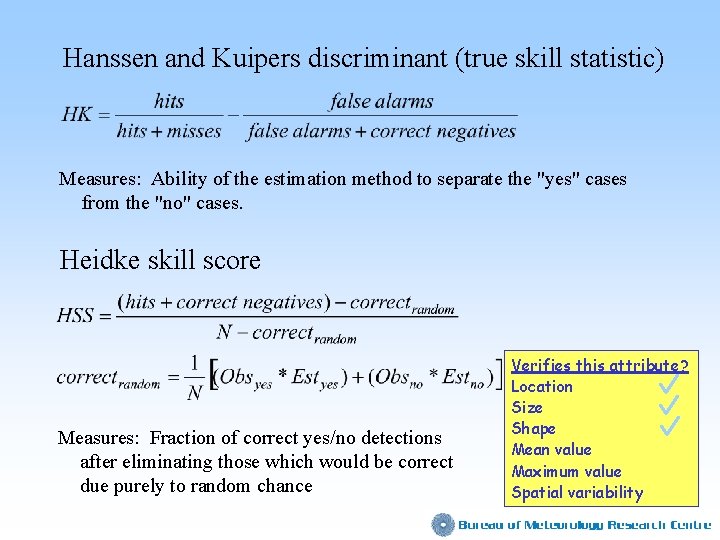
Hanssen and Kuipers discriminant (true skill statistic) Measures: Ability of the estimation method to separate the "yes" cases from the "no" cases. Heidke skill score Measures: Fraction of correct yes/no detections after eliminating those which would be correct due purely to random chance Verifies this attribute? Location Size Shape Mean value Maximum value Spatial variability
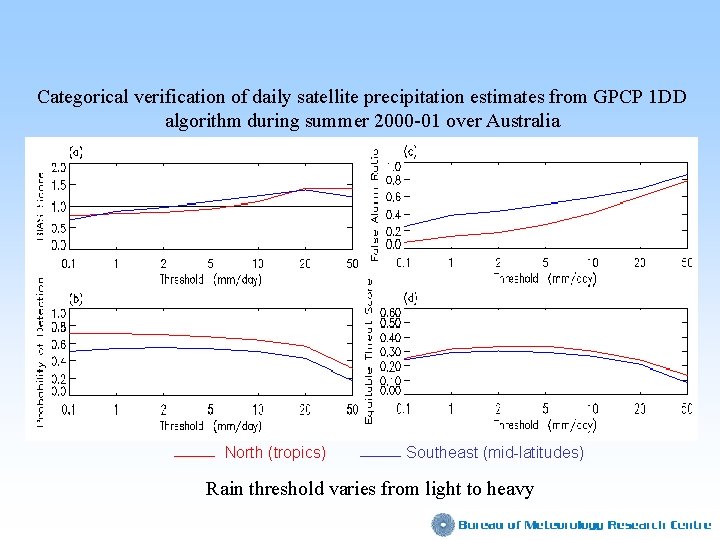
Categorical verification of daily satellite precipitation estimates from GPCP 1 DD algorithm during summer 2000 -01 over Australia North (tropics) Southeast (mid-latitudes) Rain threshold varies from light to heavy
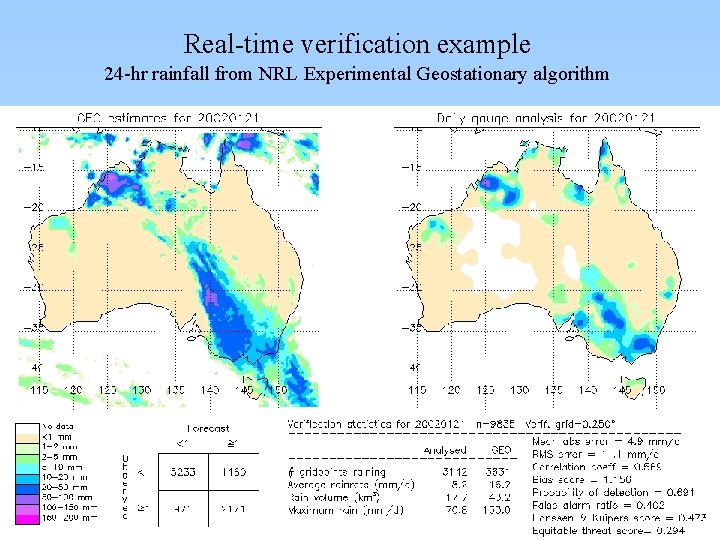
Real-time verification example 24 -hr rainfall from NRL Experimental Geostationary algorithm
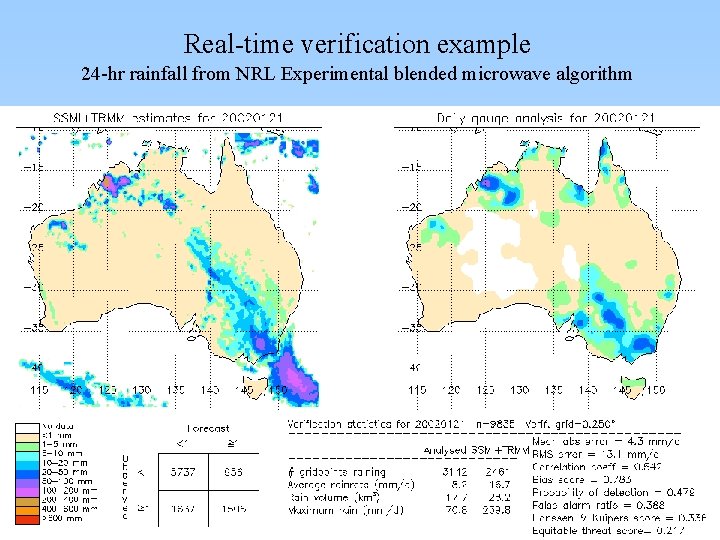
Real-time verification example 24 -hr rainfall from NRL Experimental blended microwave algorithm
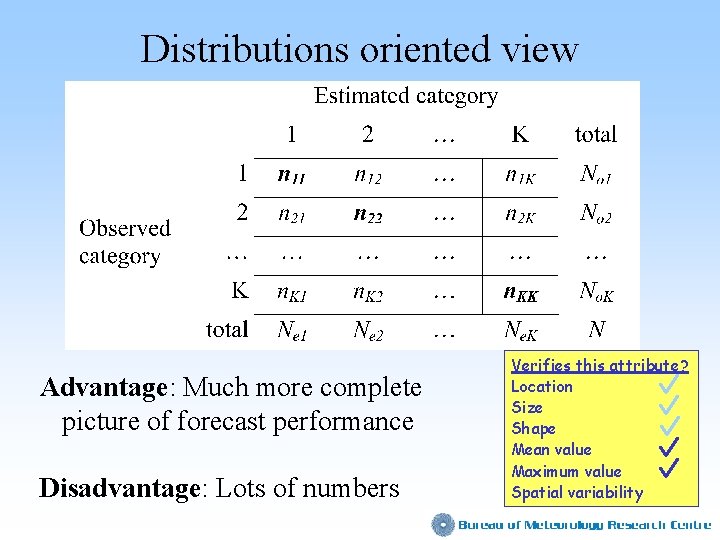
Distributions oriented view Advantage: Much more complete picture of forecast performance Disadvantage: Lots of numbers Verifies this attribute? Location Size Shape Mean value Maximum value Spatial variability
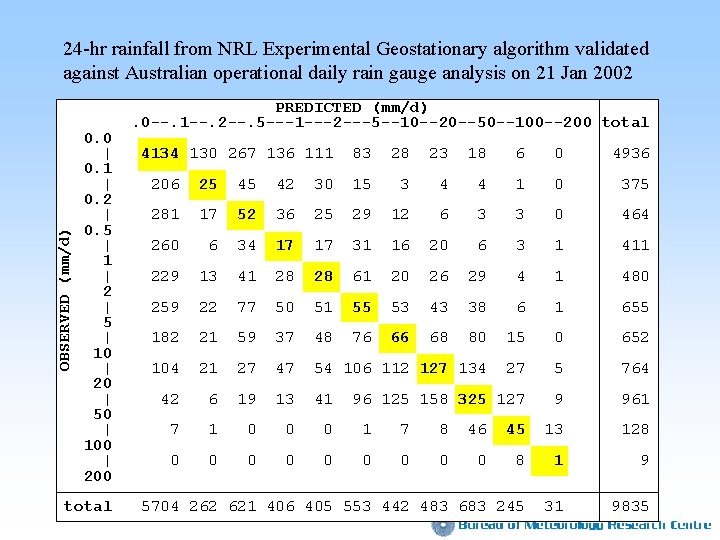
OBSERVED (mm/d) 24 -hr rainfall from NRL Experimental Geostationary algorithm validated against Australian operational daily rain gauge analysis on 21 Jan 2002 0. 0 | 0. 1 | 0. 2 | 0. 5 | 1 | 2 | 5 | 10 | 20 | 50 | 100 | 200 total PREDICTED (mm/d). 0 --. 1 --. 2 --. 5 ---1 ---2 ---5 --10 --20 --50 --100 --200 total 4134 130 267 136 111 83 28 23 18 6 0 4936 206 25 45 42 30 15 3 4 4 1 0 375 281 17 52 36 25 29 12 6 3 3 0 464 260 6 34 17 17 31 16 20 6 3 1 411 229 13 41 28 28 61 20 26 29 4 1 480 259 22 77 50 51 55 53 43 38 6 1 655 182 21 59 37 48 76 66 68 80 15 0 652 104 21 27 47 54 106 112 127 134 27 5 764 42 6 19 13 41 96 125 158 325 127 9 961 7 1 0 0 0 1 7 8 46 45 13 128 0 0 0 0 0 8 1 9 5704 262 621 406 405 553 442 483 683 245 31 9835
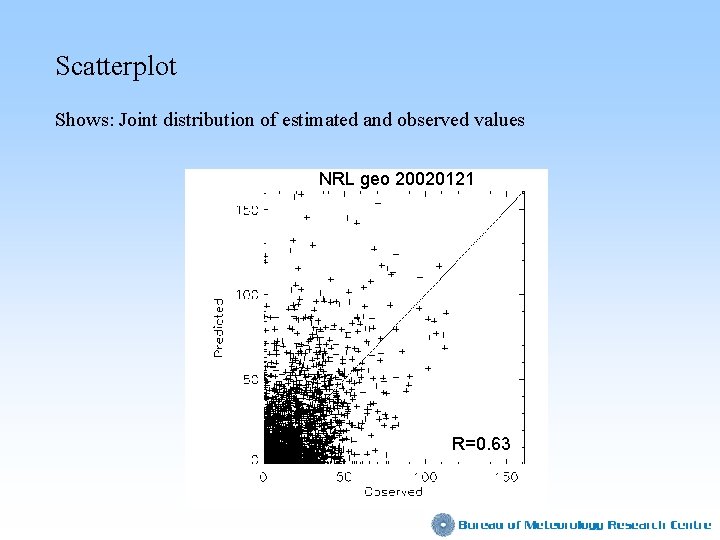
Scatterplot Shows: Joint distribution of estimated and observed values NRL geo 20020121 R=0. 63
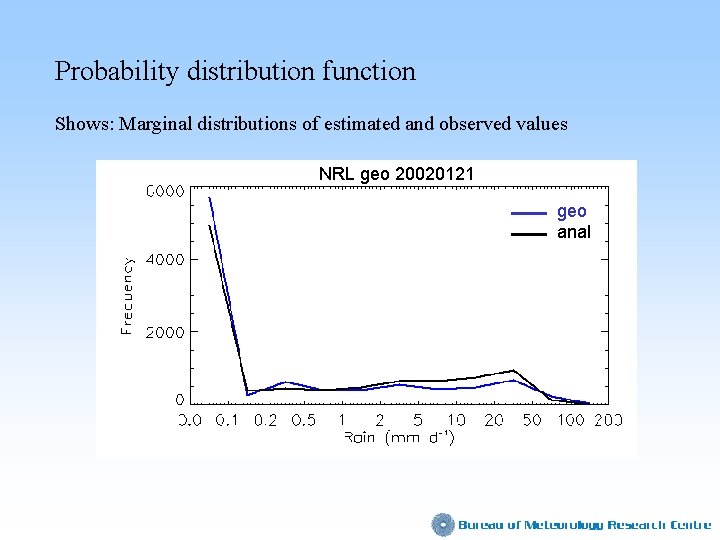
Probability distribution function Shows: Marginal distributions of estimated and observed values NRL geo 20020121 geo anal
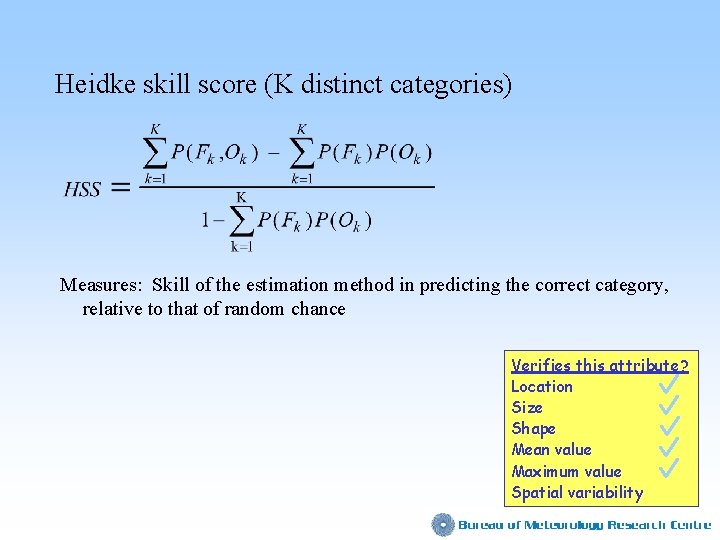
Heidke skill score (K distinct categories) Measures: Skill of the estimation method in predicting the correct category, relative to that of random chance Verifies this attribute? Location Size Shape Mean value Maximum value Spatial variability
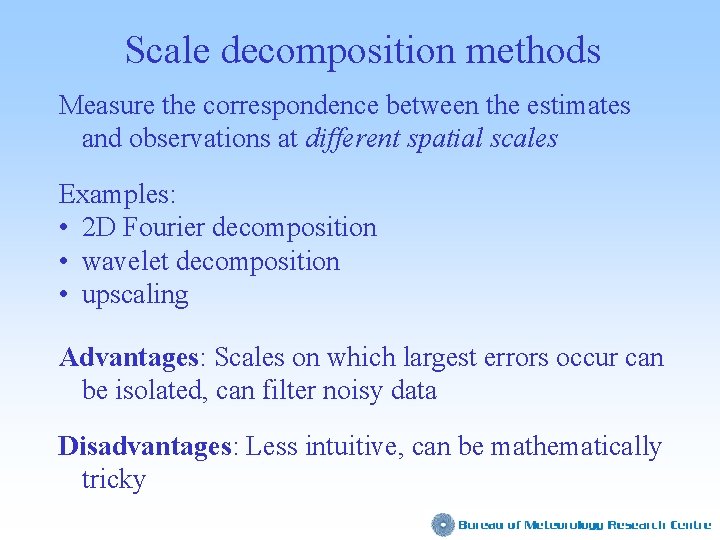
Scale decomposition methods Measure the correspondence between the estimates and observations at different spatial scales Examples: • 2 D Fourier decomposition • wavelet decomposition • upscaling Advantages: Scales on which largest errors occur can be isolated, can filter noisy data Disadvantages: Less intuitive, can be mathematically tricky
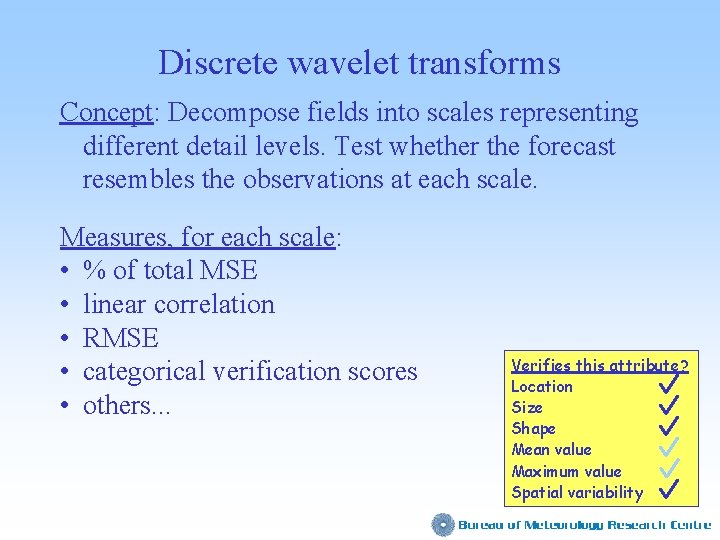
Discrete wavelet transforms Concept: Decompose fields into scales representing different detail levels. Test whether the forecast resembles the observations at each scale. Measures, for each scale: • % of total MSE • linear correlation • RMSE • categorical verification scores • others. . . Verifies this attribute? Location Size Shape Mean value Maximum value Spatial variability
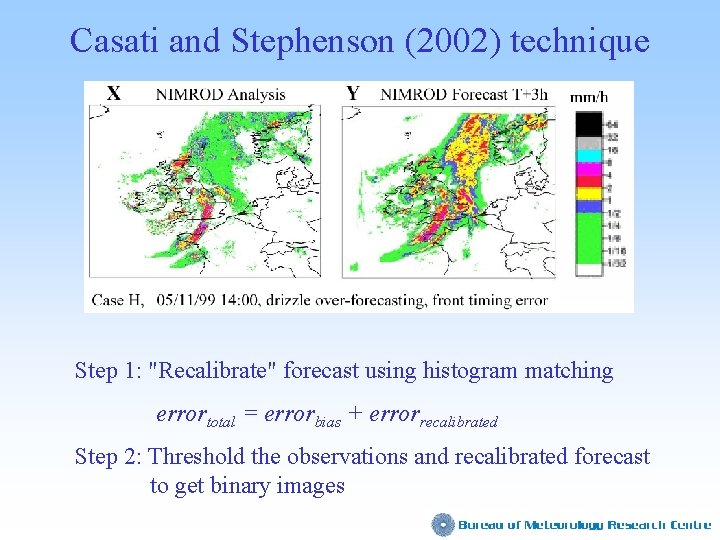
Casati and Stephenson (2002) technique Step 1: "Recalibrate" forecast using histogram matching errortotal = errorbias + errorrecalibrated Step 2: Threshold the observations and recalibrated forecast to get binary images
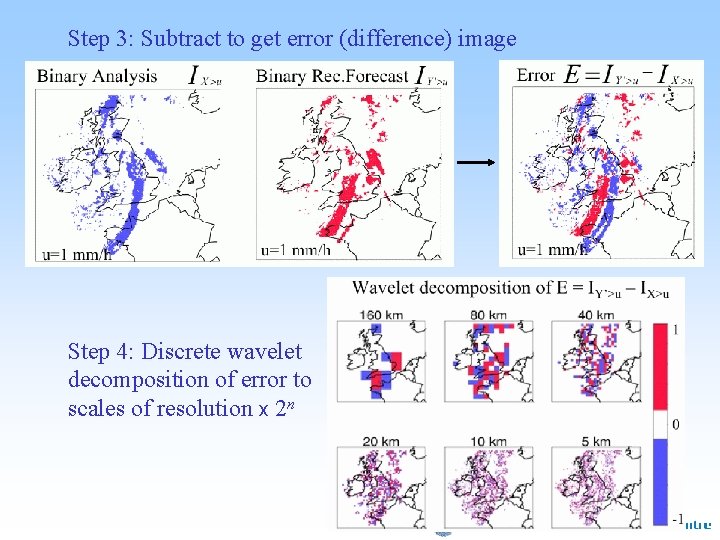
Step 3: Subtract to get error (difference) image Step 4: Discrete wavelet decomposition of error to scales of resolution x 2 n
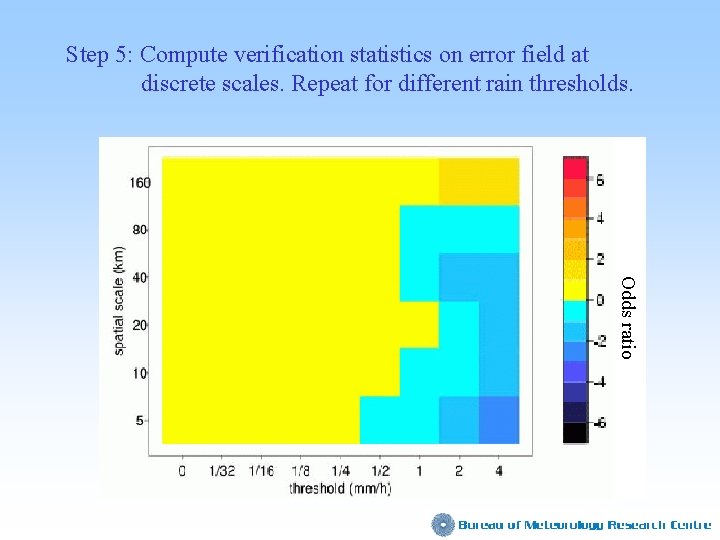
Step 5: Compute verification statistics on error field at discrete scales. Repeat for different rain thresholds. Odds ratio
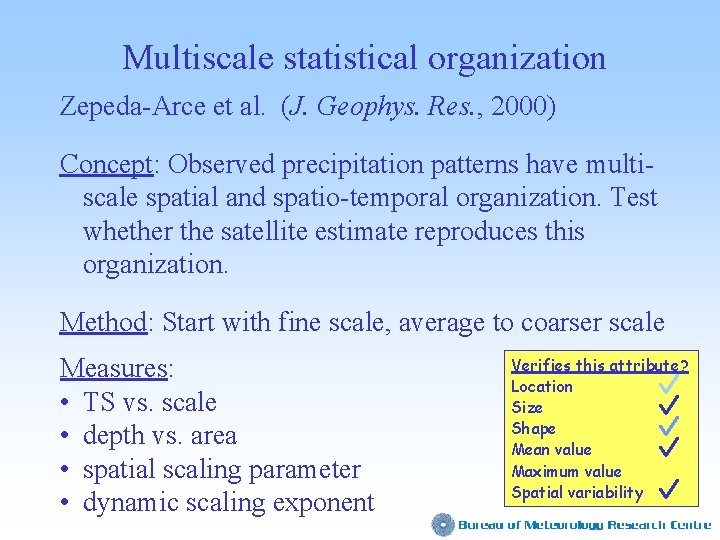
Multiscale statistical organization Zepeda-Arce et al. (J. Geophys. Res. , 2000) Concept: Observed precipitation patterns have multiscale spatial and spatio-temporal organization. Test whether the satellite estimate reproduces this organization. Method: Start with fine scale, average to coarser scale Measures: • TS vs. scale • depth vs. area • spatial scaling parameter • dynamic scaling exponent Verifies this attribute? Location Size Shape Mean value Maximum value Spatial variability
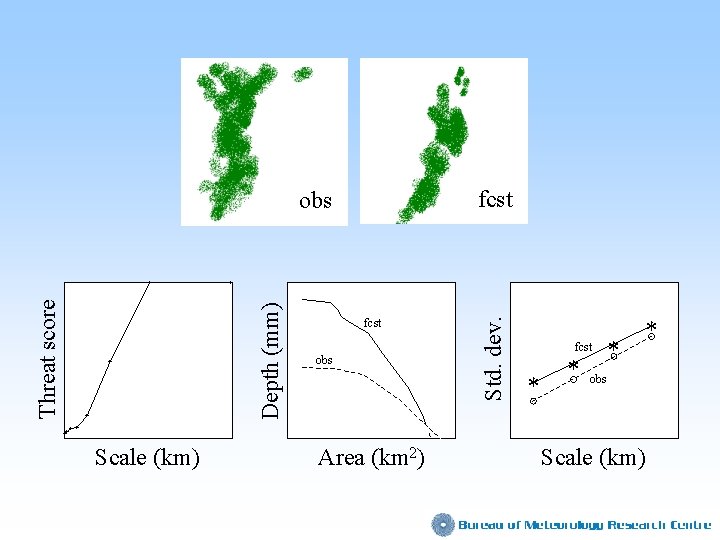
fcst obs + fcst obs Std. dev. + + Depth (mm) Threat score + fcst * * obs ++ ++ Scale (km) Area (km 2) Scale (km)
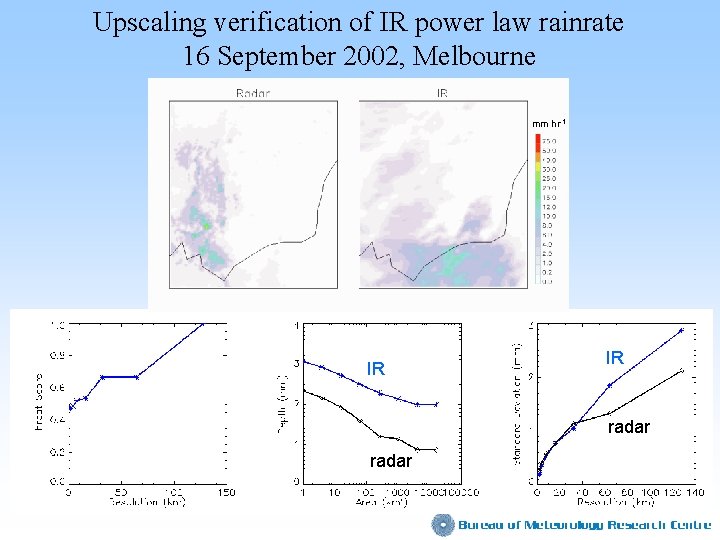
Upscaling verification of IR power law rainrate 16 September 2002, Melbourne mm hr-1 IR IR radar
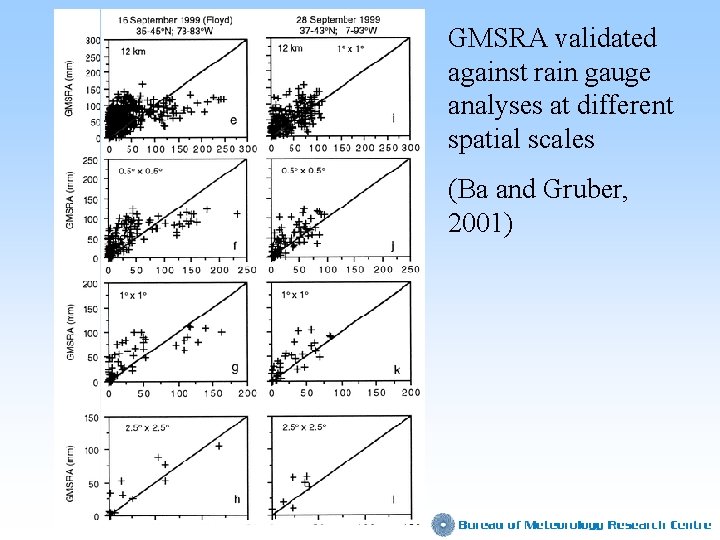
GMSRA validated against rain gauge analyses at different spatial scales (Ba and Gruber, 2001)
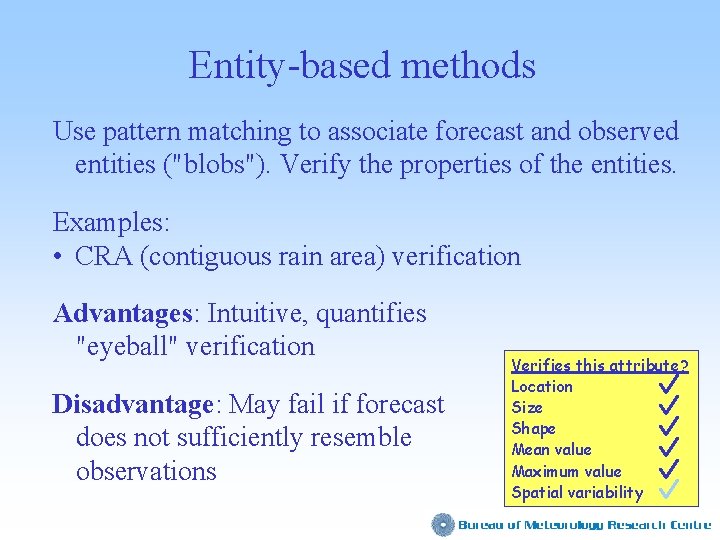
Entity-based methods Use pattern matching to associate forecast and observed entities ("blobs"). Verify the properties of the entities. Examples: • CRA (contiguous rain area) verification Advantages: Intuitive, quantifies "eyeball" verification Disadvantage: May fail if forecast does not sufficiently resemble observations Verifies this attribute? Location Size Shape Mean value Maximum value Spatial variability
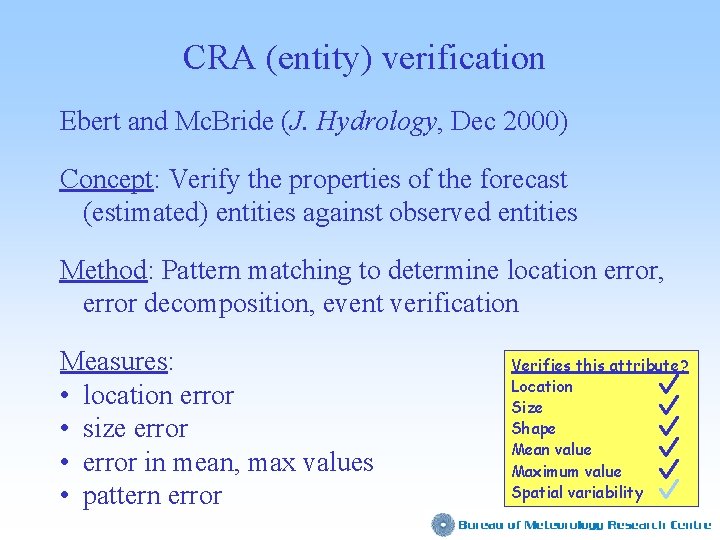
CRA (entity) verification Ebert and Mc. Bride (J. Hydrology, Dec 2000) Concept: Verify the properties of the forecast (estimated) entities against observed entities Method: Pattern matching to determine location error, error decomposition, event verification Measures: • location error • size error • error in mean, max values • pattern error Verifies this attribute? Location Size Shape Mean value Maximum value Spatial variability
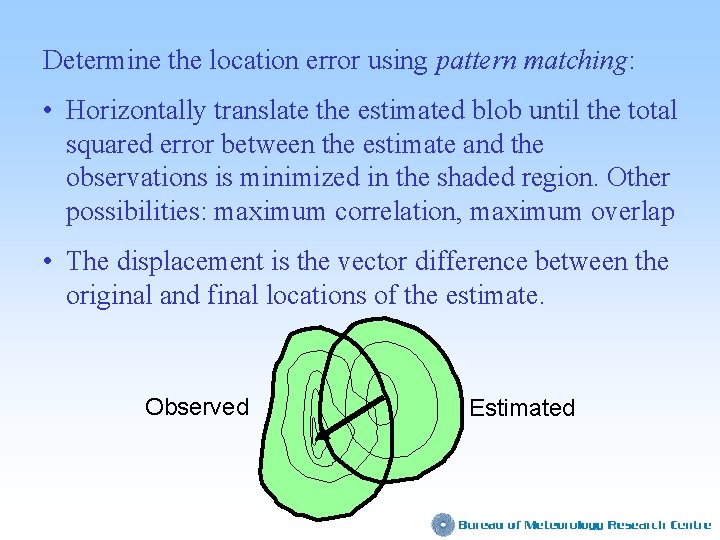
Determine the location error using pattern matching: • Horizontally translate the estimated blob until the total squared error between the estimate and the observations is minimized in the shaded region. Other possibilities: maximum correlation, maximum overlap • The displacement is the vector difference between the original and final locations of the estimate. Observed Estimated
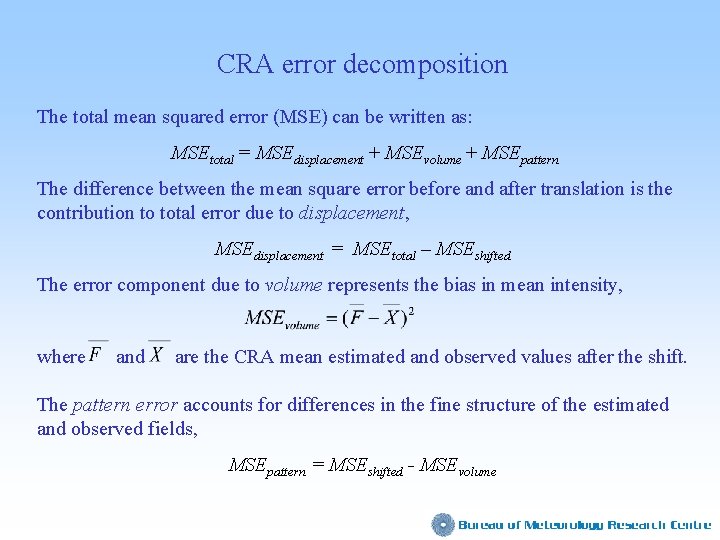
CRA error decomposition The total mean squared error (MSE) can be written as: MSEtotal = MSEdisplacement + MSEvolume + MSEpattern The difference between the mean square error before and after translation is the contribution to total error due to displacement, MSEdisplacement = MSEtotal – MSEshifted The error component due to volume represents the bias in mean intensity, where and are the CRA mean estimated and observed values after the shift. The pattern error accounts for differences in the fine structure of the estimated and observed fields, MSEpattern = MSEshifted - MSEvolume
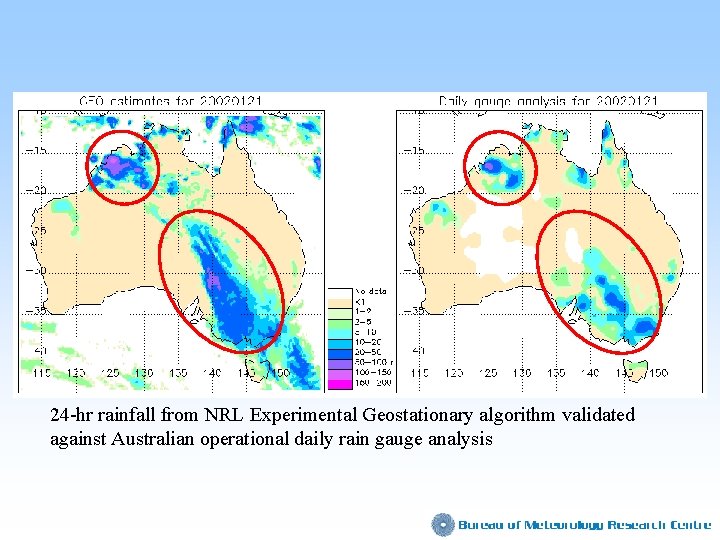
24 -hr rainfall from NRL Experimental Geostationary algorithm validated against Australian operational daily rain gauge analysis
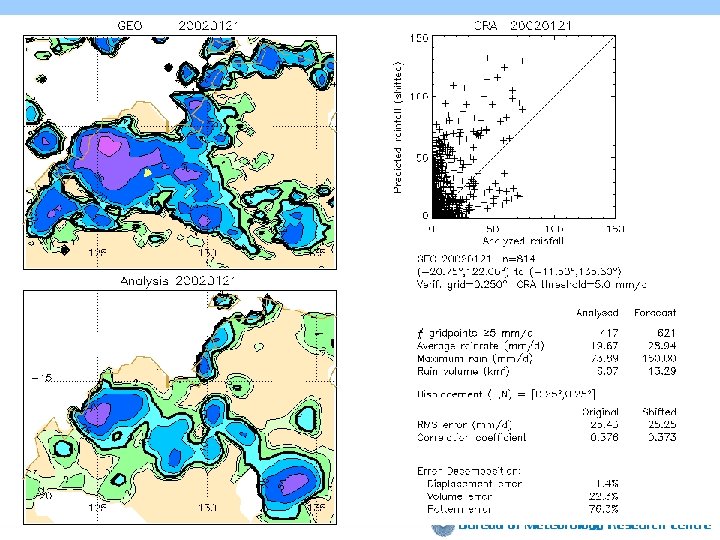
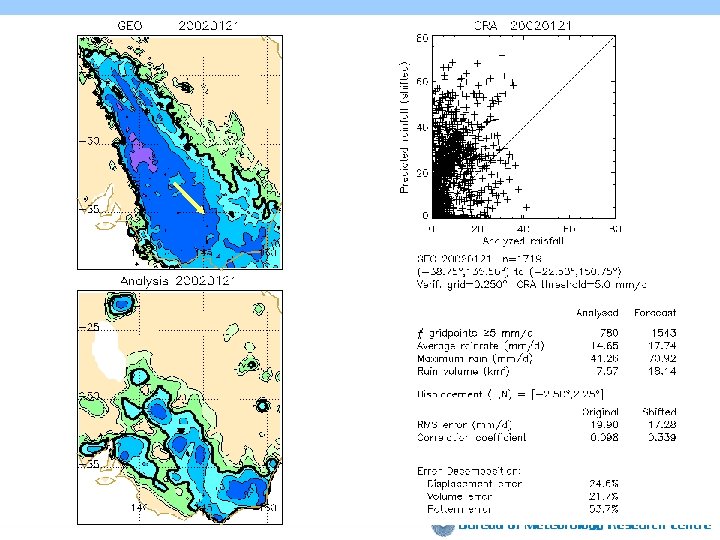
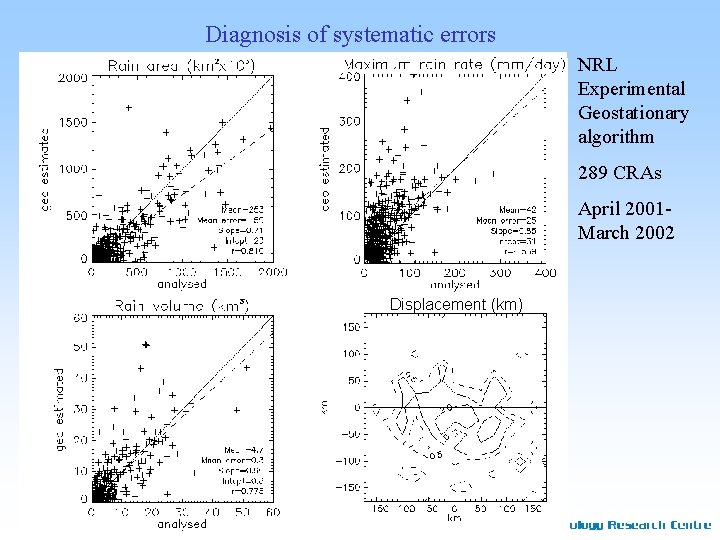
Diagnosis of systematic errors NRL Experimental Geostationary algorithm 289 CRAs April 2001 March 2002 Displacement (km)
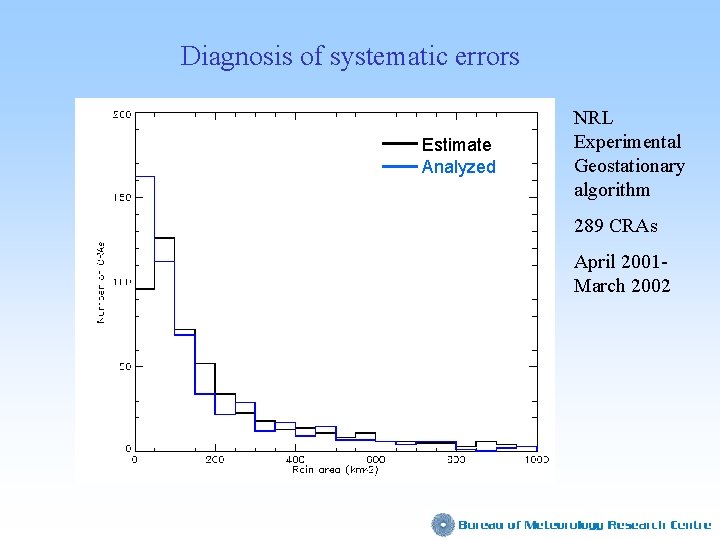
Diagnosis of systematic errors Estimate Analyzed NRL Experimental Geostationary algorithm 289 CRAs April 2001 March 2002
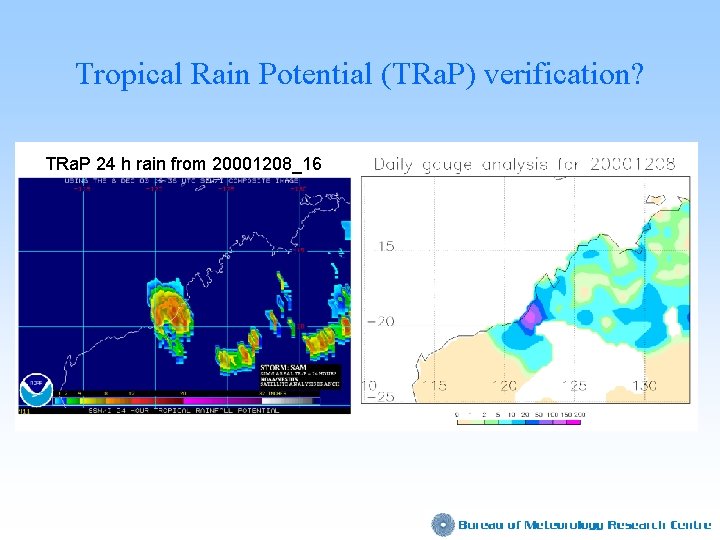
Tropical Rain Potential (TRa. P) verification? TRa. P 24 h rain from 20001208_16
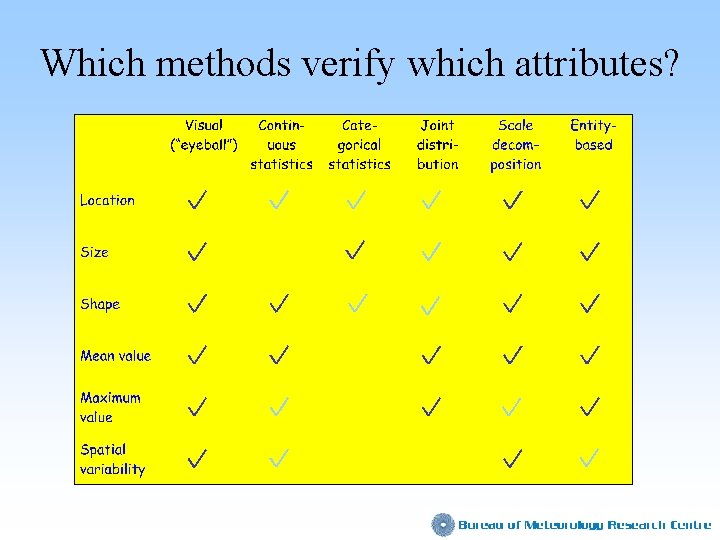
Which methods verify which attributes?
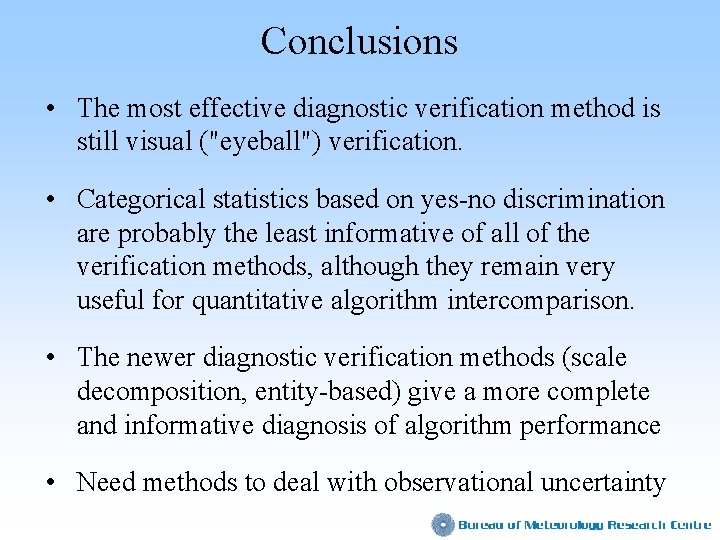
Conclusions • The most effective diagnostic verification method is still visual ("eyeball") verification. • Categorical statistics based on yes-no discrimination are probably the least informative of all of the verification methods, although they remain very useful for quantitative algorithm intercomparison. • The newer diagnostic verification methods (scale decomposition, entity-based) give a more complete and informative diagnosis of algorithm performance • Need methods to deal with observational uncertainty
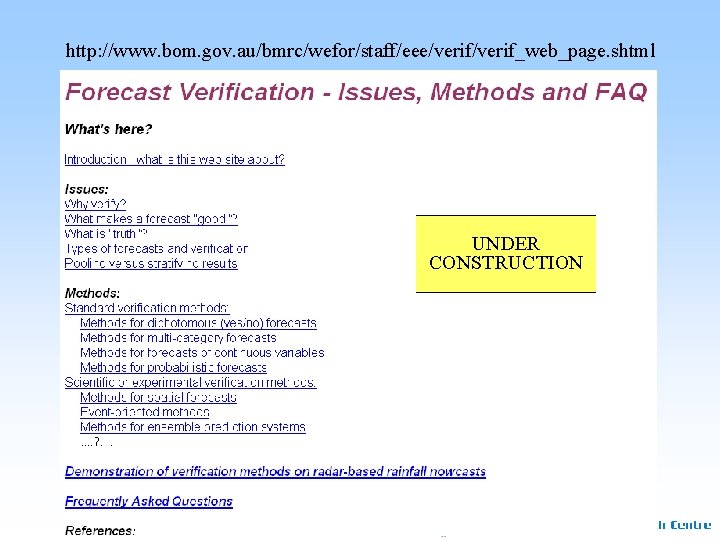
http: //www. bom. gov. au/bmrc/wefor/staff/eee/verif_web_page. shtml _________ UNDER CONSTRUCTION _________
Gravimetry steps
Co precipitation and post precipitation
National meteorological and hydrological services
Vietnam meteorological and hydrological administration
Croatian meteorological and hydrological service
Hydrological
Hydrological cycle
Hydrological cycle diagram
Hydrological prediction center
Cot2x identity
Verifying trig identities
5-2 verifying trigonometric identities
Dea number example
5-2 verifying trigonometric identities
Verifying trig functions
5-2 practice verifying trigonometric identities
Completing a death certificate geeky medics
What transformation can verify congruence
Caribbean institute for meteorology and hydrology
Who global estimates on prevalence of hearing loss 2020
Who global estimates on prevalence of hearing loss 2020
Jessie estimates the weight of her cat
Gauss markov assumptions
Dcms economic estimates
Building maintenance cost estimates
Job order costing
Fermi estimates
Fermi estimate
The account analysis method estimates cost functions
Marquis company estimates that annual manufacturing
Weather map station model light rain
Whether the weather tongue twister
Poem fashion
It's windy weather it's stormy weather
Weather and whether
Heavy weather by weather report
Capital weather gang weather wall
Fspos vägledning för kontinuitetshantering
Typiska novell drag
Nationell inriktning för artificiell intelligens
Vad står k.r.å.k.a.n för
Shingelfrisyren
En lathund för arbete med kontinuitetshantering
Särskild löneskatt för pensionskostnader
Vilotidsbok
A gastrica
Densitet vatten
Datorkunskap för nybörjare
Stig kerman
Debatt artikel mall
Autokratiskt ledarskap
Nyckelkompetenser för livslångt lärande
Påbyggnader för flakfordon
Vätsketryck formel
Offentlig förvaltning
Kyssande vind analys
Presentera för publik crossboss
Teckenspråk minoritetsspråk argument