The Regression Discontinuity Design Hctor LamadridFigueroa Center for
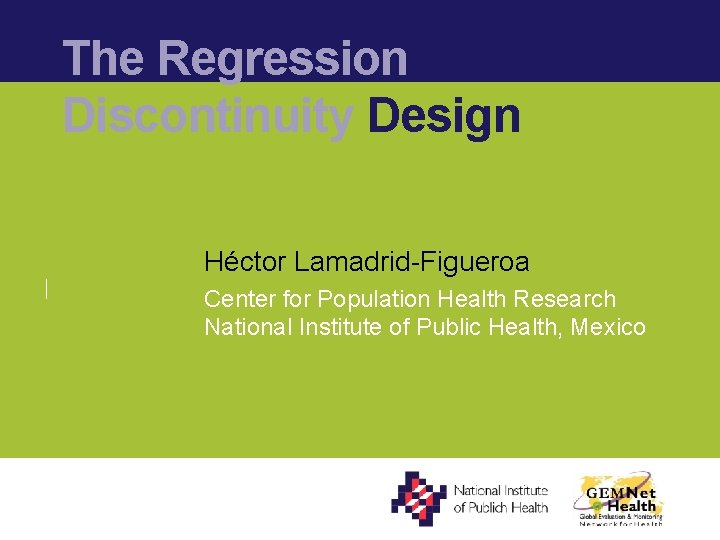
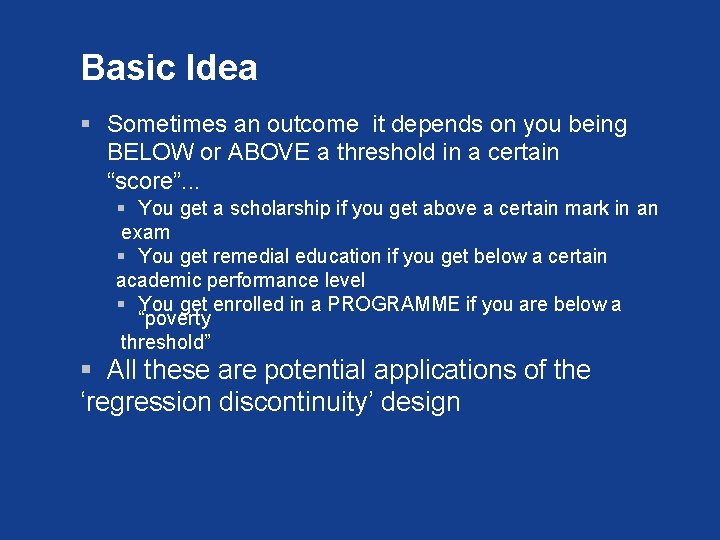
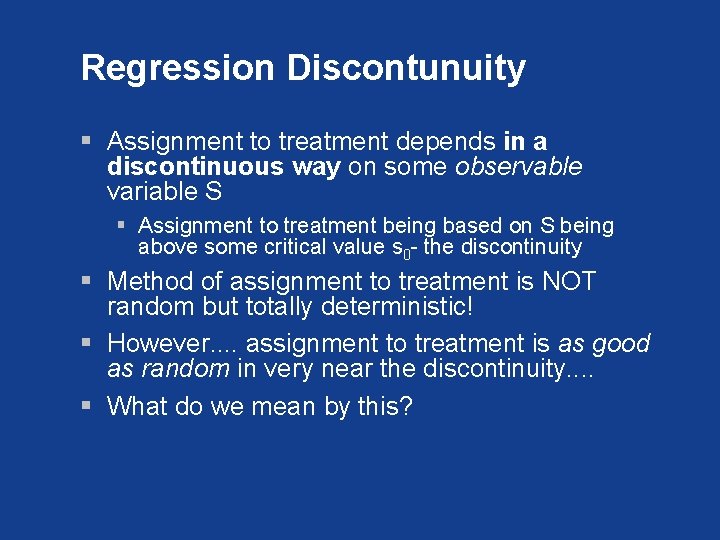
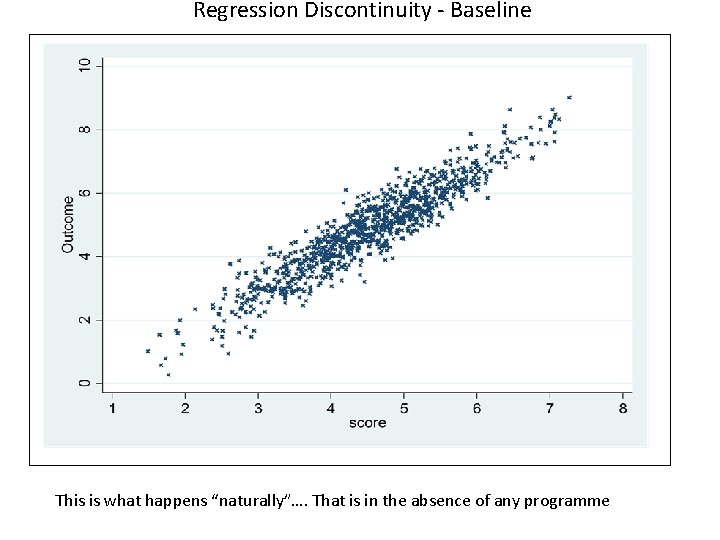
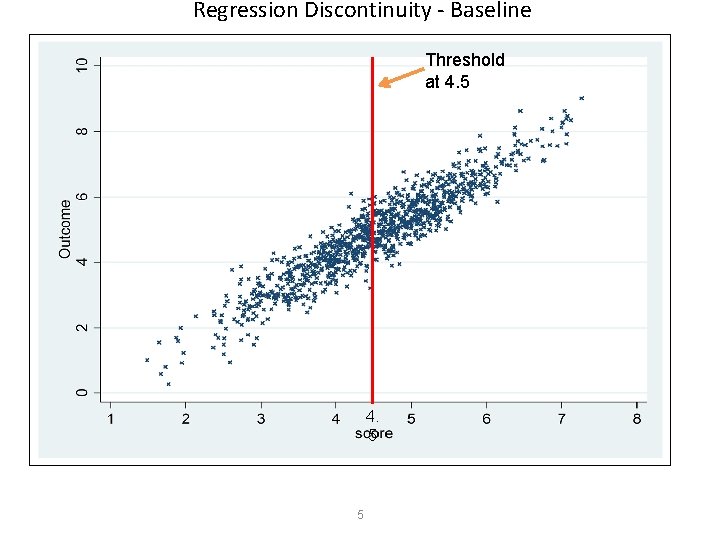
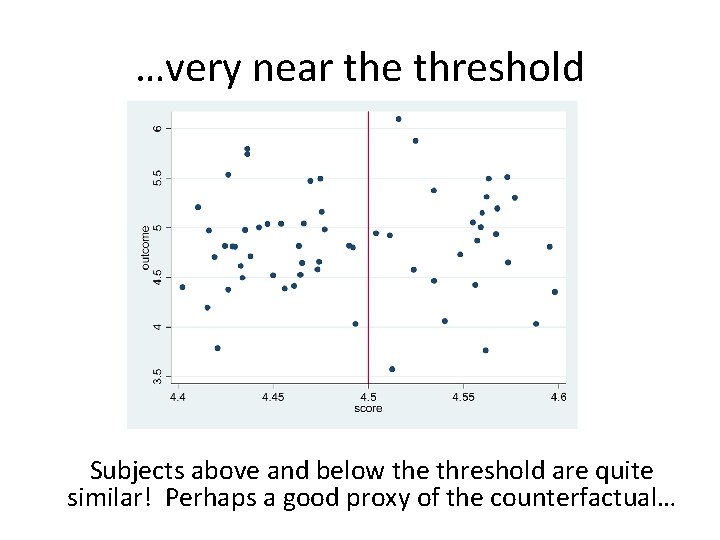
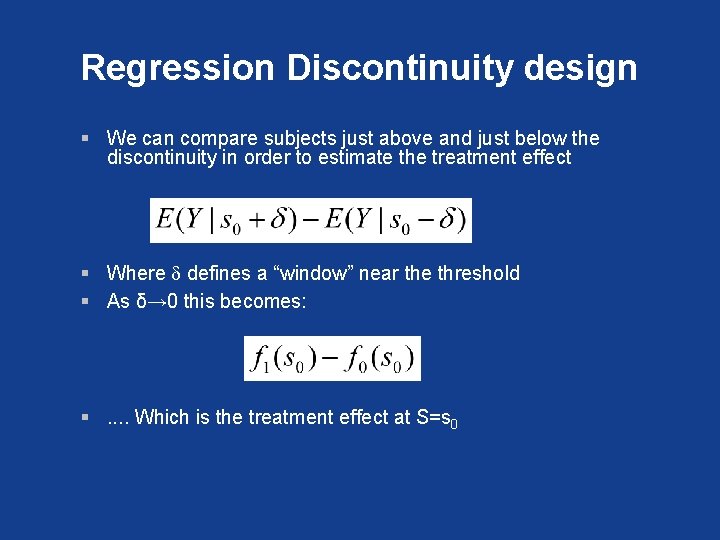
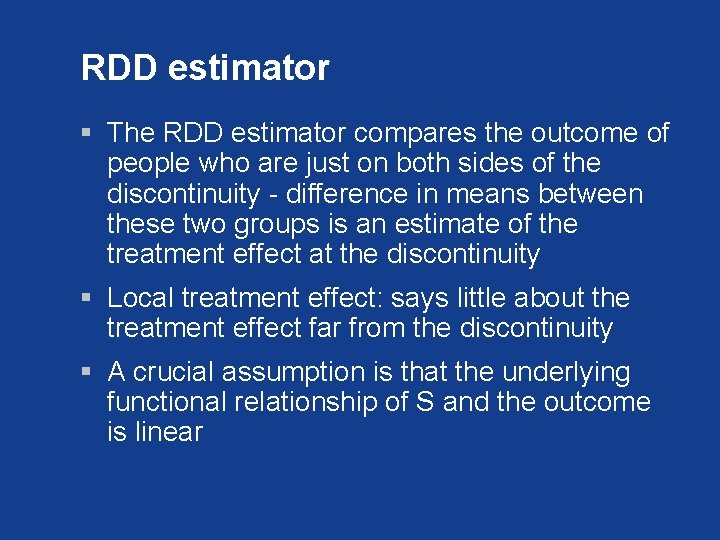
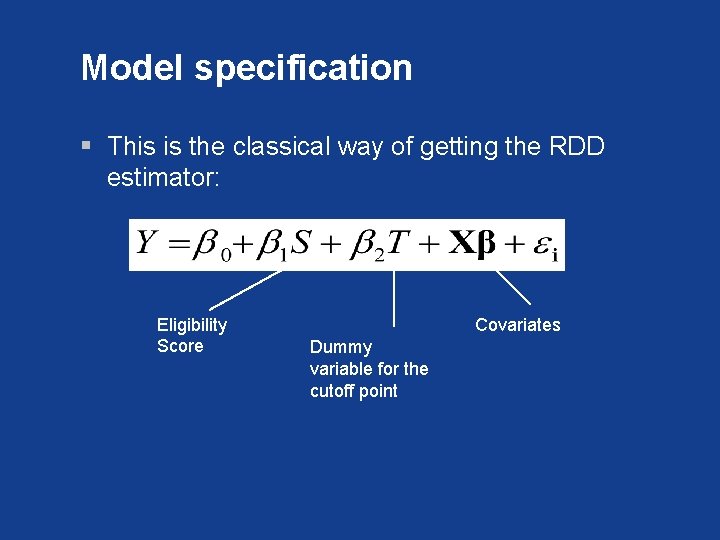
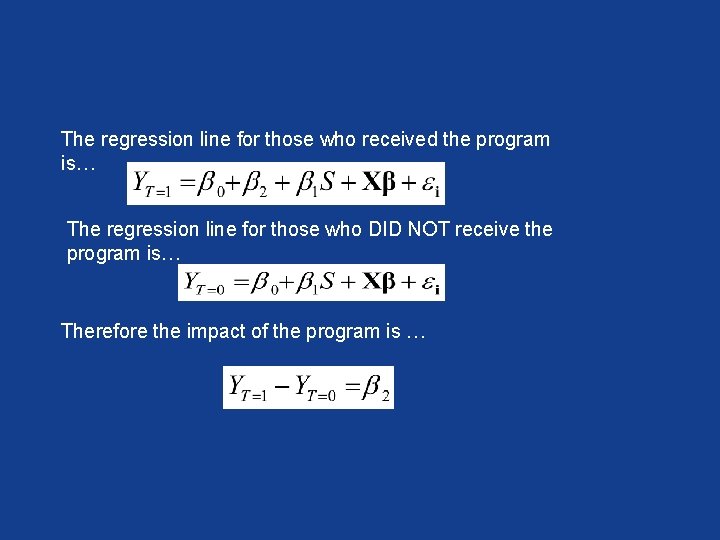
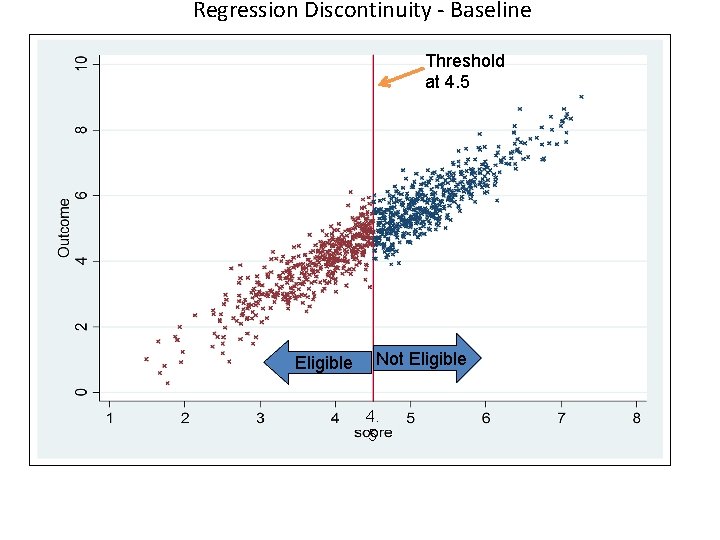
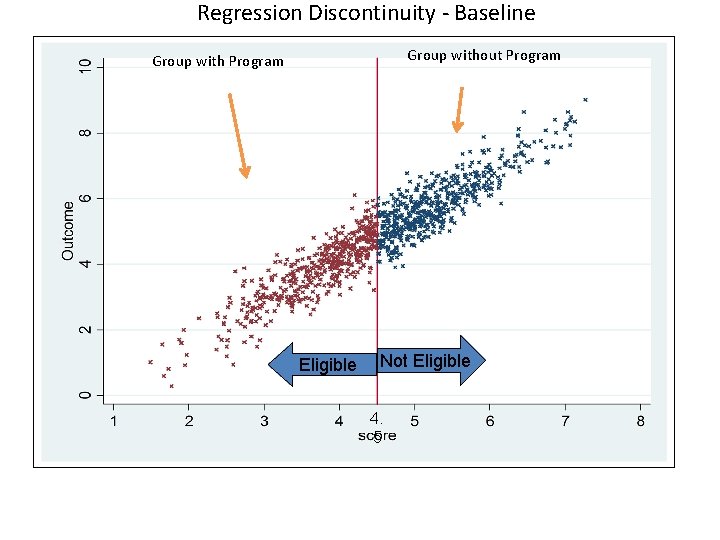
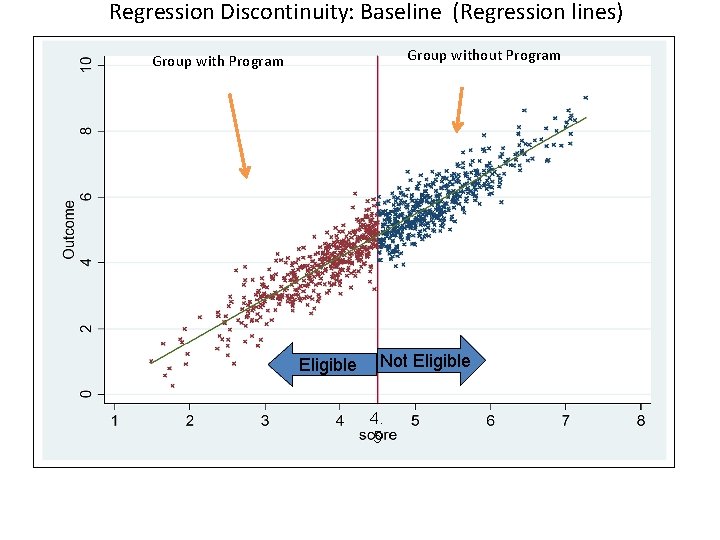
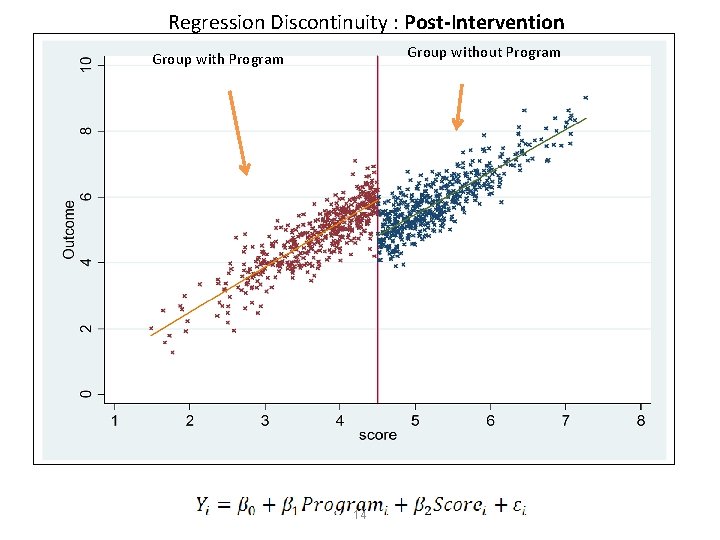
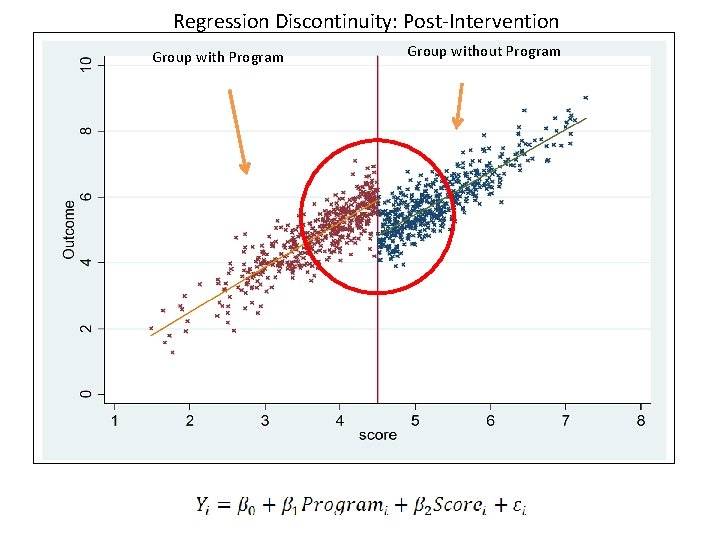
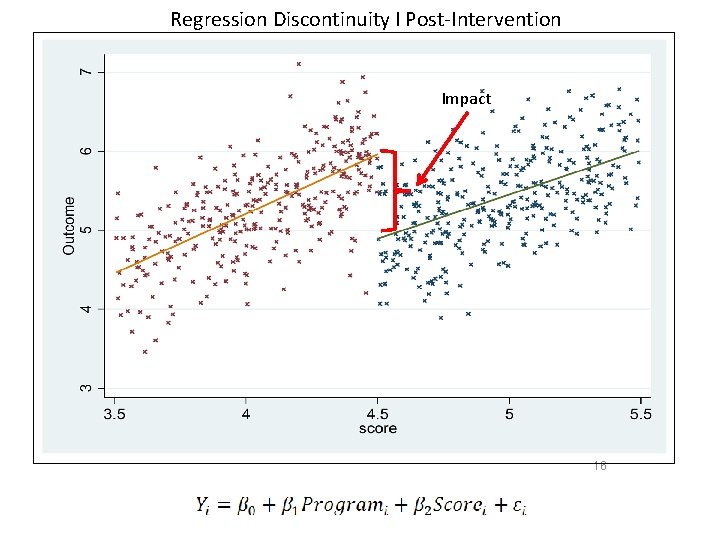
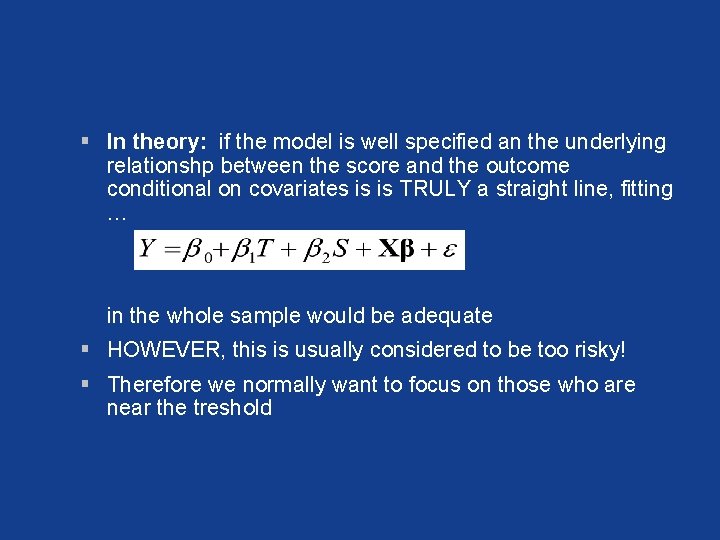
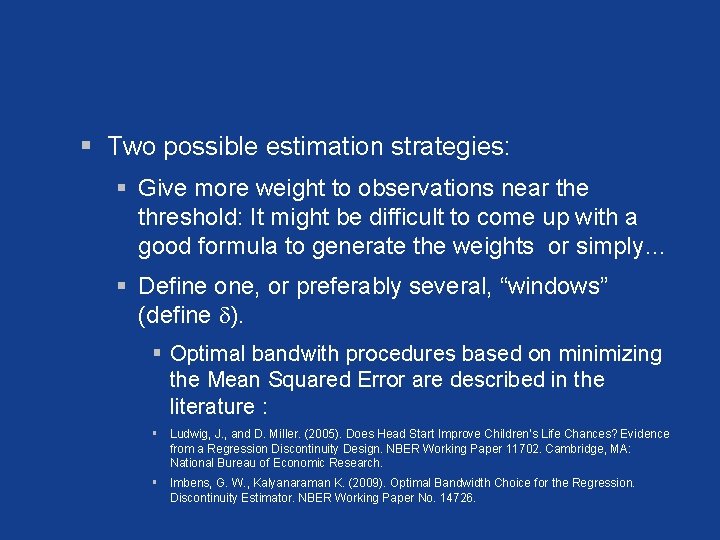
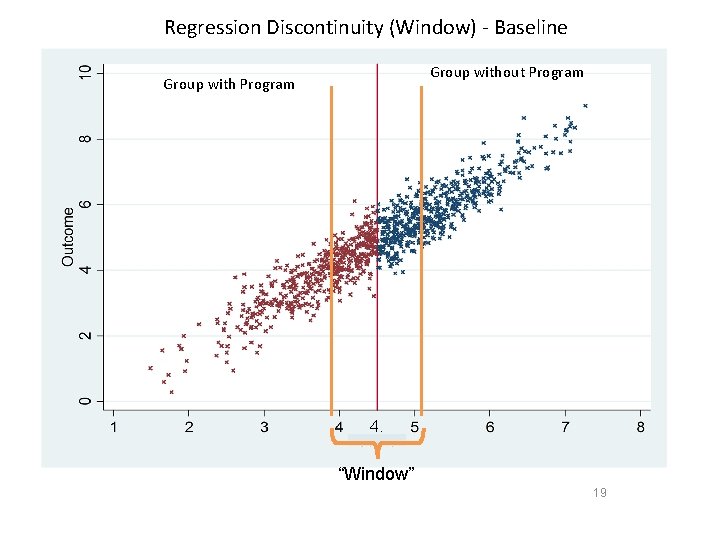
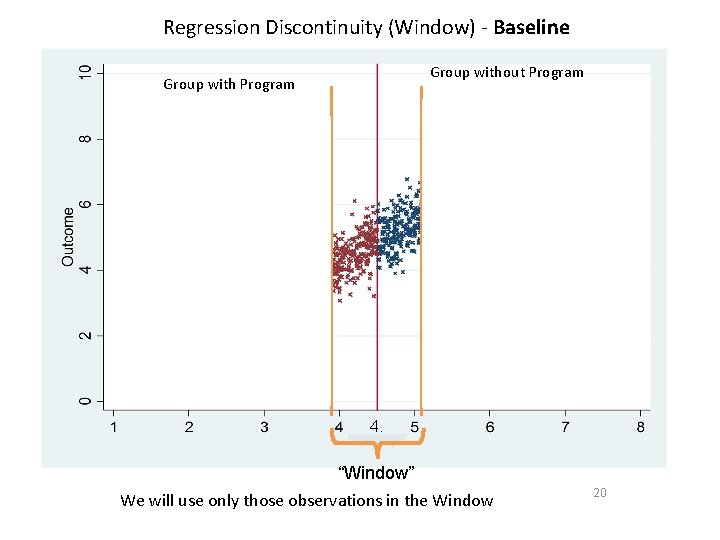
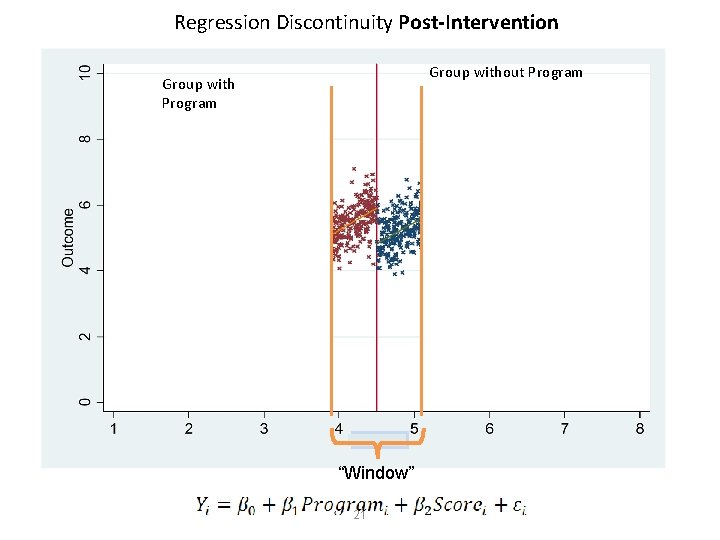
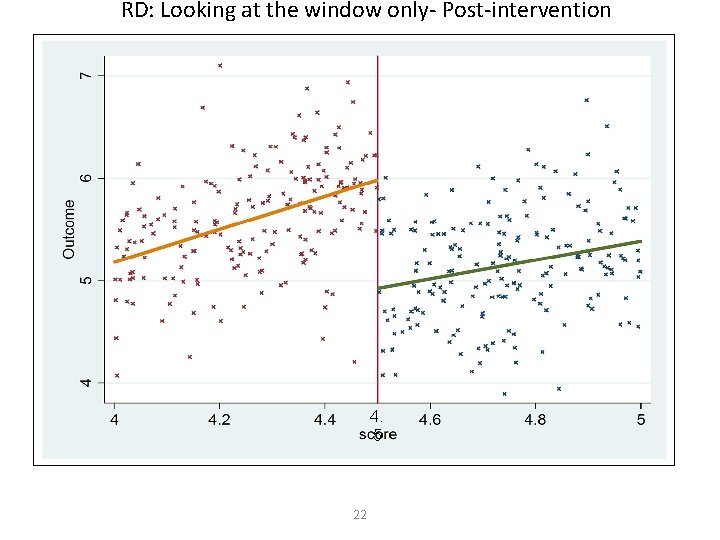
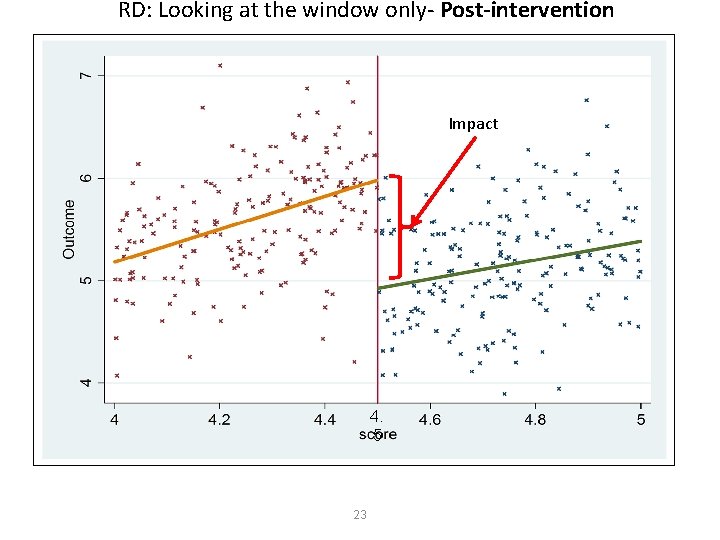
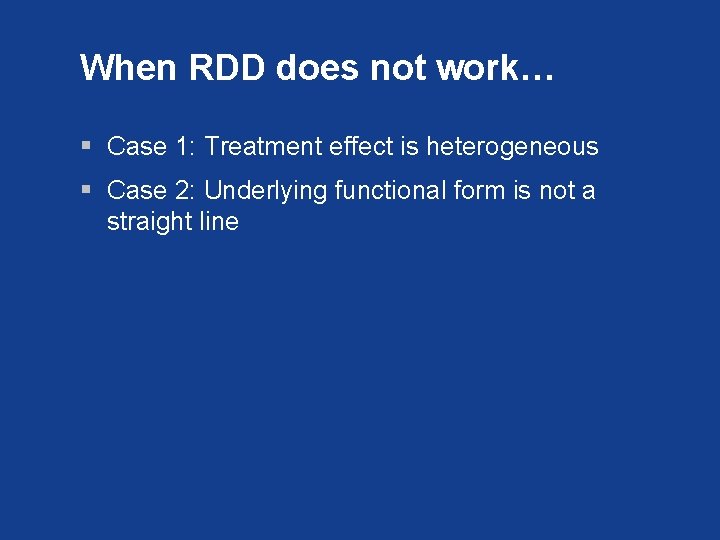
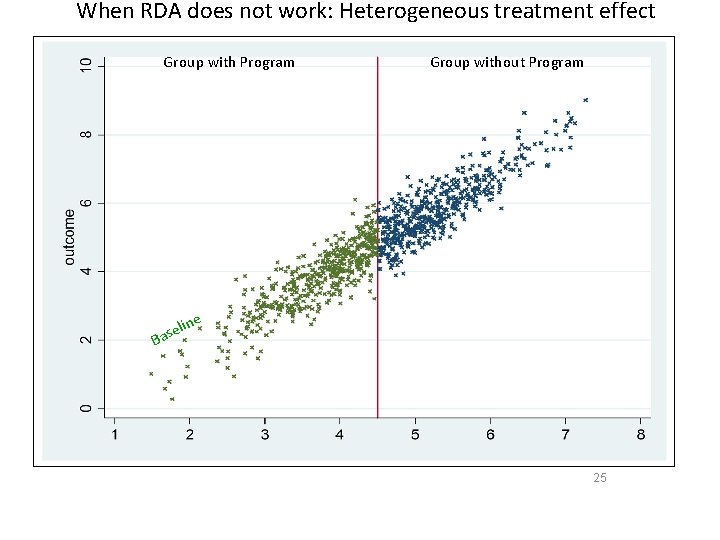
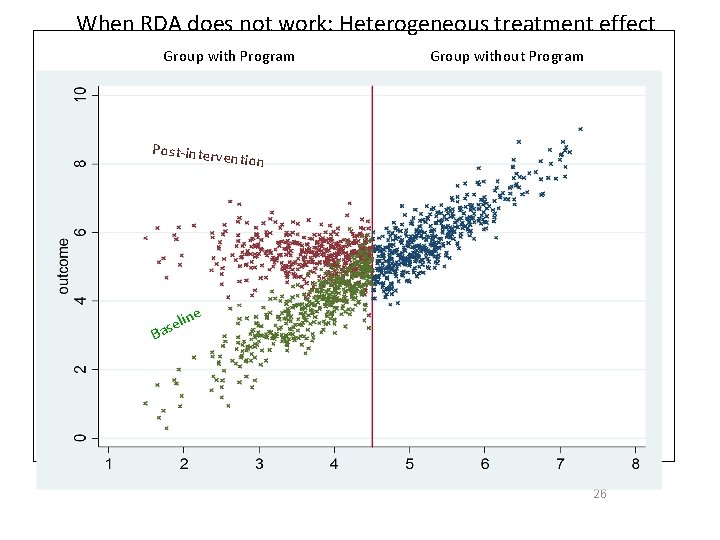
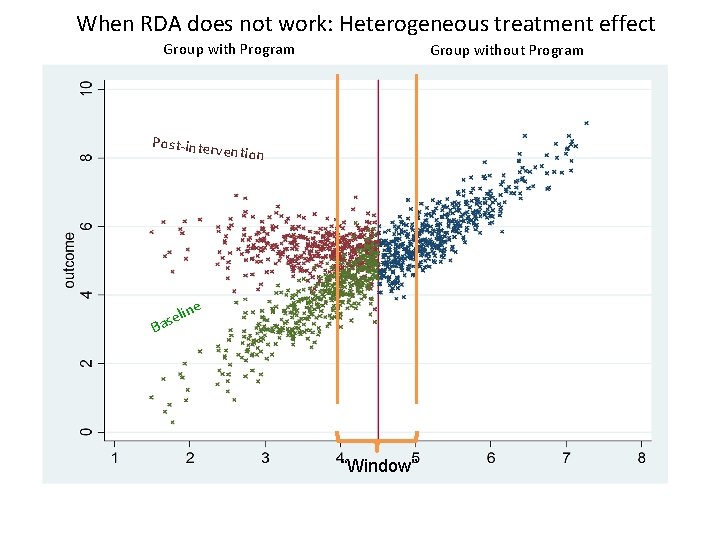
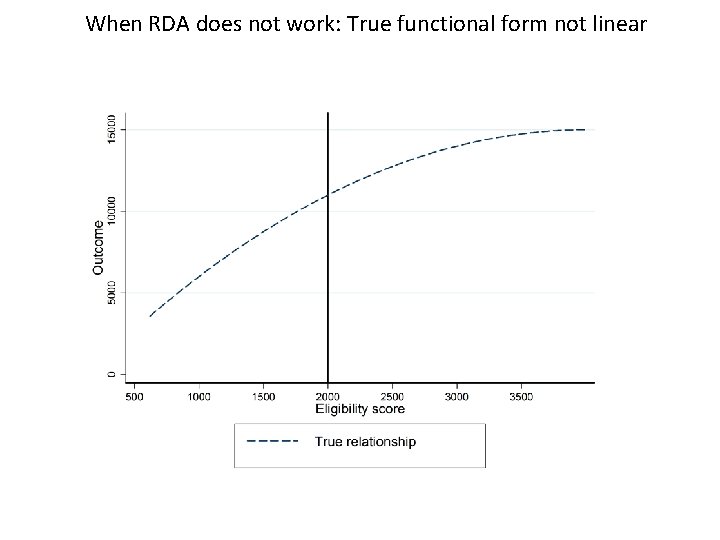
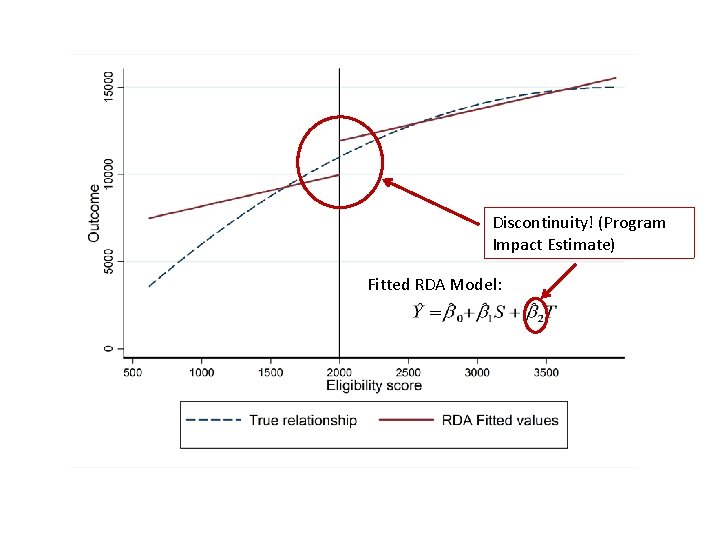
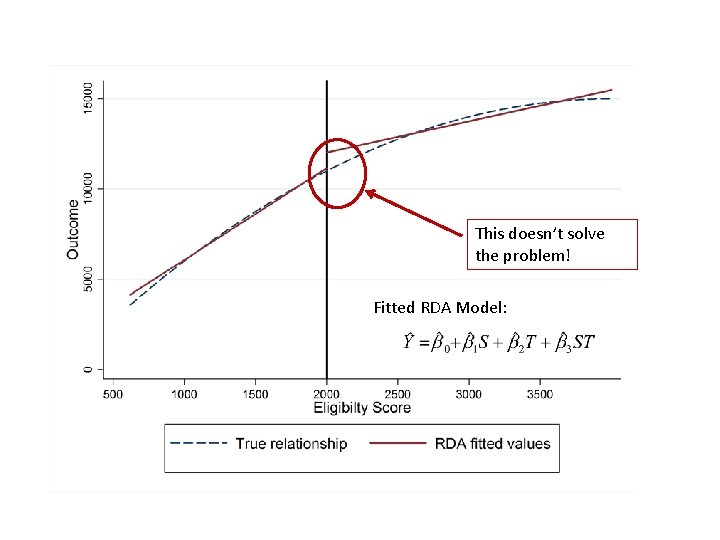
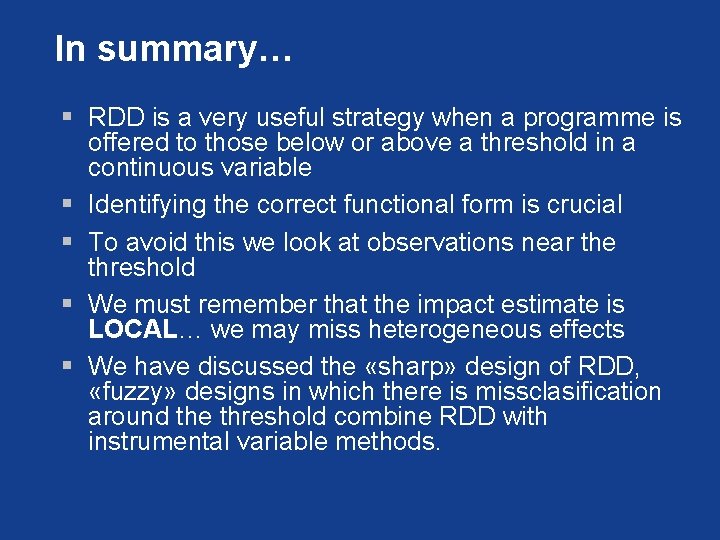
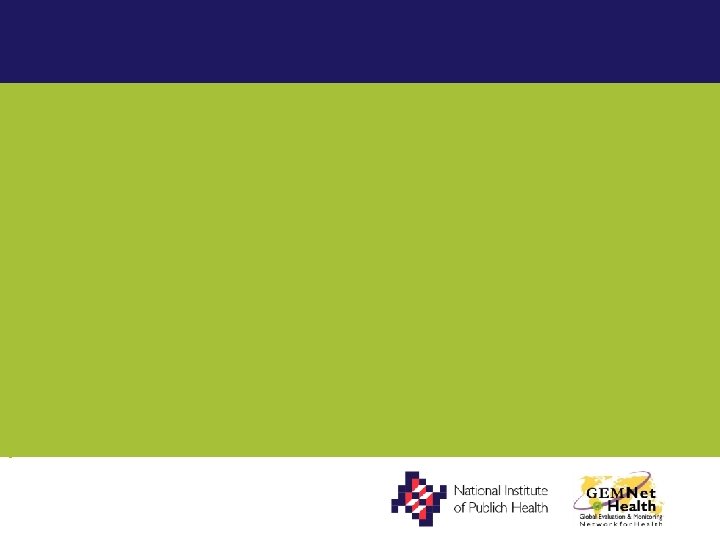
- Slides: 32
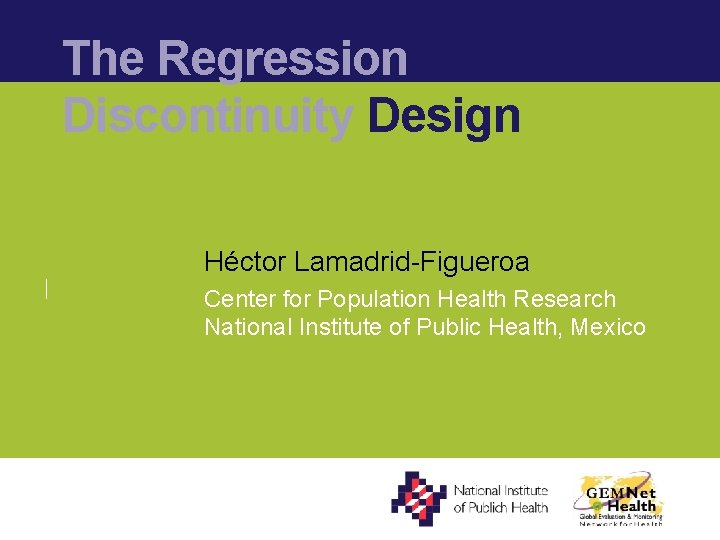
The Regression Discontinuity Design Héctor Lamadrid-Figueroa Center for Population Health Research National Institute of Public Health, Mexico
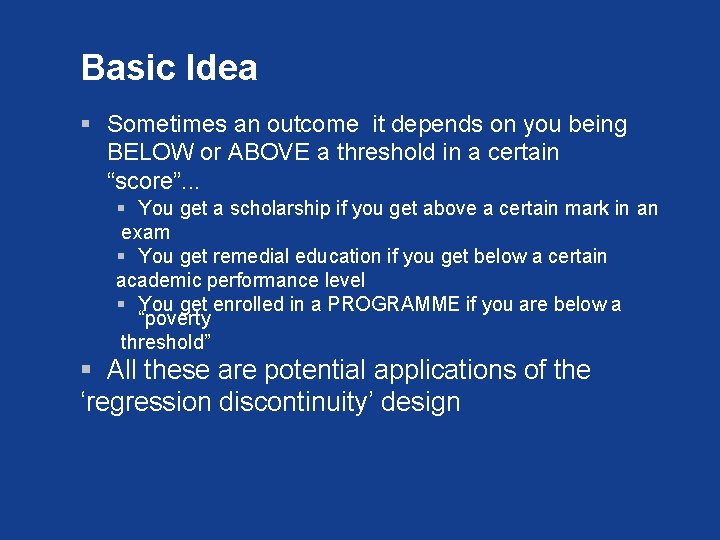
Basic Idea § Sometimes an outcome it depends on you being BELOW or ABOVE a threshold in a certain “score”. . . § You get a scholarship if you get above a certain mark in an exam § You get remedial education if you get below a certain academic performance level § You get enrolled in a PROGRAMME if you are below a “poverty threshold” § All these are potential applications of the ‘regression discontinuity’ design
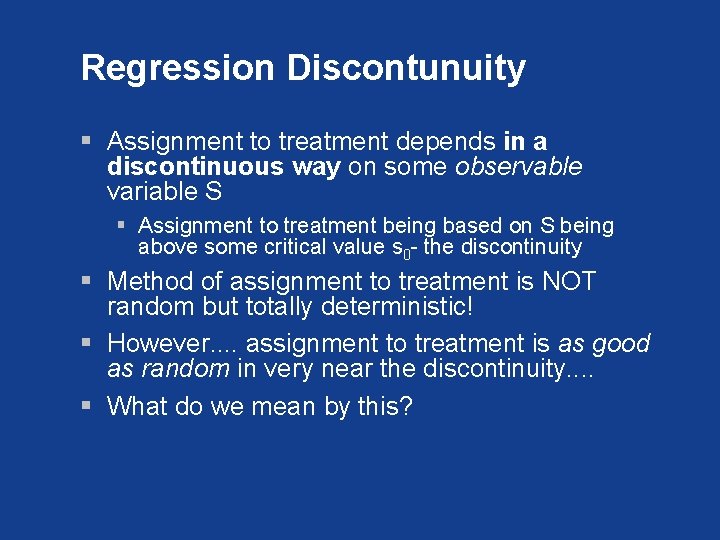
Regression Discontunuity § Assignment to treatment depends in a discontinuous way on some observable variable S § Assignment to treatment being based on S being above some critical value s 0 - the discontinuity § Method of assignment to treatment is NOT random but totally deterministic! § However. . assignment to treatment is as good as random in very near the discontinuity. . § What do we mean by this?
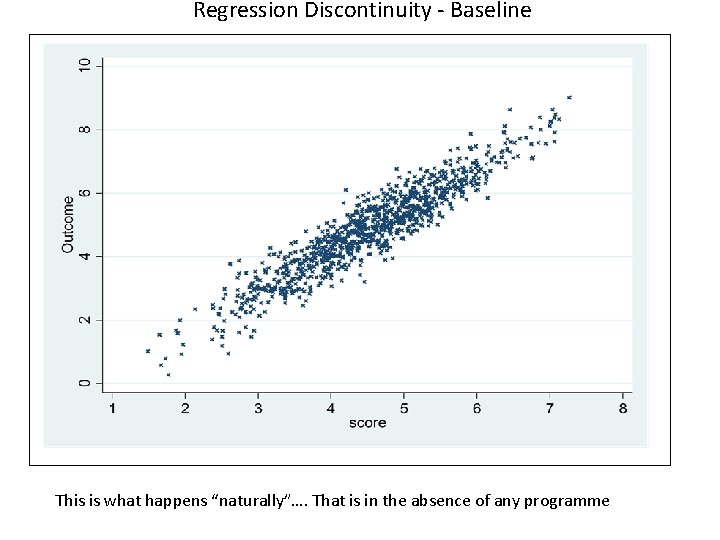
Regression Discontinuity - Baseline This is what happens “naturally”…. That is in the absence of any programme
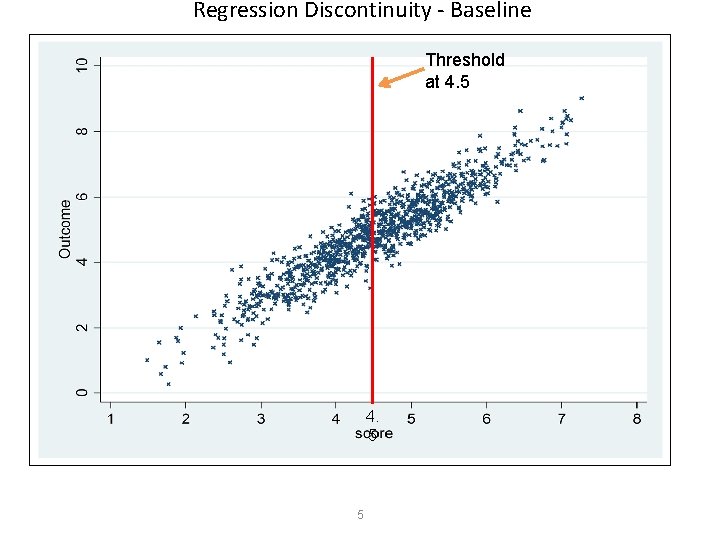
Regression Discontinuity - Baseline Threshold at 4. 5 5
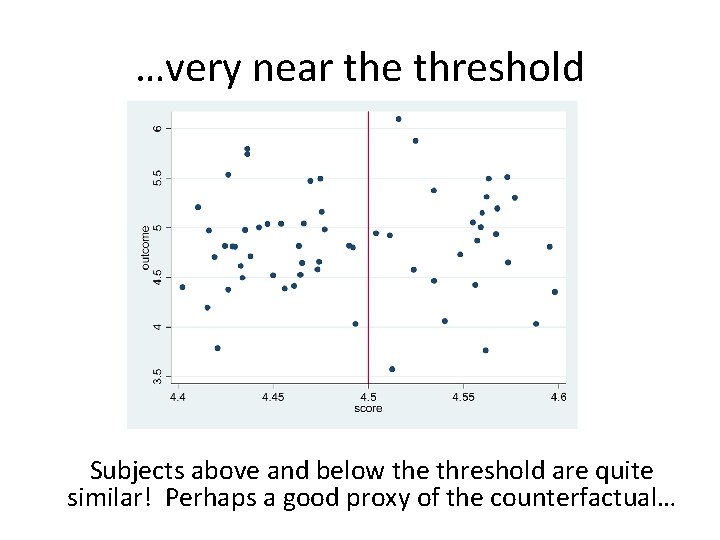
…very near the threshold Subjects above and below the threshold are quite similar! Perhaps a good proxy of the counterfactual…
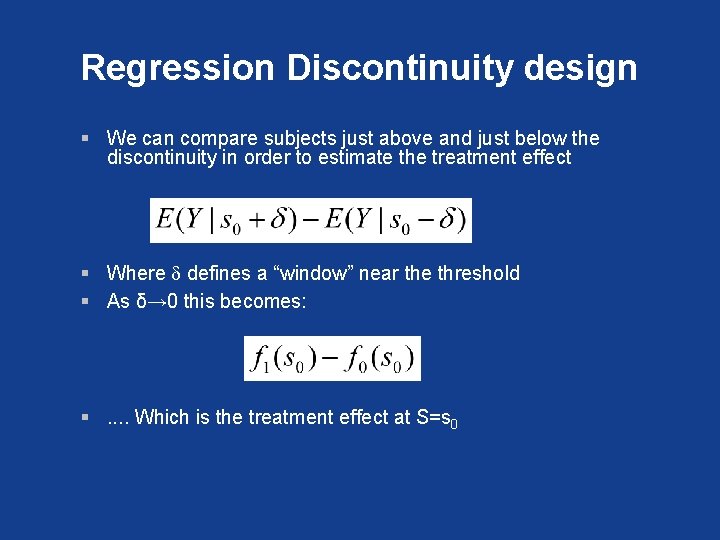
Regression Discontinuity design § We can compare subjects just above and just below the discontinuity in order to estimate the treatment effect § Where d defines a “window” near the threshold § As δ→ 0 this becomes: §. . Which is the treatment effect at S=s 0
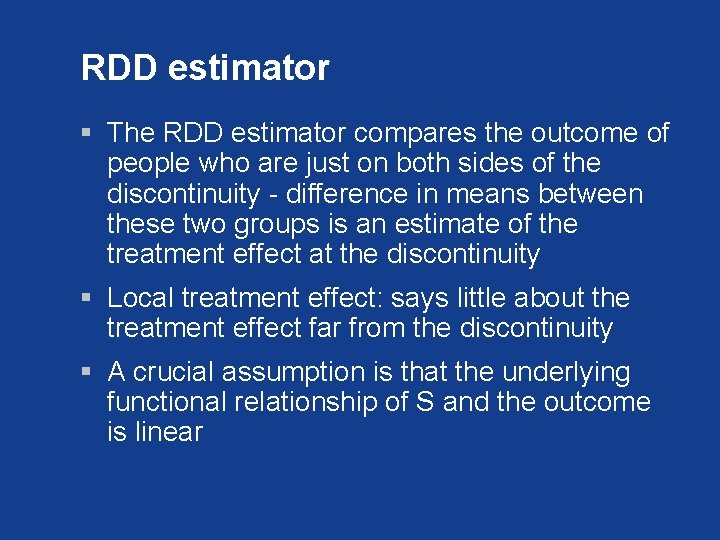
RDD estimator § The RDD estimator compares the outcome of people who are just on both sides of the discontinuity - difference in means between these two groups is an estimate of the treatment effect at the discontinuity § Local treatment effect: says little about the treatment effect far from the discontinuity § A crucial assumption is that the underlying functional relationship of S and the outcome is linear
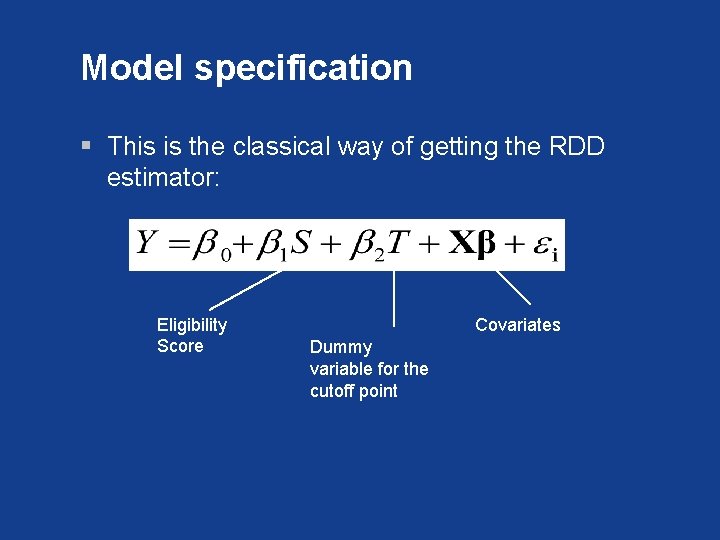
Model specification § This is the classical way of getting the RDD estimator: Eligibility Score Covariates Dummy variable for the cutoff point
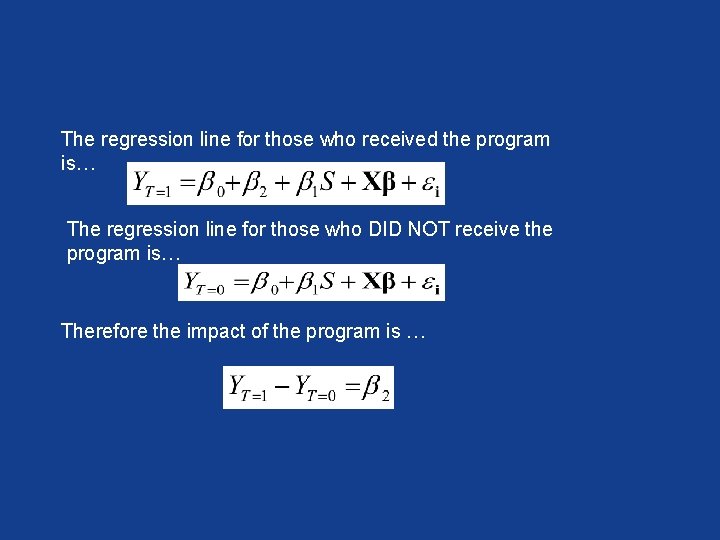
The regression line for those who received the program is… The regression line for those who DID NOT receive the program is… Therefore the impact of the program is …
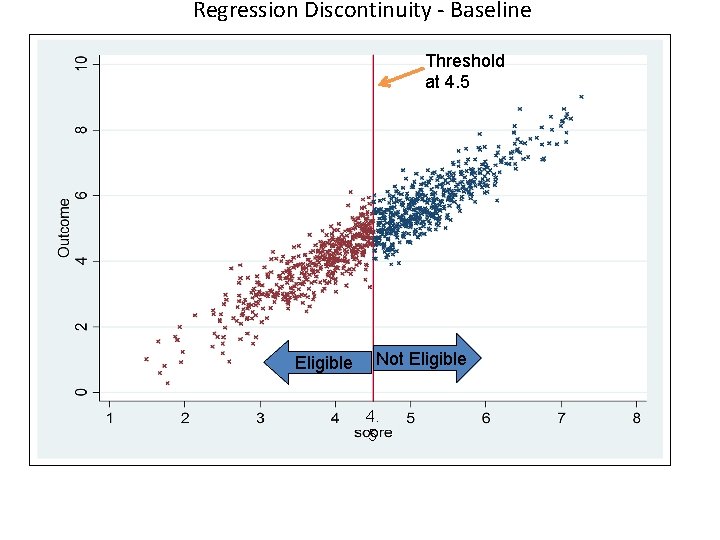
Regression Discontinuity - Baseline Threshold at 4. 5 Eligible Not Eligible 4. 5
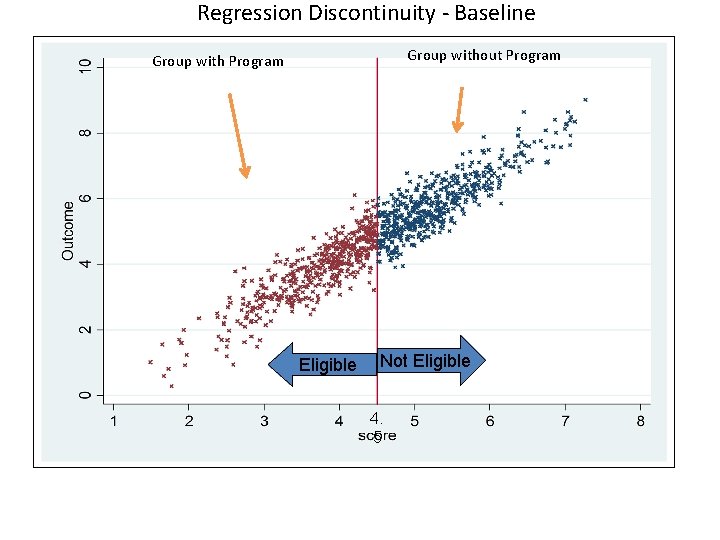
Regression Discontinuity - Baseline Group without Program Group with Program Eligible Not Eligible 4. 5
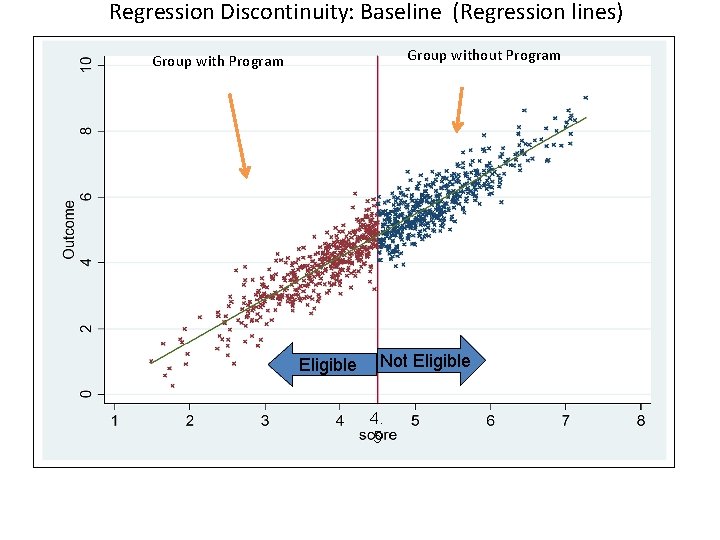
Regression Discontinuity: Baseline (Regression lines) Group without Program Group with Program Eligible Not Eligible 4. 5
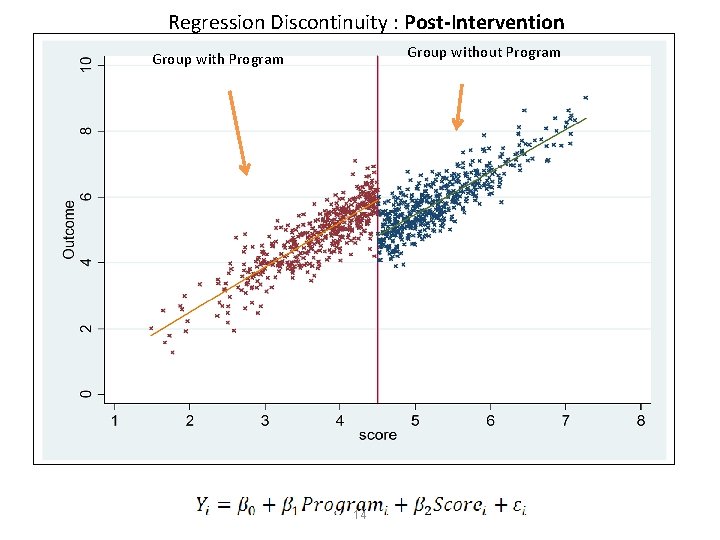
Regression Discontinuity : Post-Intervention Group without Program Group with Program 14
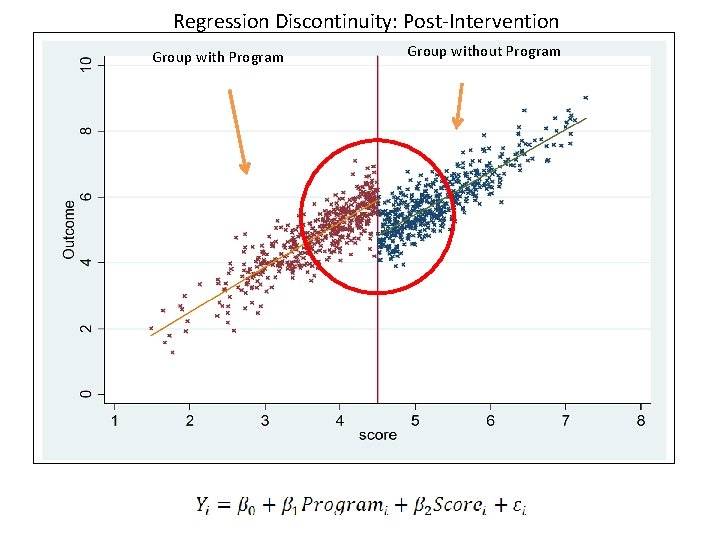
Regression Discontinuity: Post-Intervention Group with Program Group without Program
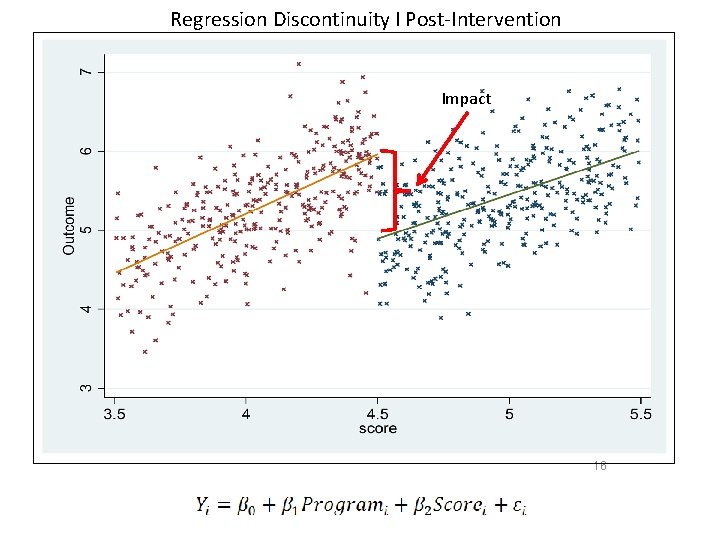
Regression Discontinuity I Post-Intervention Impact 16
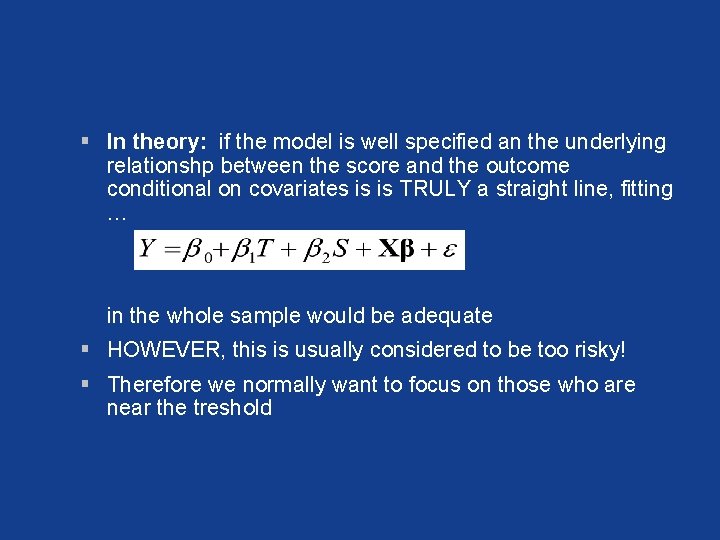
§ In theory: if the model is well specified an the underlying relationshp between the score and the outcome conditional on covariates is is TRULY a straight line, fitting … in the whole sample would be adequate § HOWEVER, this is usually considered to be too risky! § Therefore we normally want to focus on those who are near the treshold
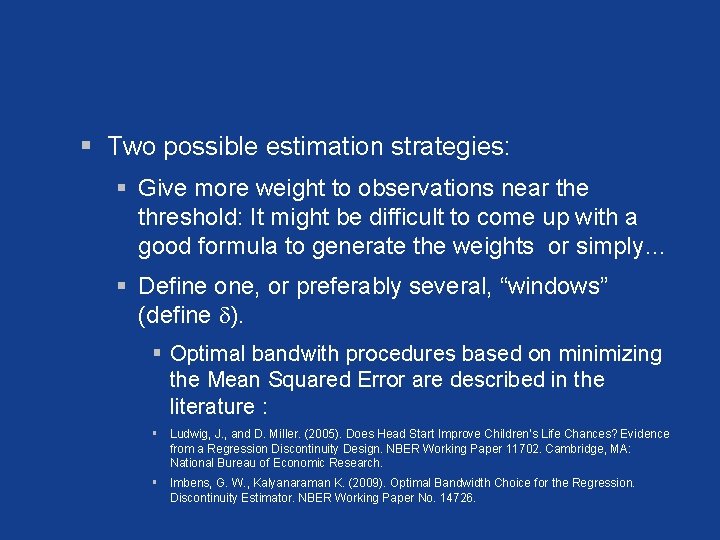
§ Two possible estimation strategies: § Give more weight to observations near the threshold: It might be difficult to come up with a good formula to generate the weights or simply… § Define one, or preferably several, “windows” (define d). § Optimal bandwith procedures based on minimizing the Mean Squared Error are described in the literature : § Ludwig, J. , and D. Miller. (2005). Does Head Start Improve Children’s Life Chances? Evidence from a Regression Discontinuity Design. NBER Working Paper 11702. Cambridge, MA: National Bureau of Economic Research. § Imbens, G. W. , Kalyanaraman K. (2009). Optimal Bandwidth Choice for the Regression. Discontinuity Estimator. NBER Working Paper No. 14726.
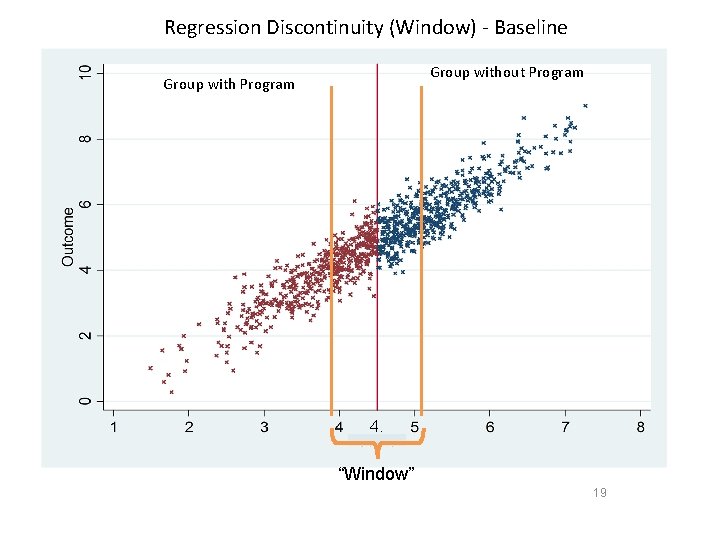
Regression Discontinuity (Window) - Baseline Group without Program Group with Program 4. 5 “Window” 19
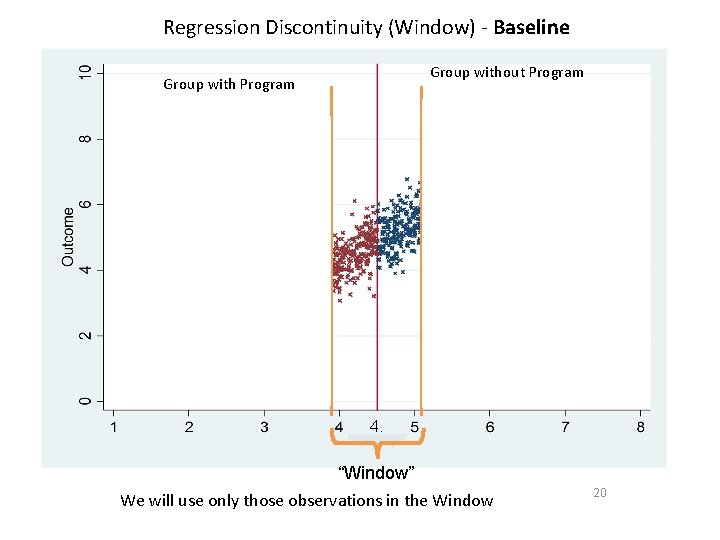
Regression Discontinuity (Window) - Baseline Group without Program Group with Program 4. 5 “Window” We will use only those observations in the Window 20
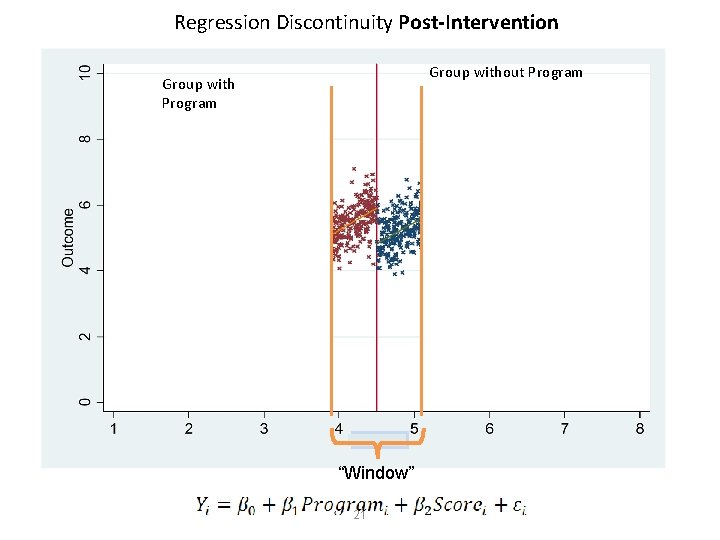
Regression Discontinuity Post-Intervention Group without Program Group with Program “Window” 21
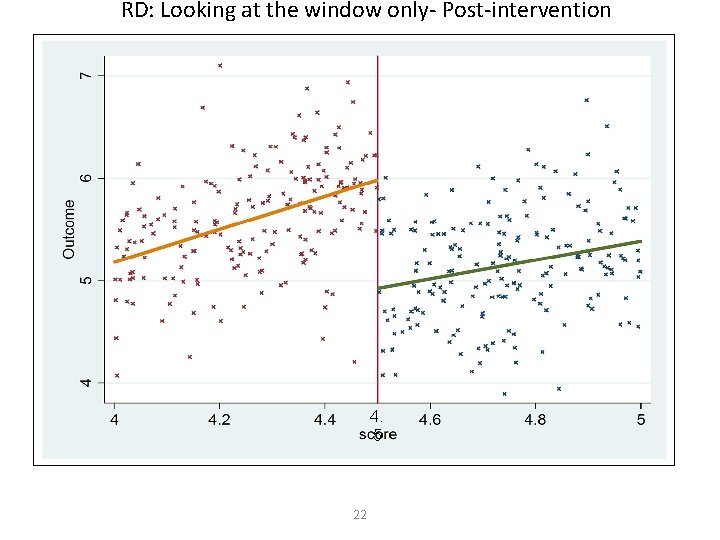
RD: Looking at the window only- Post-intervention 4. 5 22
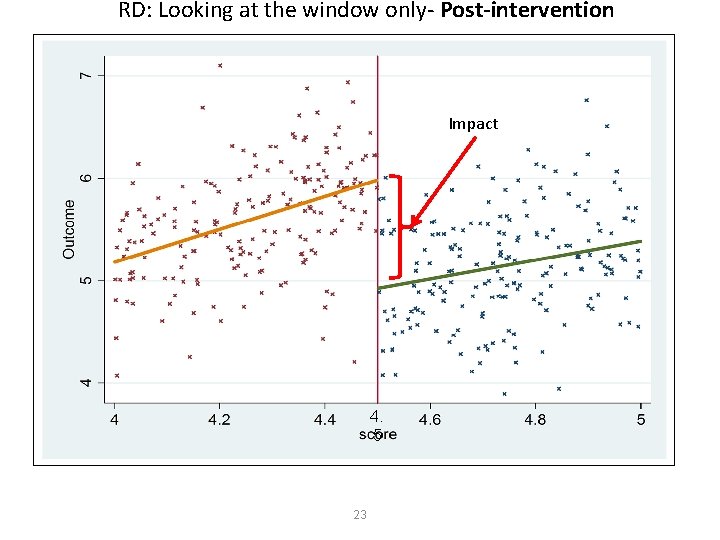
RD: Looking at the window only- Post-intervention Impact 4. 5 23
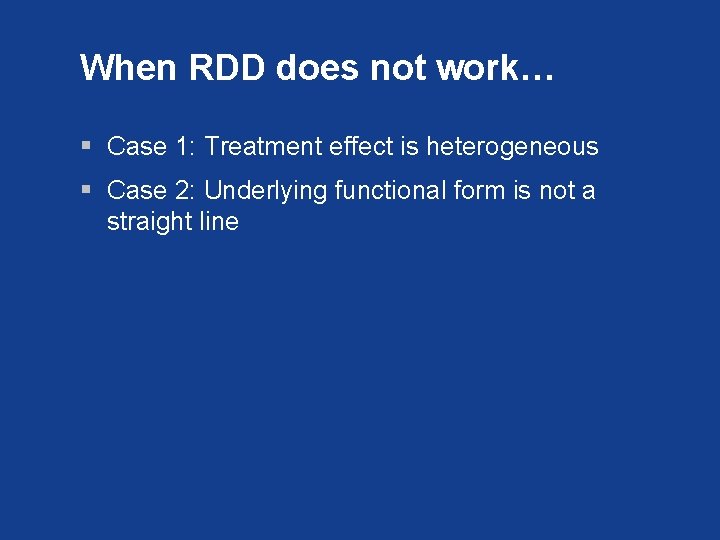
When RDD does not work… § Case 1: Treatment effect is heterogeneous § Case 2: Underlying functional form is not a straight line
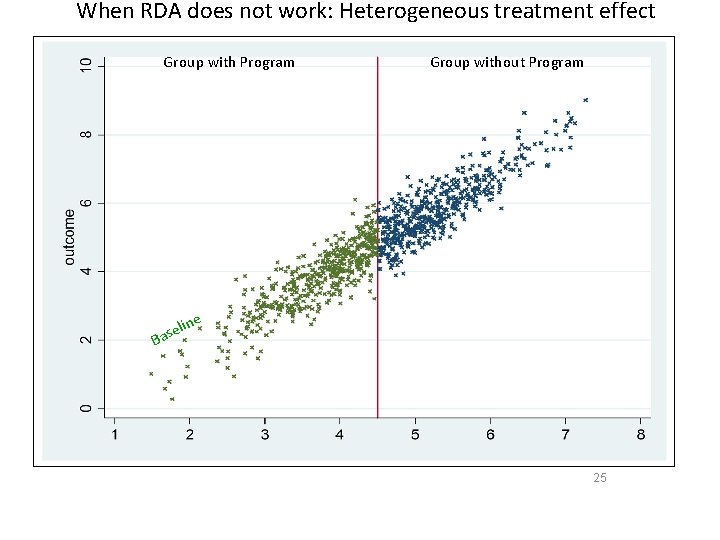
When RDA does not work: Heterogeneous treatment effect Group with Program Group without Program ine el s a B 25
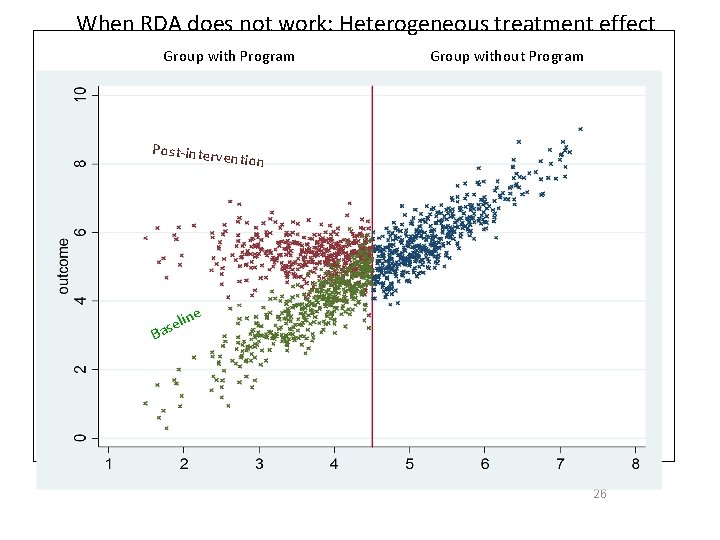
When RDA does not work: Heterogeneous treatment effect Group with Program Group without Program Post-interv ention e lin e s a B 26
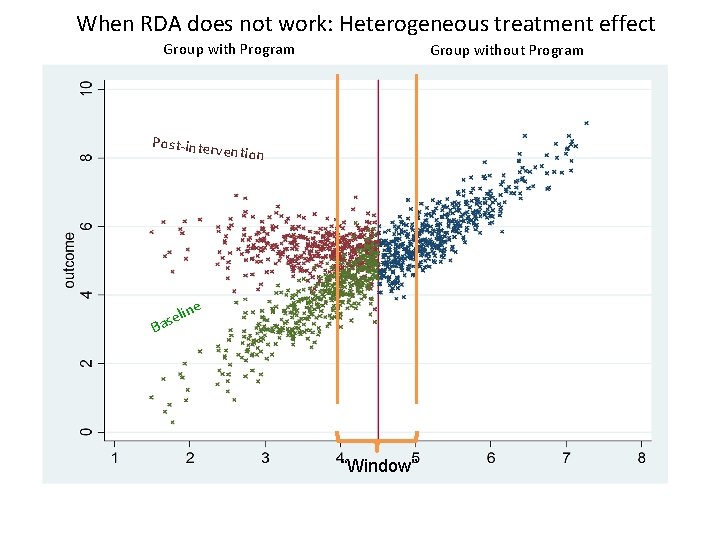
When RDA does not work: Heterogeneous treatment effect Group with Program Group without Program Post-interv ention ine el s a B “Window” 27
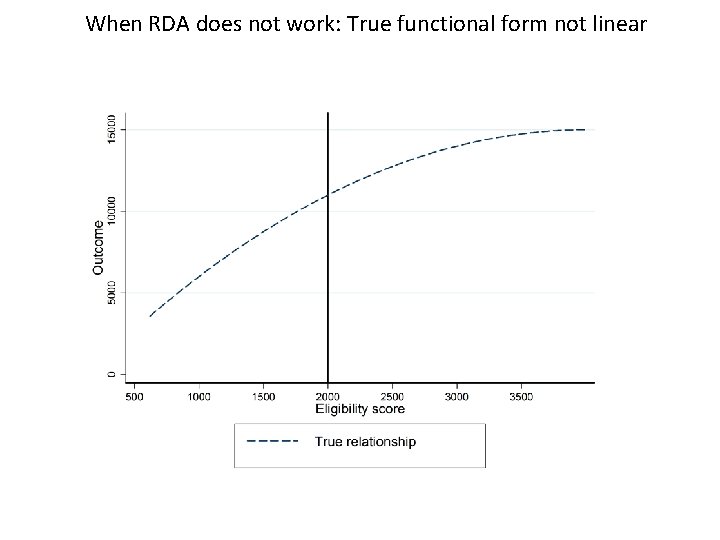
When RDA does not work: True functional form not linear
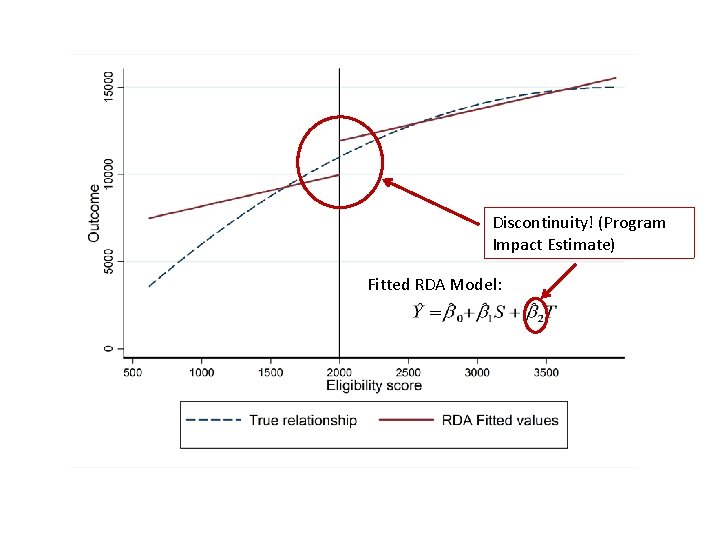
Discontinuity! (Program Impact Estimate) Fitted RDA Model:
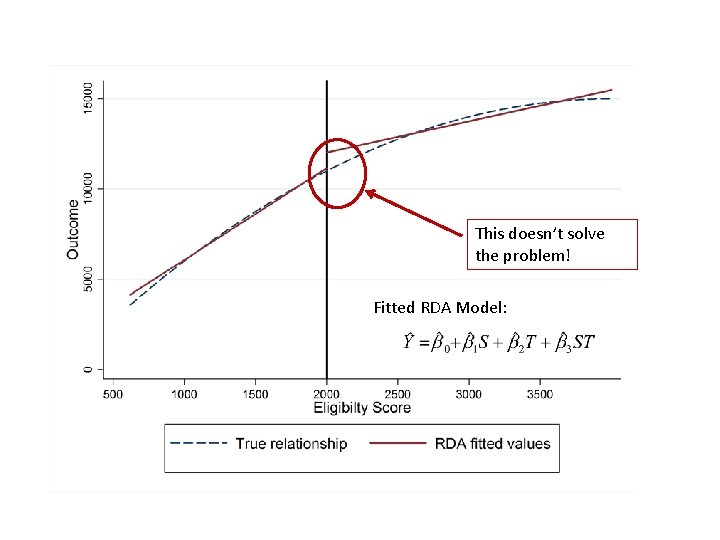
This doesn’t solve the problem! Fitted RDA Model:
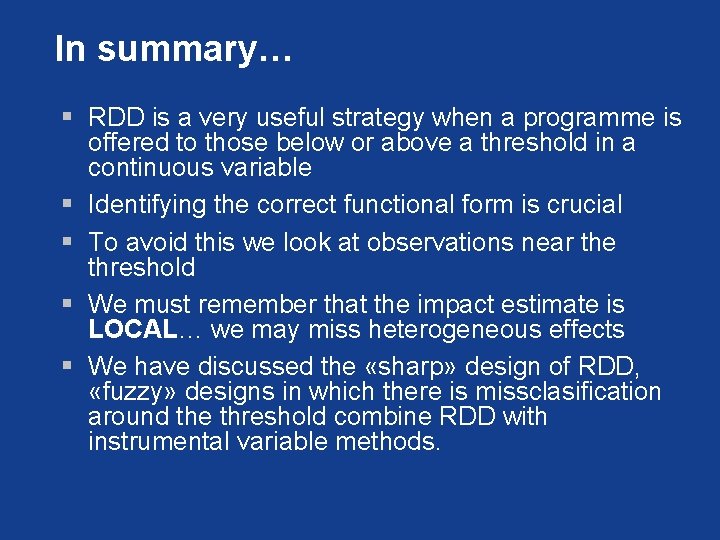
In summary… § RDD is a very useful strategy when a programme is offered to those below or above a threshold in a continuous variable § Identifying the correct functional form is crucial § To avoid this we look at observations near the threshold § We must remember that the impact estimate is LOCAL… we may miss heterogeneous effects § We have discussed the «sharp» design of RDD, «fuzzy» designs in which there is missclasification around the threshold combine RDD with instrumental variable methods.
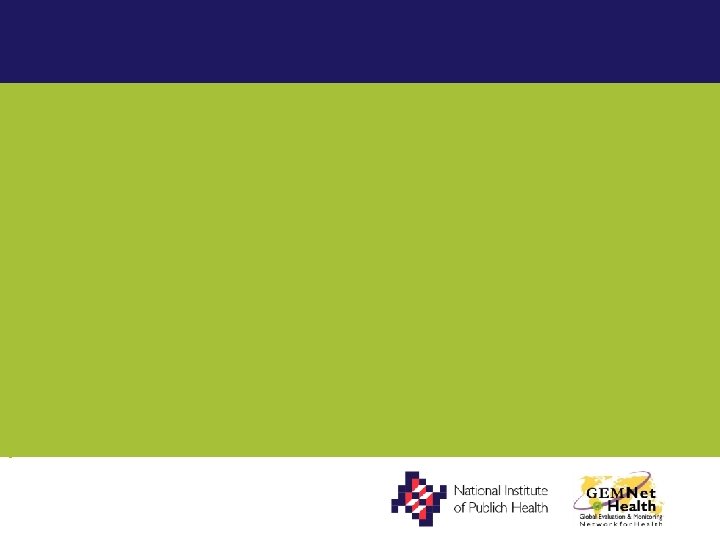
Regression discontinuity
Regression discontinuity
Interrupted time series vs regression discontinuity
Simple and multiple linear regression
Multiple regression vs linear regression
Survival analysis vs logistic regression
Logistic regression vs linear regression
Multiple regression research design
Kontinuitetshantering
Typiska drag för en novell
Tack för att ni lyssnade bild
Ekologiskt fotavtryck
Varför kallas perioden 1918-1939 för mellankrigstiden
En lathund för arbete med kontinuitetshantering
Adressändring ideell förening
Personlig tidbok för yrkesförare
Anatomi organ reproduksi
Vad är densitet
Datorkunskap för nybörjare
Stig kerman
Att skriva en debattartikel
Magnetsjukhus
Nyckelkompetenser för livslångt lärande
Påbyggnader för flakfordon
Arkimedes princip formel
Svenskt ramverk för digital samverkan
Bo bergman jag fryser om dina händer
Presentera för publik crossboss
Jiddisch
Bat mitza
Treserva lathund
Fimbrietratt
Claes martinsson