An Empirical Test of the Regression Discontinuity Design
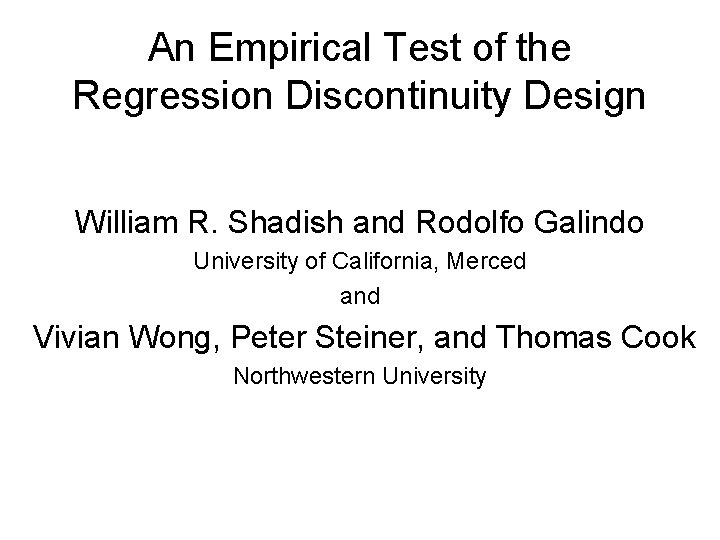
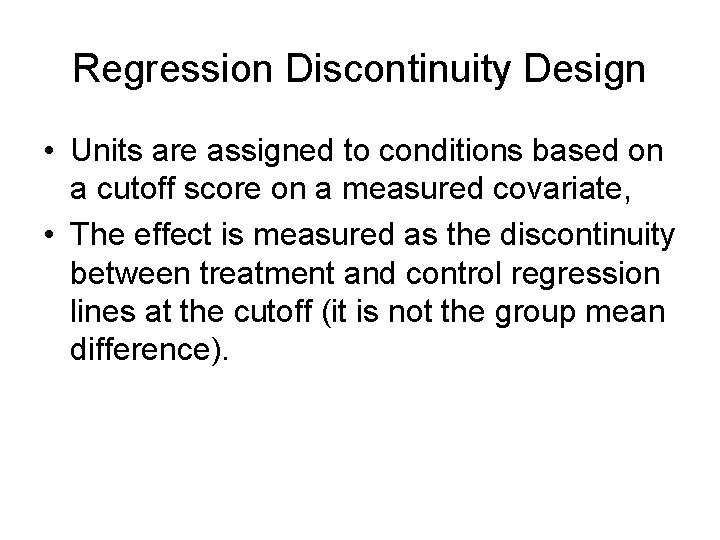
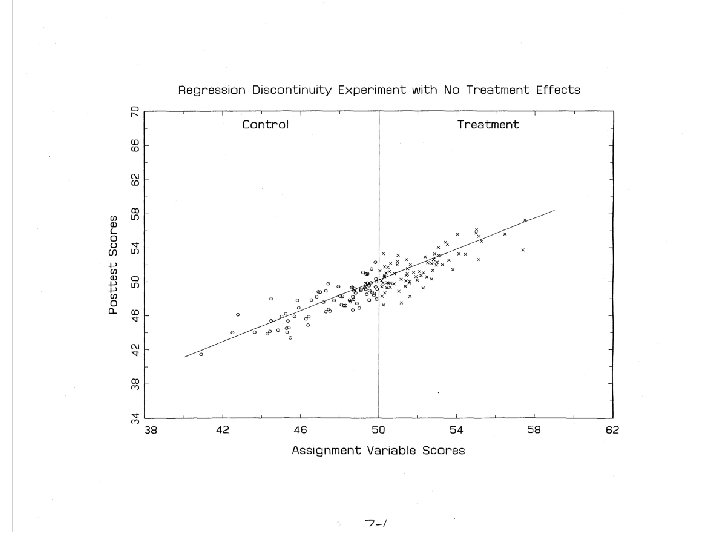
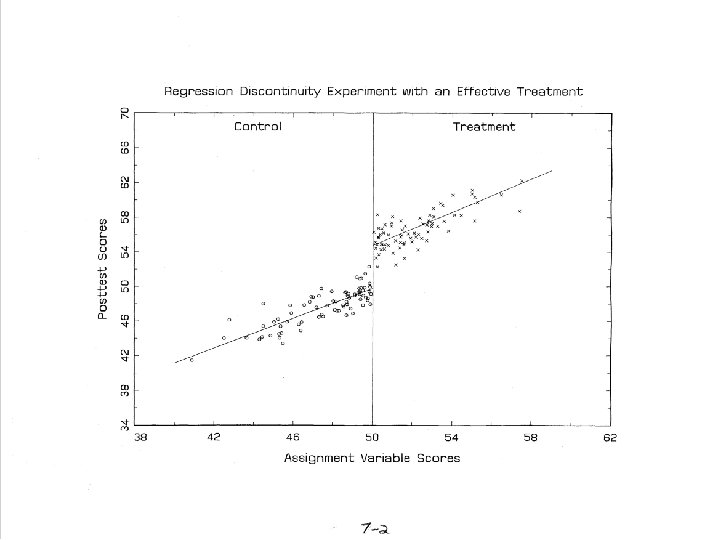
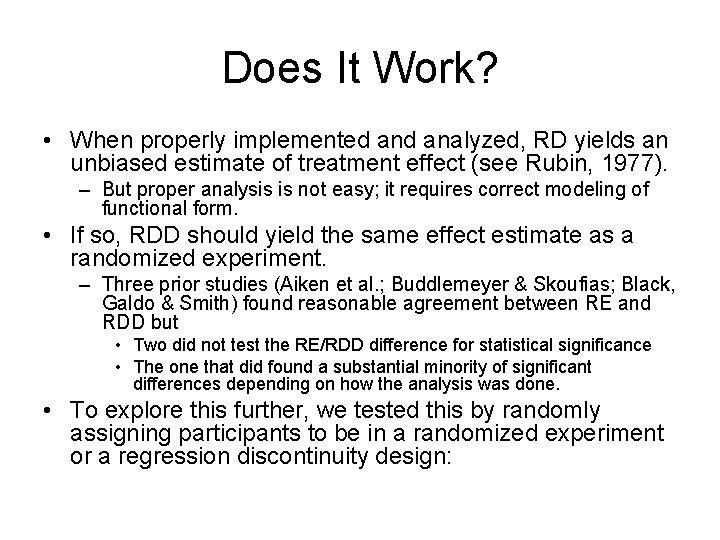
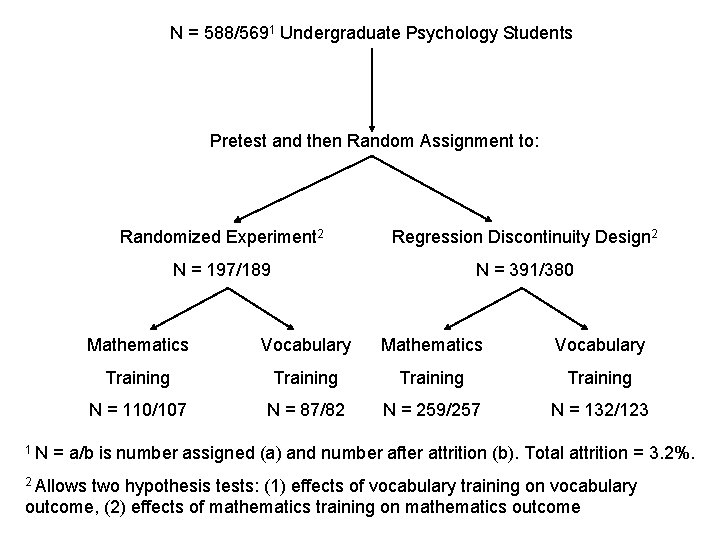
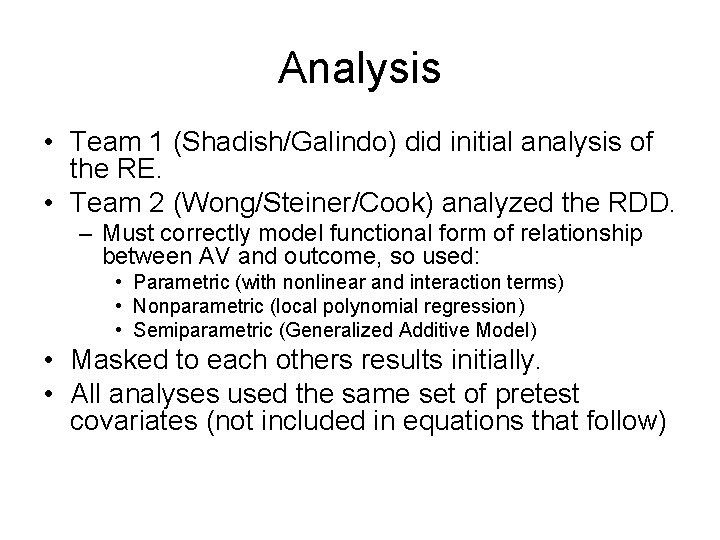
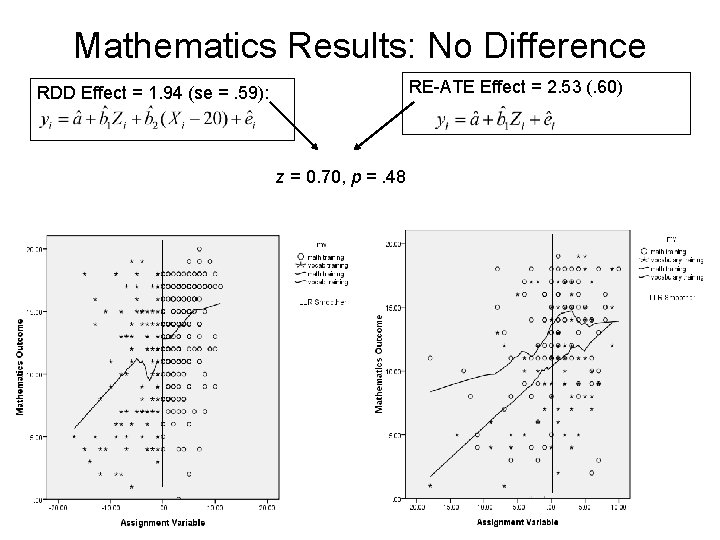
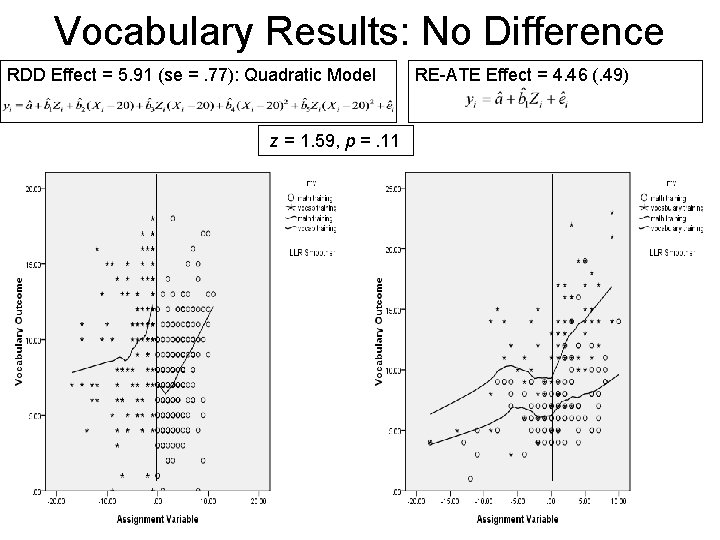
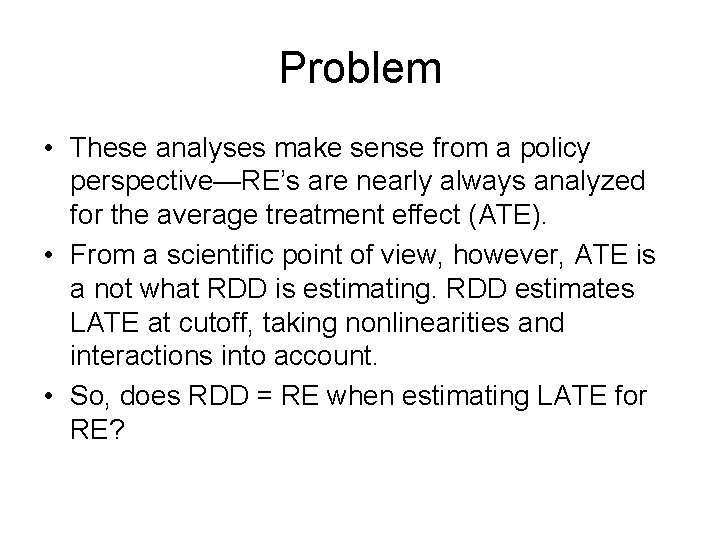
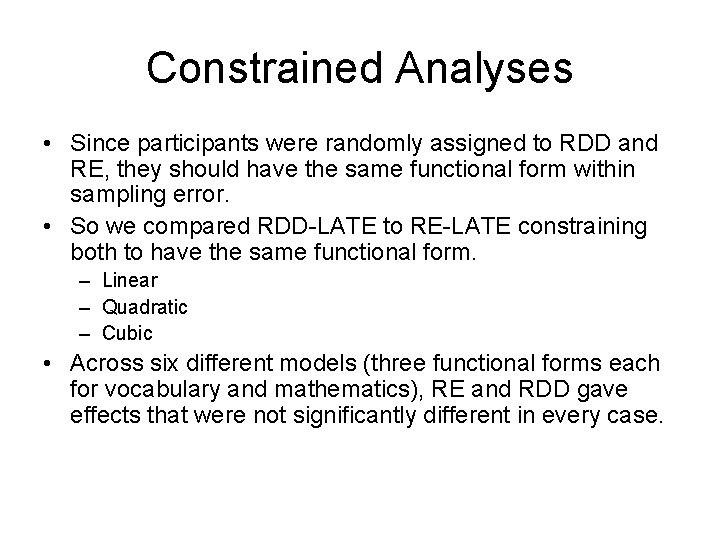
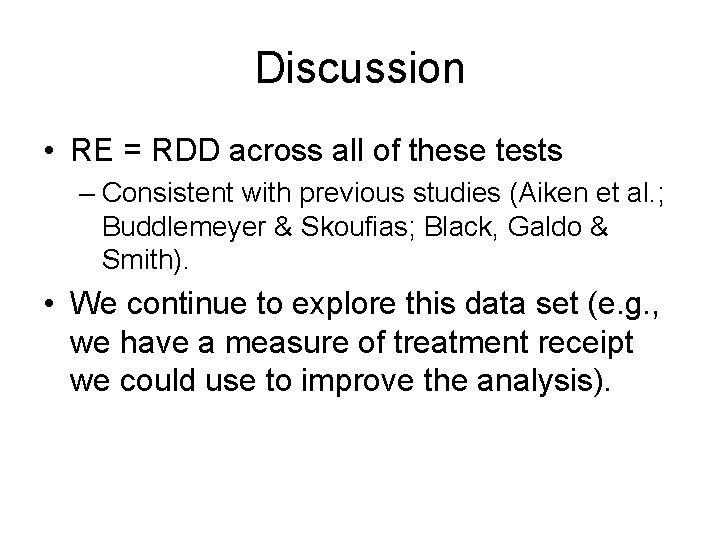
- Slides: 12
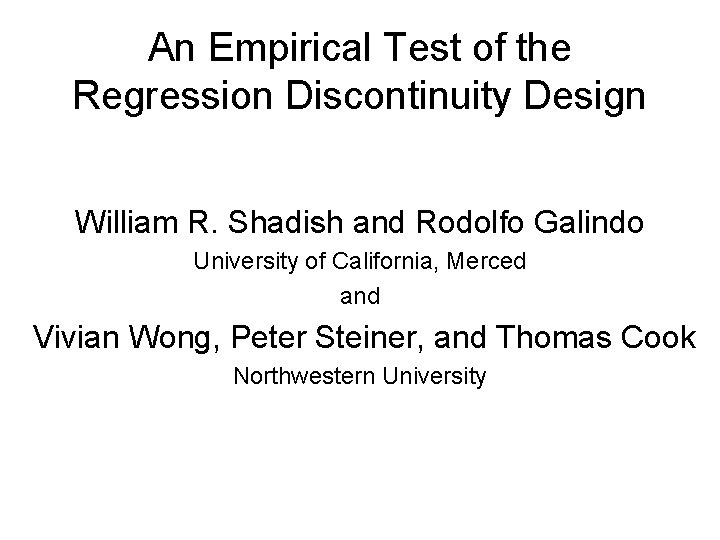
An Empirical Test of the Regression Discontinuity Design William R. Shadish and Rodolfo Galindo University of California, Merced and Vivian Wong, Peter Steiner, and Thomas Cook Northwestern University
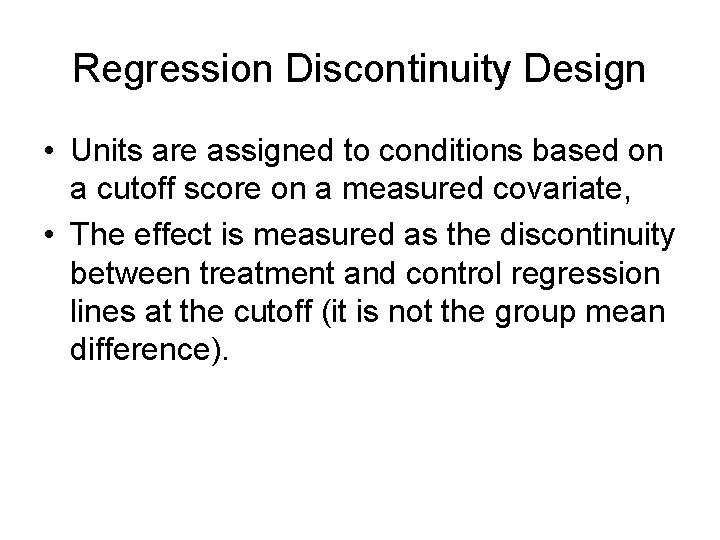
Regression Discontinuity Design • Units are assigned to conditions based on a cutoff score on a measured covariate, • The effect is measured as the discontinuity between treatment and control regression lines at the cutoff (it is not the group mean difference).
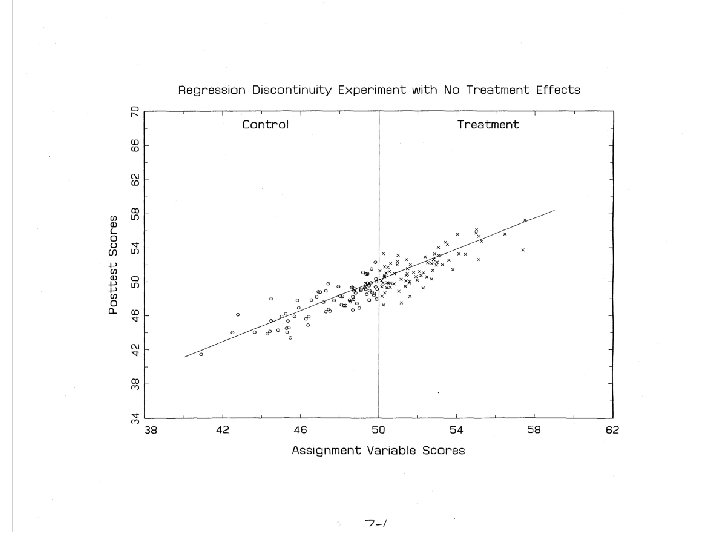
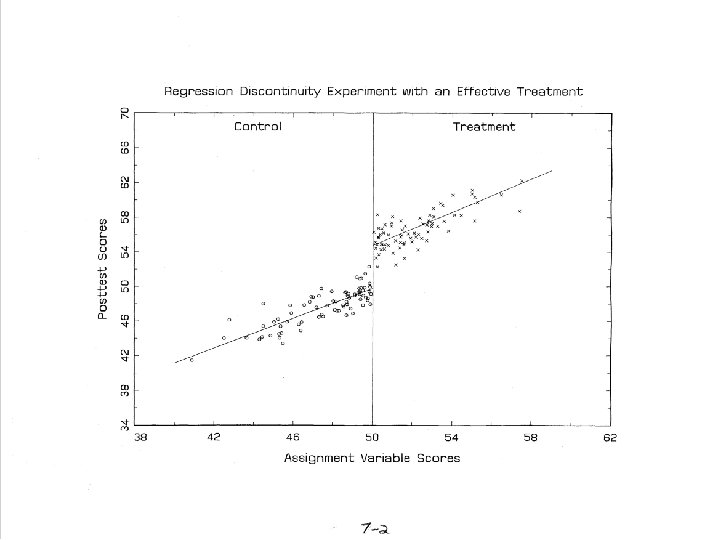
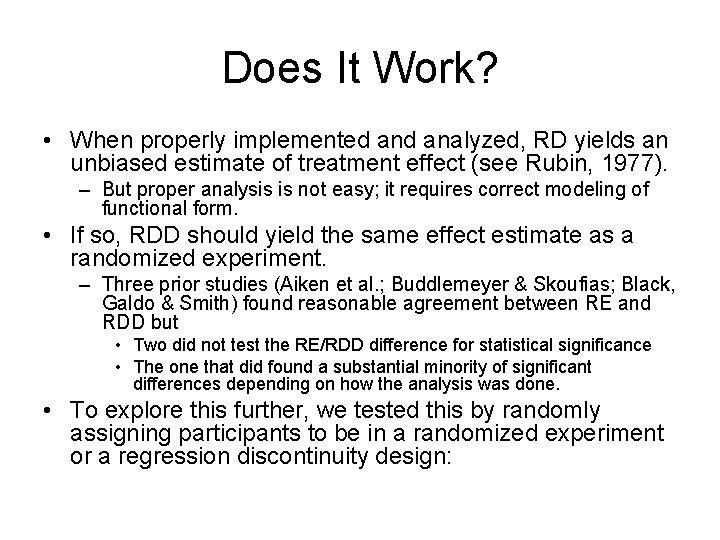
Does It Work? • When properly implemented analyzed, RD yields an unbiased estimate of treatment effect (see Rubin, 1977). – But proper analysis is not easy; it requires correct modeling of functional form. • If so, RDD should yield the same effect estimate as a randomized experiment. – Three prior studies (Aiken et al. ; Buddlemeyer & Skoufias; Black, Galdo & Smith) found reasonable agreement between RE and RDD but • Two did not test the RE/RDD difference for statistical significance • The one that did found a substantial minority of significant differences depending on how the analysis was done. • To explore this further, we tested this by randomly assigning participants to be in a randomized experiment or a regression discontinuity design:
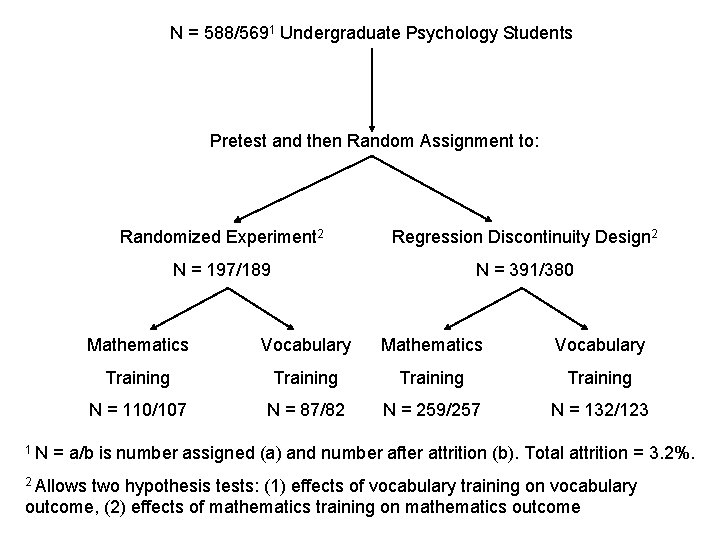
N = 588/5691 Undergraduate Psychology Students Pretest and then Random Assignment to: 1 N Randomized Experiment 2 Regression Discontinuity Design 2 N = 197/189 N = 391/380 Mathematics Vocabulary Training N = 110/107 N = 87/82 N = 259/257 N = 132/123 = a/b is number assigned (a) and number after attrition (b). Total attrition = 3. 2%. 2 Allows two hypothesis tests: (1) effects of vocabulary training on vocabulary outcome, (2) effects of mathematics training on mathematics outcome
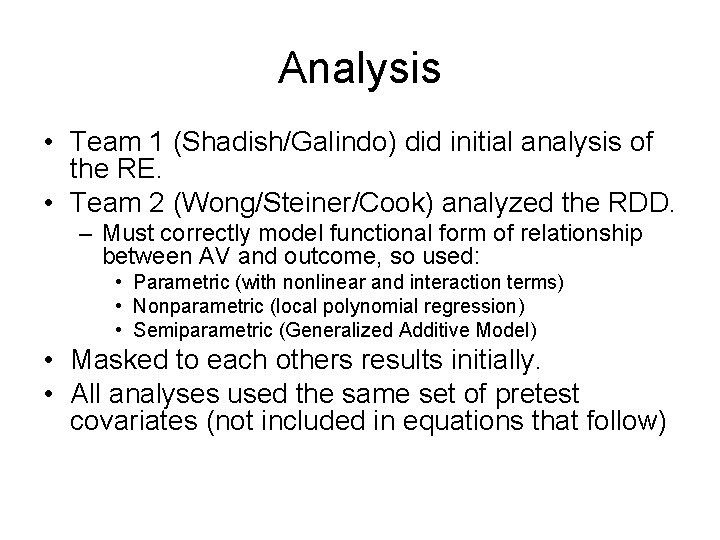
Analysis • Team 1 (Shadish/Galindo) did initial analysis of the RE. • Team 2 (Wong/Steiner/Cook) analyzed the RDD. – Must correctly model functional form of relationship between AV and outcome, so used: • Parametric (with nonlinear and interaction terms) • Nonparametric (local polynomial regression) • Semiparametric (Generalized Additive Model) • Masked to each others results initially. • All analyses used the same set of pretest covariates (not included in equations that follow)
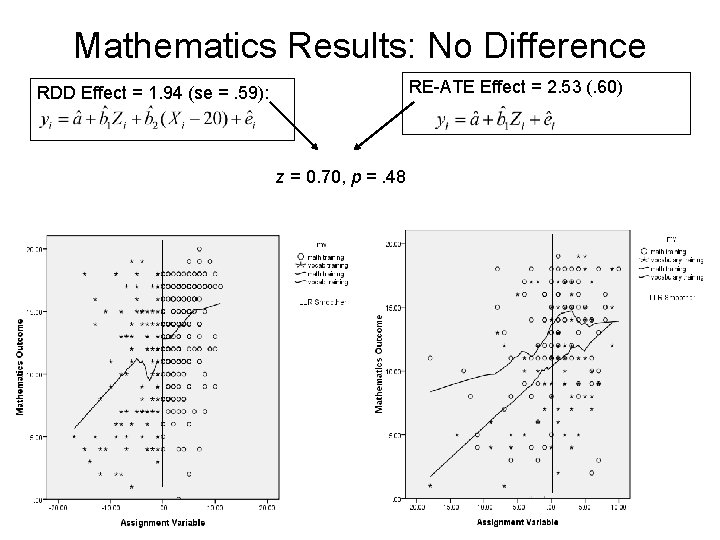
Mathematics Results: No Difference RE-ATE Effect = 2. 53 (. 60) RDD Effect = 1. 94 (se =. 59): z = 0. 70, p =. 48
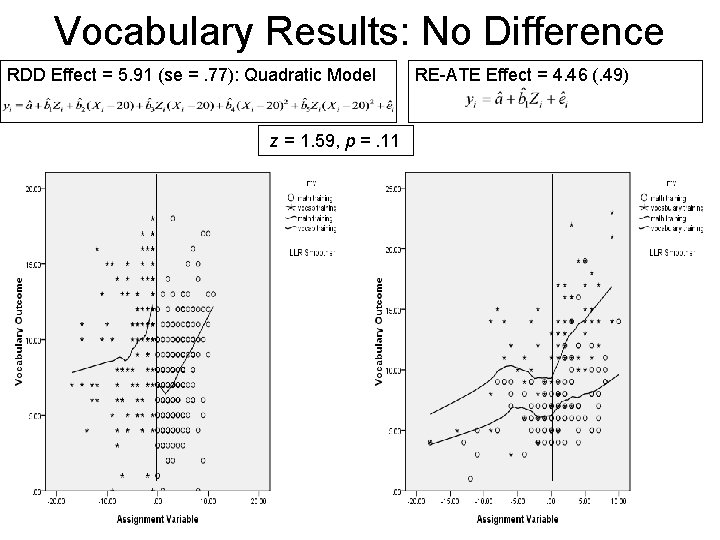
Vocabulary Results: No Difference RDD Effect = 5. 91 (se =. 77): Quadratic Model z = 1. 59, p =. 11 RE-ATE Effect = 4. 46 (. 49)
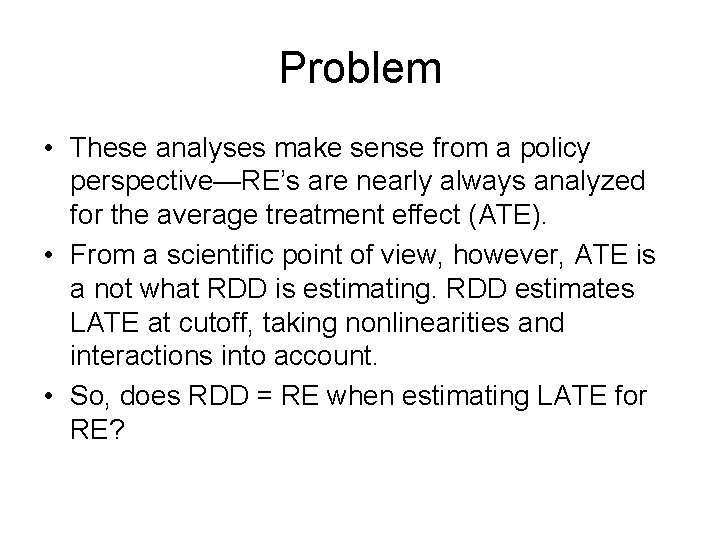
Problem • These analyses make sense from a policy perspective—RE’s are nearly always analyzed for the average treatment effect (ATE). • From a scientific point of view, however, ATE is a not what RDD is estimating. RDD estimates LATE at cutoff, taking nonlinearities and interactions into account. • So, does RDD = RE when estimating LATE for RE?
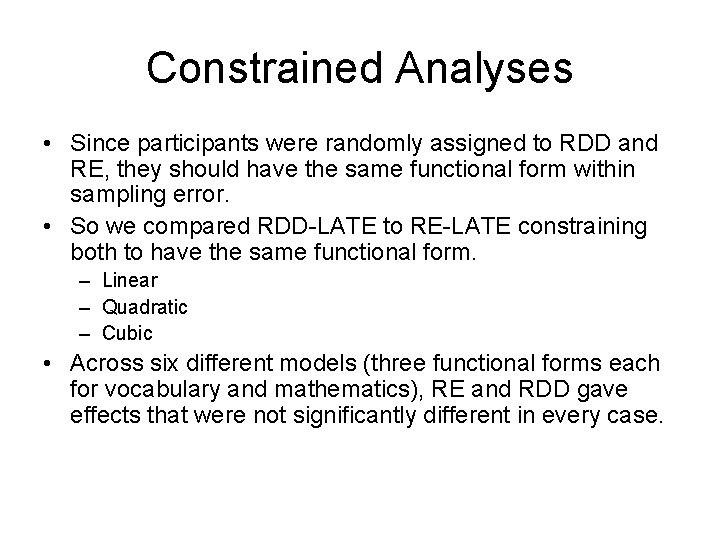
Constrained Analyses • Since participants were randomly assigned to RDD and RE, they should have the same functional form within sampling error. • So we compared RDD-LATE to RE-LATE constraining both to have the same functional form. – Linear – Quadratic – Cubic • Across six different models (three functional forms each for vocabulary and mathematics), RE and RDD gave effects that were not significantly different in every case.
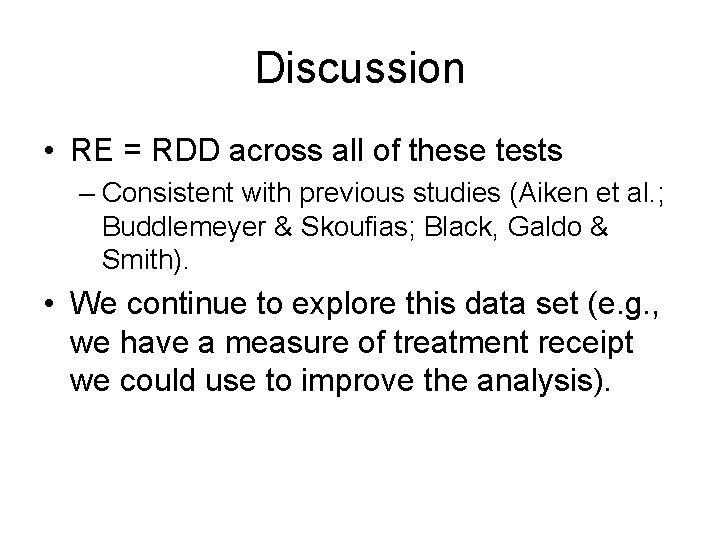
Discussion • RE = RDD across all of these tests – Consistent with previous studies (Aiken et al. ; Buddlemeyer & Skoufias; Black, Galdo & Smith). • We continue to explore this data set (e. g. , we have a measure of treatment receipt we could use to improve the analysis).
Regression discontinuity
Regression discontinuity
Interrupted time series vs regression discontinuity
Empirical bayesian kriging
Simple linear regression and multiple regression
Linear regression vs multiple regression
Logistic regression vs linear regression
Logistic regression vs linear regression
Regression analysis in quantitative research
Discontinuity of development
Technological discontinuity definition
Piaget second stage
How to find points of discontinuity