Speech and Affect in Intelligent Tutoring Dialogue Systems
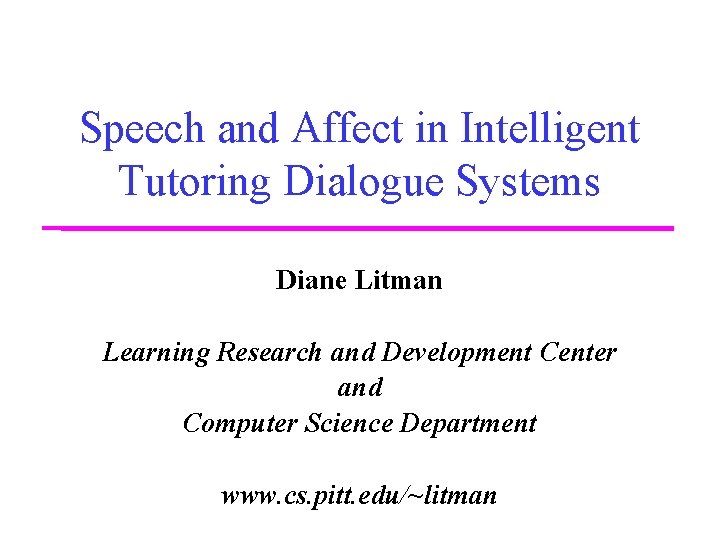
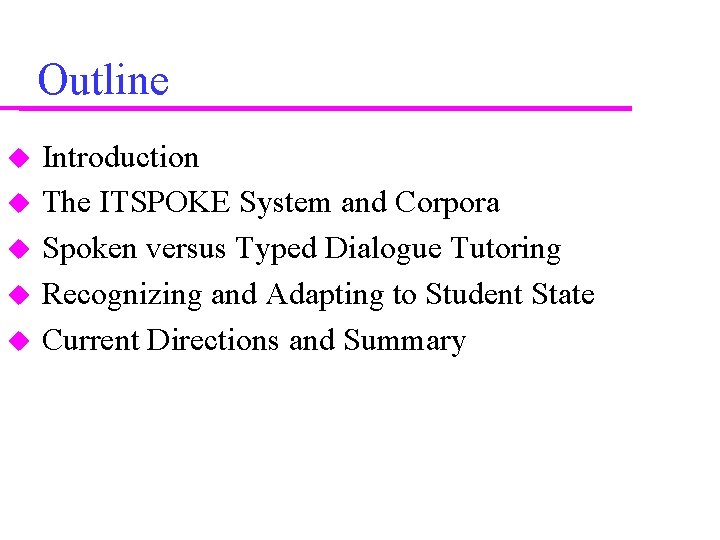
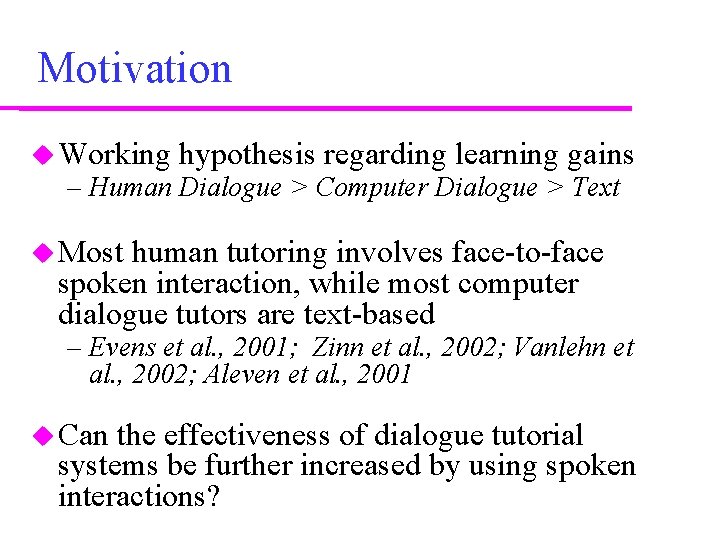
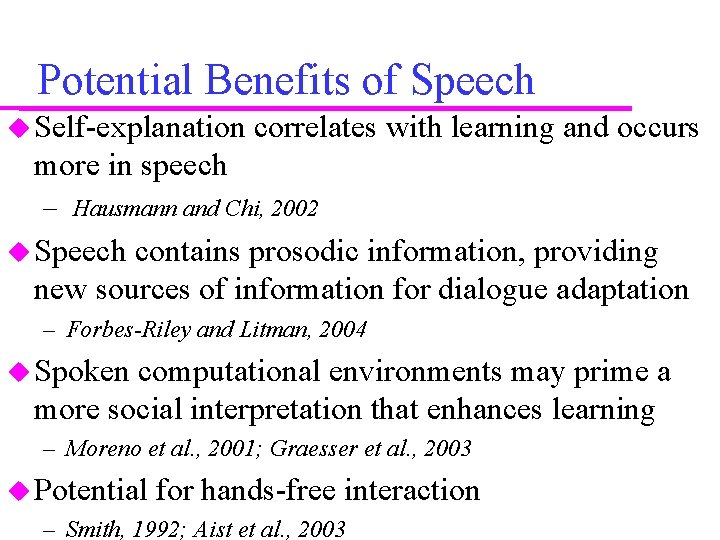
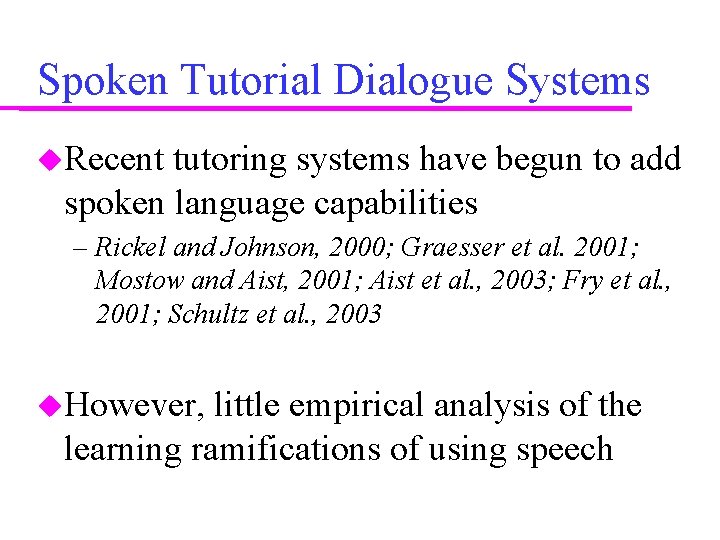
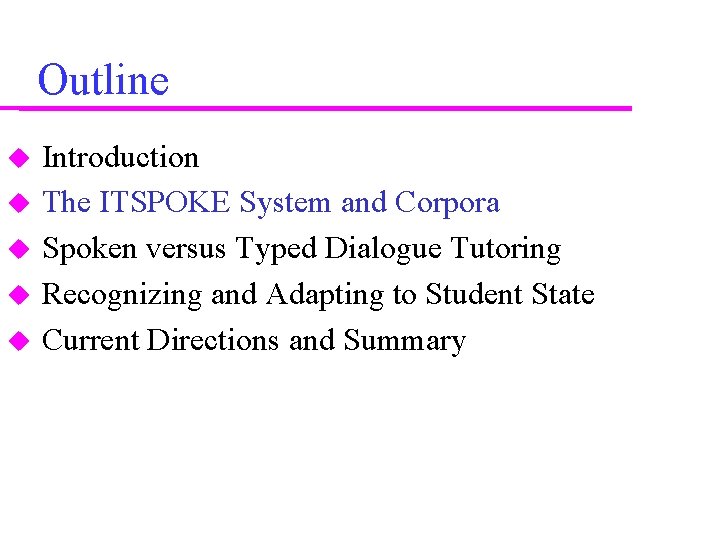
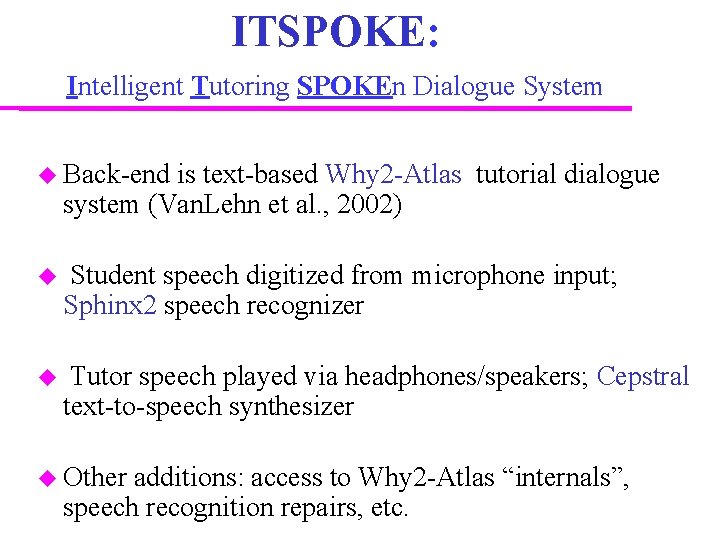
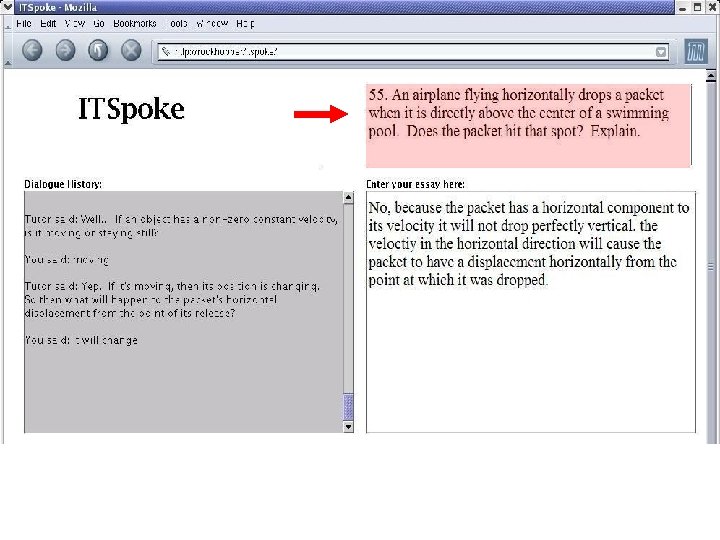
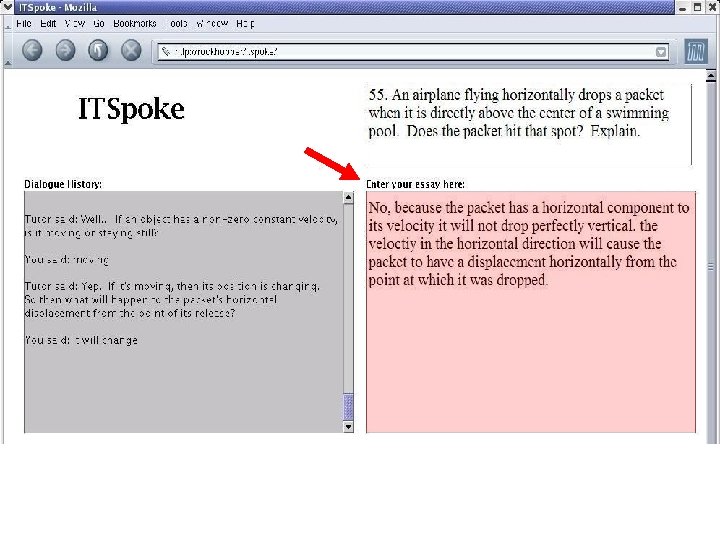
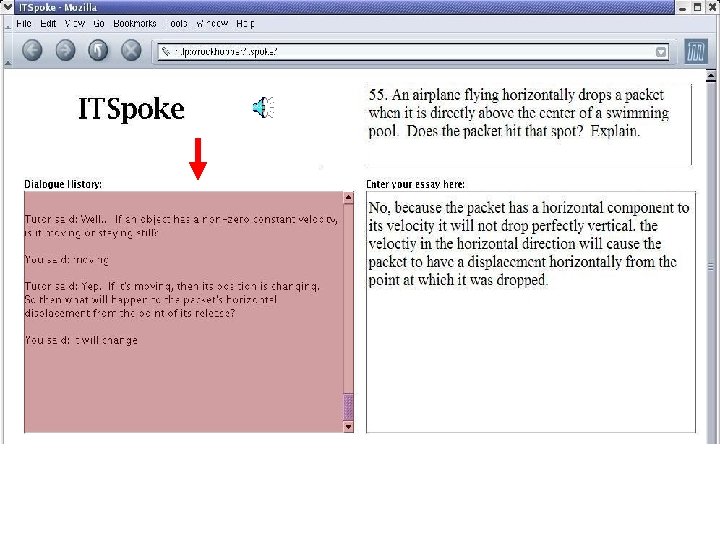
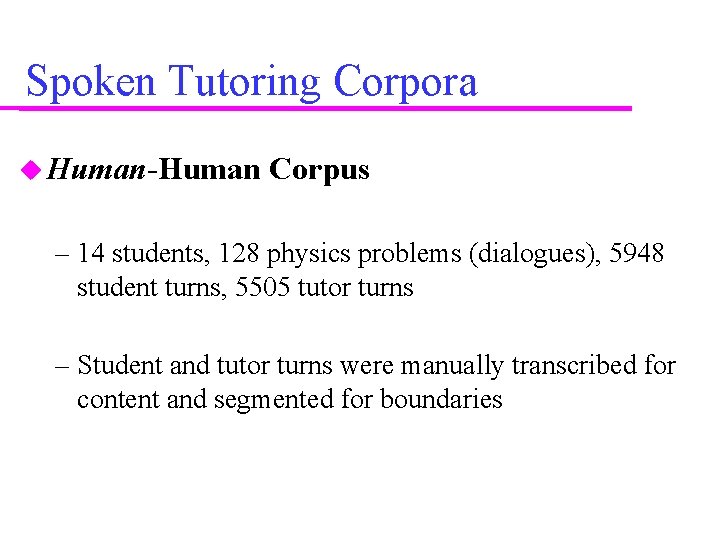
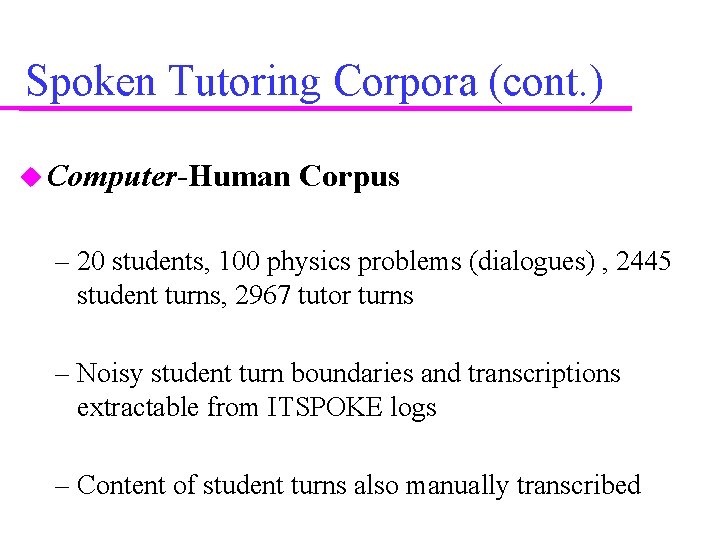
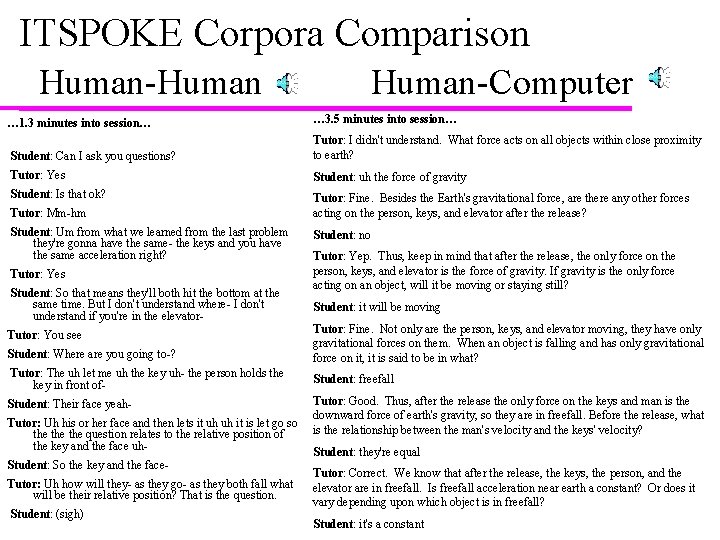
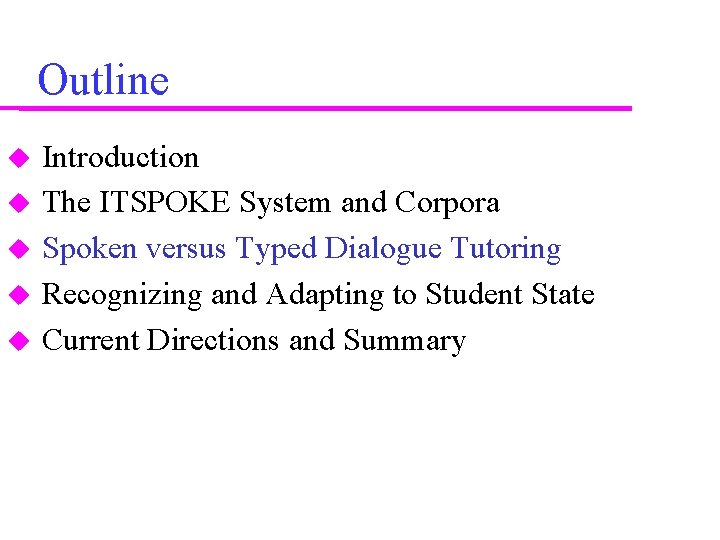
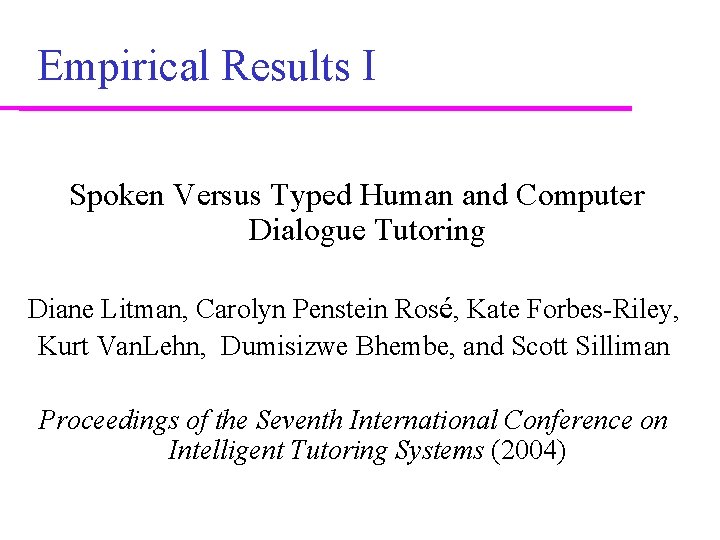
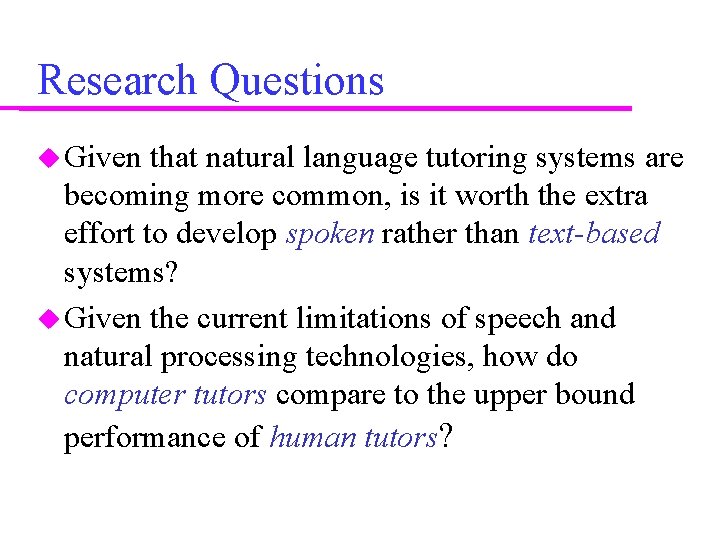
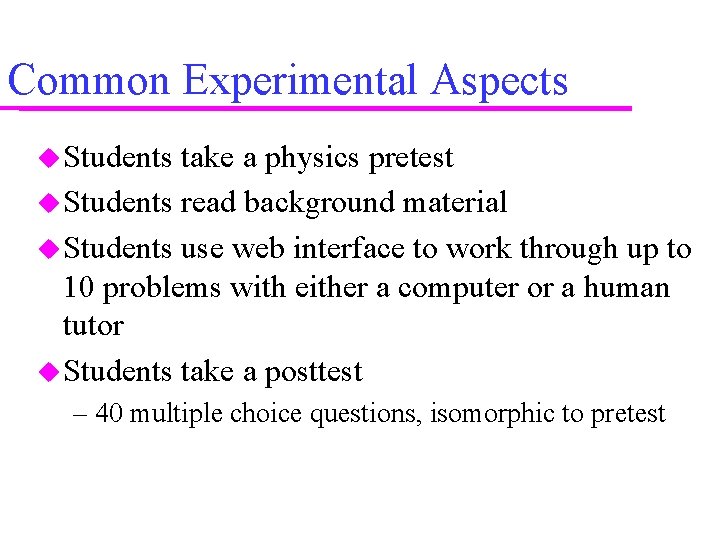
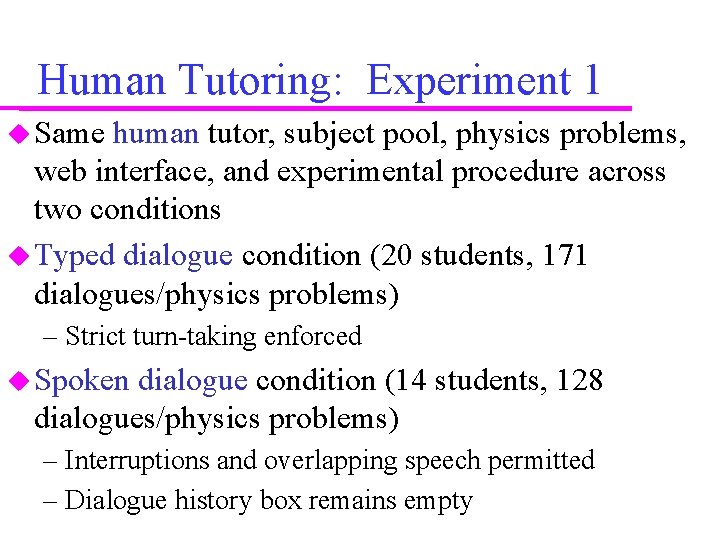
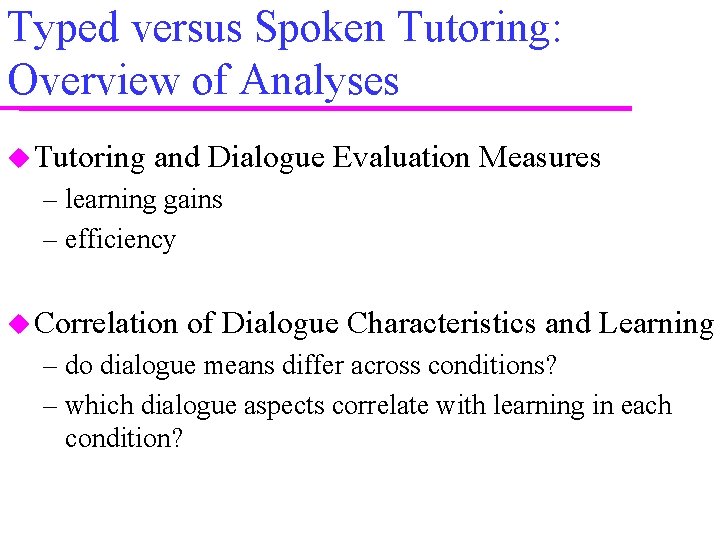
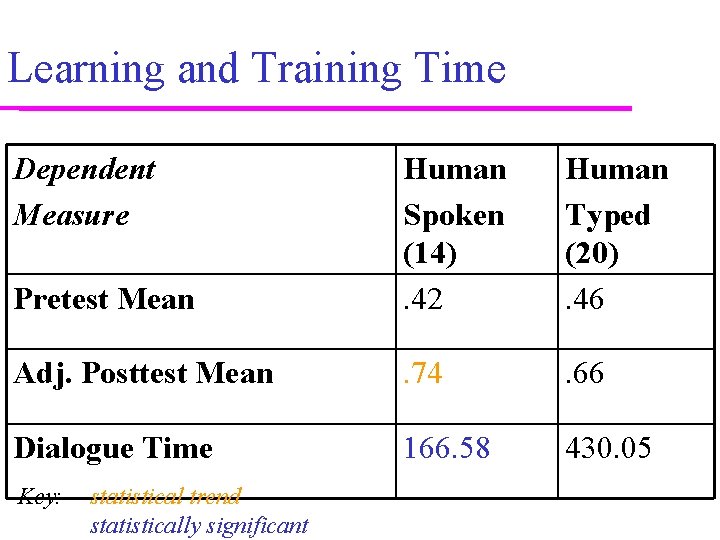
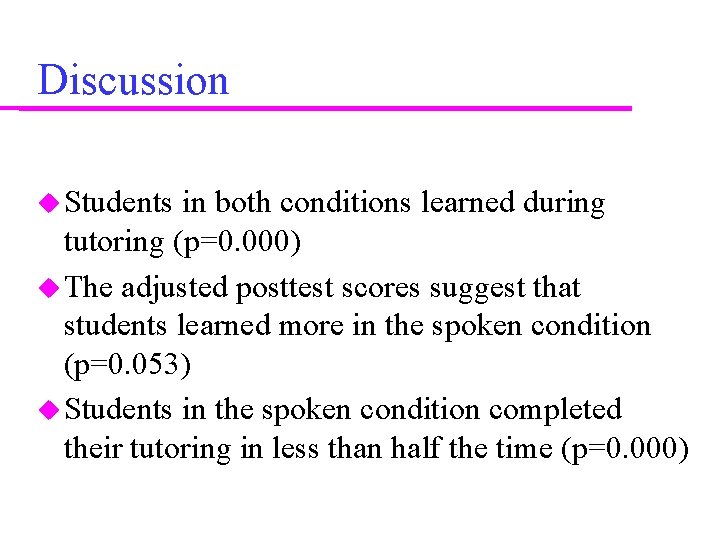
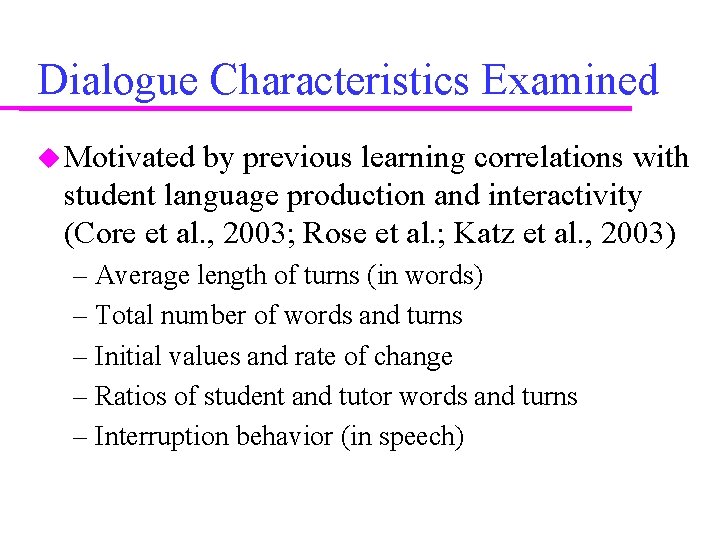
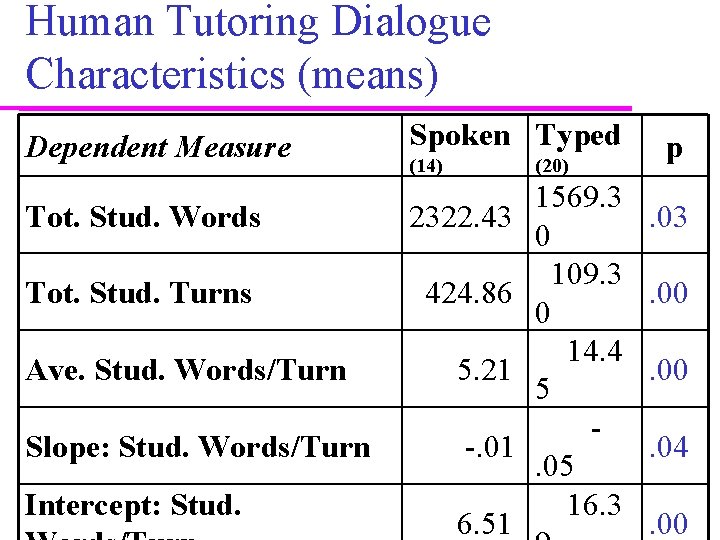
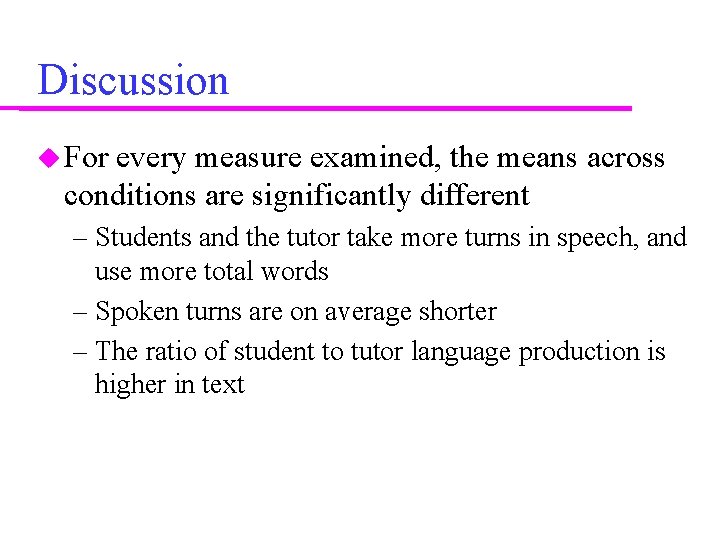
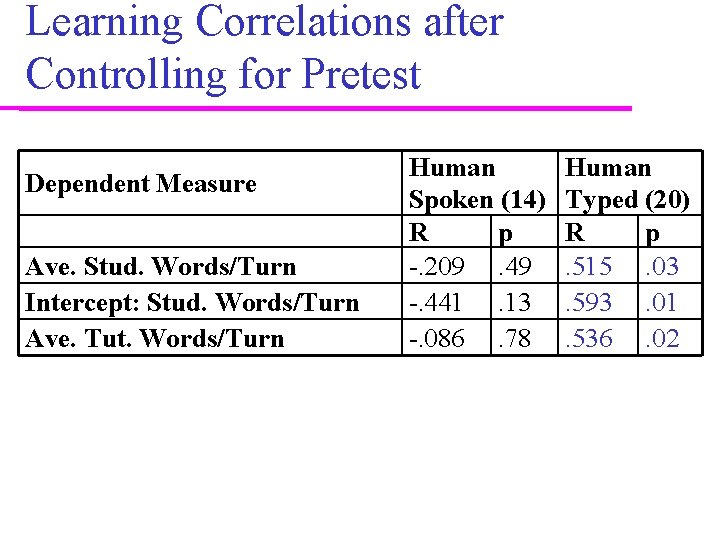
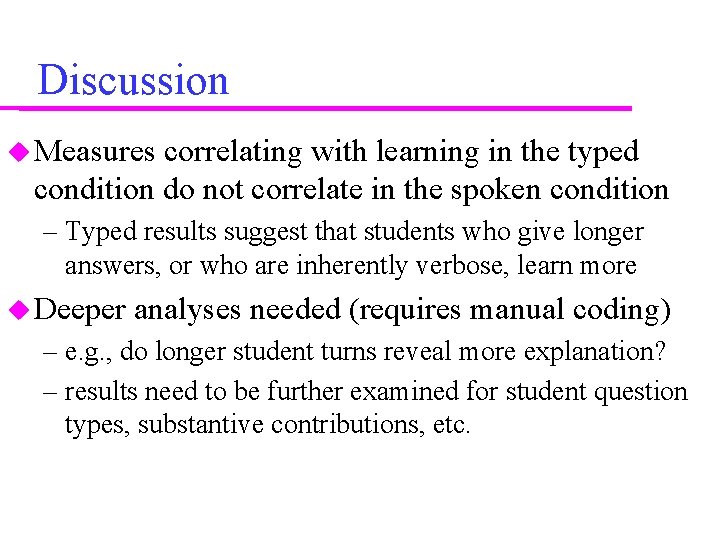
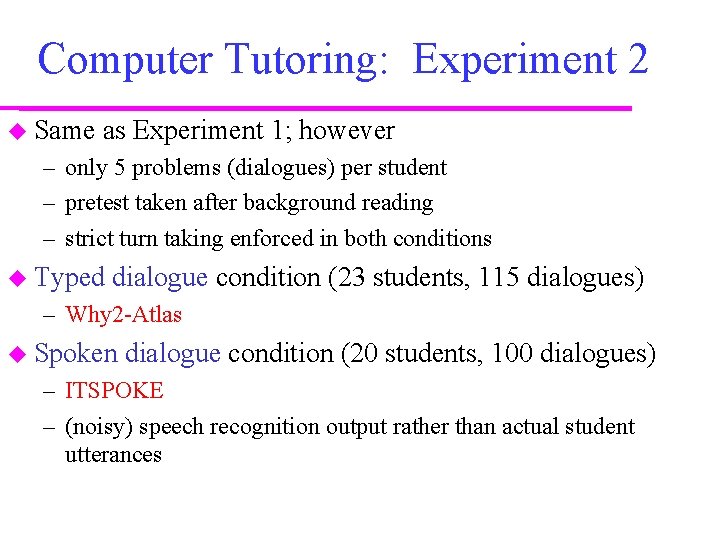
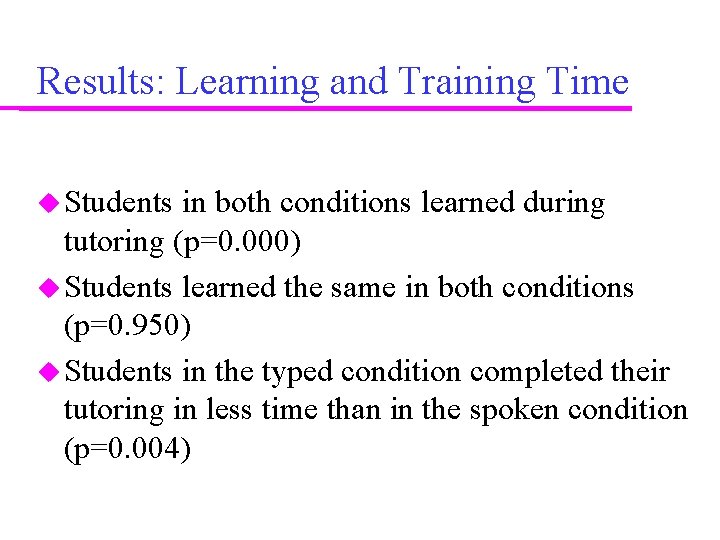
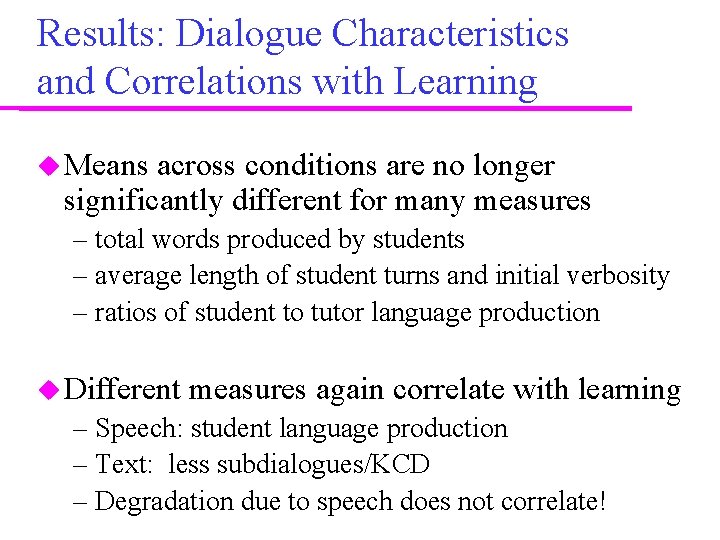
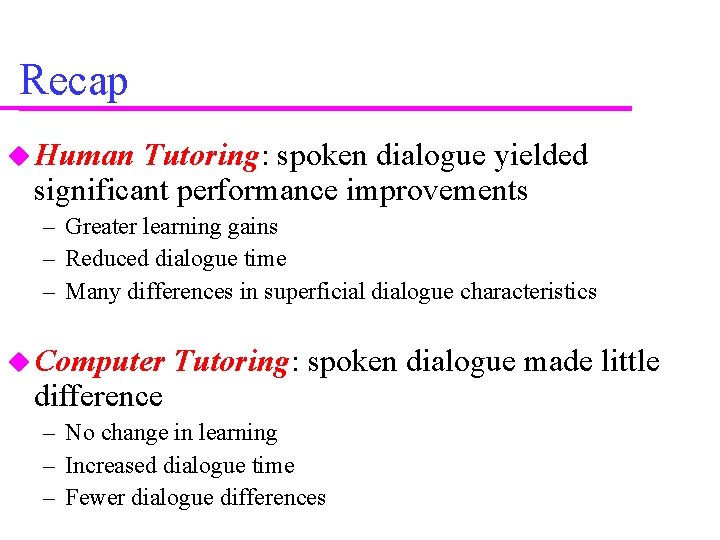
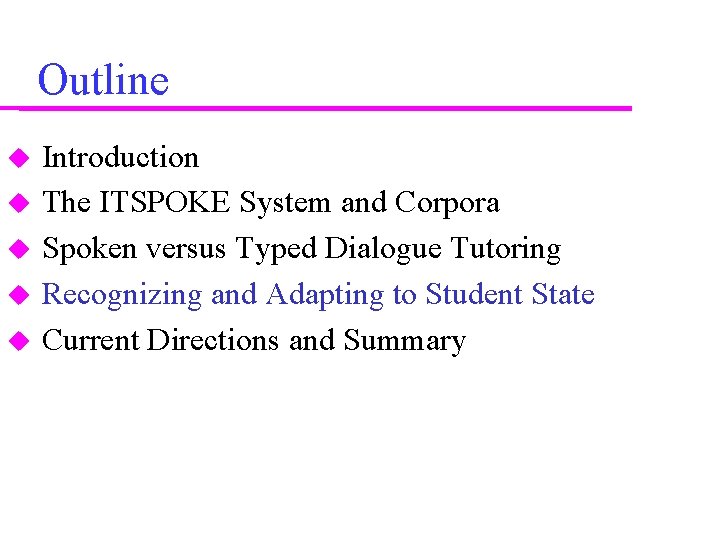
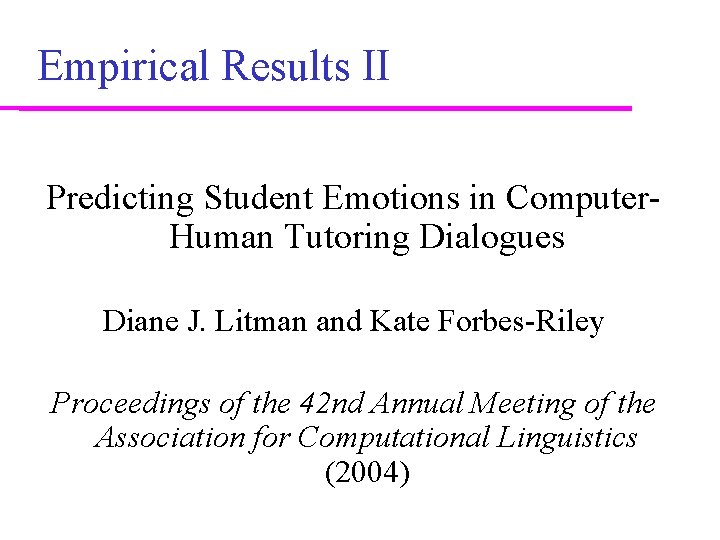
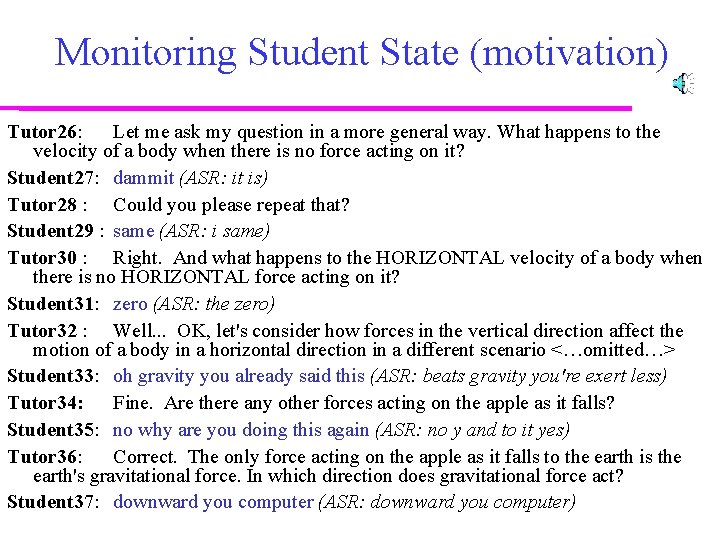
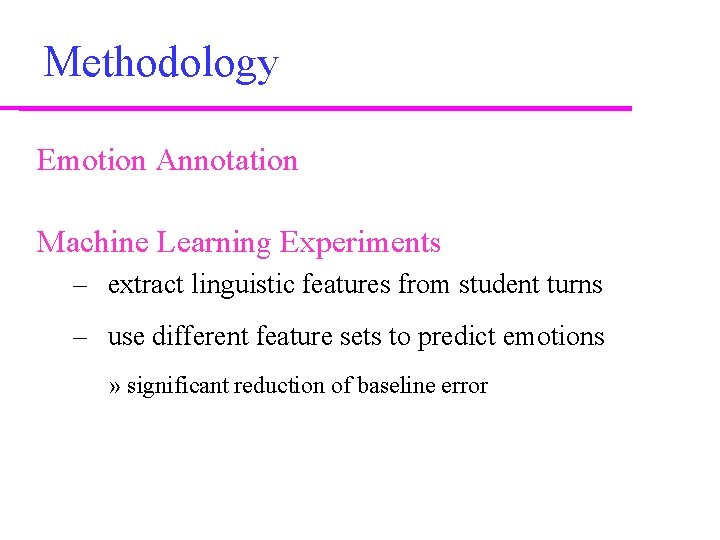
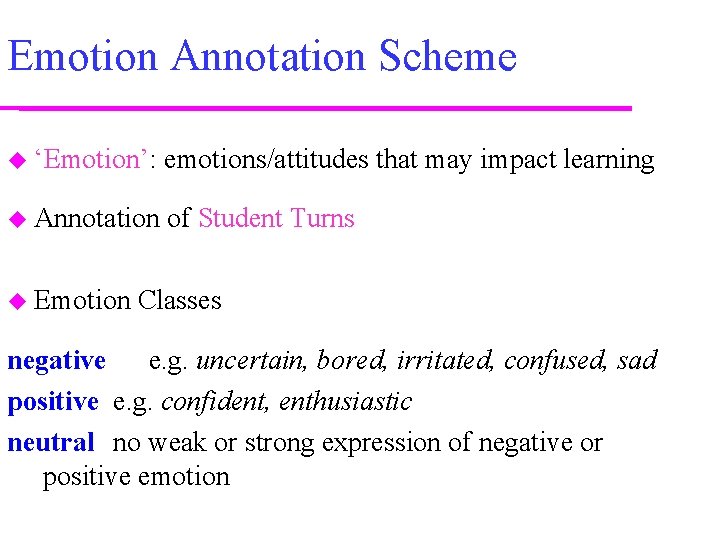
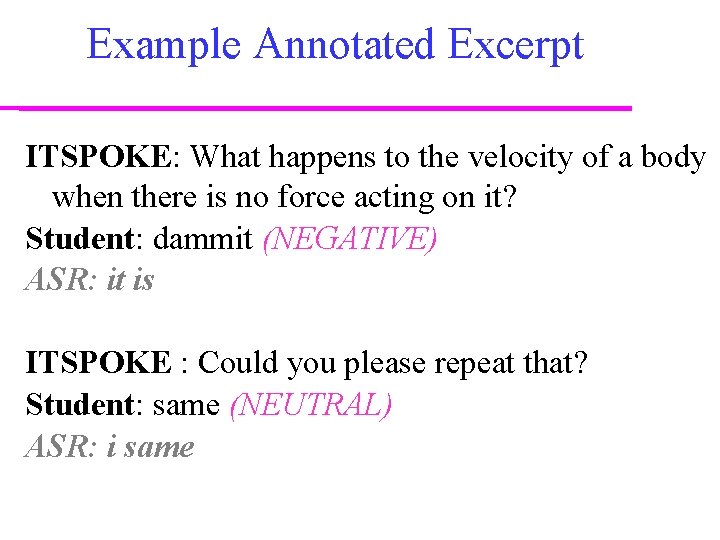
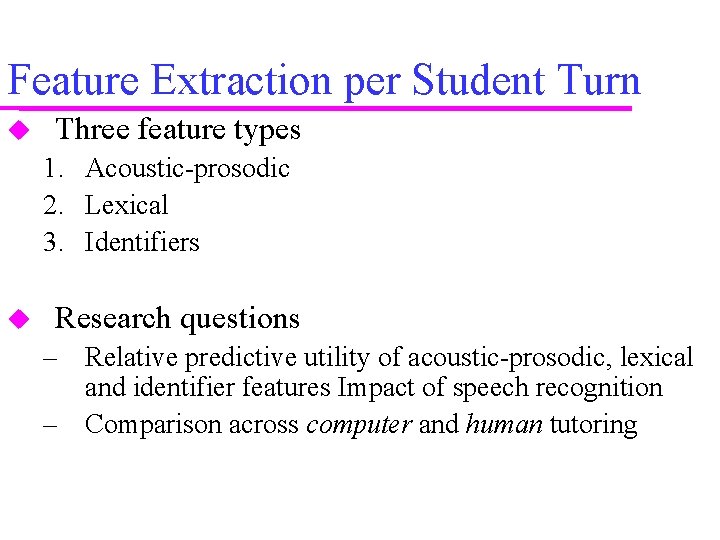
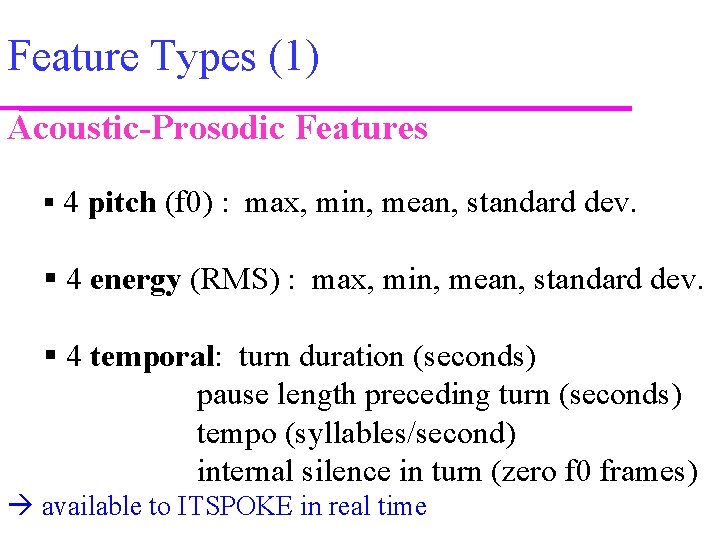
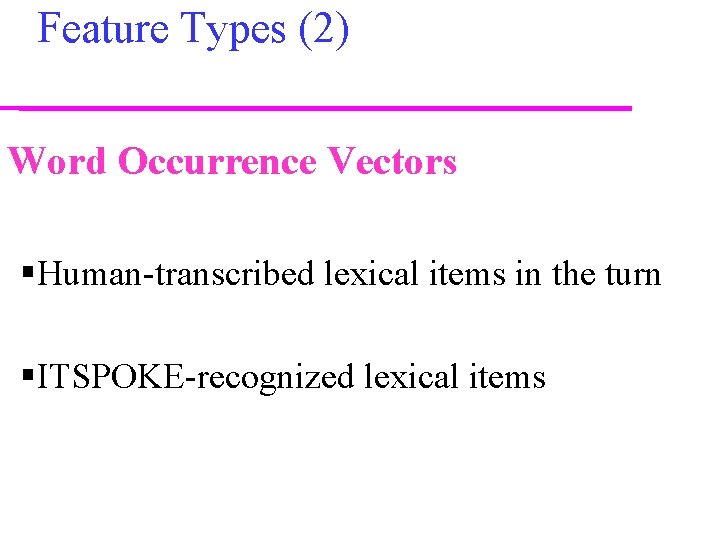
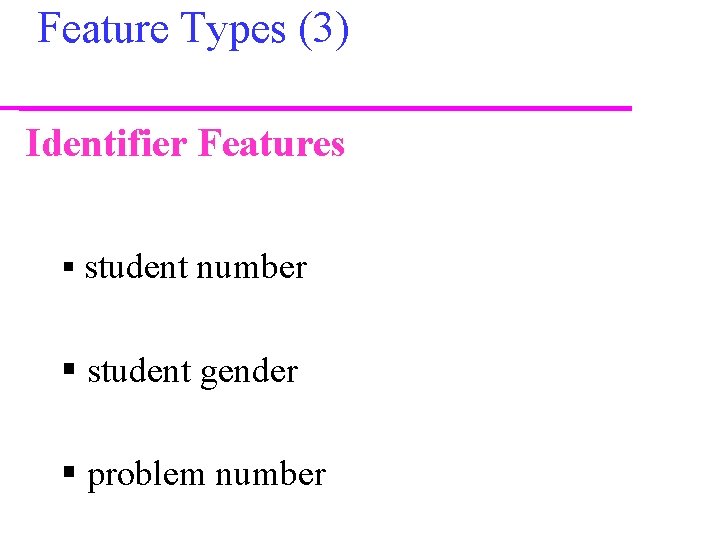
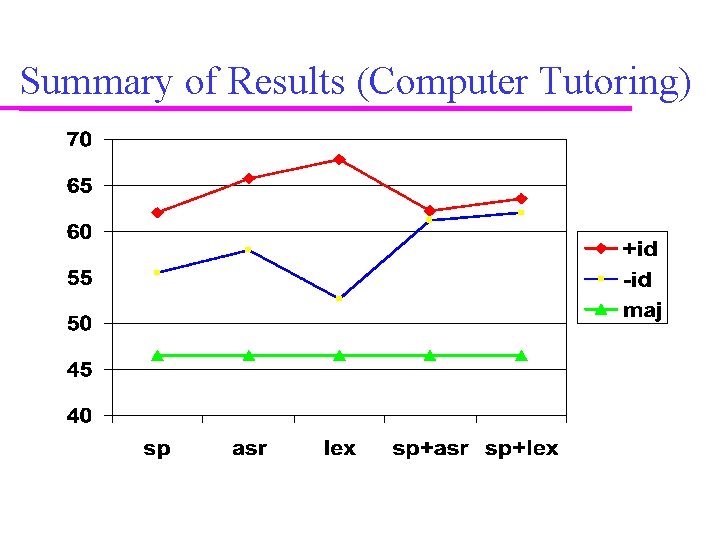
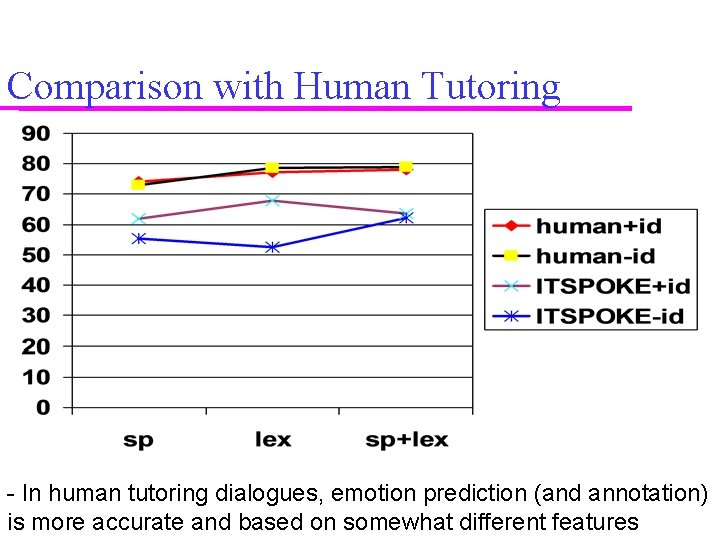
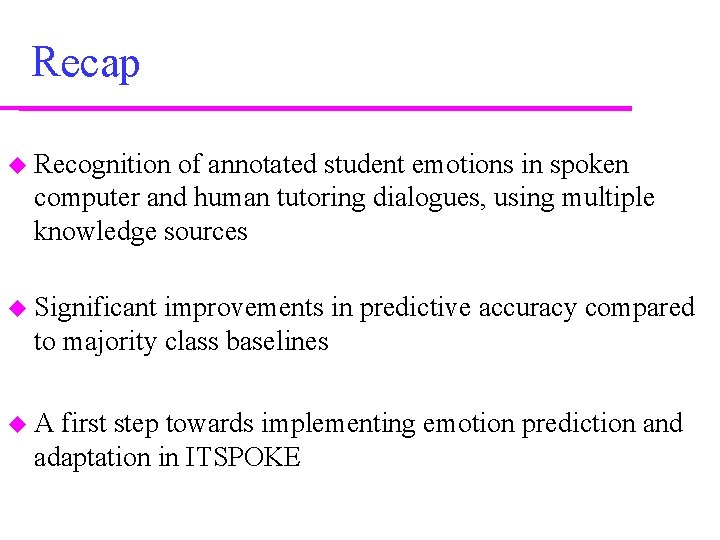
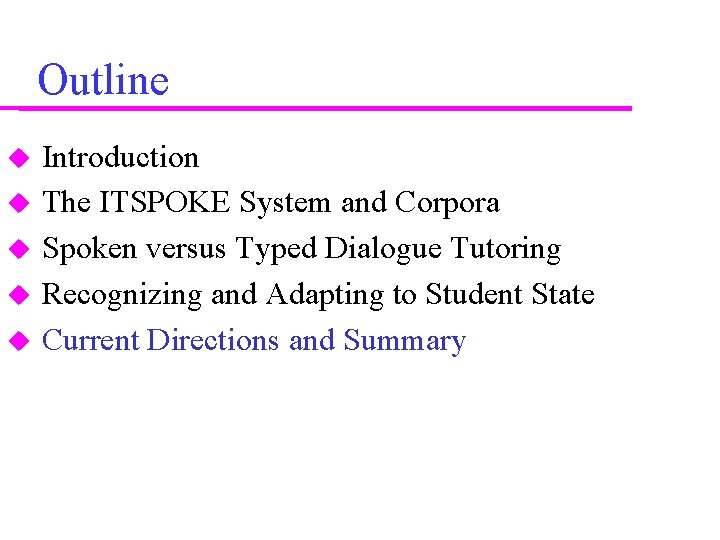
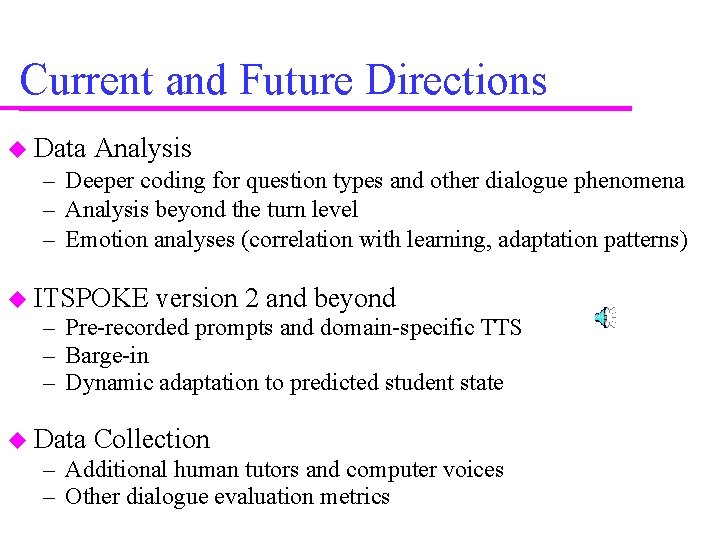
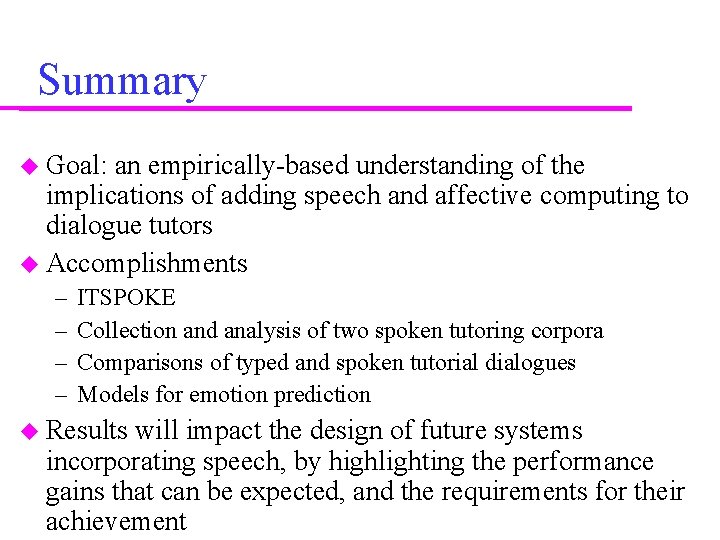
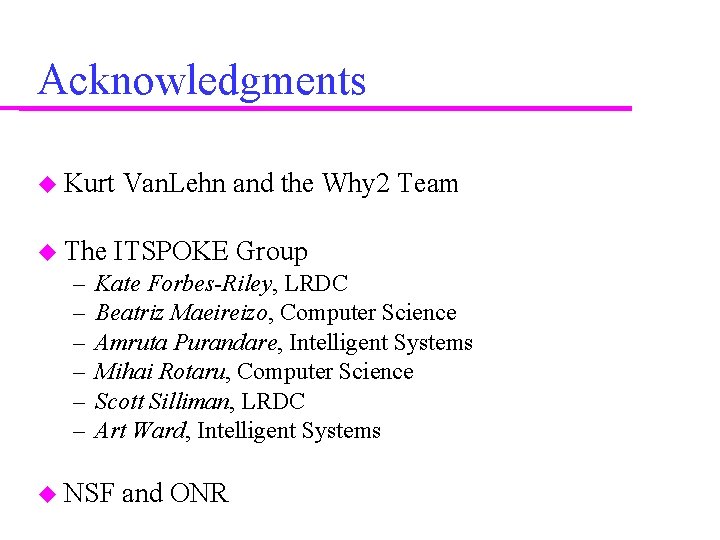
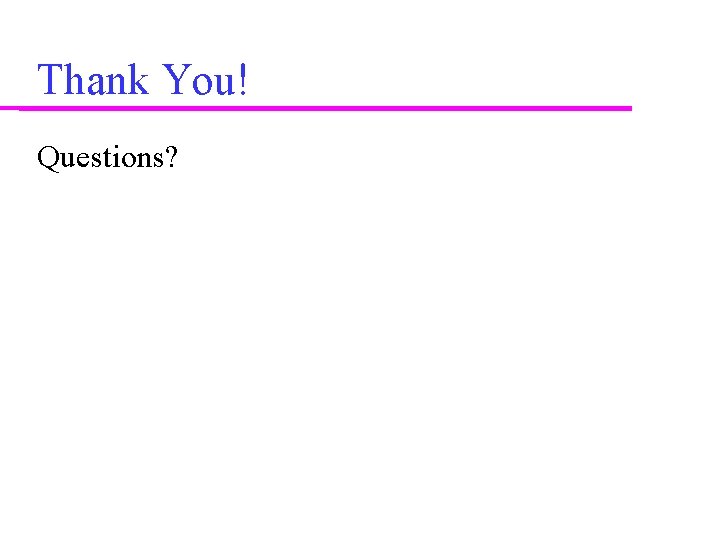
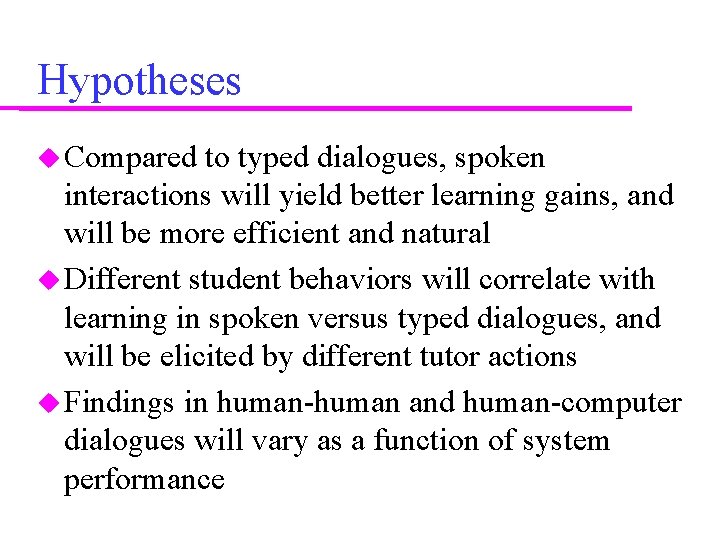
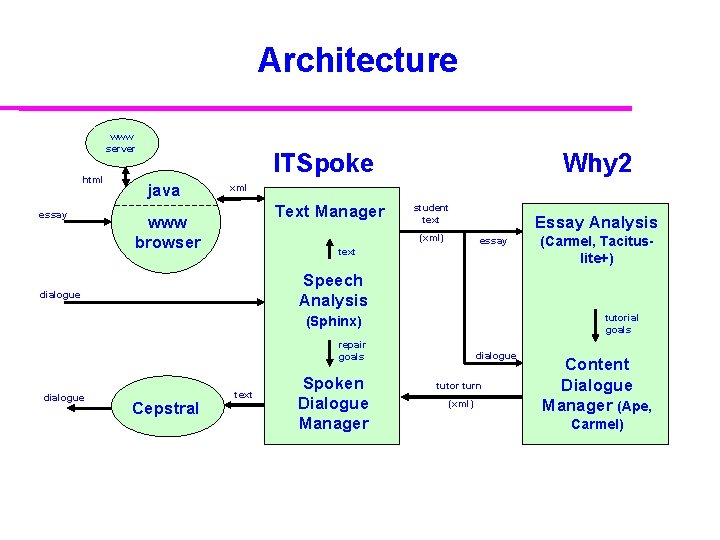
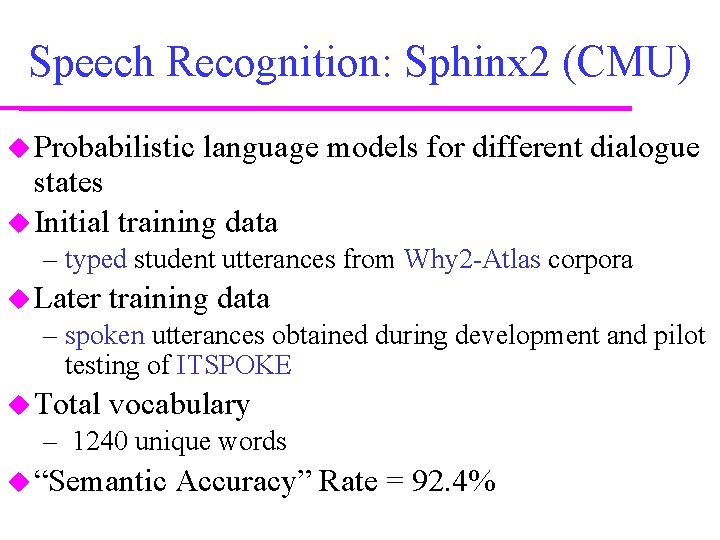
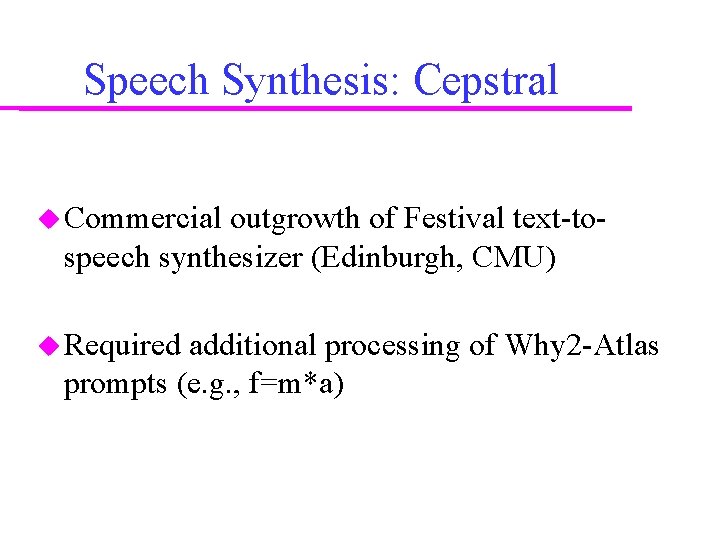
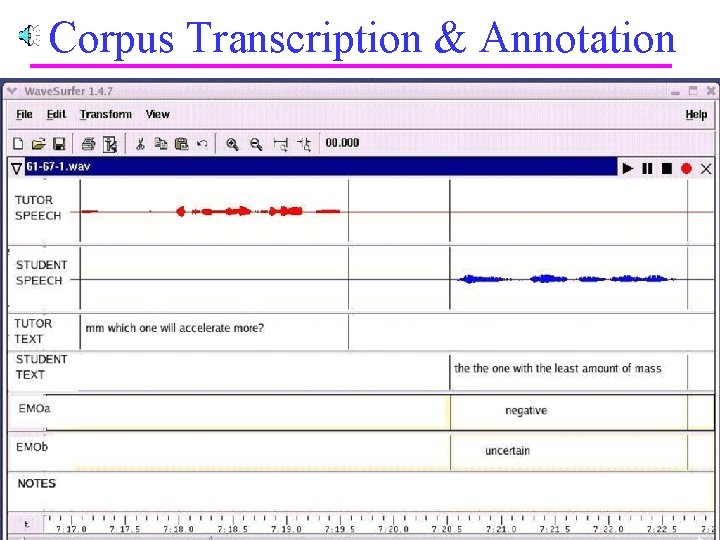
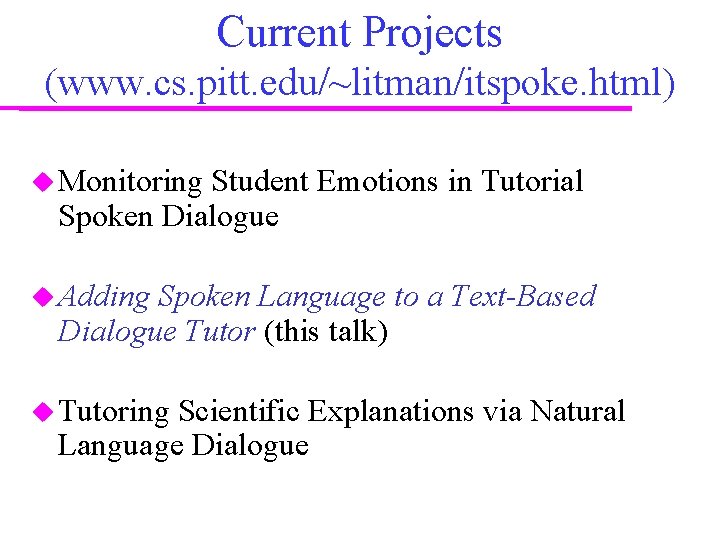
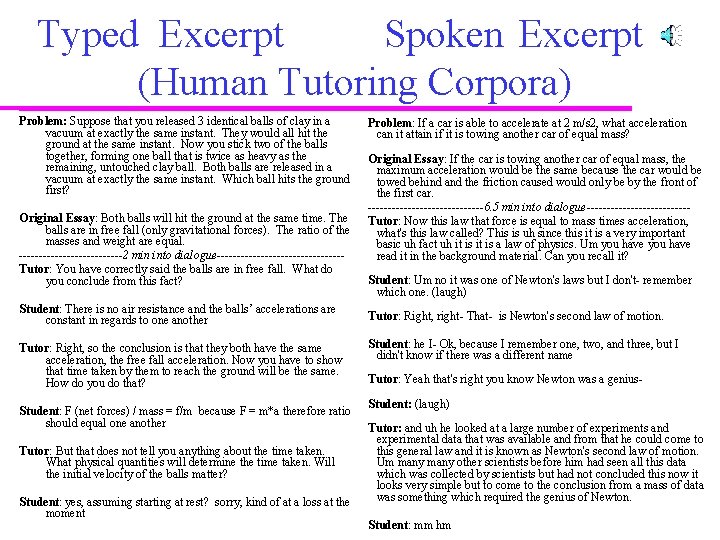
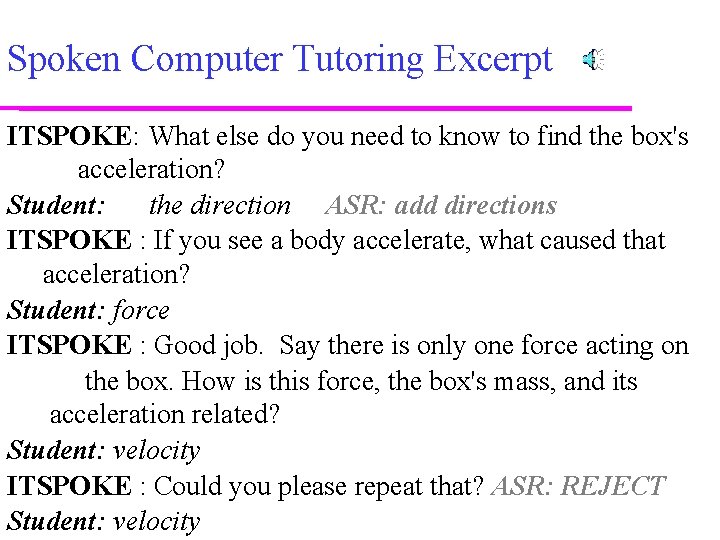
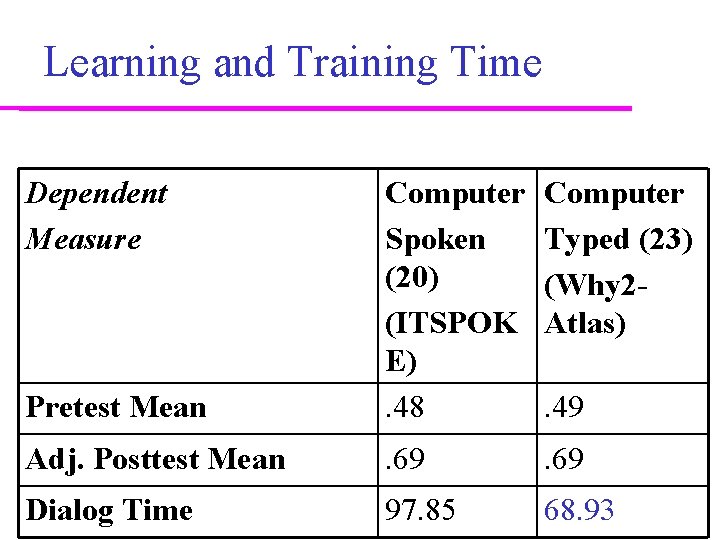
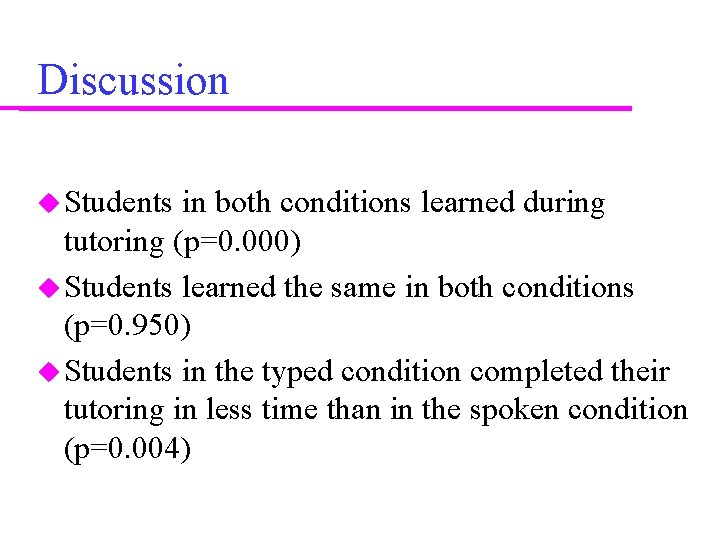
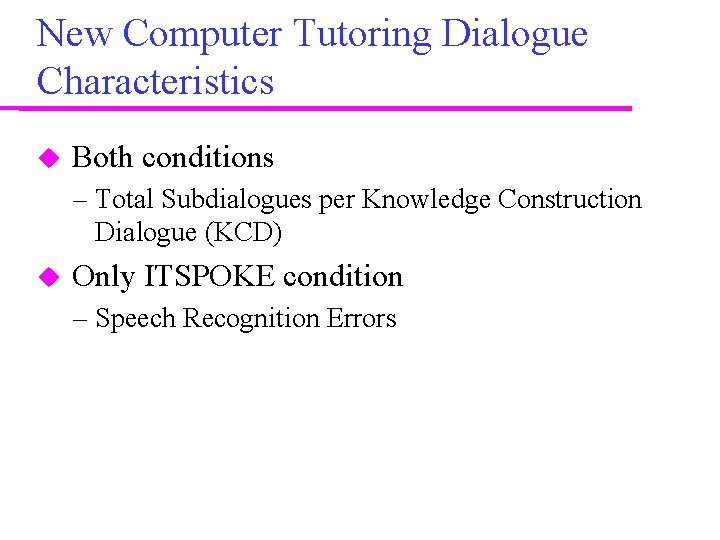
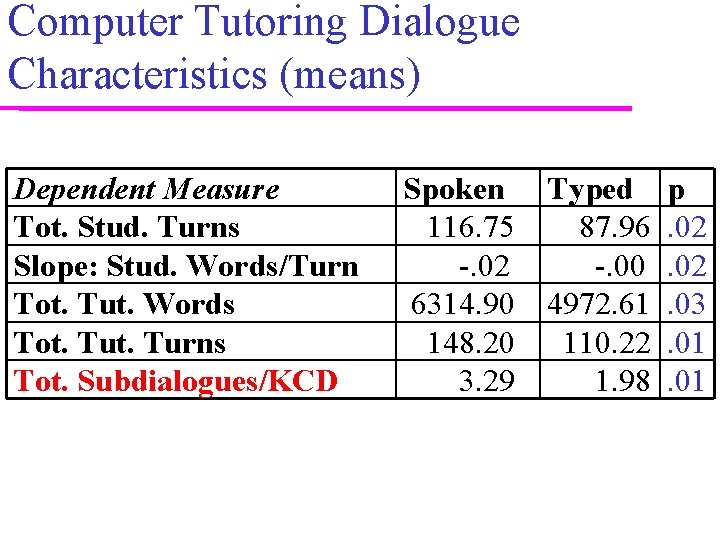
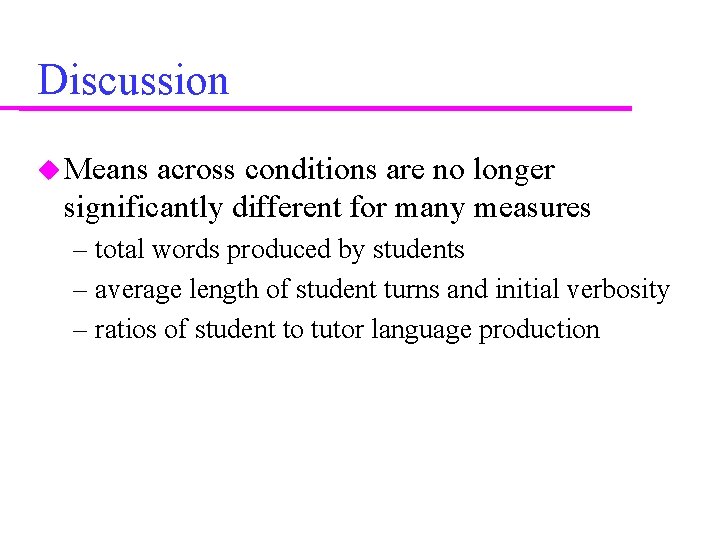
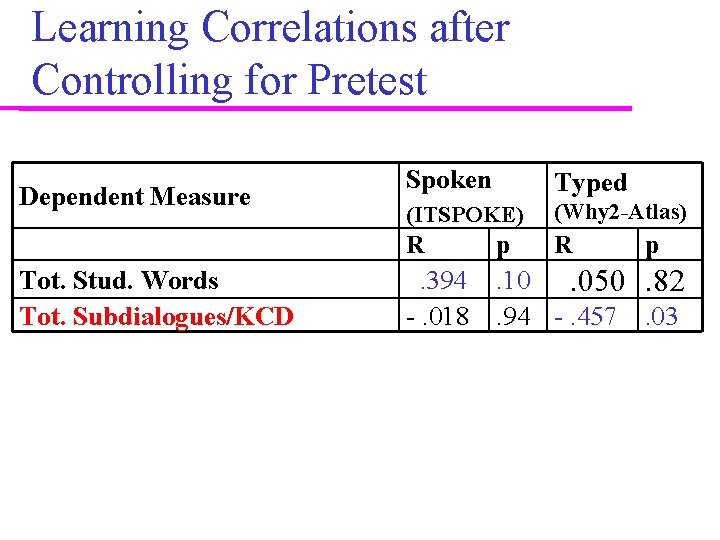
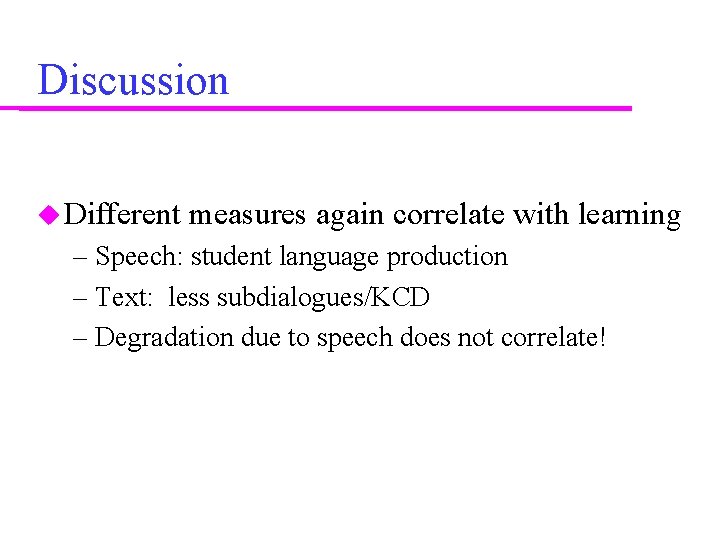
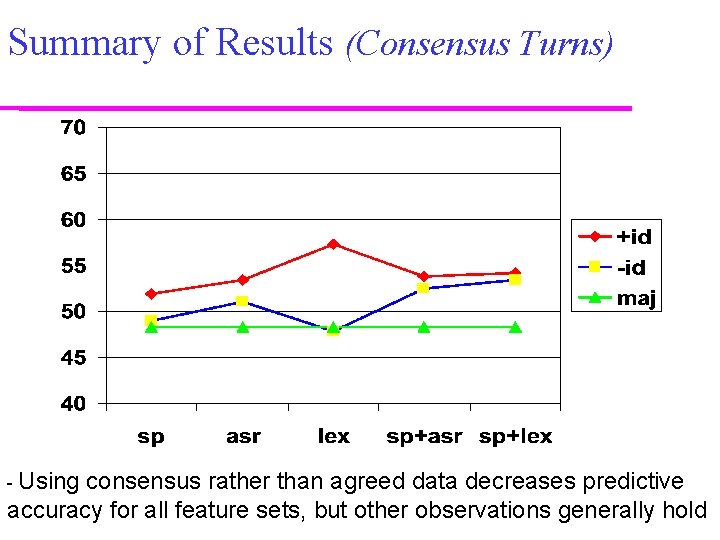
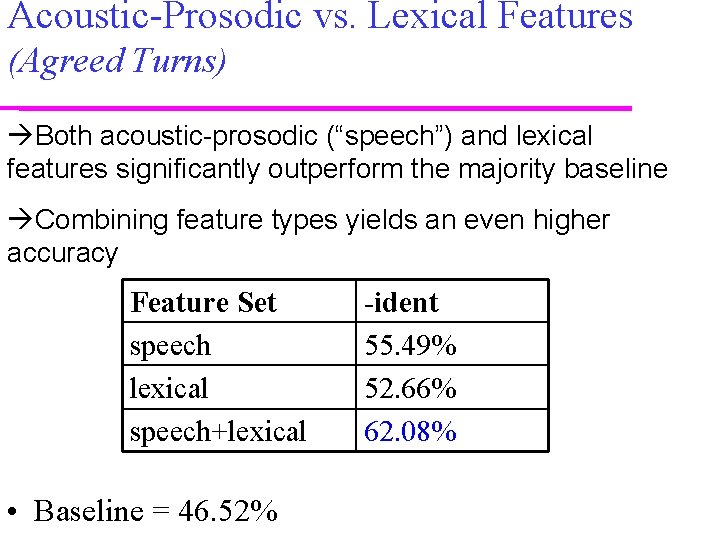
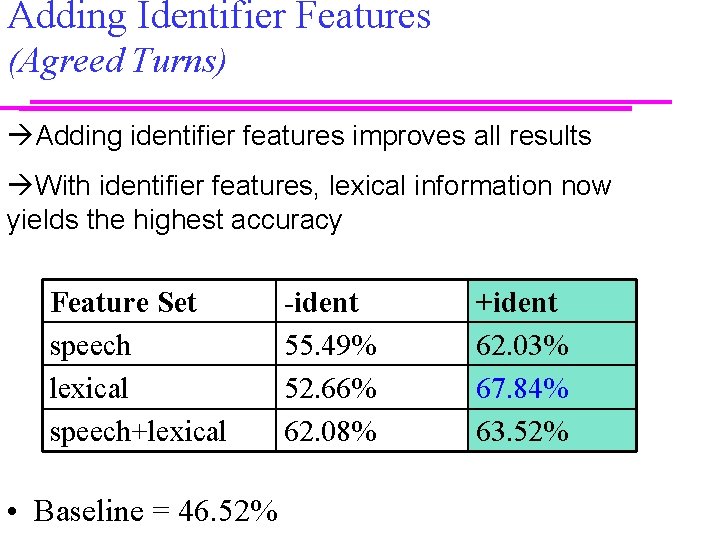
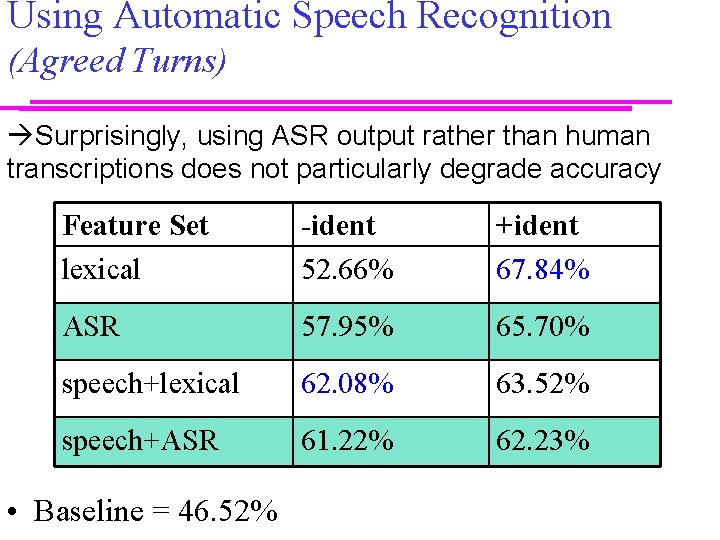
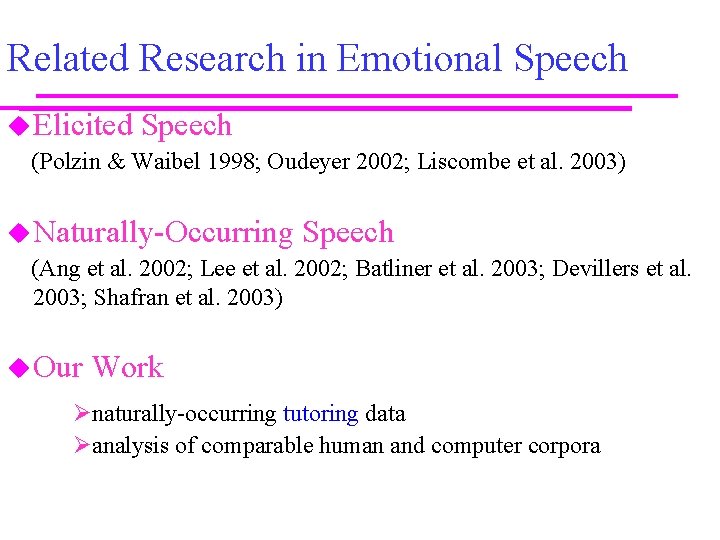
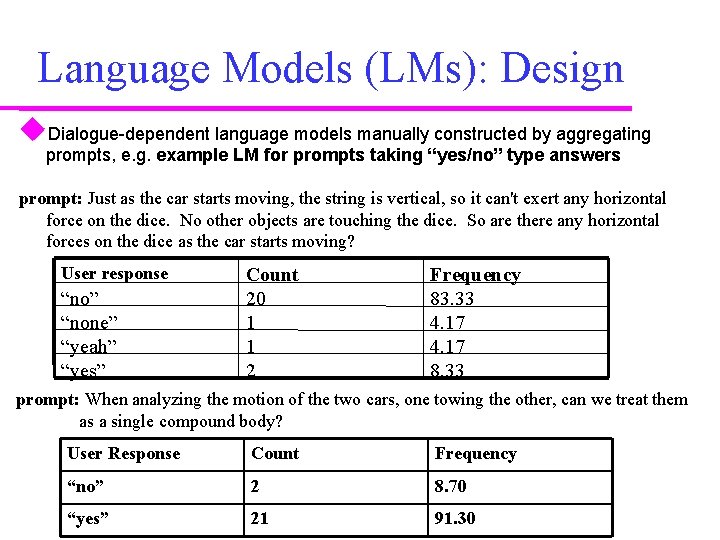
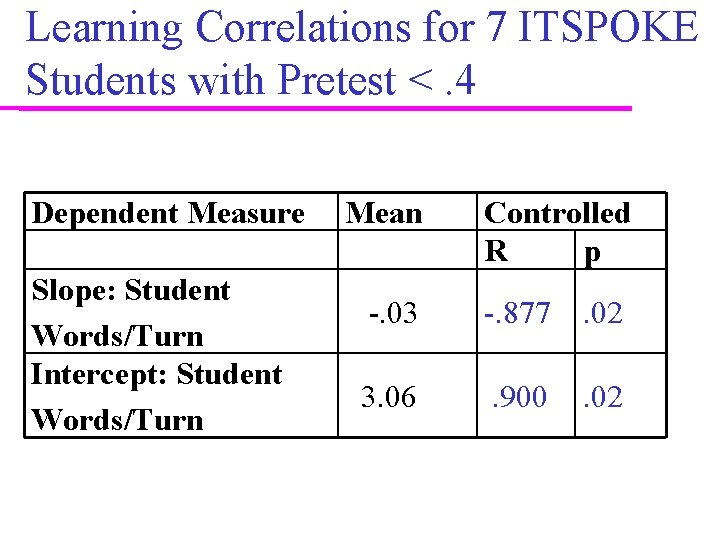
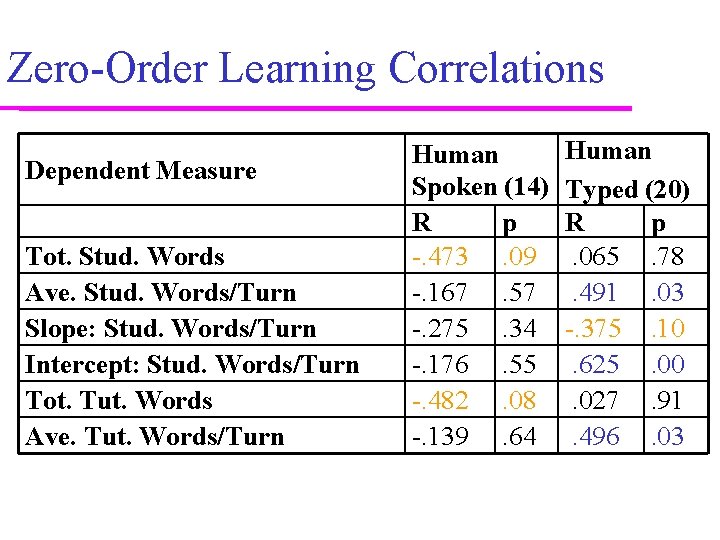
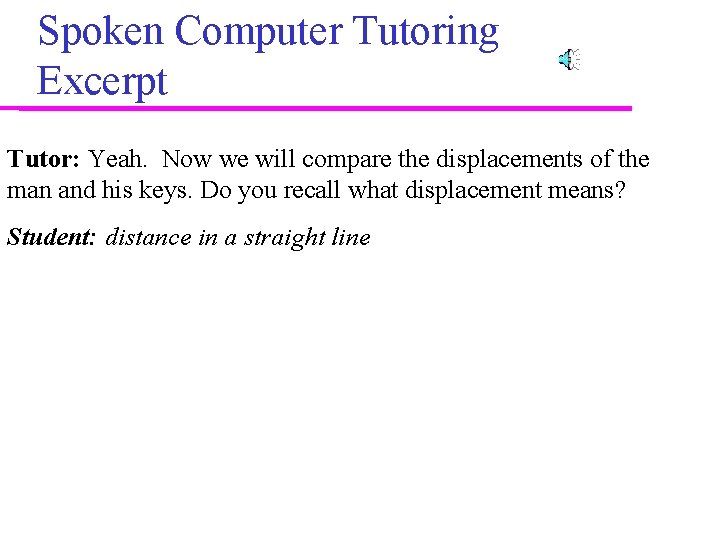
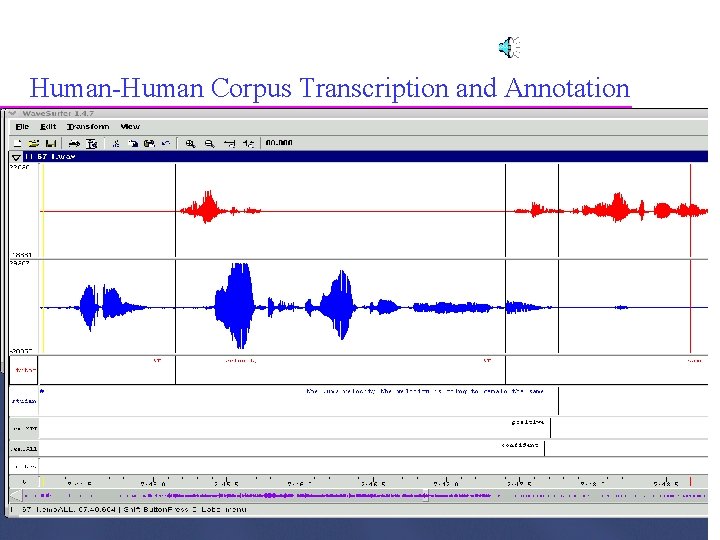
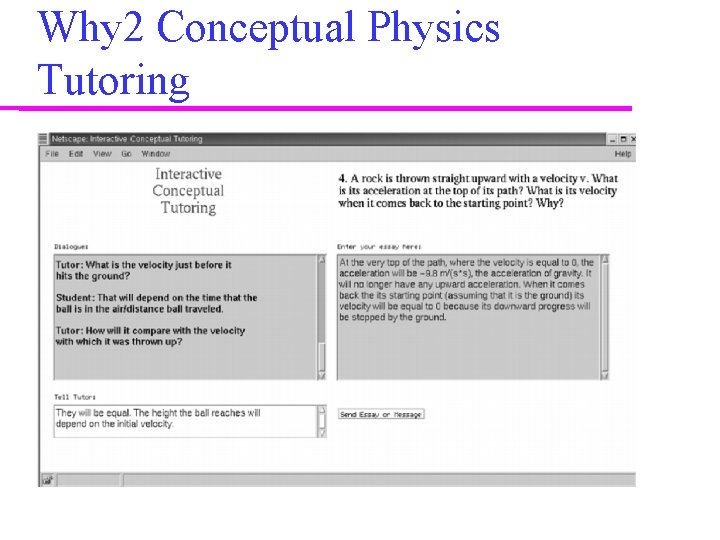
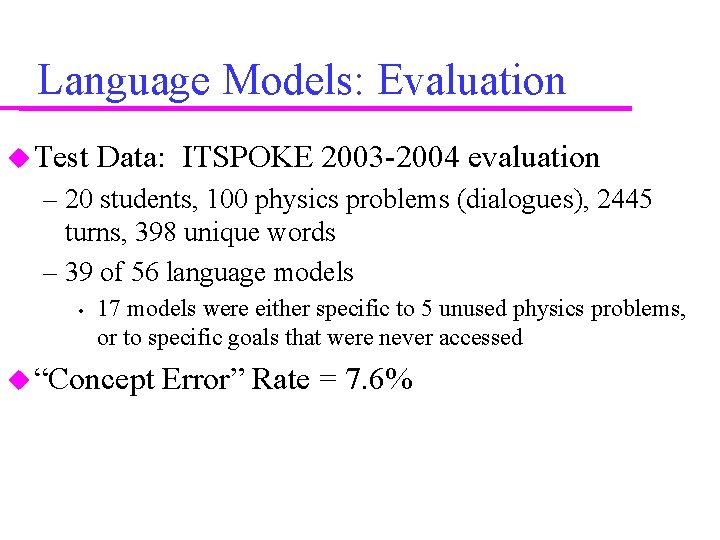
- Slides: 75
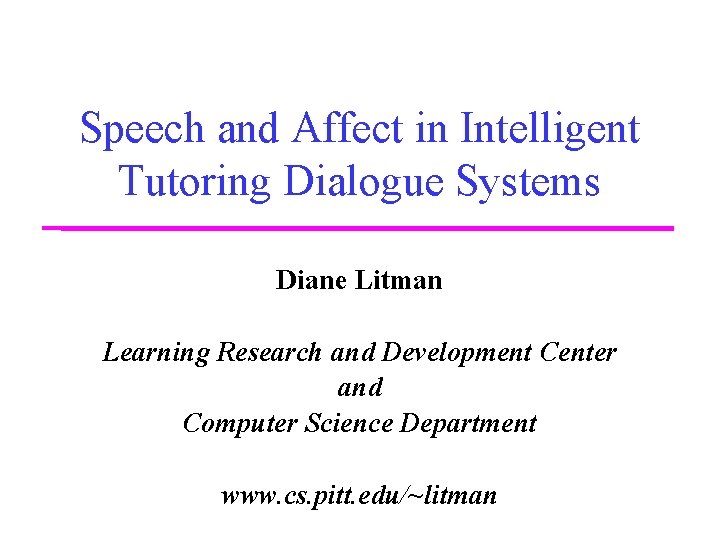
Speech and Affect in Intelligent Tutoring Dialogue Systems Diane Litman Learning Research and Development Center and Computer Science Department www. cs. pitt. edu/~litman
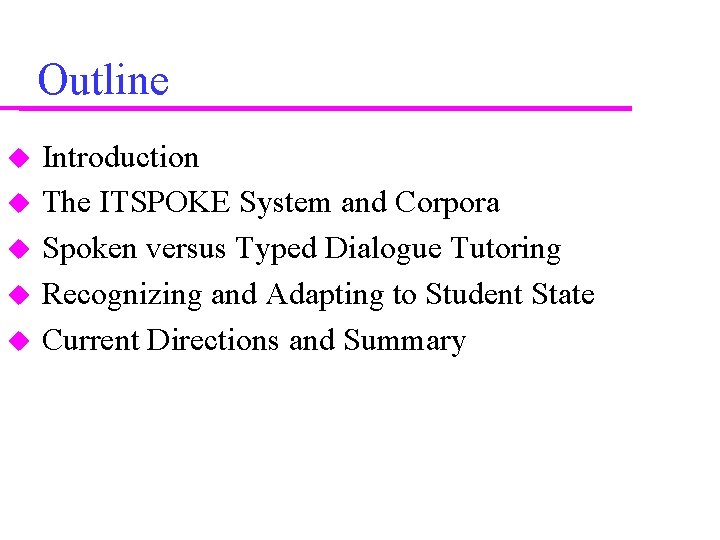
Outline Introduction The ITSPOKE System and Corpora Spoken versus Typed Dialogue Tutoring Recognizing and Adapting to Student State Current Directions and Summary
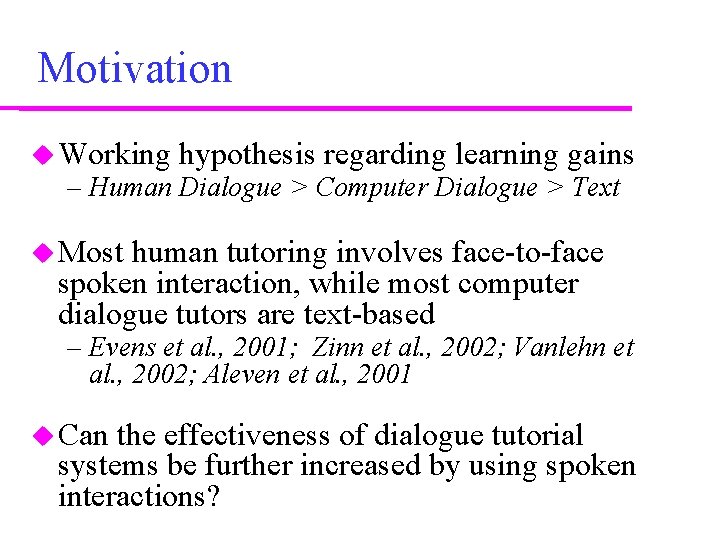
Motivation Working hypothesis regarding learning gains – Human Dialogue > Computer Dialogue > Text Most human tutoring involves face-to-face spoken interaction, while most computer dialogue tutors are text-based – Evens et al. , 2001; Zinn et al. , 2002; Vanlehn et al. , 2002; Aleven et al. , 2001 Can the effectiveness of dialogue tutorial systems be further increased by using spoken interactions?
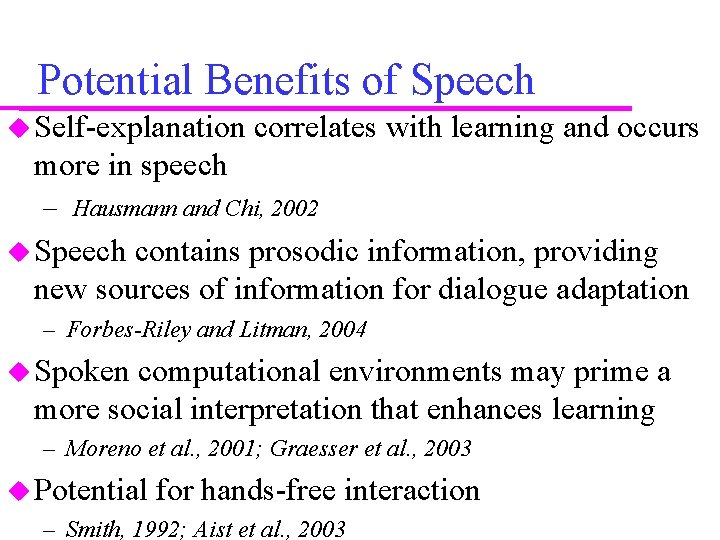
Potential Benefits of Speech Self-explanation correlates with learning and occurs more in speech – Hausmann and Chi, 2002 Speech contains prosodic information, providing new sources of information for dialogue adaptation – Forbes-Riley and Litman, 2004 Spoken computational environments may prime a more social interpretation that enhances learning – Moreno et al. , 2001; Graesser et al. , 2003 Potential for hands-free interaction – Smith, 1992; Aist et al. , 2003
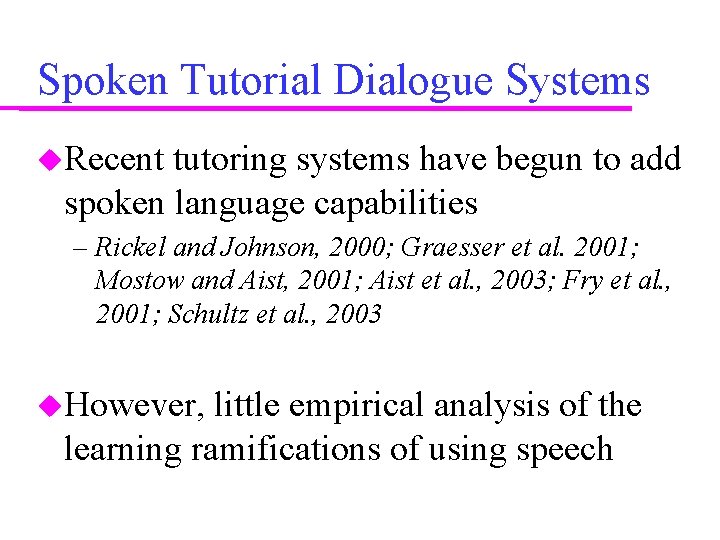
Spoken Tutorial Dialogue Systems Recent tutoring systems have begun to add spoken language capabilities – Rickel and Johnson, 2000; Graesser et al. 2001; Mostow and Aist, 2001; Aist et al. , 2003; Fry et al. , 2001; Schultz et al. , 2003 However, little empirical analysis of the learning ramifications of using speech
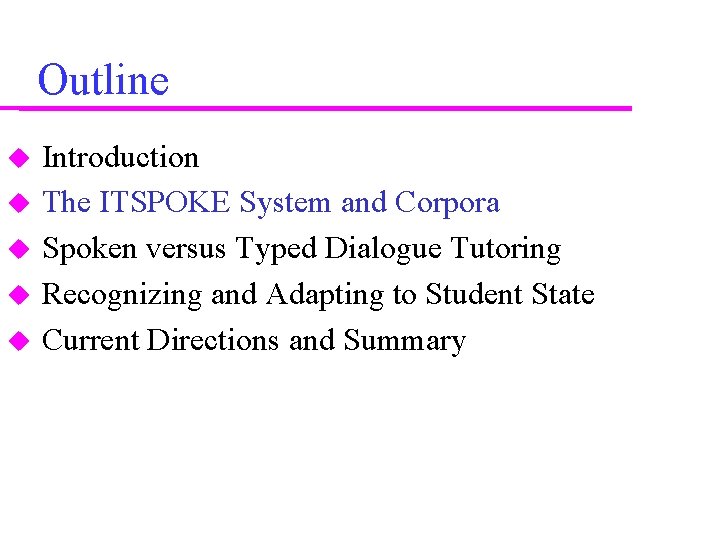
Outline Introduction The ITSPOKE System and Corpora Spoken versus Typed Dialogue Tutoring Recognizing and Adapting to Student State Current Directions and Summary
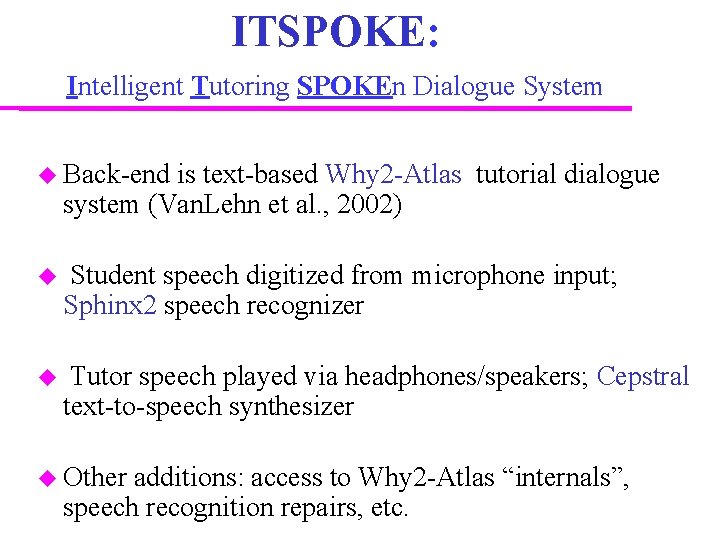
ITSPOKE: Intelligent Tutoring SPOKEn Dialogue System Back-end is text-based Why 2 -Atlas tutorial dialogue system (Van. Lehn et al. , 2002) Student speech digitized from microphone input; Sphinx 2 speech recognizer Tutor speech played via headphones/speakers; Cepstral text-to-speech synthesizer Other additions: access to Why 2 -Atlas “internals”, speech recognition repairs, etc.
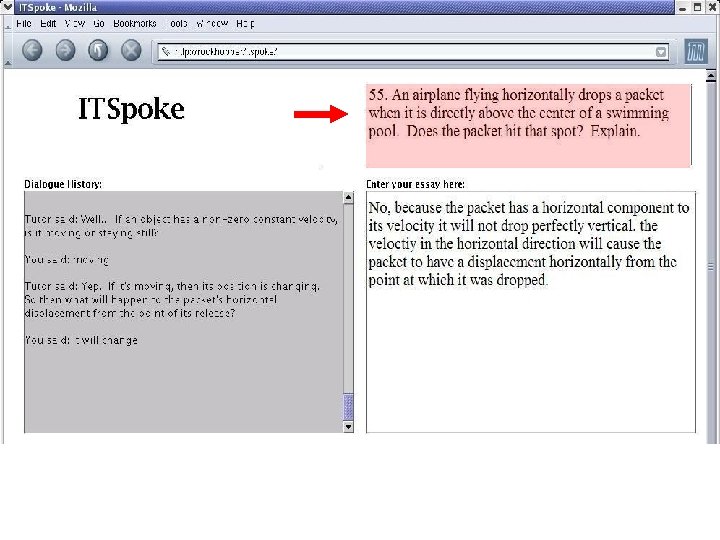
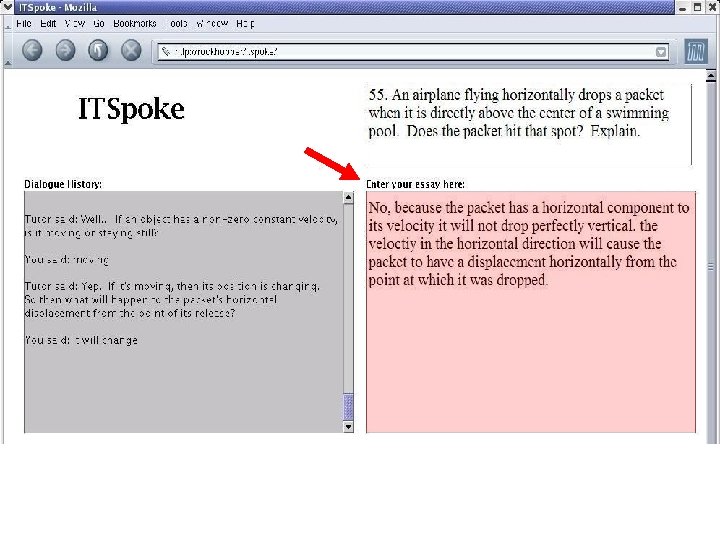
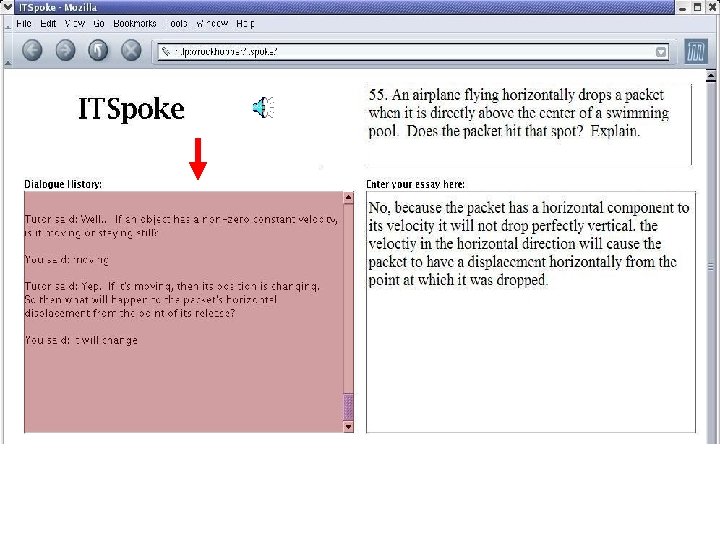
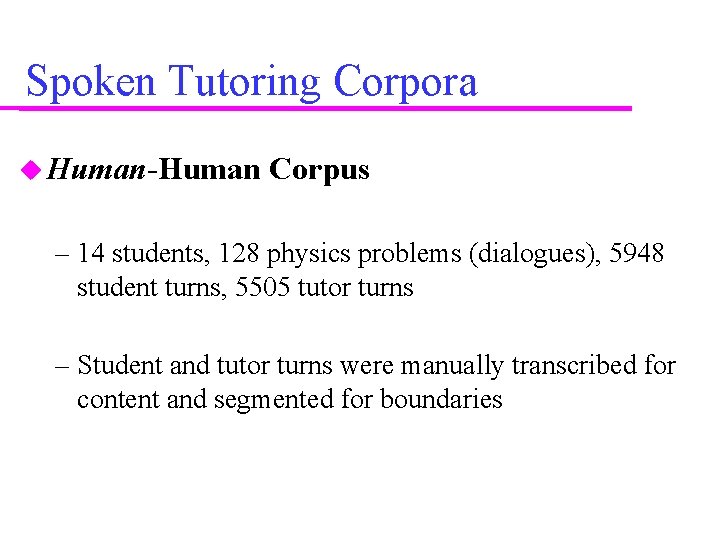
Spoken Tutoring Corpora Human-Human Corpus – 14 students, 128 physics problems (dialogues), 5948 student turns, 5505 tutor turns – Student and tutor turns were manually transcribed for content and segmented for boundaries
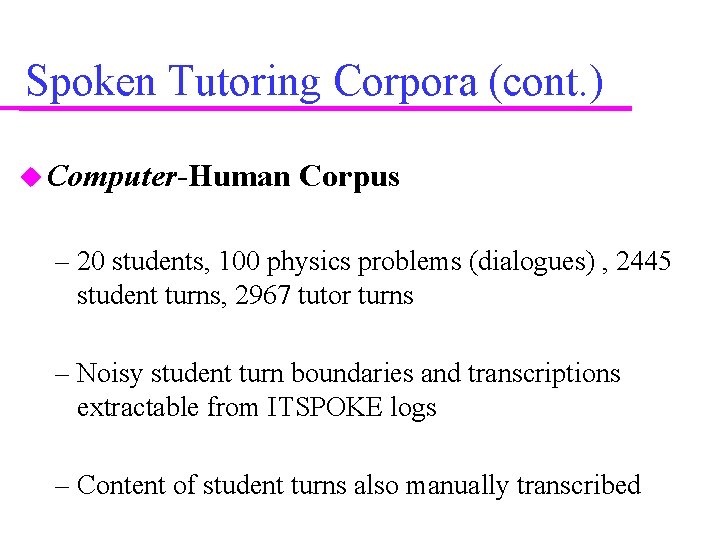
Spoken Tutoring Corpora (cont. ) Computer-Human Corpus – 20 students, 100 physics problems (dialogues) , 2445 student turns, 2967 tutor turns – Noisy student turn boundaries and transcriptions extractable from ITSPOKE logs – Content of student turns also manually transcribed
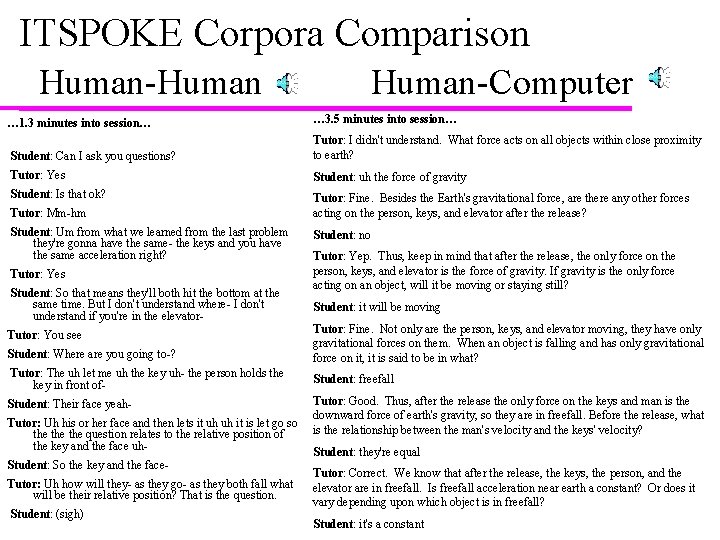
ITSPOKE Corpora Comparison Human-Human-Computer … 1. 3 minutes into session… … 3. 5 minutes into session… Student: Can I ask you questions? Tutor: I didn't understand. What force acts on all objects within close proximity to earth? Tutor: Yes Student: uh the force of gravity Student: Is that ok? Tutor: Fine. Besides the Earth's gravitational force, are there any other forces acting on the person, keys, and elevator after the release? Tutor: Mm-hm Student: Um from what we learned from the last problem they're gonna have the same- the keys and you have the same acceleration right? Tutor: Yes Student: So that means they'll both hit the bottom at the same time. But I don't understand where- I don't understand if you're in the elevator- Student: no Tutor: Yep. Thus, keep in mind that after the release, the only force on the person, keys, and elevator is the force of gravity. If gravity is the only force acting on an object, will it be moving or staying still? Student: it will be moving Student: Where are you going to-? Tutor: Fine. Not only are the person, keys, and elevator moving, they have only gravitational forces on them. When an object is falling and has only gravitational force on it, it is said to be in what? Tutor: The uh let me uh the key uh- the person holds the key in front of- Student: freefall Tutor: You see Student: Their face yeah. Tutor: Uh his or her face and then lets it uh uh it is let go so the the question relates to the relative position of the key and the face uh. Student: So the key and the face. Tutor: Uh how will they- as they go- as they both fall what will be their relative position? That is the question. Student: (sigh) Tutor: Good. Thus, after the release the only force on the keys and man is the downward force of earth's gravity, so they are in freefall. Before the release, what is the relationship between the man's velocity and the keys' velocity? Student: they're equal Tutor: Correct. We know that after the release, the keys, the person, and the elevator are in freefall. Is freefall acceleration near earth a constant? Or does it vary depending upon which object is in freefall? Student: it's a constant
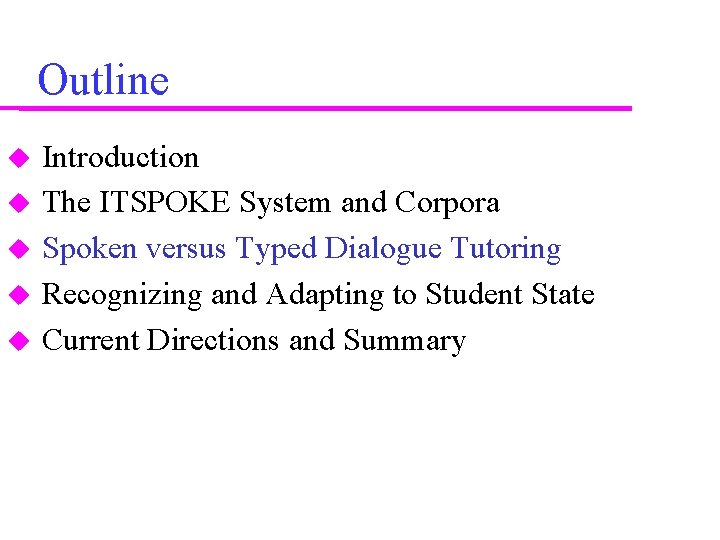
Outline Introduction The ITSPOKE System and Corpora Spoken versus Typed Dialogue Tutoring Recognizing and Adapting to Student State Current Directions and Summary
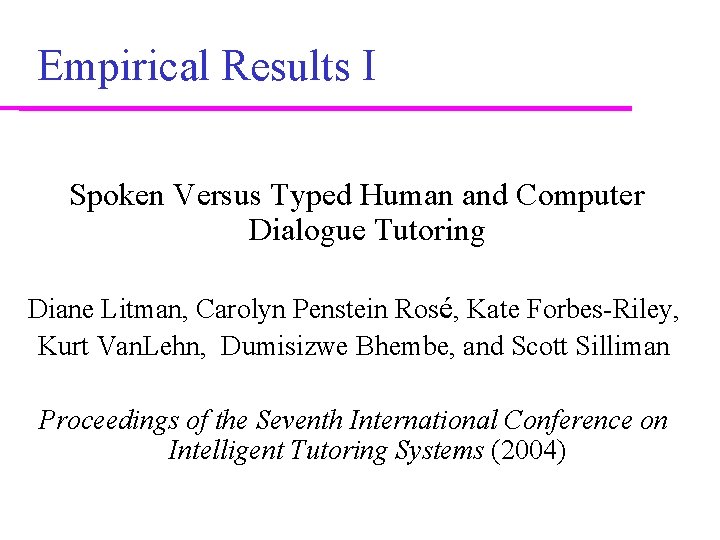
Empirical Results I Spoken Versus Typed Human and Computer Dialogue Tutoring Diane Litman, Carolyn Penstein Rosé, Kate Forbes-Riley, Kurt Van. Lehn, Dumisizwe Bhembe, and Scott Silliman Proceedings of the Seventh International Conference on Intelligent Tutoring Systems (2004)
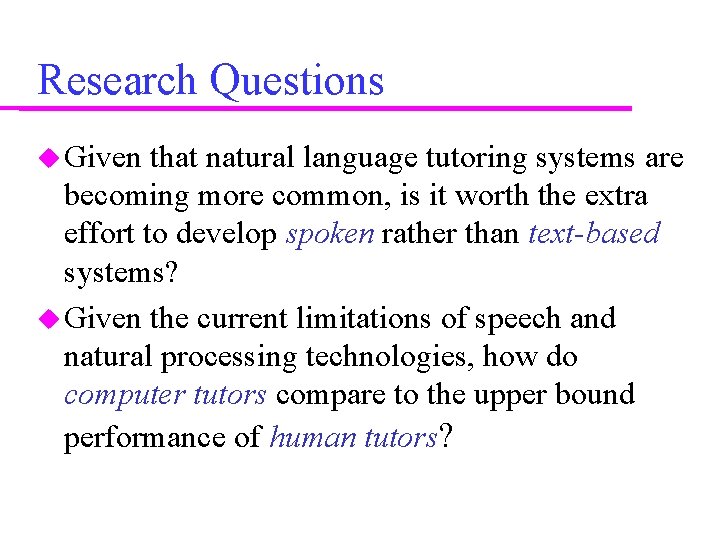
Research Questions Given that natural language tutoring systems are becoming more common, is it worth the extra effort to develop spoken rather than text-based systems? Given the current limitations of speech and natural processing technologies, how do computer tutors compare to the upper bound performance of human tutors?
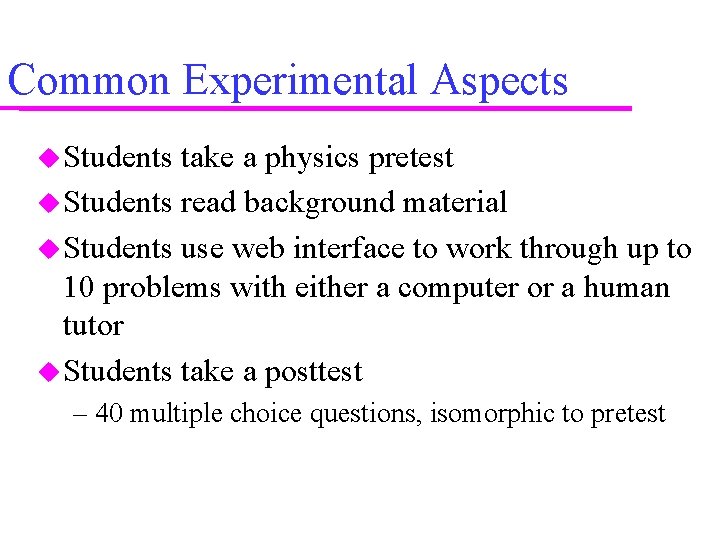
Common Experimental Aspects Students take a physics pretest Students read background material Students use web interface to work through up to 10 problems with either a computer or a human tutor Students take a posttest – 40 multiple choice questions, isomorphic to pretest
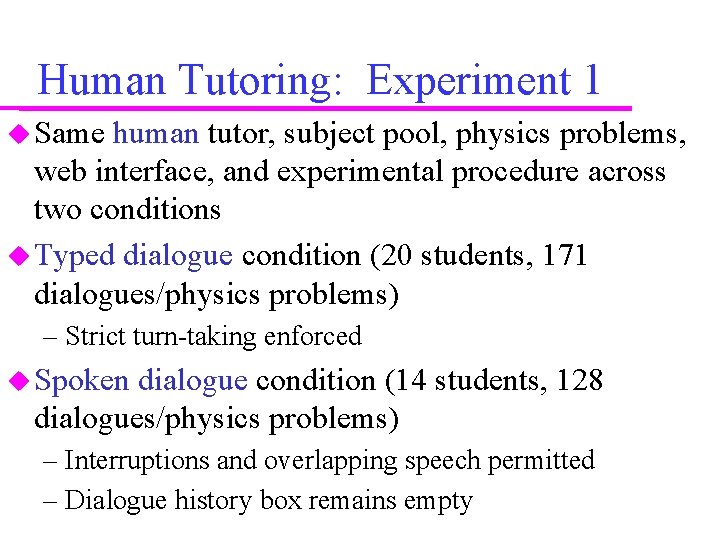
Human Tutoring: Experiment 1 Same human tutor, subject pool, physics problems, web interface, and experimental procedure across two conditions Typed dialogue condition (20 students, 171 dialogues/physics problems) – Strict turn-taking enforced Spoken dialogue condition (14 students, 128 dialogues/physics problems) – Interruptions and overlapping speech permitted – Dialogue history box remains empty
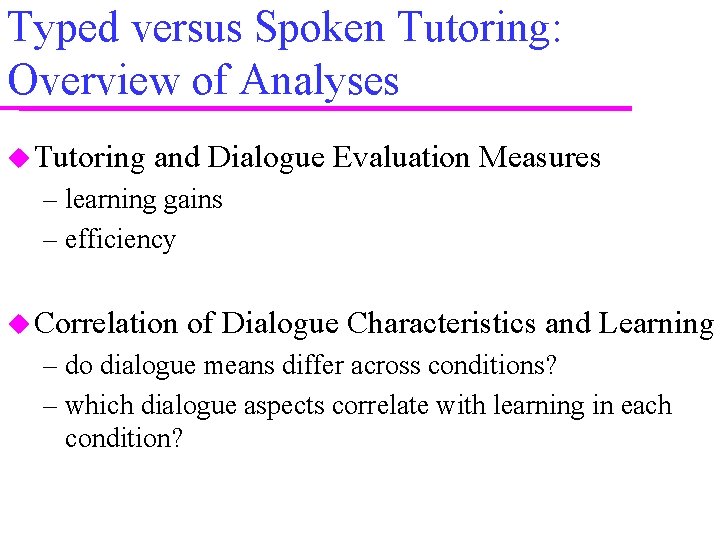
Typed versus Spoken Tutoring: Overview of Analyses Tutoring and Dialogue Evaluation Measures – learning gains – efficiency Correlation of Dialogue Characteristics and Learning – do dialogue means differ across conditions? – which dialogue aspects correlate with learning in each condition?
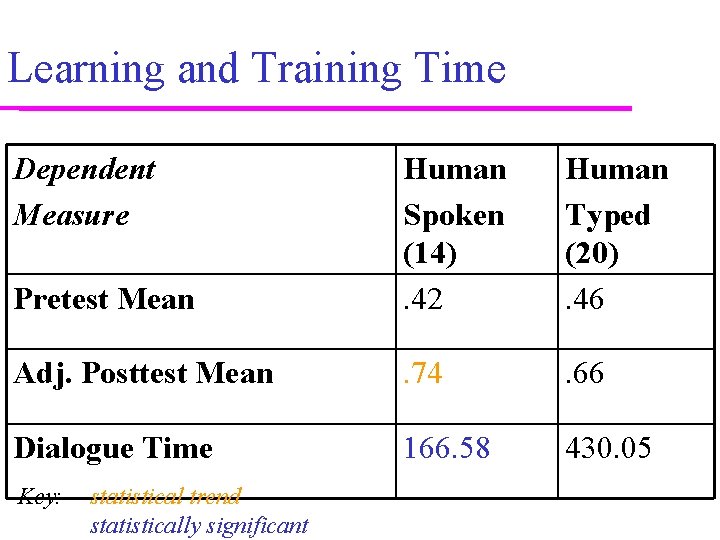
Learning and Training Time Dependent Measure Pretest Mean Human Spoken (14). 42 Human Typed (20). 46 Adj. Posttest Mean . 74 . 66 Dialogue Time 166. 58 430. 05 Key: statistical trend statistically significant
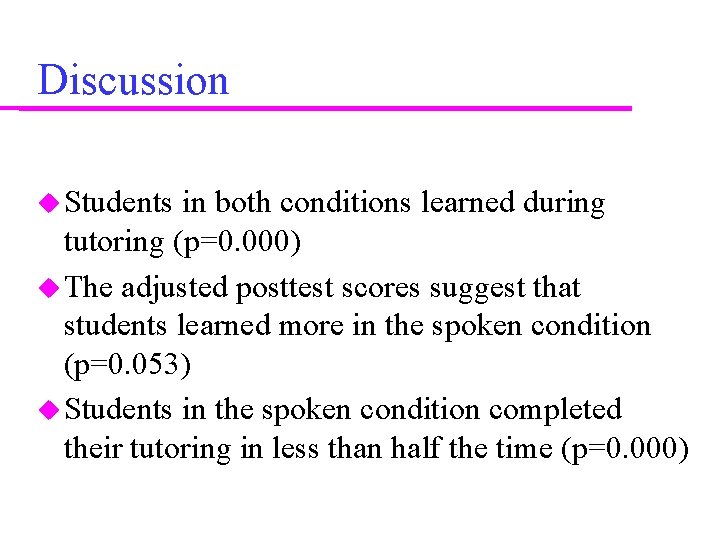
Discussion Students in both conditions learned during tutoring (p=0. 000) The adjusted posttest scores suggest that students learned more in the spoken condition (p=0. 053) Students in the spoken condition completed their tutoring in less than half the time (p=0. 000)
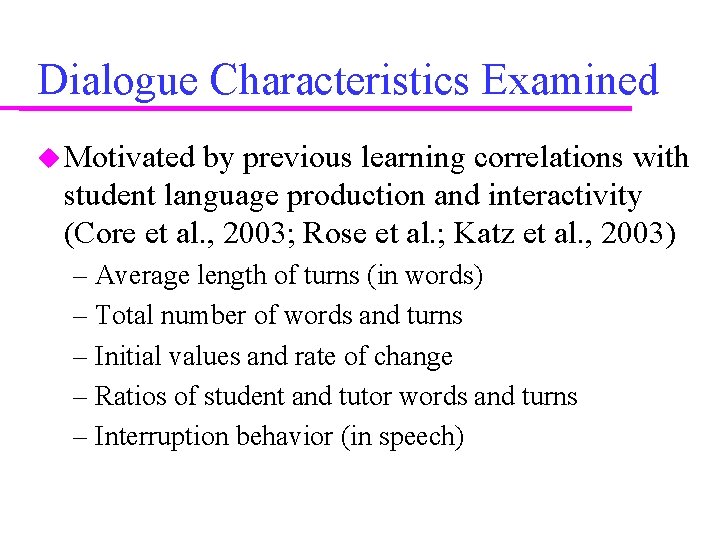
Dialogue Characteristics Examined Motivated by previous learning correlations with student language production and interactivity (Core et al. , 2003; Rose et al. ; Katz et al. , 2003) – Average length of turns (in words) – Total number of words and turns – Initial values and rate of change – Ratios of student and tutor words and turns – Interruption behavior (in speech)
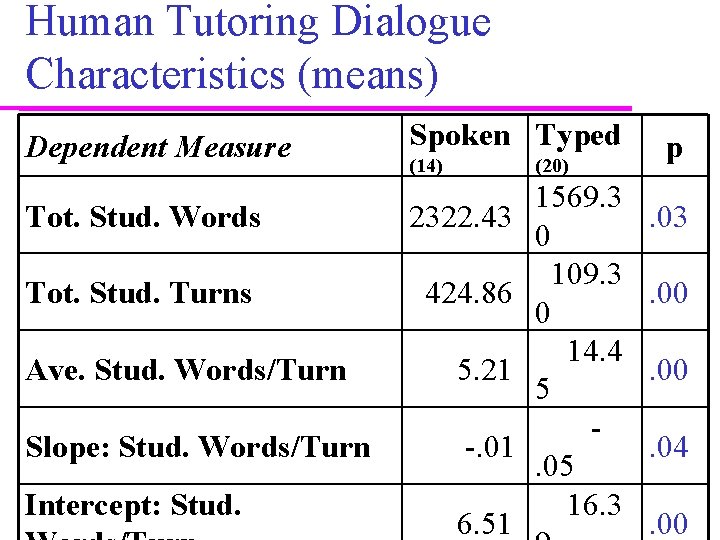
Human Tutoring Dialogue Characteristics (means) Dependent Measure Spoken Typed (14) (20) Tot. Stud. Words 2322. 43 Tot. Stud. Turns 424. 86 Ave. Stud. Words/Turn 5. 21 Slope: Stud. Words/Turn -. 01 Intercept: Stud. 6. 51 1569. 3 0 109. 3 0 14. 4 5. 05 16. 3 p. 03. 00. 04. 00
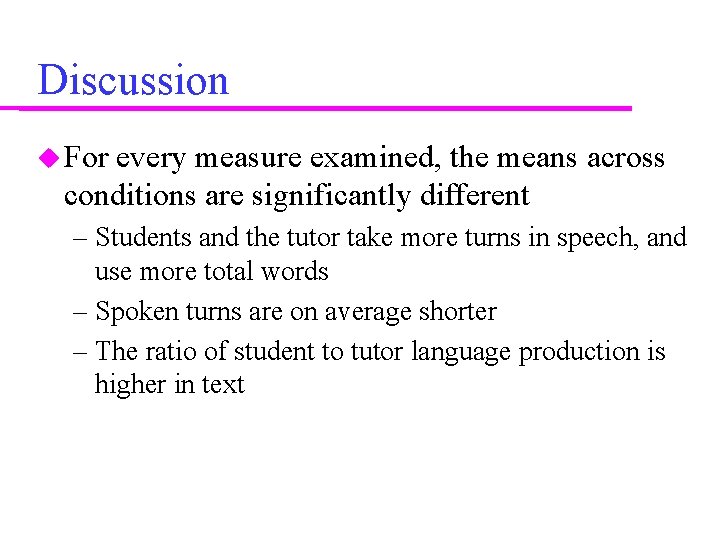
Discussion For every measure examined, the means across conditions are significantly different – Students and the tutor take more turns in speech, and use more total words – Spoken turns are on average shorter – The ratio of student to tutor language production is higher in text
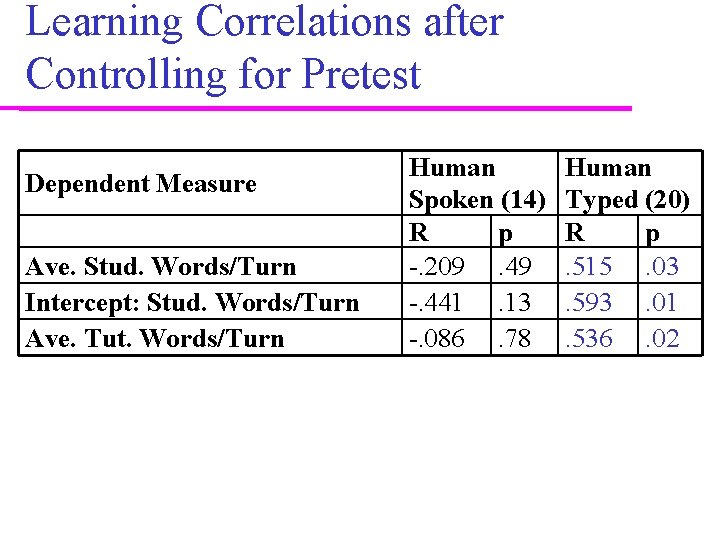
Learning Correlations after Controlling for Pretest Dependent Measure Ave. Stud. Words/Turn Intercept: Stud. Words/Turn Ave. Tut. Words/Turn Human Spoken (14) R p -. 209. 49 -. 441. 13 -. 086. 78 Human Typed (20) R p. 515. 03. 593. 01. 536. 02
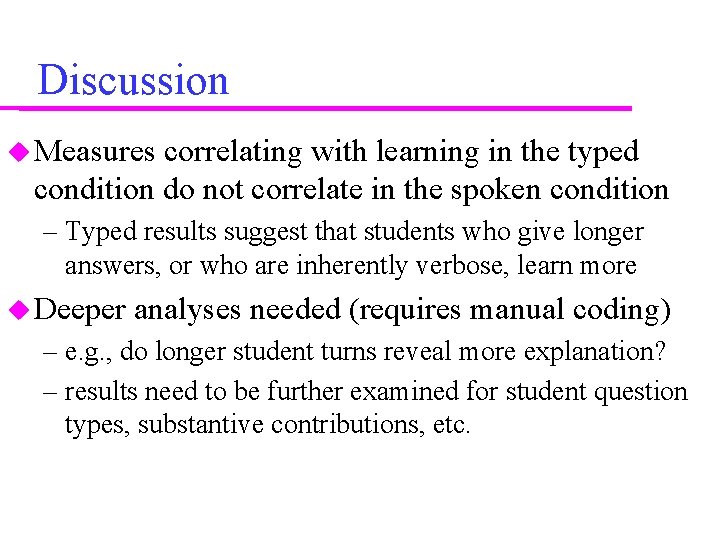
Discussion Measures correlating with learning in the typed condition do not correlate in the spoken condition – Typed results suggest that students who give longer answers, or who are inherently verbose, learn more Deeper analyses needed (requires manual coding) – e. g. , do longer student turns reveal more explanation? – results need to be further examined for student question types, substantive contributions, etc.
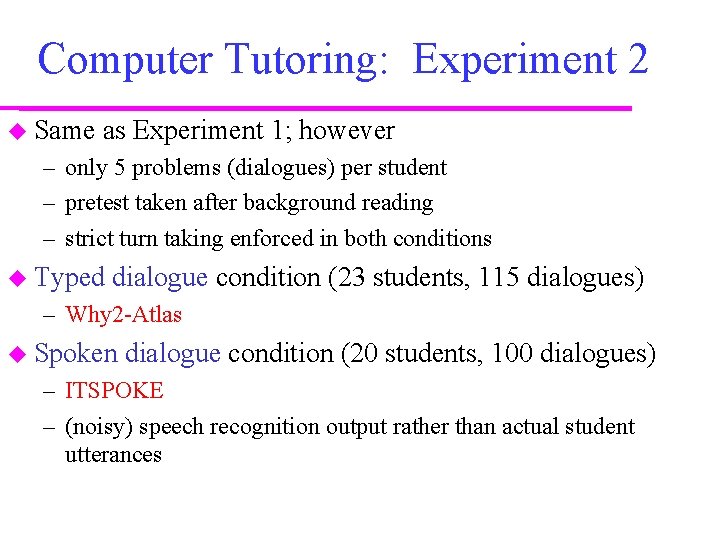
Computer Tutoring: Experiment 2 Same as Experiment 1; however – only 5 problems (dialogues) per student – pretest taken after background reading – strict turn taking enforced in both conditions Typed dialogue condition (23 students, 115 dialogues) – Why 2 -Atlas Spoken dialogue condition (20 students, 100 dialogues) – ITSPOKE – (noisy) speech recognition output rather than actual student utterances
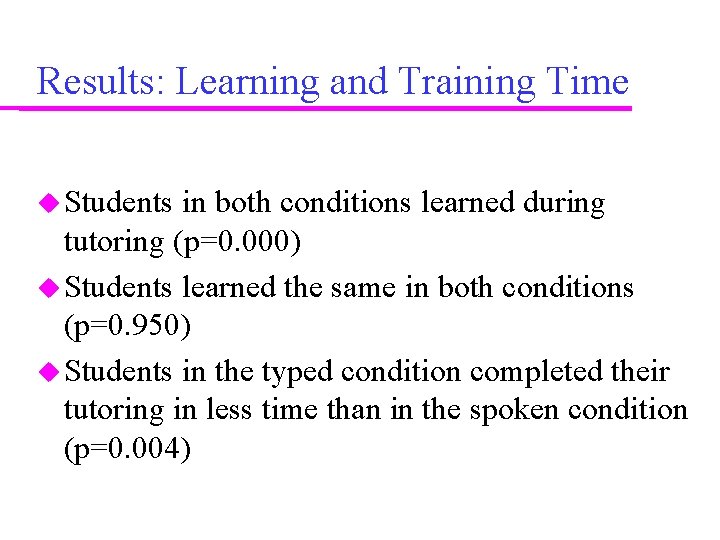
Results: Learning and Training Time Students in both conditions learned during tutoring (p=0. 000) Students learned the same in both conditions (p=0. 950) Students in the typed condition completed their tutoring in less time than in the spoken condition (p=0. 004)
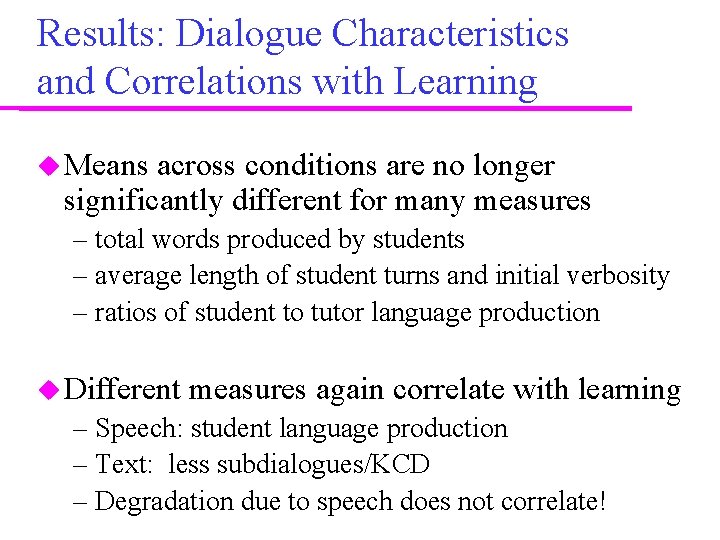
Results: Dialogue Characteristics and Correlations with Learning Means across conditions are no longer significantly different for many measures – total words produced by students – average length of student turns and initial verbosity – ratios of student to tutor language production Different measures again correlate with learning – Speech: student language production – Text: less subdialogues/KCD – Degradation due to speech does not correlate!
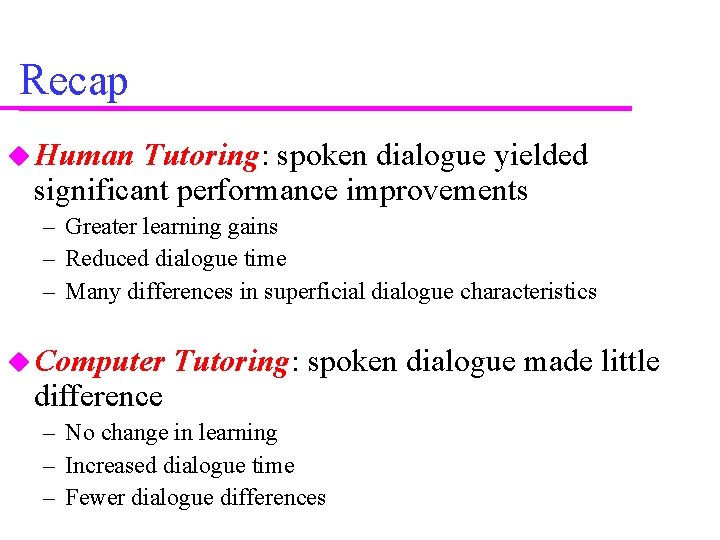
Recap Human Tutoring: spoken dialogue yielded significant performance improvements – Greater learning gains – Reduced dialogue time – Many differences in superficial dialogue characteristics Computer difference Tutoring: spoken dialogue made little – No change in learning – Increased dialogue time – Fewer dialogue differences
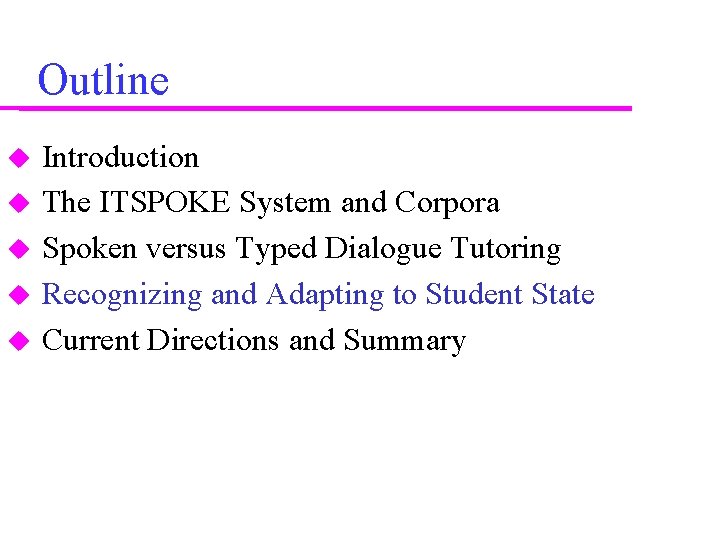
Outline Introduction The ITSPOKE System and Corpora Spoken versus Typed Dialogue Tutoring Recognizing and Adapting to Student State Current Directions and Summary
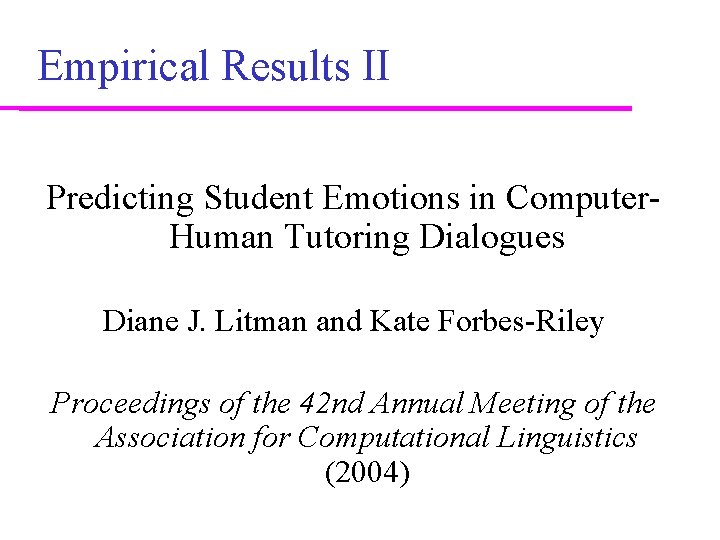
Empirical Results II Predicting Student Emotions in Computer. Human Tutoring Dialogues Diane J. Litman and Kate Forbes-Riley Proceedings of the 42 nd Annual Meeting of the Association for Computational Linguistics (2004)
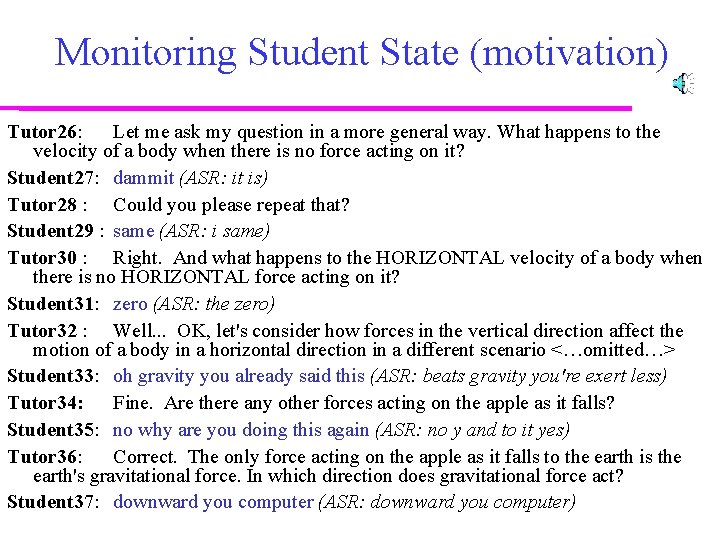
Monitoring Student State (motivation) Tutor 26: Let me ask my question in a more general way. What happens to the velocity of a body when there is no force acting on it? Student 27: dammit (ASR: it is) Tutor 28 : Could you please repeat that? Student 29 : same (ASR: i same) Tutor 30 : Right. And what happens to the HORIZONTAL velocity of a body when there is no HORIZONTAL force acting on it? Student 31: zero (ASR: the zero) Tutor 32 : Well. . . OK, let's consider how forces in the vertical direction affect the motion of a body in a horizontal direction in a different scenario <…omitted…> Student 33: oh gravity you already said this (ASR: beats gravity you're exert less) Tutor 34: Fine. Are there any other forces acting on the apple as it falls? Student 35: no why are you doing this again (ASR: no y and to it yes) Tutor 36: Correct. The only force acting on the apple as it falls to the earth is the earth's gravitational force. In which direction does gravitational force act? Student 37: downward you computer (ASR: downward you computer)
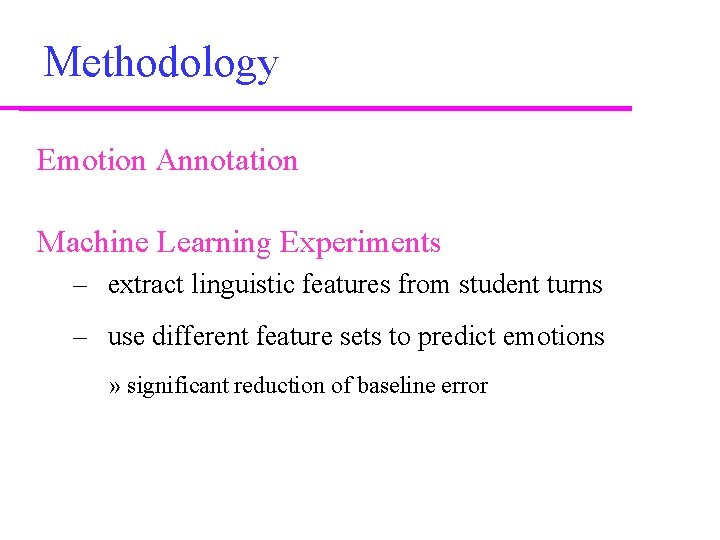
Methodology Emotion Annotation Machine Learning Experiments – extract linguistic features from student turns – use different feature sets to predict emotions » significant reduction of baseline error
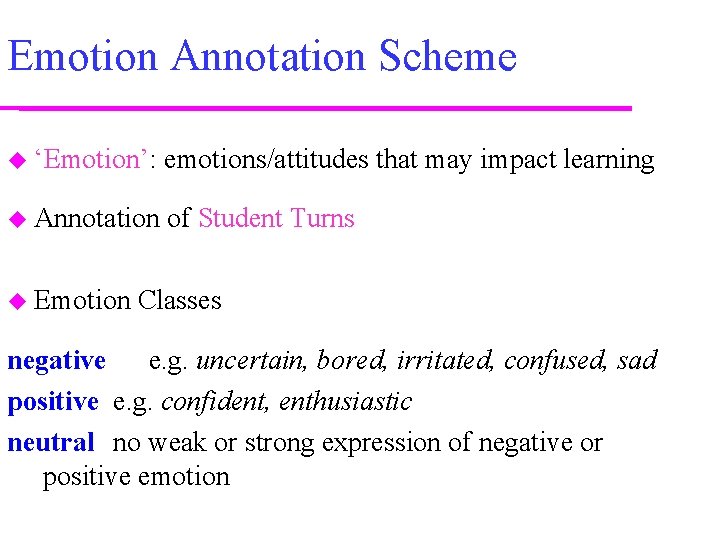
Emotion Annotation Scheme ‘Emotion’: emotions/attitudes that may impact learning Annotation of Student Turns Emotion Classes negative e. g. uncertain, bored, irritated, confused, sad positive e. g. confident, enthusiastic neutral no weak or strong expression of negative or positive emotion
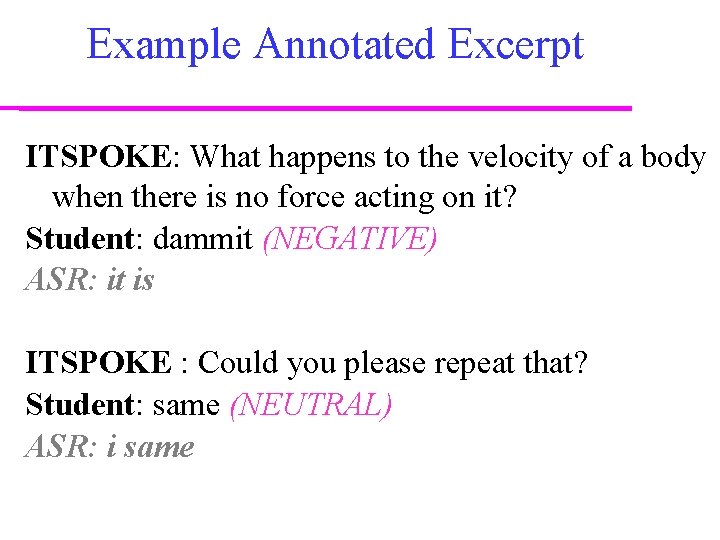
Example Annotated Excerpt ITSPOKE: What happens to the velocity of a body when there is no force acting on it? Student: dammit (NEGATIVE) ASR: it is ITSPOKE : Could you please repeat that? Student: same (NEUTRAL) ASR: i same
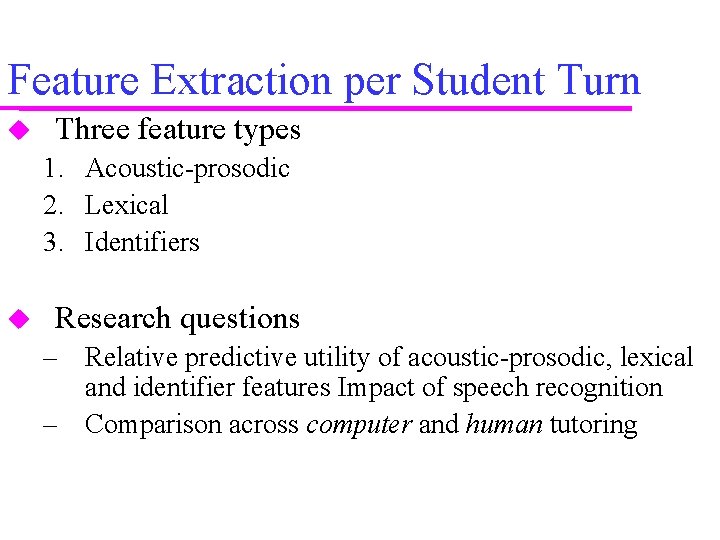
Feature Extraction per Student Turn Three feature types 1. Acoustic-prosodic 2. Lexical 3. Identifiers Research questions – Relative predictive utility of acoustic-prosodic, lexical and identifier features Impact of speech recognition – Comparison across computer and human tutoring
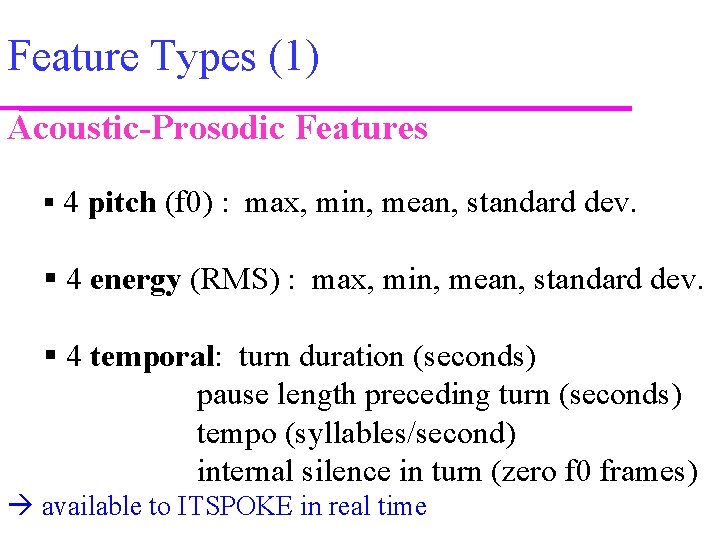
Feature Types (1) Acoustic-Prosodic Features § 4 pitch (f 0) : max, min, mean, standard dev. § 4 energy (RMS) : max, min, mean, standard dev. § 4 temporal: turn duration (seconds) pause length preceding turn (seconds) tempo (syllables/second) internal silence in turn (zero f 0 frames) available to ITSPOKE in real time
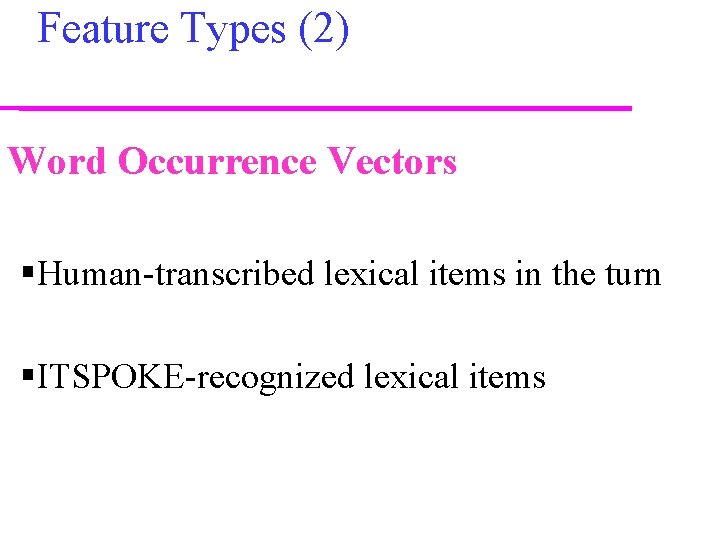
Feature Types (2) Word Occurrence Vectors §Human-transcribed lexical items in the turn §ITSPOKE-recognized lexical items
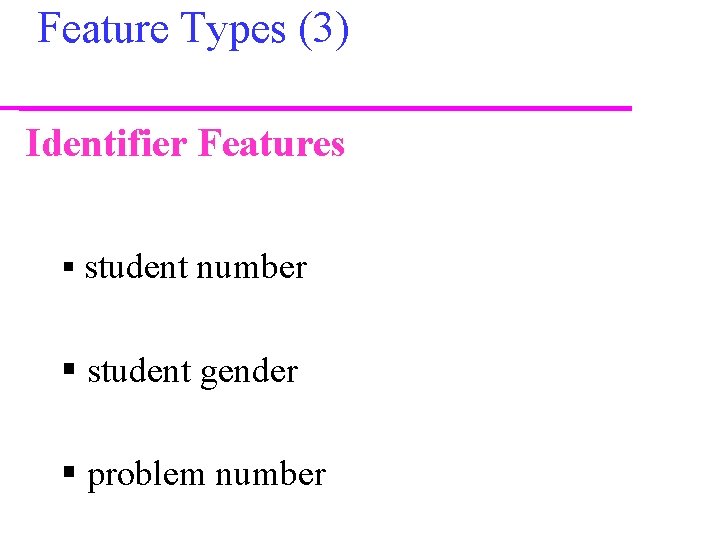
Feature Types (3) Identifier Features § student number § student gender § problem number
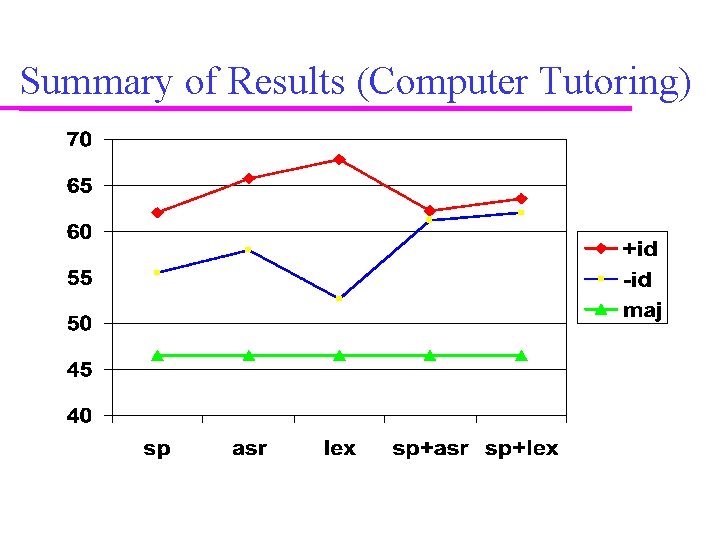
Summary of Results (Computer Tutoring)
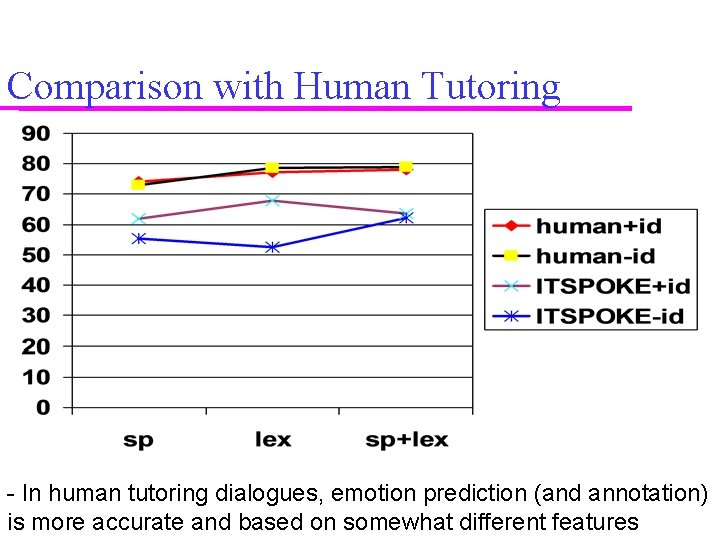
Comparison with Human Tutoring - In human tutoring dialogues, emotion prediction (and annotation) is more accurate and based on somewhat different features
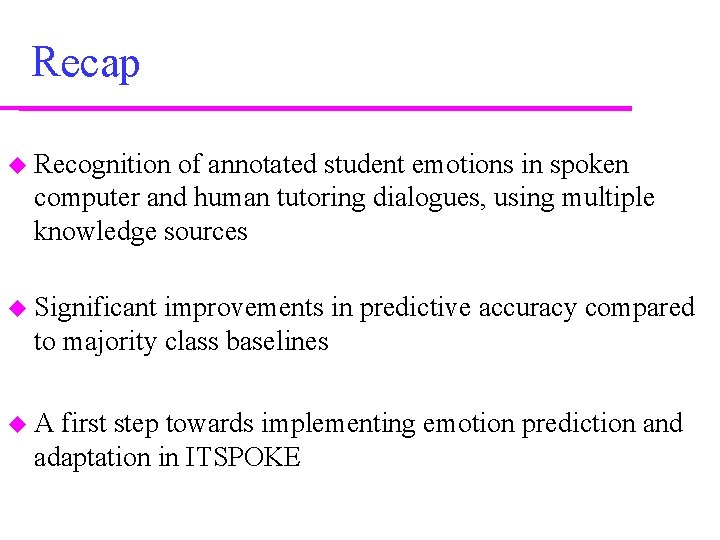
Recap Recognition of annotated student emotions in spoken computer and human tutoring dialogues, using multiple knowledge sources Significant improvements in predictive accuracy compared to majority class baselines A first step towards implementing emotion prediction and adaptation in ITSPOKE
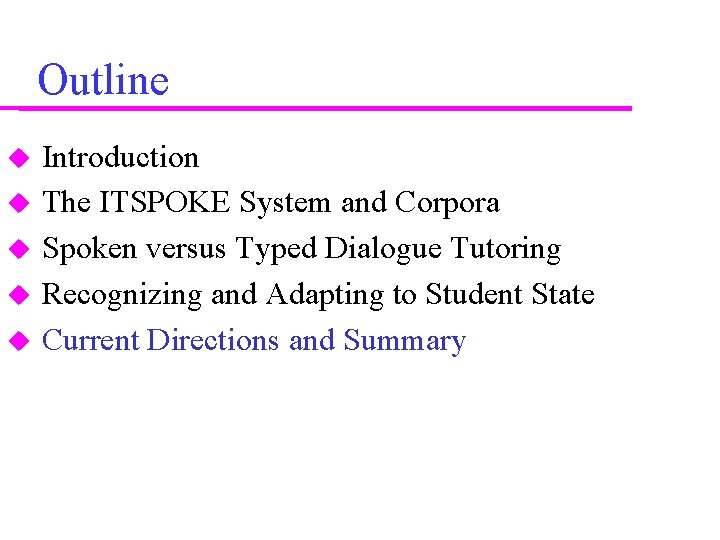
Outline Introduction The ITSPOKE System and Corpora Spoken versus Typed Dialogue Tutoring Recognizing and Adapting to Student State Current Directions and Summary
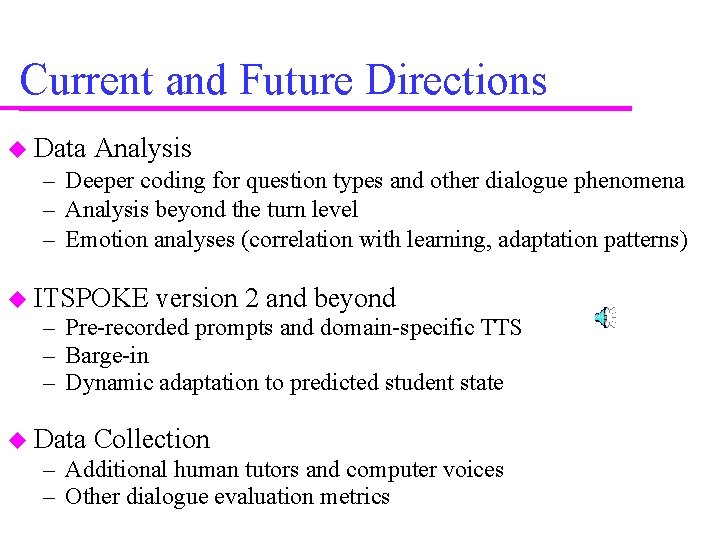
Current and Future Directions Data Analysis – Deeper coding for question types and other dialogue phenomena – Analysis beyond the turn level – Emotion analyses (correlation with learning, adaptation patterns) ITSPOKE version 2 and beyond – Pre-recorded prompts and domain-specific TTS – Barge-in – Dynamic adaptation to predicted student state Data Collection – Additional human tutors and computer voices – Other dialogue evaluation metrics
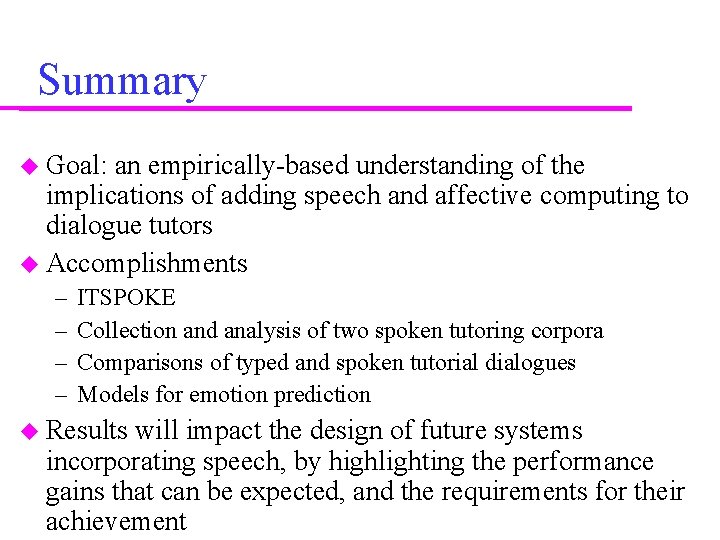
Summary Goal: an empirically-based understanding of the implications of adding speech and affective computing to dialogue tutors Accomplishments – – ITSPOKE Collection and analysis of two spoken tutoring corpora Comparisons of typed and spoken tutorial dialogues Models for emotion prediction Results will impact the design of future systems incorporating speech, by highlighting the performance gains that can be expected, and the requirements for their achievement
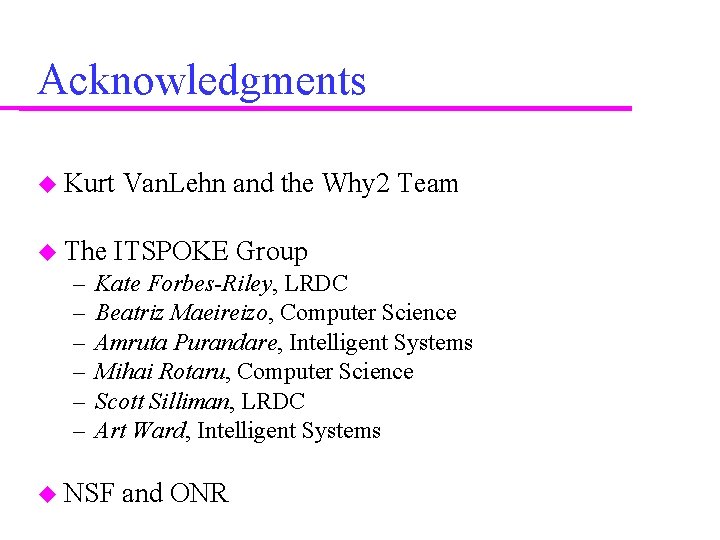
Acknowledgments Kurt The – – – Van. Lehn and the Why 2 Team ITSPOKE Group Kate Forbes-Riley, LRDC Beatriz Maeireizo, Computer Science Amruta Purandare, Intelligent Systems Mihai Rotaru, Computer Science Scott Silliman, LRDC Art Ward, Intelligent Systems NSF and ONR
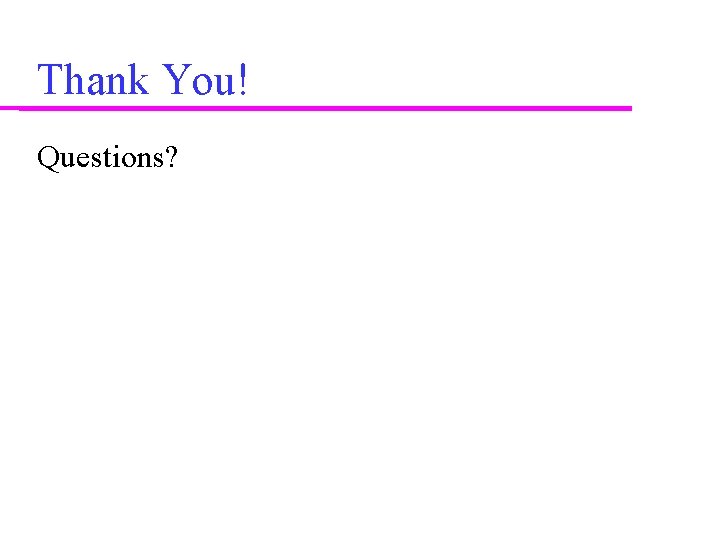
Thank You! Questions?
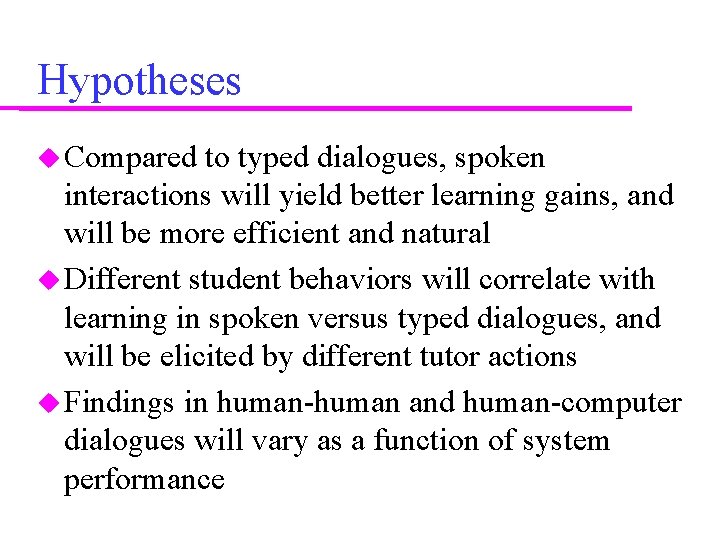
Hypotheses Compared to typed dialogues, spoken interactions will yield better learning gains, and will be more efficient and natural Different student behaviors will correlate with learning in spoken versus typed dialogues, and will be elicited by different tutor actions Findings in human-human and human-computer dialogues will vary as a function of system performance
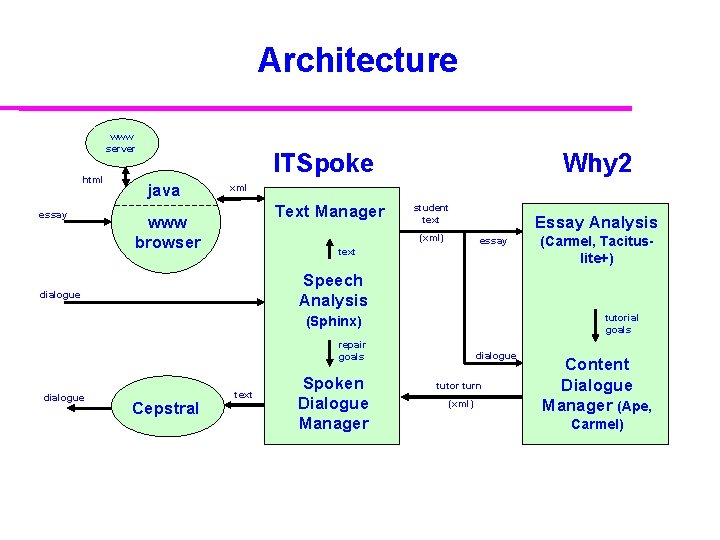
Architecture www server html essay ITSpoke java Why 2 xml Text Manager www browser student text (xml) Essay Analysis essay text Speech Analysis dialogue tutorial goals (Sphinx) repair goals dialogue (Carmel, Tacituslite+) Cepstral text Spoken Dialogue Manager dialogue tutor turn (xml) Content Dialogue Manager (Ape, Carmel)
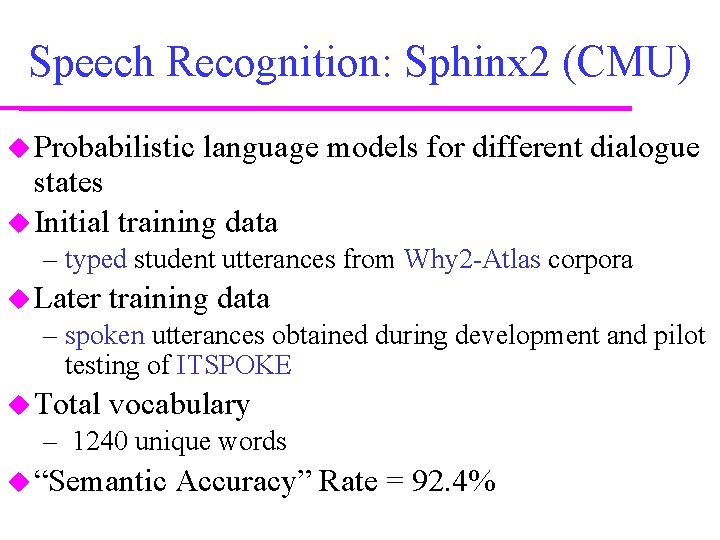
Speech Recognition: Sphinx 2 (CMU) Probabilistic language models for different dialogue states Initial training data – typed student utterances from Why 2 -Atlas corpora Later training data – spoken utterances obtained during development and pilot testing of ITSPOKE Total vocabulary – 1240 unique words “Semantic Accuracy” Rate = 92. 4%
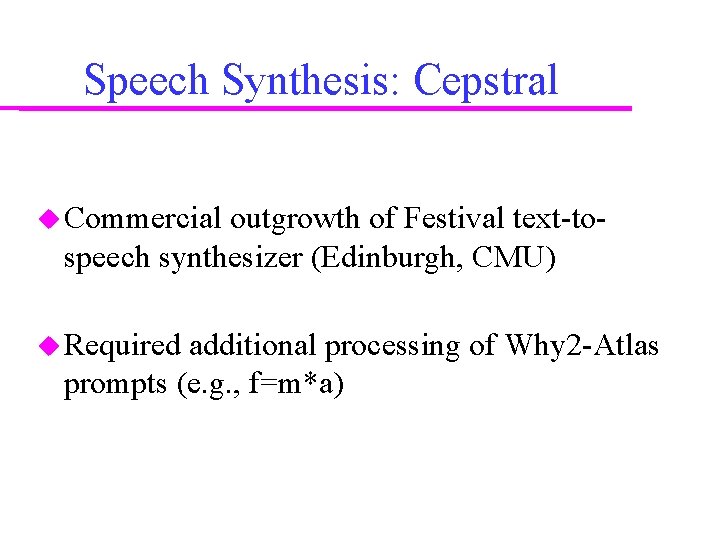
Speech Synthesis: Cepstral Commercial outgrowth of Festival text-tospeech synthesizer (Edinburgh, CMU) Required additional processing of Why 2 -Atlas prompts (e. g. , f=m*a)
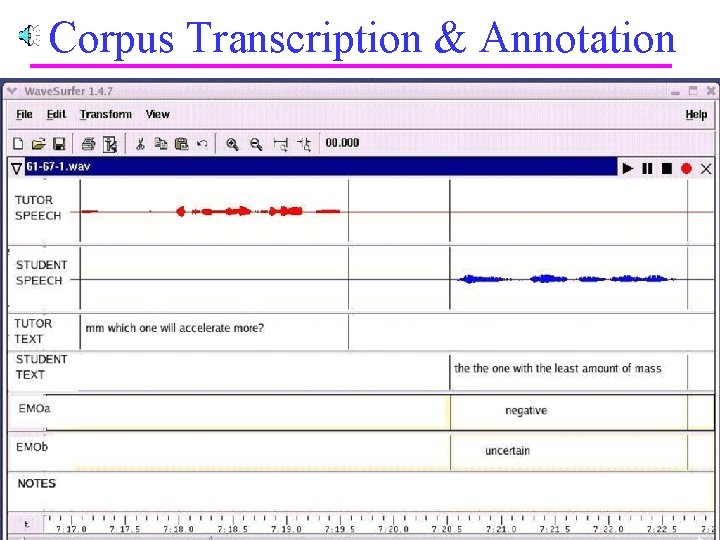
Corpus Transcription & Annotation
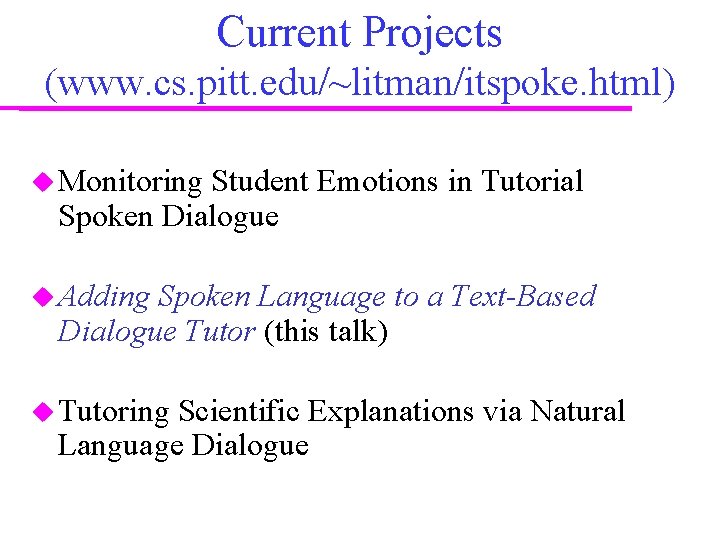
Current Projects (www. cs. pitt. edu/~litman/itspoke. html) Monitoring Student Emotions in Tutorial Spoken Dialogue Adding Spoken Language to a Text-Based Dialogue Tutor (this talk) Tutoring Scientific Explanations via Natural Language Dialogue
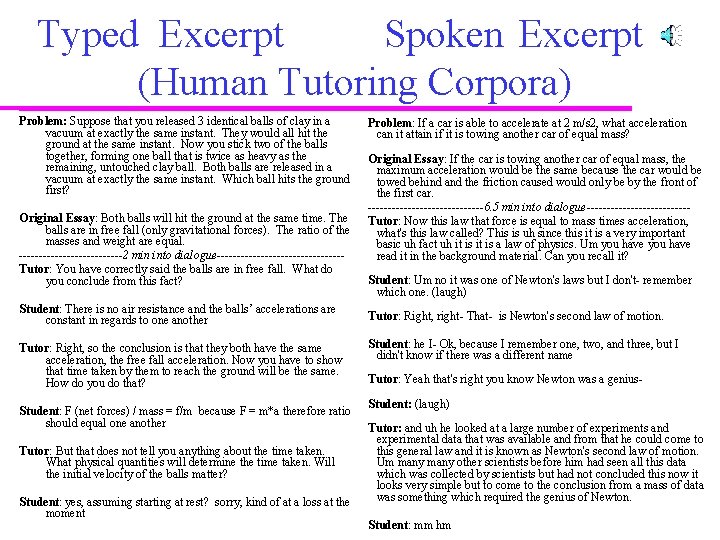
Typed Excerpt Spoken Excerpt (Human Tutoring Corpora) Problem: Suppose that you released 3 identical balls of clay in a vacuum at exactly the same instant. They would all hit the ground at the same instant. Now you stick two of the balls together, forming one ball that is twice as heavy as the remaining, untouched clay ball. Both balls are released in a vacuum at exactly the same instant. Which ball hits the ground first? Original Essay: Both balls will hit the ground at the same time. The balls are in free fall (only gravitational forces). The ratio of the masses and weight are equal. -------------2 min into dialogue----------------Tutor: You have correctly said the balls are in free fall. What do you conclude from this fact? Student: There is no air resistance and the balls’ accelerations are constant in regards to one another Tutor: Right, so the conclusion is that they both have the same acceleration, the free fall acceleration. Now you have to show that time taken by them to reach the ground will be the same. How do you do that? Student: F (net forces) / mass = f/m because F = m*a therefore ratio should equal one another Tutor: But that does not tell you anything about the time taken. What physical quantities will determine the time taken. Will the initial velocity of the balls matter? Student: yes, assuming starting at rest? sorry, kind of at a loss at the moment Problem: If a car is able to accelerate at 2 m/s 2, what acceleration can it attain if it is towing another car of equal mass? Original Essay: If the car is towing another car of equal mass, the maximum acceleration would be the same because the car would be towed behind and the friction caused would only be by the front of the first car. ---------------6. 5 min into dialogue-------------Tutor: Now this law that force is equal to mass times acceleration, what's this law called? This is uh since this it is a very important basic uh fact uh it is a law of physics. Um you have read it in the background material. Can you recall it? Student: Um no it was one of Newton's laws but I don't- remember which one. (laugh) Tutor: Right, right- That- is Newton's second law of motion. Student: he I- Ok, because I remember one, two, and three, but I didn't know if there was a different name Tutor: Yeah that's right you know Newton was a genius. Student: (laugh) Tutor: and uh he looked at a large number of experiments and experimental data that was available and from that he could come to this general law and it is known as Newton's second law of motion. Um many other scientists before him had seen all this data which was collected by scientists but had not concluded this now it looks very simple but to come to the conclusion from a mass of data was something which required the genius of Newton. Student: mm hm
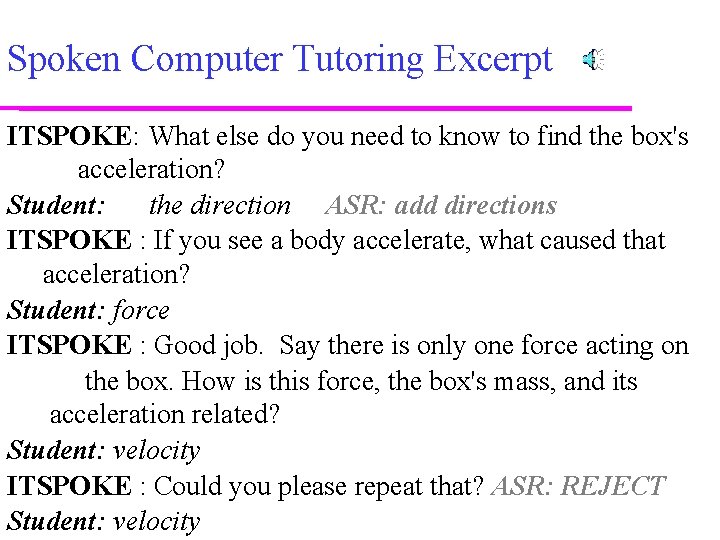
Spoken Computer Tutoring Excerpt ITSPOKE: What else do you need to know to find the box's acceleration? Student: the direction ASR: add directions ITSPOKE : If you see a body accelerate, what caused that acceleration? Student: force ITSPOKE : Good job. Say there is only one force acting on the box. How is this force, the box's mass, and its acceleration related? Student: velocity ITSPOKE : Could you please repeat that? ASR: REJECT Student: velocity
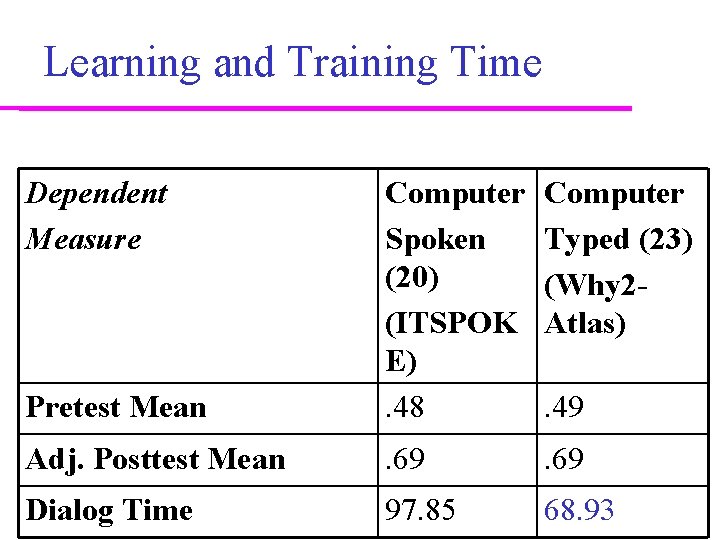
Learning and Training Time Dependent Measure Computer Typed (23) (Why 2 Atlas) Pretest Mean Computer Spoken (20) (ITSPOK E). 48 Adj. Posttest Mean . 69 Dialog Time 97. 85 68. 93 . 49
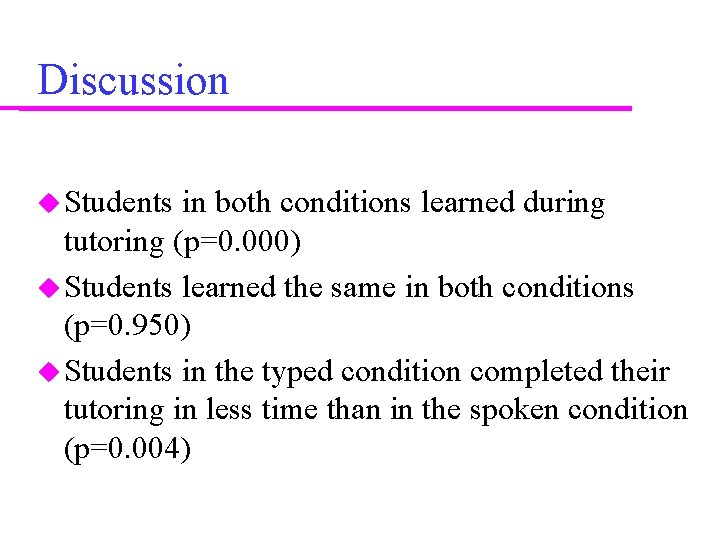
Discussion Students in both conditions learned during tutoring (p=0. 000) Students learned the same in both conditions (p=0. 950) Students in the typed condition completed their tutoring in less time than in the spoken condition (p=0. 004)
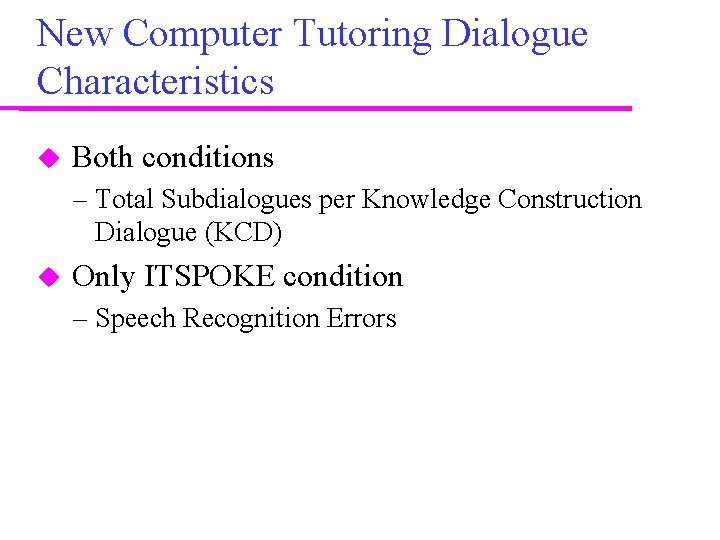
New Computer Tutoring Dialogue Characteristics Both conditions – Total Subdialogues per Knowledge Construction Dialogue (KCD) Only ITSPOKE condition – Speech Recognition Errors
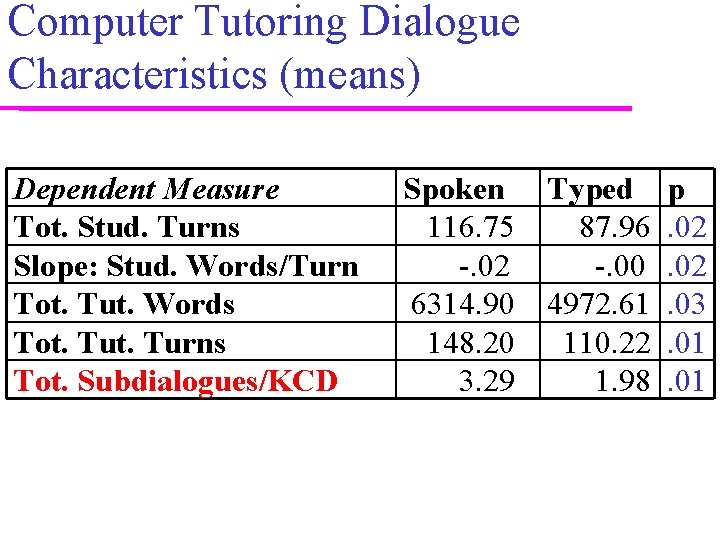
Computer Tutoring Dialogue Characteristics (means) Dependent Measure Tot. Stud. Turns Slope: Stud. Words/Turn Tot. Tut. Words Tot. Turns Tot. Subdialogues/KCD Spoken 116. 75 -. 02 6314. 90 148. 20 3. 29 Typed 87. 96 -. 00 4972. 61 110. 22 1. 98 p. 02. 03. 01
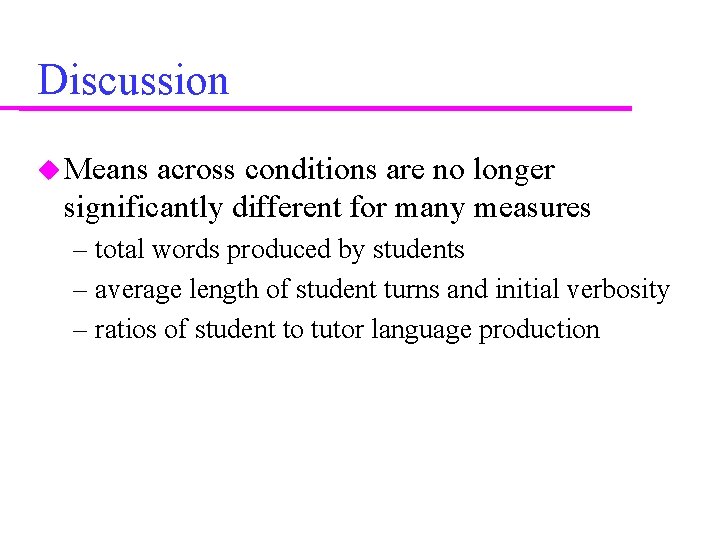
Discussion Means across conditions are no longer significantly different for many measures – total words produced by students – average length of student turns and initial verbosity – ratios of student to tutor language production
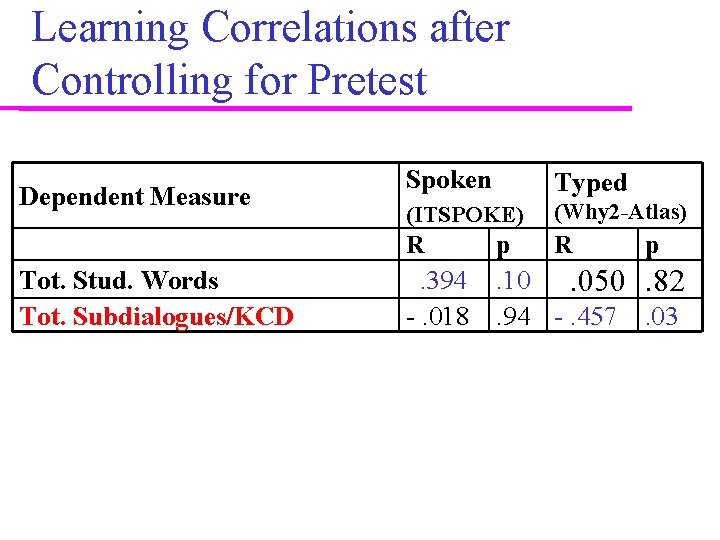
Learning Correlations after Controlling for Pretest Dependent Measure Tot. Stud. Words Tot. Subdialogues/KCD Spoken Typed (ITSPOKE) (Why 2 -Atlas) R. 394 -. 018 p R p. 10. 050. 82. 94 -. 457. 03
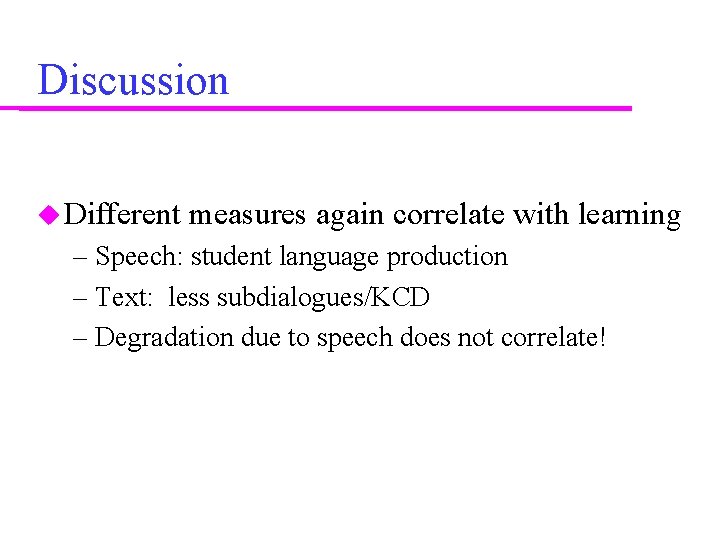
Discussion Different measures again correlate with learning – Speech: student language production – Text: less subdialogues/KCD – Degradation due to speech does not correlate!
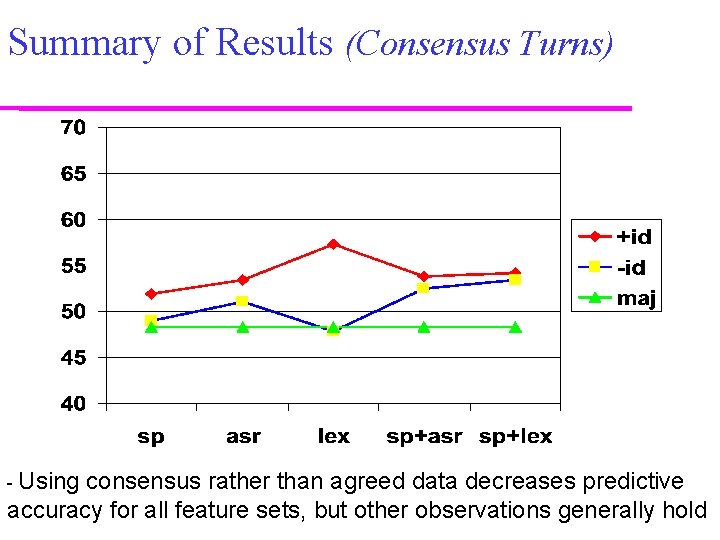
Summary of Results (Consensus Turns) - Using consensus rather than agreed data decreases predictive accuracy for all feature sets, but other observations generally hold
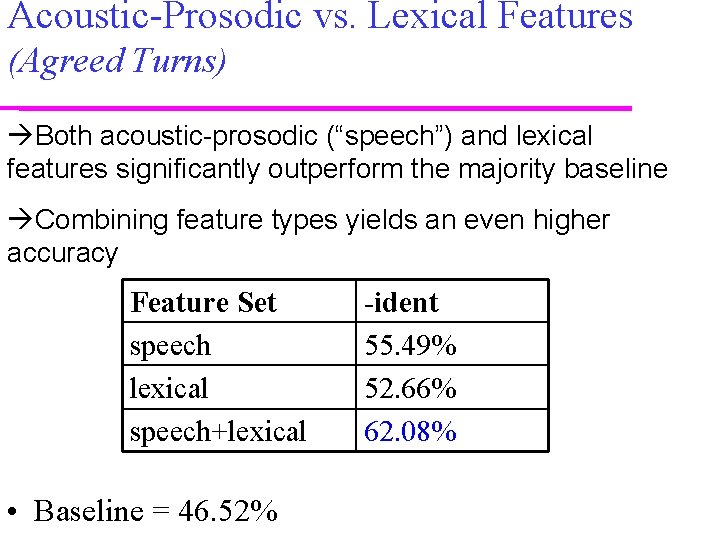
Acoustic-Prosodic vs. Lexical Features (Agreed Turns) Both acoustic-prosodic (“speech”) and lexical features significantly outperform the majority baseline Combining feature types yields an even higher accuracy Feature Set speech lexical speech+lexical • Baseline = 46. 52% -ident 55. 49% 52. 66% 62. 08%
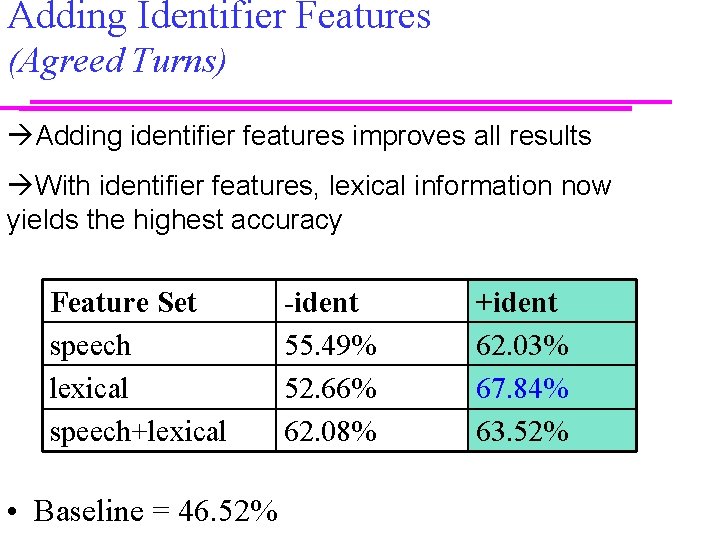
Adding Identifier Features (Agreed Turns) Adding identifier features improves all results With identifier features, lexical information now yields the highest accuracy Feature Set speech lexical speech+lexical • Baseline = 46. 52% -ident 55. 49% 52. 66% 62. 08% +ident 62. 03% 67. 84% 63. 52%
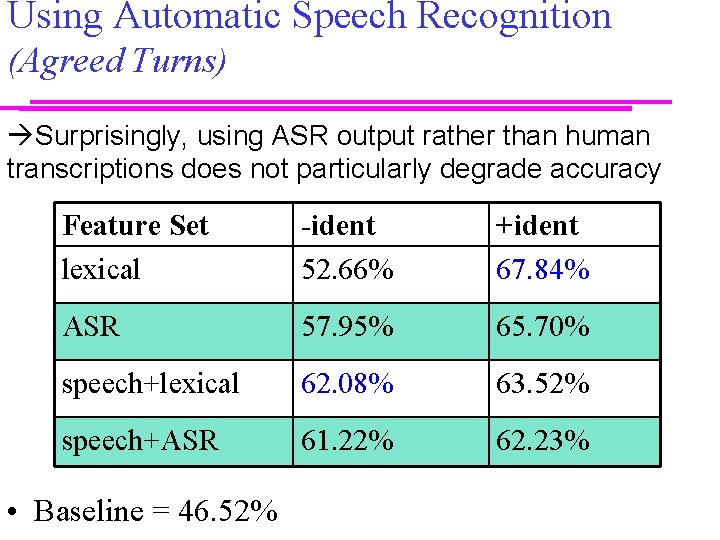
Using Automatic Speech Recognition (Agreed Turns) Surprisingly, using ASR output rather than human transcriptions does not particularly degrade accuracy Feature Set lexical -ident 52. 66% +ident 67. 84% ASR 57. 95% 65. 70% speech+lexical 62. 08% 63. 52% speech+ASR 61. 22% 62. 23% • Baseline = 46. 52%
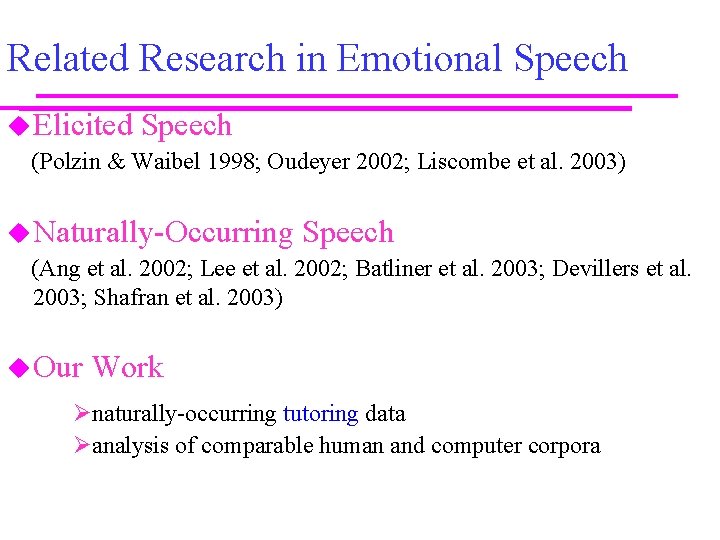
Related Research in Emotional Speech Elicited Speech (Polzin & Waibel 1998; Oudeyer 2002; Liscombe et al. 2003) Naturally-Occurring Speech (Ang et al. 2002; Lee et al. 2002; Batliner et al. 2003; Devillers et al. 2003; Shafran et al. 2003) Our Work Ønaturally-occurring tutoring data Øanalysis of comparable human and computer corpora
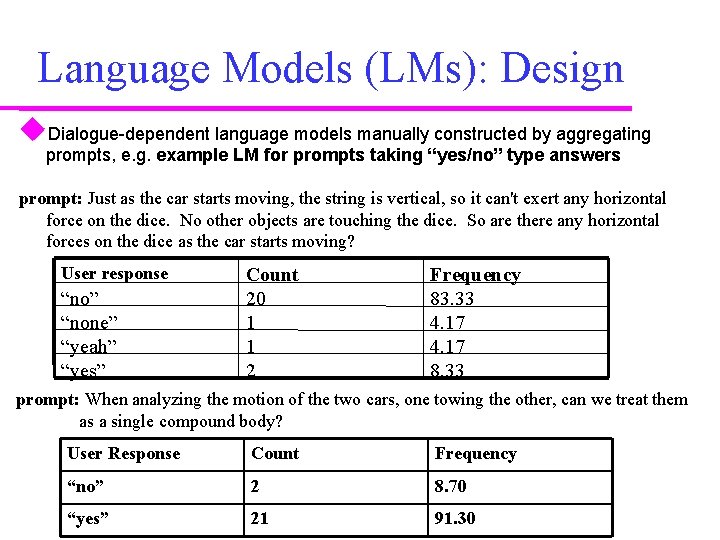
Language Models (LMs): Design Dialogue-dependent language models manually constructed by aggregating prompts, e. g. example LM for prompts taking “yes/no” type answers prompt: Just as the car starts moving, the string is vertical, so it can't exert any horizontal force on the dice. No other objects are touching the dice. So are there any horizontal forces on the dice as the car starts moving? User response “no” “none” “yeah” “yes” Count 20 1 1 2 Frequency 83. 33 4. 17 8. 33 prompt: When analyzing the motion of the two cars, one towing the other, can we treat them as a single compound body? User Response Count Frequency “no” 2 8. 70 “yes” 21 91. 30
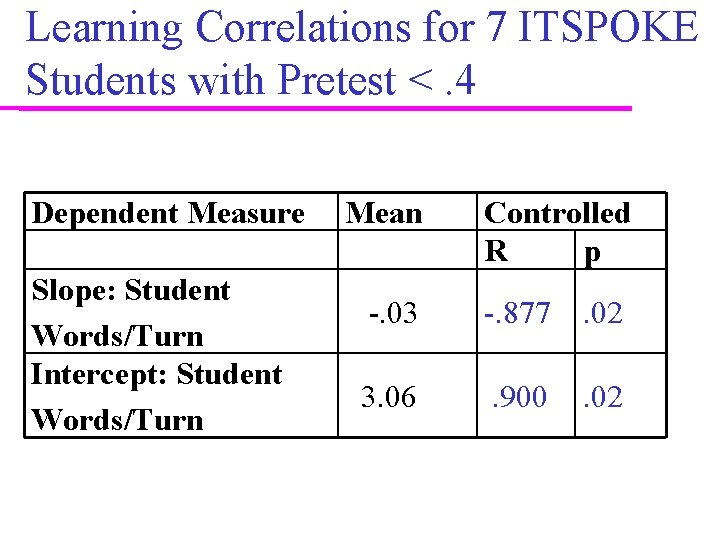
Learning Correlations for 7 ITSPOKE Students with Pretest <. 4 Dependent Measure Slope: Student Words/Turn Intercept: Student Words/Turn Mean Controlled R p -. 03 -. 877. 02 3. 06 . 900 . 02
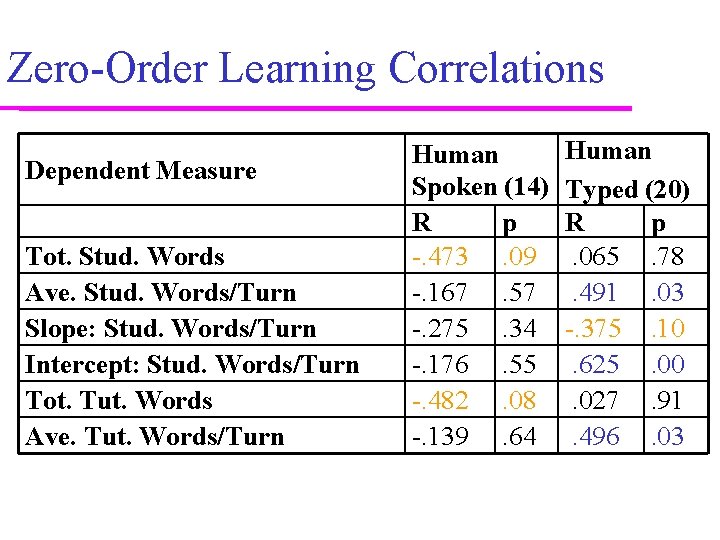
Zero-Order Learning Correlations Dependent Measure Tot. Stud. Words Ave. Stud. Words/Turn Slope: Stud. Words/Turn Intercept: Stud. Words/Turn Tot. Tut. Words Ave. Tut. Words/Turn Human Spoken (14) R p -. 473. 09 -. 167. 57 -. 275. 34 -. 176. 55 -. 482. 08 -. 139. 64 Human Typed (20) R p. 065. 78. 491. 03 -. 375. 10. 625. 00. 027. 91. 496. 03
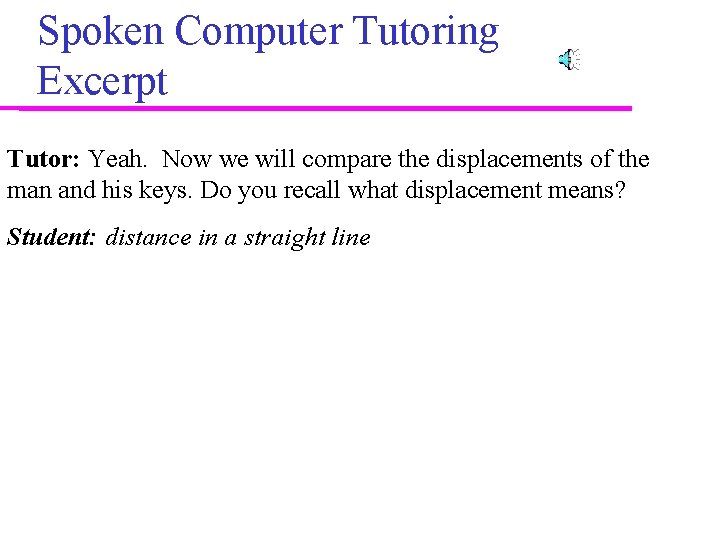
Spoken Computer Tutoring Excerpt Tutor: Yeah. Now we will compare the displacements of the man and his keys. Do you recall what displacement means? Student: distance in a straight line
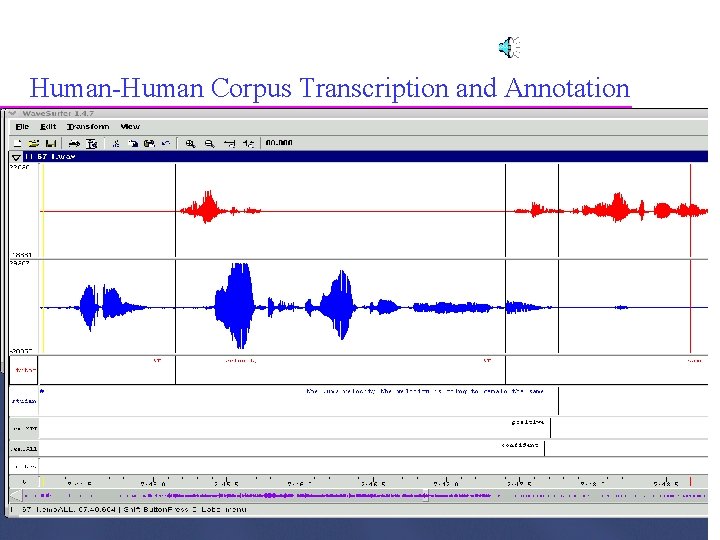
Human-Human Corpus Transcription and Annotation
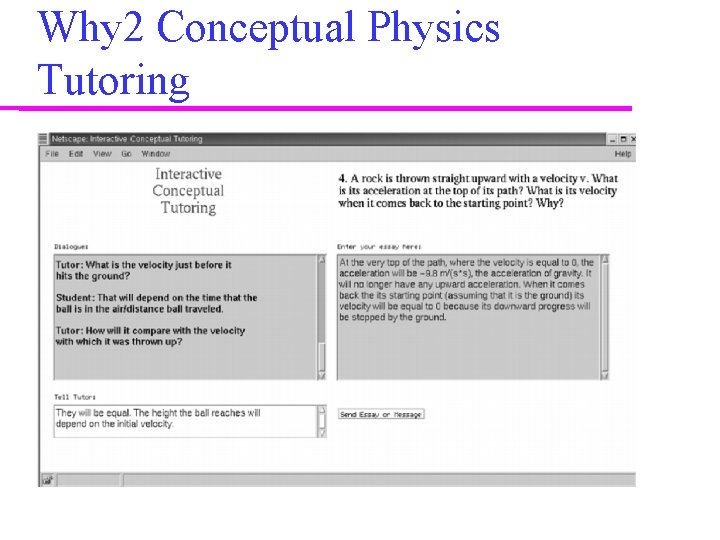
Why 2 Conceptual Physics Tutoring
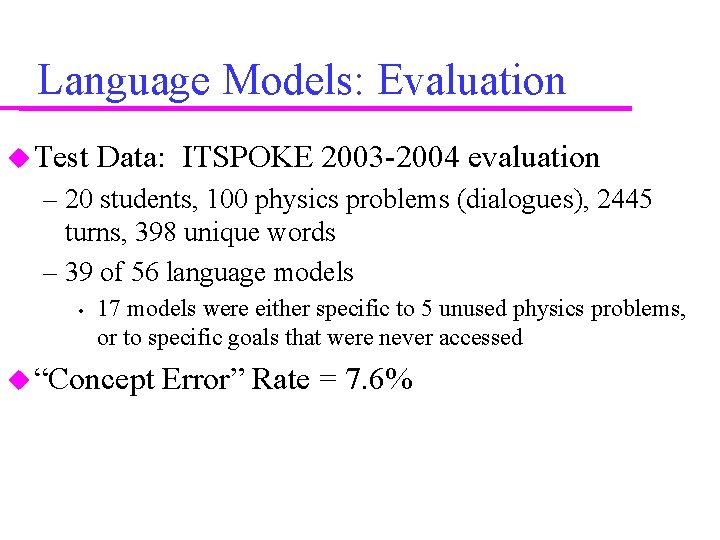
Language Models: Evaluation Test Data: ITSPOKE 2003 -2004 evaluation – 20 students, 100 physics problems (dialogues), 2445 turns, 398 unique words – 39 of 56 language models • 17 models were either specific to 5 unused physics problems, or to specific goals that were never accessed “Concept Error” Rate = 7. 6%
Decision support systems and intelligent systems
What is congratulation expression
Intelligent lectern systems
Ipmi architecture
Isys intelligent systems
Core intelligent systems platform
Intelligent systems for molecular biology
How will information systems affect business careers
How will information systems affect business careers
Richard song uri
Smarty cats drop in tutoring
Scuola leonardo da vinci parigi
Tutoring definicja
Ksu ccse tutoring
Scholarly tutoring
Stanford vptl tutoring
Tutoring
Tutoring
Msi tutoring ucsc
Mutuo insegnamento scuola primaria
Tutoring
Bcc tutoring
Accuplacer tutoring
Tutoring
Media hopper replay
Small group tutoring
Youtube.com
Wise tutoring
Academic engagement
Tutoring cycle
Aspire tutoring
Connective tutoring
Tutoring čechy
Uci antplanner
Wise ant tutoring
Tutoring cycle
Tutoring shawlands
Kindergarten tutoring
Cdli tutoring
Rpi alac tutoring
Uri aec tutoring
Chinese tutoring
Reported speech matching exercises
Pure speech vs symbolic speech
Phrasing on reported speech (1) answers
Reported speech present simple to past simple
What is direct and indirect speech with examples
Reported speech exercises doc
Quoted speech to reported speech
New en comparative and superlative
Comparative and superlative warm
Conventional computing and intelligent computing
Speech to the progress toward
Informative vs persuasive
Wh direct and indirect speech
We to indirect speech
Speech to the progress toward
Direct speech act example
Present simple in reported speech
Direct speech examples
Persuasive speech vs informative speech
Reported speech ask
Direct speech into reported speech
They are playing football
50 examples of direct and indirect speech
Reported speech sujetos
Speech privacy systems llc
Dicapine
Embedded systems vs cyber physical systems
Engineering elegant systems: theory of systems engineering
Any time interrogation call flow
Definition of intelligence
Vni2140
Intelligent sharing of power is done among:
Intelligent customer routing
Contoh peas