PSY 368 Human Memory Semantic Memory Announcements Due
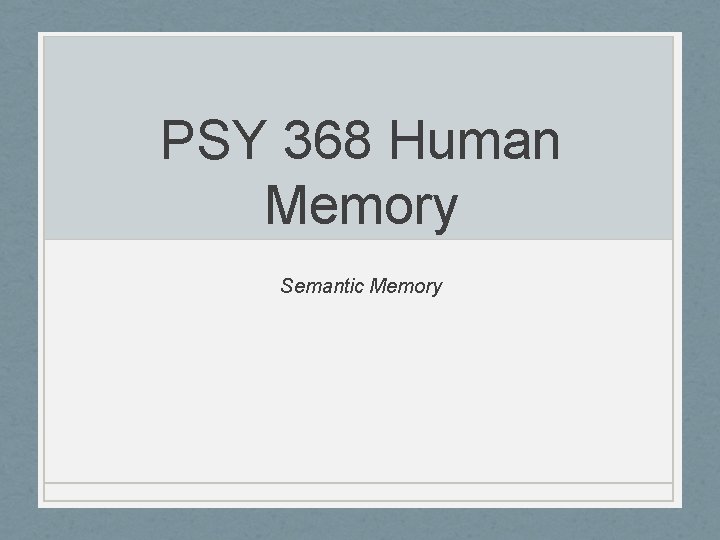
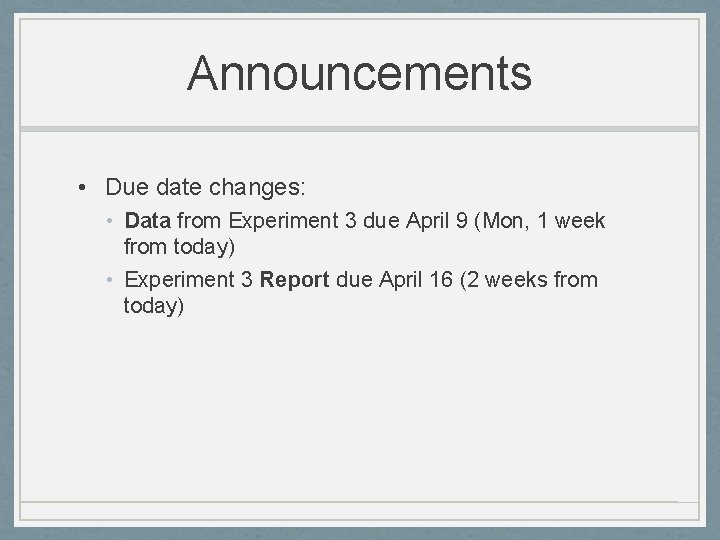
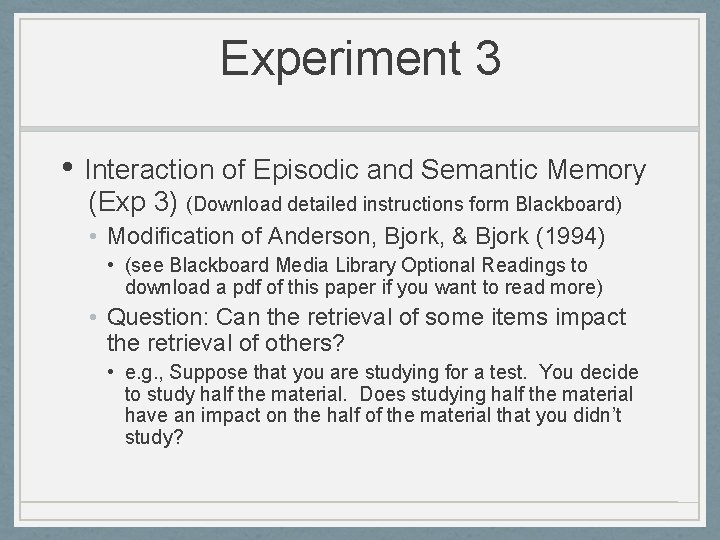
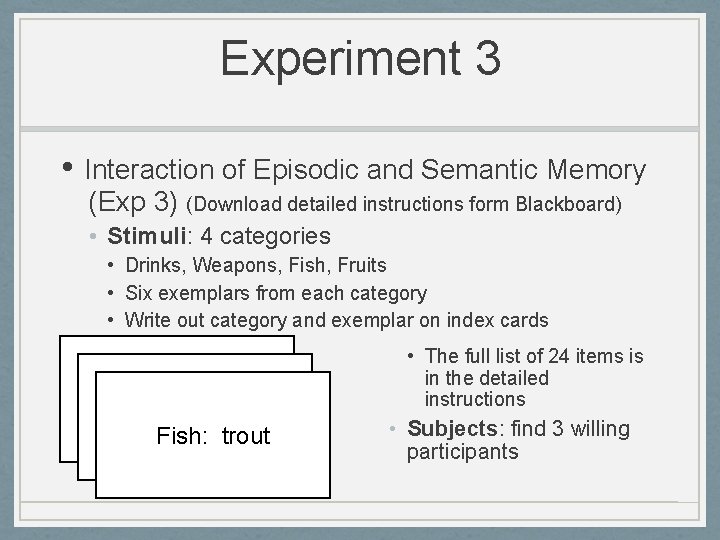
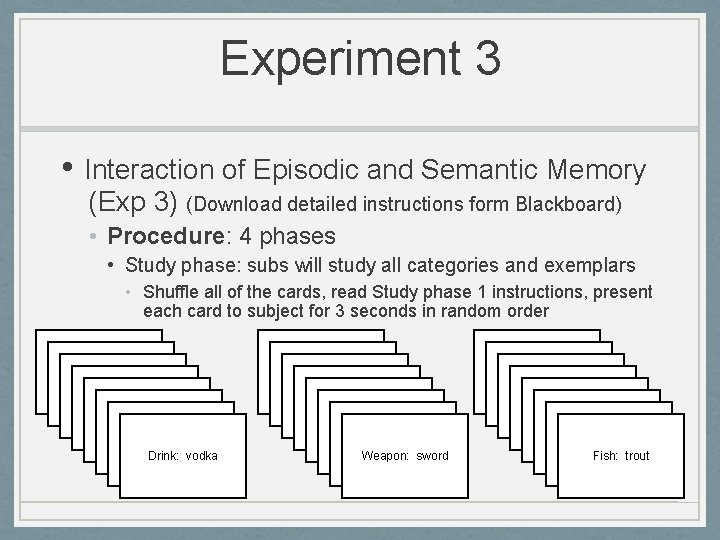
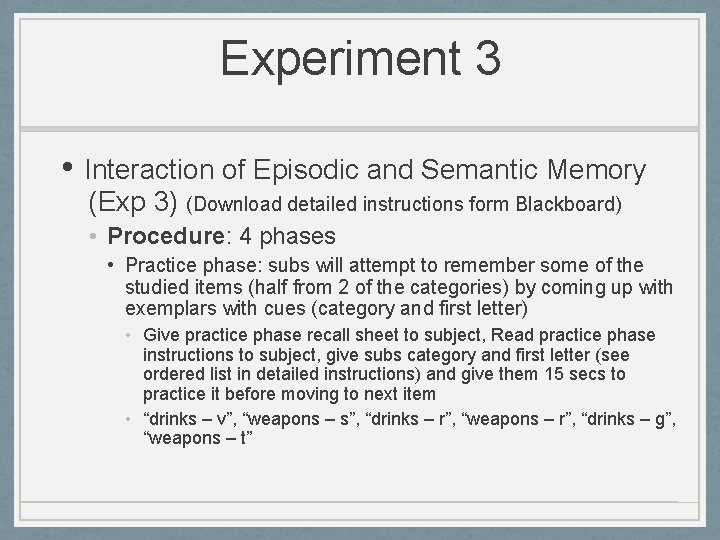
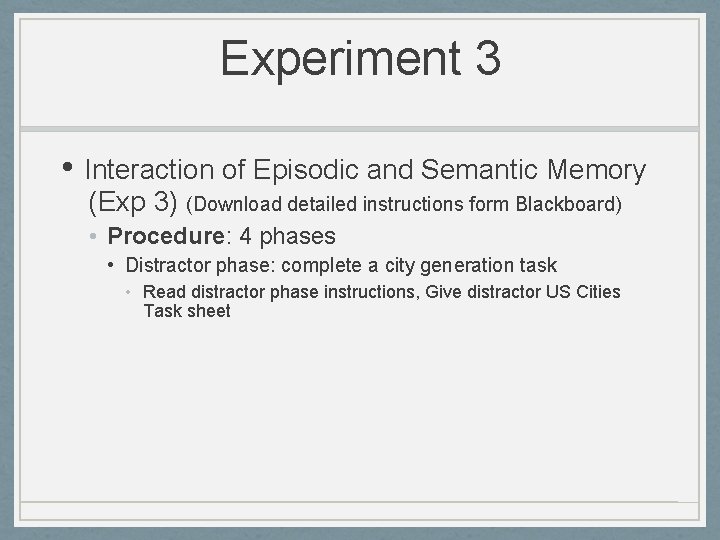
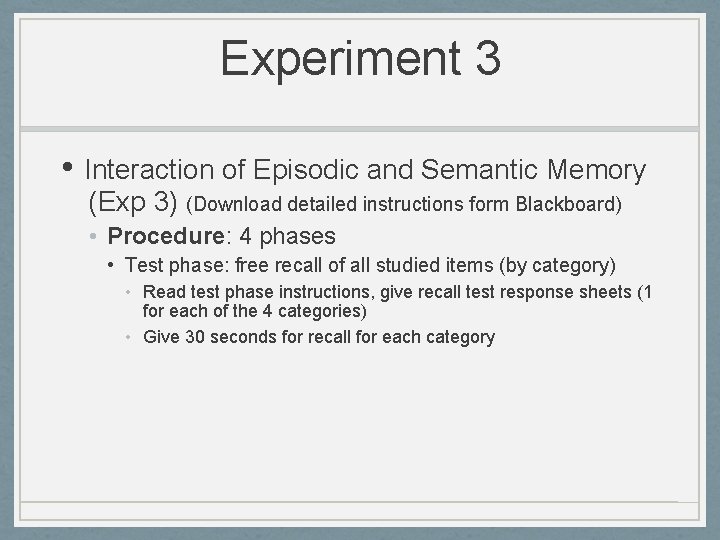
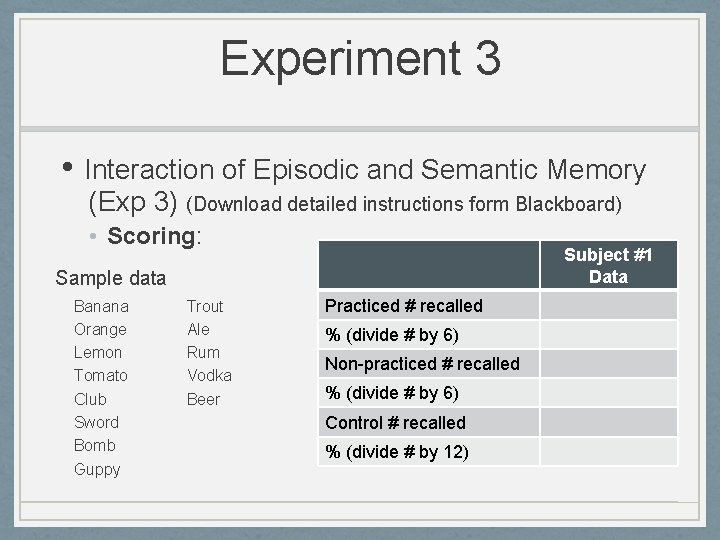
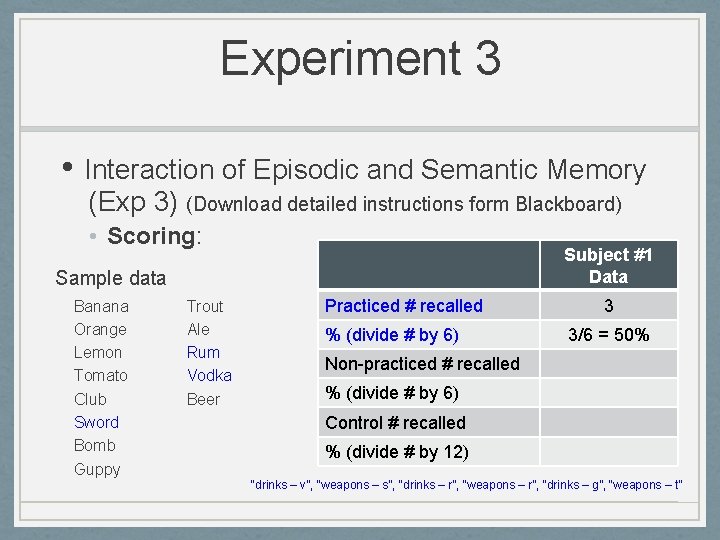
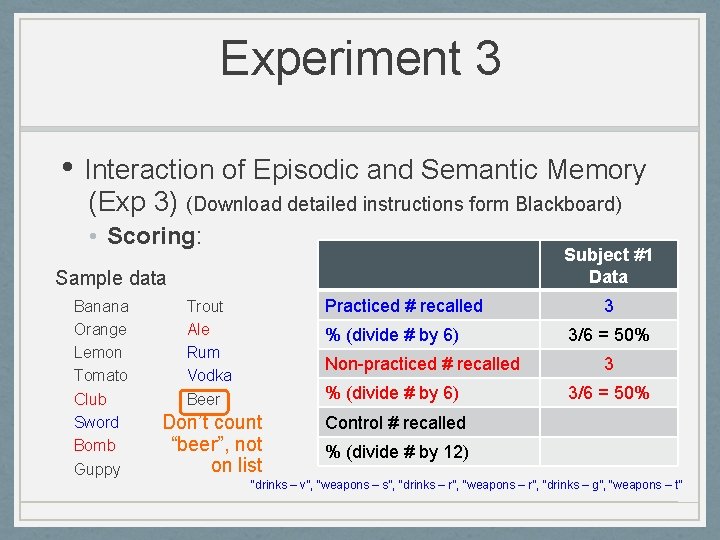
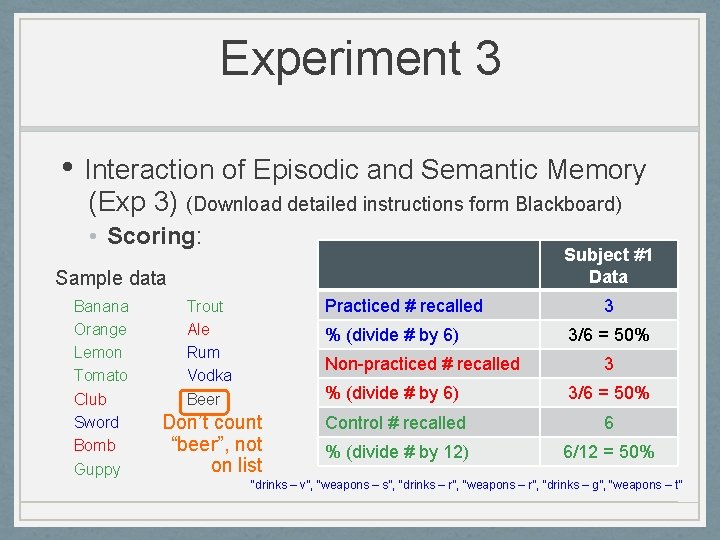
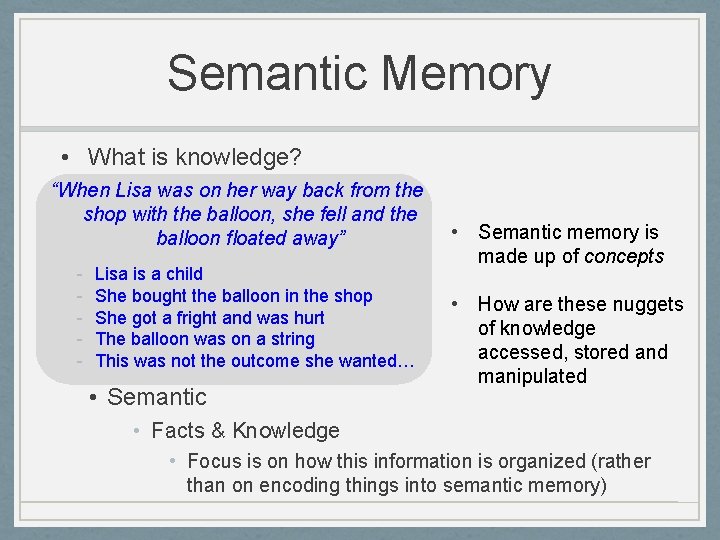
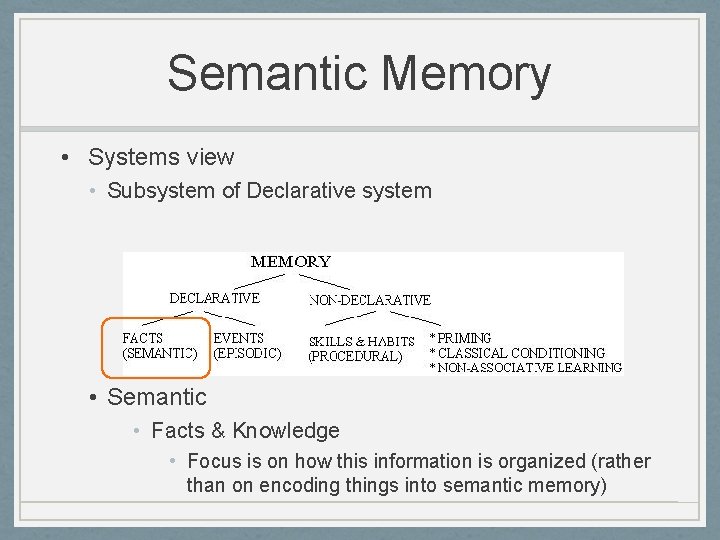
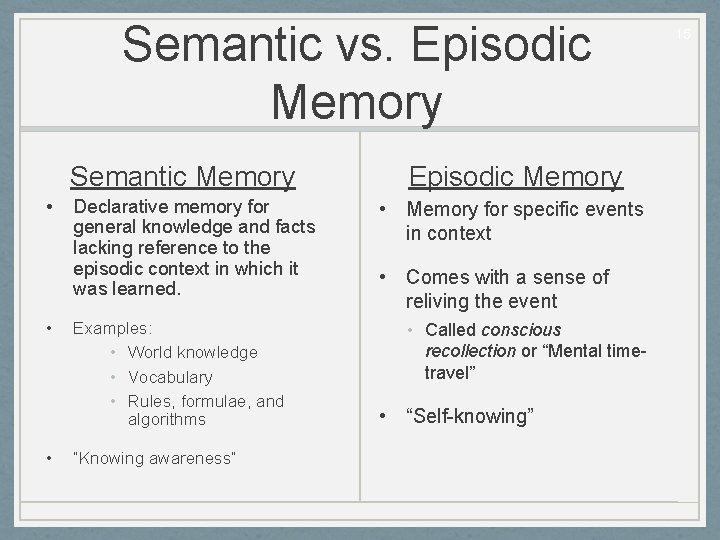
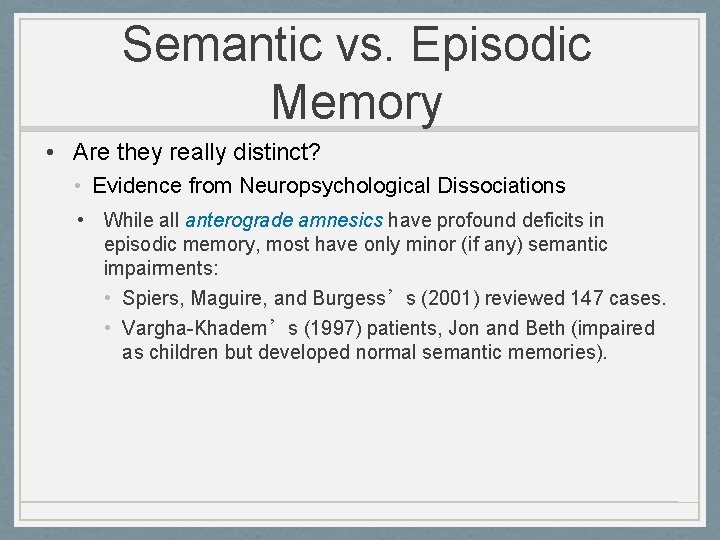
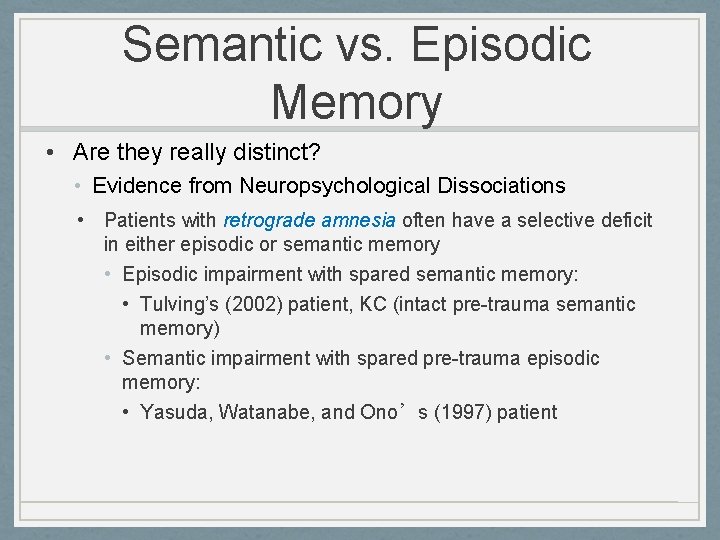
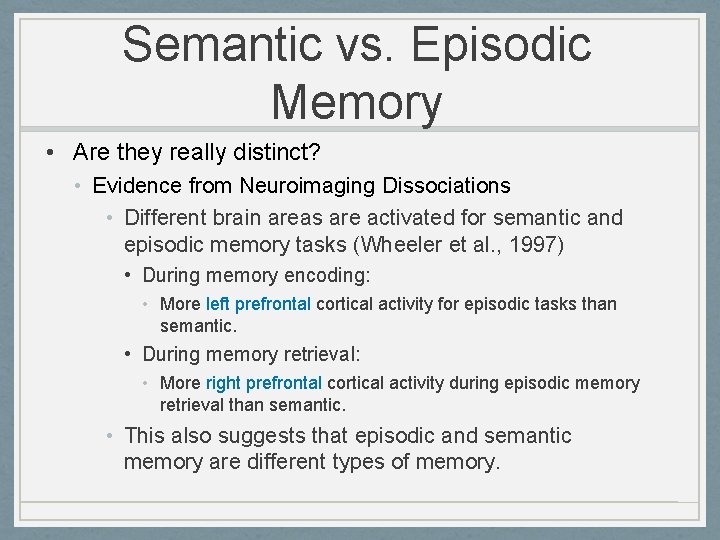
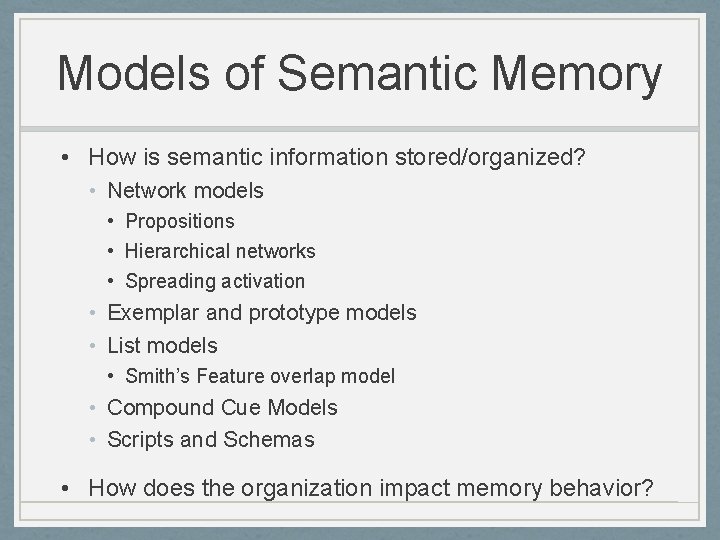
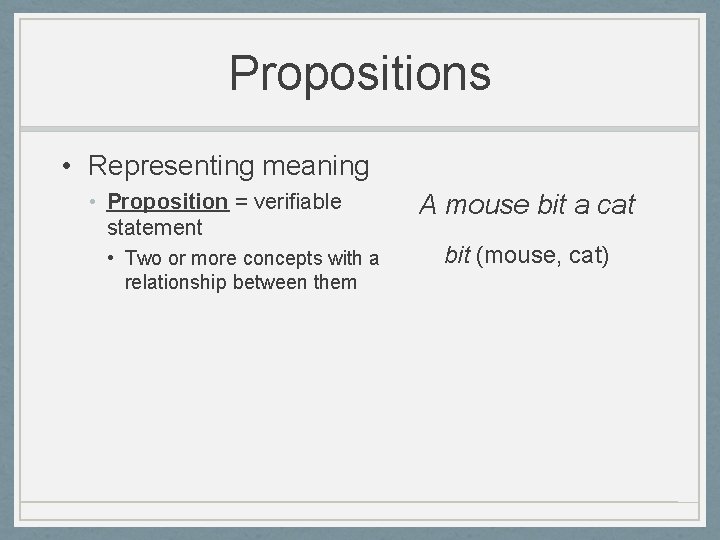
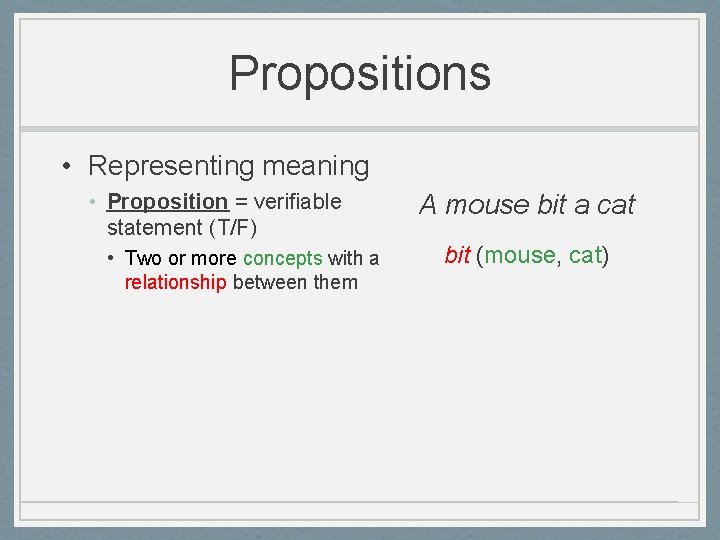
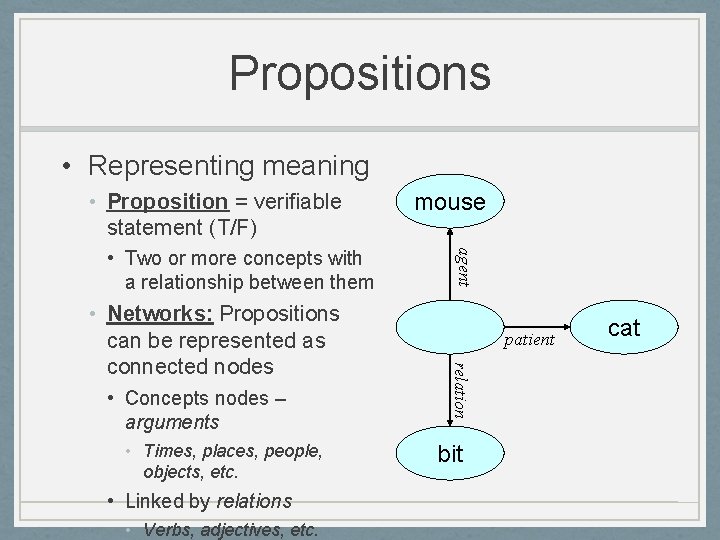
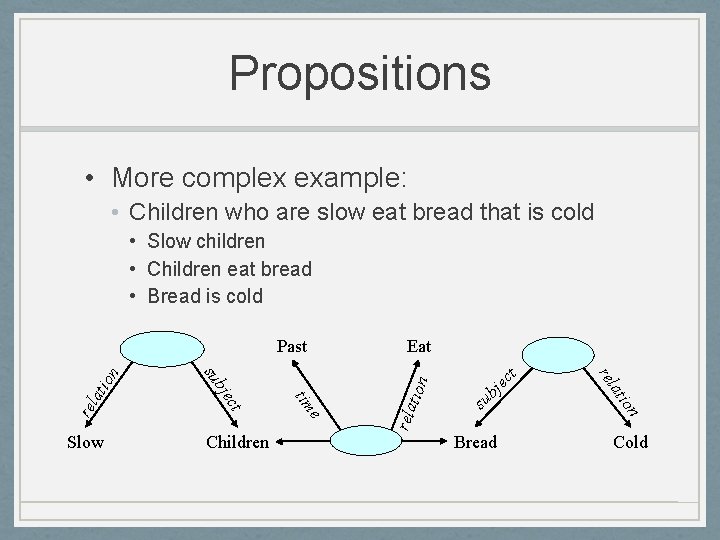
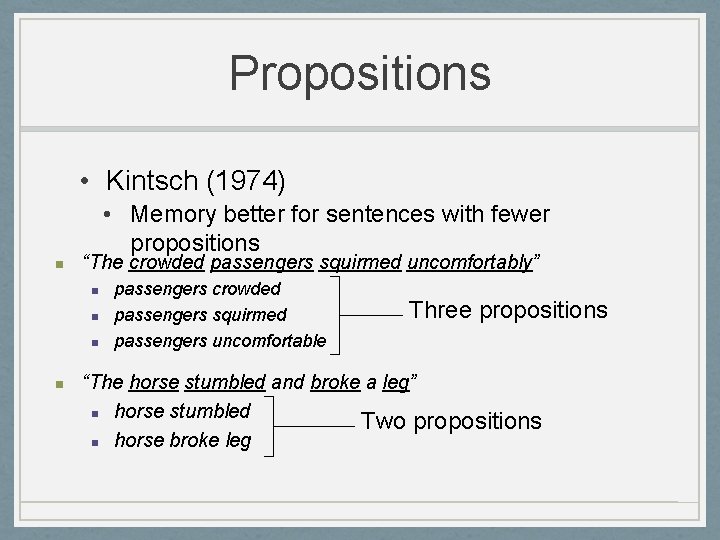
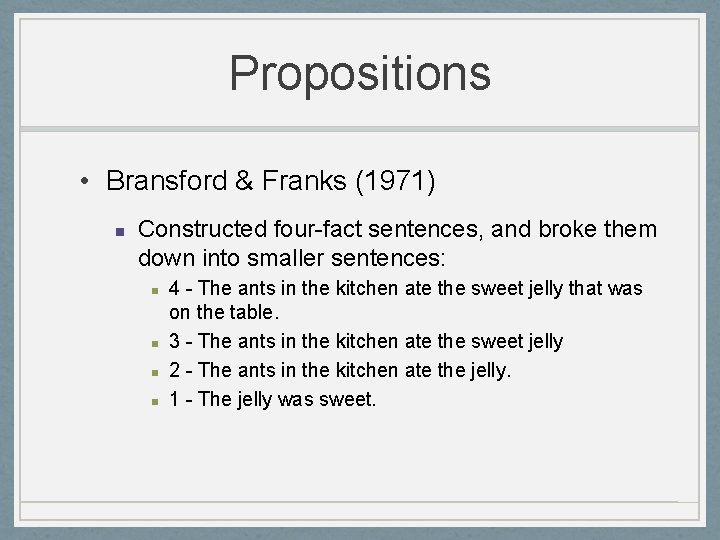
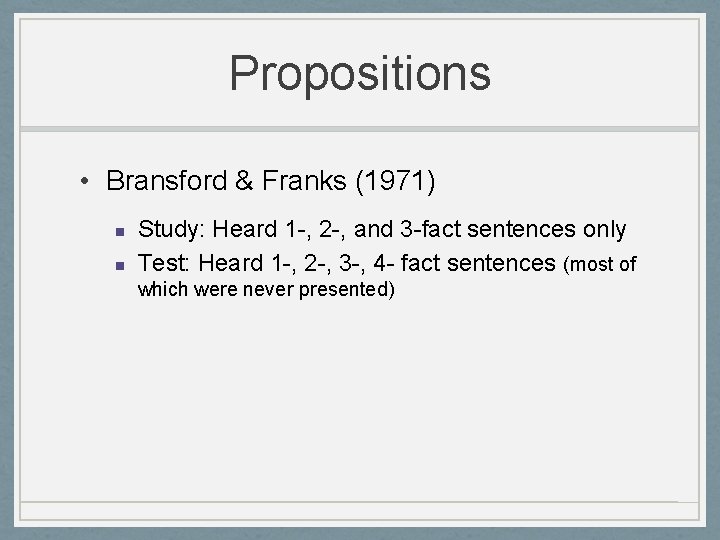
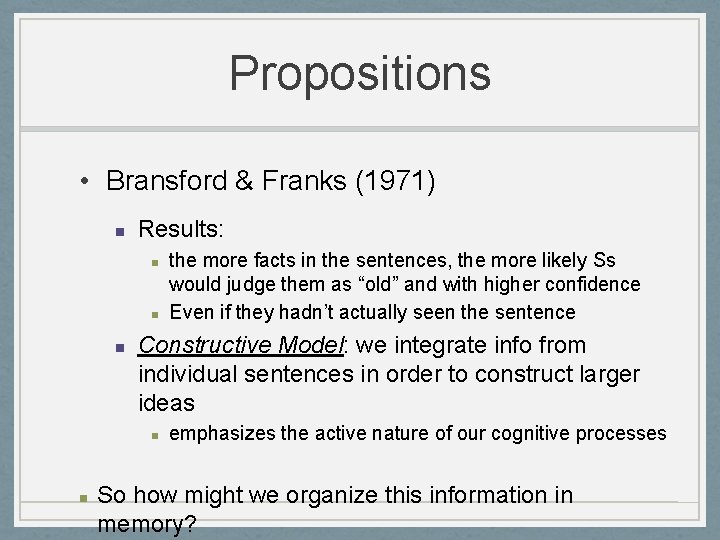
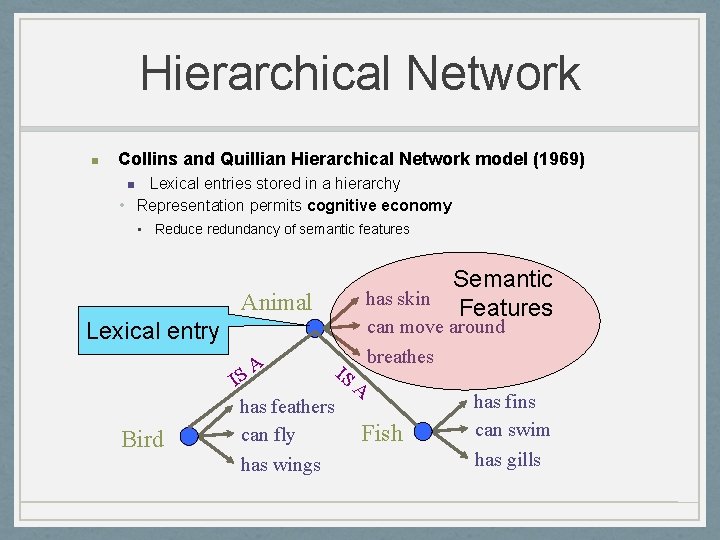
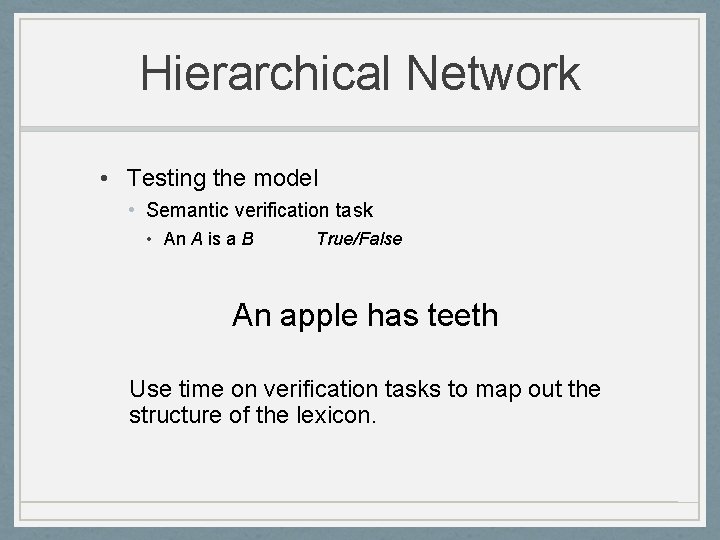
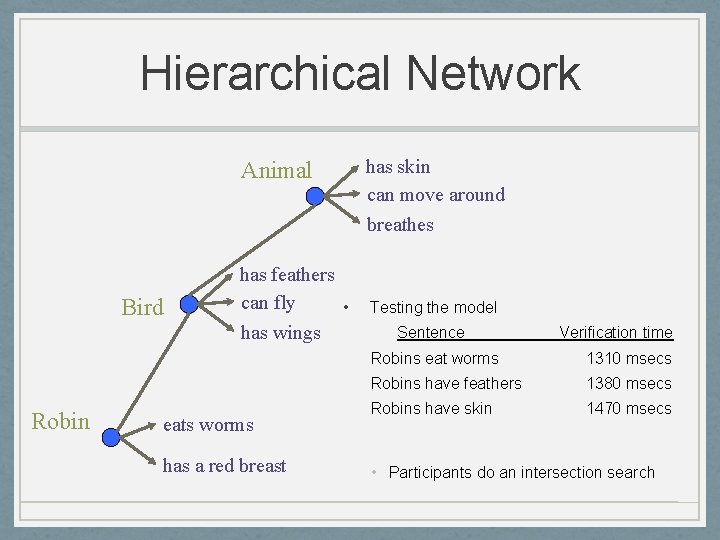
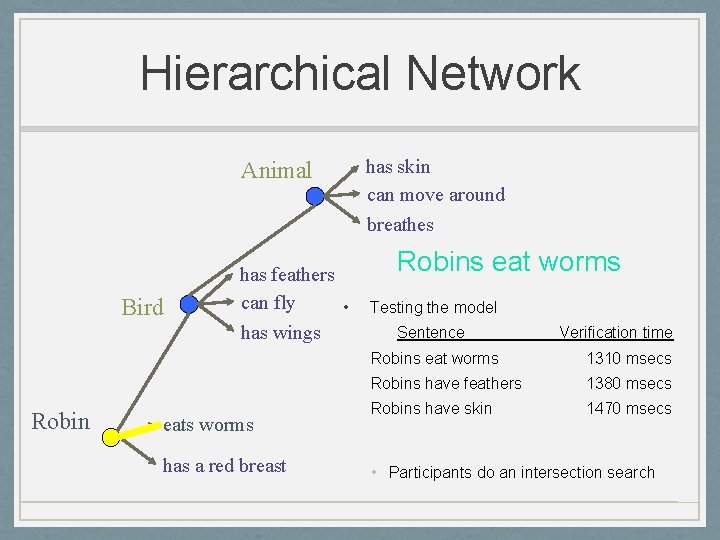
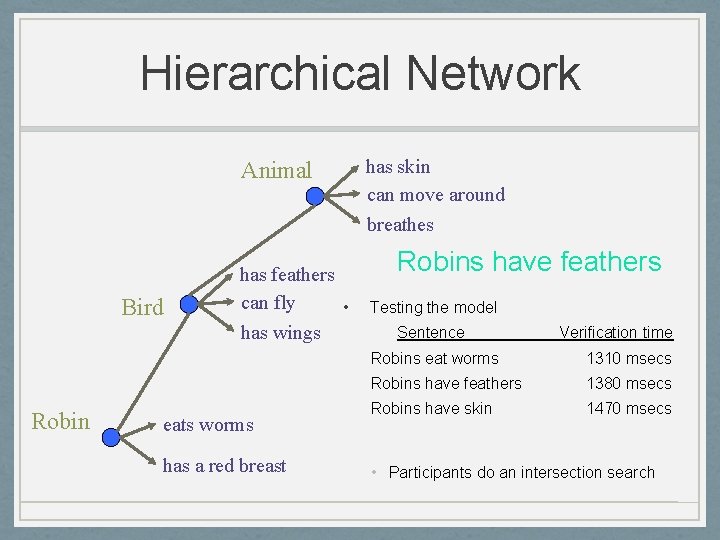
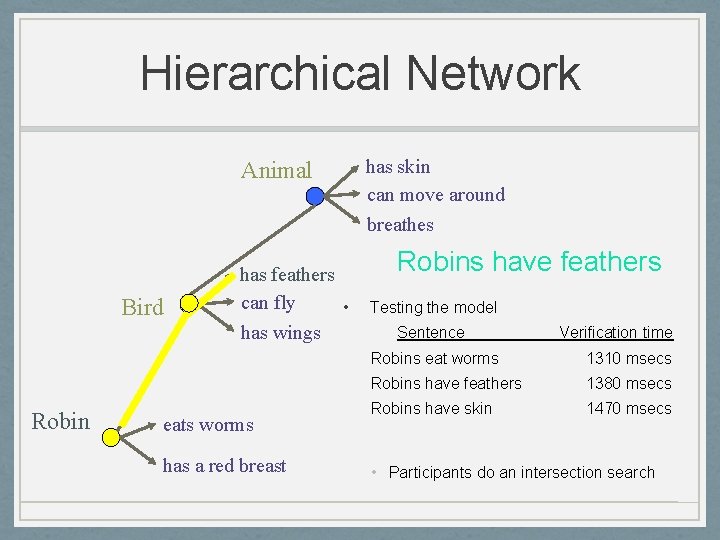
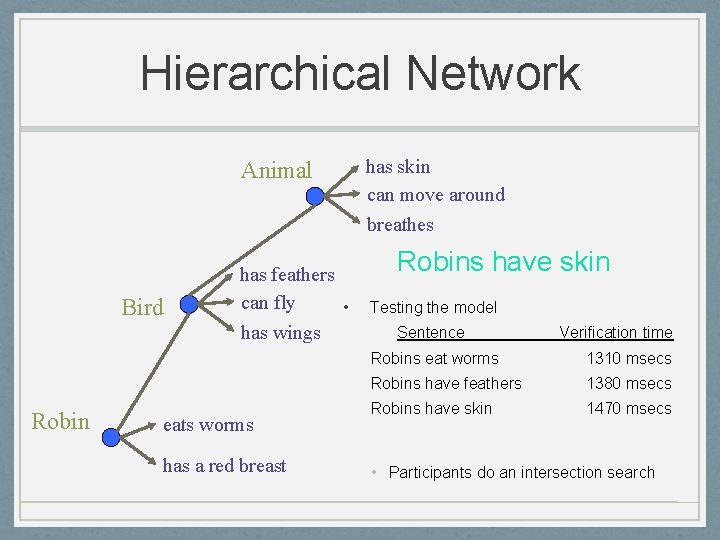
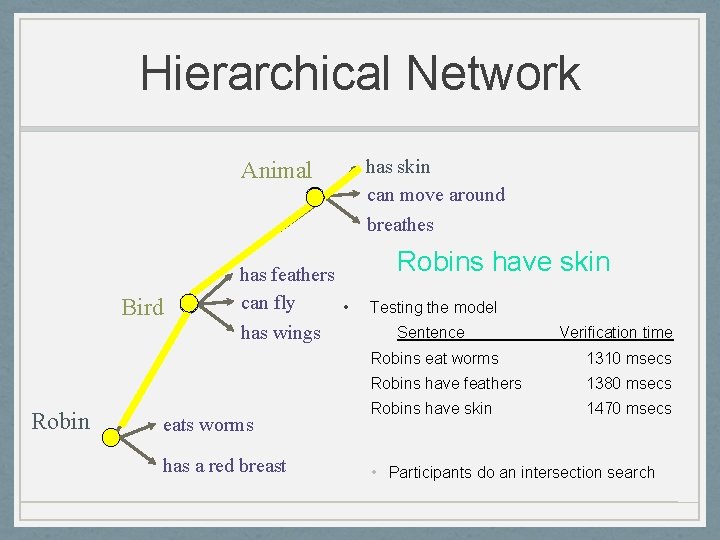
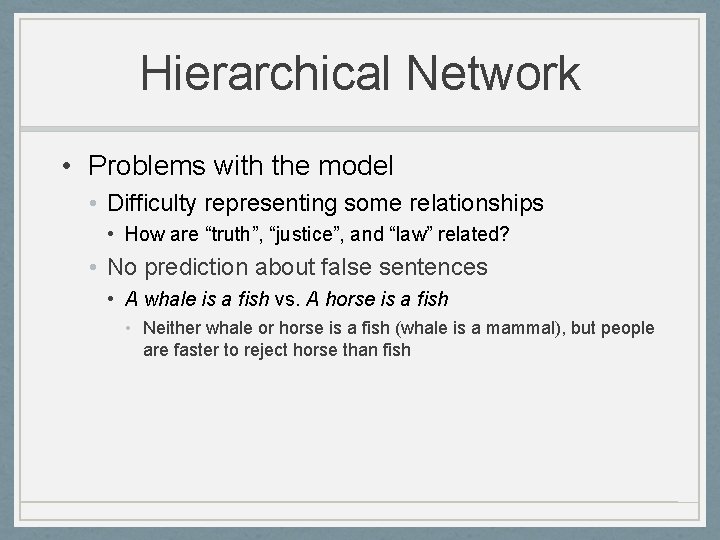
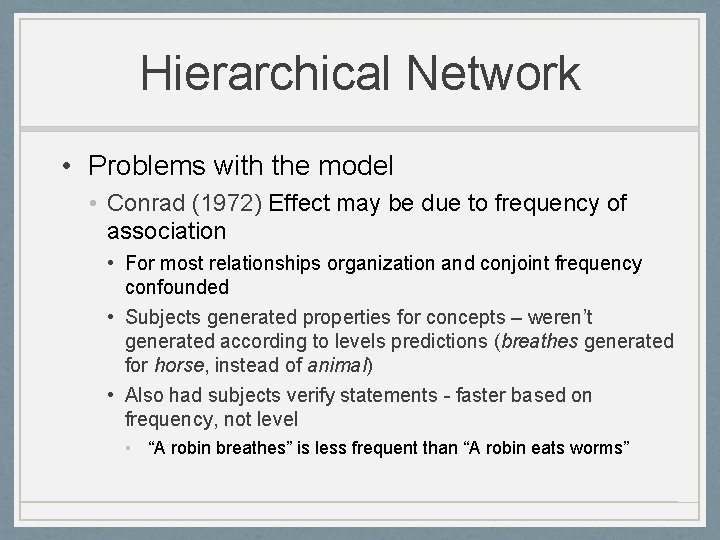
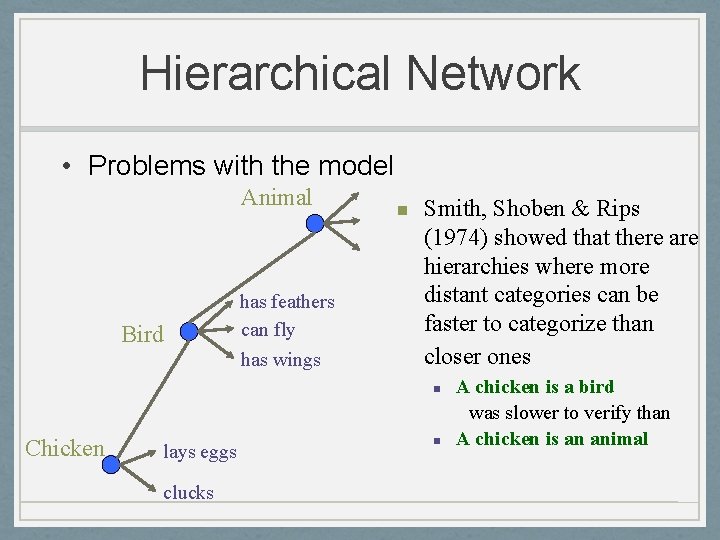
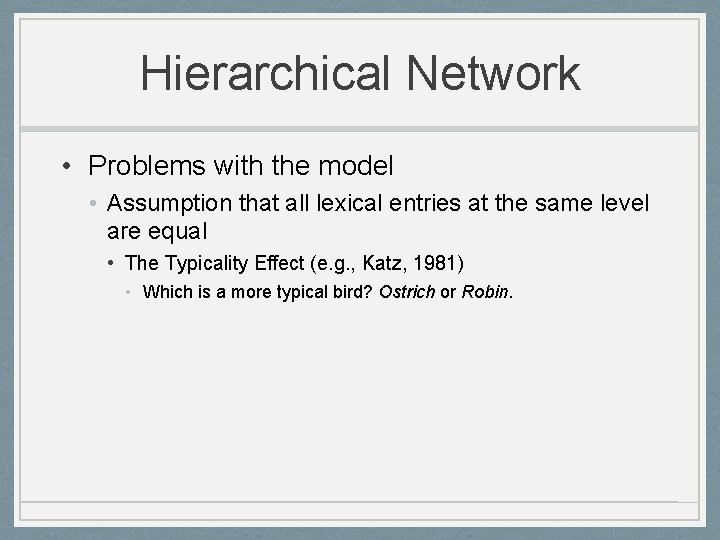
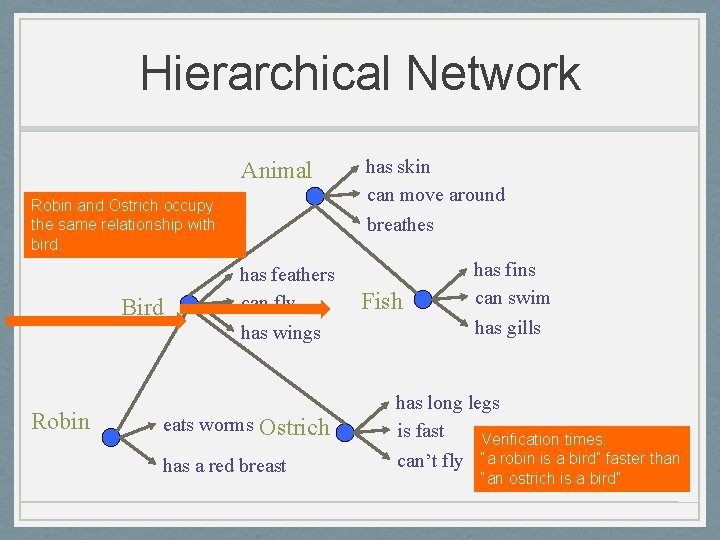
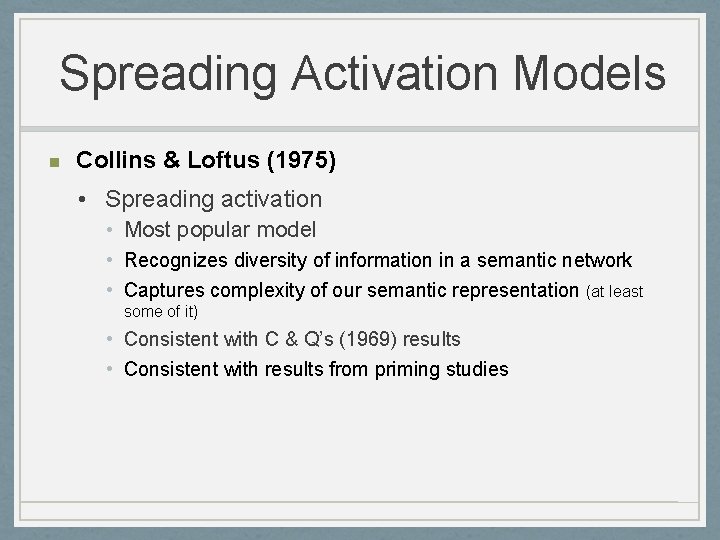
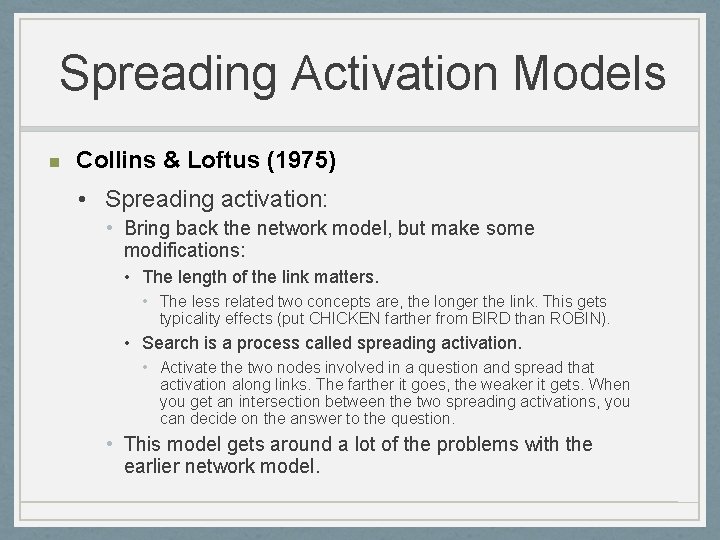
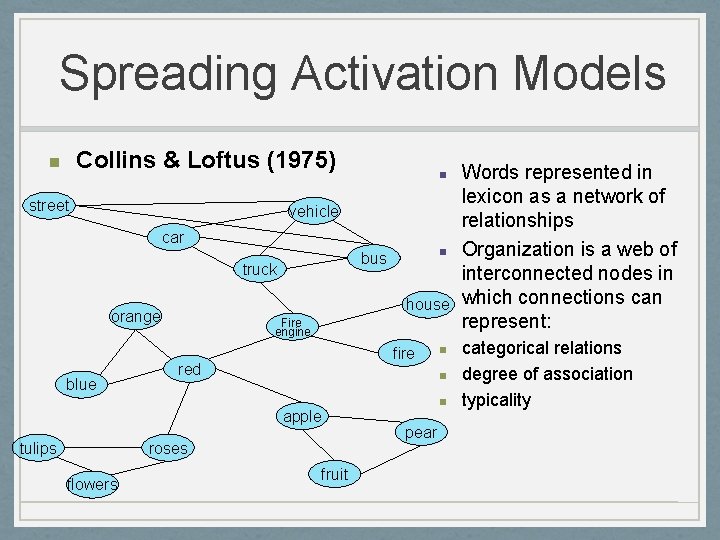
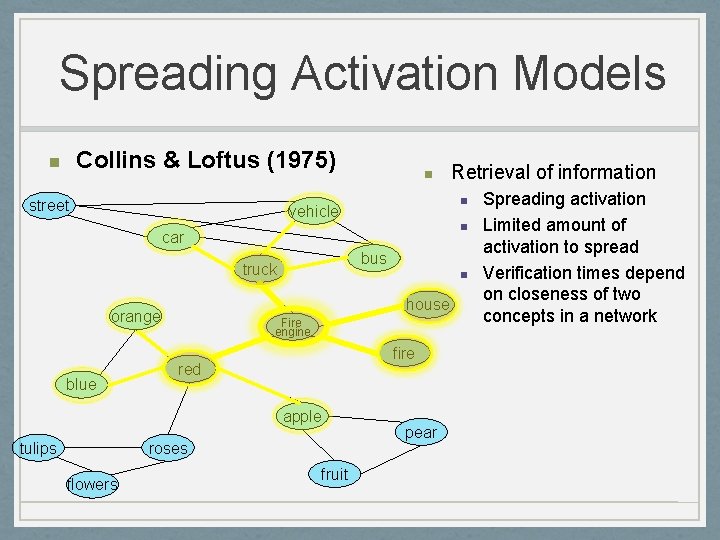
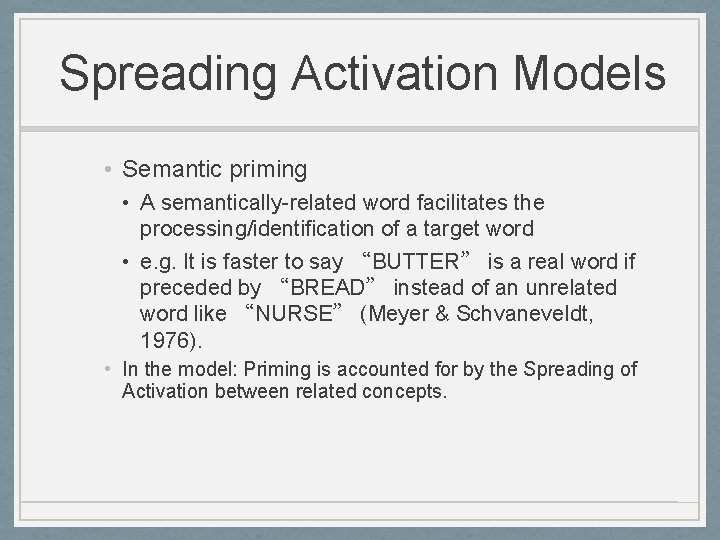
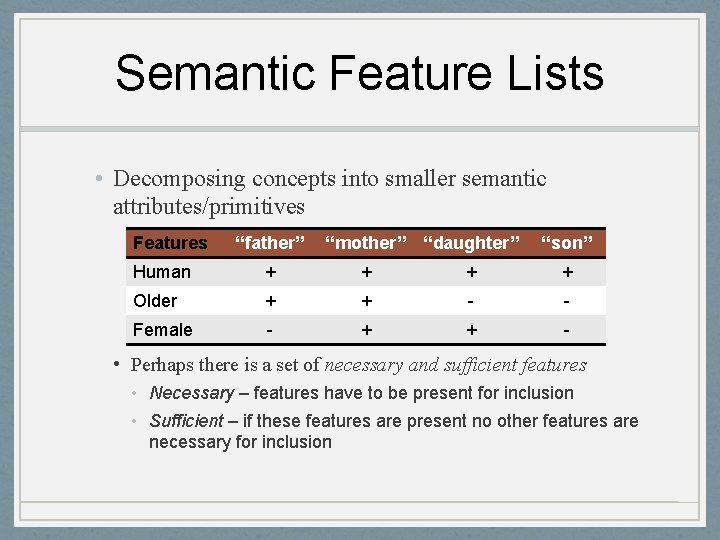
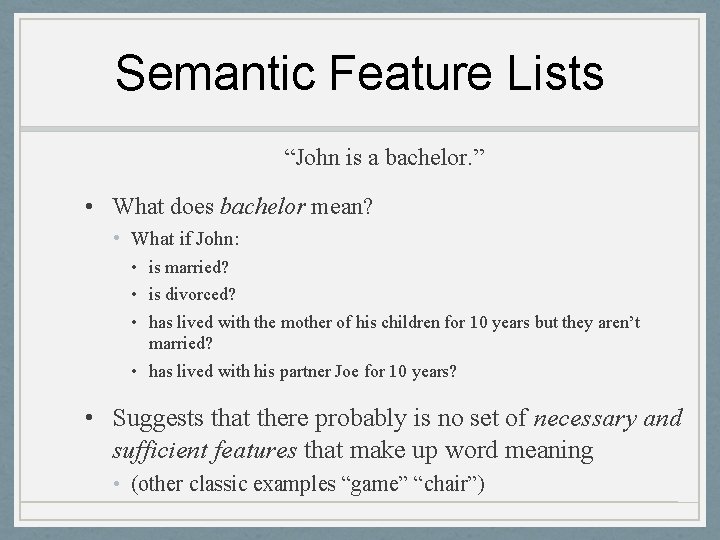
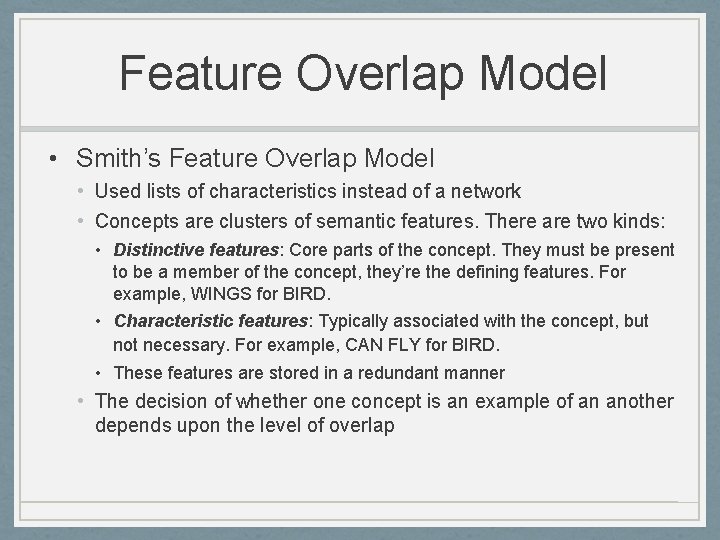
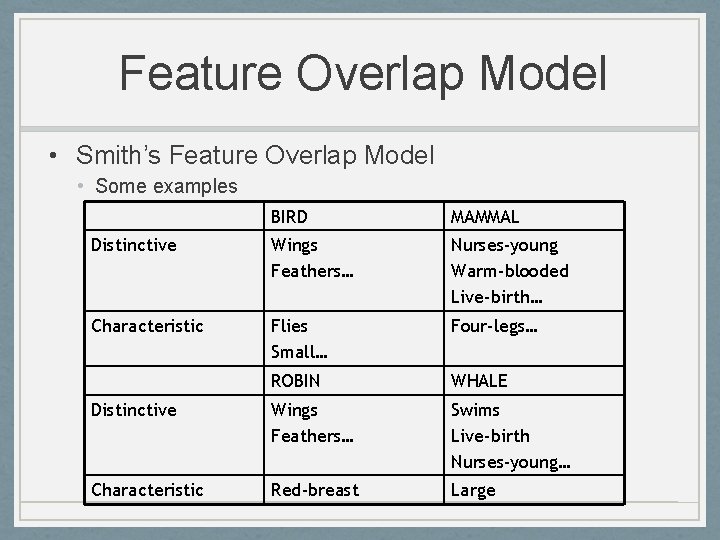
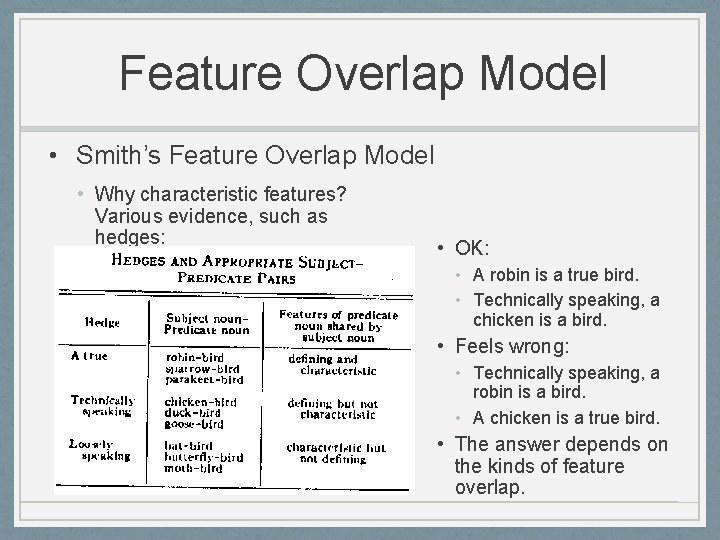
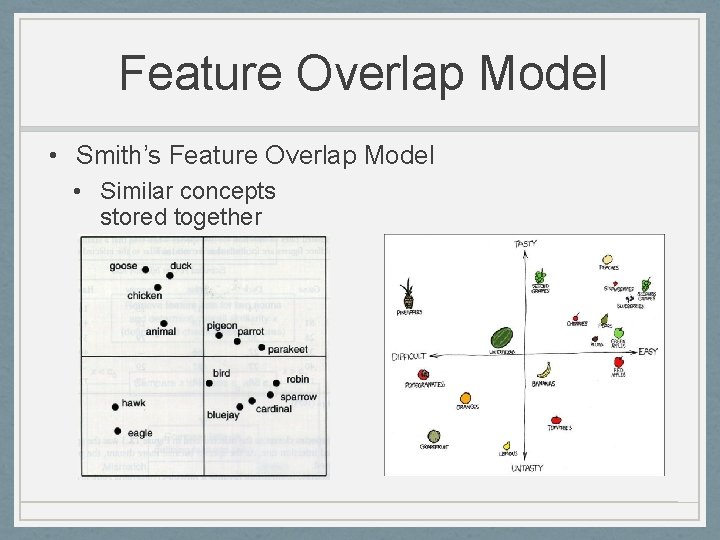
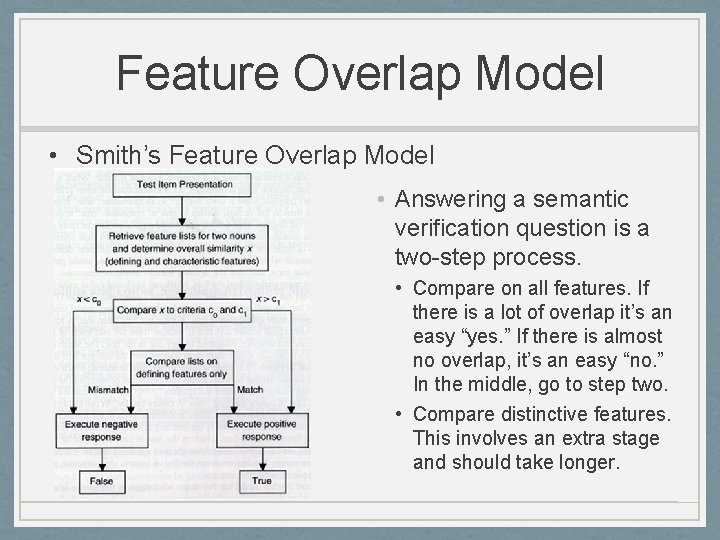
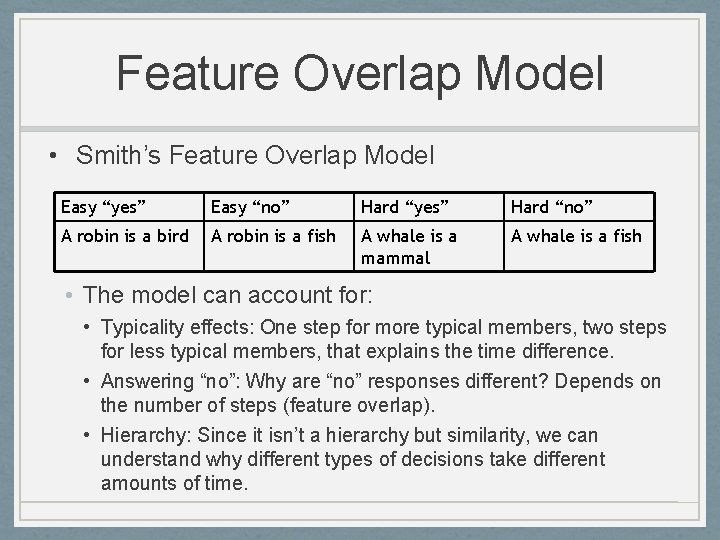
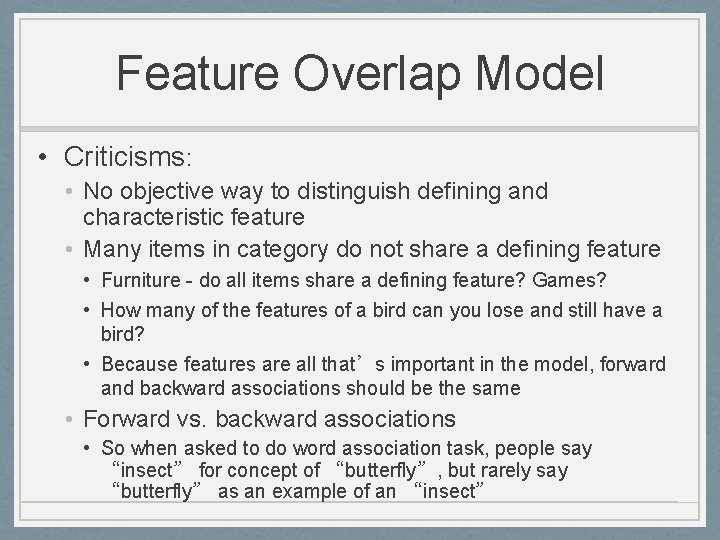
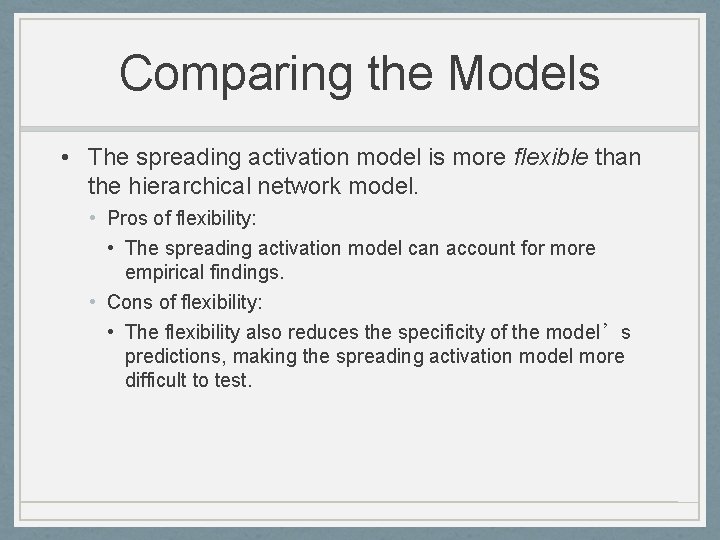
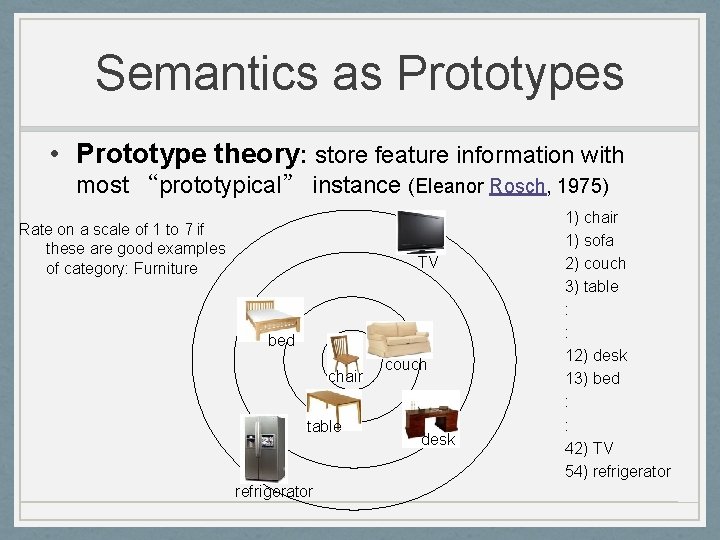
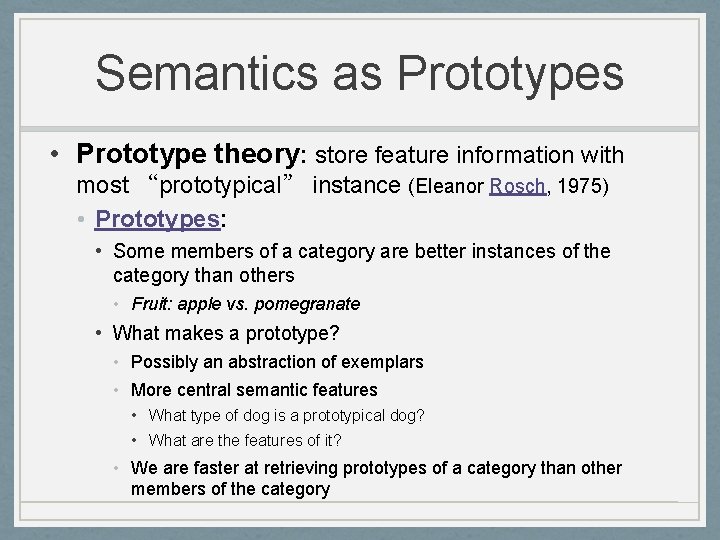
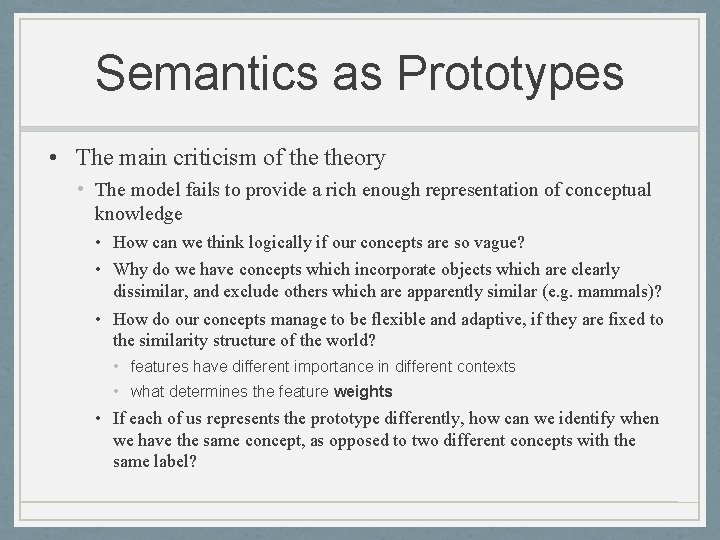
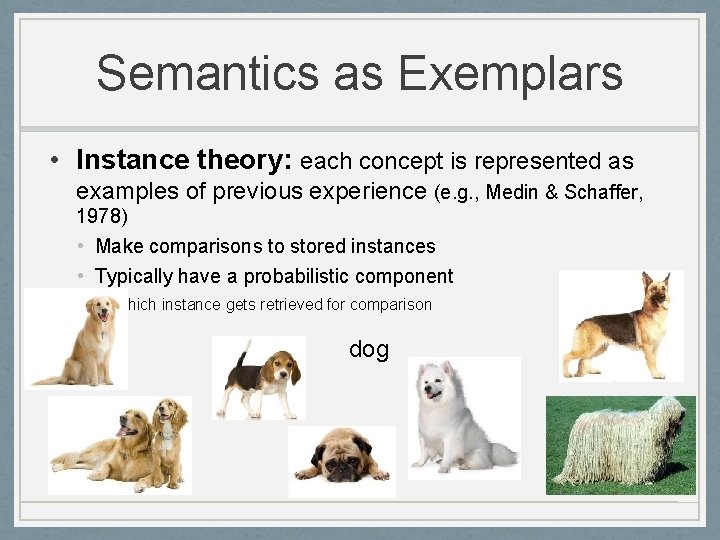
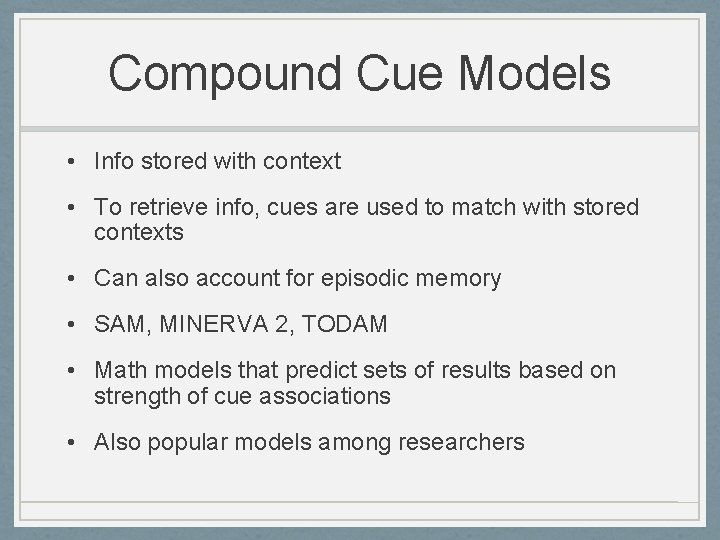
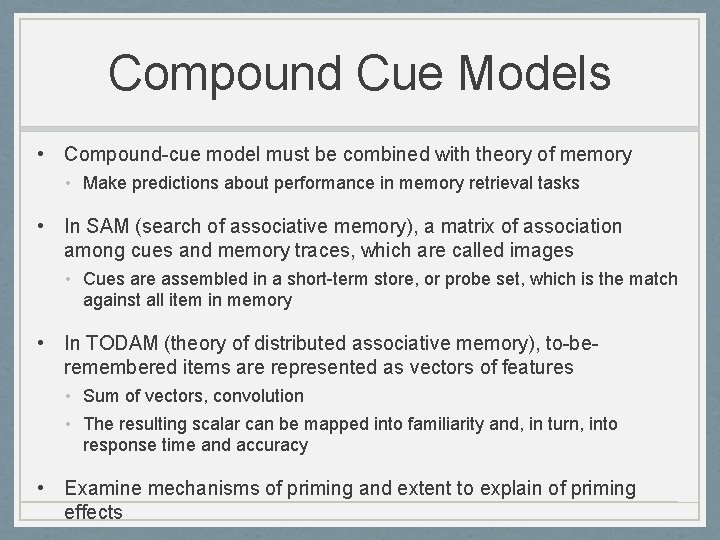
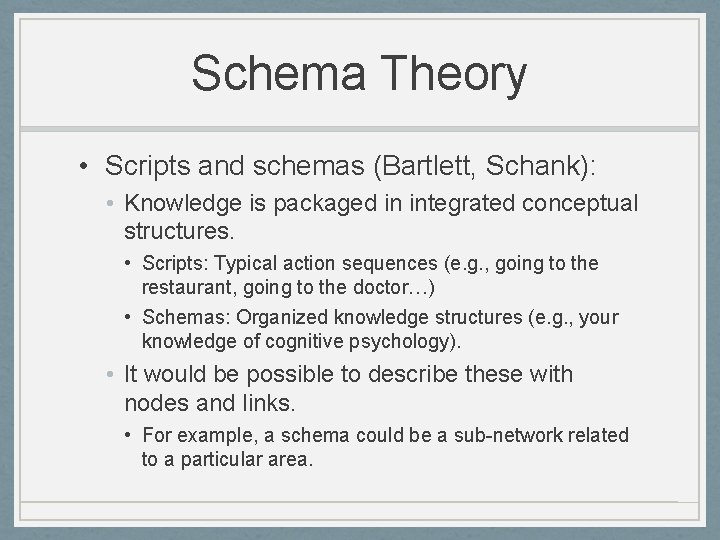
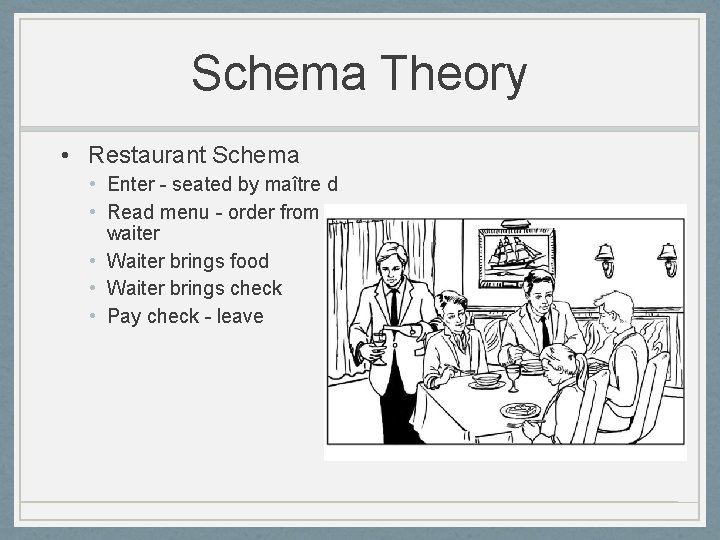
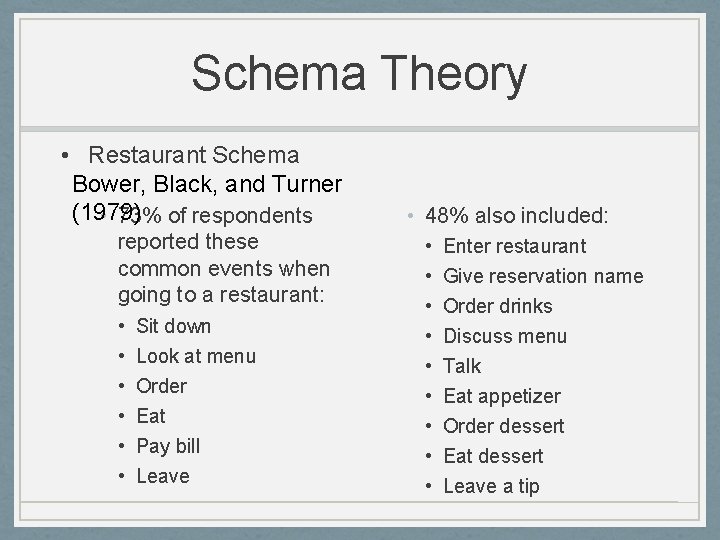
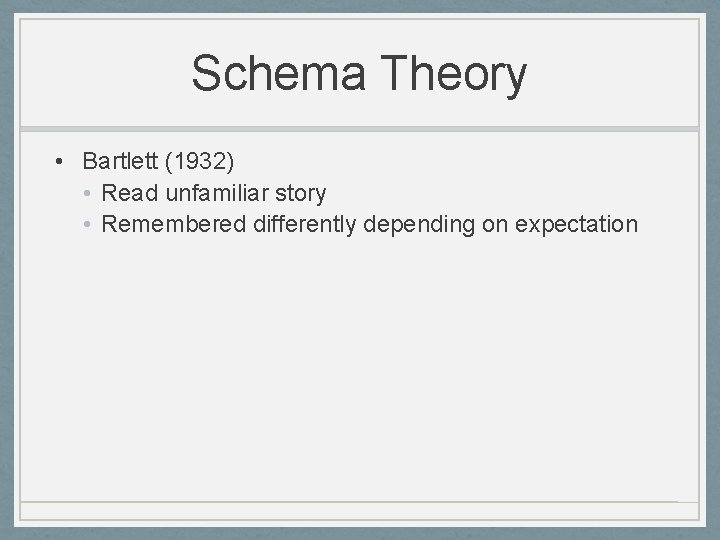
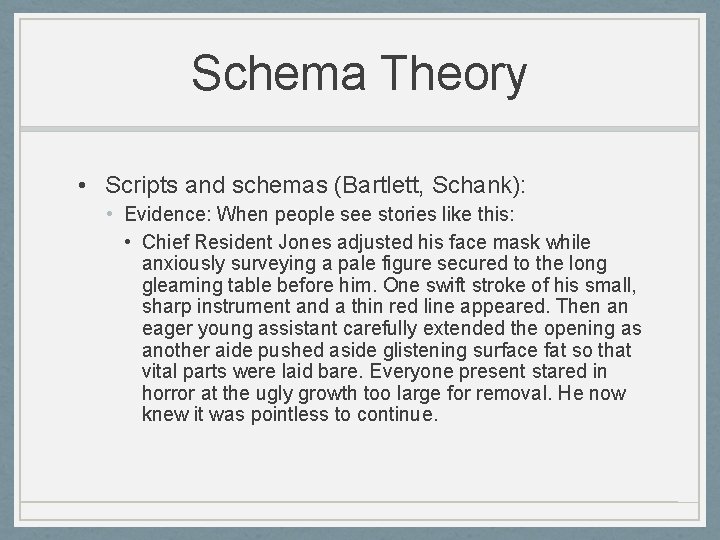
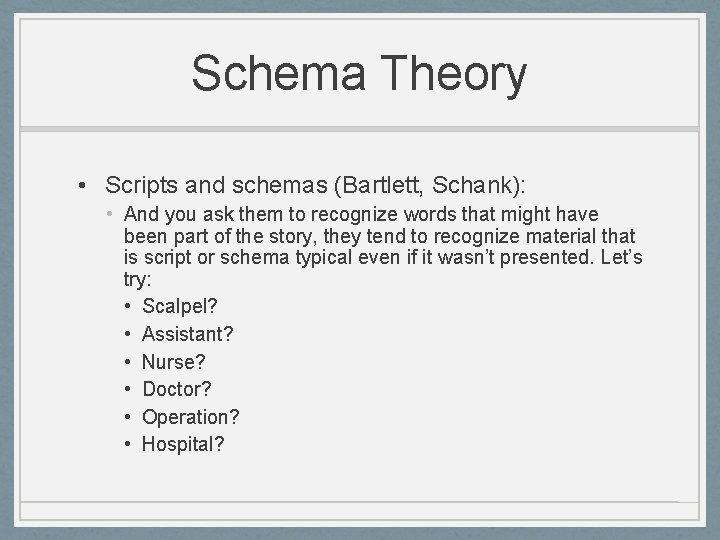
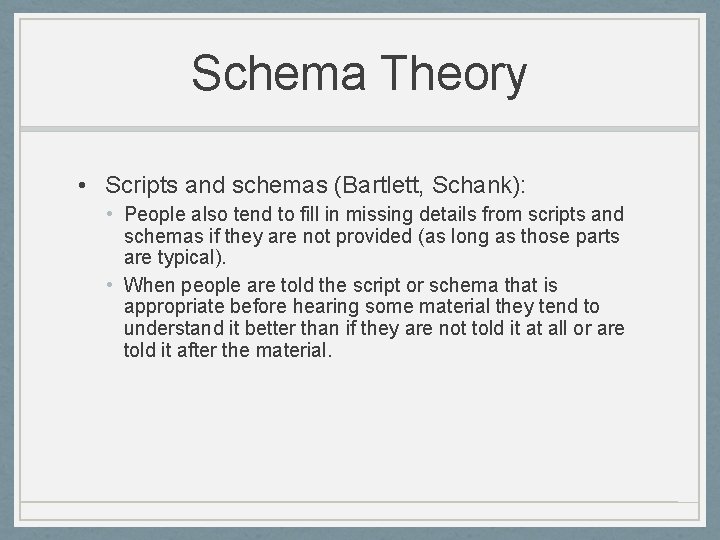
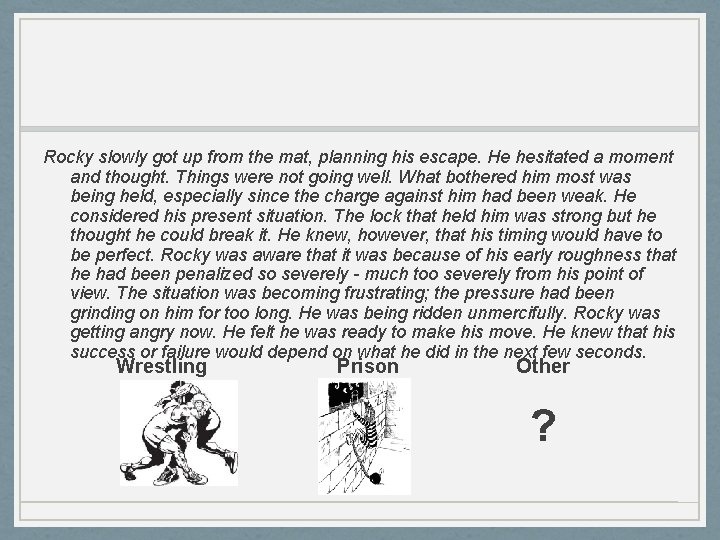
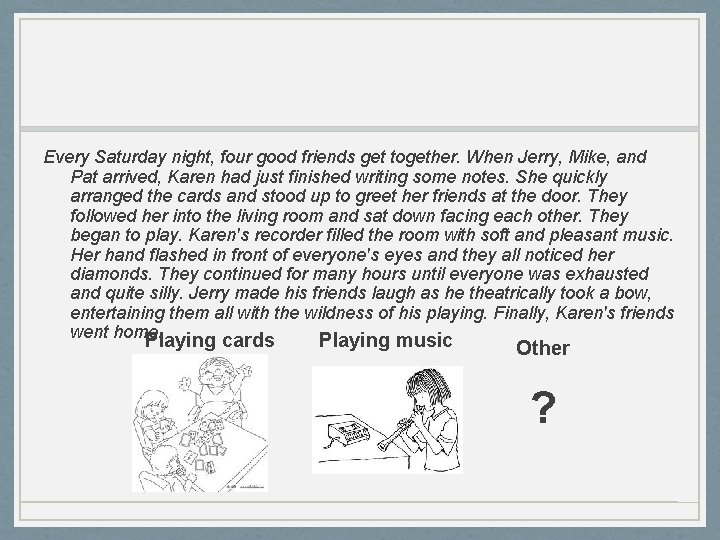
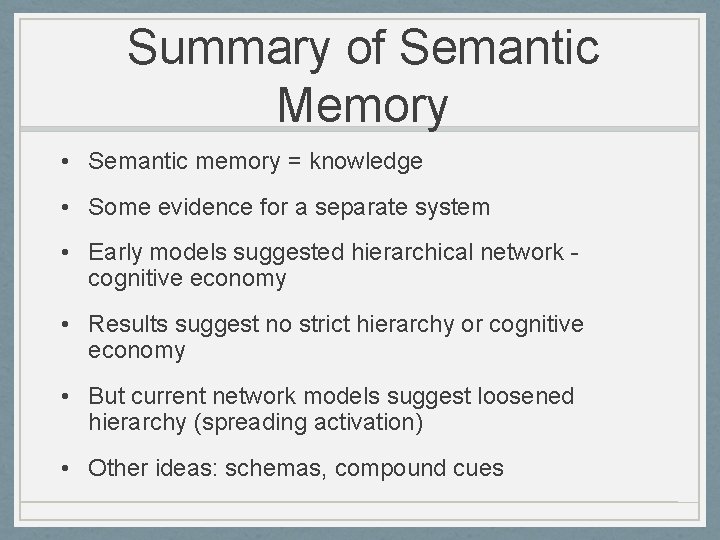
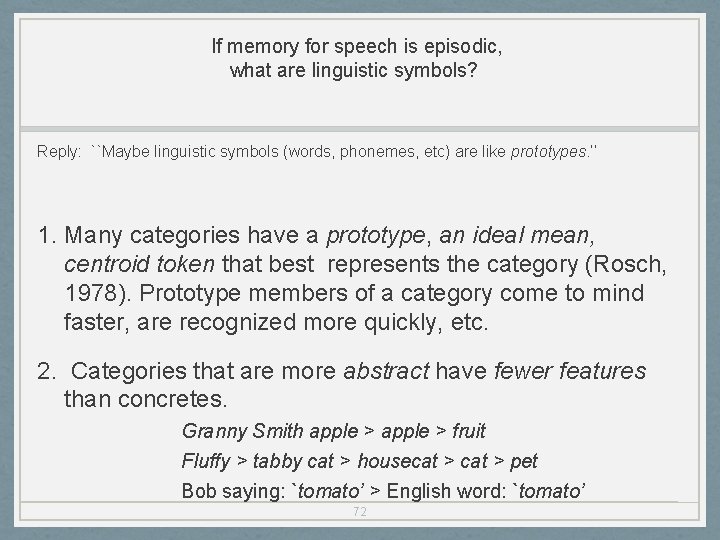
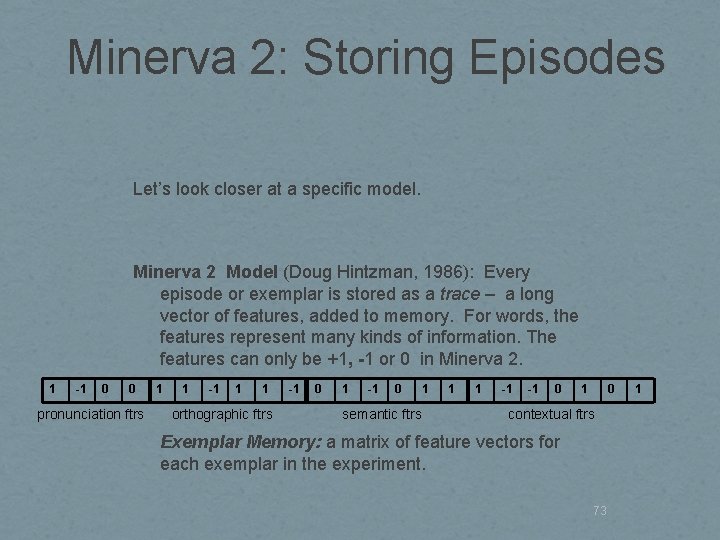
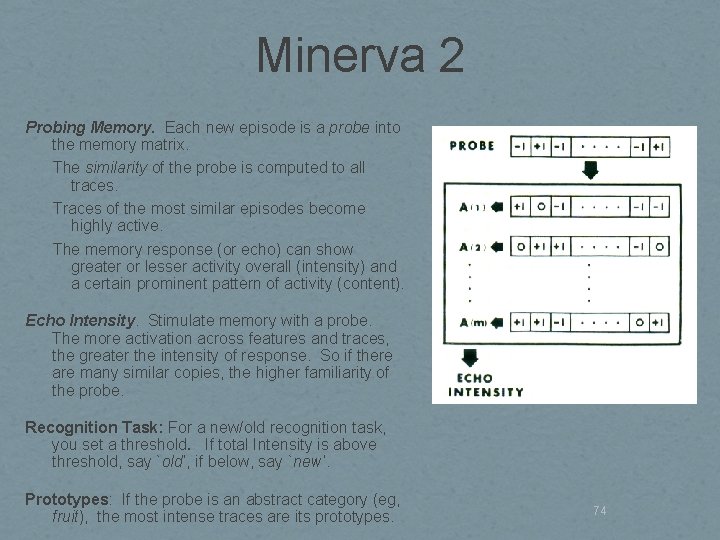
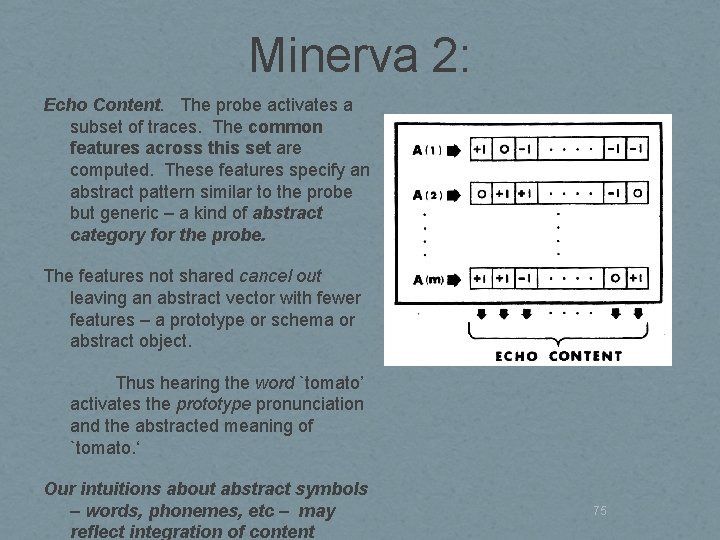
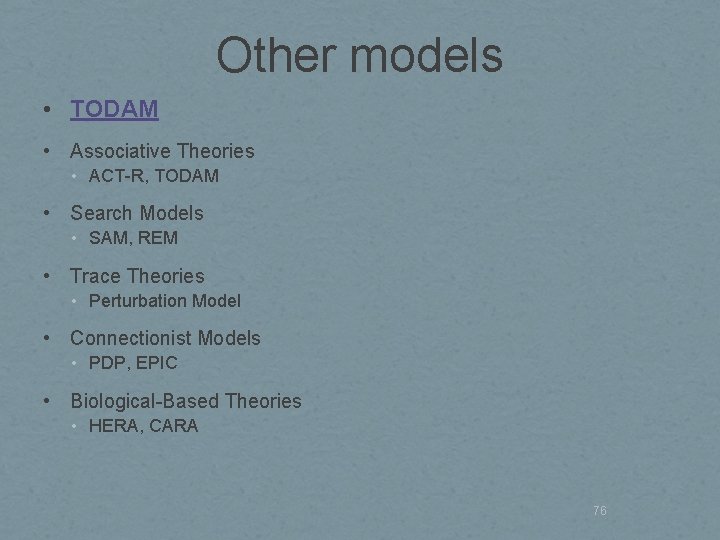
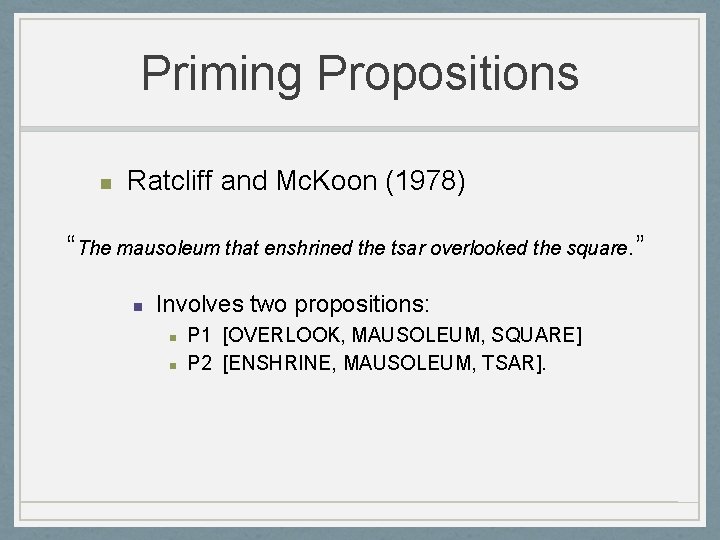
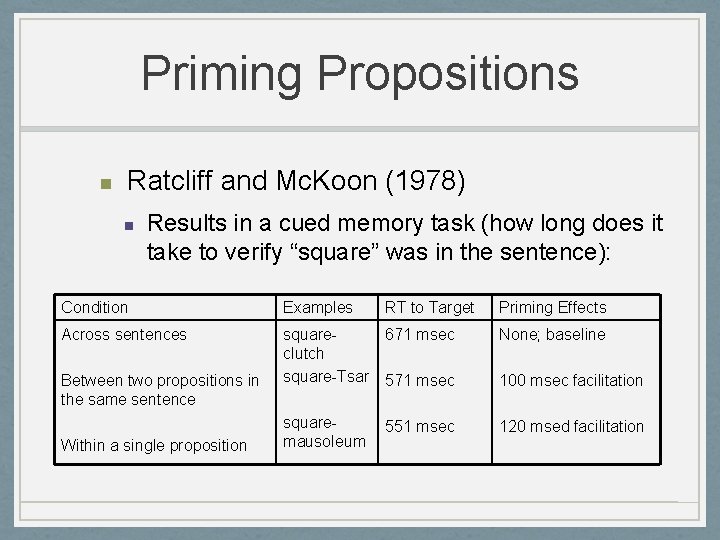
- Slides: 78
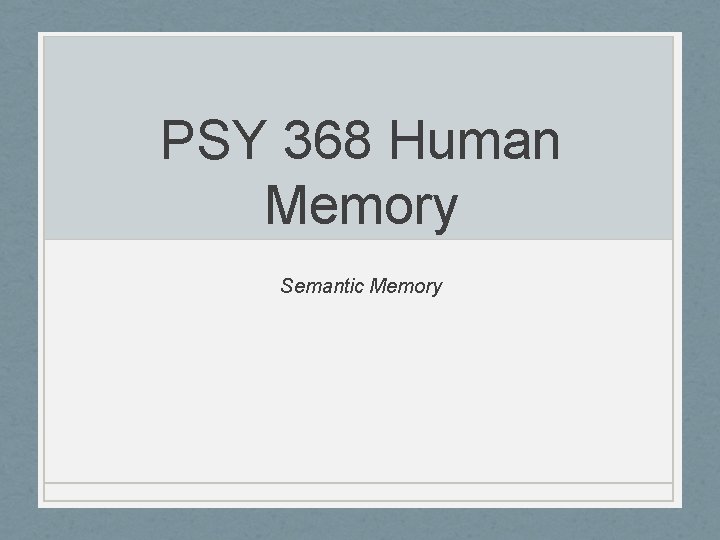
PSY 368 Human Memory Semantic Memory
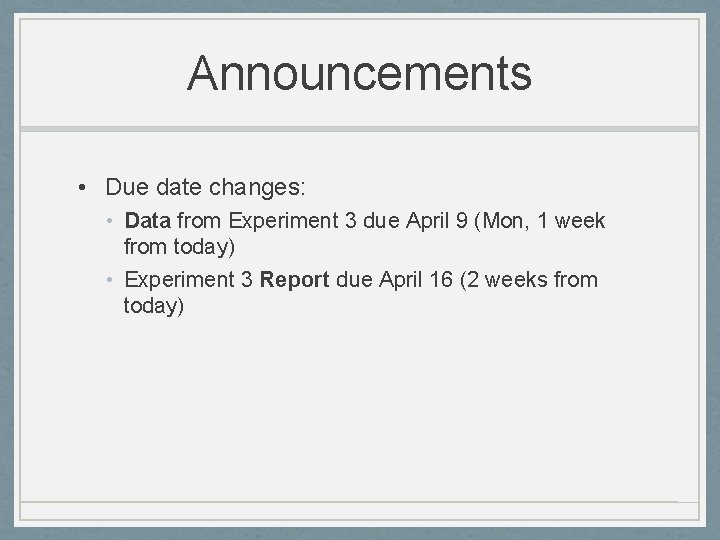
Announcements • Due date changes: • Data from Experiment 3 due April 9 (Mon, 1 week from today) • Experiment 3 Report due April 16 (2 weeks from today)
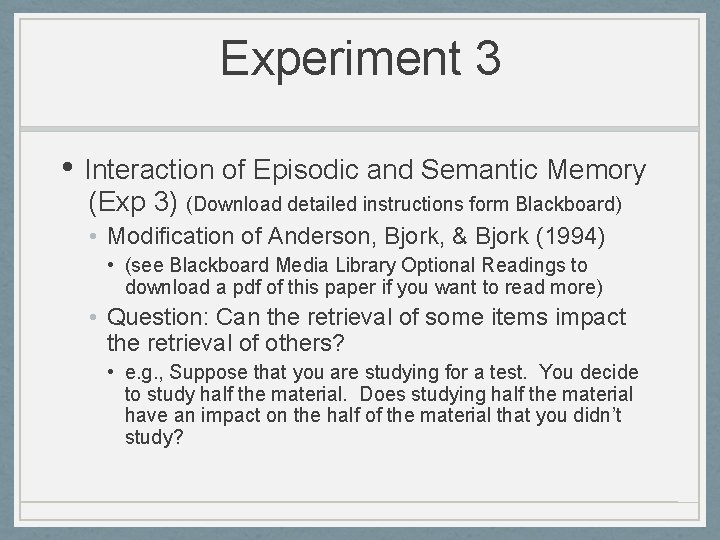
Experiment 3 • Interaction of Episodic and Semantic Memory (Exp 3) (Download detailed instructions form Blackboard) • Modification of Anderson, Bjork, & Bjork (1994) • (see Blackboard Media Library Optional Readings to download a pdf of this paper if you want to read more) • Question: Can the retrieval of some items impact the retrieval of others? • e. g. , Suppose that you are studying for a test. You decide to study half the material. Does studying half the material have an impact on the half of the material that you didn’t study?
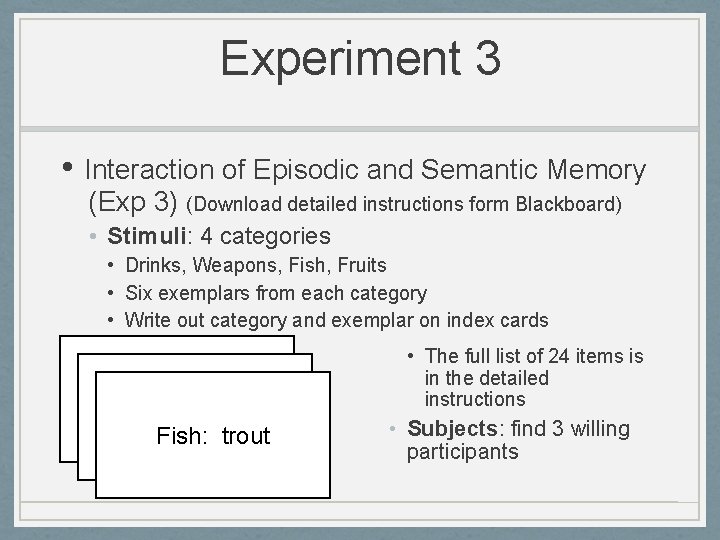
Experiment 3 • Interaction of Episodic and Semantic Memory (Exp 3) (Download detailed instructions form Blackboard) • Stimuli: 4 categories • Drinks, Weapons, Fish, Fruits • Six exemplars from each category • Write out category and exemplar on index cards Drink: vodka Weapon: sword Fish: trout • The full list of 24 items is in the detailed instructions • Subjects: find 3 willing participants
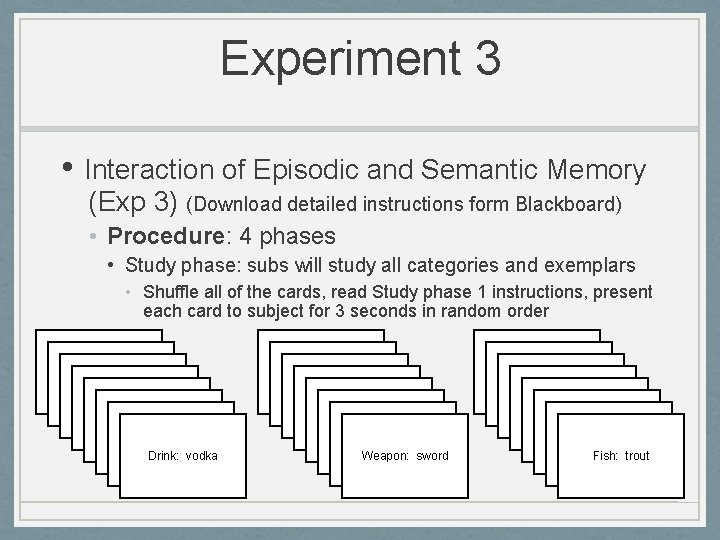
Experiment 3 • Interaction of Episodic and Semantic Memory (Exp 3) (Download detailed instructions form Blackboard) • Procedure: 4 phases • Study phase: subs will study all categories and exemplars • Shuffle all of the cards, read Study phase 1 instructions, present each card to subject for 3 seconds in random order Drink: vodka Drink: vodka Weapon: sword Weapon: sword Weapon: sword Weapon: sword Fish: trout
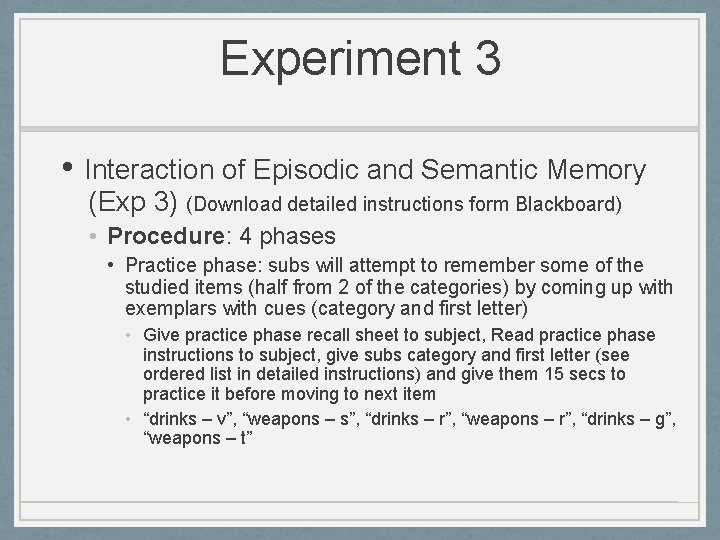
Experiment 3 • Interaction of Episodic and Semantic Memory (Exp 3) (Download detailed instructions form Blackboard) • Procedure: 4 phases • Practice phase: subs will attempt to remember some of the studied items (half from 2 of the categories) by coming up with exemplars with cues (category and first letter) • Give practice phase recall sheet to subject, Read practice phase instructions to subject, give subs category and first letter (see ordered list in detailed instructions) and give them 15 secs to practice it before moving to next item • “drinks – v”, “weapons – s”, “drinks – r”, “weapons – r”, “drinks – g”, “weapons – t”
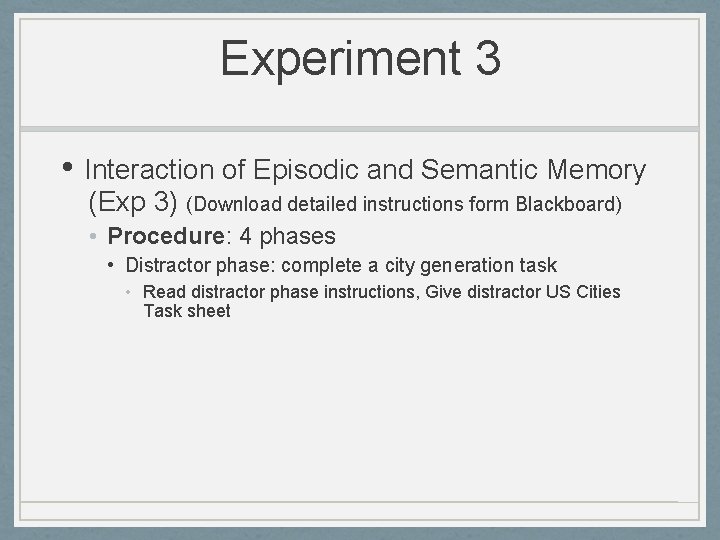
Experiment 3 • Interaction of Episodic and Semantic Memory (Exp 3) (Download detailed instructions form Blackboard) • Procedure: 4 phases • Distractor phase: complete a city generation task • Read distractor phase instructions, Give distractor US Cities Task sheet
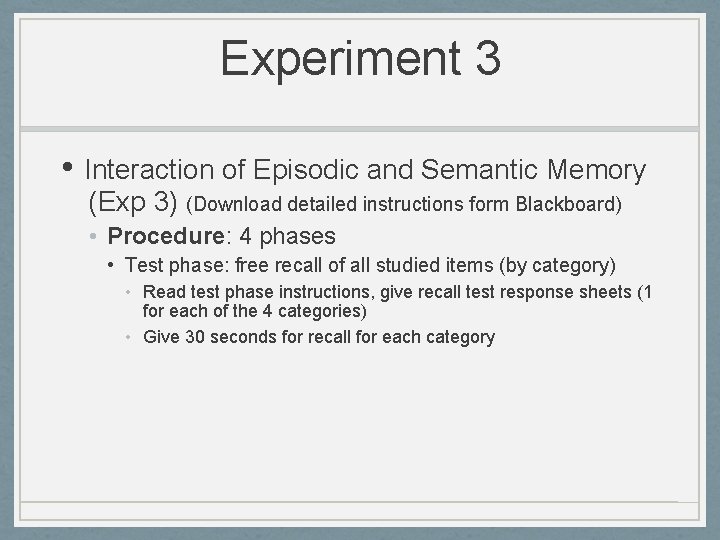
Experiment 3 • Interaction of Episodic and Semantic Memory (Exp 3) (Download detailed instructions form Blackboard) • Procedure: 4 phases • Test phase: free recall of all studied items (by category) • Read test phase instructions, give recall test response sheets (1 for each of the 4 categories) • Give 30 seconds for recall for each category
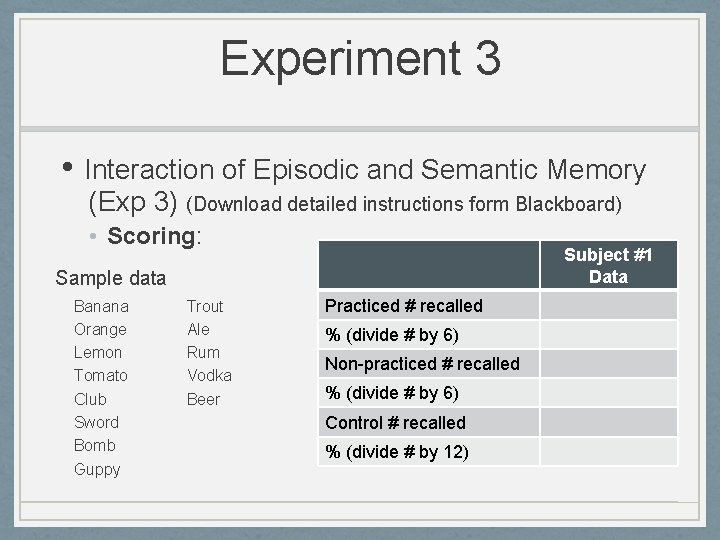
Experiment 3 • Interaction of Episodic and Semantic Memory (Exp 3) (Download detailed instructions form Blackboard) • Scoring: Subject #1 Data Sample data Banana Orange Lemon Tomato Club Sword Bomb Guppy Trout Ale Rum Vodka Beer Practiced # recalled % (divide # by 6) Non-practiced # recalled % (divide # by 6) Control # recalled % (divide # by 12)
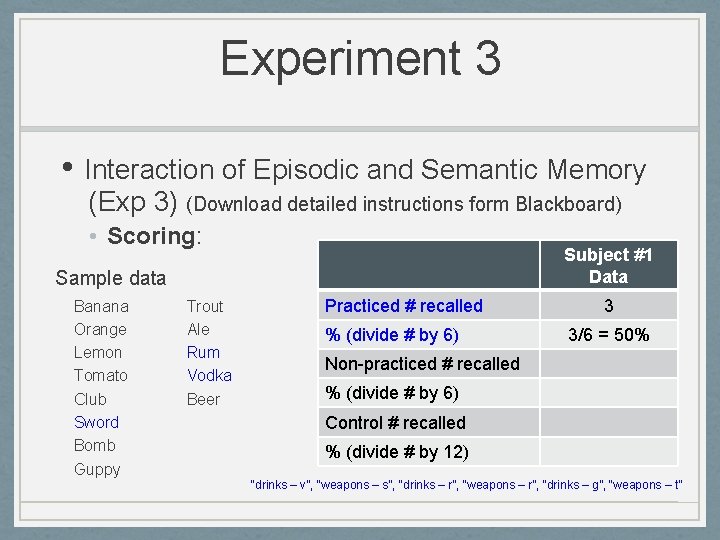
Experiment 3 • Interaction of Episodic and Semantic Memory (Exp 3) (Download detailed instructions form Blackboard) • Scoring: Subject #1 Data Sample data Banana Orange Lemon Tomato Club Sword Bomb Guppy Trout Ale Rum Vodka Beer Practiced # recalled % (divide # by 6) 3 3/6 = 50% Non-practiced # recalled % (divide # by 6) Control # recalled % (divide # by 12) “drinks – v”, “weapons – s”, “drinks – r”, “weapons – r”, “drinks – g”, “weapons – t”
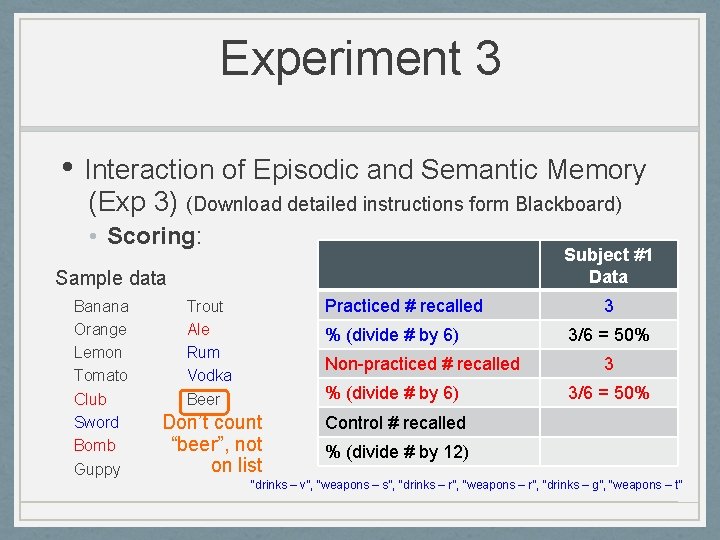
Experiment 3 • Interaction of Episodic and Semantic Memory (Exp 3) (Download detailed instructions form Blackboard) • Scoring: Subject #1 Data Sample data Banana Orange Lemon Tomato Club Sword Bomb Guppy Practiced # recalled Trout Ale Rum Vodka Beer % (divide # by 6) Non-practiced # recalled % (divide # by 6) Don’t count “beer”, not on list 3 3/6 = 50% Control # recalled % (divide # by 12) “drinks – v”, “weapons – s”, “drinks – r”, “weapons – r”, “drinks – g”, “weapons – t”
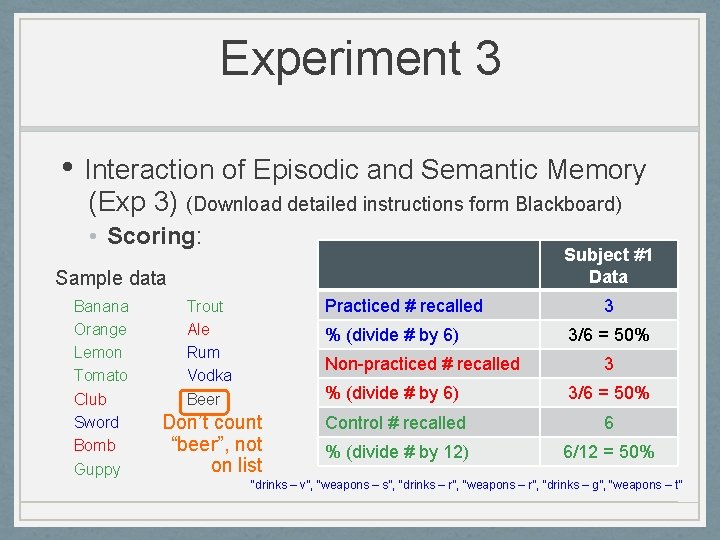
Experiment 3 • Interaction of Episodic and Semantic Memory (Exp 3) (Download detailed instructions form Blackboard) • Scoring: Subject #1 Data Sample data Banana Orange Lemon Tomato Club Sword Bomb Guppy Practiced # recalled Trout Ale Rum Vodka Beer % (divide # by 6) Non-practiced # recalled Don’t count “beer”, not on list 3 3/6 = 50% 3 % (divide # by 6) 3/6 = 50% Control # recalled 6 % (divide # by 12) 6/12 = 50% “drinks – v”, “weapons – s”, “drinks – r”, “weapons – r”, “drinks – g”, “weapons – t”
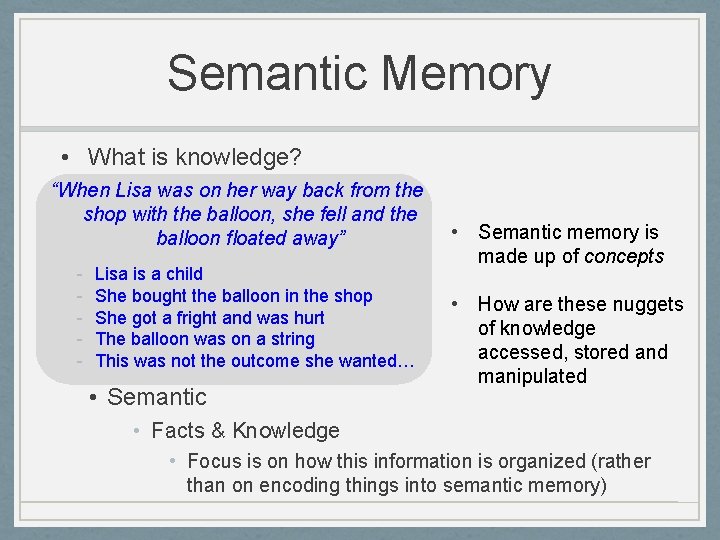
Semantic Memory • What is knowledge? “When Lisa was on her way back from the shop with the balloon, she fell and the balloon floated away” - Lisa is a child She bought the balloon in the shop She got a fright and was hurt The balloon was on a string This was not the outcome she wanted… • Semantic memory is made up of concepts • How are these nuggets of knowledge accessed, stored and manipulated • Facts & Knowledge • Focus is on how this information is organized (rather than on encoding things into semantic memory)
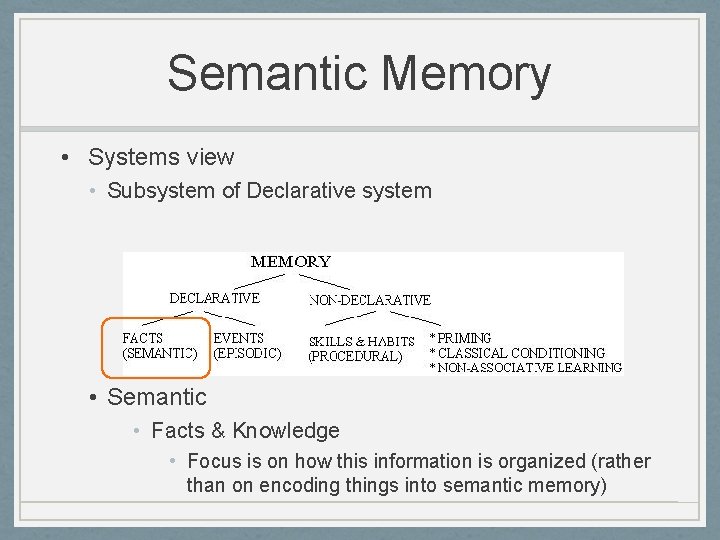
Semantic Memory • Systems view • Subsystem of Declarative system • Semantic • Facts & Knowledge • Focus is on how this information is organized (rather than on encoding things into semantic memory)
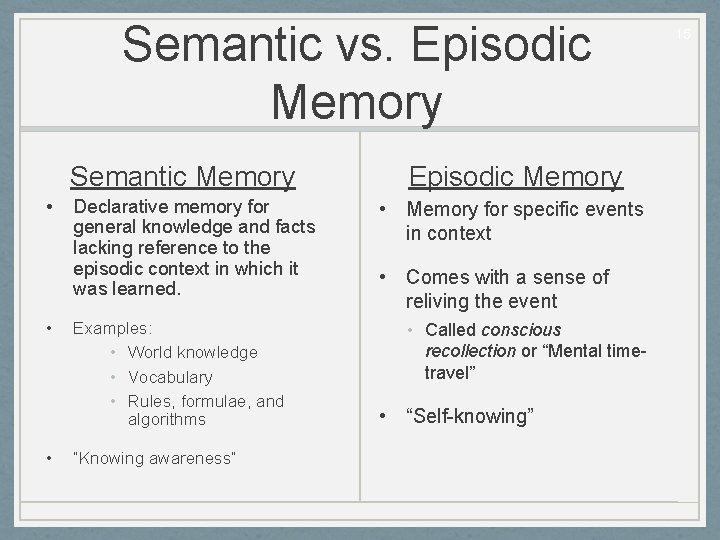
Semantic vs. Episodic Memory Semantic Memory • • • Declarative memory for general knowledge and facts lacking reference to the episodic context in which it was learned. Examples: • World knowledge • Vocabulary • Rules, formulae, and algorithms “Knowing awareness” Episodic Memory • Memory for specific events in context • Comes with a sense of reliving the event • Called conscious recollection or “Mental timetravel” • “Self-knowing” 15
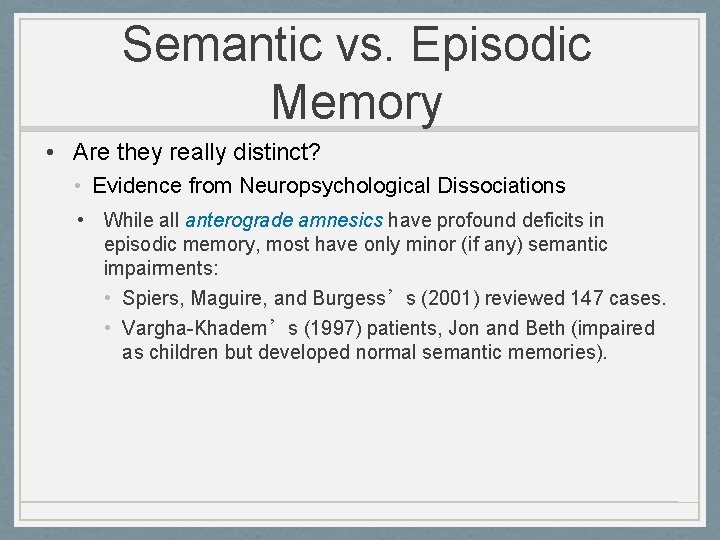
Semantic vs. Episodic Memory • Are they really distinct? • Evidence from Neuropsychological Dissociations • While all anterograde amnesics have profound deficits in episodic memory, most have only minor (if any) semantic impairments: • Spiers, Maguire, and Burgess’s (2001) reviewed 147 cases. • Vargha-Khadem’s (1997) patients, Jon and Beth (impaired as children but developed normal semantic memories).
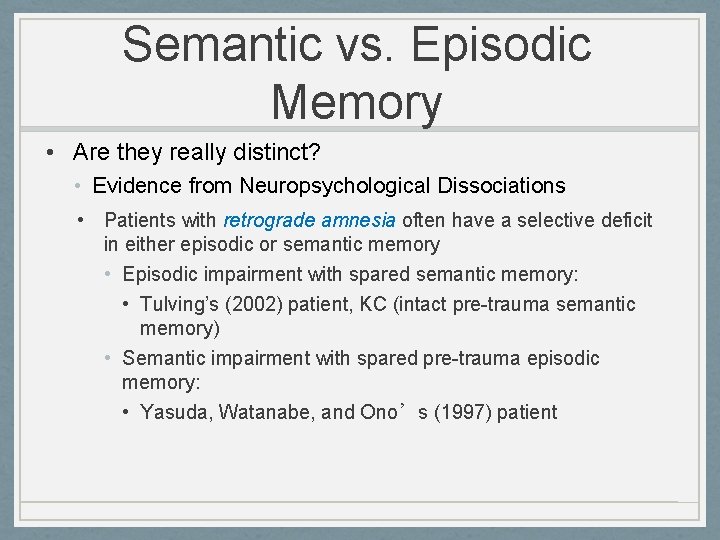
Semantic vs. Episodic Memory • Are they really distinct? • Evidence from Neuropsychological Dissociations • Patients with retrograde amnesia often have a selective deficit in either episodic or semantic memory • Episodic impairment with spared semantic memory: • Tulving’s (2002) patient, KC (intact pre-trauma semantic memory) • Semantic impairment with spared pre-trauma episodic memory: • Yasuda, Watanabe, and Ono’s (1997) patient
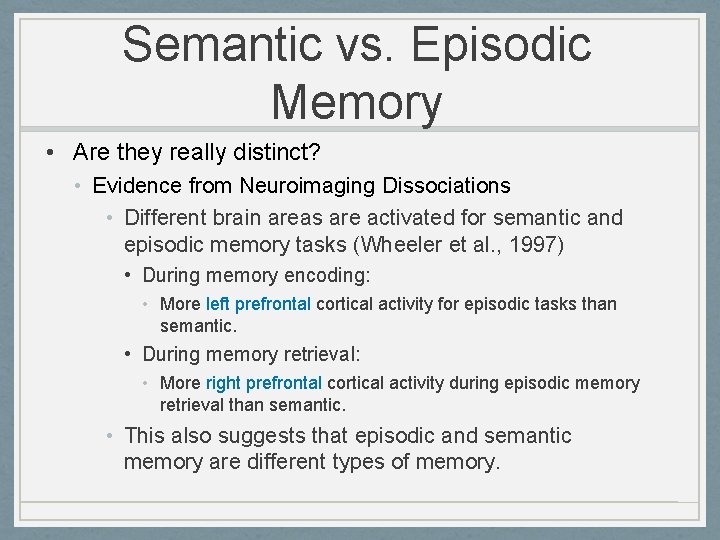
Semantic vs. Episodic Memory • Are they really distinct? • Evidence from Neuroimaging Dissociations • Different brain areas are activated for semantic and episodic memory tasks (Wheeler et al. , 1997) • During memory encoding: • More left prefrontal cortical activity for episodic tasks than semantic. • During memory retrieval: • More right prefrontal cortical activity during episodic memory retrieval than semantic. • This also suggests that episodic and semantic memory are different types of memory.
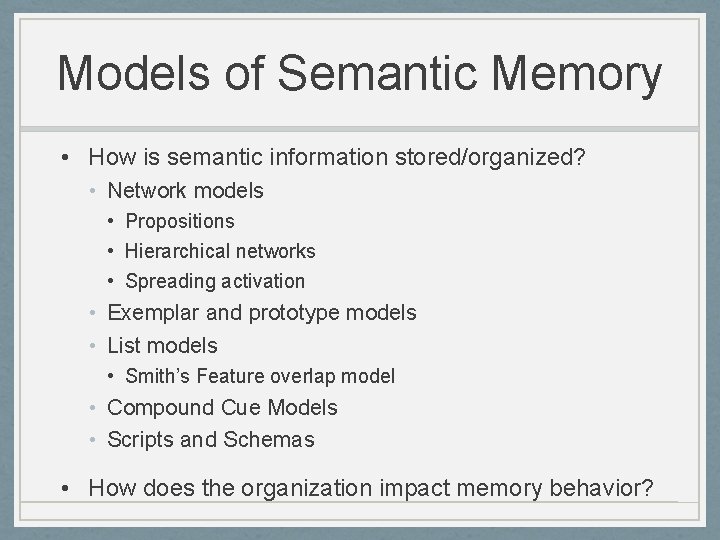
Models of Semantic Memory • How is semantic information stored/organized? • Network models • Propositions • Hierarchical networks • Spreading activation • Exemplar and prototype models • List models • Smith’s Feature overlap model • Compound Cue Models • Scripts and Schemas • How does the organization impact memory behavior?
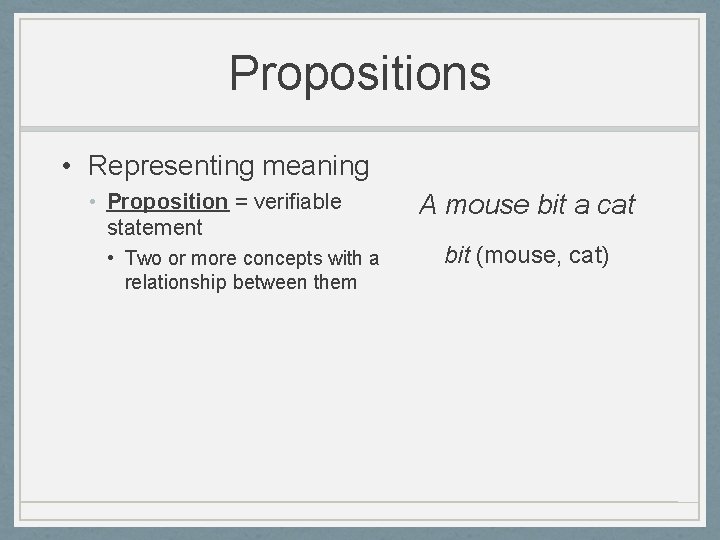
Propositions • Representing meaning • Proposition = verifiable statement • Two or more concepts with a relationship between them A mouse bit a cat bit (mouse, cat)
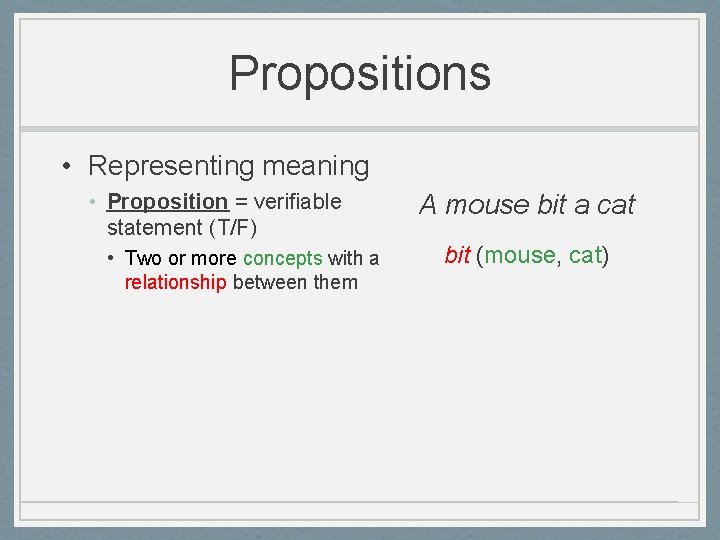
Propositions • Representing meaning • Proposition = verifiable statement (T/F) • Two or more concepts with a relationship between them A mouse bit a cat bit (mouse, cat)
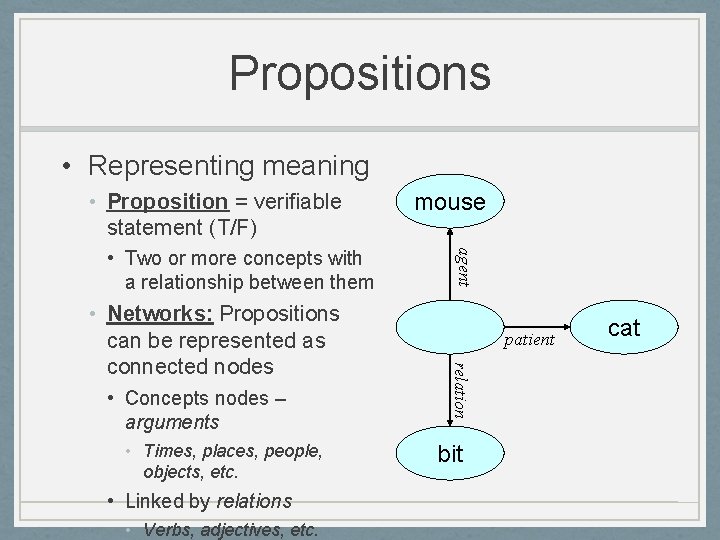
Propositions • Representing meaning • Proposition = verifiable statement (T/F) • Concepts nodes – arguments • Times, places, people, objects, etc. • Linked by relations • Verbs, adjectives, etc. patient relation • Networks: Propositions can be represented as connected nodes agent • Two or more concepts with a relationship between them mouse bit cat
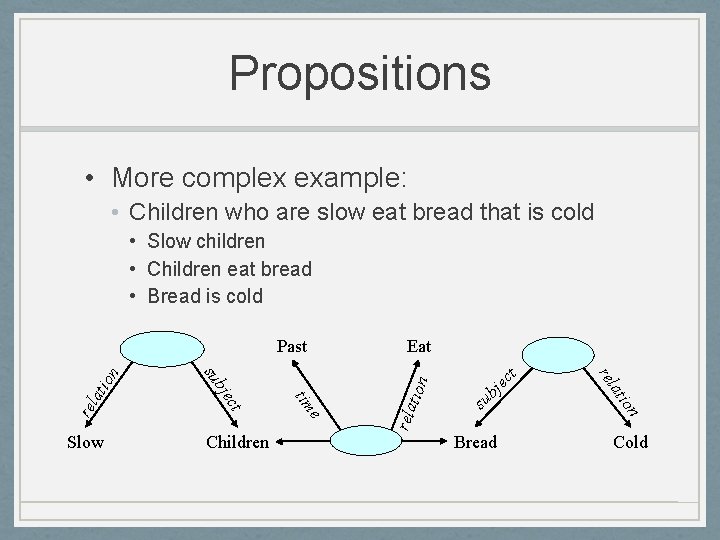
Propositions • More complex example: • Children who are slow eat bread that is cold • Slow children • Children eat bread • Bread is cold t ec su bj tion rela tio rel a on Bread ati e Children Eat rel tim ct bje Slow su n Past Cold
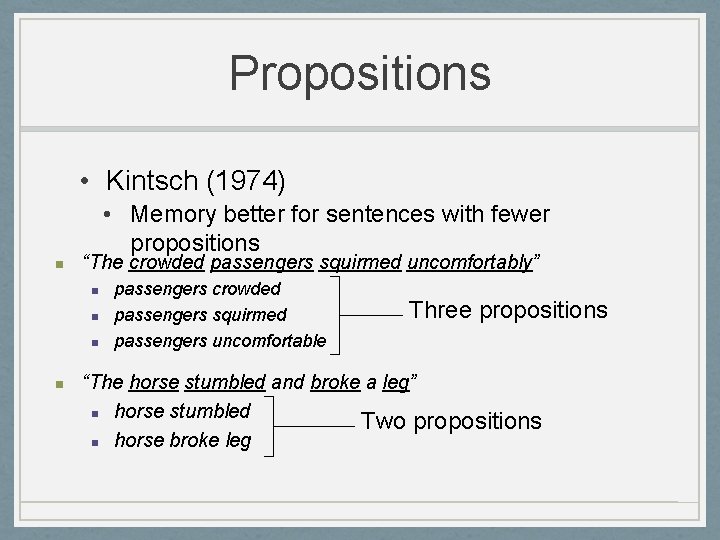
Propositions • Kintsch (1974) • Memory better for sentences with fewer propositions n “The crowded passengers squirmed uncomfortably” n n passengers crowded passengers squirmed passengers uncomfortable Three propositions “The horse stumbled and broke a leg” n horse stumbled Two propositions n horse broke leg
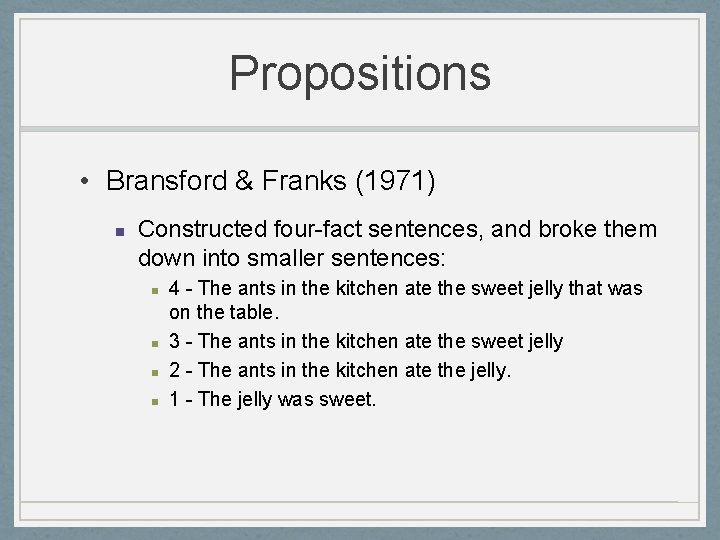
Propositions • Bransford & Franks (1971) n Constructed four-fact sentences, and broke them down into smaller sentences: n n 4 - The ants in the kitchen ate the sweet jelly that was on the table. 3 - The ants in the kitchen ate the sweet jelly 2 - The ants in the kitchen ate the jelly. 1 - The jelly was sweet.
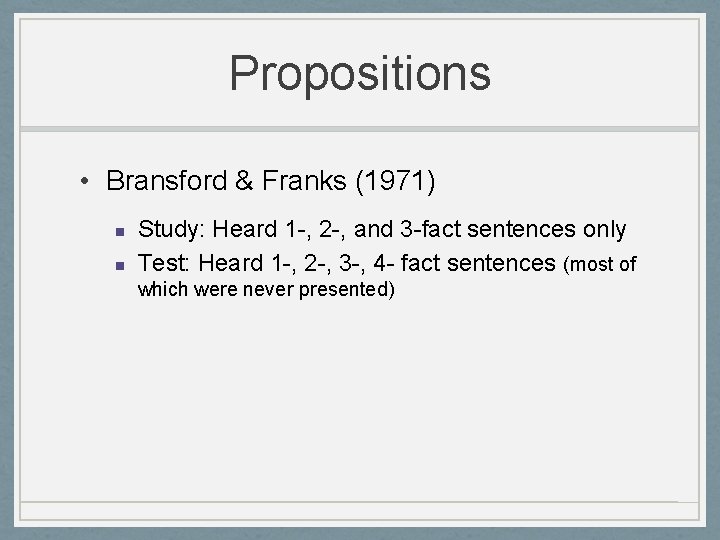
Propositions • Bransford & Franks (1971) n n Study: Heard 1 -, 2 -, and 3 -fact sentences only Test: Heard 1 -, 2 -, 3 -, 4 - fact sentences (most of which were never presented)
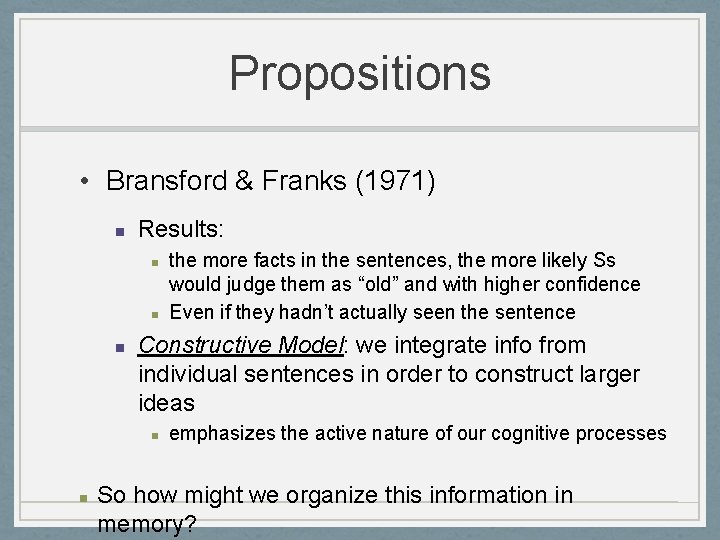
Propositions • Bransford & Franks (1971) n Results: n n n Constructive Model: we integrate info from individual sentences in order to construct larger ideas n n the more facts in the sentences, the more likely Ss would judge them as “old” and with higher confidence Even if they hadn’t actually seen the sentence emphasizes the active nature of our cognitive processes So how might we organize this information in memory?
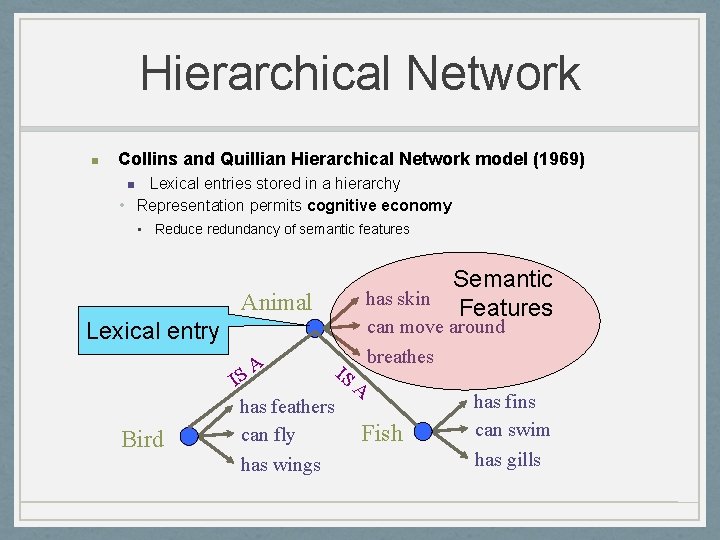
Hierarchical Network n Collins and Quillian Hierarchical Network model (1969) Lexical entries stored in a hierarchy • Representation permits cognitive economy n • Reduce redundancy of semantic features Animal Lexical entry Bird Semantic has skin Features can move around breathes A IS IS A has feathers can fly Fish has wings has fins can swim has gills
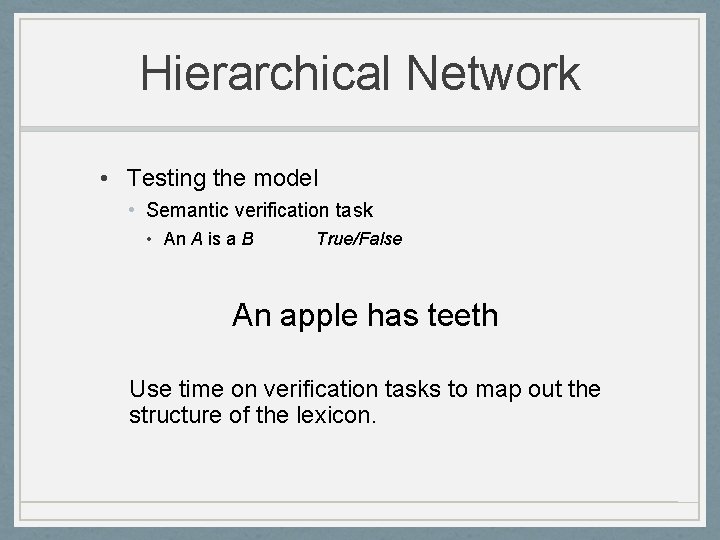
Hierarchical Network • Testing the model • Semantic verification task • An A is a B True/False An apple has teeth Use time on verification tasks to map out the structure of the lexicon.
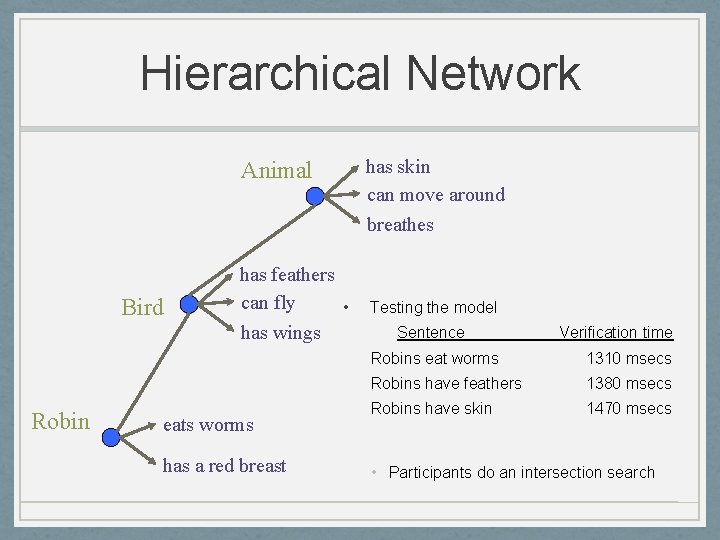
Hierarchical Network Animal Bird Robin has feathers can fly • has wings eats worms has a red breast has skin can move around breathes Testing the model Sentence Verification time Robins eat worms 1310 msecs Robins have feathers 1380 msecs Robins have skin 1470 msecs • Participants do an intersection search
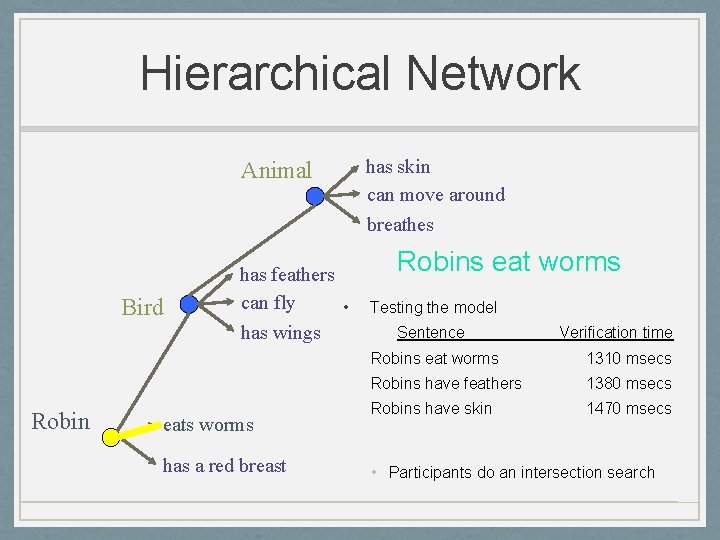
Hierarchical Network Animal Bird Robin has feathers can fly • has wings eats worms has a red breast has skin can move around breathes Robins eat worms Testing the model Sentence Verification time Robins eat worms 1310 msecs Robins have feathers 1380 msecs Robins have skin 1470 msecs • Participants do an intersection search
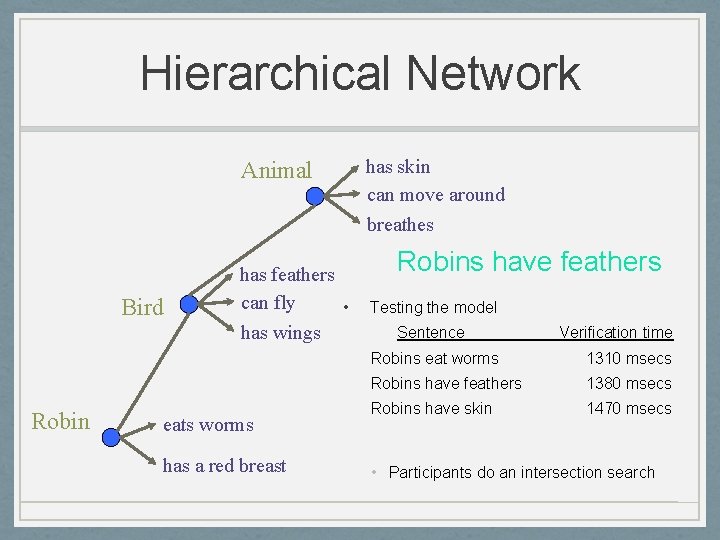
Hierarchical Network Animal Bird Robin has feathers can fly • has wings eats worms has a red breast has skin can move around breathes Robins have feathers Testing the model Sentence Verification time Robins eat worms 1310 msecs Robins have feathers 1380 msecs Robins have skin 1470 msecs • Participants do an intersection search
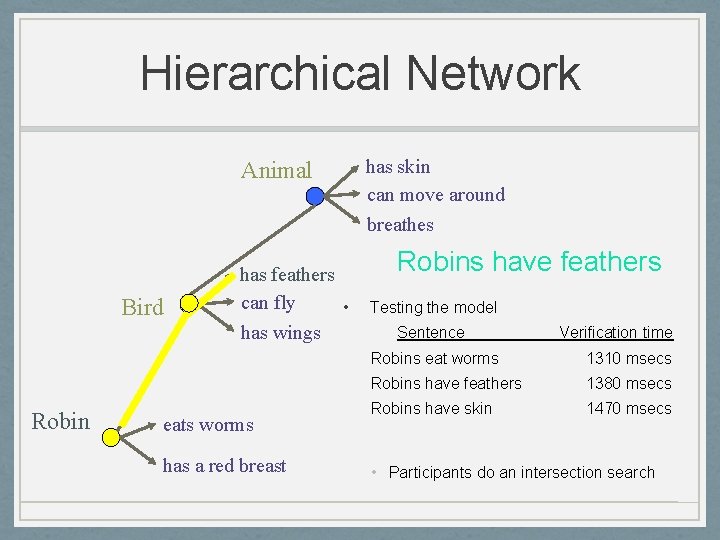
Hierarchical Network Animal Bird Robin has feathers can fly • has wings eats worms has a red breast has skin can move around breathes Robins have feathers Testing the model Sentence Verification time Robins eat worms 1310 msecs Robins have feathers 1380 msecs Robins have skin 1470 msecs • Participants do an intersection search
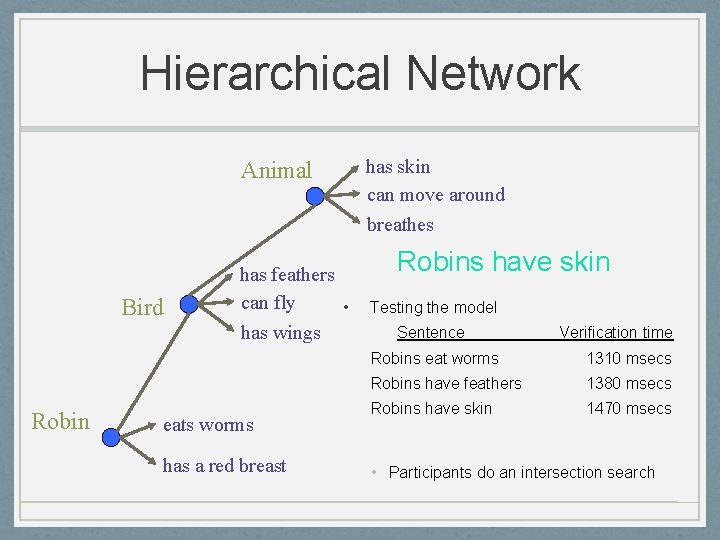
Hierarchical Network Animal Bird Robin has feathers can fly • has wings eats worms has a red breast has skin can move around breathes Robins have skin Testing the model Sentence Verification time Robins eat worms 1310 msecs Robins have feathers 1380 msecs Robins have skin 1470 msecs • Participants do an intersection search
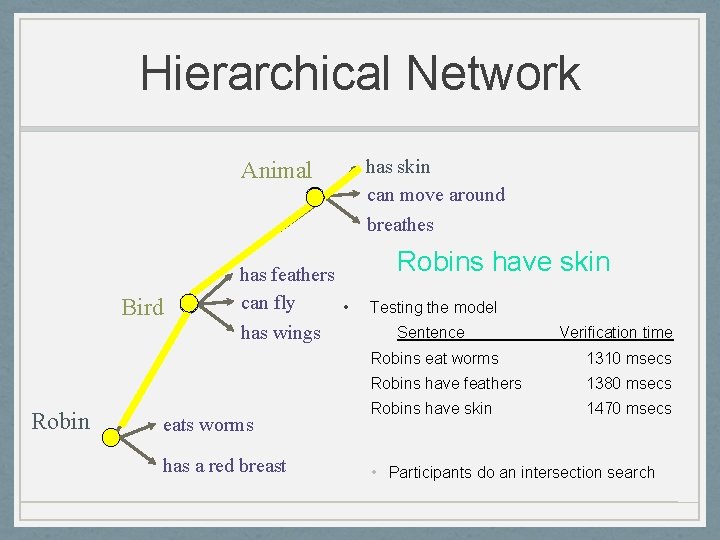
Hierarchical Network Animal Bird Robin has feathers can fly • has wings eats worms has a red breast has skin can move around breathes Robins have skin Testing the model Sentence Verification time Robins eat worms 1310 msecs Robins have feathers 1380 msecs Robins have skin 1470 msecs • Participants do an intersection search
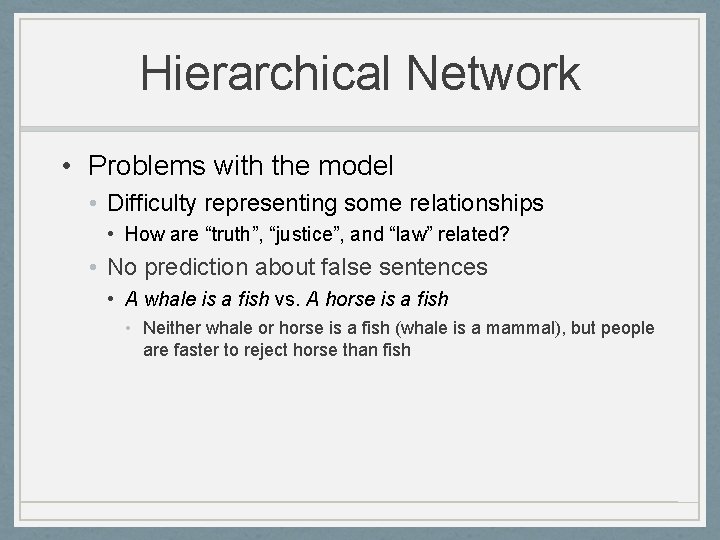
Hierarchical Network • Problems with the model • Difficulty representing some relationships • How are “truth”, “justice”, and “law” related? • No prediction about false sentences • A whale is a fish vs. A horse is a fish • Neither whale or horse is a fish (whale is a mammal), but people are faster to reject horse than fish
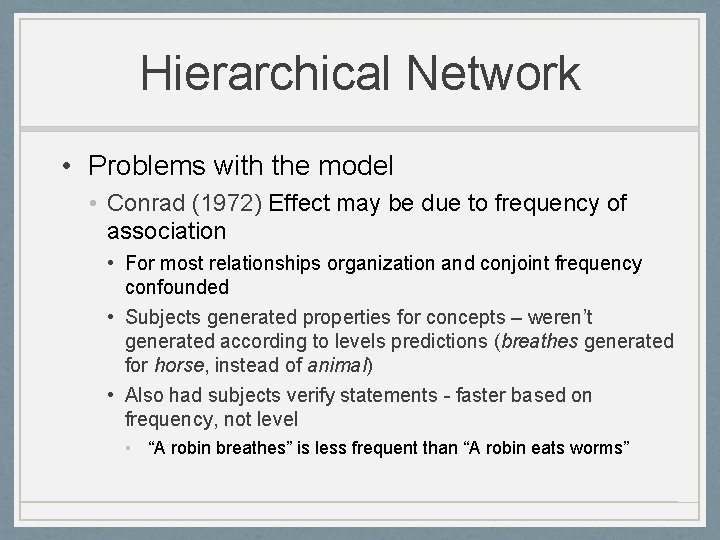
Hierarchical Network • Problems with the model • Conrad (1972) Effect may be due to frequency of association • For most relationships organization and conjoint frequency confounded • Subjects generated properties for concepts – weren’t generated according to levels predictions (breathes generated for horse, instead of animal) • Also had subjects verify statements - faster based on frequency, not level • “A robin breathes” is less frequent than “A robin eats worms”
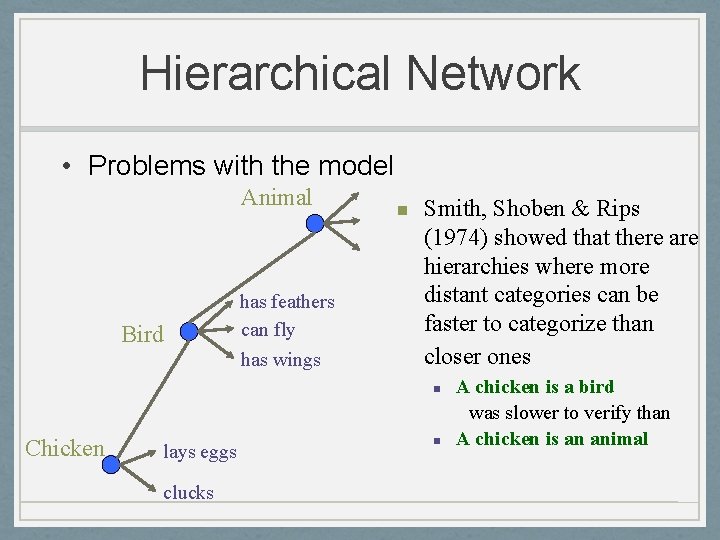
Hierarchical Network • Problems with the model Animal Bird has feathers can fly has wings n Smith, Shoben & Rips (1974) showed that there are hierarchies where more distant categories can be faster to categorize than closer ones n Chicken lays eggs clucks n A chicken is a bird was slower to verify than A chicken is an animal
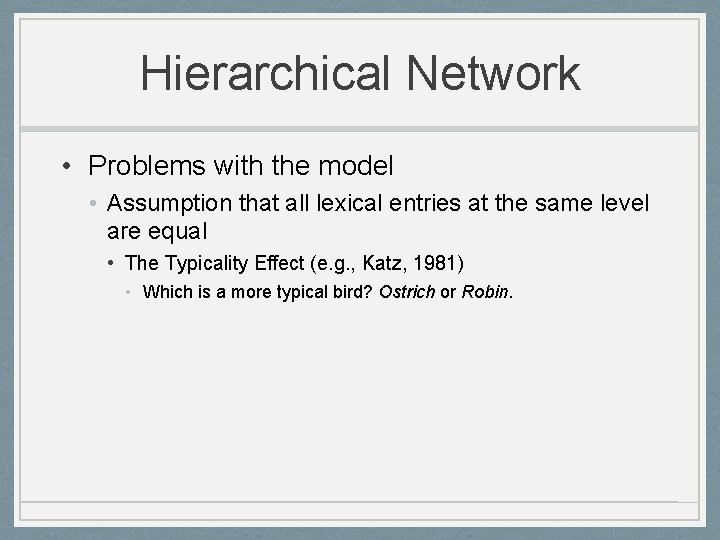
Hierarchical Network • Problems with the model • Assumption that all lexical entries at the same level are equal • The Typicality Effect (e. g. , Katz, 1981) • Which is a more typical bird? Ostrich or Robin.
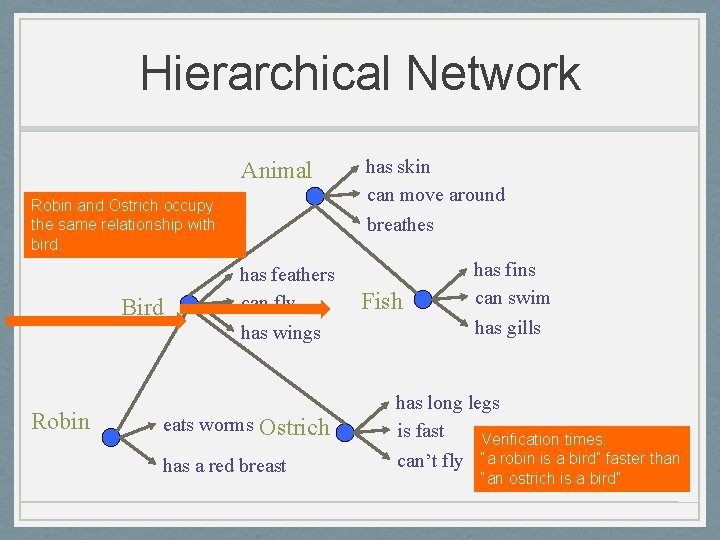
Hierarchical Network Animal Robin and Ostrich occupy the same relationship with bird. Bird Robin has feathers can fly has wings eats worms Ostrich has a red breast has skin can move around breathes Fish has fins can swim has gills has long legs is fast Verification times: can’t fly “a robin is a bird” faster than “an ostrich is a bird”
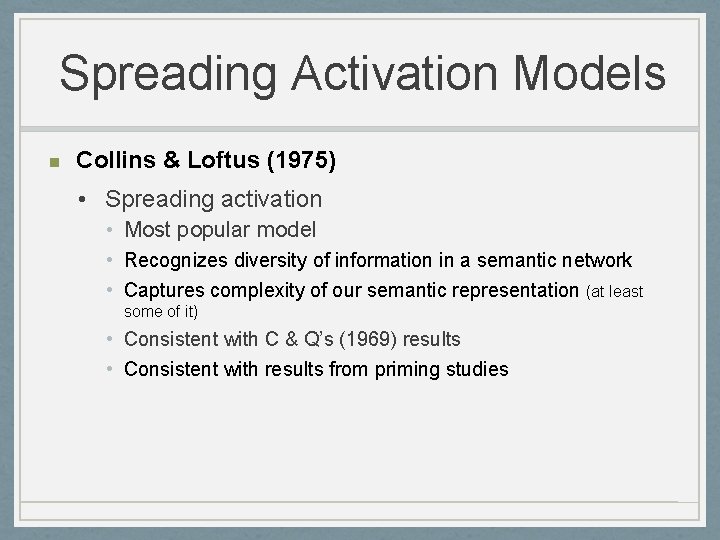
Spreading Activation Models n Collins & Loftus (1975) • Spreading activation • Most popular model • Recognizes diversity of information in a semantic network • Captures complexity of our semantic representation (at least some of it) • Consistent with C & Q’s (1969) results • Consistent with results from priming studies
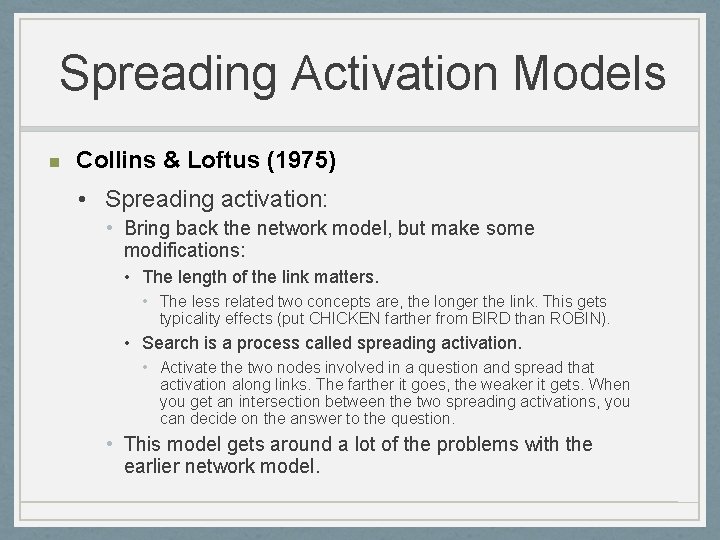
Spreading Activation Models n Collins & Loftus (1975) • Spreading activation: • Bring back the network model, but make some modifications: • The length of the link matters. • The less related two concepts are, the longer the link. This gets typicality effects (put CHICKEN farther from BIRD than ROBIN). • Search is a process called spreading activation. • Activate the two nodes involved in a question and spread that activation along links. The farther it goes, the weaker it gets. When you get an intersection between the two spreading activations, you can decide on the answer to the question. • This model gets around a lot of the problems with the earlier network model.
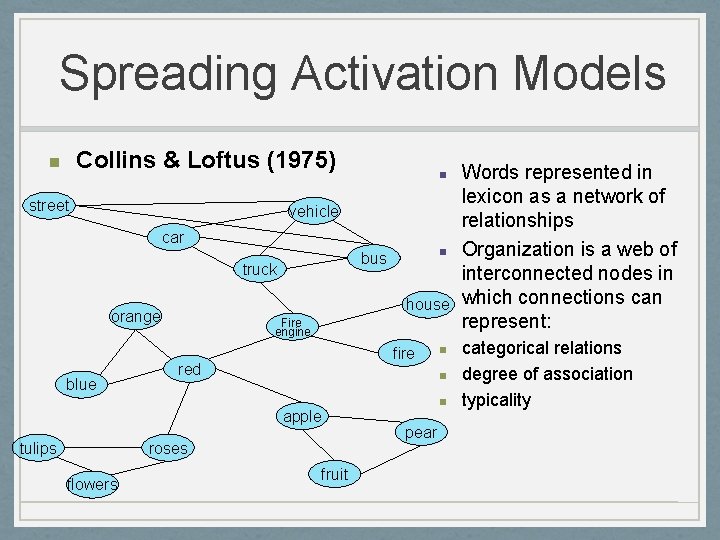
Spreading Activation Models Collins & Loftus (1975) n street n vehicle car truck house orange blue Fire engine fire red roses flowers n n apple tulips n bus fruit n pear Words represented in lexicon as a network of relationships Organization is a web of interconnected nodes in which connections can represent: categorical relations degree of association typicality
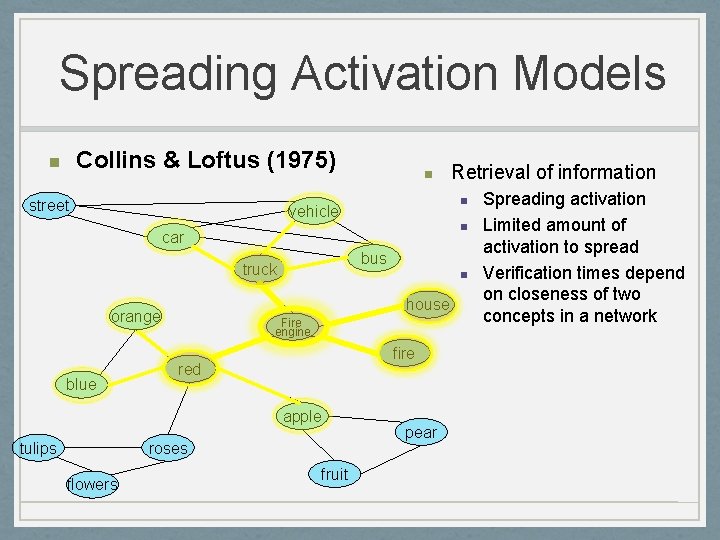
Spreading Activation Models Collins & Loftus (1975) n street n n vehicle n car bus truck blue n house orange Fire engine fire red apple tulips roses flowers Retrieval of information fruit pear Spreading activation Limited amount of activation to spread Verification times depend on closeness of two concepts in a network
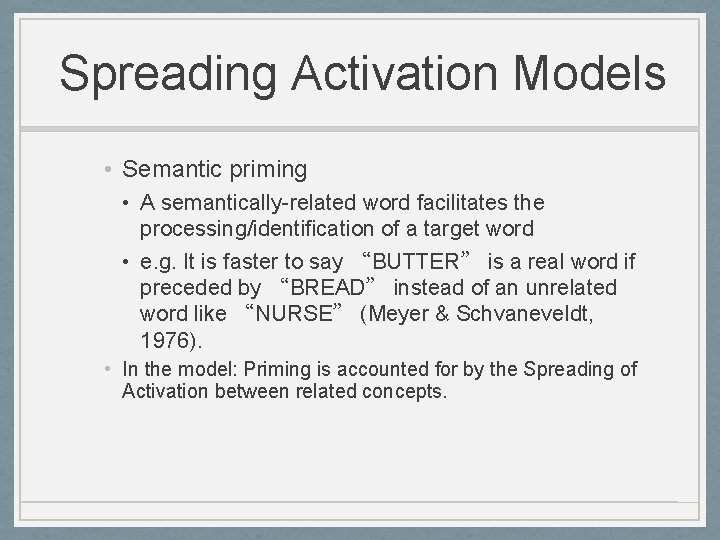
Spreading Activation Models • Semantic priming • A semantically-related word facilitates the processing/identification of a target word • e. g. It is faster to say “BUTTER” is a real word if preceded by “BREAD” instead of an unrelated word like “NURSE” (Meyer & Schvaneveldt, 1976). • In the model: Priming is accounted for by the Spreading of Activation between related concepts.
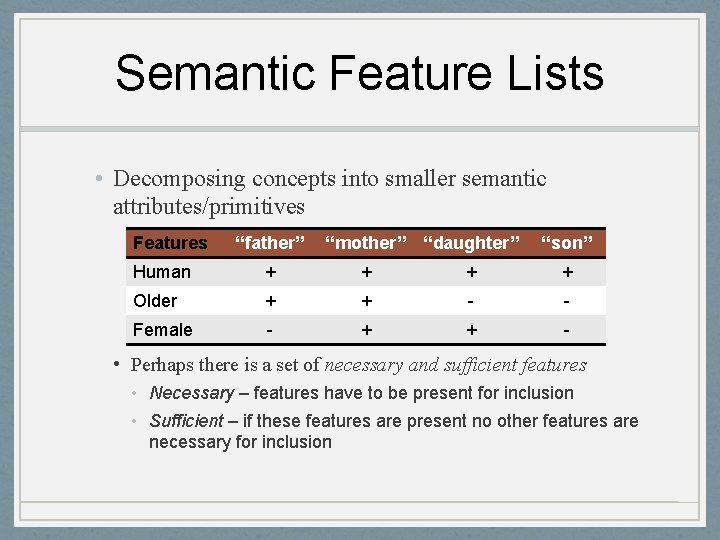
Semantic Feature Lists • Decomposing concepts into smaller semantic attributes/primitives Features “father” “mother” “daughter” “son” Human + + Older + + - - Female - + + - • Perhaps there is a set of necessary and sufficient features • Necessary – features have to be present for inclusion • Sufficient – if these features are present no other features are necessary for inclusion
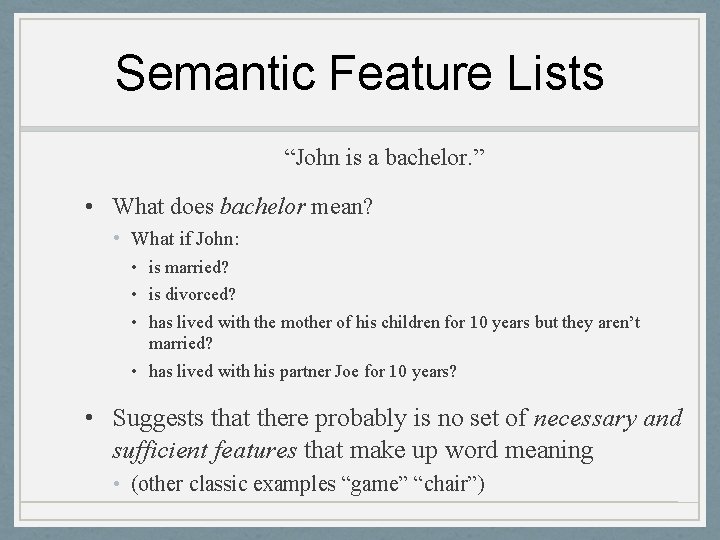
Semantic Feature Lists “John is a bachelor. ” • What does bachelor mean? • What if John: • is married? • is divorced? • has lived with the mother of his children for 10 years but they aren’t married? • has lived with his partner Joe for 10 years? • Suggests that there probably is no set of necessary and sufficient features that make up word meaning • (other classic examples “game” “chair”)
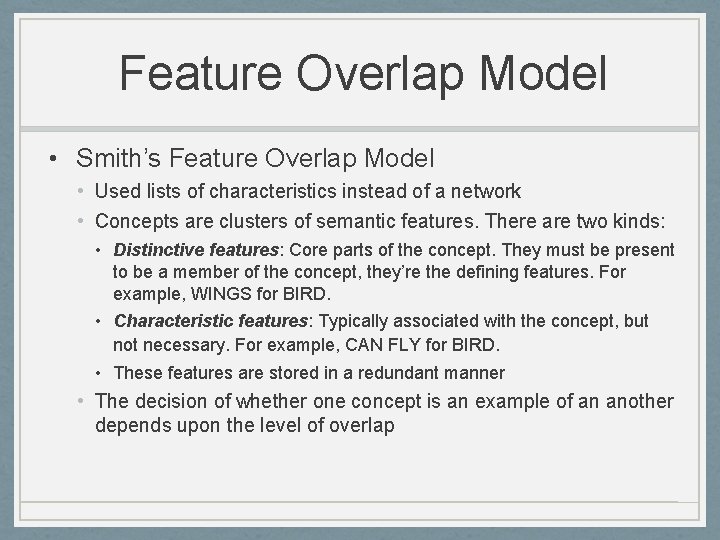
Feature Overlap Model • Smith’s Feature Overlap Model • Used lists of characteristics instead of a network • Concepts are clusters of semantic features. There are two kinds: • Distinctive features: Core parts of the concept. They must be present to be a member of the concept, they’re the defining features. For example, WINGS for BIRD. • Characteristic features: Typically associated with the concept, but not necessary. For example, CAN FLY for BIRD. • These features are stored in a redundant manner • The decision of whether one concept is an example of an another depends upon the level of overlap
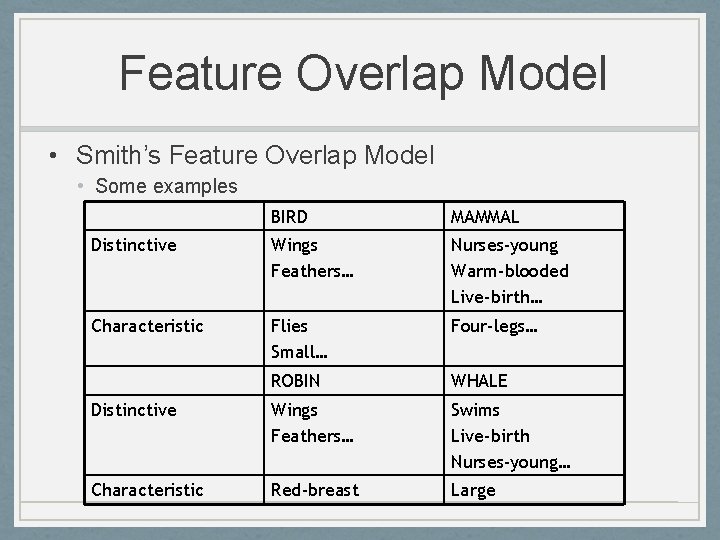
Feature Overlap Model • Smith’s Feature Overlap Model • Some examples BIRD MAMMAL Distinctive Wings Feathers… Nurses-young Warm-blooded Live-birth… Characteristic Flies Small… Four-legs… ROBIN WHALE Distinctive Wings Feathers… Swims Live-birth Nurses-young… Characteristic Red-breast Large
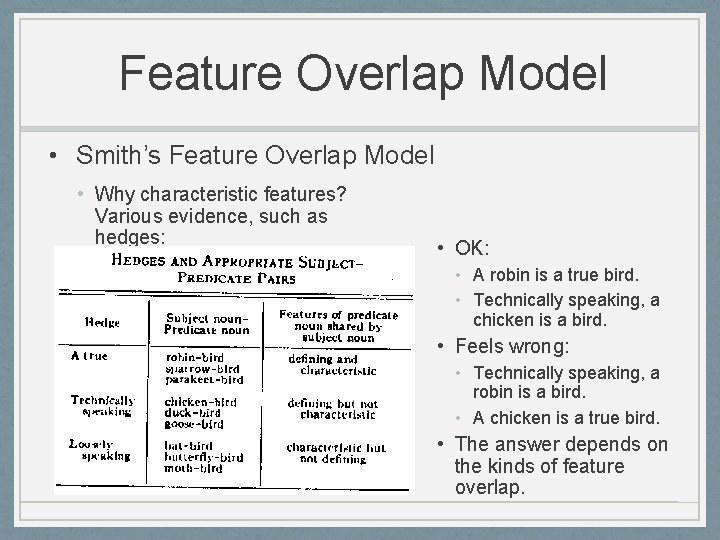
Feature Overlap Model • Smith’s Feature Overlap Model • Why characteristic features? Various evidence, such as hedges: • OK: • A robin is a true bird. • Technically speaking, a chicken is a bird. • Feels wrong: • Technically speaking, a robin is a bird. • A chicken is a true bird. • The answer depends on the kinds of feature overlap.
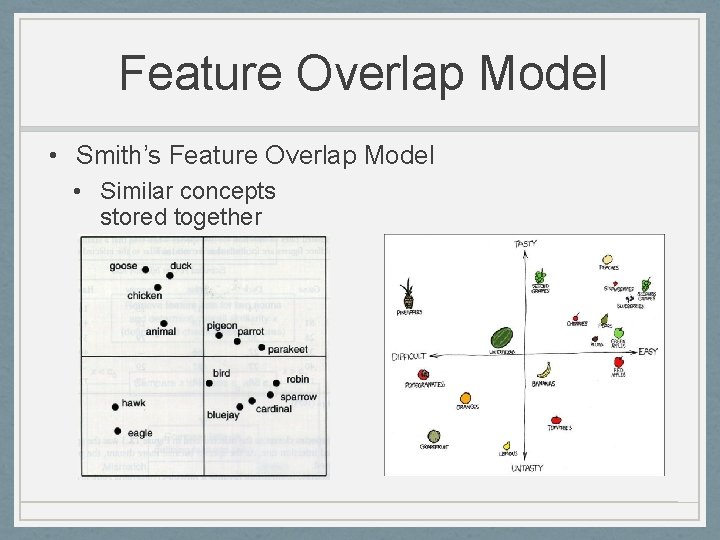
Feature Overlap Model • Smith’s Feature Overlap Model • Similar concepts stored together
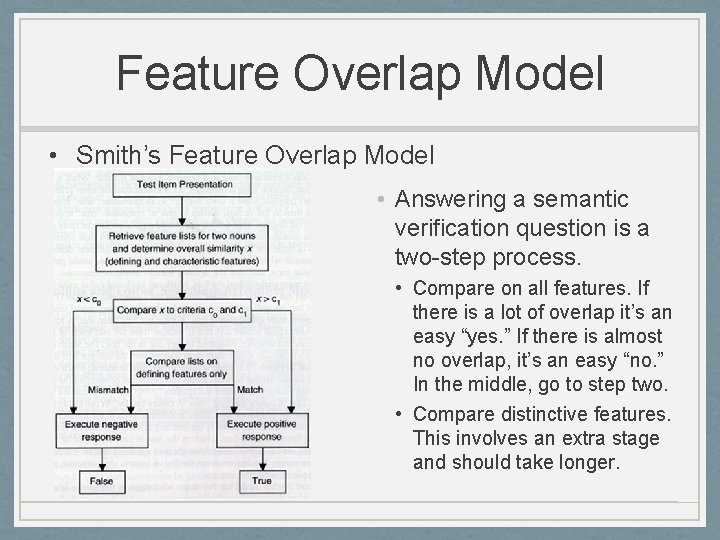
Feature Overlap Model • Smith’s Feature Overlap Model • Answering a semantic verification question is a two-step process. • Compare on all features. If there is a lot of overlap it’s an easy “yes. ” If there is almost no overlap, it’s an easy “no. ” In the middle, go to step two. • Compare distinctive features. This involves an extra stage and should take longer.
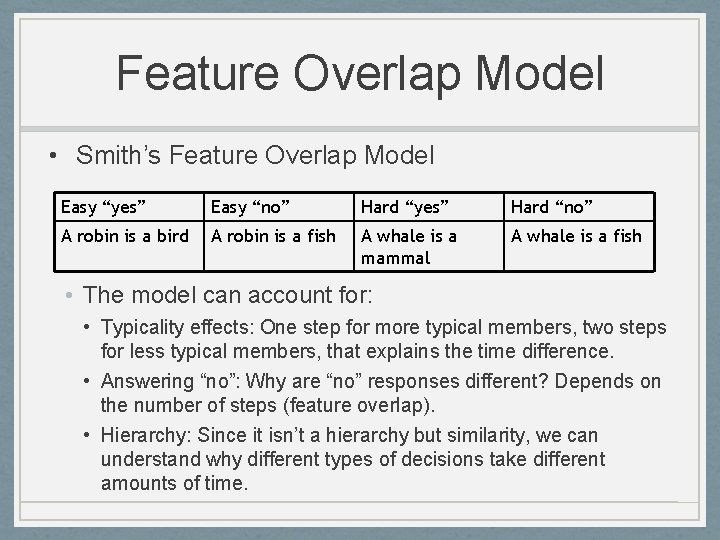
Feature Overlap Model • Smith’s Feature Overlap Model Easy “yes” Easy “no” Hard “yes” Hard “no” A robin is a bird A robin is a fish A whale is a mammal A whale is a fish • The model can account for: • Typicality effects: One step for more typical members, two steps for less typical members, that explains the time difference. • Answering “no”: Why are “no” responses different? Depends on the number of steps (feature overlap). • Hierarchy: Since it isn’t a hierarchy but similarity, we can understand why different types of decisions take different amounts of time.
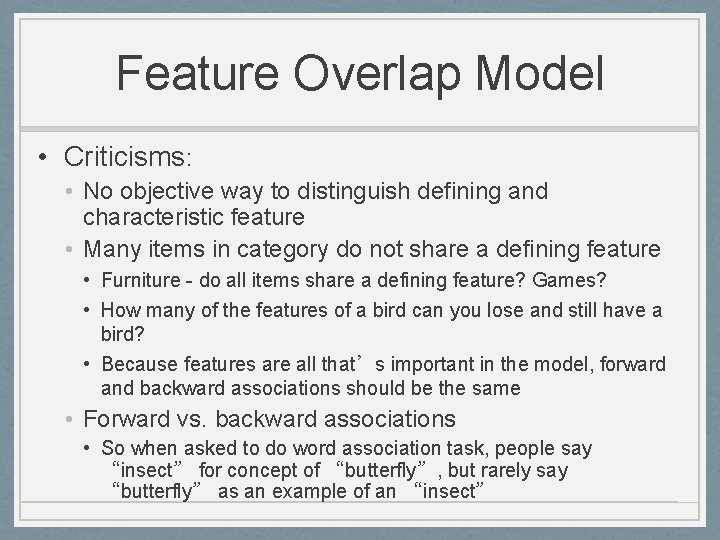
Feature Overlap Model • Criticisms: • No objective way to distinguish defining and characteristic feature • Many items in category do not share a defining feature • Furniture - do all items share a defining feature? Games? • How many of the features of a bird can you lose and still have a bird? • Because features are all that’s important in the model, forward and backward associations should be the same • Forward vs. backward associations • So when asked to do word association task, people say “insect” for concept of “butterfly”, but rarely say “butterfly” as an example of an “insect”
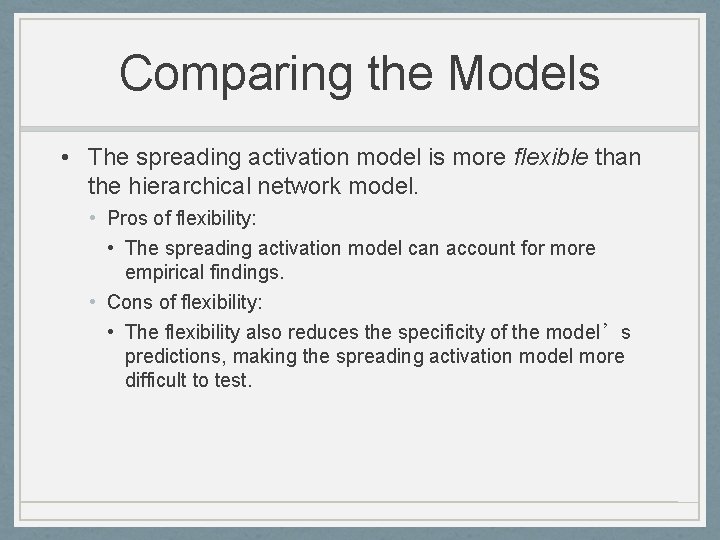
Comparing the Models • The spreading activation model is more flexible than the hierarchical network model. • Pros of flexibility: • The spreading activation model can account for more empirical findings. • Cons of flexibility: • The flexibility also reduces the specificity of the model’s predictions, making the spreading activation model more difficult to test.
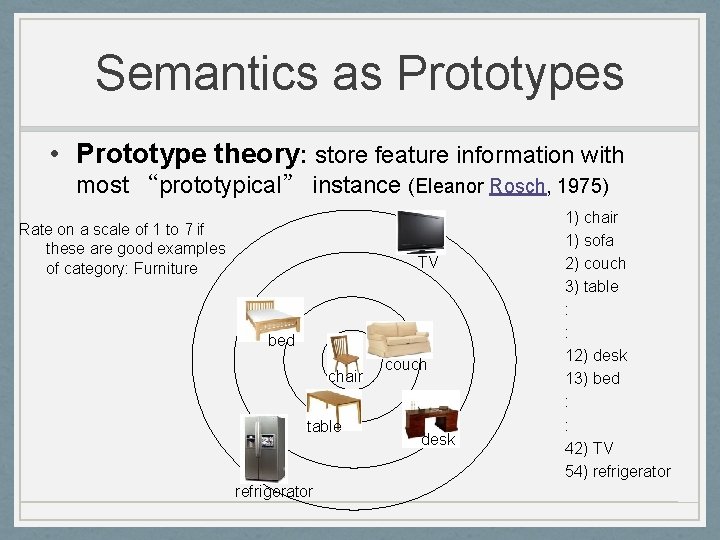
Semantics as Prototypes • Prototype theory: store feature information with most “prototypical” instance (Eleanor Rosch, 1975) Rate on a scale of 1 to 7 if these are good examples of category: Furniture TV bed chair table refrigerator couch desk 1) chair 1) sofa 2) couch 3) table : : 12) desk 13) bed : : 42) TV 54) refrigerator
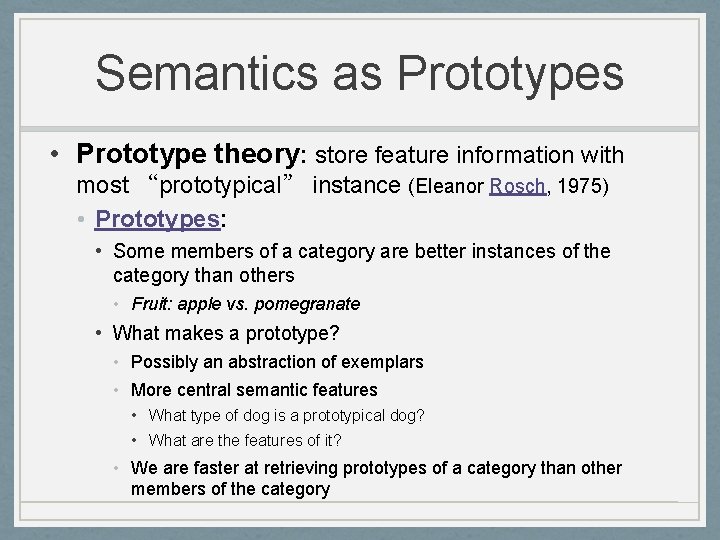
Semantics as Prototypes • Prototype theory: store feature information with most “prototypical” instance (Eleanor Rosch, 1975) • Prototypes: • Some members of a category are better instances of the category than others • Fruit: apple vs. pomegranate • What makes a prototype? • Possibly an abstraction of exemplars • More central semantic features • What type of dog is a prototypical dog? • What are the features of it? • We are faster at retrieving prototypes of a category than other members of the category
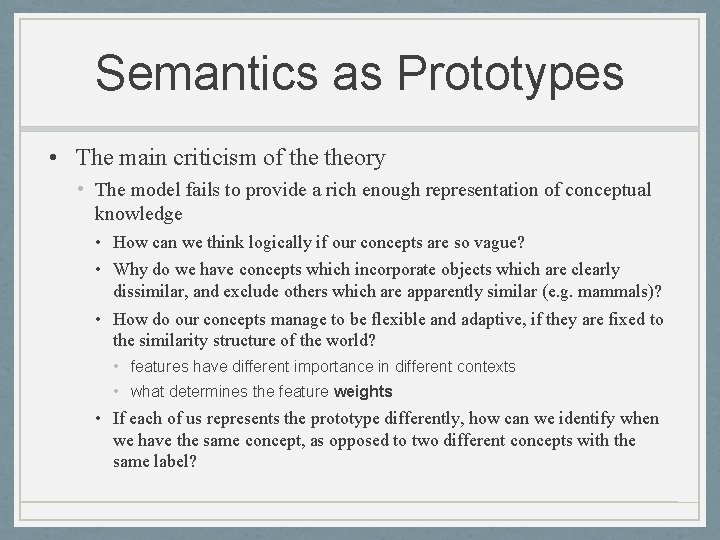
Semantics as Prototypes • The main criticism of theory • The model fails to provide a rich enough representation of conceptual knowledge • How can we think logically if our concepts are so vague? • Why do we have concepts which incorporate objects which are clearly dissimilar, and exclude others which are apparently similar (e. g. mammals)? • How do our concepts manage to be flexible and adaptive, if they are fixed to the similarity structure of the world? • features have different importance in different contexts • what determines the feature weights • If each of us represents the prototype differently, how can we identify when we have the same concept, as opposed to two different concepts with the same label?
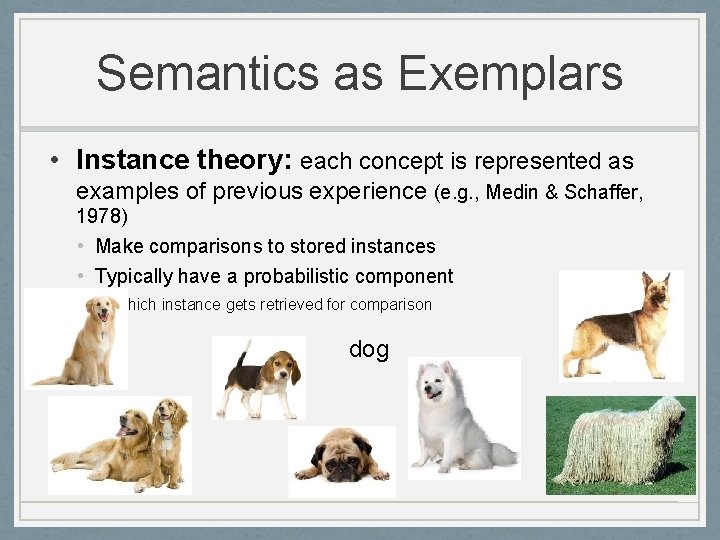
Semantics as Exemplars • Instance theory: each concept is represented as examples of previous experience (e. g. , Medin & Schaffer, 1978) • Make comparisons to stored instances • Typically have a probabilistic component • Which instance gets retrieved for comparison dog
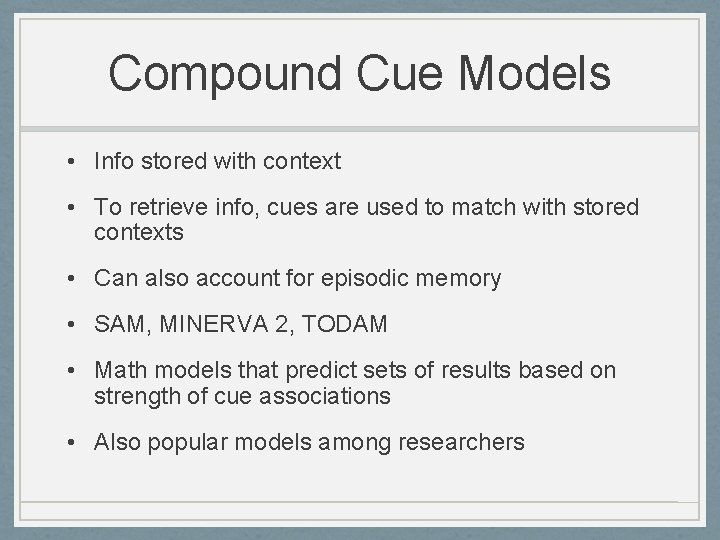
Compound Cue Models • Info stored with context • To retrieve info, cues are used to match with stored contexts • Can also account for episodic memory • SAM, MINERVA 2, TODAM • Math models that predict sets of results based on strength of cue associations • Also popular models among researchers
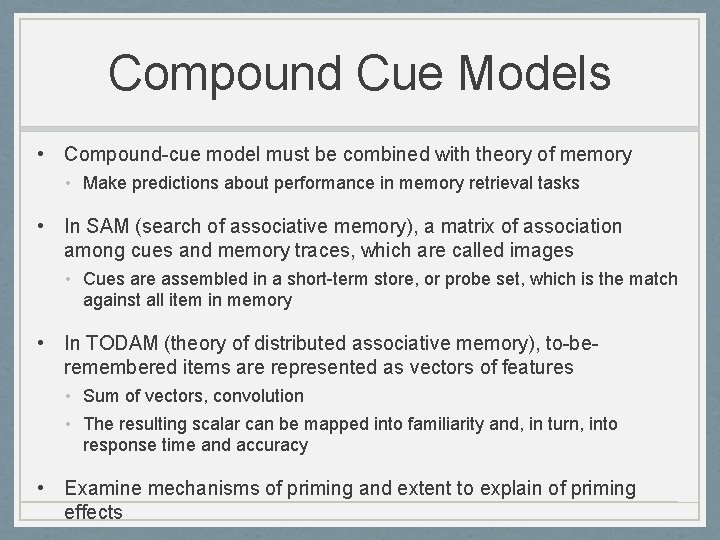
Compound Cue Models • Compound-cue model must be combined with theory of memory • Make predictions about performance in memory retrieval tasks • In SAM (search of associative memory), a matrix of association among cues and memory traces, which are called images • Cues are assembled in a short-term store, or probe set, which is the match against all item in memory • In TODAM (theory of distributed associative memory), to-beremembered items are represented as vectors of features • Sum of vectors, convolution • The resulting scalar can be mapped into familiarity and, in turn, into response time and accuracy • Examine mechanisms of priming and extent to explain of priming effects
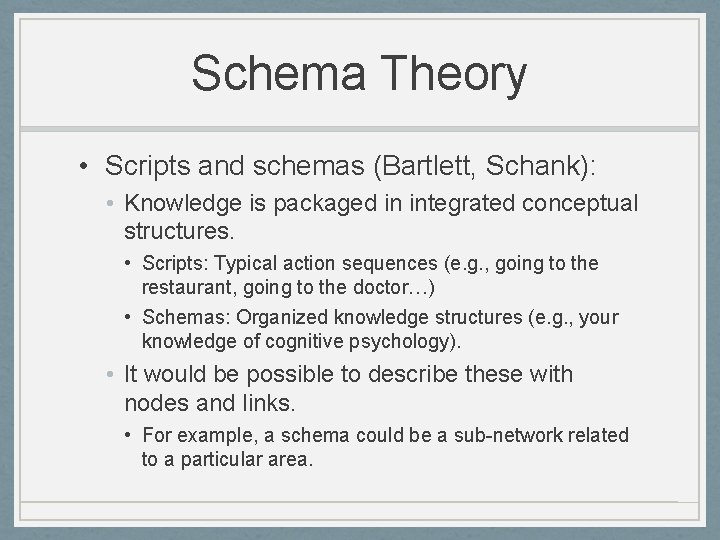
Schema Theory • Scripts and schemas (Bartlett, Schank): • Knowledge is packaged in integrated conceptual structures. • Scripts: Typical action sequences (e. g. , going to the restaurant, going to the doctor…) • Schemas: Organized knowledge structures (e. g. , your knowledge of cognitive psychology). • It would be possible to describe these with nodes and links. • For example, a schema could be a sub-network related to a particular area.
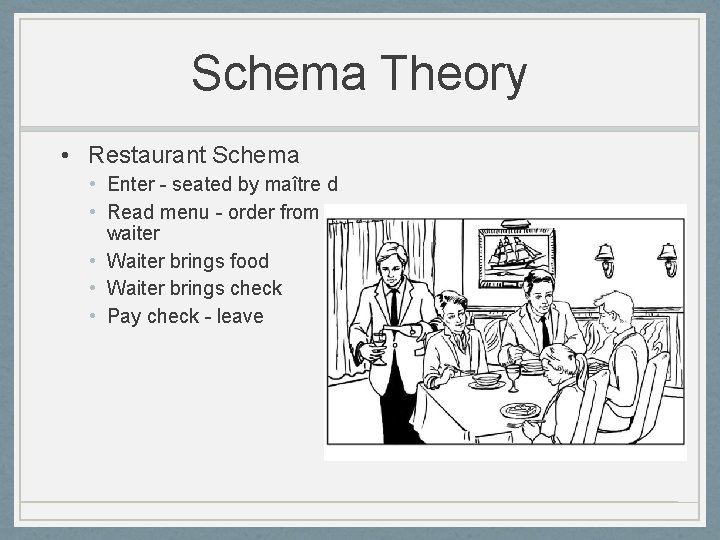
Schema Theory • Restaurant Schema • Enter - seated by maître d • Read menu - order from waiter • Waiter brings food • Waiter brings check • Pay check - leave
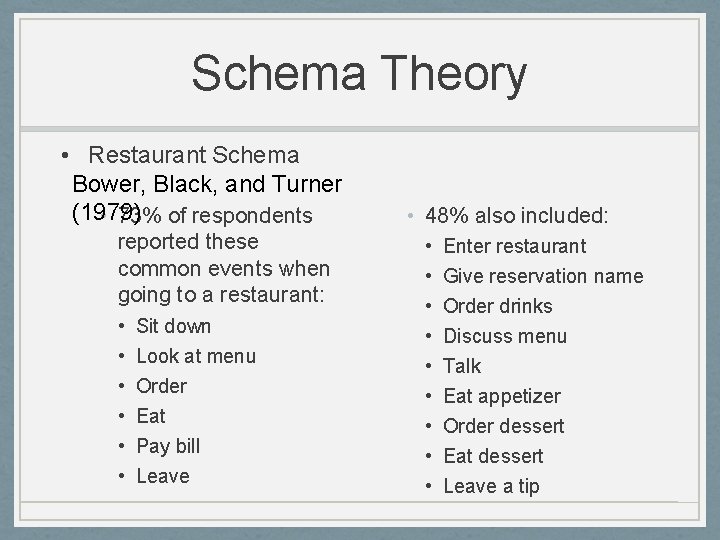
Schema Theory • Restaurant Schema Bower, Black, and Turner (1979) • 73% of respondents reported these common events when going to a restaurant: • • • Sit down Look at menu Order Eat Pay bill Leave • 48% also included: • • • Enter restaurant Give reservation name Order drinks Discuss menu Talk Eat appetizer Order dessert Eat dessert Leave a tip
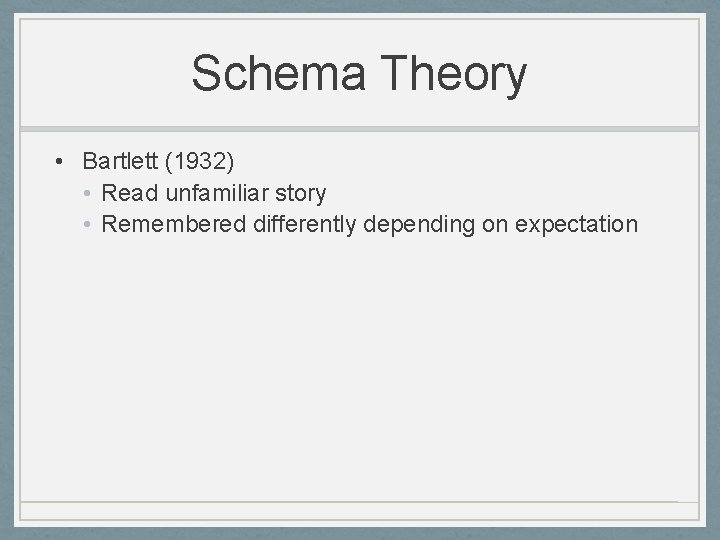
Schema Theory • Bartlett (1932) • Read unfamiliar story • Remembered differently depending on expectation
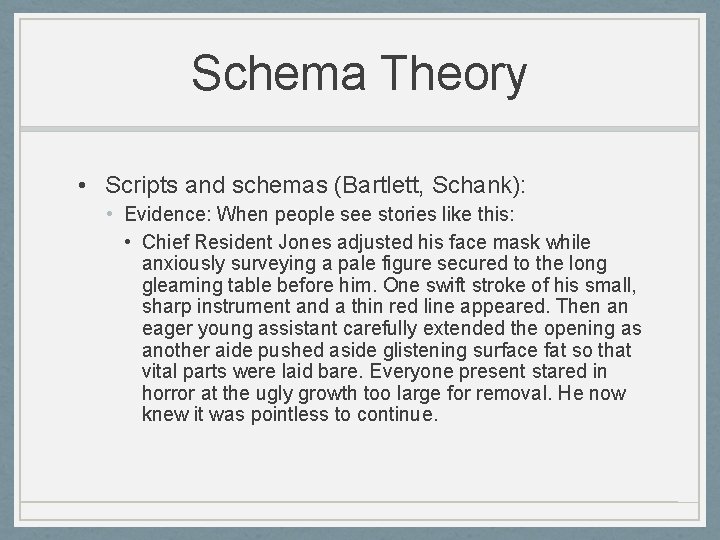
Schema Theory • Scripts and schemas (Bartlett, Schank): • Evidence: When people see stories like this: • Chief Resident Jones adjusted his face mask while anxiously surveying a pale figure secured to the long gleaming table before him. One swift stroke of his small, sharp instrument and a thin red line appeared. Then an eager young assistant carefully extended the opening as another aide pushed aside glistening surface fat so that vital parts were laid bare. Everyone present stared in horror at the ugly growth too large for removal. He now knew it was pointless to continue.
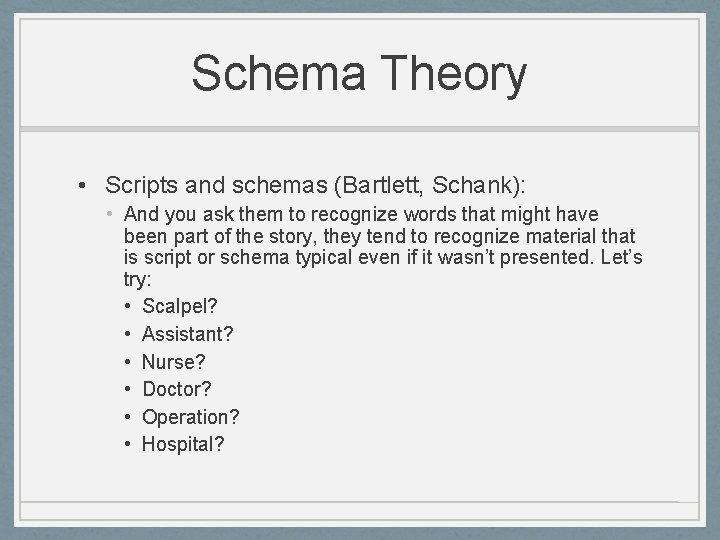
Schema Theory • Scripts and schemas (Bartlett, Schank): • And you ask them to recognize words that might have been part of the story, they tend to recognize material that is script or schema typical even if it wasn’t presented. Let’s try: • Scalpel? • Assistant? • Nurse? • Doctor? • Operation? • Hospital?
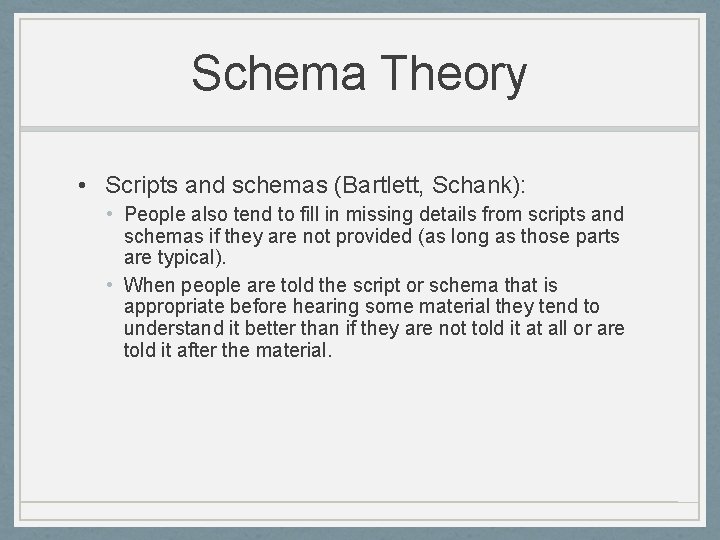
Schema Theory • Scripts and schemas (Bartlett, Schank): • People also tend to fill in missing details from scripts and schemas if they are not provided (as long as those parts are typical). • When people are told the script or schema that is appropriate before hearing some material they tend to understand it better than if they are not told it at all or are told it after the material.
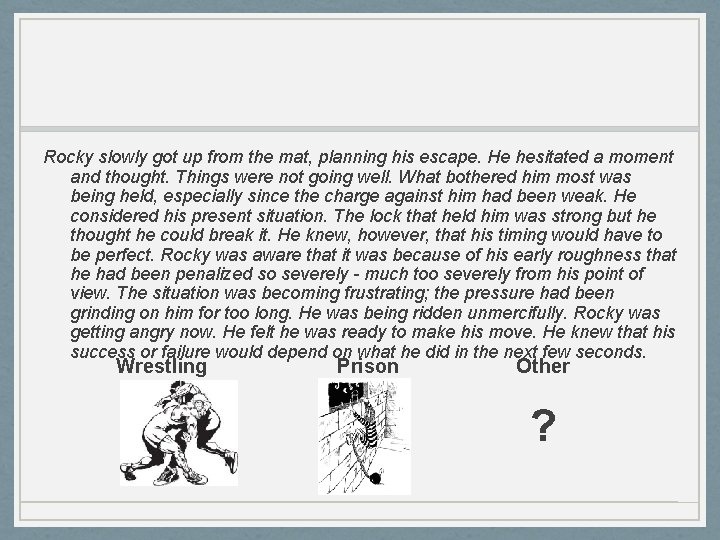
Rocky slowly got up from the mat, planning his escape. He hesitated a moment and thought. Things were not going well. What bothered him most was being held, especially since the charge against him had been weak. He considered his present situation. The lock that held him was strong but he thought he could break it. He knew, however, that his timing would have to be perfect. Rocky was aware that it was because of his early roughness that he had been penalized so severely - much too severely from his point of view. The situation was becoming frustrating; the pressure had been grinding on him for too long. He was being ridden unmercifully. Rocky was getting angry now. He felt he was ready to make his move. He knew that his success or failure would depend on what he did in the next few seconds. Wrestling Prison Other ?
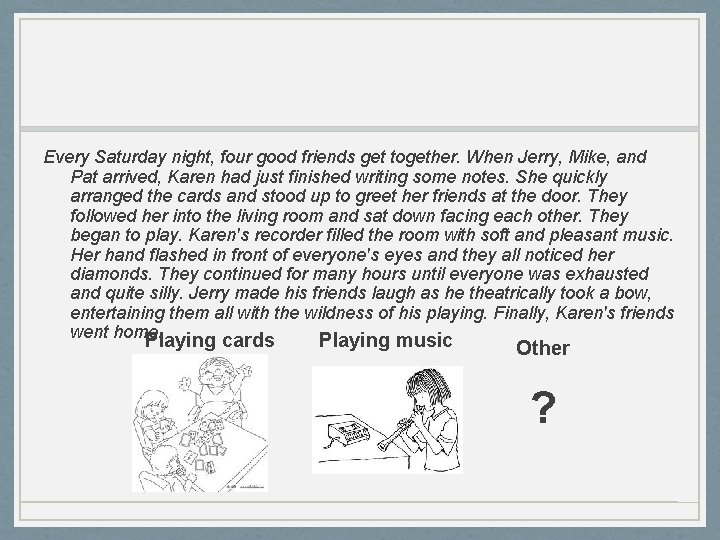
Every Saturday night, four good friends get together. When Jerry, Mike, and Pat arrived, Karen had just finished writing some notes. She quickly arranged the cards and stood up to greet her friends at the door. They followed her into the living room and sat down facing each other. They began to play. Karen's recorder filled the room with soft and pleasant music. Her hand flashed in front of everyone's eyes and they all noticed her diamonds. They continued for many hours until everyone was exhausted and quite silly. Jerry made his friends laugh as he theatrically took a bow, entertaining them all with the wildness of his playing. Finally, Karen's friends went home. Playing cards Playing music Other ?
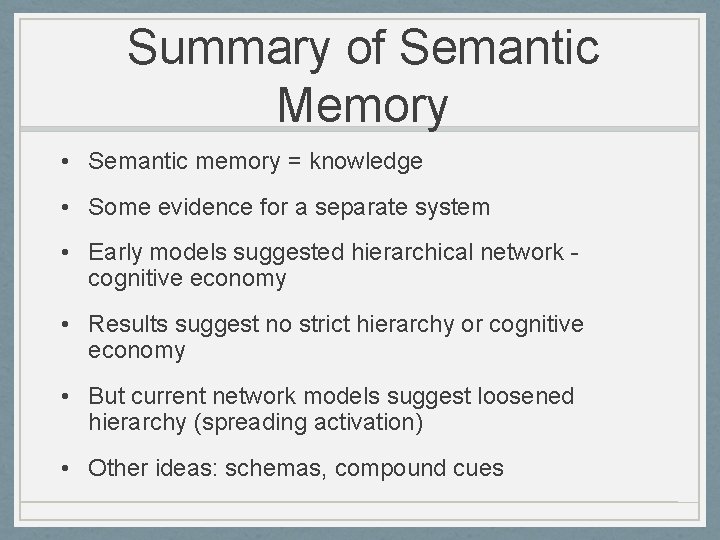
Summary of Semantic Memory • Semantic memory = knowledge • Some evidence for a separate system • Early models suggested hierarchical network cognitive economy • Results suggest no strict hierarchy or cognitive economy • But current network models suggest loosened hierarchy (spreading activation) • Other ideas: schemas, compound cues
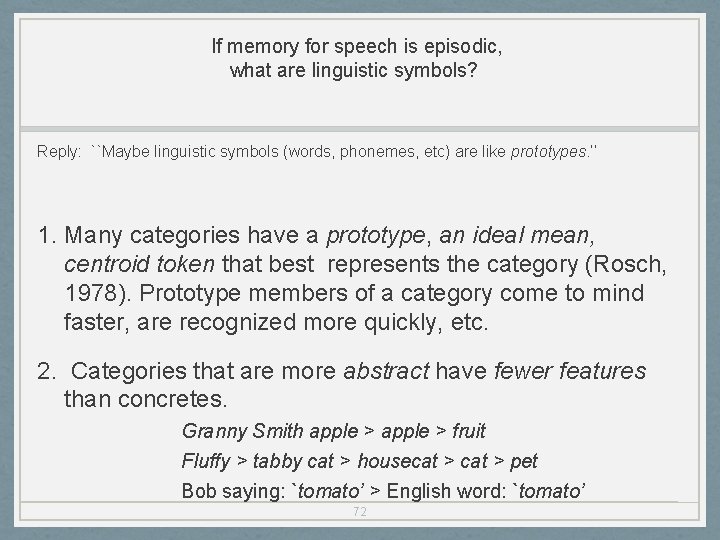
If memory for speech is episodic, what are linguistic symbols? Reply: ``Maybe linguistic symbols (words, phonemes, etc) are like prototypes. ’’ 1. Many categories have a prototype, an ideal mean, centroid token that best represents the category (Rosch, 1978). Prototype members of a category come to mind faster, are recognized more quickly, etc. 2. Categories that are more abstract have fewer features than concretes. Granny Smith apple > fruit Fluffy > tabby cat > housecat > pet Bob saying: `tomato’ > English word: `tomato’ 72
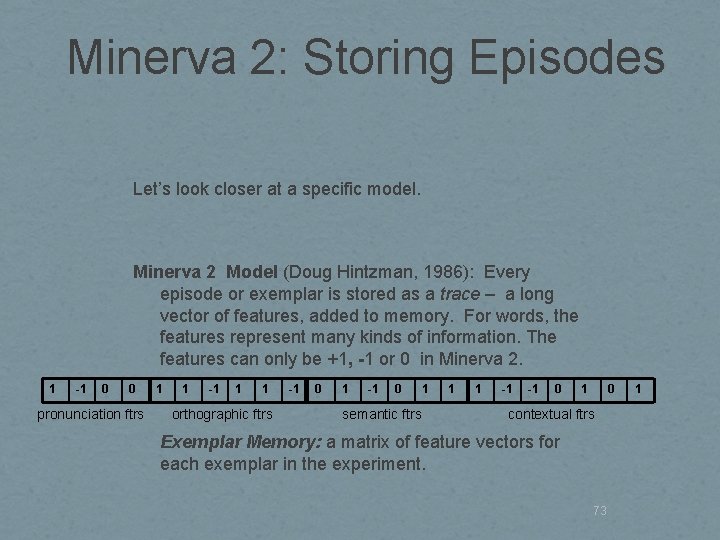
Minerva 2: Storing Episodes Let’s look closer at a specific model. Minerva 2 Model (Doug Hintzman, 1986): Every episode or exemplar is stored as a trace – a long vector of features, added to memory. For words, the features represent many kinds of information. The features can only be +1, -1 or 0 in Minerva 2. 1 -1 0 0 pronunciation ftrs 1 1 -1 1 1 orthographic ftrs -1 0 1 semantic ftrs 1 1 -1 -1 0 contextual ftrs Exemplar Memory: a matrix of feature vectors for each exemplar in the experiment. 73 1
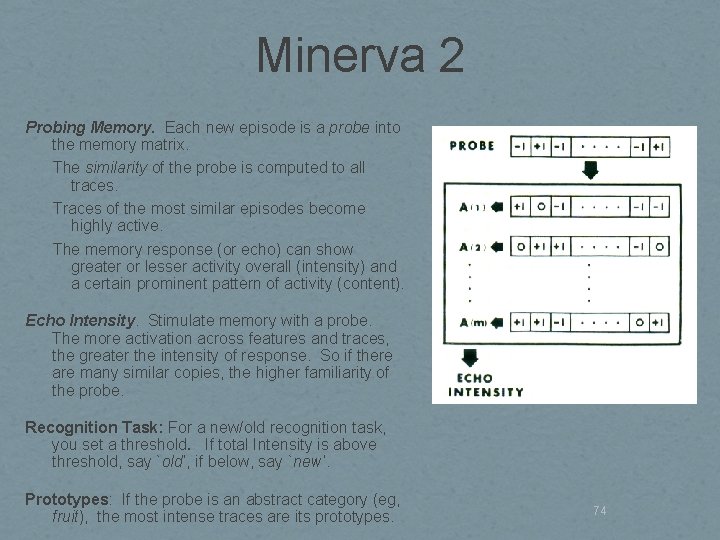
Minerva 2 Probing Memory. Each new episode is a probe into the memory matrix. The similarity of the probe is computed to all traces. Traces of the most similar episodes become highly active. The memory response (or echo) can show greater or lesser activity overall (intensity) and a certain prominent pattern of activity (content). Echo Intensity. Stimulate memory with a probe. The more activation across features and traces, the greater the intensity of response. So if there are many similar copies, the higher familiarity of the probe. Recognition Task: For a new/old recognition task, you set a threshold. If total Intensity is above threshold, say `old’, if below, say `new’. Prototypes: If the probe is an abstract category (eg, fruit), the most intense traces are its prototypes. 74
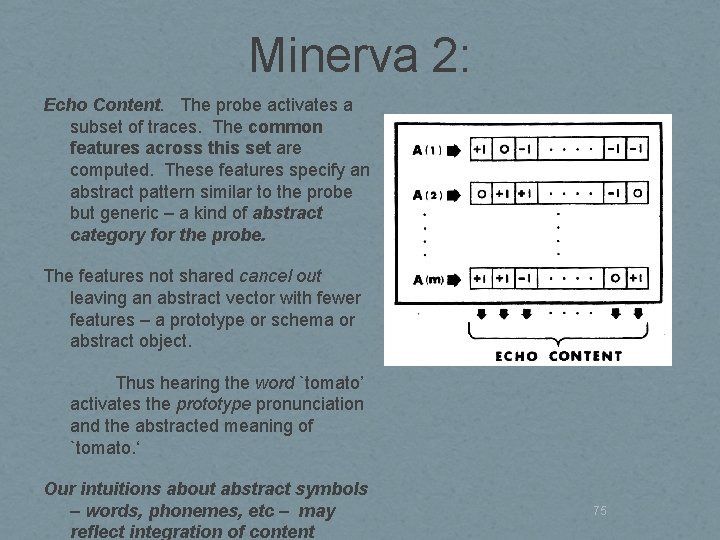
Minerva 2: Echo Content. The probe activates a subset of traces. The common features across this set are computed. These features specify an abstract pattern similar to the probe but generic – a kind of abstract category for the probe. The features not shared cancel out leaving an abstract vector with fewer features – a prototype or schema or abstract object. Thus hearing the word `tomato’ activates the prototype pronunciation and the abstracted meaning of `tomato. ‘ Our intuitions about abstract symbols – words, phonemes, etc – may reflect integration of content 75
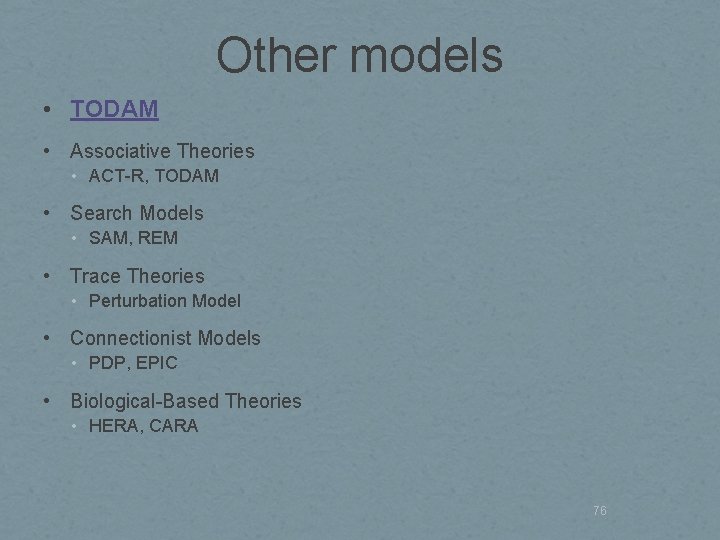
Other models • TODAM • Associative Theories • ACT-R, TODAM • Search Models • SAM, REM • Trace Theories • Perturbation Model • Connectionist Models • PDP, EPIC • Biological-Based Theories • HERA, CARA 76
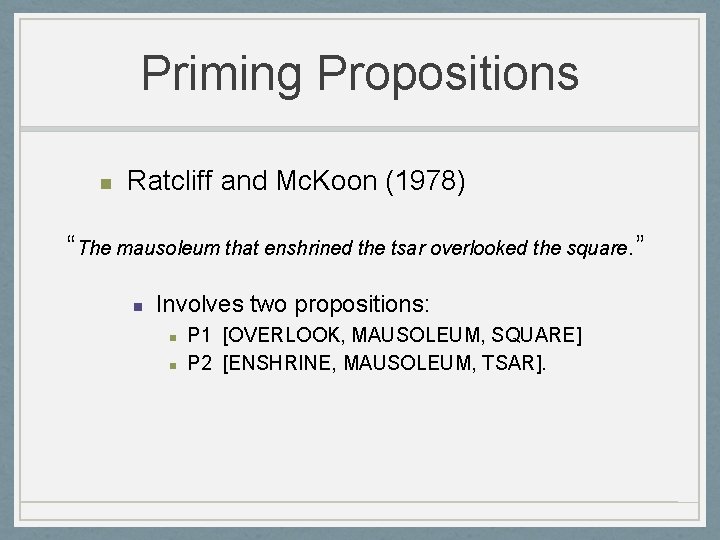
Priming Propositions n Ratcliff and Mc. Koon (1978) “The mausoleum that enshrined the tsar overlooked the square. ” n Involves two propositions: n n P 1 [OVERLOOK, MAUSOLEUM, SQUARE] P 2 [ENSHRINE, MAUSOLEUM, TSAR].
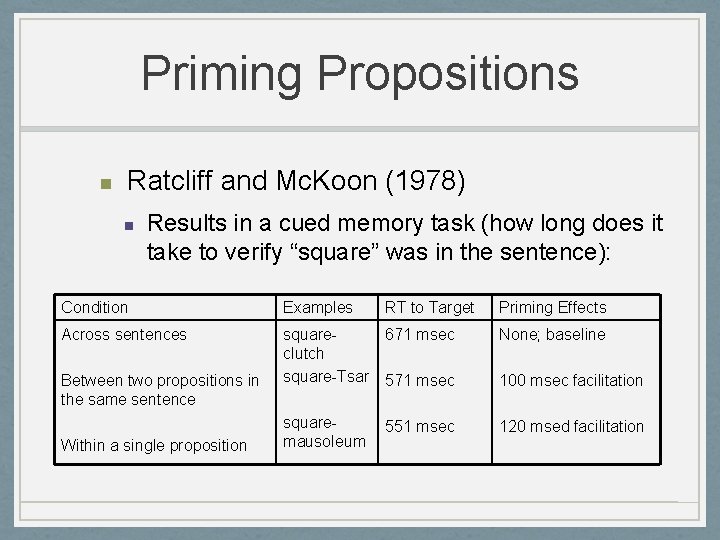
Priming Propositions n Ratcliff and Mc. Koon (1978) n Results in a cued memory task (how long does it take to verify “square” was in the sentence): Condition Examples Across sentences square 671 msec clutch square-Tsar 571 msec None; baseline squaremausoleum 120 msed facilitation Between two propositions in the same sentence Within a single proposition RT to Target 551 msec Priming Effects 100 msec facilitation
Episodic vs semantic memory
Ogle 2007 blg 368 lb
Sta 302
Stars-368 av
Ece 368
Ece 368
Ece 368
Ece 368
Ece 465
Cs 368 uw madison
Bc 368
Pvu official announcement
R/announcements!
Church announcements
Fahrenheit 451 pages
Kayl announcements
General announcements
Euro psy
Ucf psychology major
Psy2055
Psy 2055
Psy2055
Psy
Daniel sanin
Psy
Psy
Phonological loop
Psy
Psy 226
Psy
Psy academy
Damasio
Psy walsh
Nenarocny pes
Kuriatko skloňovanie
11 psy
Regression psy
Psy
What is adoloscence
Forma geometrica 4 lati
Procedural vs substantive due process
Grande rhetra
Conservazione del moto
Eleanor rosch carrot
Implicit memory vs explicit memory
Long term memory vs short term memory
Internal memory and external memory
Primary memory and secondary memory
Logical memory is broken into
Which memory is the actual working memory?
Virtual memory and cache memory
Virtual memory in memory hierarchy consists of
Eidetic memory vs iconic memory
Symmetric shared memory architecture
Human memory management
Types of human memory
Human vs non human bones
Cna chapter 8 human needs and human development
Chapter 8 human needs and human development
Human development index definition ap human geography
Human nouns
Semantic understanding
Semantic vector
Semantic web stack
Examples of sense and reference in semantics
Scope of semantics
Graphophonic cues definition
Semantic mismatch example
Contoh word for word translation
Syntax vs semantic
Swrl tutorial
Stylistic semasiology
Semantic mismatch example
Hyponymy
Semantic primes
Syntax vs semantic
Semantic answers
Semantic primes
Semantics example