Statistical Approaches to Analysis of Traditional Chinese Medicine
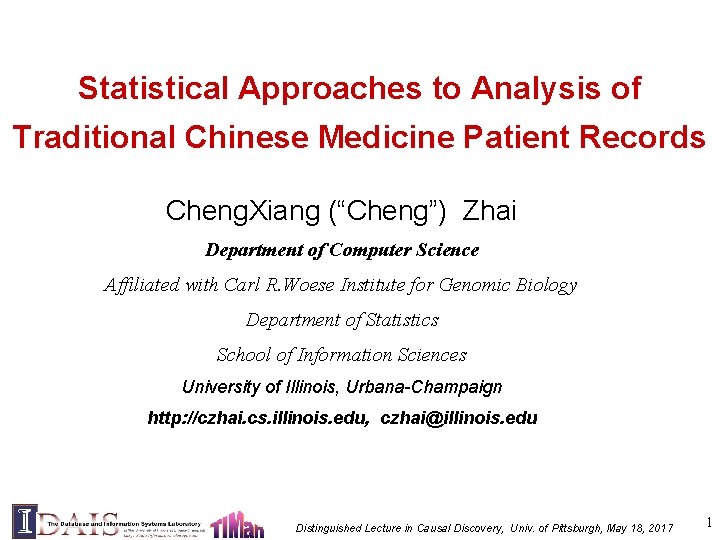
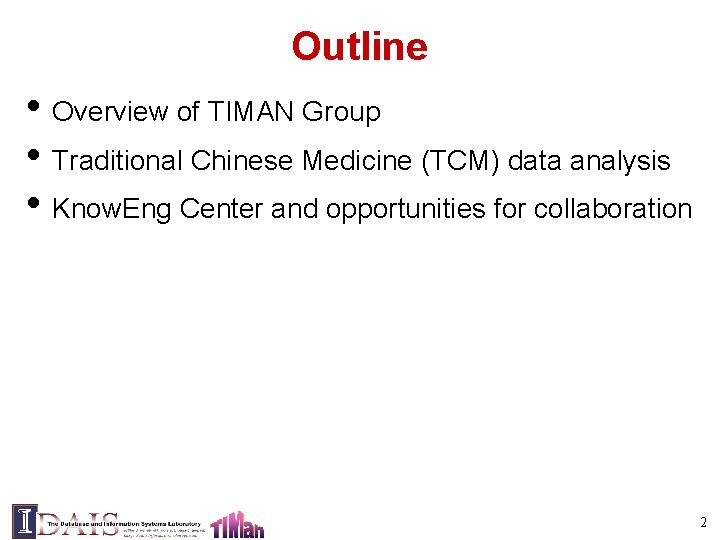
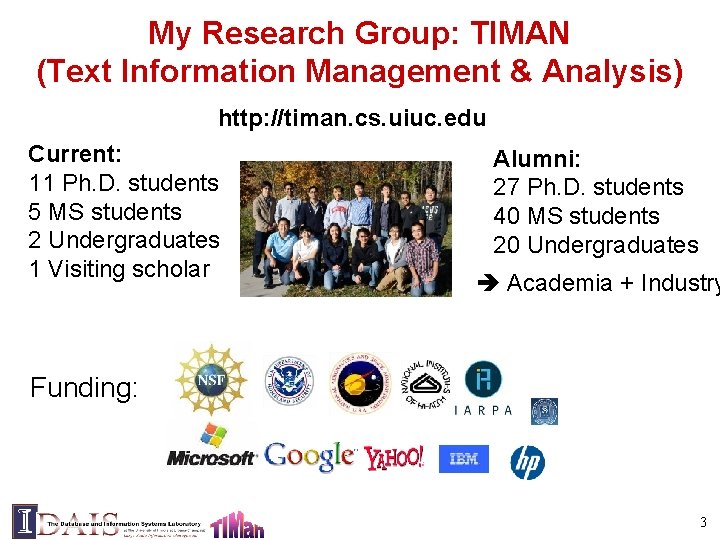
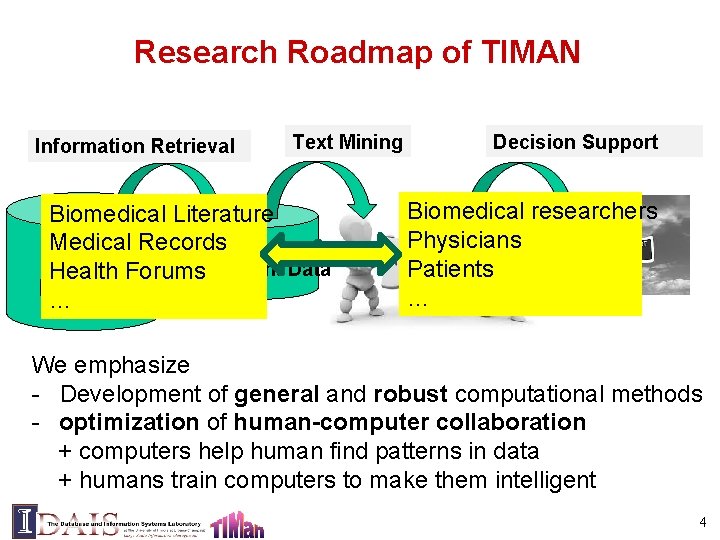
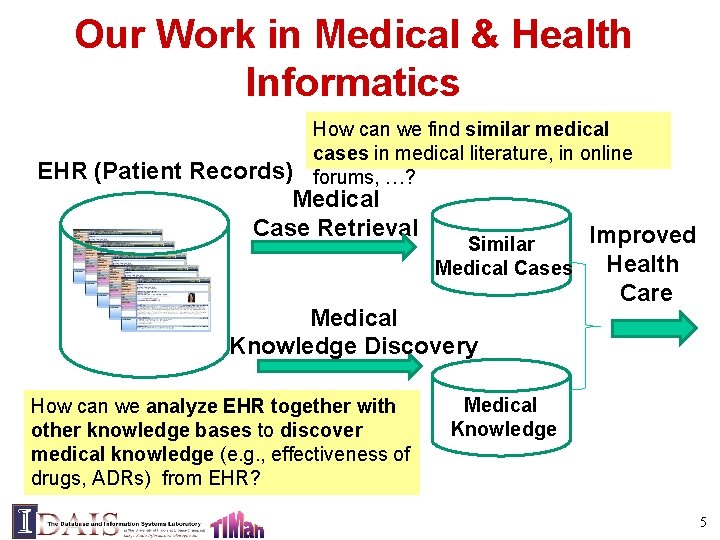
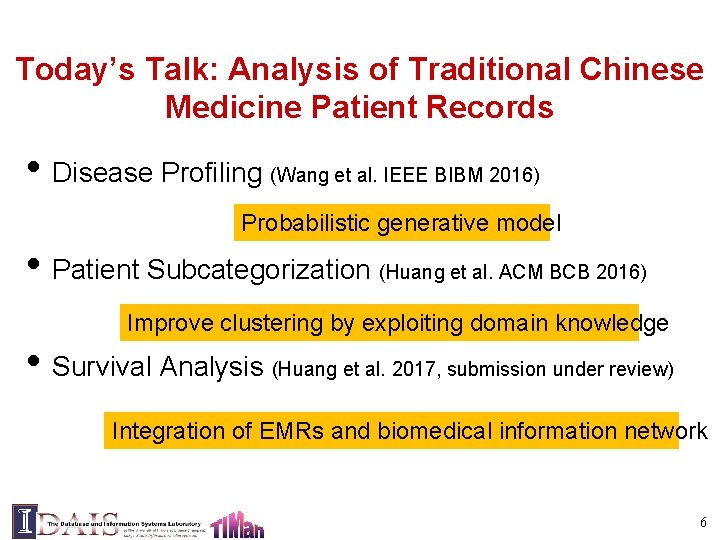
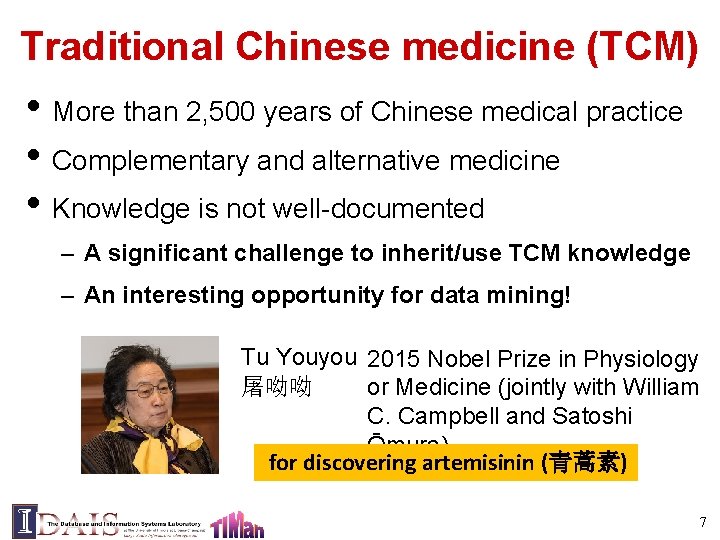
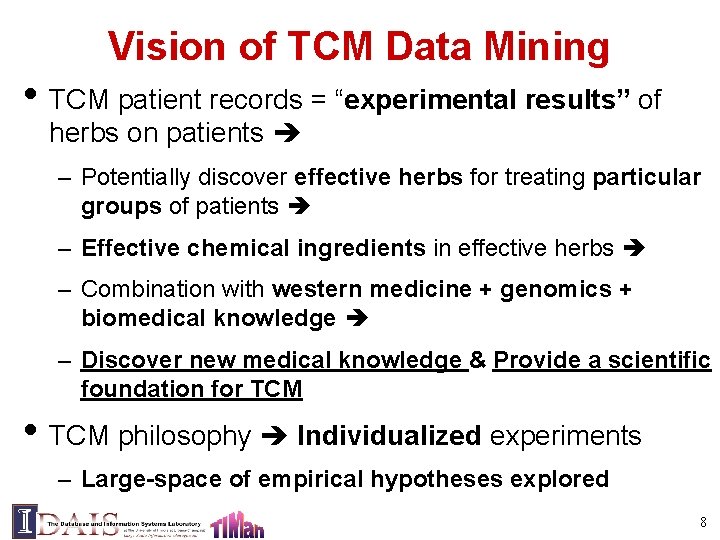
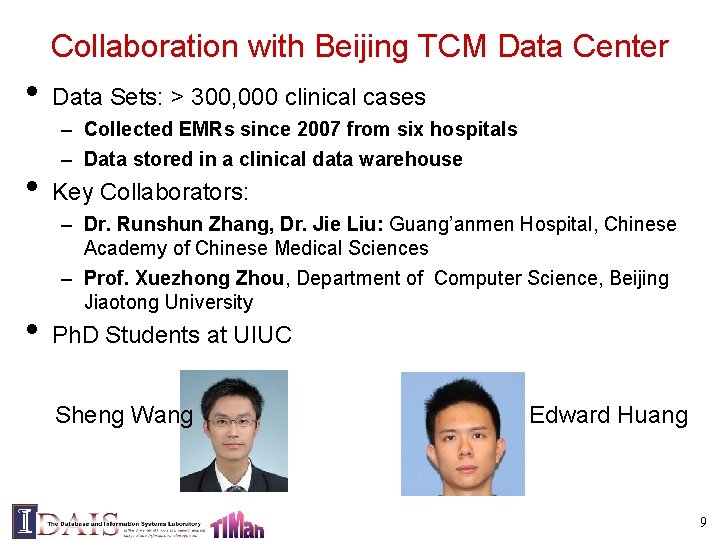
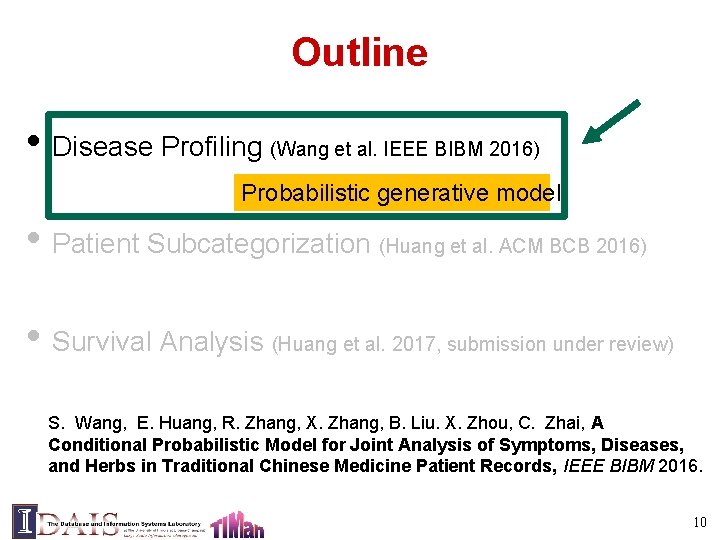
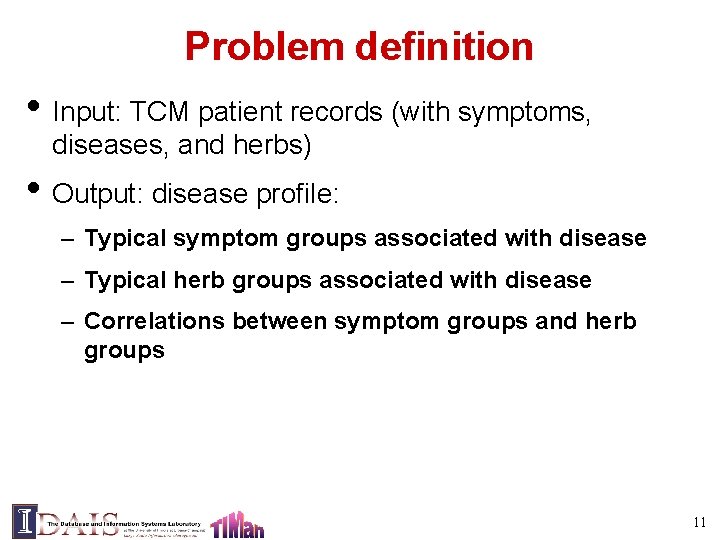
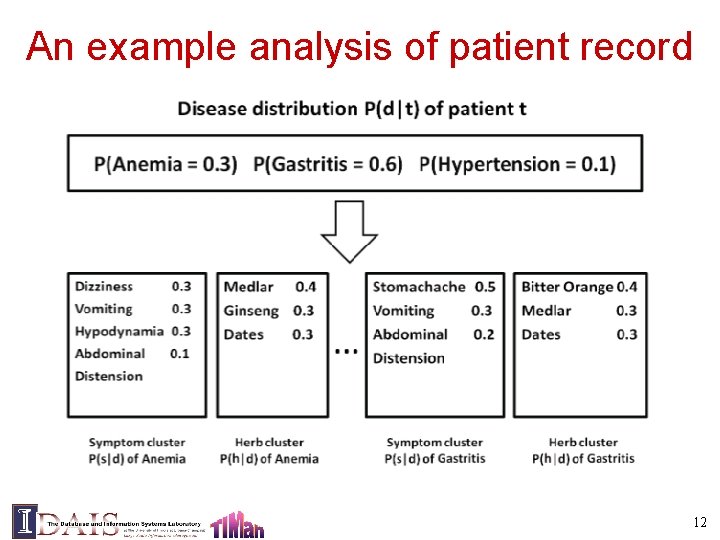
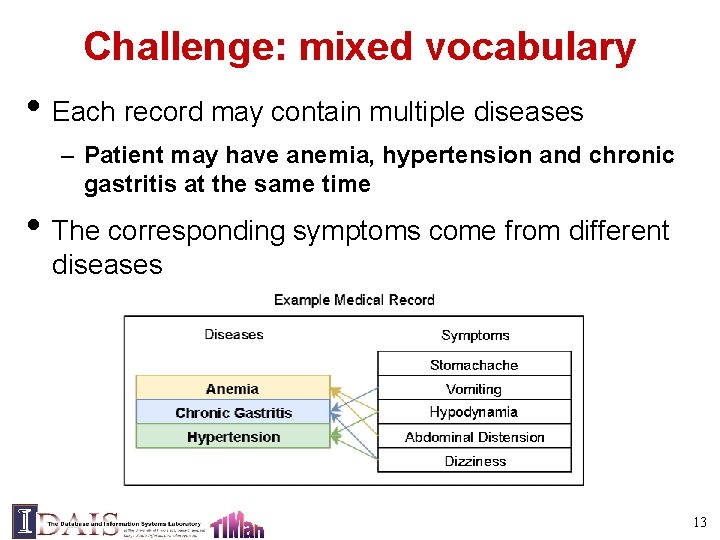
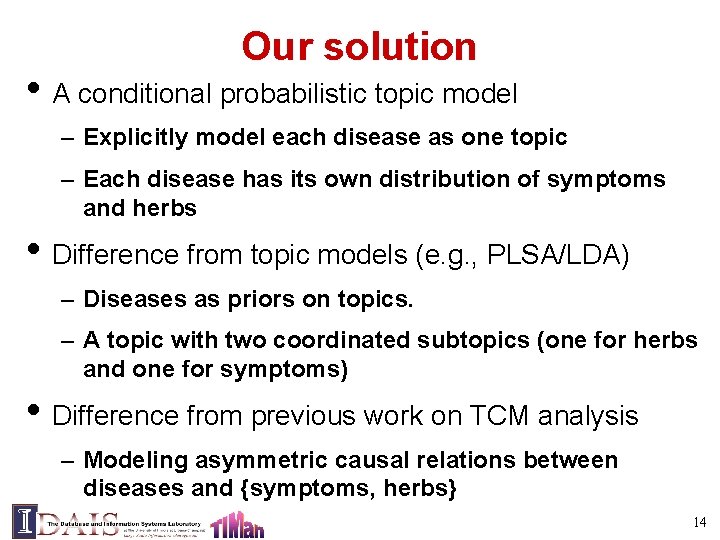
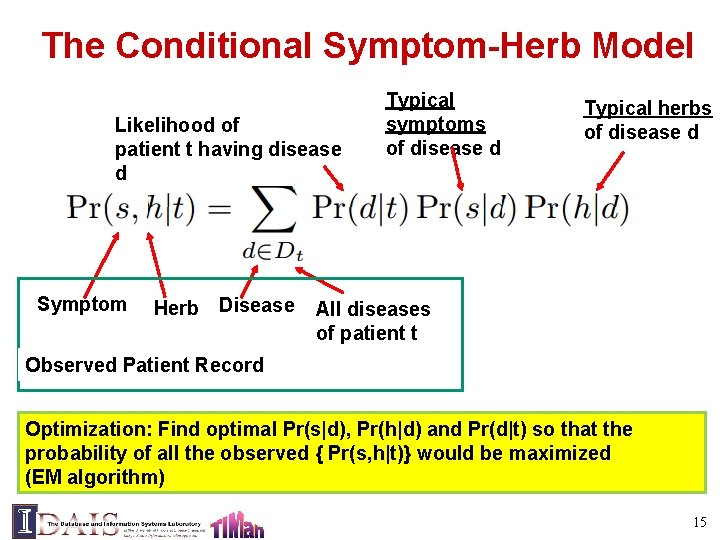
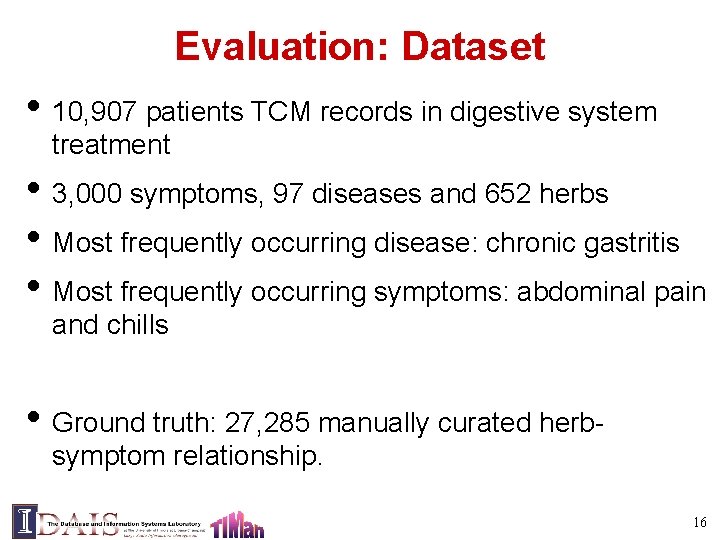
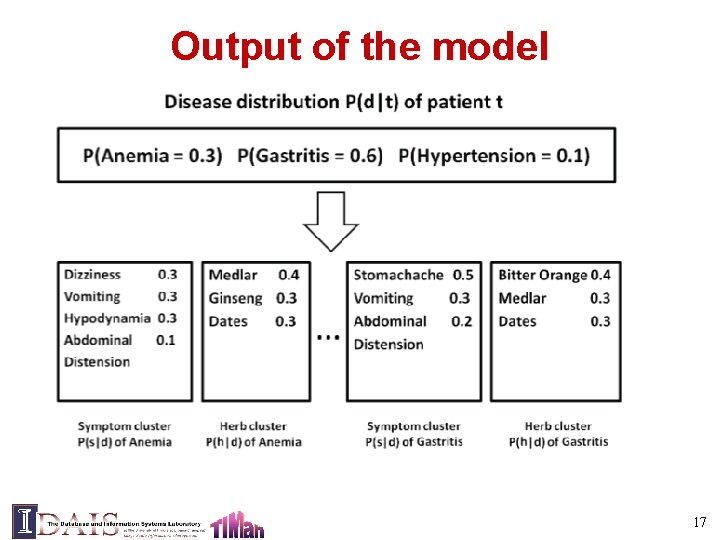
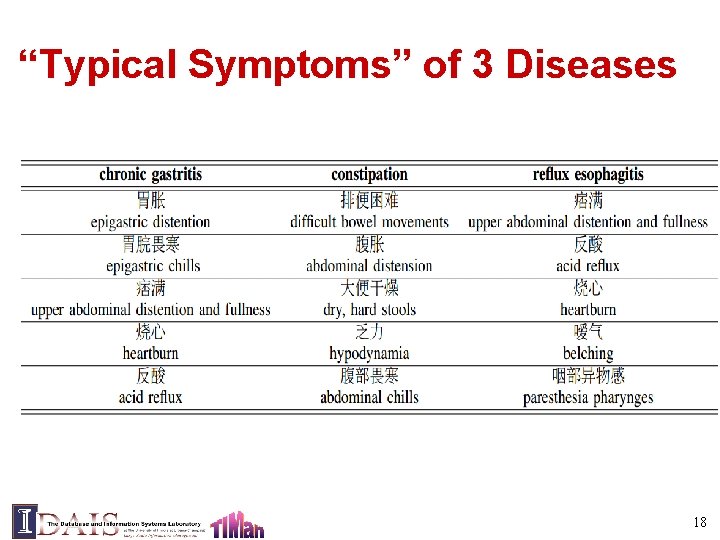
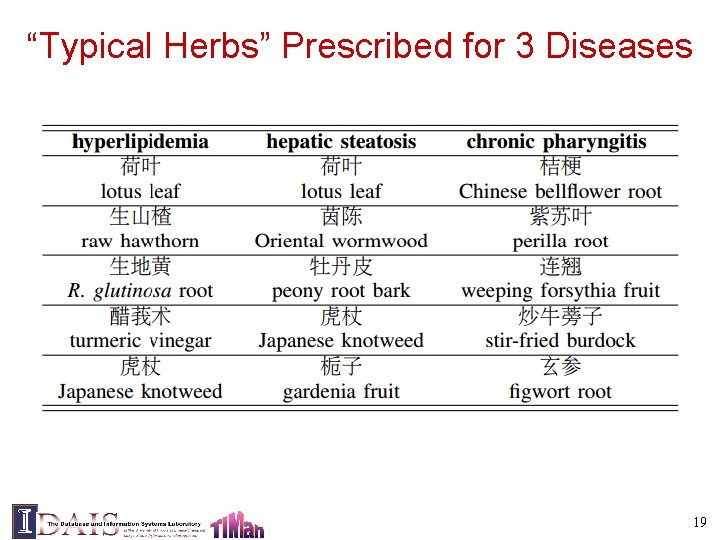
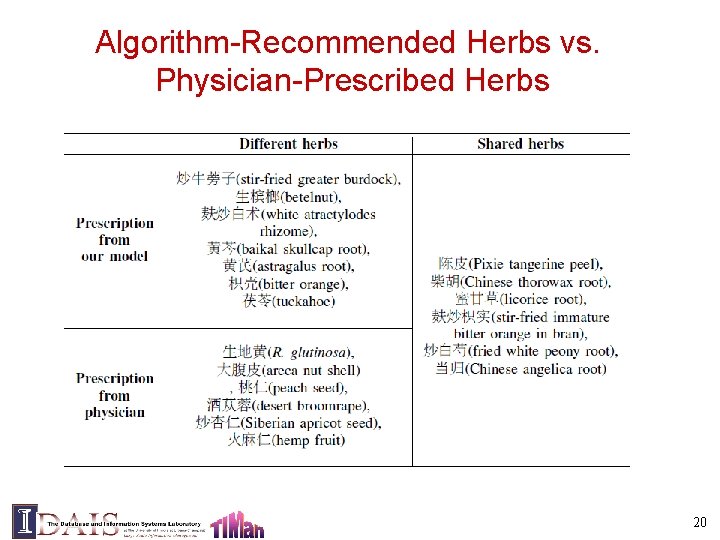
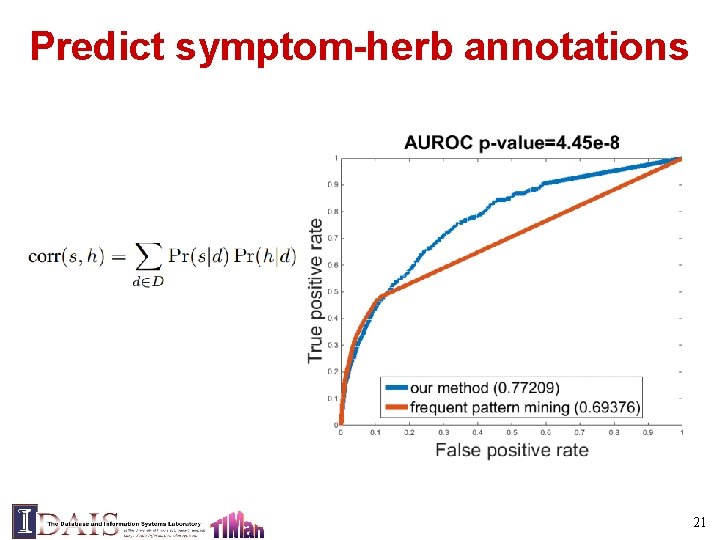
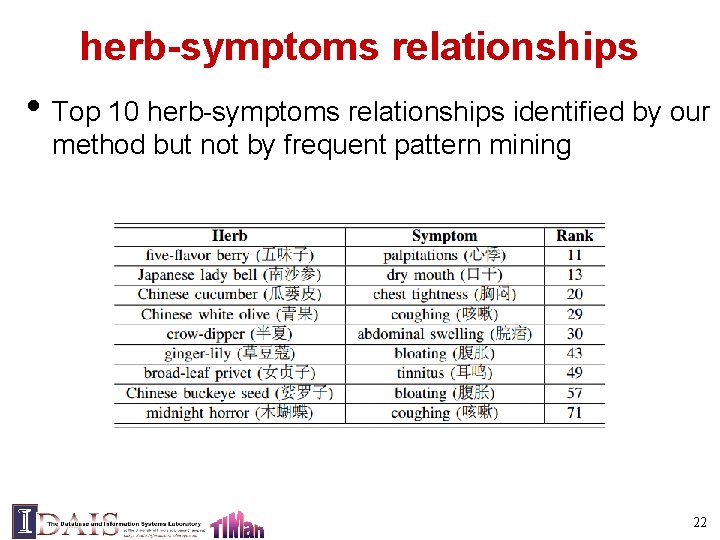
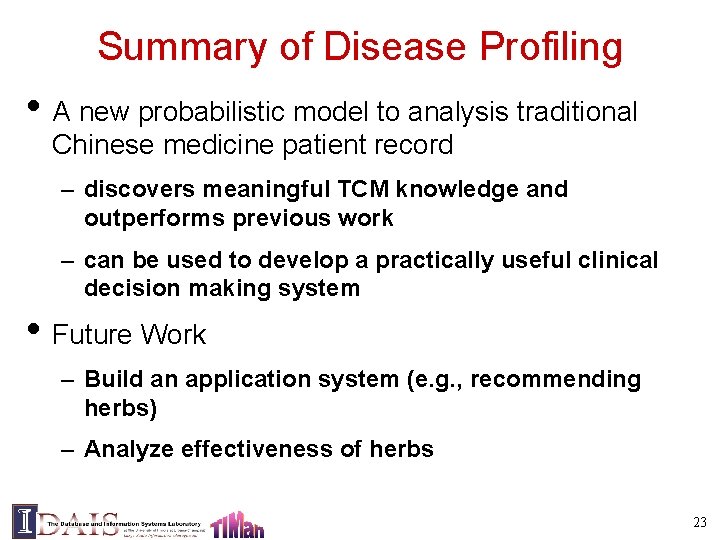
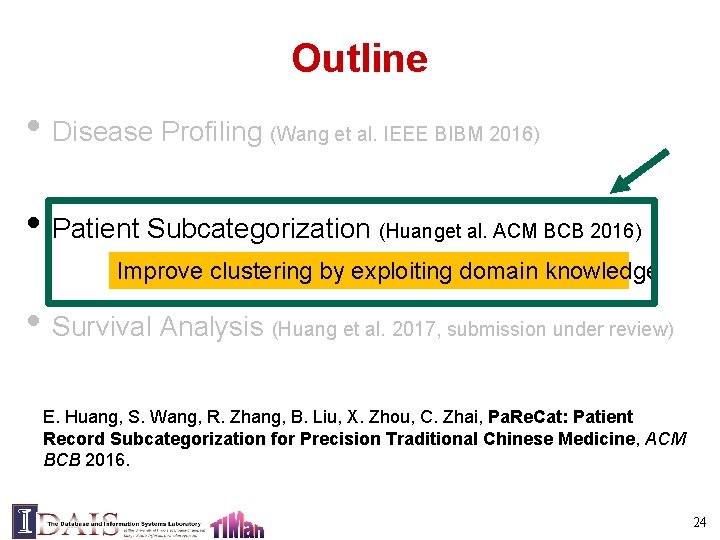
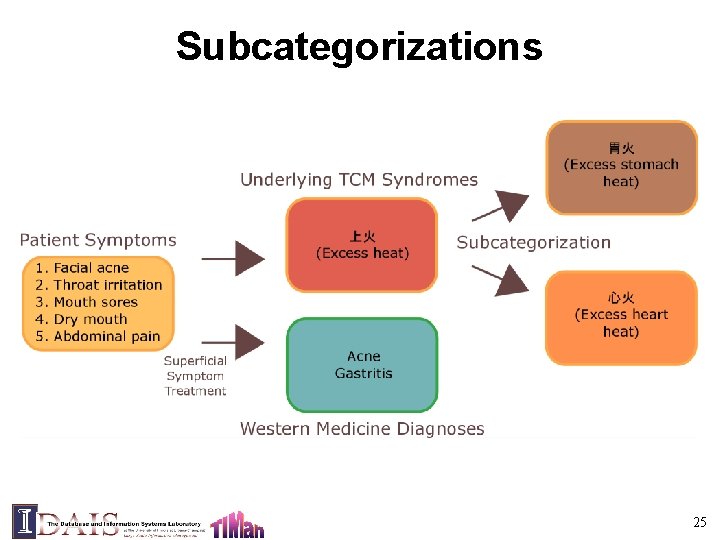
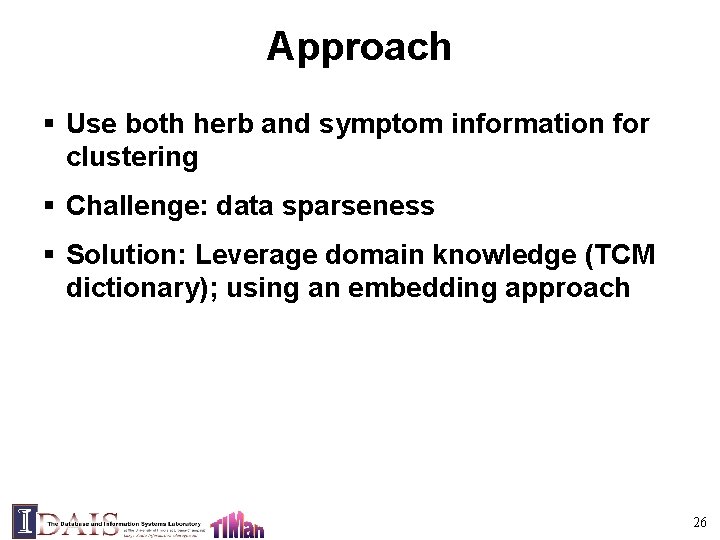
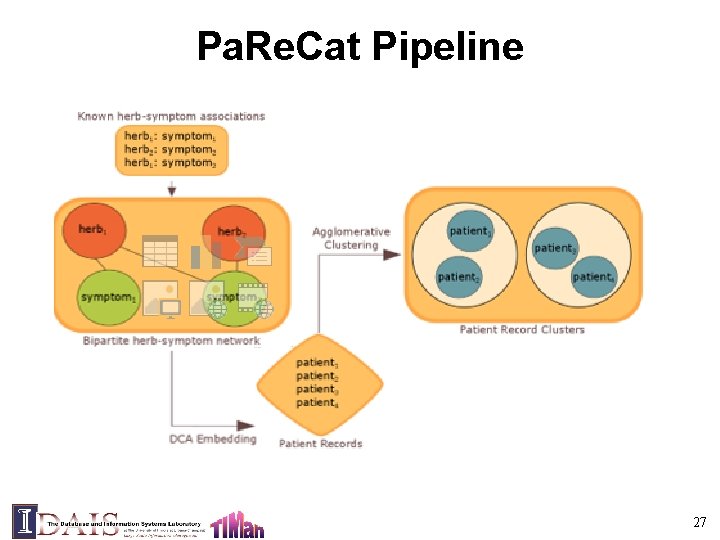
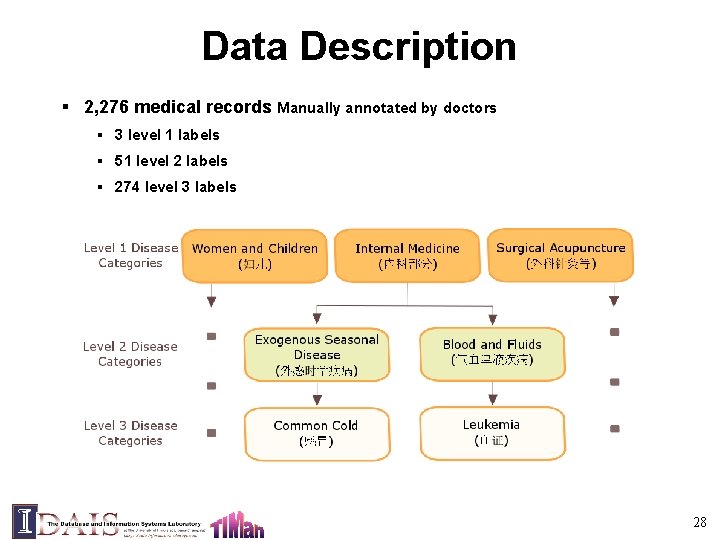
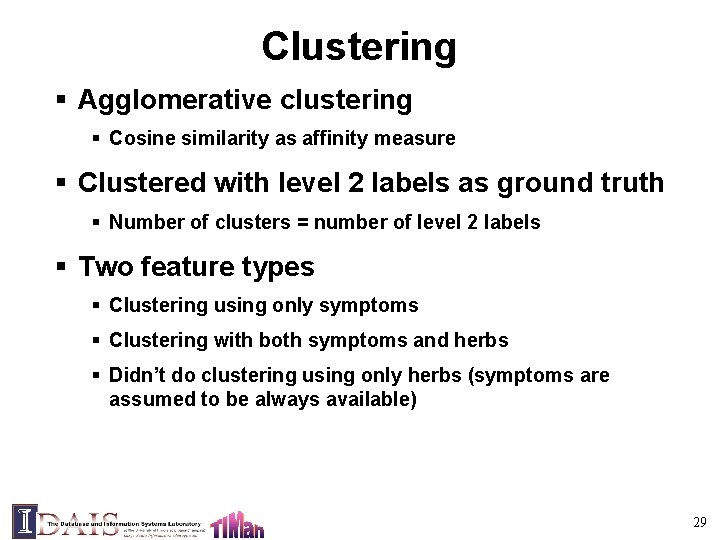
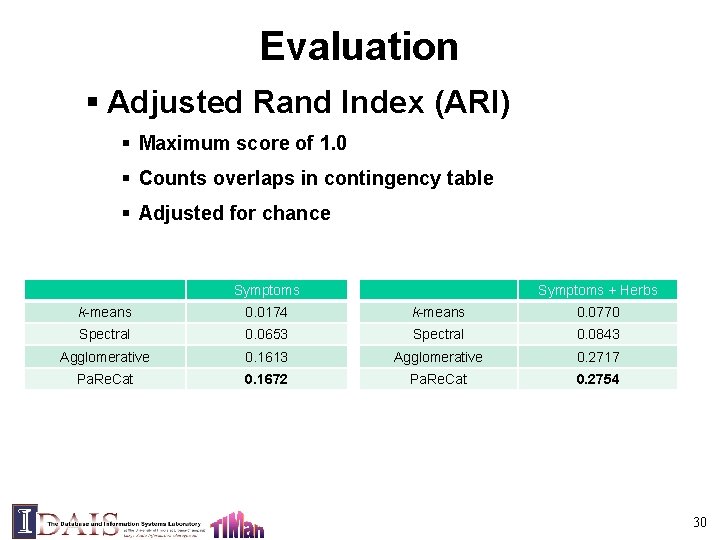
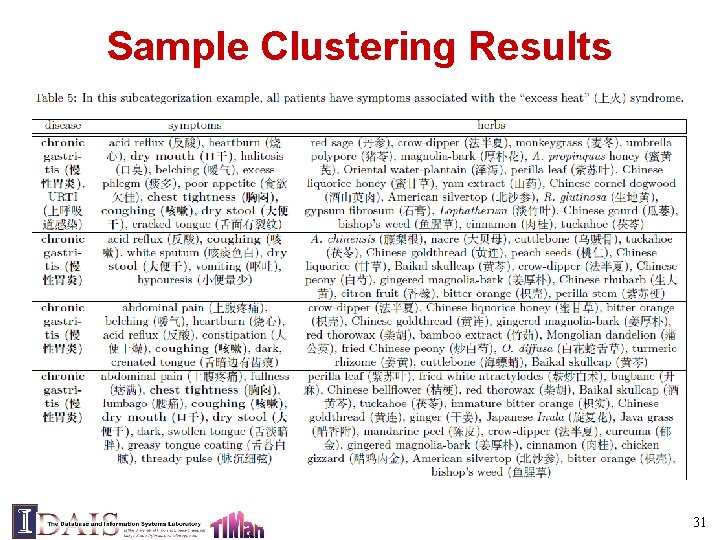
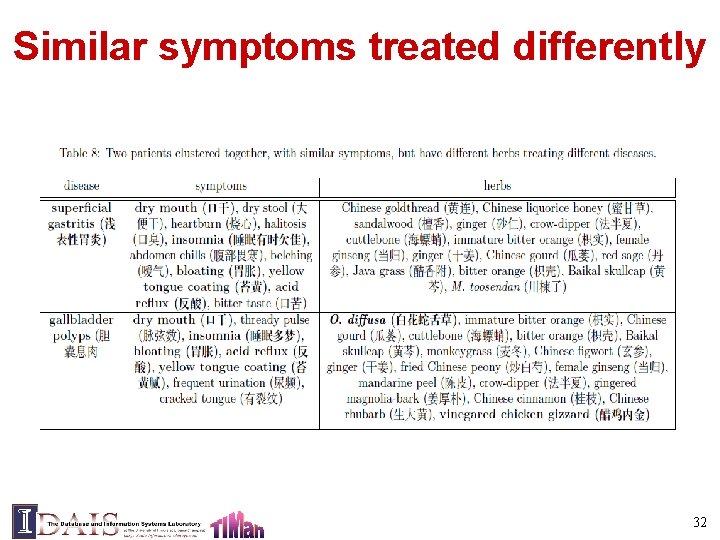
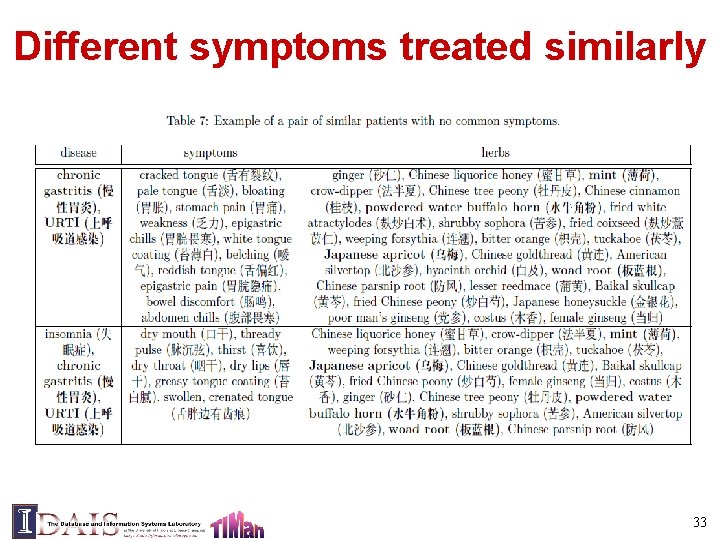
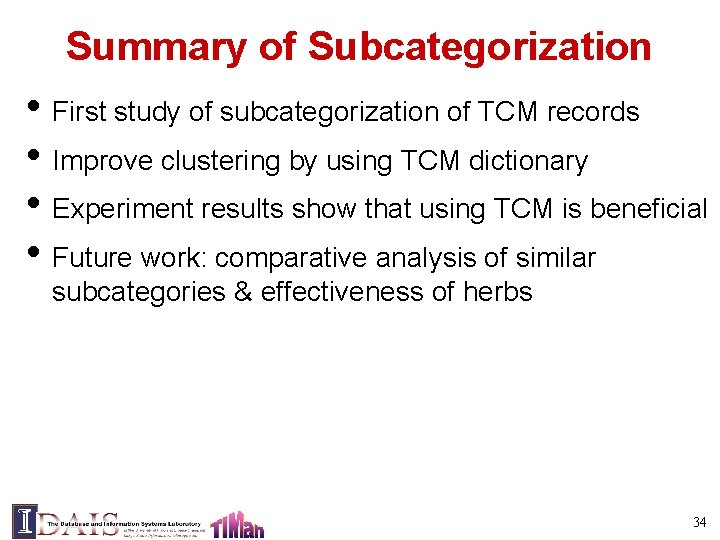
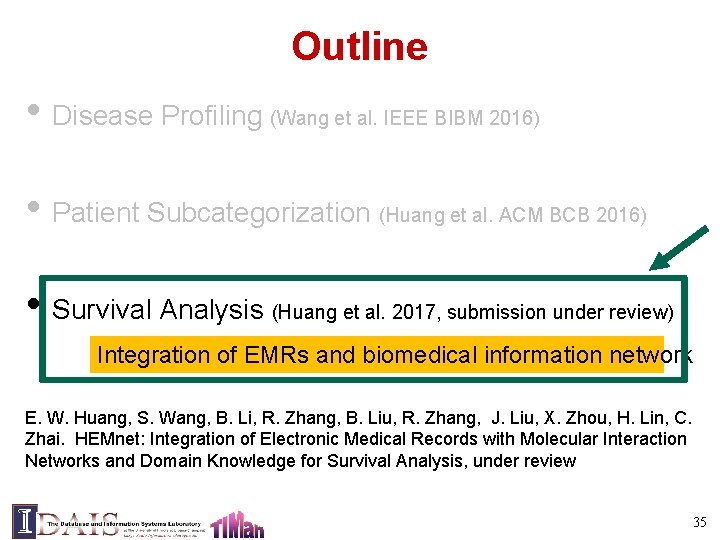
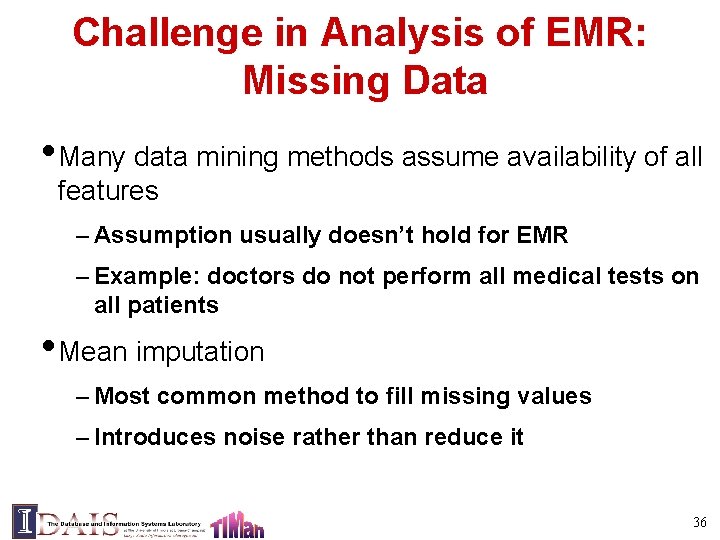
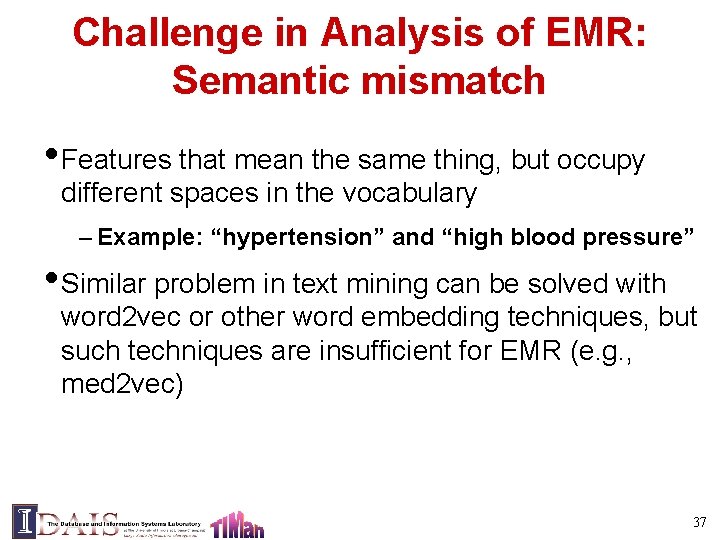
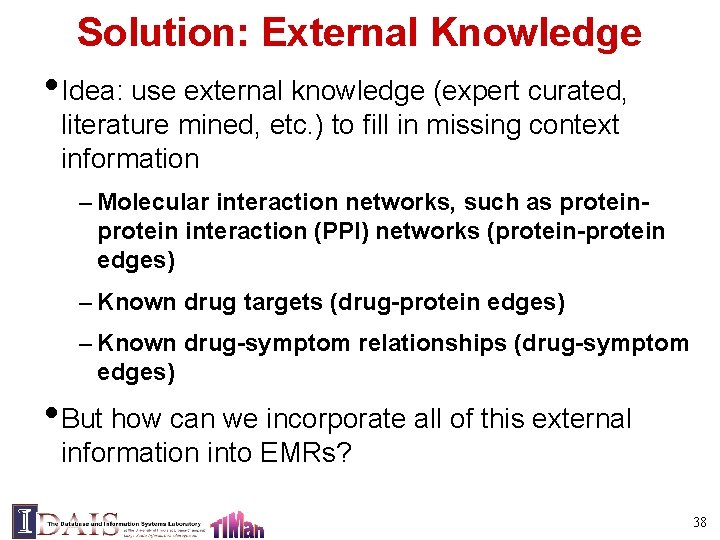
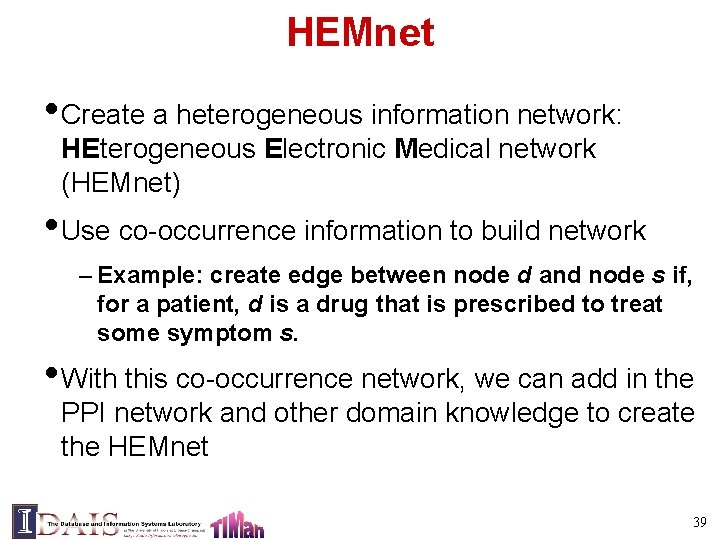
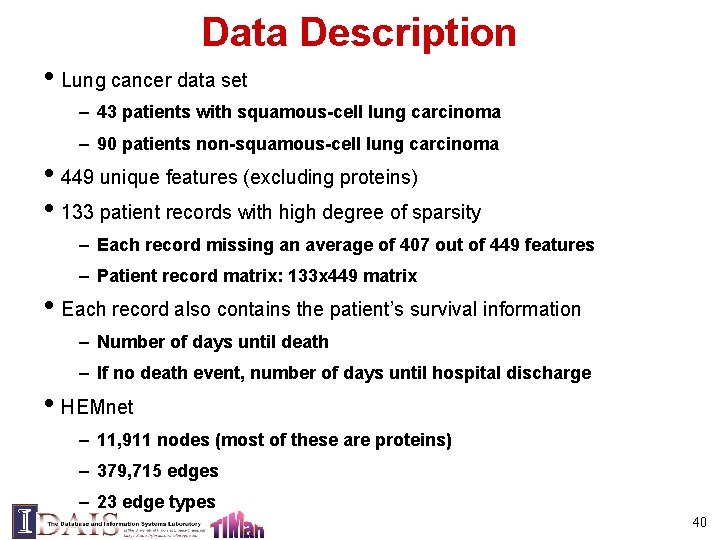
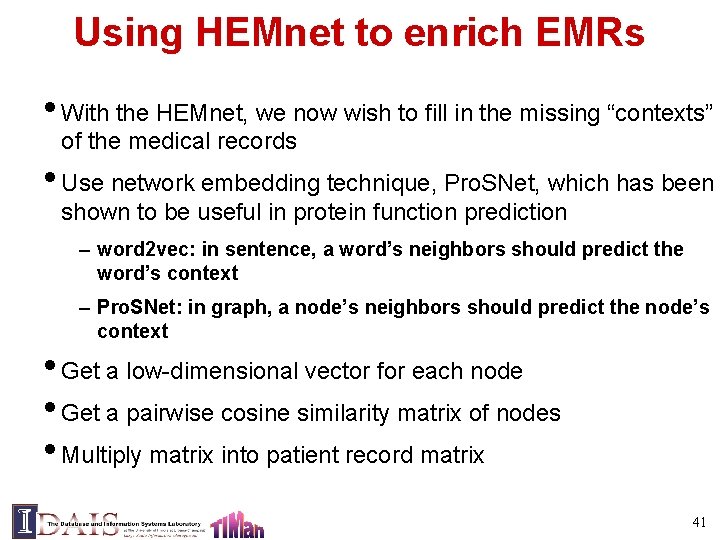
![Pos. Network Embedding [Wang et al. 17] Heterogenous network Node/Entity vector space z E Pos. Network Embedding [Wang et al. 17] Heterogenous network Node/Entity vector space z E](https://slidetodoc.com/presentation_image_h/13462b34239b5c73abfaf13e7c2e02d0/image-42.jpg)
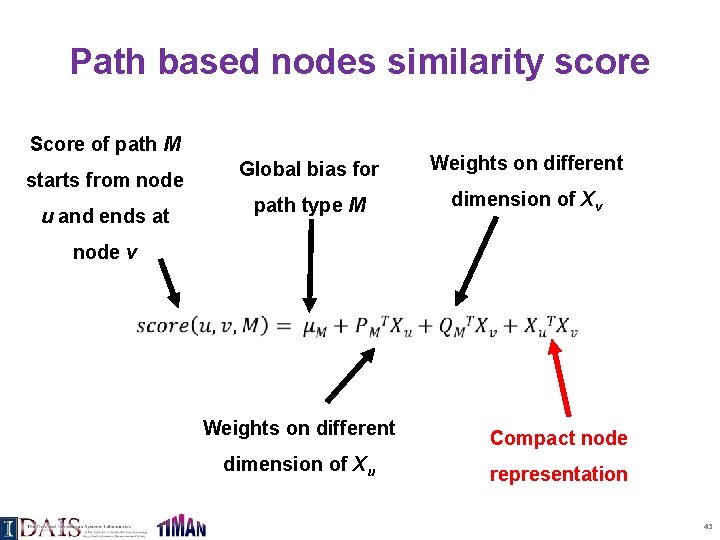
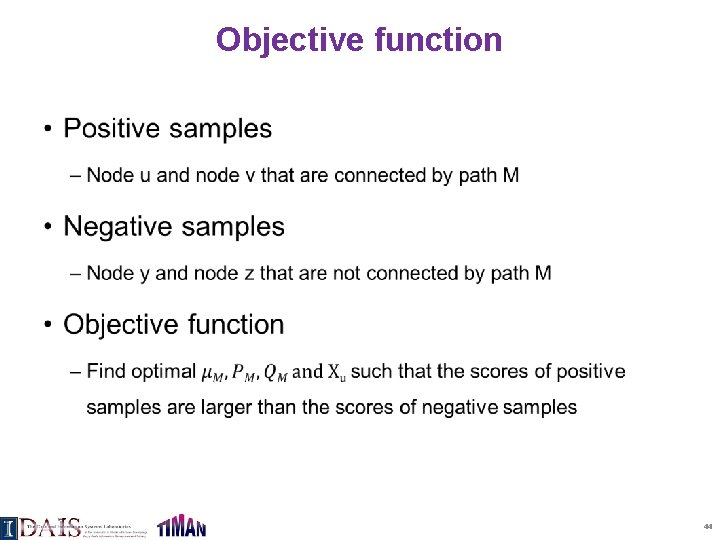
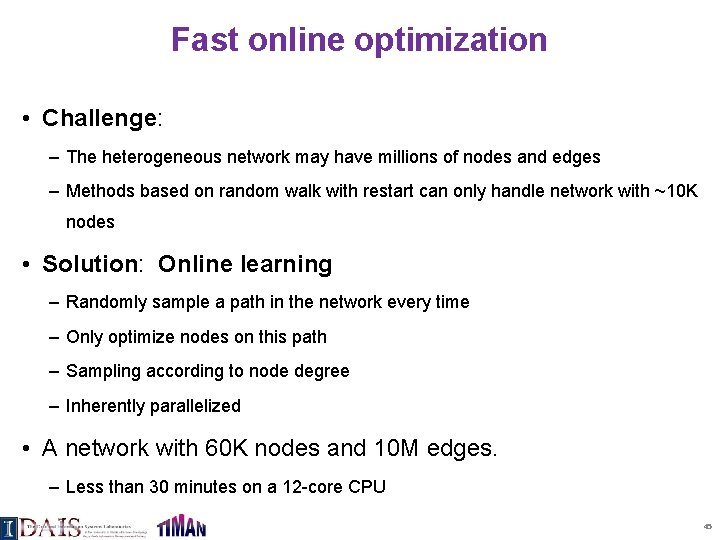
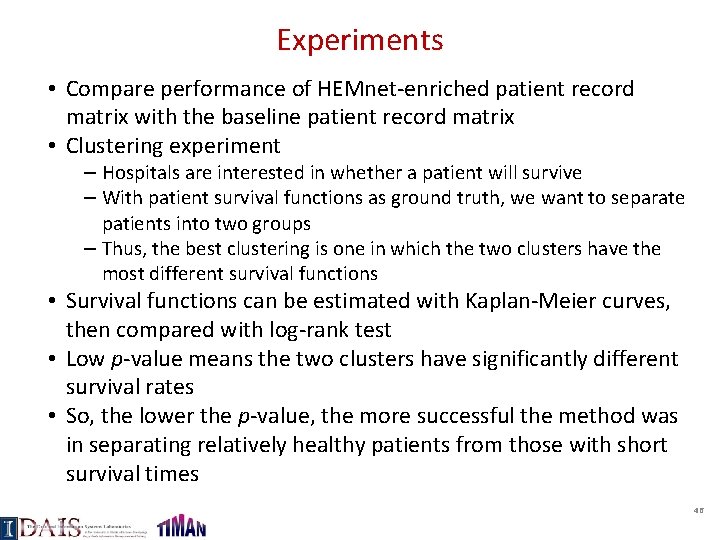
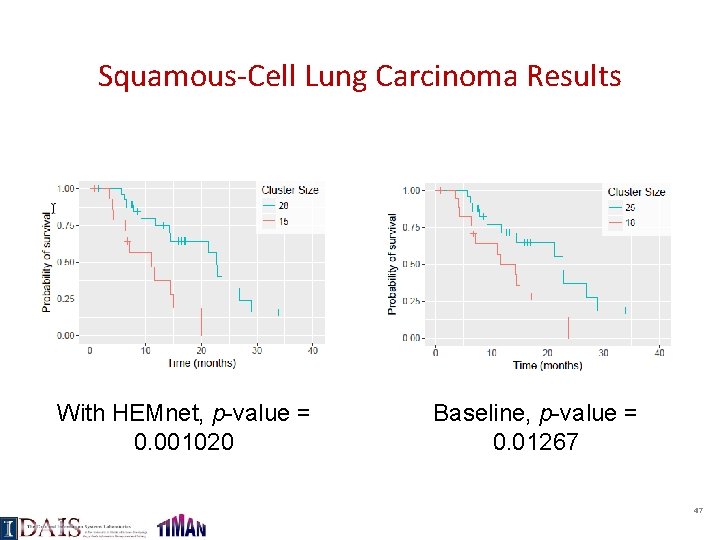
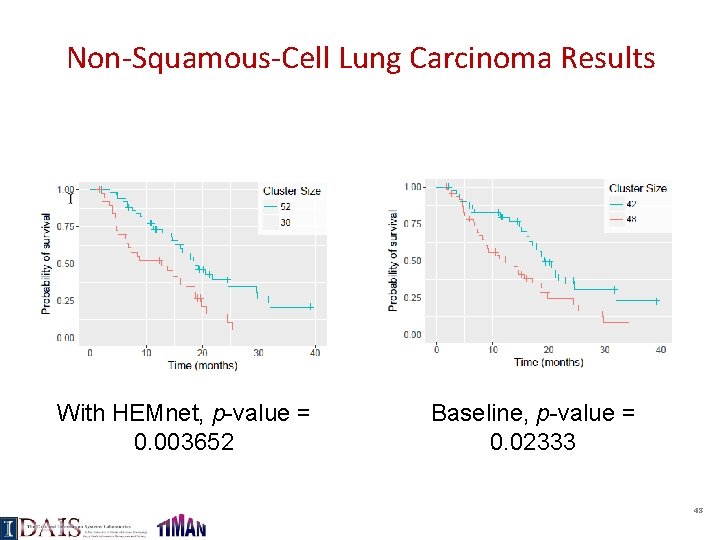
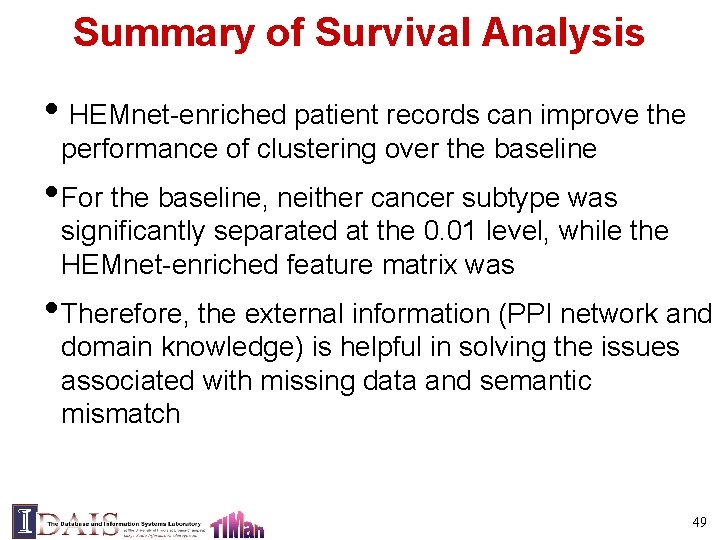
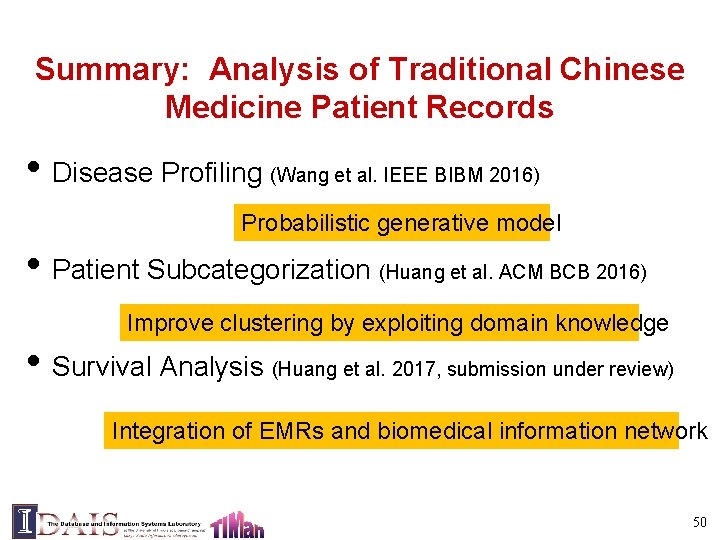
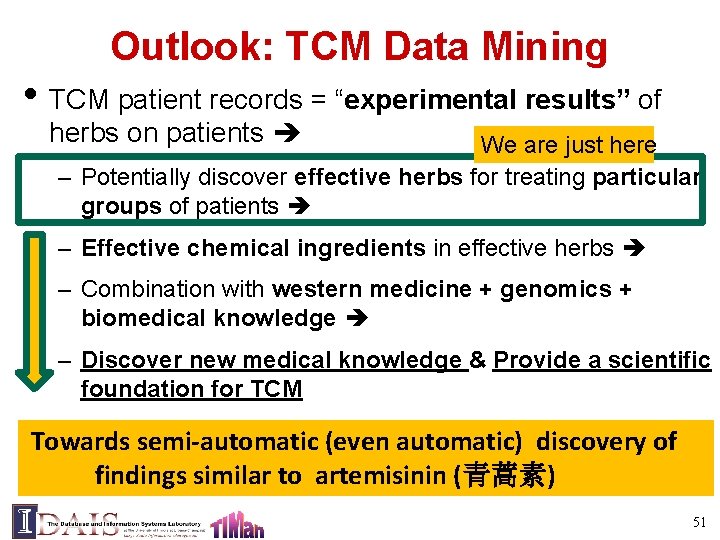
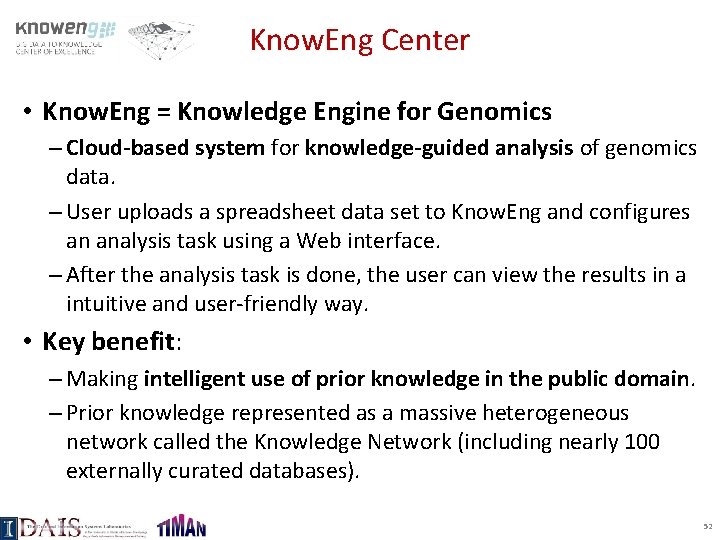
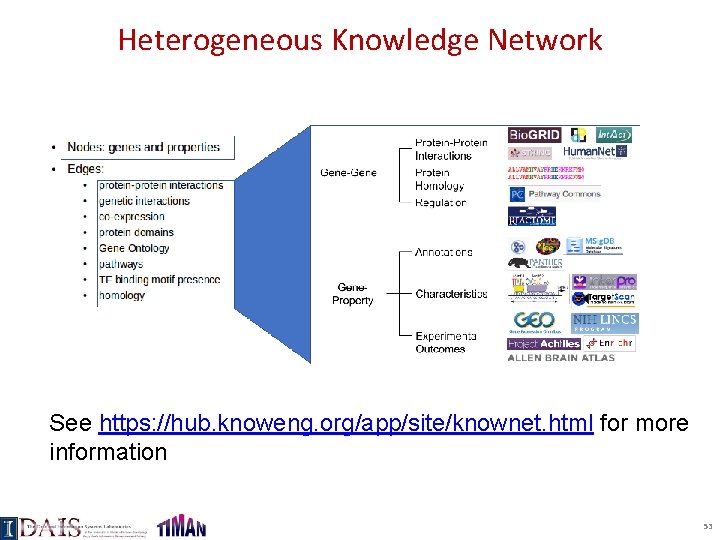
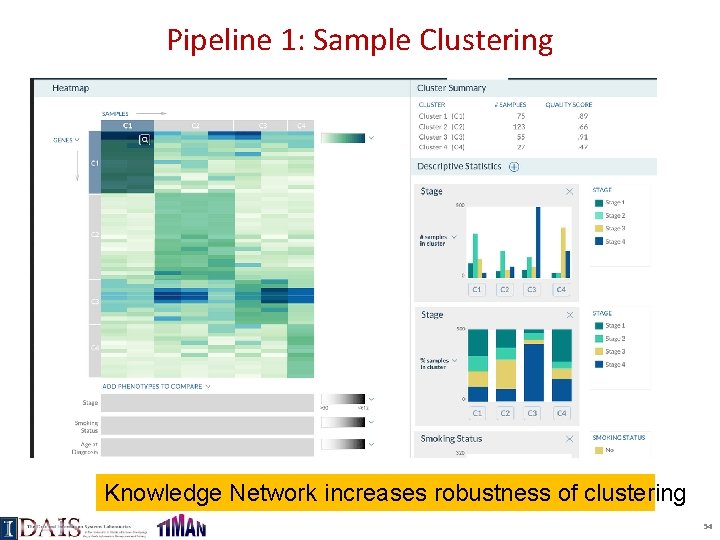
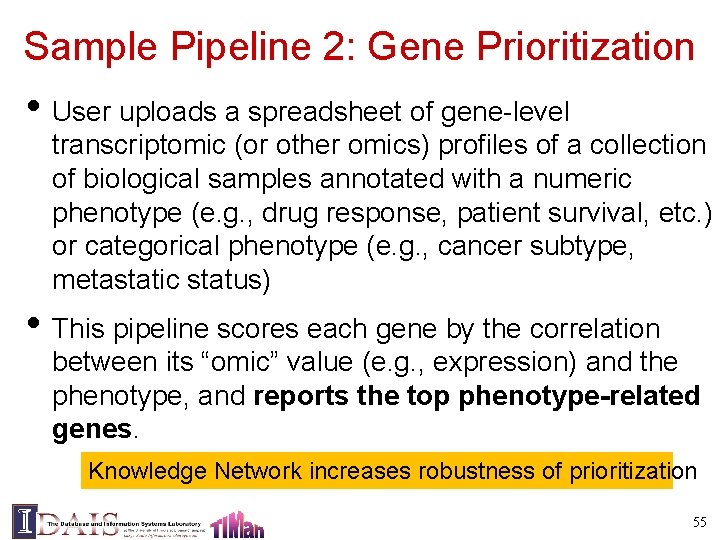
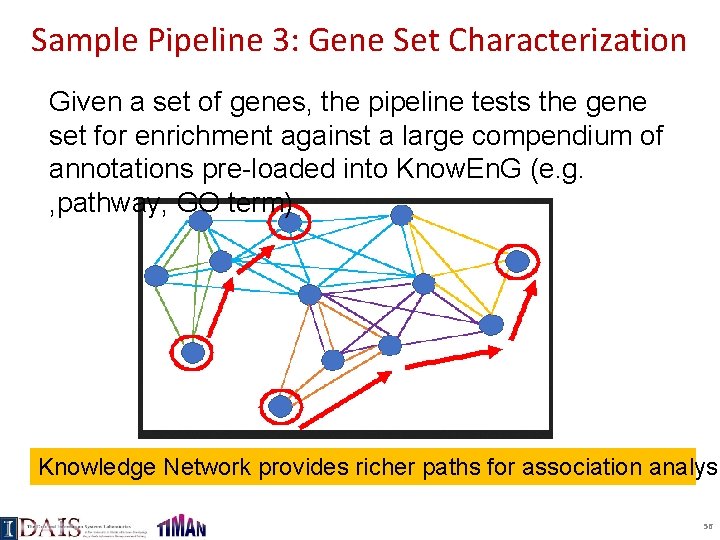
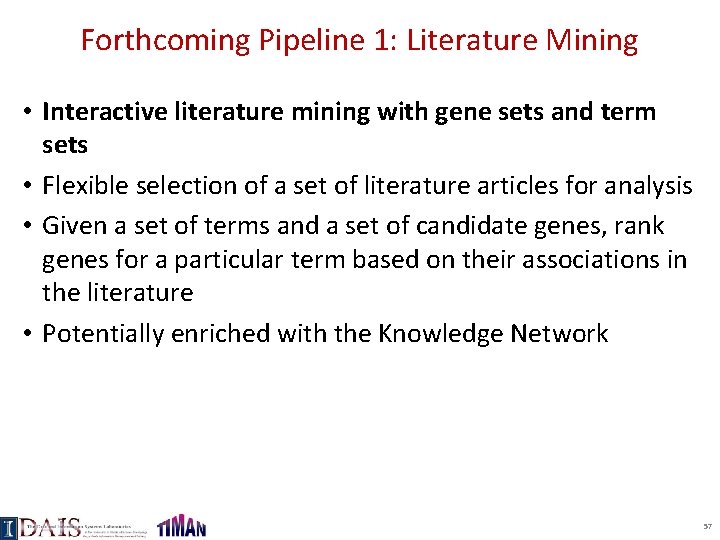
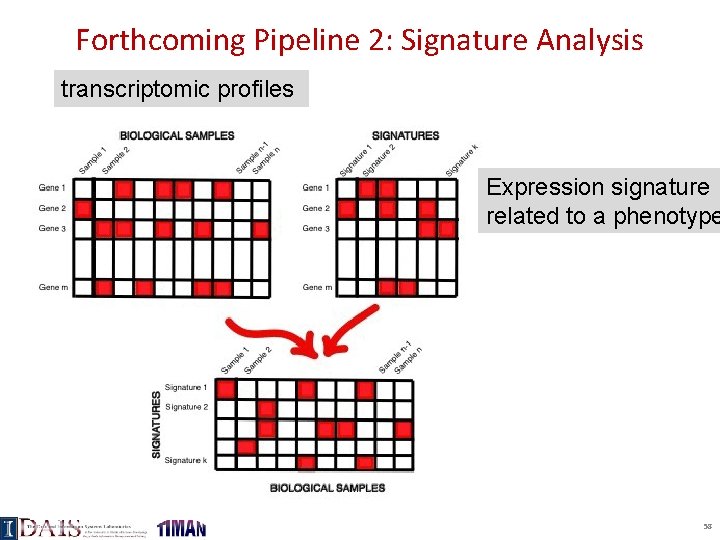
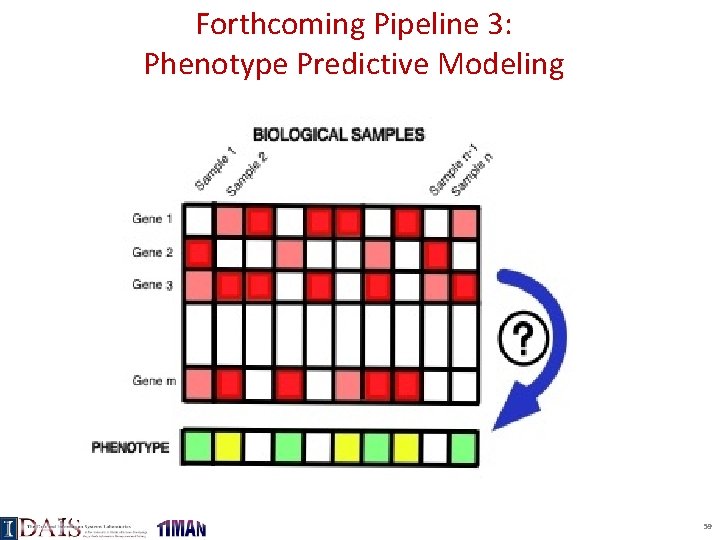
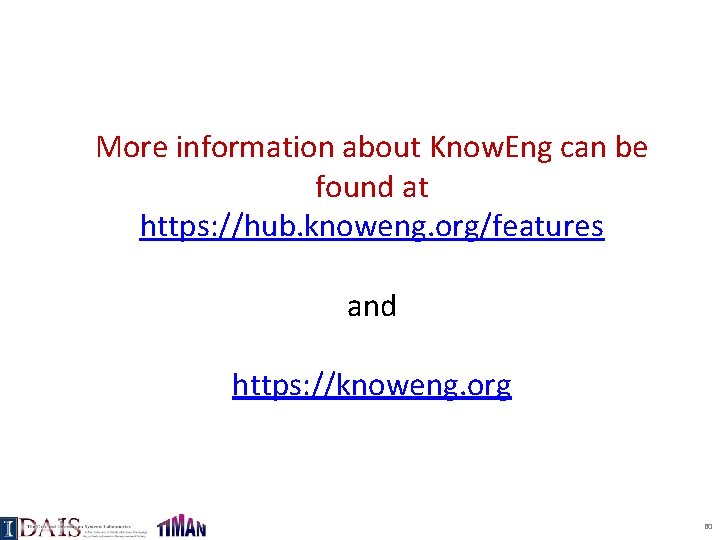
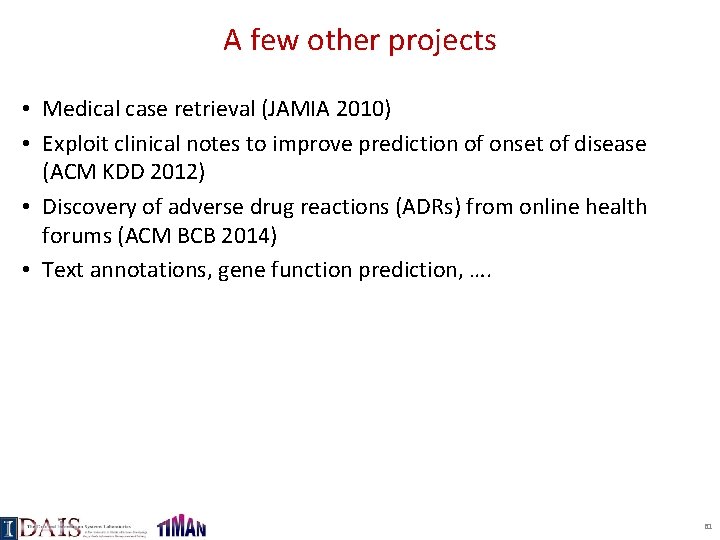
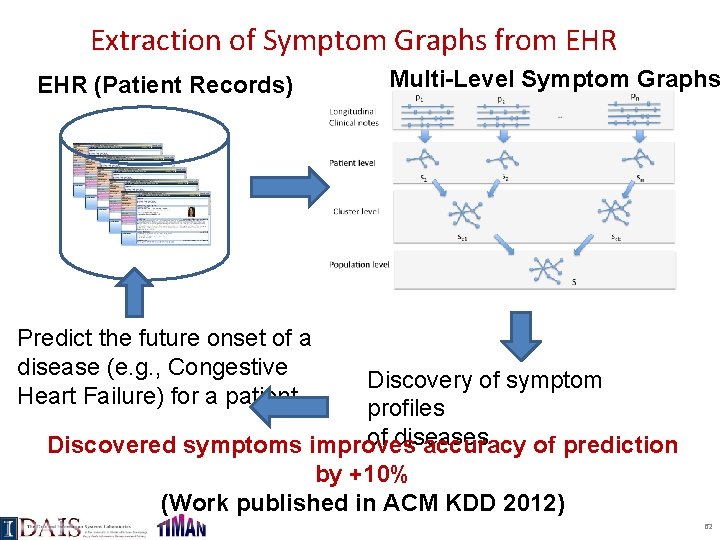
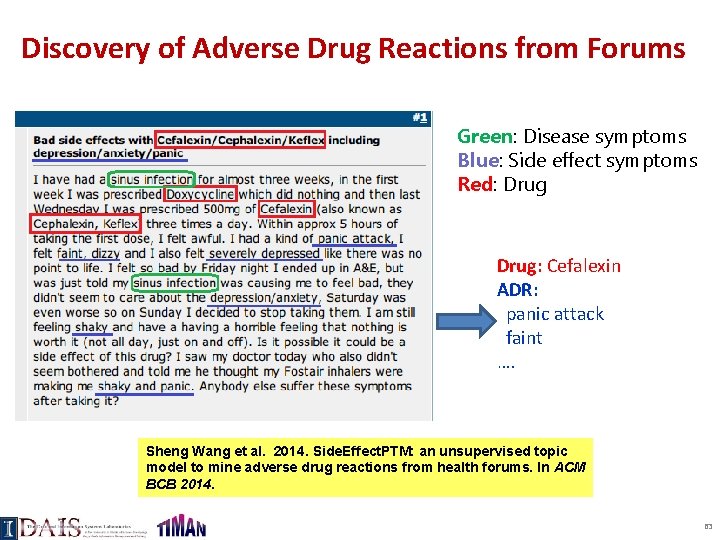
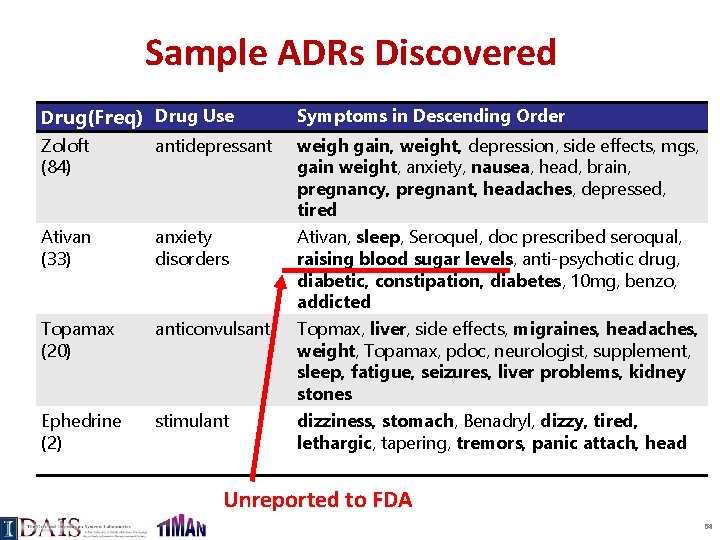
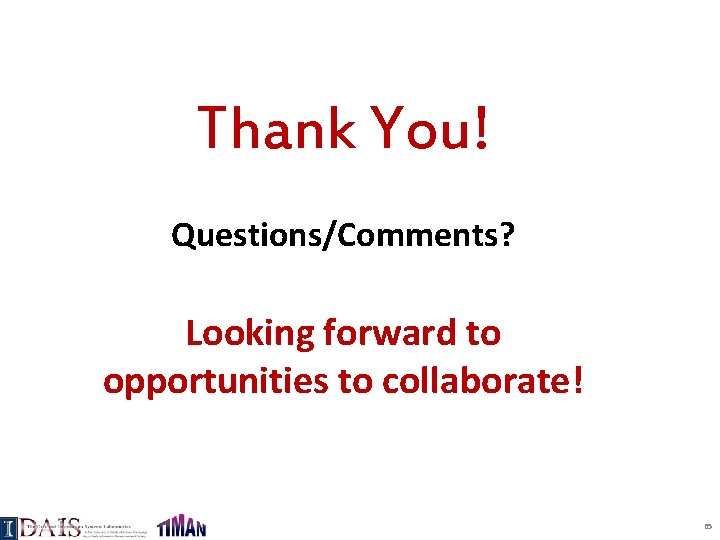
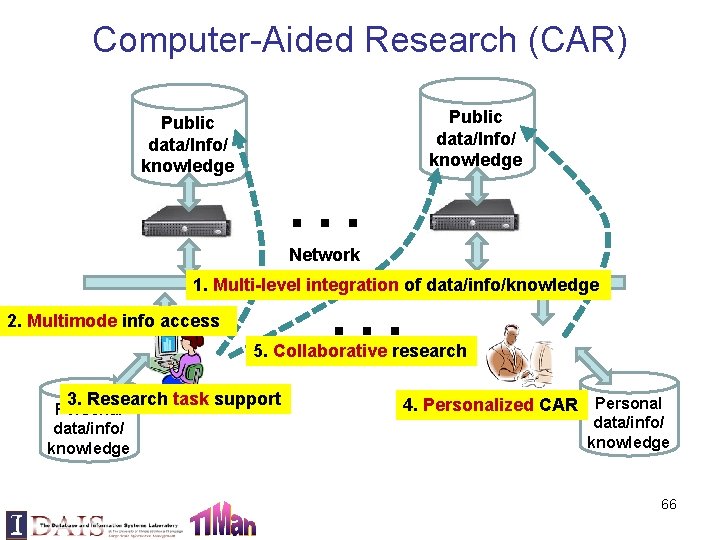
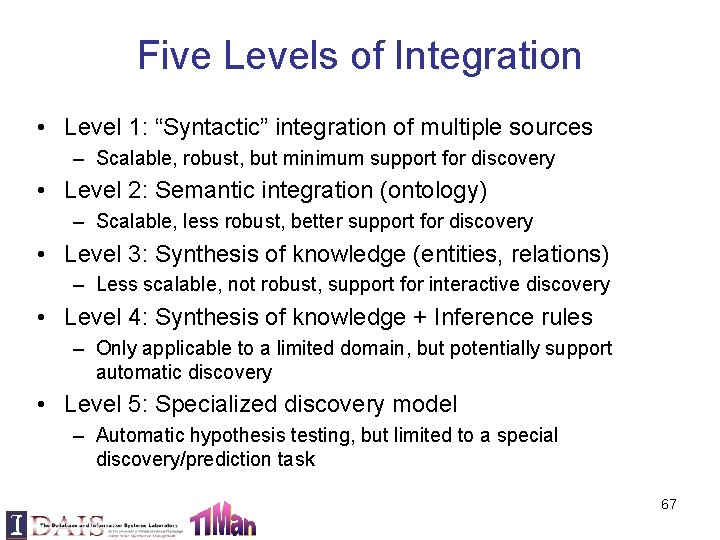
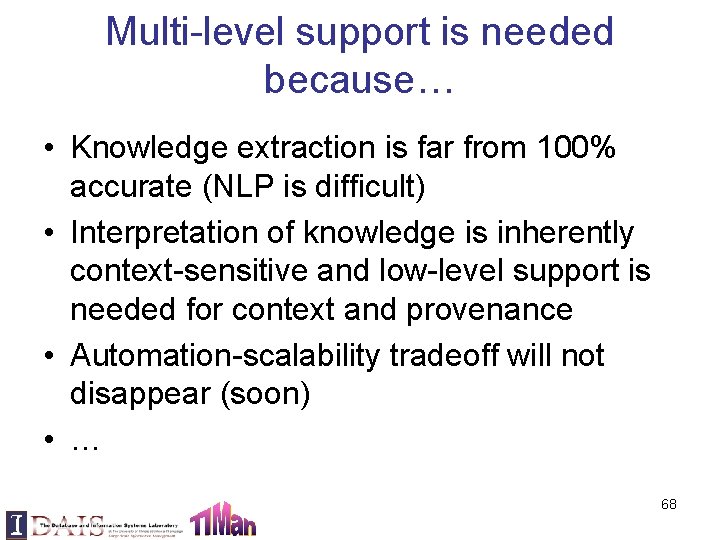
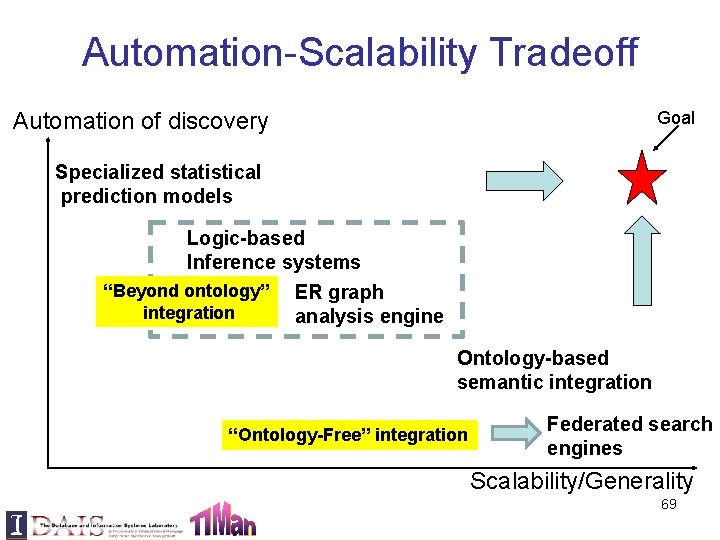
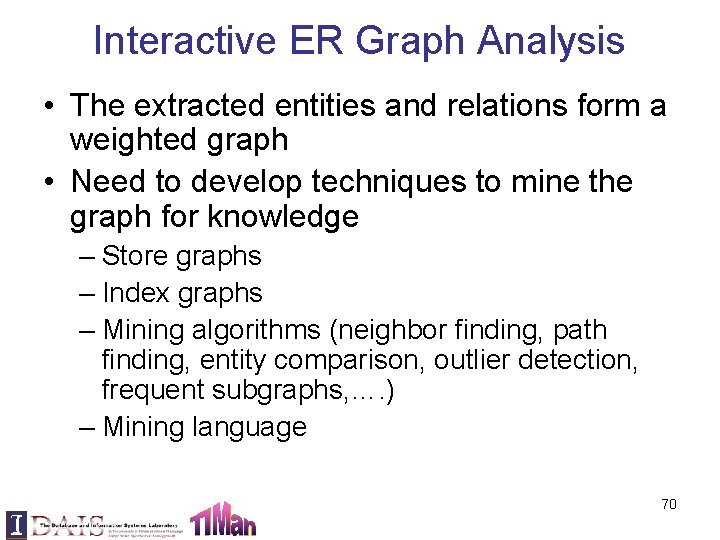
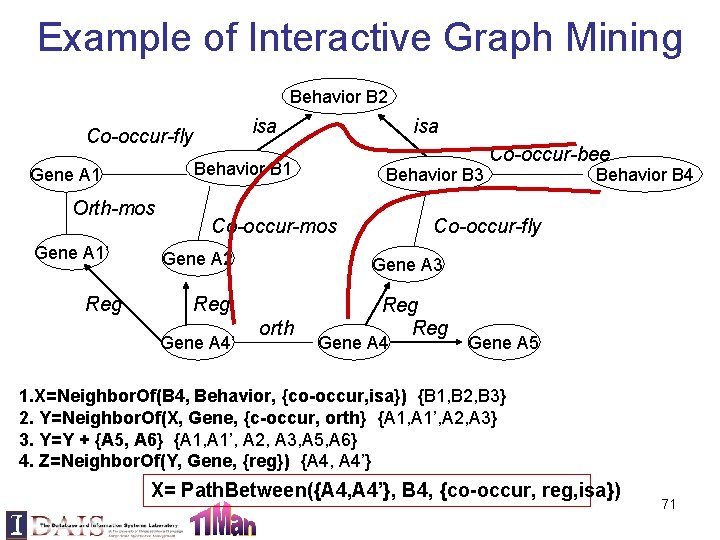
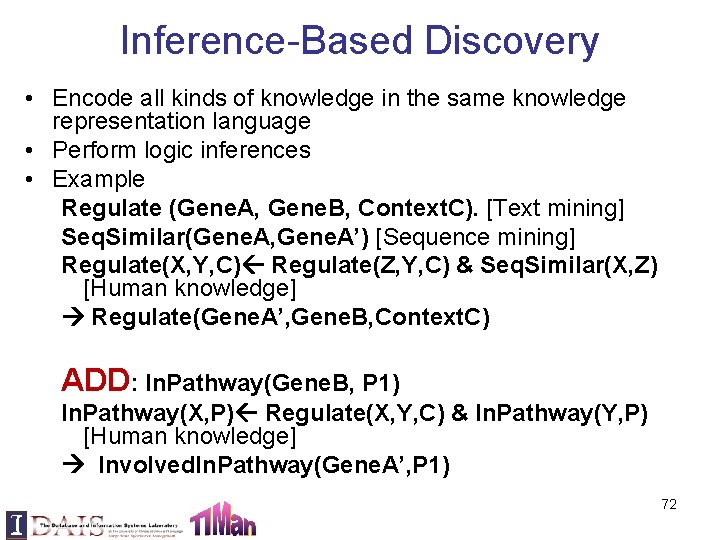
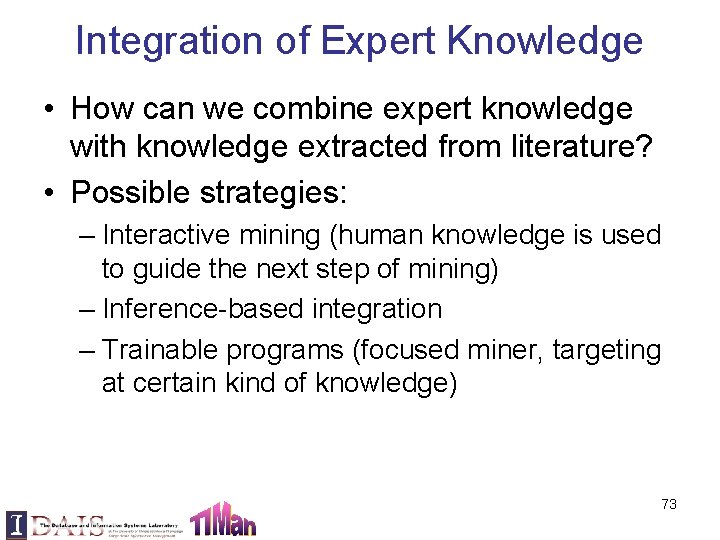
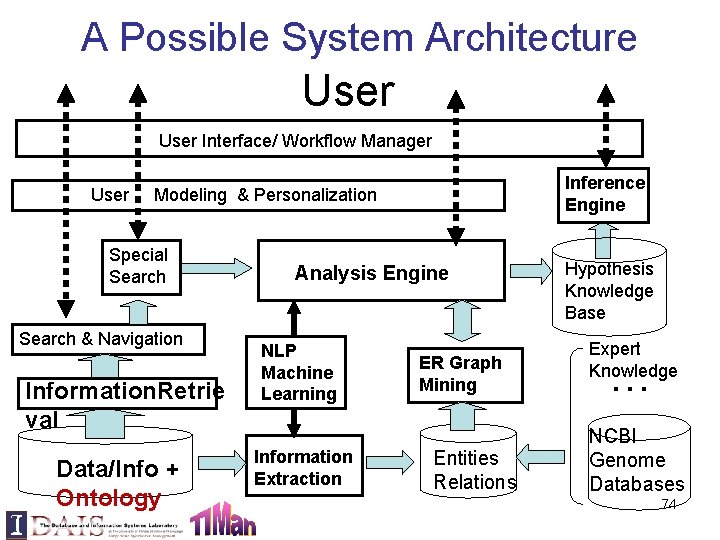
- Slides: 74
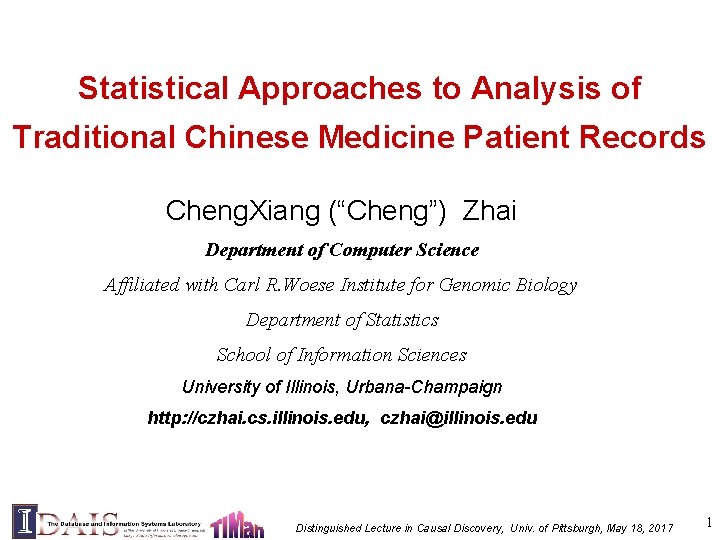
Statistical Approaches to Analysis of Traditional Chinese Medicine Patient Records Cheng. Xiang (“Cheng”) Zhai Department of Computer Science Affiliated with Carl R. Woese Institute for Genomic Biology Department of Statistics School of Information Sciences University of Illinois, Urbana-Champaign http: //czhai. cs. illinois. edu, czhai@illinois. edu Distinguished Lecture in Causal Discovery, Univ. of Pittsburgh, May 18, 2017 1
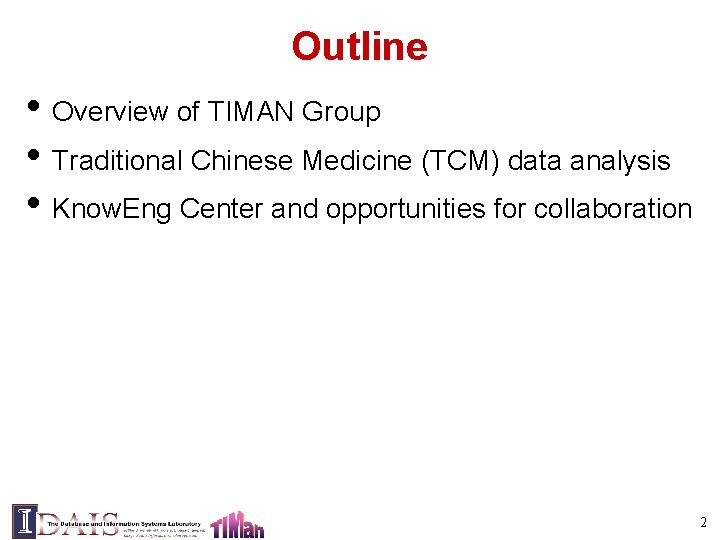
Outline • Overview of TIMAN Group • Traditional Chinese Medicine (TCM) data analysis • Know. Eng Center and opportunities for collaboration 2
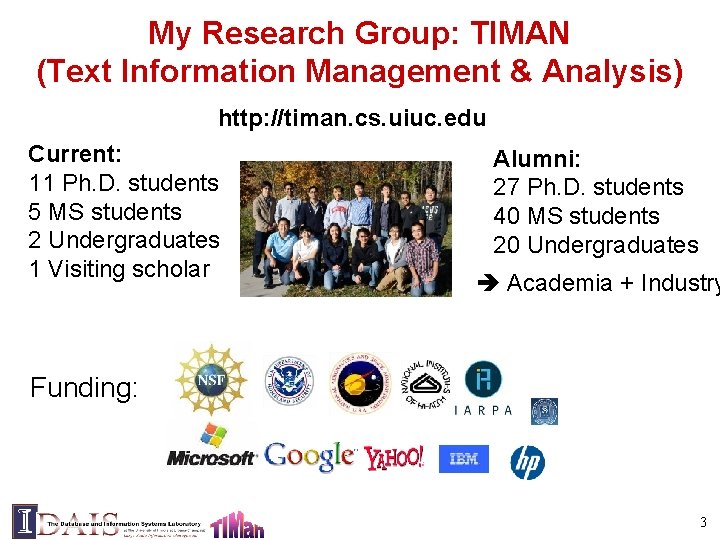
My Research Group: TIMAN (Text Information Management & Analysis) http: //timan. cs. uiuc. edu Current: 11 Ph. D. students 5 MS students 2 Undergraduates 1 Visiting scholar Alumni: 27 Ph. D. students 40 MS students 20 Undergraduates Academia + Industry Funding: 3
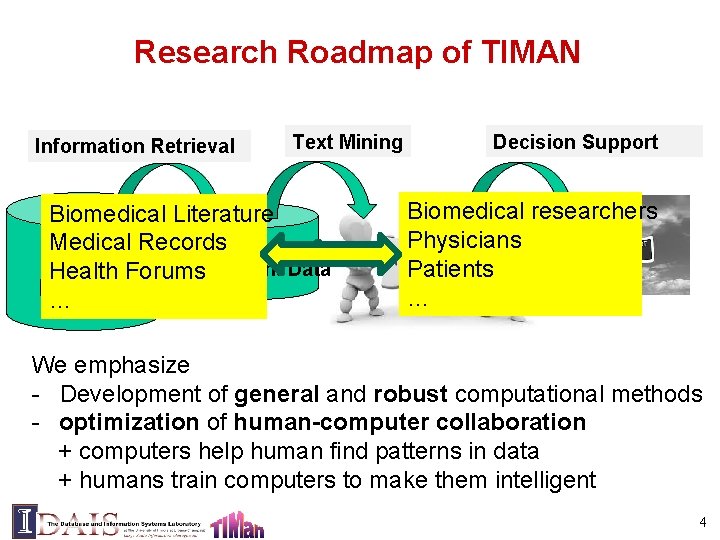
Research Roadmap of TIMAN Information Retrieval Text Mining Biomedical Literature Medical Records Small Relevant Data Health Forums Raw Data … Big Decision Support Biomedical researchers Physicians Patients … We emphasize - Development of general and robust computational methods - optimization of human-computer collaboration + computers help human find patterns in data + humans train computers to make them intelligent 4
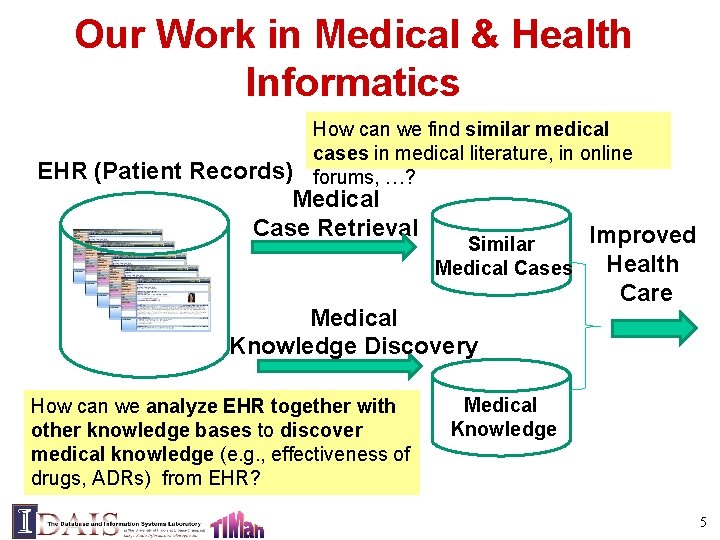
Our Work in Medical & Health Informatics How can we find similar medical cases in medical literature, in online forums, …? EHR (Patient Records) Medical Case Retrieval Similar Medical Cases Medical Knowledge Discovery How can we analyze EHR together with other knowledge bases to discover medical knowledge (e. g. , effectiveness of drugs, ADRs) from EHR? Improved Health Care Medical Knowledge 5
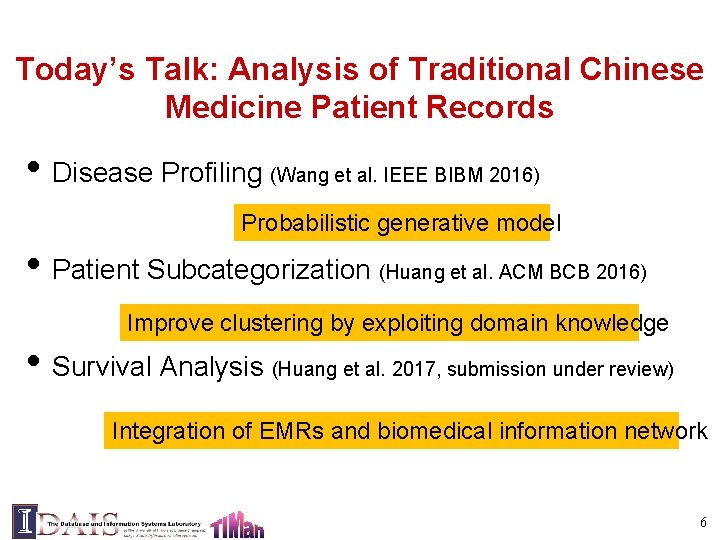
Today’s Talk: Analysis of Traditional Chinese Medicine Patient Records • Disease Profiling (Wang et al. IEEE BIBM 2016) Probabilistic generative model • Patient Subcategorization (Huang et al. ACM BCB 2016) Improve clustering by exploiting domain knowledge • Survival Analysis (Huang et al. 2017, submission under review) Integration of EMRs and biomedical information network 6
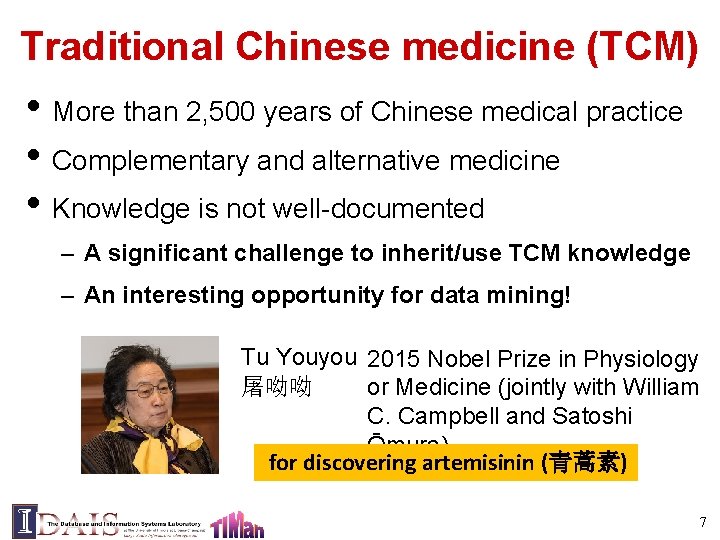
Traditional Chinese medicine (TCM) • More than 2, 500 years of Chinese medical practice • Complementary and alternative medicine • Knowledge is not well-documented – A significant challenge to inherit/use TCM knowledge – An interesting opportunity for data mining! Tu Youyou 2015 Nobel Prize in Physiology 屠呦呦 or Medicine (jointly with William C. Campbell and Satoshi Ōmura) for discovering artemisinin (青蒿素) 7
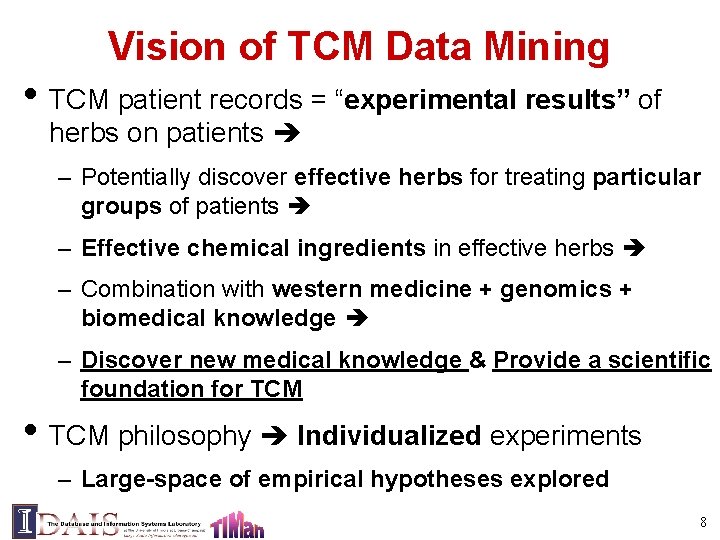
Vision of TCM Data Mining • TCM patient records = “experimental results” of herbs on patients – Potentially discover effective herbs for treating particular groups of patients – Effective chemical ingredients in effective herbs – Combination with western medicine + genomics + biomedical knowledge – Discover new medical knowledge & Provide a scientific foundation for TCM • TCM philosophy Individualized experiments – Large-space of empirical hypotheses explored 8
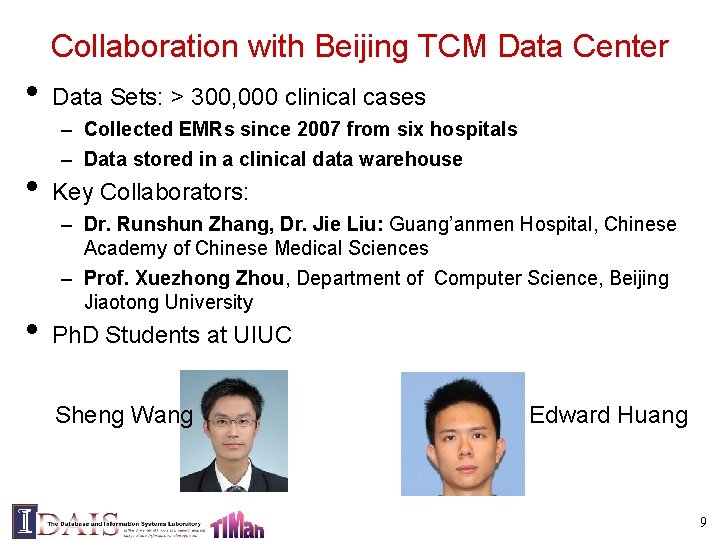
Collaboration with Beijing TCM Data Center • • • Data Sets: > 300, 000 clinical cases – Collected EMRs since 2007 from six hospitals – Data stored in a clinical data warehouse Key Collaborators: – Dr. Runshun Zhang, Dr. Jie Liu: Guang’anmen Hospital, Chinese Academy of Chinese Medical Sciences – Prof. Xuezhong Zhou, Department of Computer Science, Beijing Jiaotong University Ph. D Students at UIUC Sheng Wang Edward Huang 9
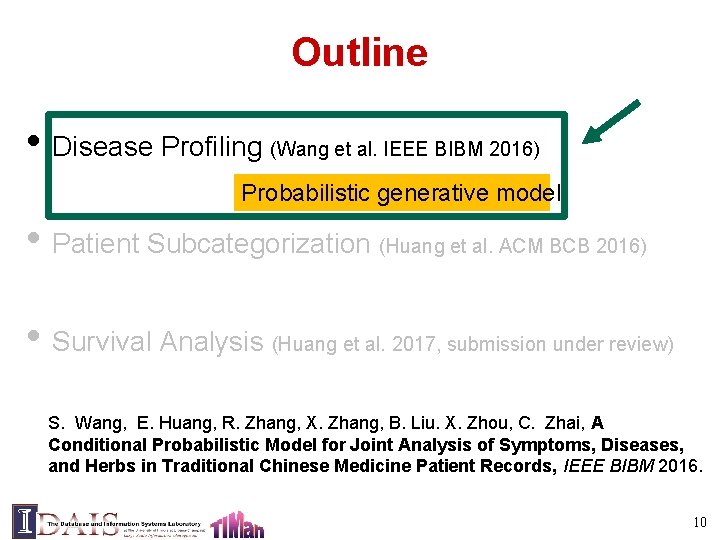
Outline • Disease Profiling (Wang et al. IEEE BIBM 2016) Probabilistic generative model • Patient Subcategorization (Huang et al. ACM BCB 2016) • Survival Analysis (Huang et al. 2017, submission under review) S. Wang, E. Huang, R. Zhang, X. Zhang, B. Liu. X. Zhou, C. Zhai, A Conditional Probabilistic Model for Joint Analysis of Symptoms, Diseases, and Herbs in Traditional Chinese Medicine Patient Records, IEEE BIBM 2016. 10
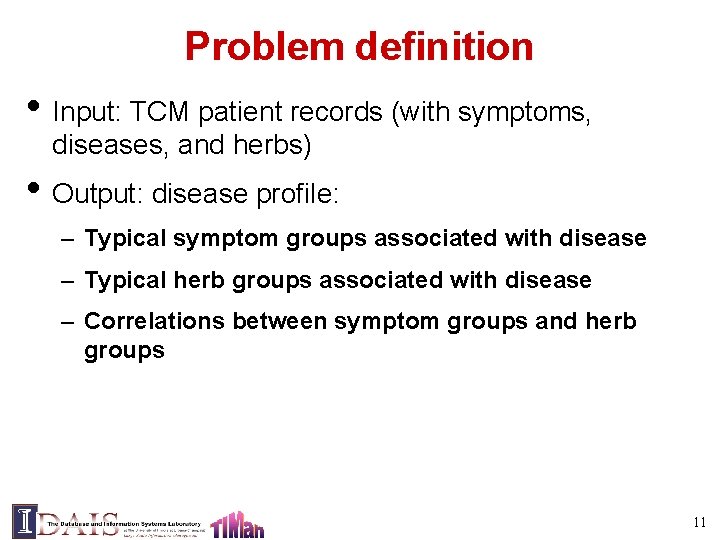
Problem definition • Input: TCM patient records (with symptoms, diseases, and herbs) • Output: disease profile: – Typical symptom groups associated with disease – Typical herb groups associated with disease – Correlations between symptom groups and herb groups 11
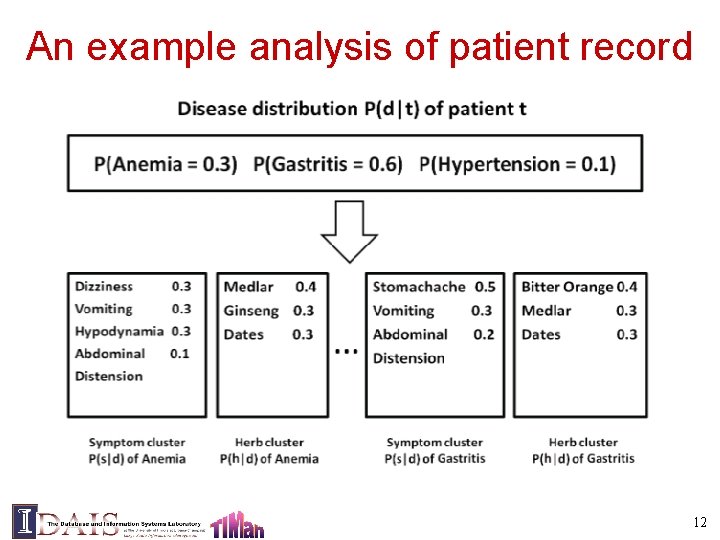
An example analysis of patient record 12
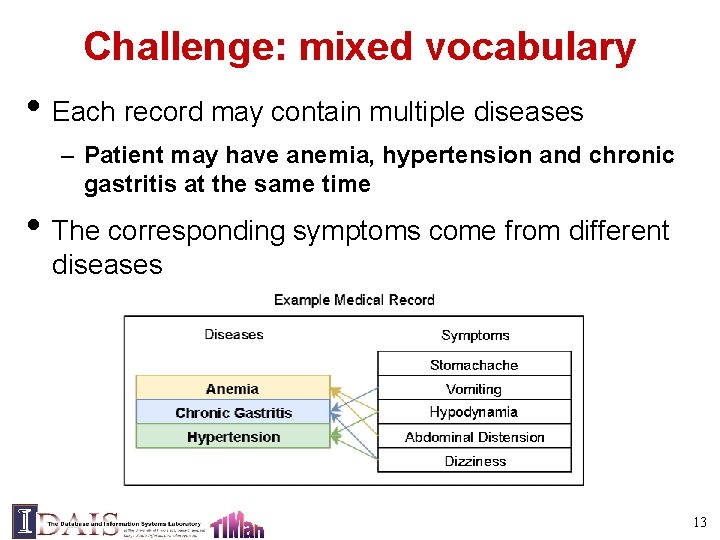
Challenge: mixed vocabulary • Each record may contain multiple diseases – Patient may have anemia, hypertension and chronic gastritis at the same time • The corresponding symptoms come from different diseases 13
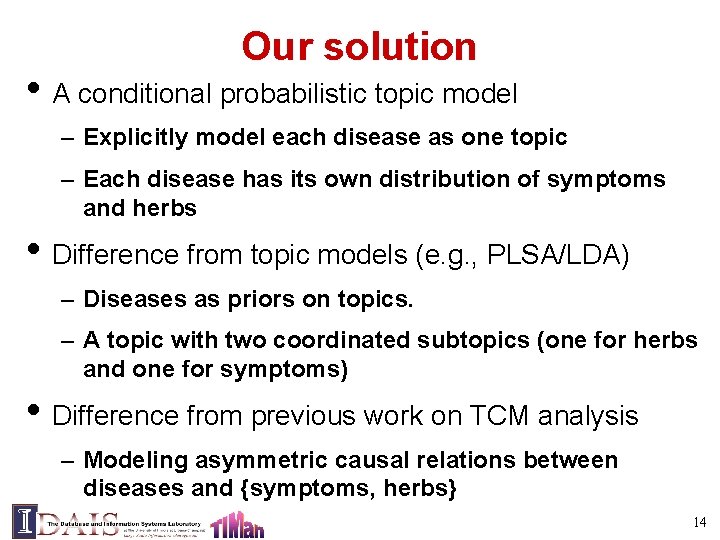
Our solution • A conditional probabilistic topic model – Explicitly model each disease as one topic – Each disease has its own distribution of symptoms and herbs • Difference from topic models (e. g. , PLSA/LDA) – Diseases as priors on topics. – A topic with two coordinated subtopics (one for herbs and one for symptoms) • Difference from previous work on TCM analysis – Modeling asymmetric causal relations between diseases and {symptoms, herbs} 14
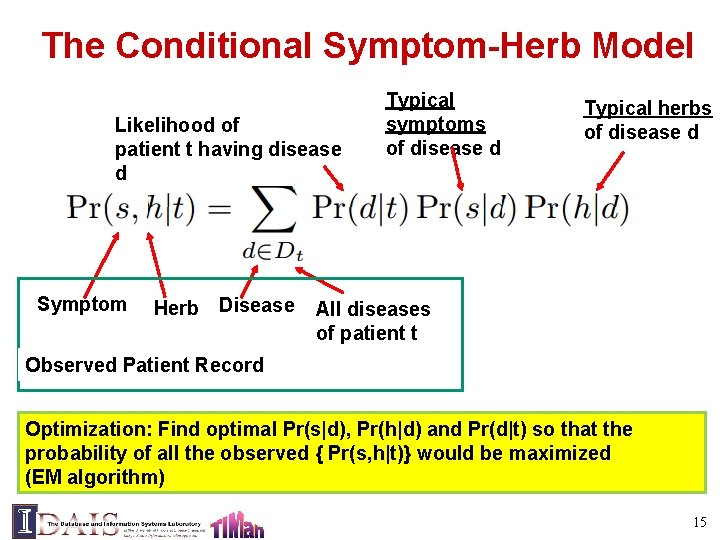
The Conditional Symptom-Herb Model Likelihood of patient t having disease d Symptom Herb Disease Typical symptoms of disease d Typical herbs of disease d All diseases of patient t Observed Patient Record Optimization: Find optimal Pr(s|d), Pr(h|d) and Pr(d|t) so that the probability of all the observed { Pr(s, h|t)} would be maximized (EM algorithm) 15
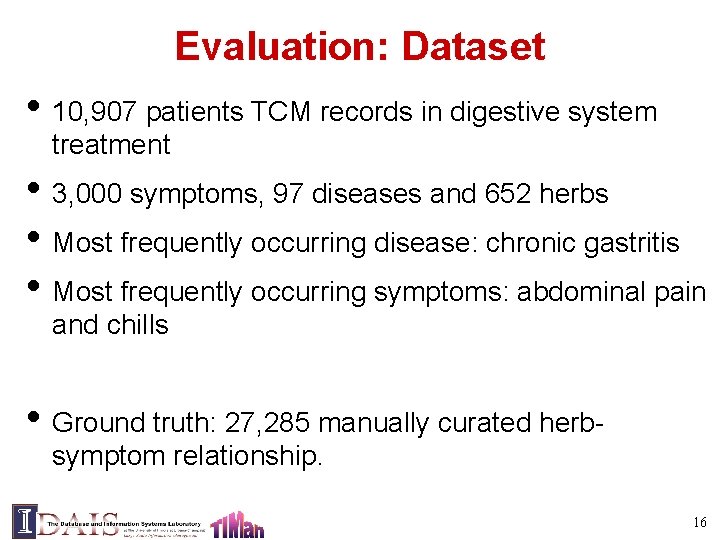
Evaluation: Dataset • 10, 907 patients TCM records in digestive system treatment • 3, 000 symptoms, 97 diseases and 652 herbs • Most frequently occurring disease: chronic gastritis • Most frequently occurring symptoms: abdominal pain and chills • Ground truth: 27, 285 manually curated herbsymptom relationship. 16
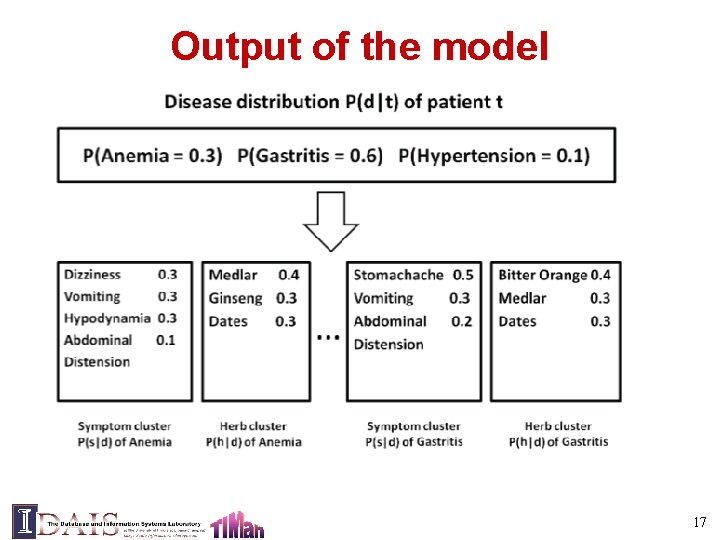
Output of the model 17
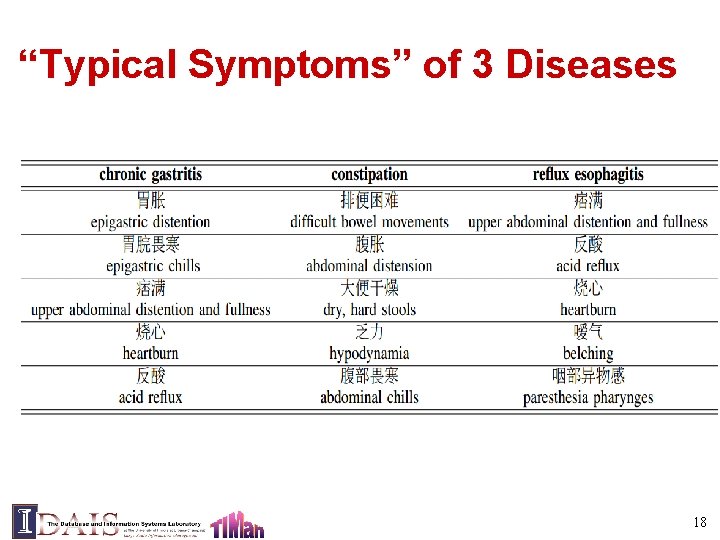
“Typical Symptoms” of 3 Diseases 18
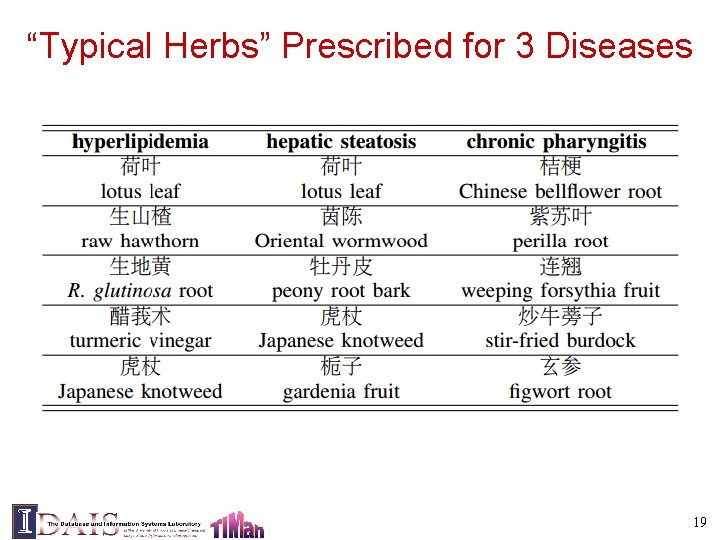
“Typical Herbs” Prescribed for 3 Diseases 19
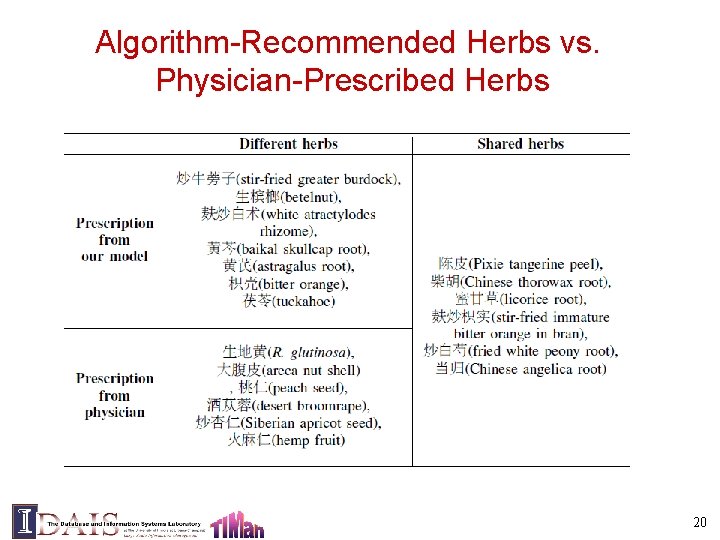
Algorithm-Recommended Herbs vs. Physician-Prescribed Herbs 20
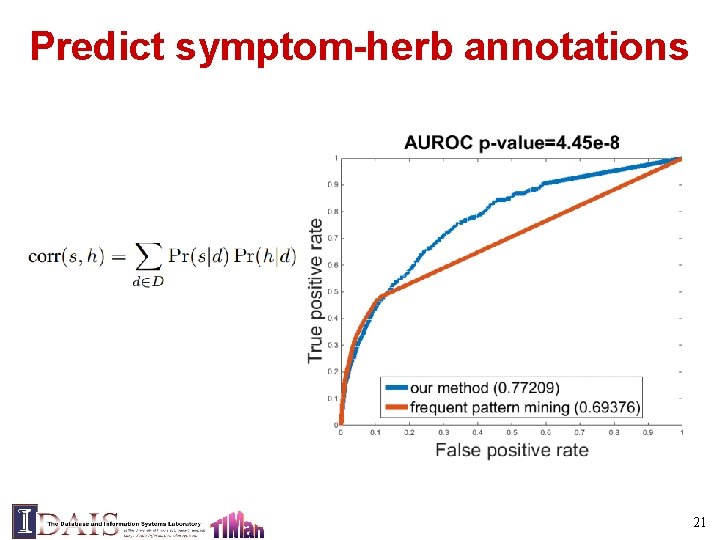
Predict symptom-herb annotations 21
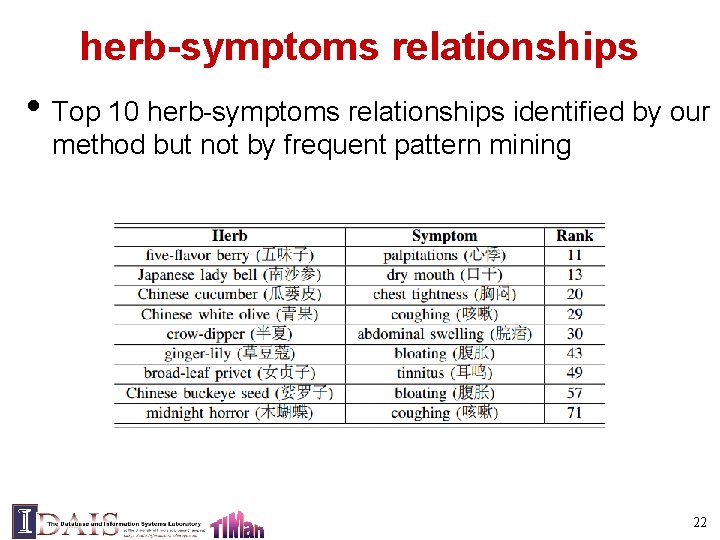
herb-symptoms relationships • Top 10 herb-symptoms relationships identified by our method but not by frequent pattern mining 22
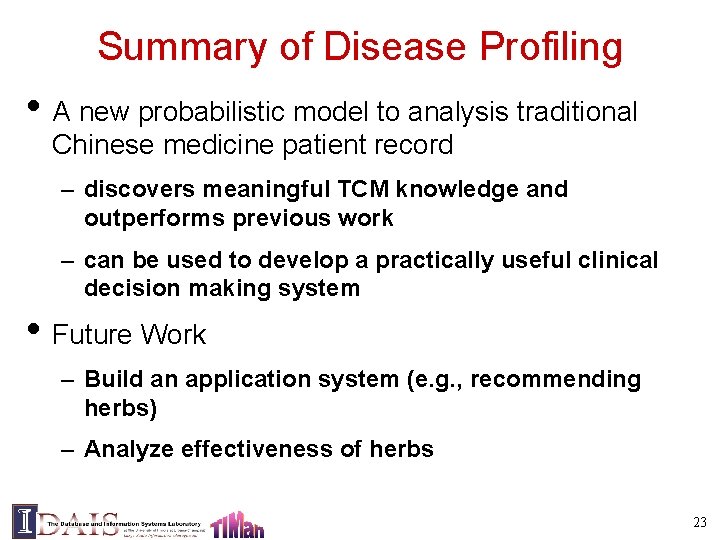
Summary of Disease Profiling • A new probabilistic model to analysis traditional Chinese medicine patient record – discovers meaningful TCM knowledge and outperforms previous work – can be used to develop a practically useful clinical decision making system • Future Work – Build an application system (e. g. , recommending herbs) – Analyze effectiveness of herbs 23
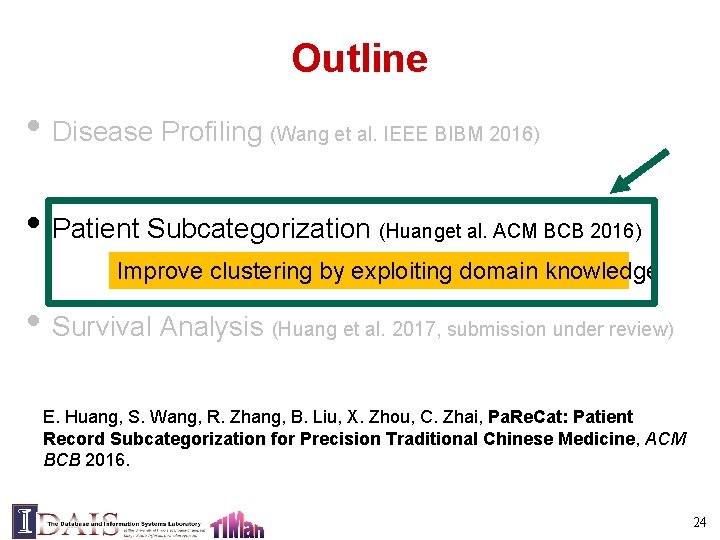
Outline • Disease Profiling (Wang et al. IEEE BIBM 2016) • Patient Subcategorization (Huanget al. ACM BCB 2016) Improve clustering by exploiting domain knowledge • Survival Analysis (Huang et al. 2017, submission under review) E. Huang, S. Wang, R. Zhang, B. Liu, X. Zhou, C. Zhai, Pa. Re. Cat: Patient Record Subcategorization for Precision Traditional Chinese Medicine, ACM BCB 2016. 24
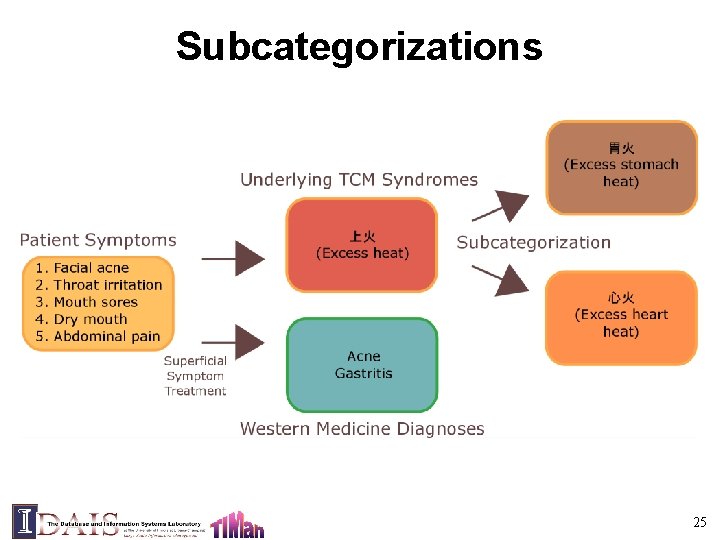
Subcategorizations 25
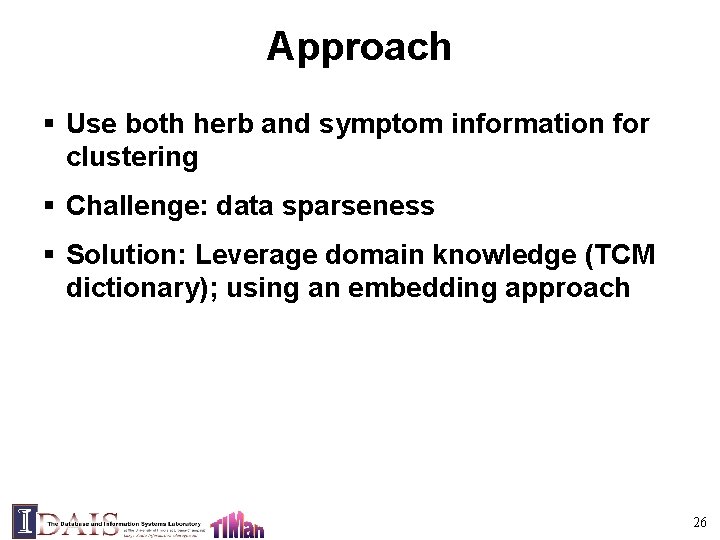
Approach § Use both herb and symptom information for clustering § Challenge: data sparseness § Solution: Leverage domain knowledge (TCM dictionary); using an embedding approach 26
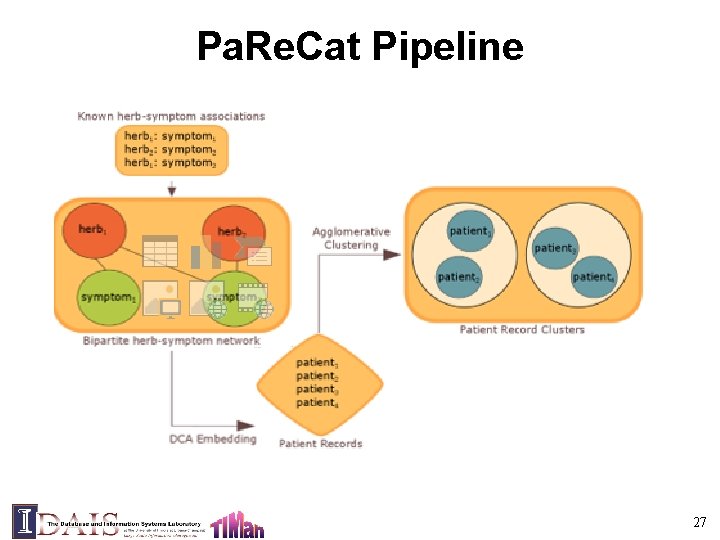
Pa. Re. Cat Pipeline 27
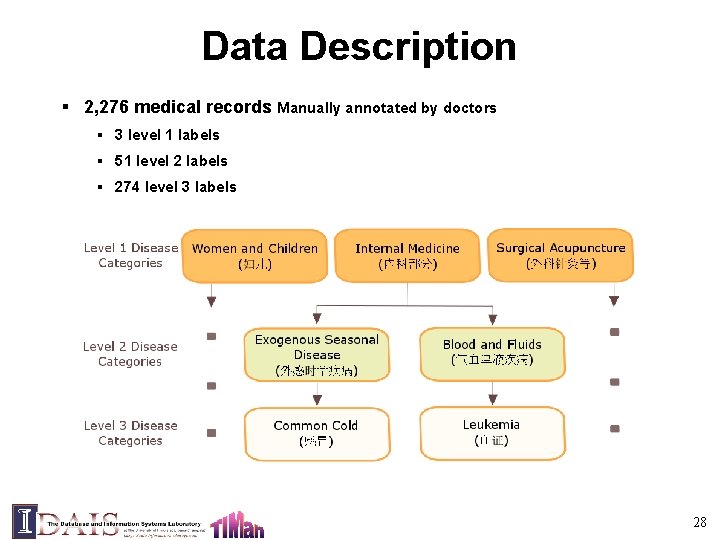
Data Description § 2, 276 medical records Manually annotated by doctors § 3 level 1 labels § 51 level 2 labels § 274 level 3 labels 28
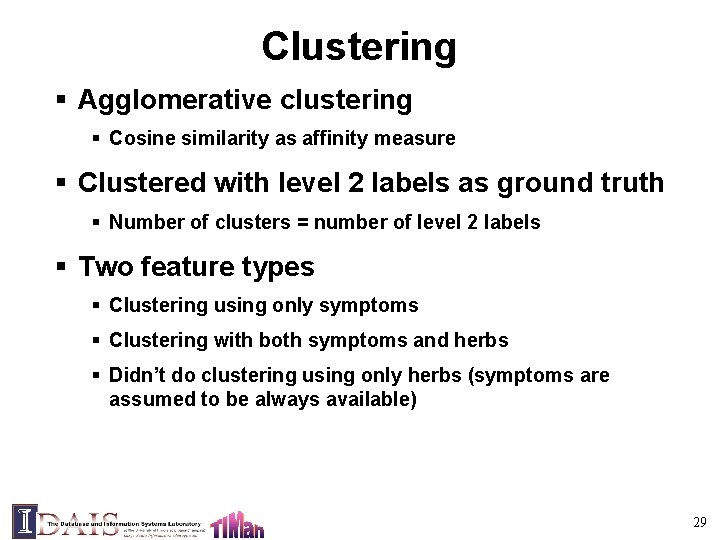
Clustering § Agglomerative clustering § Cosine similarity as affinity measure § Clustered with level 2 labels as ground truth § Number of clusters = number of level 2 labels § Two feature types § Clustering using only symptoms § Clustering with both symptoms and herbs § Didn’t do clustering using only herbs (symptoms are assumed to be always available) 29
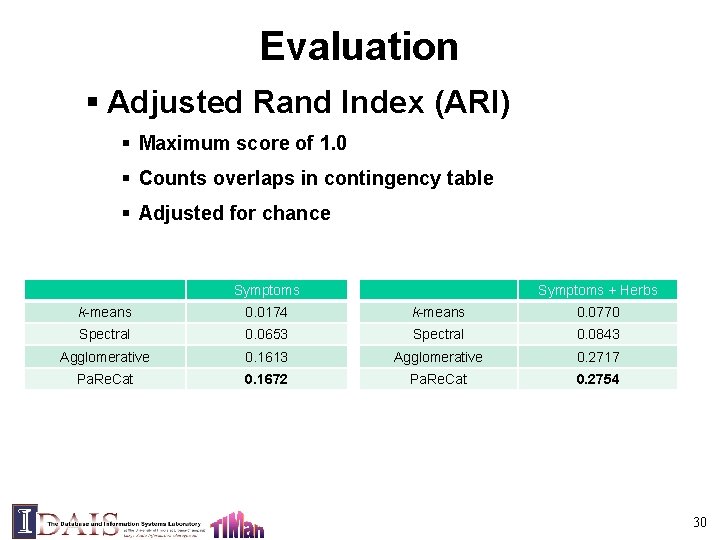
Evaluation § Adjusted Rand Index (ARI) § Maximum score of 1. 0 § Counts overlaps in contingency table § Adjusted for chance Symptoms + Herbs k-means 0. 0174 k-means 0. 0770 Spectral 0. 0653 Spectral 0. 0843 Agglomerative 0. 1613 Agglomerative 0. 2717 Pa. Re. Cat 0. 1672 Pa. Re. Cat 0. 2754 30
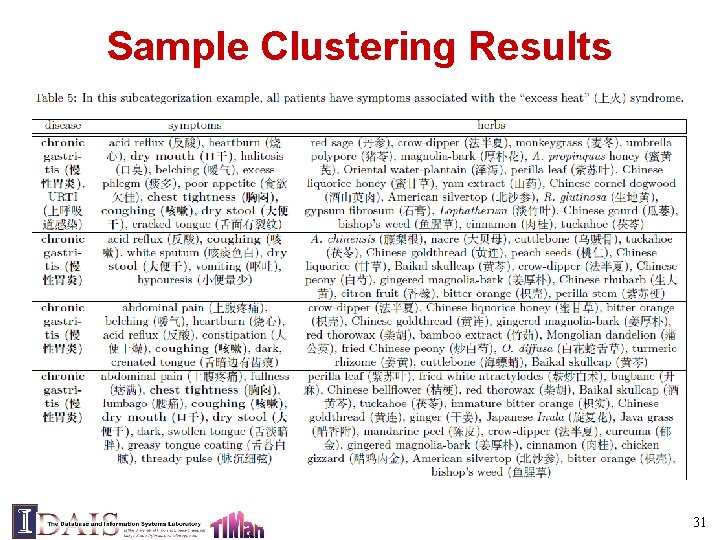
Sample Clustering Results 31
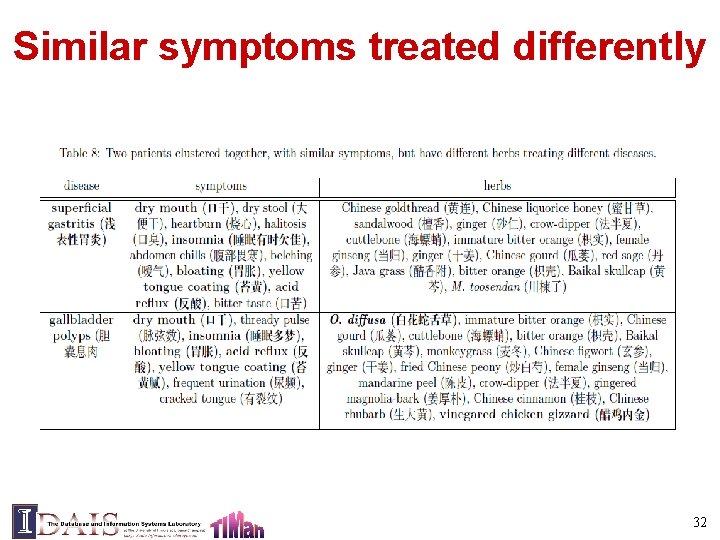
Similar symptoms treated differently 32
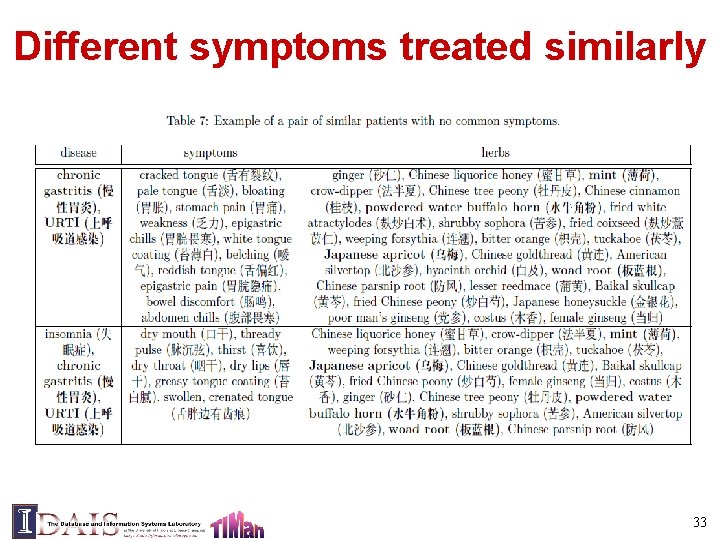
Different symptoms treated similarly 33
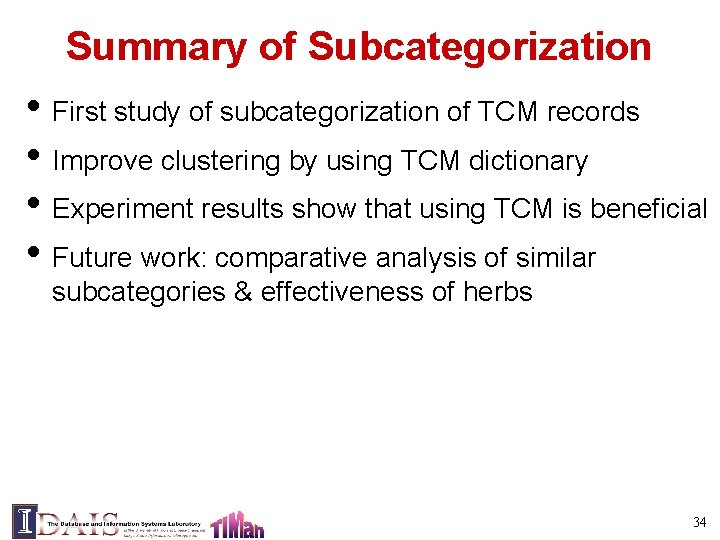
Summary of Subcategorization • First study of subcategorization of TCM records • Improve clustering by using TCM dictionary • Experiment results show that using TCM is beneficial • Future work: comparative analysis of similar subcategories & effectiveness of herbs 34
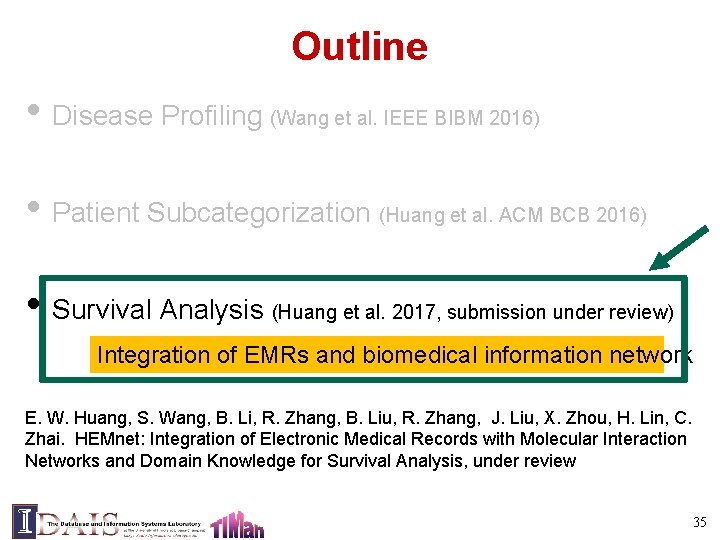
Outline • Disease Profiling (Wang et al. IEEE BIBM 2016) • Patient Subcategorization (Huang et al. ACM BCB 2016) • Survival Analysis (Huang et al. 2017, submission under review) Integration of EMRs and biomedical information network E. W. Huang, S. Wang, B. Li, R. Zhang, B. Liu, R. Zhang, J. Liu, X. Zhou, H. Lin, C. Zhai. HEMnet: Integration of Electronic Medical Records with Molecular Interaction Networks and Domain Knowledge for Survival Analysis, under review 35
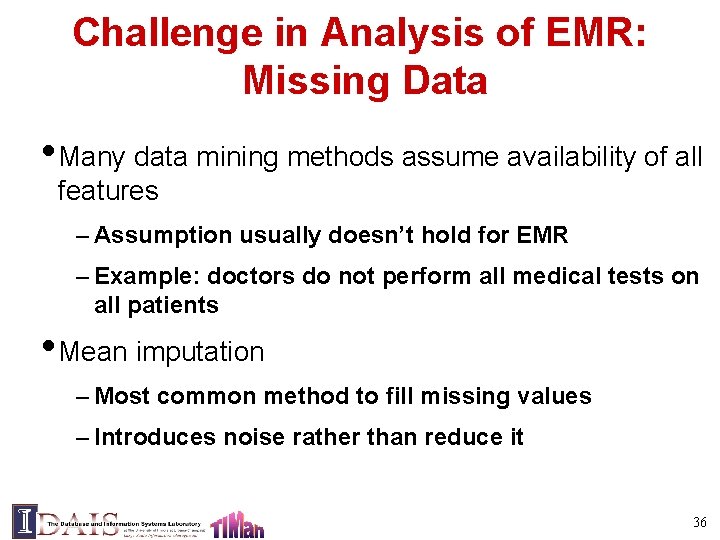
Challenge in Analysis of EMR: Missing Data • Many data mining methods assume availability of all features – Assumption usually doesn’t hold for EMR – Example: doctors do not perform all medical tests on all patients • Mean imputation – Most common method to fill missing values – Introduces noise rather than reduce it 36
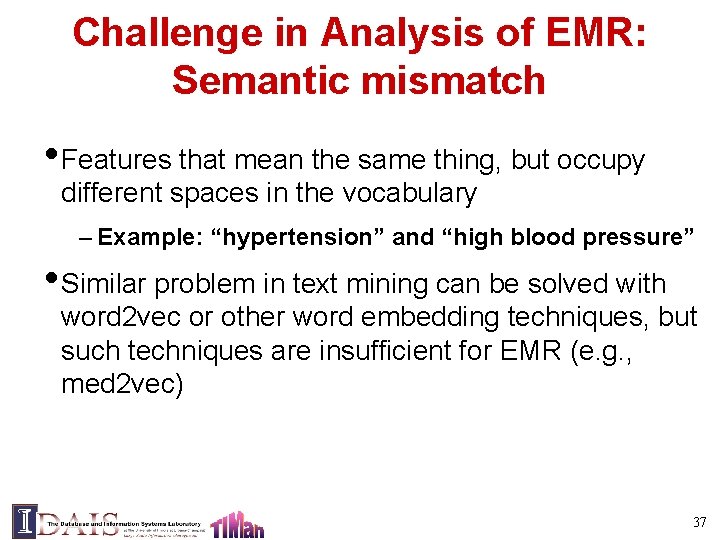
Challenge in Analysis of EMR: Semantic mismatch • Features that mean the same thing, but occupy different spaces in the vocabulary – Example: “hypertension” and “high blood pressure” • Similar problem in text mining can be solved with word 2 vec or other word embedding techniques, but such techniques are insufficient for EMR (e. g. , med 2 vec) 37
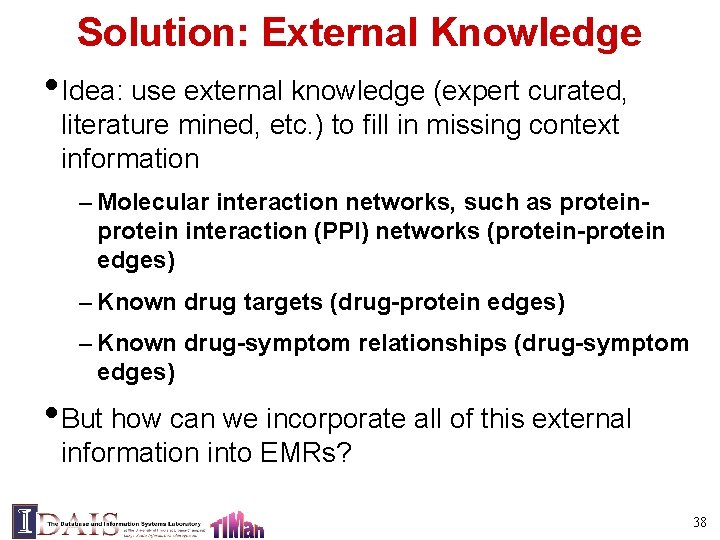
Solution: External Knowledge • Idea: use external knowledge (expert curated, literature mined, etc. ) to fill in missing context information – Molecular interaction networks, such as protein interaction (PPI) networks (protein-protein edges) – Known drug targets (drug-protein edges) – Known drug-symptom relationships (drug-symptom edges) • But how can we incorporate all of this external information into EMRs? 38
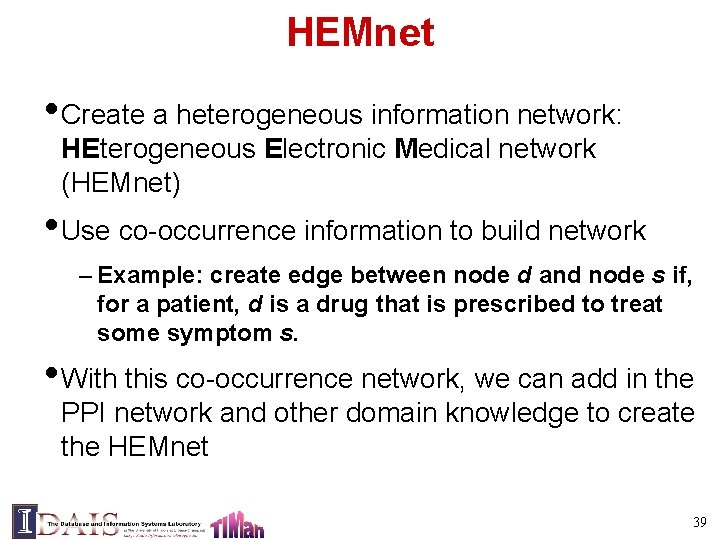
HEMnet • Create a heterogeneous information network: HEterogeneous Electronic Medical network (HEMnet) • Use co-occurrence information to build network – Example: create edge between node d and node s if, for a patient, d is a drug that is prescribed to treat some symptom s. • With this co-occurrence network, we can add in the PPI network and other domain knowledge to create the HEMnet 39
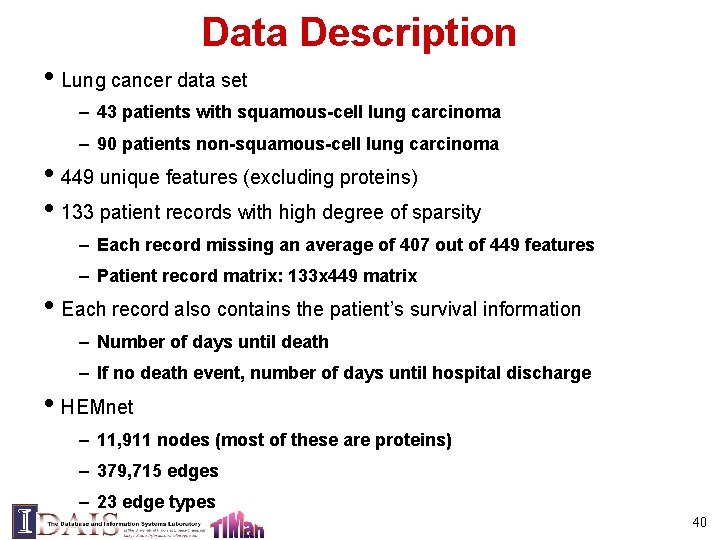
Data Description • Lung cancer data set – 43 patients with squamous-cell lung carcinoma – 90 patients non-squamous-cell lung carcinoma • 449 unique features (excluding proteins) • 133 patient records with high degree of sparsity – Each record missing an average of 407 out of 449 features – Patient record matrix: 133 x 449 matrix • Each record also contains the patient’s survival information – Number of days until death – If no death event, number of days until hospital discharge • HEMnet – 11, 911 nodes (most of these are proteins) – 379, 715 edges – 23 edge types 40
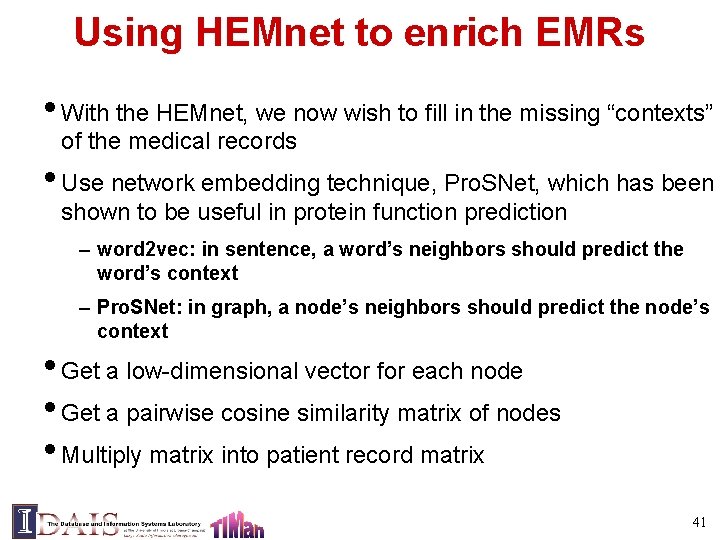
Using HEMnet to enrich EMRs • With the HEMnet, we now wish to fill in the missing “contexts” of the medical records • Use network embedding technique, Pro. SNet, which has been shown to be useful in protein function prediction – word 2 vec: in sentence, a word’s neighbors should predict the word’s context – Pro. SNet: in graph, a node’s neighbors should predict the node’s context • Get a low-dimensional vector for each node • Get a pairwise cosine similarity matrix of nodes • Multiply matrix into patient record matrix 41
![Pos Network Embedding Wang et al 17 Heterogenous network NodeEntity vector space z E Pos. Network Embedding [Wang et al. 17] Heterogenous network Node/Entity vector space z E](https://slidetodoc.com/presentation_image_h/13462b34239b5c73abfaf13e7c2e02d0/image-42.jpg)
Pos. Network Embedding [Wang et al. 17] Heterogenous network Node/Entity vector space z E 3 E 4 E 5 E 2 E 1 Input Pros. Net Dimensionality Reduction y x Output • General: applicable to any heterogeneous network – e. g. , gene, drug, disease network • Efficient: – 60 K nodes and 10 M edges: less than 30 minutes on a 12 -core CPU 42
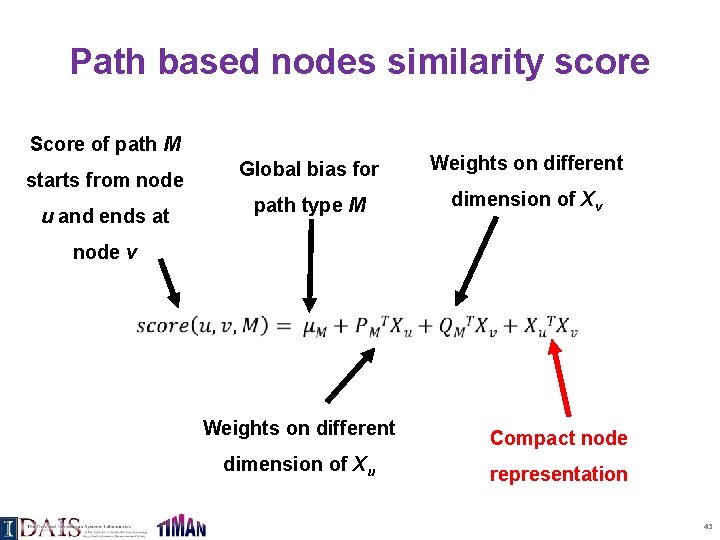
Path based nodes similarity score Score of path M starts from node u and ends at Global bias for Weights on different path type M dimension of Xv node v Weights on different dimension of Xu Compact node representation 43
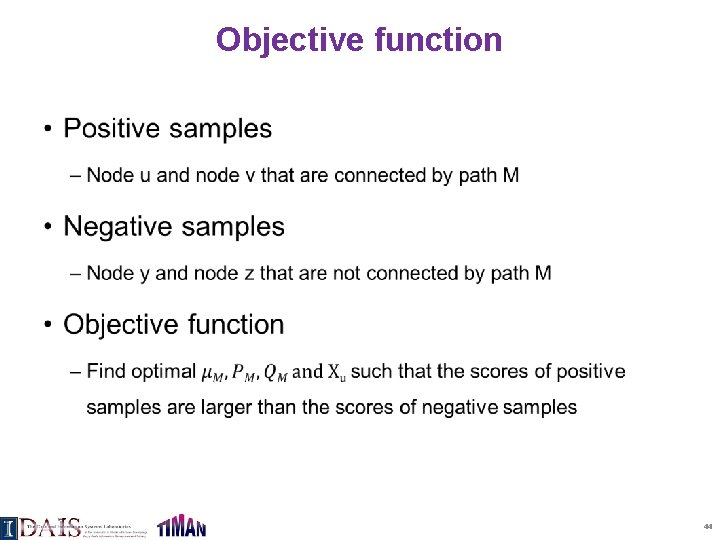
Objective function • 44
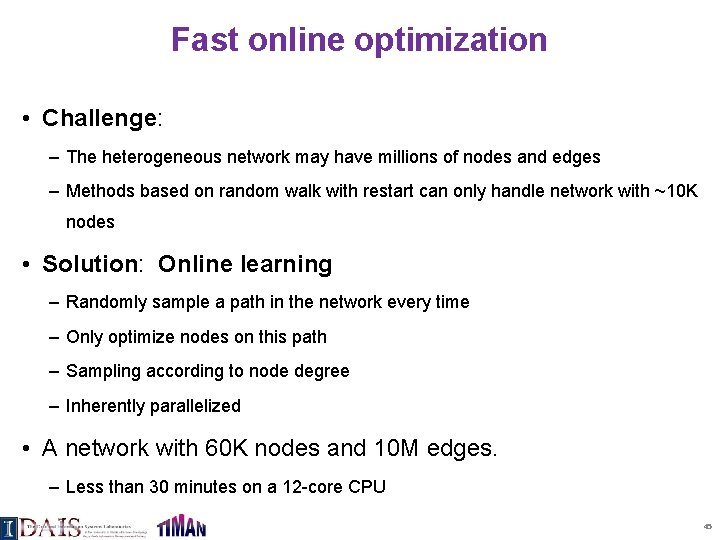
Fast online optimization • Challenge: – The heterogeneous network may have millions of nodes and edges – Methods based on random walk with restart can only handle network with ~10 K nodes • Solution: Online learning – Randomly sample a path in the network every time – Only optimize nodes on this path – Sampling according to node degree – Inherently parallelized • A network with 60 K nodes and 10 M edges. – Less than 30 minutes on a 12 -core CPU 45
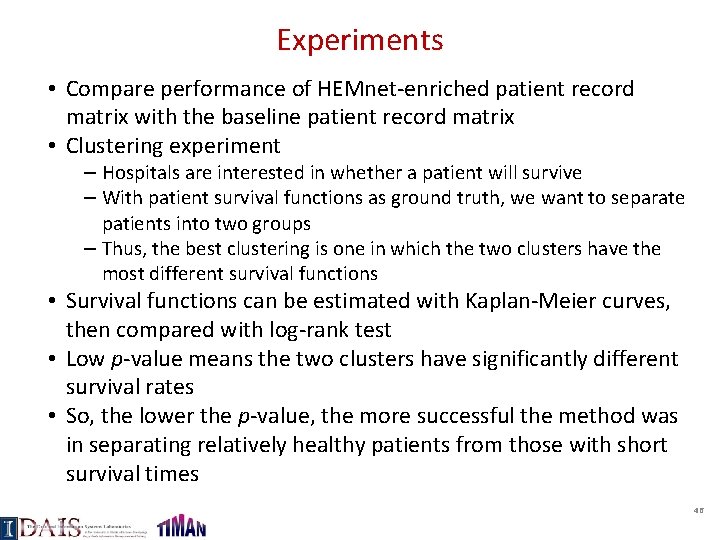
Experiments • Compare performance of HEMnet-enriched patient record matrix with the baseline patient record matrix • Clustering experiment – Hospitals are interested in whether a patient will survive – With patient survival functions as ground truth, we want to separate patients into two groups – Thus, the best clustering is one in which the two clusters have the most different survival functions • Survival functions can be estimated with Kaplan-Meier curves, then compared with log-rank test • Low p-value means the two clusters have significantly different survival rates • So, the lower the p-value, the more successful the method was in separating relatively healthy patients from those with short survival times 46
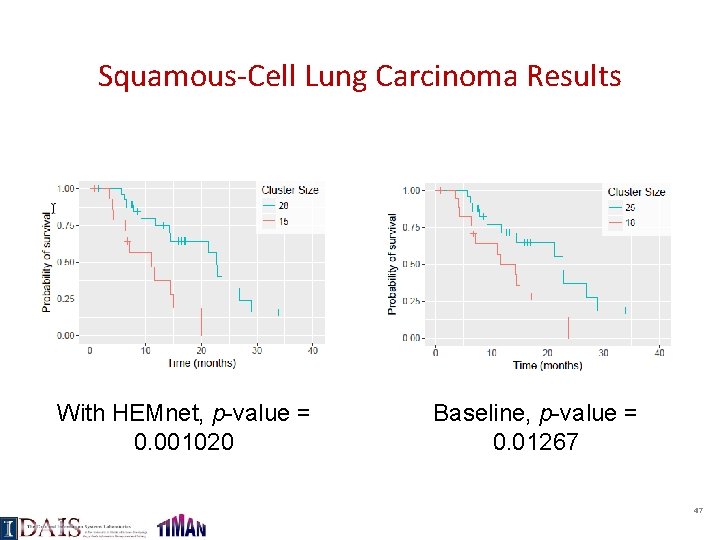
Squamous-Cell Lung Carcinoma Results With HEMnet, p-value = 0. 001020 Baseline, p-value = 0. 01267 47
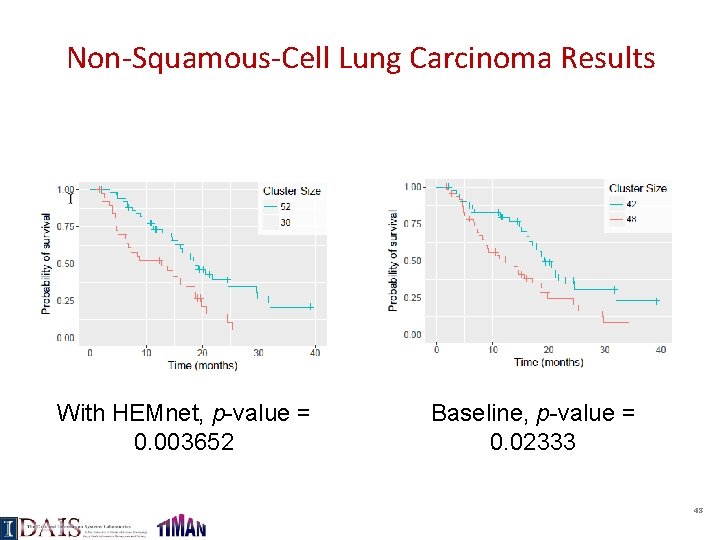
Non-Squamous-Cell Lung Carcinoma Results With HEMnet, p-value = 0. 003652 Baseline, p-value = 0. 02333 48
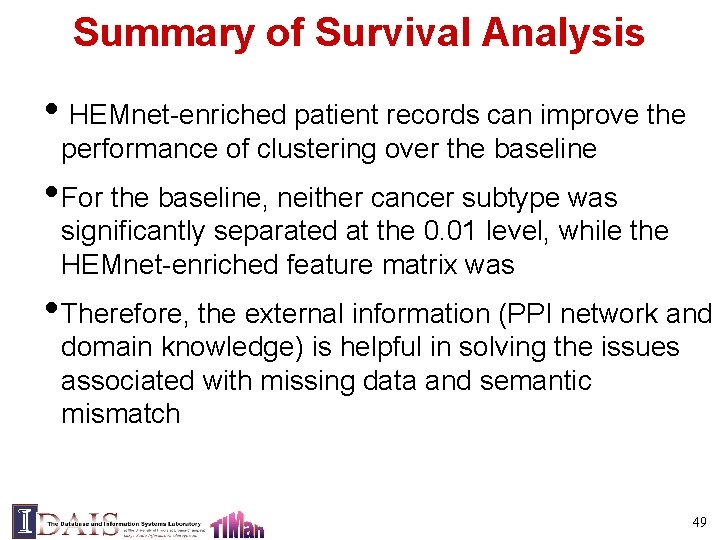
Summary of Survival Analysis • HEMnet-enriched patient records can improve the performance of clustering over the baseline • For the baseline, neither cancer subtype was significantly separated at the 0. 01 level, while the HEMnet-enriched feature matrix was • Therefore, the external information (PPI network and domain knowledge) is helpful in solving the issues associated with missing data and semantic mismatch 49
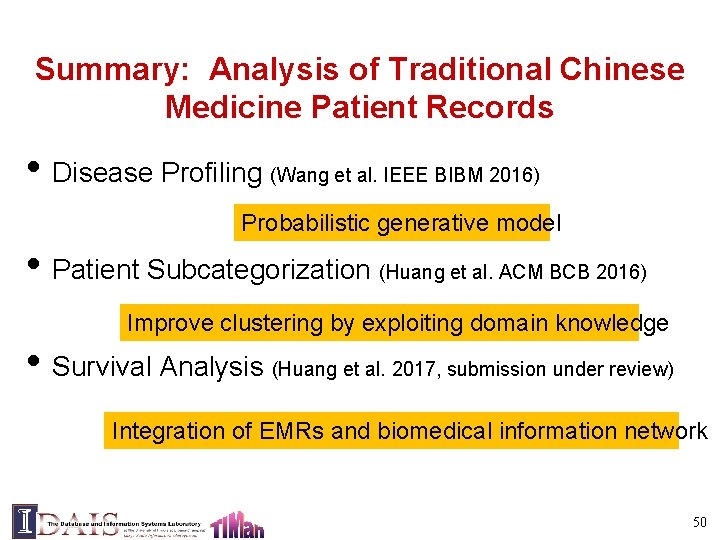
Summary: Analysis of Traditional Chinese Medicine Patient Records • Disease Profiling (Wang et al. IEEE BIBM 2016) Probabilistic generative model • Patient Subcategorization (Huang et al. ACM BCB 2016) Improve clustering by exploiting domain knowledge • Survival Analysis (Huang et al. 2017, submission under review) Integration of EMRs and biomedical information network 50
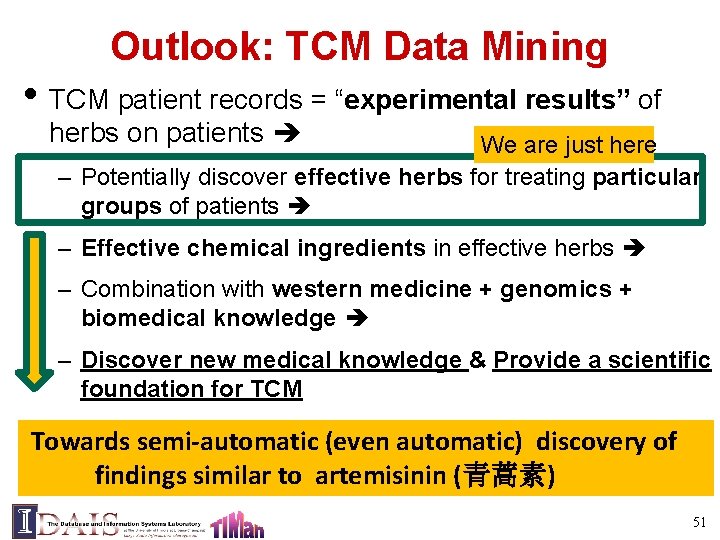
Outlook: TCM Data Mining • TCM patient records = “experimental results” of herbs on patients We are just here – Potentially discover effective herbs for treating particular groups of patients – Effective chemical ingredients in effective herbs – Combination with western medicine + genomics + biomedical knowledge – Discover new medical knowledge & Provide a scientific foundation for TCM Towards semi-automatic (even automatic) discovery of findings similar to artemisinin (青蒿素) 51
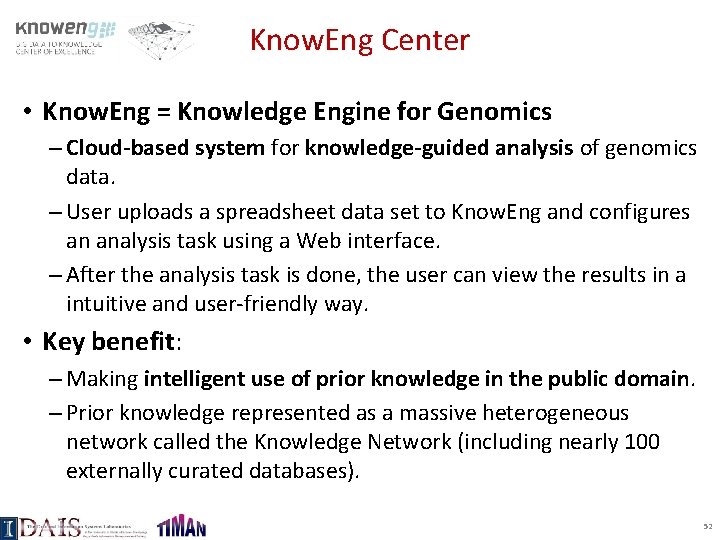
Know. Eng Center • Know. Eng = Knowledge Engine for Genomics – Cloud-based system for knowledge-guided analysis of genomics data. – User uploads a spreadsheet data set to Know. Eng and configures an analysis task using a Web interface. – After the analysis task is done, the user can view the results in a intuitive and user-friendly way. • Key benefit: – Making intelligent use of prior knowledge in the public domain. – Prior knowledge represented as a massive heterogeneous network called the Knowledge Network (including nearly 100 externally curated databases). 52
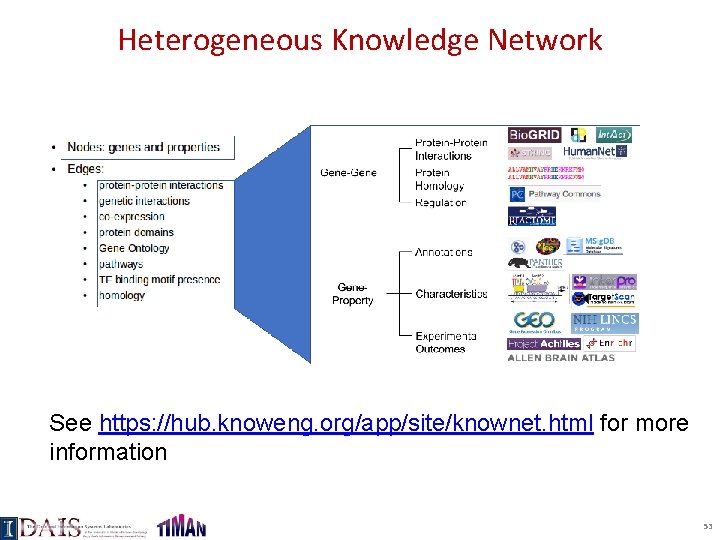
Heterogeneous Knowledge Network See https: //hub. knoweng. org/app/site/knownet. html for more information 53
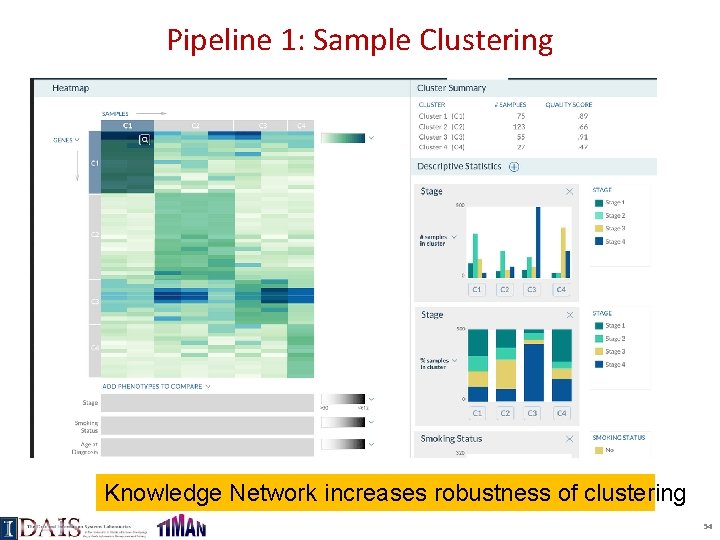
Pipeline 1: Sample Clustering Knowledge Network increases robustness of clustering 54
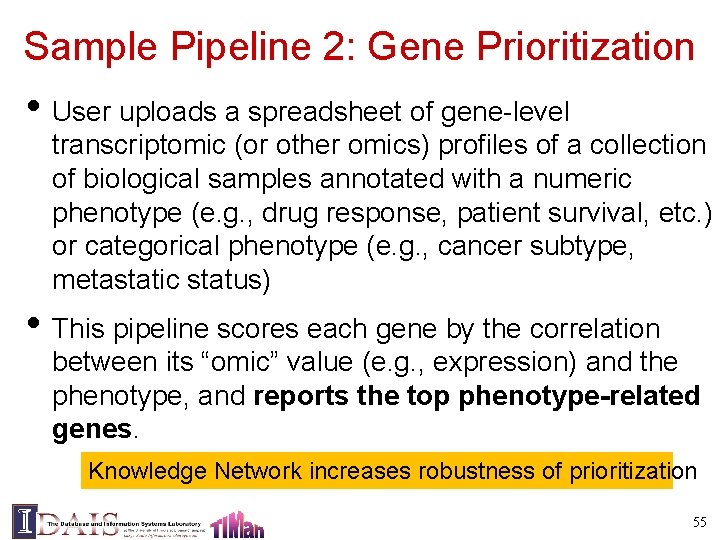
Sample Pipeline 2: Gene Prioritization • User uploads a spreadsheet of gene-level transcriptomic (or other omics) profiles of a collection of biological samples annotated with a numeric phenotype (e. g. , drug response, patient survival, etc. ) or categorical phenotype (e. g. , cancer subtype, metastatic status) • This pipeline scores each gene by the correlation between its “omic” value (e. g. , expression) and the phenotype, and reports the top phenotype-related genes. Knowledge Network increases robustness of prioritization 55
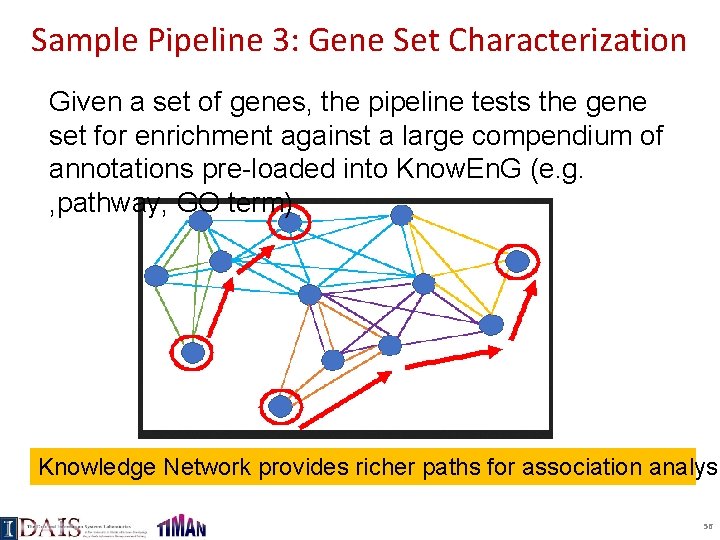
Sample Pipeline 3: Gene Set Characterization Given a set of genes, the pipeline tests the gene set for enrichment against a large compendium of annotations pre-loaded into Know. En. G (e. g. , pathway, GO term) Knowledge Network provides richer paths for association analysi 56
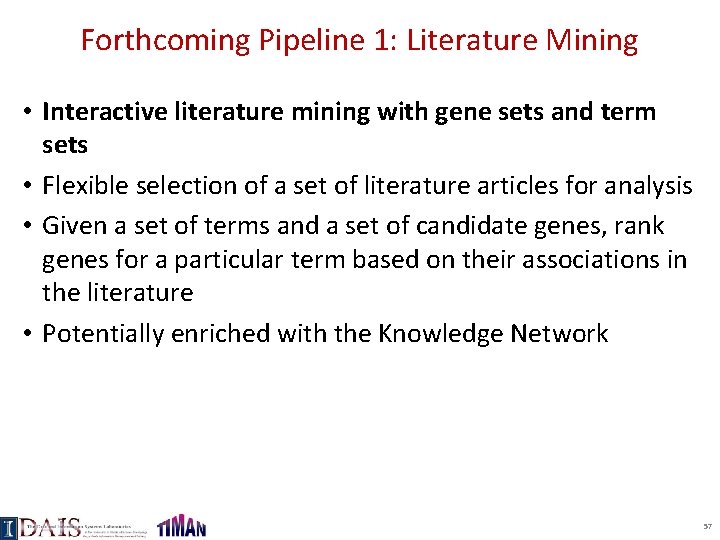
Forthcoming Pipeline 1: Literature Mining • Interactive literature mining with gene sets and term sets • Flexible selection of a set of literature articles for analysis • Given a set of terms and a set of candidate genes, rank genes for a particular term based on their associations in the literature • Potentially enriched with the Knowledge Network 57
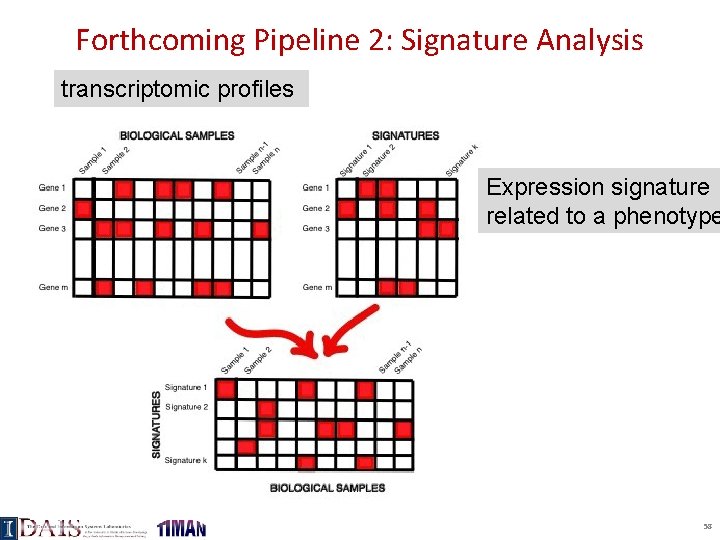
Forthcoming Pipeline 2: Signature Analysis transcriptomic profiles Expression signature related to a phenotype 58
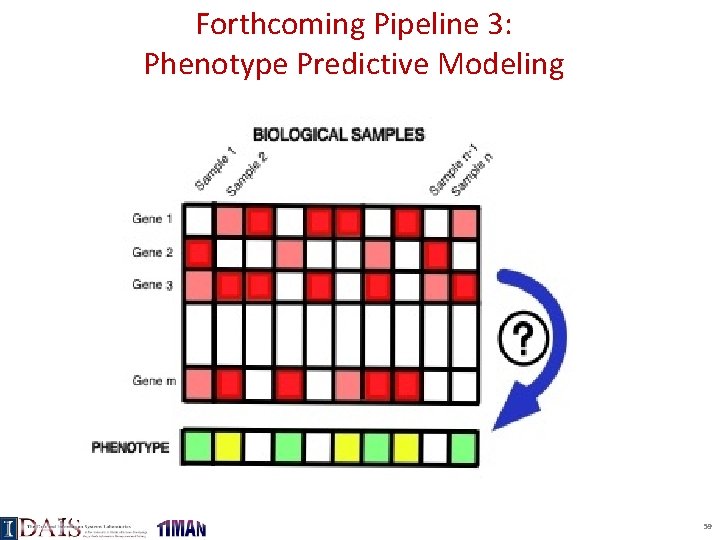
Forthcoming Pipeline 3: Phenotype Predictive Modeling 59
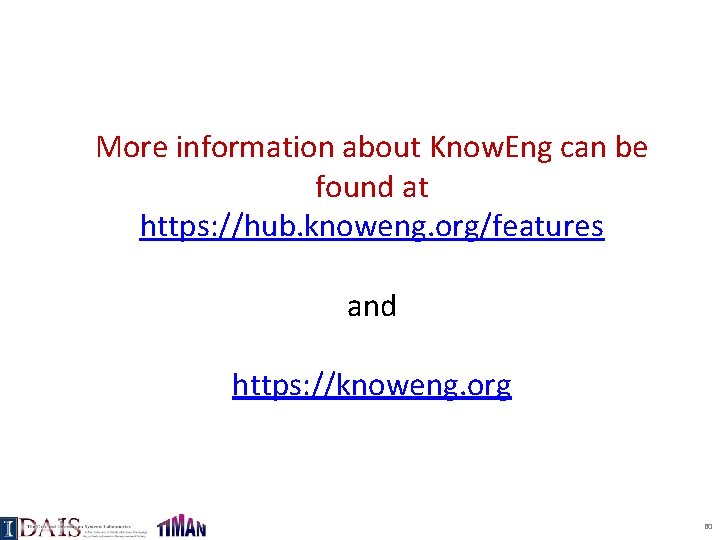
More information about Know. Eng can be found at https: //hub. knoweng. org/features and https: //knoweng. org 60
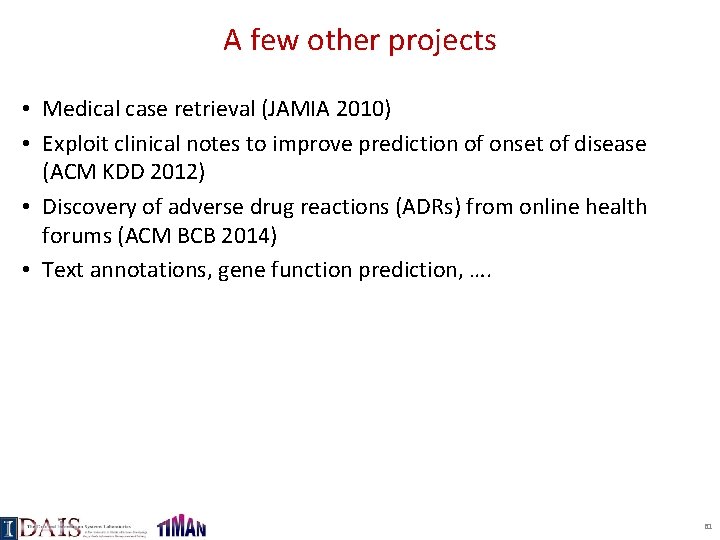
A few other projects • Medical case retrieval (JAMIA 2010) • Exploit clinical notes to improve prediction of onset of disease (ACM KDD 2012) • Discovery of adverse drug reactions (ADRs) from online health forums (ACM BCB 2014) • Text annotations, gene function prediction, …. 61
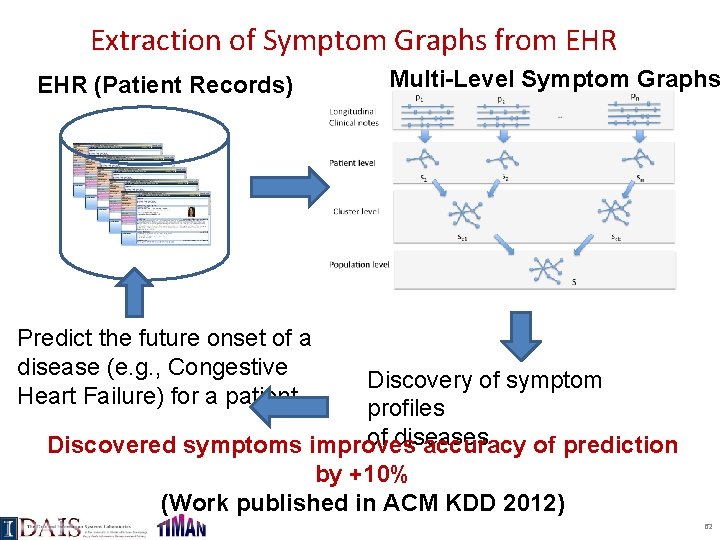
Extraction of Symptom Graphs from EHR (Patient Records) Multi-Level Symptom Graphs Predict the future onset of a disease (e. g. , Congestive Heart Failure) for a patient Discovery of symptom profiles of diseases Discovered symptoms improves accuracy of prediction by +10% (Work published in ACM KDD 2012) 62
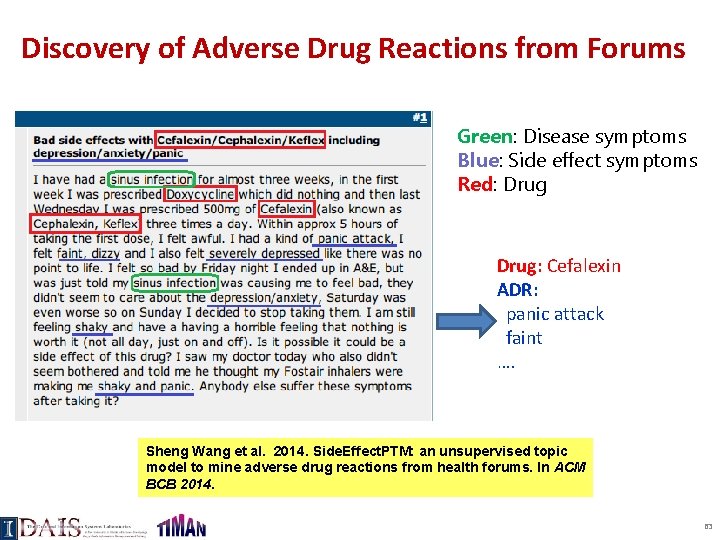
Discovery of Adverse Drug Reactions from Forums Green: Disease symptoms Blue: Side effect symptoms Red: Drug: Cefalexin ADR: panic attack faint …. Sheng Wang et al. 2014. Side. Effect. PTM: an unsupervised topic model to mine adverse drug reactions from health forums. In ACM BCB 2014. 63
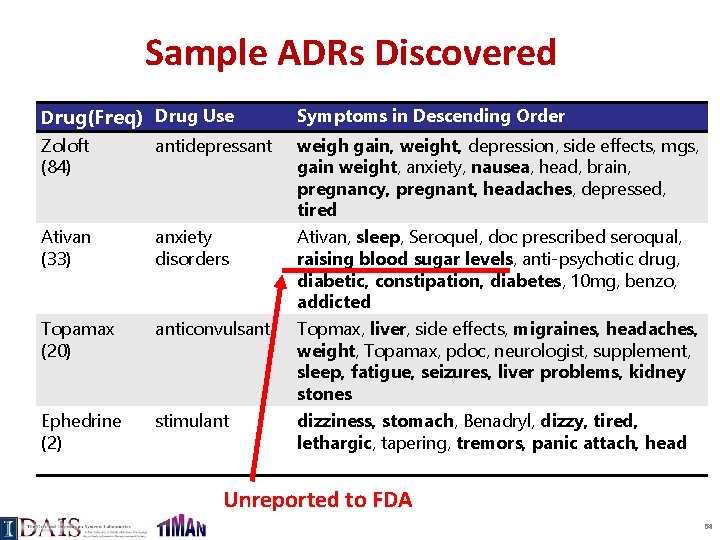
Sample ADRs Discovered Drug(Freq) Drug Use Symptoms in Descending Order Zoloft (84) antidepressant Ativan (33) anxiety disorders Topamax (20) anticonvulsant Ephedrine (2) stimulant weigh gain, weight, depression, side effects, mgs, gain weight, anxiety, nausea, head, brain, pregnancy, pregnant, headaches, depressed, tired Ativan, sleep, Seroquel, doc prescribed seroqual, raising blood sugar levels, anti-psychotic drug, diabetic, constipation, diabetes, 10 mg, benzo, addicted Topmax, liver, side effects, migraines, headaches, weight, Topamax, pdoc, neurologist, supplement, sleep, fatigue, seizures, liver problems, kidney stones dizziness, stomach, Benadryl, dizzy, tired, lethargic, tapering, tremors, panic attach, head Unreported to FDA 64
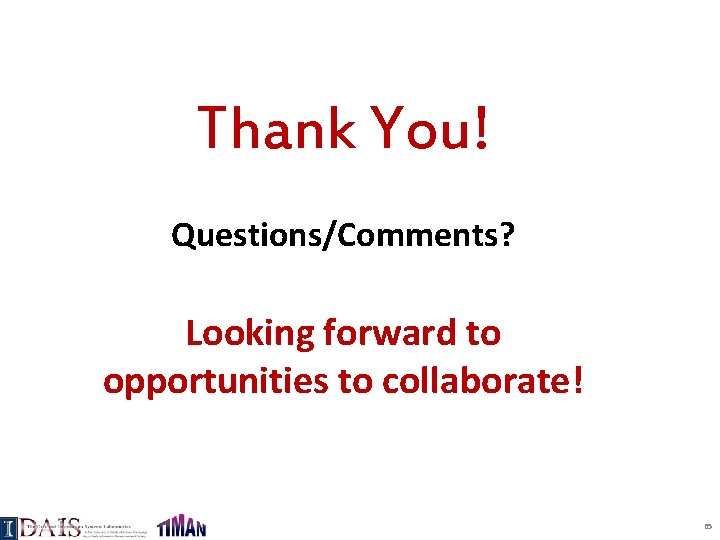
Thank You! Questions/Comments? Looking forward to opportunities to collaborate! 65
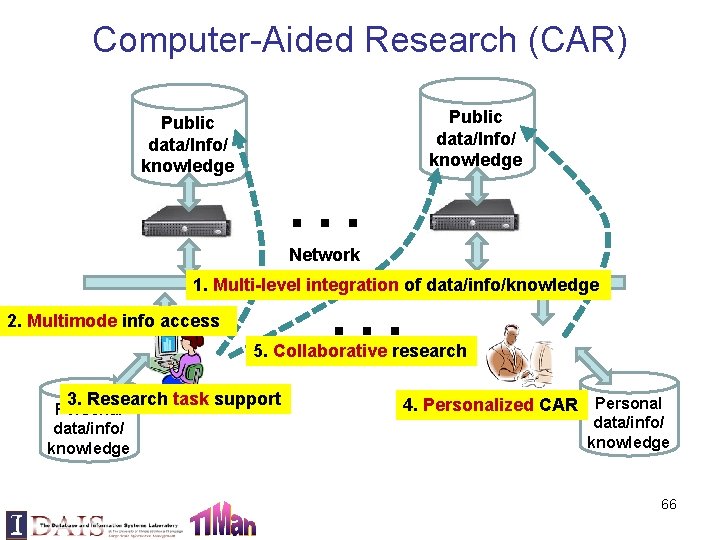
Computer-Aided Research (CAR) Public data/Info/ knowledge … … Public data/Info/ knowledge Network 1. Multi-level integration of data/info/knowledge 2. Multimode info access 5. Collaborative research 3. Research task support Personal data/info/ knowledge 4. Personalized CAR Personal data/info/ knowledge 66
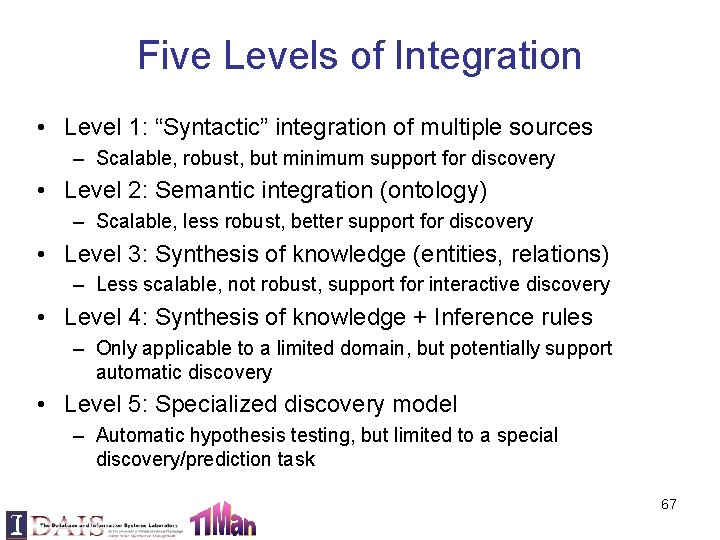
Five Levels of Integration • Level 1: “Syntactic” integration of multiple sources – Scalable, robust, but minimum support for discovery • Level 2: Semantic integration (ontology) – Scalable, less robust, better support for discovery • Level 3: Synthesis of knowledge (entities, relations) – Less scalable, not robust, support for interactive discovery • Level 4: Synthesis of knowledge + Inference rules – Only applicable to a limited domain, but potentially support automatic discovery • Level 5: Specialized discovery model – Automatic hypothesis testing, but limited to a special discovery/prediction task 67
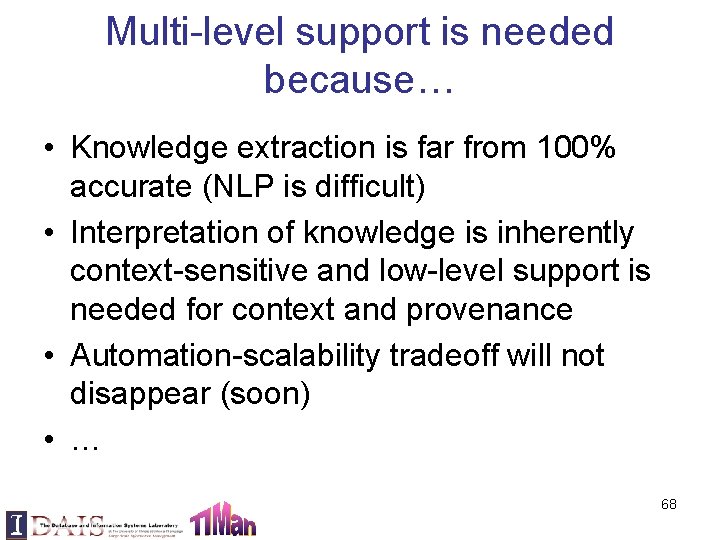
Multi-level support is needed because… • Knowledge extraction is far from 100% accurate (NLP is difficult) • Interpretation of knowledge is inherently context-sensitive and low-level support is needed for context and provenance • Automation-scalability tradeoff will not disappear (soon) • … 68
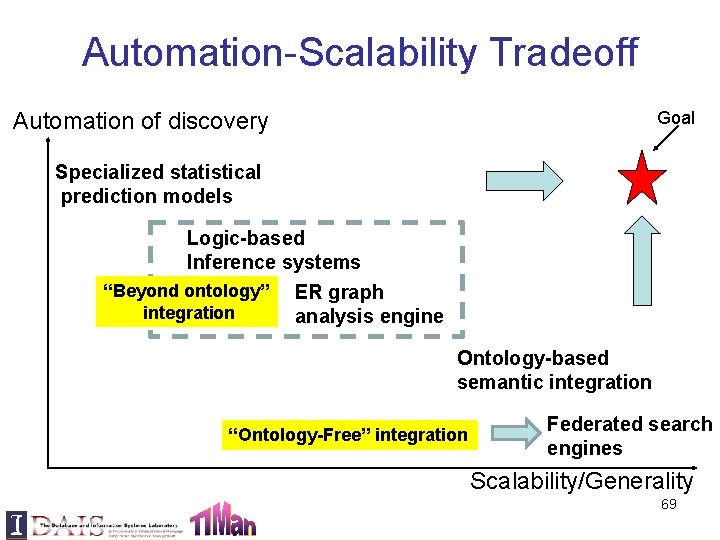
Automation-Scalability Tradeoff Automation of discovery Goal Specialized statistical prediction models Logic-based Inference systems “Beyond ontology” ER graph integration analysis engine Ontology-based semantic integration “Ontology-Free” integration Federated search engines Scalability/Generality 69
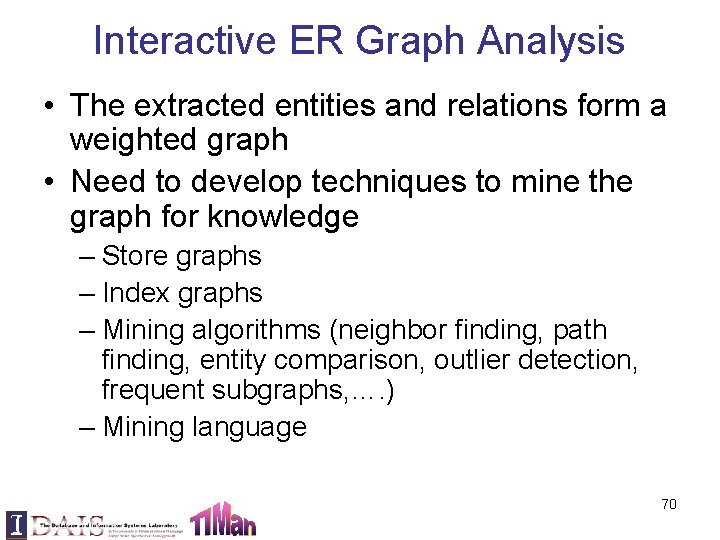
Interactive ER Graph Analysis • The extracted entities and relations form a weighted graph • Need to develop techniques to mine the graph for knowledge – Store graphs – Index graphs – Mining algorithms (neighbor finding, path finding, entity comparison, outlier detection, frequent subgraphs, …. ) – Mining language 70
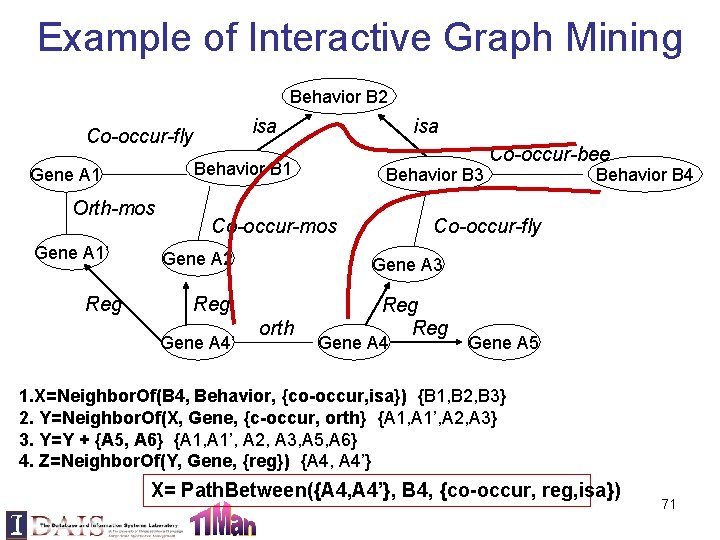
Example of Interactive Graph Mining Behavior B 2 isa Co-occur-fly Gene A 1 Orth-mos Gene A 1’ Reg isa Behavior B 1 Behavior B 3 Co-occur-mos Gene A 3 Reg Reg Gene A 4’ Behavior B 4 Co-occur-fly Gene A 2 orth Co-occur-bee Gene A 4 Gene A 5 1. X=Neighbor. Of(B 4, Behavior, {co-occur, isa}) {B 1, B 2, B 3} 2. Y=Neighbor. Of(X, Gene, {c-occur, orth} {A 1, A 1’, A 2, A 3} 3. Y=Y + {A 5, A 6} {A 1, A 1’, A 2, A 3, A 5, A 6} 4. Z=Neighbor. Of(Y, Gene, {reg}) {A 4, A 4’} X= Path. Between({A 4, A 4’}, B 4, {co-occur, reg, isa}) 71
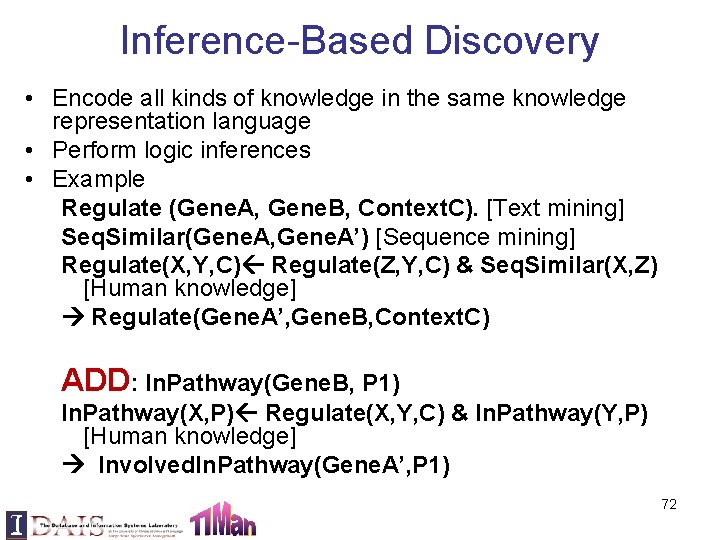
Inference-Based Discovery • Encode all kinds of knowledge in the same knowledge representation language • Perform logic inferences • Example Regulate (Gene. A, Gene. B, Context. C). [Text mining] Seq. Similar(Gene. A, Gene. A’) [Sequence mining] Regulate(X, Y, C) Regulate(Z, Y, C) & Seq. Similar(X, Z) [Human knowledge] Regulate(Gene. A’, Gene. B, Context. C) ADD: In. Pathway(Gene. B, P 1) In. Pathway(X, P) Regulate(X, Y, C) & In. Pathway(Y, P) [Human knowledge] Involved. In. Pathway(Gene. A’, P 1) 72
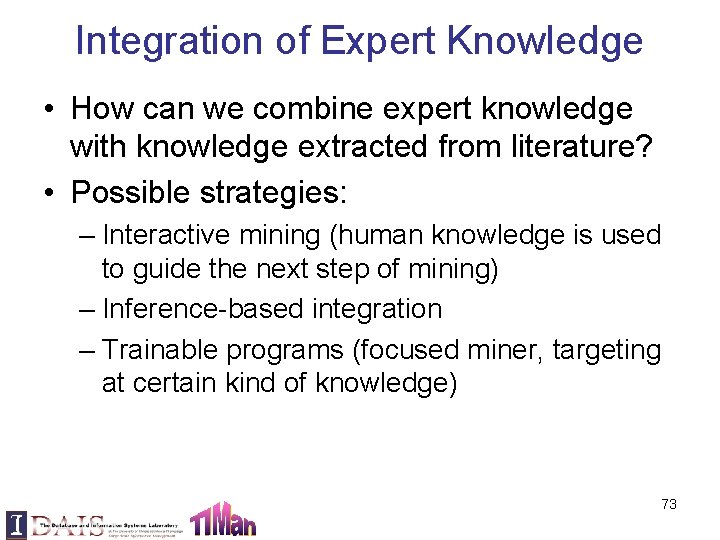
Integration of Expert Knowledge • How can we combine expert knowledge with knowledge extracted from literature? • Possible strategies: – Interactive mining (human knowledge is used to guide the next step of mining) – Inference-based integration – Trainable programs (focused miner, targeting at certain kind of knowledge) 73
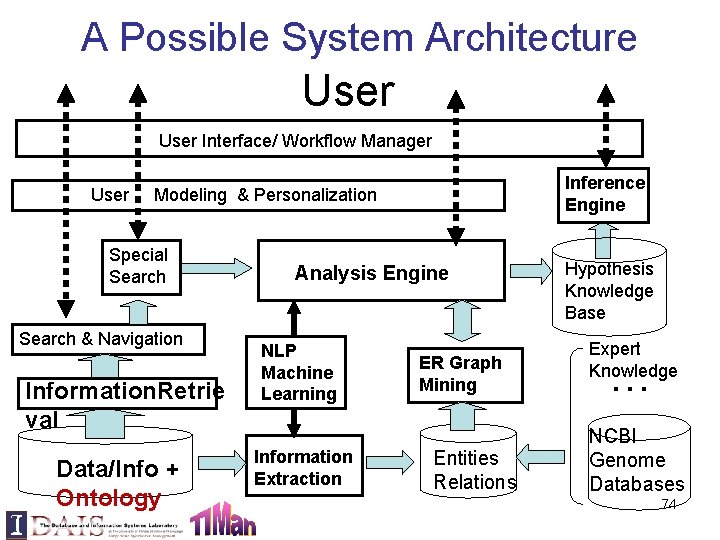
A Possible System Architecture User Interface/ Workflow Manager Inference Engine User Modeling & Personalization Special Search & Navigation Information. Retrie val Data/Info + Ontology Analysis Engine NLP Machine Learning Information Extraction ER Graph Mining Entities Relations Hypothesis Knowledge Base Expert Knowledge … NCBI Genome Databases 74
New york college of traditional chinese medicine manhattan
Anglo chinese school primary
Gong chinese instrument
Psalm 23 in chinese (traditional)
Chinese traditional festival
Happy mothers day chinese
Chinese traditional wedding
Pien fu traditional chinese clothing
Role of pharmacognosy in allopathy
Health systems building blocks
Traditional mi'kmaq medicine wheel
Statistical analysis system
On the statistical analysis of dirty pictures
Preserving statistical validity in adaptive data analysis
Multivariate statistical analysis
Cowan statistical data analysis
Statistical business analysis
Conjoint analysis in r
Cowan statistical data analysis pdf
Statistical analysis of experimental data
Approaches to literary criticism
Applying critical approaches to literary analysis quiz
Corpus approaches to discourse analysis
Discourse and register analysis approaches
A comparison of approaches to large-scale data analysis
Applying critical approaches to literary analysis
Applying critical approaches to literary analysis
American born chinese transformer
Traditional development approach
Job analysis and competency models
Traditional analysis
Traditional job analysis vs competency approach
Gss graduate route
Using statistical measures to compare populations
Stat
Statistical mechanics
Equipartition theorem proof
Statistical displays
Types of statistical questions
Statistical tdm
Statistical thermodynamics in chemistry
What is microcanonical ensemble
Univariate analysis tests
In continuous statistical surfaces the z values occur
Generating alpha meaning
Statistical power table
Statistical natural language processing
What do you understand by statistical investigation
Statistical package for the social sciences
Statistical process control ppt
Statistical symbols and meanings
Chebyshev's inequality
Probability and statistical inference 9th solution pdf
National academy of statistical administration
Misleading graphs and statistics
3 sigma
Statistical methods of demand forecasting
Statistical versus deterministic relationship
Partition function
Statistical algorithms
Statistical computing environment
Statistical hypothesis formula
Statistical test flow chart
Government statistical officer
Statistical methods of demand forecasting
Unit 3 statistical studies answers
Statistical journal entry example
Statistical investigations examples
Partition function in statistical mechanics
Appropriate statistical chart
What is a statistical syllogism
Operations management quality control
Distinguish between synchronous and statistical tdm.
Statistical treatment of data example
Introduction to statistical quality control montgomery