Power laws scalefree networks the structure of the
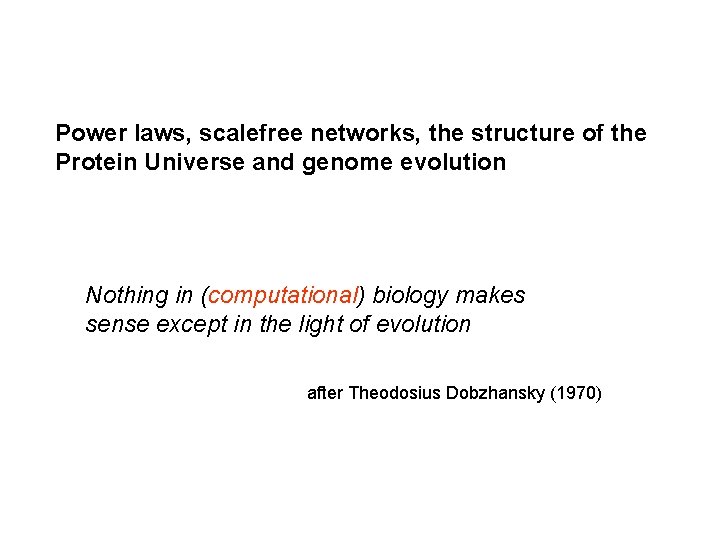
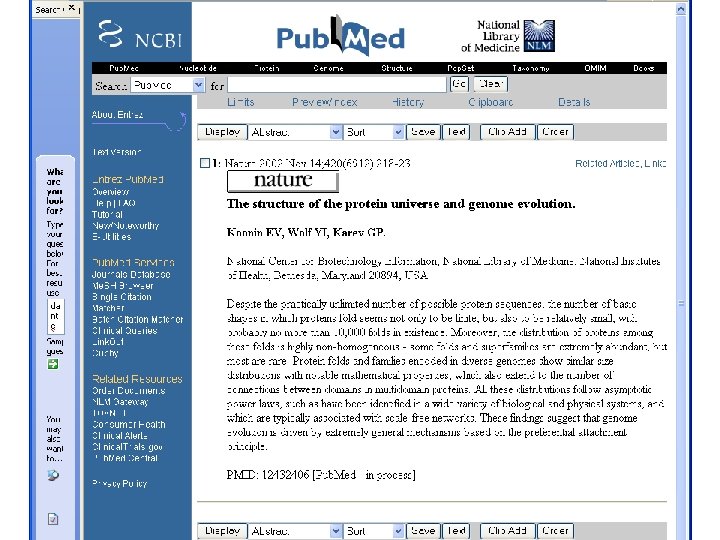
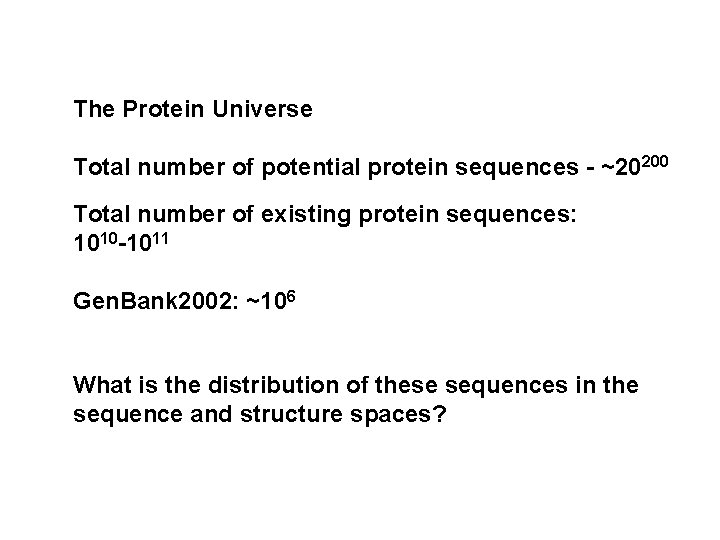
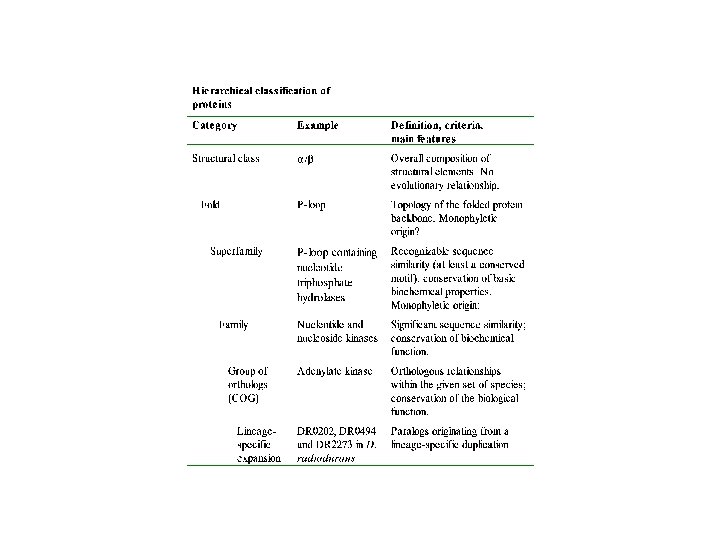
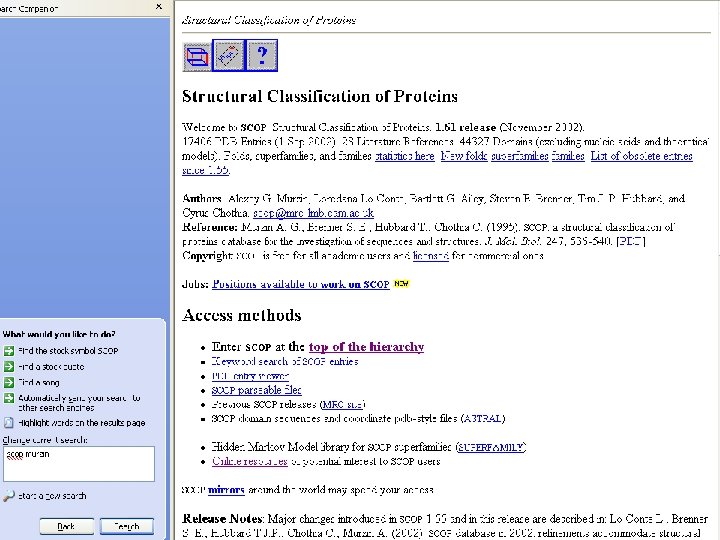
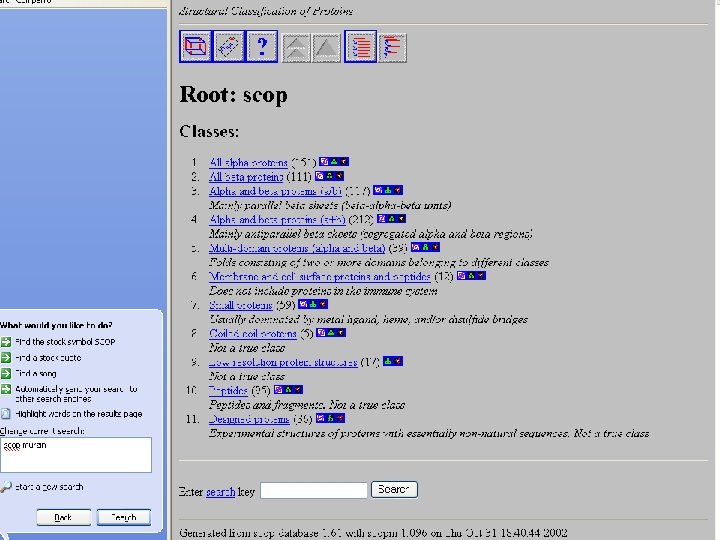
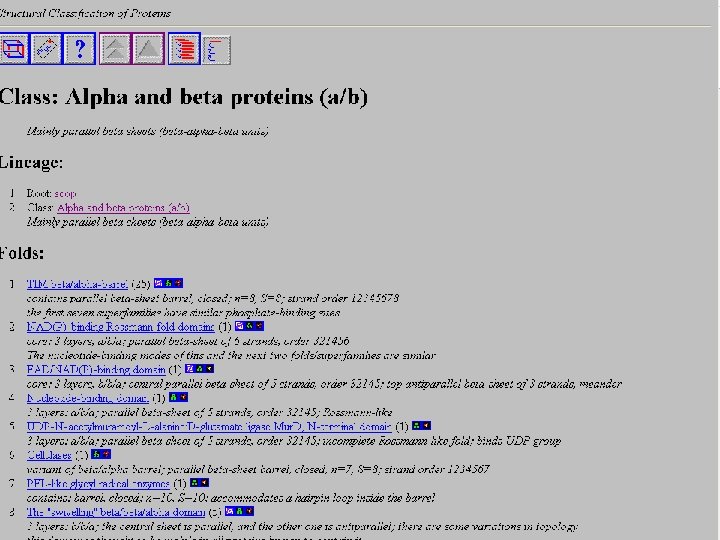
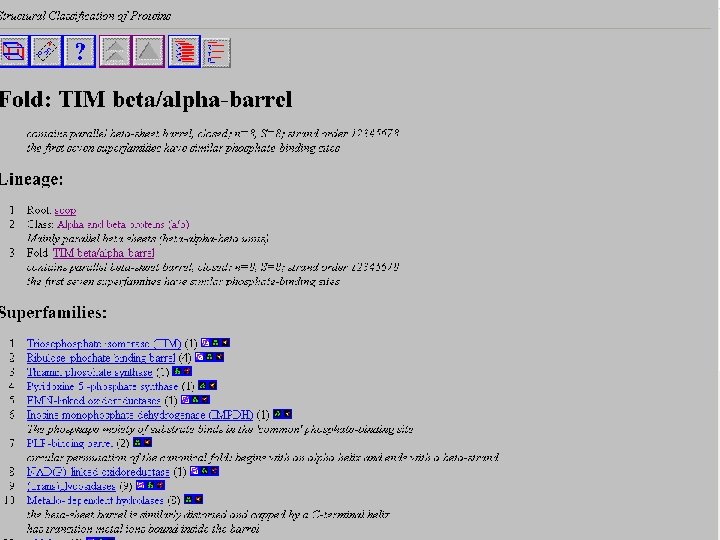
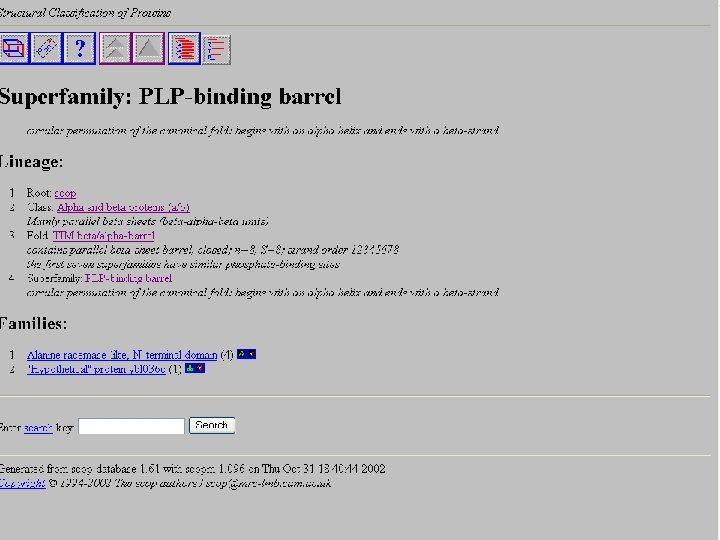
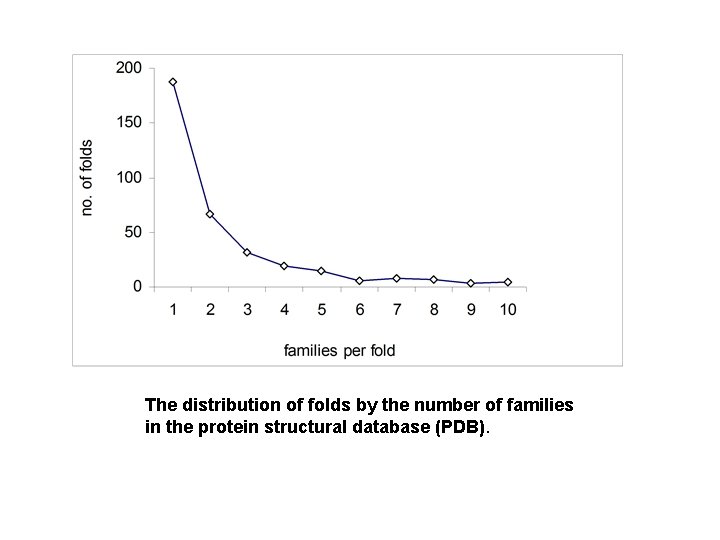
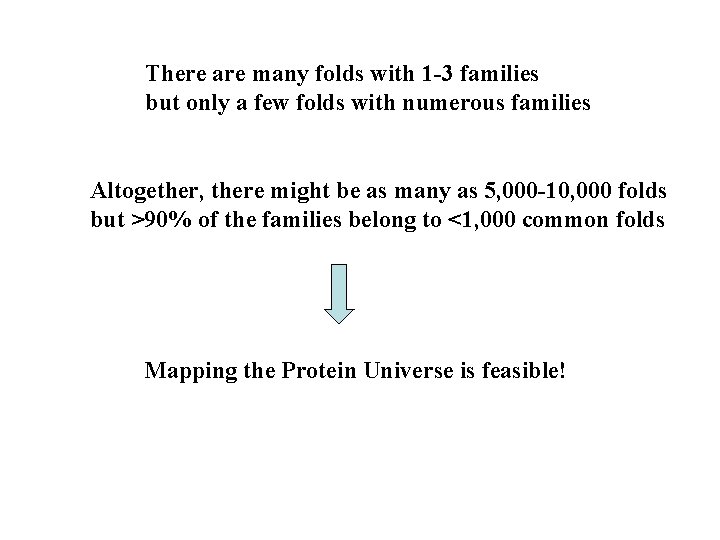
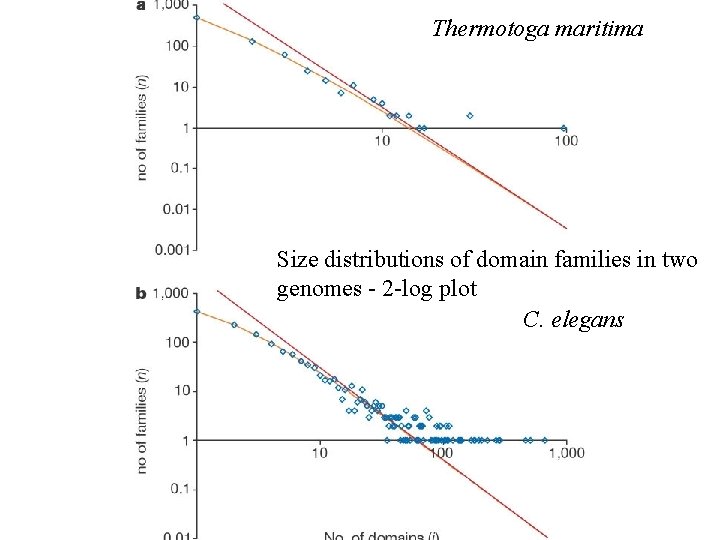
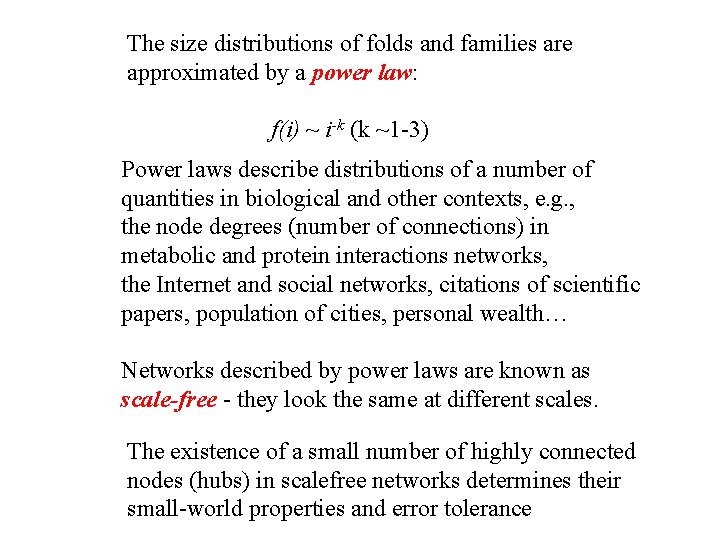
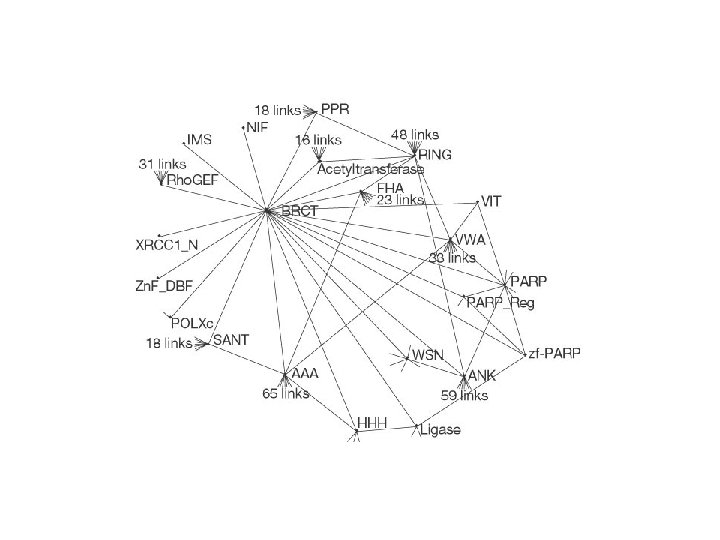
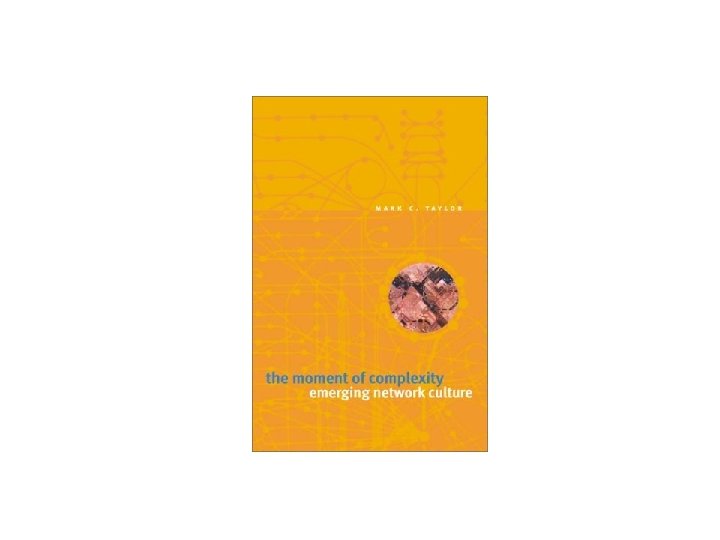
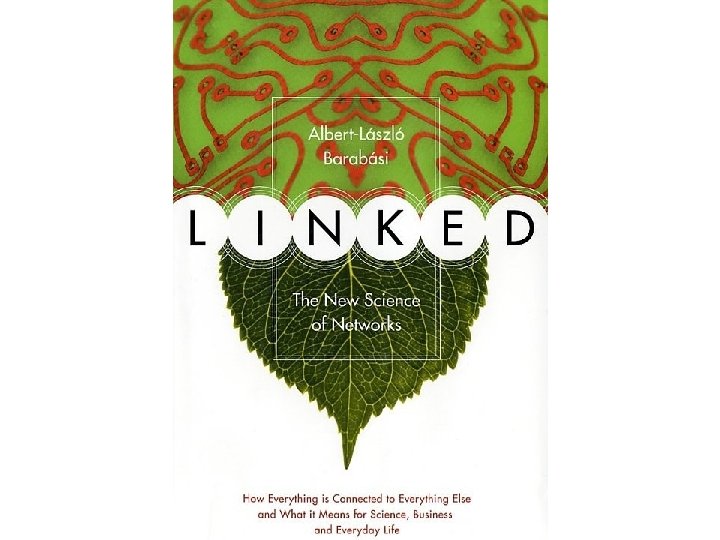
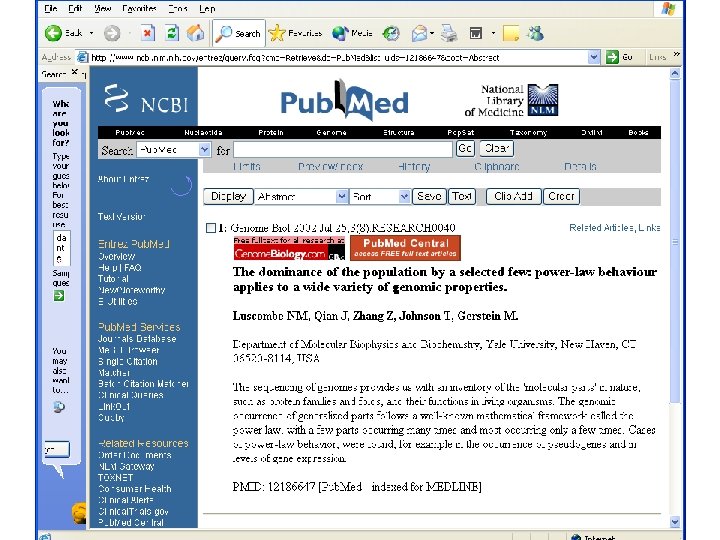
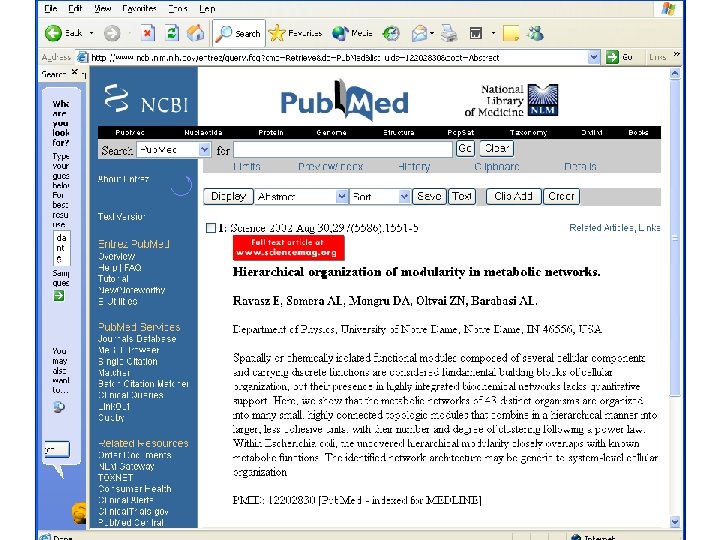
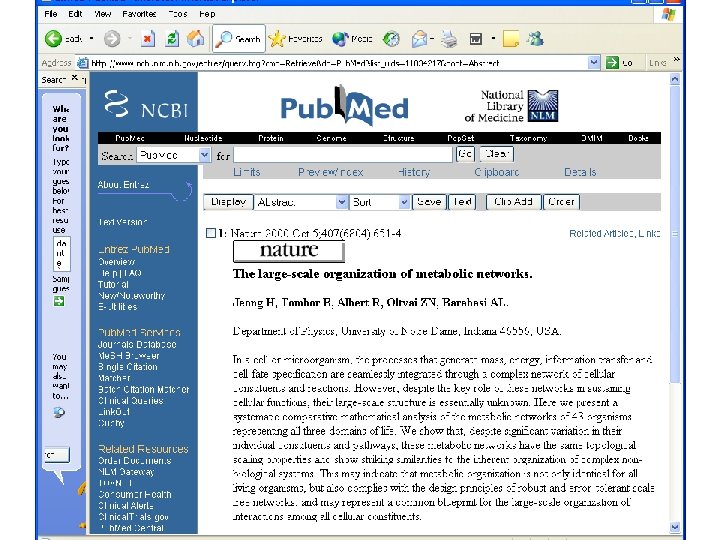
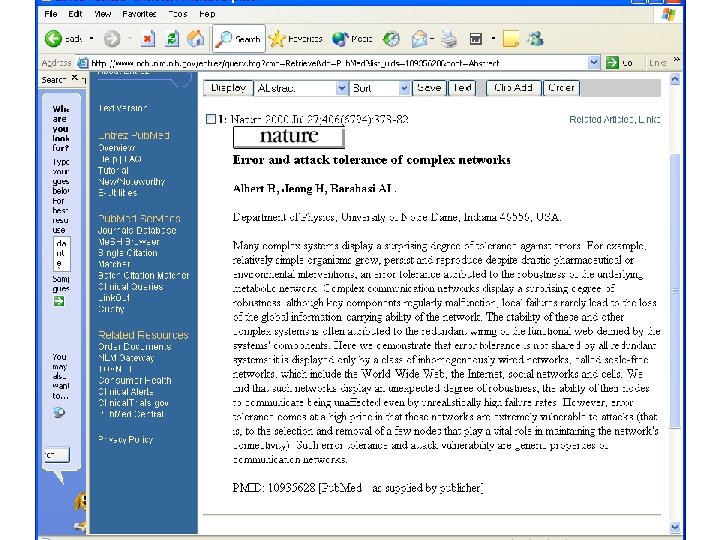
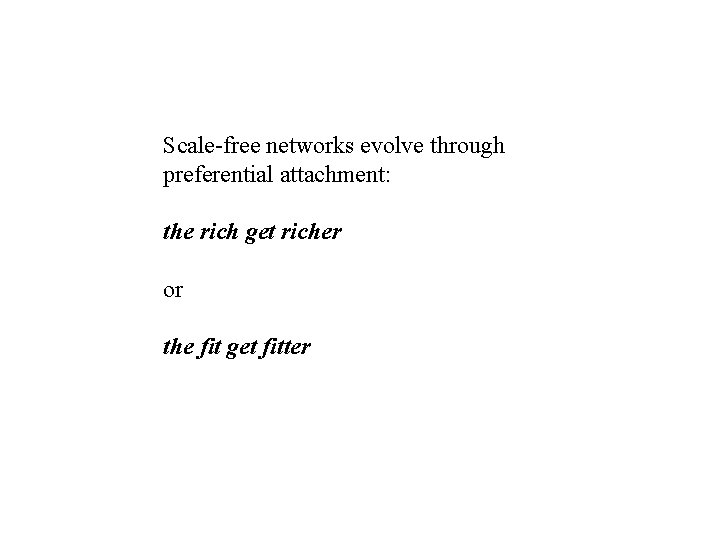
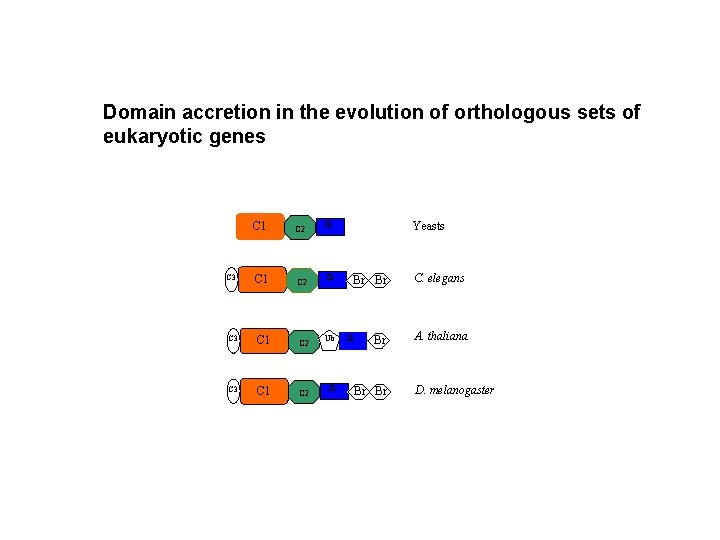
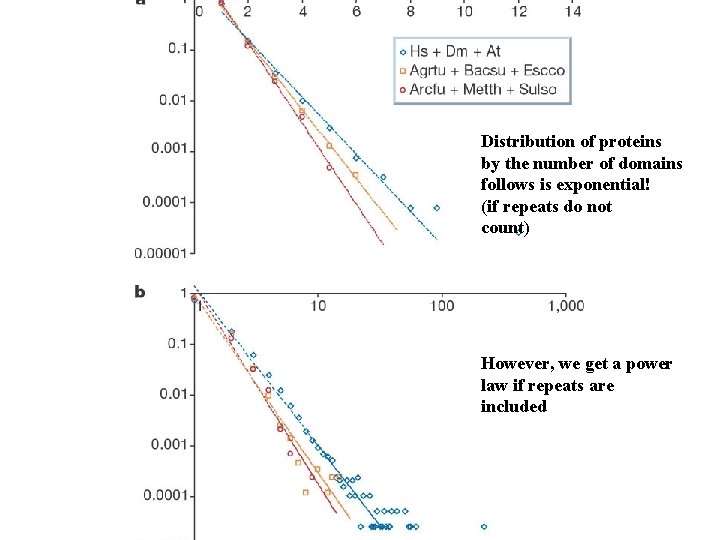
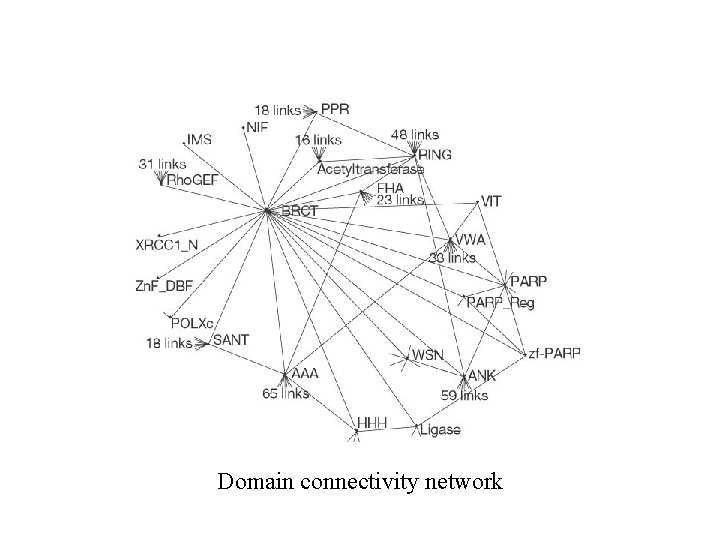
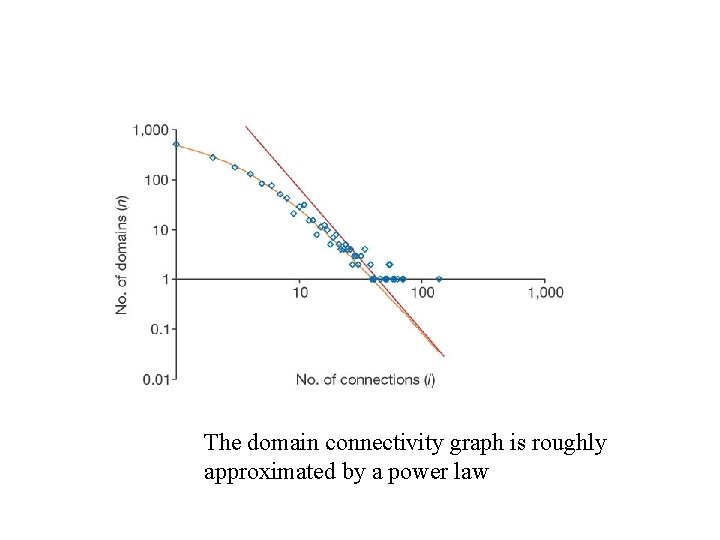
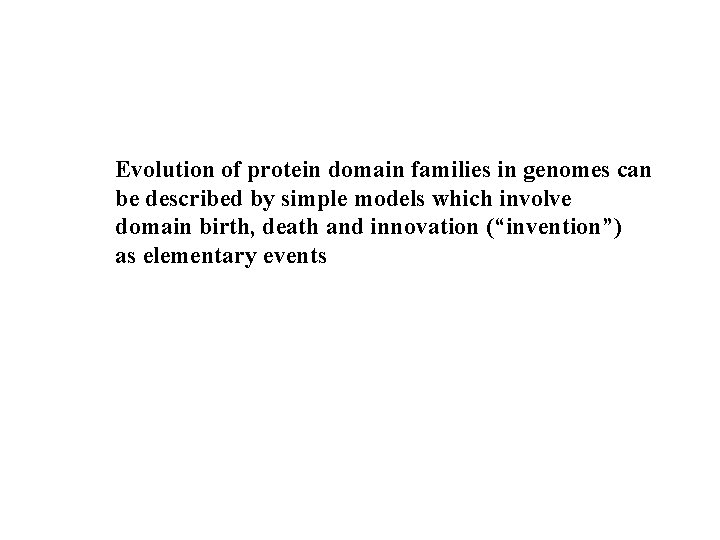
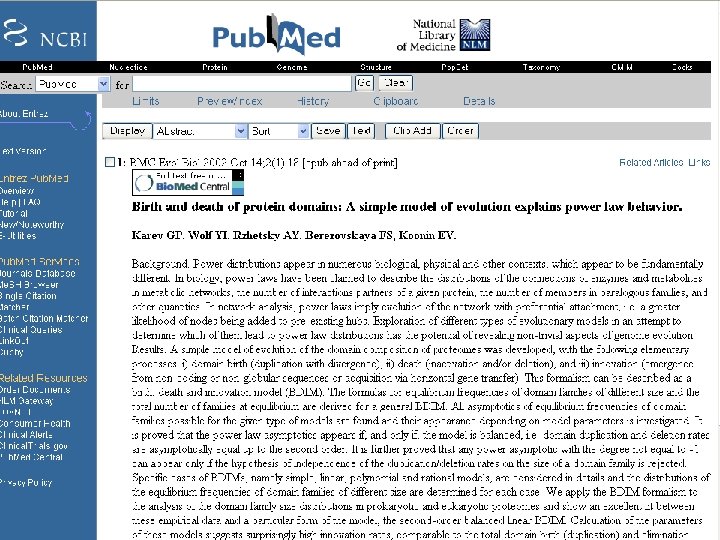
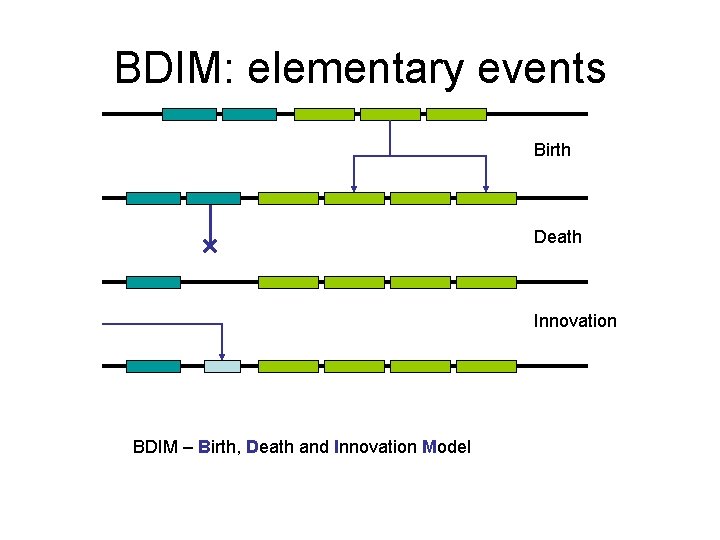
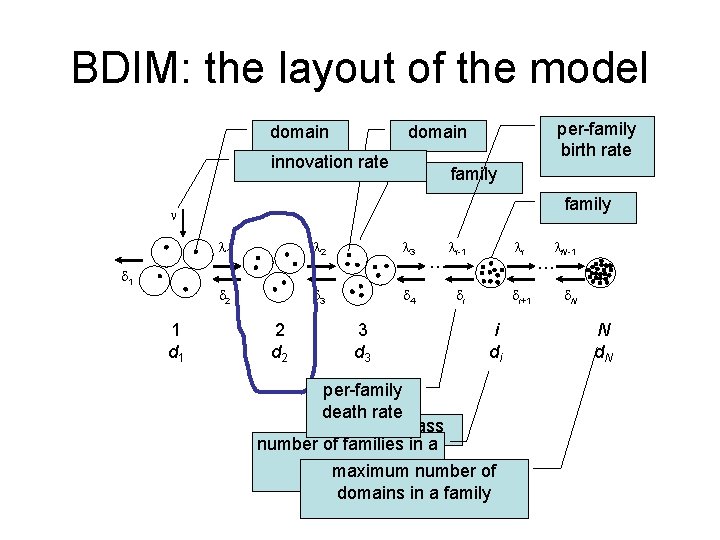
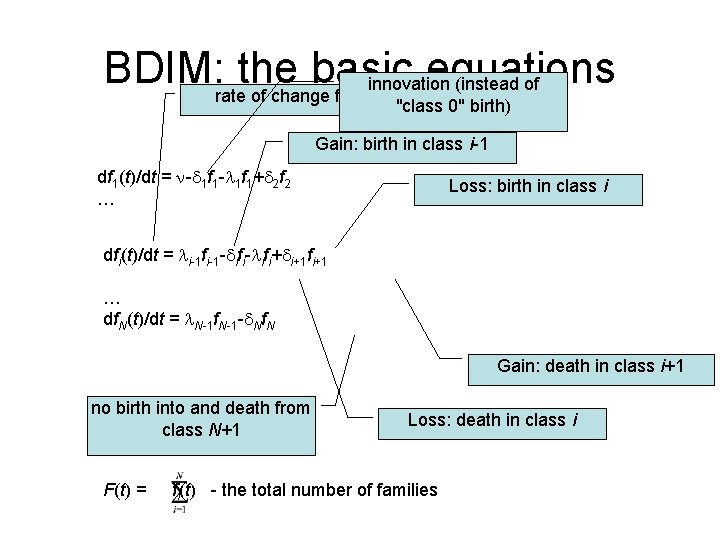
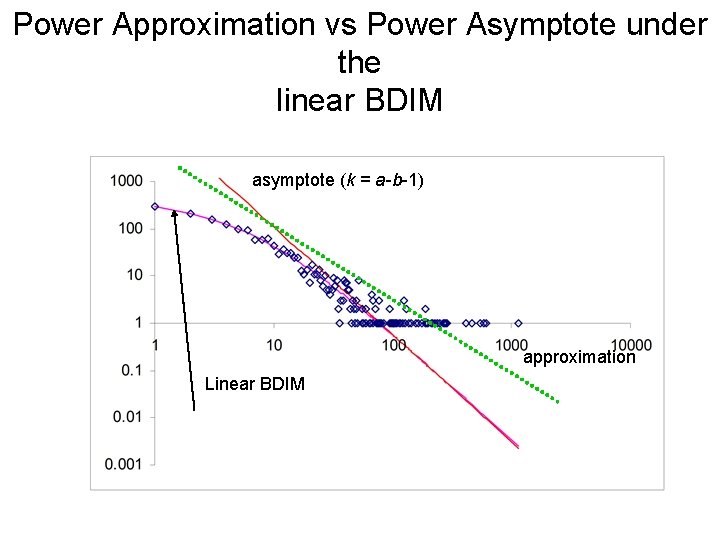
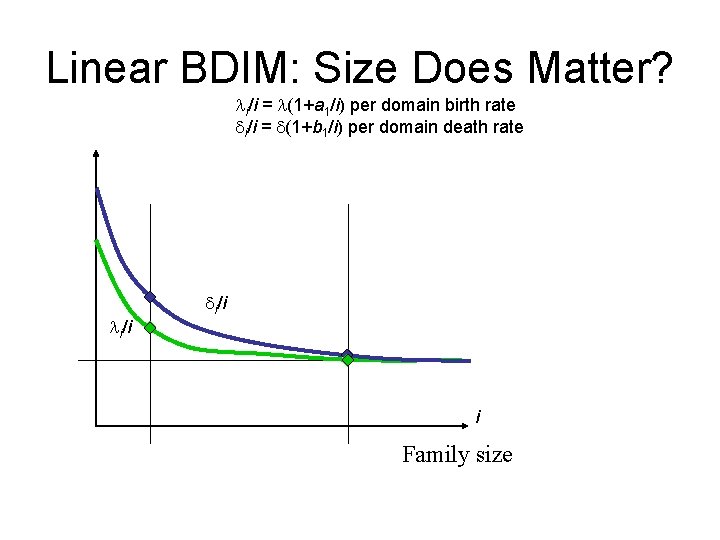
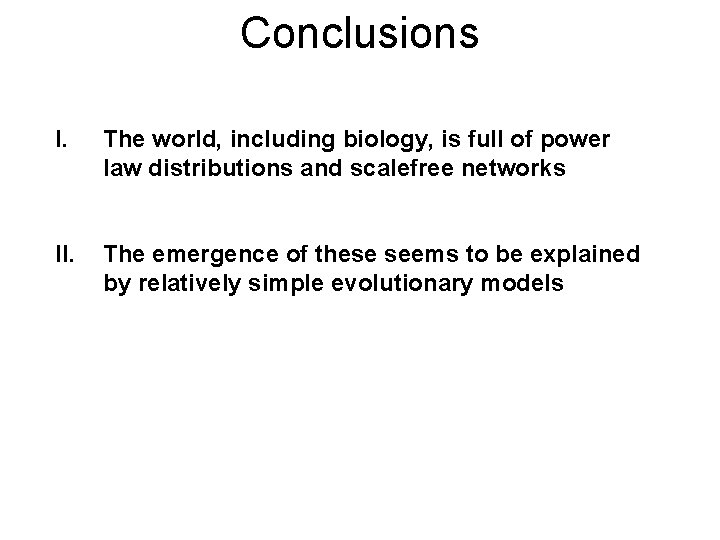
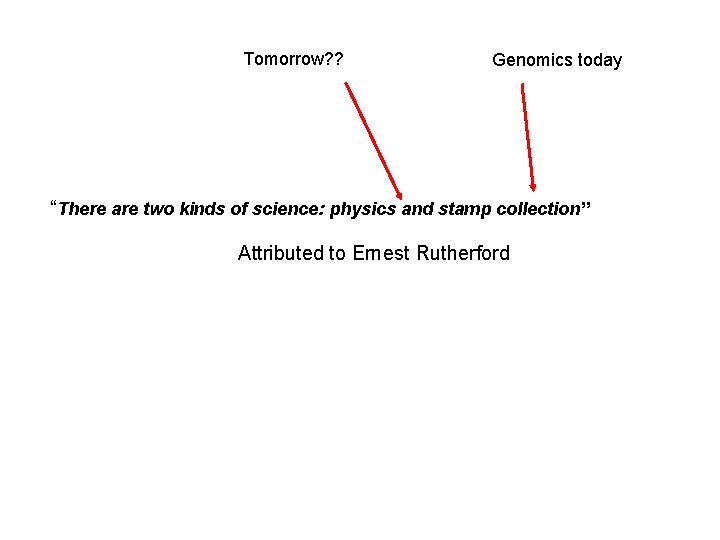
- Slides: 34
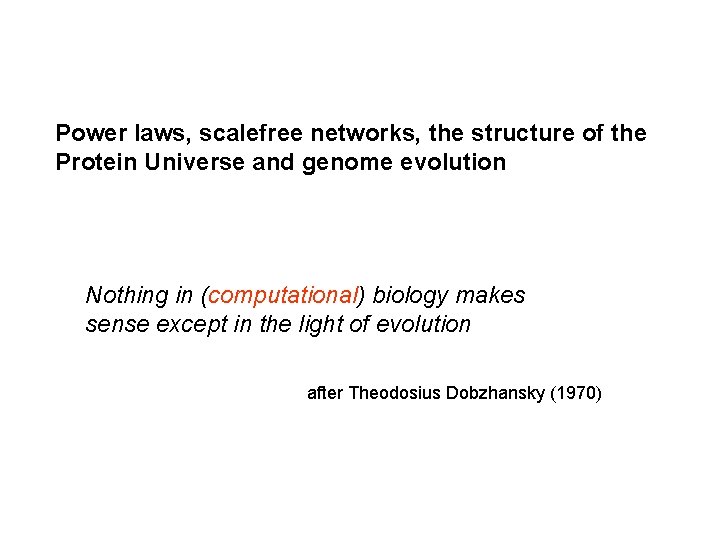
Power laws, scalefree networks, the structure of the Protein Universe and genome evolution Nothing in (computational) biology makes sense except in the light of evolution after Theodosius Dobzhansky (1970)
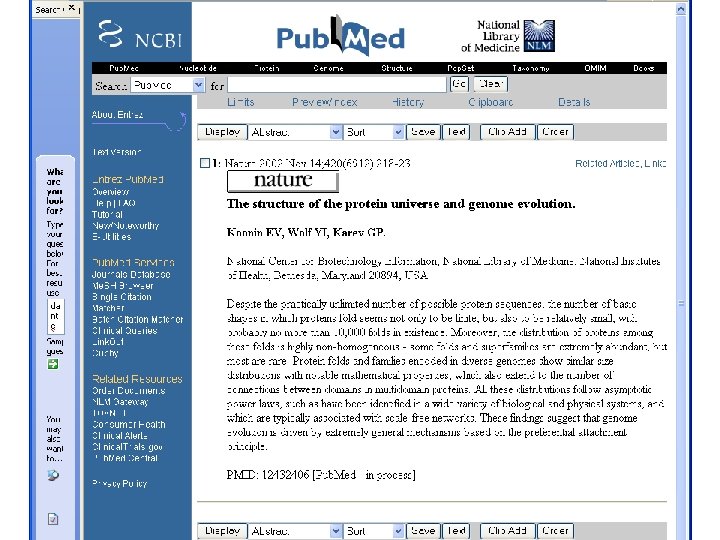
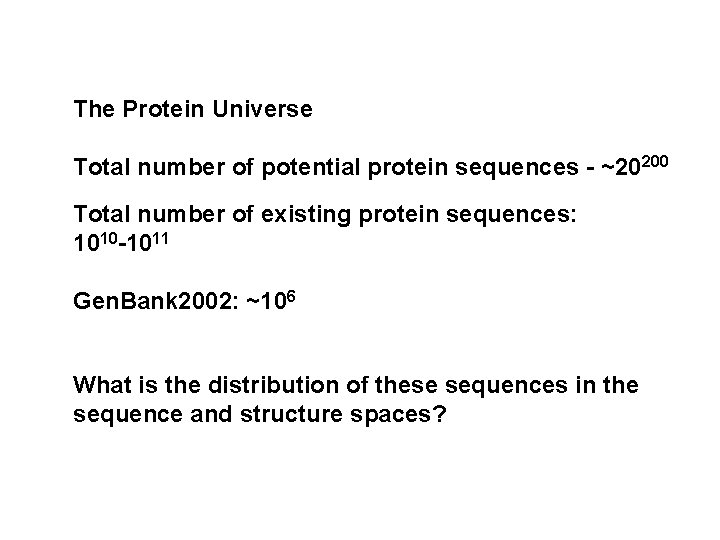
The Protein Universe Total number of potential protein sequences - ~20200 Total number of existing protein sequences: 1010 -1011 Gen. Bank 2002: ~106 What is the distribution of these sequences in the sequence and structure spaces?
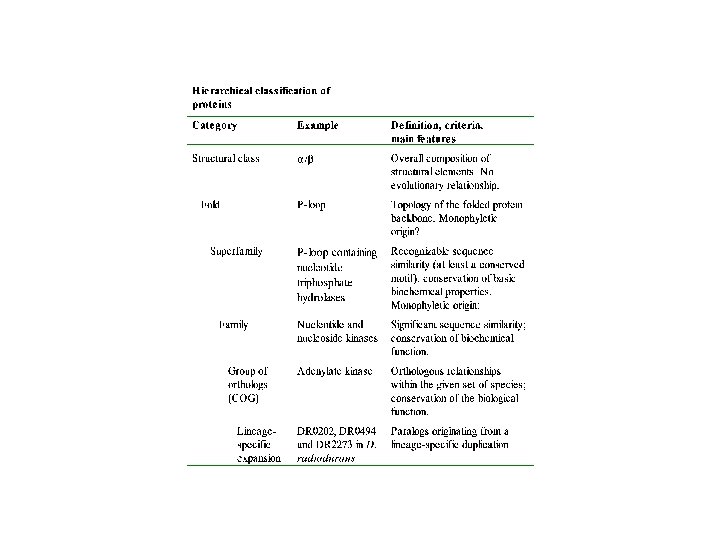
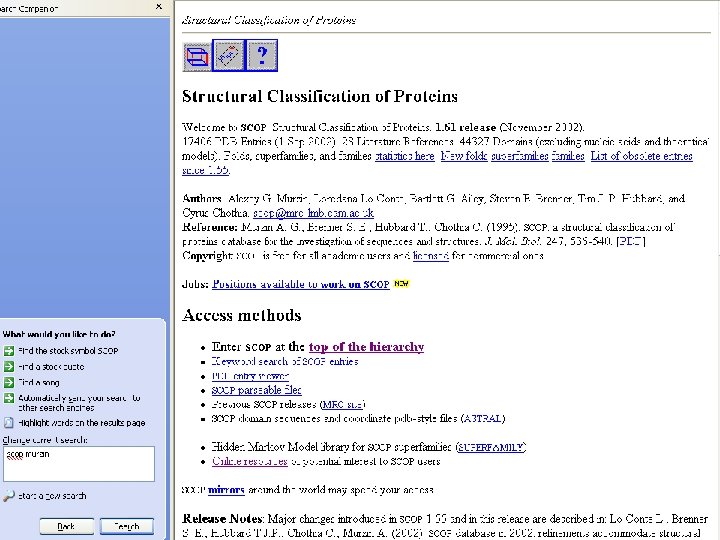
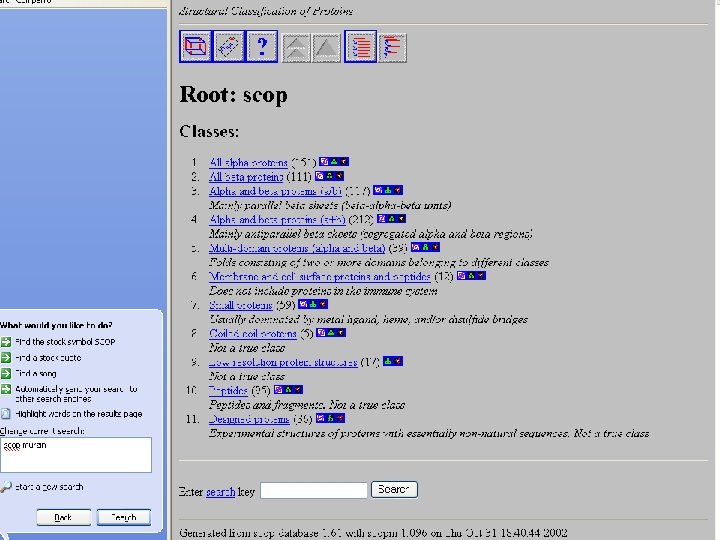
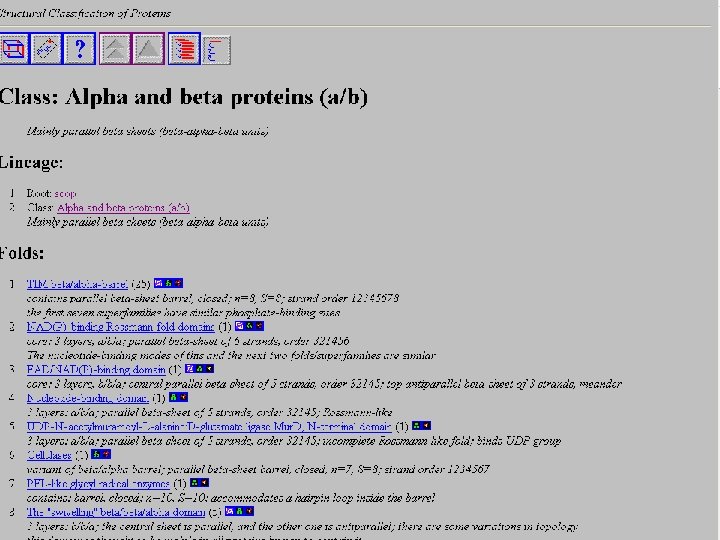
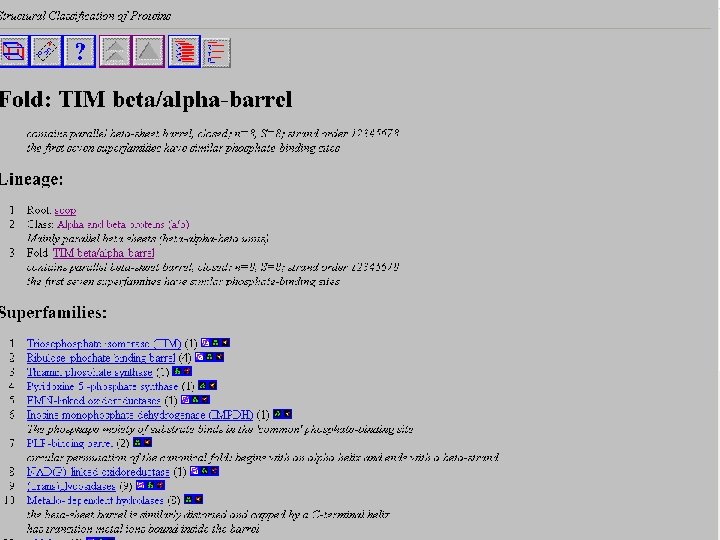
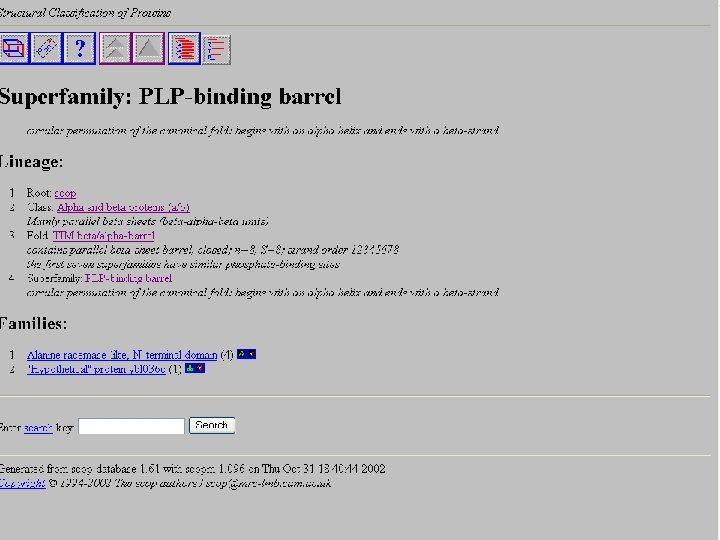
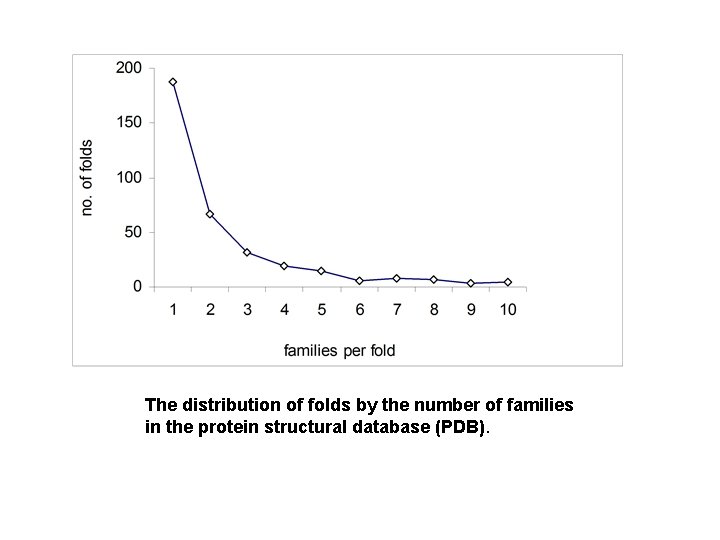
The distribution of folds by the number of families in the protein structural database (PDB).
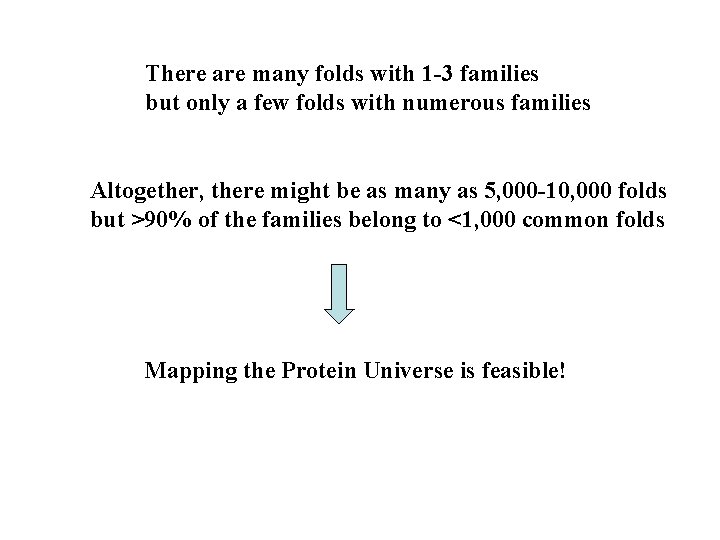
There are many folds with 1 -3 families but only a few folds with numerous families Altogether, there might be as many as 5, 000 -10, 000 folds but >90% of the families belong to <1, 000 common folds Mapping the Protein Universe is feasible!
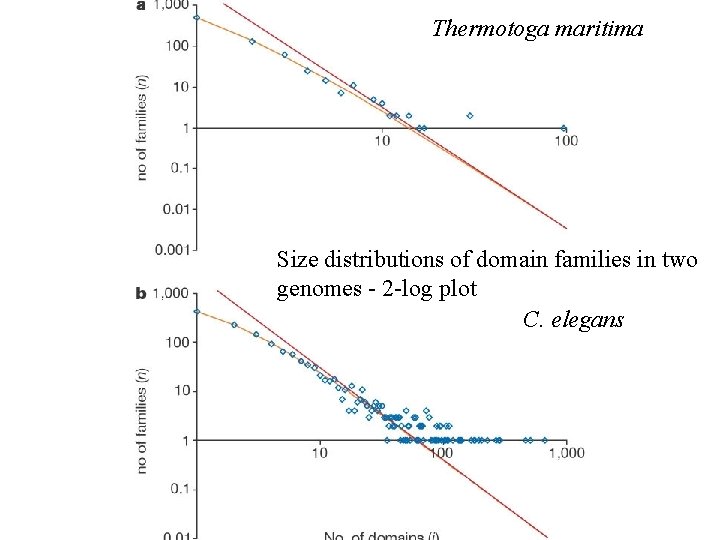
Thermotoga maritima Size distributions of domain families in two genomes - 2 -log plot C. elegans
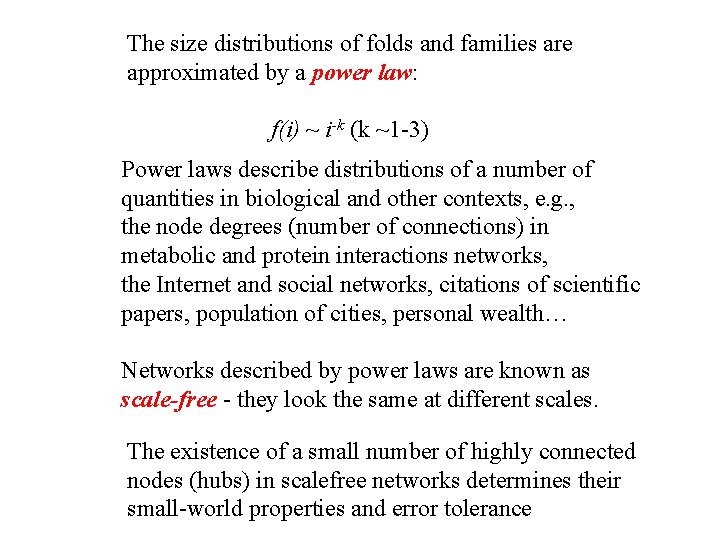
The size distributions of folds and families are approximated by a power law: f(i) ~ i-k (k ~1 -3) Power laws describe distributions of a number of quantities in biological and other contexts, e. g. , the node degrees (number of connections) in metabolic and protein interactions networks, the Internet and social networks, citations of scientific papers, population of cities, personal wealth… Networks described by power laws are known as scale-free - they look the same at different scales. The existence of a small number of highly connected nodes (hubs) in scalefree networks determines their small-world properties and error tolerance
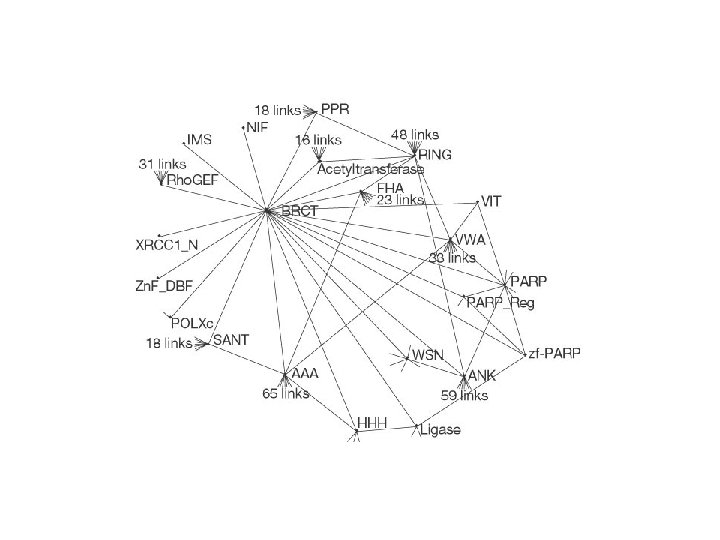
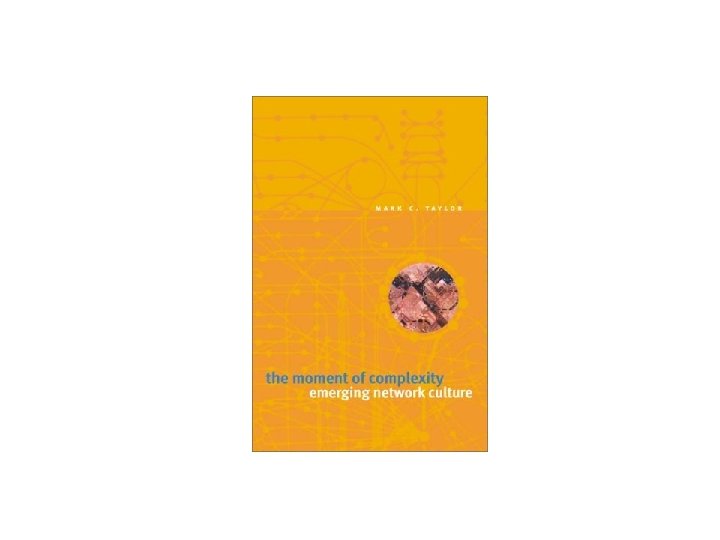
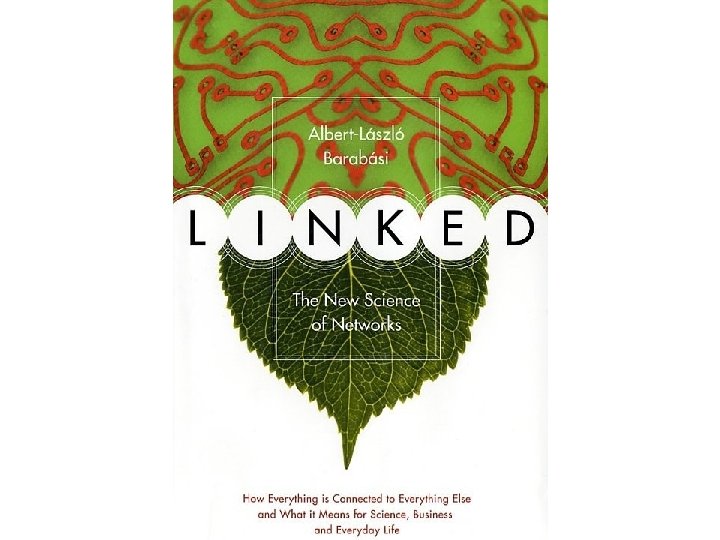
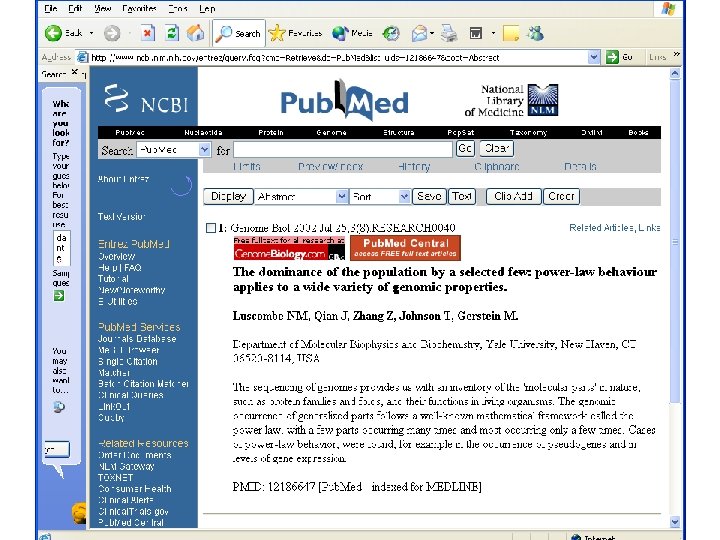
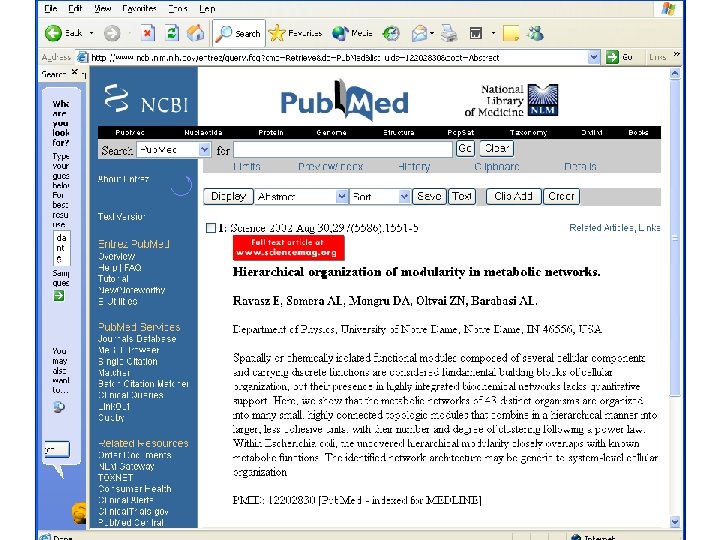
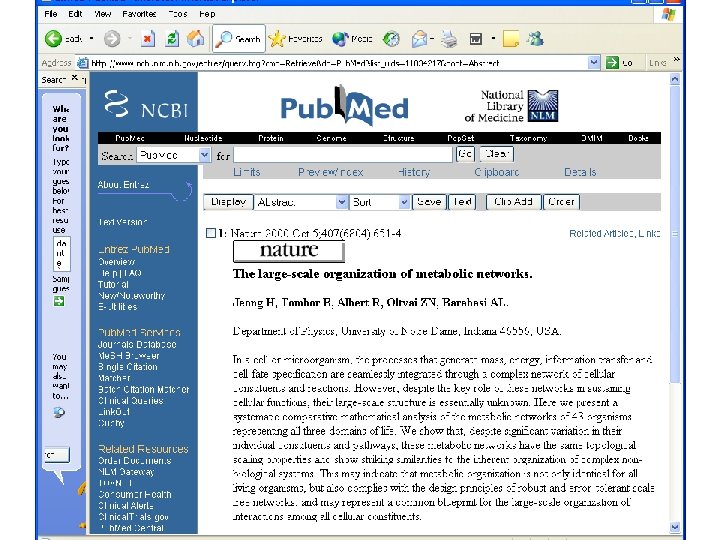
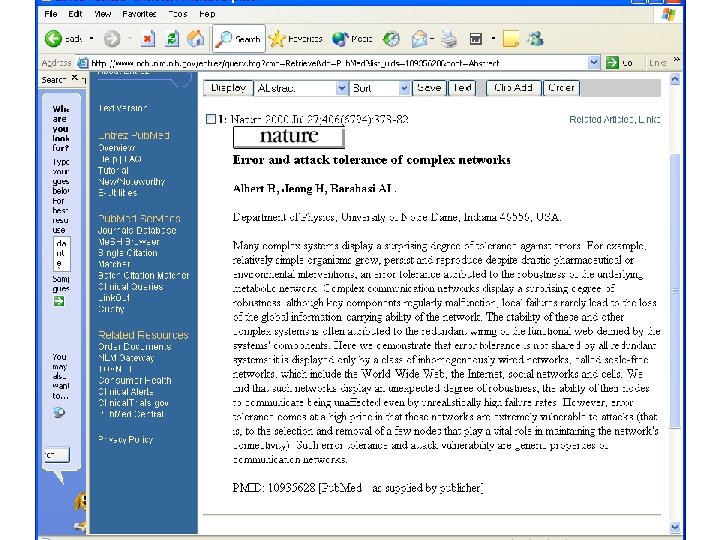
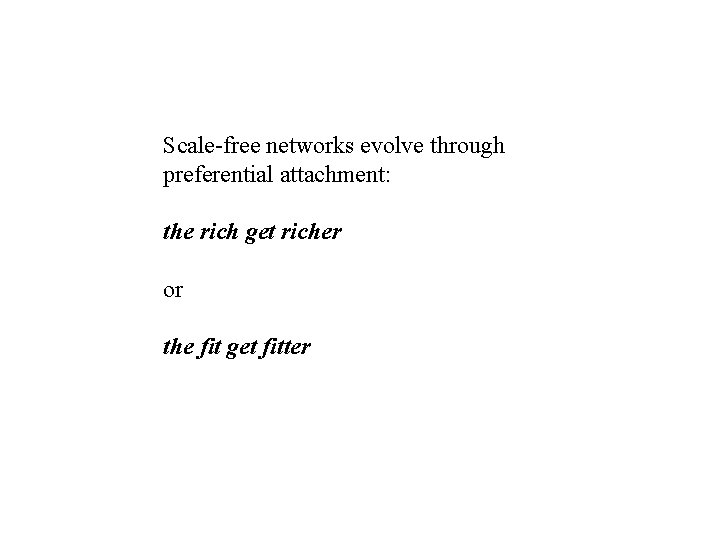
Scale-free networks evolve through preferential attachment: the rich get richer or the fit get fitter
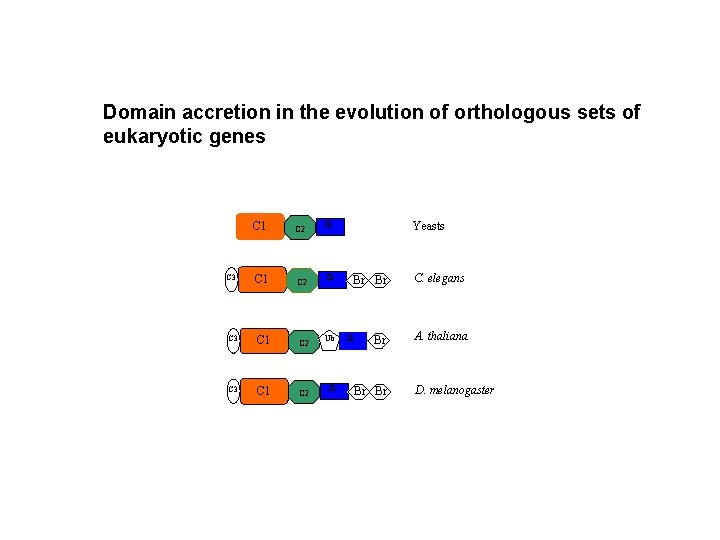
Domain accretion in the evolution of orthologous sets of eukaryotic genes C 1 C 2 Zk C 3 C 1 C 2 Ub C 3 C 1 C 2 Zk Yeasts Br Br Zk Br Br Br C. elegans A. thaliana D. melanogaster
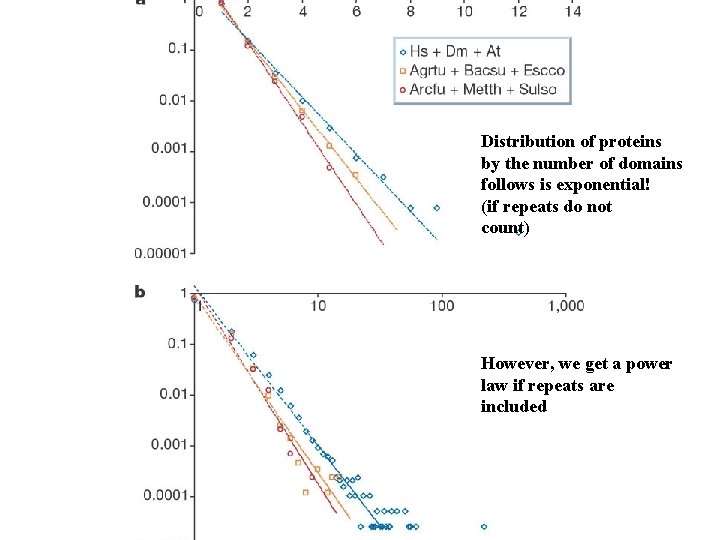
Distribution of proteins by the number of domains follows is exponential! (if repeats do not count) However, we get a power law if repeats are included
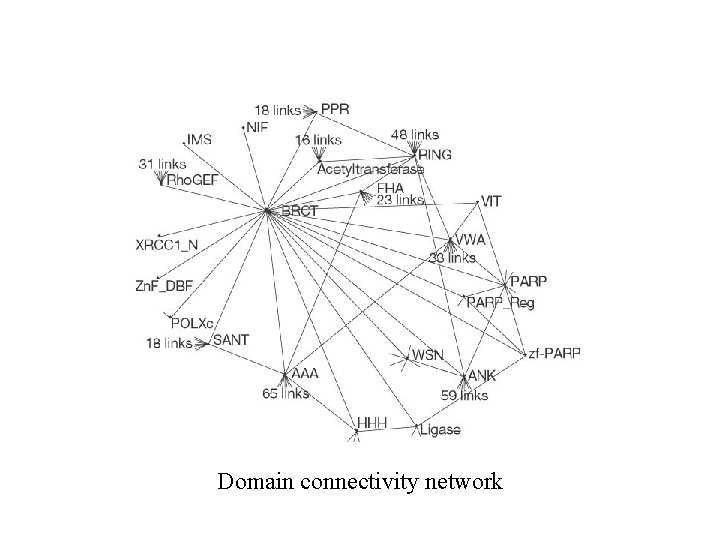
Domain connectivity network
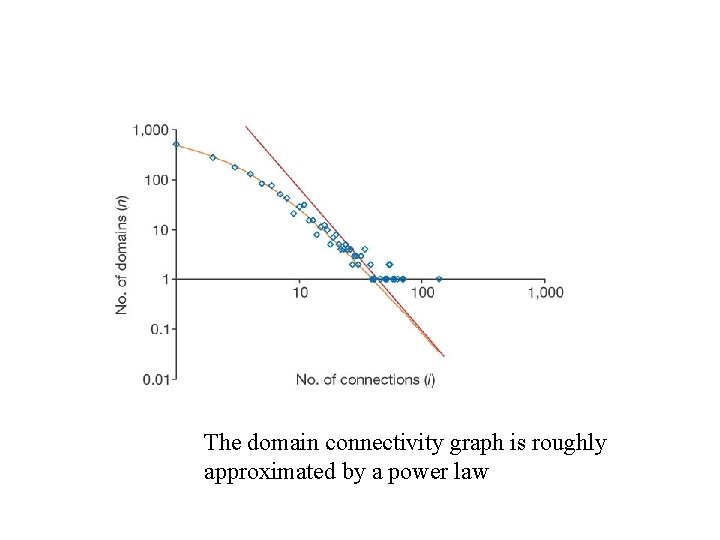
The domain connectivity graph is roughly approximated by a power law
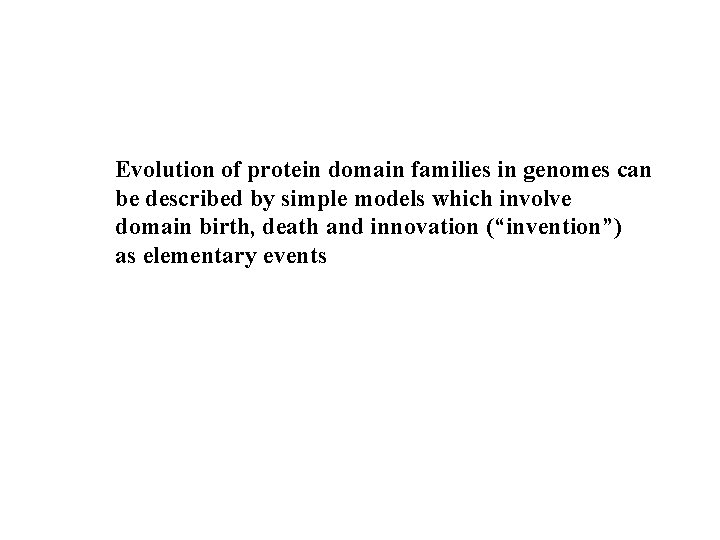
Evolution of protein domain families in genomes can be described by simple models which involve domain birth, death and innovation (“invention”) as elementary events
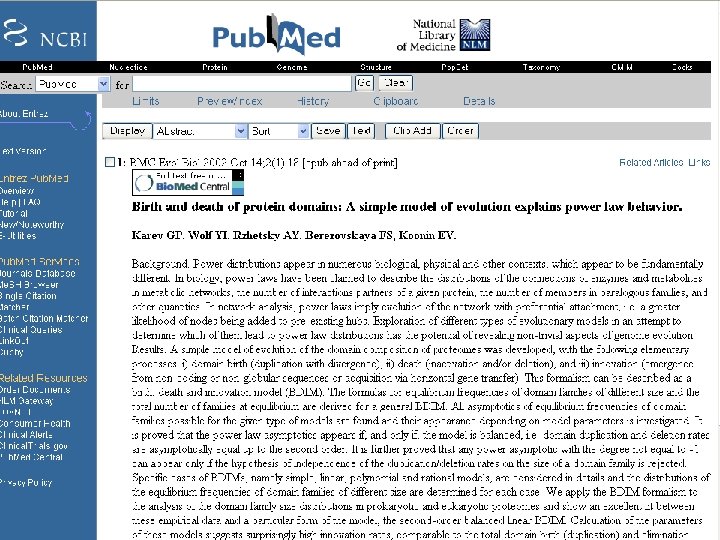
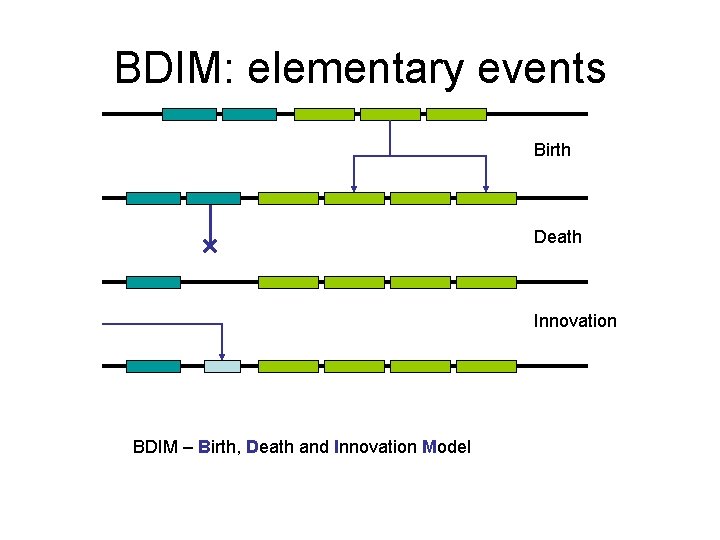
BDIM: elementary events Birth Death Innovation BDIM – Birth, Death and Innovation Model
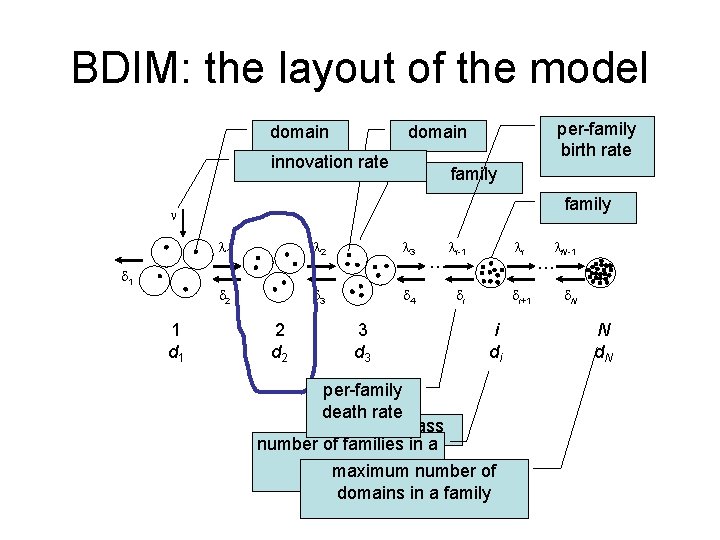
BDIM: the layout of the model domain per-family birth rate domain innovation rate family 1 1 d 1 1 2 3 4 2 d 2 3 d 3 … i-1 i i i+1 i di per-family death rate size class number of families in a size class maximum number of domains in a family N-1 … N N d. N
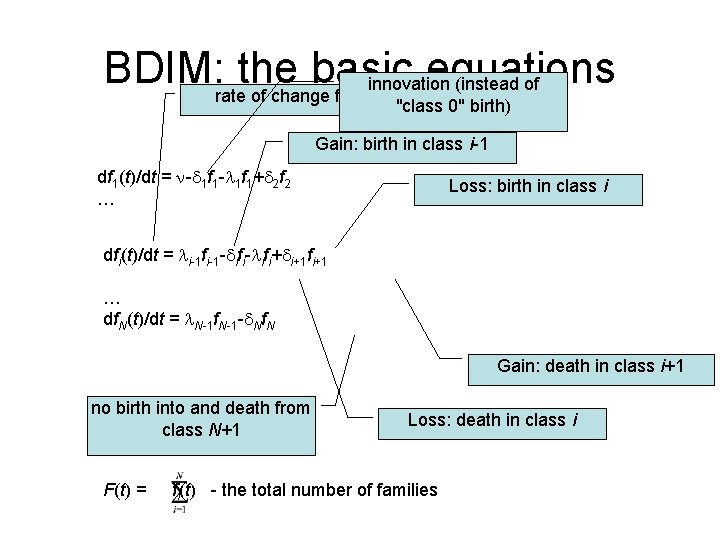
BDIM: ratethe basic equations innovation (instead of of change for d i "class 0" birth) Gain: birth in class i-1 df 1(t)/dt = - 1 f 1+ 2 f 2 … Loss: birth in class i dfi(t)/dt = i-1 fi-1 - ifi+ i+1 fi+1 … df. N(t)/dt = N-1 f. N-1 - Nf. N Gain: death in class i+1 no birth into and death from class N+1 F(t) = Loss: death in class i fi(t) - the total number of families
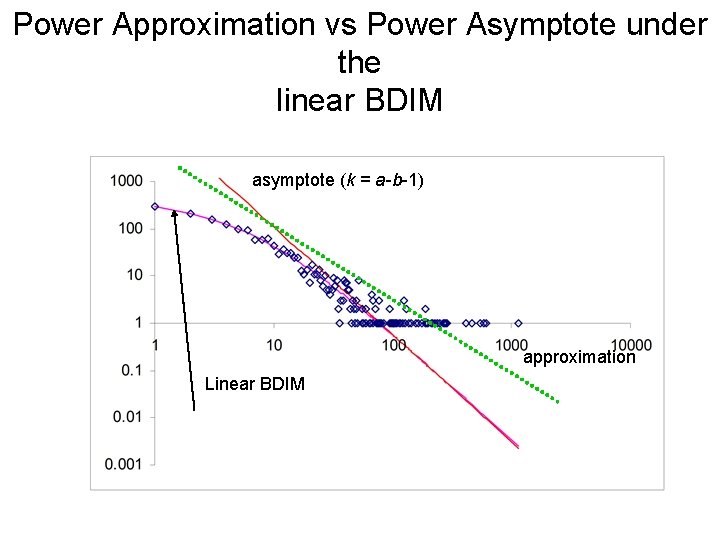
Power Approximation vs Power Asymptote under the linear BDIM asymptote (k = a-b-1) approximation Linear BDIM
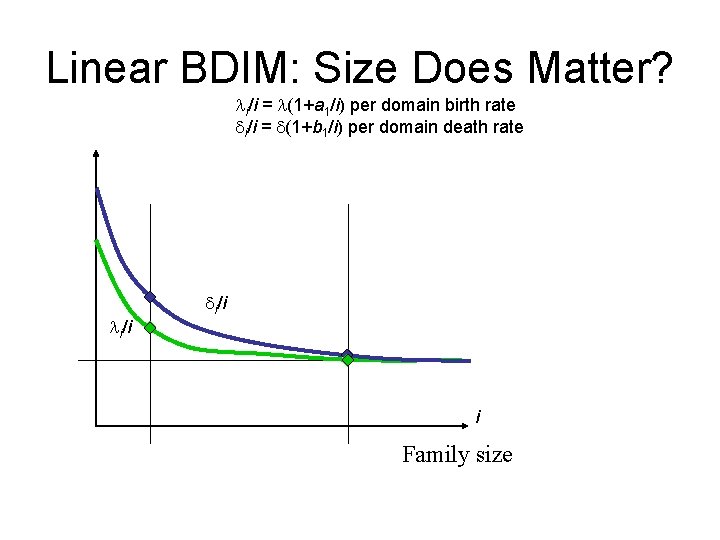
Linear BDIM: Size Does Matter? i/i = (1+a 1/i) per domain birth rate i/i = (1+b 1/i) per domain death rate i/i i Family size
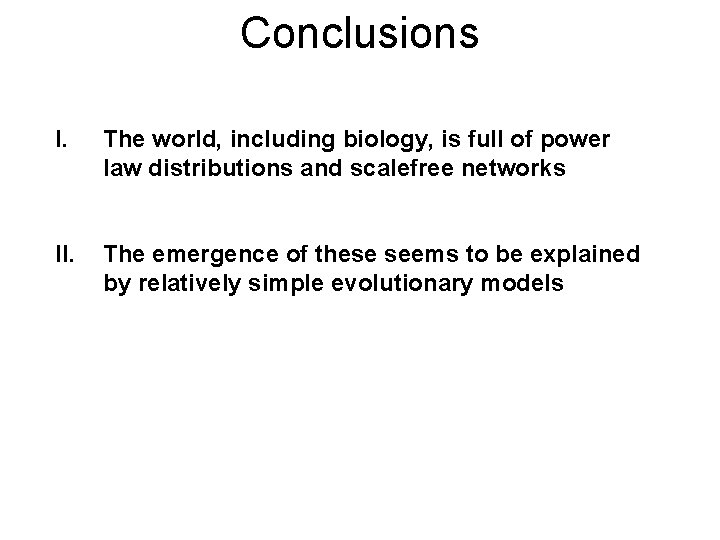
Conclusions I. The world, including biology, is full of power law distributions and scalefree networks II. The emergence of these seems to be explained by relatively simple evolutionary models
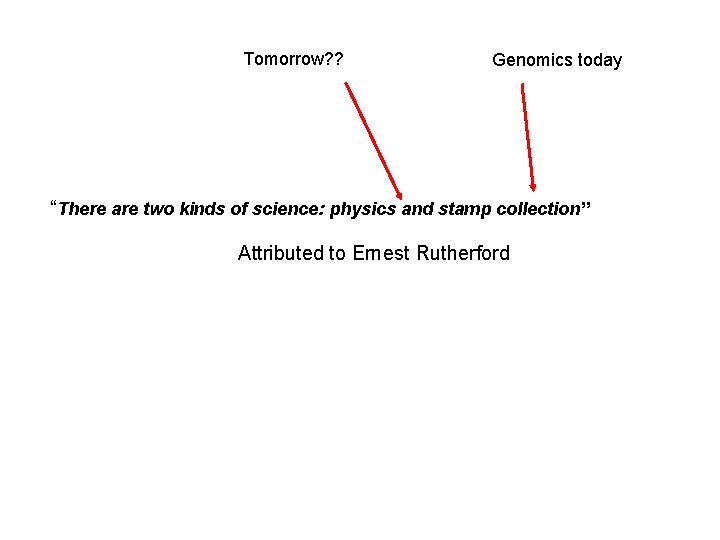
Tomorrow? ? Genomics today “There are two kinds of science: physics and stamp collection” Attributed to Ernest Rutherford
Difference between virtual circuit and datagram subnet
Basestore iptv
Useless laws weaken the necessary laws
Power triangle
Service and installation rules
Elements in traditional fantasy
Tier 3 isp
Internet structure network of networks
Finding community structure in very large networks
Community detection in networks
Solar power satellites and microwave power transmission
Actual power and potential power
Flex28024a
Dispersive power of grating is inversely proportional
Power of a power property
Chain rule範例
Power angle curve in power system stability
Informsu
Power absorbed or supplied
Hát kết hợp bộ gõ cơ thể
Bổ thể
Tỉ lệ cơ thể trẻ em
Gấu đi như thế nào
Tư thế worms-breton
Hát lên người ơi
Kể tên các môn thể thao
Thế nào là hệ số cao nhất
Các châu lục và đại dương trên thế giới
Công thức tiính động năng
Trời xanh đây là của chúng ta thể thơ
Cách giải mật thư tọa độ
Phép trừ bù
độ dài liên kết
Các châu lục và đại dương trên thế giới