Core Percolation in Scalefree Cross Layer Networks Nan
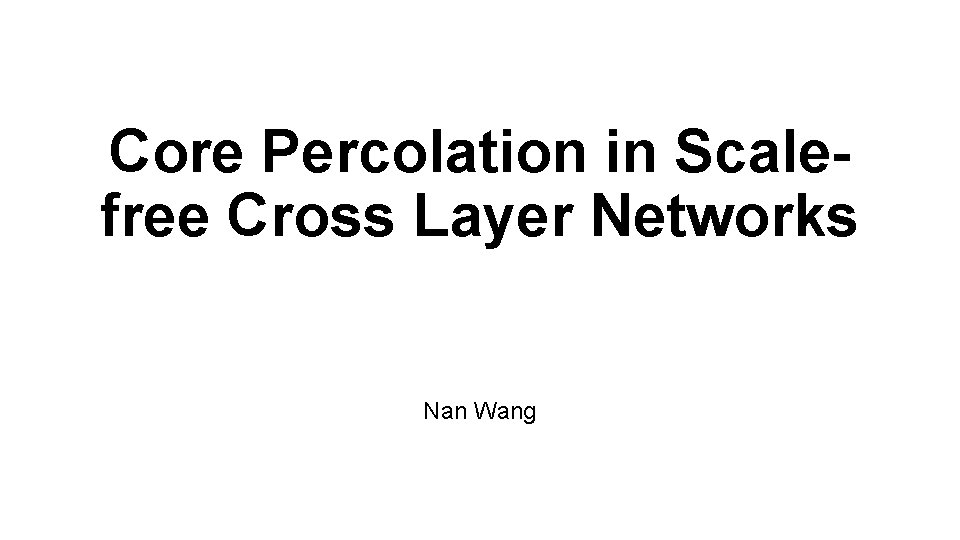
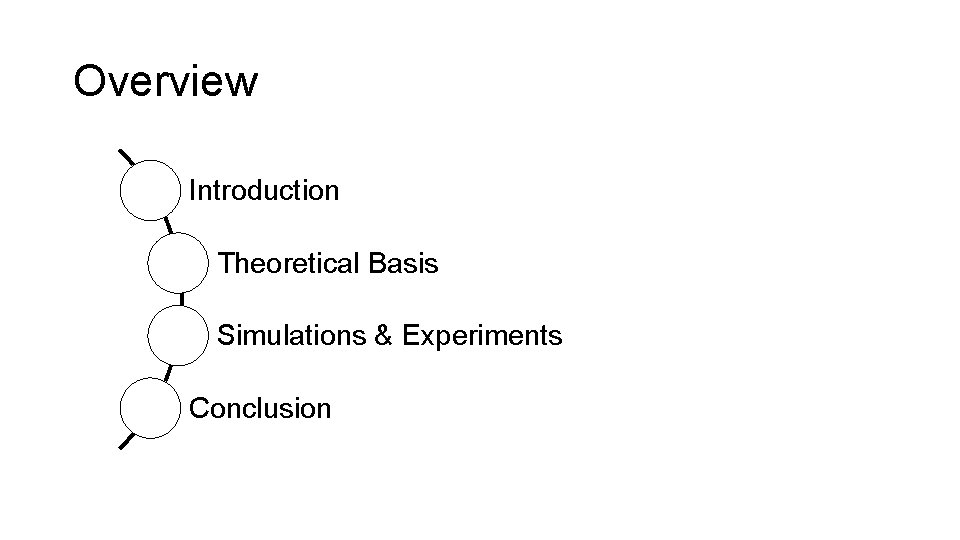
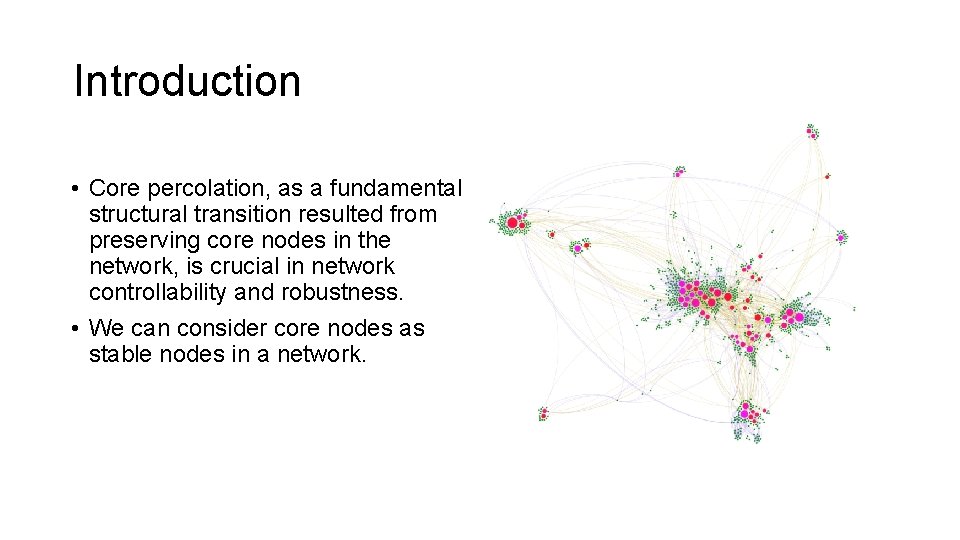
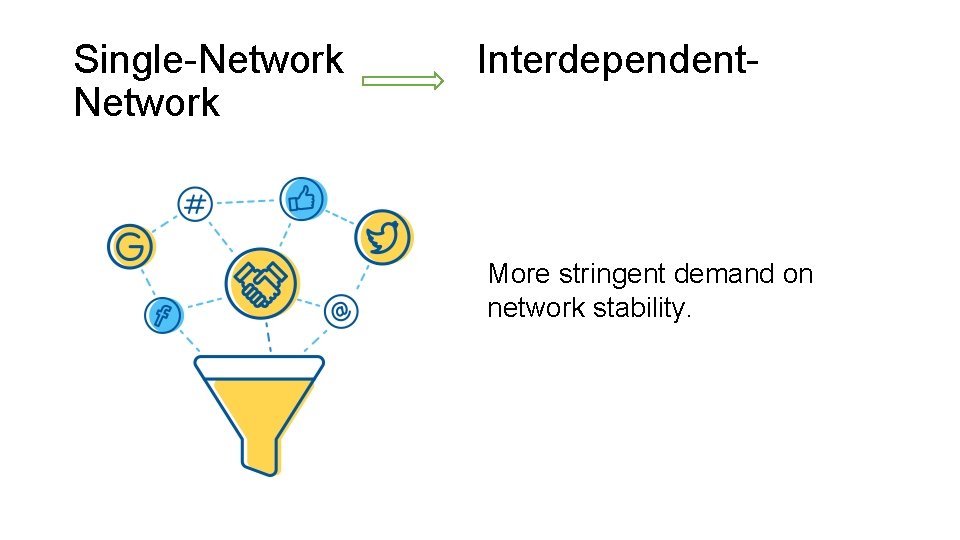
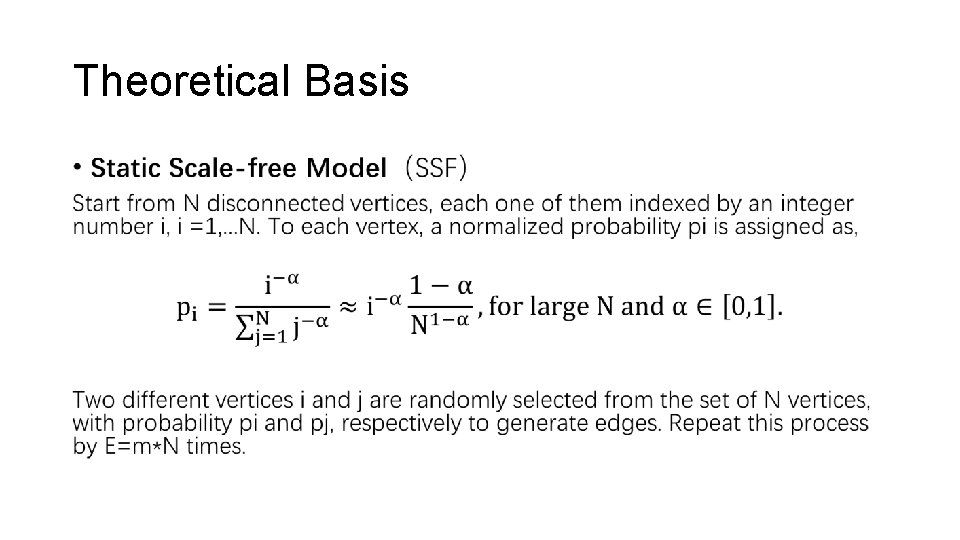
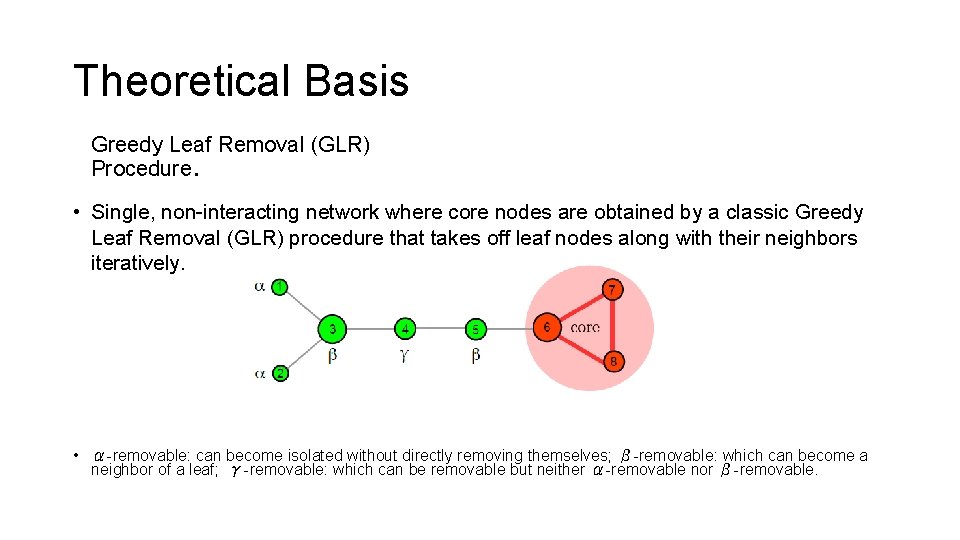
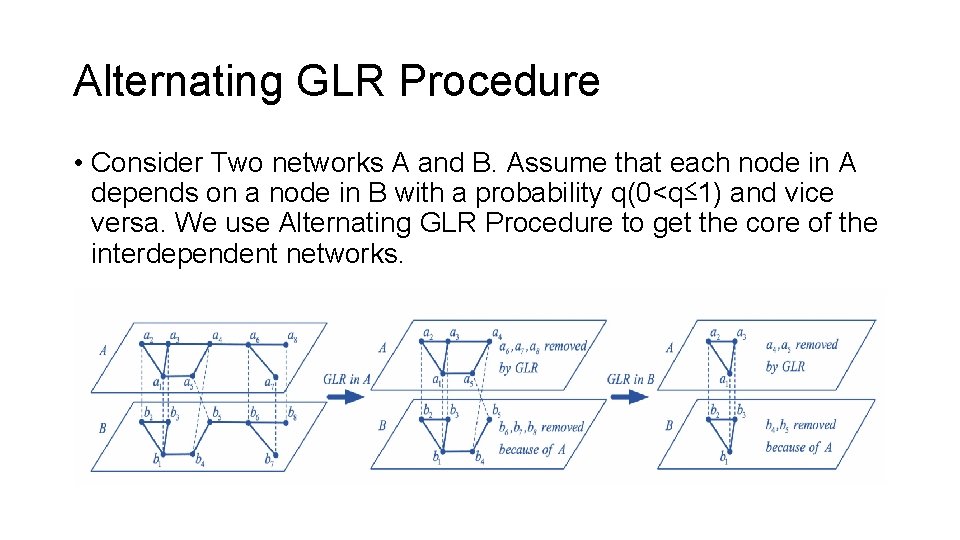
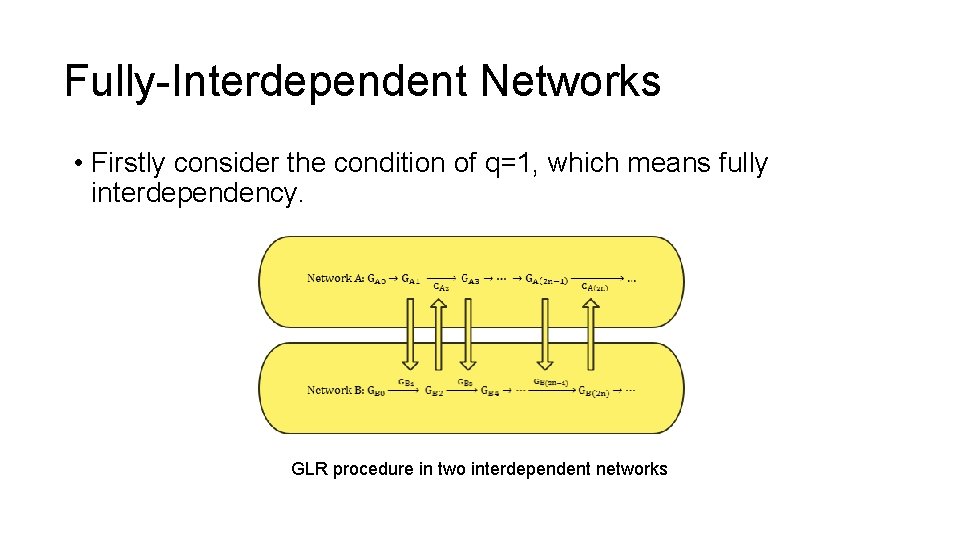
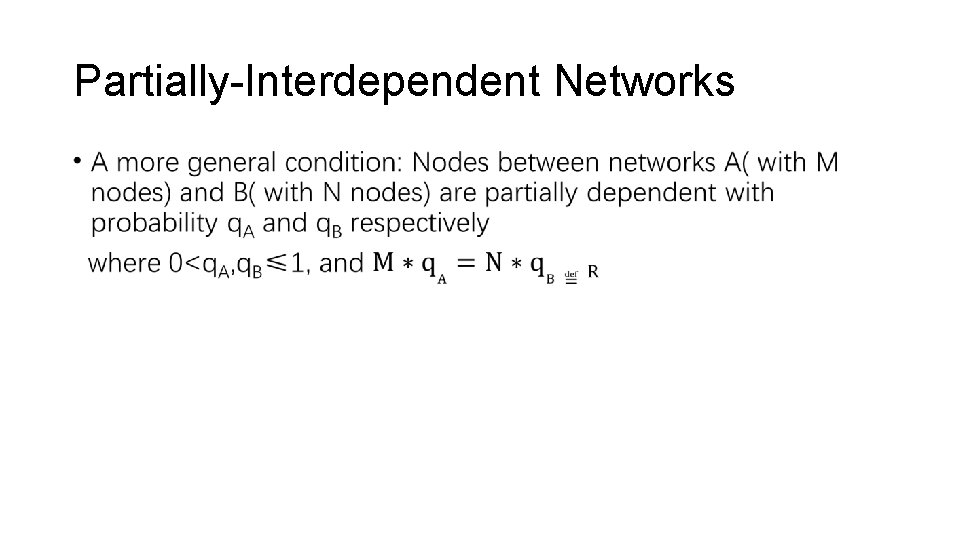
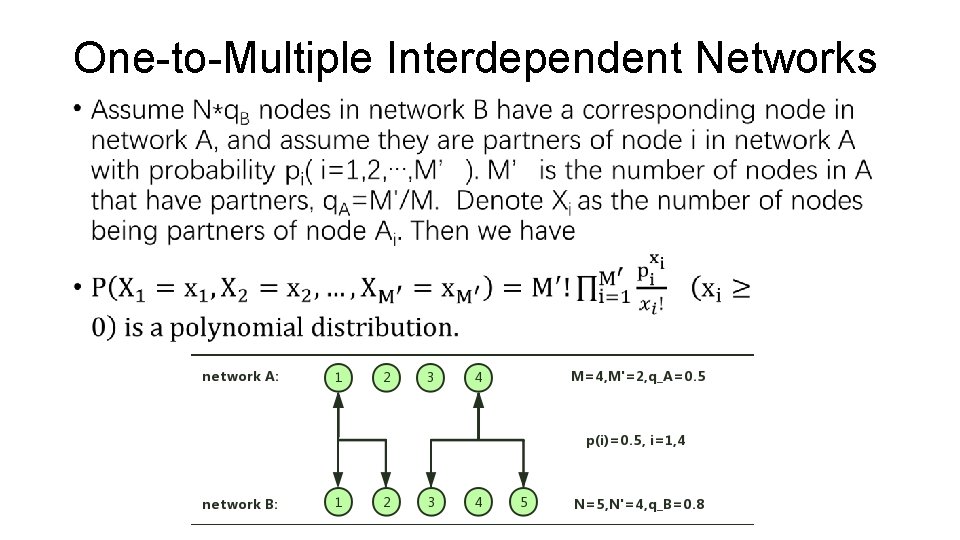
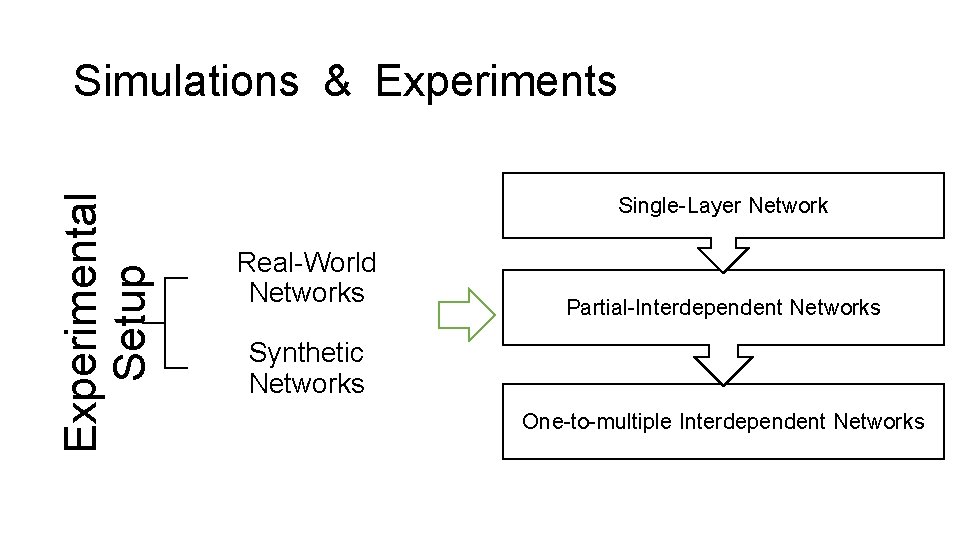
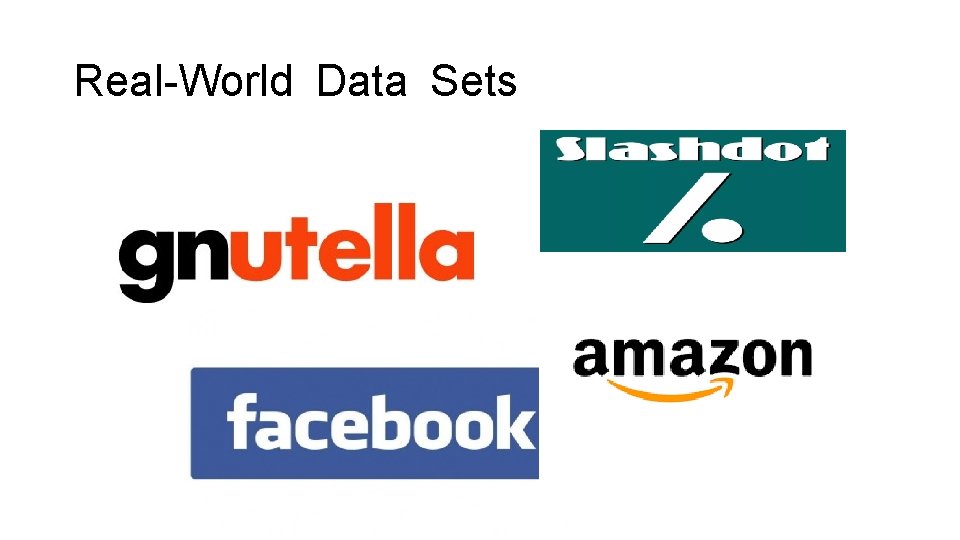
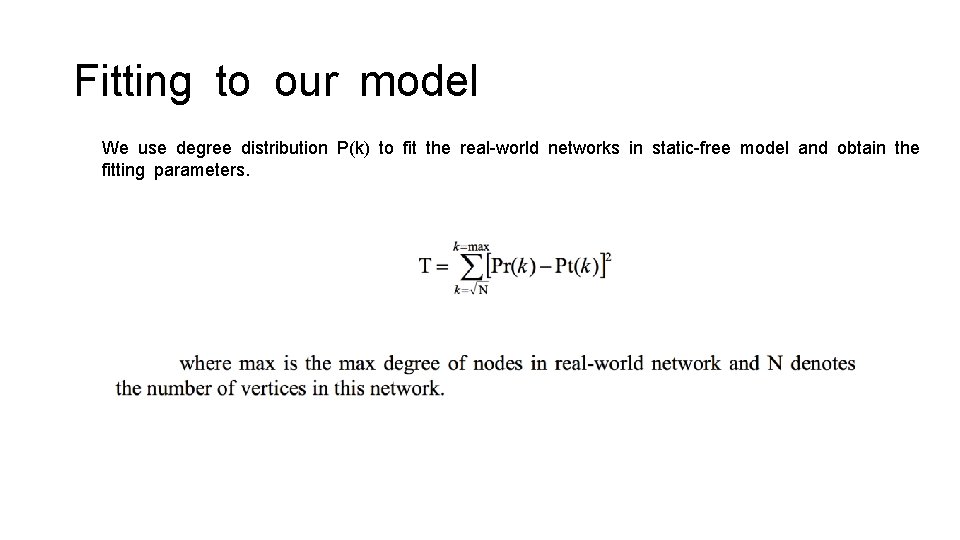
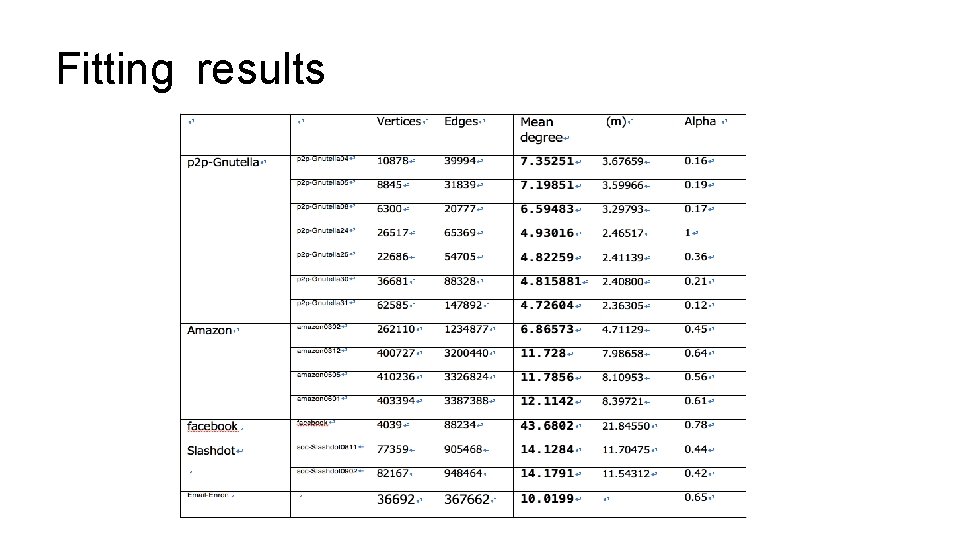
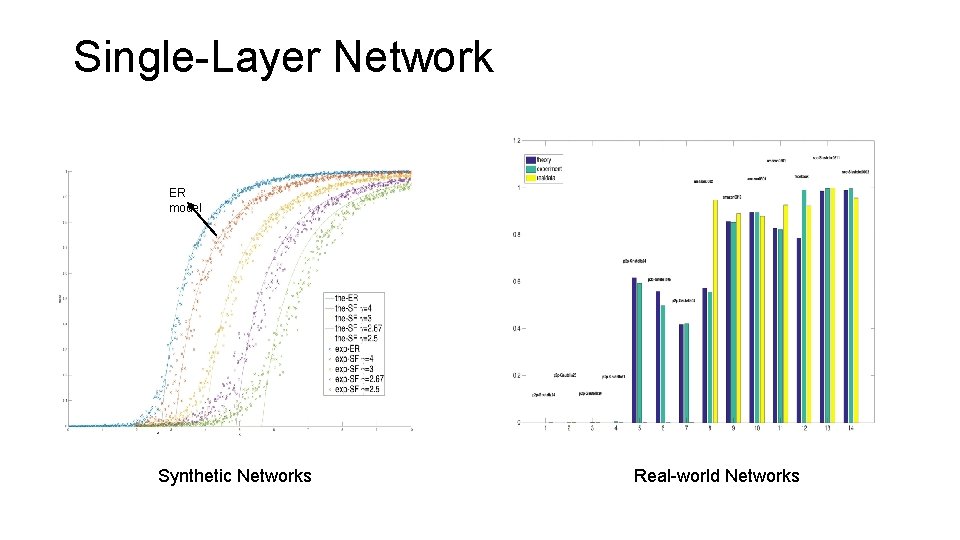
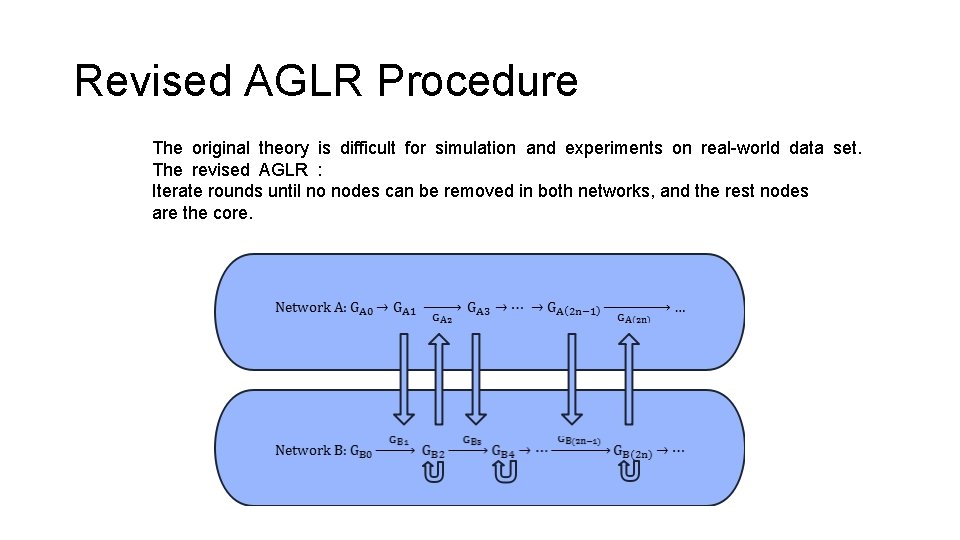
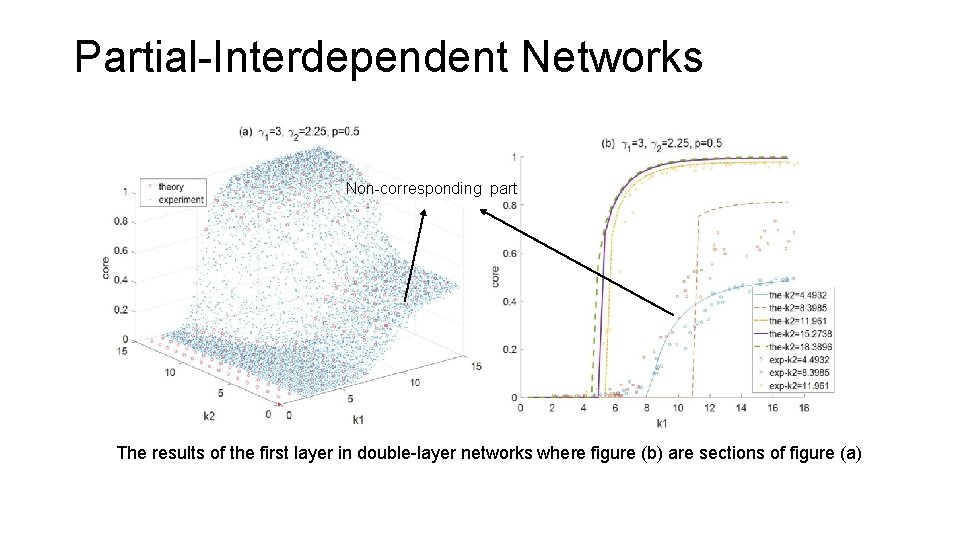
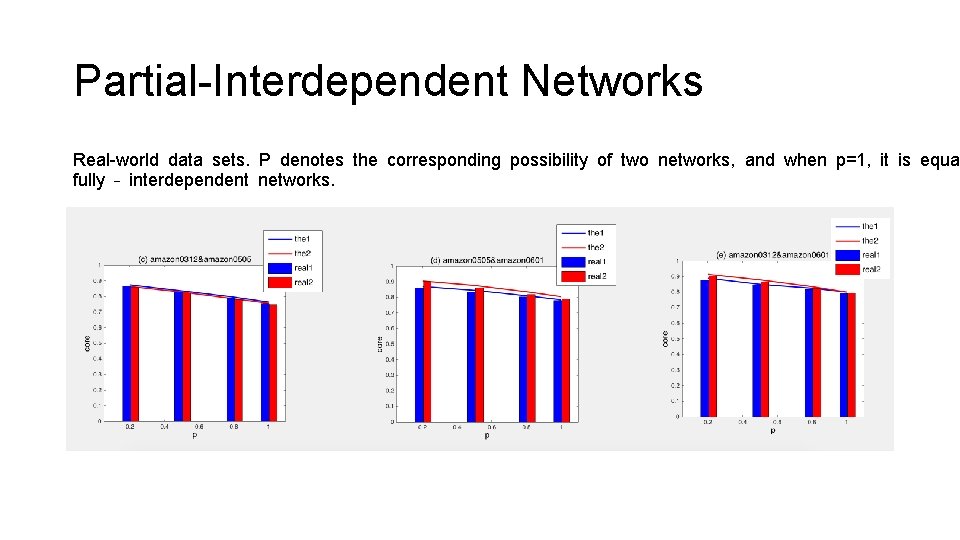
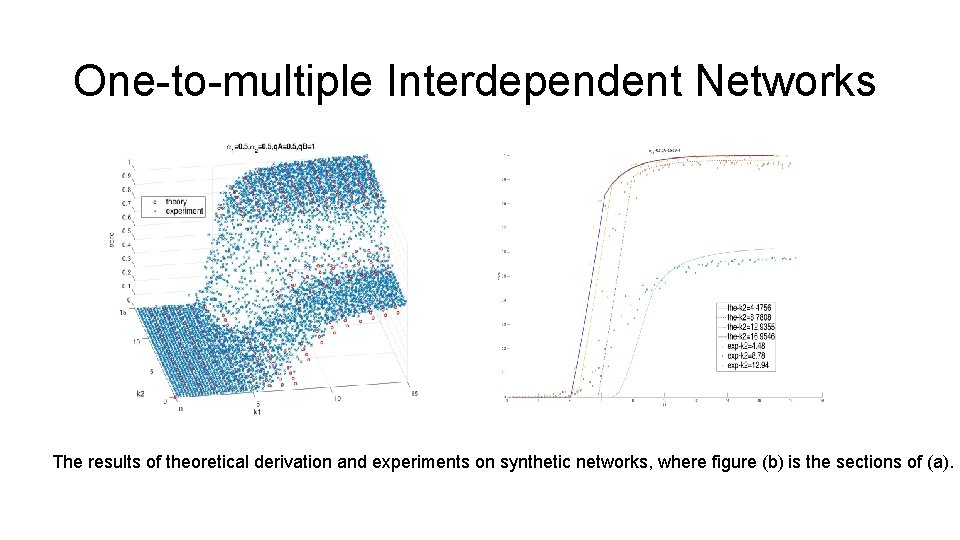
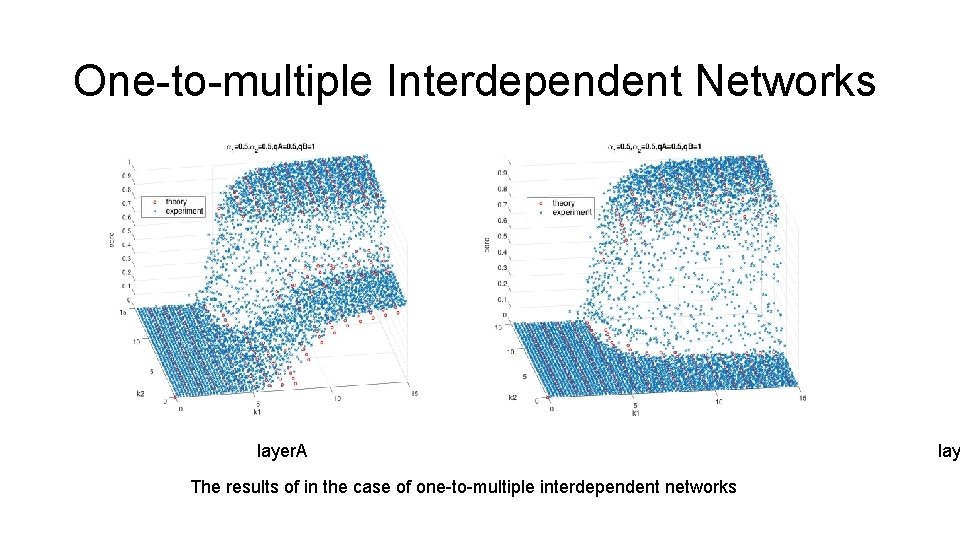
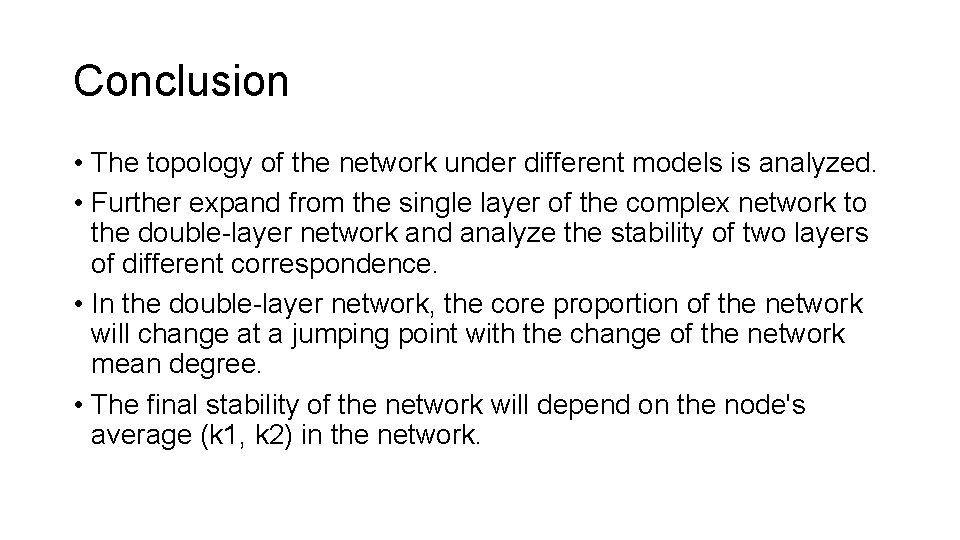
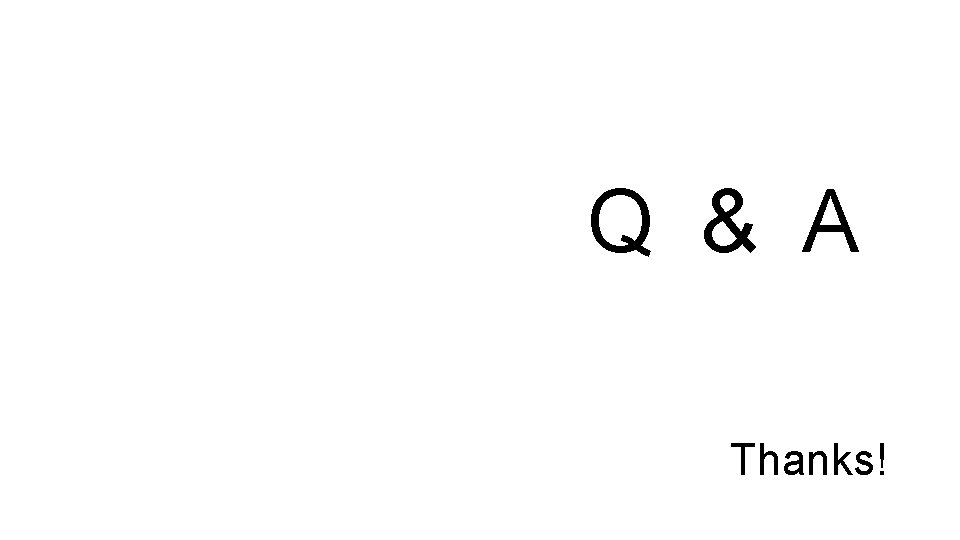
- Slides: 22
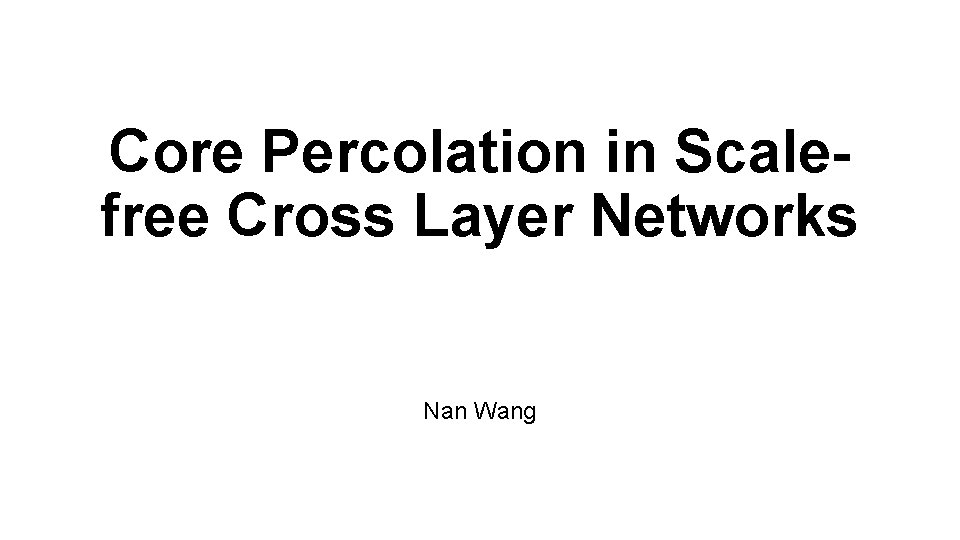
Core Percolation in Scalefree Cross Layer Networks Nan Wang
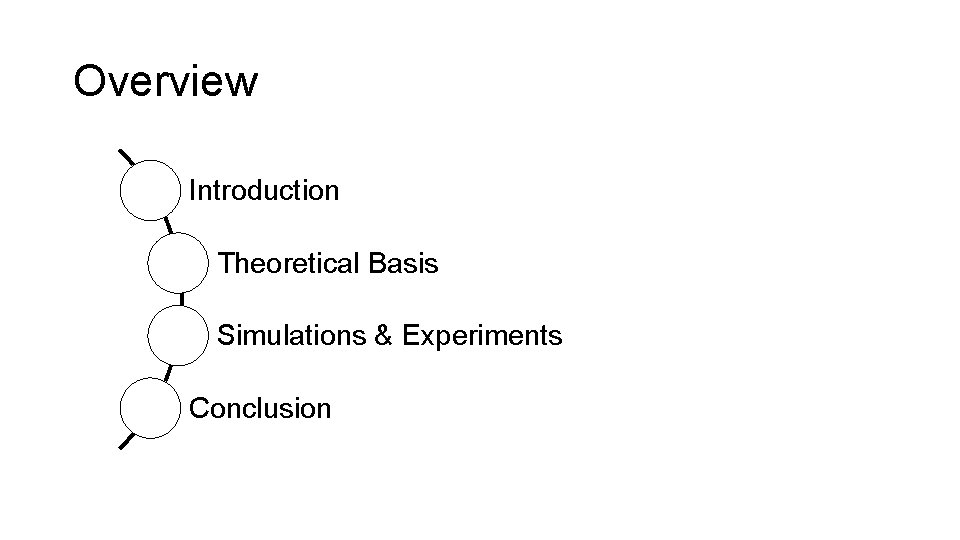
Overview Introduction Theoretical Basis Simulations & Experiments Conclusion
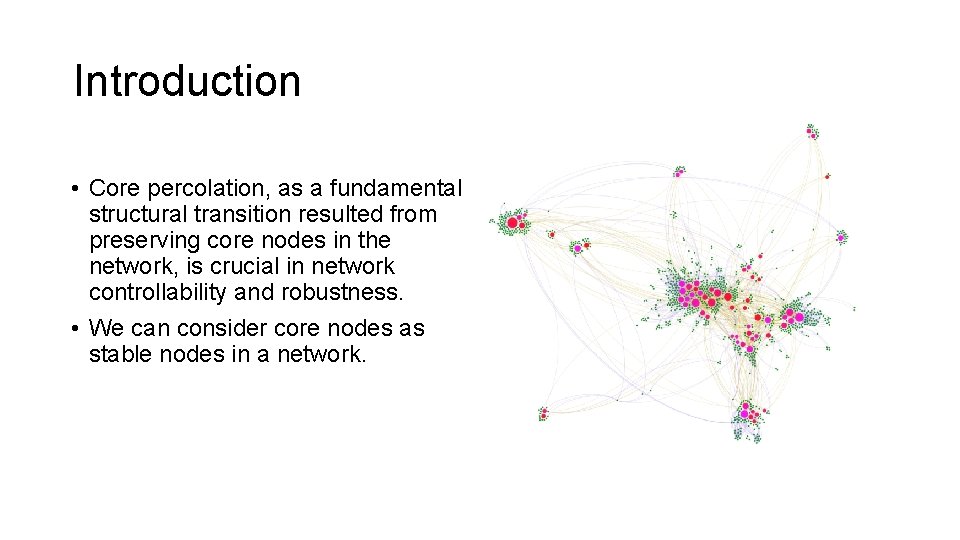
Introduction • Core percolation, as a fundamental structural transition resulted from preserving core nodes in the network, is crucial in network controllability and robustness. • We can consider core nodes as stable nodes in a network.
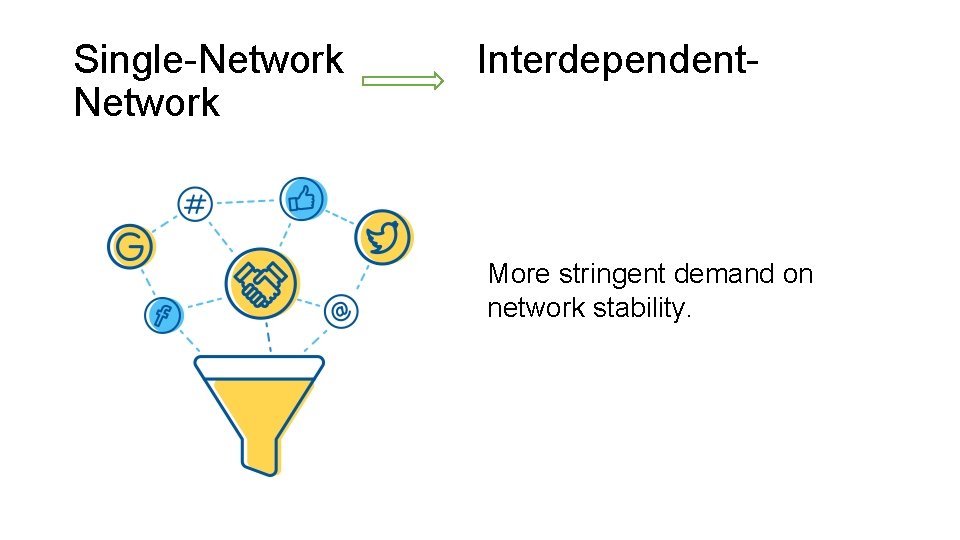
Single-Network Interdependent- More stringent demand on network stability.
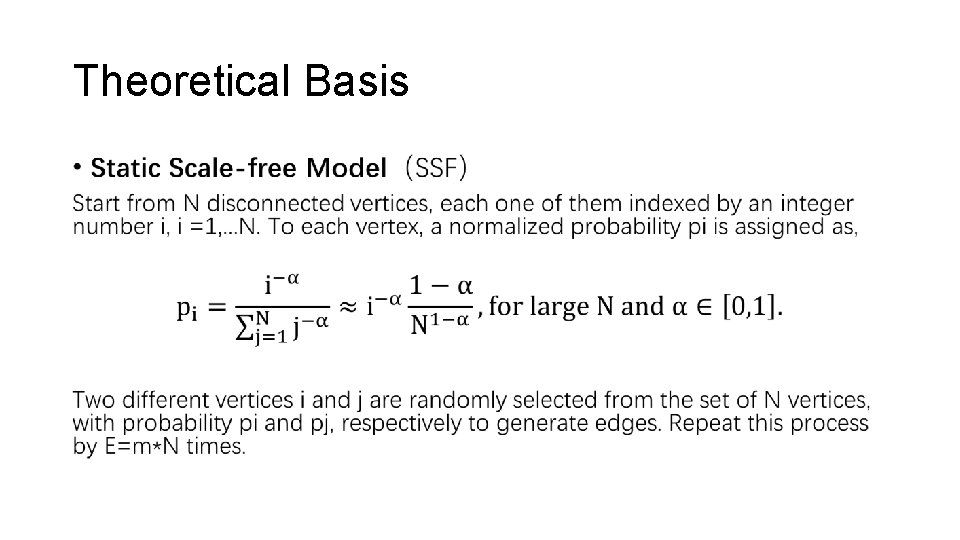
Theoretical Basis •
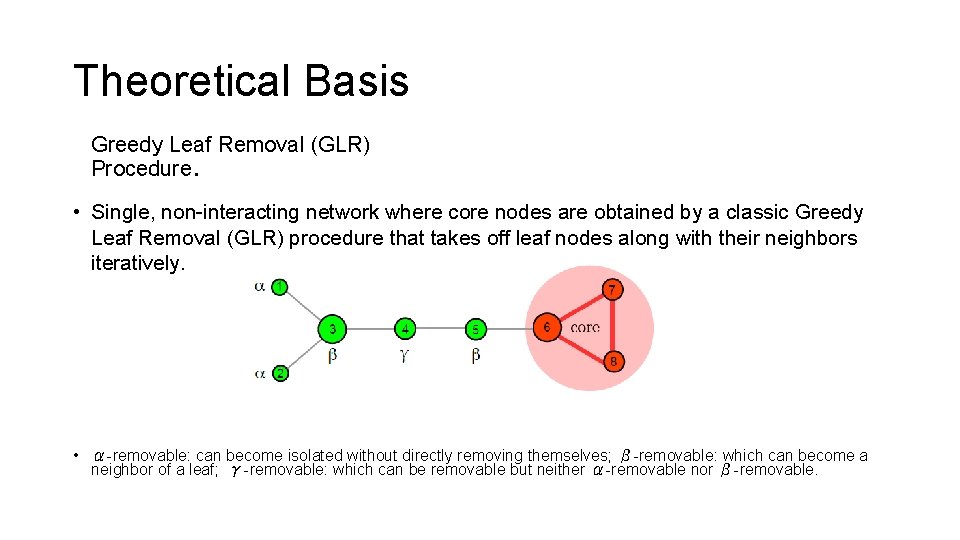
Theoretical Basis Greedy Leaf Removal (GLR) Procedure. • Single, non-interacting network where core nodes are obtained by a classic Greedy Leaf Removal (GLR) procedure that takes off leaf nodes along with their neighbors iteratively. • α-removable: can become isolated without directly removing themselves; β-removable: which can become a neighbor of a leaf; γ-removable: which can be removable but neither α-removable nor β-removable.
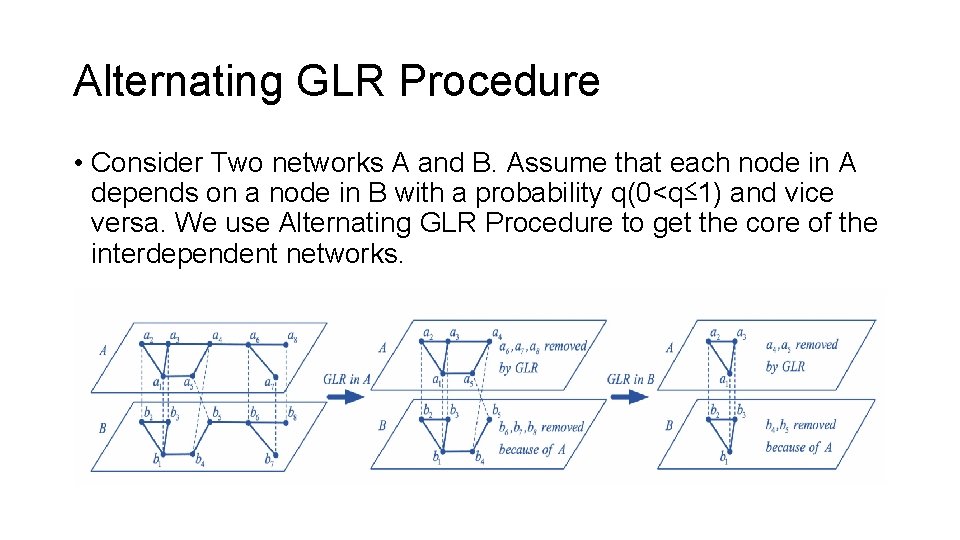
Alternating GLR Procedure • Consider Two networks A and B. Assume that each node in A depends on a node in B with a probability q(0<q≤ 1) and vice versa. We use Alternating GLR Procedure to get the core of the interdependent networks.
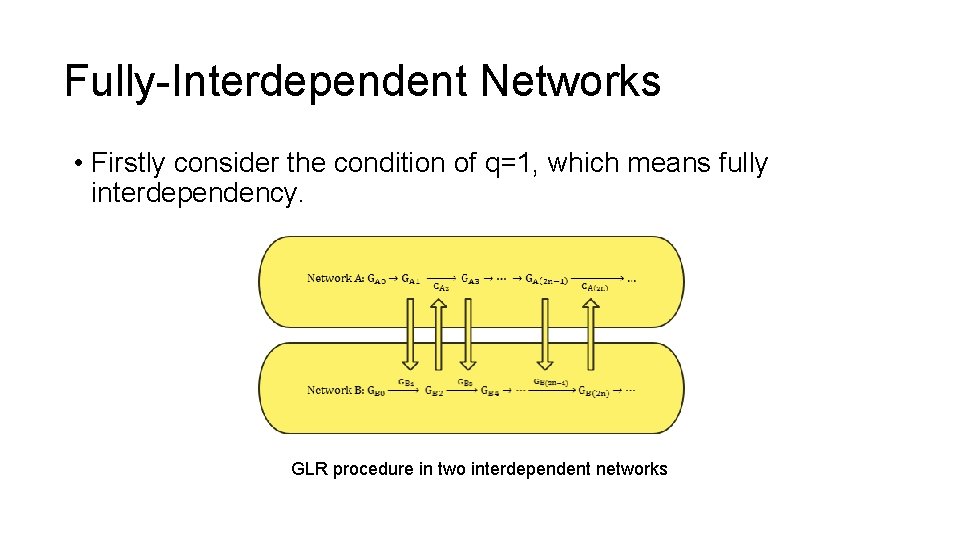
Fully-Interdependent Networks • Firstly consider the condition of q=1, which means fully interdependency. GLR procedure in two interdependent networks
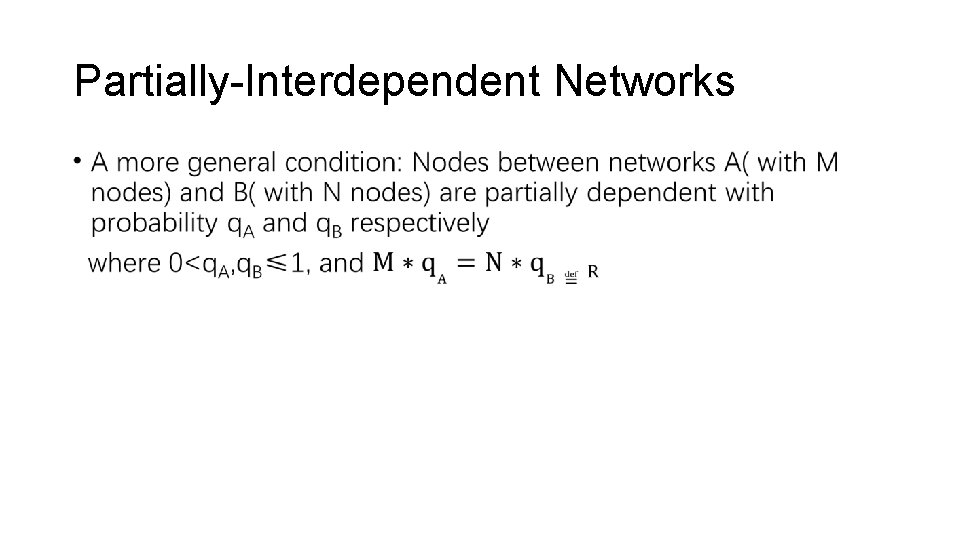
Partially-Interdependent Networks •
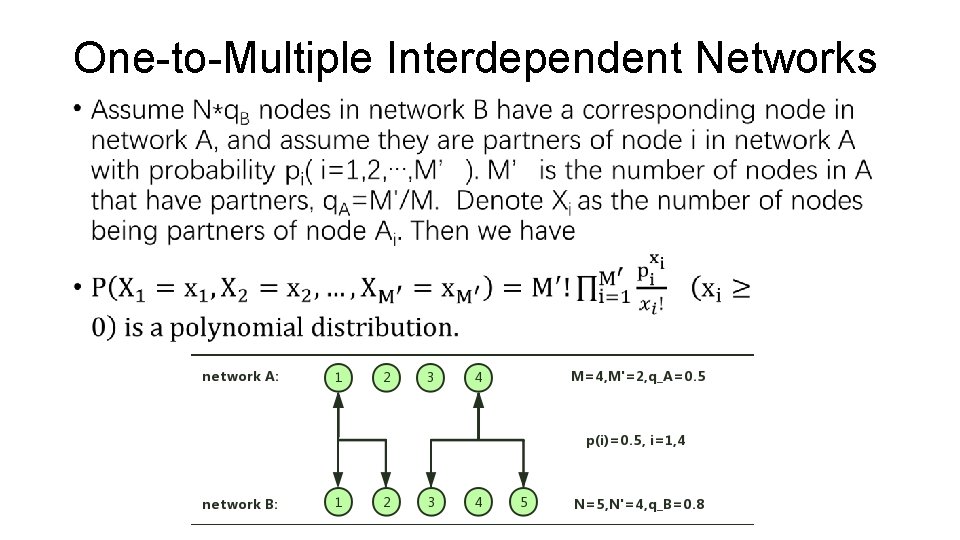
One-to-Multiple Interdependent Networks
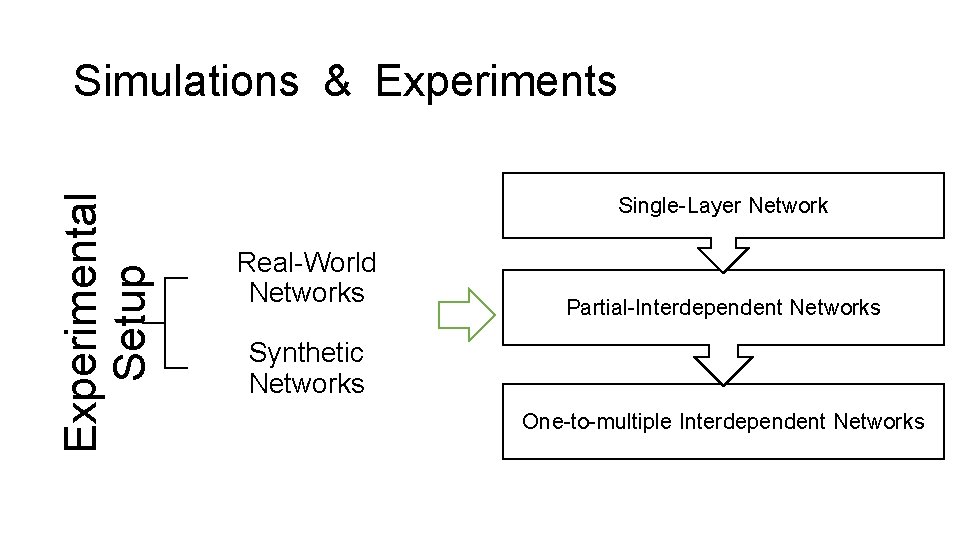
Experimental Setup Simulations & Experiments Single-Layer Network Real-World Networks Partial-Interdependent Networks Synthetic Networks One-to-multiple Interdependent Networks
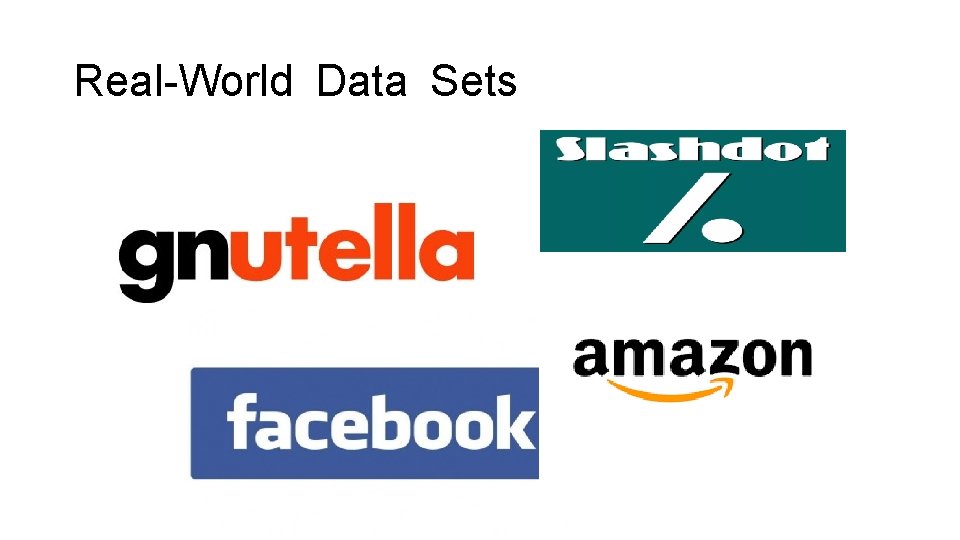
Real-World Data Sets
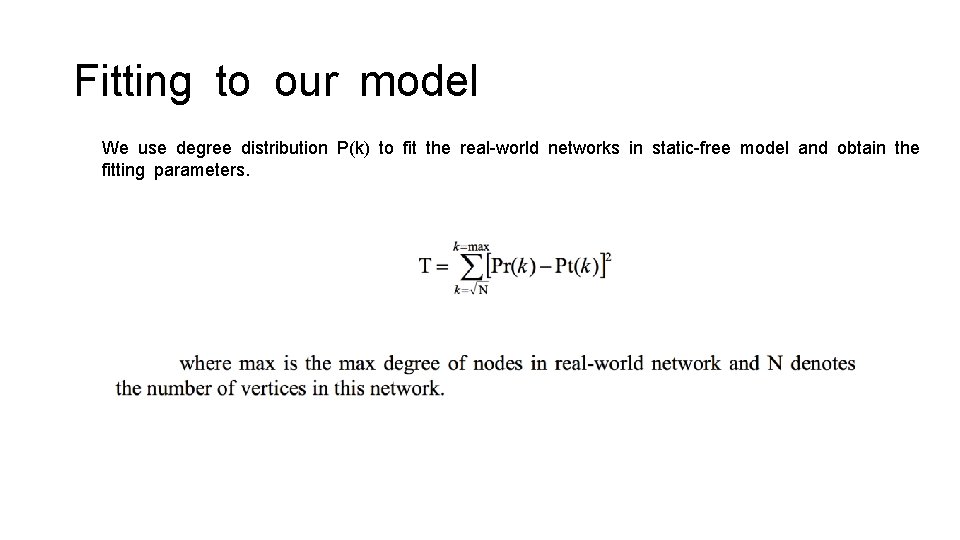
Fitting to our model We use degree distribution P(k) to fit the real-world networks in static-free model and obtain the fitting parameters.
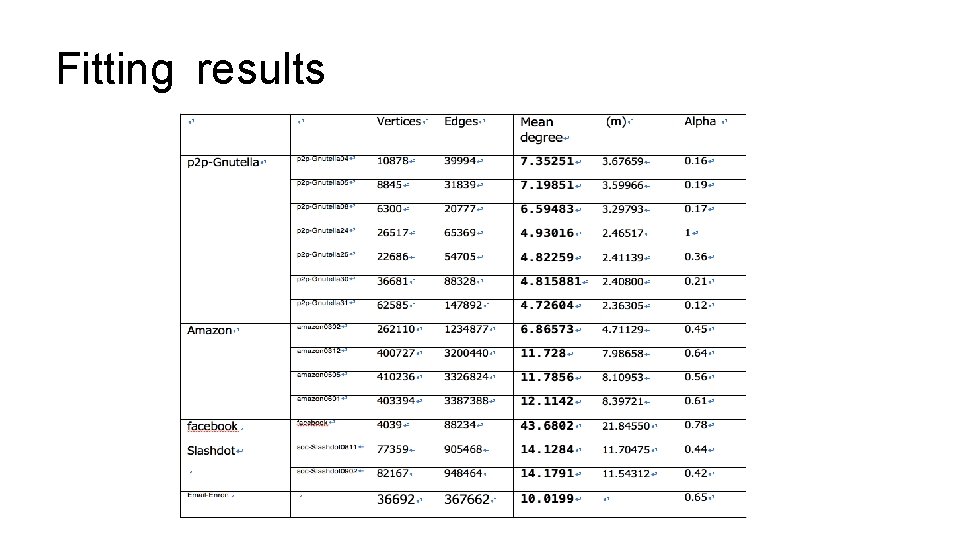
Fitting results
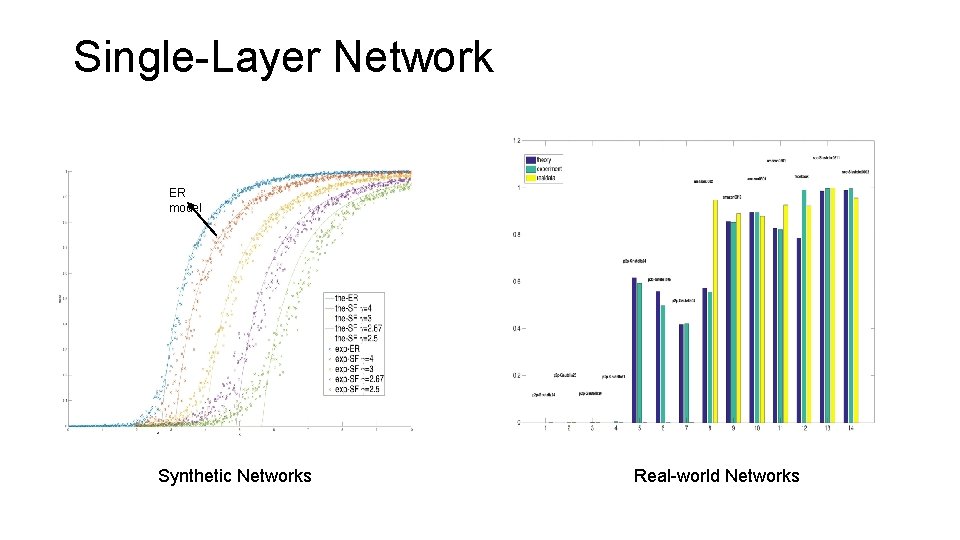
Single-Layer Network ER model Synthetic Networks Real-world Networks
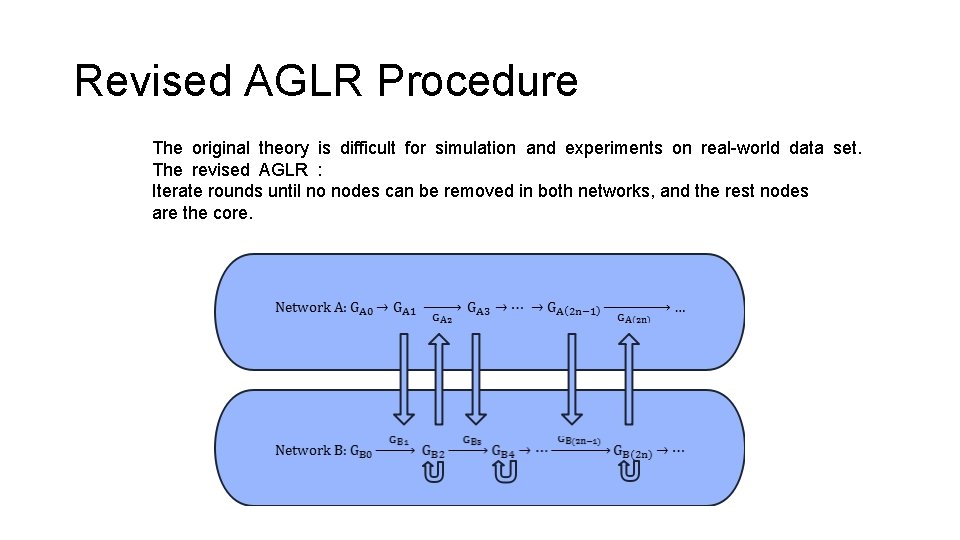
Revised AGLR Procedure The original theory is difficult for simulation and experiments on real-world data set. The revised AGLR : Iterate rounds until no nodes can be removed in both networks, and the rest nodes are the core.
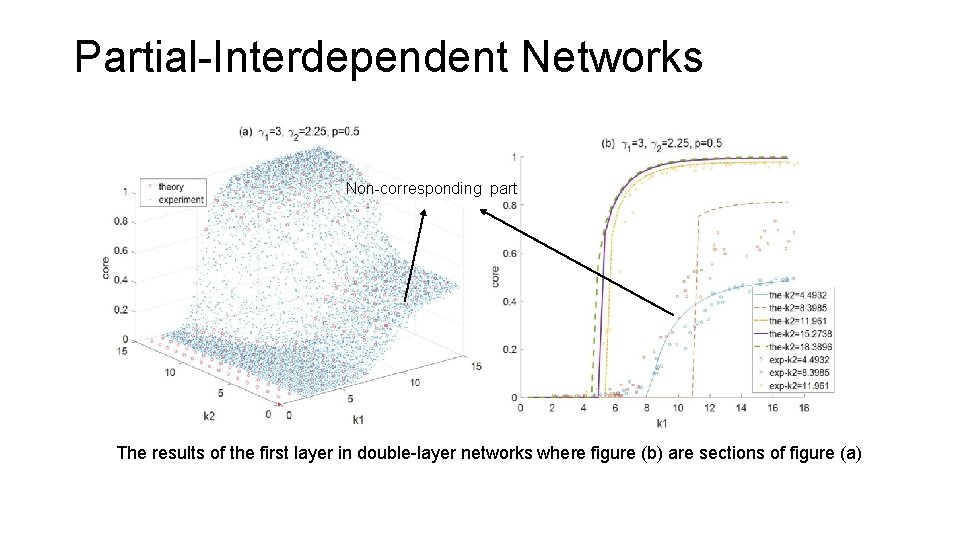
Partial-Interdependent Networks Non-corresponding part The results of the first layer in double-layer networks where figure (b) are sections of figure (a)
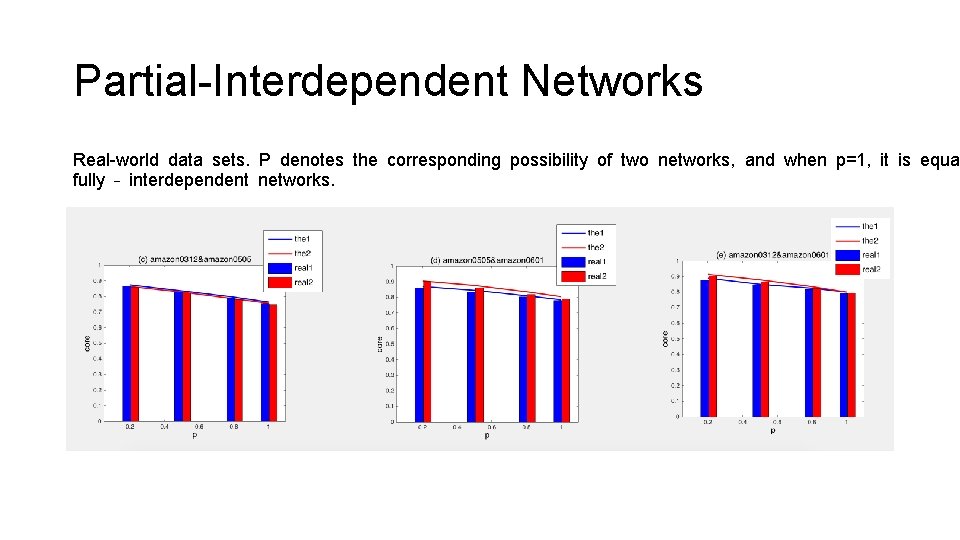
Partial-Interdependent Networks Real-world data sets. P denotes the corresponding possibility of two networks, and when p=1, it is equa fully – interdependent networks.
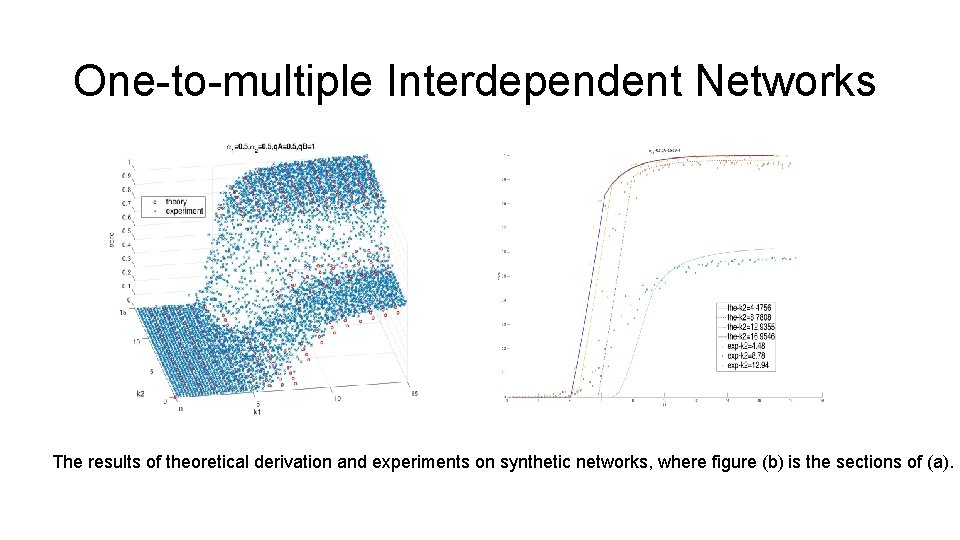
One-to-multiple Interdependent Networks The results of theoretical derivation and experiments on synthetic networks, where figure (b) is the sections of (a).
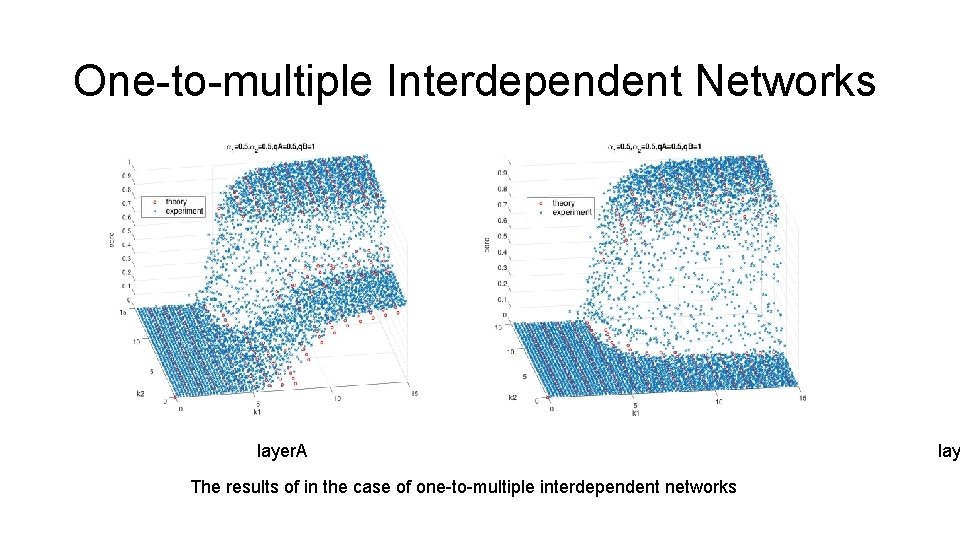
One-to-multiple Interdependent Networks layer. A The results of in the case of one-to-multiple interdependent networks lay
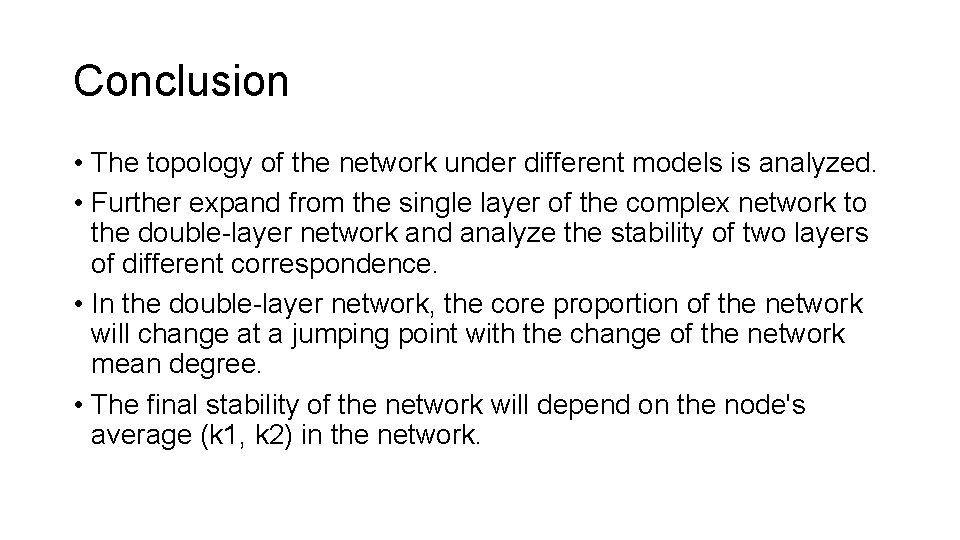
Conclusion • The topology of the network under different models is analyzed. • Further expand from the single layer of the complex network to the double-layer network and analyze the stability of two layers of different correspondence. • In the double-layer network, the core proportion of the network will change at a jumping point with the change of the network mean degree. • The final stability of the network will depend on the node's average (k 1, k 2) in the network.
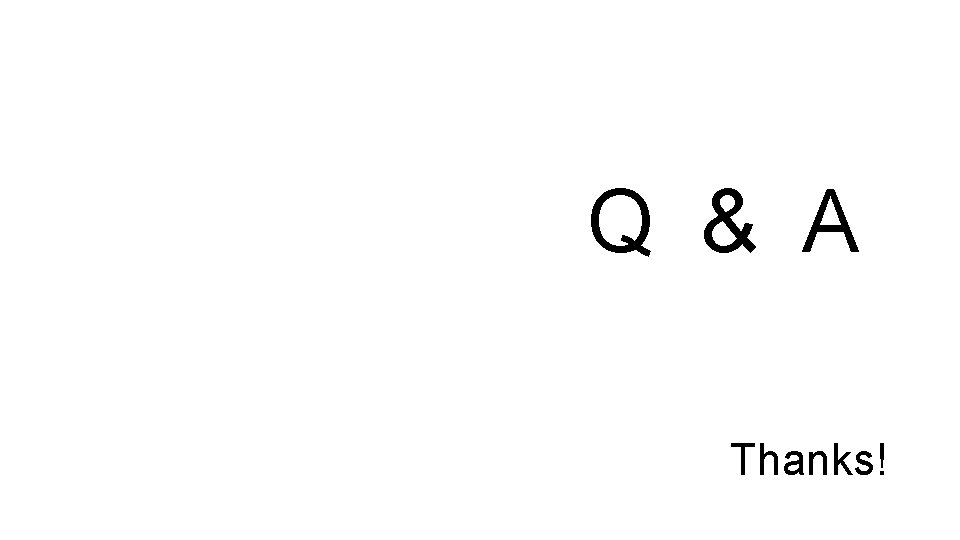
Q & A Thanks!
Infiltration water cycle
Infiltration vs percolation
Elen johad
Design of percolation tank
Java percolation
Percolation
Maceration pharmaceutics
Chemical properties of dental materials
Difference between datagram and virtual circuit operation
Backbone networks in computer networks
Switching in data link layer
Presentation layer
Network layer is concerned with
A link layer protocol for quantum networks
Utopian simplex protocol
Data link control
Greedy layer wise training of deep networks
Design issues of network layer
Deva nan
Nan-f--app8 -site:youtube.com
Billy elliot grandmother
Nǐ jiā yǒu jí kǒu rén?
Amanda o'nan