Air quality and haze weather forecast for 2010
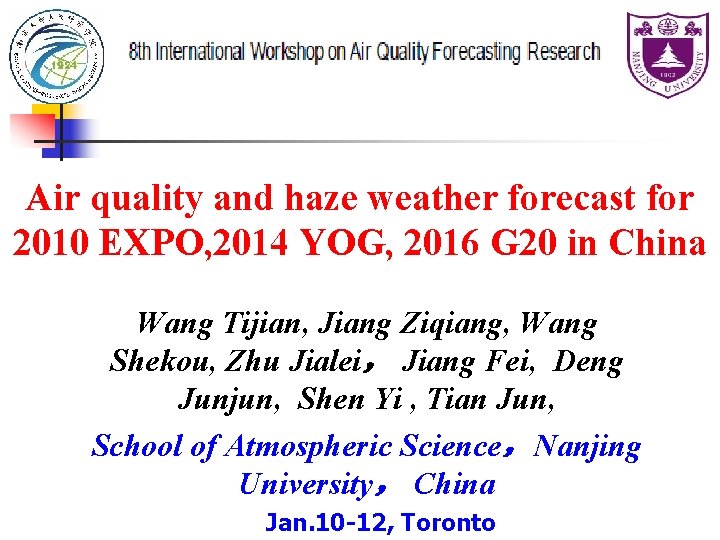
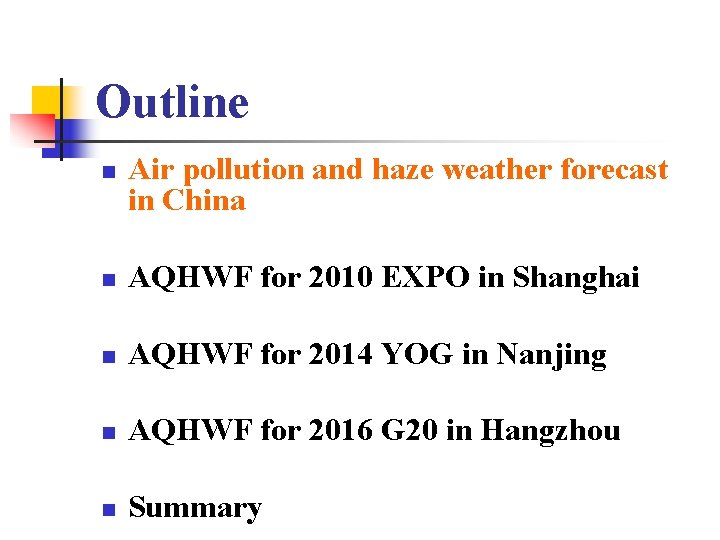
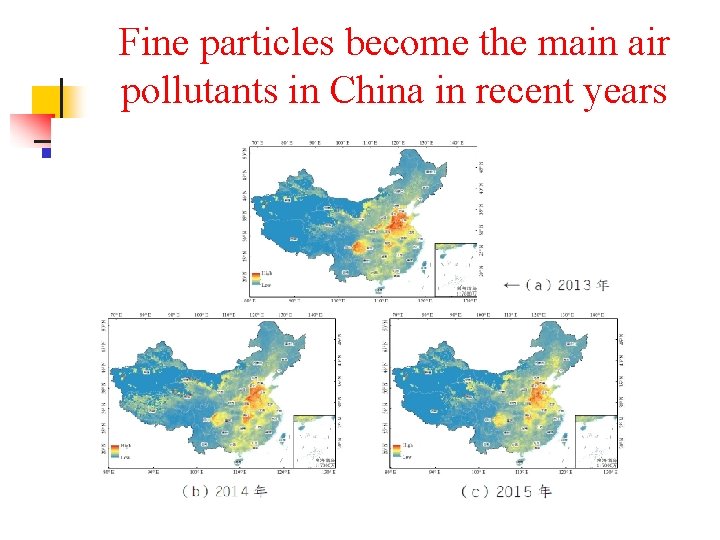
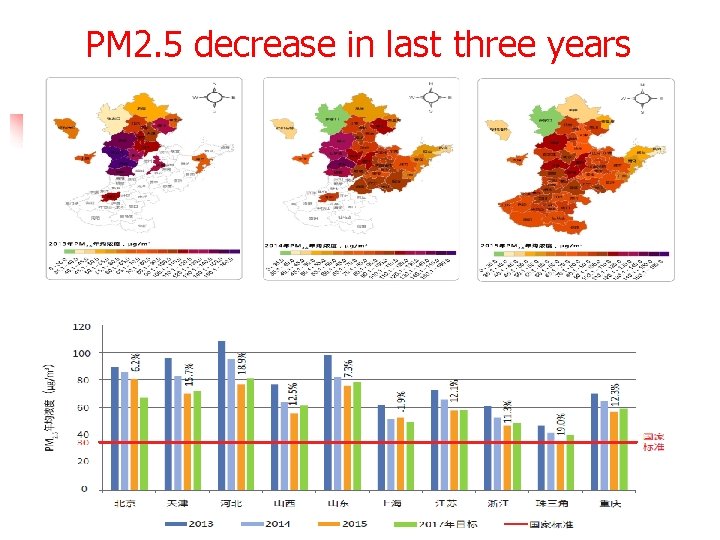
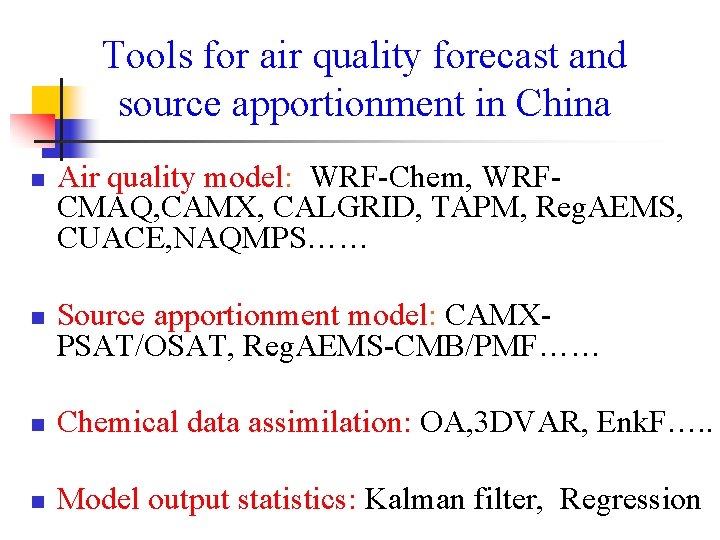
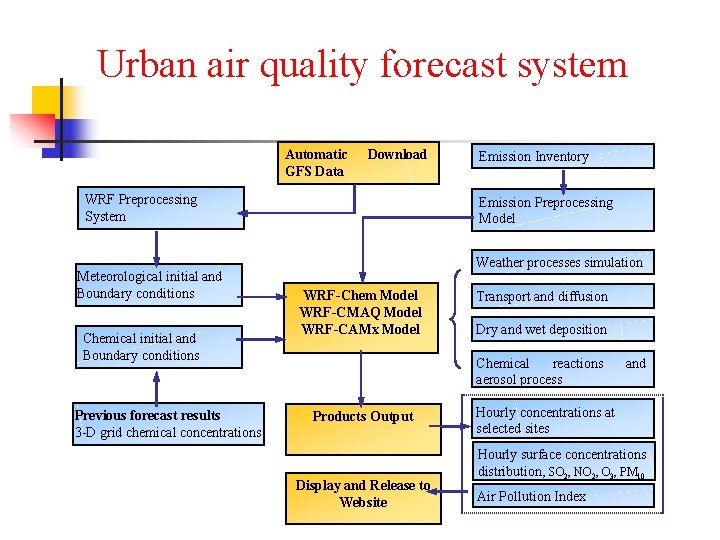
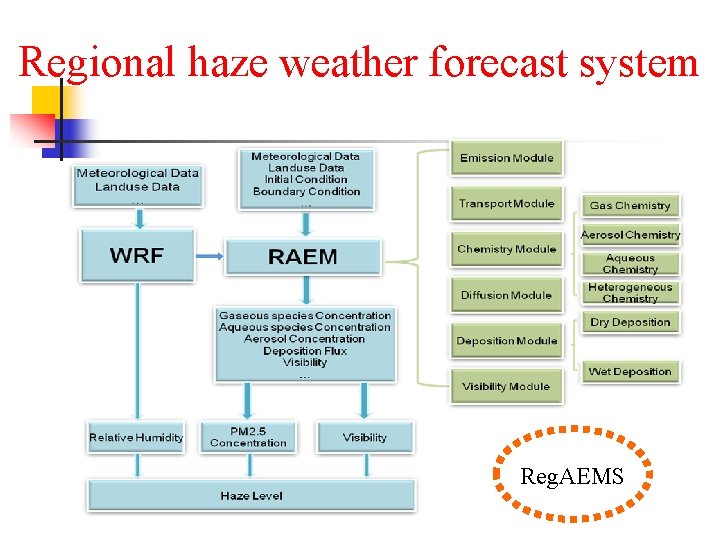
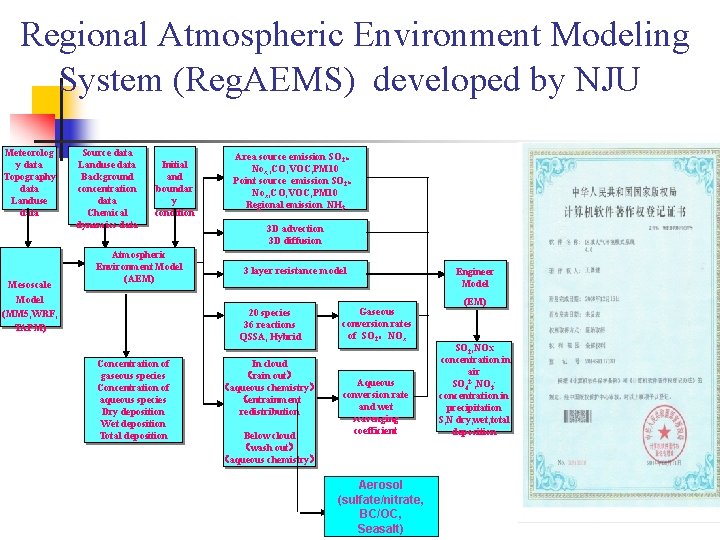
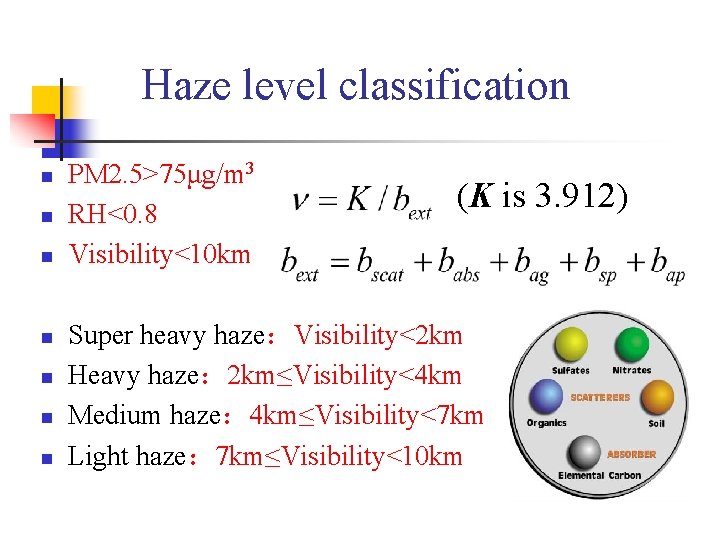
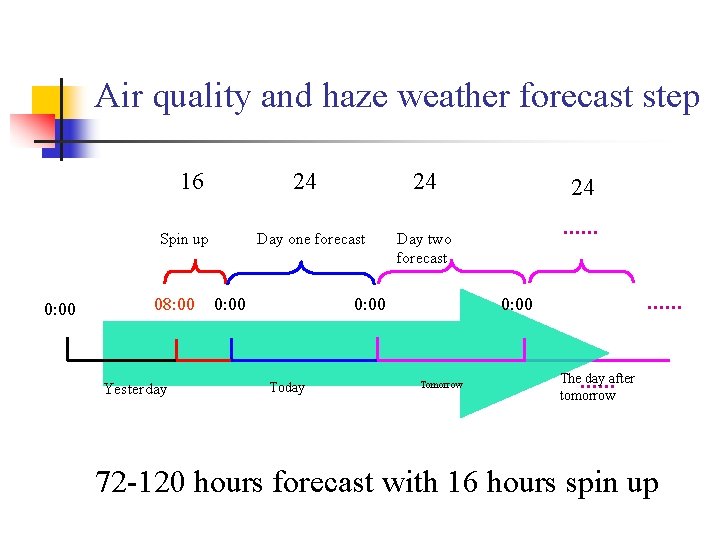
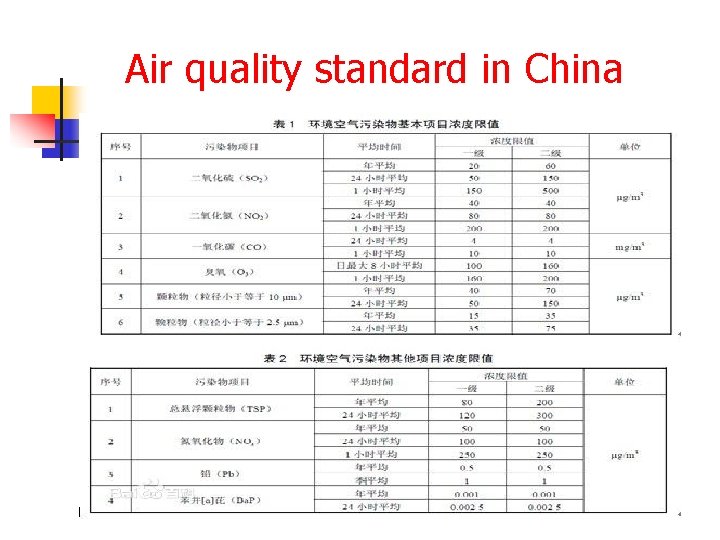
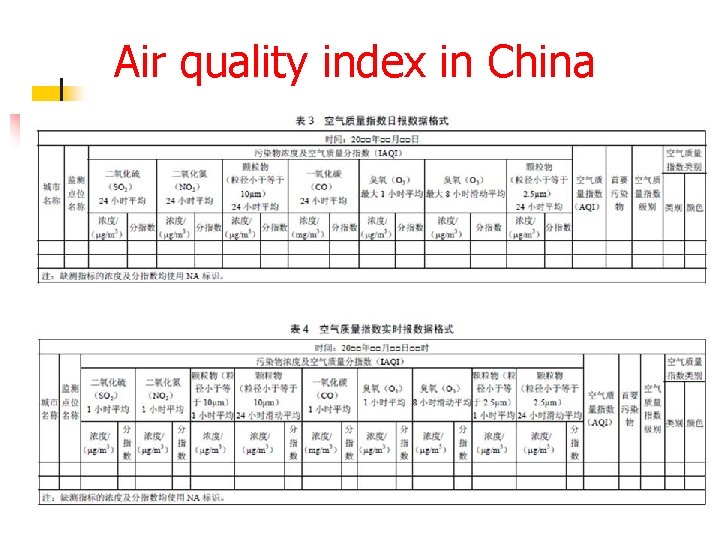
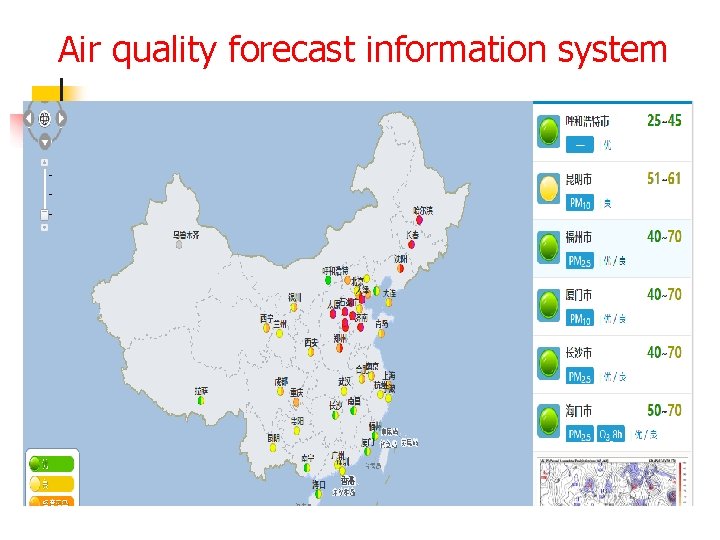
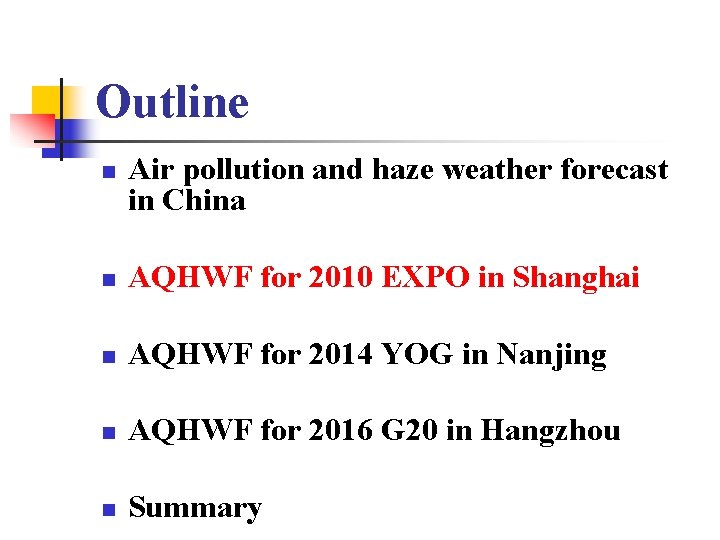
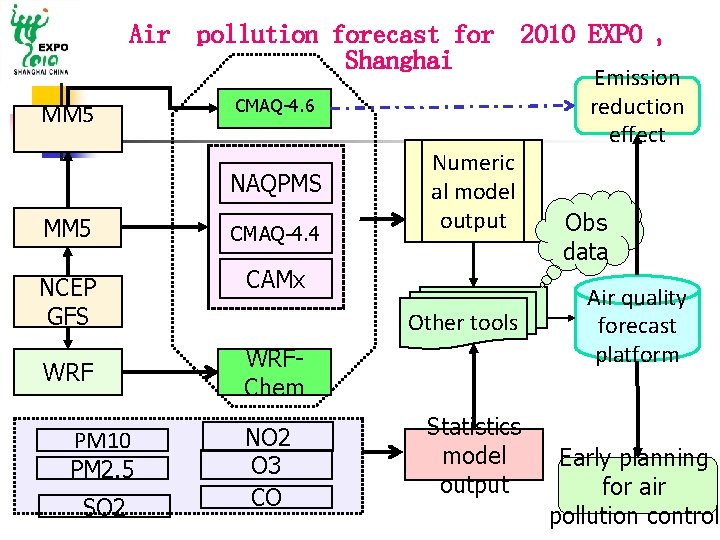
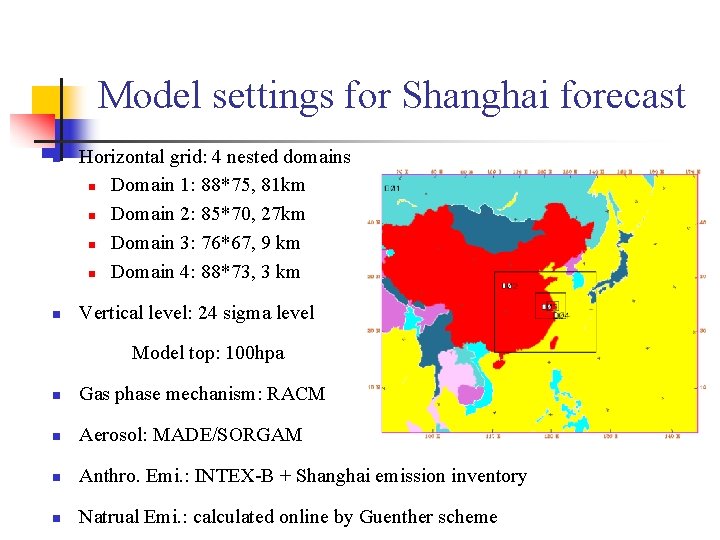
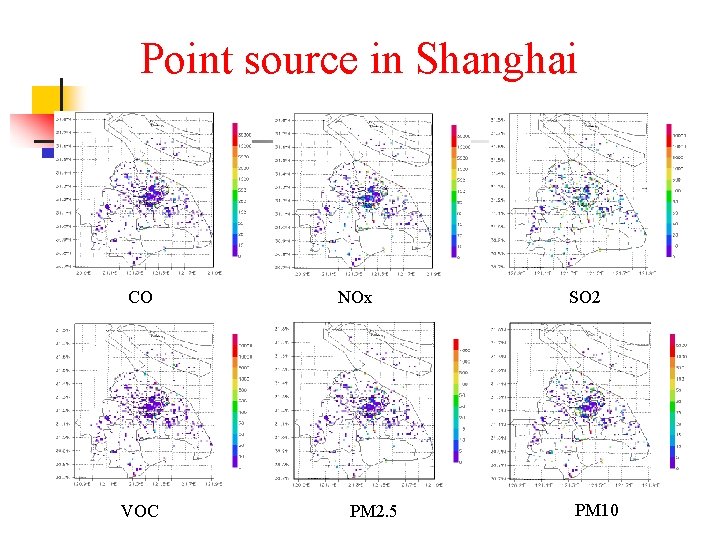
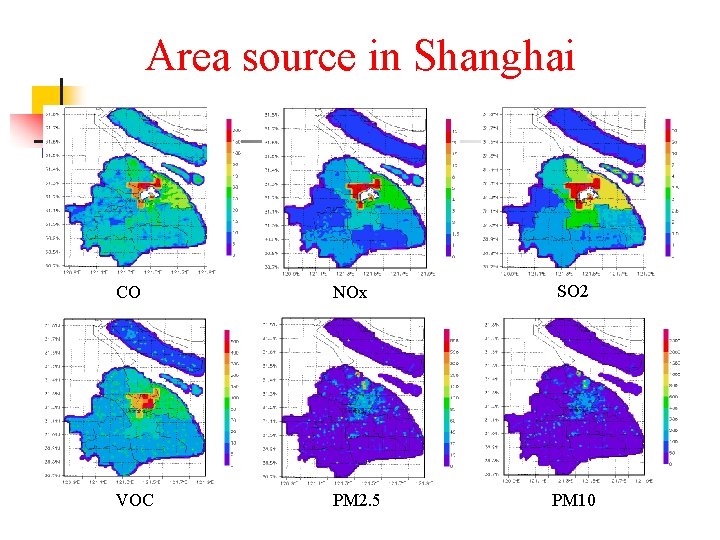
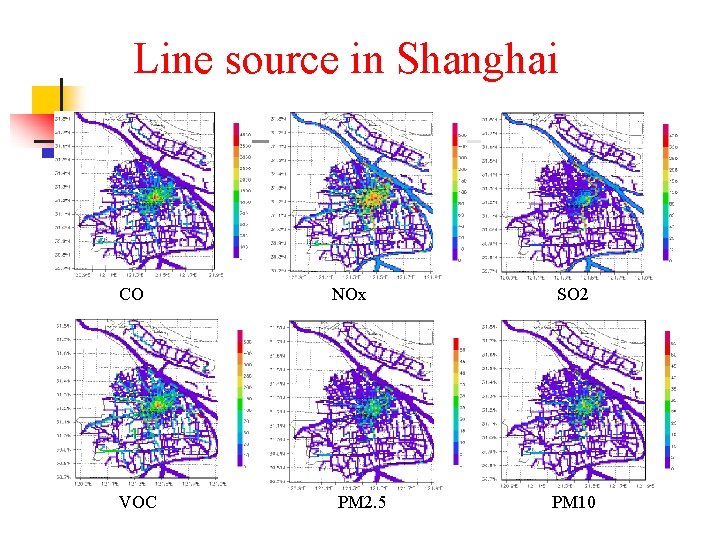
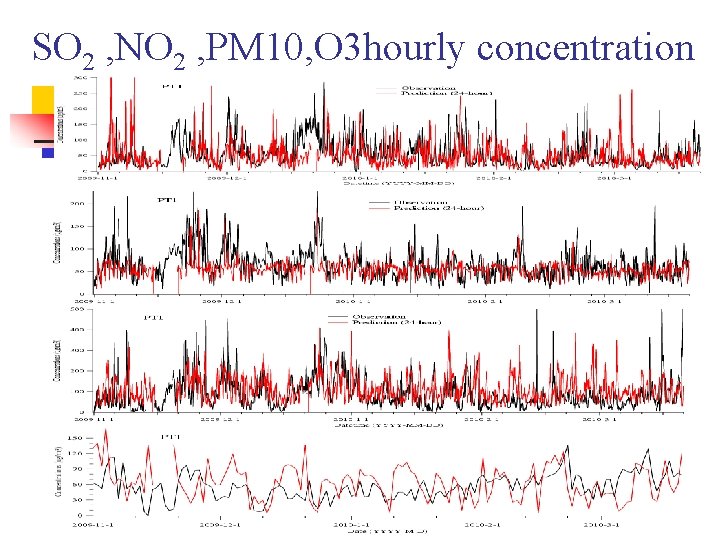
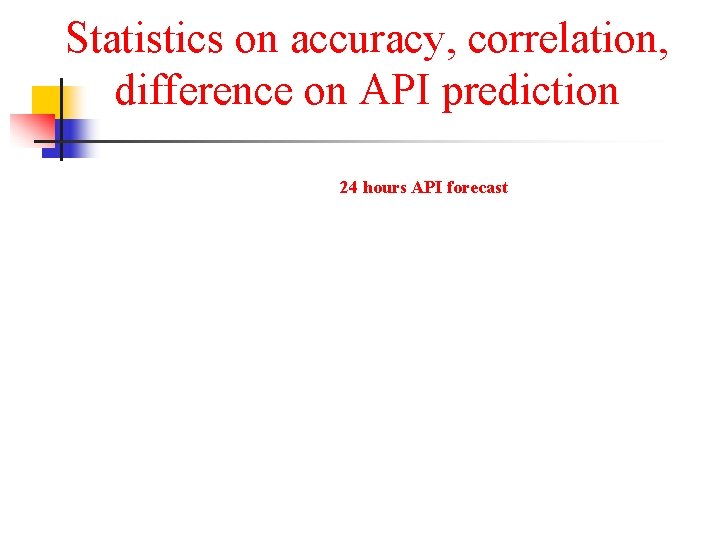
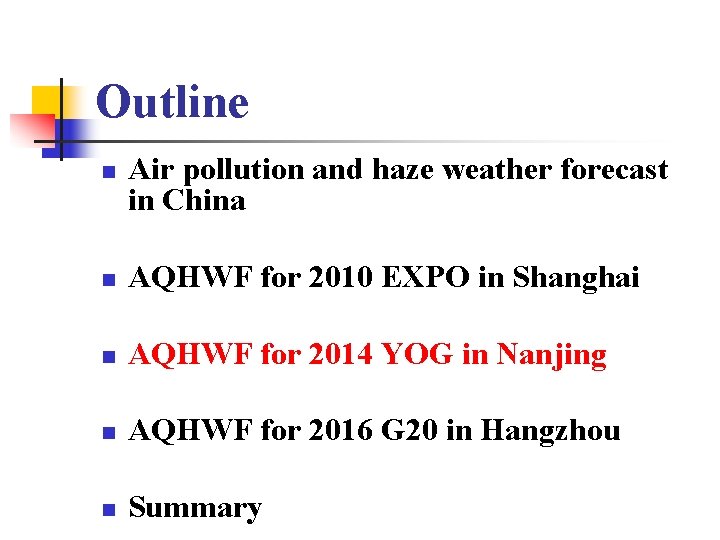
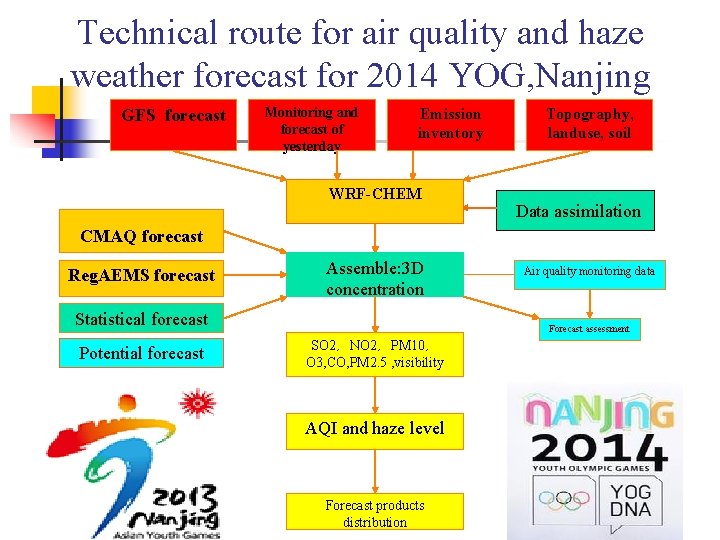
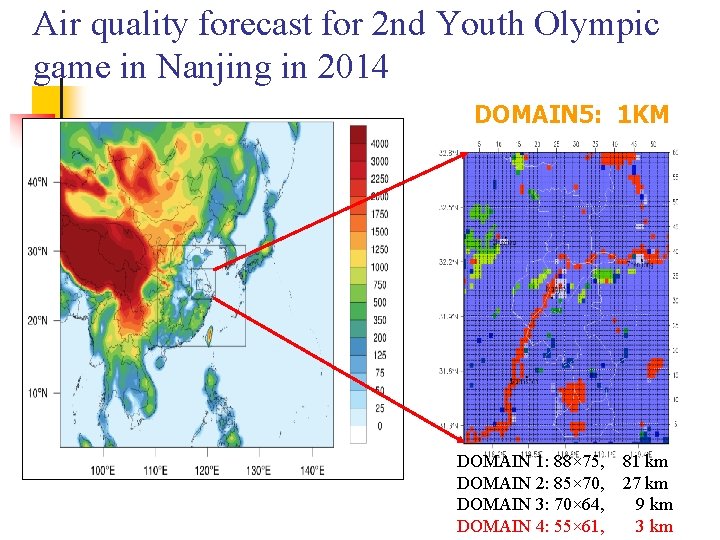
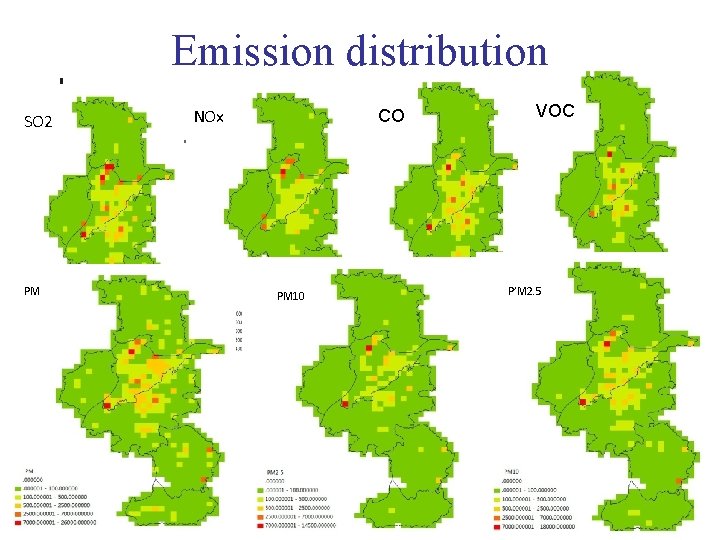
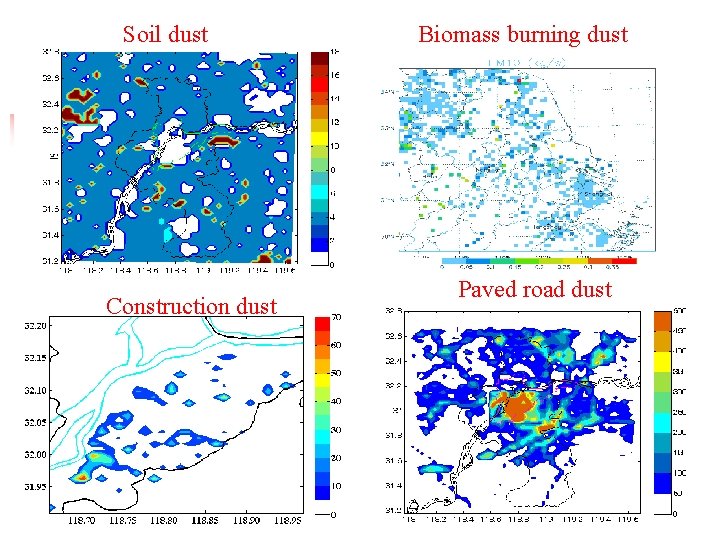
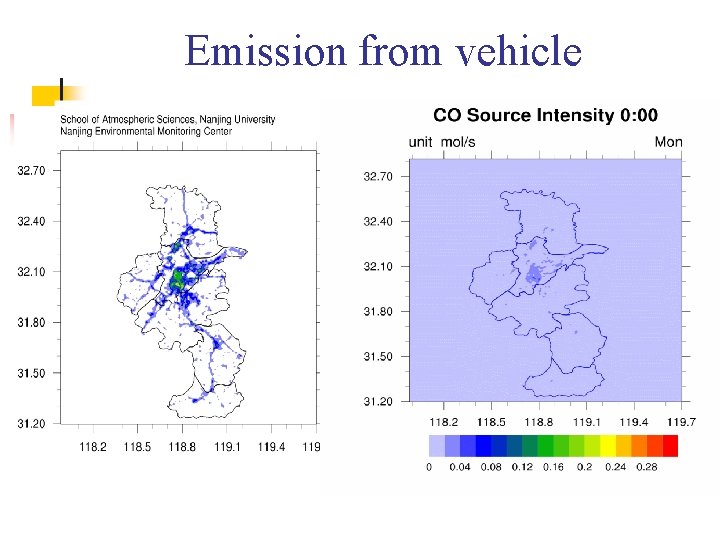
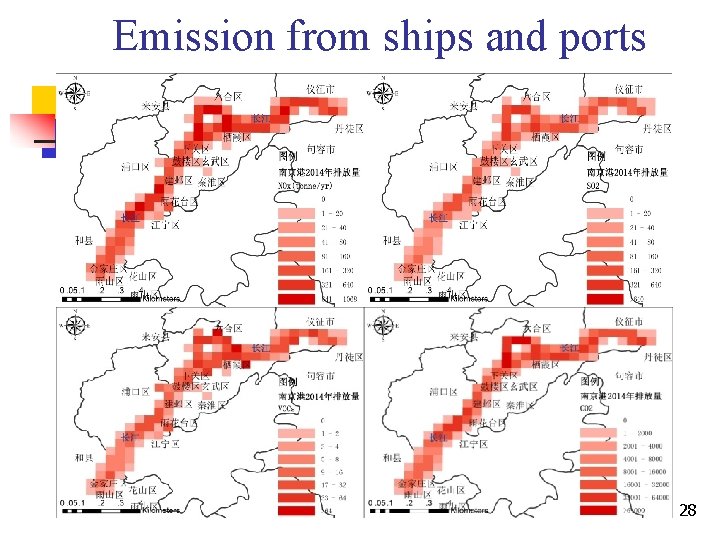
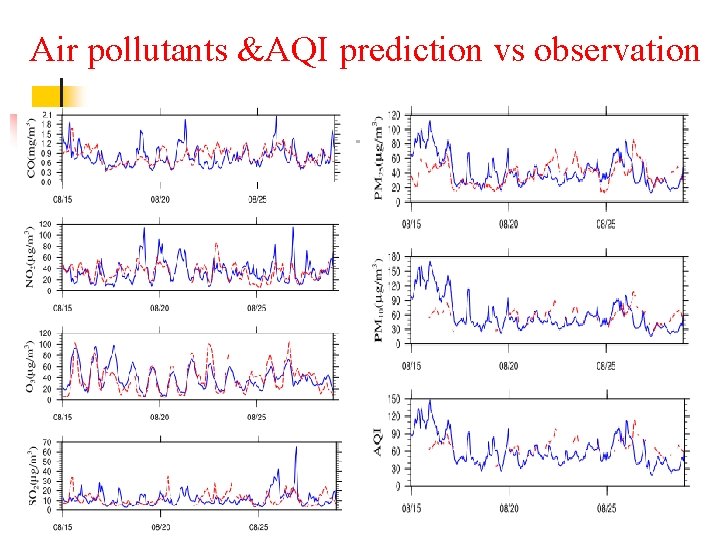
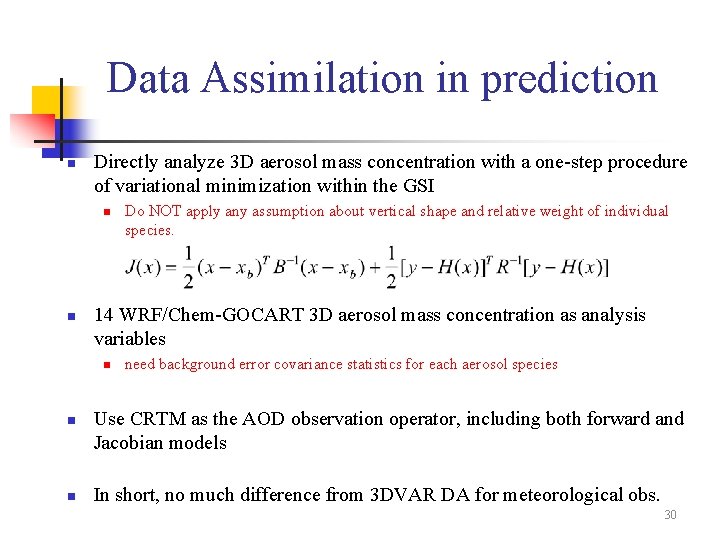
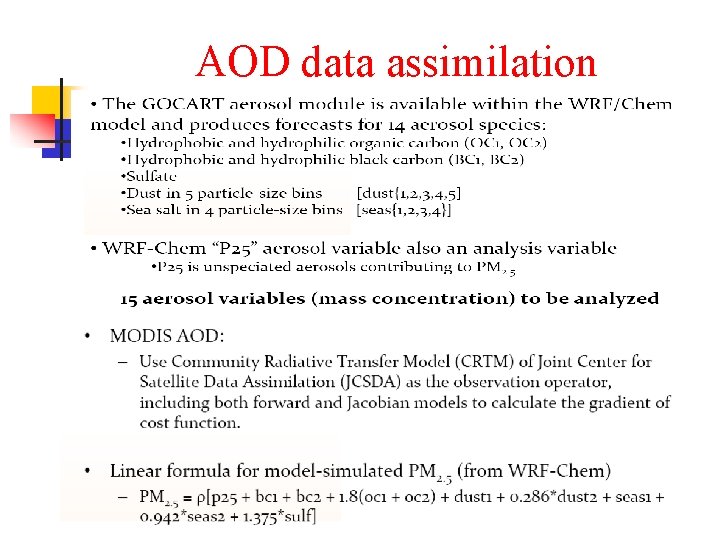
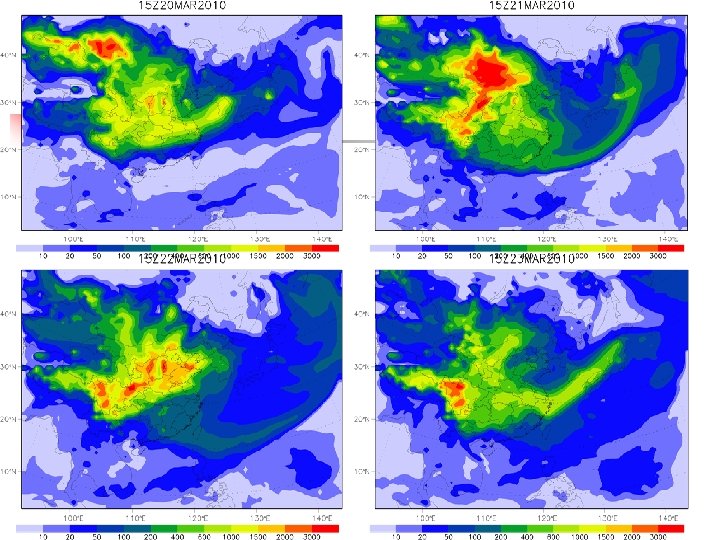
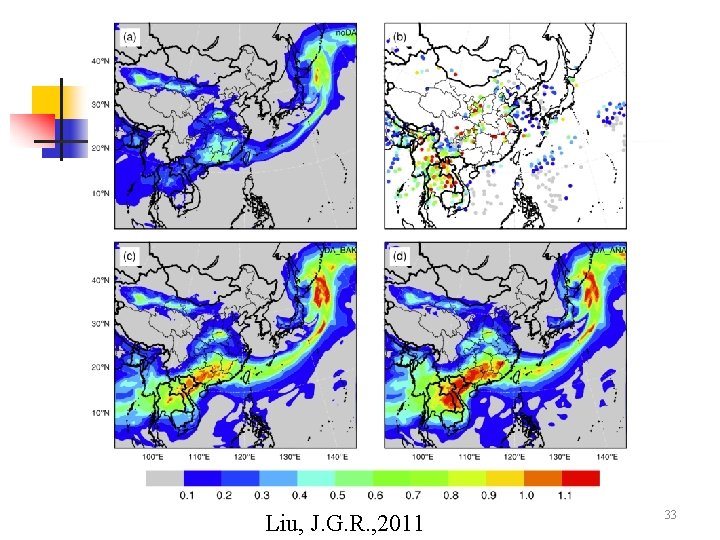
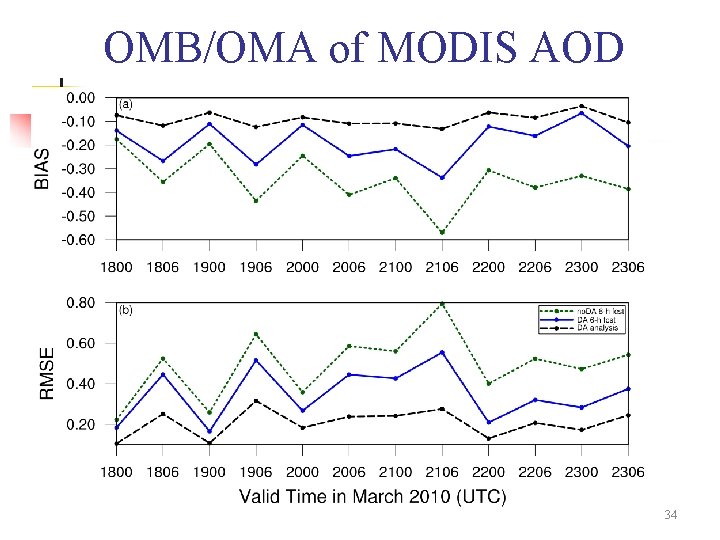
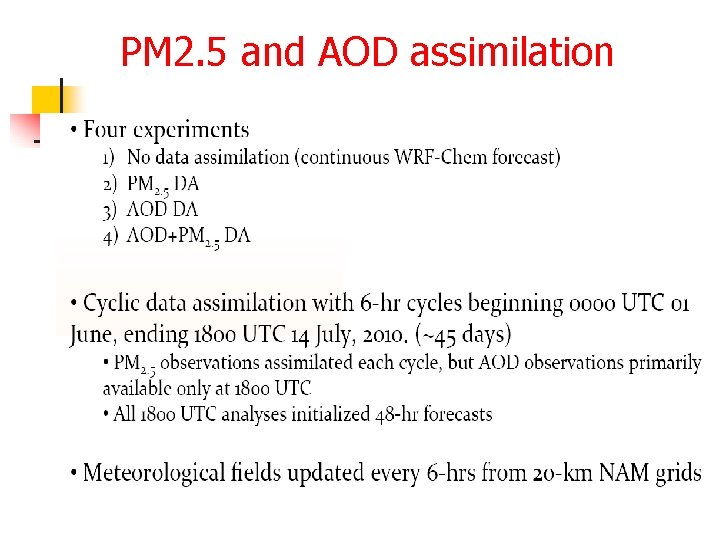
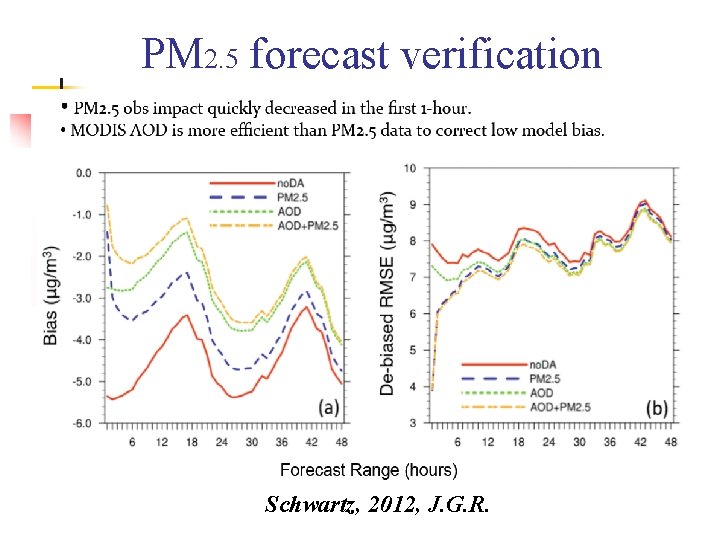
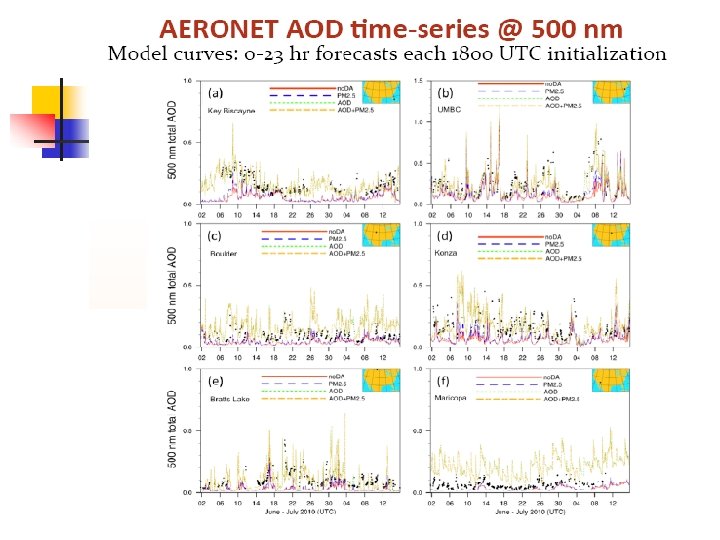
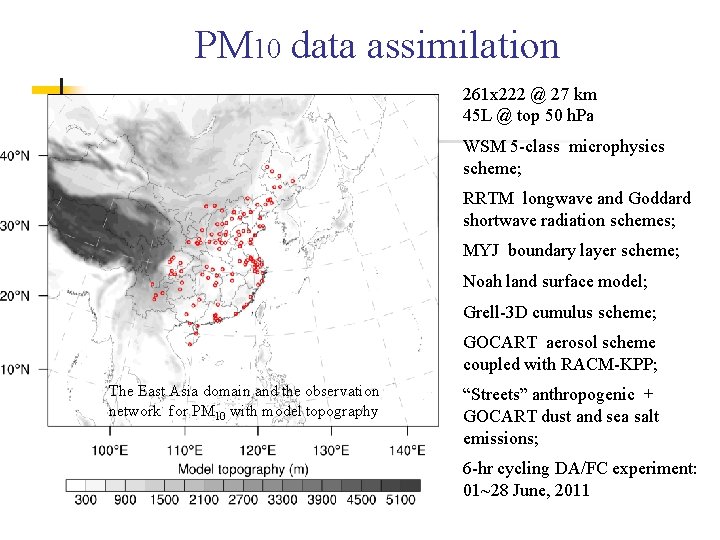
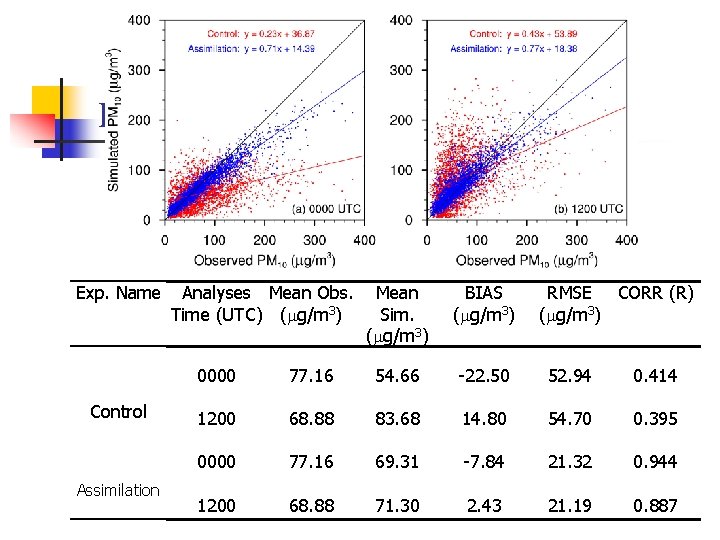
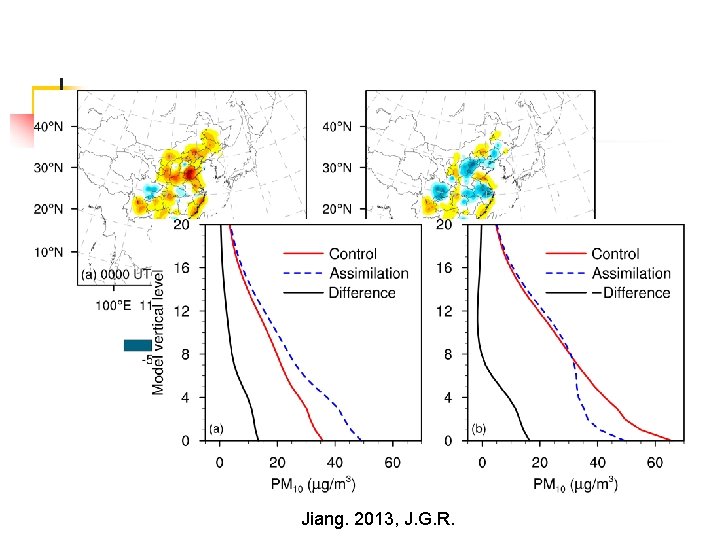
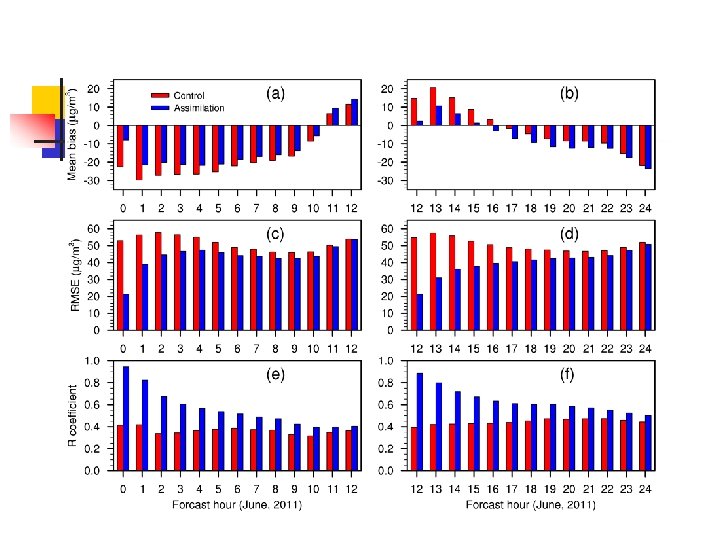
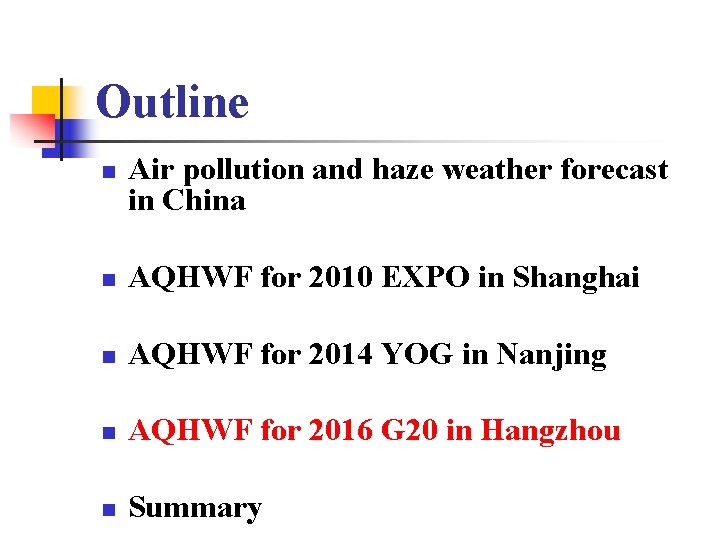
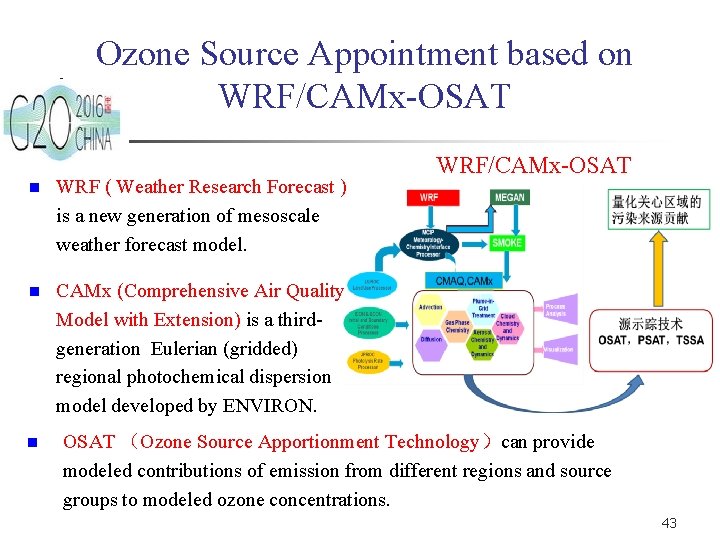
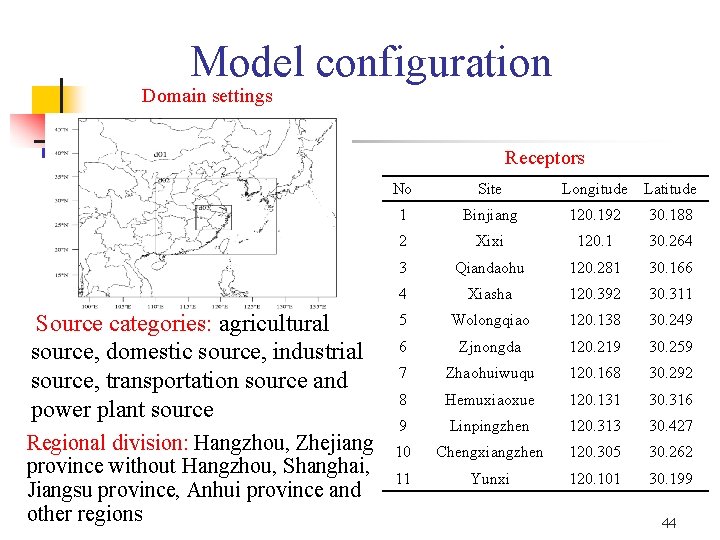
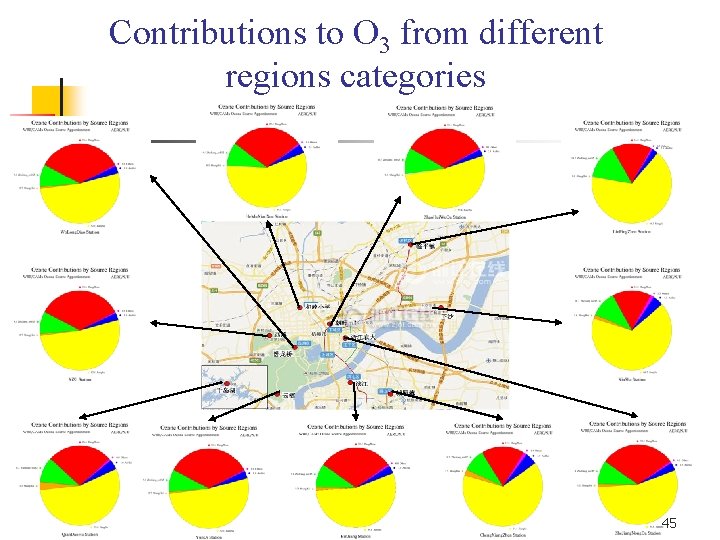
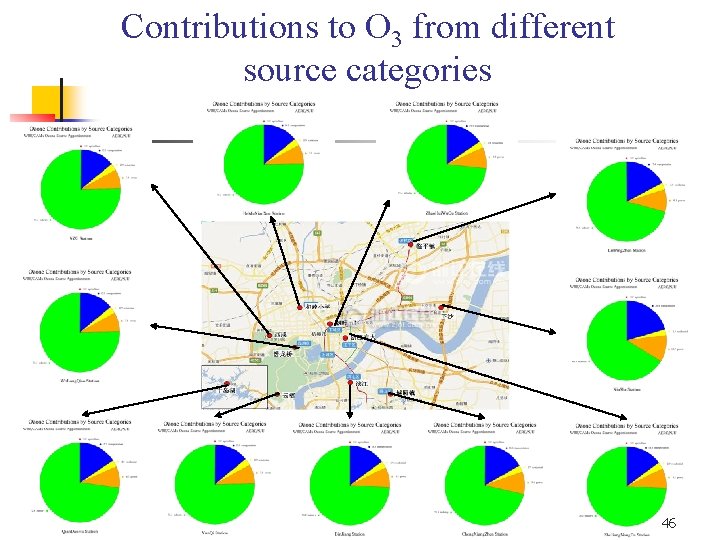
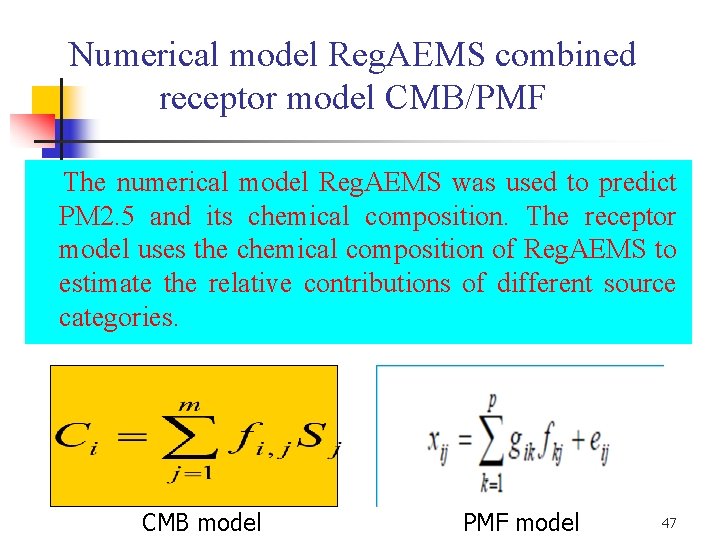
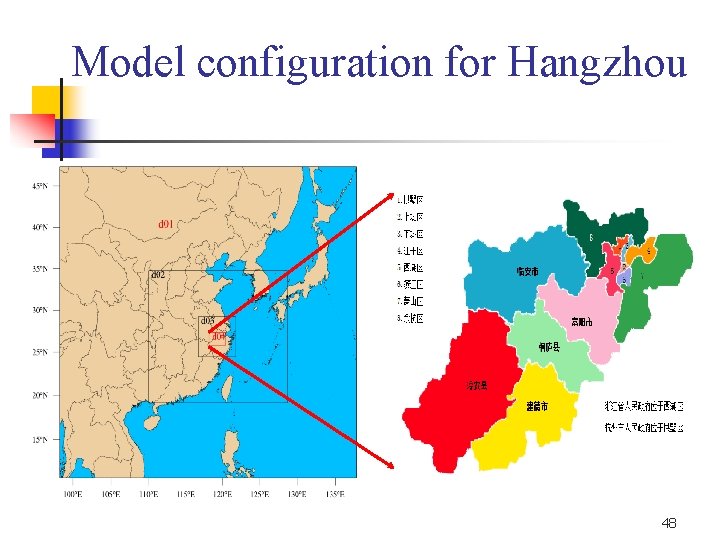
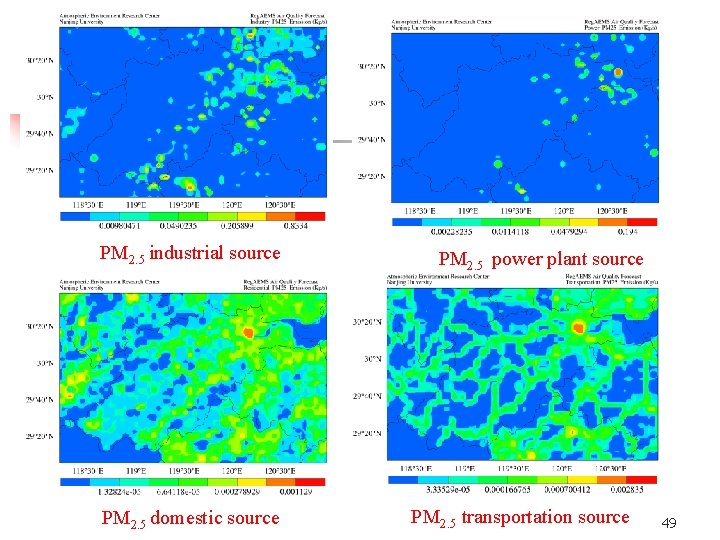
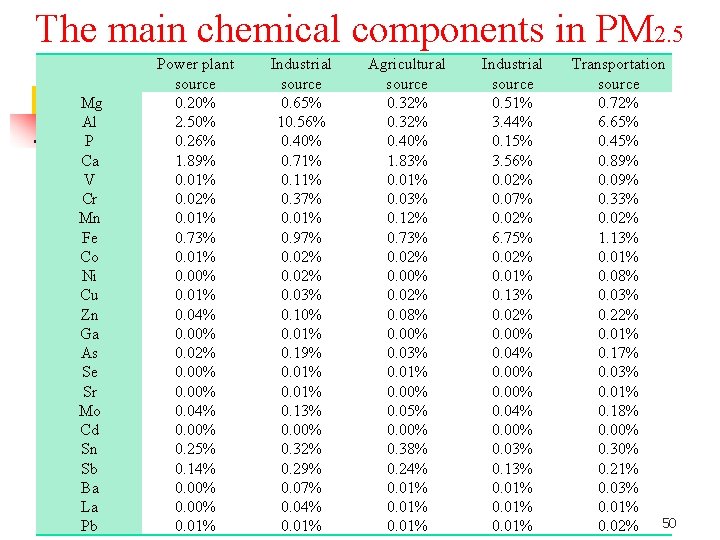
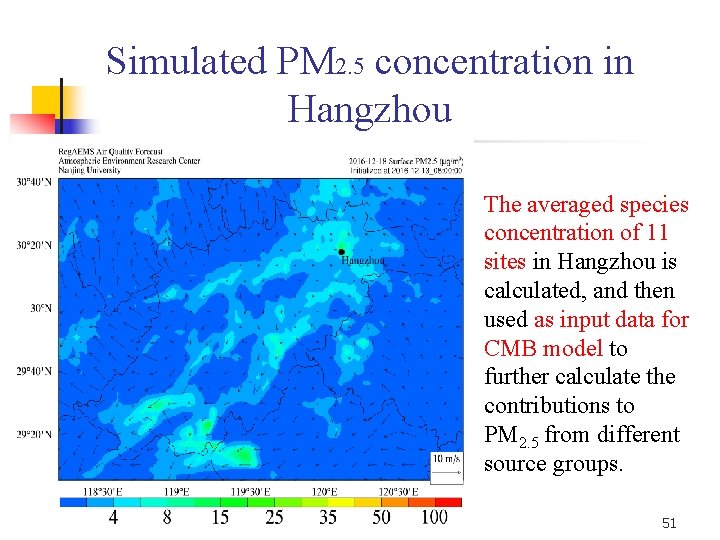
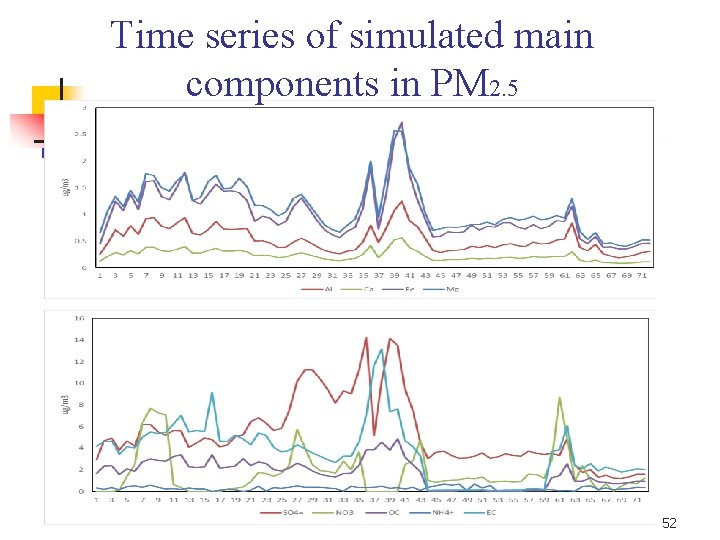
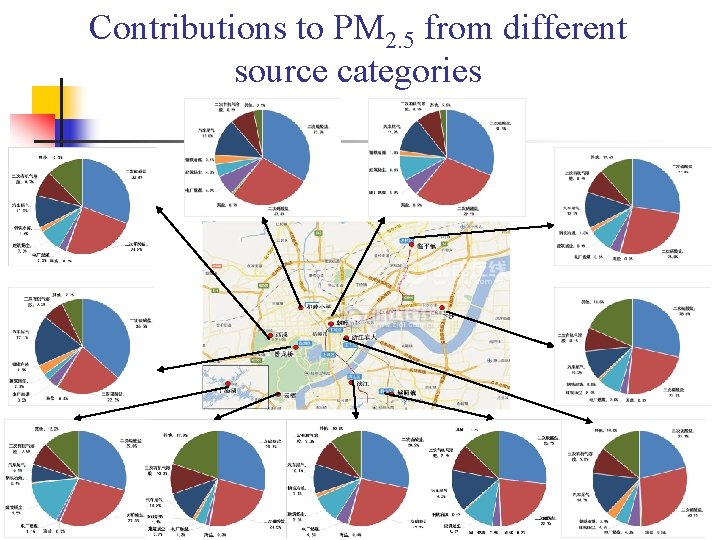
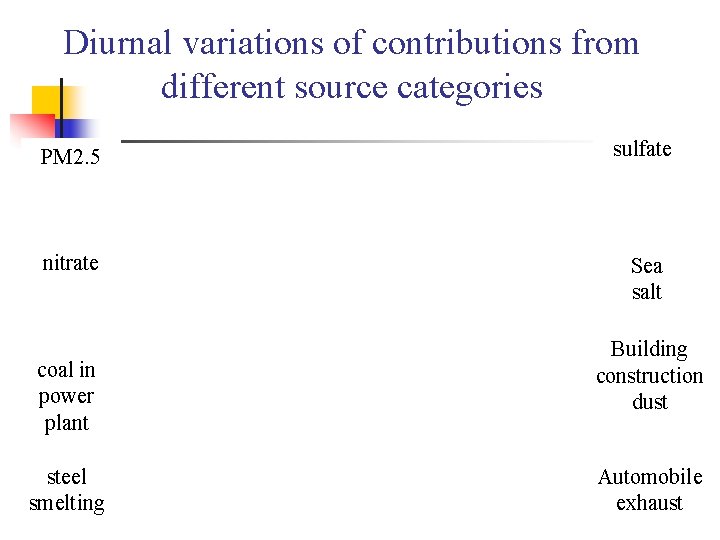
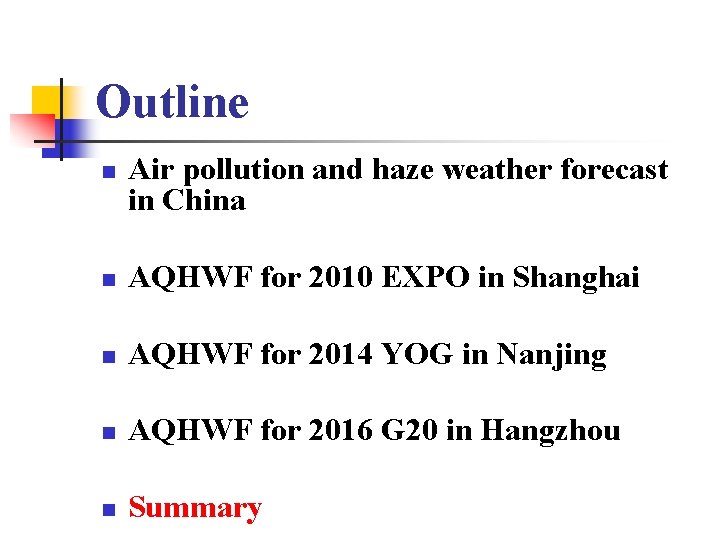
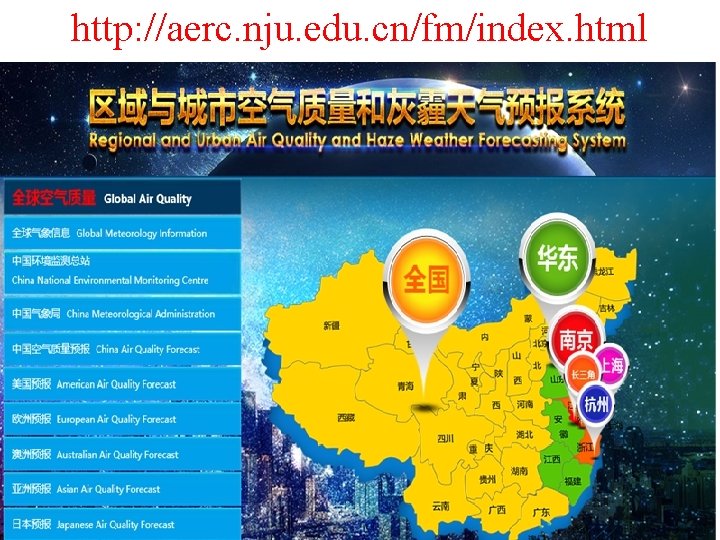
- Slides: 56
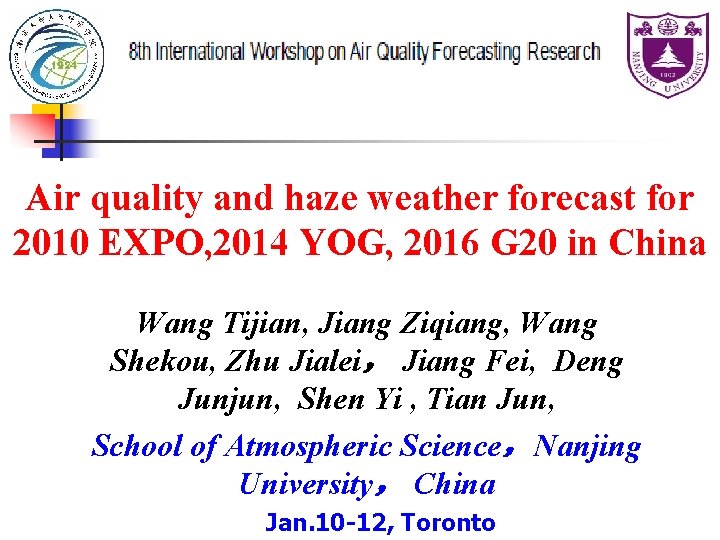
Air quality and haze weather forecast for 2010 EXPO, 2014 YOG, 2016 G 20 in China Wang Tijian, Jiang Ziqiang, Wang Shekou, Zhu Jialei, Jiang Fei, Deng Junjun, Shen Yi , Tian Jun, School of Atmospheric Science,Nanjing University, China Jan. 10 -12, Toronto
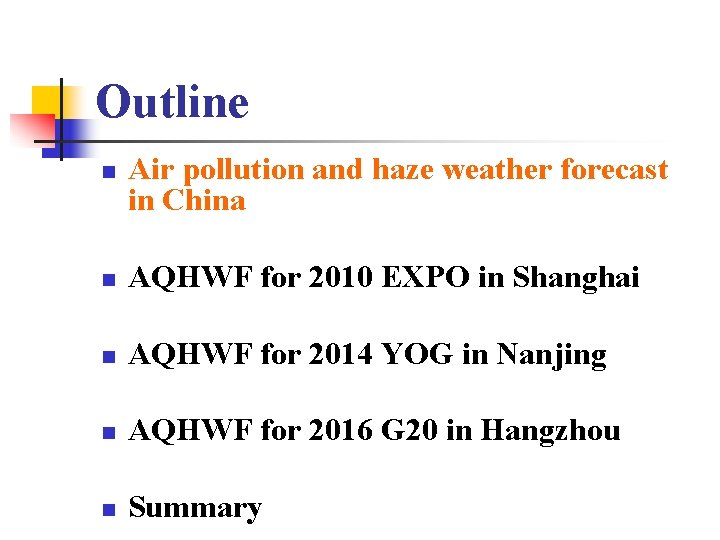
Outline n Air pollution and haze weather forecast in China n AQHWF for 2010 EXPO in Shanghai n AQHWF for 2014 YOG in Nanjing n AQHWF for 2016 G 20 in Hangzhou n Summary
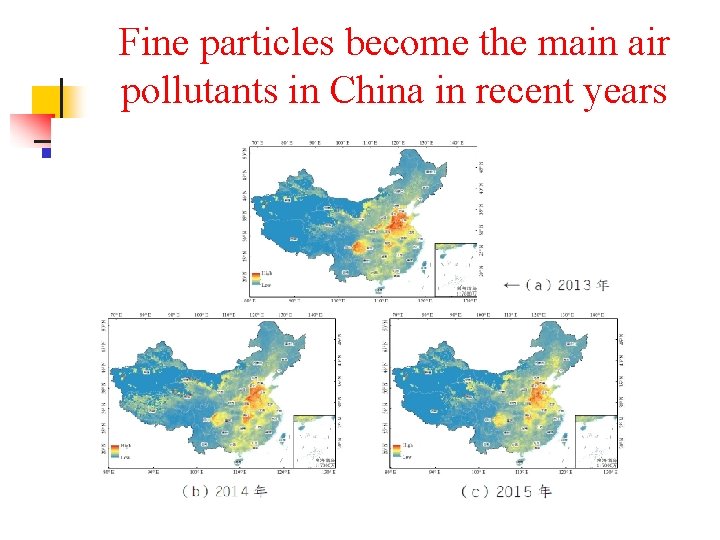
Fine particles become the main air pollutants in China in recent years
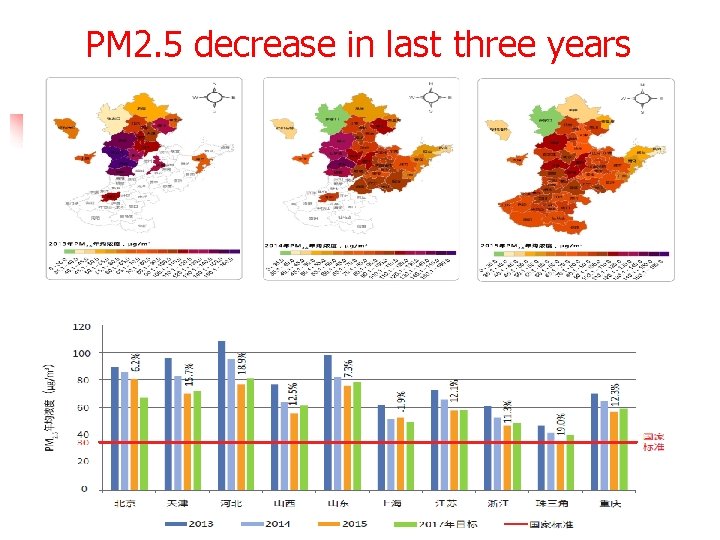
PM 2. 5 decrease in last three years
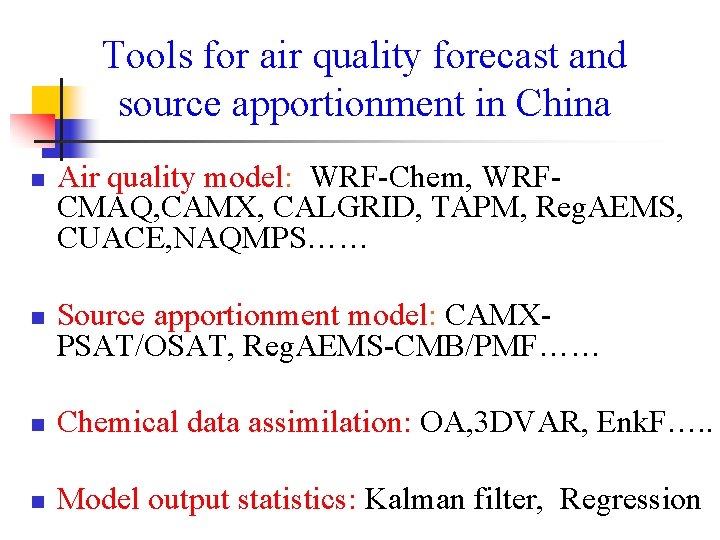
Tools for air quality forecast and source apportionment in China n n Air quality model: WRF-Chem, WRFCMAQ, CAMX, CALGRID, TAPM, Reg. AEMS, CUACE, NAQMPS…… Source apportionment model: CAMXPSAT/OSAT, Reg. AEMS-CMB/PMF…… n Chemical data assimilation: OA, 3 DVAR, Enk. F…. . n Model output statistics: Kalman filter, Regression
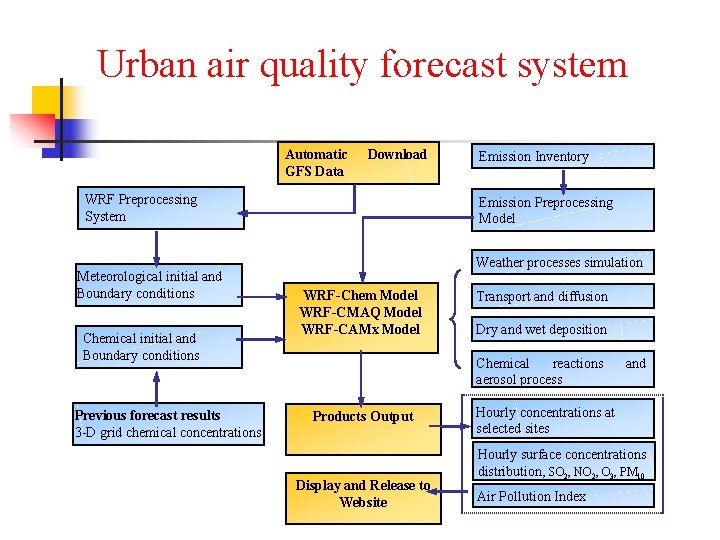
Urban air quality forecast system Automatic GFS Data Download WRF Preprocessing System Meteorological initial and Boundary conditions Chemical initial and Boundary conditions Previous forecast results 3 -D grid chemical concentrations Emission Inventory Emission Preprocessing Model Weather processes simulation WRF-Chem Model WRF-CMAQ Model WRF-CAMx Model Transport and diffusion Dry and wet deposition Chemical reactions aerosol process Products Output Display and Release to Website and Hourly concentrations at selected sites Hourly surface concentrations distribution, SO 2, NO 2, O 3, PM 10 Air Pollution Index
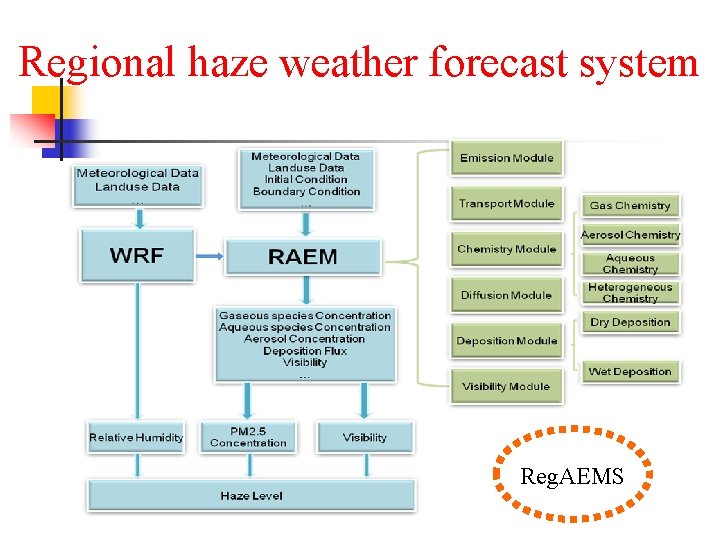
Regional haze weather forecast system Reg. AEMS
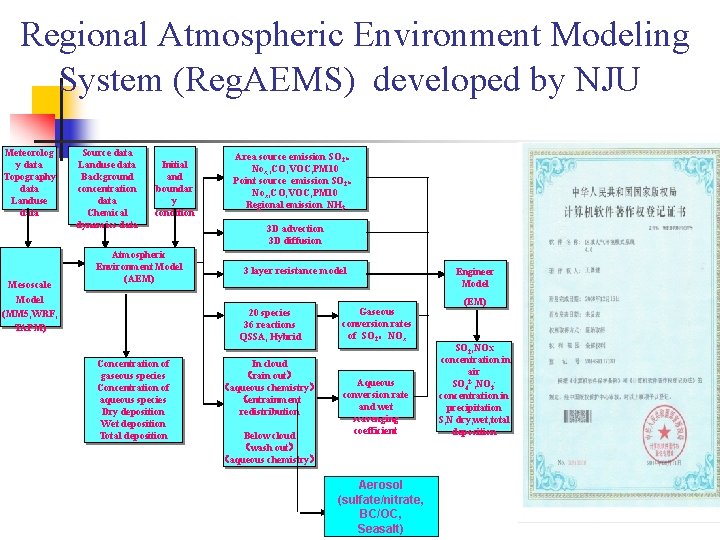
Regional Atmospheric Environment Modeling System (Reg. AEMS) developed by NJU Meteorolog y data Topography data Landuse data Mesoscale Model (MM 5, WRF, TAPM) Source data Landuse data Background concentration data Chemical dynamics data Initial and boundar y condition Atmospheric Environment Model (AEM) Area source emission SO 2、 Nox, , CO, VOC, PM 10 Point source emission SO 2、 Nox, CO, VOC, PM 10 Regional emission NH 3 3 D advection 3 D diffusion 3 layer resistance model 20 species 36 reactions QSSA, Hybrid Concentration of gaseous species Concentration of aqueous species Dry deposition Wet deposition Total deposition In cloud (rain out) (aqueous chemistry) (entrainment redistribution Below cloud (wash out) (aqueous chemistry) Gaseous conversion rates of SO 2、NOx Aqueous conversion rate and wet scavenging coefficient Aerosol (sulfate/nitrate, BC/OC, Seasalt) Engineer Model (EM) SO 2, NOx concentration in air SO 42 -, NO 3 concentration in precipitation S, N dry, wet, total deposition
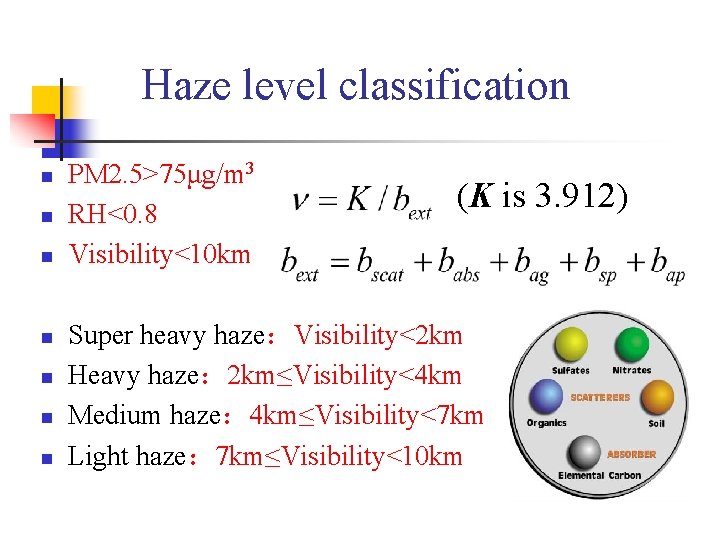
Haze level classification n n n PM 2. 5>75μg/m 3 RH<0. 8 Visibility<10 km (K is 3. 912) Super heavy haze:Visibility<2 km Heavy haze: 2 km≤Visibility<4 km Medium haze: 4 km≤Visibility<7 km Light haze: 7 km≤Visibility<10 km
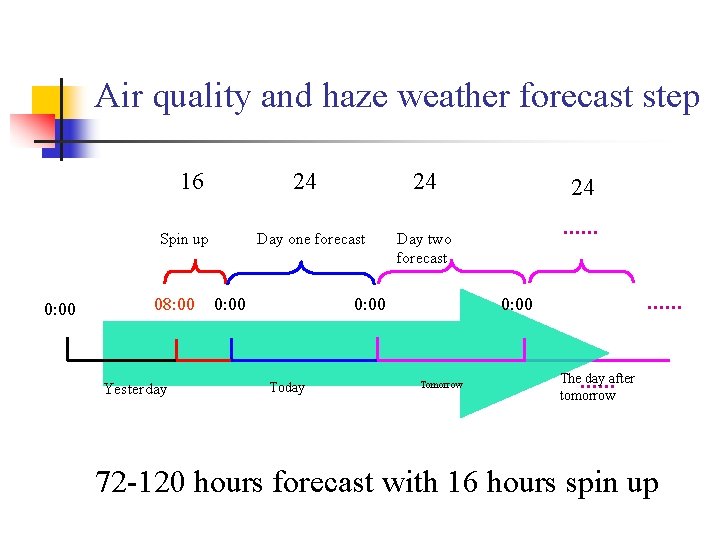
Air quality and haze weather forecast step 16 24 Spin up 0: 00 08: 00 Yesterday 24 Day one forecast 0: 00 …… Day two forecast 0: 00 Today 24 …… 0: 00 Tomorrow The day after …… tomorrow 72 -120 hours forecast with 16 hours spin up
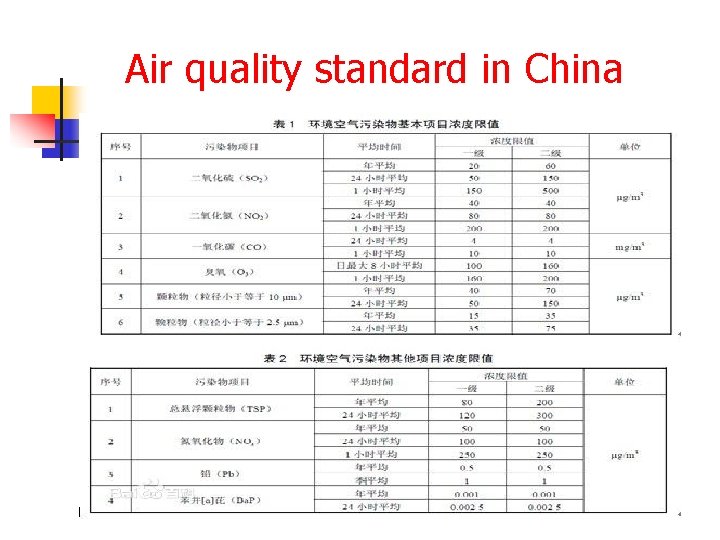
Air quality standard in China
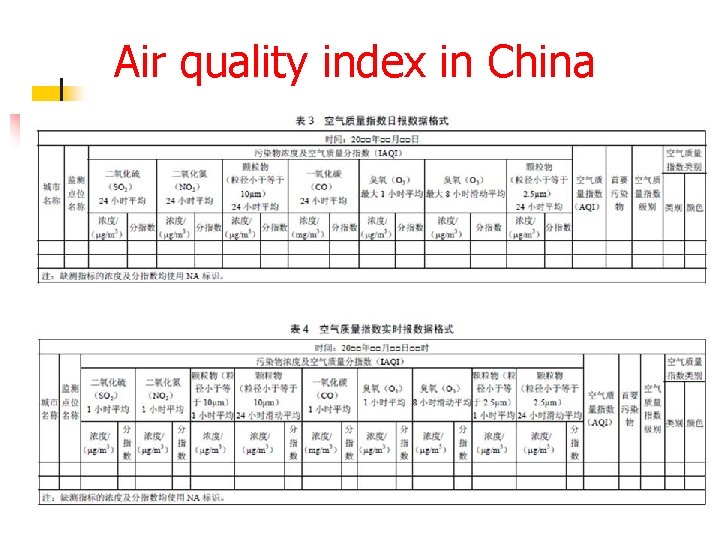
Air quality index in China
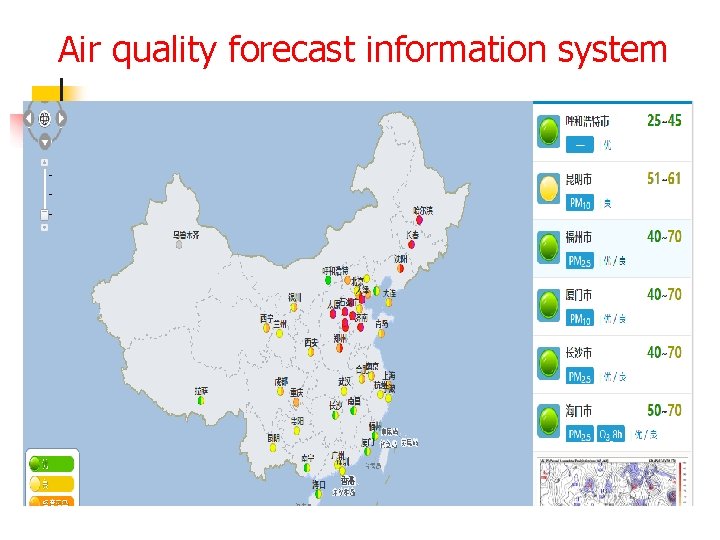
Air quality forecast information system
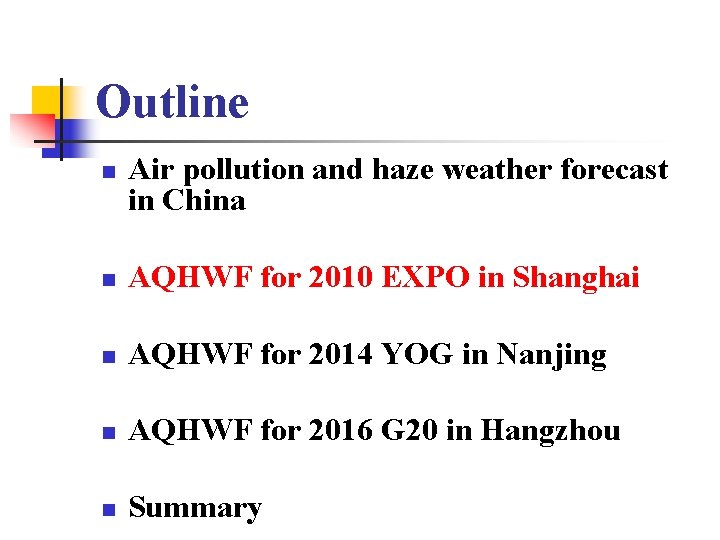
Outline n Air pollution and haze weather forecast in China n AQHWF for 2010 EXPO in Shanghai n AQHWF for 2014 YOG in Nanjing n AQHWF for 2016 G 20 in Hangzhou n Summary
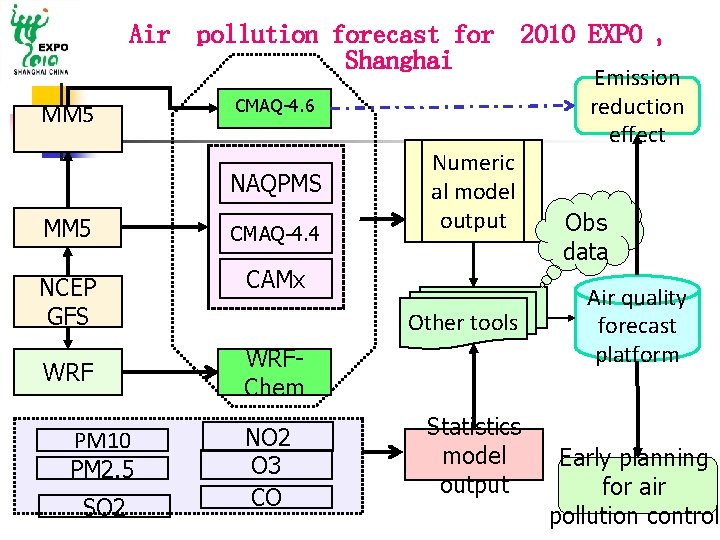
Air MM 5 pollution forecast for 2010 EXPO , Shanghai CMAQ-4. 6 NAQPMS MM 5 CMAQ-4. 4 NCEP GFS CAMx WRF PM 10 PM 2. 5 SO 2 Numeric al model output Other tools WRFChem NO 2 O 3 CO Statistics model output Emission reduction effect Obs data Air quality forecast platform Early planning for air pollution control
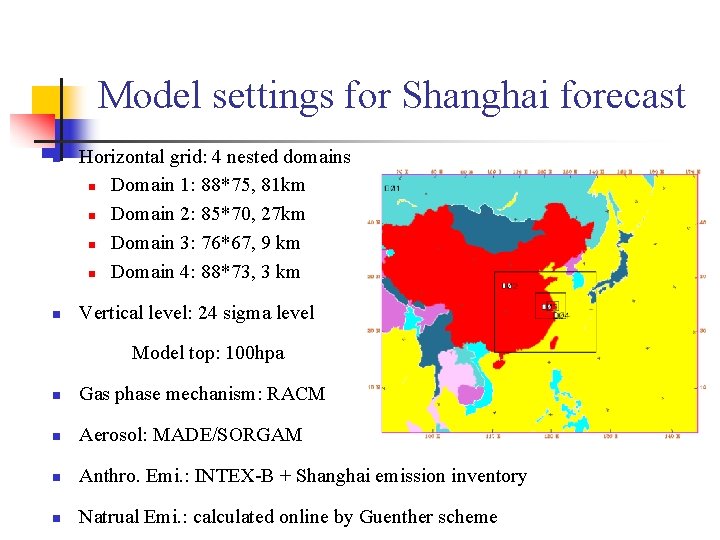
Model settings for Shanghai forecast n n Horizontal grid: 4 nested domains n Domain 1: 88*75, 81 km n Domain 2: 85*70, 27 km n Domain 3: 76*67, 9 km n Domain 4: 88*73, 3 km Vertical level: 24 sigma level Model top: 100 hpa n Gas phase mechanism: RACM n Aerosol: MADE/SORGAM n Anthro. Emi. : INTEX-B + Shanghai emission inventory n Natrual Emi. : calculated online by Guenther scheme
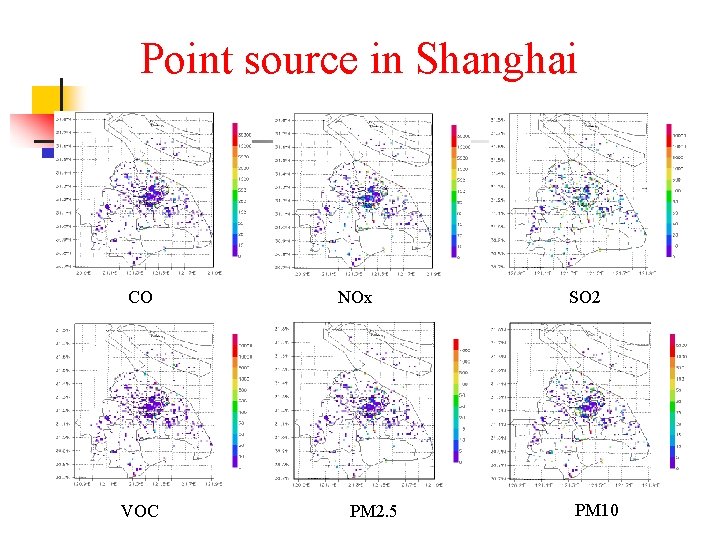
Point source in Shanghai CO VOC NOx PM 2. 5 SO 2 PM 10
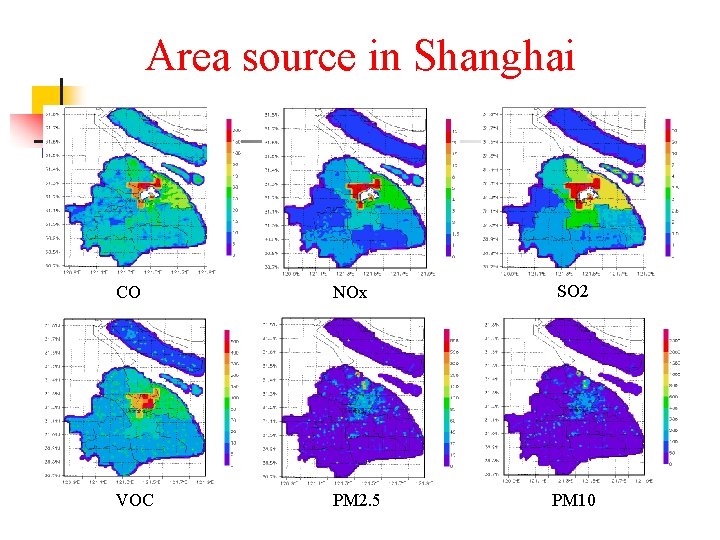
Area source in Shanghai CO NOx VOC PM 2. 5 SO 2 PM 10
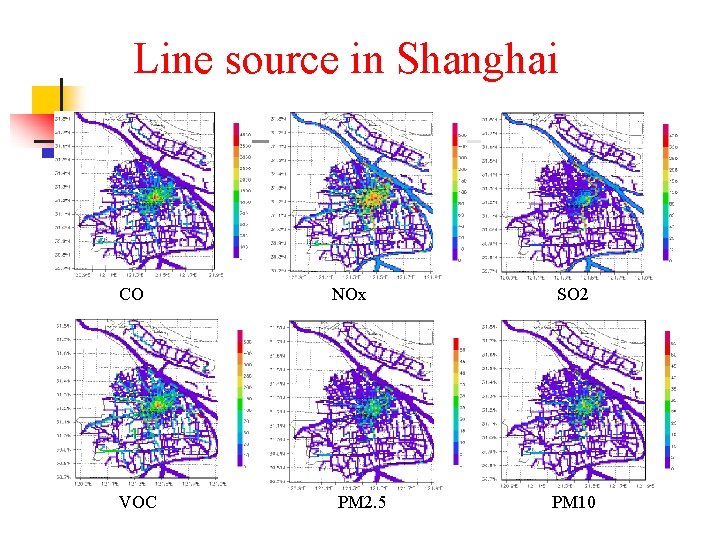
Line source in Shanghai CO VOC NOx PM 2. 5 SO 2 PM 10
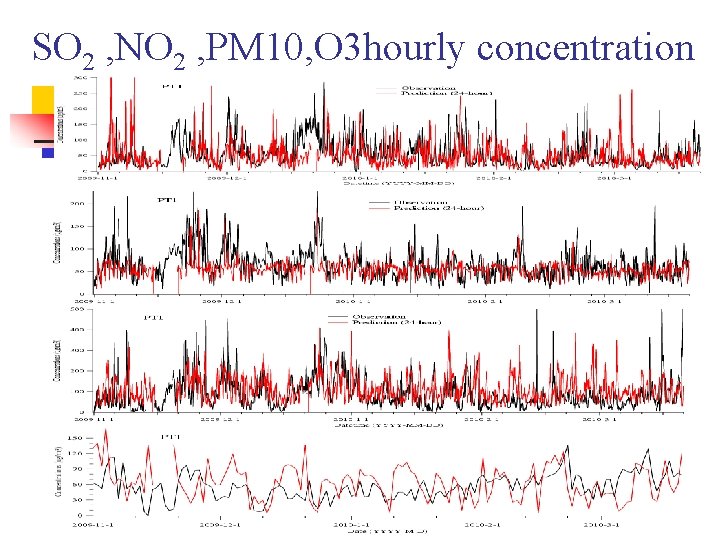
SO 2 , NO 2 , PM 10, O 3 hourly concentration
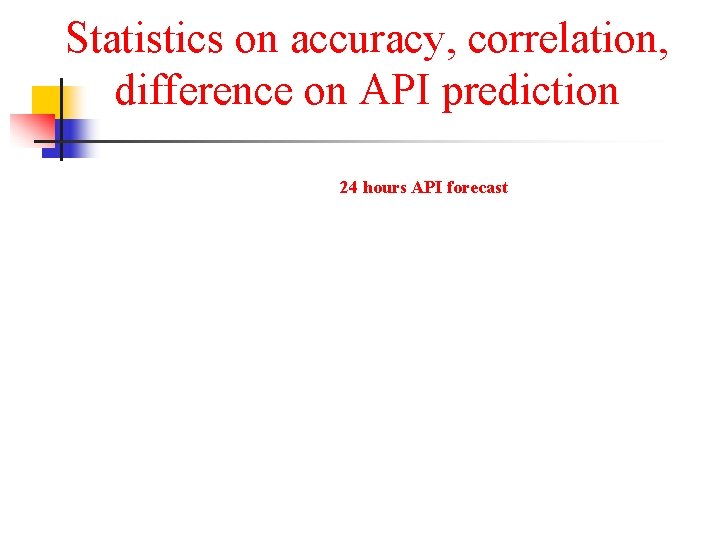
Statistics on accuracy, correlation, difference on API prediction 24 hours API forecast
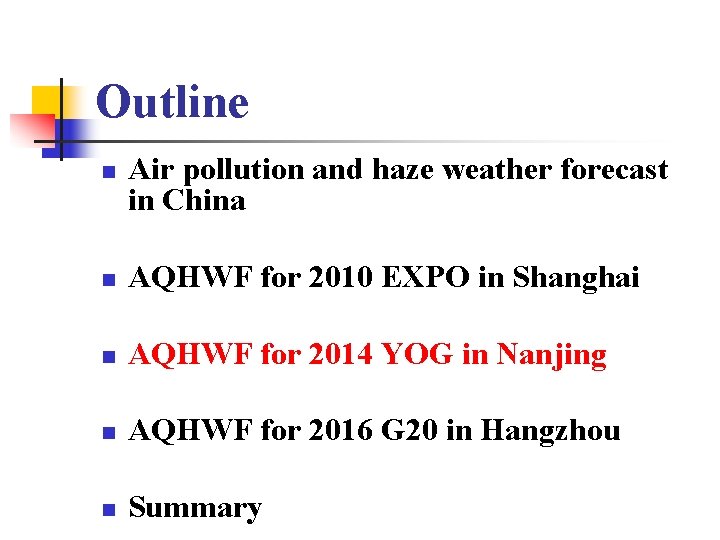
Outline n Air pollution and haze weather forecast in China n AQHWF for 2010 EXPO in Shanghai n AQHWF for 2014 YOG in Nanjing n AQHWF for 2016 G 20 in Hangzhou n Summary
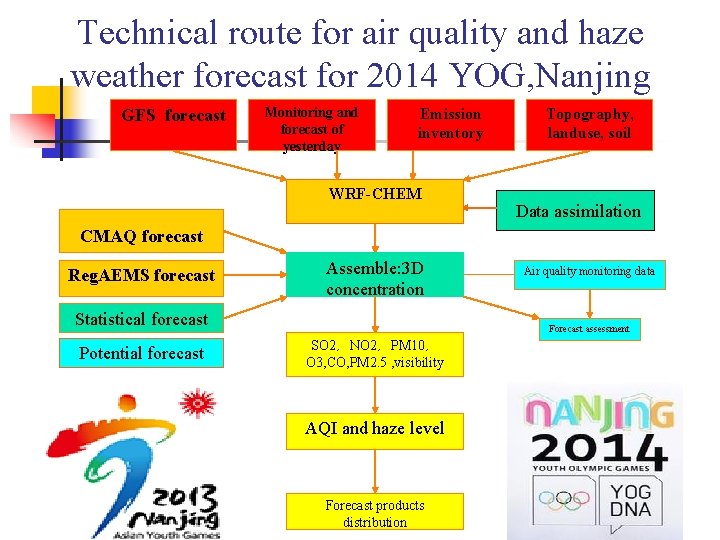
Technical route for air quality and haze weather forecast for 2014 YOG, Nanjing GFS forecast Monitoring and forecast of yesterday Emission inventory WRF-CHEM Topography, landuse, soil Data assimilation CMAQ forecast Reg. AEMS forecast Assemble: 3 D concentration Forecast from statistical Statistical forecast Potential forecast Air quality monitoring data Forecast assessment SO 2,NO 2,PM 10, O 3, CO, PM 2. 5 , visibility AQI and haze level Forecast products distribution
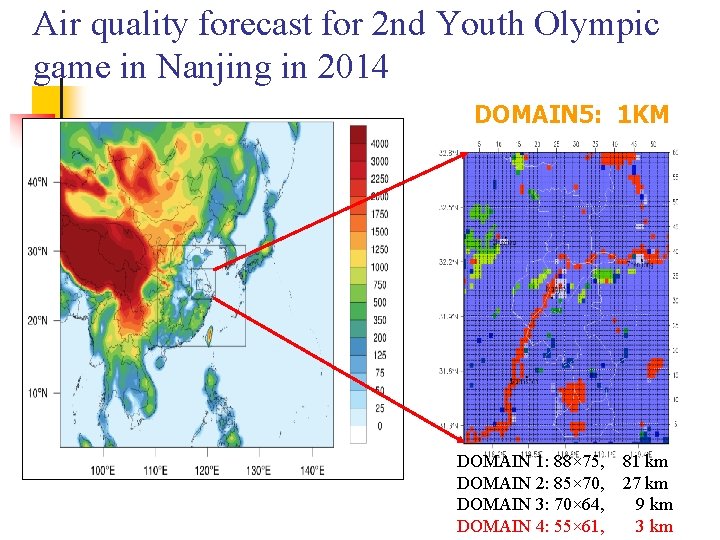
Air quality forecast for 2 nd Youth Olympic game in Nanjing in 2014 DOMAIN 5: 1 KM DOMAIN 1: 88× 75, 81 km DOMAIN 2: 85× 70, 27 km DOMAIN 3: 70× 64, 9 km DOMAIN 4: 55× 61, 3 km
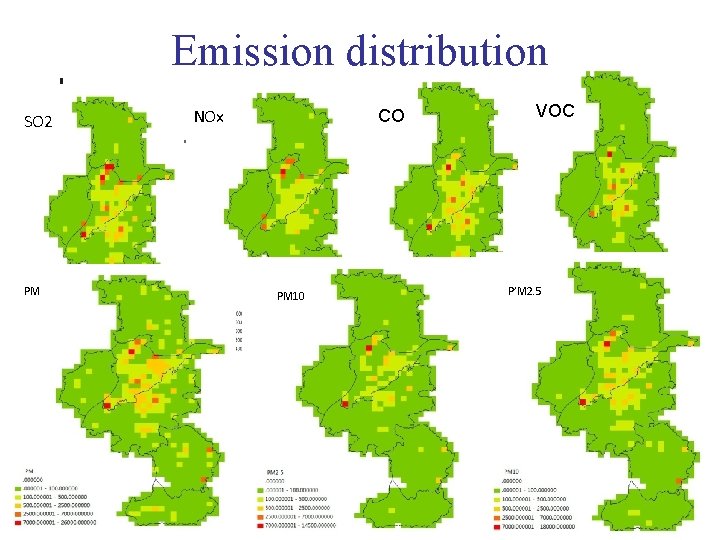
Emission distribution SOSO 2 2 PM NOx CO PM 10 VOC P’M 2. 5
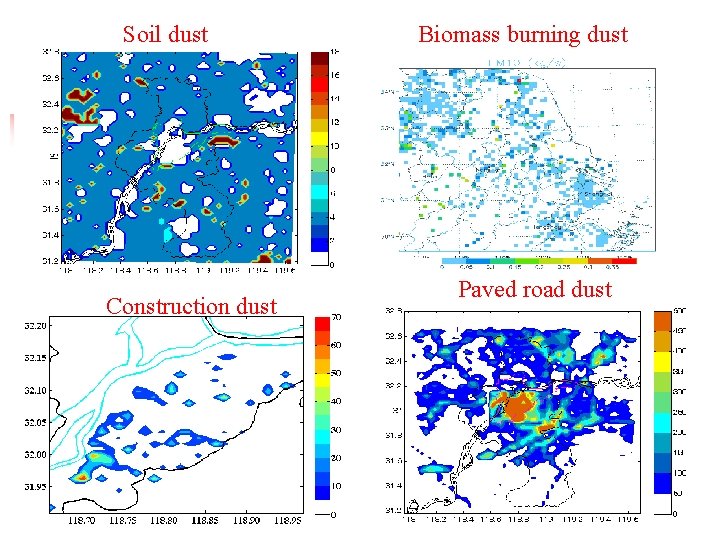
Soil dust Construction dust Biomass burning dust Paved road dust
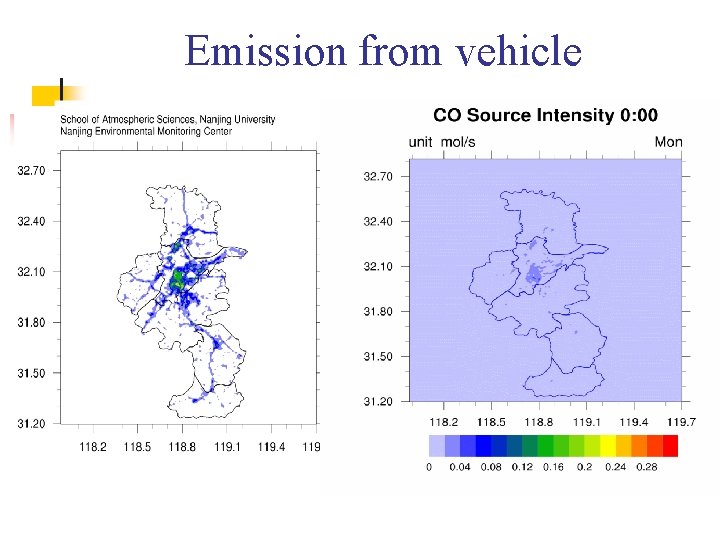
Emission from vehicle
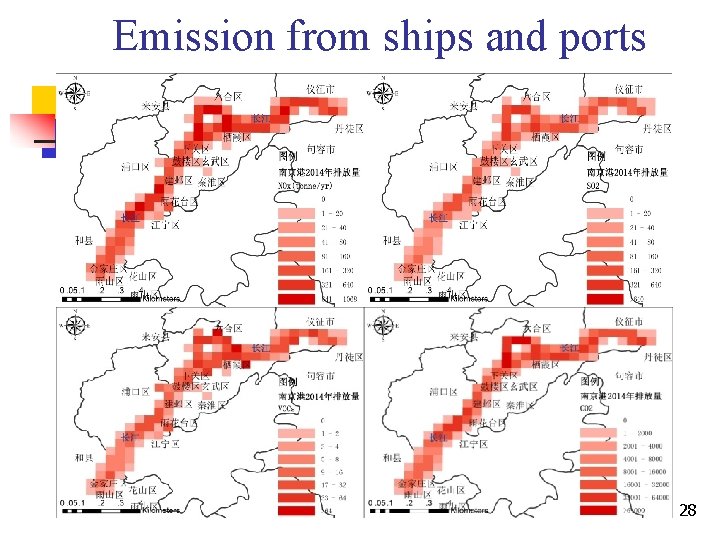
Emission from ships and ports 28
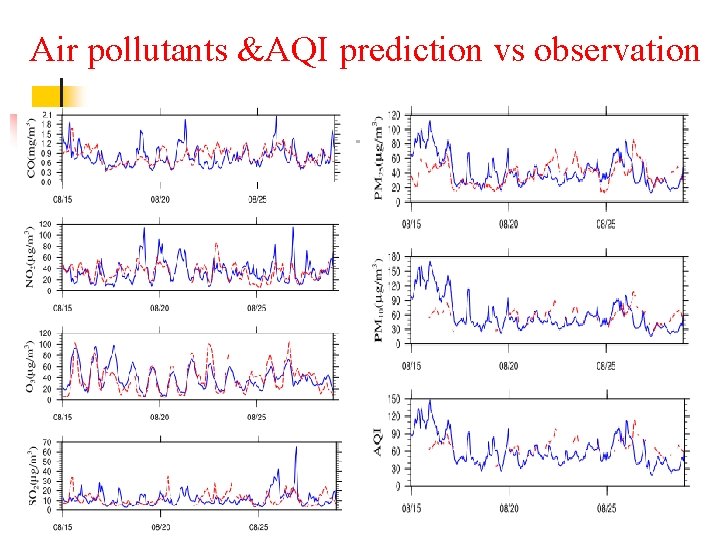
Air pollutants &AQI prediction vs observation
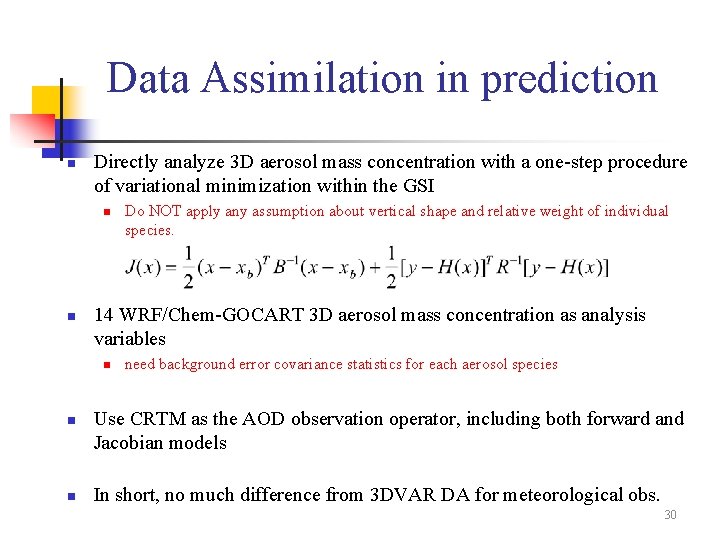
Data Assimilation in prediction n Directly analyze 3 D aerosol mass concentration with a one-step procedure of variational minimization within the GSI n n 14 WRF/Chem-GOCART 3 D aerosol mass concentration as analysis variables n n n Do NOT apply any assumption about vertical shape and relative weight of individual species. need background error covariance statistics for each aerosol species Use CRTM as the AOD observation operator, including both forward and Jacobian models In short, no much difference from 3 DVAR DA for meteorological obs. 30
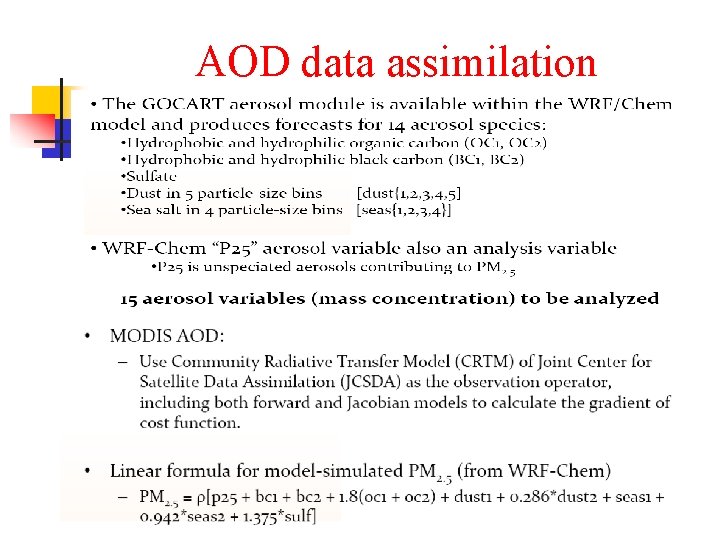
AOD data assimilation
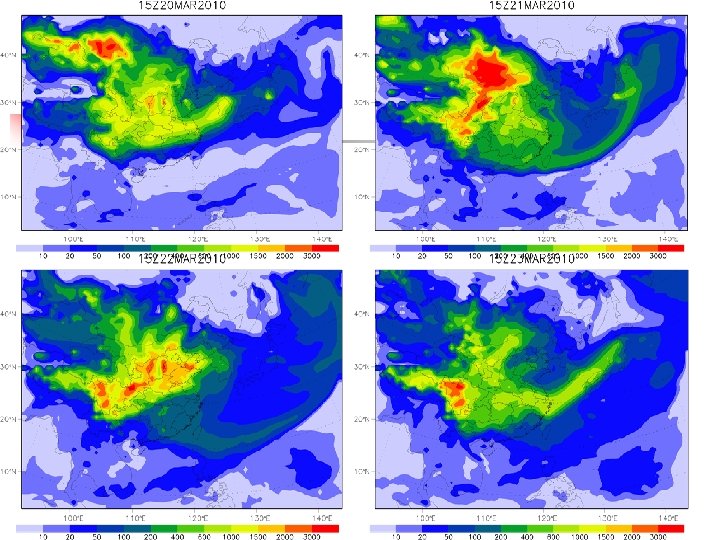
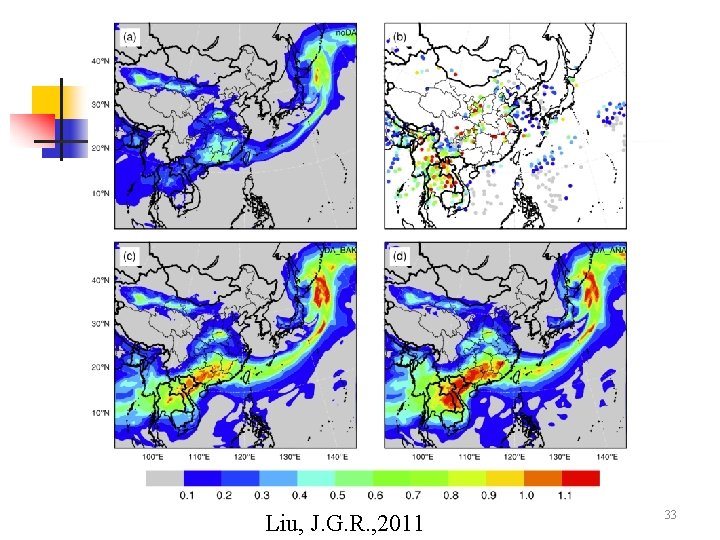
Liu, J. G. R. , 2011 33
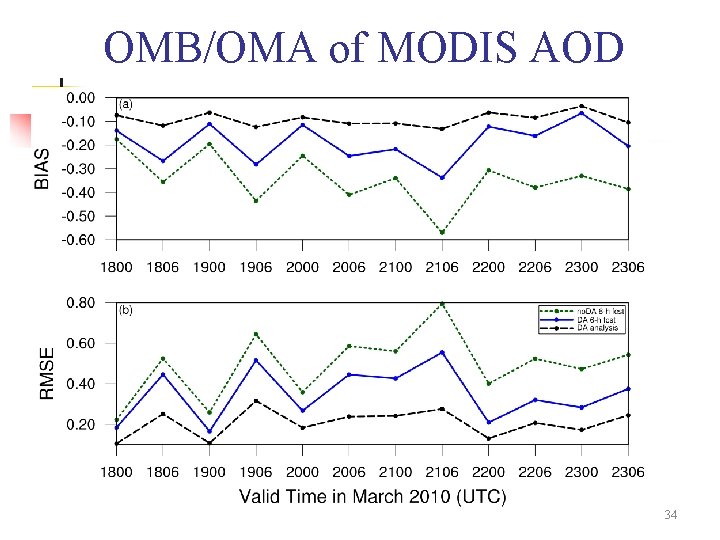
OMB/OMA of MODIS AOD 34
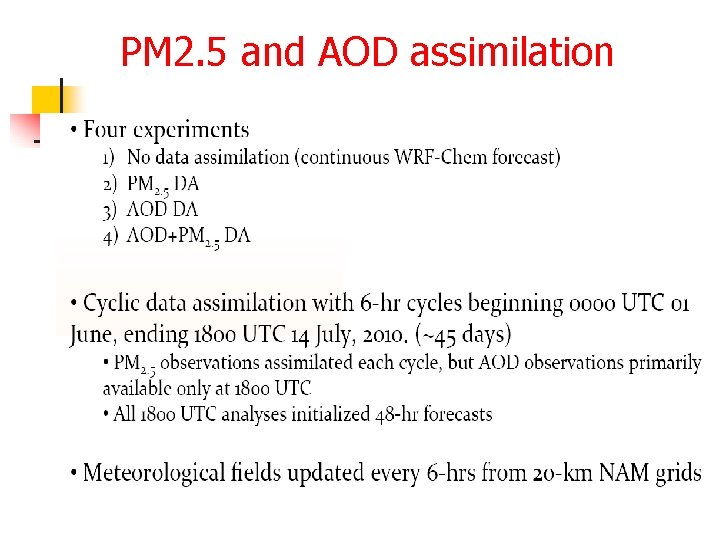
PM 2. 5 and AOD assimilation
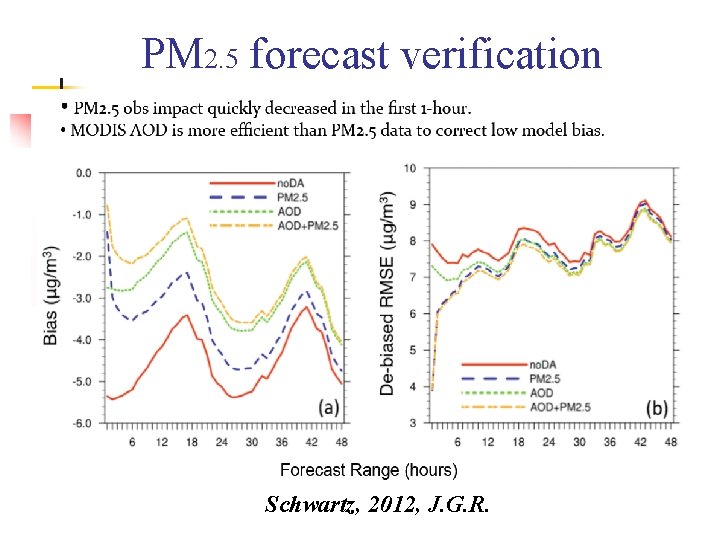
PM 2. 5 forecast verification Schwartz, 2012, J. G. R.
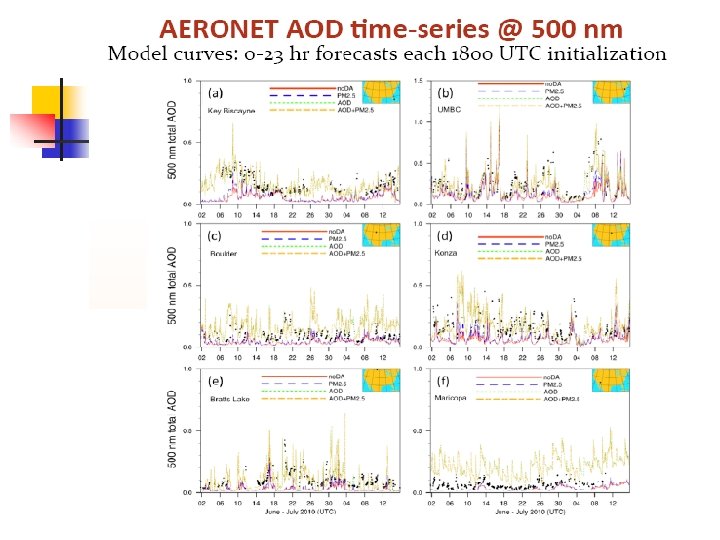
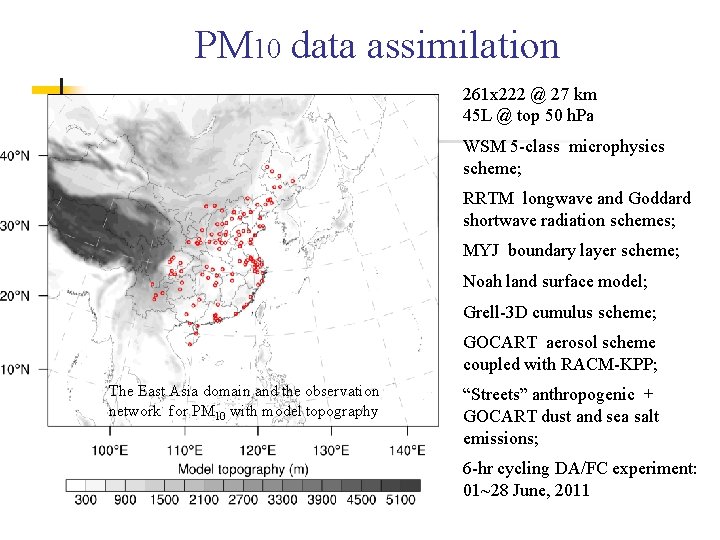
PM 10 data assimilation 261 x 222 @ 27 km 45 L @ top 50 h. Pa WSM 5 -class microphysics scheme; RRTM longwave and Goddard shortwave radiation schemes; MYJ boundary layer scheme; Noah land surface model; Grell-3 D cumulus scheme; GOCART aerosol scheme coupled with RACM-KPP; The East Asia domain and the observation network for PM 10 with model topography “Streets” anthropogenic + GOCART dust and sea salt emissions; 6 -hr cycling DA/FC experiment: 01~28 June, 2011
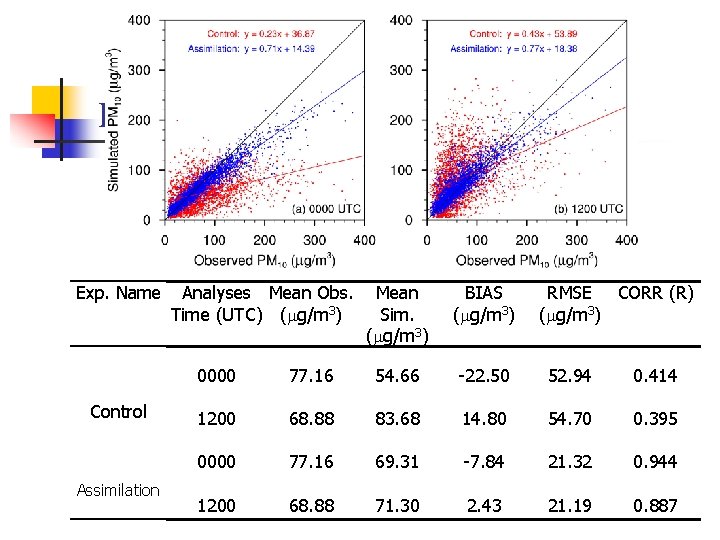
Impacts on aerosol ICs Exp. Name Control Assimilation Analyses Mean Obs. Time (UTC) ( g/m 3) Mean Sim. ( g/m 3) BIAS ( g/m 3) RMSE CORR (R) ( g/m 3) 0000 77. 16 54. 66 -22. 50 52. 94 0. 414 1200 68. 88 83. 68 14. 80 54. 70 0. 395 0000 77. 16 69. 31 -7. 84 21. 32 0. 944 1200 68. 88 71. 30 2. 43 21. 19 0. 887
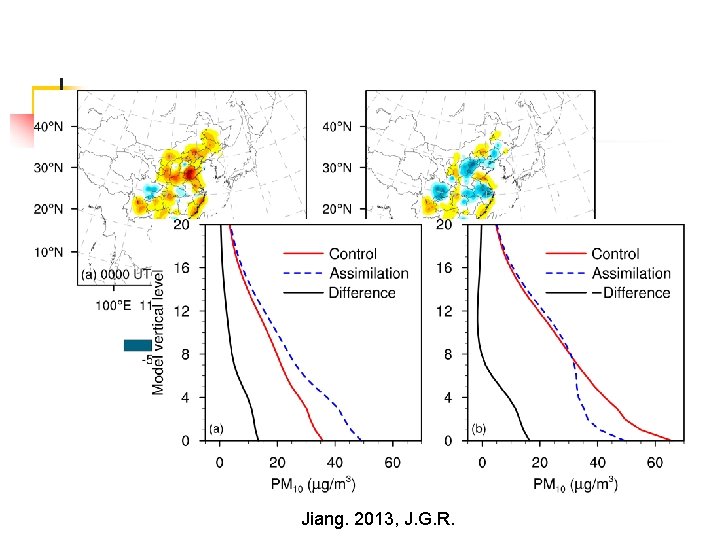
Impacts on aerosol ICs difference = assimilation minus control Jiang. 2013, J. G. R.
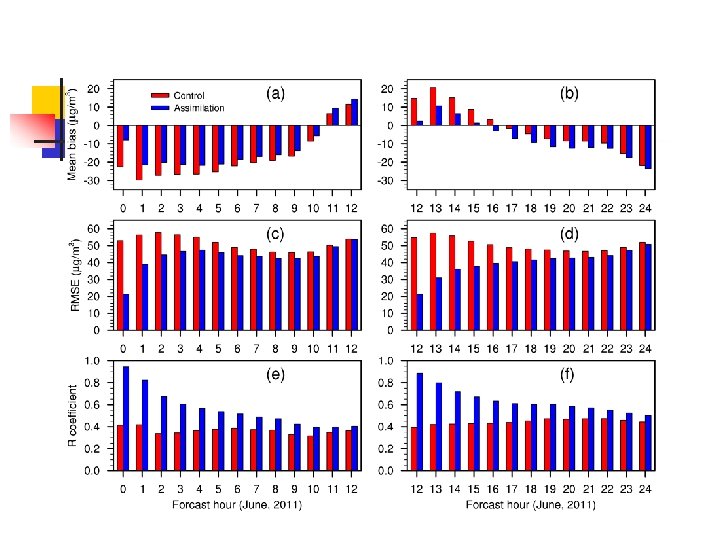
Forecast verification
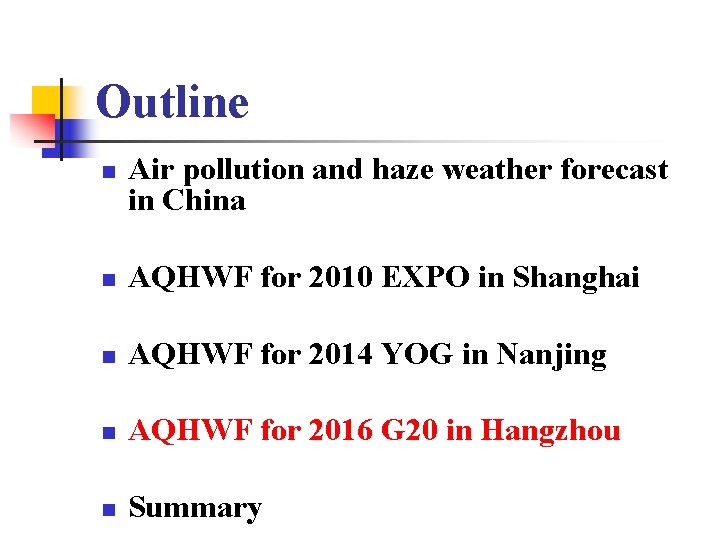
Outline n Air pollution and haze weather forecast in China n AQHWF for 2010 EXPO in Shanghai n AQHWF for 2014 YOG in Nanjing n AQHWF for 2016 G 20 in Hangzhou n Summary
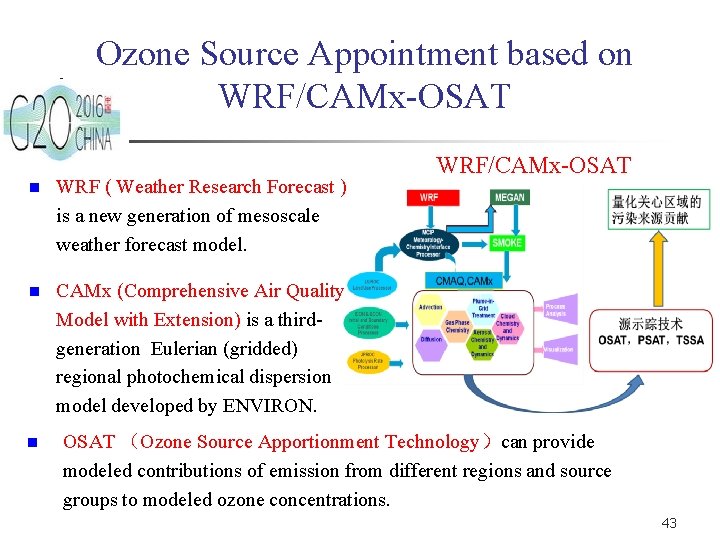
Ozone Source Appointment based on WRF/CAMx-OSAT n WRF ( Weather Research Forecast ) is a new generation of mesoscale weather forecast model. n CAMx (Comprehensive Air Quality Model with Extension) is a thirdgeneration Eulerian (gridded) regional photochemical dispersion model developed by ENVIRON. n WRF/CAMx-OSAT (Ozone Source Apportionment Technology)can provide modeled contributions of emission from different regions and source groups to modeled ozone concentrations. 43
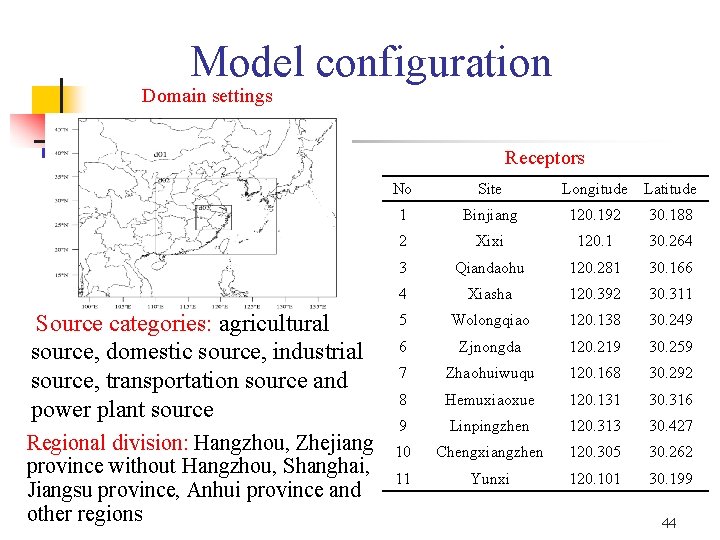
Model configuration Domain settings Receptors Source categories: agricultural source, domestic source, industrial source, transportation source and power plant source Regional division: Hangzhou, Zhejiang province without Hangzhou, Shanghai, Jiangsu province, Anhui province and other regions No Site Longitude Latitude 1 Binjiang 120. 192 30. 188 2 Xixi 120. 1 30. 264 3 Qiandaohu 120. 281 30. 166 4 Xiasha 120. 392 30. 311 5 Wolongqiao 120. 138 30. 249 6 Zjnongda 120. 219 30. 259 7 Zhaohuiwuqu 120. 168 30. 292 8 Hemuxiaoxue 120. 131 30. 316 9 Linpingzhen 120. 313 30. 427 10 Chengxiangzhen 120. 305 30. 262 11 Yunxi 120. 101 30. 199 44
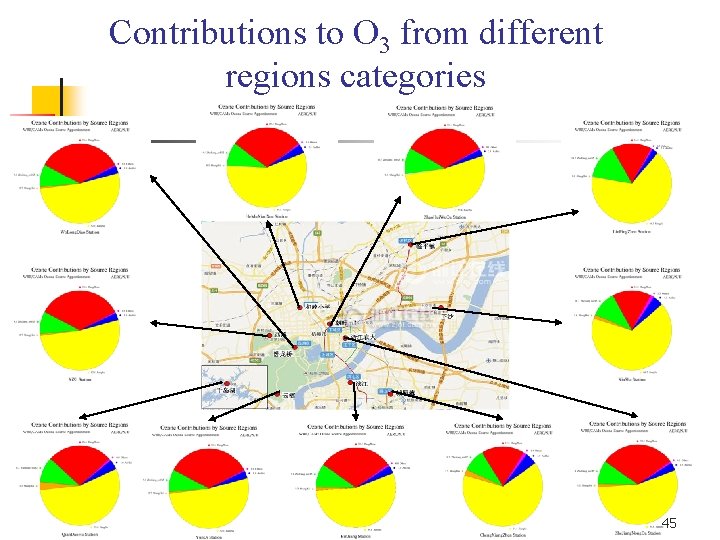
Contributions to O 3 from different regions categories 45
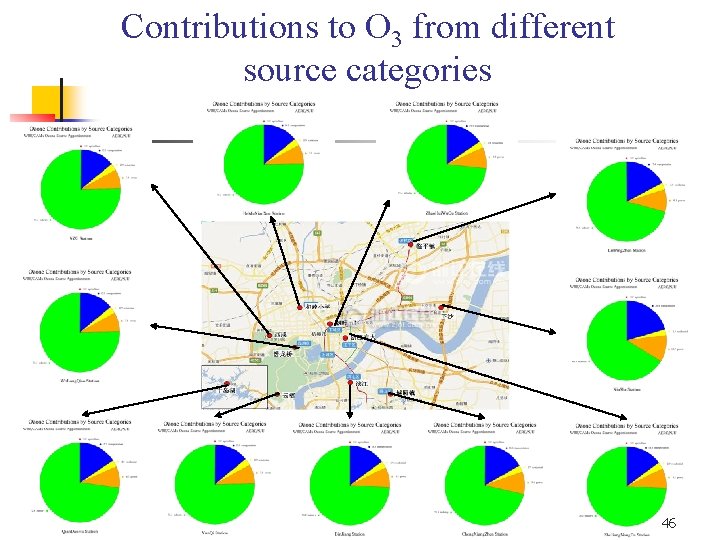
Contributions to O 3 from different source categories 46
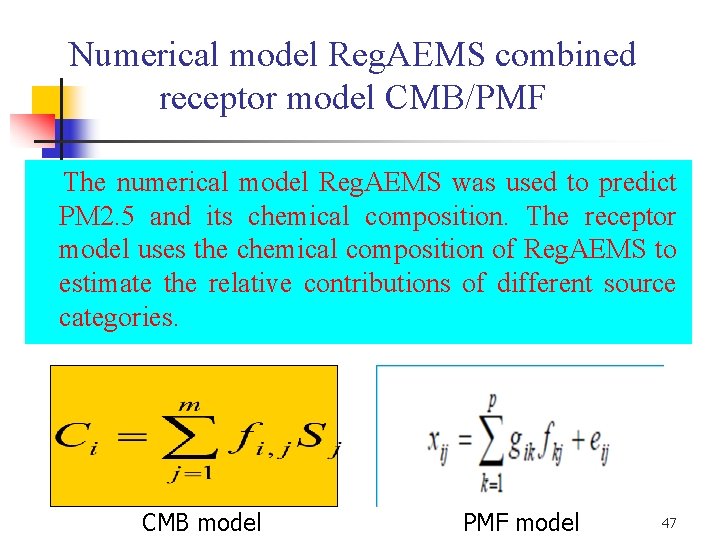
Numerical model Reg. AEMS combined receptor model CMB/PMF The numerical model Reg. AEMS was used to predict PM 2. 5 and its chemical composition. The receptor model uses the chemical composition of Reg. AEMS to estimate the relative contributions of different source categories. CMB model PMF model 47
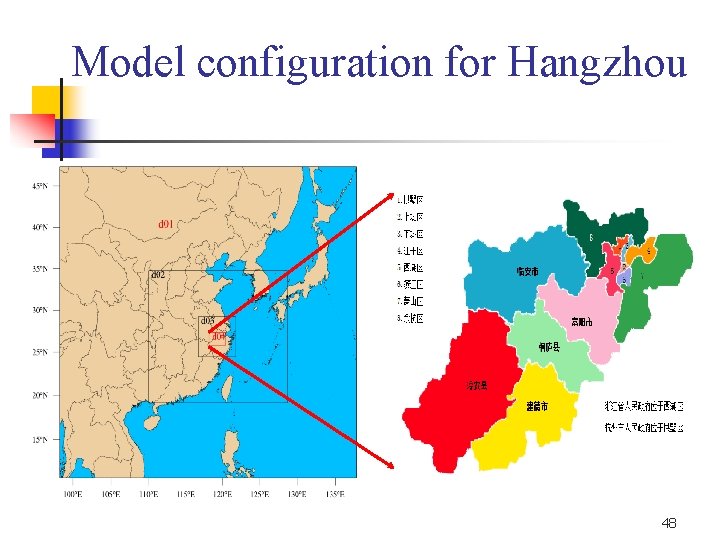
Model configuration for Hangzhou 48
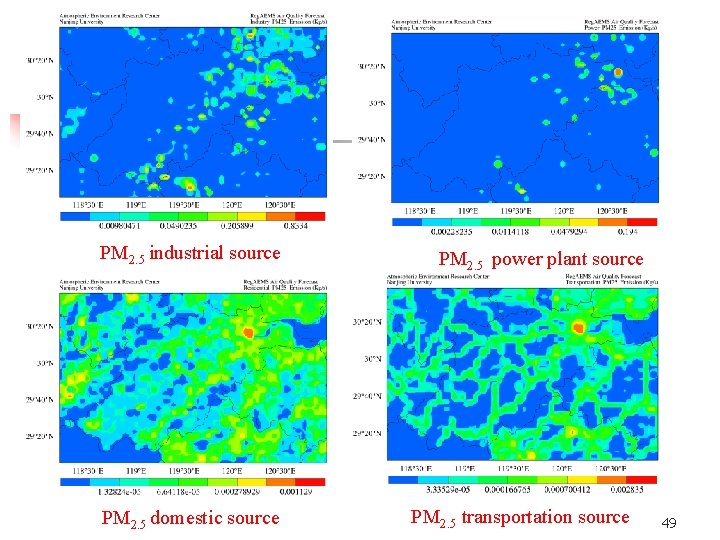
PM 2. 5 industrial source PM 2. 5 domestic source PM 2. 5 power plant source PM 2. 5 transportation source 49
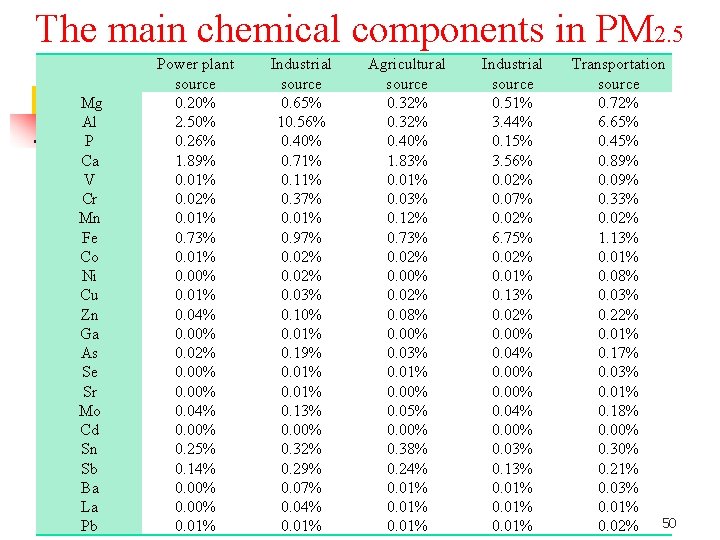
The main chemical components in PM 2. 5 Mg Al P Ca V Cr Mn Fe Co Ni Cu Zn Ga As Se Sr Mo Cd Sn Sb Ba La Pb Power plant source 0. 20% 2. 50% 0. 26% 1. 89% 0. 01% 0. 02% 0. 01% 0. 73% 0. 01% 0. 00% 0. 01% 0. 04% 0. 00% 0. 02% 0. 00% 0. 04% 0. 00% 0. 25% 0. 14% 0. 00% 0. 01% Industrial source 0. 65% 10. 56% 0. 40% 0. 71% 0. 11% 0. 37% 0. 01% 0. 97% 0. 02% 0. 03% 0. 10% 0. 01% 0. 19% 0. 01% 0. 13% 0. 00% 0. 32% 0. 29% 0. 07% 0. 04% 0. 01% Agricultural source 0. 32% 0. 40% 1. 83% 0. 01% 0. 03% 0. 12% 0. 73% 0. 02% 0. 00% 0. 02% 0. 08% 0. 00% 0. 03% 0. 01% 0. 00% 0. 05% 0. 00% 0. 38% 0. 24% 0. 01% Industrial source 0. 51% 3. 44% 0. 15% 3. 56% 0. 02% 0. 07% 0. 02% 6. 75% 0. 02% 0. 01% 0. 13% 0. 02% 0. 00% 0. 04% 0. 00% 0. 03% 0. 13% 0. 01% Transportation source 0. 72% 6. 65% 0. 45% 0. 89% 0. 09% 0. 33% 0. 02% 1. 13% 0. 01% 0. 08% 0. 03% 0. 22% 0. 01% 0. 17% 0. 03% 0. 01% 0. 18% 0. 00% 0. 30% 0. 21% 0. 03% 0. 01% 0. 02% 50
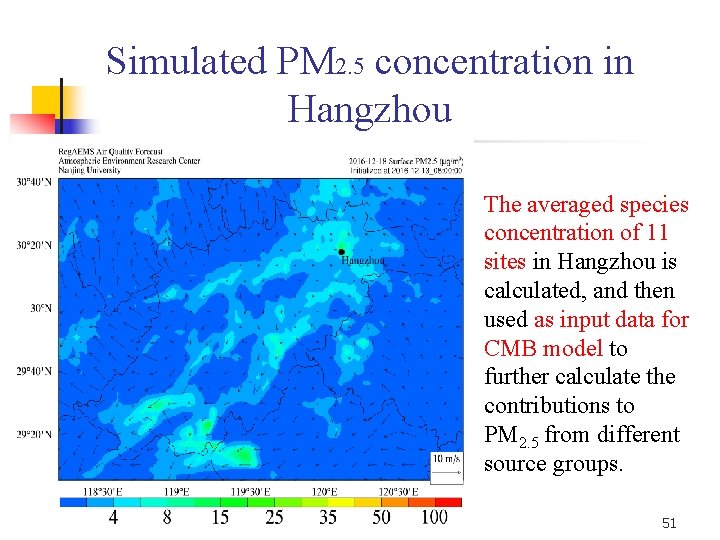
Simulated PM 2. 5 concentration in Hangzhou The averaged species concentration of 11 sites in Hangzhou is calculated, and then used as input data for CMB model to further calculate the contributions to PM 2. 5 from different source groups. 51
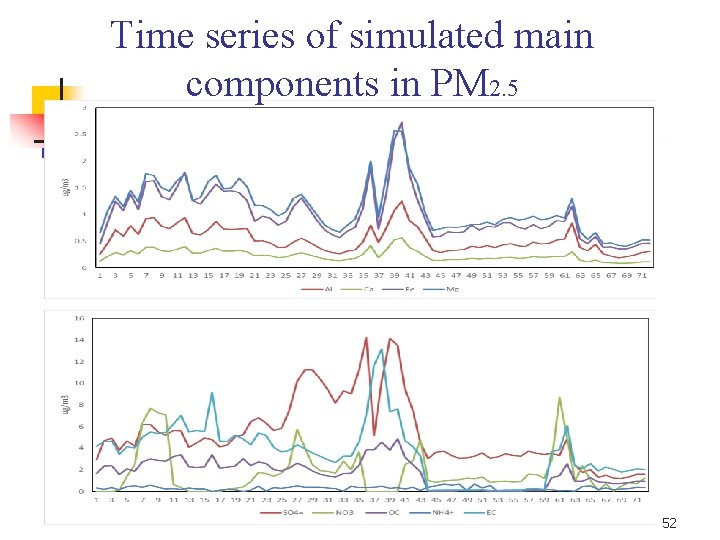
Time series of simulated main components in PM 2. 5 52
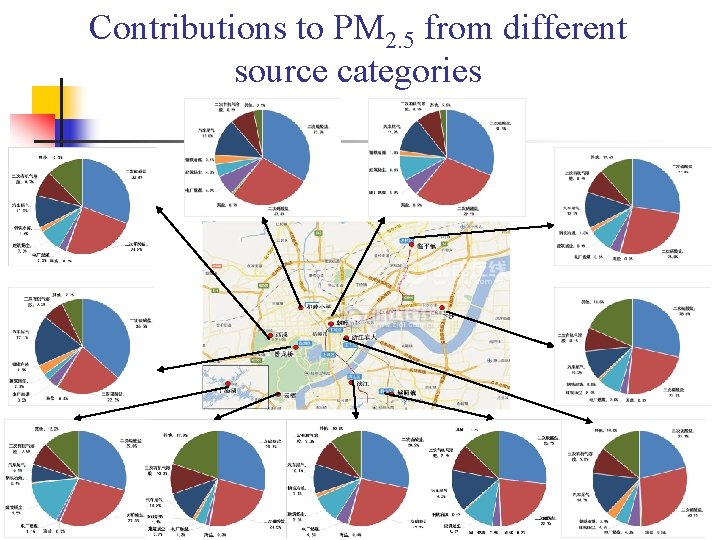
Contributions to PM 2. 5 from different source categories 53
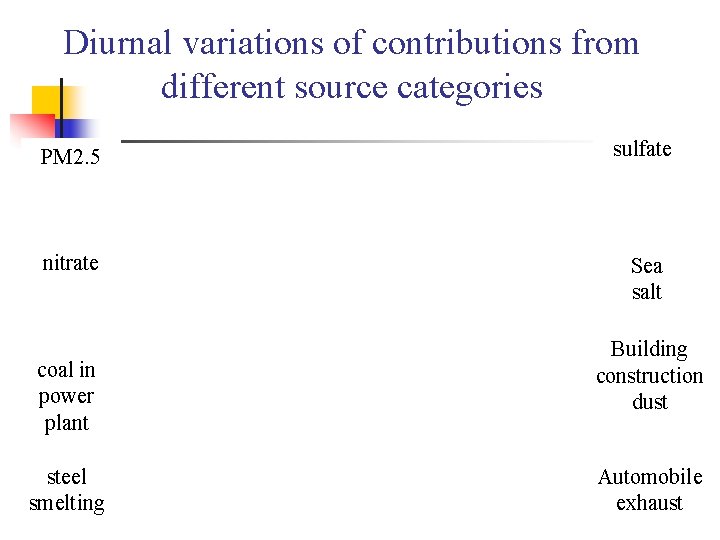
Diurnal variations of contributions from different source categories PM 2. 5 sulfate nitrate Sea salt coal in power plant steel smelting Building construction dust Automobile exhaust
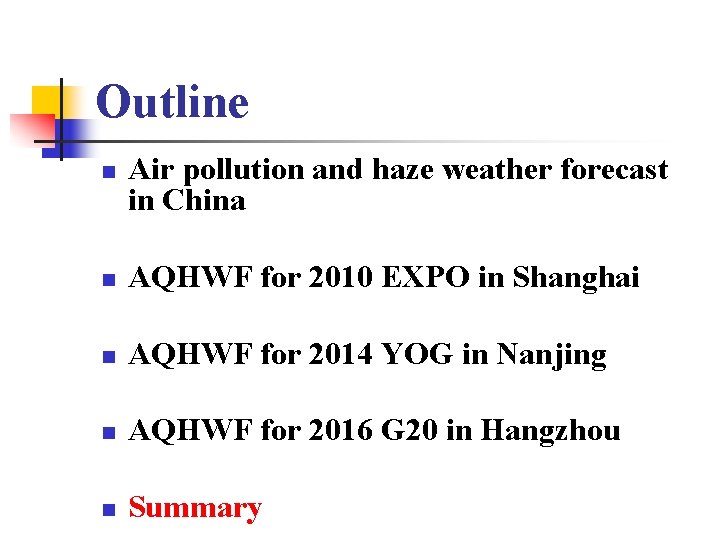
Outline n Air pollution and haze weather forecast in China n AQHWF for 2010 EXPO in Shanghai n AQHWF for 2014 YOG in Nanjing n AQHWF for 2016 G 20 in Hangzhou n Summary
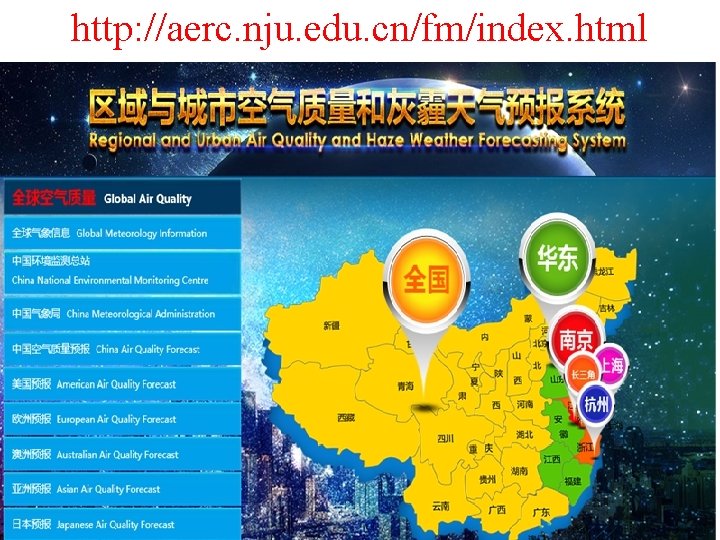
http: //aerc. nju. edu. cn/fm/index. html
The gray-brown haze often found over large cities is called
Natural haze
Single image haze removal using dark channel prior
Weather forecast words and phrases
Helicopter weather forecast
Disaster scenario media and information literacy
Weather forecast lesson 3 outline answers
Wtic weather forecast
How's the weather
Imt 556
Create your own weather forecast template
Spot weather monitor
Graphical weather forecast
Governing equation
Weather forecast udumalpet next 14 days
Millersville university weather forecast
Southern hemisphere weather forecast
Weather in ubc
Predictive services weather forecast products
Air higroskopis air kapiler dan air gravitasi
Weather station symbols
Tongue twister spring
What's the weather poem
Noun of windy
Whether the weather is fine or whether the weather is not
Heavy weather by weather report
Capital weather gang weather wall
Perform quality assurance
Basic concepts of quality
Indirect control of single acting cylinder
Return air and supply air
Flow control valve direction
Supply air throttling in pneumatic system
An instrument used to measure temperature
Why does low air pressure usually indicate stormy weather
Plan quality management pmp
Pmbok quality management
Quality assurance cycle in nursing
Quality improvement vs quality assurance
David a garvin 8 dimensions of quality
Quality is free: the art of making quality certain
Old quality vs new quality
Formuö
Typiska novell drag
Nationell inriktning för artificiell intelligens
Vad står k.r.å.k.a.n för
Varför kallas perioden 1918-1939 för mellankrigstiden
En lathund för arbete med kontinuitetshantering
Underlag för särskild löneskatt på pensionskostnader
Personlig tidbok fylla i
Sura för anatom
Vad är densitet
Datorkunskap för nybörjare
Boverket ka
Hur skriver man en tes
Delegerande ledarskap
Nyckelkompetenser för livslångt lärande