SWISS Score Nice Graphical Introduction SWISS Score Toy
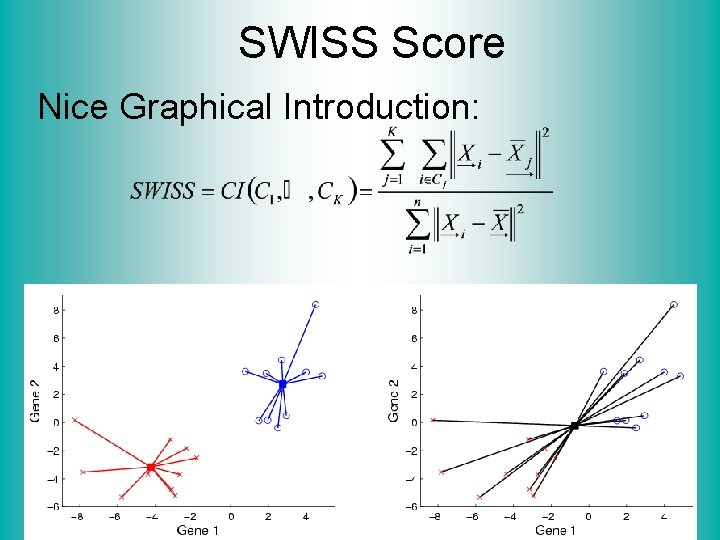
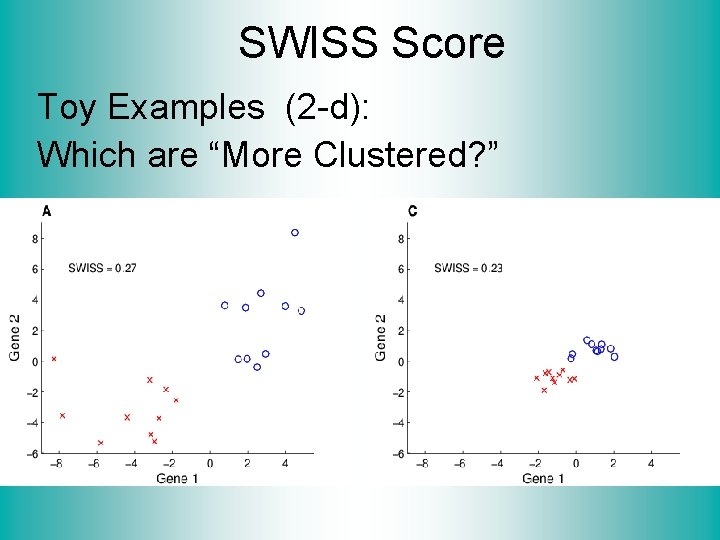
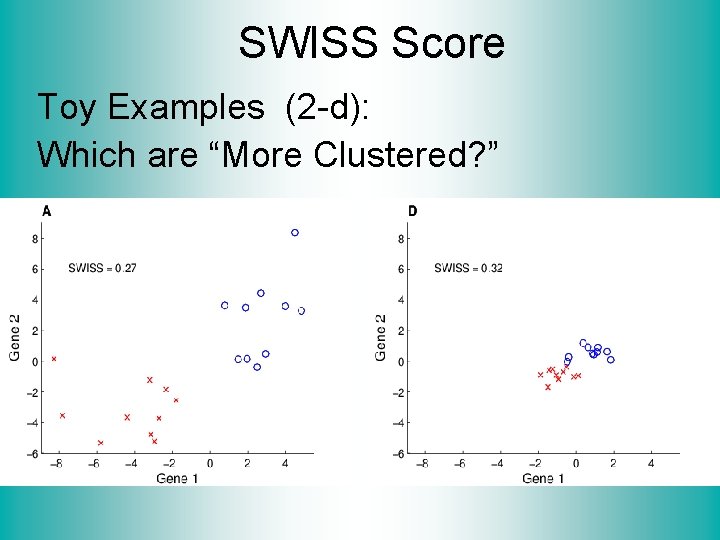
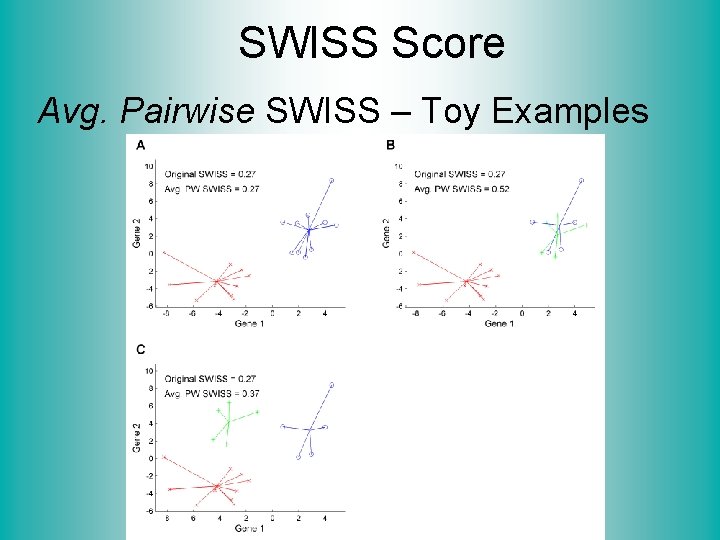
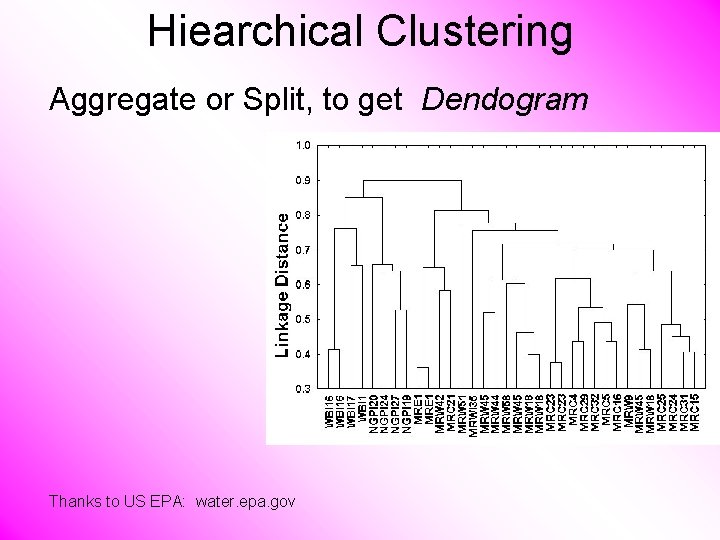
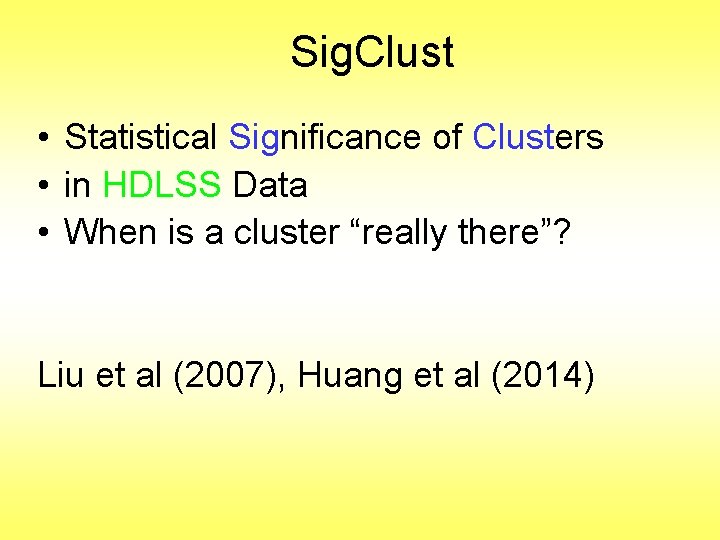
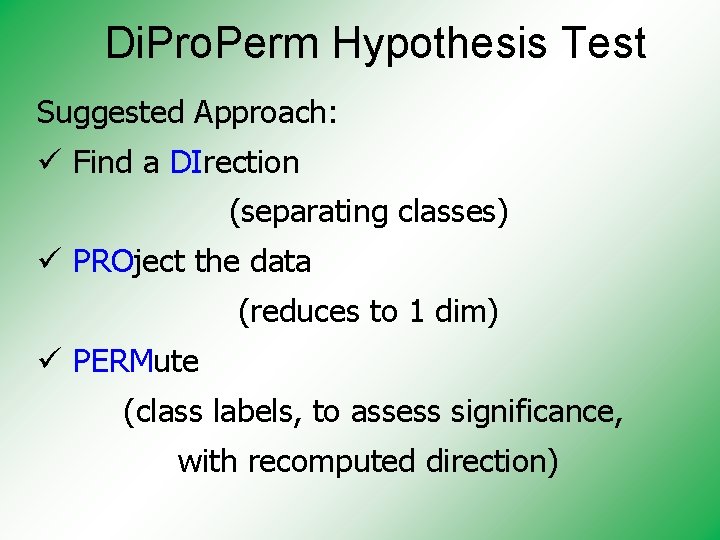
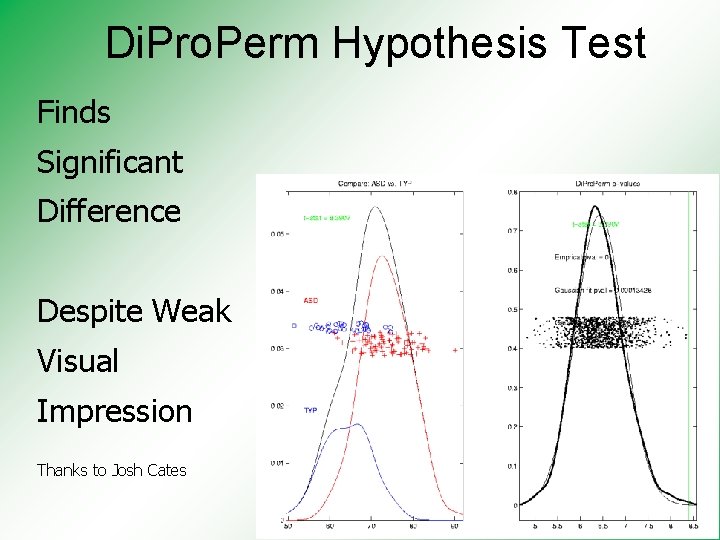
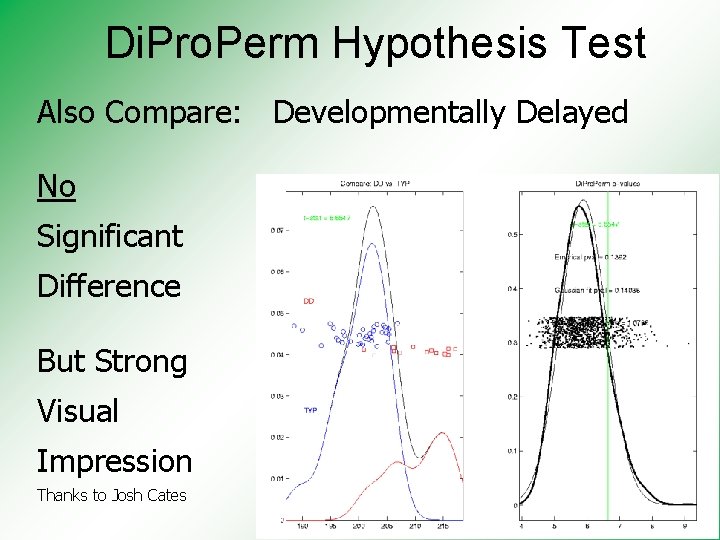
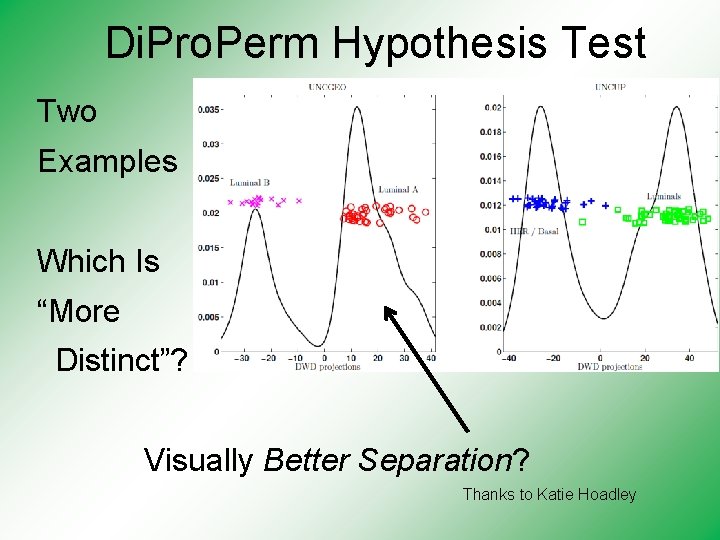
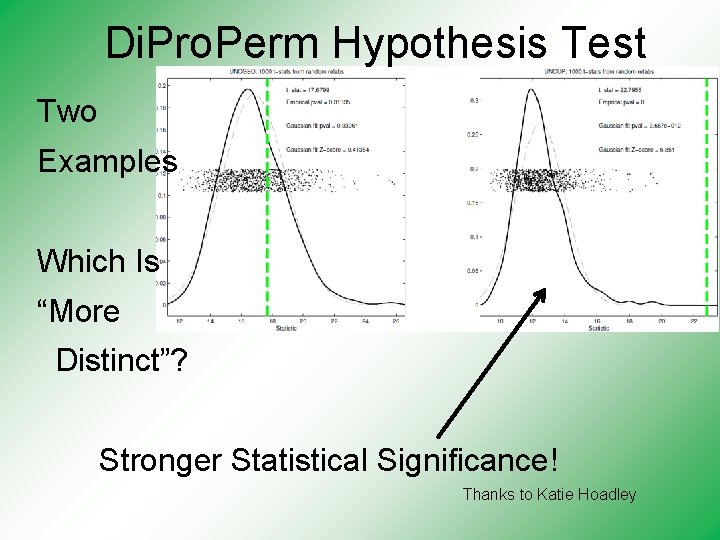
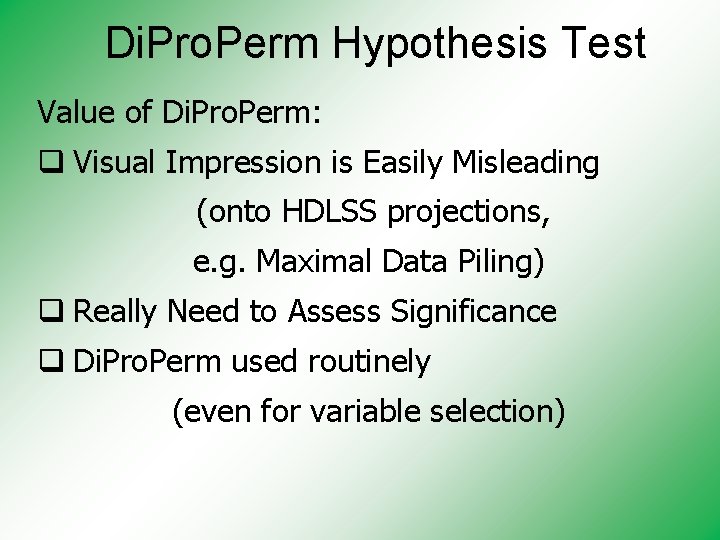
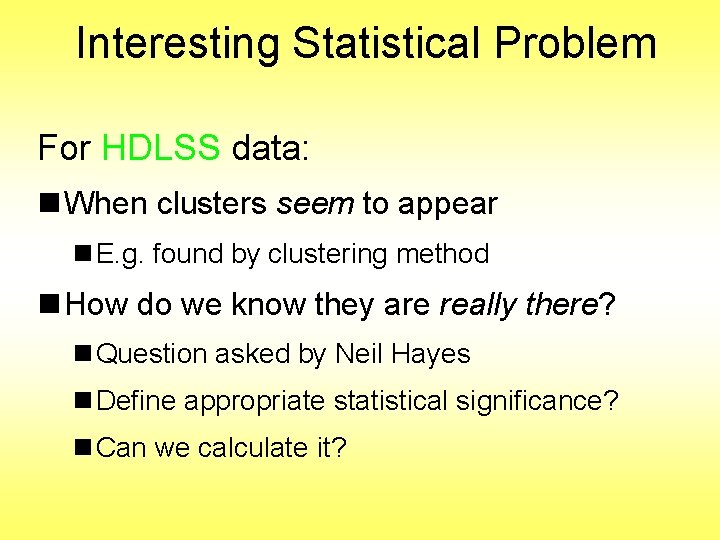
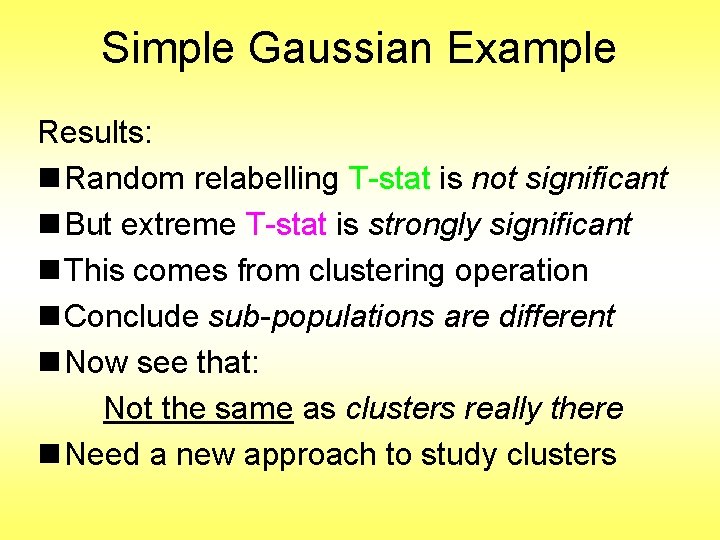
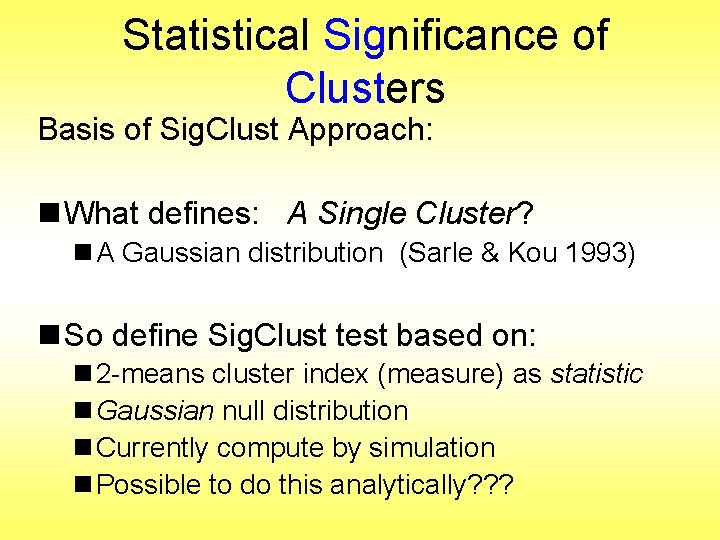
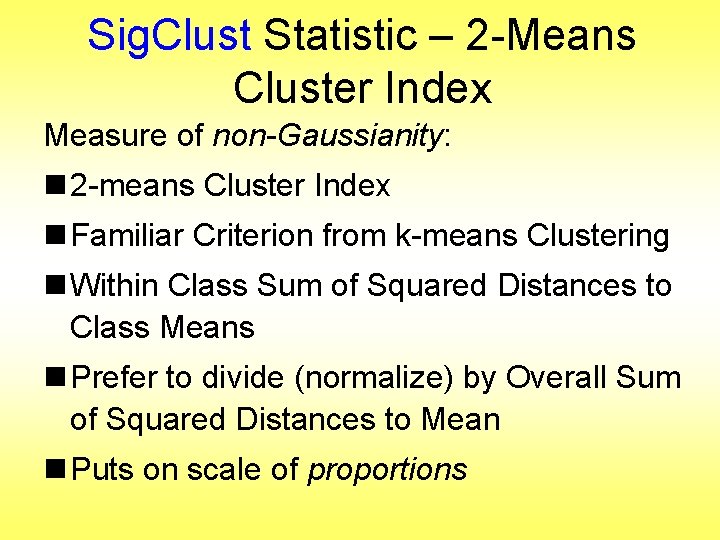
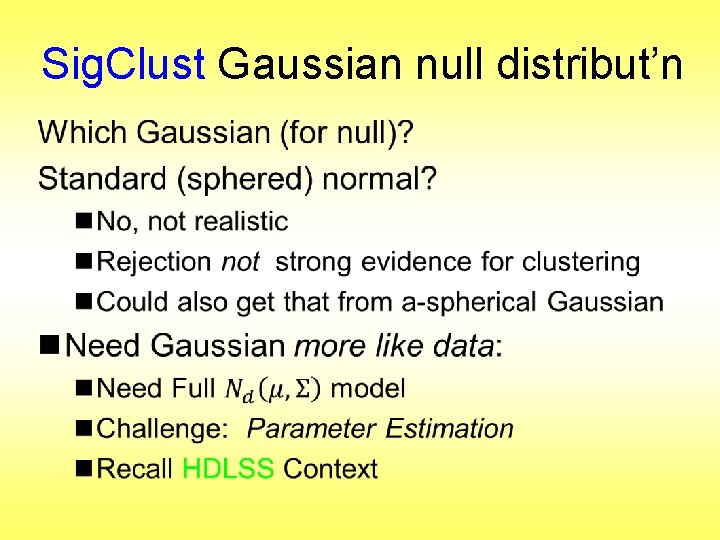
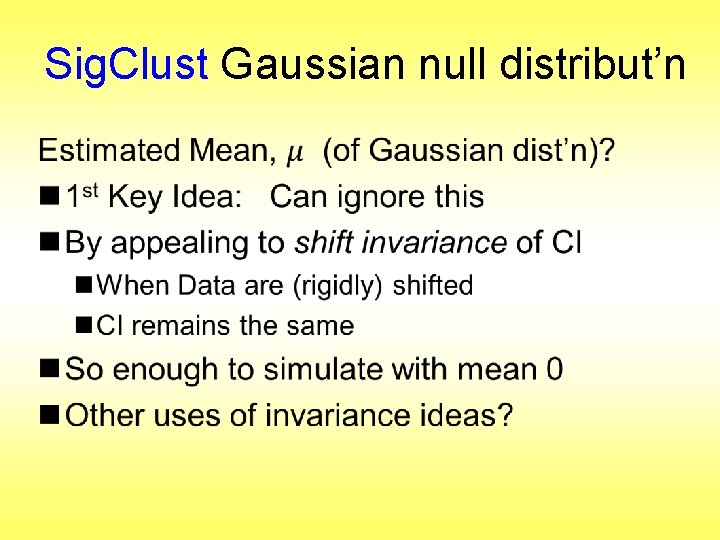
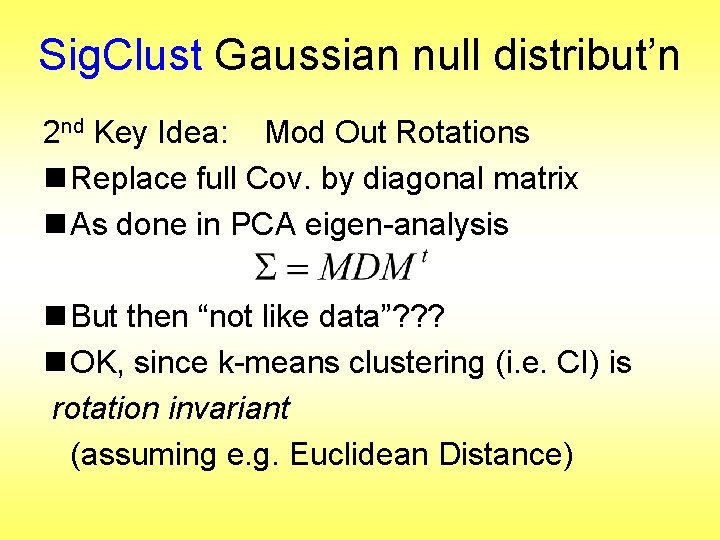
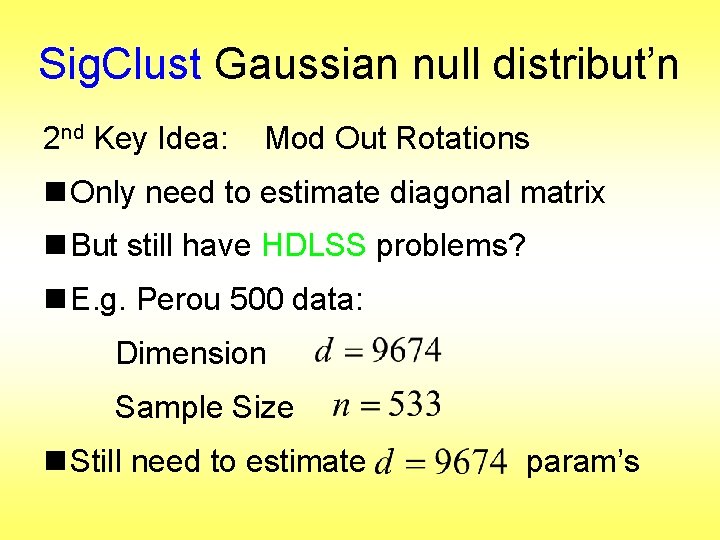
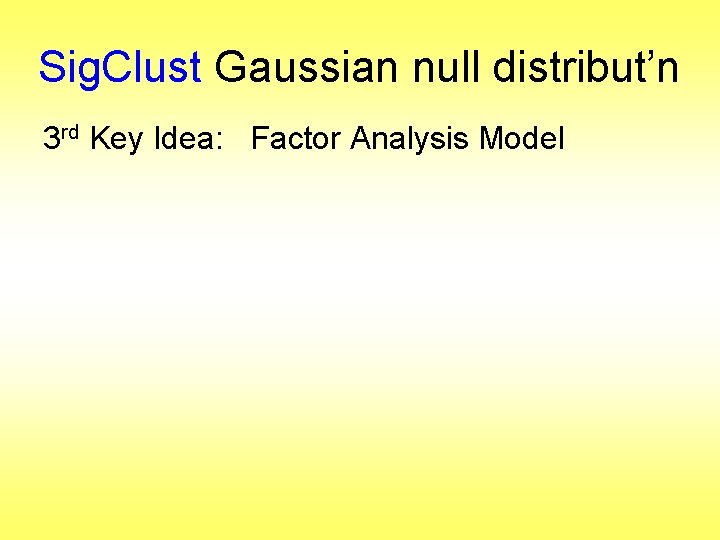
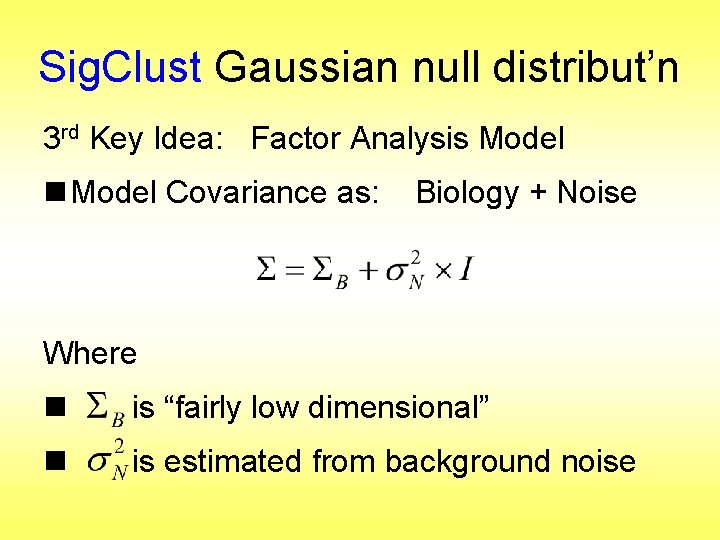
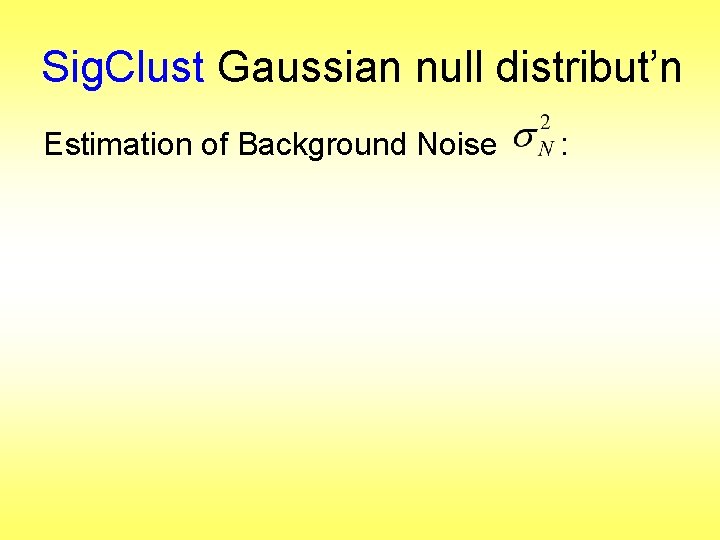
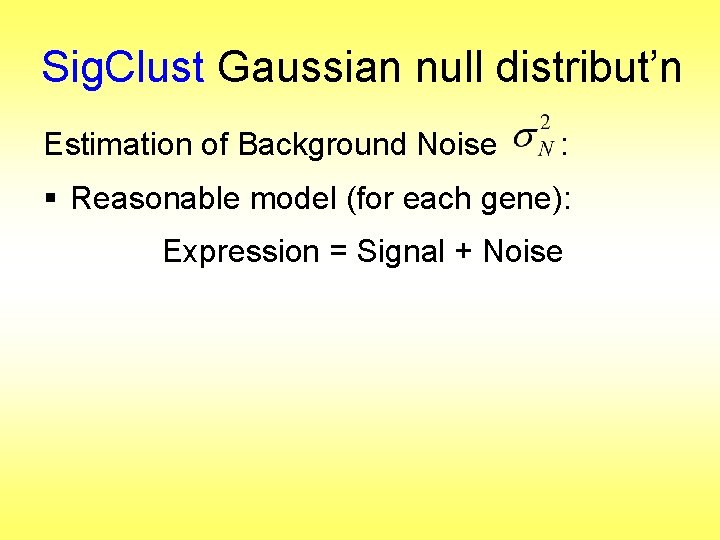
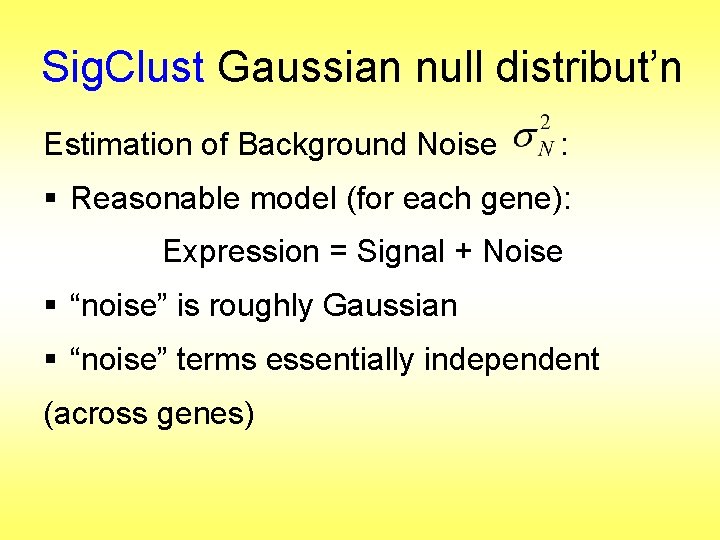
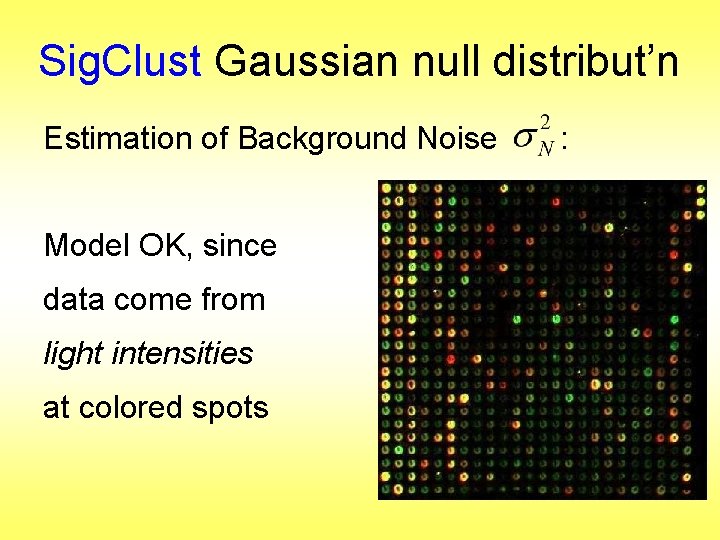
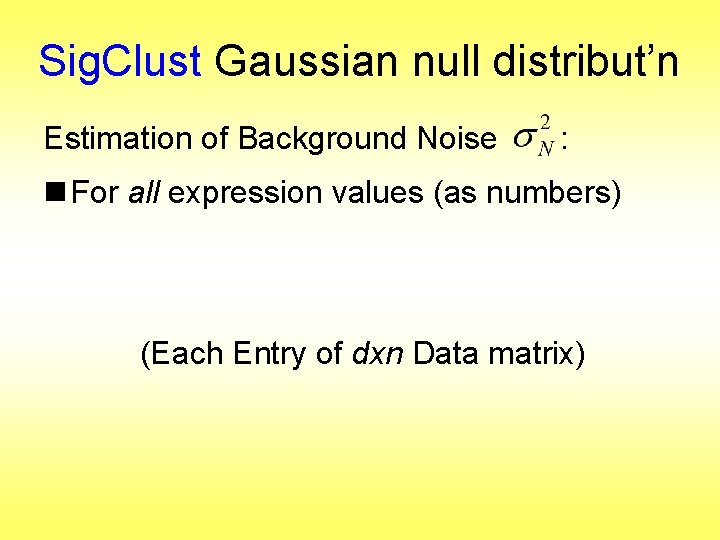
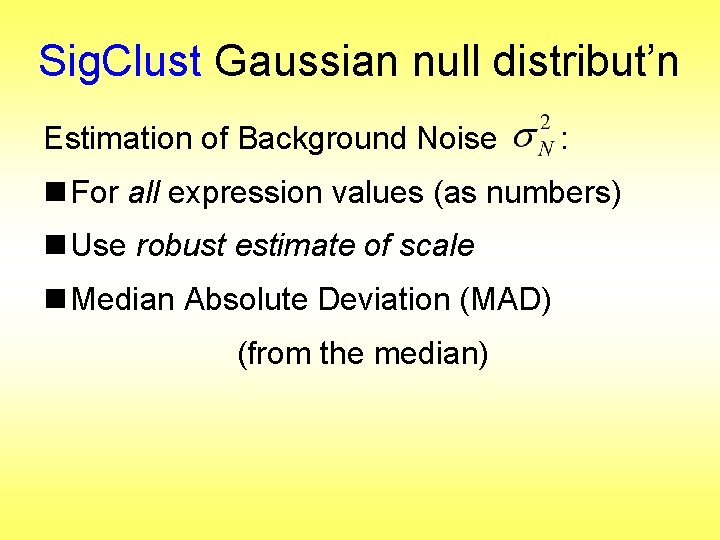
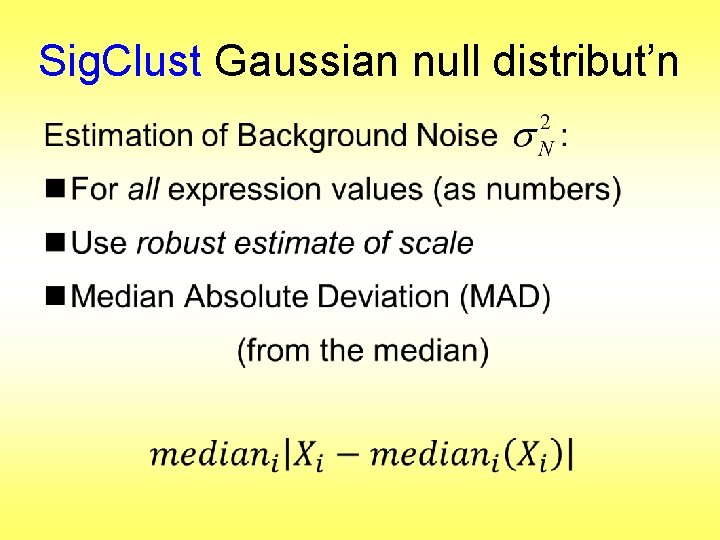
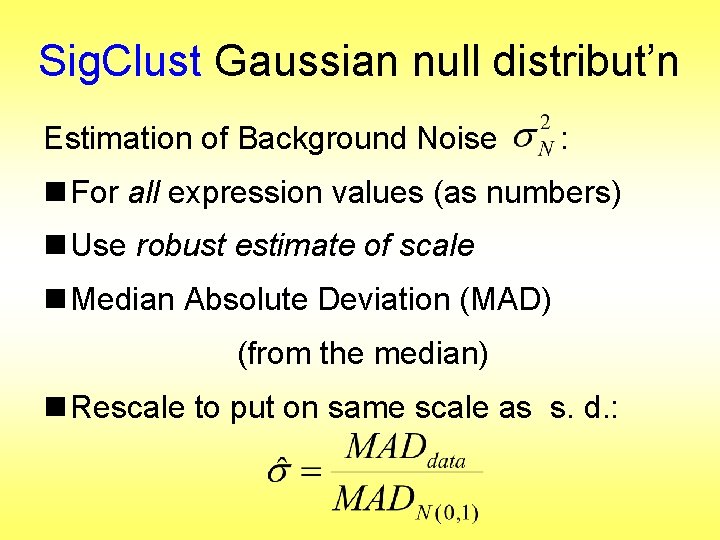
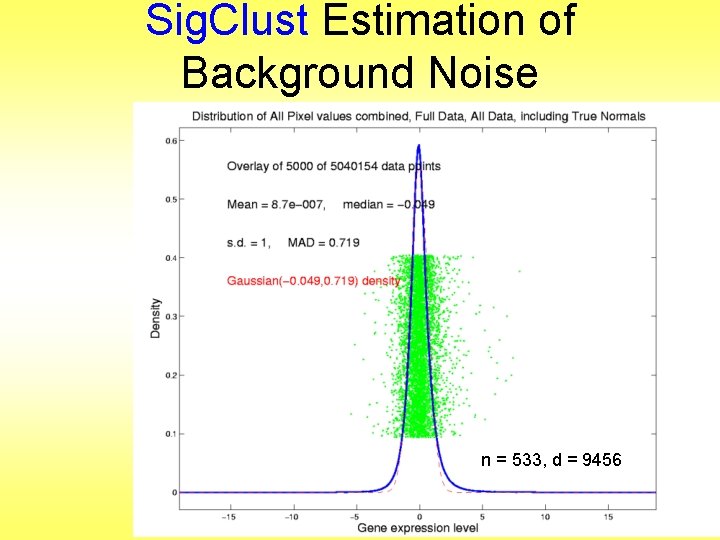
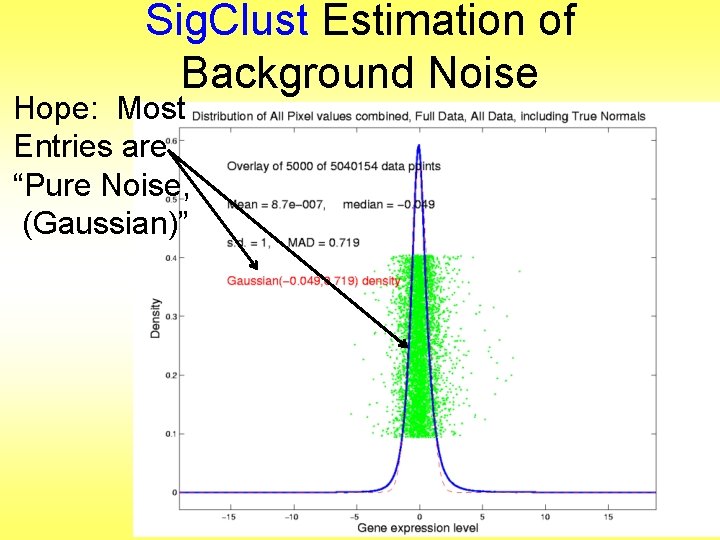
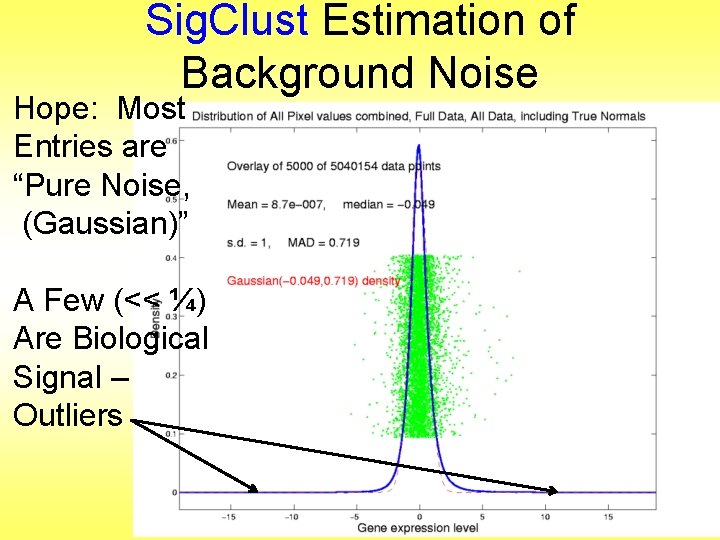
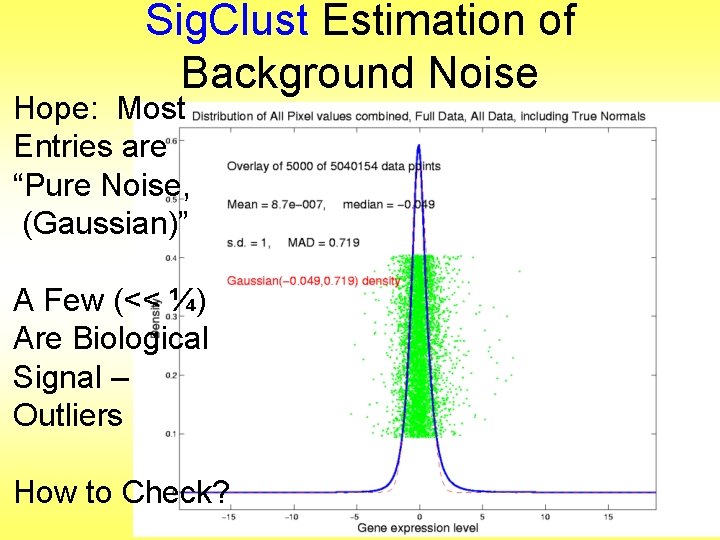
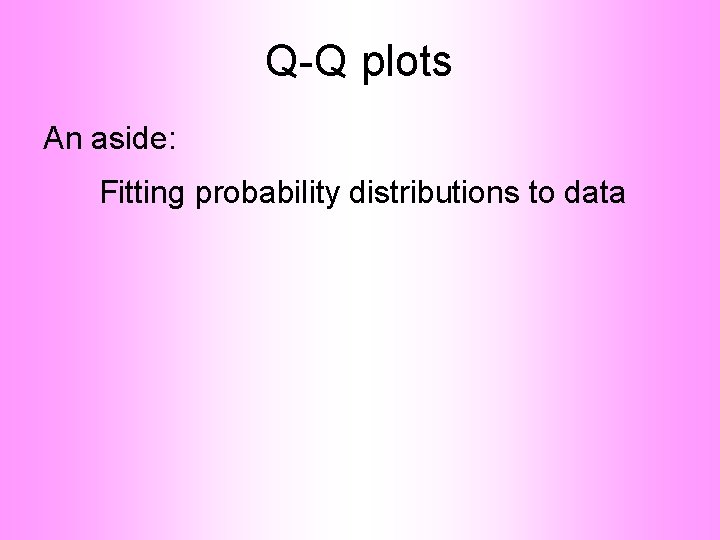
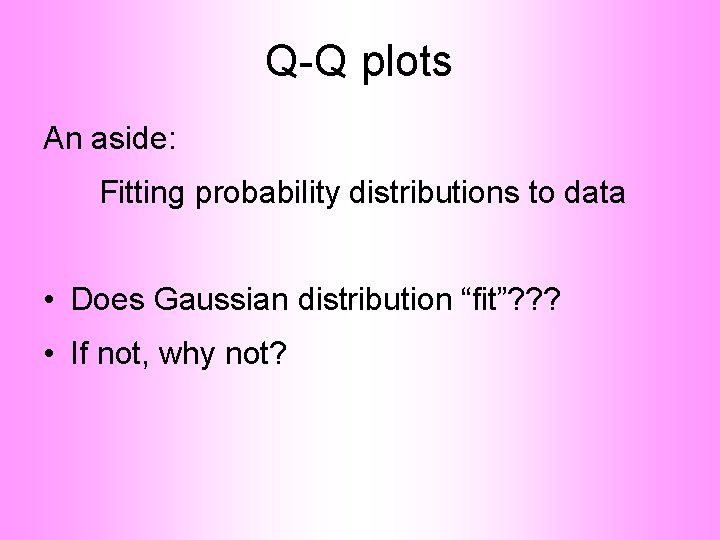
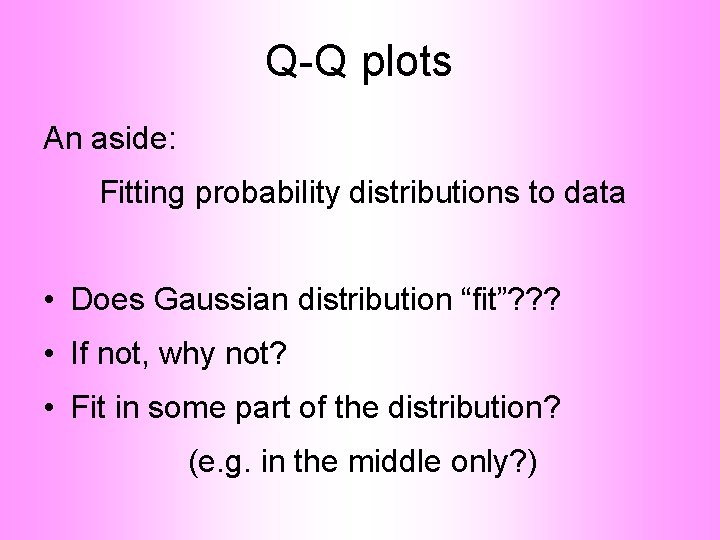
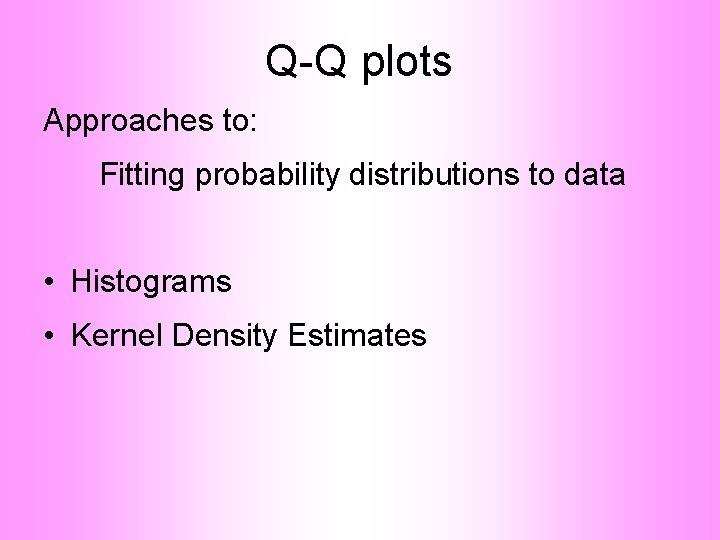
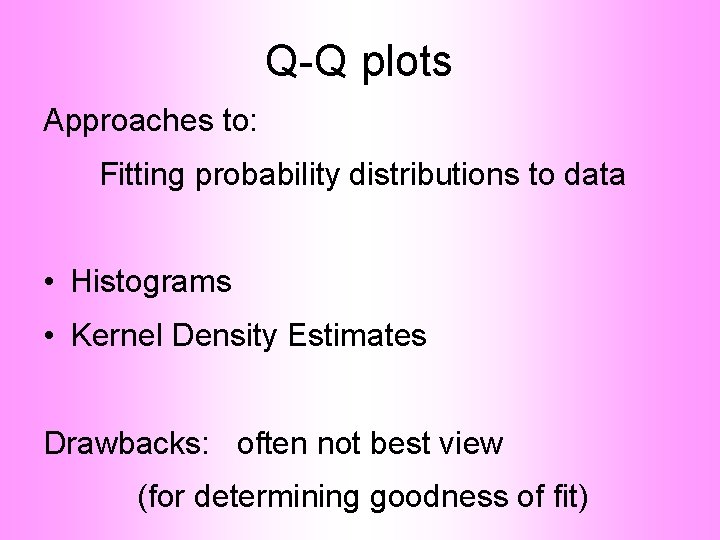
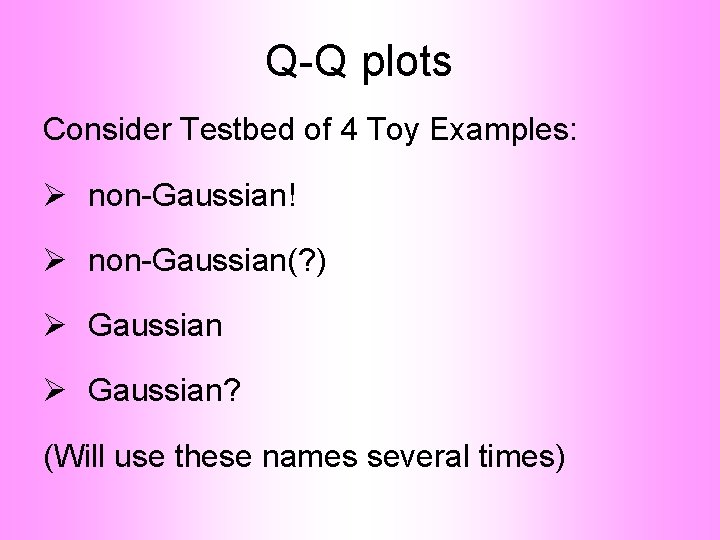
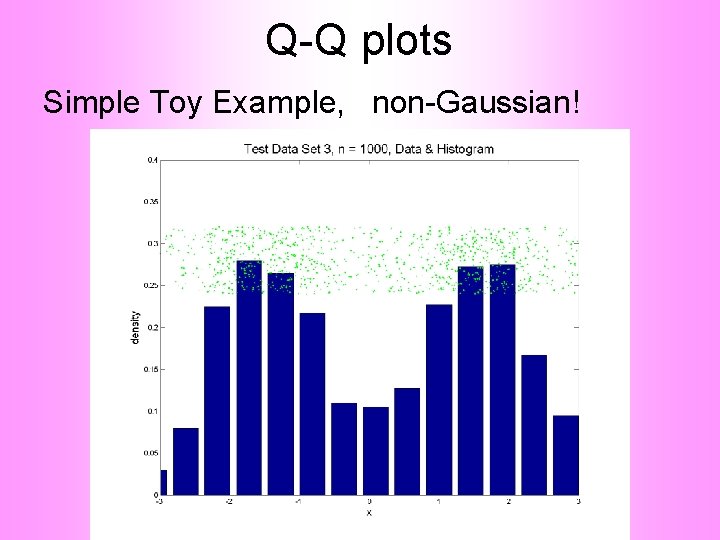
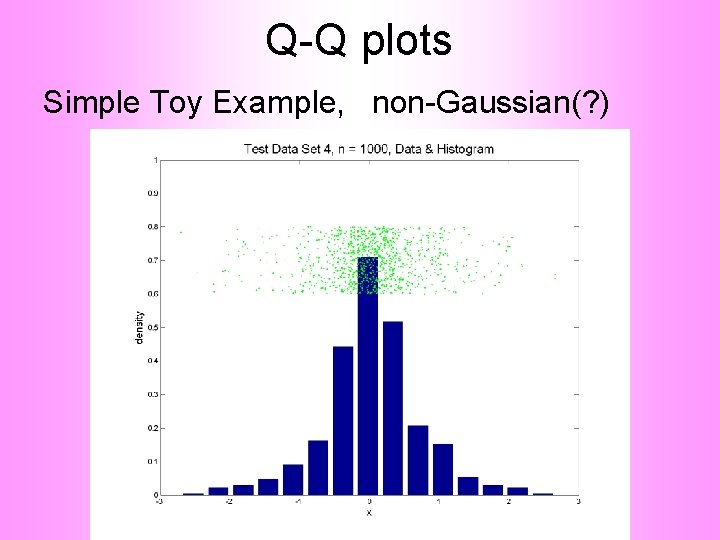
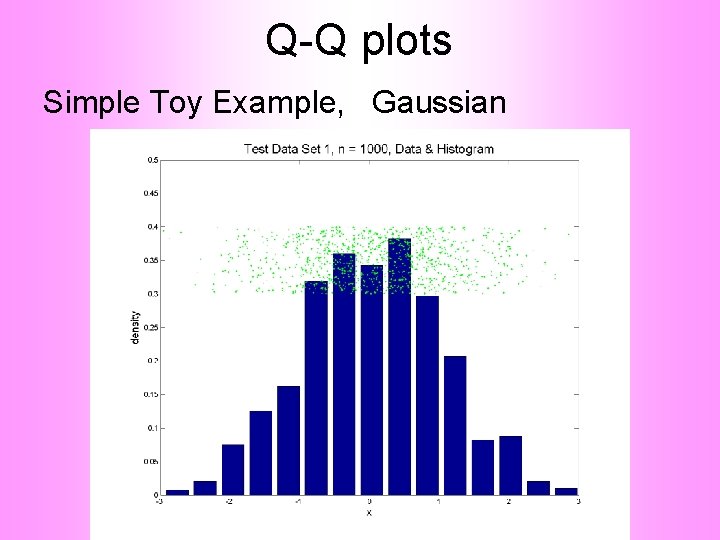
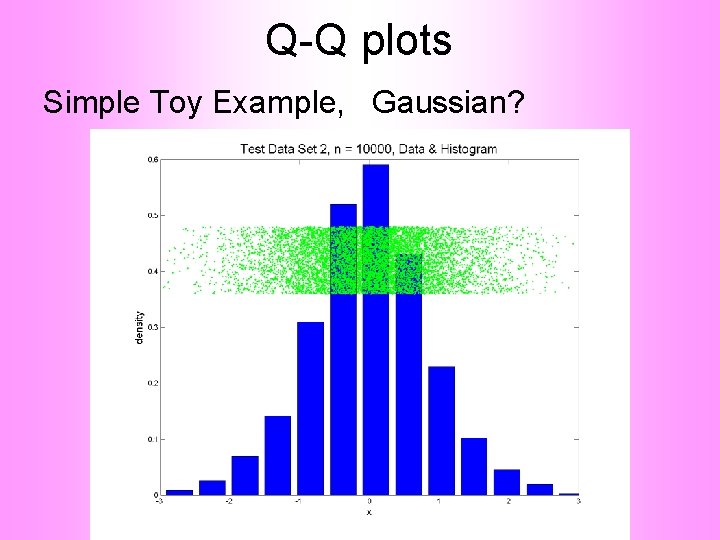
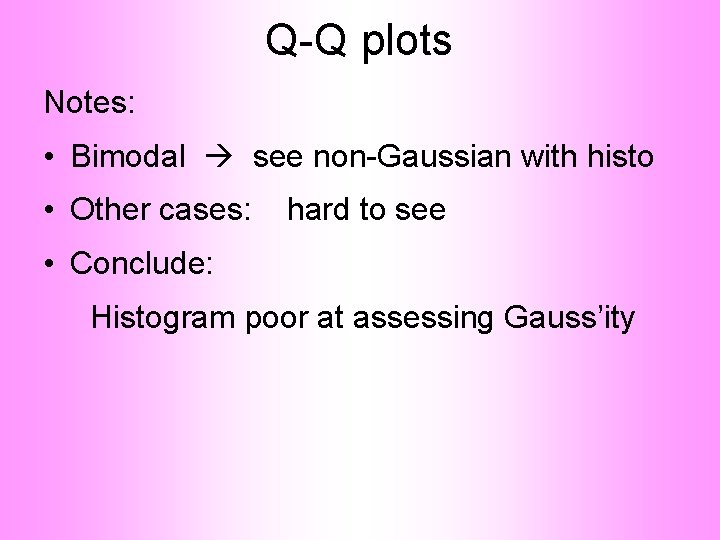
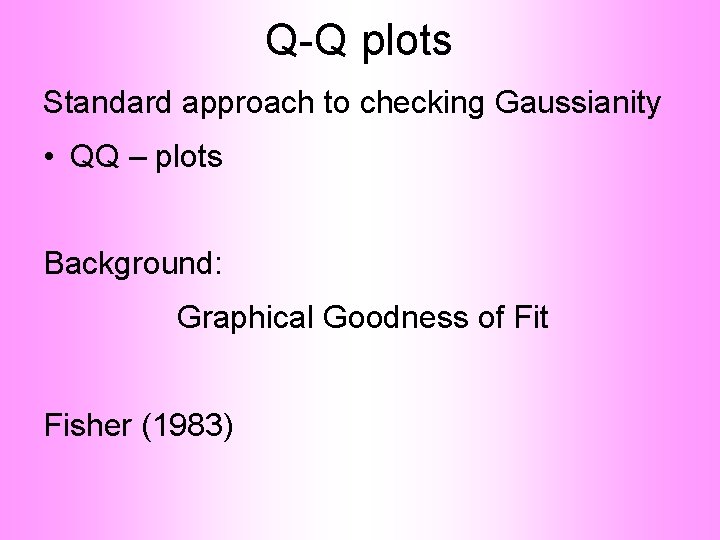
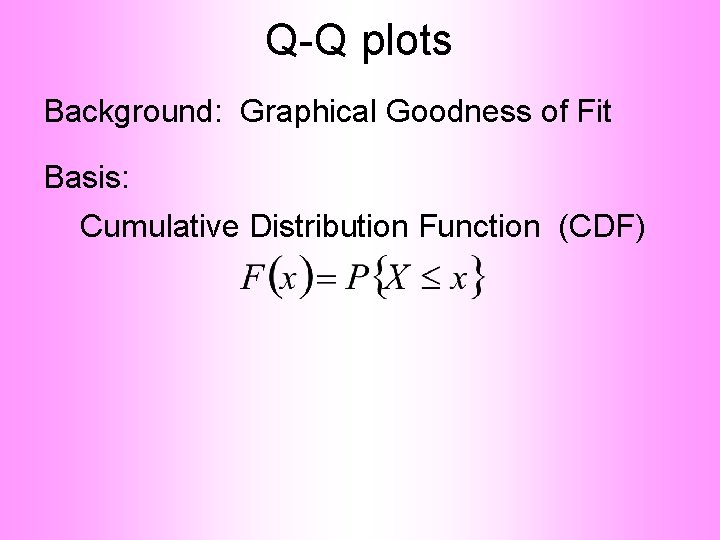
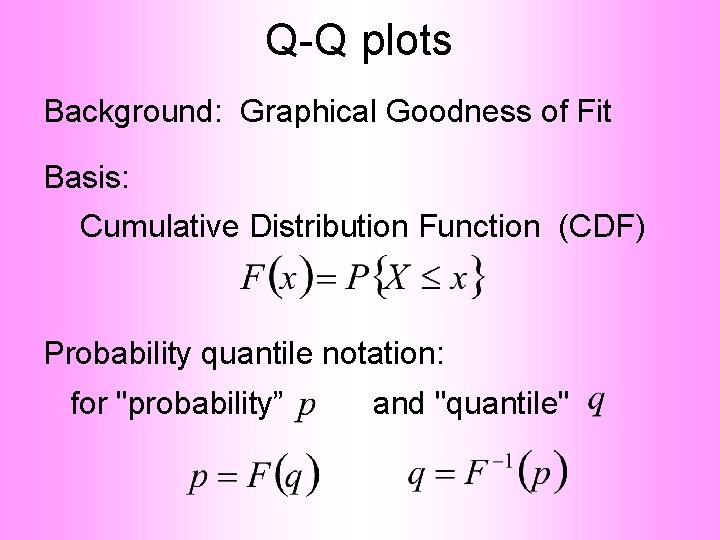
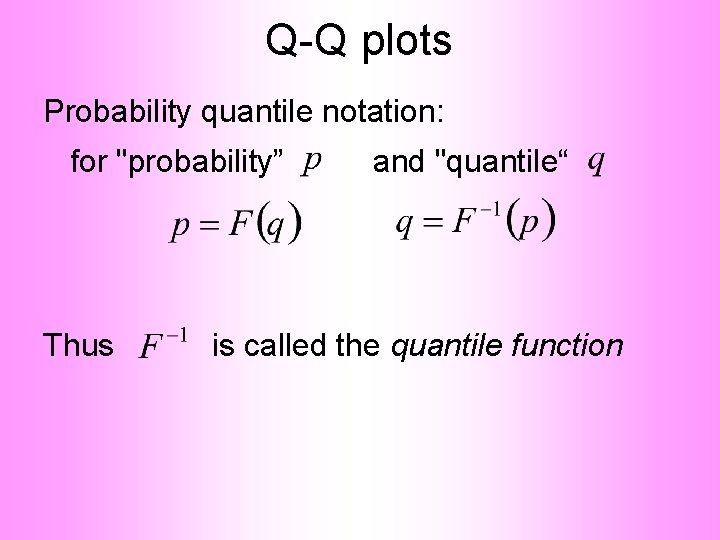
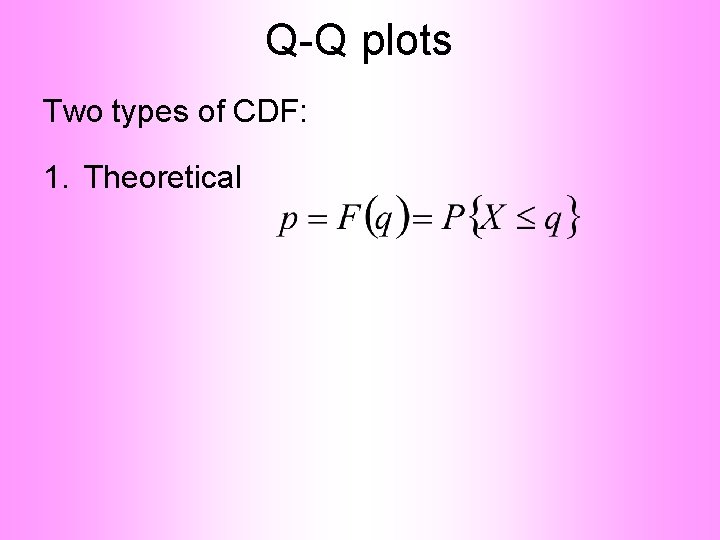
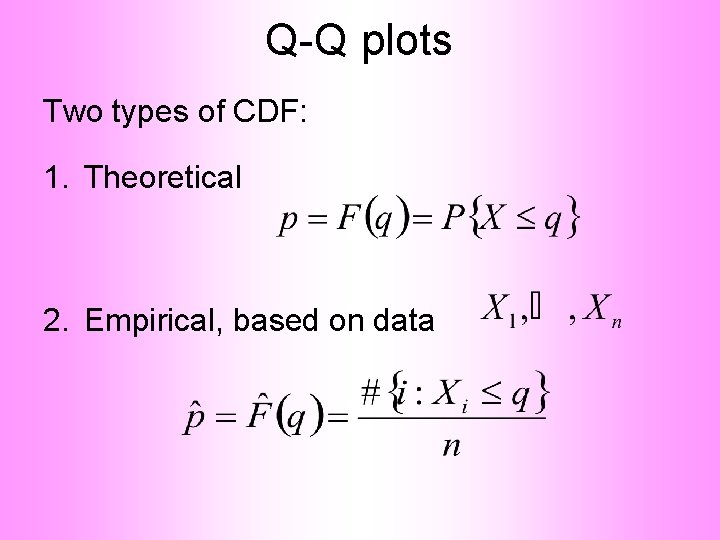
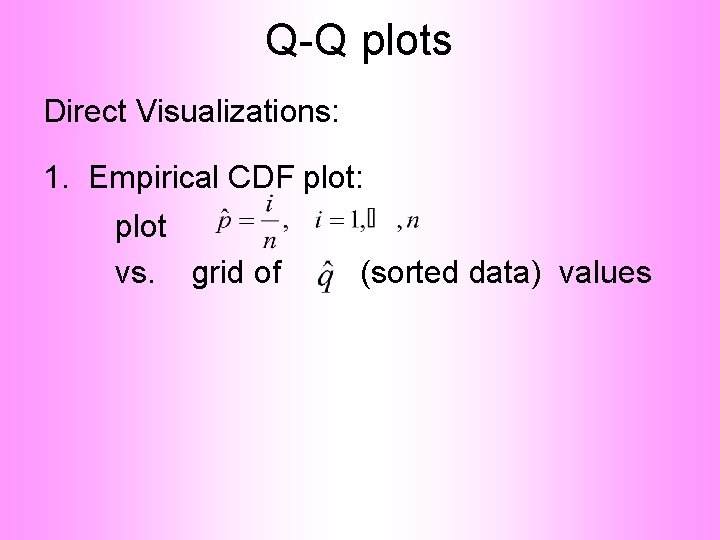
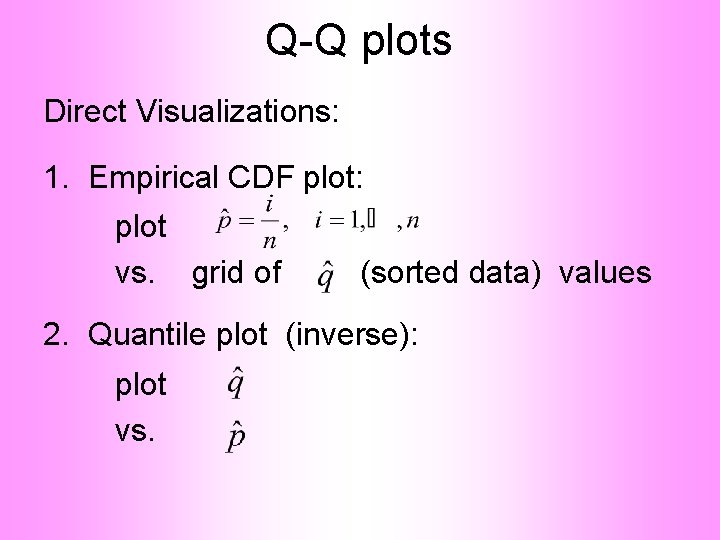
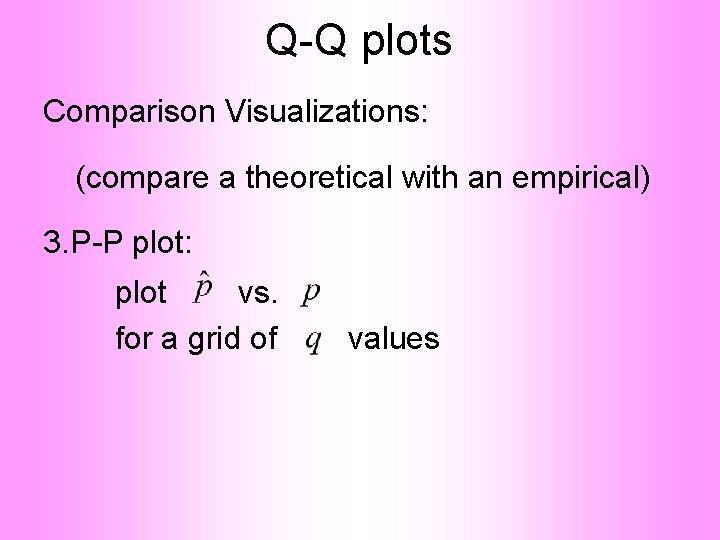
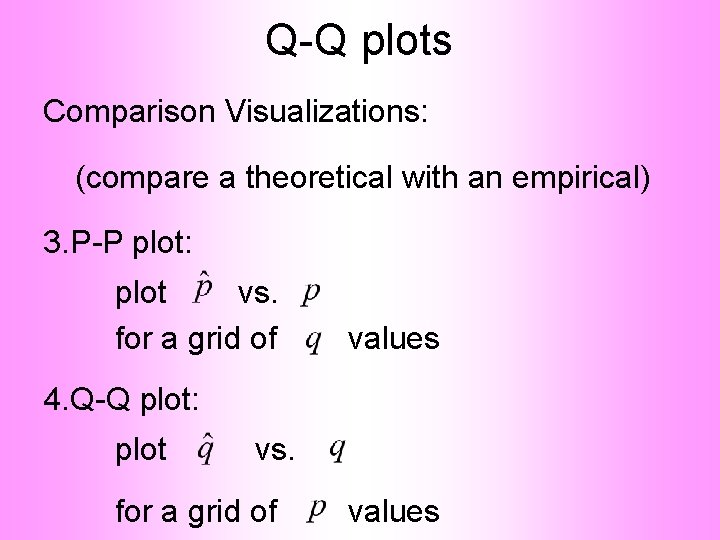
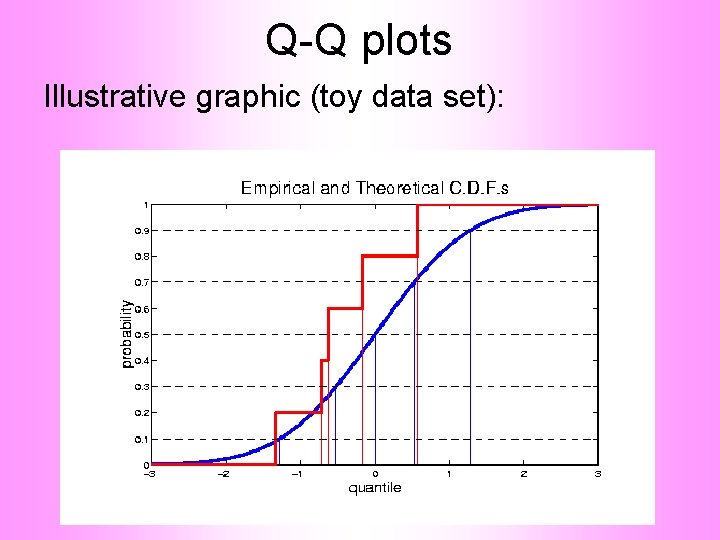
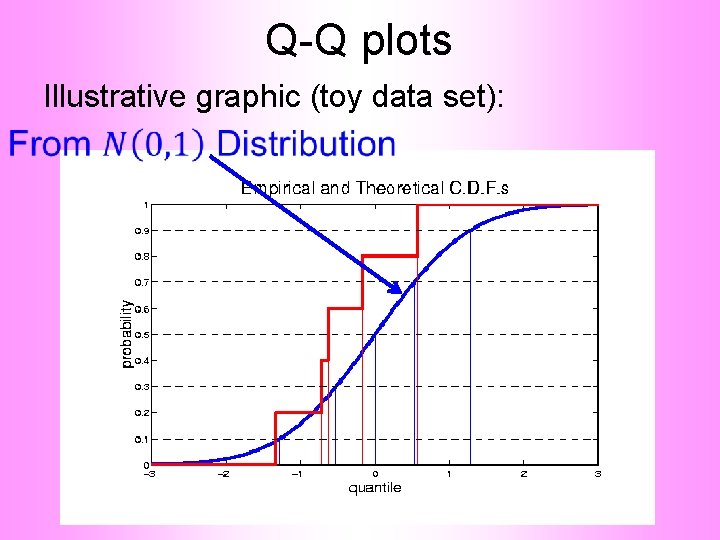
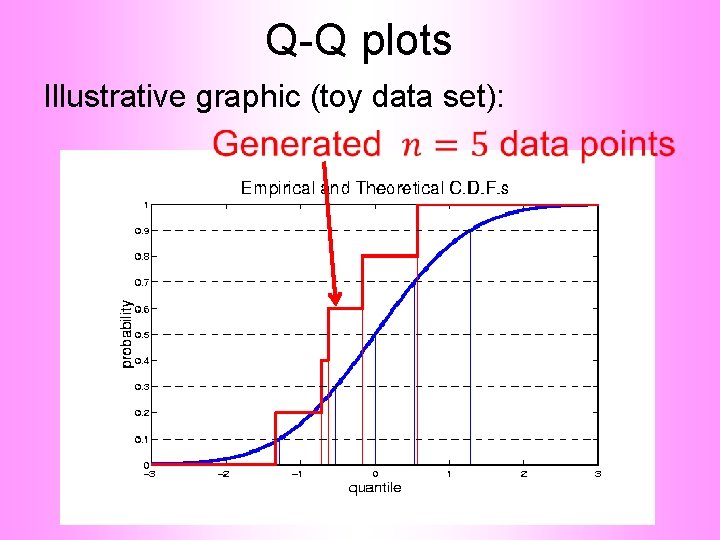
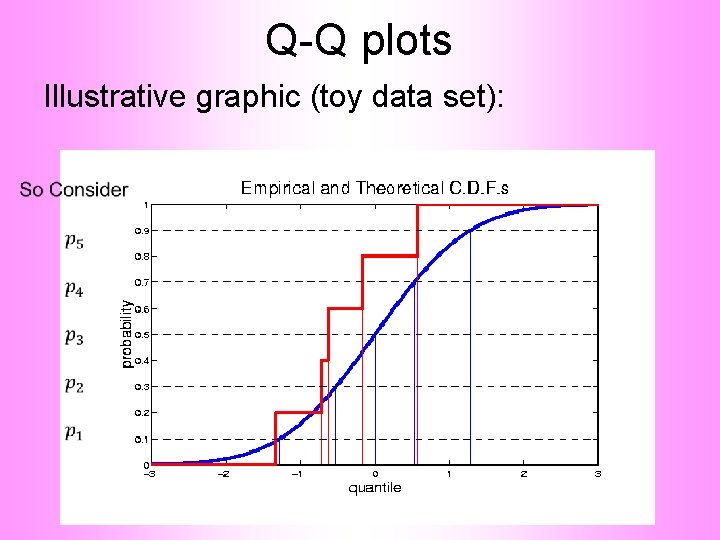
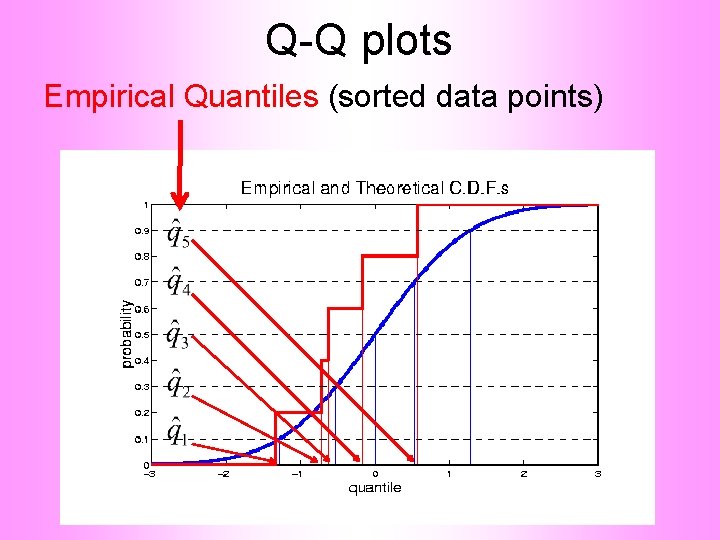
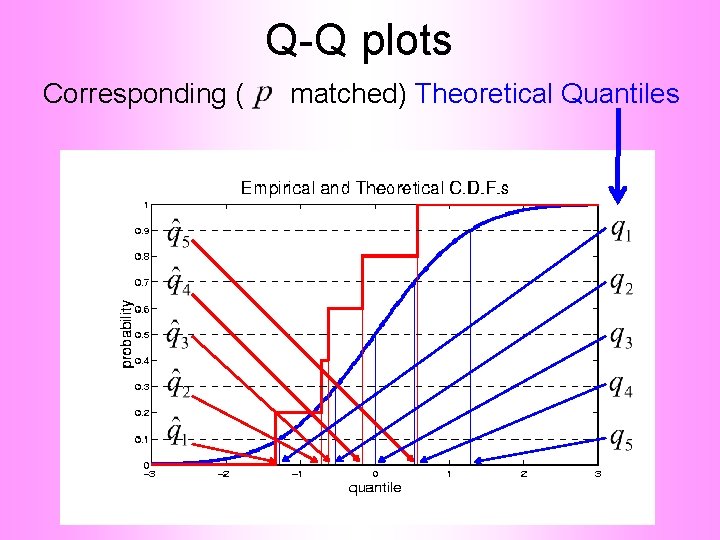
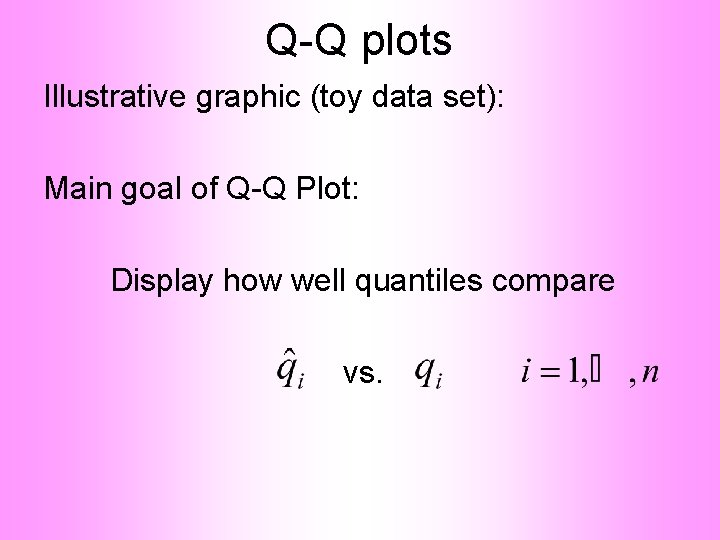
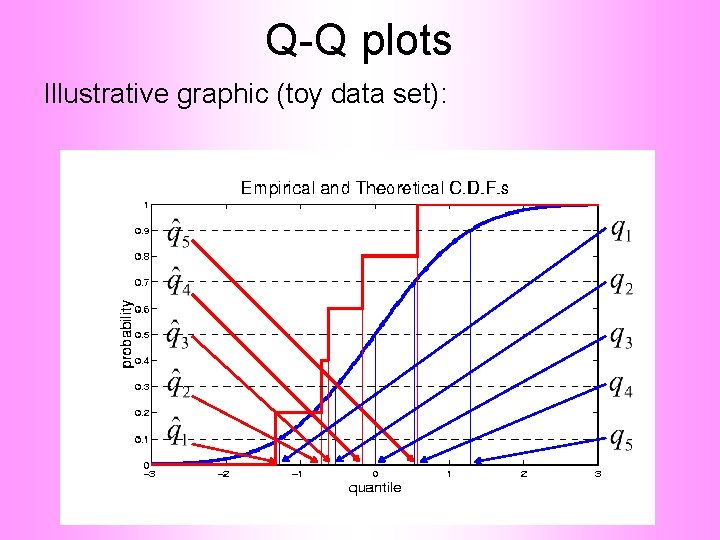
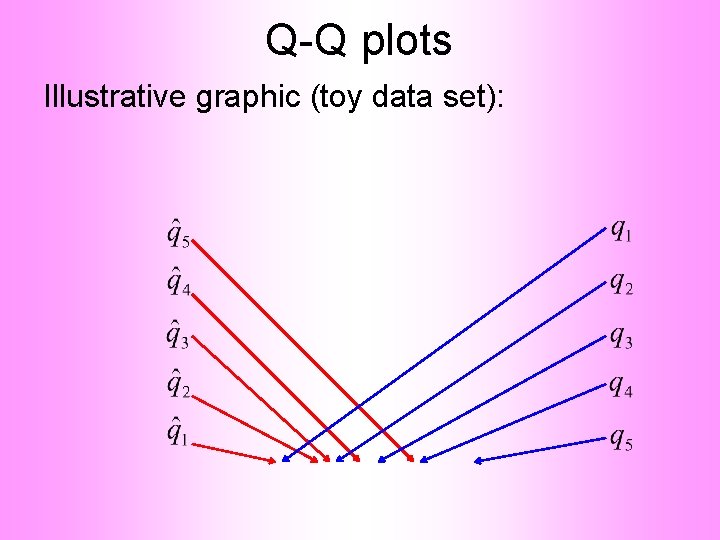
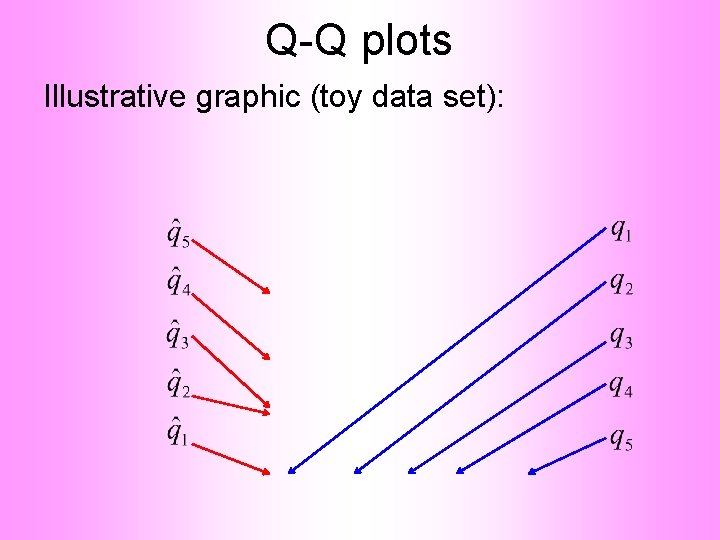
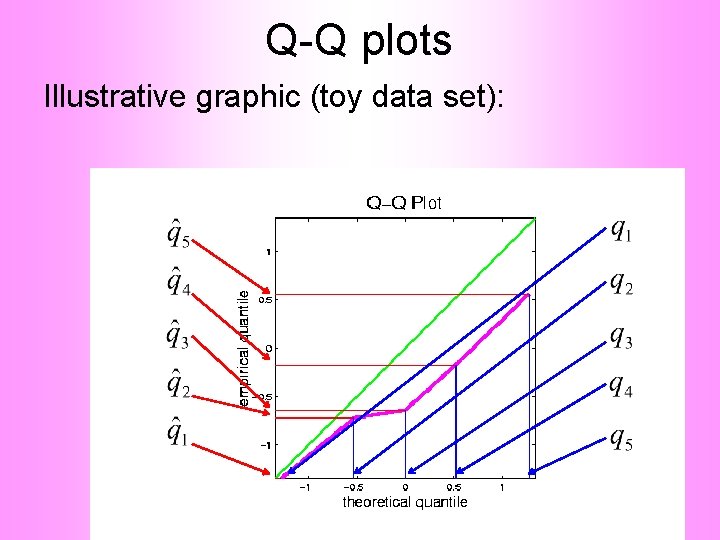
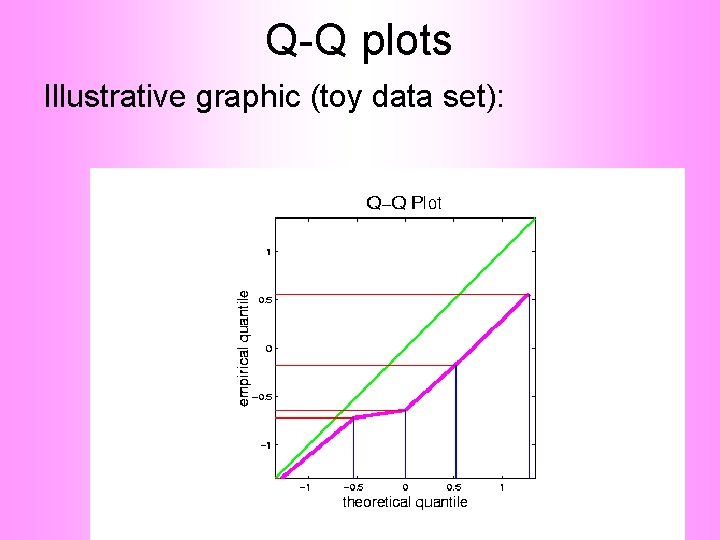
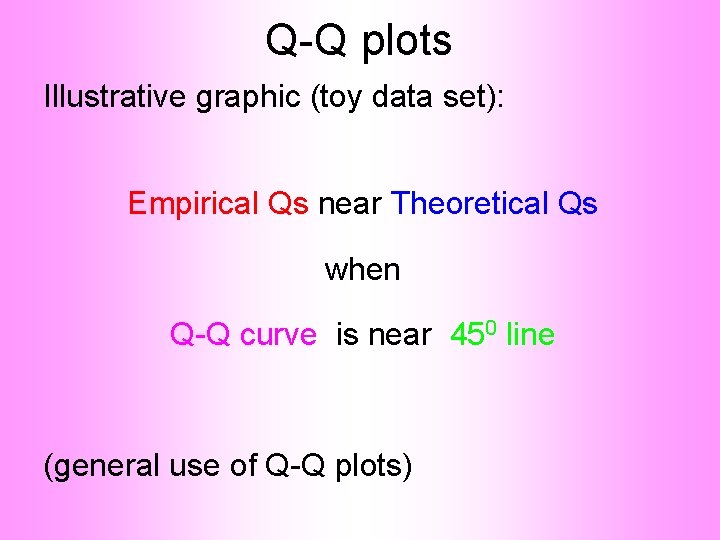
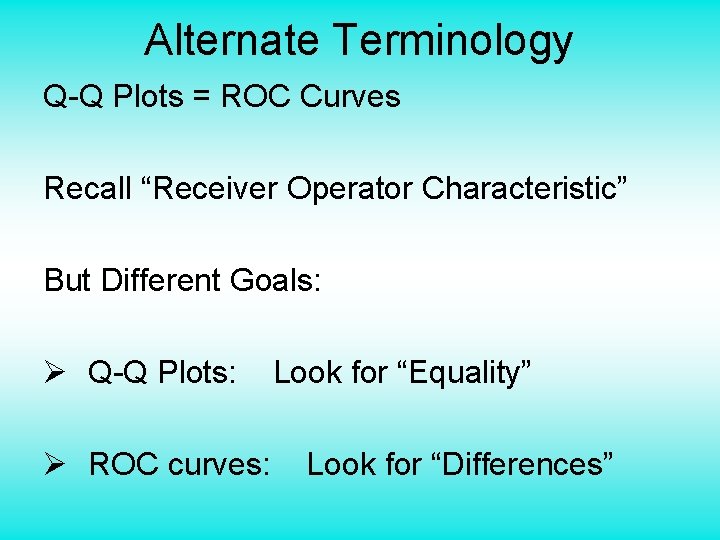
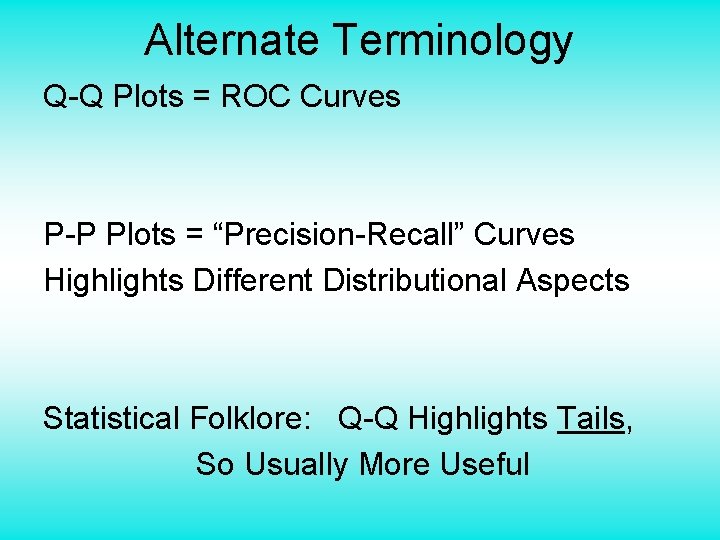
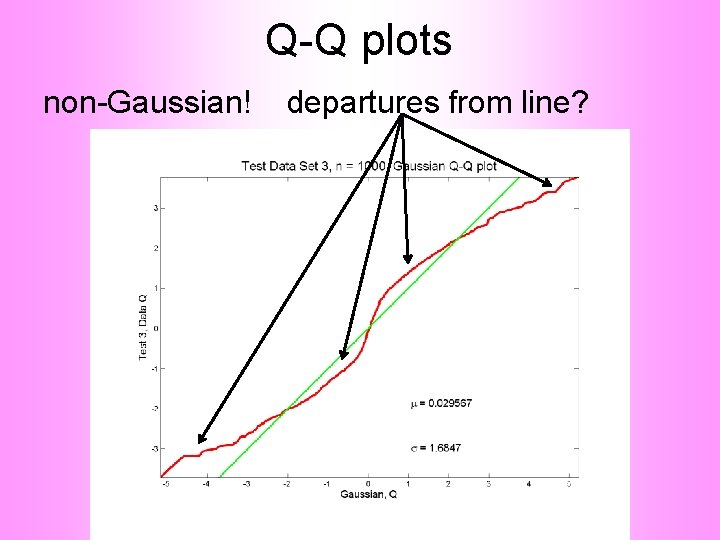
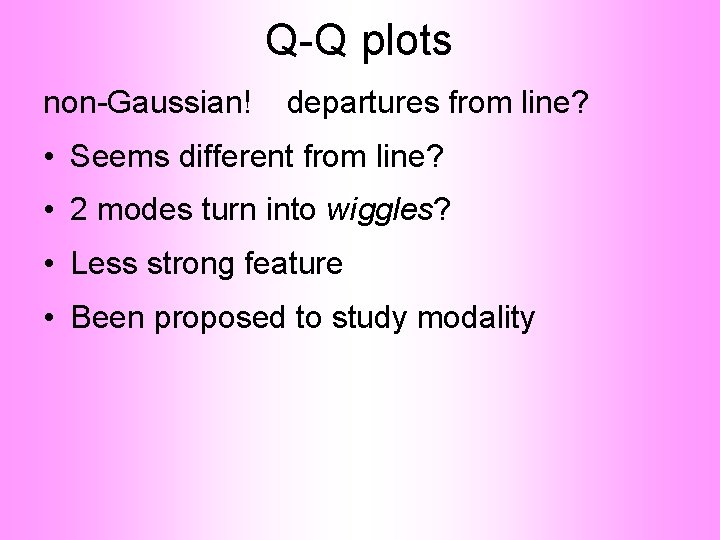
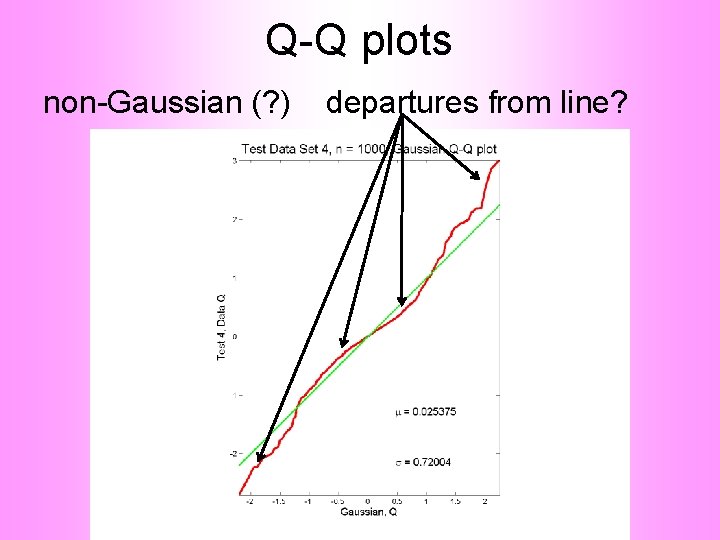
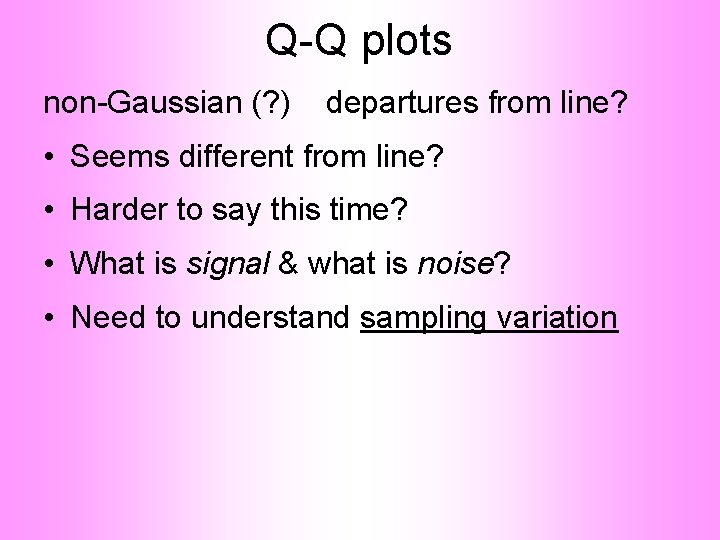
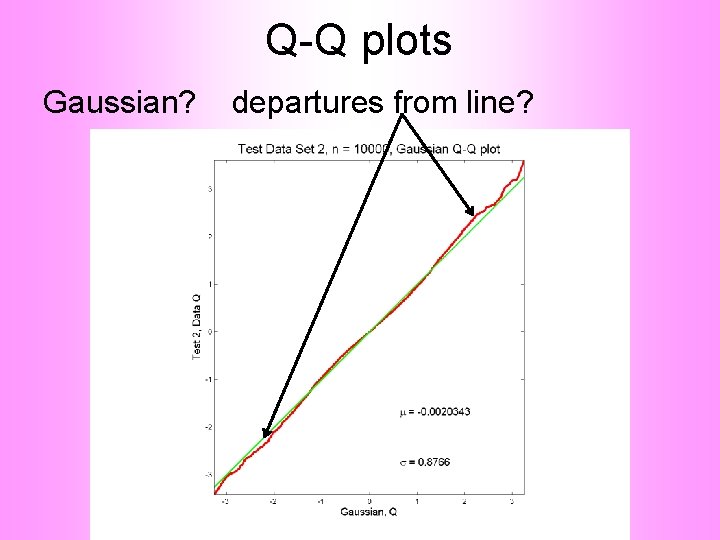
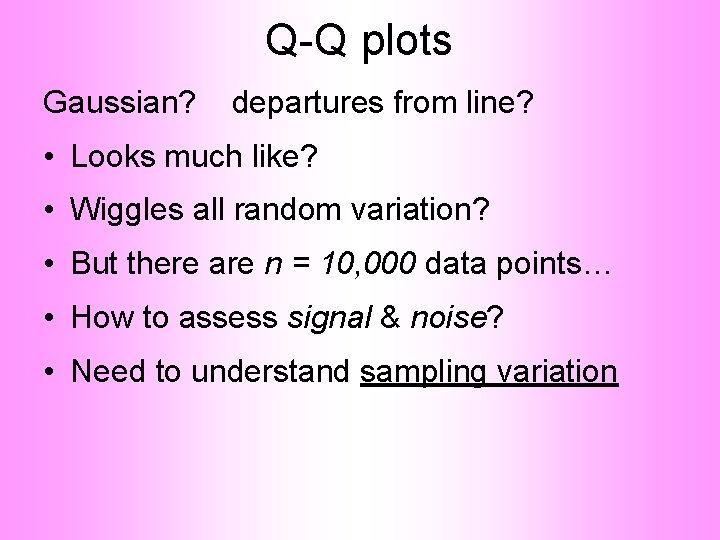
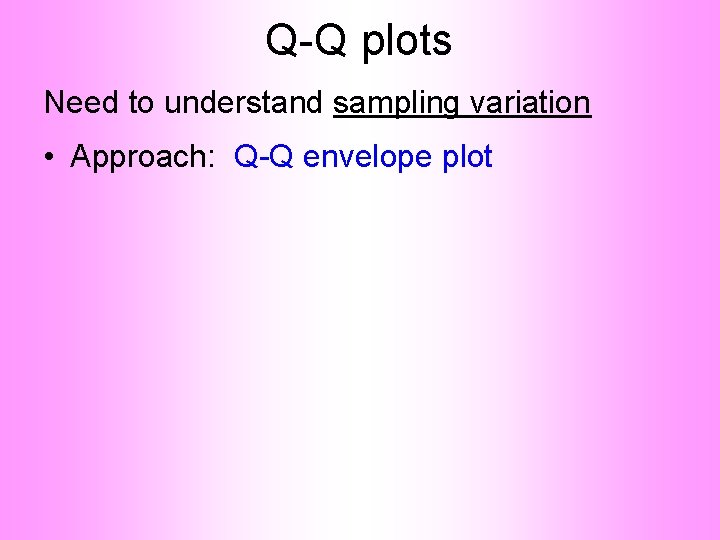
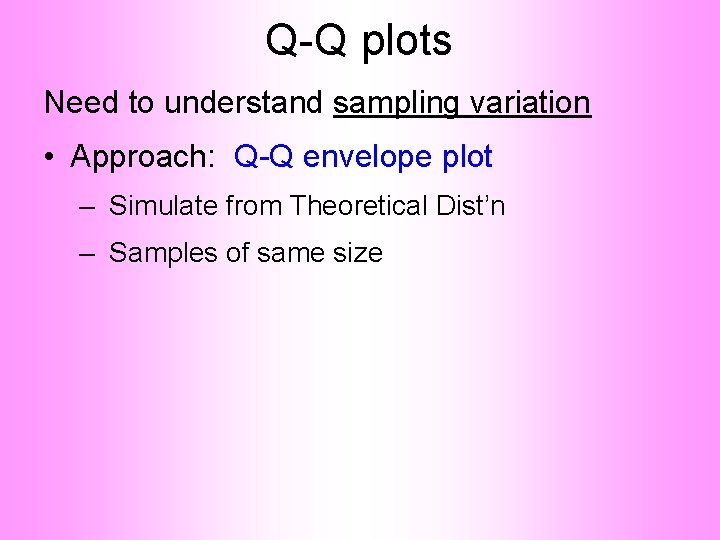
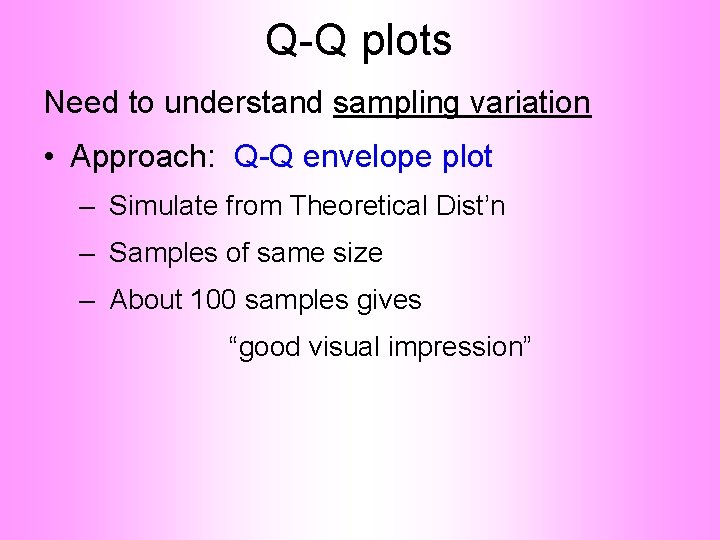
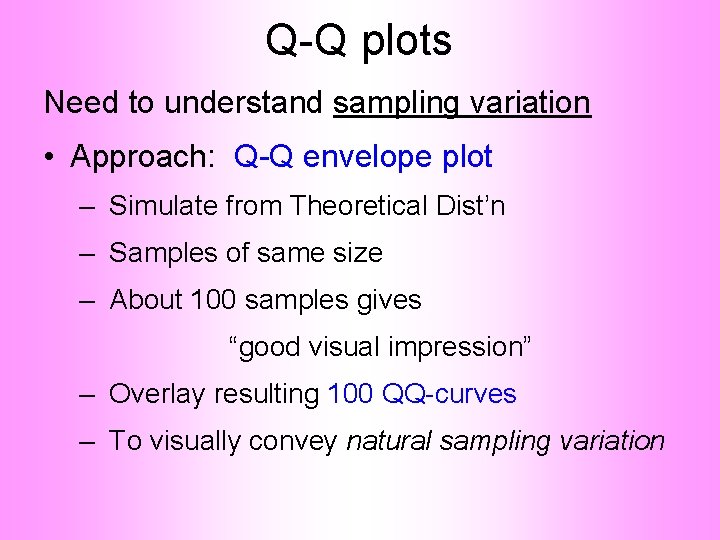
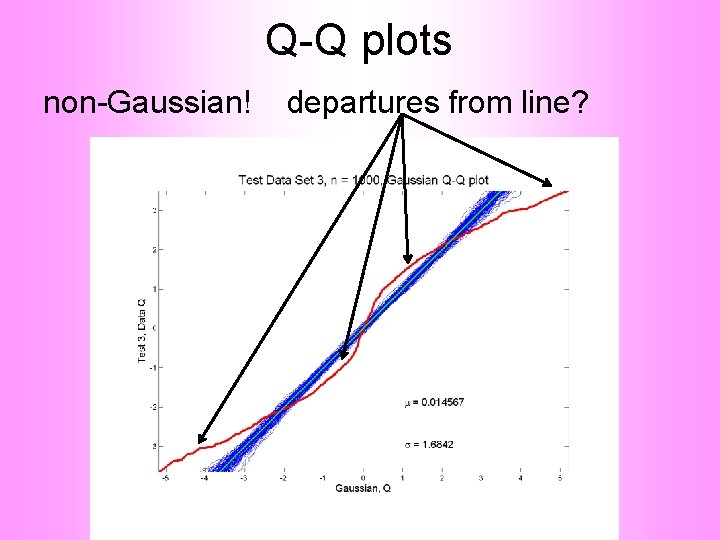
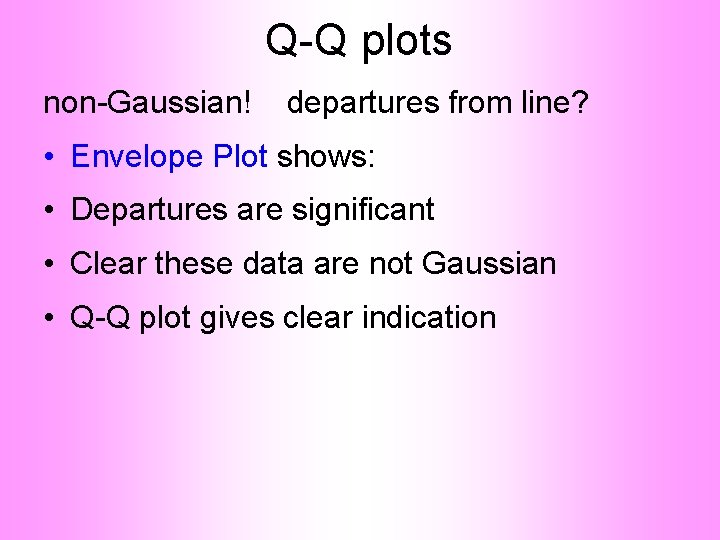
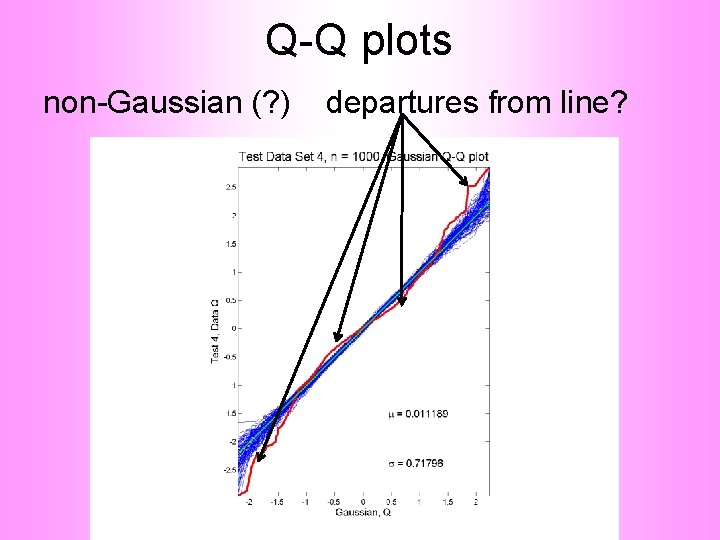
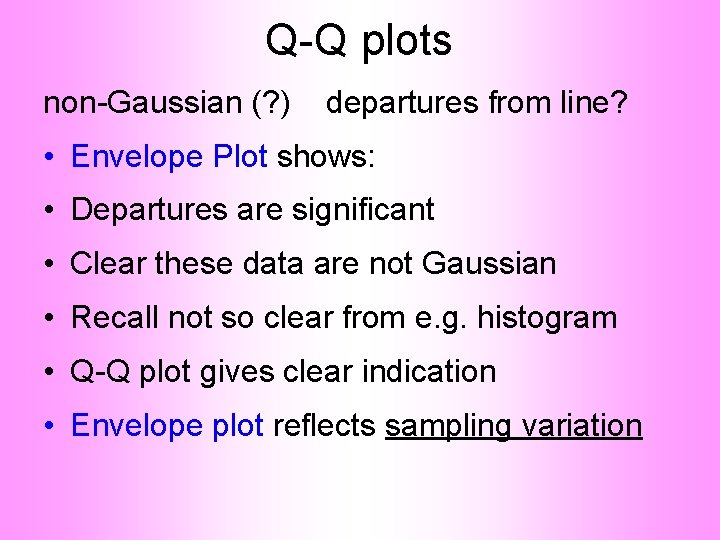
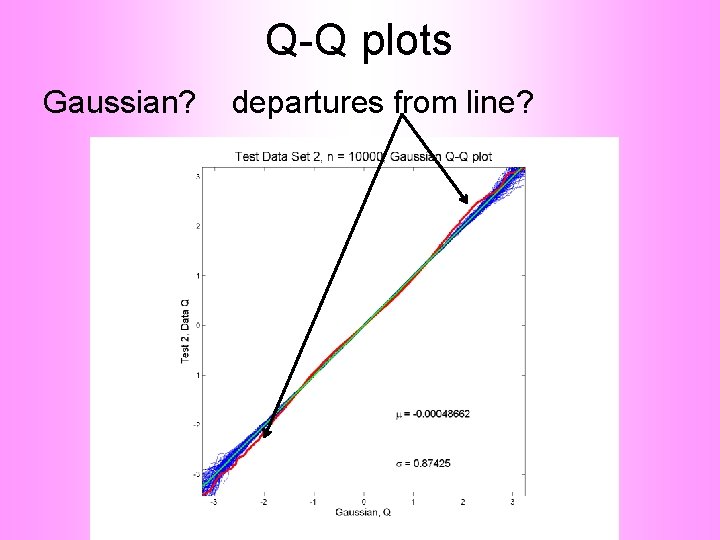
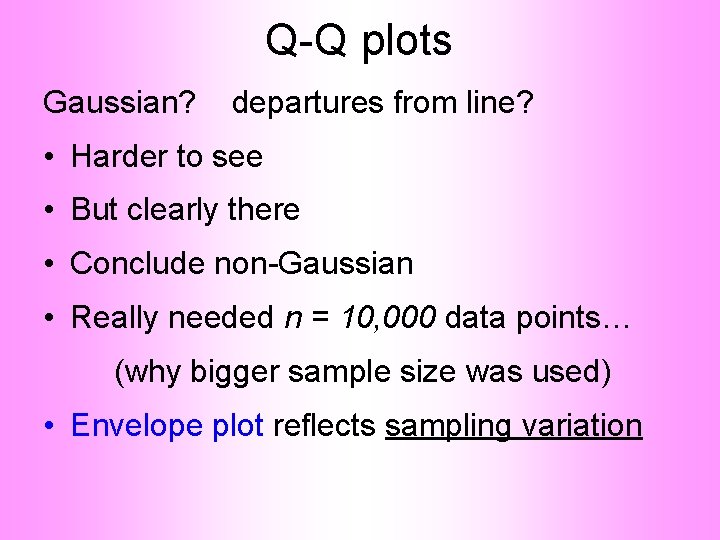
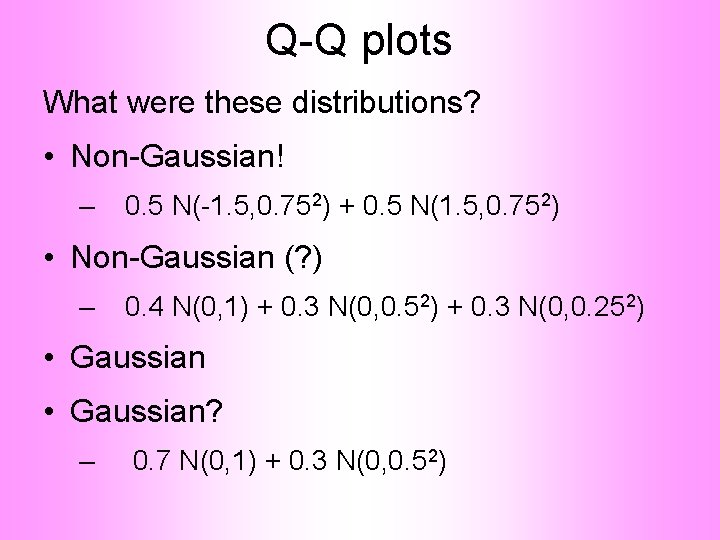
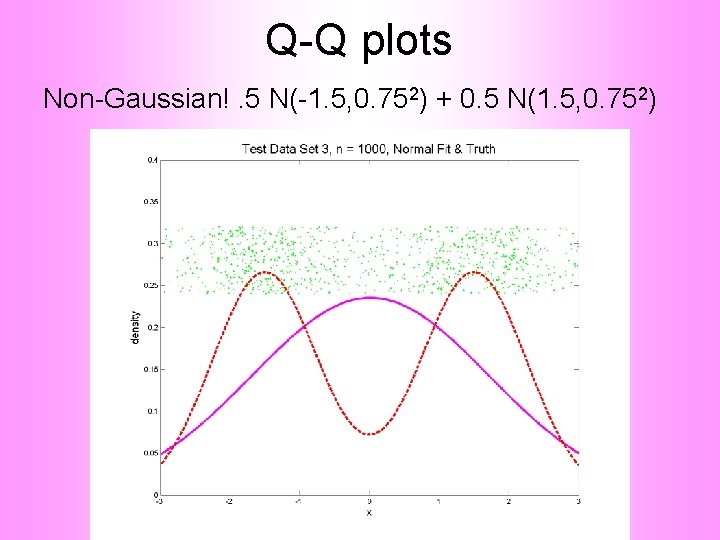
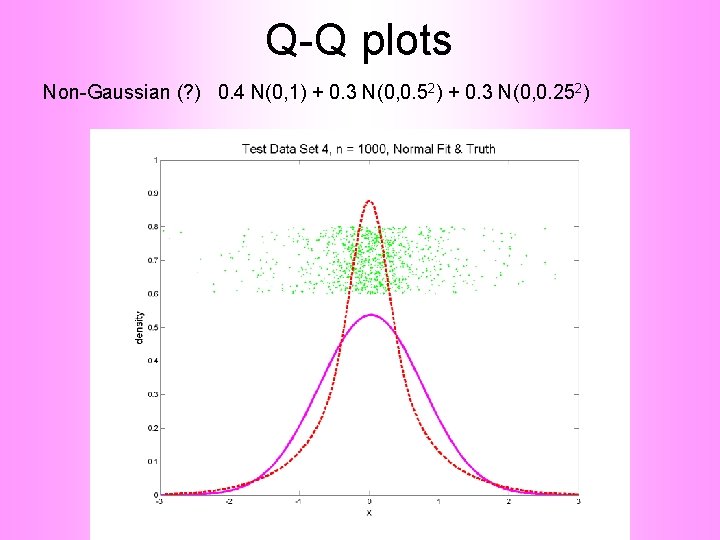
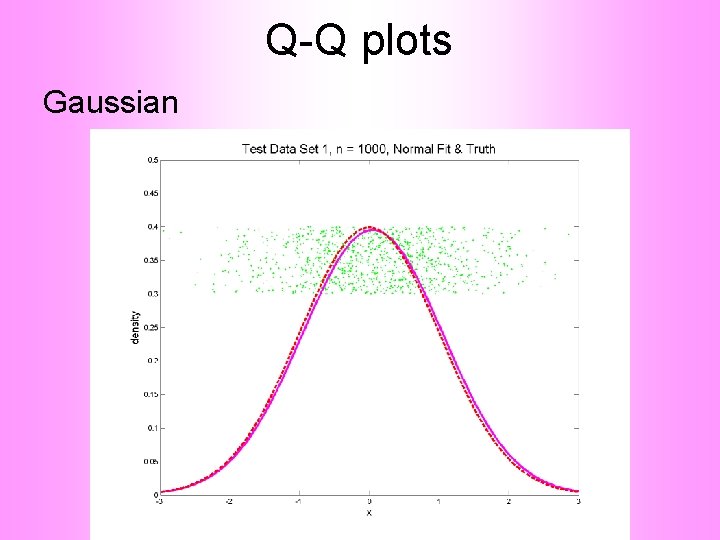
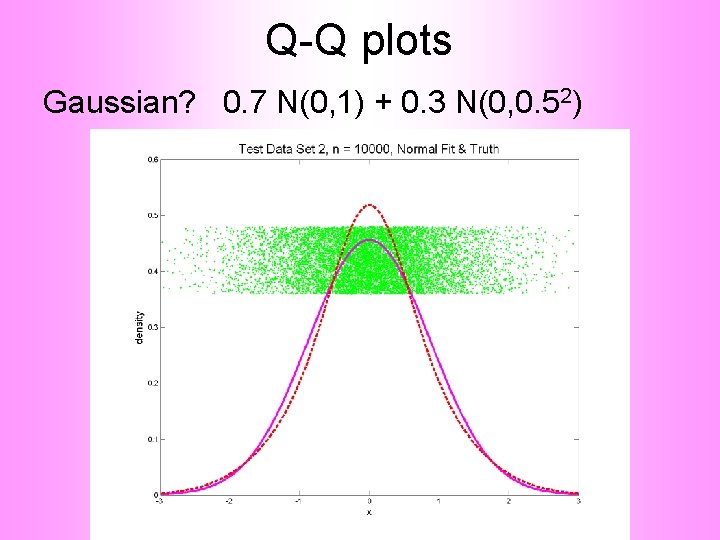
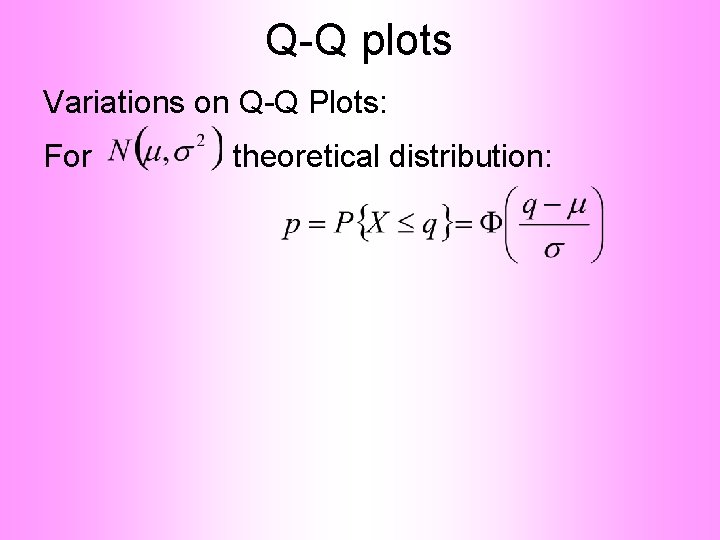
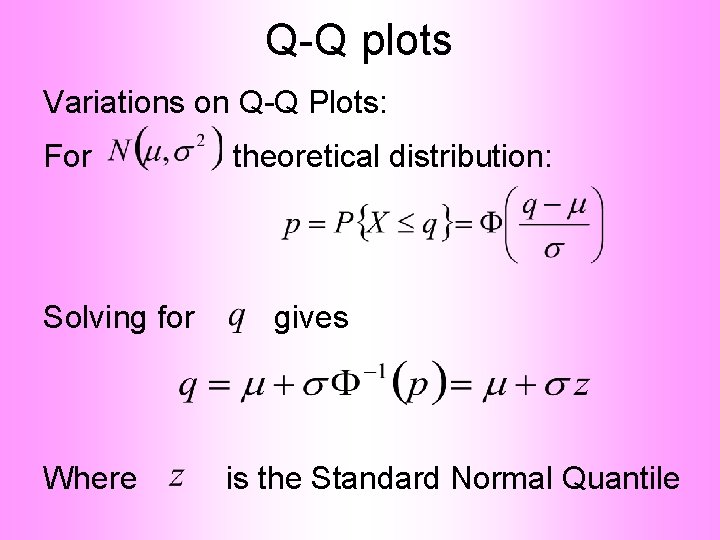
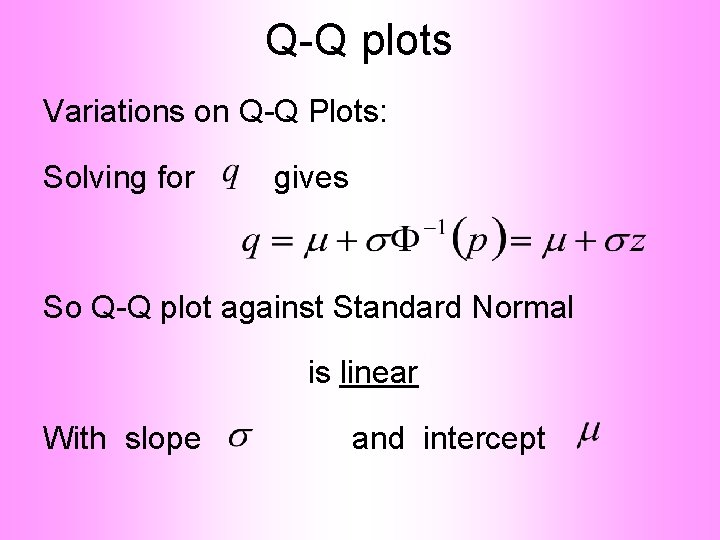
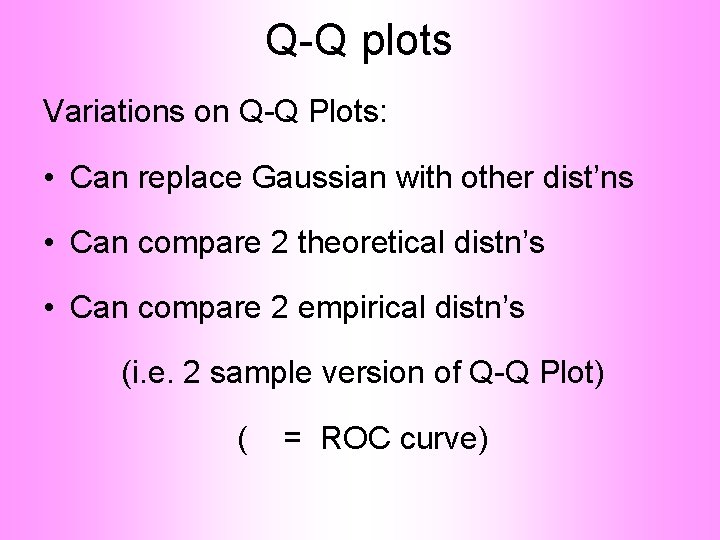
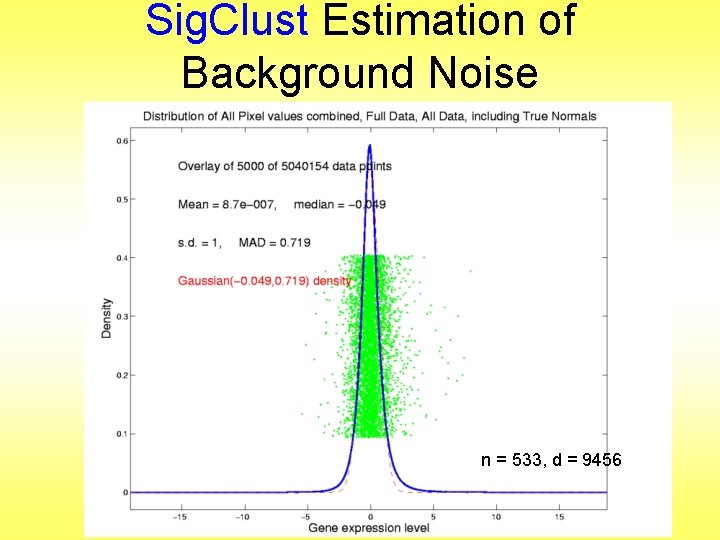
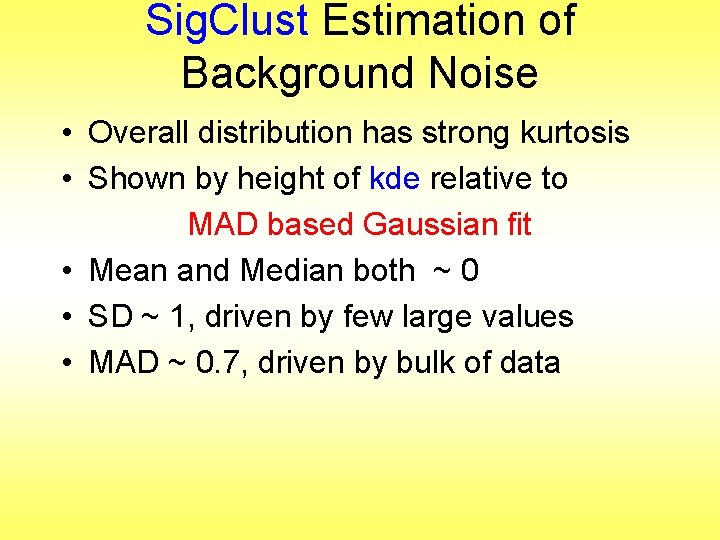
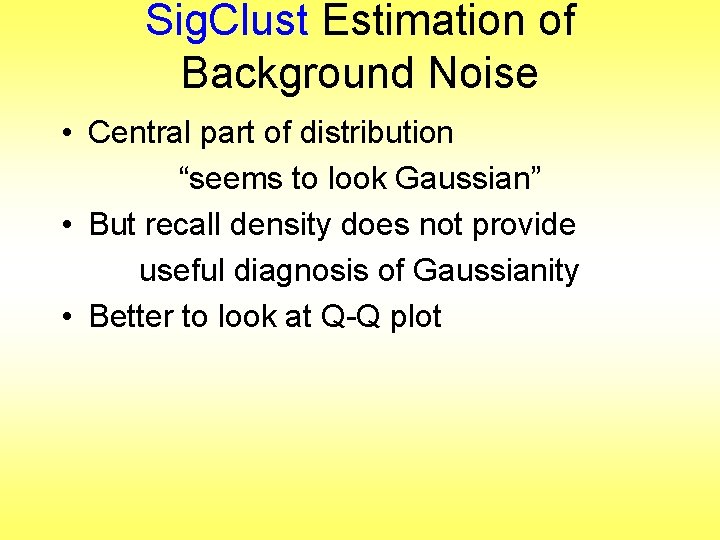
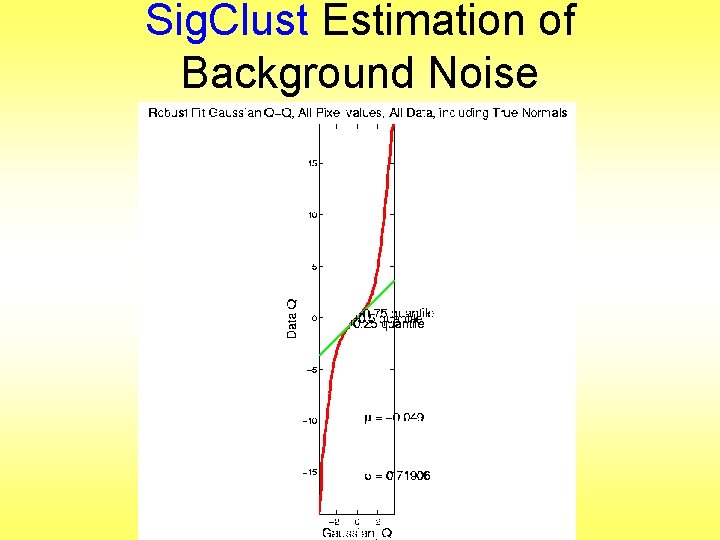
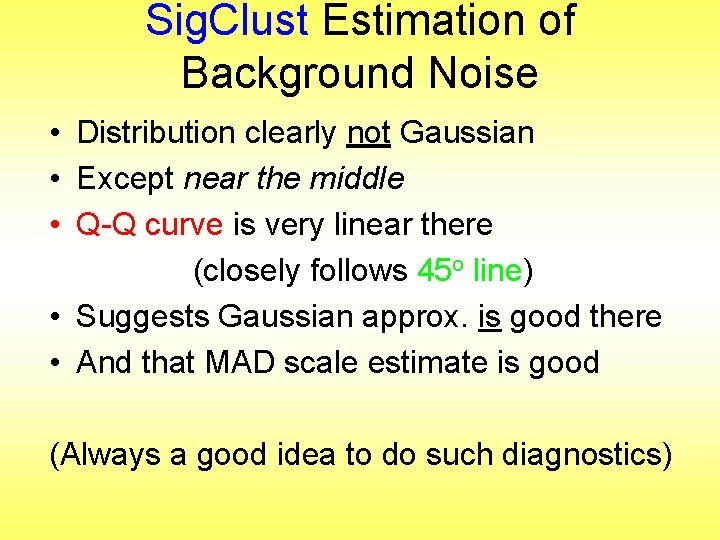
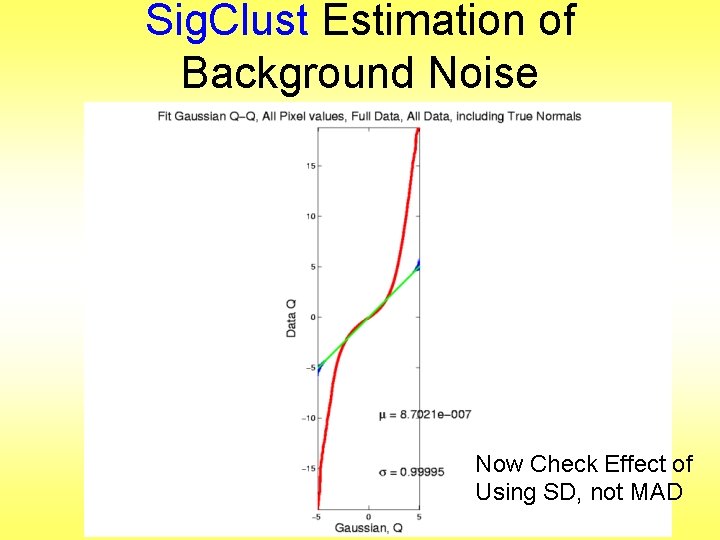
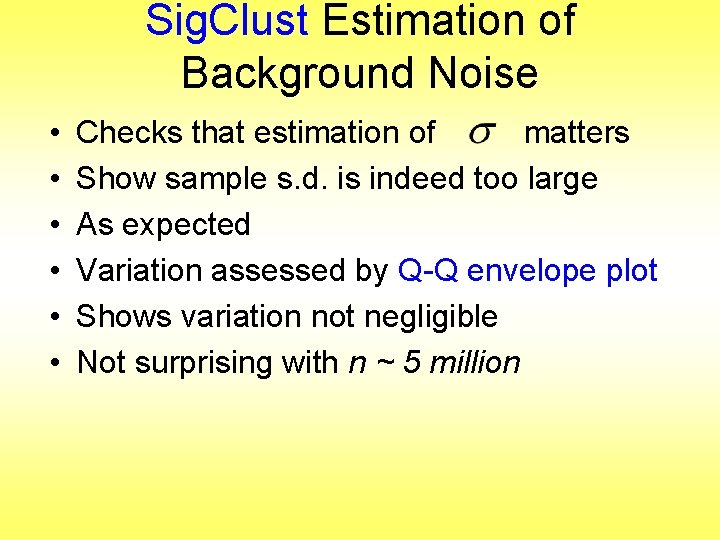
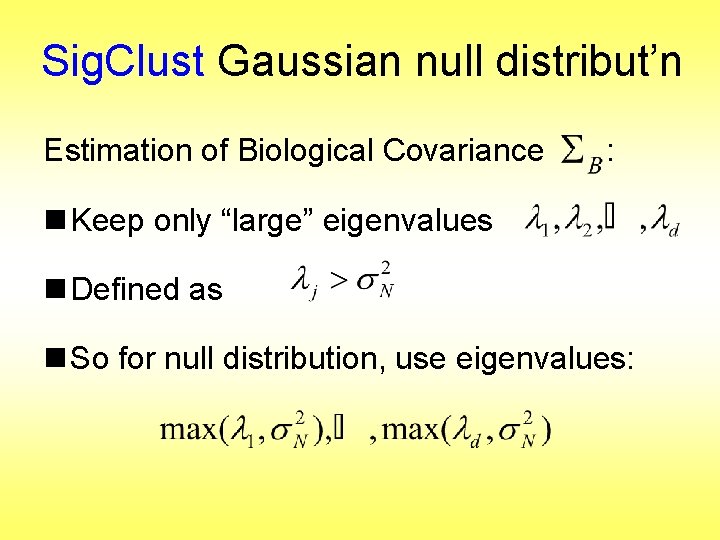
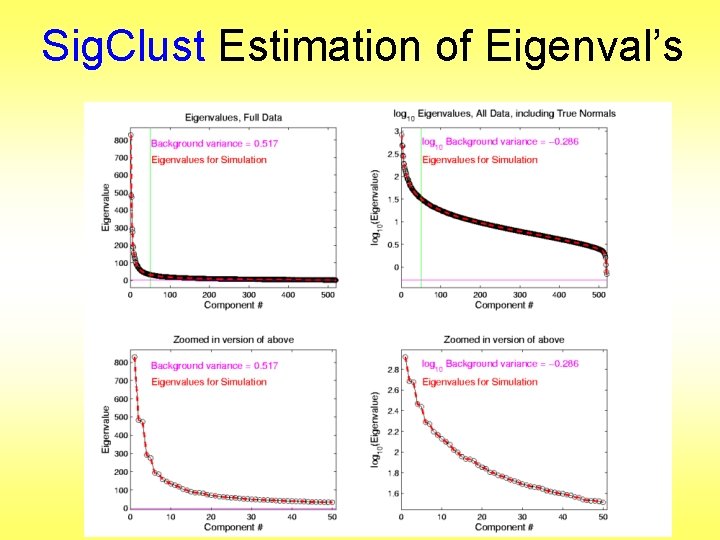
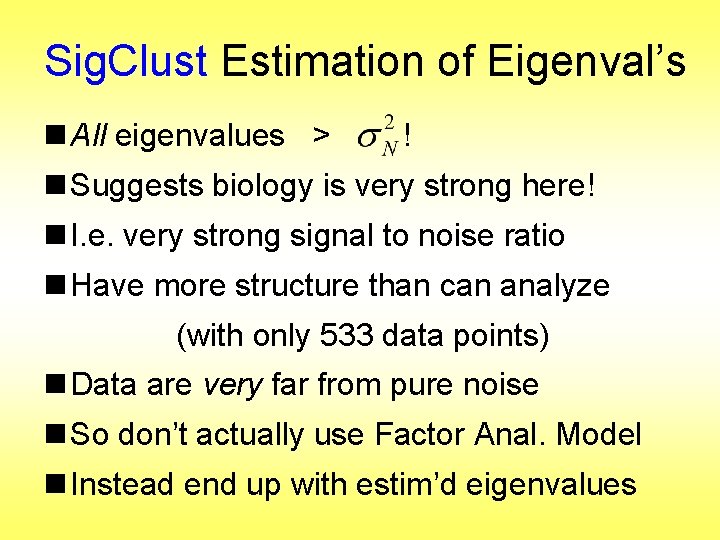
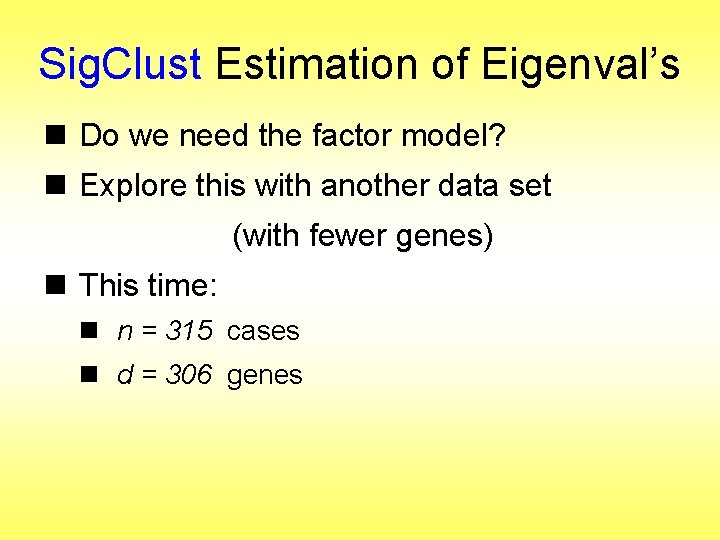
- Slides: 106
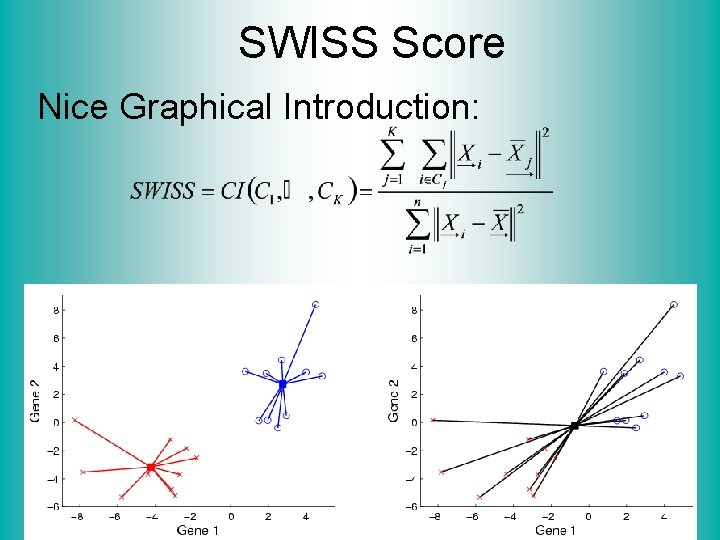
SWISS Score Nice Graphical Introduction:
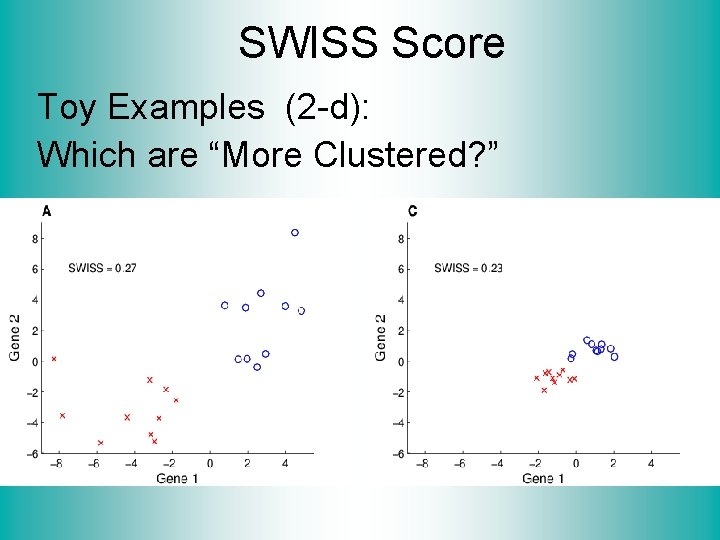
SWISS Score Toy Examples (2 -d): Which are “More Clustered? ”
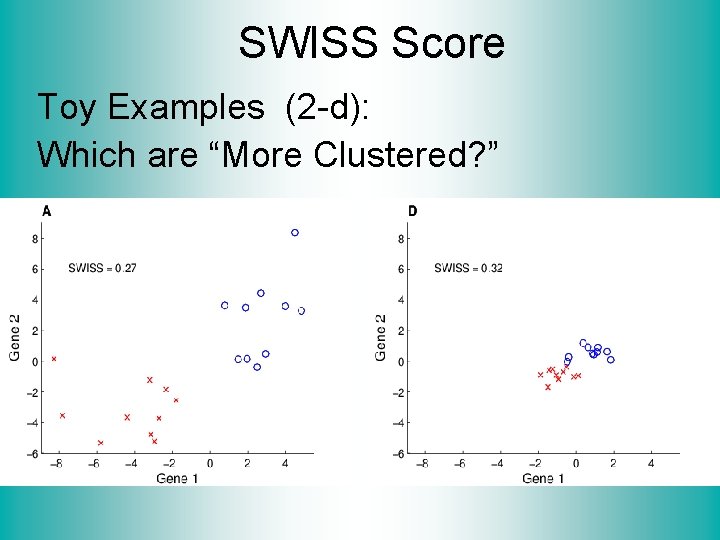
SWISS Score Toy Examples (2 -d): Which are “More Clustered? ”
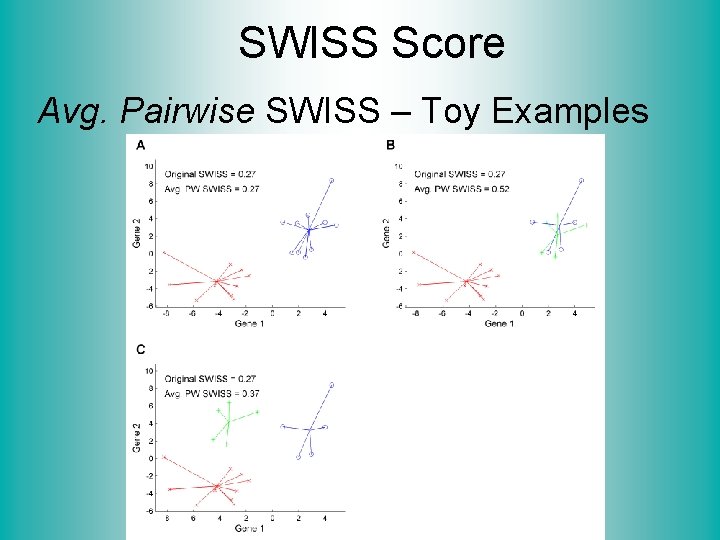
SWISS Score Avg. Pairwise SWISS – Toy Examples
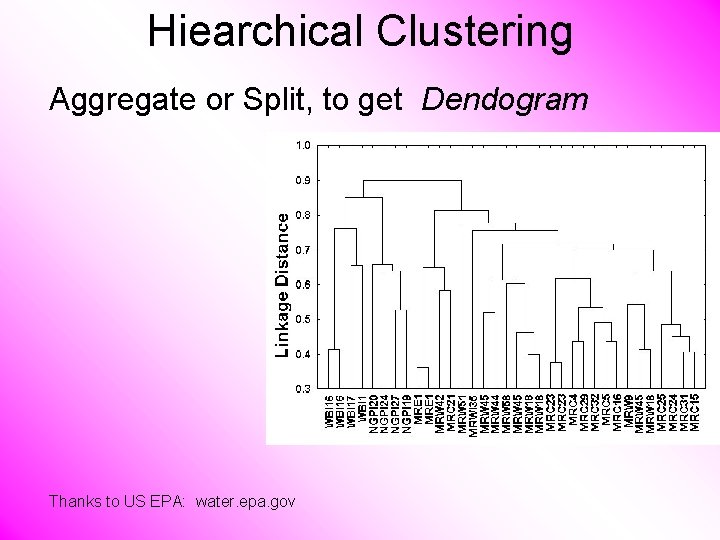
Hiearchical Clustering Aggregate or Split, to get Dendogram Thanks to US EPA: water. epa. gov
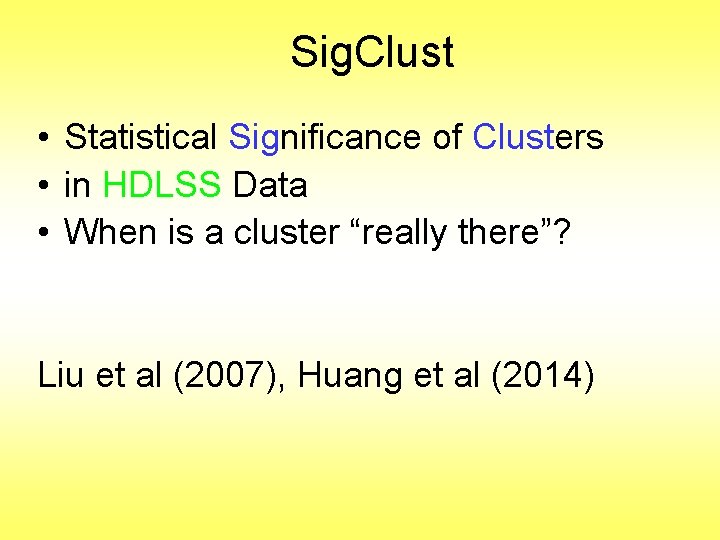
Sig. Clust • Statistical Significance of Clusters • in HDLSS Data • When is a cluster “really there”? Liu et al (2007), Huang et al (2014)
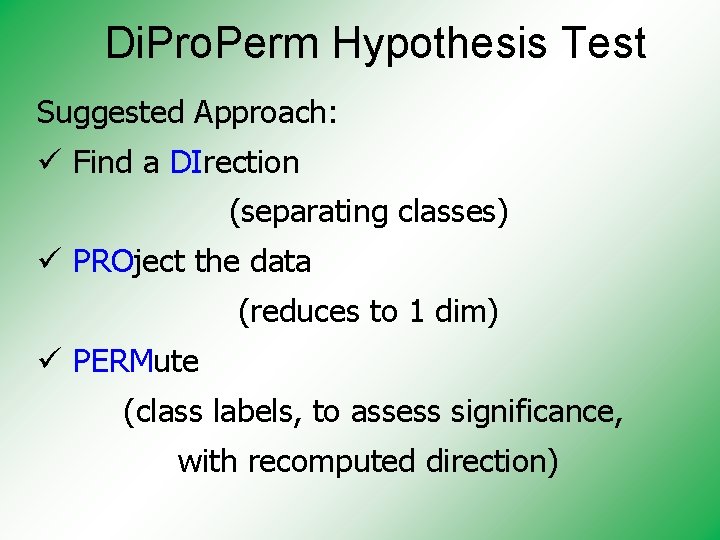
Di. Pro. Perm Hypothesis Test Suggested Approach: ü Find a DIrection (separating classes) ü PROject the data (reduces to 1 dim) ü PERMute (class labels, to assess significance, with recomputed direction)
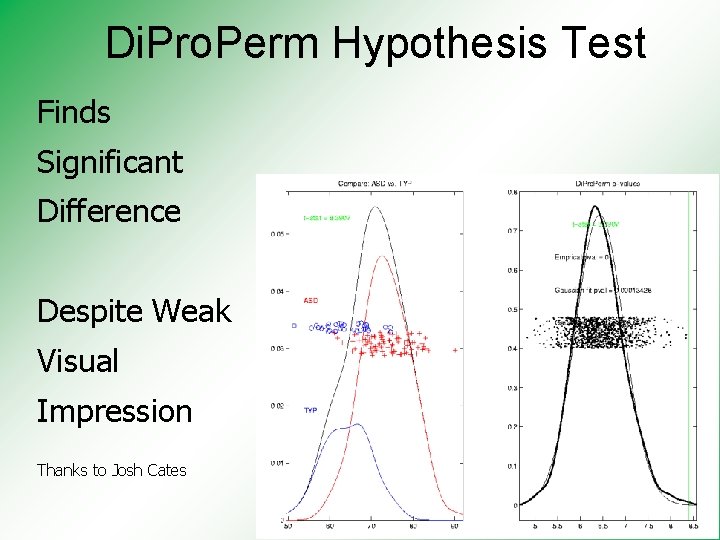
Di. Pro. Perm Hypothesis Test Finds Significant Difference Despite Weak Visual Impression Thanks to Josh Cates
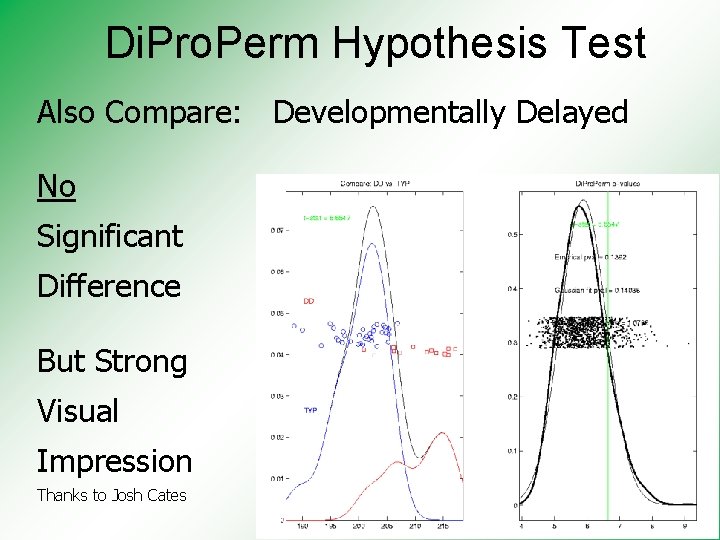
Di. Pro. Perm Hypothesis Test Also Compare: Developmentally Delayed No Significant Difference But Strong Visual Impression Thanks to Josh Cates
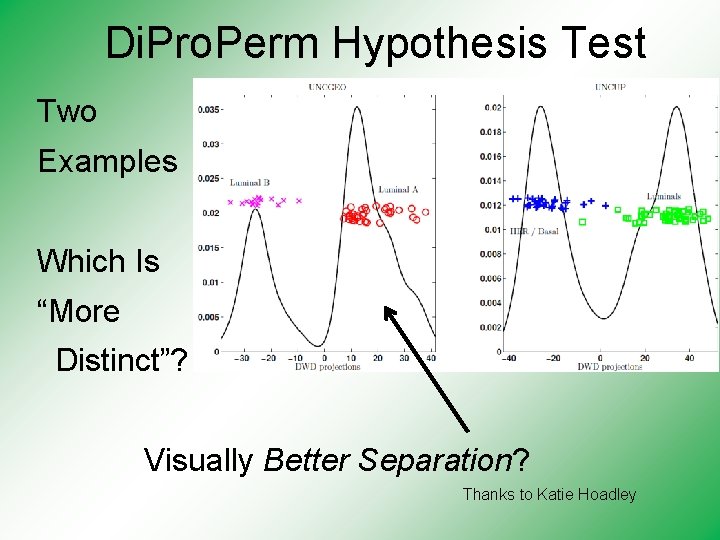
Di. Pro. Perm Hypothesis Test Two Examples Which Is “More Distinct”? Visually Better Separation? Thanks to Katie Hoadley
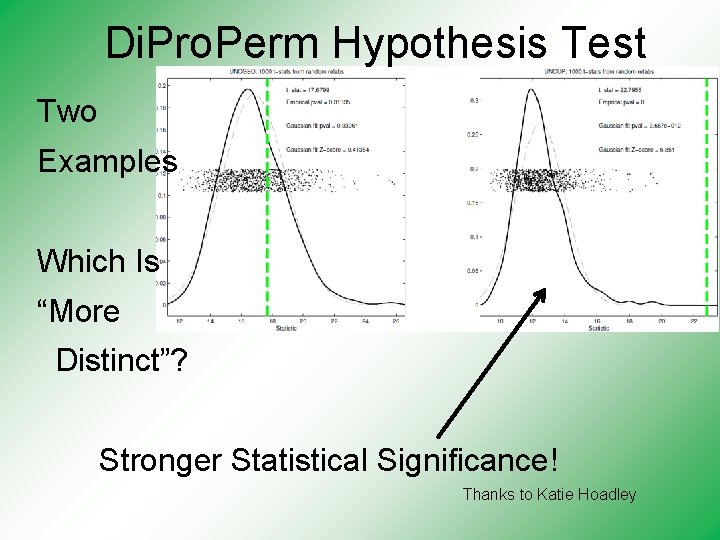
Di. Pro. Perm Hypothesis Test Two Examples Which Is “More Distinct”? Stronger Statistical Significance! Thanks to Katie Hoadley
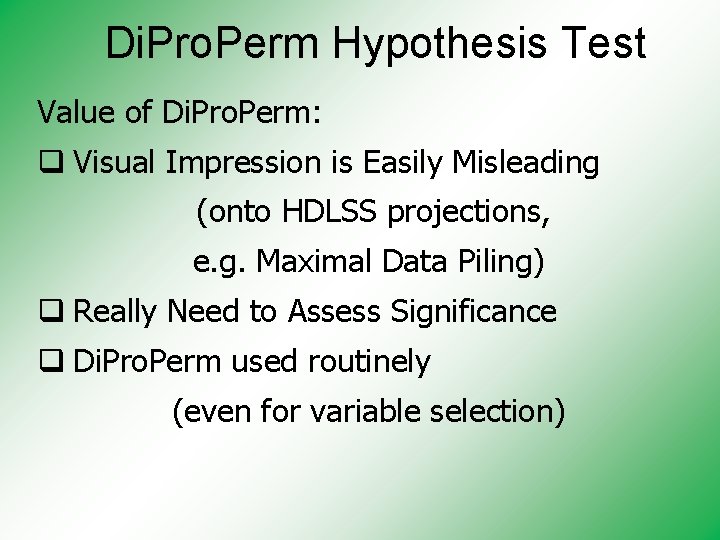
Di. Pro. Perm Hypothesis Test Value of Di. Pro. Perm: q Visual Impression is Easily Misleading (onto HDLSS projections, e. g. Maximal Data Piling) q Really Need to Assess Significance q Di. Pro. Perm used routinely (even for variable selection)
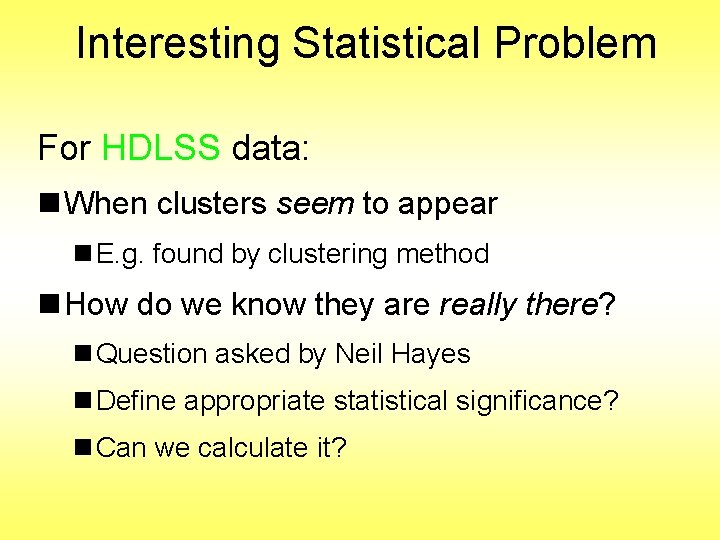
Interesting Statistical Problem For HDLSS data: n When clusters seem to appear n E. g. found by clustering method n How do we know they are really there? n Question asked by Neil Hayes n Define appropriate statistical significance? n Can we calculate it?
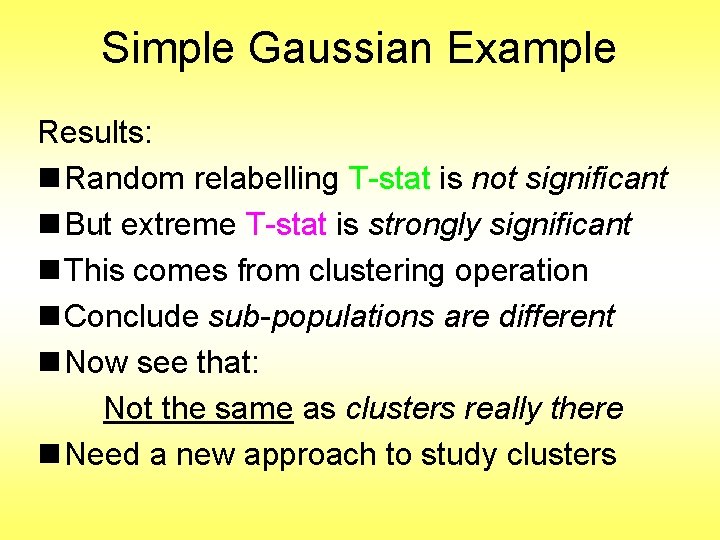
Simple Gaussian Example Results: n Random relabelling T-stat is not significant n But extreme T-stat is strongly significant n This comes from clustering operation n Conclude sub-populations are different n Now see that: Not the same as clusters really there n Need a new approach to study clusters
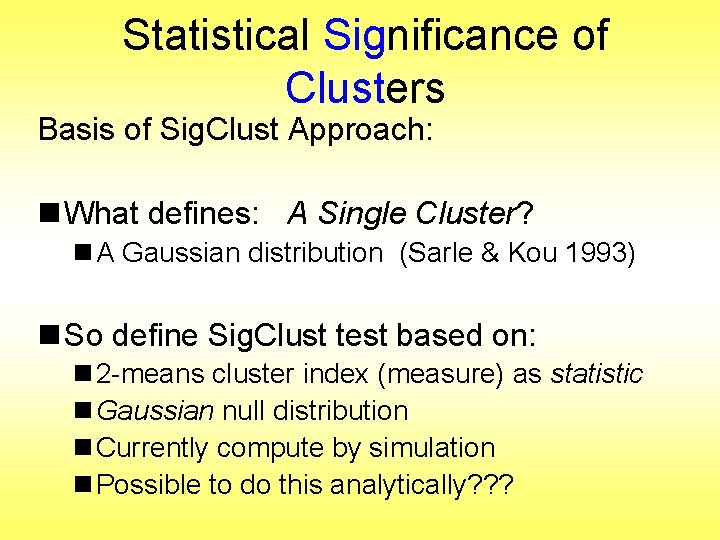
Statistical Significance of Clusters Basis of Sig. Clust Approach: n What defines: A Single Cluster? n A Gaussian distribution (Sarle & Kou 1993) n So define Sig. Clust test based on: n 2 -means cluster index (measure) as statistic n Gaussian null distribution n Currently compute by simulation n Possible to do this analytically? ? ?
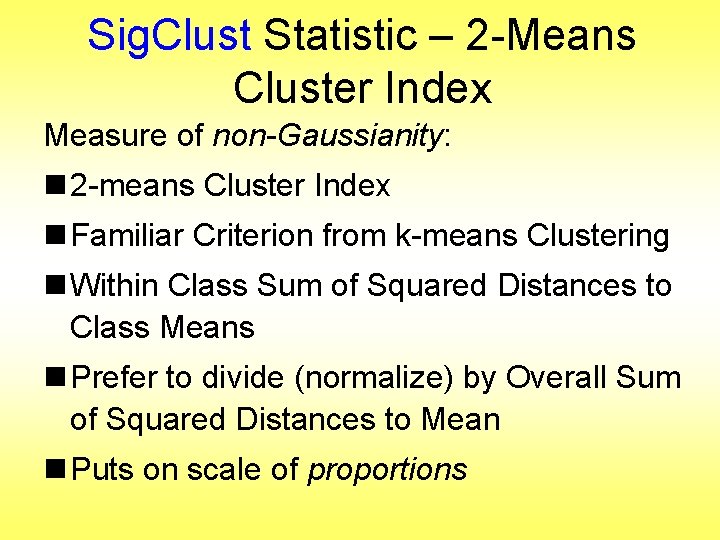
Sig. Clust Statistic – 2 -Means Cluster Index Measure of non-Gaussianity: n 2 -means Cluster Index n Familiar Criterion from k-means Clustering n Within Class Sum of Squared Distances to Class Means n Prefer to divide (normalize) by Overall Sum of Squared Distances to Mean n Puts on scale of proportions
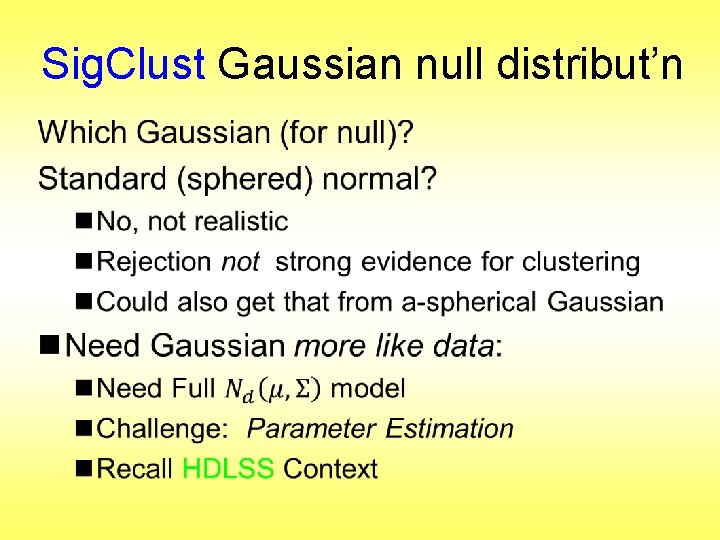
Sig. Clust Gaussian null distribut’n •
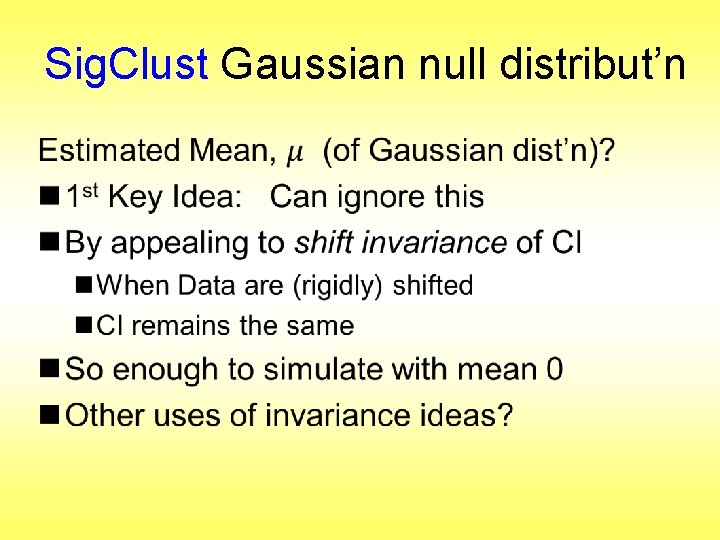
Sig. Clust Gaussian null distribut’n •
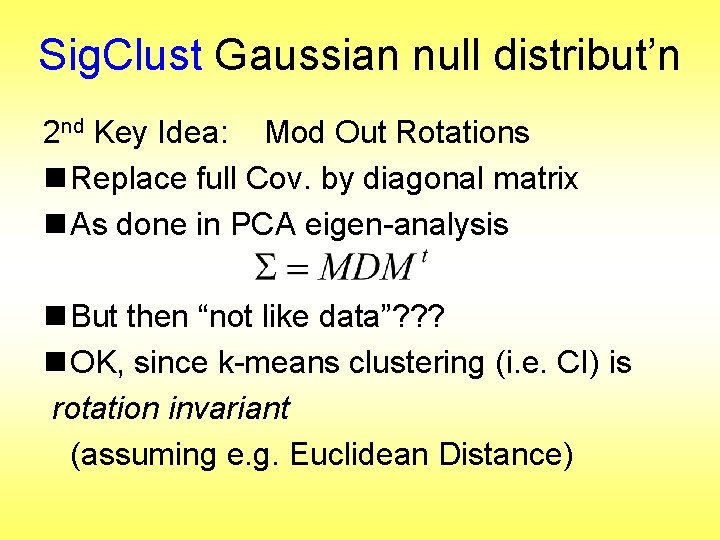
Sig. Clust Gaussian null distribut’n 2 nd Key Idea: Mod Out Rotations n Replace full Cov. by diagonal matrix n As done in PCA eigen-analysis n But then “not like data”? ? ? n OK, since k-means clustering (i. e. CI) is rotation invariant (assuming e. g. Euclidean Distance)
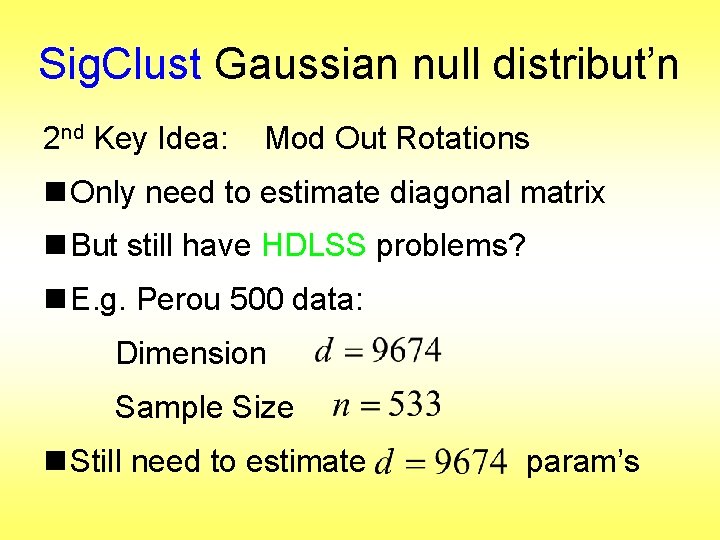
Sig. Clust Gaussian null distribut’n 2 nd Key Idea: Mod Out Rotations n Only need to estimate diagonal matrix n But still have HDLSS problems? n E. g. Perou 500 data: Dimension Sample Size n Still need to estimate param’s
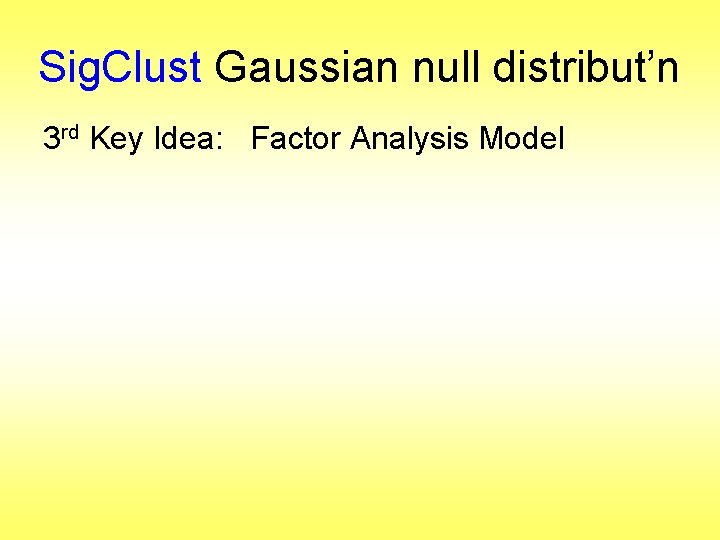
Sig. Clust Gaussian null distribut’n 3 rd Key Idea: Factor Analysis Model
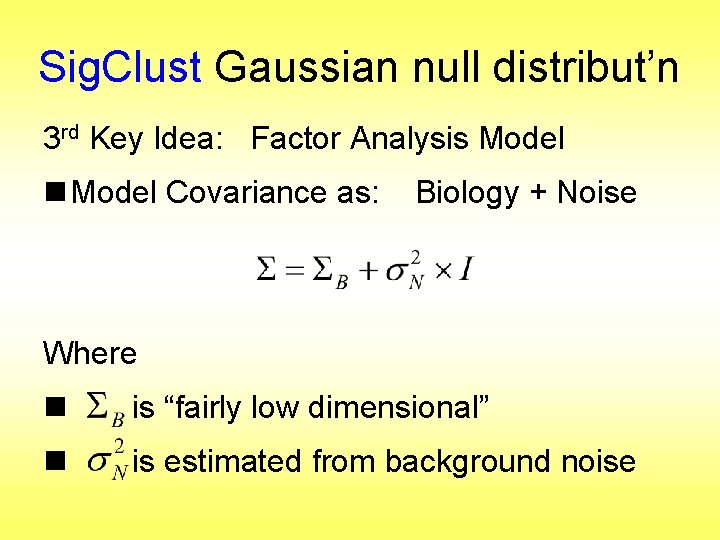
Sig. Clust Gaussian null distribut’n 3 rd Key Idea: Factor Analysis Model n Model Covariance as: Biology + Noise Where n is “fairly low dimensional” n is estimated from background noise
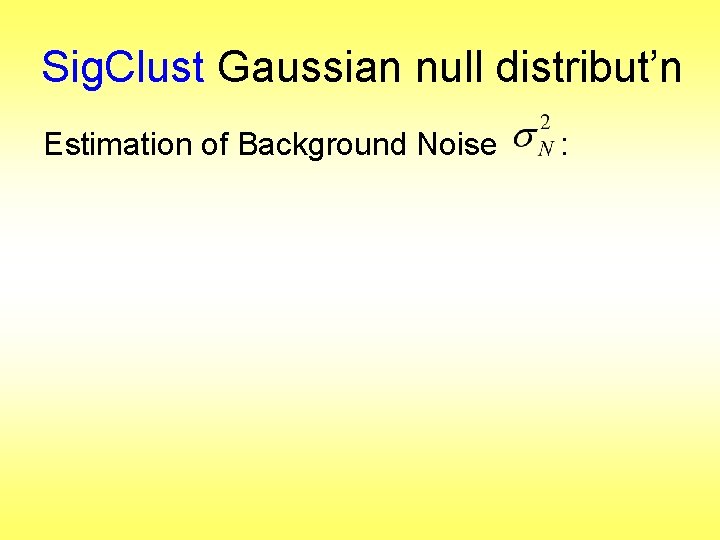
Sig. Clust Gaussian null distribut’n Estimation of Background Noise :
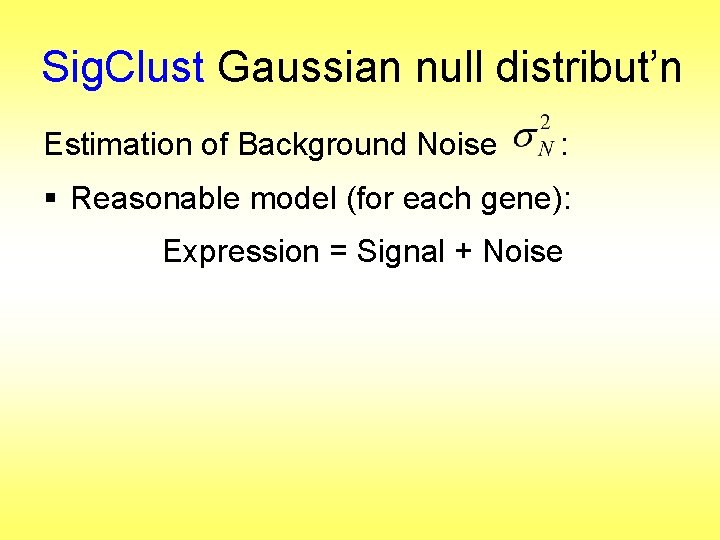
Sig. Clust Gaussian null distribut’n Estimation of Background Noise : § Reasonable model (for each gene): Expression = Signal + Noise
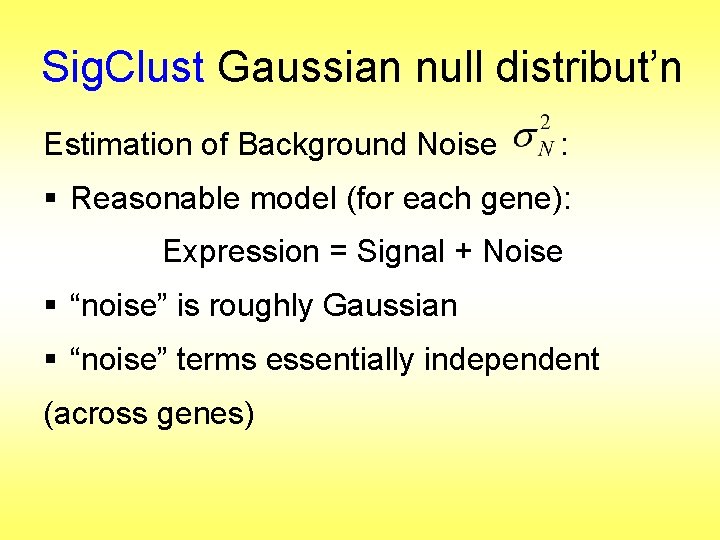
Sig. Clust Gaussian null distribut’n Estimation of Background Noise : § Reasonable model (for each gene): Expression = Signal + Noise § “noise” is roughly Gaussian § “noise” terms essentially independent (across genes)
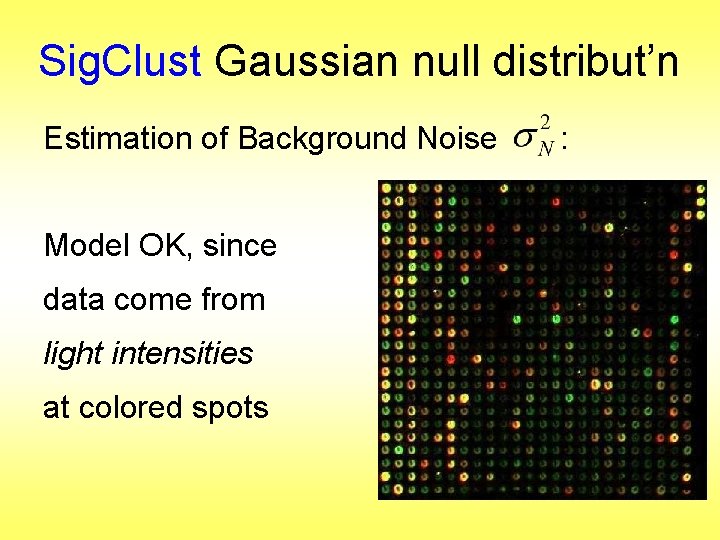
Sig. Clust Gaussian null distribut’n Estimation of Background Noise Model OK, since data come from light intensities at colored spots :
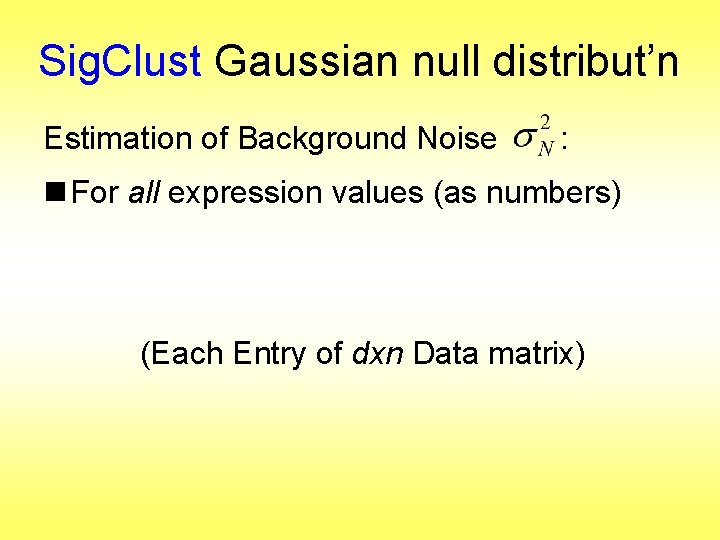
Sig. Clust Gaussian null distribut’n Estimation of Background Noise : n For all expression values (as numbers) (Each Entry of dxn Data matrix)
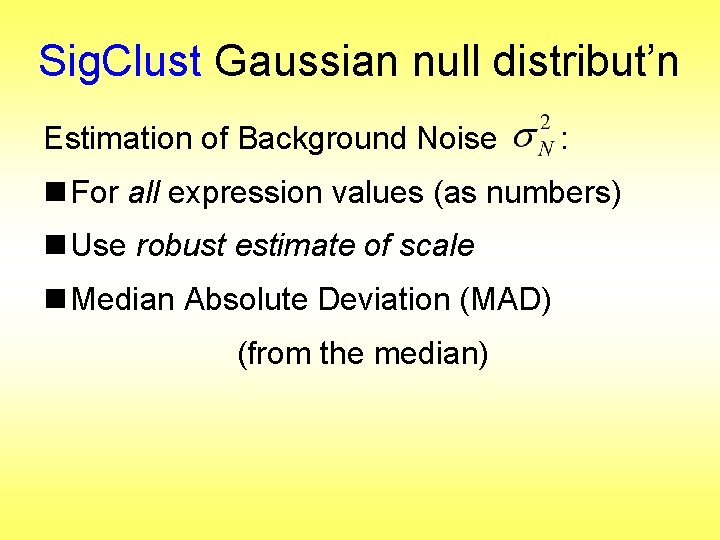
Sig. Clust Gaussian null distribut’n Estimation of Background Noise : n For all expression values (as numbers) n Use robust estimate of scale n Median Absolute Deviation (MAD) (from the median)
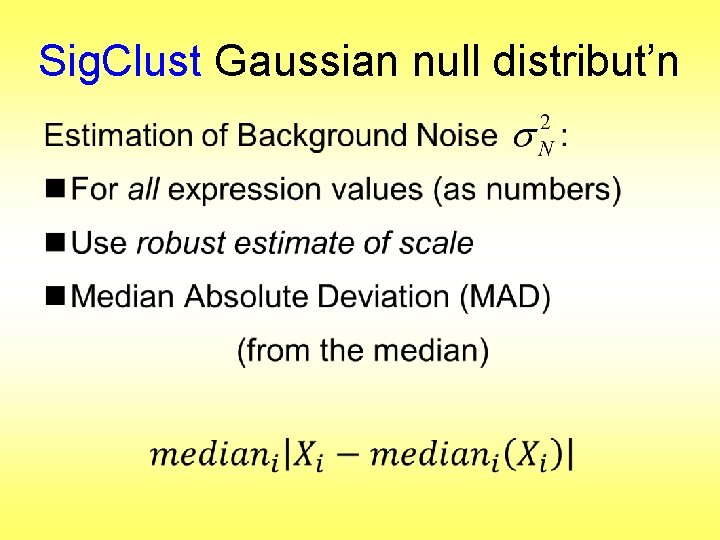
Sig. Clust Gaussian null distribut’n •
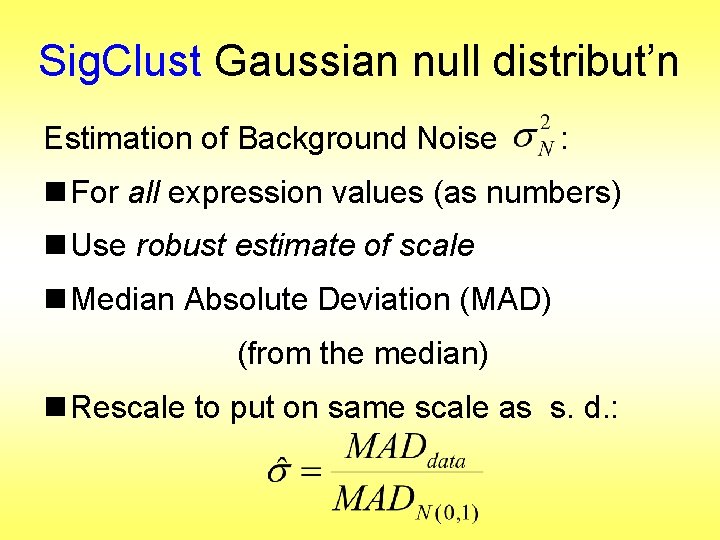
Sig. Clust Gaussian null distribut’n Estimation of Background Noise : n For all expression values (as numbers) n Use robust estimate of scale n Median Absolute Deviation (MAD) (from the median) n Rescale to put on same scale as s. d. :
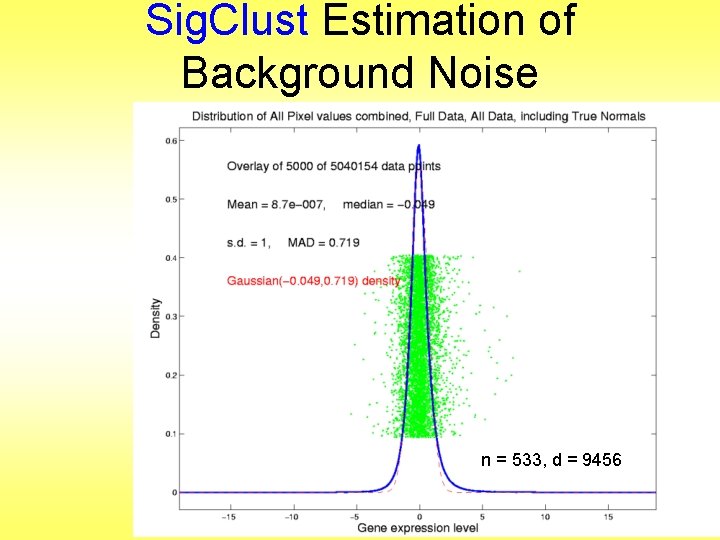
Sig. Clust Estimation of Background Noise n = 533, d = 9456
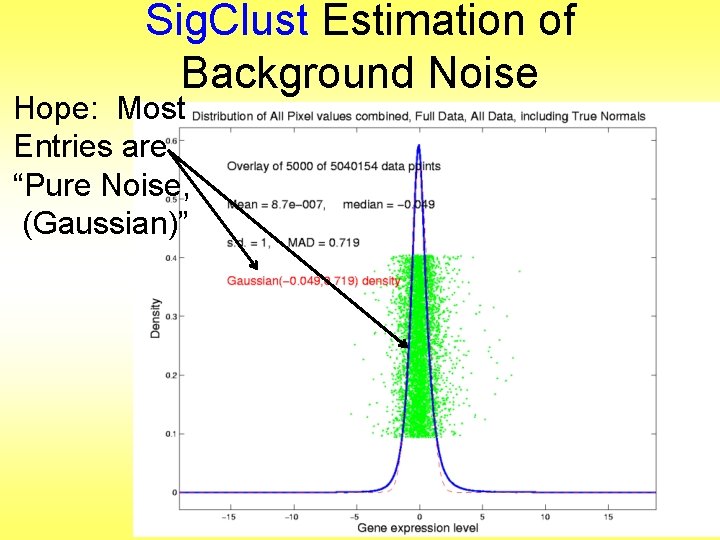
Sig. Clust Estimation of Background Noise Hope: Most Entries are “Pure Noise, (Gaussian)”
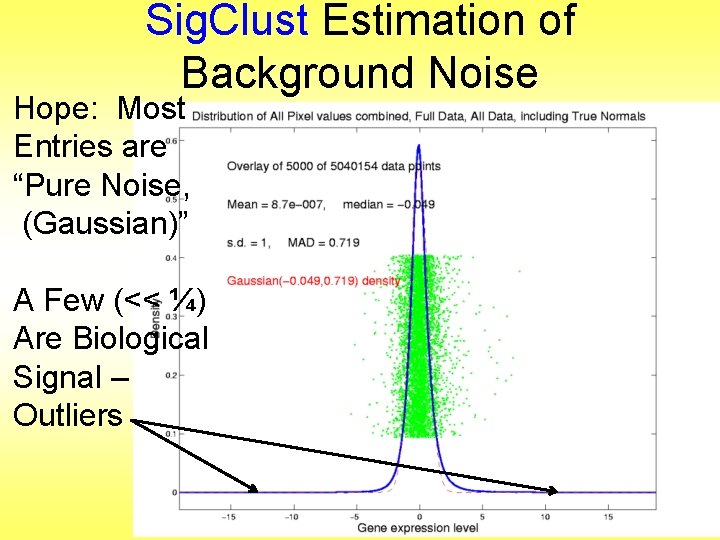
Sig. Clust Estimation of Background Noise Hope: Most Entries are “Pure Noise, (Gaussian)” A Few (<< ¼) Are Biological Signal – Outliers
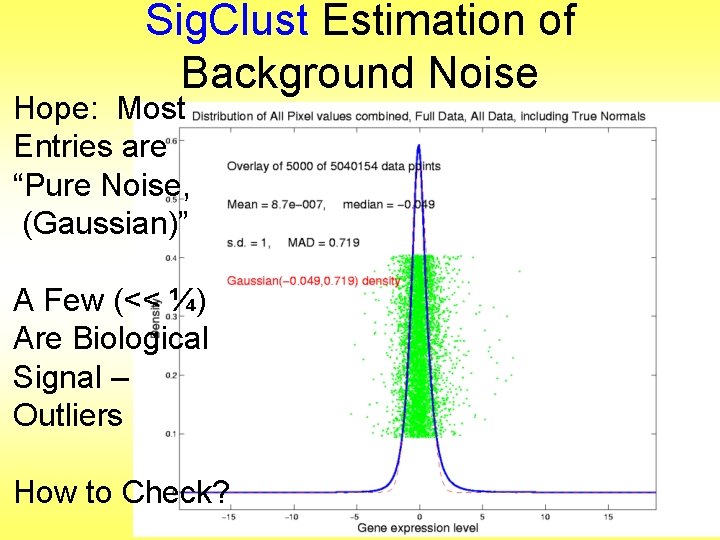
Sig. Clust Estimation of Background Noise Hope: Most Entries are “Pure Noise, (Gaussian)” A Few (<< ¼) Are Biological Signal – Outliers How to Check?
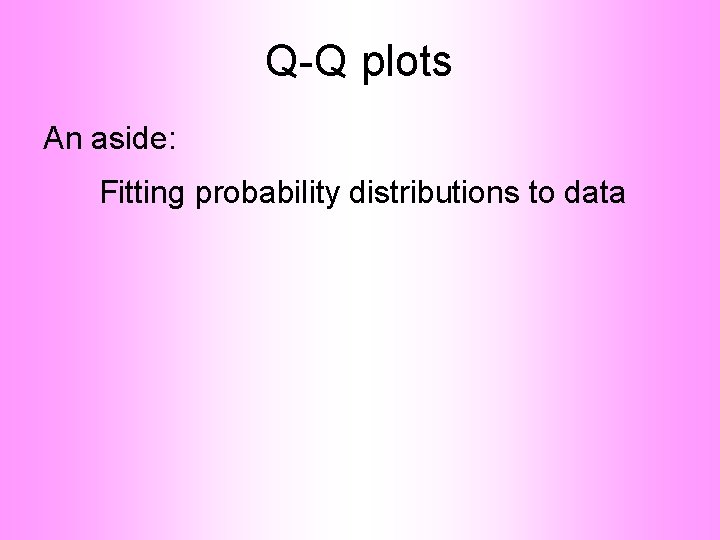
Q-Q plots An aside: Fitting probability distributions to data
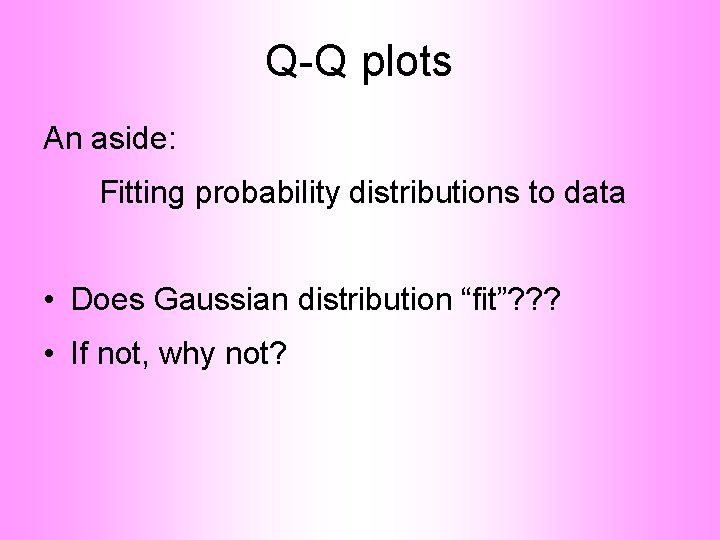
Q-Q plots An aside: Fitting probability distributions to data • Does Gaussian distribution “fit”? ? ? • If not, why not?
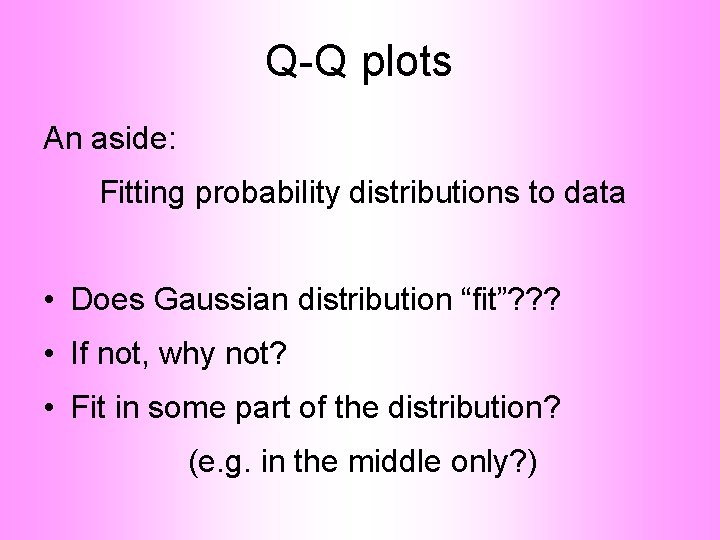
Q-Q plots An aside: Fitting probability distributions to data • Does Gaussian distribution “fit”? ? ? • If not, why not? • Fit in some part of the distribution? (e. g. in the middle only? )
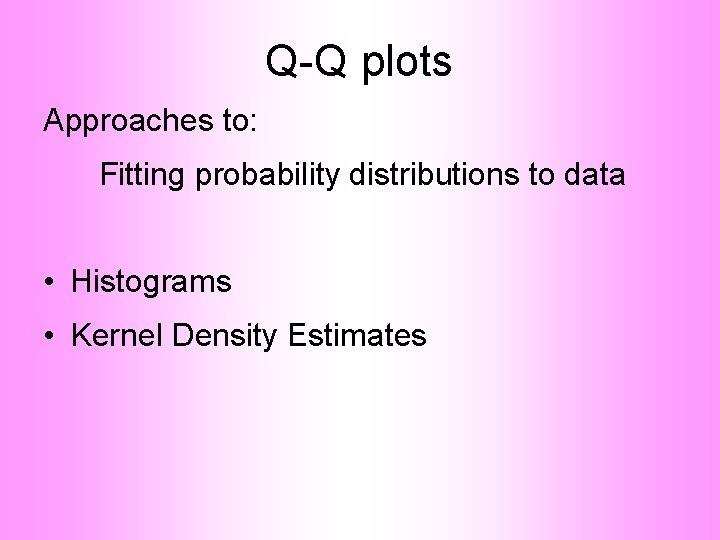
Q-Q plots Approaches to: Fitting probability distributions to data • Histograms • Kernel Density Estimates
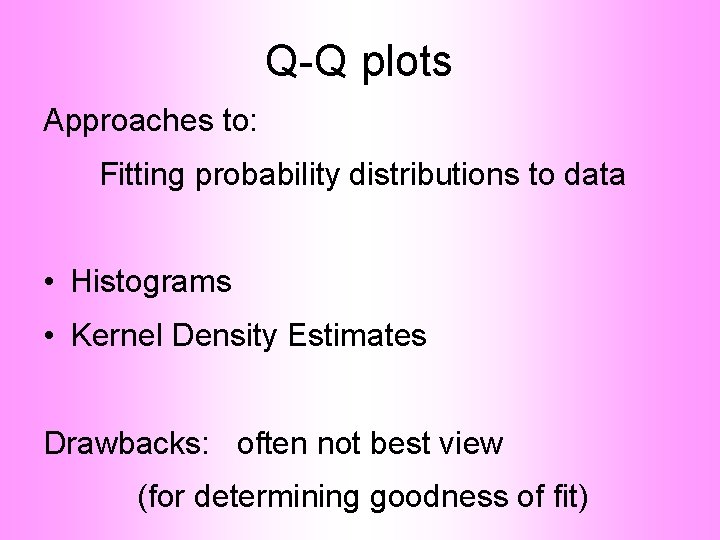
Q-Q plots Approaches to: Fitting probability distributions to data • Histograms • Kernel Density Estimates Drawbacks: often not best view (for determining goodness of fit)
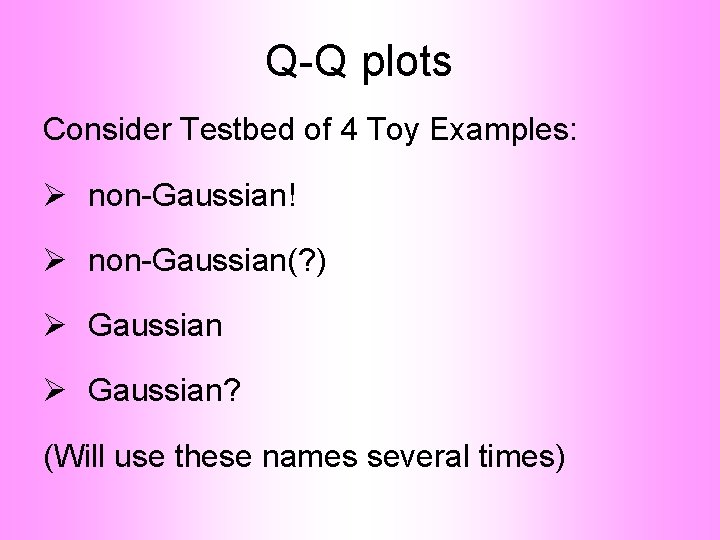
Q-Q plots Consider Testbed of 4 Toy Examples: Ø non-Gaussian! Ø non-Gaussian(? ) Ø Gaussian? (Will use these names several times)
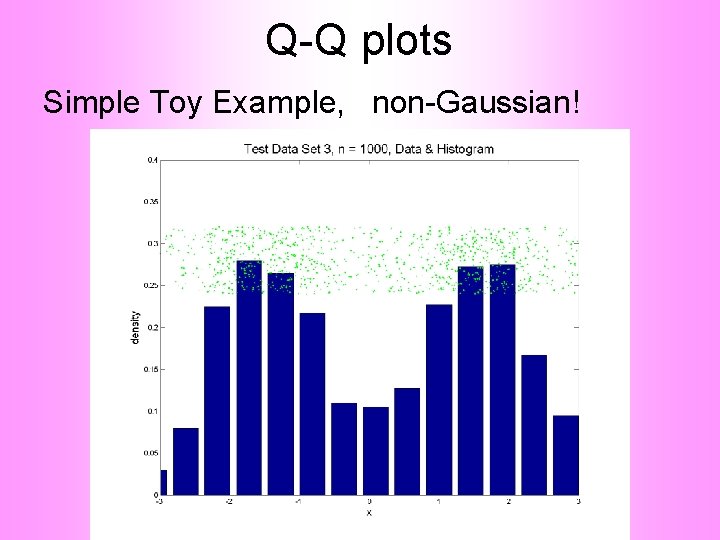
Q-Q plots Simple Toy Example, non-Gaussian!
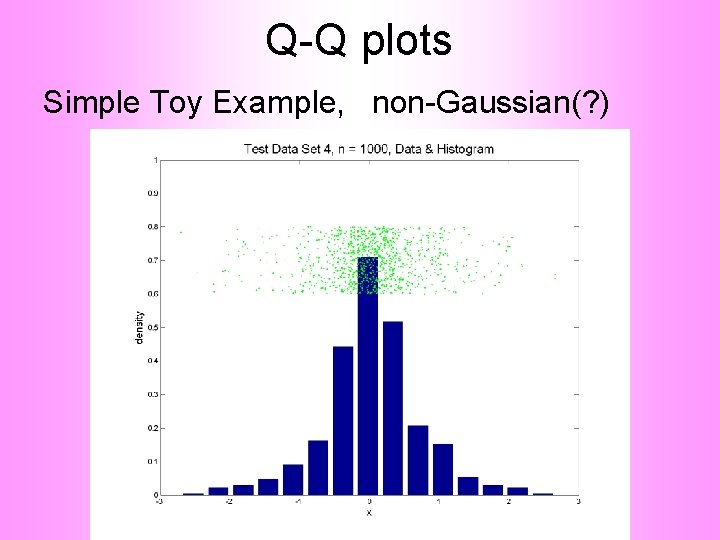
Q-Q plots Simple Toy Example, non-Gaussian(? )
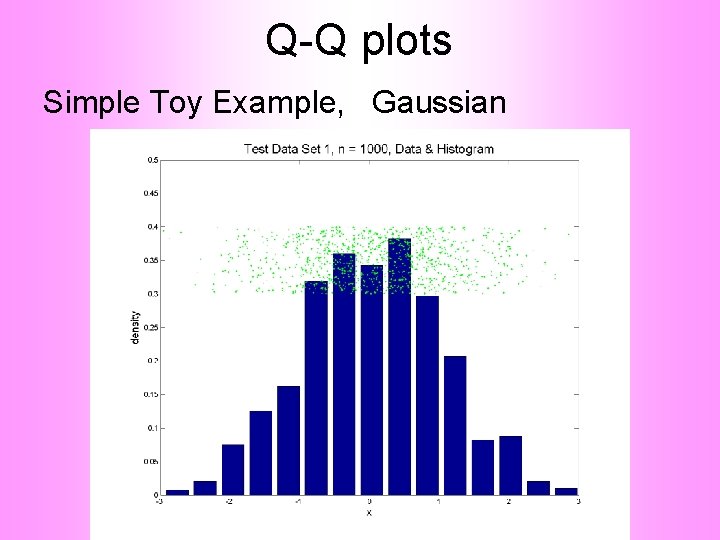
Q-Q plots Simple Toy Example, Gaussian
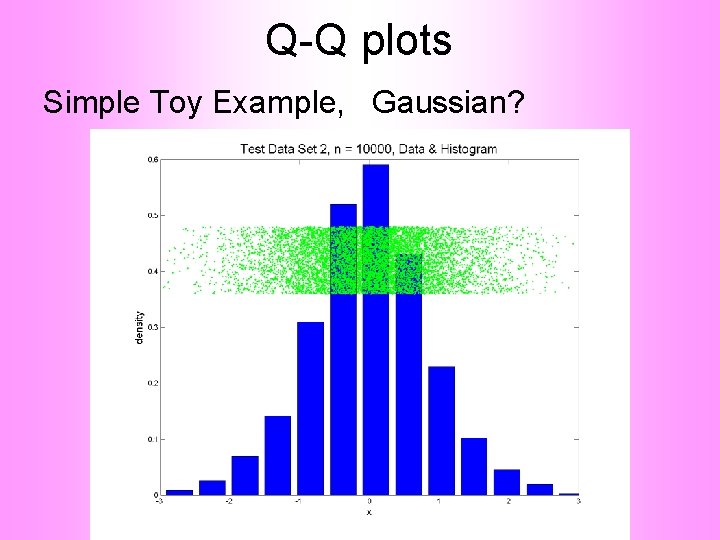
Q-Q plots Simple Toy Example, Gaussian?
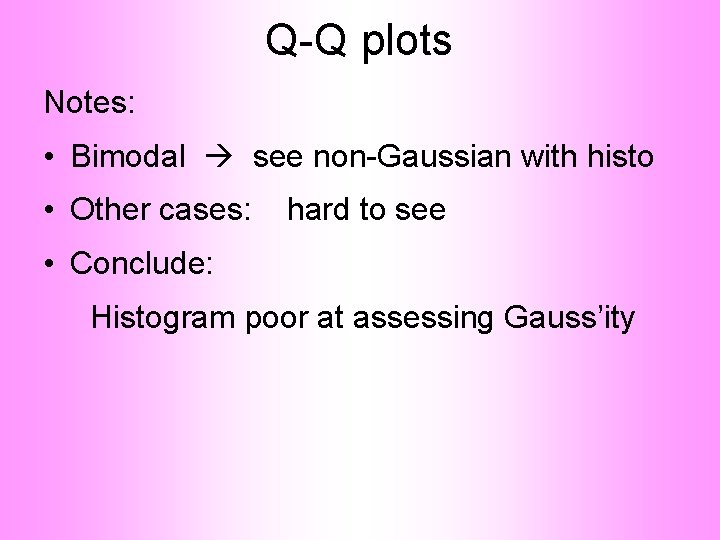
Q-Q plots Notes: • Bimodal see non-Gaussian with histo • Other cases: hard to see • Conclude: Histogram poor at assessing Gauss’ity
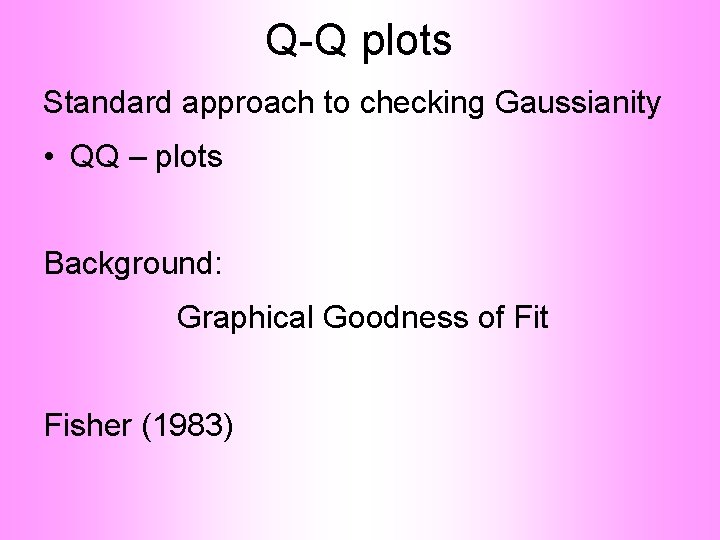
Q-Q plots Standard approach to checking Gaussianity • QQ – plots Background: Graphical Goodness of Fit Fisher (1983)
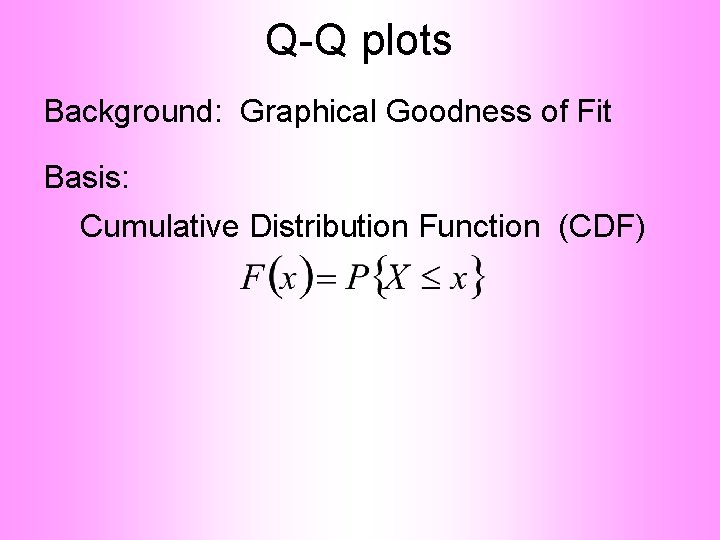
Q-Q plots Background: Graphical Goodness of Fit Basis: Cumulative Distribution Function (CDF)
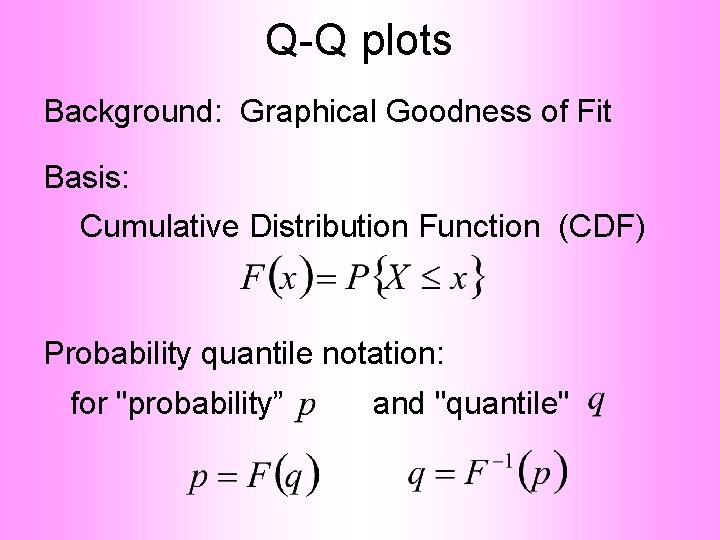
Q-Q plots Background: Graphical Goodness of Fit Basis: Cumulative Distribution Function (CDF) Probability quantile notation: for "probability” and "quantile"
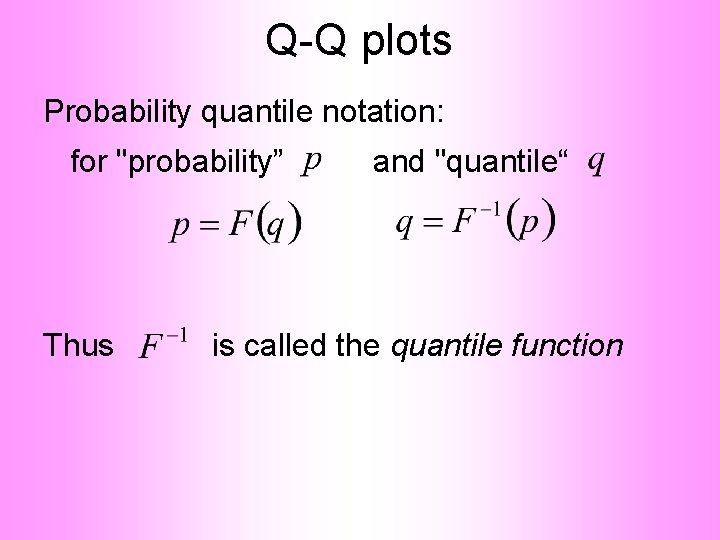
Q-Q plots Probability quantile notation: for "probability” Thus and "quantile“ is called the quantile function
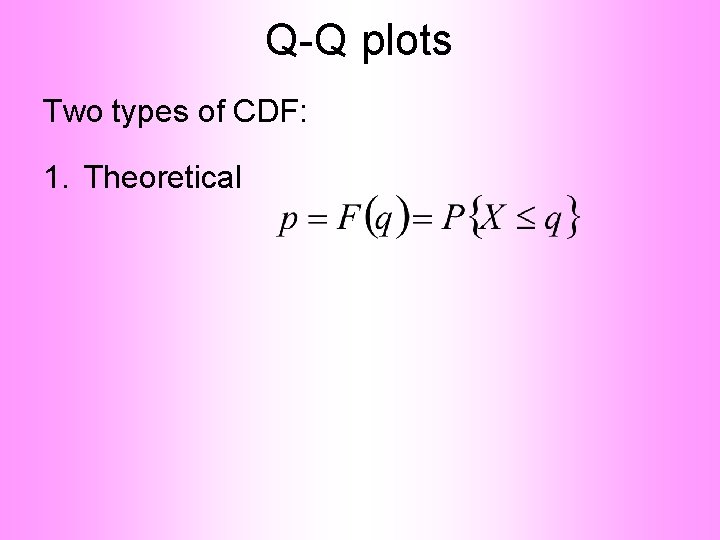
Q-Q plots Two types of CDF: 1. Theoretical
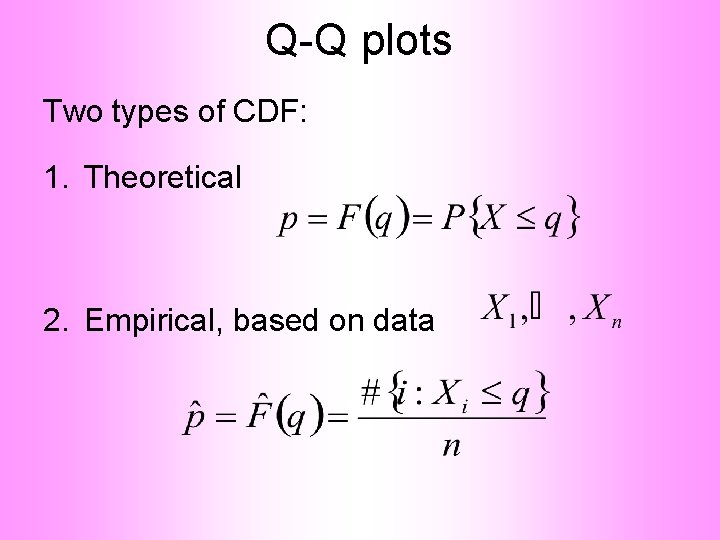
Q-Q plots Two types of CDF: 1. Theoretical 2. Empirical, based on data
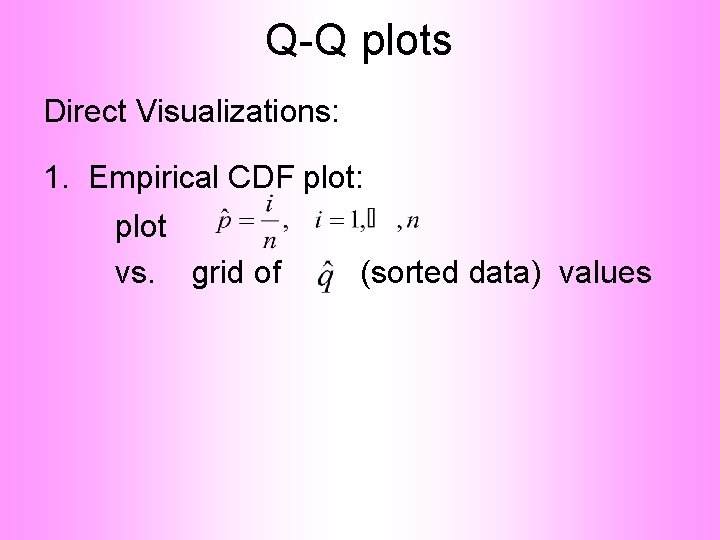
Q-Q plots Direct Visualizations: 1. Empirical CDF plot: plot vs. grid of (sorted data) values
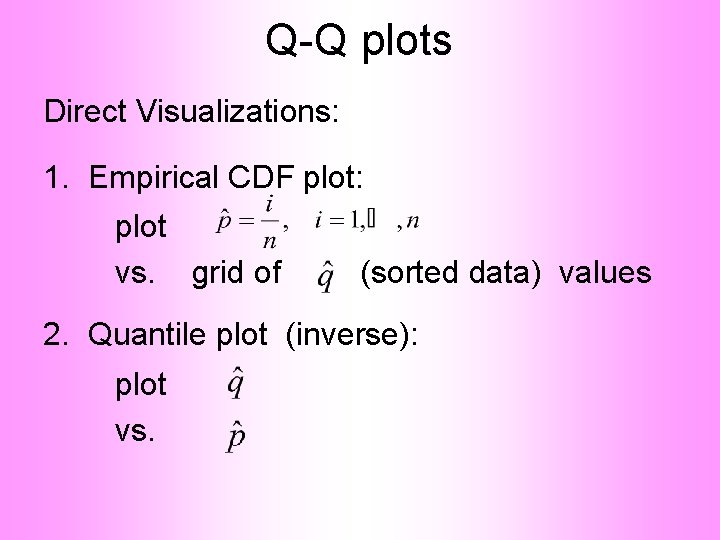
Q-Q plots Direct Visualizations: 1. Empirical CDF plot: plot vs. grid of (sorted data) values 2. Quantile plot (inverse): plot vs.
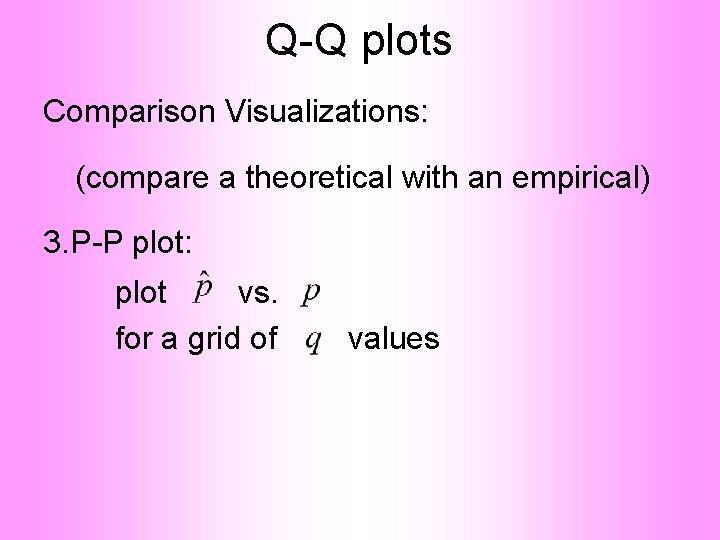
Q-Q plots Comparison Visualizations: (compare a theoretical with an empirical) 3. P-P plot: plot vs. for a grid of values
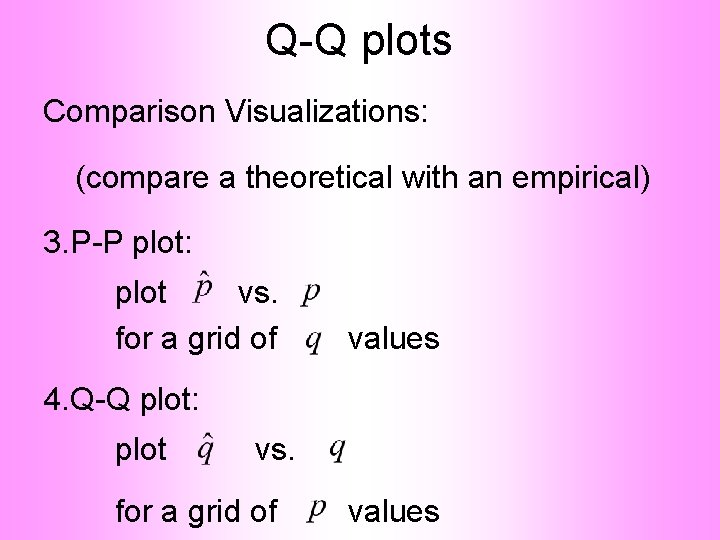
Q-Q plots Comparison Visualizations: (compare a theoretical with an empirical) 3. P-P plot: plot vs. for a grid of values 4. Q-Q plot: plot vs. for a grid of values
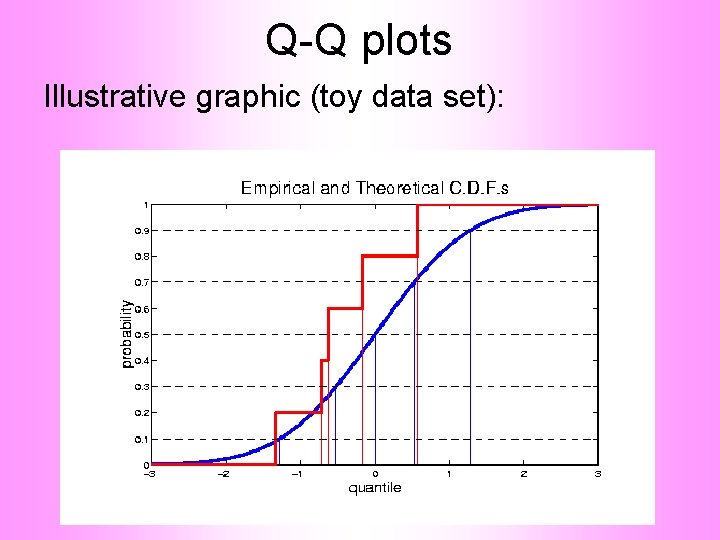
Q-Q plots Illustrative graphic (toy data set):
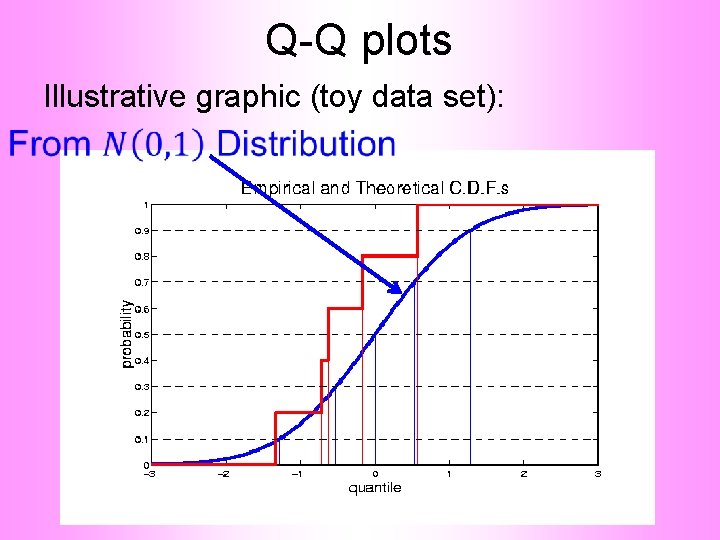
Q-Q plots Illustrative graphic (toy data set):
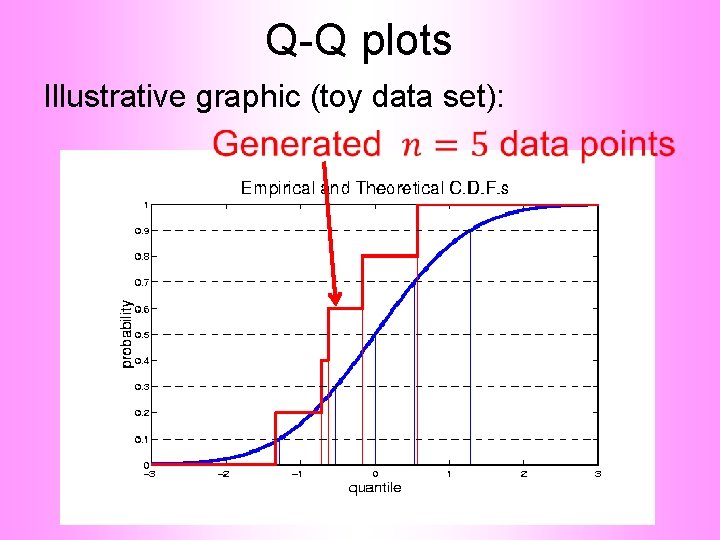
Q-Q plots Illustrative graphic (toy data set):
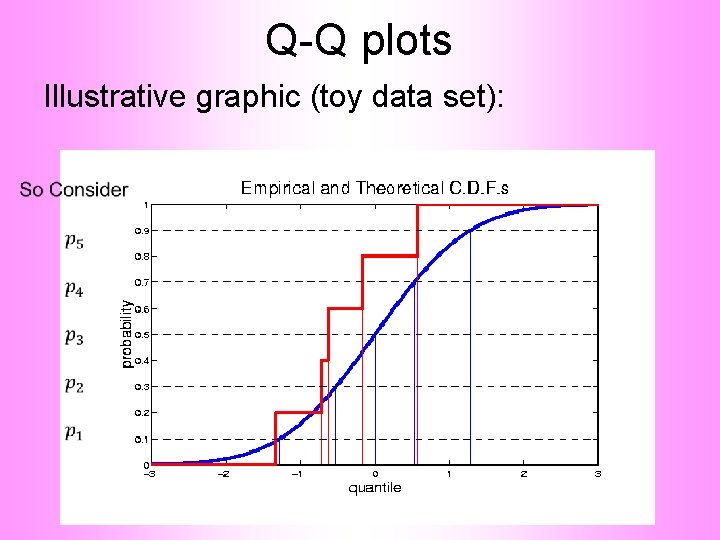
Q-Q plots Illustrative graphic (toy data set):
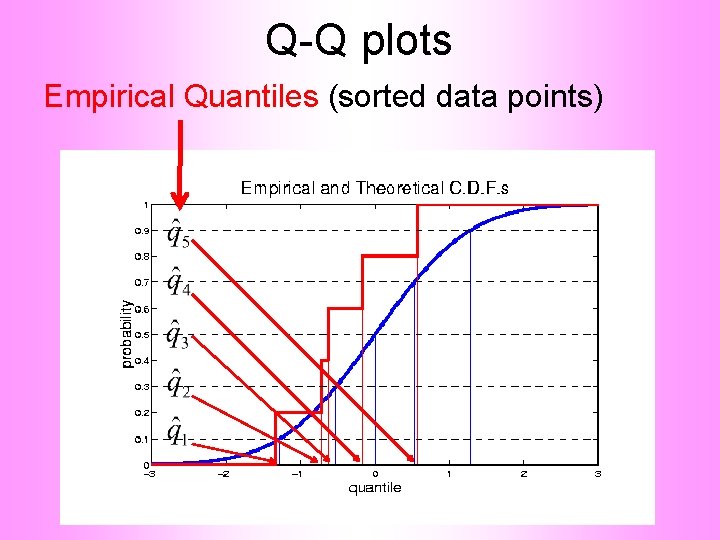
Q-Q plots Empirical Quantiles (sorted data points)
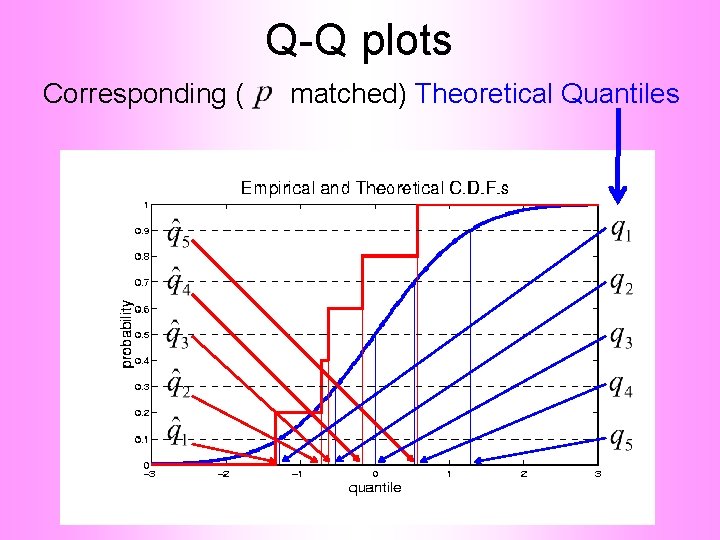
Q-Q plots Corresponding ( matched) Theoretical Quantiles
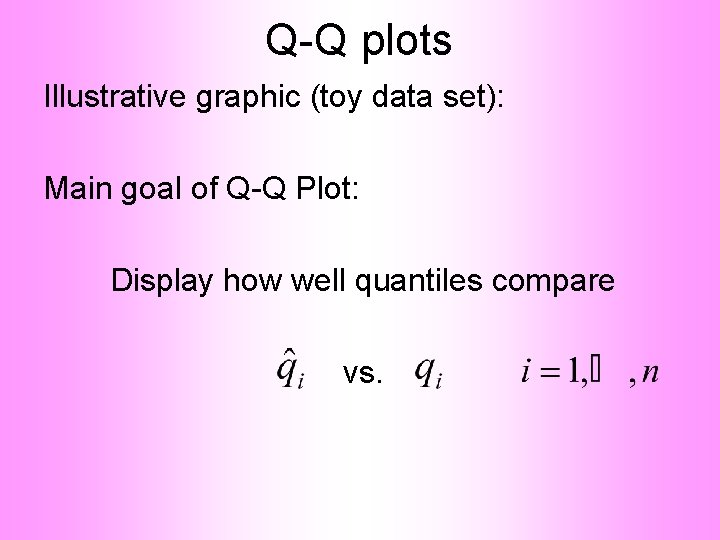
Q-Q plots Illustrative graphic (toy data set): Main goal of Q-Q Plot: Display how well quantiles compare vs.
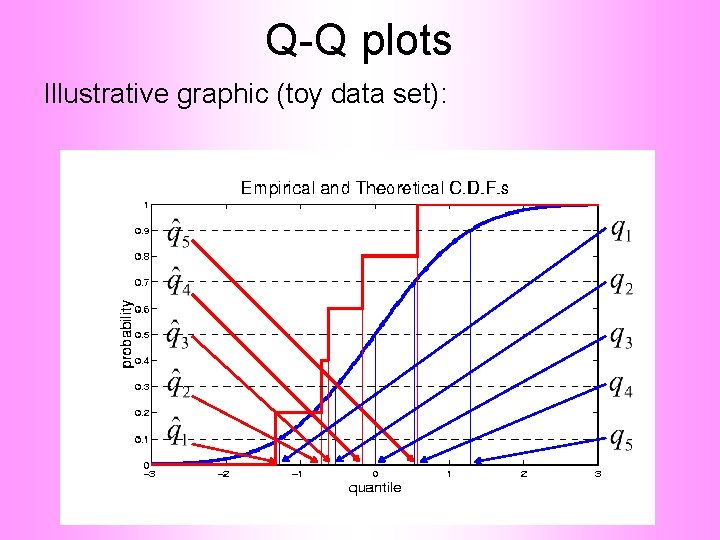
Q-Q plots Illustrative graphic (toy data set):
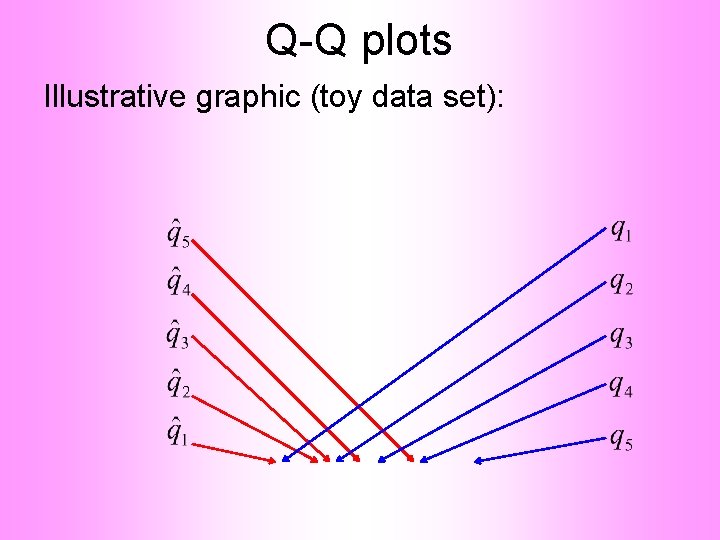
Q-Q plots Illustrative graphic (toy data set):
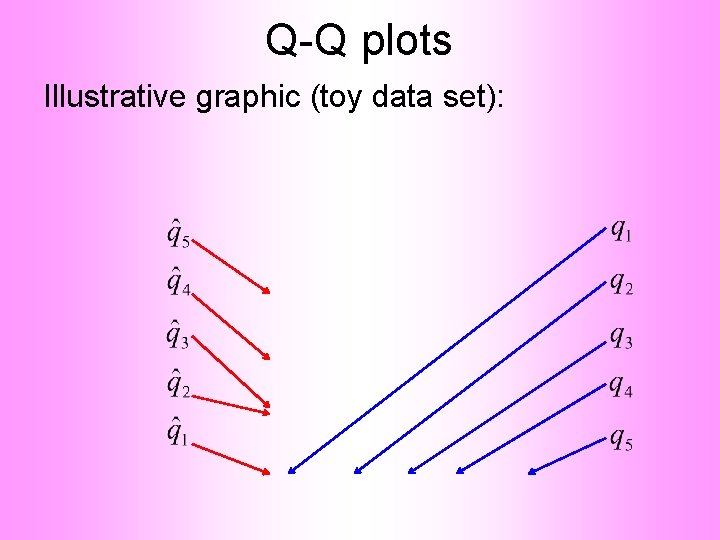
Q-Q plots Illustrative graphic (toy data set):
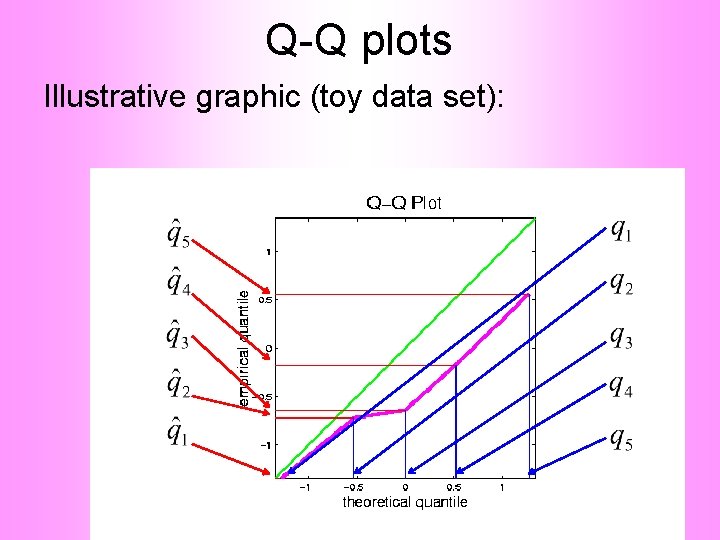
Q-Q plots Illustrative graphic (toy data set):
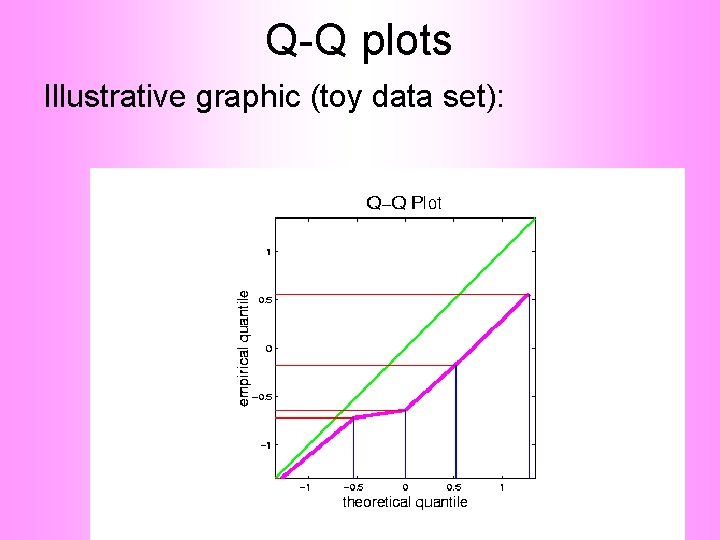
Q-Q plots Illustrative graphic (toy data set):
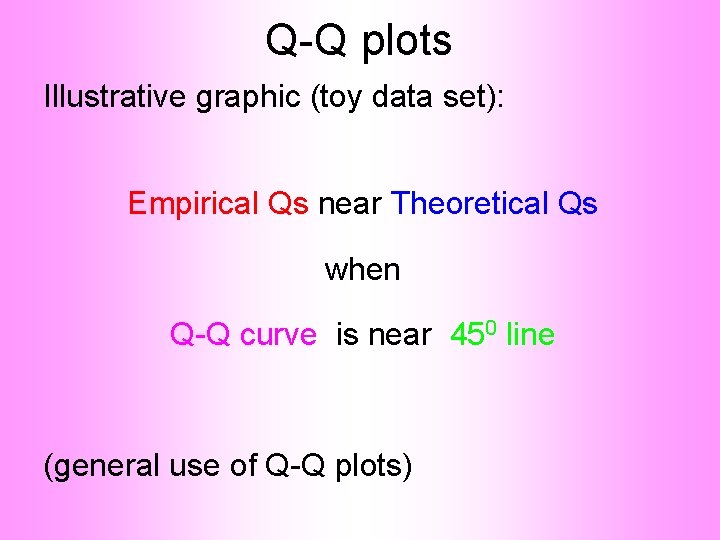
Q-Q plots Illustrative graphic (toy data set): Empirical Qs near Theoretical Qs when Q-Q curve is near 450 line (general use of Q-Q plots)
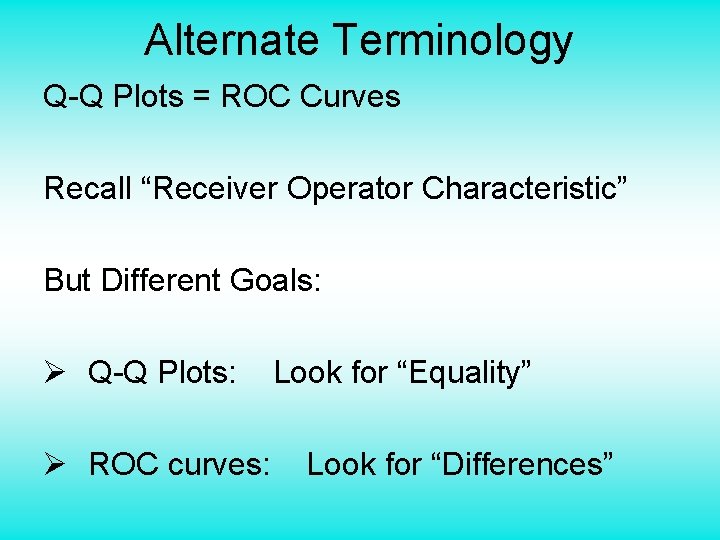
Alternate Terminology Q-Q Plots = ROC Curves Recall “Receiver Operator Characteristic” But Different Goals: Ø Q-Q Plots: Ø ROC curves: Look for “Equality” Look for “Differences”
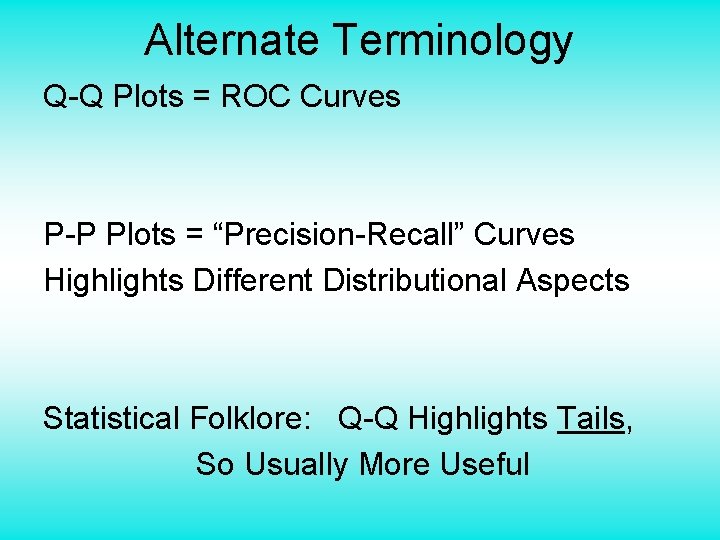
Alternate Terminology Q-Q Plots = ROC Curves P-P Plots = “Precision-Recall” Curves Highlights Different Distributional Aspects Statistical Folklore: Q-Q Highlights Tails, So Usually More Useful
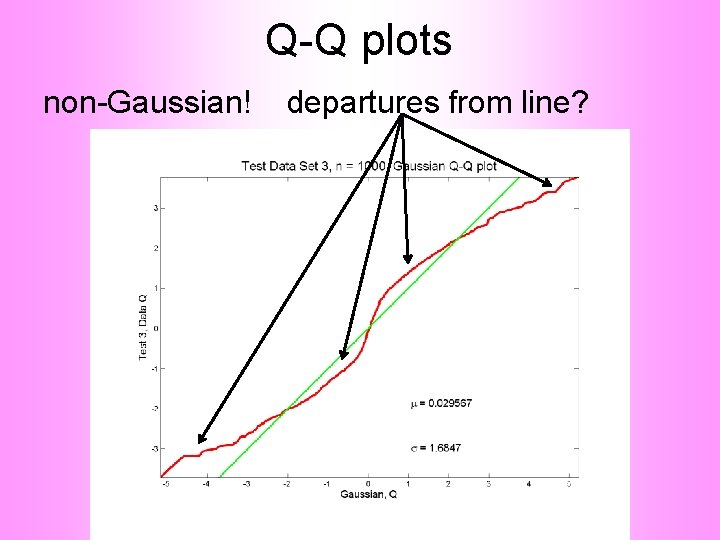
Q-Q plots non-Gaussian! departures from line?
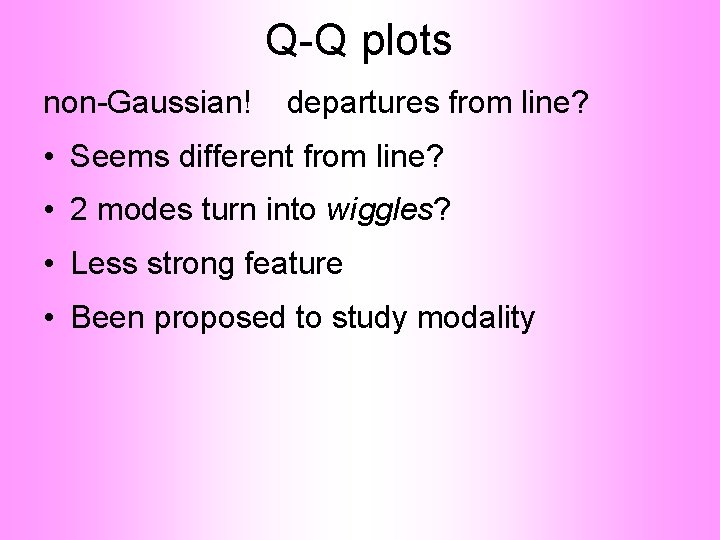
Q-Q plots non-Gaussian! departures from line? • Seems different from line? • 2 modes turn into wiggles? • Less strong feature • Been proposed to study modality
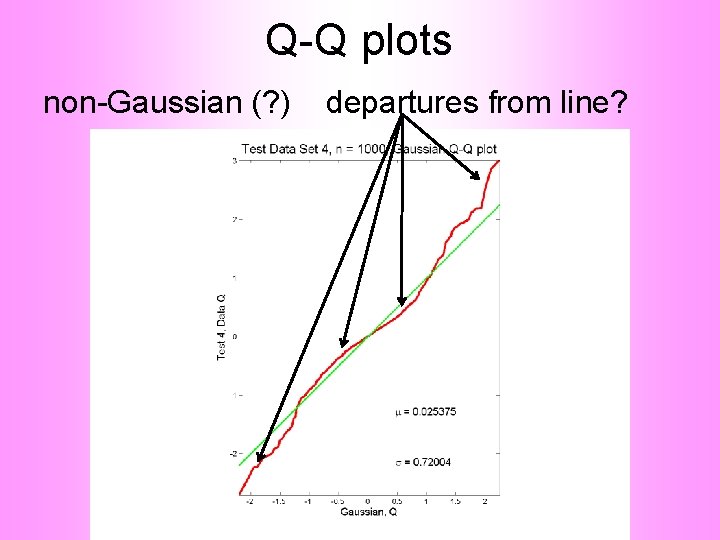
Q-Q plots non-Gaussian (? ) departures from line?
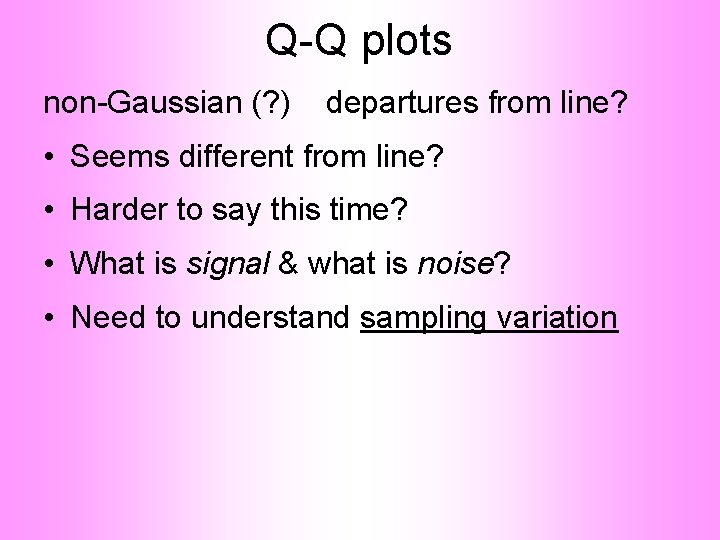
Q-Q plots non-Gaussian (? ) departures from line? • Seems different from line? • Harder to say this time? • What is signal & what is noise? • Need to understand sampling variation
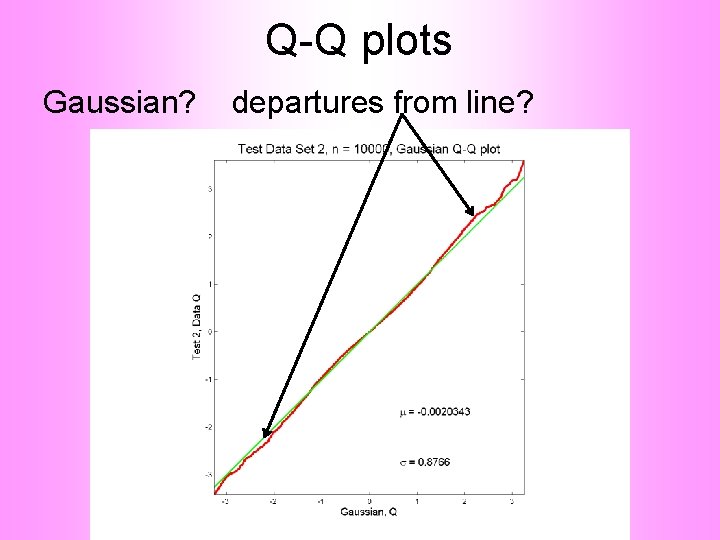
Q-Q plots Gaussian? departures from line?
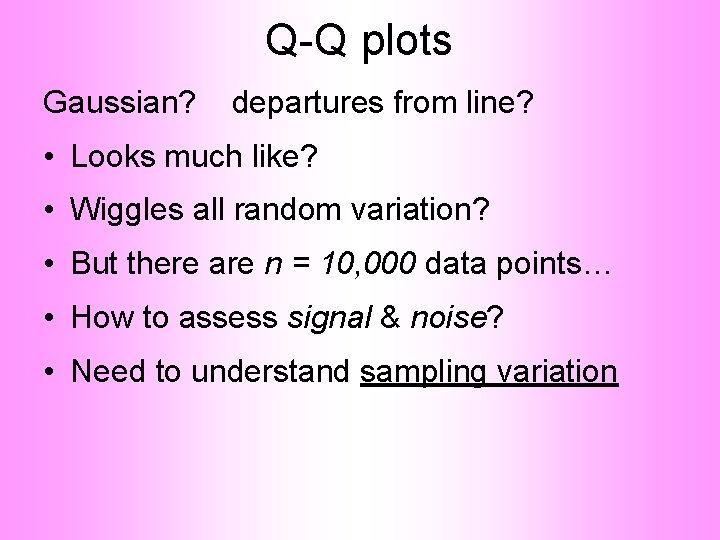
Q-Q plots Gaussian? departures from line? • Looks much like? • Wiggles all random variation? • But there are n = 10, 000 data points… • How to assess signal & noise? • Need to understand sampling variation
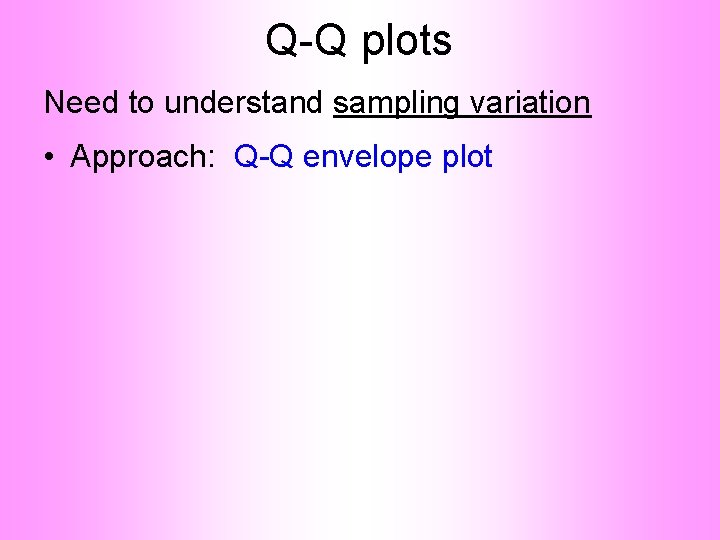
Q-Q plots Need to understand sampling variation • Approach: Q-Q envelope plot
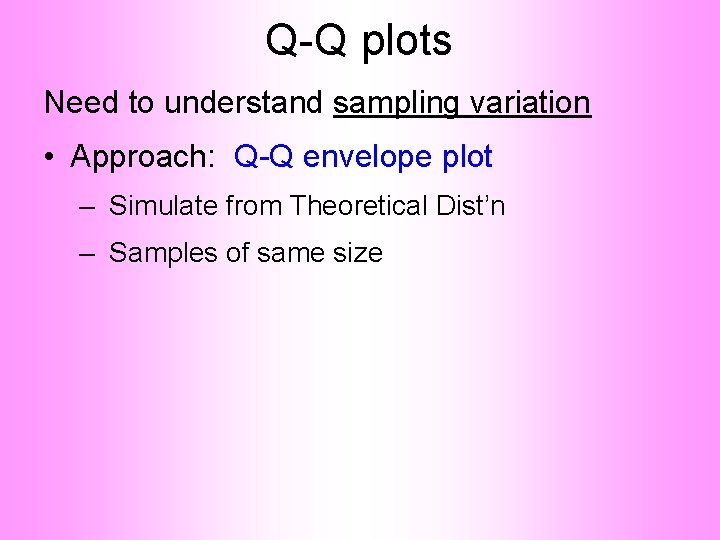
Q-Q plots Need to understand sampling variation • Approach: Q-Q envelope plot – Simulate from Theoretical Dist’n – Samples of same size
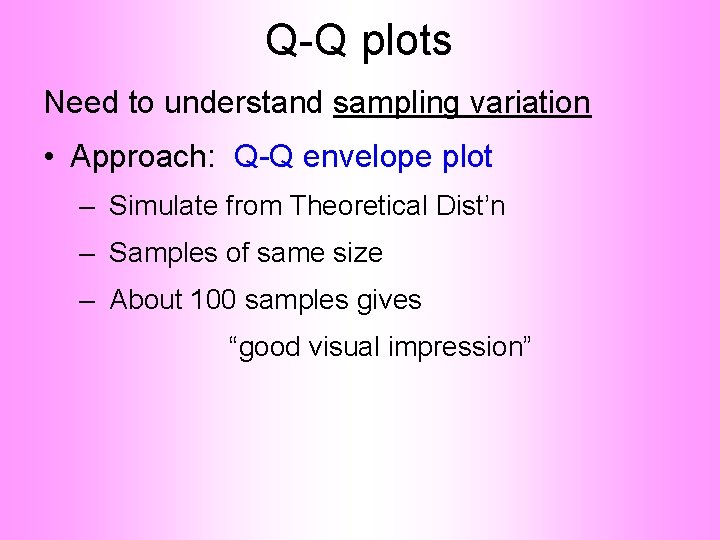
Q-Q plots Need to understand sampling variation • Approach: Q-Q envelope plot – Simulate from Theoretical Dist’n – Samples of same size – About 100 samples gives “good visual impression”
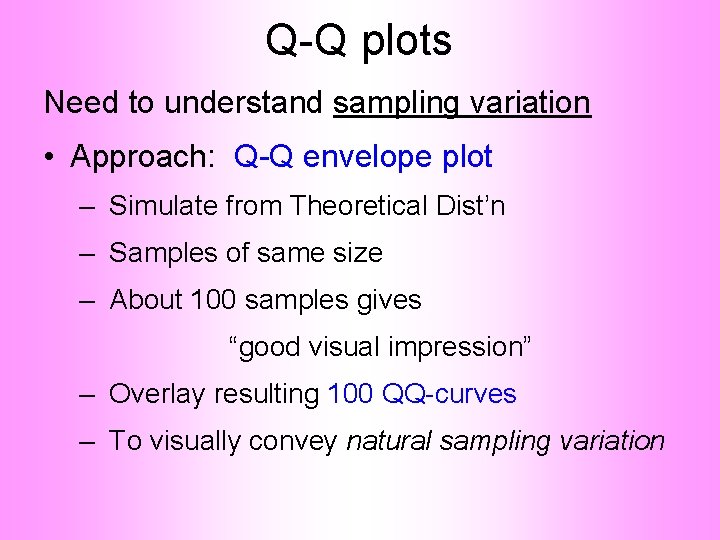
Q-Q plots Need to understand sampling variation • Approach: Q-Q envelope plot – Simulate from Theoretical Dist’n – Samples of same size – About 100 samples gives “good visual impression” – Overlay resulting 100 QQ-curves – To visually convey natural sampling variation
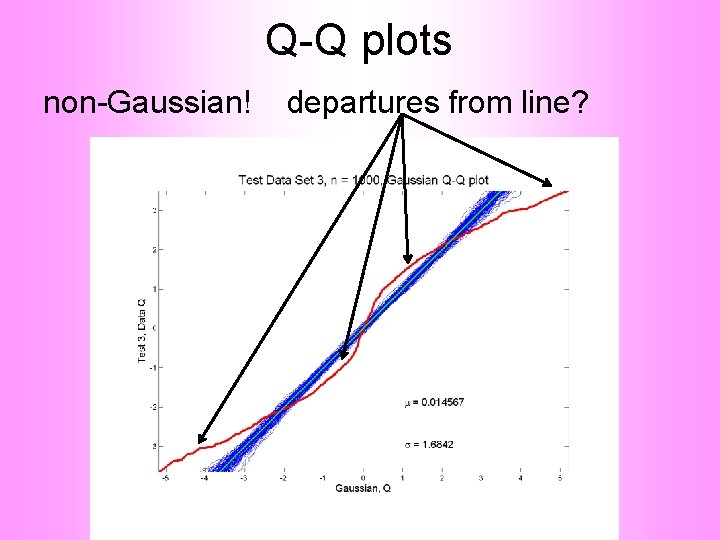
Q-Q plots non-Gaussian! departures from line?
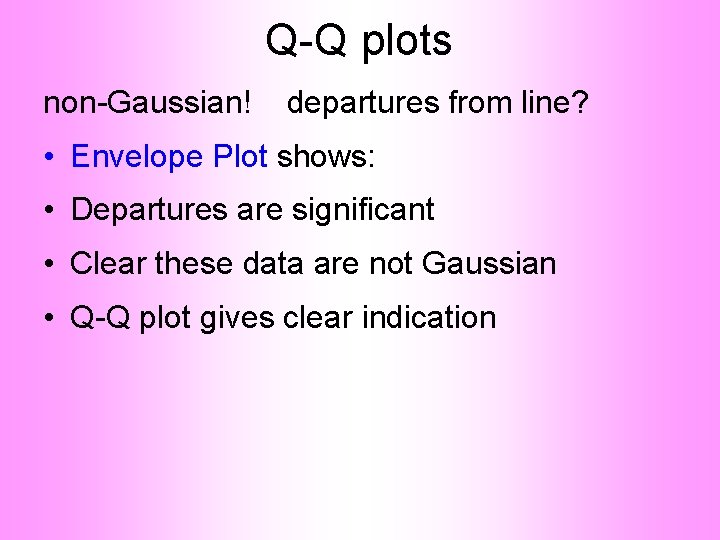
Q-Q plots non-Gaussian! departures from line? • Envelope Plot shows: • Departures are significant • Clear these data are not Gaussian • Q-Q plot gives clear indication
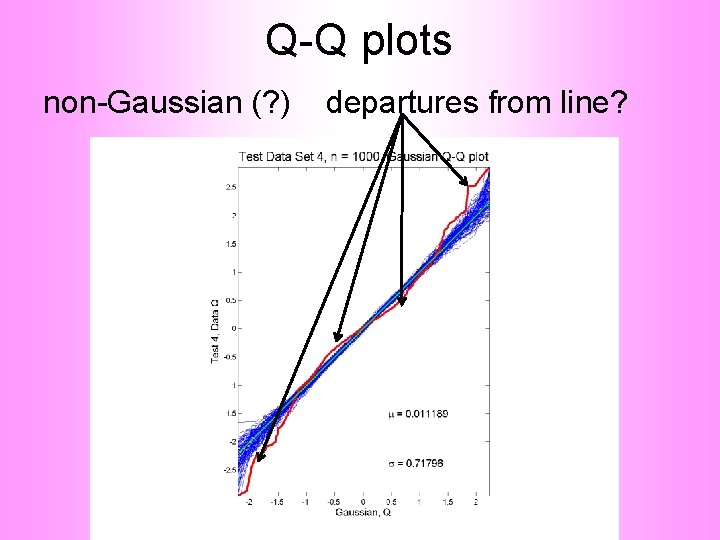
Q-Q plots non-Gaussian (? ) departures from line?
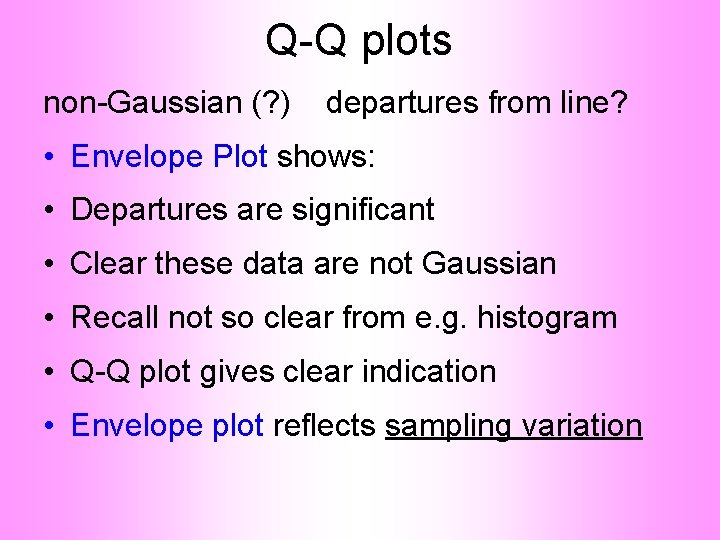
Q-Q plots non-Gaussian (? ) departures from line? • Envelope Plot shows: • Departures are significant • Clear these data are not Gaussian • Recall not so clear from e. g. histogram • Q-Q plot gives clear indication • Envelope plot reflects sampling variation
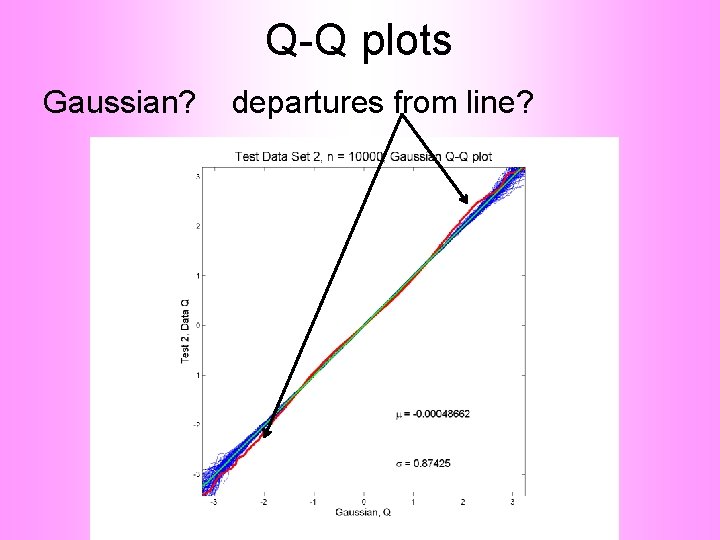
Q-Q plots Gaussian? departures from line?
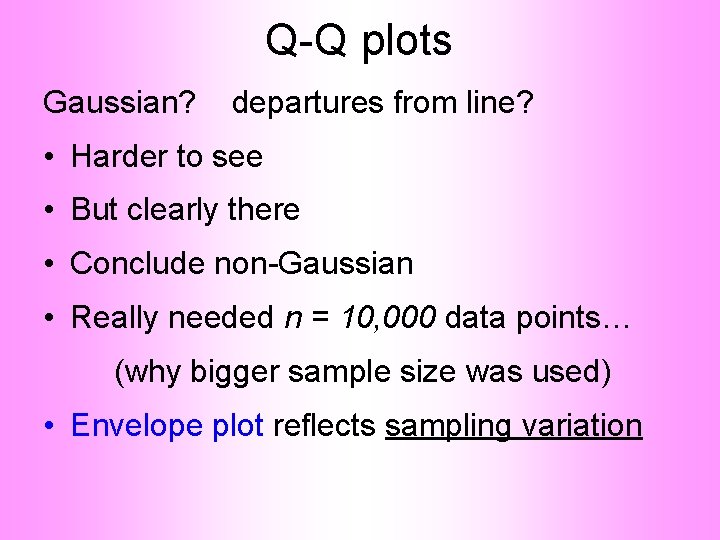
Q-Q plots Gaussian? departures from line? • Harder to see • But clearly there • Conclude non-Gaussian • Really needed n = 10, 000 data points… (why bigger sample size was used) • Envelope plot reflects sampling variation
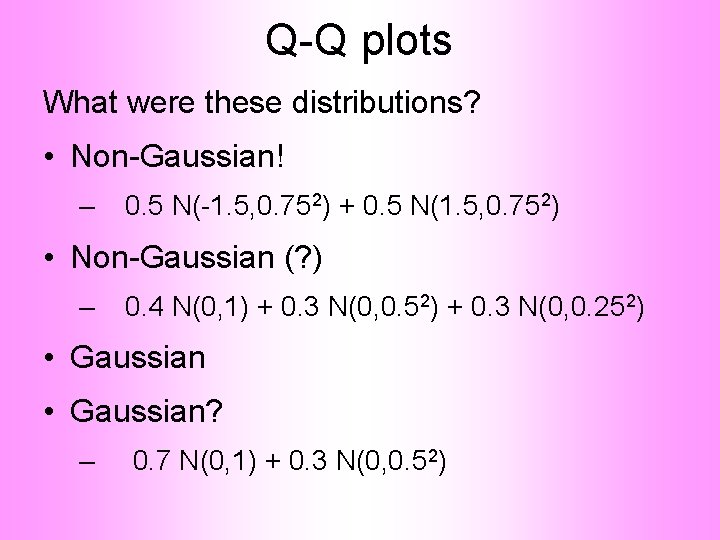
Q-Q plots What were these distributions? • Non-Gaussian! – 0. 5 N(-1. 5, 0. 752) + 0. 5 N(1. 5, 0. 752) • Non-Gaussian (? ) – 0. 4 N(0, 1) + 0. 3 N(0, 0. 52) + 0. 3 N(0, 0. 252) • Gaussian? – 0. 7 N(0, 1) + 0. 3 N(0, 0. 52)
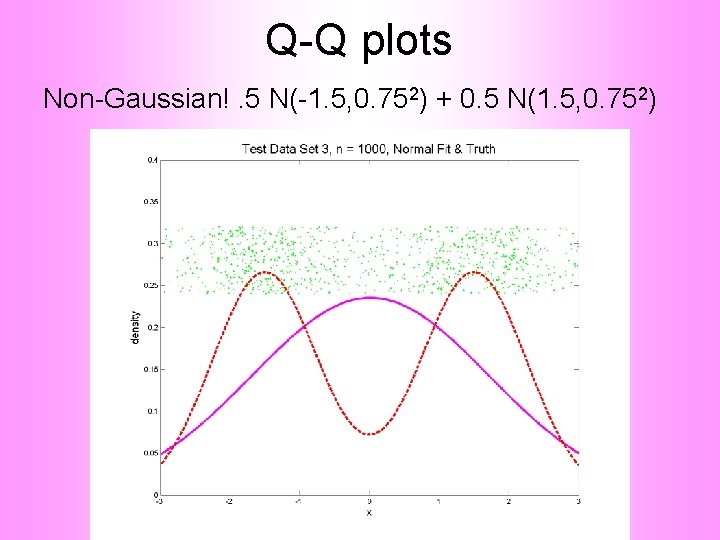
Q-Q plots Non-Gaussian!. 5 N(-1. 5, 0. 752) + 0. 5 N(1. 5, 0. 752)
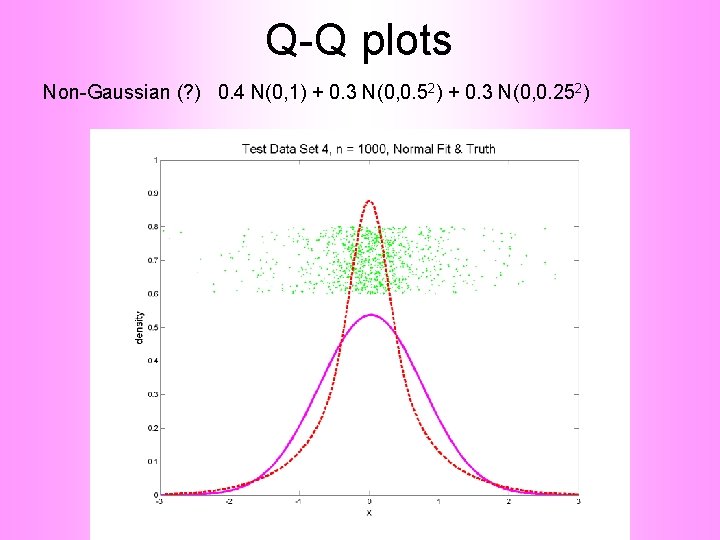
Q-Q plots Non-Gaussian (? ) 0. 4 N(0, 1) + 0. 3 N(0, 0. 52) + 0. 3 N(0, 0. 252)
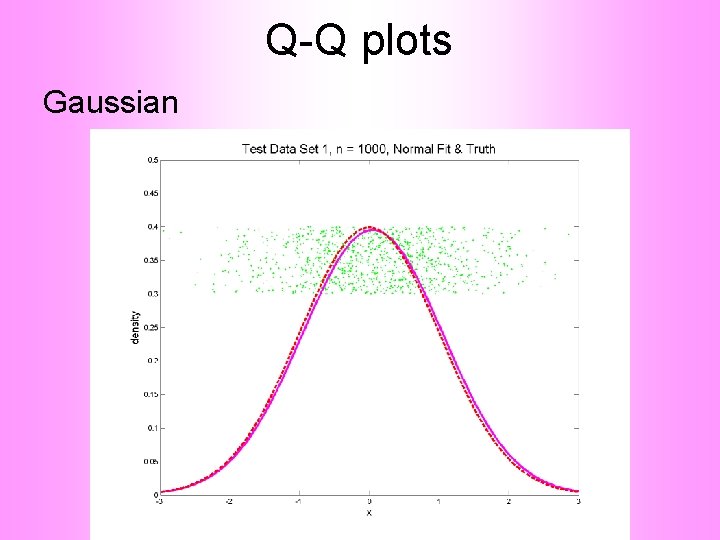
Q-Q plots Gaussian
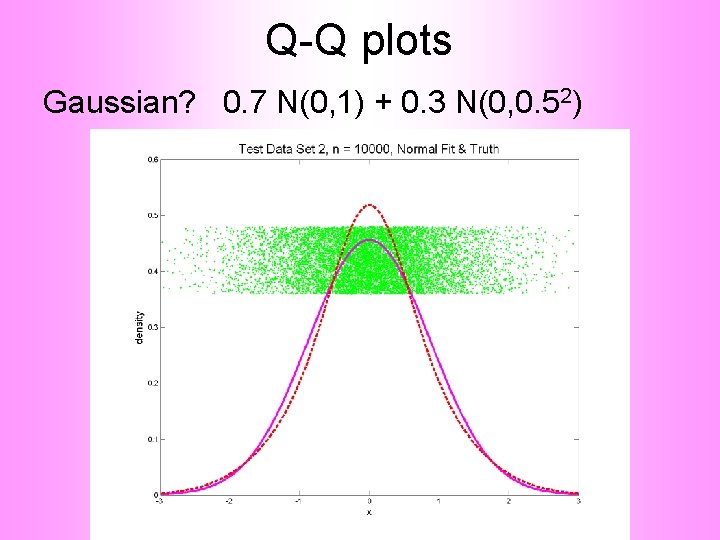
Q-Q plots Gaussian? 0. 7 N(0, 1) + 0. 3 N(0, 0. 52)
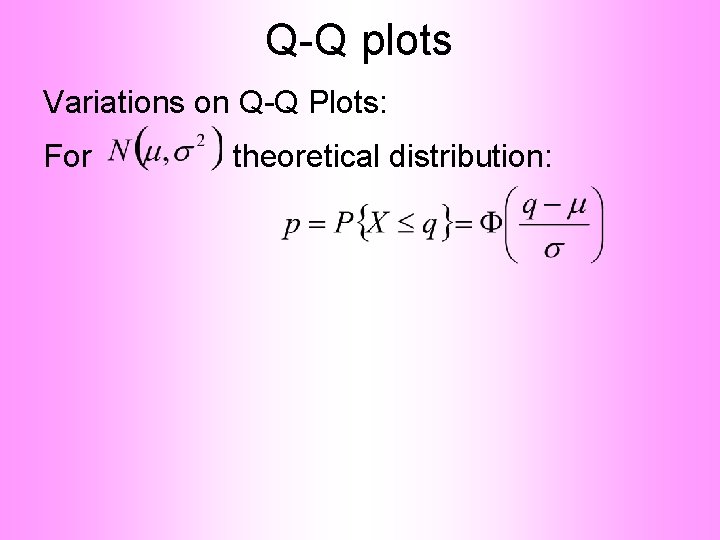
Q-Q plots Variations on Q-Q Plots: For theoretical distribution:
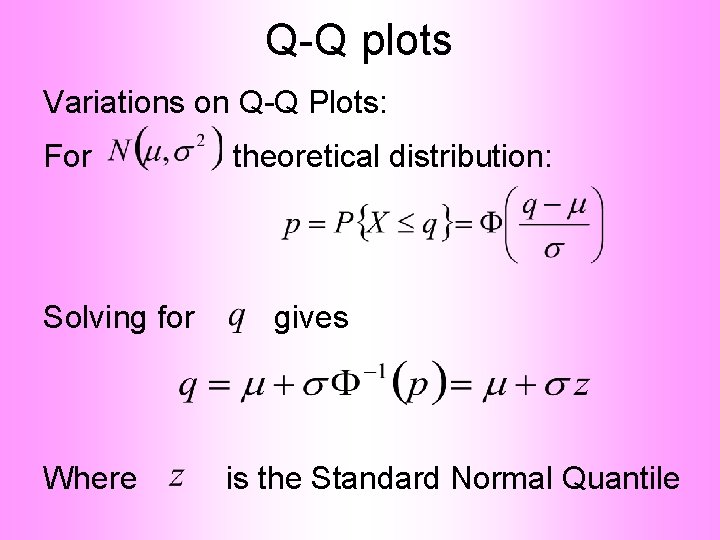
Q-Q plots Variations on Q-Q Plots: For Solving for Where theoretical distribution: gives is the Standard Normal Quantile
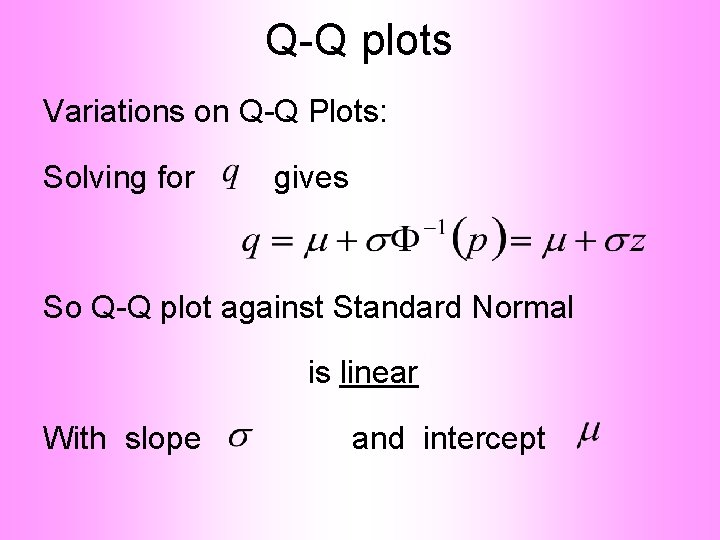
Q-Q plots Variations on Q-Q Plots: Solving for gives So Q-Q plot against Standard Normal is linear With slope and intercept
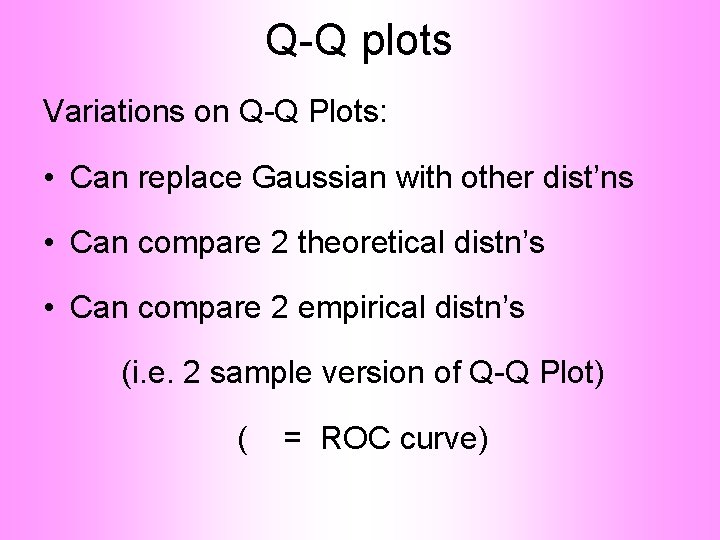
Q-Q plots Variations on Q-Q Plots: • Can replace Gaussian with other dist’ns • Can compare 2 theoretical distn’s • Can compare 2 empirical distn’s (i. e. 2 sample version of Q-Q Plot) ( = ROC curve)
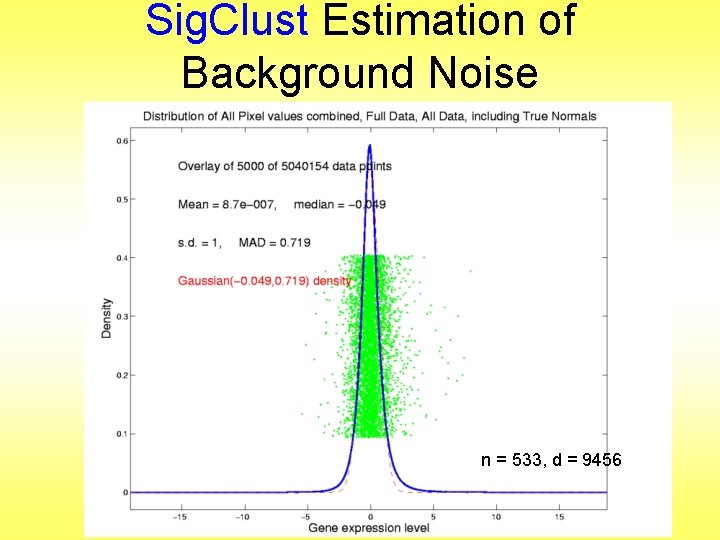
Sig. Clust Estimation of Background Noise n = 533, d = 9456
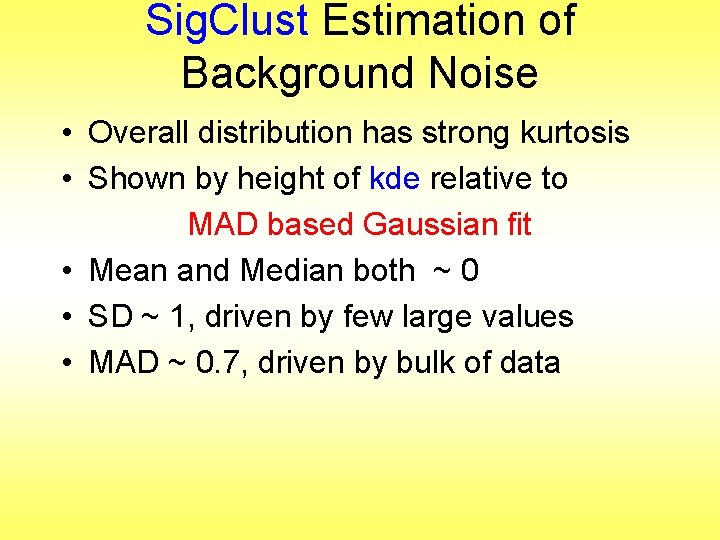
Sig. Clust Estimation of Background Noise • Overall distribution has strong kurtosis • Shown by height of kde relative to MAD based Gaussian fit • Mean and Median both ~ 0 • SD ~ 1, driven by few large values • MAD ~ 0. 7, driven by bulk of data
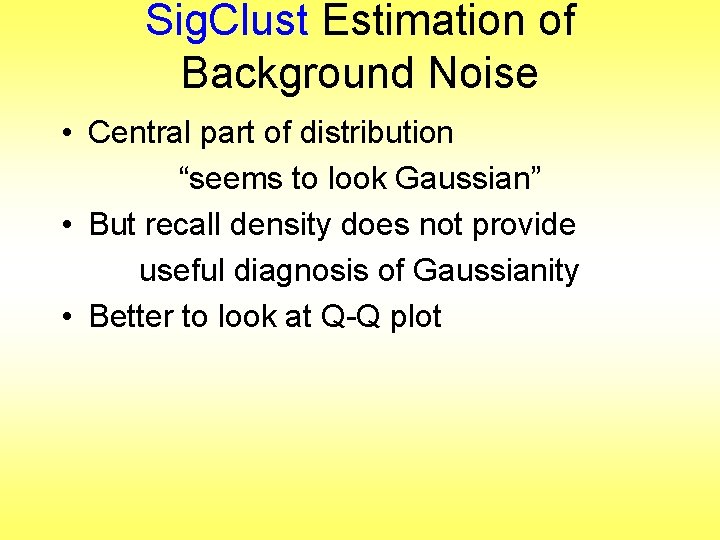
Sig. Clust Estimation of Background Noise • Central part of distribution “seems to look Gaussian” • But recall density does not provide useful diagnosis of Gaussianity • Better to look at Q-Q plot
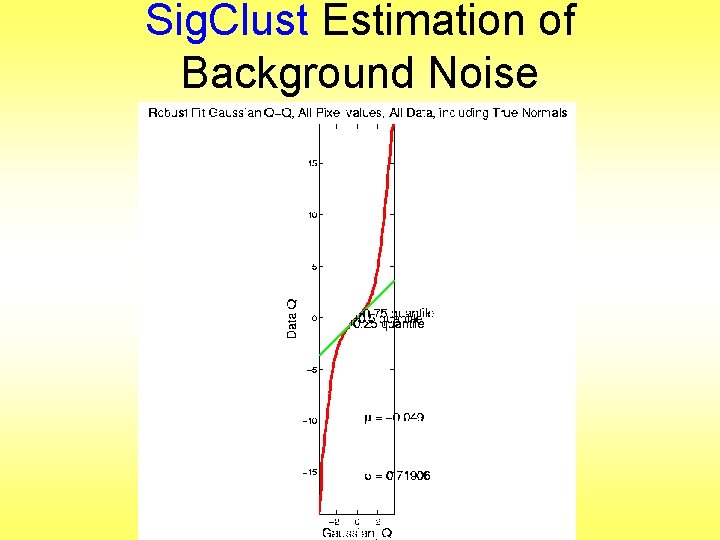
Sig. Clust Estimation of Background Noise
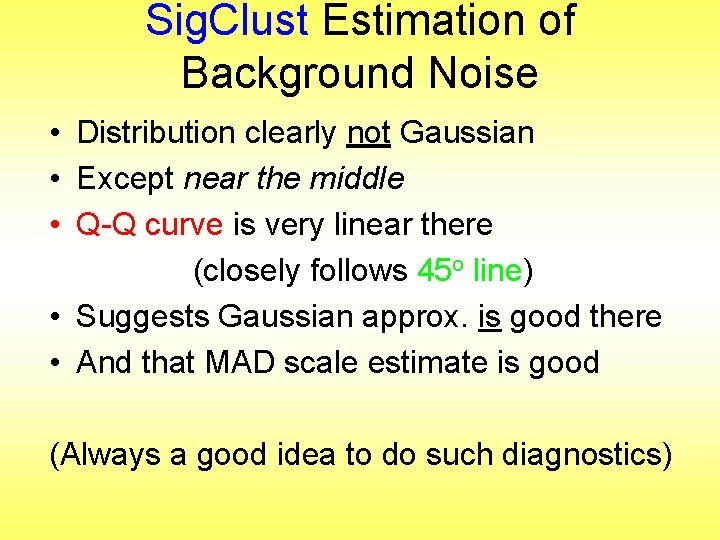
Sig. Clust Estimation of Background Noise • Distribution clearly not Gaussian • Except near the middle • Q-Q curve is very linear there (closely follows 45 o line) • Suggests Gaussian approx. is good there • And that MAD scale estimate is good (Always a good idea to do such diagnostics)
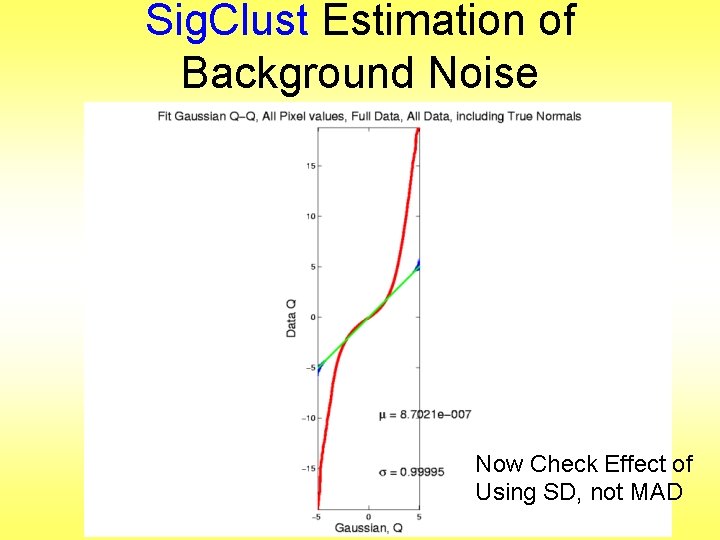
Sig. Clust Estimation of Background Noise Now Check Effect of Using SD, not MAD
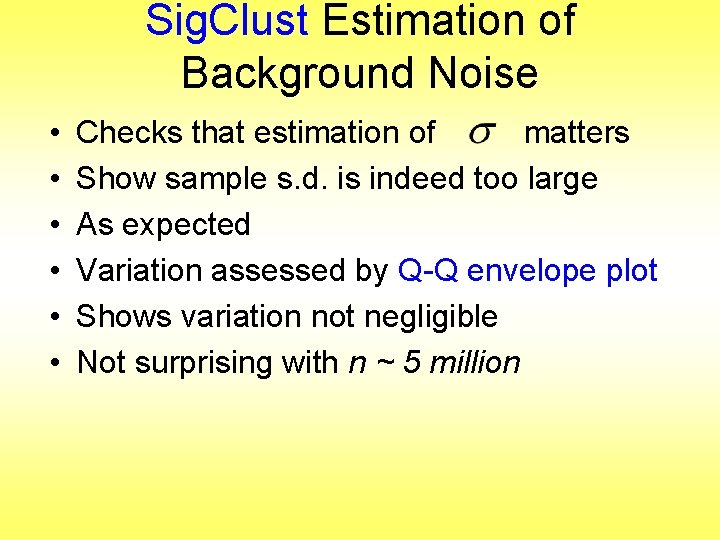
Sig. Clust Estimation of Background Noise • • • Checks that estimation of matters Show sample s. d. is indeed too large As expected Variation assessed by Q-Q envelope plot Shows variation not negligible Not surprising with n ~ 5 million
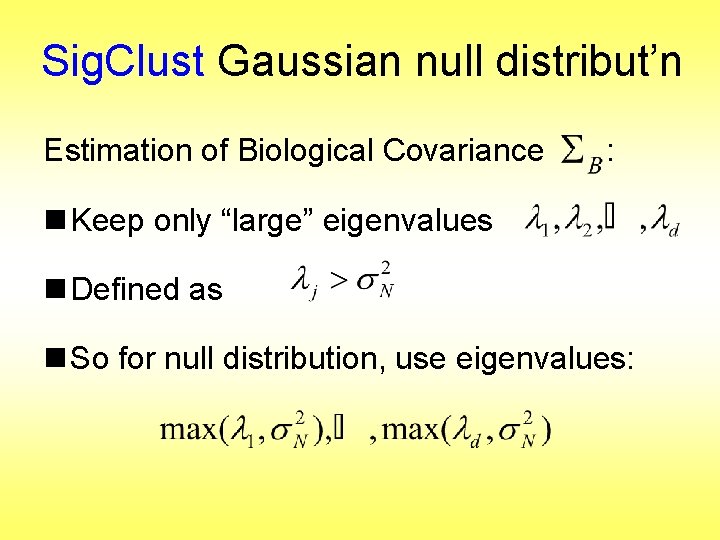
Sig. Clust Gaussian null distribut’n Estimation of Biological Covariance : n Keep only “large” eigenvalues n Defined as n So for null distribution, use eigenvalues:
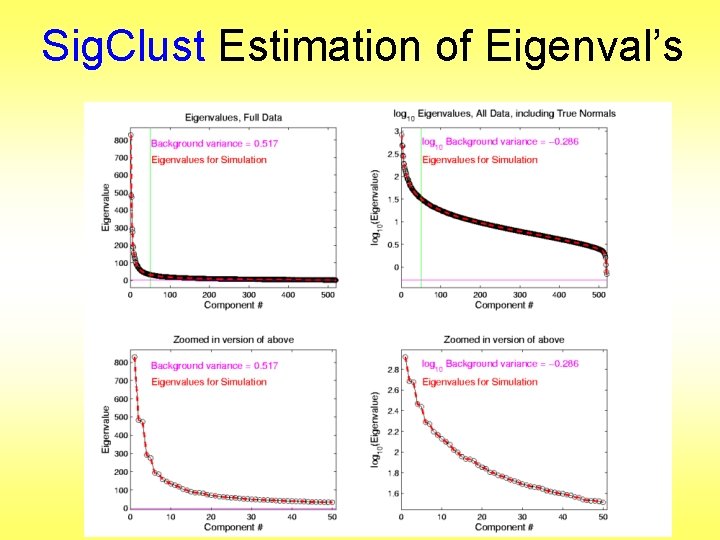
Sig. Clust Estimation of Eigenval’s
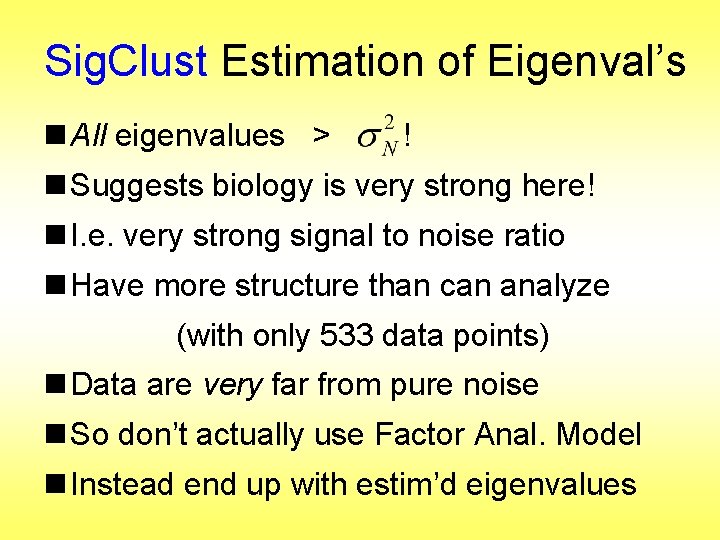
Sig. Clust Estimation of Eigenval’s n All eigenvalues > ! n Suggests biology is very strong here! n I. e. very strong signal to noise ratio n Have more structure than can analyze (with only 533 data points) n Data are very far from pure noise n So don’t actually use Factor Anal. Model n Instead end up with estim’d eigenvalues
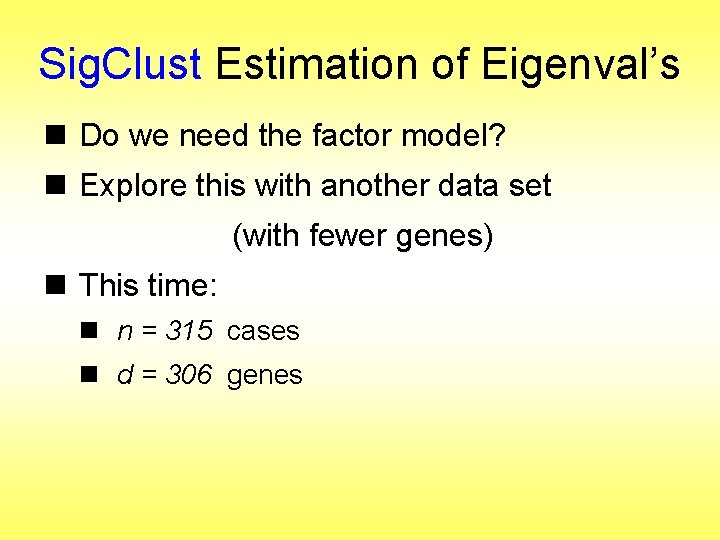
Sig. Clust Estimation of Eigenval’s n Do we need the factor model? n Explore this with another data set (with fewer genes) n This time: n n = 315 cases n d = 306 genes
Hercules scar rug
Toytoy thai
Graphical user interface examples
An introduction to variational methods for graphical models
An introduction to probabilistic graphical models
Toy machine simulator
Teddy bear museum naples
Plural of paper
Scalar is to vector as regents
Potatis minerva
Forbidden toy paradigma
Toy story foreshadowing
Carlo seneca
Carl gustav jung 12 archetypes
Types of mechanical toys
Vik muniz toy soldier
Brush plural nouns
Planet zeist
Is a toy car a conductor or insulator
Forbidden toy experiment
Vex pull toy designs
Two parents have each built a toy catapult
Quantum angular momentum toy
Hcf questions for class 4
Child protection and toy safety act
Wumpus toy
A plastic toy submarine is held 15
A toy car with essentially frictionless
Akc toy breeds
Natural science term 3 grade 7
Gcf problem solving with answers
Chapter 6 motion in two dimensions
A model for the path of a toy rocket is given by
My toy cars am is are in the box
A wind up toy is fully wound and at rest
Toy truck
Geri's game climax
Lady diana rey
Gregory is my beautiful gray persian cat
Acme toy company prints baseball cards
Purpose
A toy rocket that weighs 10 n
Toy presentation
Toy assembly language
Mark of purity toy
Richard james slinky
Making silicone toys
A toy train engine rests motionless on a track
Bone density z score
T score and z score difference
Conectados swiss medical beneficios
Swiss ice hockey league
Swiss knife vs. radio
Swiss medical organigrama
Swiss snow happening 2023
Gaya desain futurisme
Daniel bernoulli
Three swiss witches
Deepview swiss pdb viewer
Swiss psychologist
Anovulatory bleeding
Swiss pdb viewer
Arbeit.swiss jobroom
Swiss international style
Swiss sheet model
Swiss snowsports
Franchising prio
Swiss gear luggage
Swiss miss mascot
Swiss volley schiedsrichter spesen
Switched witches
Hoseline brandmeister
Immopulse objekte
Swiss ski kwo terminkalender
Swiss meteo
Swiss point middle school
Swiss fel
Adme
Spoilersync
Reporter swiss ice hockey
Language symbol in word
Carol ann duffy nostalgia analysis
Structuralism swiss
Ikea switzerland
Participants in a formal discussion use language that is .
Führungscredo
Mathematician cramer
Swiss pdb viewer download
Switzerland point middle school dress code
Osi inglin
Swiss dns
Swinog
Swiss excellence scholarship
Swiss solvency test
Politeknik mekanik swiss itb
Hockey stick lie
Alternative reference rates
Swiss protection
Swiss life sciences
Swiss vbs
Swisspdb viewer
The very hungry caterpillar stomachache
Suez canal map
Swiss ice hockey association
Swiss ice hockey federation
Theory of probability is applied to in surveying
Pfadi swiss