REGRESSION Tuesday April 14 REGRESSION ANALYSIS Estimates the
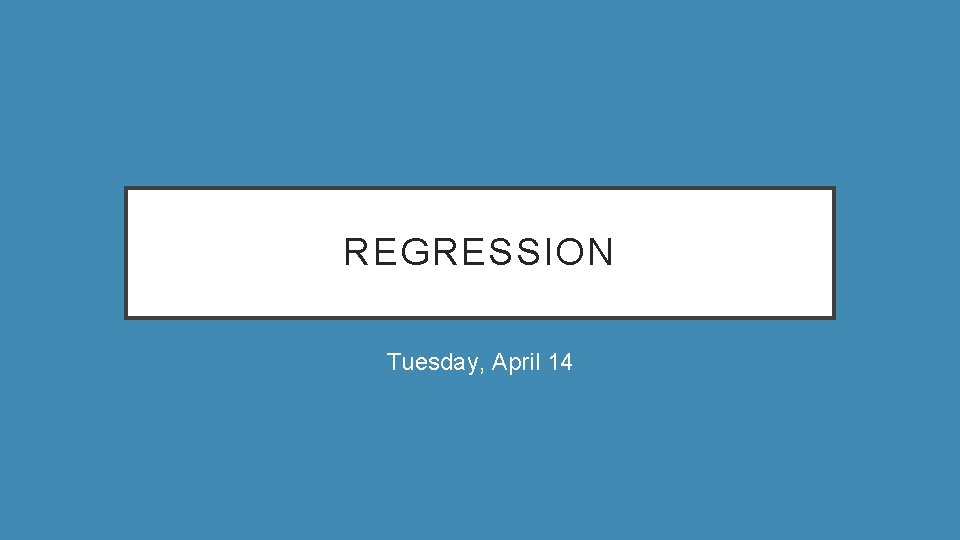
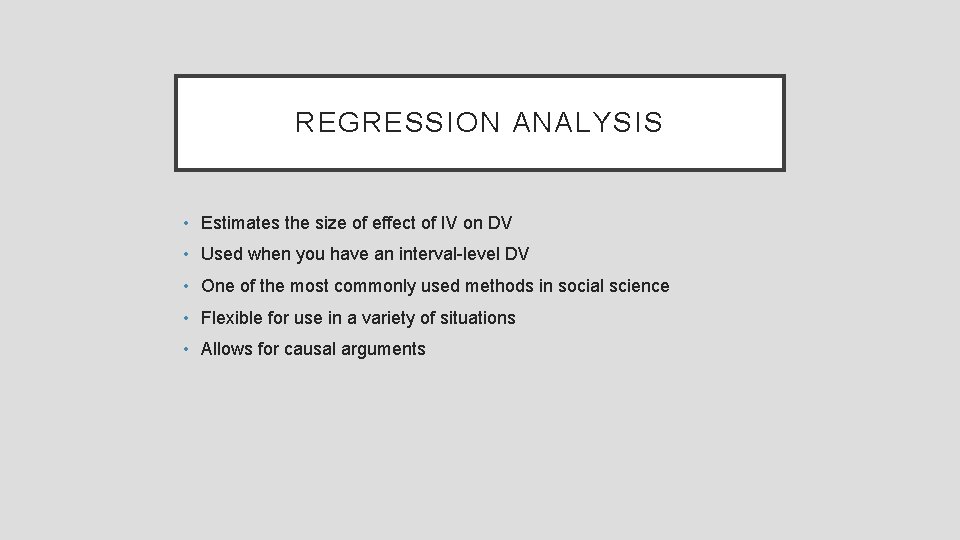
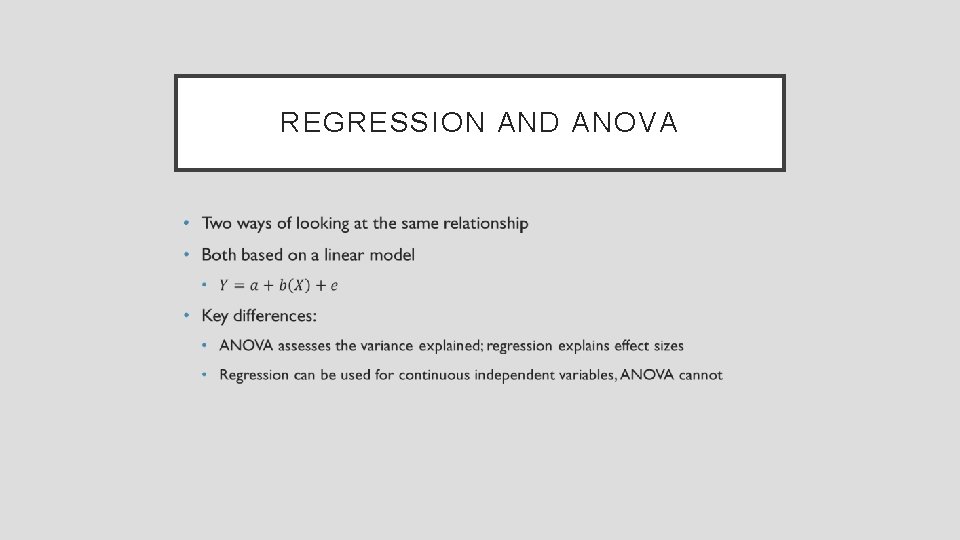
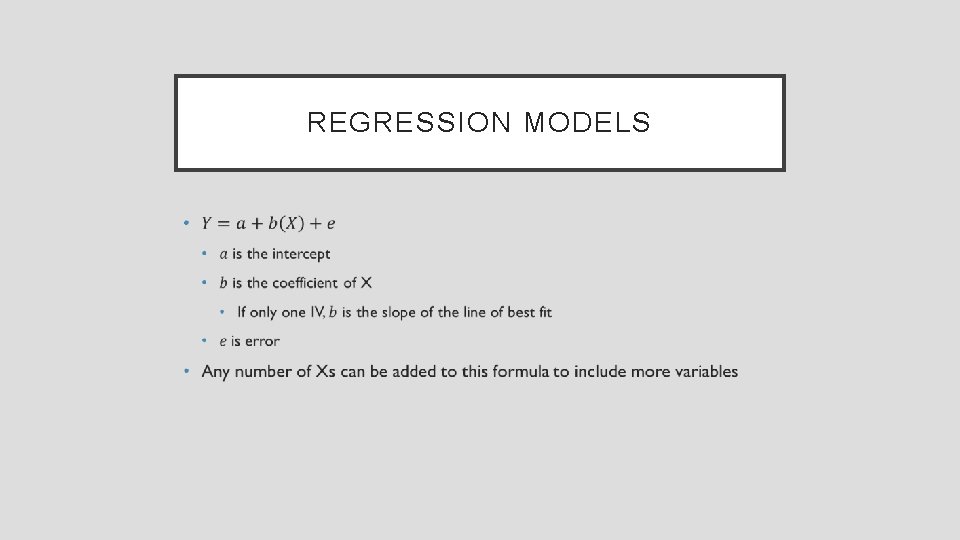
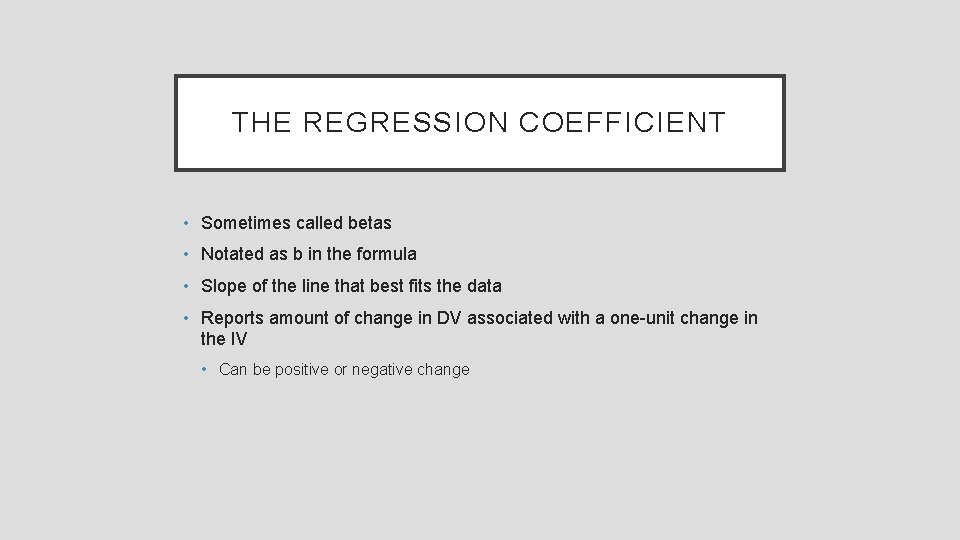
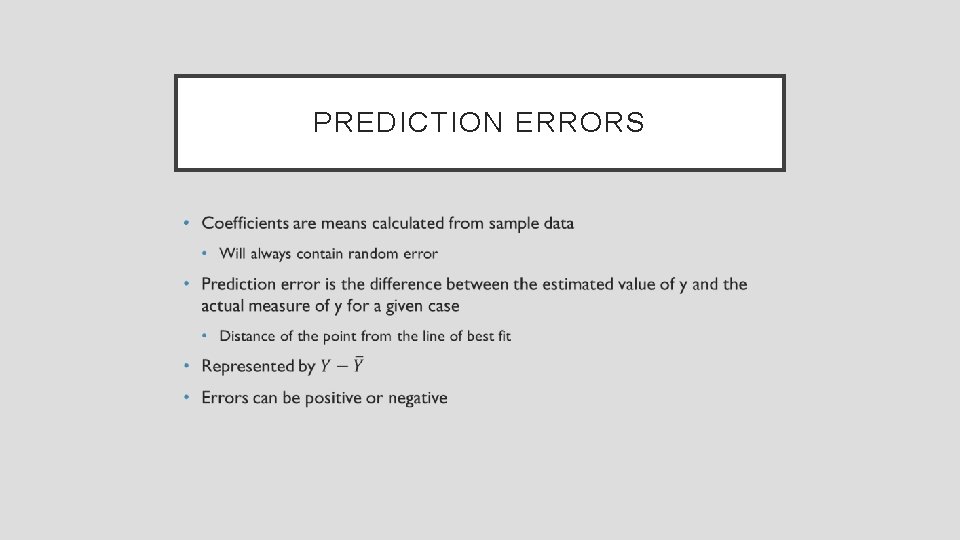
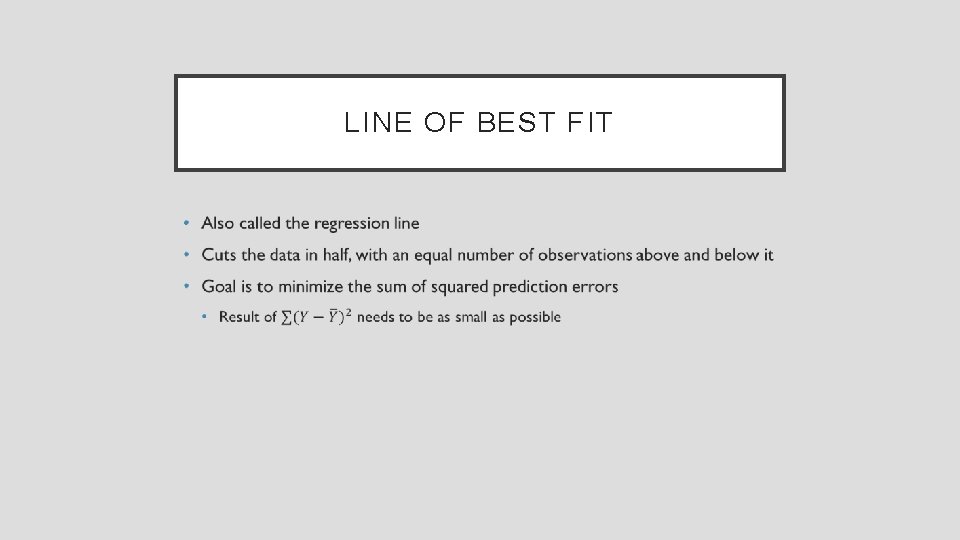
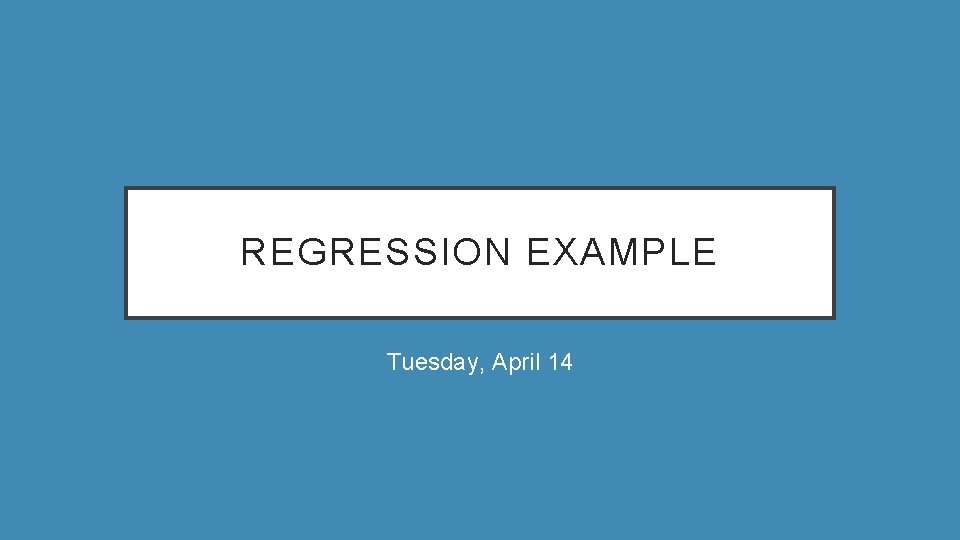
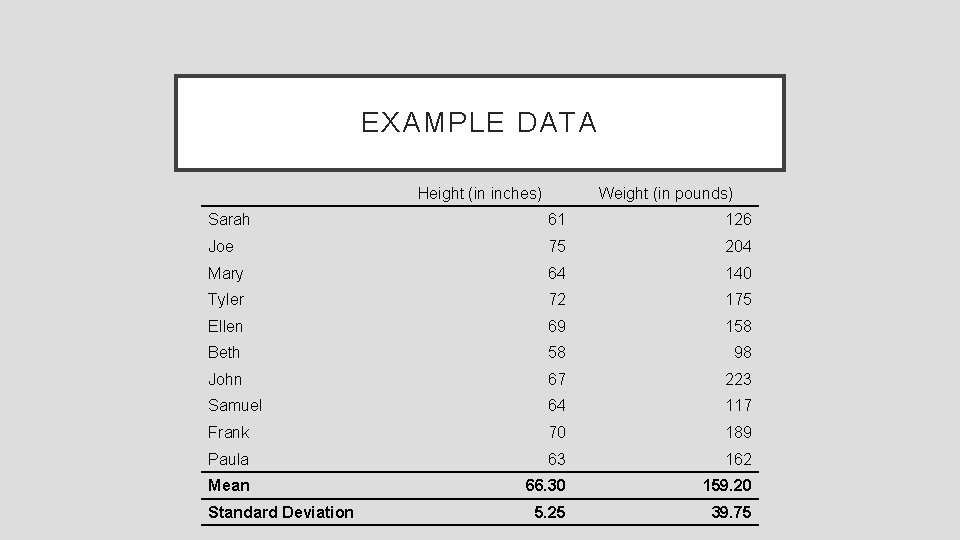
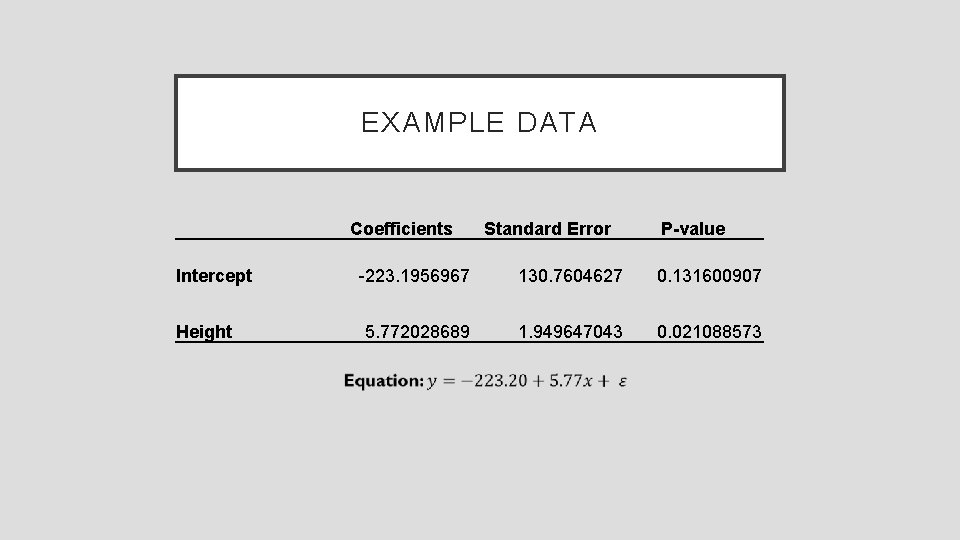
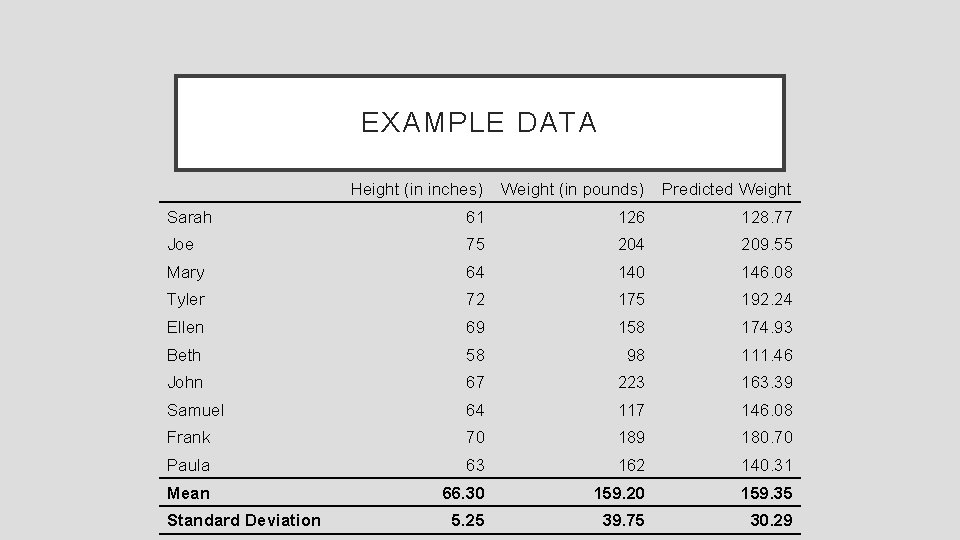
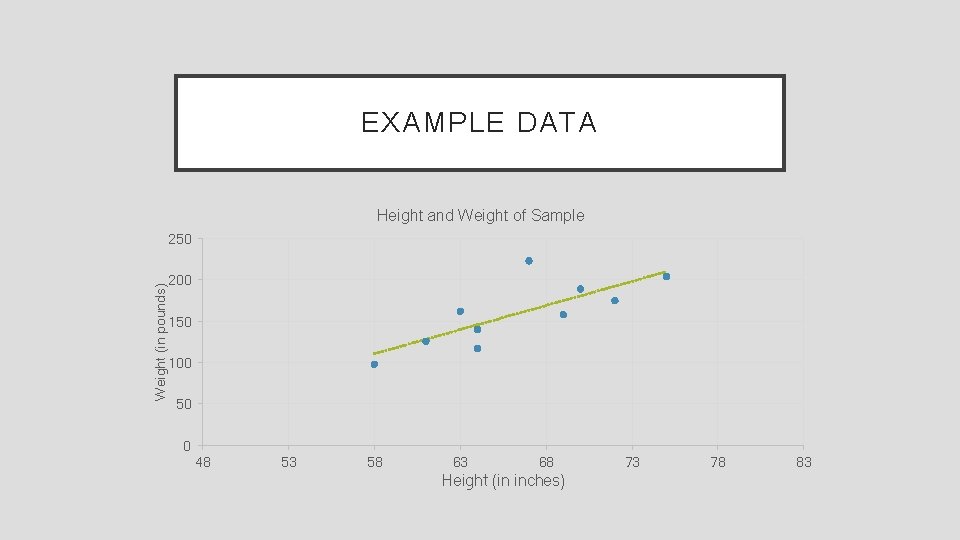
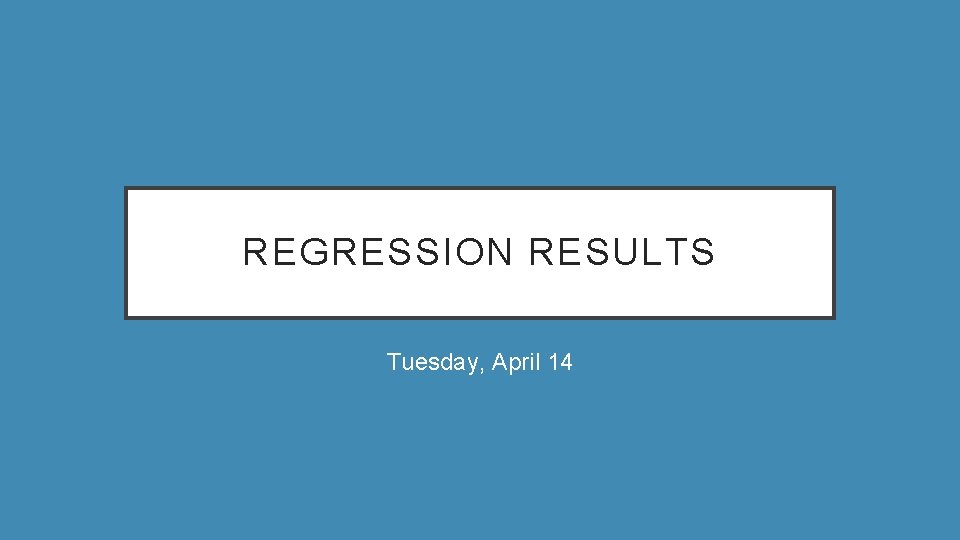
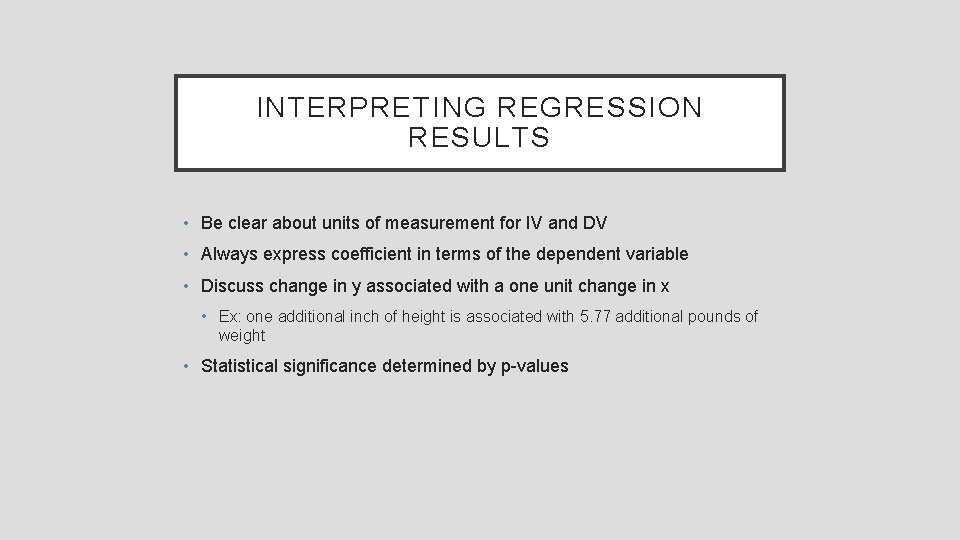
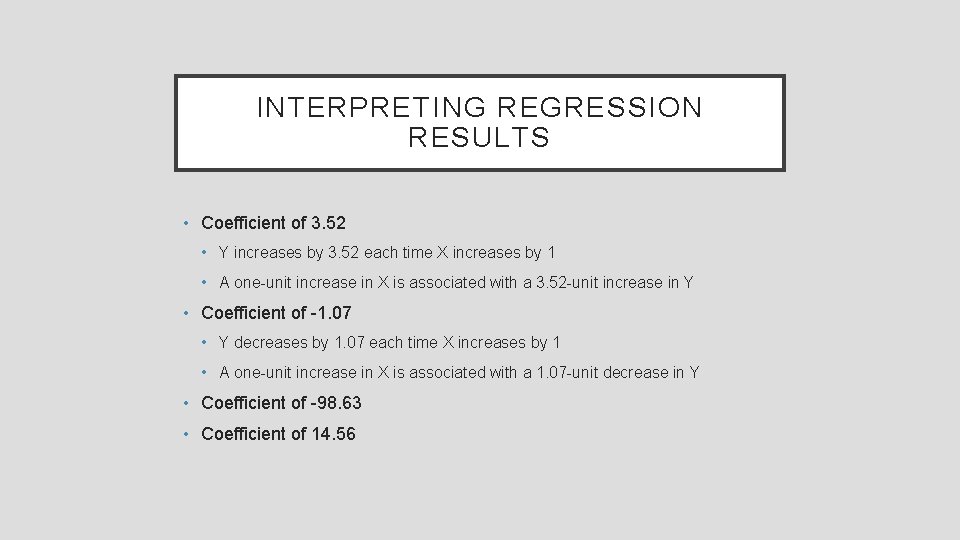
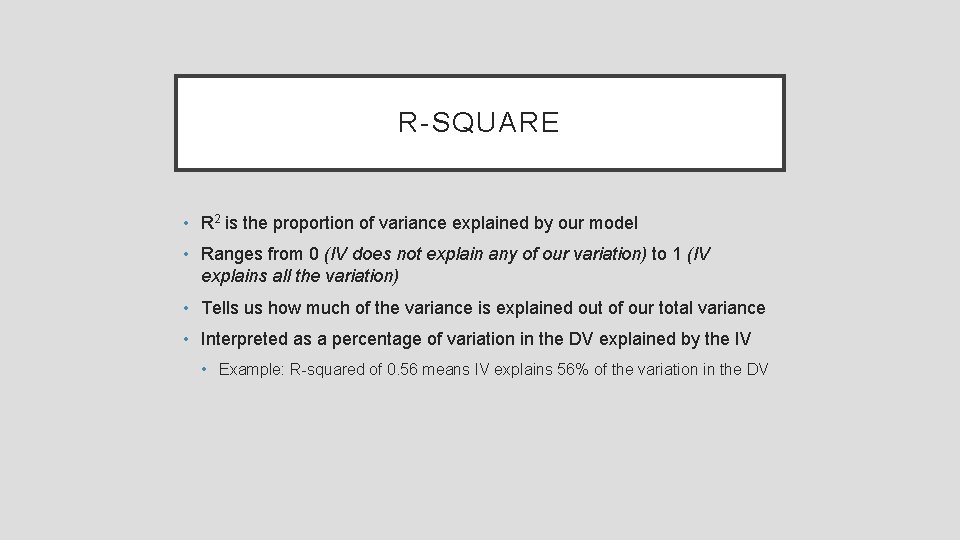
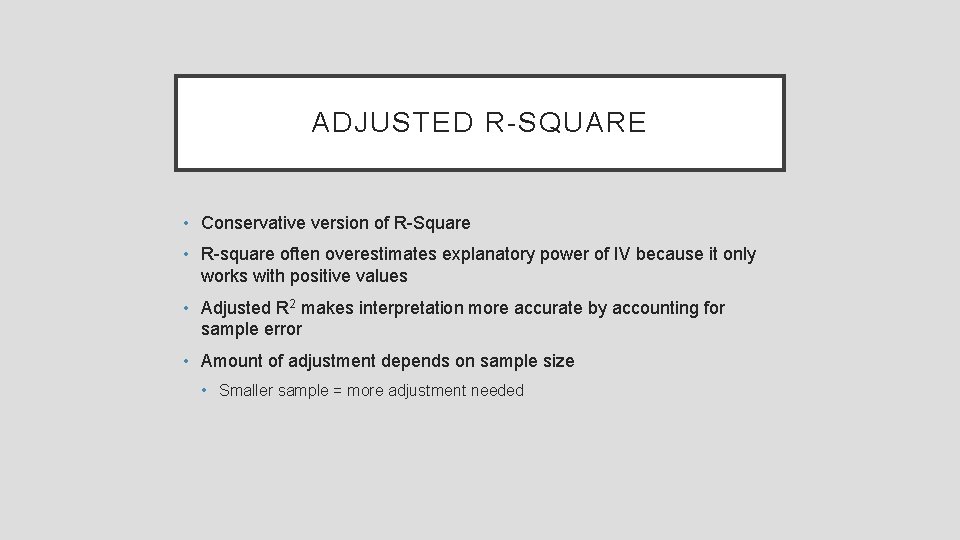
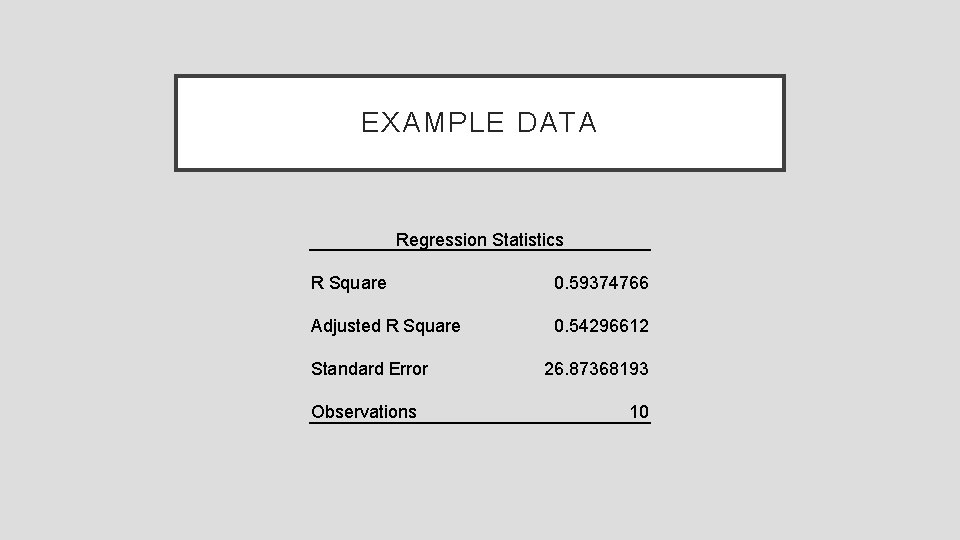
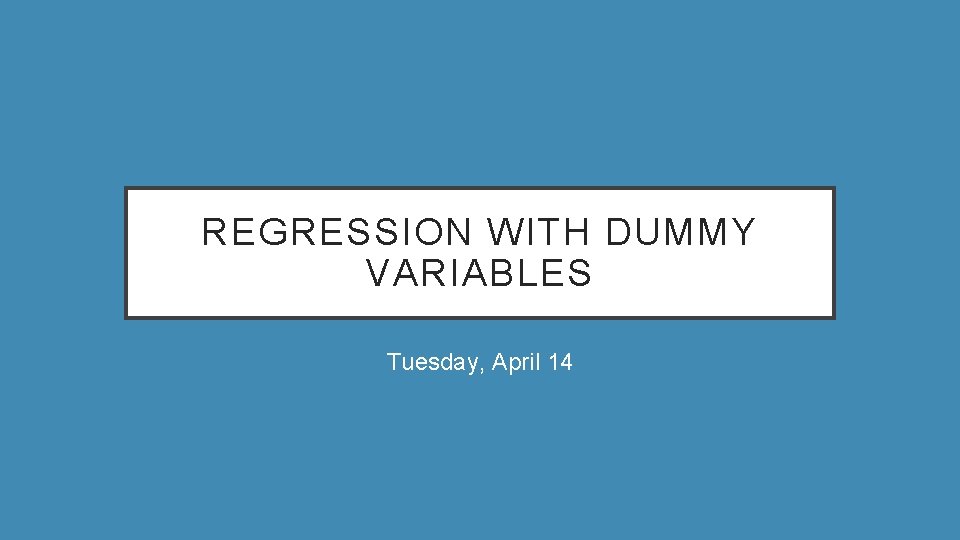
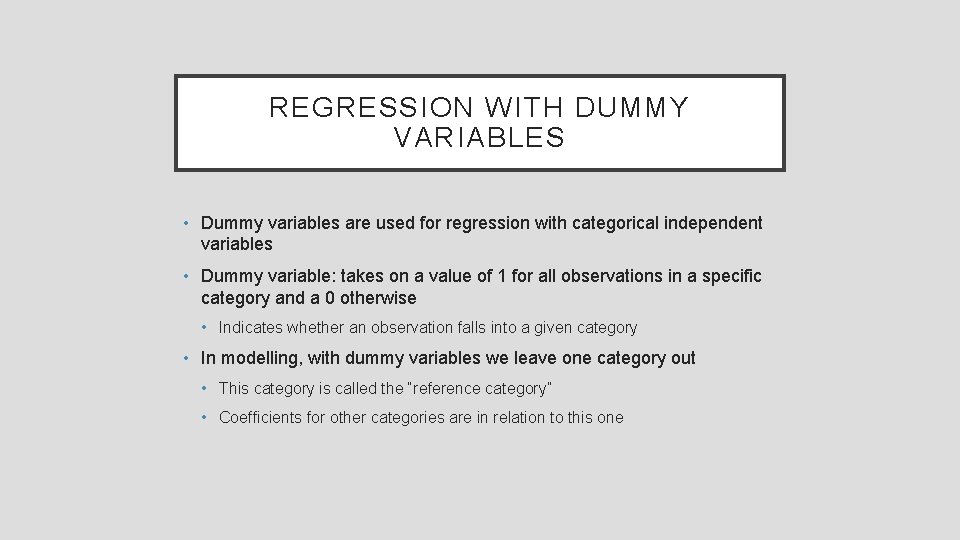
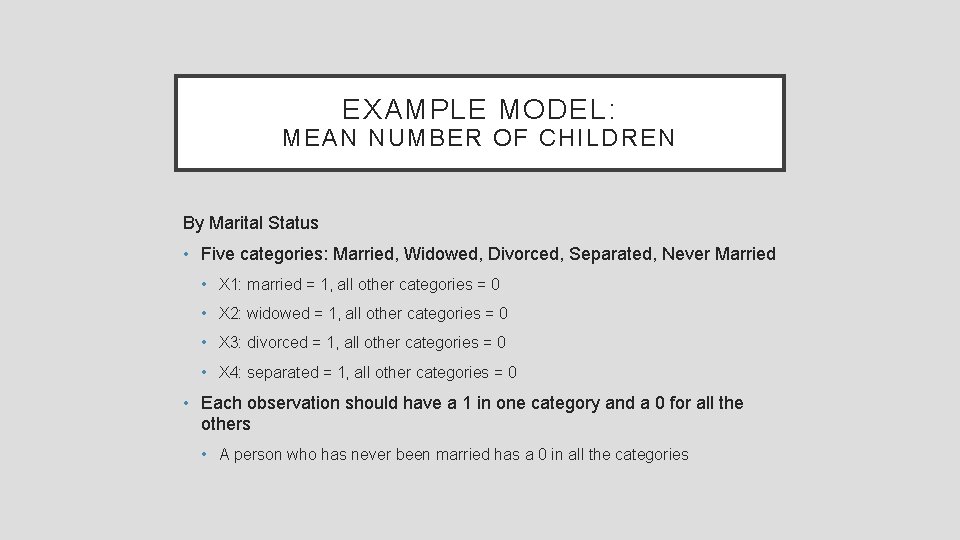
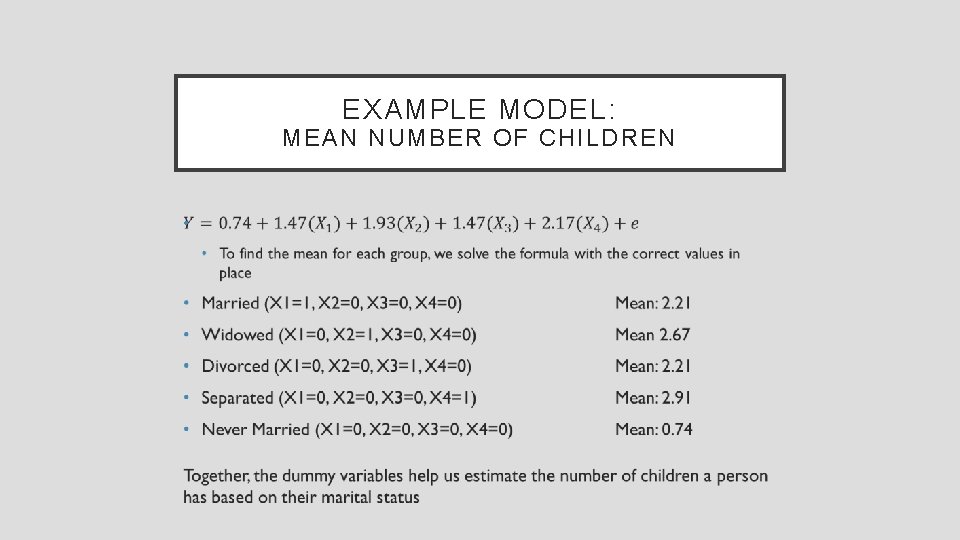
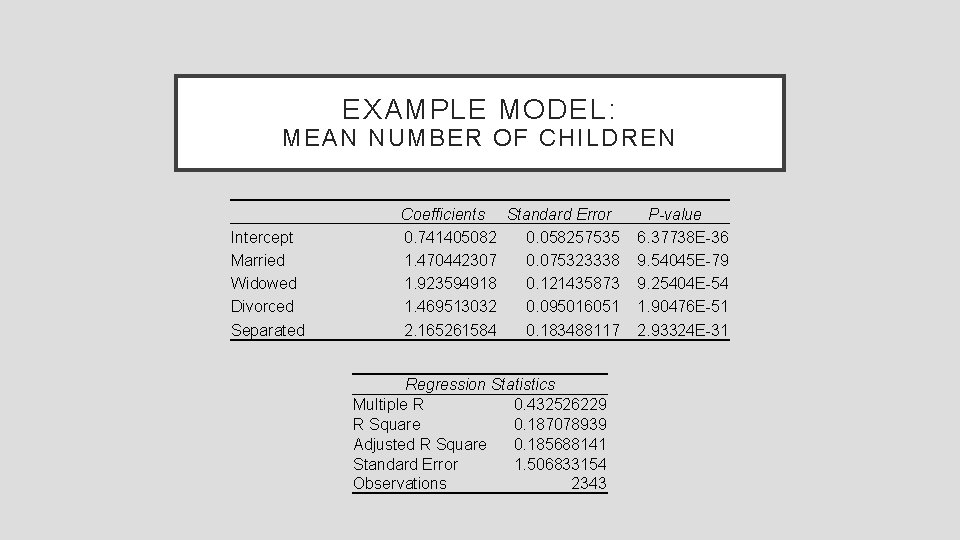
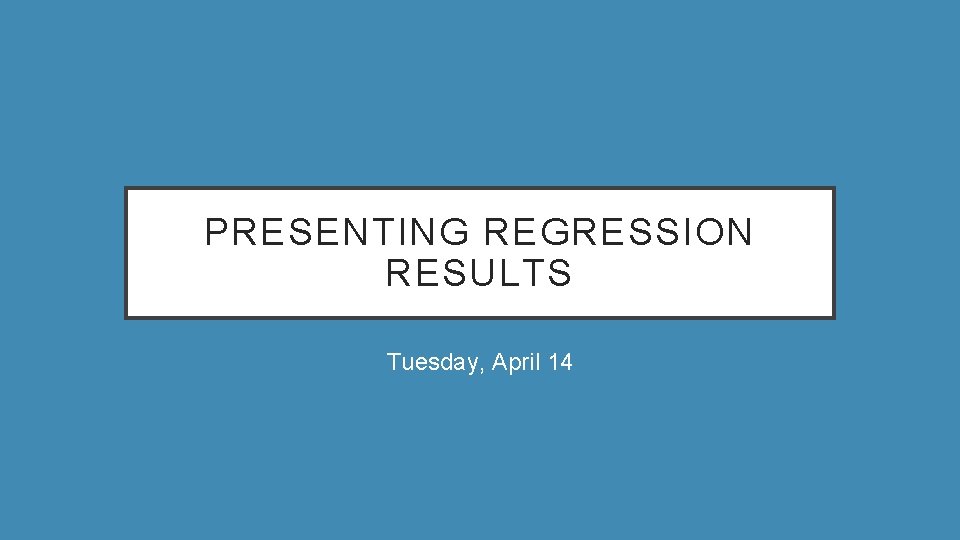
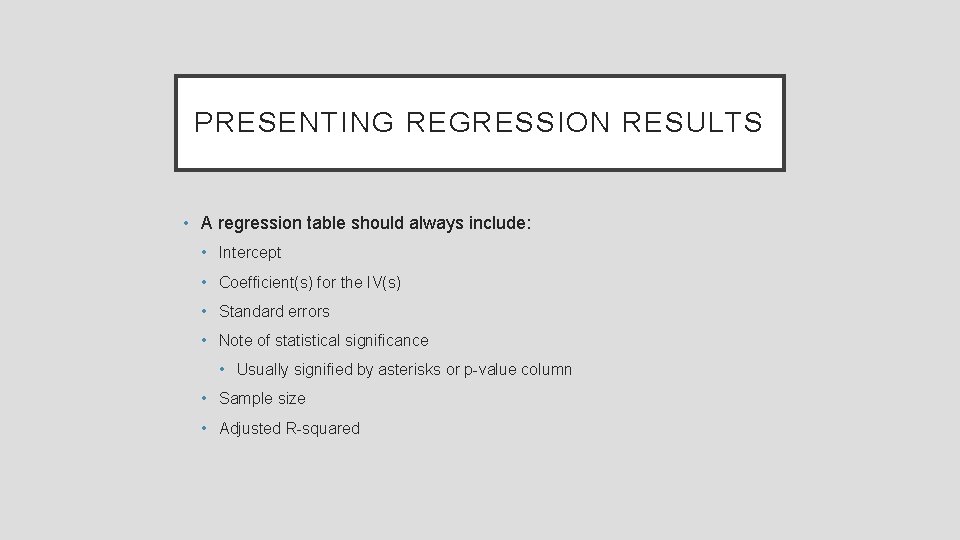
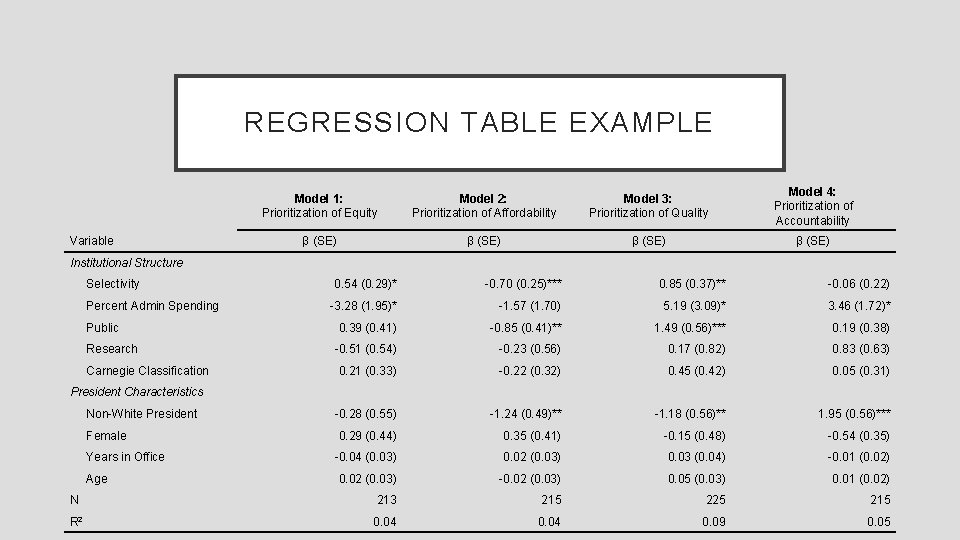
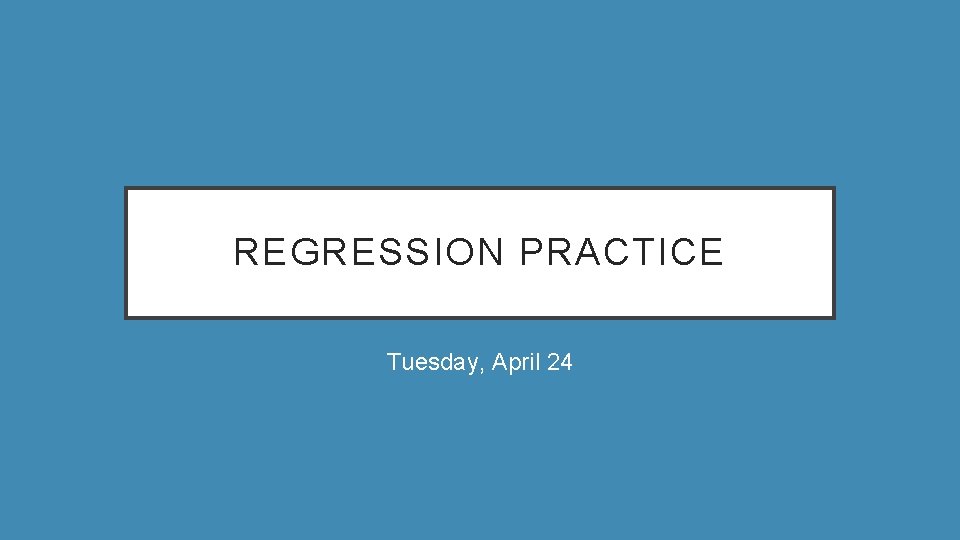
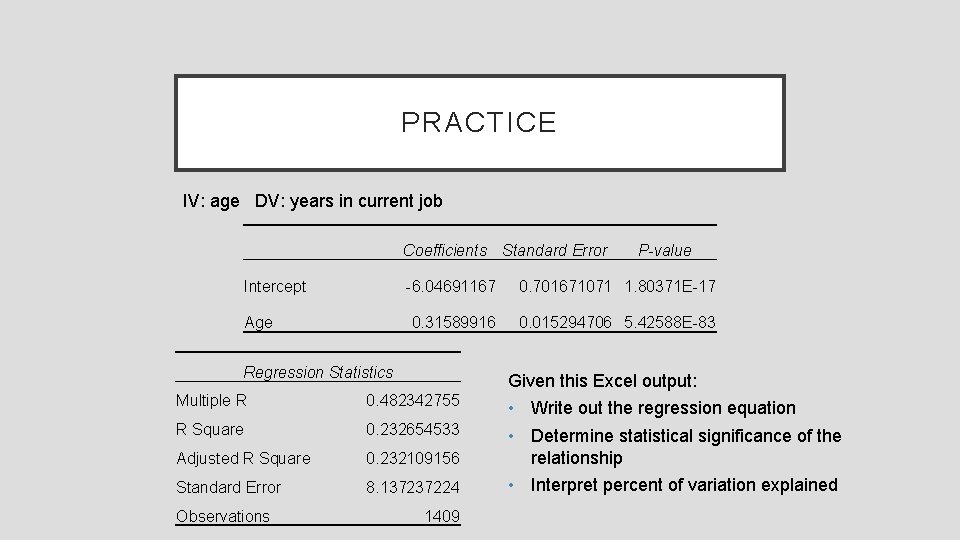
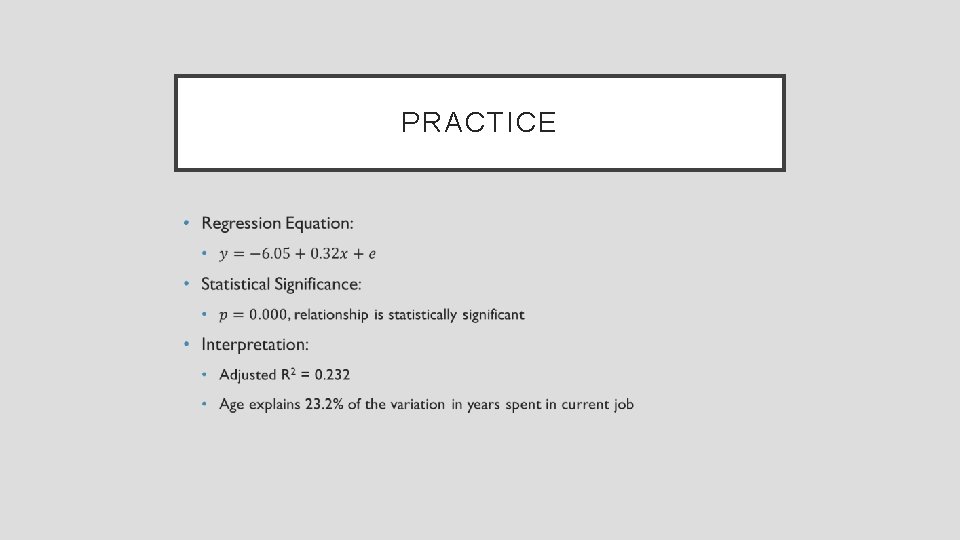
- Slides: 29
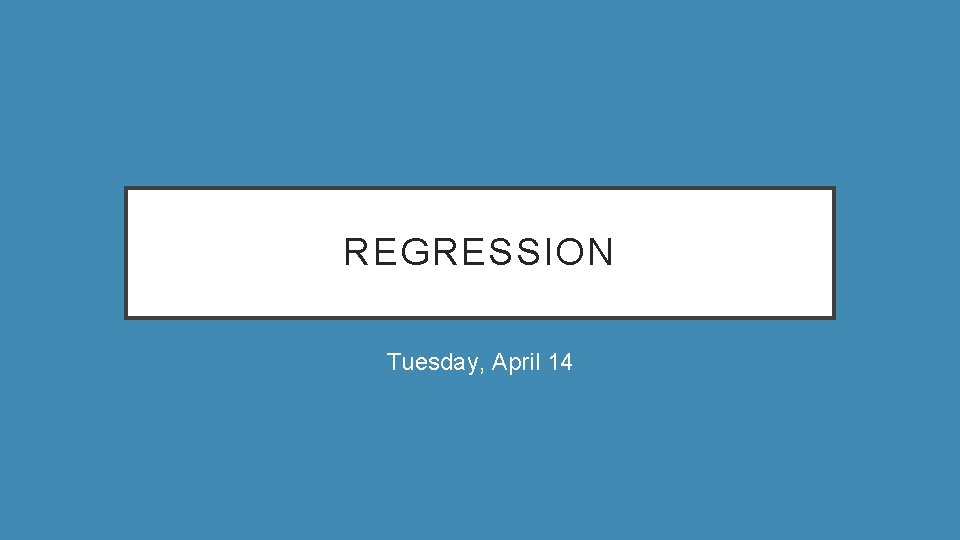
REGRESSION Tuesday, April 14
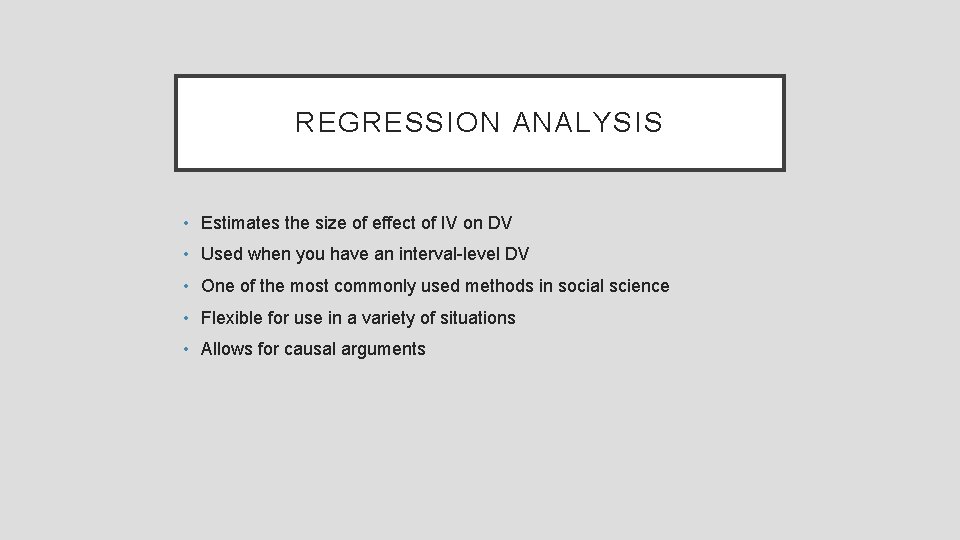
REGRESSION ANALYSIS • Estimates the size of effect of IV on DV • Used when you have an interval-level DV • One of the most commonly used methods in social science • Flexible for use in a variety of situations • Allows for causal arguments
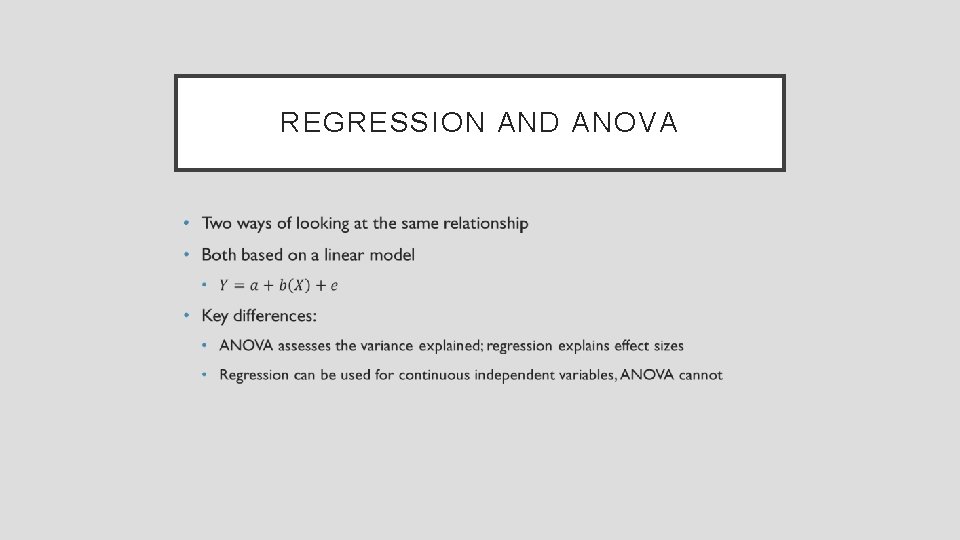
REGRESSION AND ANOVA •
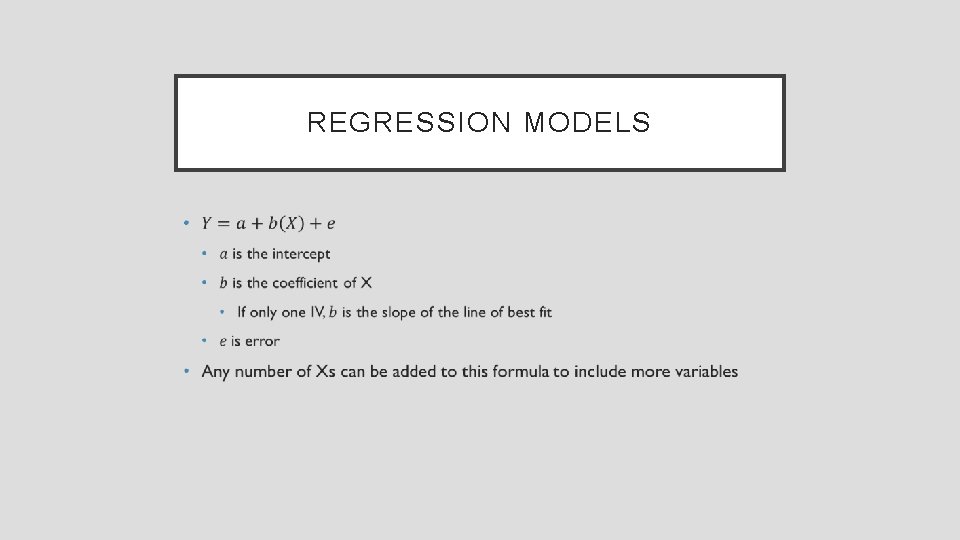
REGRESSION MODELS •
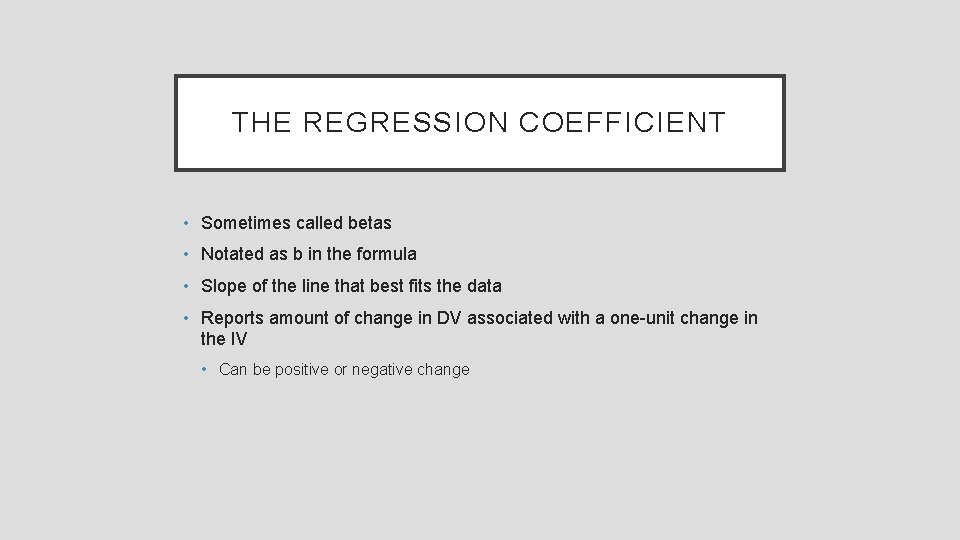
THE REGRESSION COEFFICIENT • Sometimes called betas • Notated as b in the formula • Slope of the line that best fits the data • Reports amount of change in DV associated with a one-unit change in the IV • Can be positive or negative change
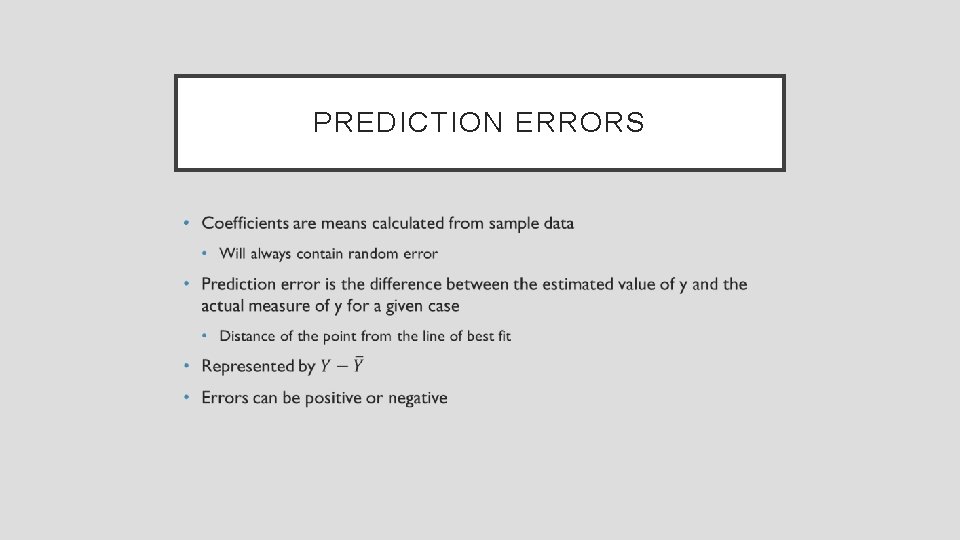
PREDICTION ERRORS •
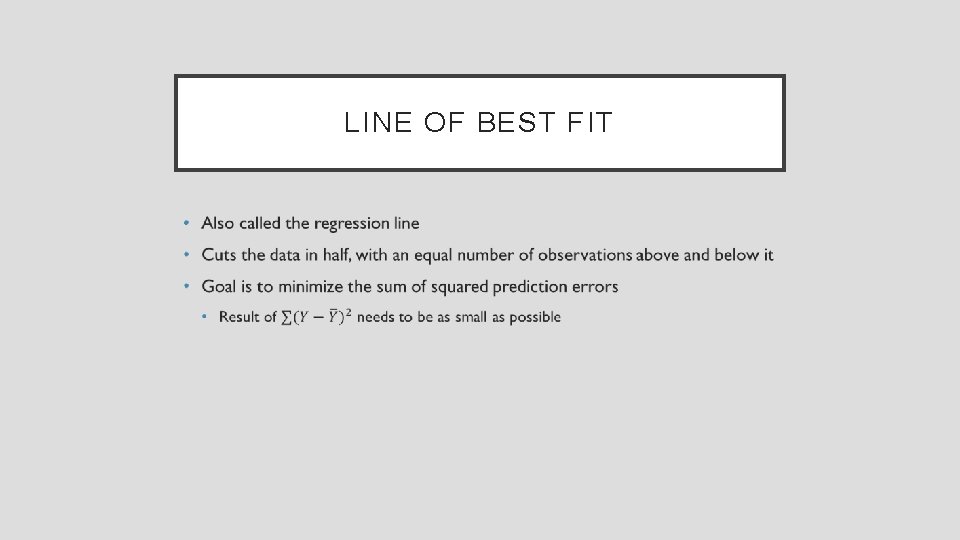
LINE OF BEST FIT •
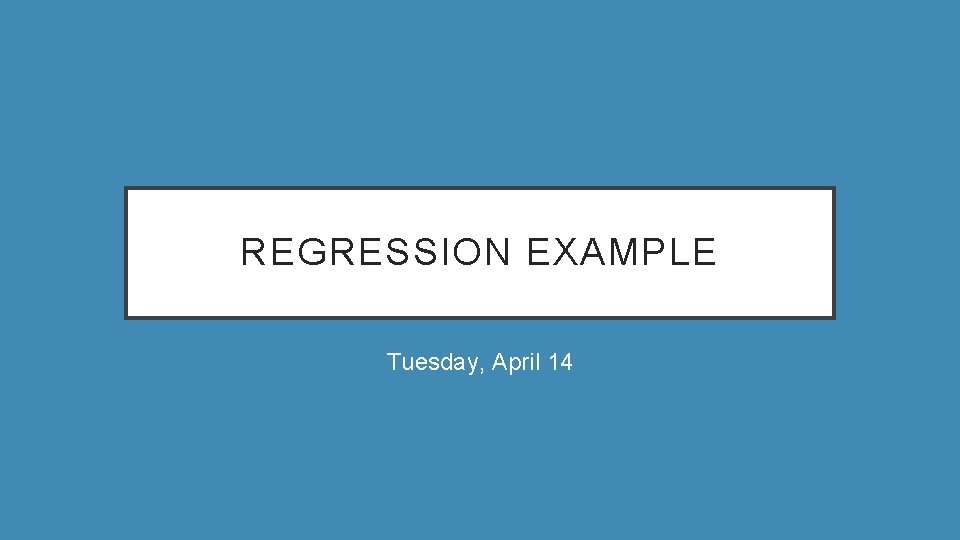
REGRESSION EXAMPLE Tuesday, April 14
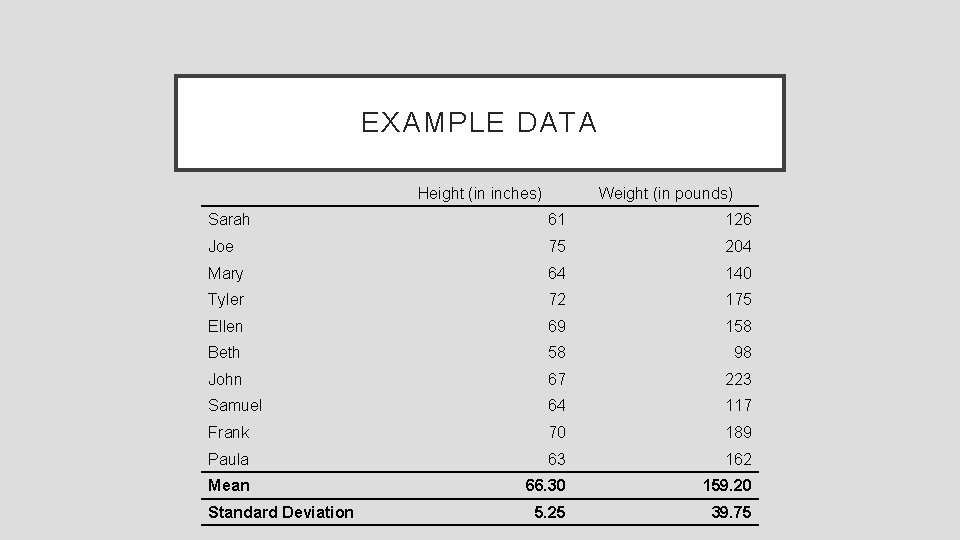
EXAMPLE DATA Height (in inches) Weight (in pounds) Sarah 61 126 Joe 75 204 Mary 64 140 Tyler 72 175 Ellen 69 158 Beth 58 98 John 67 223 Samuel 64 117 Frank 70 189 Paula 63 162 Mean 66. 30 159. 20 5. 25 39. 75 Standard Deviation
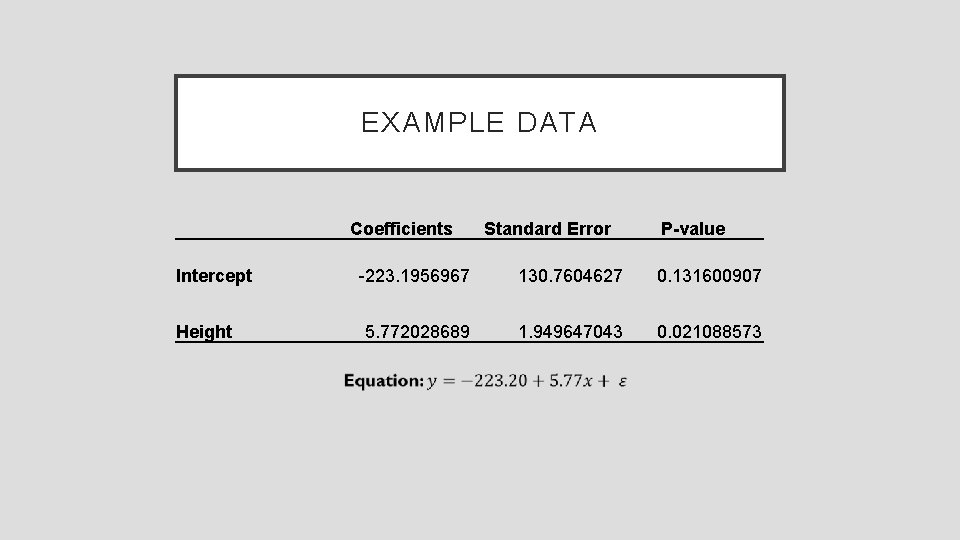
EXAMPLE DATA Coefficients Intercept Height Standard Error P-value -223. 1956967 130. 7604627 0. 131600907 5. 772028689 1. 949647043 0. 021088573
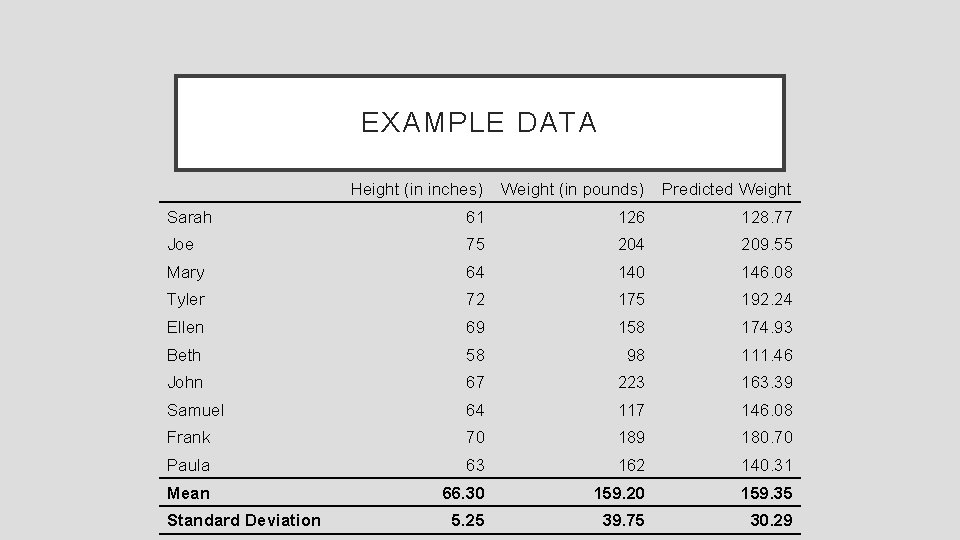
EXAMPLE DATA Height (in inches) Weight (in pounds) Predicted Weight Sarah 61 126 128. 77 Joe 75 204 209. 55 Mary 64 140 146. 08 Tyler 72 175 192. 24 Ellen 69 158 174. 93 Beth 58 98 111. 46 John 67 223 163. 39 Samuel 64 117 146. 08 Frank 70 189 180. 70 Paula 63 162 140. 31 Mean 66. 30 159. 20 159. 35 5. 25 39. 75 30. 29 Standard Deviation
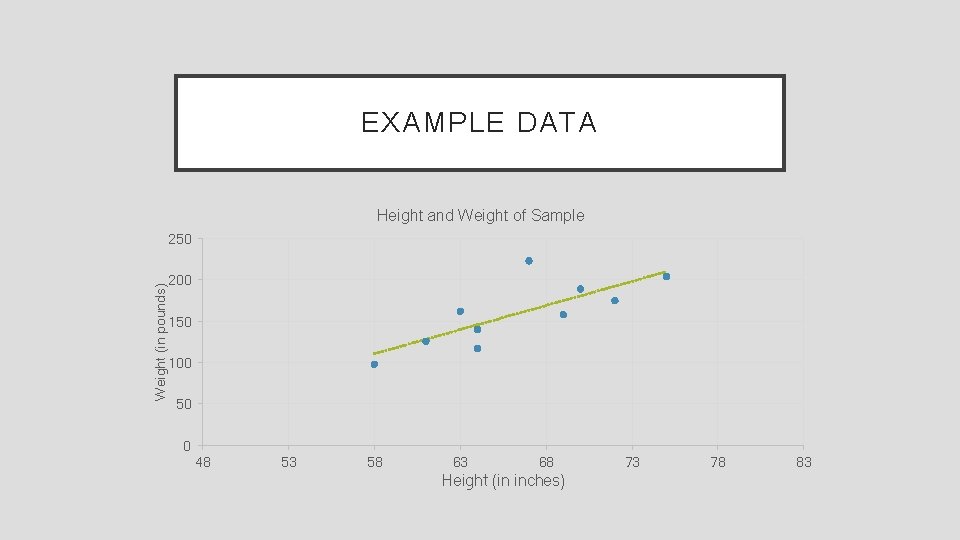
EXAMPLE DATA Height and Weight of Sample Weight (in pounds) 250 200 150 100 50 0 48 53 58 63 68 Height (in inches) 73 78 83
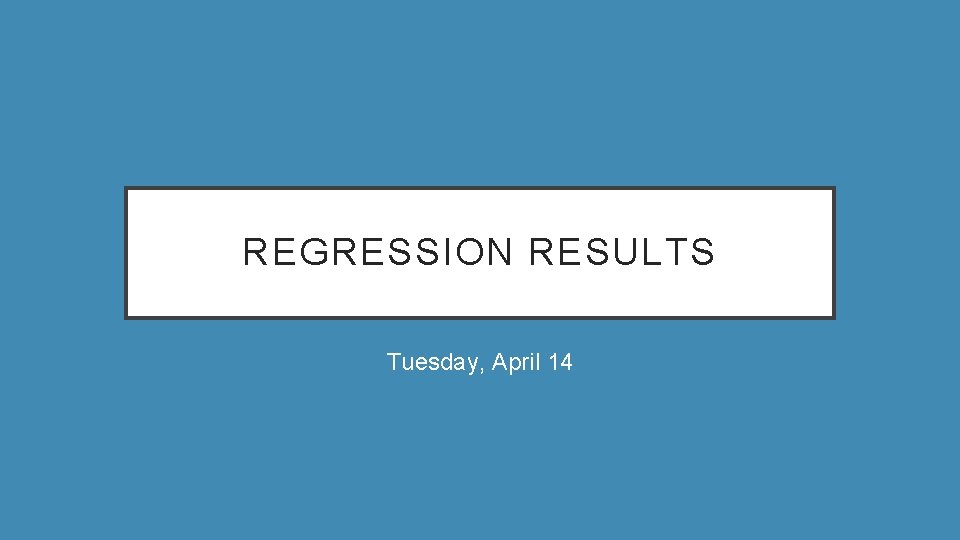
REGRESSION RESULTS Tuesday, April 14
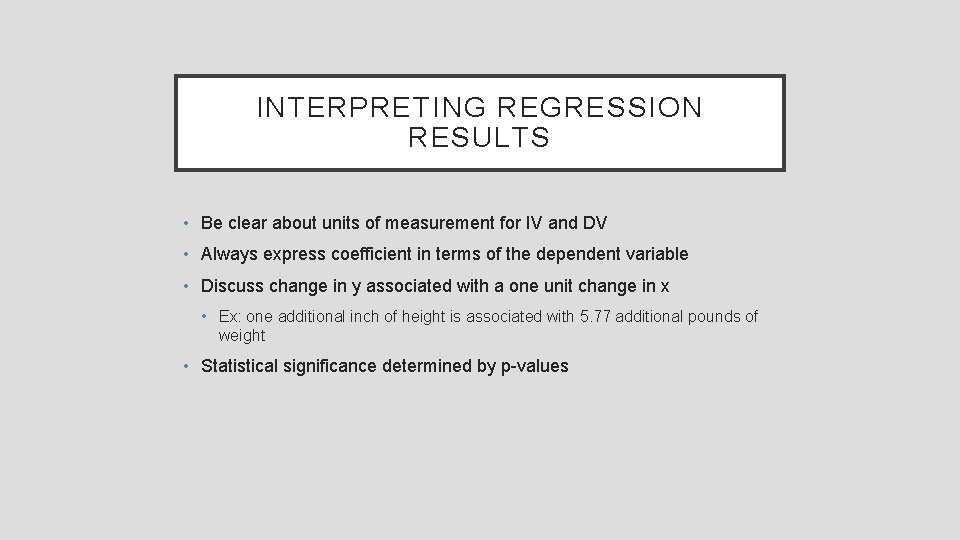
INTERPRETING REGRESSION RESULTS • Be clear about units of measurement for IV and DV • Always express coefficient in terms of the dependent variable • Discuss change in y associated with a one unit change in x • Ex: one additional inch of height is associated with 5. 77 additional pounds of weight • Statistical significance determined by p-values
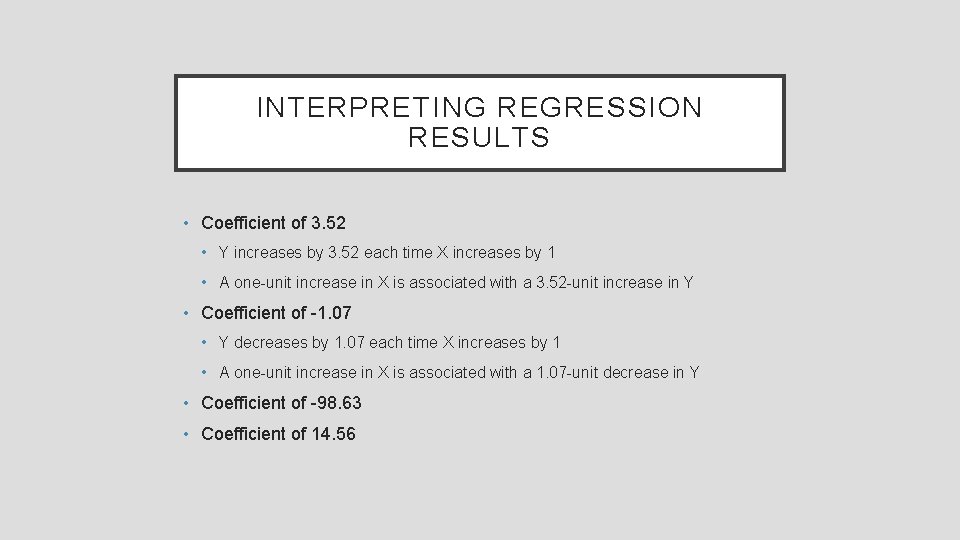
INTERPRETING REGRESSION RESULTS • Coefficient of 3. 52 • Y increases by 3. 52 each time X increases by 1 • A one-unit increase in X is associated with a 3. 52 -unit increase in Y • Coefficient of -1. 07 • Y decreases by 1. 07 each time X increases by 1 • A one-unit increase in X is associated with a 1. 07 -unit decrease in Y • Coefficient of -98. 63 • Coefficient of 14. 56
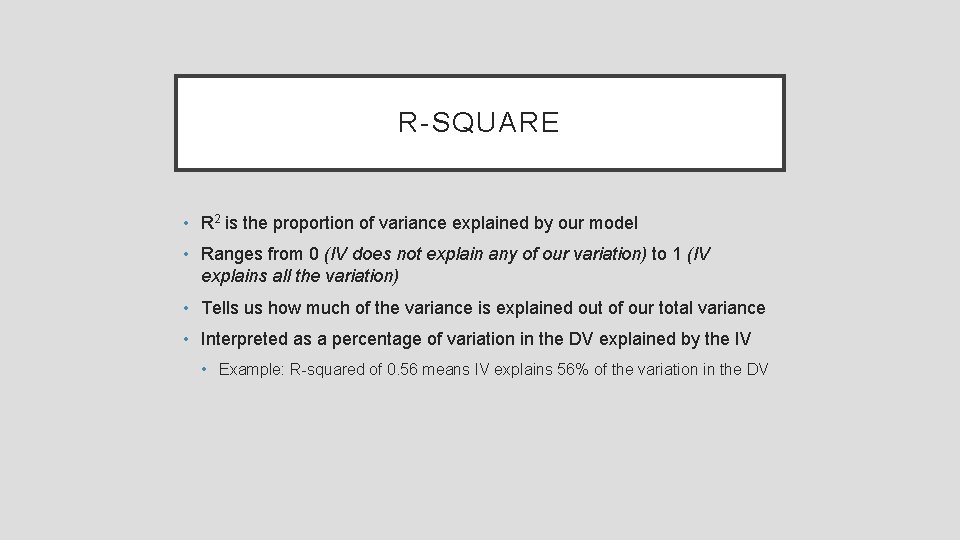
R-SQUARE • R 2 is the proportion of variance explained by our model • Ranges from 0 (IV does not explain any of our variation) to 1 (IV explains all the variation) • Tells us how much of the variance is explained out of our total variance • Interpreted as a percentage of variation in the DV explained by the IV • Example: R-squared of 0. 56 means IV explains 56% of the variation in the DV
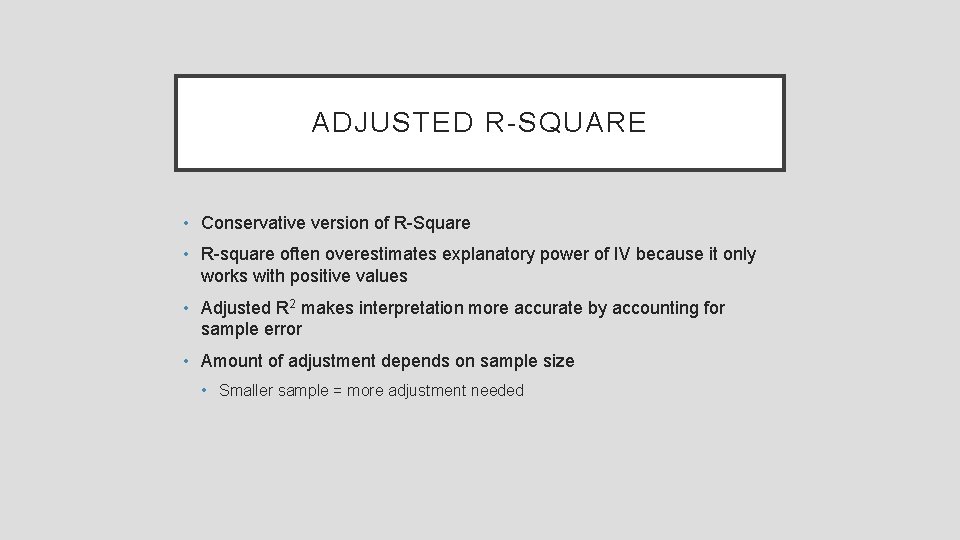
ADJUSTED R-SQUARE • Conservative version of R-Square • R-square often overestimates explanatory power of IV because it only works with positive values • Adjusted R 2 makes interpretation more accurate by accounting for sample error • Amount of adjustment depends on sample size • Smaller sample = more adjustment needed
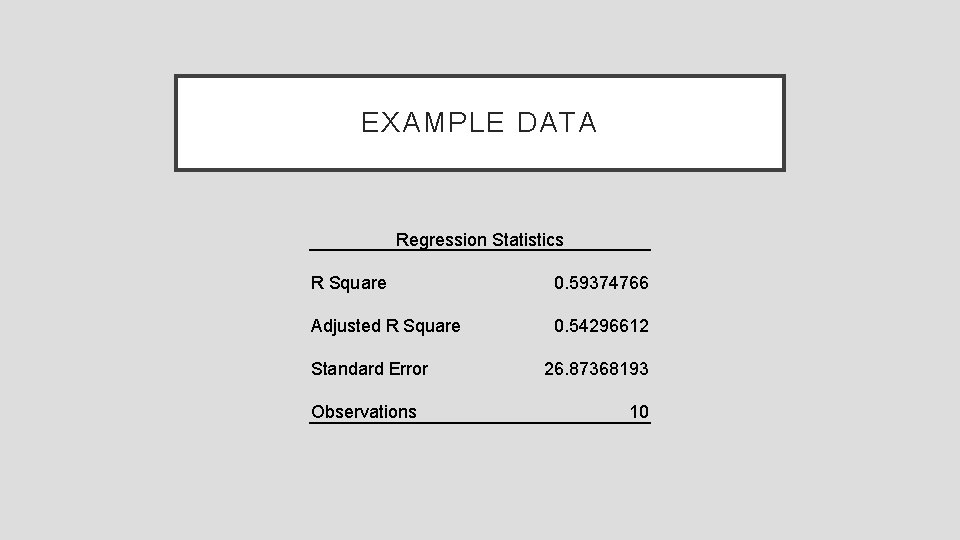
EXAMPLE DATA Regression Statistics R Square 0. 59374766 Adjusted R Square 0. 54296612 Standard Error Observations 26. 87368193 10
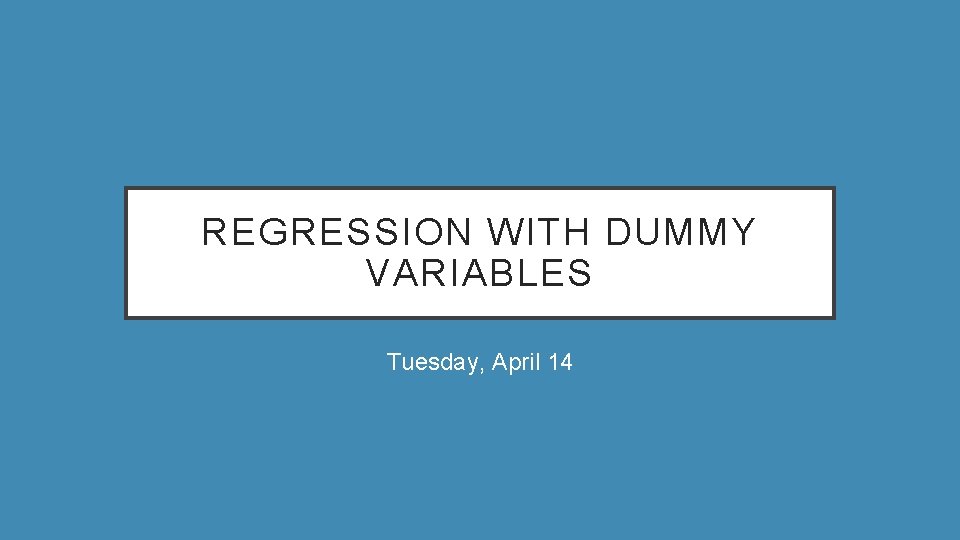
REGRESSION WITH DUMMY VARIABLES Tuesday, April 14
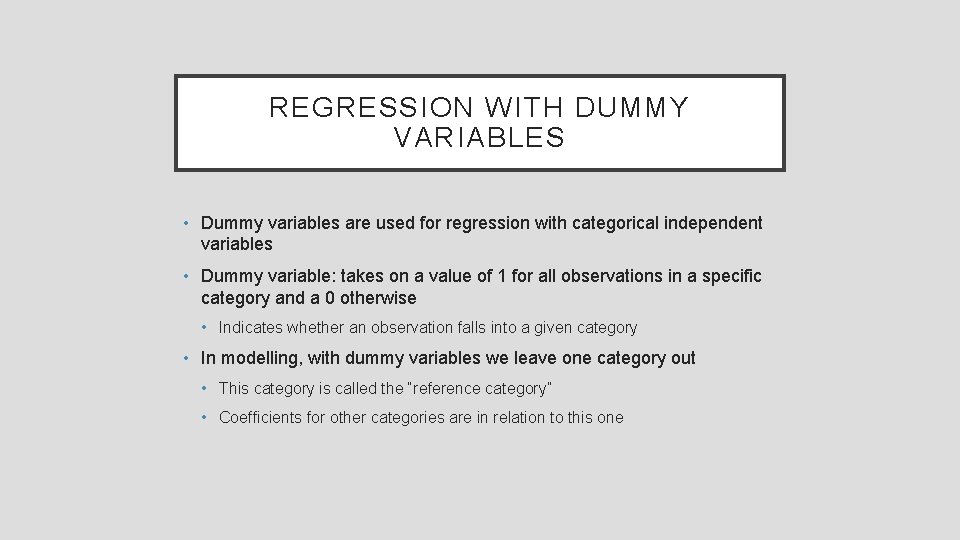
REGRESSION WITH DUMMY VARIABLES • Dummy variables are used for regression with categorical independent variables • Dummy variable: takes on a value of 1 for all observations in a specific category and a 0 otherwise • Indicates whether an observation falls into a given category • In modelling, with dummy variables we leave one category out • This category is called the “reference category” • Coefficients for other categories are in relation to this one
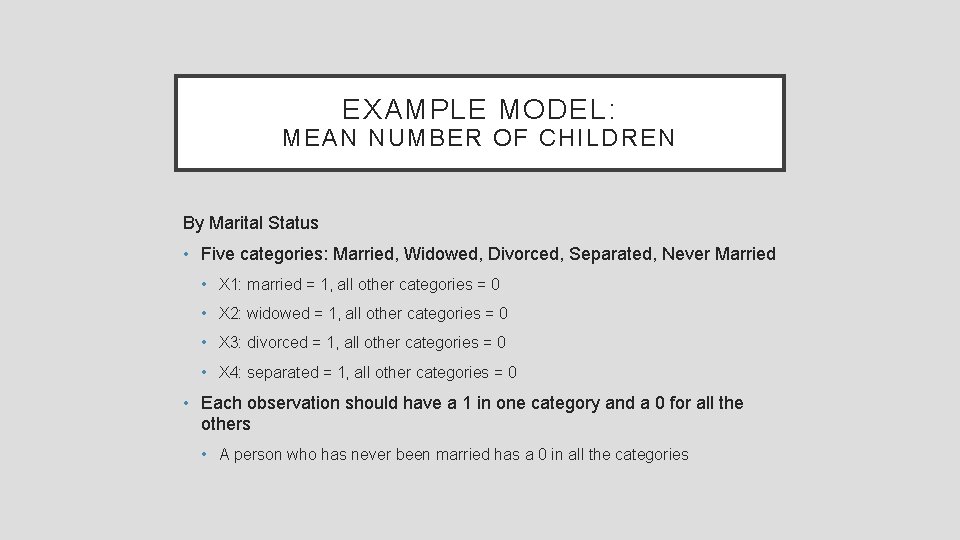
EXAMPLE MODEL: MEAN NUMBER OF CHILDREN By Marital Status • Five categories: Married, Widowed, Divorced, Separated, Never Married • X 1: married = 1, all other categories = 0 • X 2: widowed = 1, all other categories = 0 • X 3: divorced = 1, all other categories = 0 • X 4: separated = 1, all other categories = 0 • Each observation should have a 1 in one category and a 0 for all the others • A person who has never been married has a 0 in all the categories
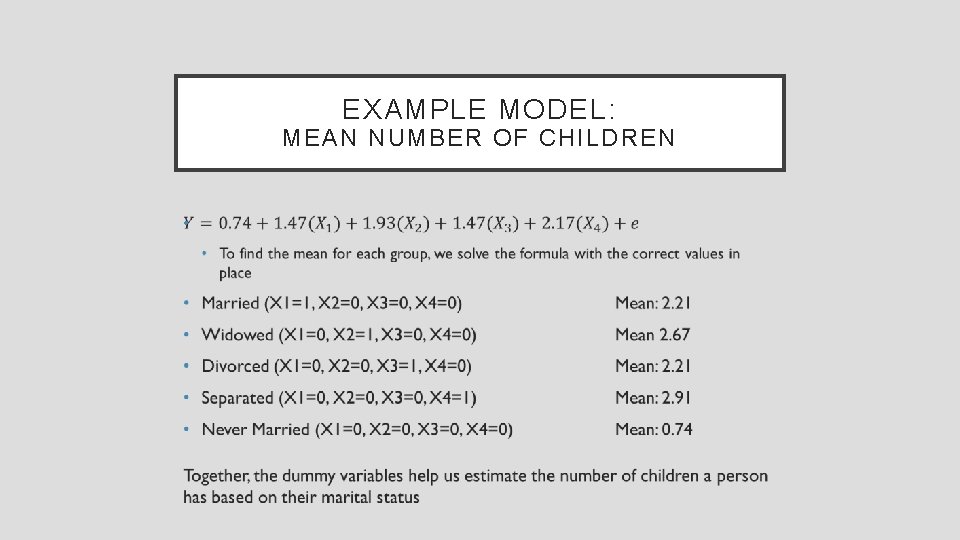
EXAMPLE MODEL: MEAN NUMBER OF CHILDREN •
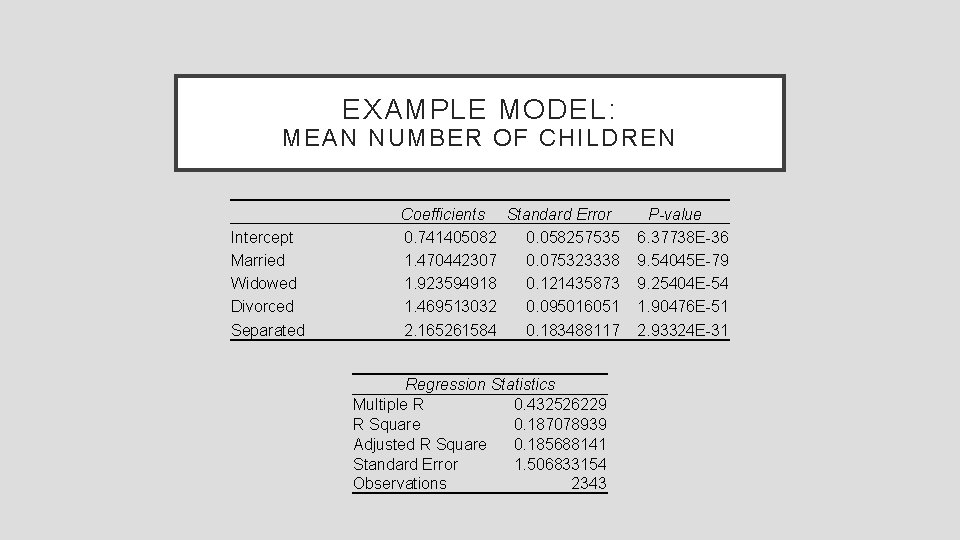
EXAMPLE MODEL: MEAN NUMBER OF CHILDREN Intercept Married Widowed Divorced Coefficients Standard Error 0. 741405082 0. 058257535 1. 470442307 0. 075323338 1. 923594918 0. 121435873 1. 469513032 0. 095016051 P-value 6. 37738 E-36 9. 54045 E-79 9. 25404 E-54 1. 90476 E-51 Separated 2. 165261584 2. 93324 E-31 0. 183488117 Regression Statistics Multiple R 0. 432526229 R Square 0. 187078939 Adjusted R Square 0. 185688141 Standard Error 1. 506833154 Observations 2343
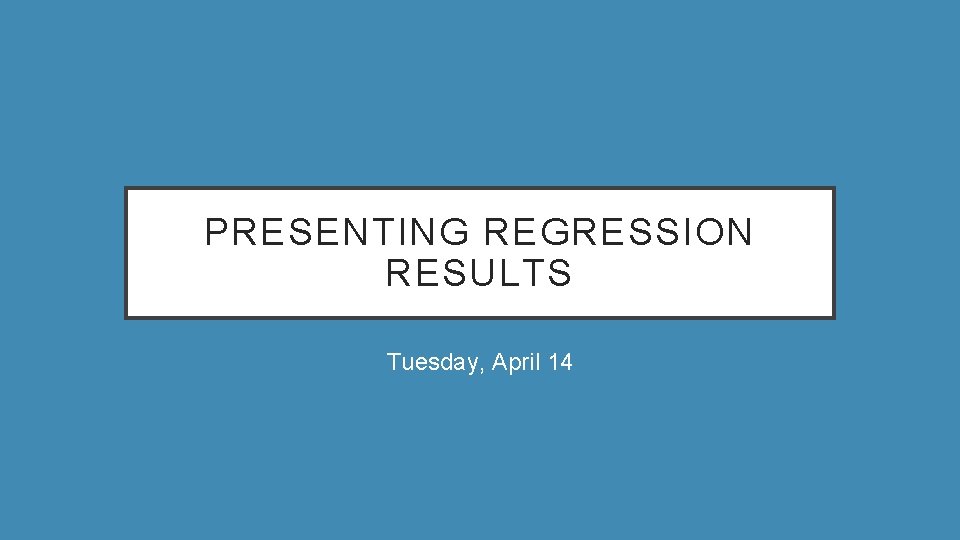
PRESENTING REGRESSION RESULTS Tuesday, April 14
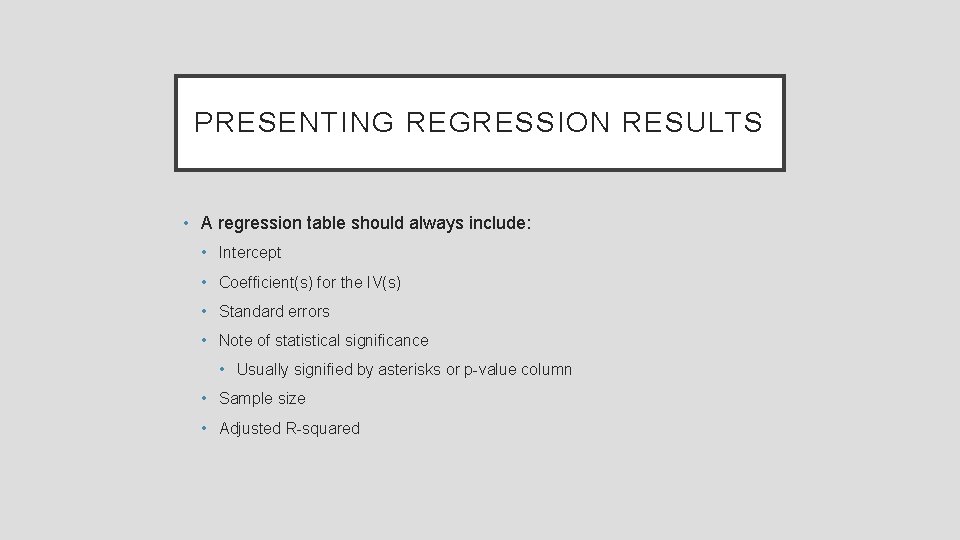
PRESENTING REGRESSION RESULTS • A regression table should always include: • Intercept • Coefficient(s) for the IV(s) • Standard errors • Note of statistical significance • Usually signified by asterisks or p-value column • Sample size • Adjusted R-squared
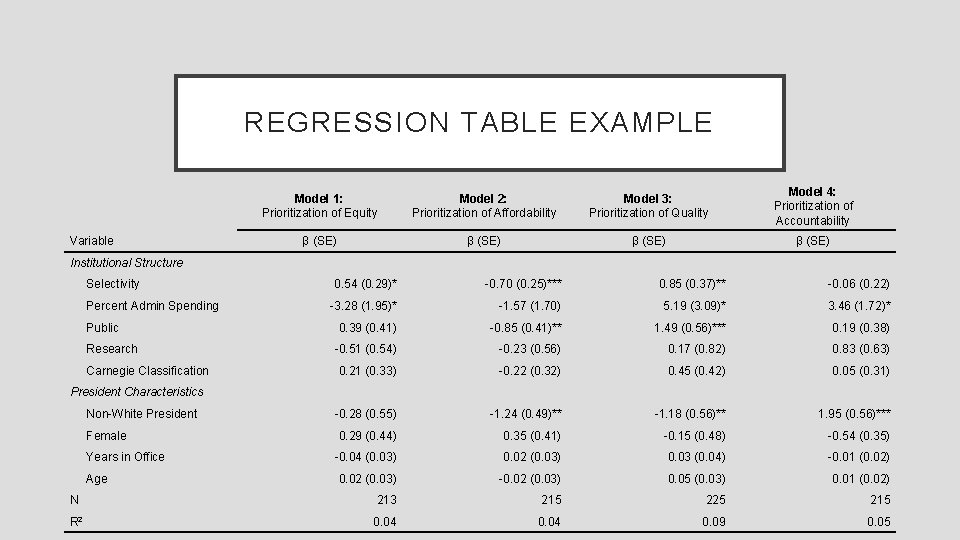
REGRESSION TABLE EXAMPLE Variable Model 1: Prioritization of Equity Model 2: Prioritization of Affordability Model 3: Prioritization of Quality Model 4: Prioritization of Accountability β (SE) Institutional Structure Selectivity 0. 54 (0. 29)* -0. 70 (0. 25)*** 0. 85 (0. 37)** -0. 06 (0. 22) -3. 28 (1. 95)* -1. 57 (1. 70) 5. 19 (3. 09)* 3. 46 (1. 72)* 0. 39 (0. 41) -0. 85 (0. 41)** 1. 49 (0. 56)*** 0. 19 (0. 38) -0. 51 (0. 54) -0. 23 (0. 56) 0. 17 (0. 82) 0. 83 (0. 63) 0. 21 (0. 33) -0. 22 (0. 32) 0. 45 (0. 42) 0. 05 (0. 31) -0. 28 (0. 55) -1. 24 (0. 49)** -1. 18 (0. 56)** 1. 95 (0. 56)*** 0. 29 (0. 44) 0. 35 (0. 41) -0. 15 (0. 48) -0. 54 (0. 35) -0. 04 (0. 03) 0. 02 (0. 03) 0. 03 (0. 04) -0. 01 (0. 02) 0. 02 (0. 03) -0. 02 (0. 03) 0. 05 (0. 03) 0. 01 (0. 02) N 213 215 225 215 R 2 0. 04 0. 09 0. 05 Percent Admin Spending Public Research Carnegie Classification President Characteristics Non-White President Female Years in Office Age
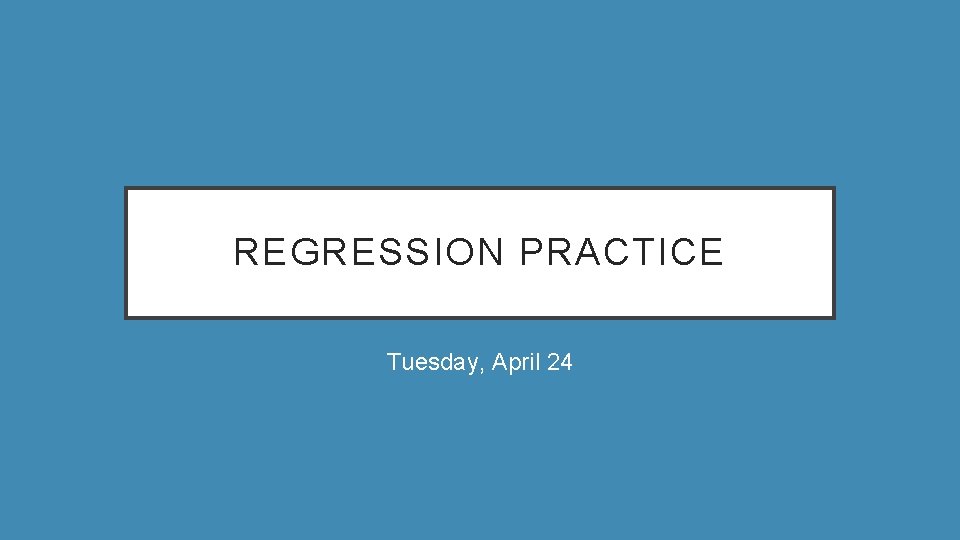
REGRESSION PRACTICE Tuesday, April 24
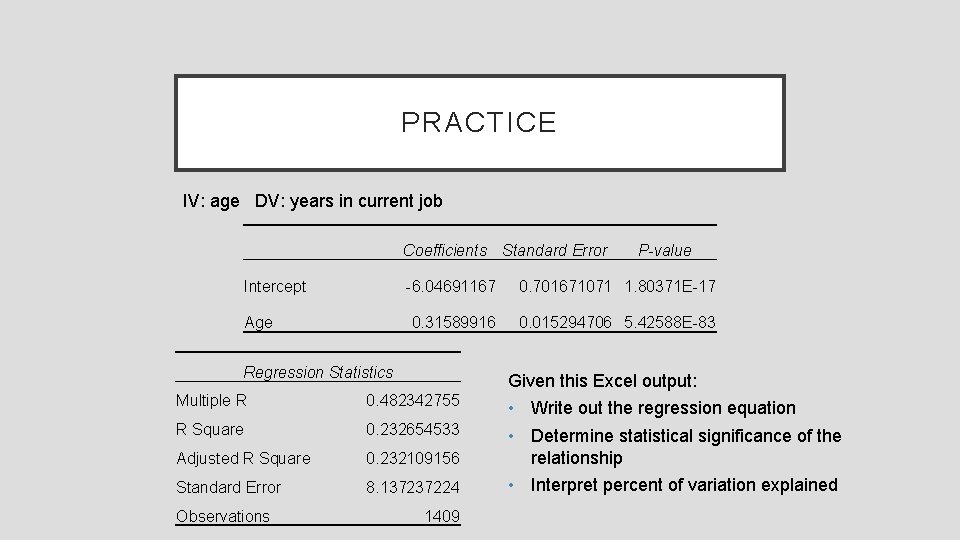
PRACTICE IV: age DV: years in current job Coefficients Standard Error Intercept Age -6. 04691167 0. 701671071 1. 80371 E-17 0. 31589916 0. 015294706 5. 42588 E-83 Regression Statistics Given this Excel output: Multiple R 0. 482342755 R Square 0. 232654533 Adjusted R Square 0. 232109156 Standard Error 8. 137237224 Observations P-value 1409 • Write out the regression equation • Determine statistical significance of the relationship • Interpret percent of variation explained
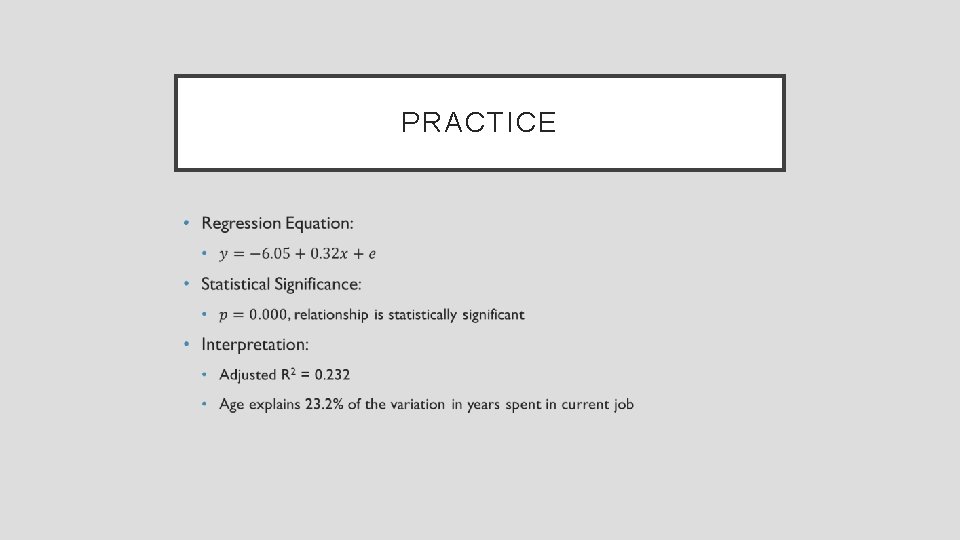
PRACTICE •
The account analysis method estimates cost functions
Who global estimates on prevalence of hearing loss 2020
Who global estimates on prevalence of hearing loss 2020
Eva estimates that 475 songs
Properties of least squares estimators
Creative industries economic estimates
Building maintenance cost estimates
Overapplied manufacturing overhead means that
Fermi estimate
Fermi estimate
Marquis company estimates that annual manufacturing
Linear regression vs multiple regression
Linear model regression
Logistic regression vs linear regression
Logistic regression vs linear regression
Hát kết hợp bộ gõ cơ thể
Slidetodoc
Bổ thể
Tỉ lệ cơ thể trẻ em
Chó sói
Thang điểm glasgow
Hát lên người ơi
Các môn thể thao bắt đầu bằng từ đua
Thế nào là hệ số cao nhất
Các châu lục và đại dương trên thế giới
Công của trọng lực
Trời xanh đây là của chúng ta thể thơ
Mật thư tọa độ 5x5
101012 bằng
Phản ứng thế ankan