Instructor Shengyu Zhang Statistical inference n Statistical inference
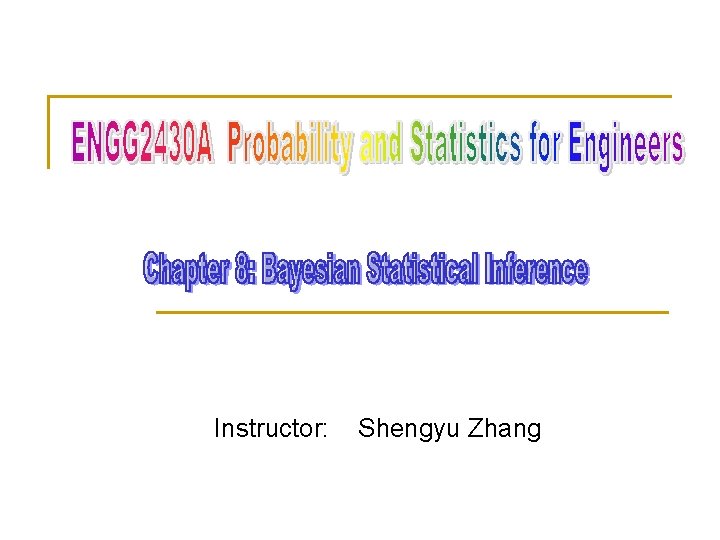
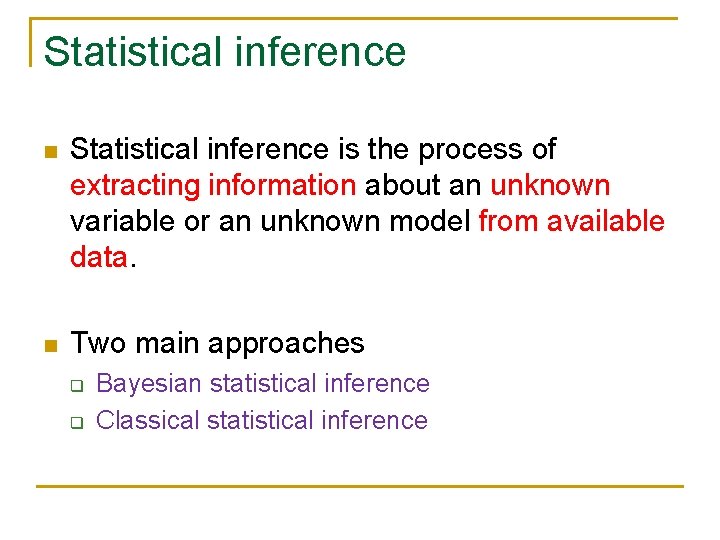
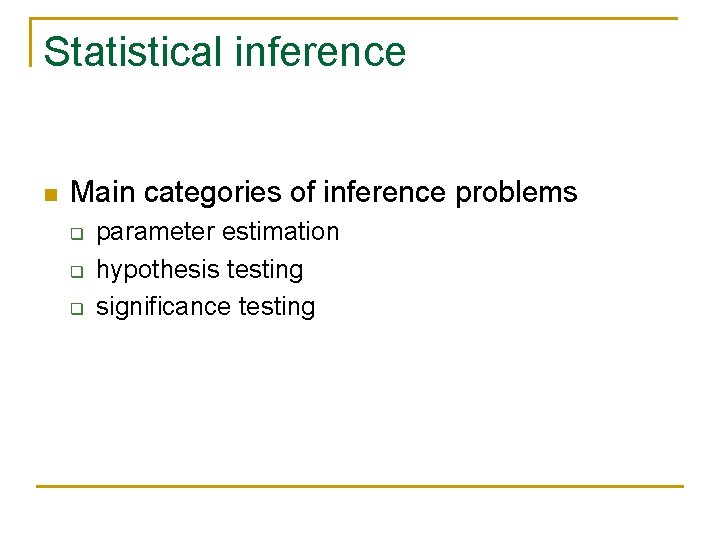
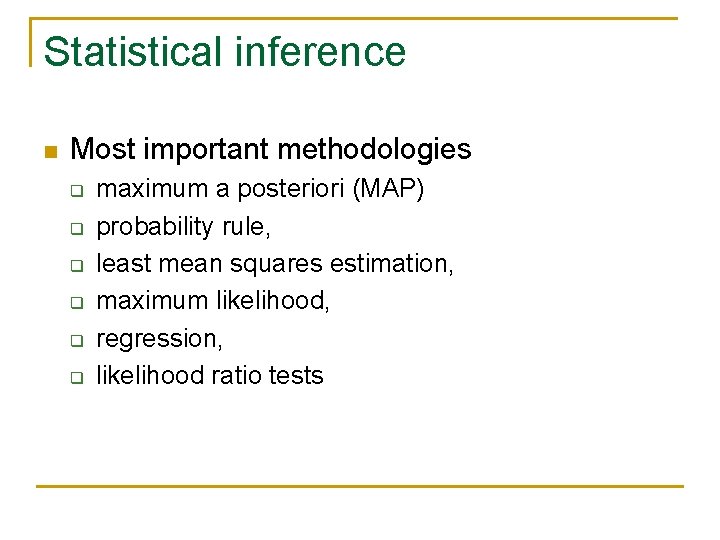
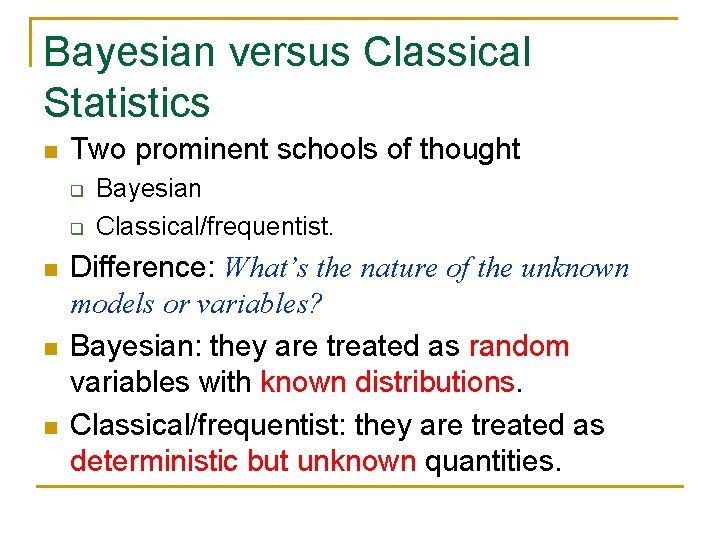
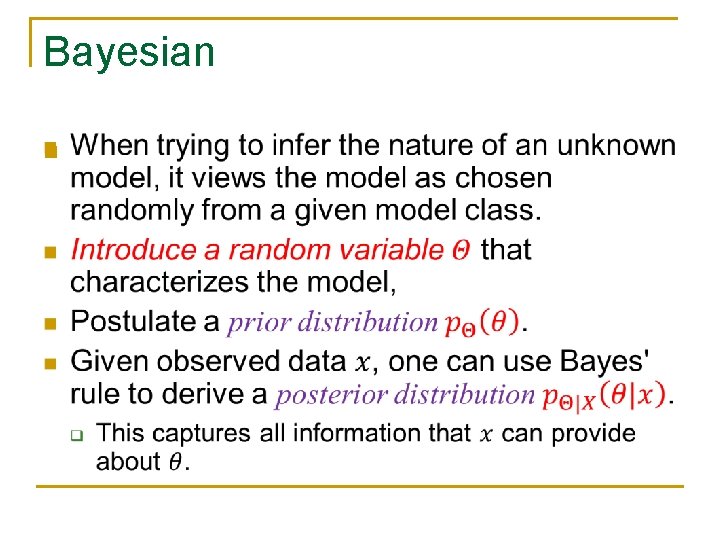
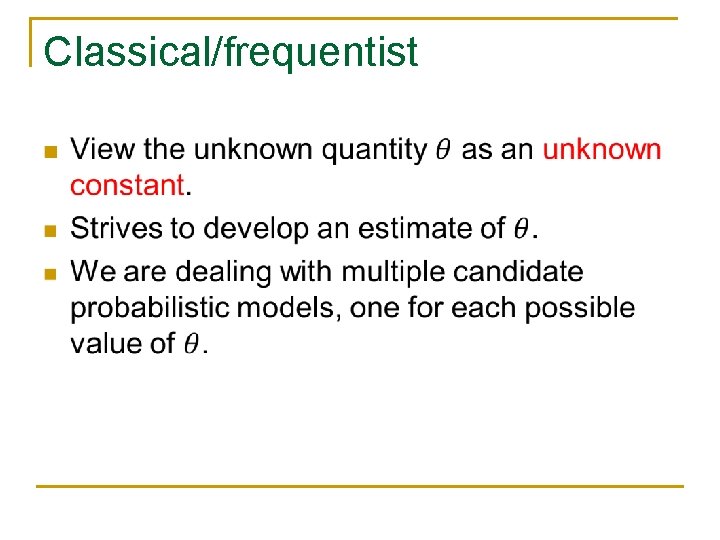
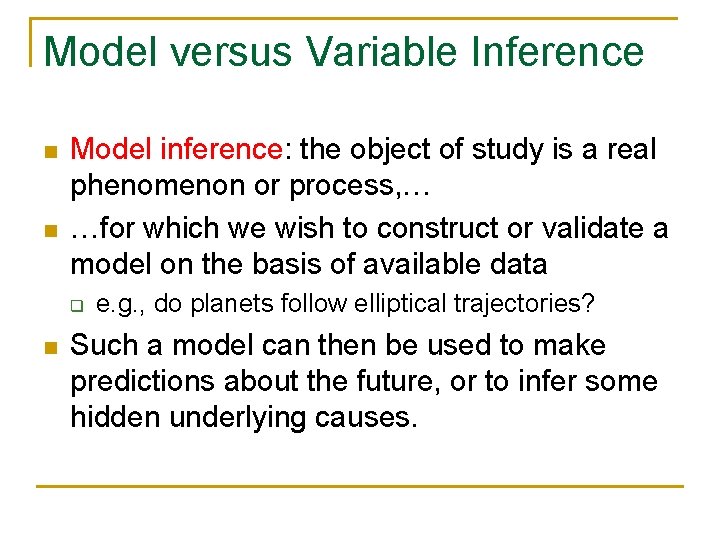
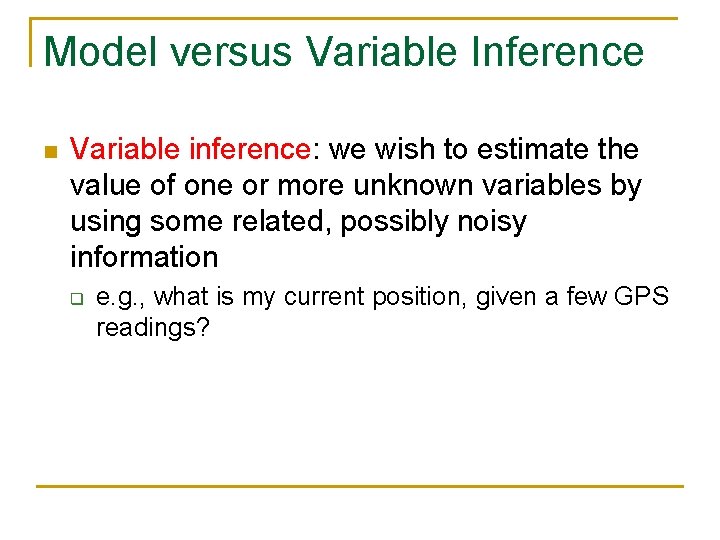
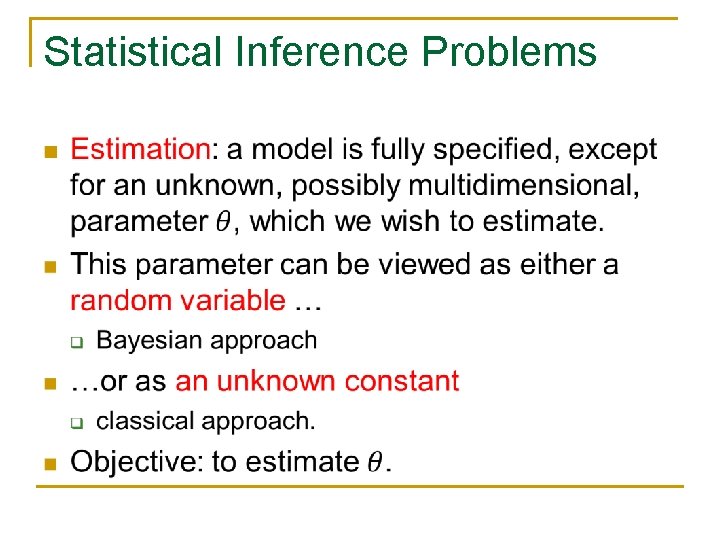
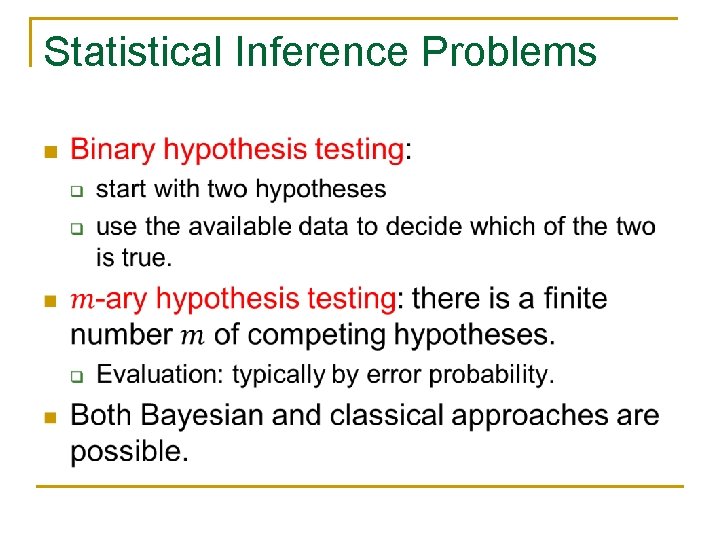
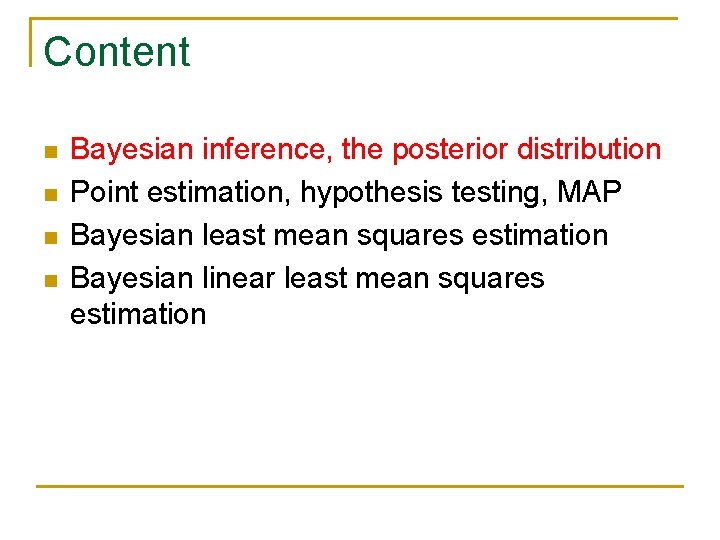
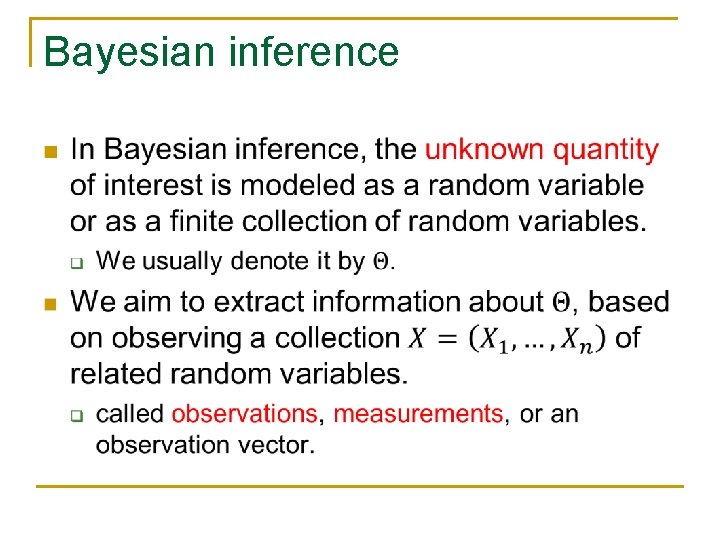
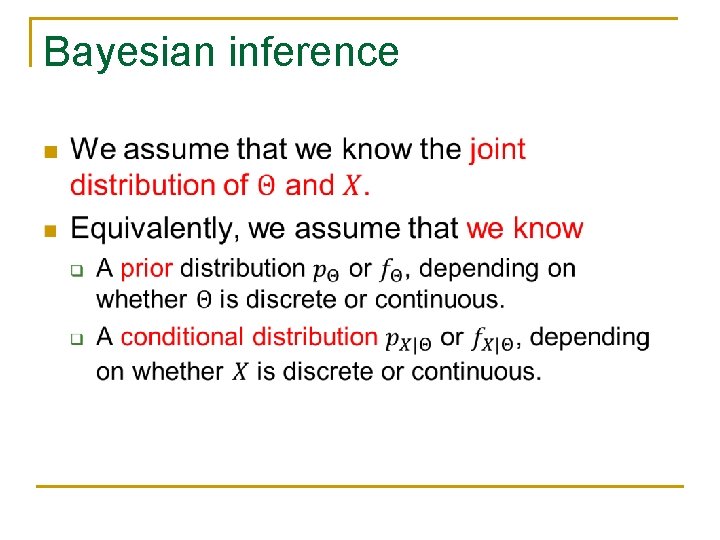
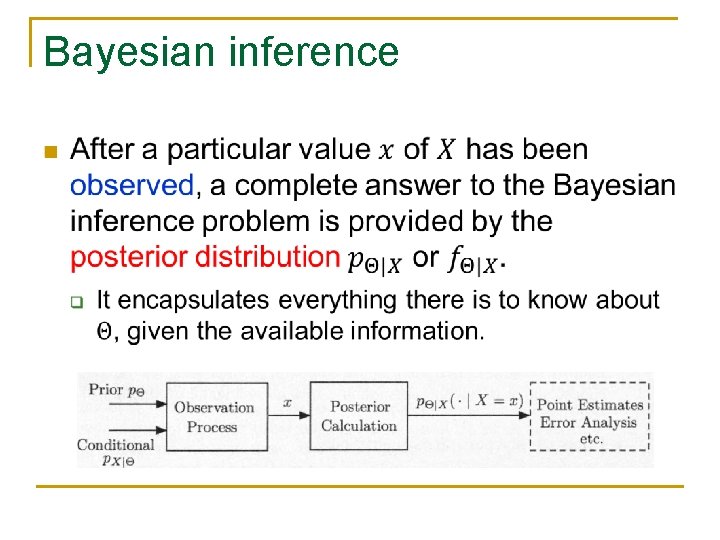
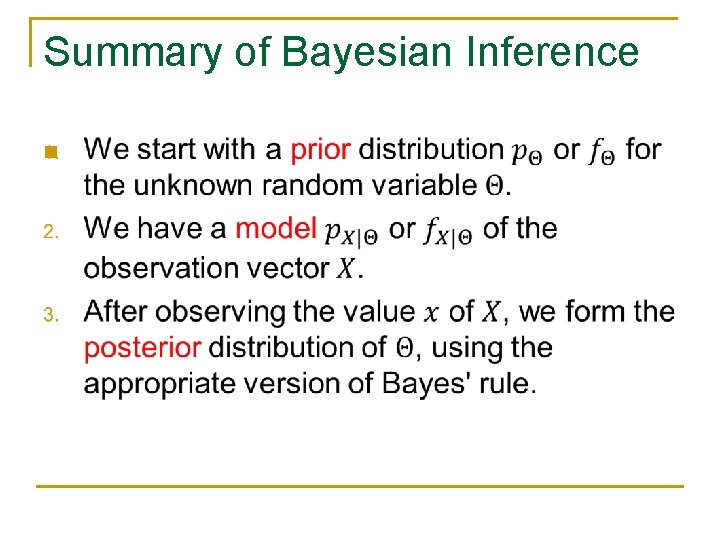
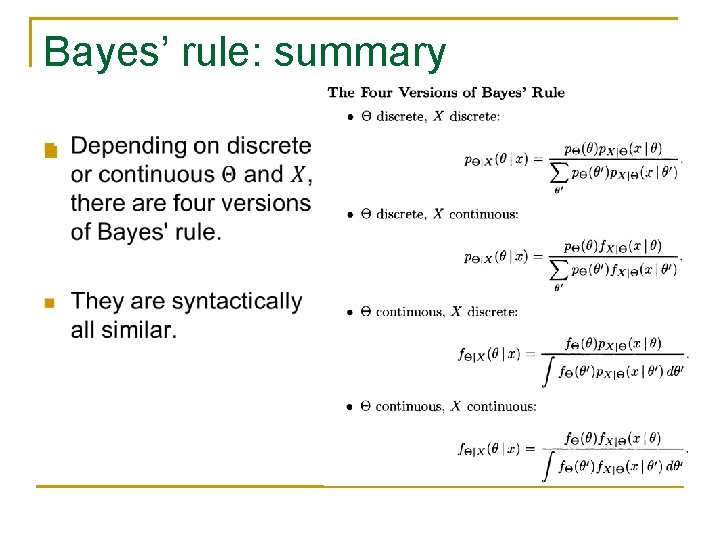
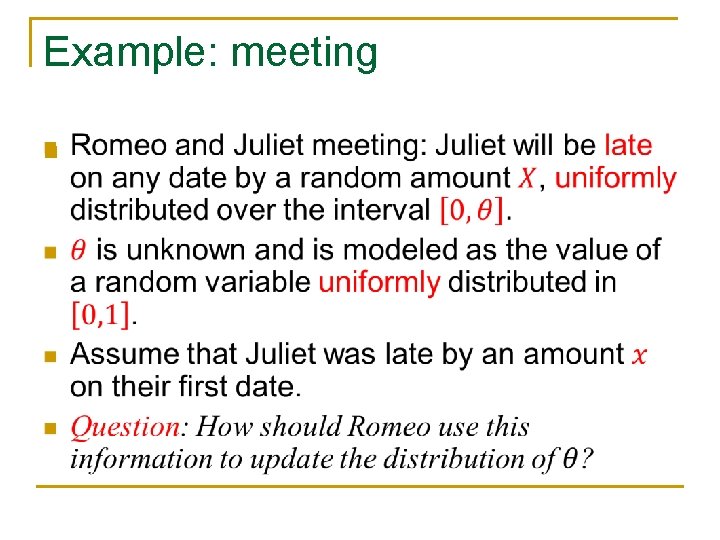
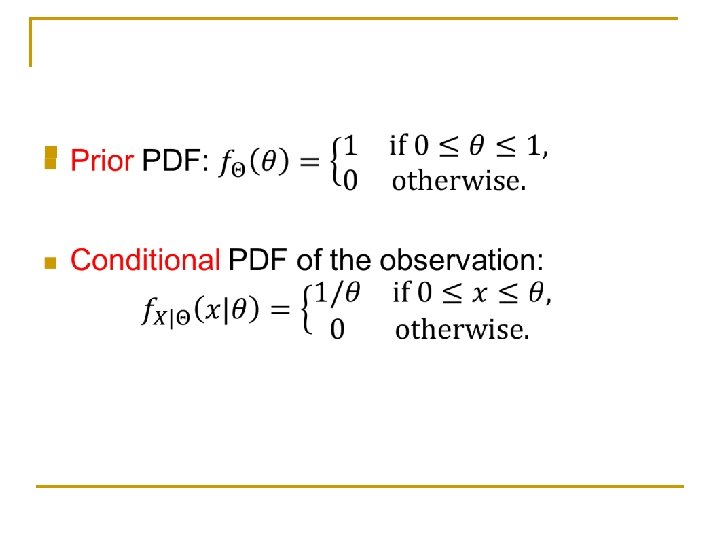
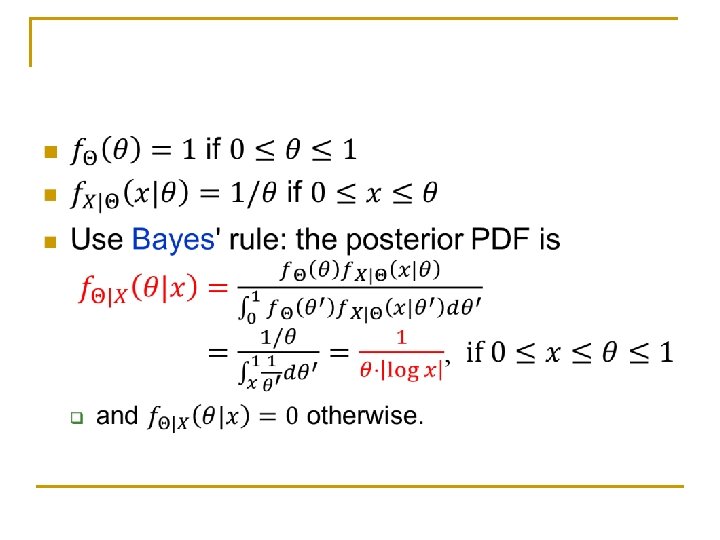
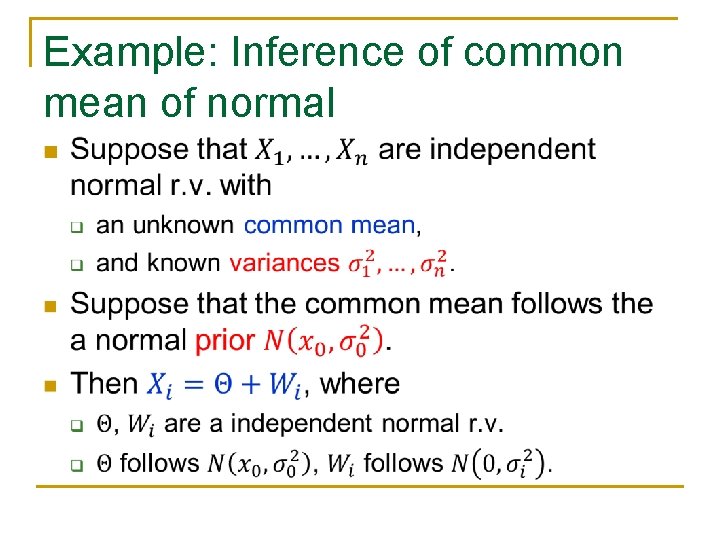
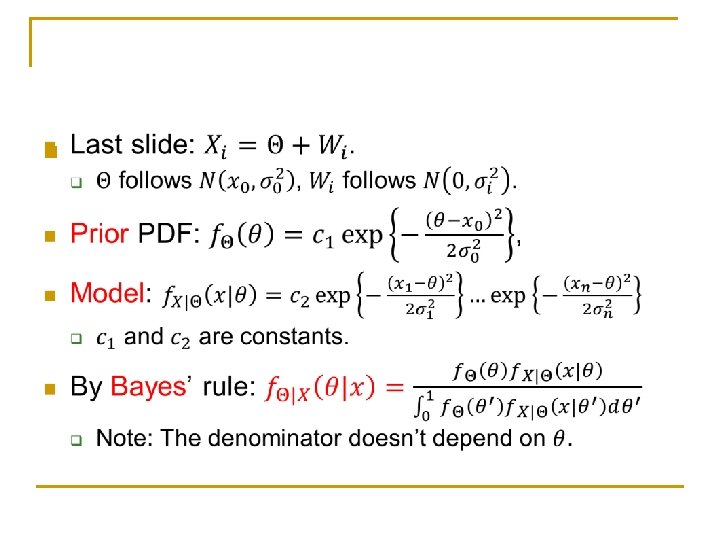
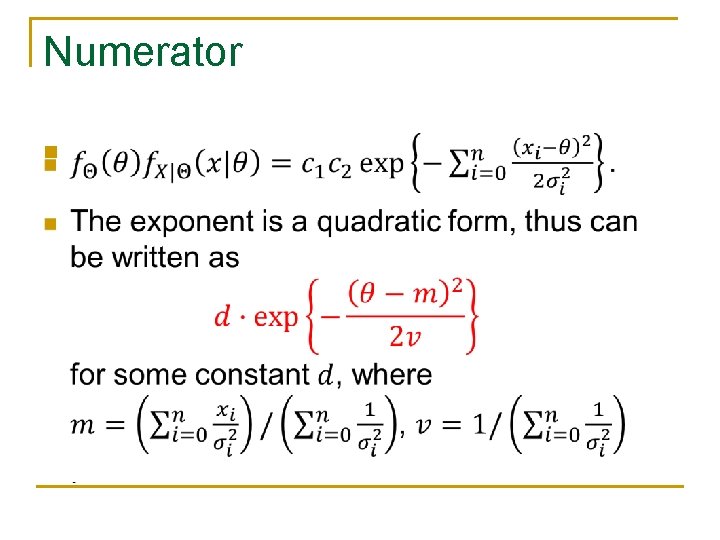
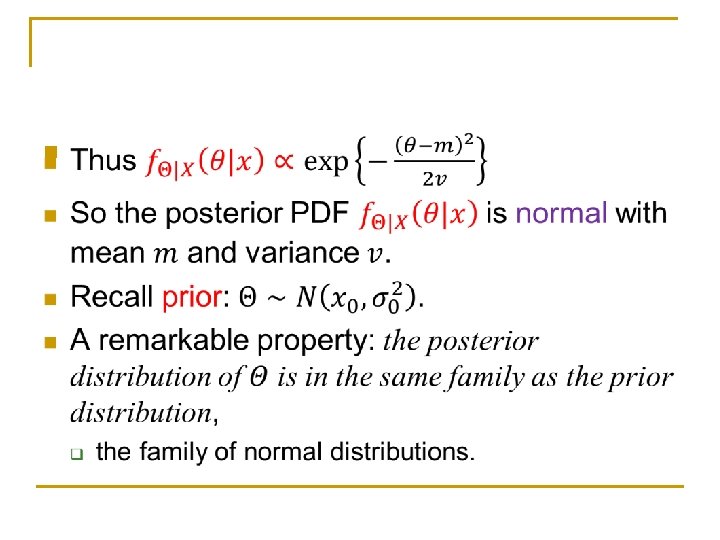
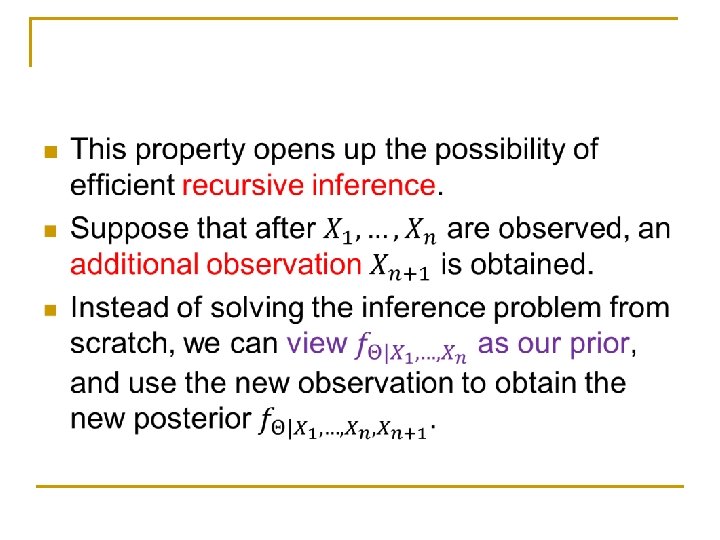
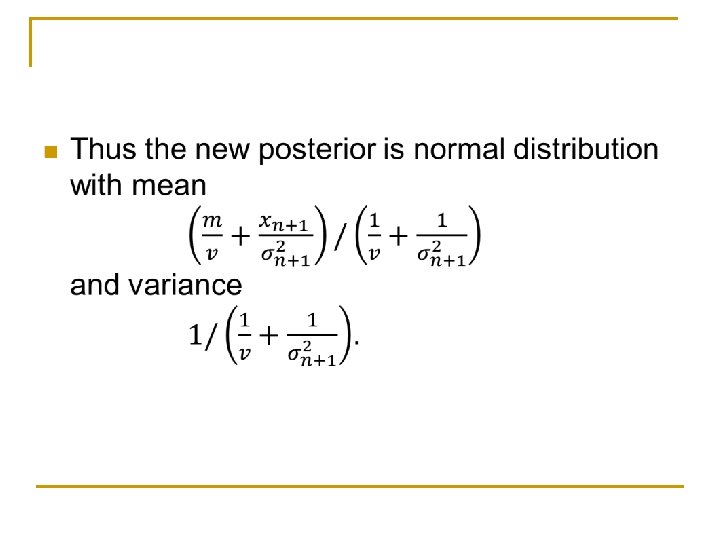
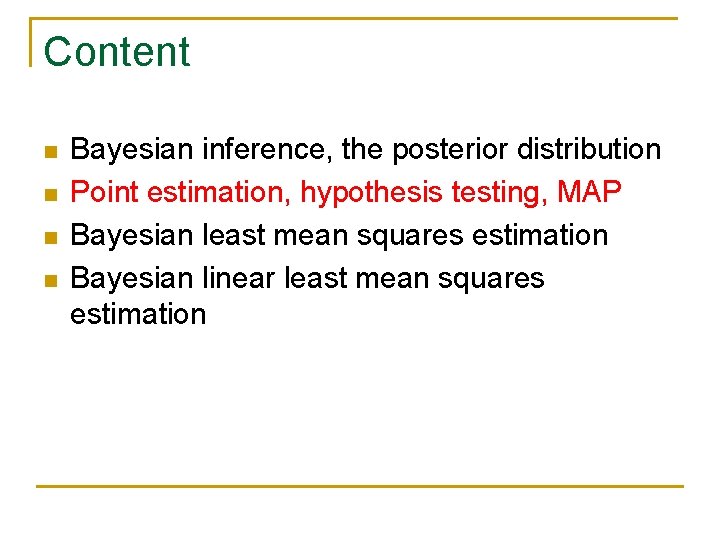
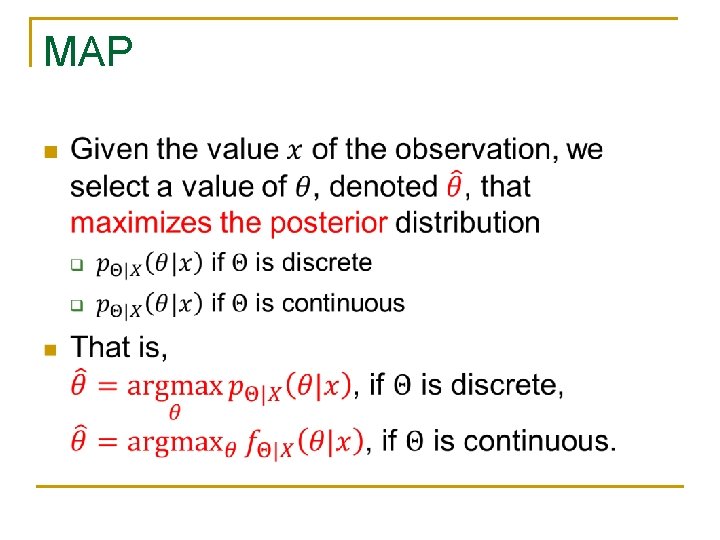
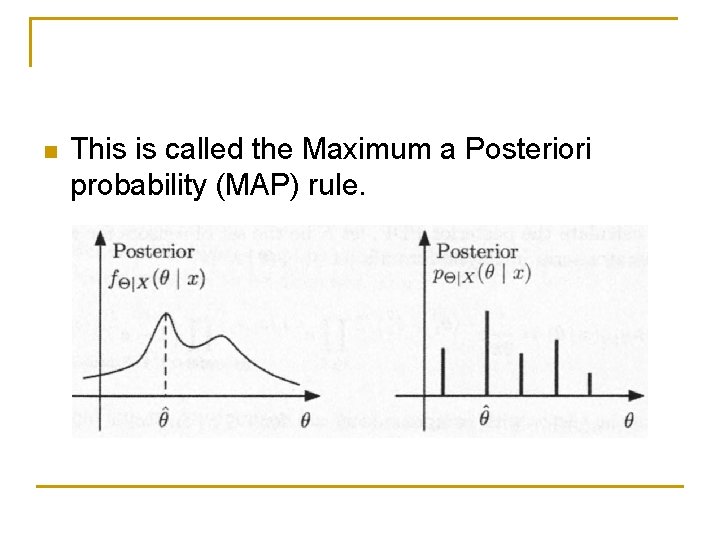
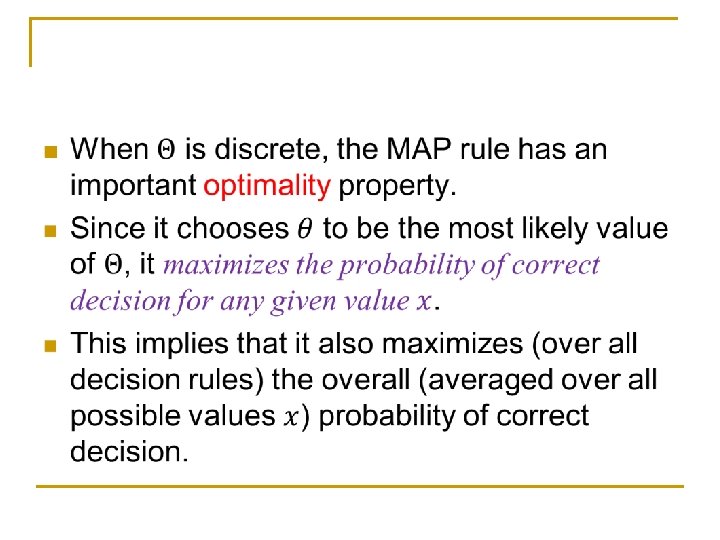
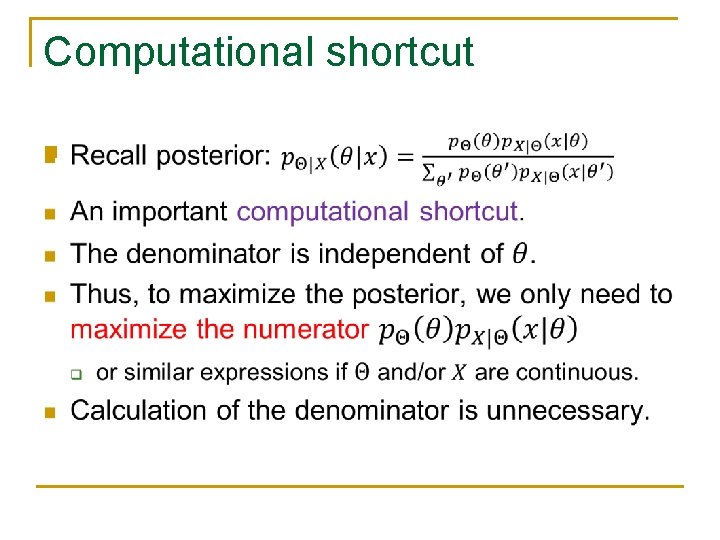
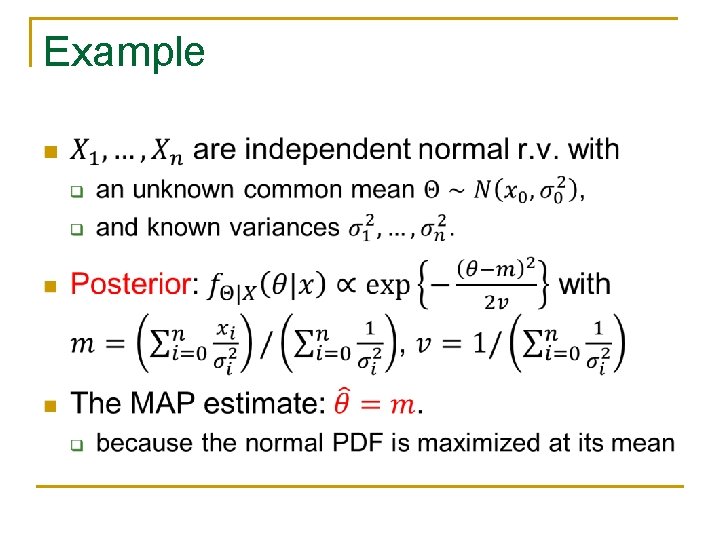
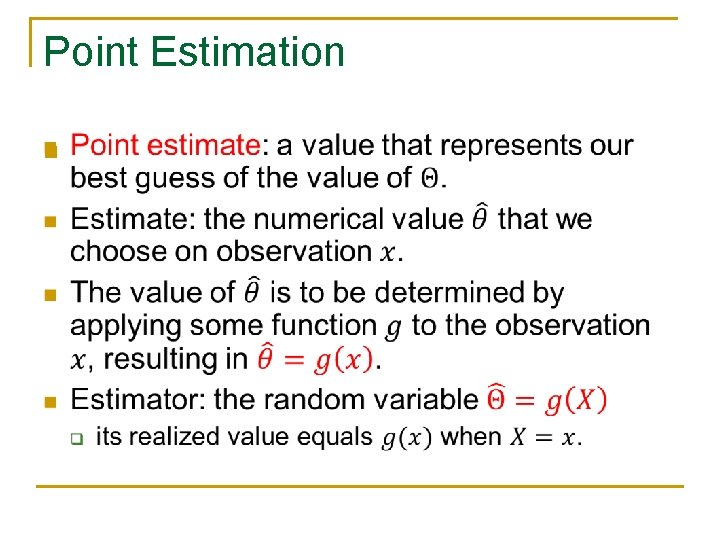
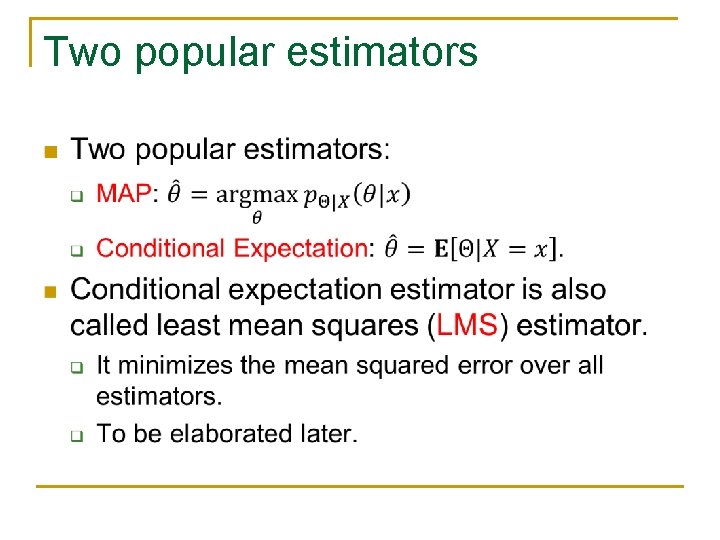
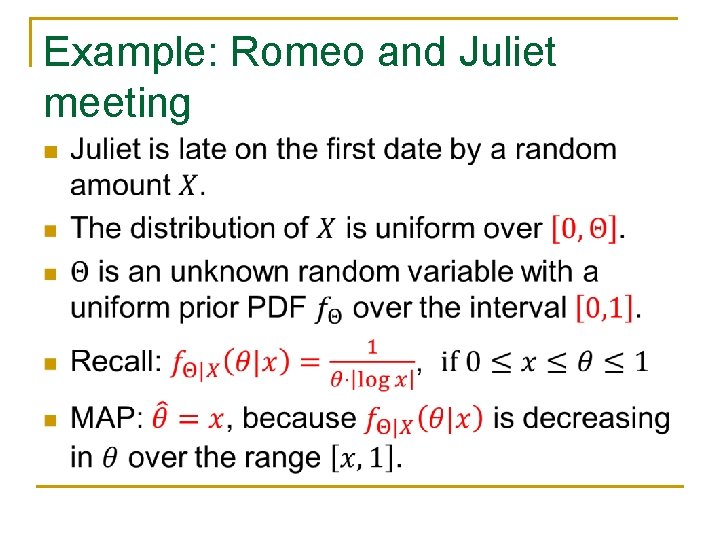
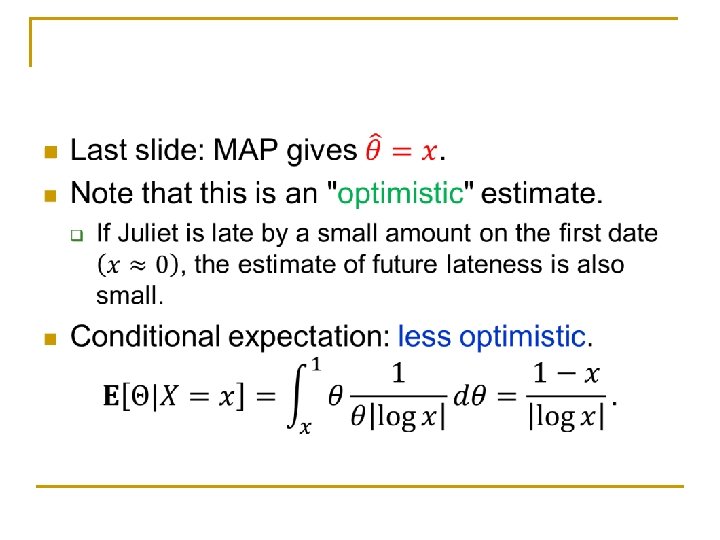
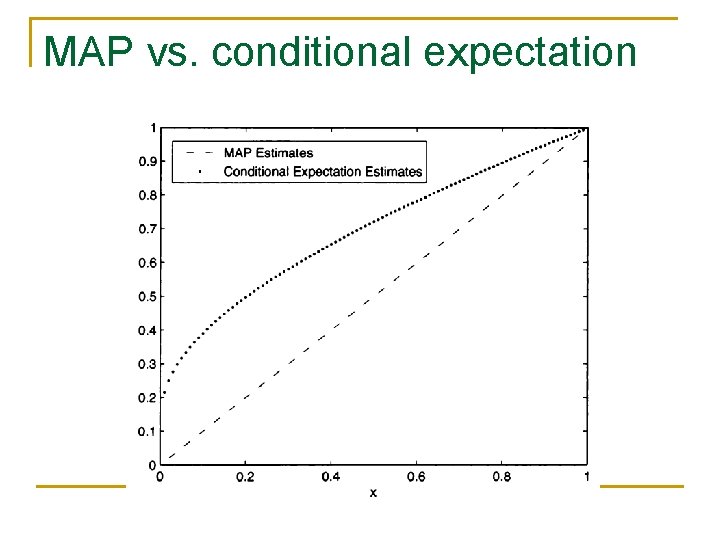
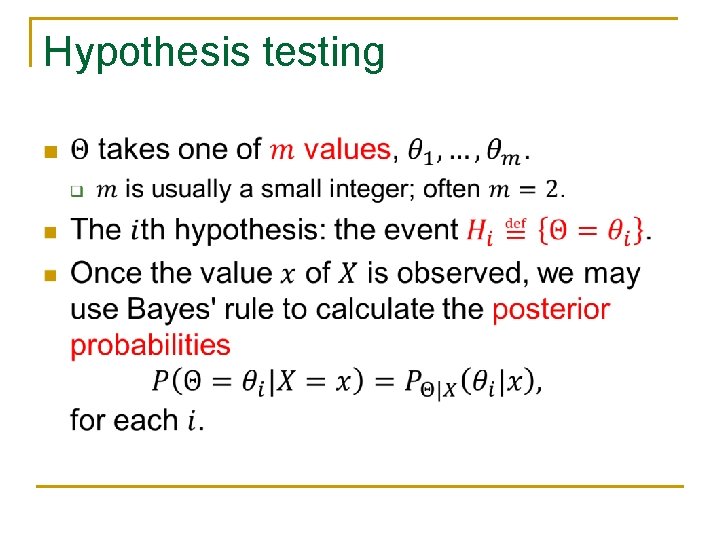
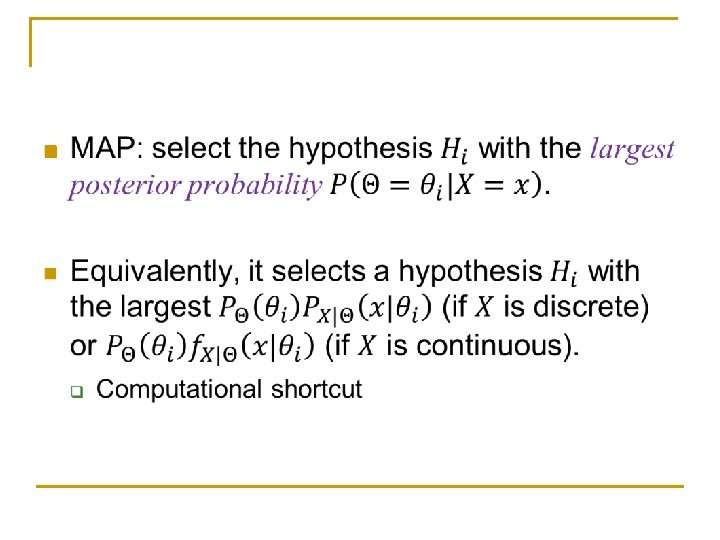
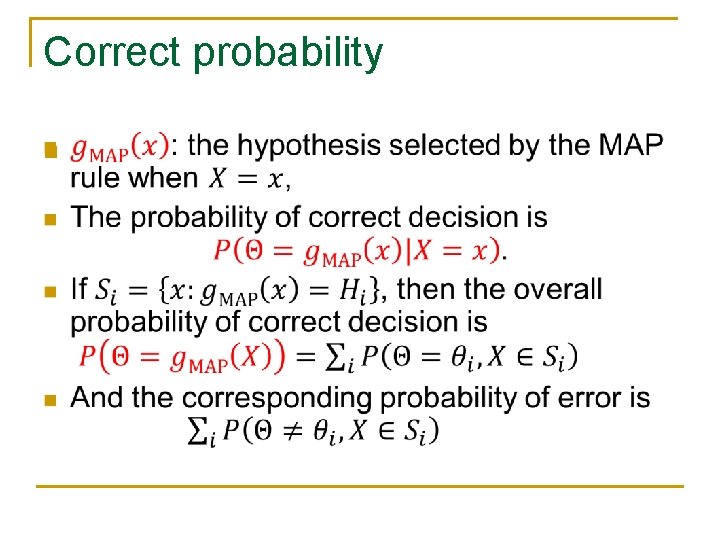
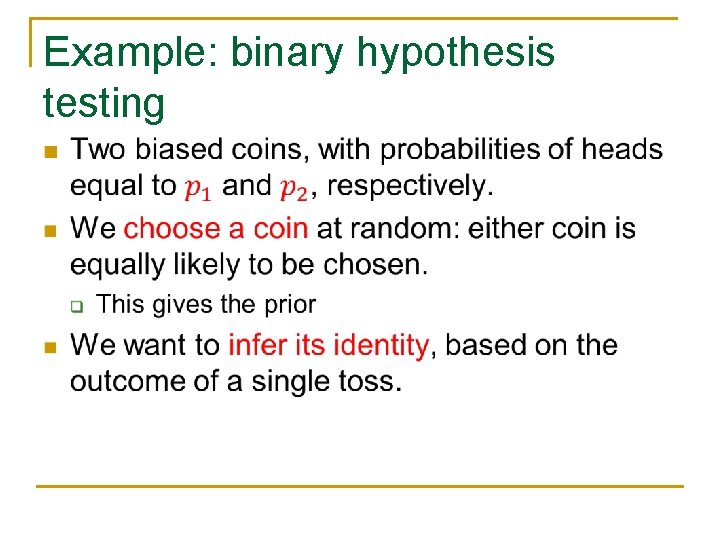
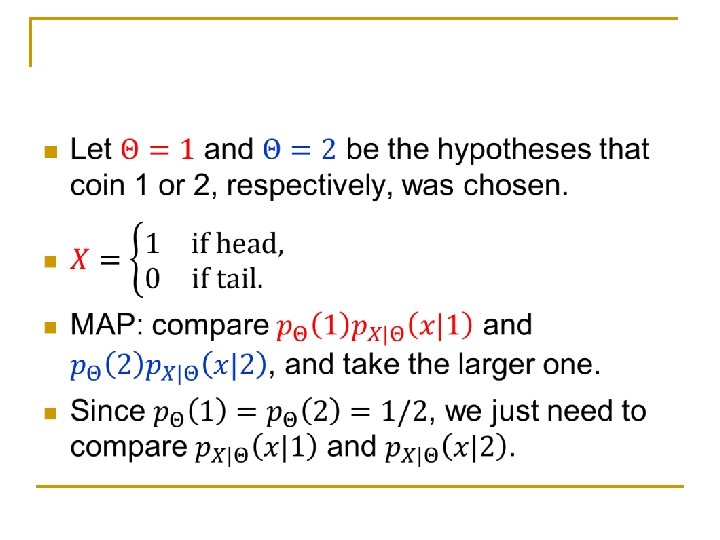
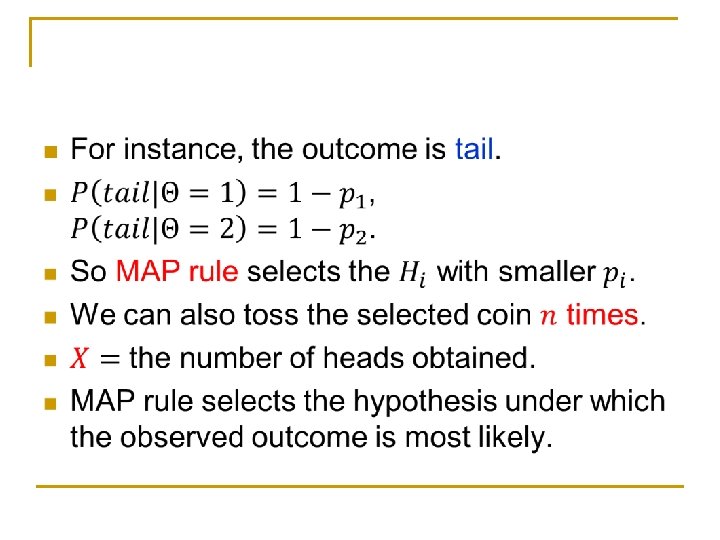
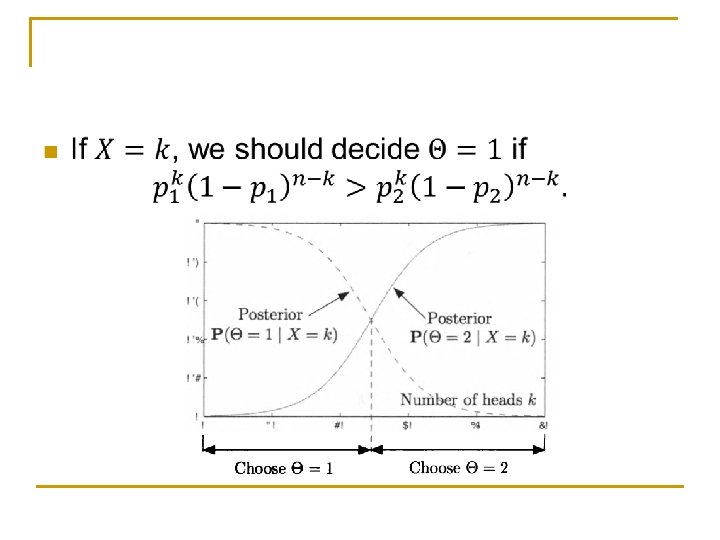
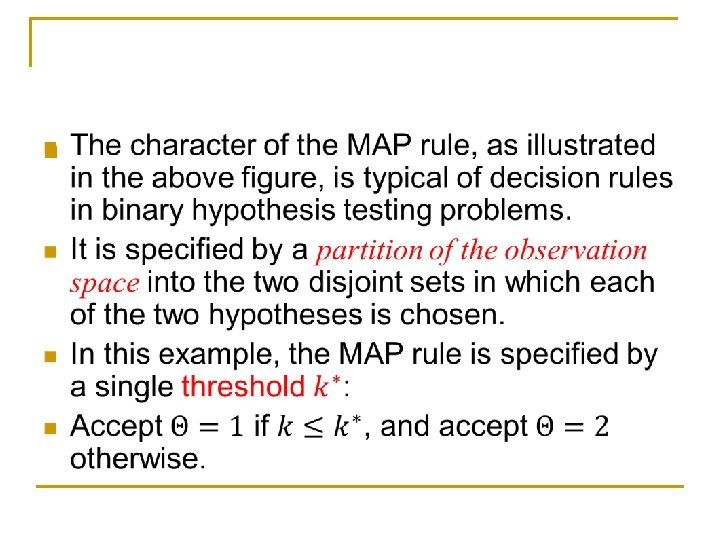
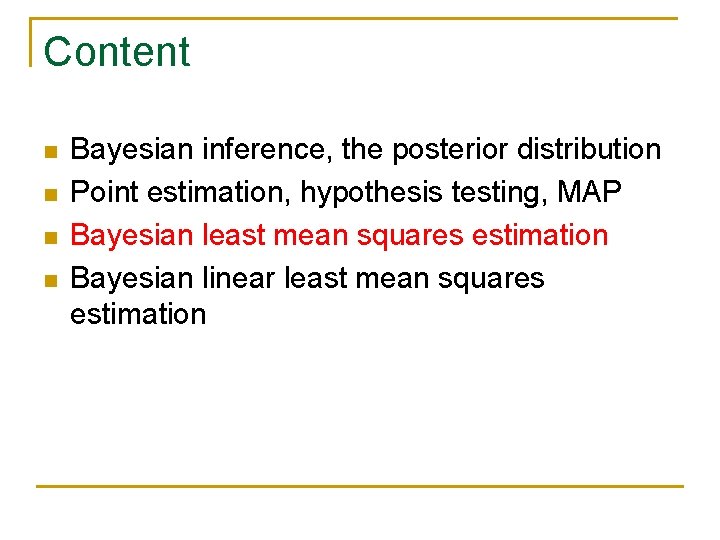
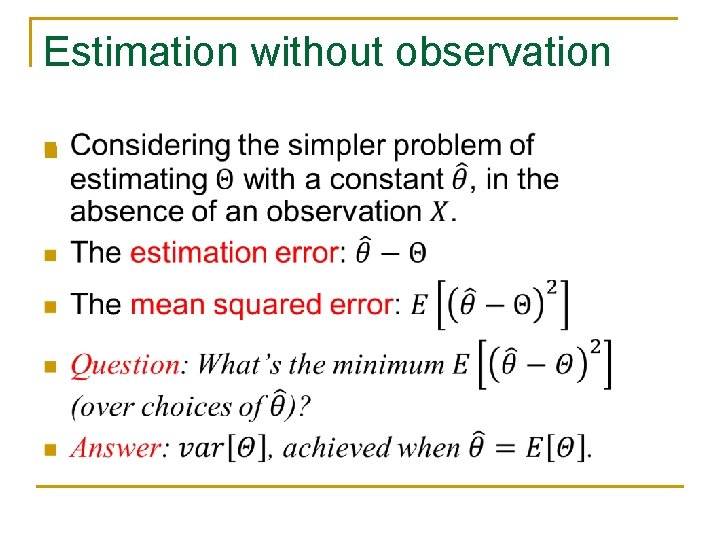
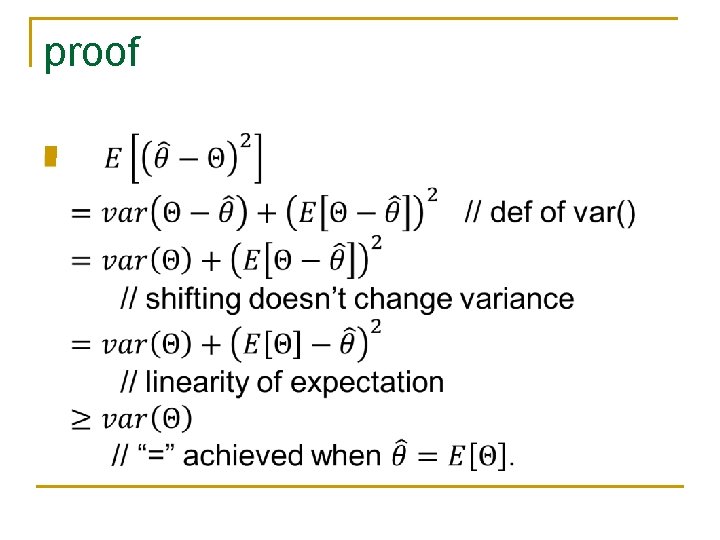
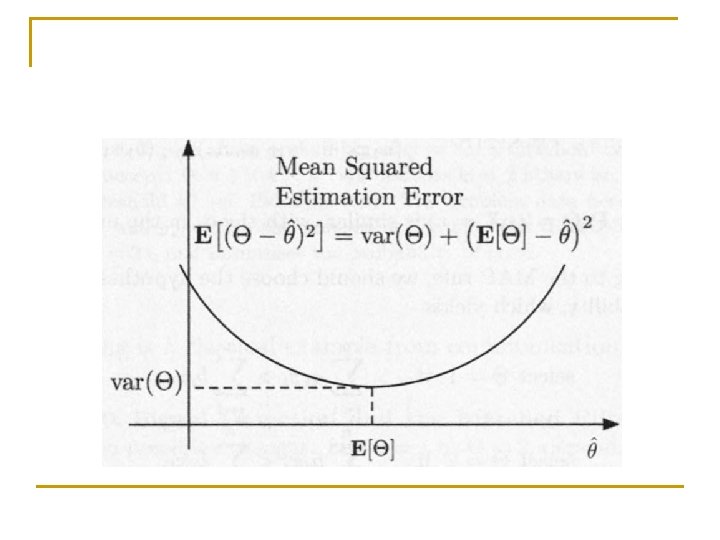
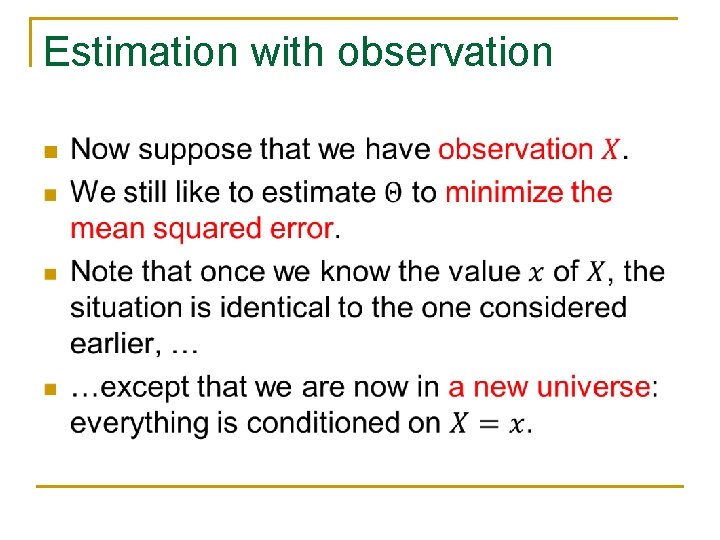
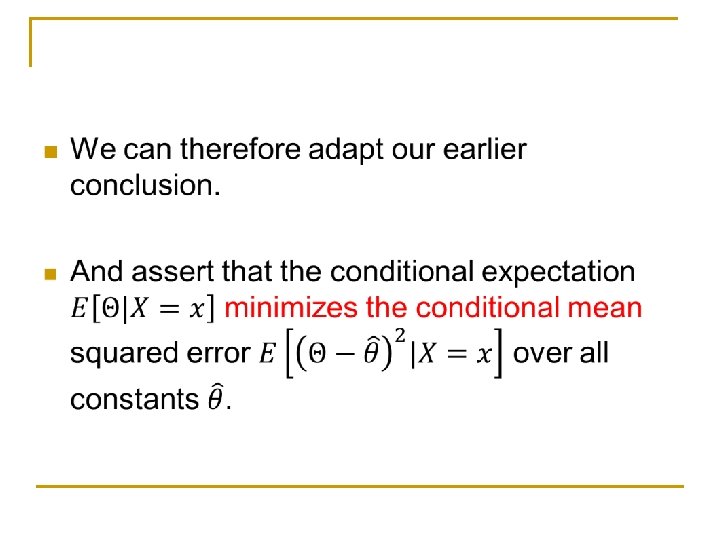
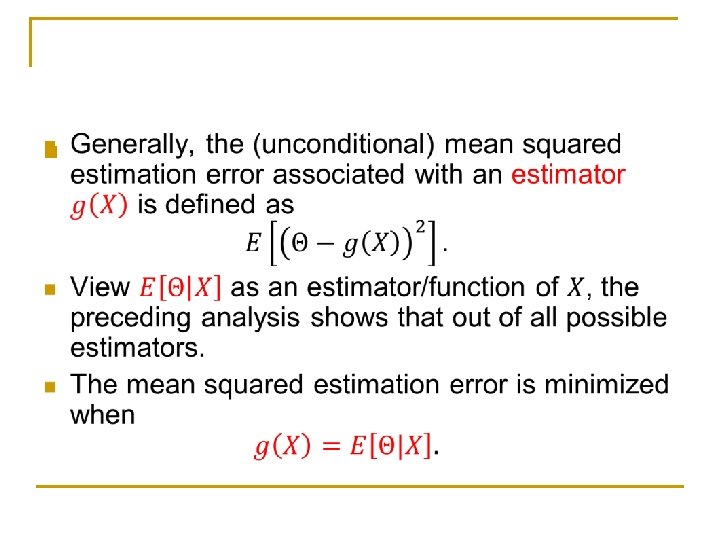
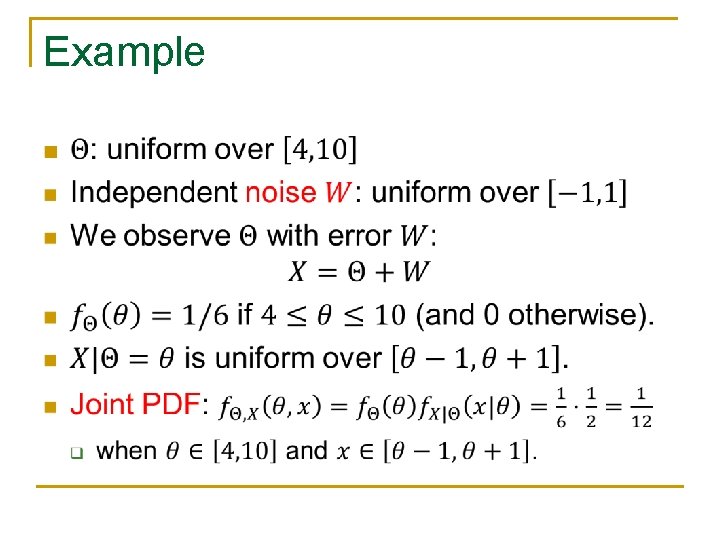
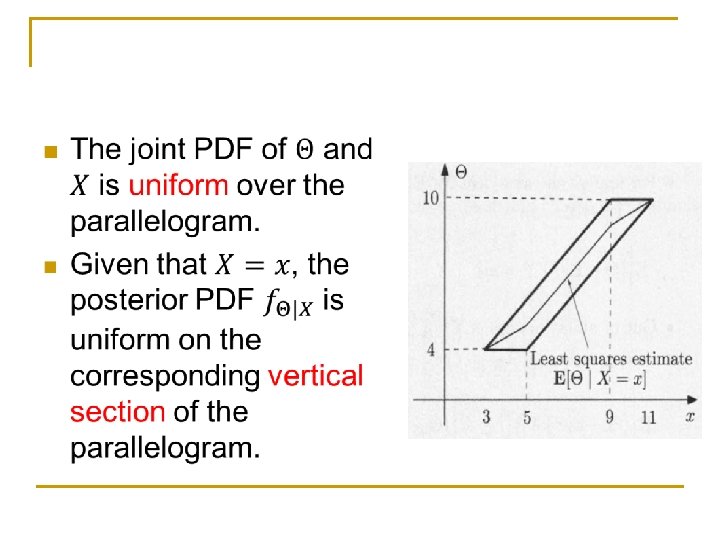
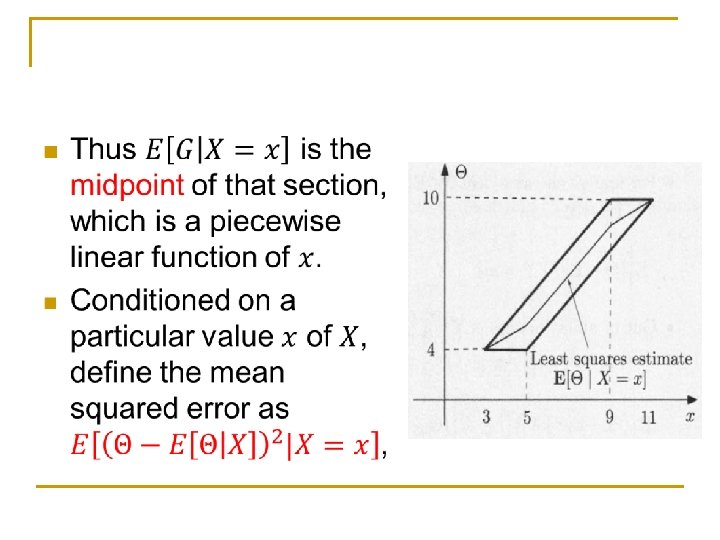
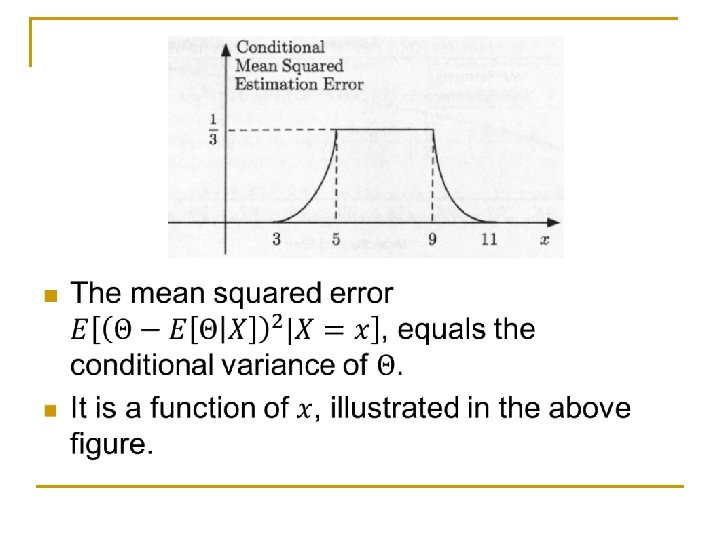
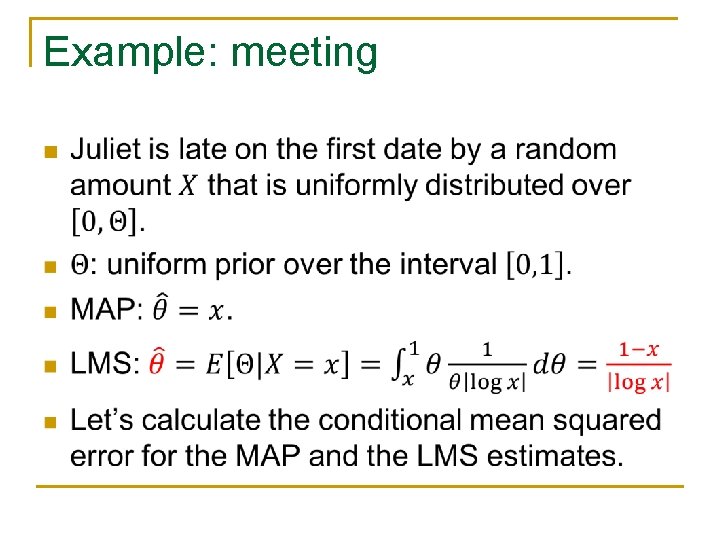
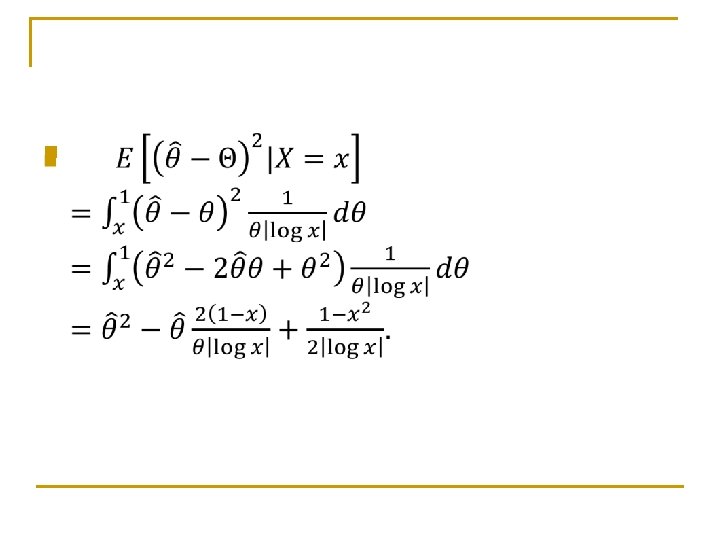
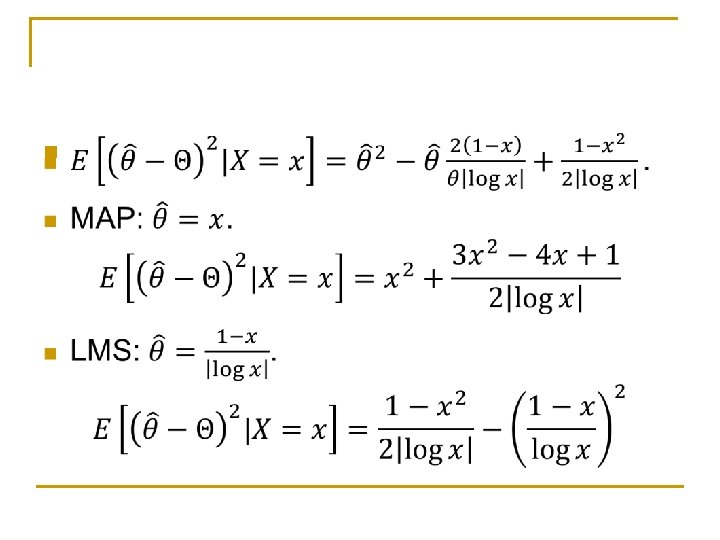
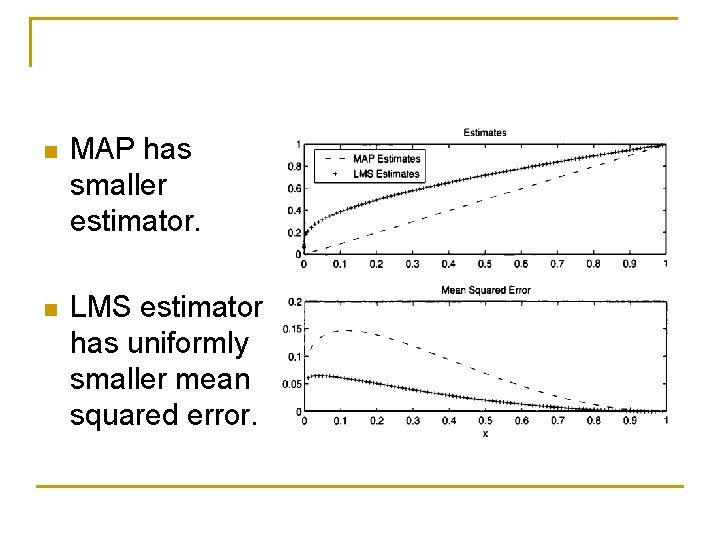
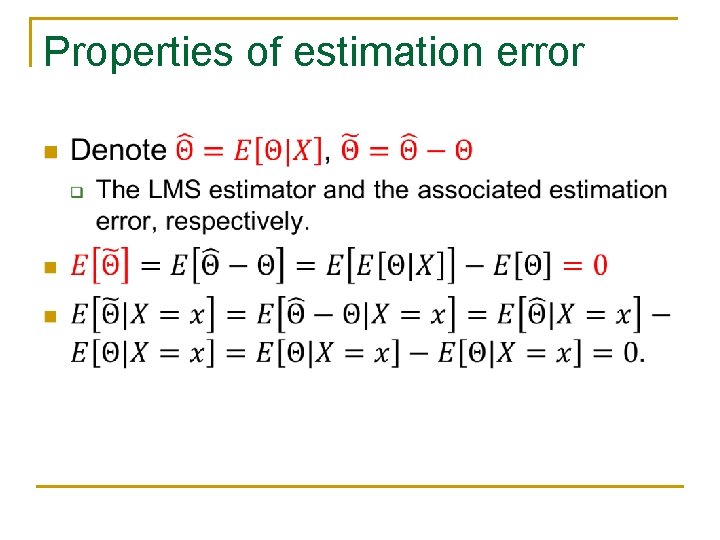
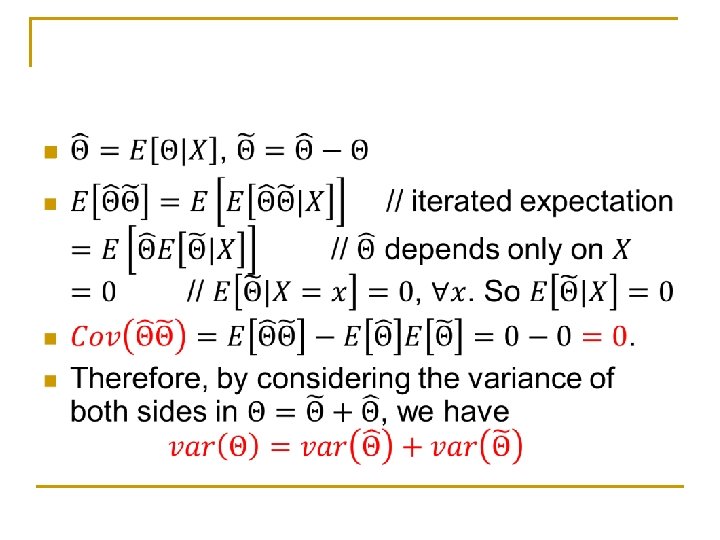
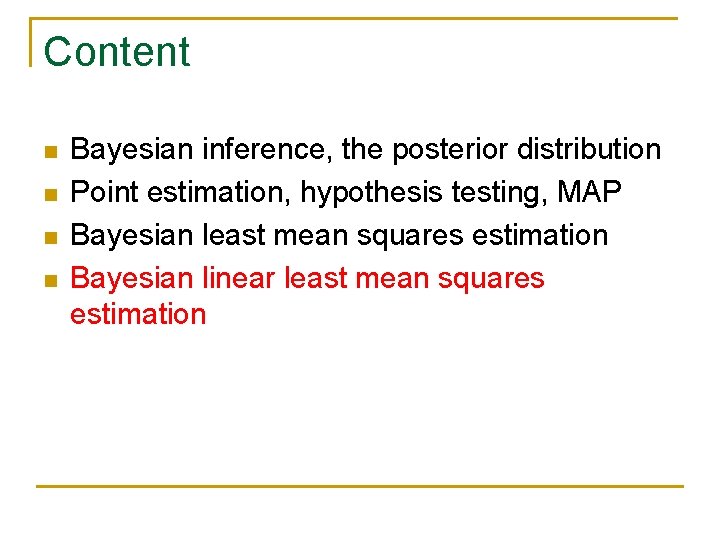
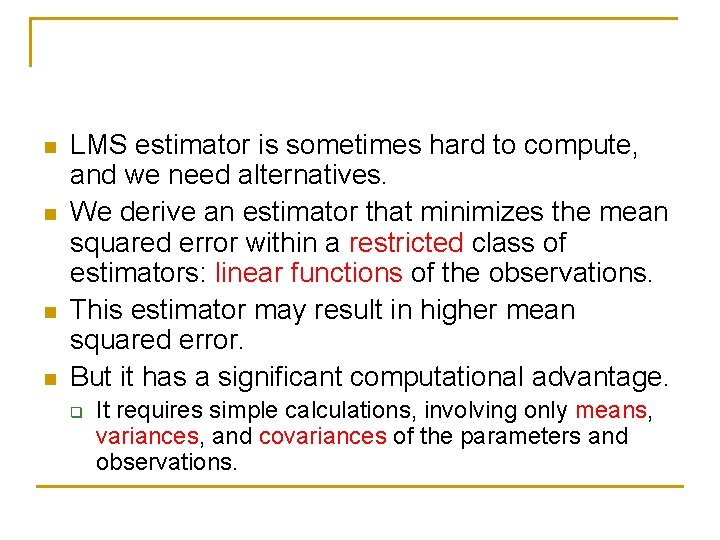
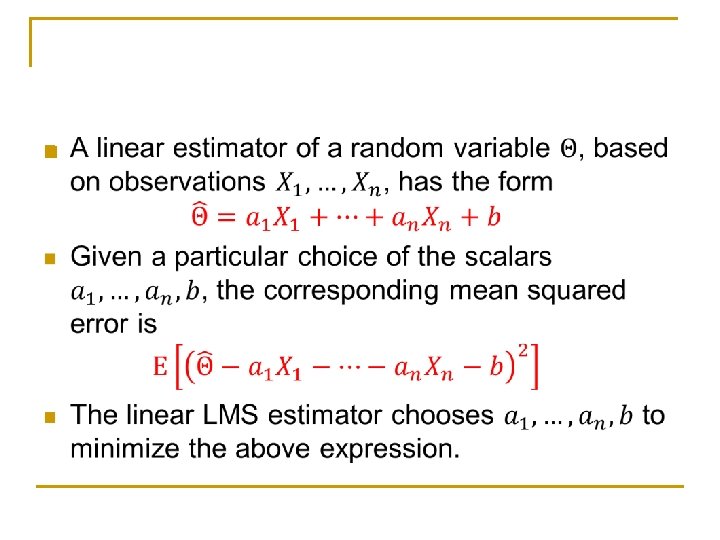
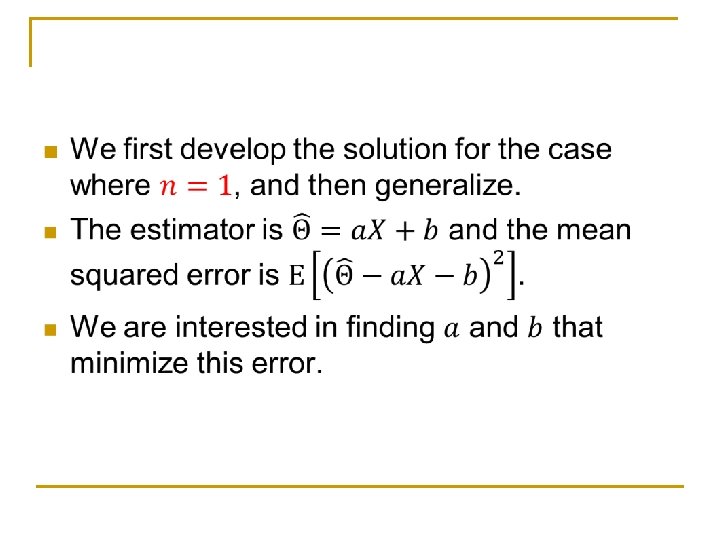
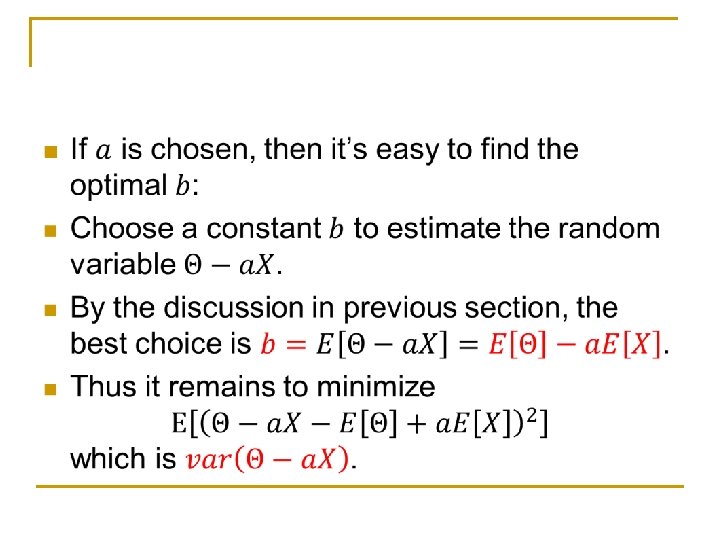
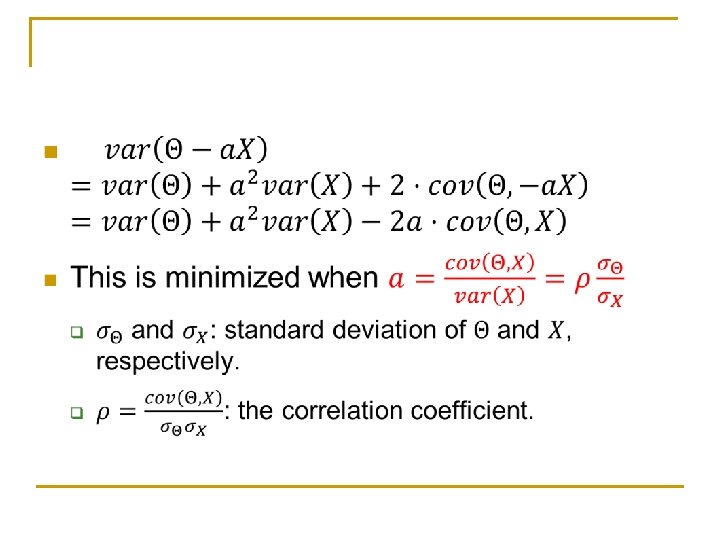
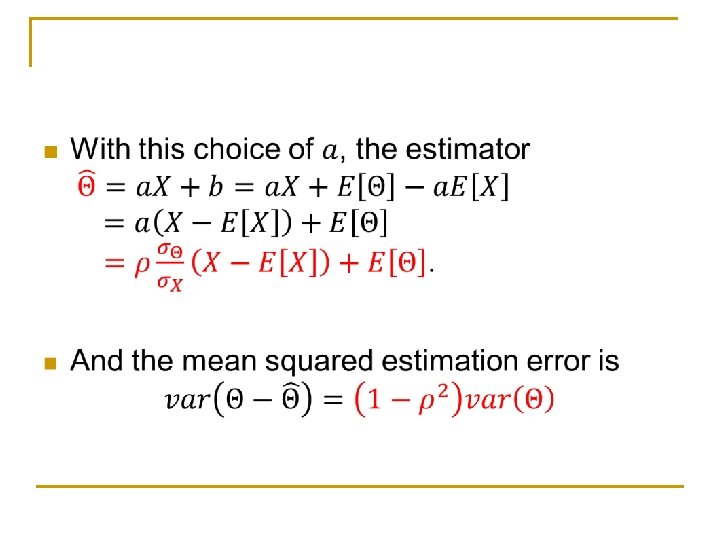
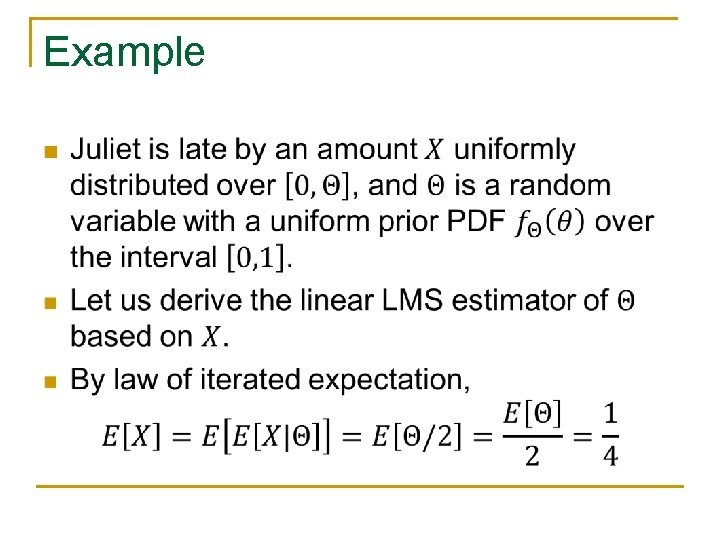
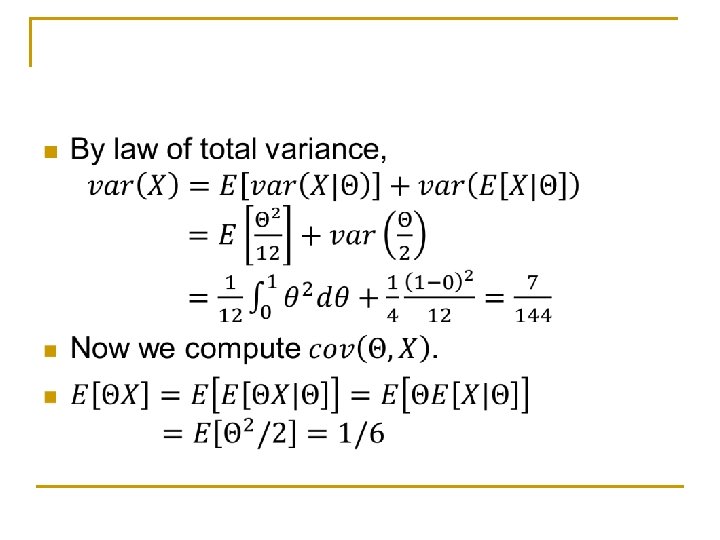
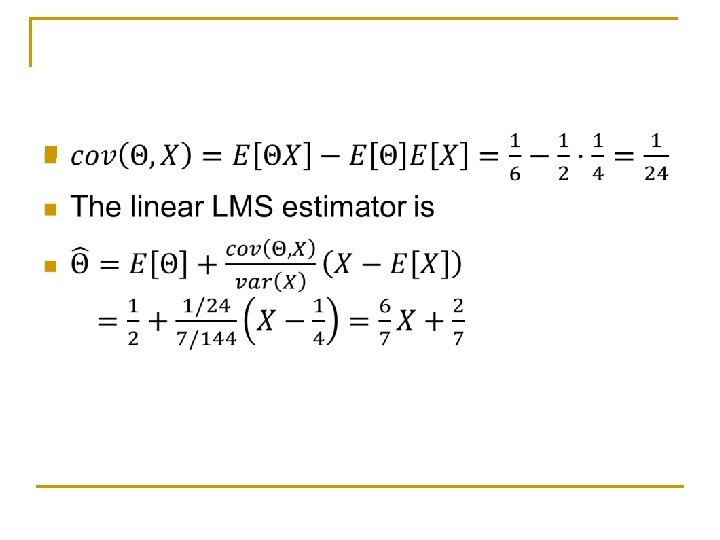
- Slides: 72
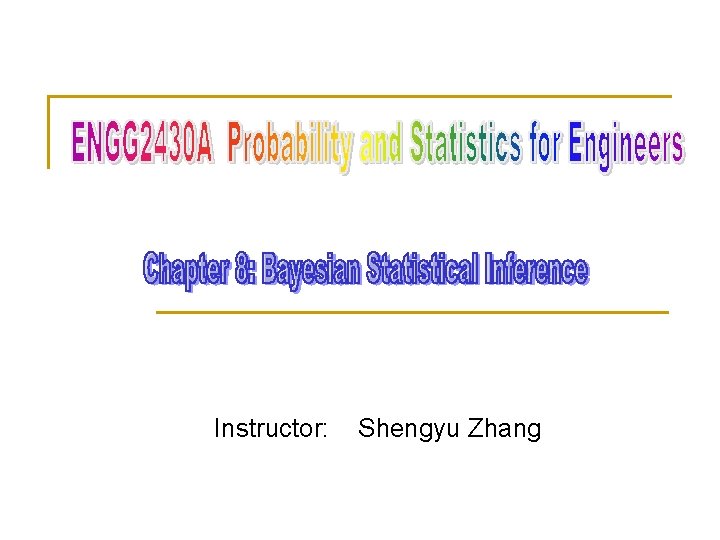
Instructor: Shengyu Zhang
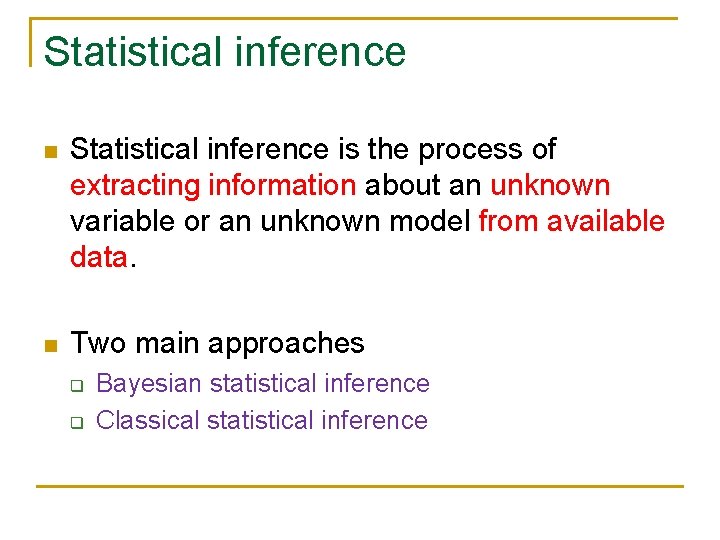
Statistical inference n Statistical inference is the process of extracting information about an unknown variable or an unknown model from available data. n Two main approaches q q Bayesian statistical inference Classical statistical inference
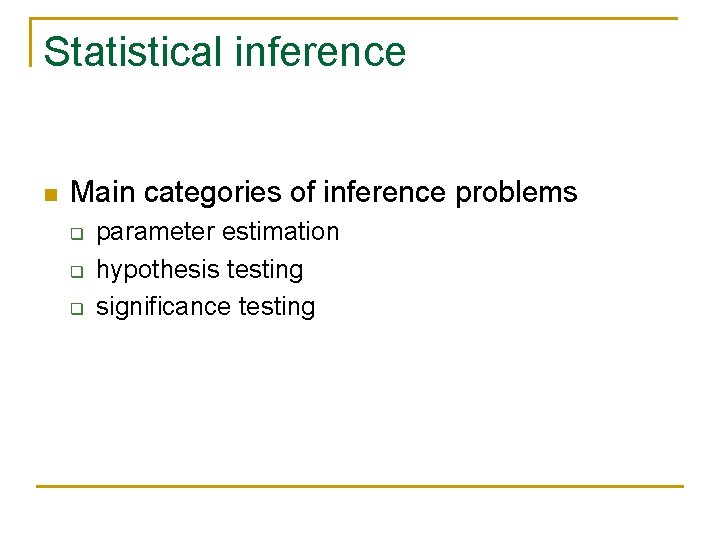
Statistical inference n Main categories of inference problems q q q parameter estimation hypothesis testing significance testing
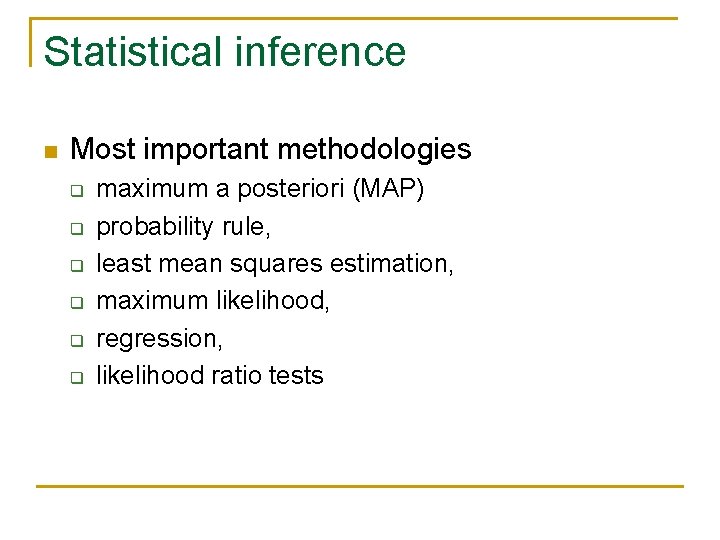
Statistical inference n Most important methodologies q q q maximum a posteriori (MAP) probability rule, least mean squares estimation, maximum likelihood, regression, likelihood ratio tests
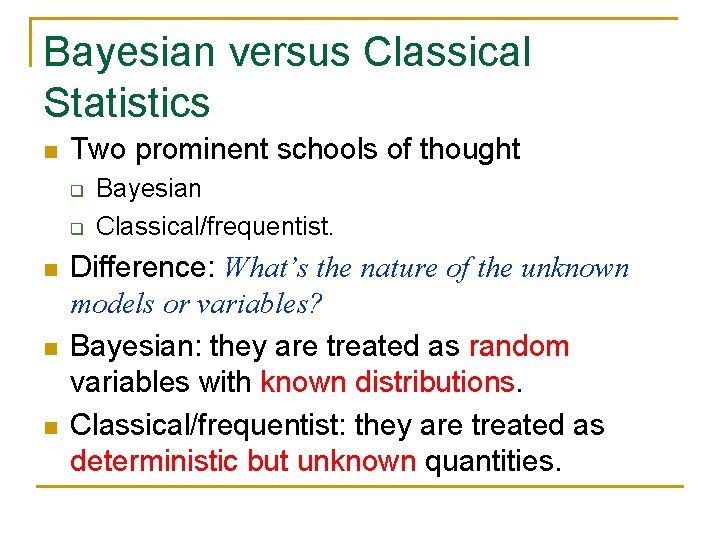
Bayesian versus Classical Statistics n Two prominent schools of thought q q n n n Bayesian Classical/frequentist. Difference: What’s the nature of the unknown models or variables? Bayesian: they are treated as random variables with known distributions. Classical/frequentist: they are treated as deterministic but unknown quantities.
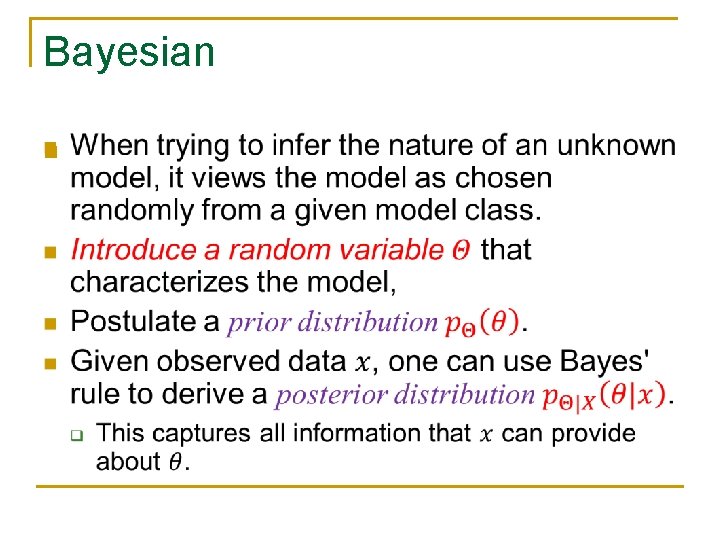
Bayesian n
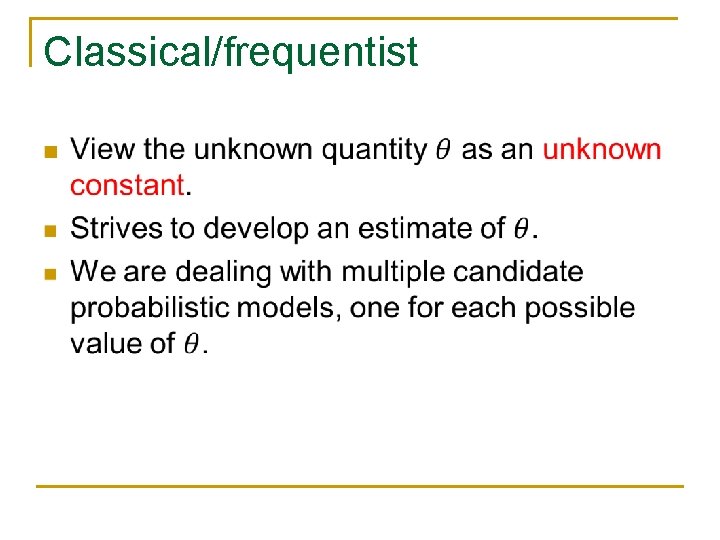
Classical/frequentist n
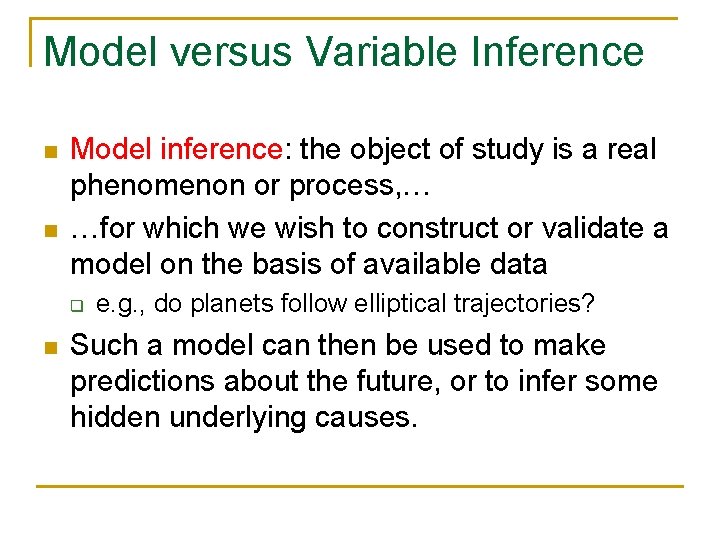
Model versus Variable Inference n n Model inference: the object of study is a real phenomenon or process, … …for which we wish to construct or validate a model on the basis of available data q n e. g. , do planets follow elliptical trajectories? Such a model can then be used to make predictions about the future, or to infer some hidden underlying causes.
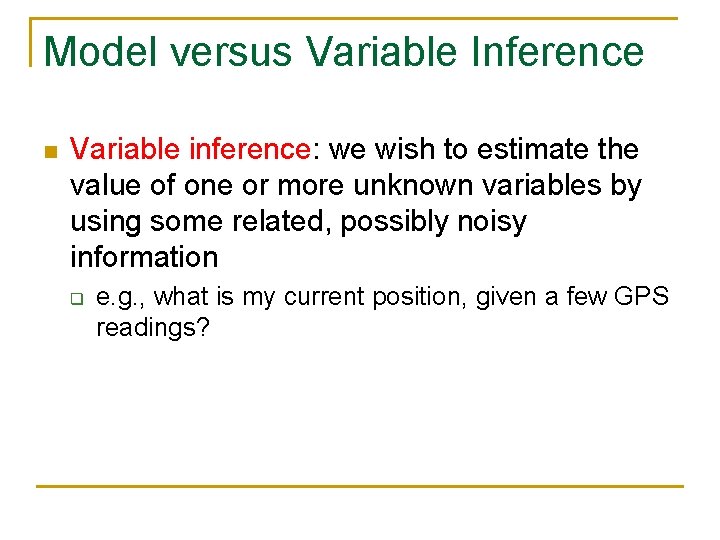
Model versus Variable Inference n Variable inference: we wish to estimate the value of one or more unknown variables by using some related, possibly noisy information q e. g. , what is my current position, given a few GPS readings?
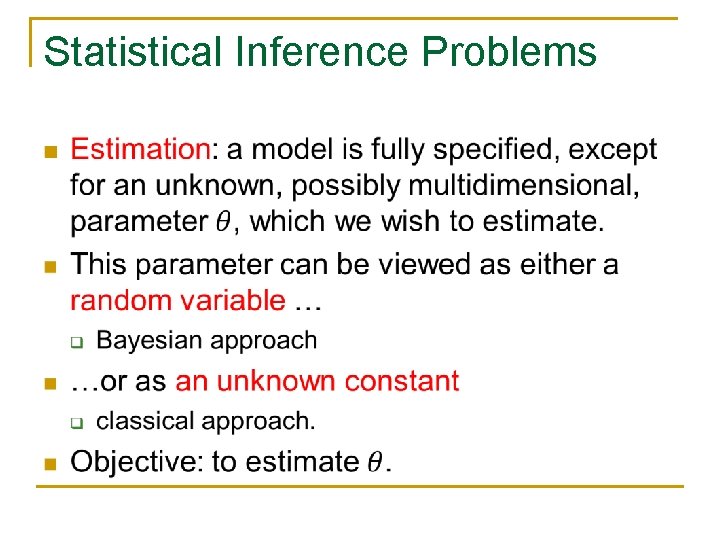
Statistical Inference Problems n
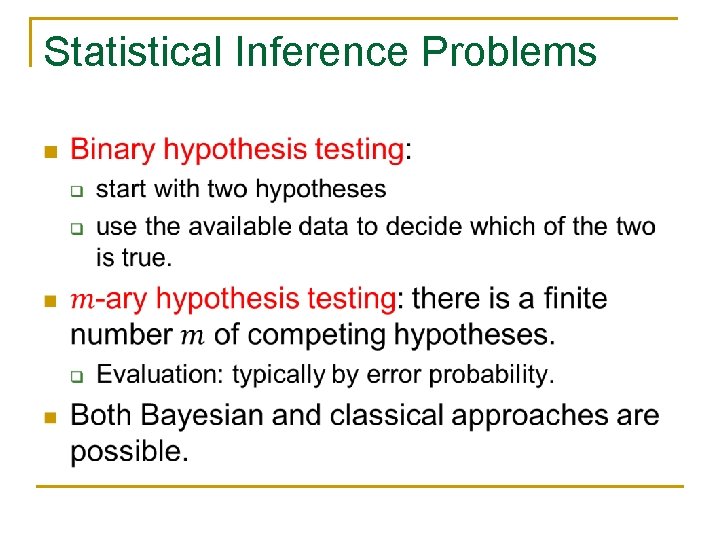
Statistical Inference Problems n
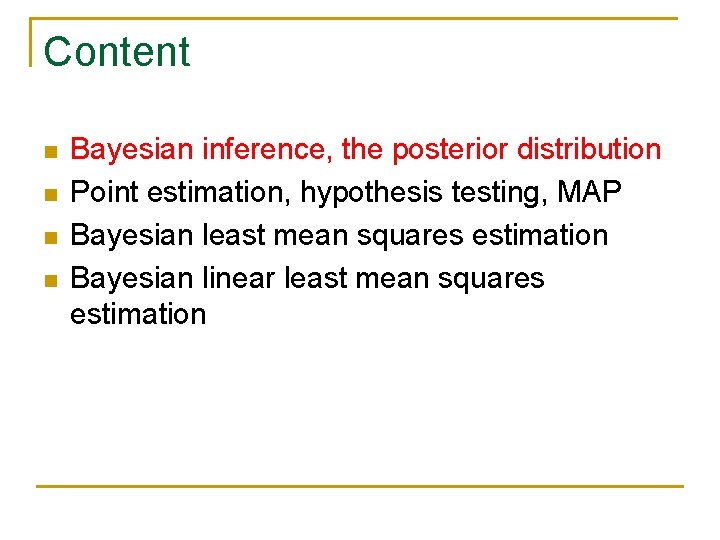
Content n n Bayesian inference, the posterior distribution Point estimation, hypothesis testing, MAP Bayesian least mean squares estimation Bayesian linear least mean squares estimation
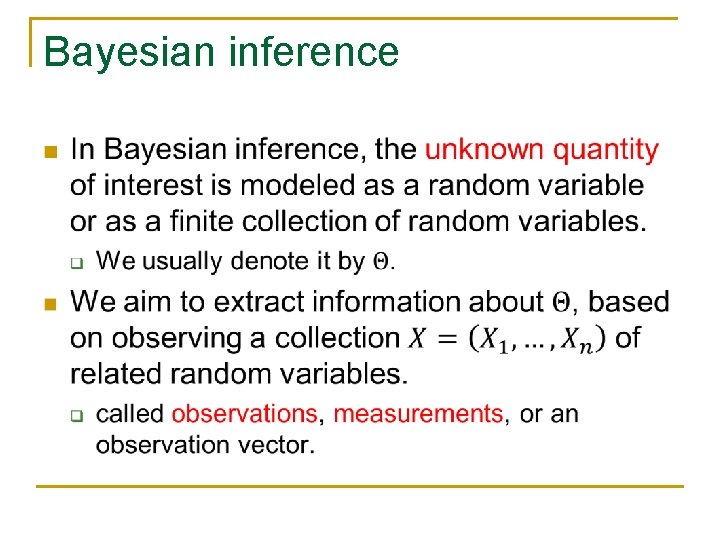
Bayesian inference n
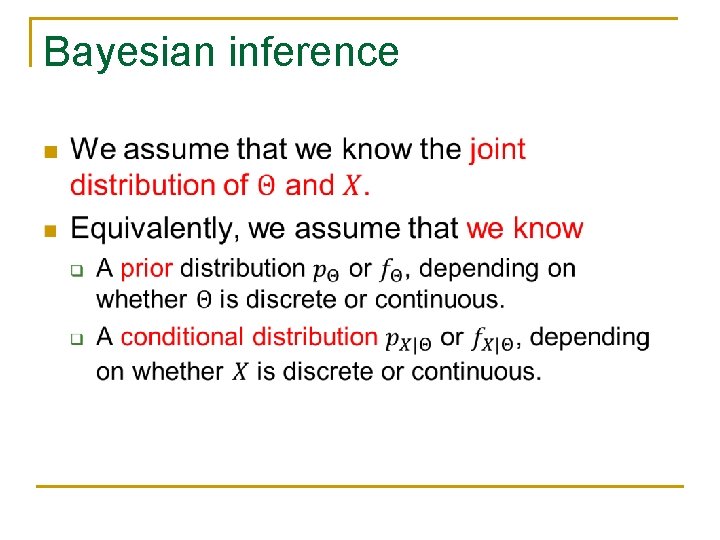
Bayesian inference n
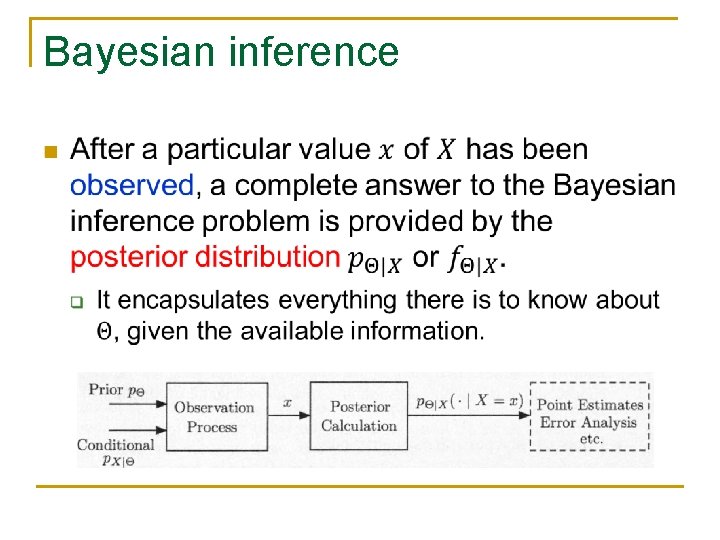
Bayesian inference n
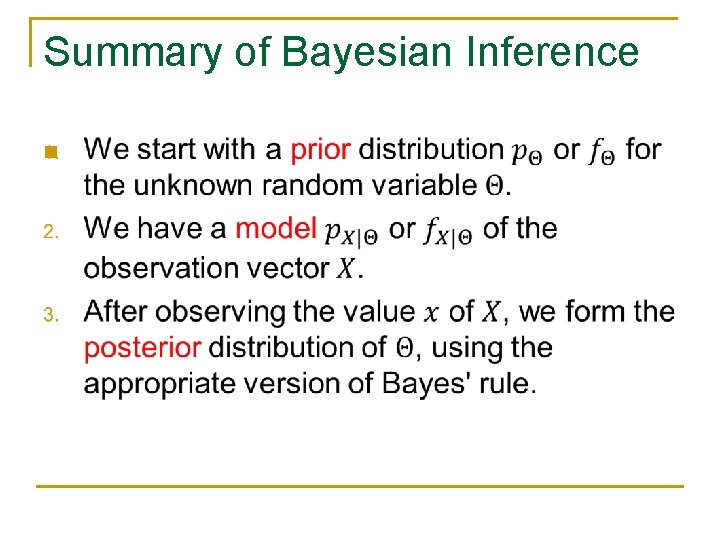
Summary of Bayesian Inference n
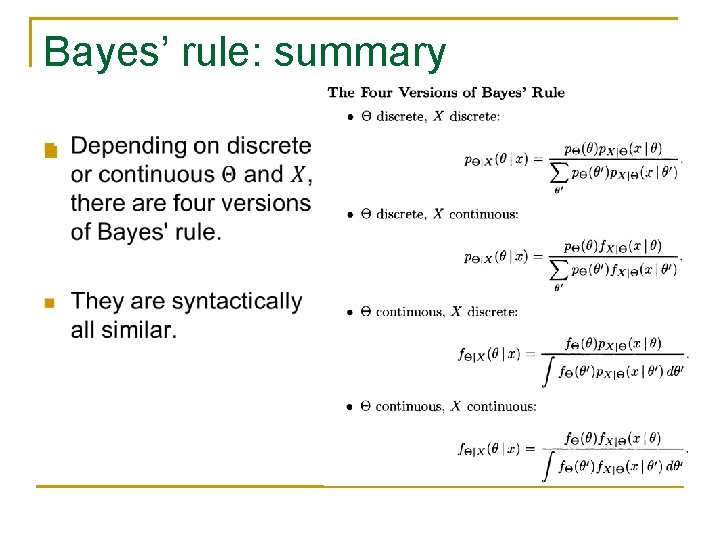
Bayes’ rule: summary n
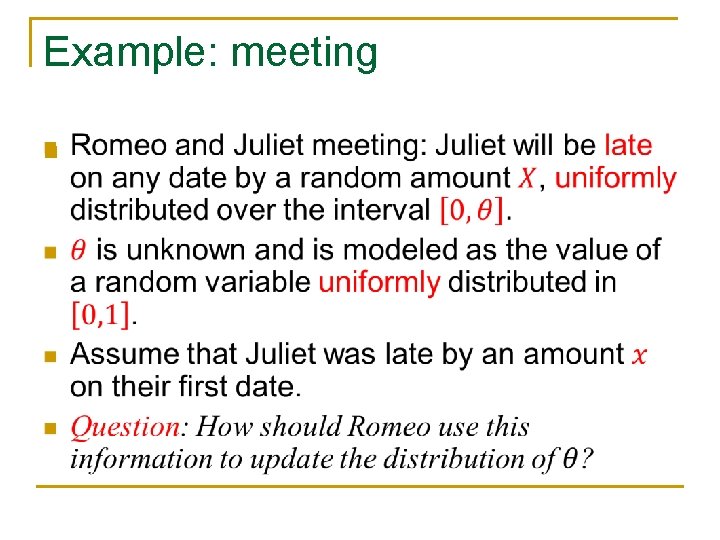
Example: meeting n
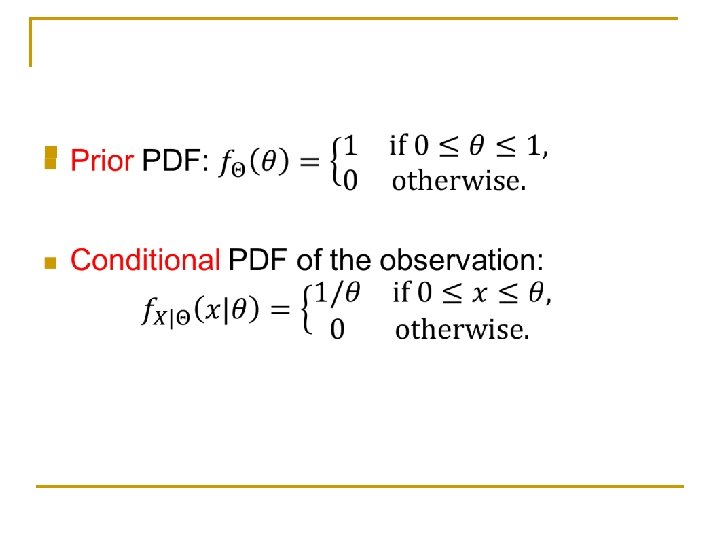
n
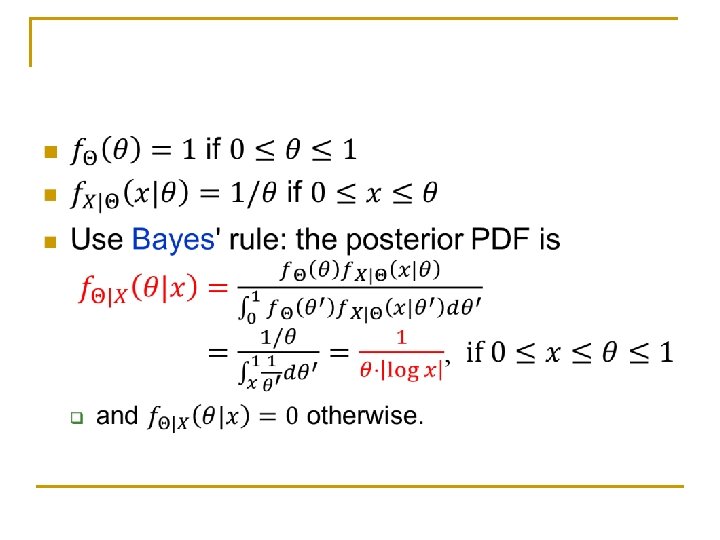
n
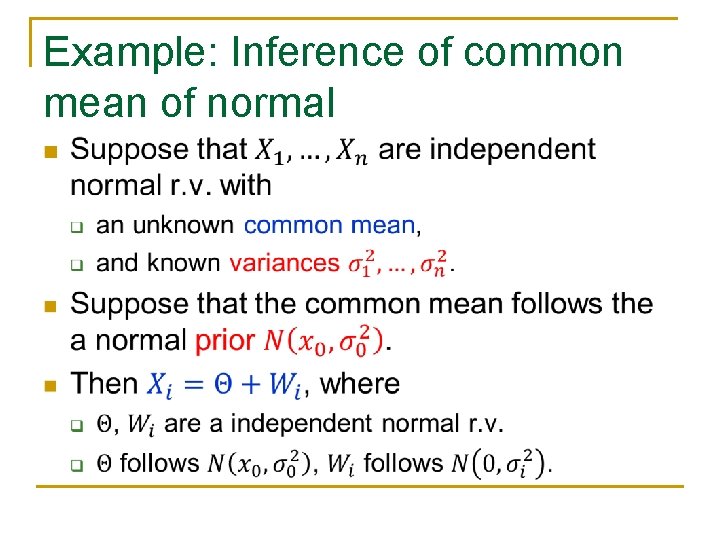
Example: Inference of common mean of normal n
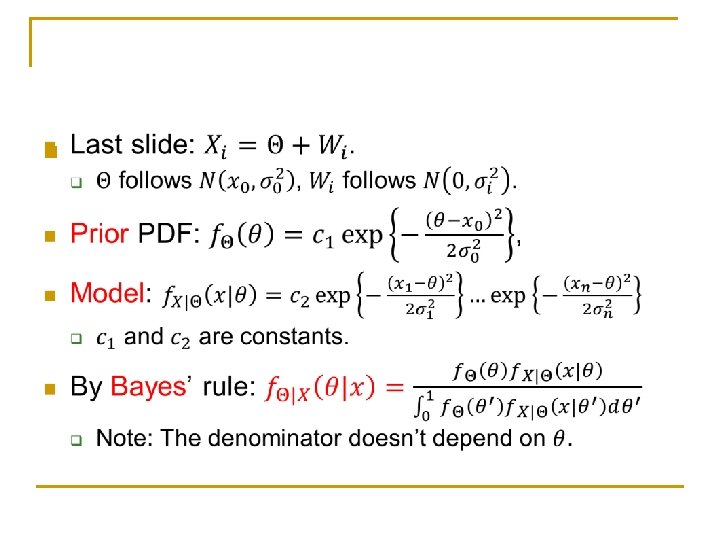
n
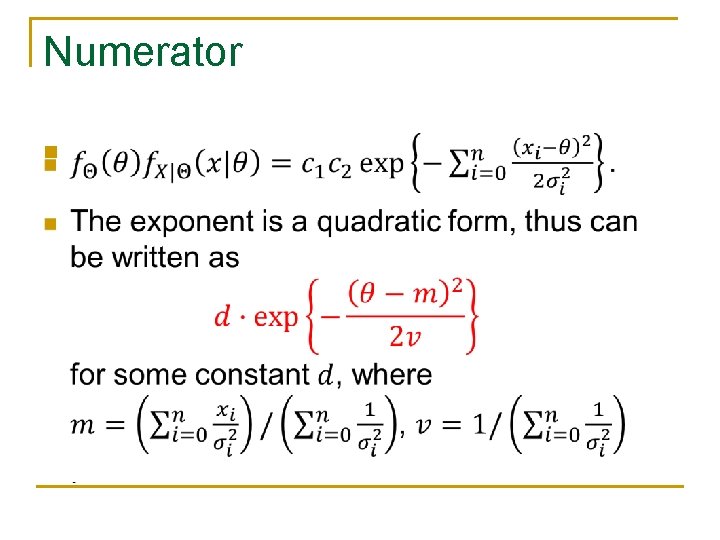
Numerator n
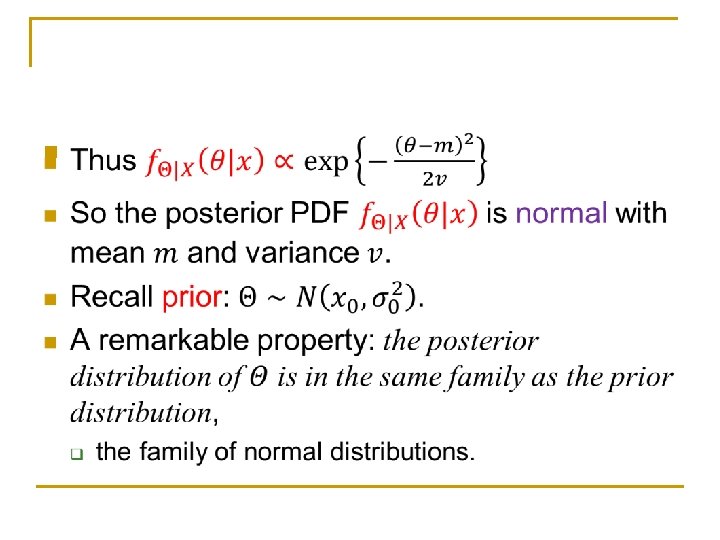
n
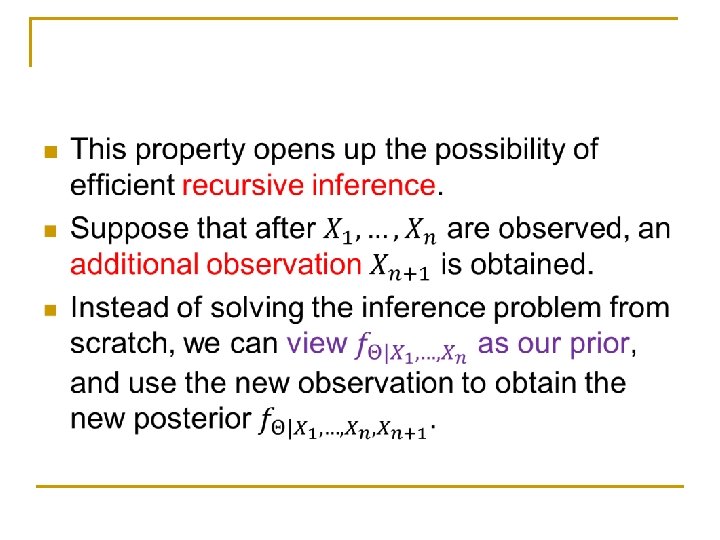
n
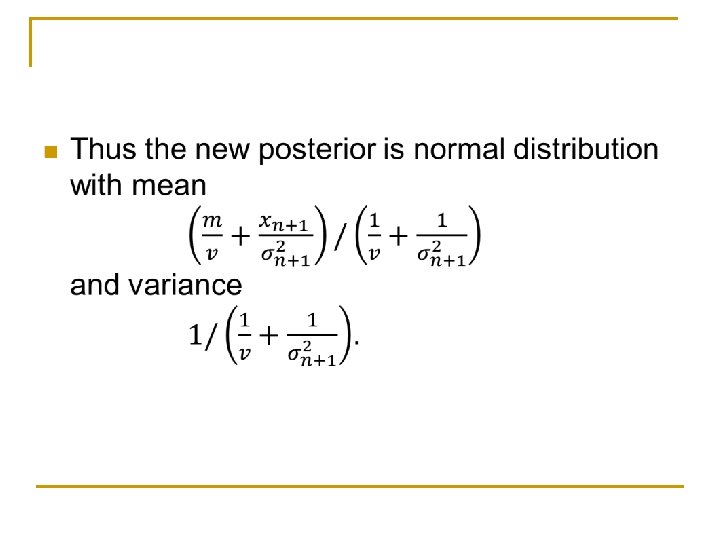
n
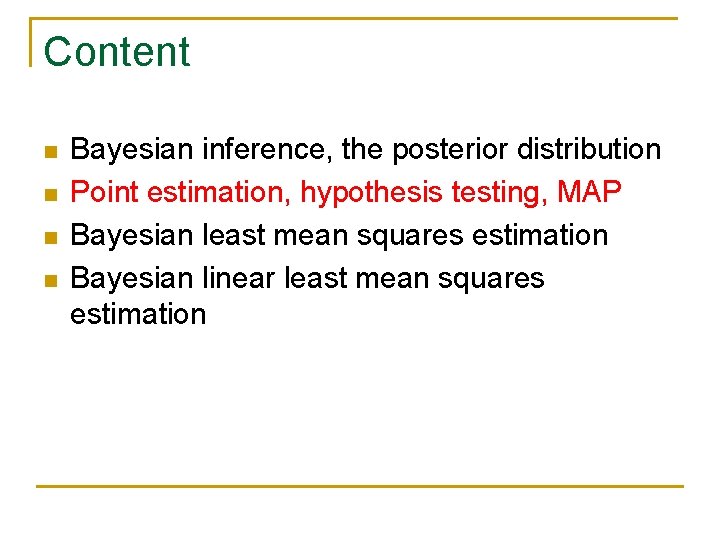
Content n n Bayesian inference, the posterior distribution Point estimation, hypothesis testing, MAP Bayesian least mean squares estimation Bayesian linear least mean squares estimation
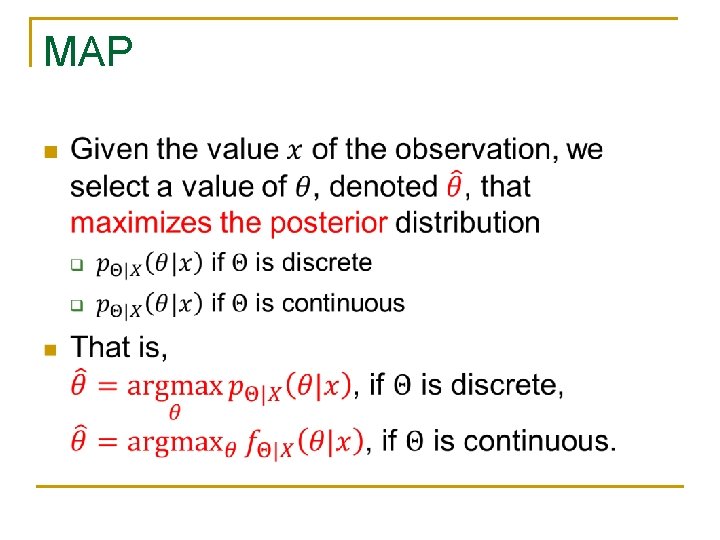
MAP n
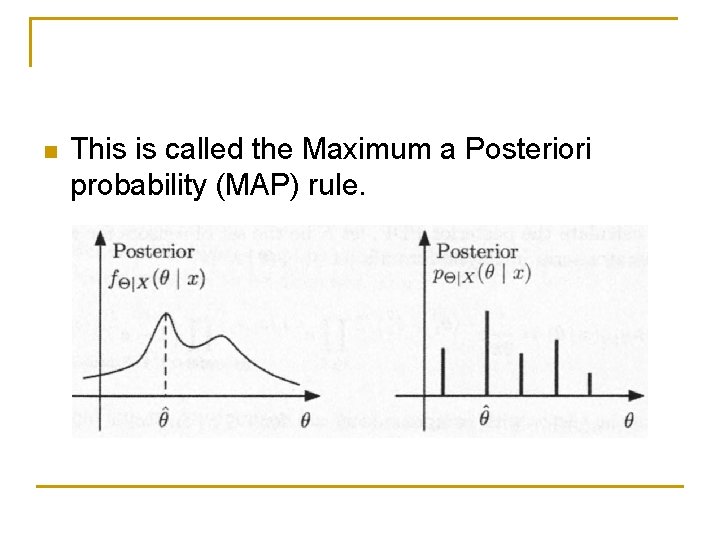
n This is called the Maximum a Posteriori probability (MAP) rule.
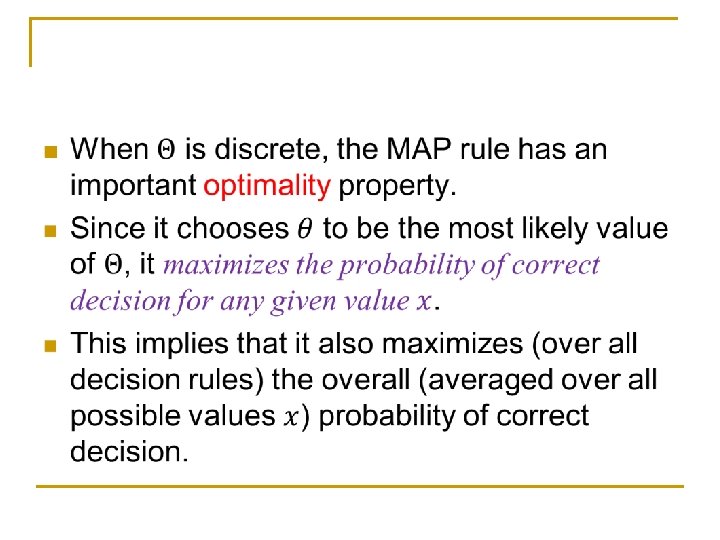
n
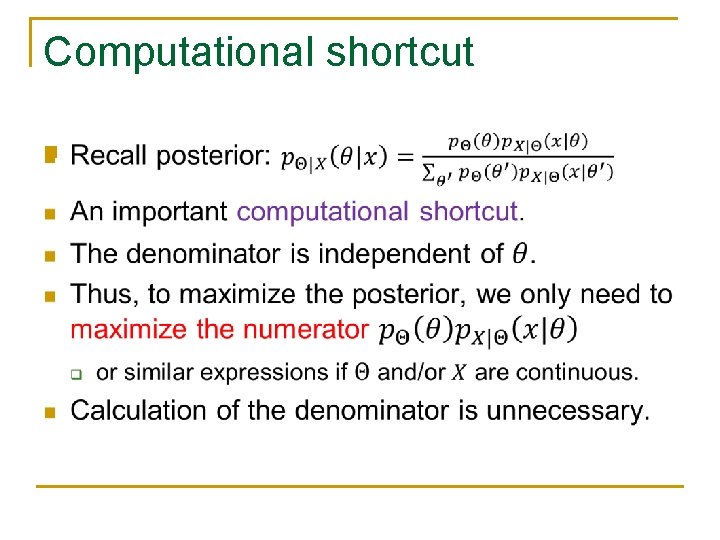
Computational shortcut n
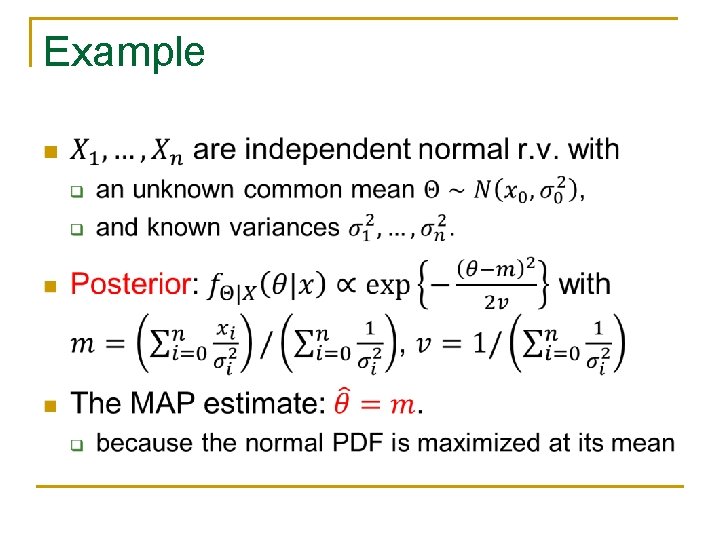
Example n
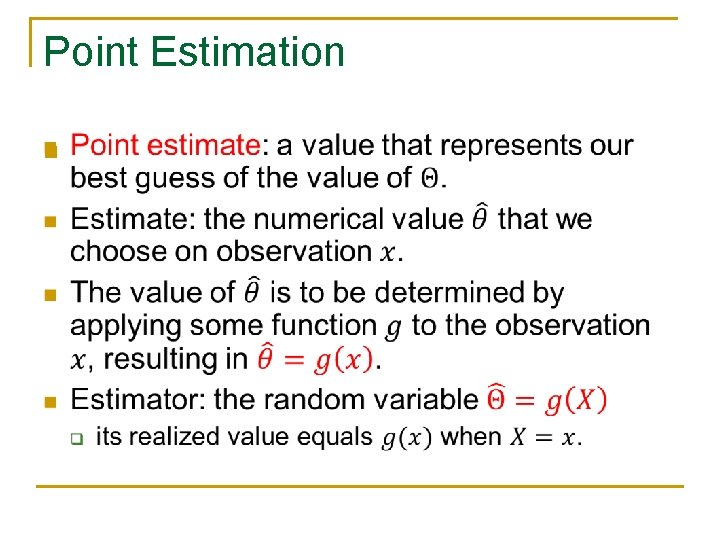
Point Estimation n
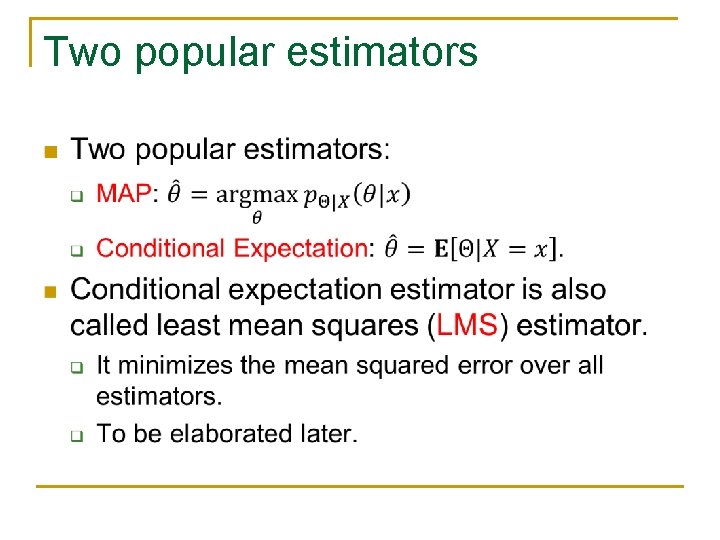
Two popular estimators n
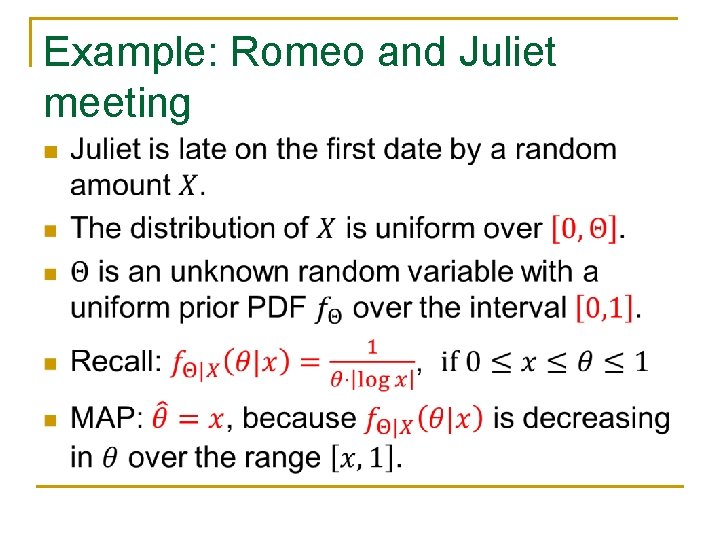
Example: Romeo and Juliet meeting n
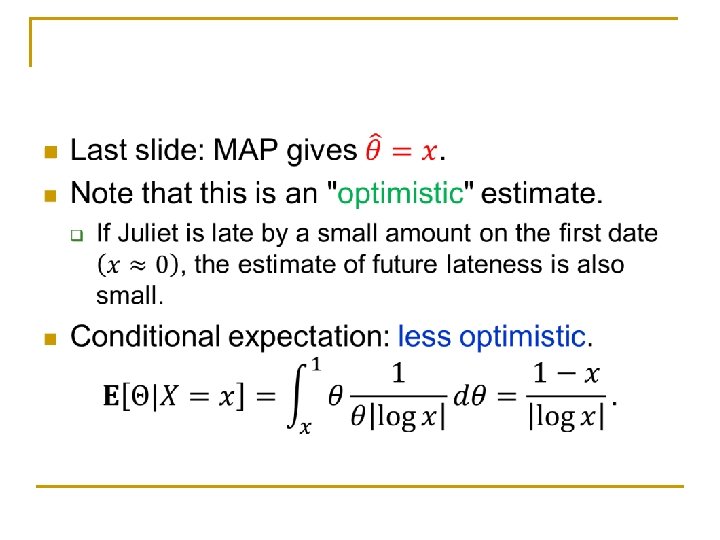
n
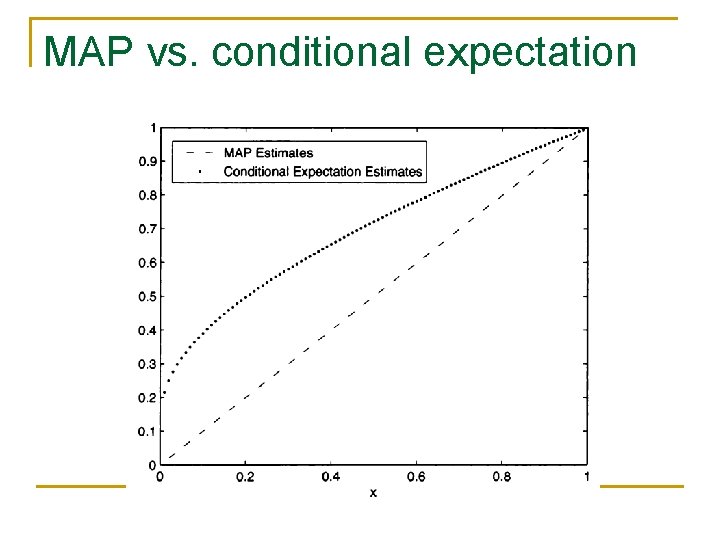
MAP vs. conditional expectation
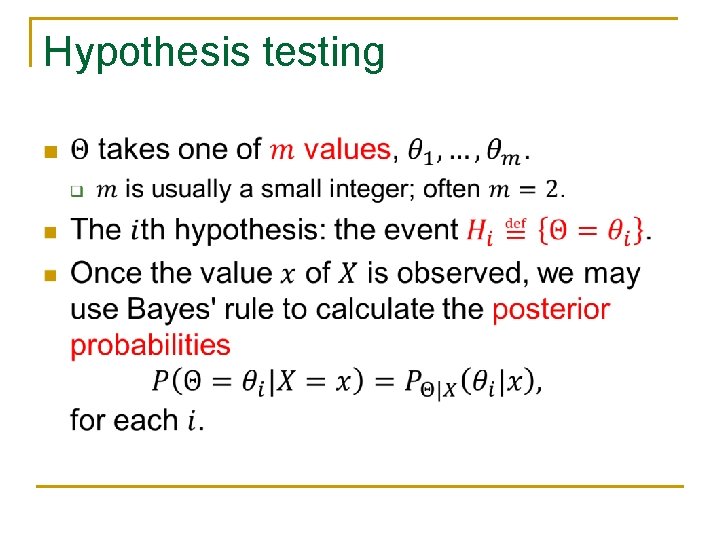
Hypothesis testing n
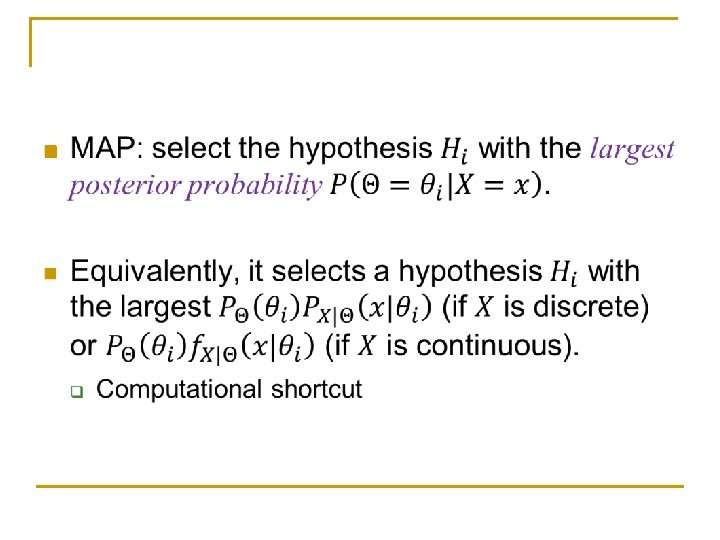
n
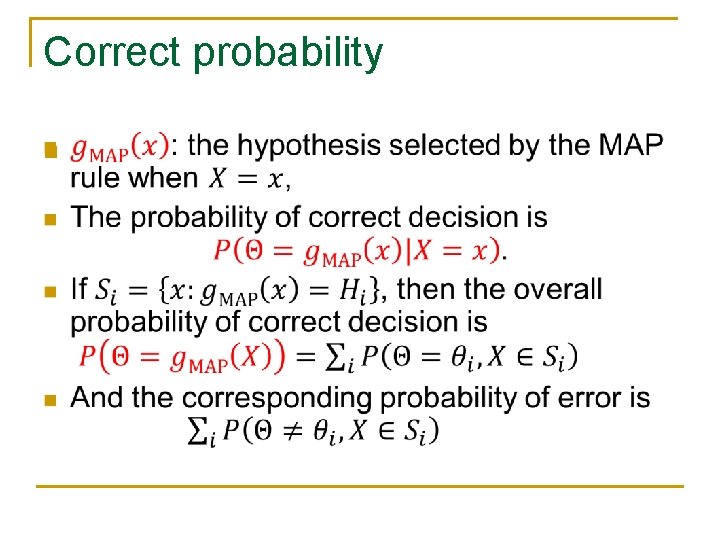
Correct probability n
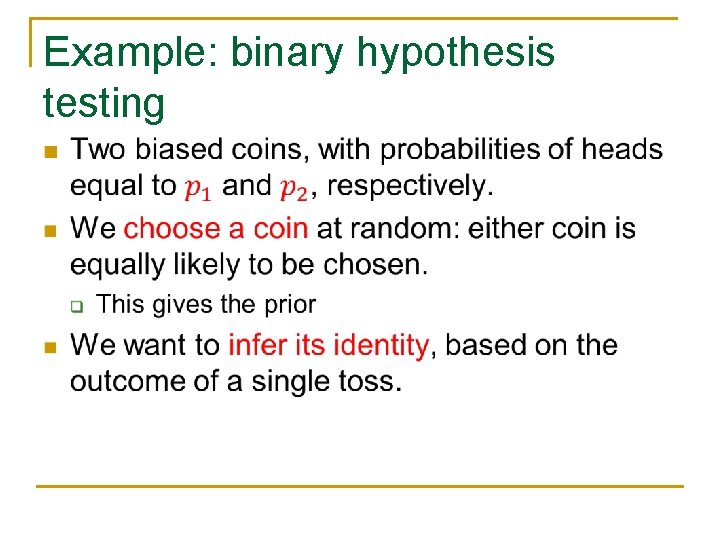
Example: binary hypothesis testing n
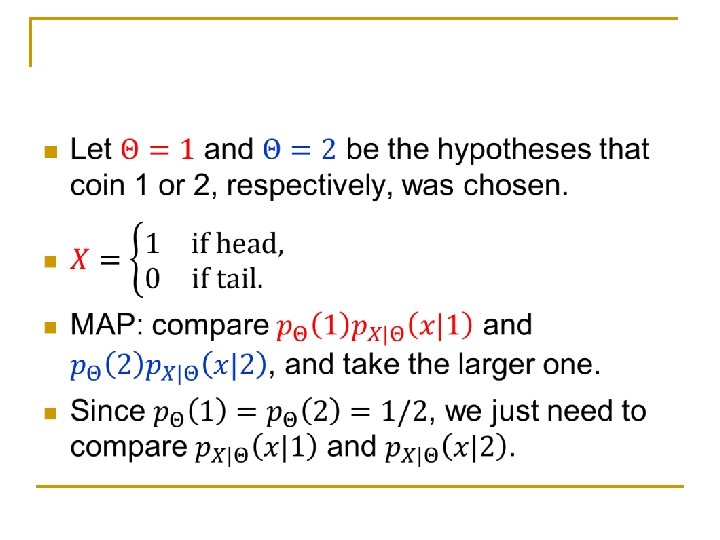
n
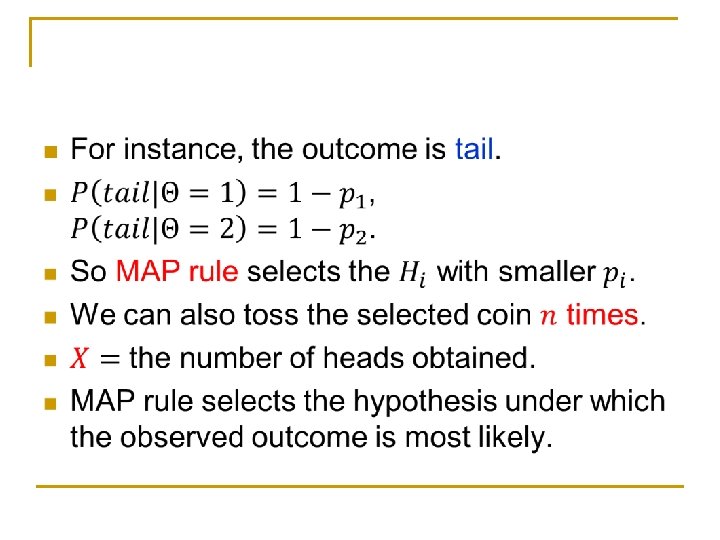
n
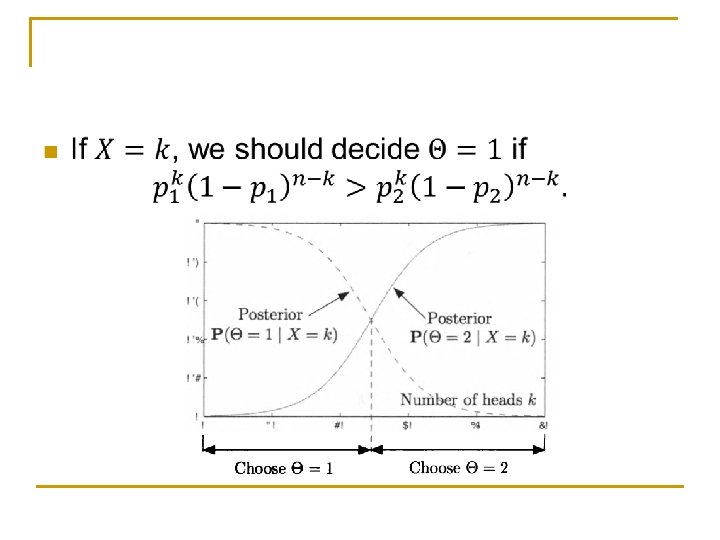
n
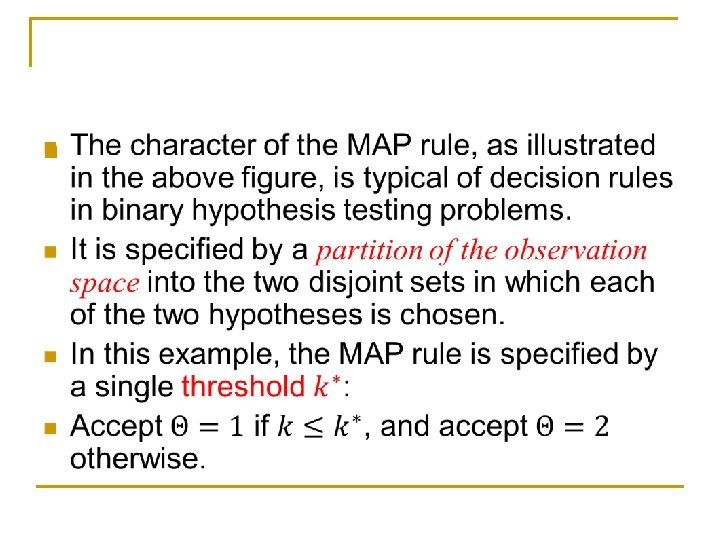
n
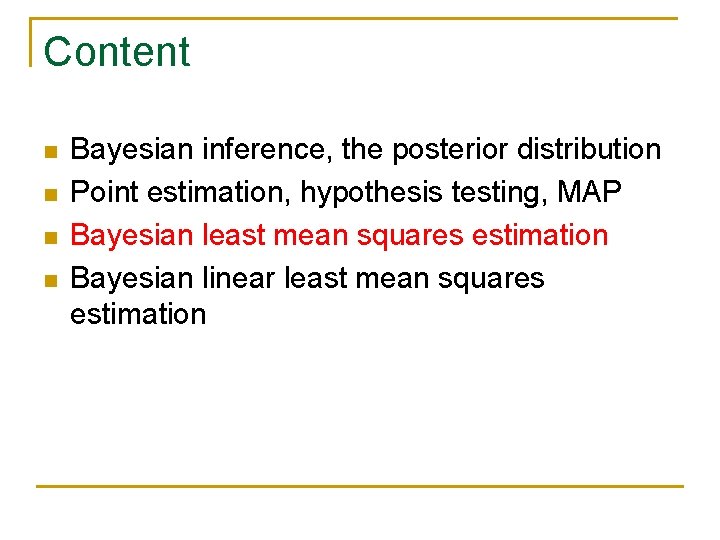
Content n n Bayesian inference, the posterior distribution Point estimation, hypothesis testing, MAP Bayesian least mean squares estimation Bayesian linear least mean squares estimation
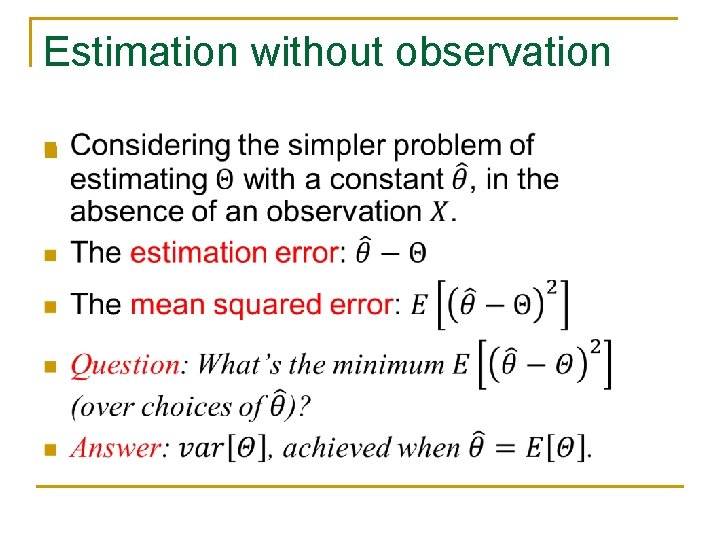
Estimation without observation n
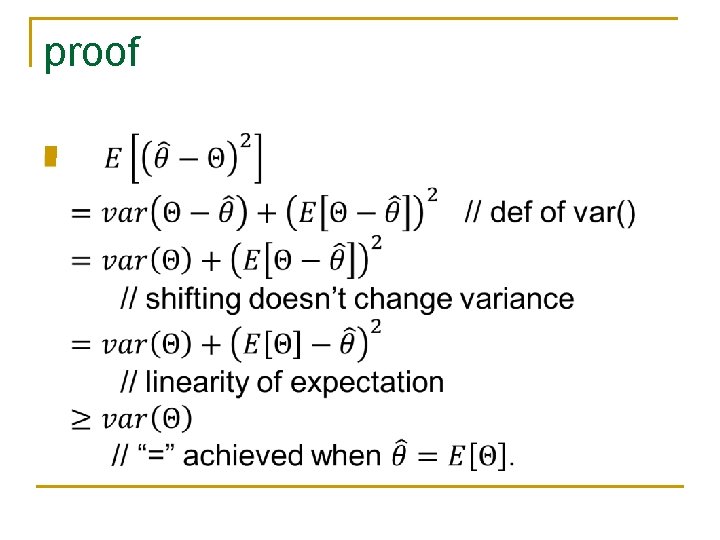
proof n
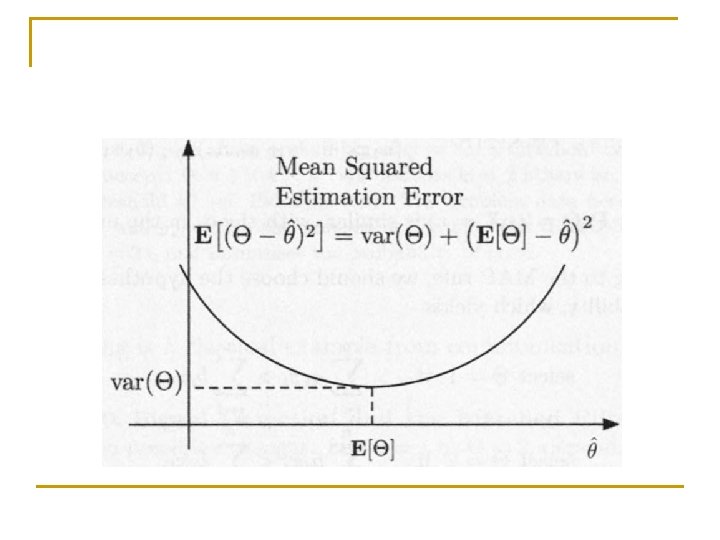
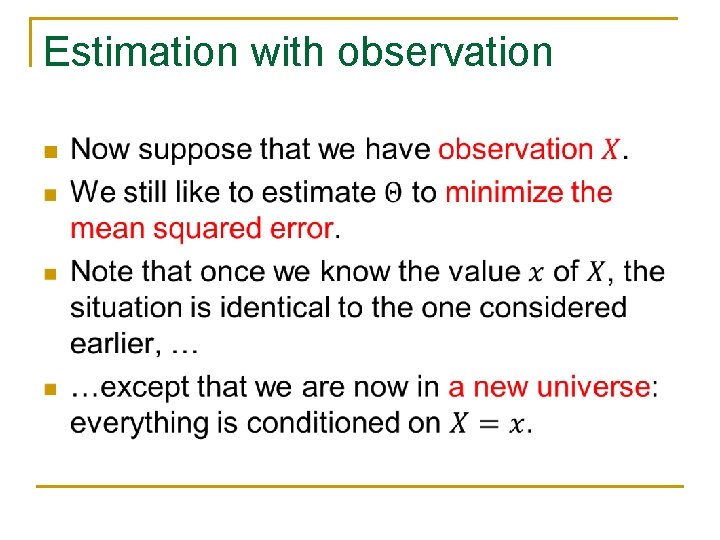
Estimation with observation n
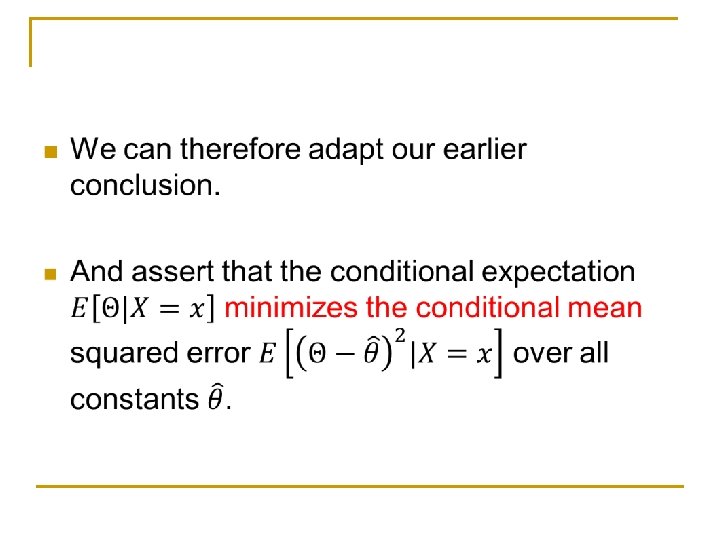
n
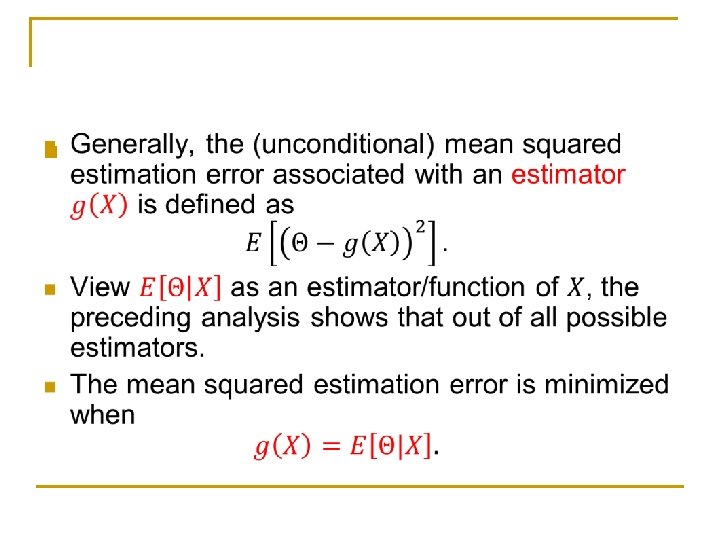
n
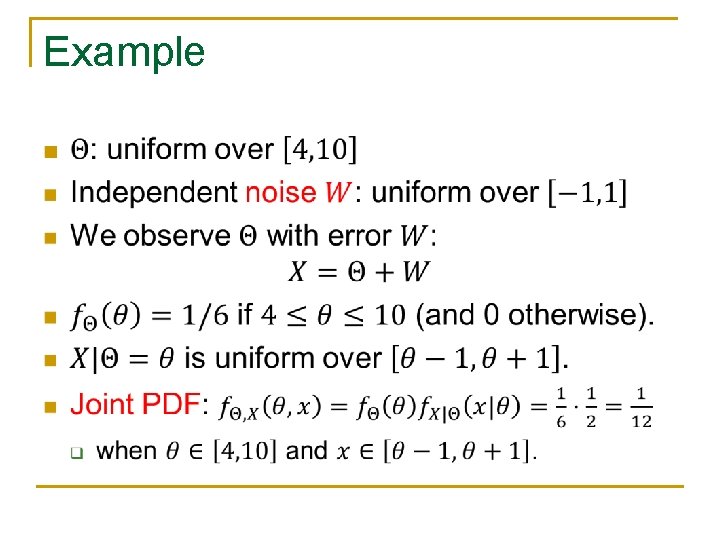
Example n
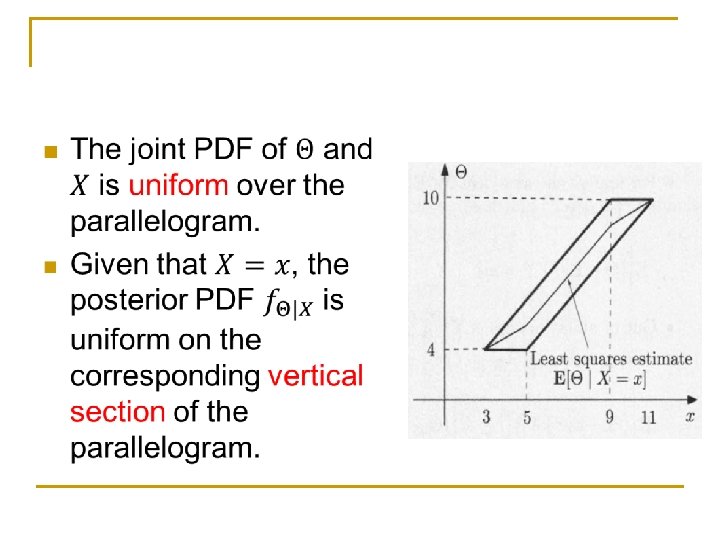
n
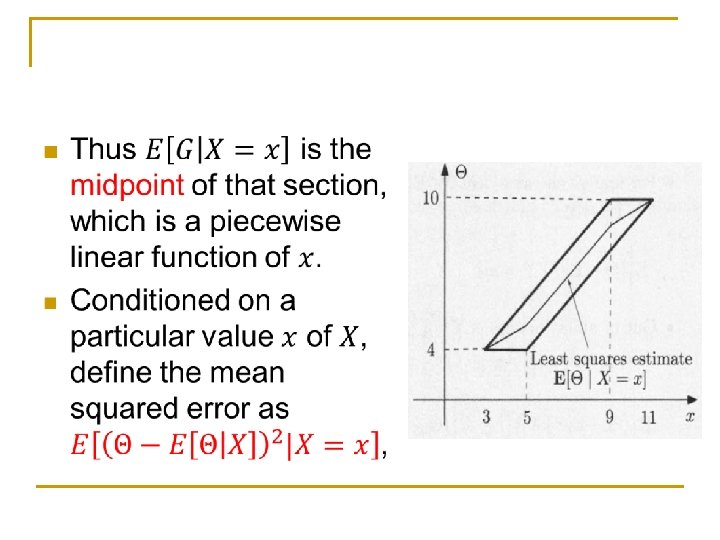
n
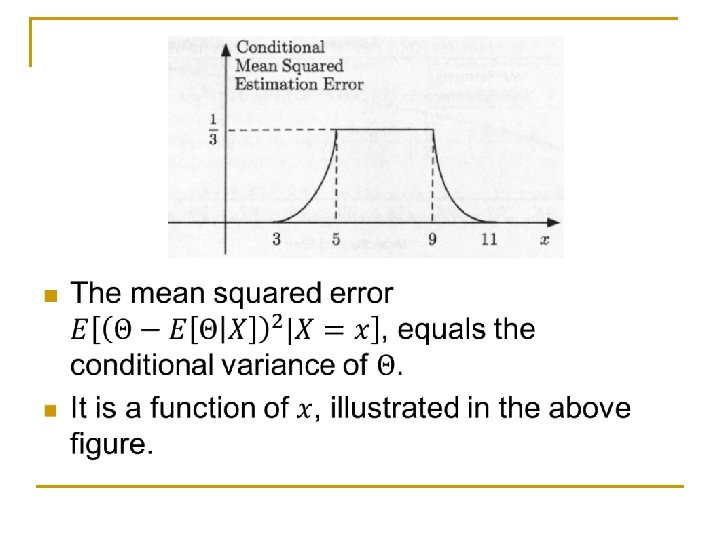
n
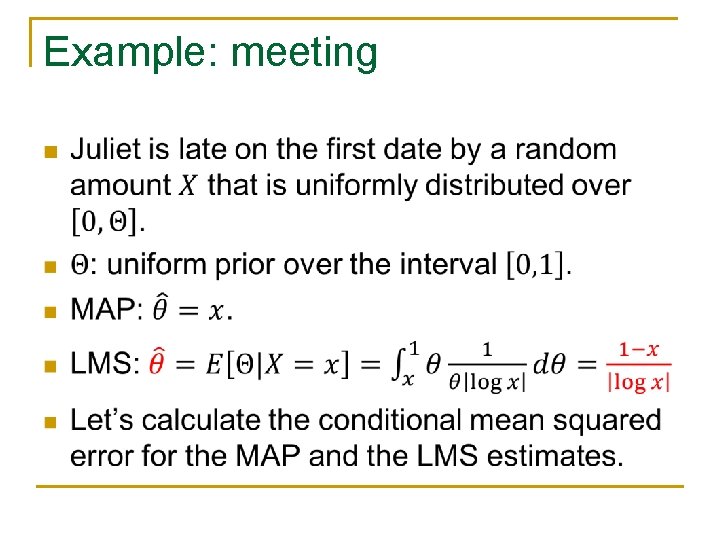
Example: meeting n
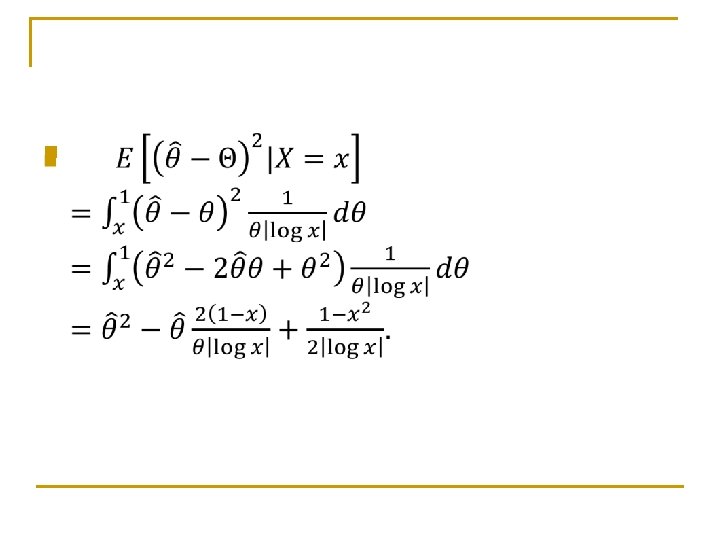
n
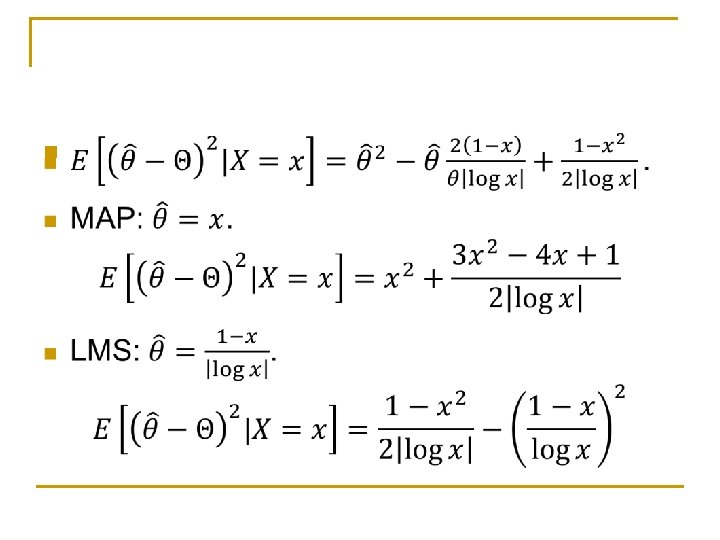
n
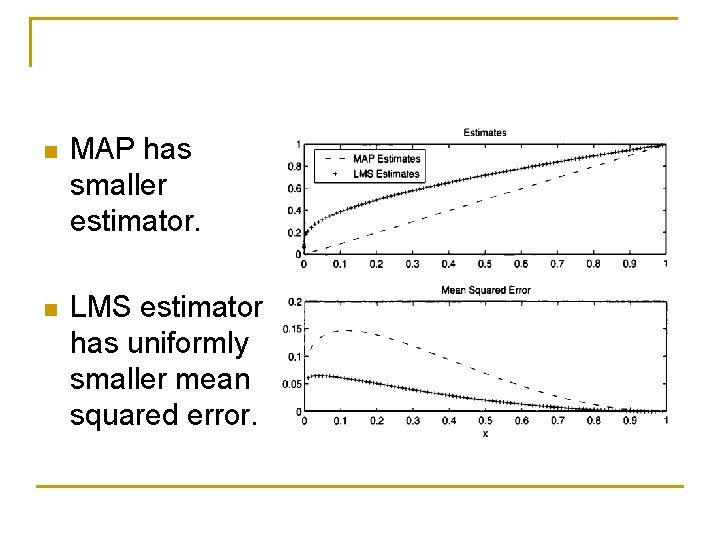
n MAP has smaller estimator. n LMS estimator has uniformly smaller mean squared error.
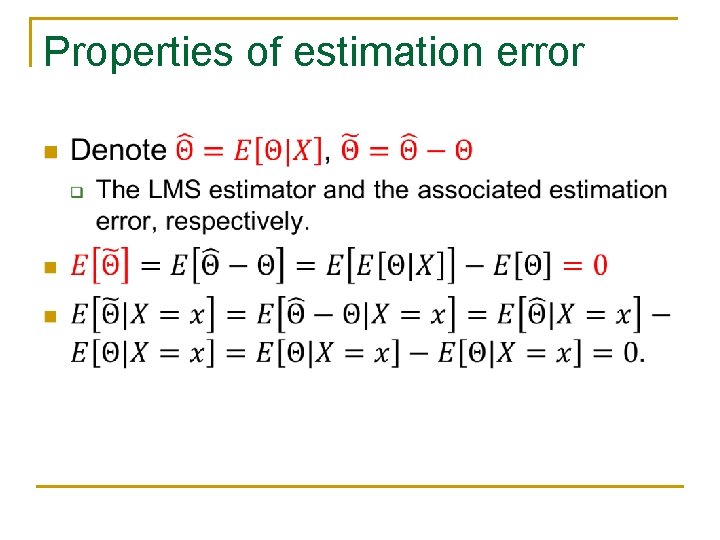
Properties of estimation error n
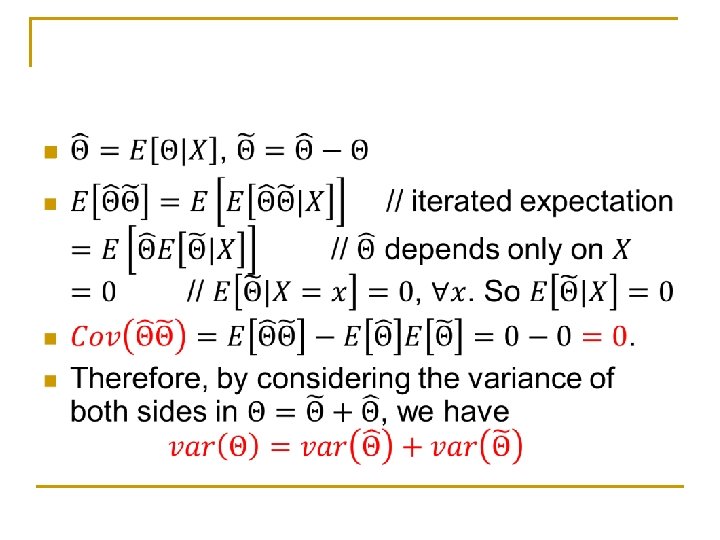
n
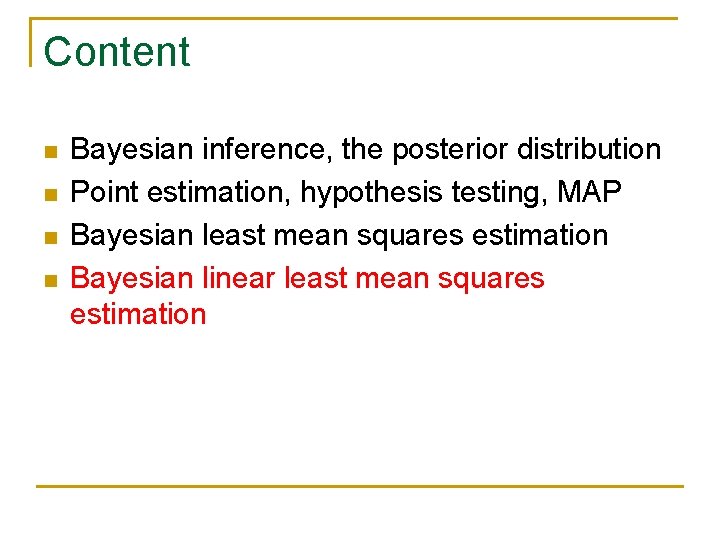
Content n n Bayesian inference, the posterior distribution Point estimation, hypothesis testing, MAP Bayesian least mean squares estimation Bayesian linear least mean squares estimation
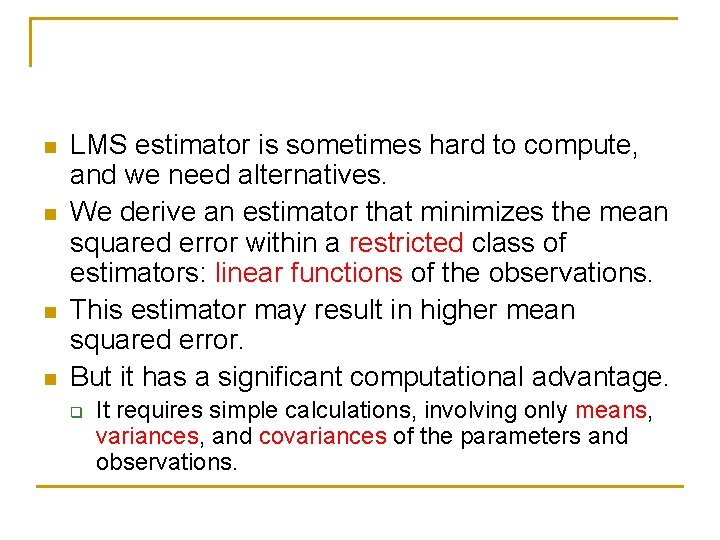
n n LMS estimator is sometimes hard to compute, and we need alternatives. We derive an estimator that minimizes the mean squared error within a restricted class of estimators: linear functions of the observations. This estimator may result in higher mean squared error. But it has a significant computational advantage. q It requires simple calculations, involving only means, variances, and covariances of the parameters and observations.
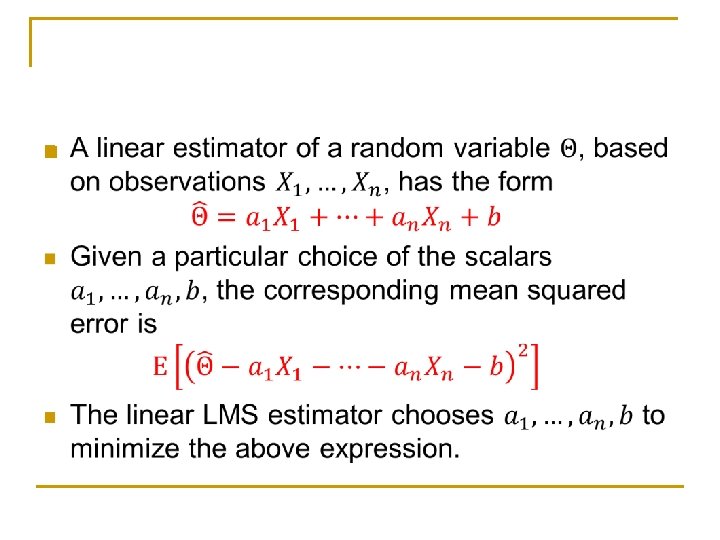
n
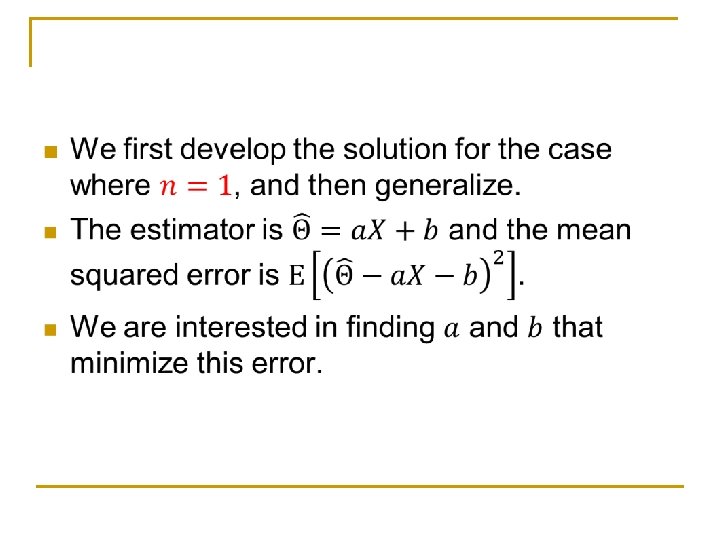
n
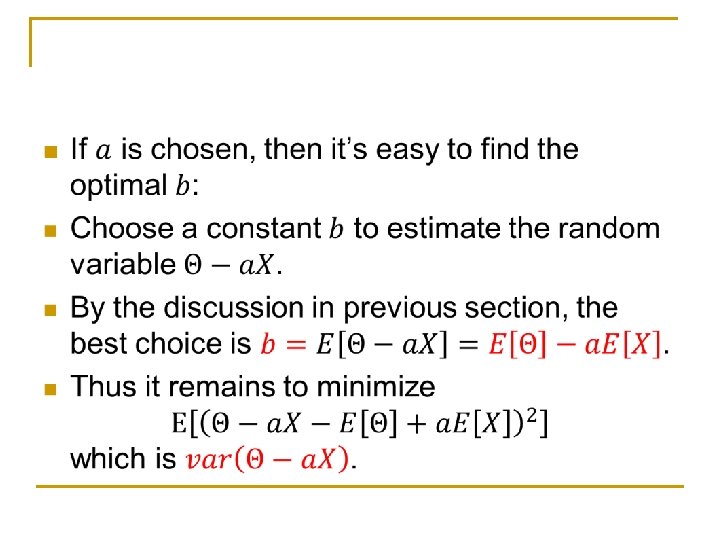
n
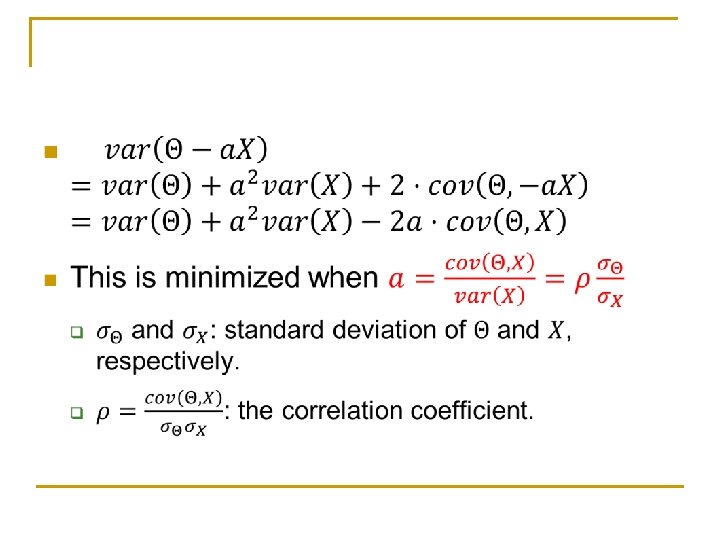
n
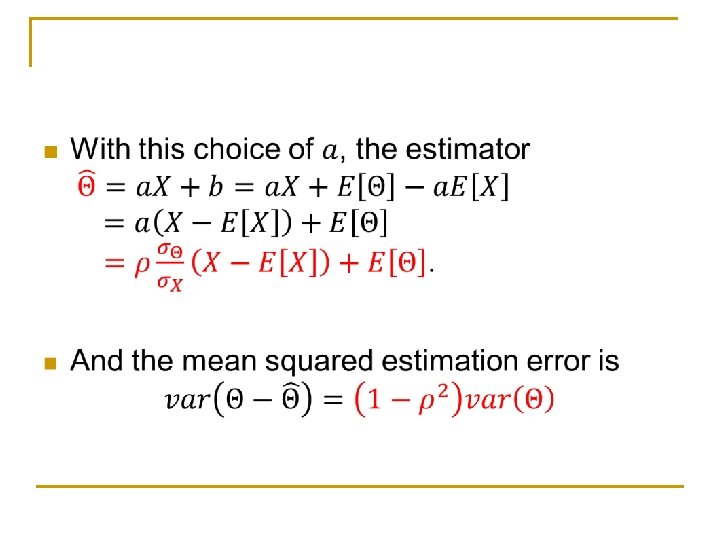
n
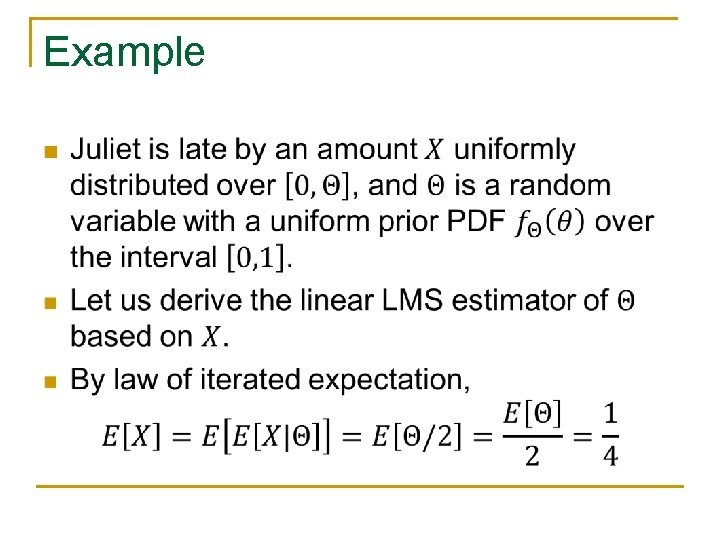
Example n
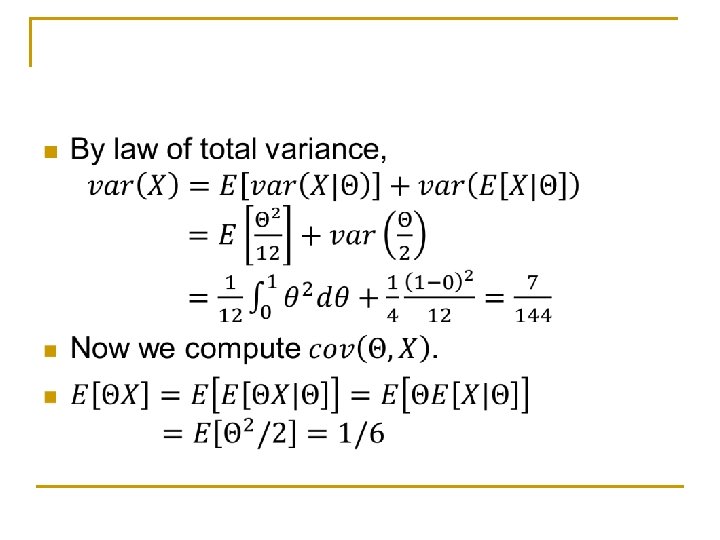
n
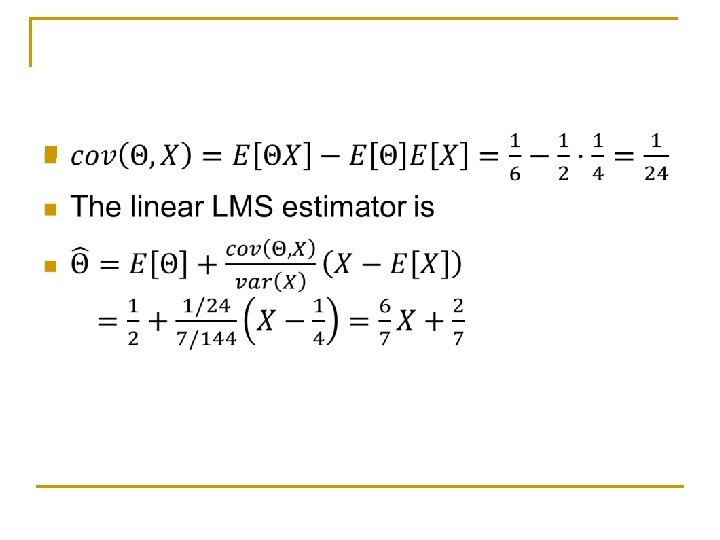
n
Shengyu zhang
Shengyu zhang
Shengyu zhang
Interval estimate example
Chebyshev inequality proof
Statistical inference is concerned with
Probability and statistical inference 9th solution pdf
Statistical inference is divided into
Statistical inference
Instructor
Basic instructor course texas
Medical terminology instructor
Nrp instructor toolkit
Basic instructor course tcole
Nra certified instructor logo
Tp 12863
Instructor vs teacher
Instructor office hours
Neither of my two suitcases are adequate for this trip
Tcole advanced instructor course
Ac 61-65
Basic instructor course #1014
Basic instructor course #1014
Basic instructor course texas
Naismith was an instructor of
Instructor operating station
Cisco certified instructor
Jrotc marksmanship instructor course online
Cisco instructor certification
Tcole 1014 basic instructor course
The virtual instructor elements of art
Tcole basic instructor course
Basic instructor course texas
If your instructor were to ask if you cleaned up your room
Catia instructor
Mptc firearms
Patricia mirror instructor
Lo más importante en la tipología de los participantes
Cbrf registry wi
Marksmanship instructor
Nfpa 1403 instructor to student ratio
Delmar cengage learning instructor resources
Pepperball training manual
Tcole advanced instructor course
Google zhang liang games
Boliang zhang
Zhiwu zhang
Guoxing sun
Hui zhang indiana
Lixia zhang ucla
Red means courage devotion uprightness bravery and loyalty
Ikaye zhang
Jingxuan zhang
Zilin zhang
Yupu zhang
Ginger zhang
Grace zhang morgan stanley
Zuofeng zhang
Zuofeng zhang
Yundong zhang
Deep learning for dummies
Squid
Yunxi zhang
Zike zhang
Ikaye zhang
Pst2005
Zhang wang leukemia
Local lymph node assay
Jiagu 360
Yanci zhang
Tong zhang
Hui zhang cmu
Intellectual giftedness