Crosslingual Name Tagging and Linking for 282 Xiaoman
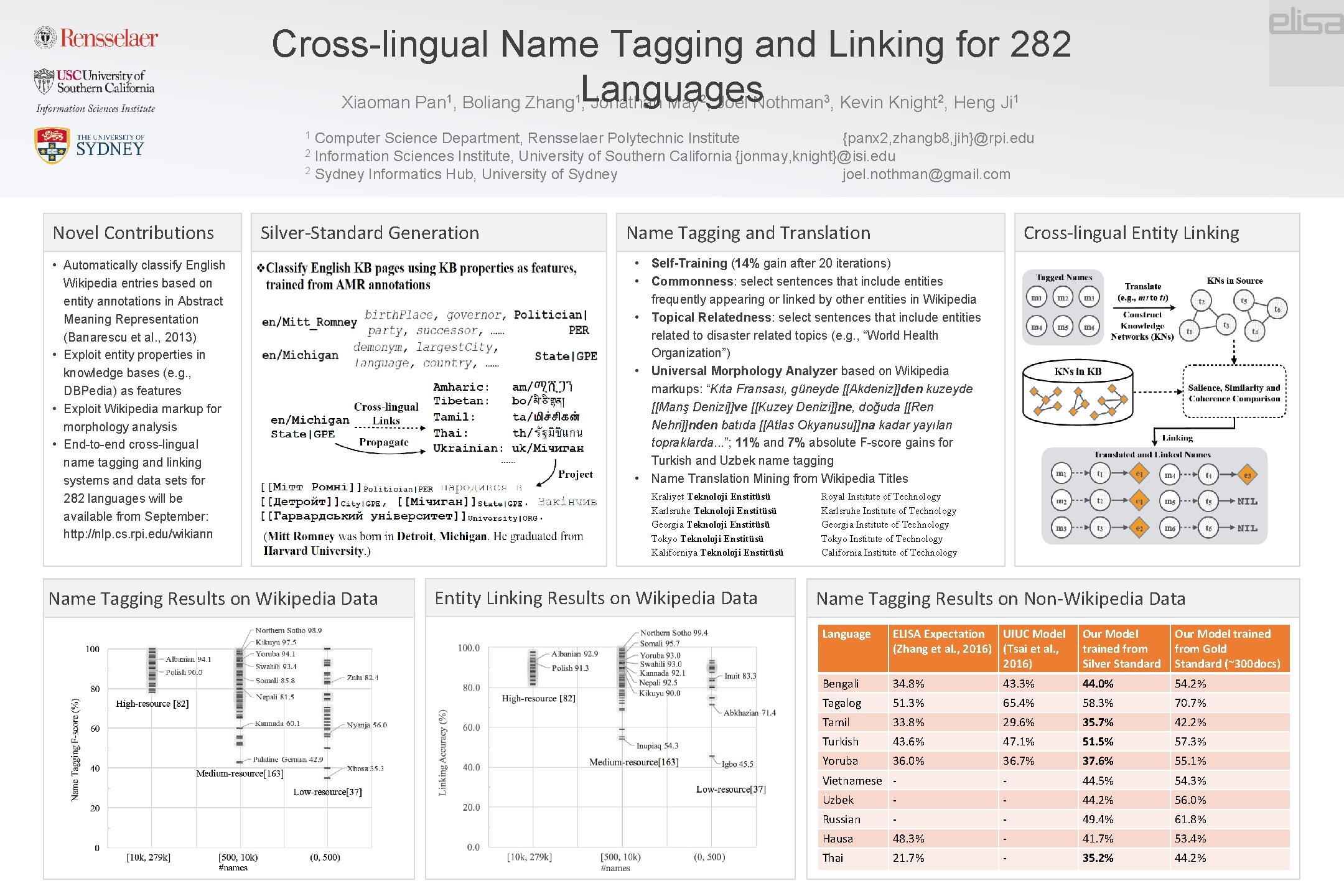
- Slides: 1
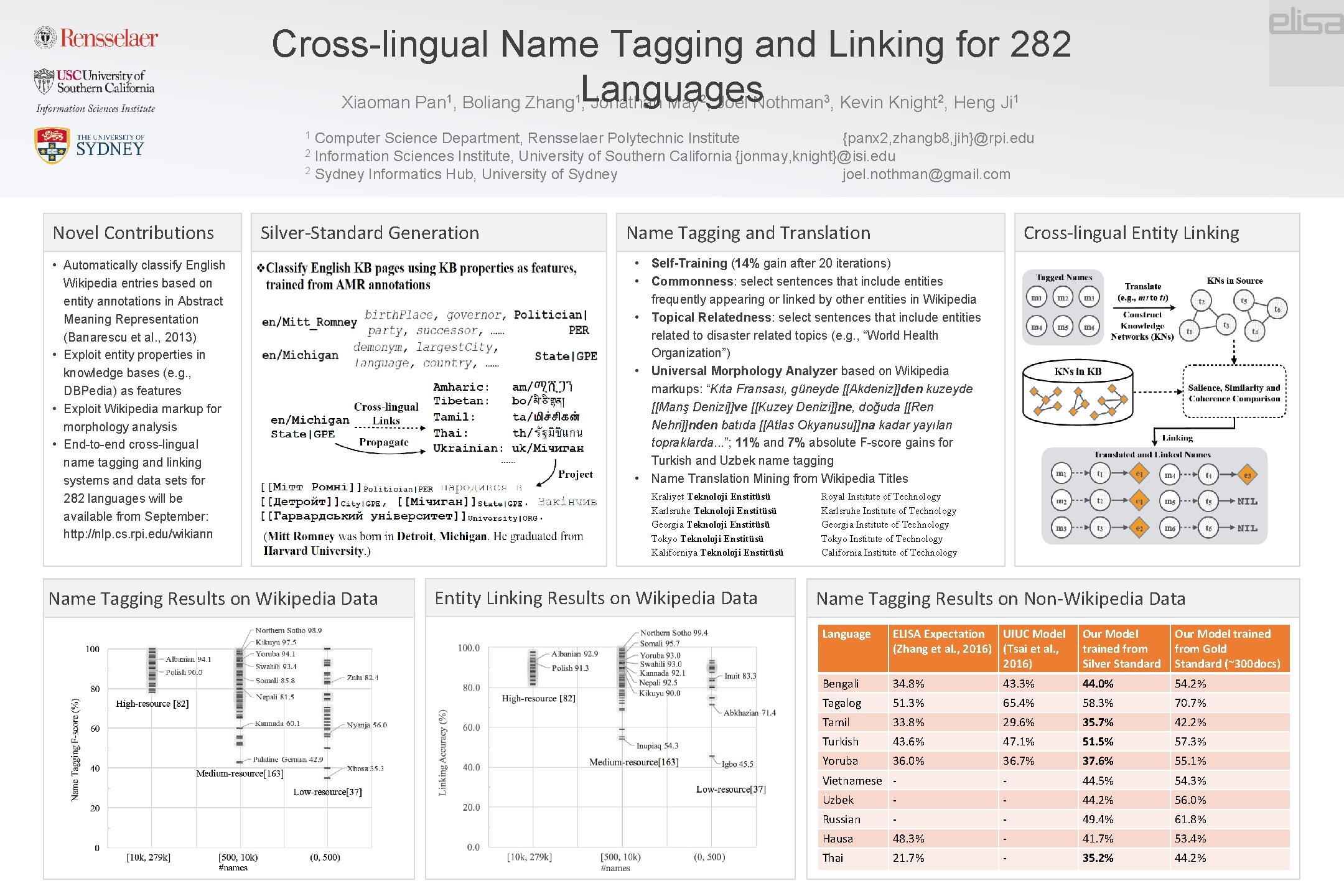
Cross-lingual Name Tagging and Linking for 282 Xiaoman Pan , Boliang Zhang Languages , Jonathan May , Joel Nothman , Kevin Knight , Heng Ji 1 1 2 3 2 1 Computer Science Department, Rensselaer Polytechnic Institute {panx 2, zhangb 8, jih}@rpi. edu 2 Information Sciences Institute, University of Southern California {jonmay, knight}@isi. edu 2 Sydney Informatics Hub, University of Sydney joel. nothman@gmail. com 1 Novel Contributions Silver-Standard Generation Cross-lingual Entity Linking Name Tagging and Translation –– • Automatically classify English Wikipedia entries based on entity annotations in Abstract Meaning Representation (Banarescu et al. , 2013) • Exploit entity properties in knowledge bases (e. g. , DBPedia) as features • Exploit Wikipedia markup for morphology analysis • End-to-end cross-lingual name tagging and linking systems and data sets for 282 languages will be available from September: http: //nlp. cs. rpi. edu/wikiann Name Tagging Results on Wikipedia Data • Self-Training (14% gain after 20 iterations) • Commonness: select sentences that include entities frequently appearing or linked by other entities in Wikipedia • Topical Relatedness: select sentences that include entities related to disaster related topics (e. g. , “World Health Organization”) • Universal Morphology Analyzer based on Wikipedia markups: “Kıta Fransası, güneyde [[Akdeniz]]den kuzeyde [[Manş Denizi]]ve [[Kuzey Denizi]]ne, doğuda [[Ren Nehri]]nden batıda [[Atlas Okyanusu]]na kadar yayılan topraklarda. . . ”; 11% and 7% absolute F-score gains for Turkish and Uzbek name tagging • Name Translation Mining from Wikipedia Titles Kraliyet Teknoloji Enstitüsü Karlsruhe Teknoloji Enstitüsü Georgia Teknoloji Enstitüsü Tokyo Teknoloji Enstitüsü Kaliforniya Teknoloji Enstitüsü Entity Linking Results on Wikipedia Data Royal Institute of Technology Karlsruhe Institute of Technology Georgia Institute of Technology Tokyo Institute of Technology California Institute of Technology Name Tagging Results on Non-Wikipedia Data Language ELISA Expectation UIUC Model (Zhang et al. , 2016) (Tsai et al. , 2016) Our Model trained from Silver Standard Our Model trained from Gold Standard (~300 docs) Bengali 34. 8% 43. 3% 44. 0% 54. 2% Tagalog 51. 3% 65. 4% 58. 3% 70. 7% Tamil 33. 8% 29. 6% 35. 7% 42. 2% Turkish 43. 6% 47. 1% 51. 5% 57. 3% Yoruba 36. 0% 36. 7% 37. 6% 55. 1% Vietnamese - - 44. 5% 54. 3% Uzbek - - 44. 2% 56. 0% Russian - - 49. 4% 61. 8% Hausa 48. 3% - 41. 7% 53. 4% Thai 21. 7% - 35. 2% 44. 2%