Data integration for candidate gene prioritization Yves Moreau
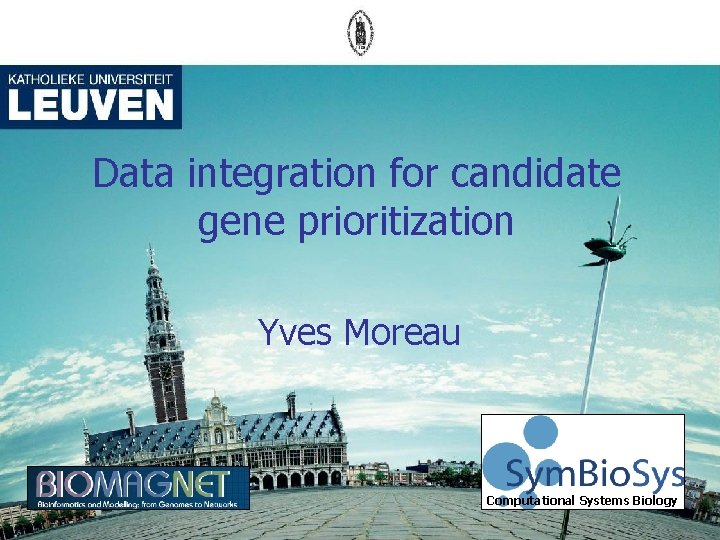
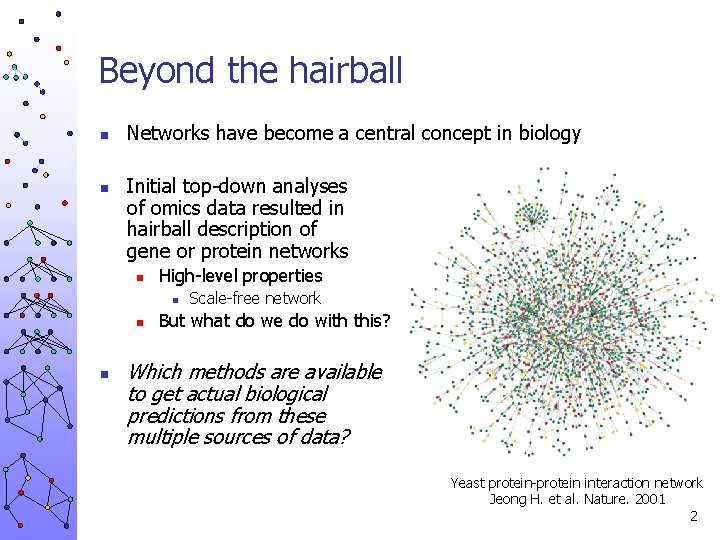
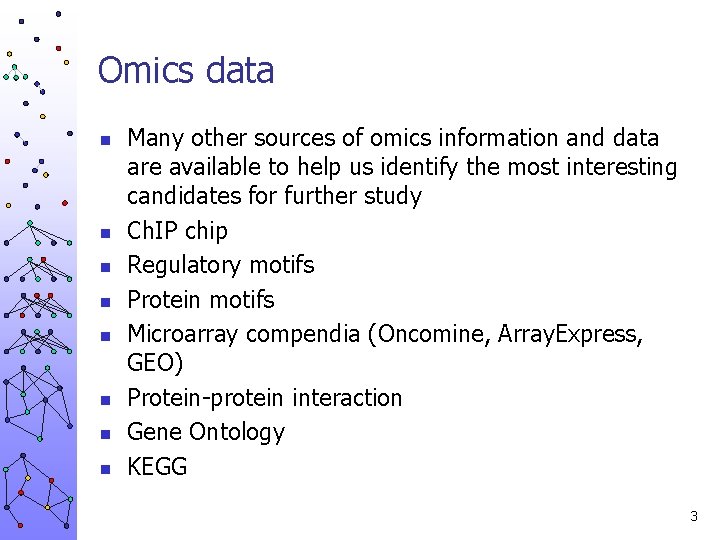
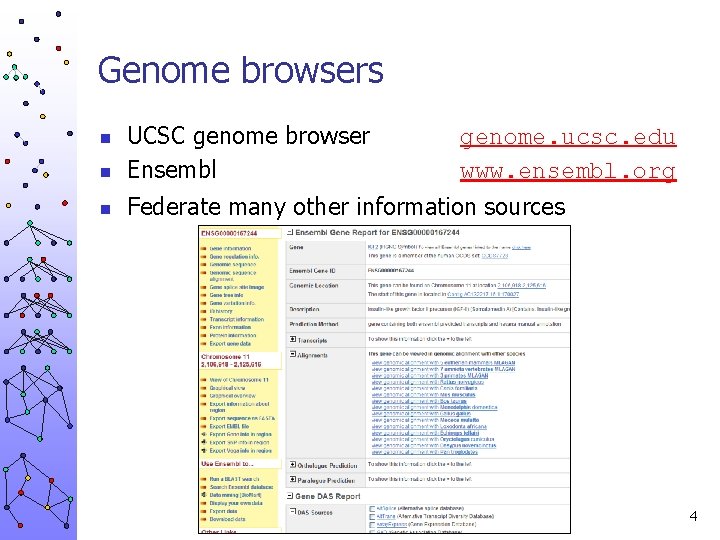
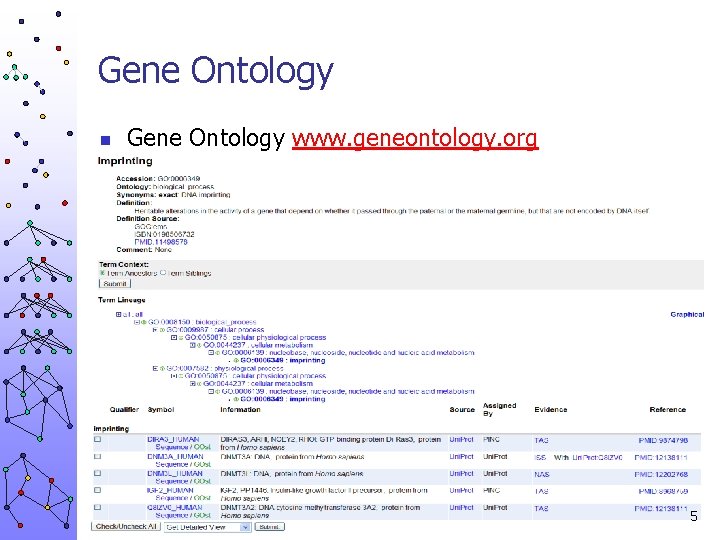
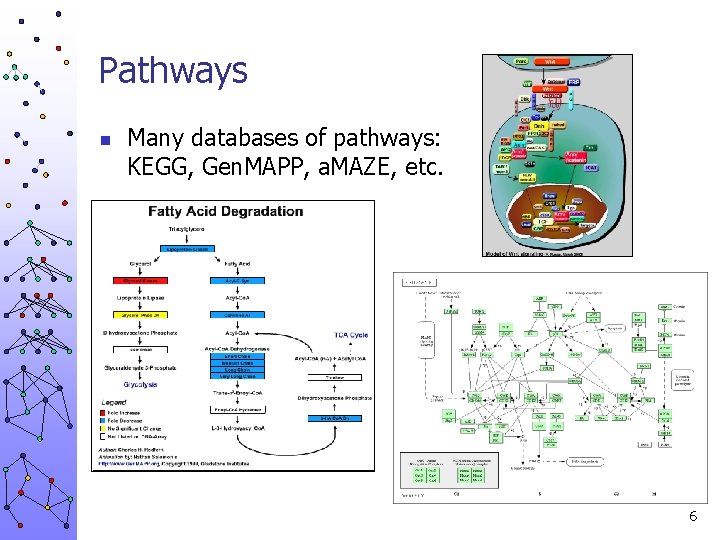
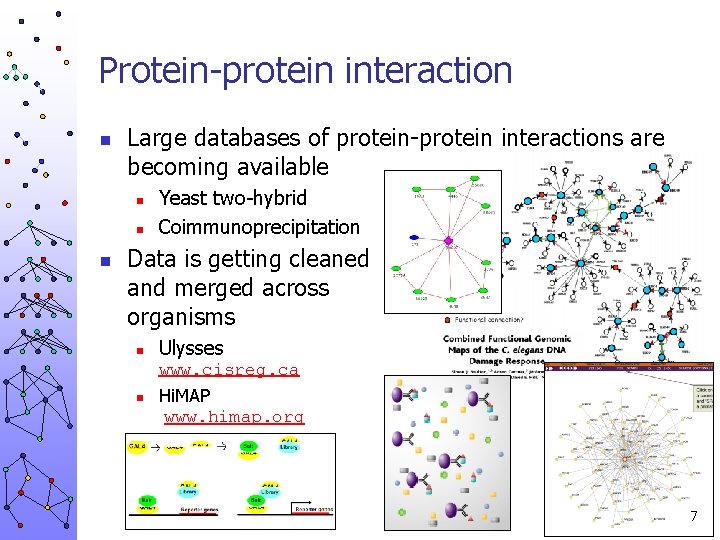
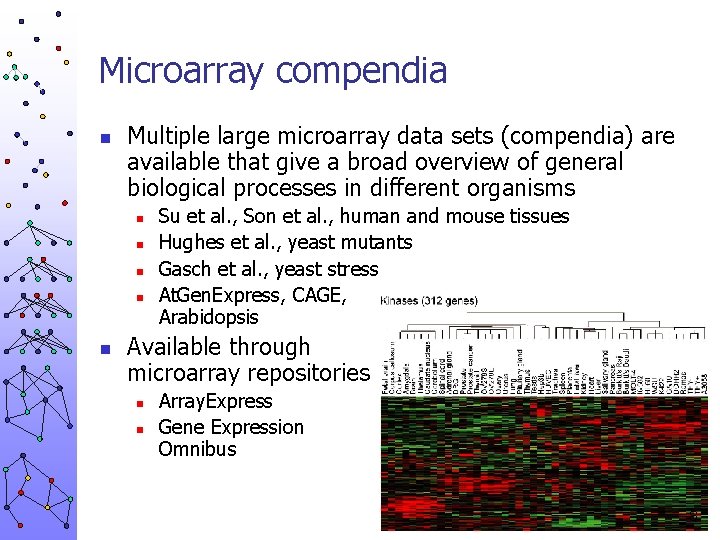
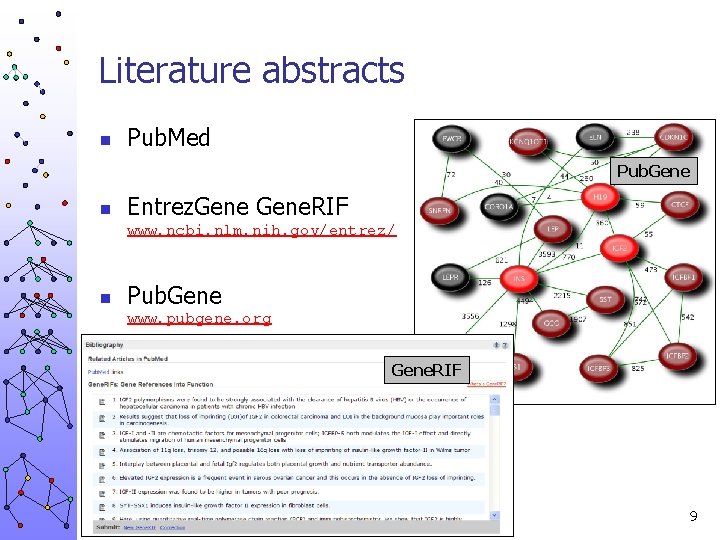
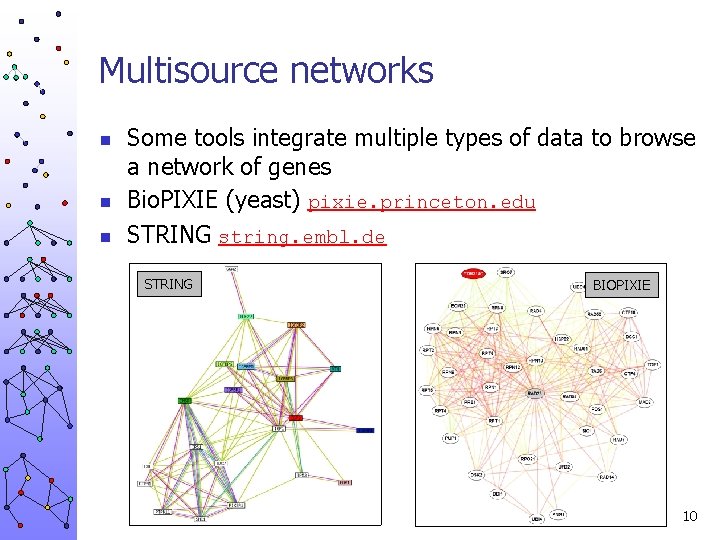
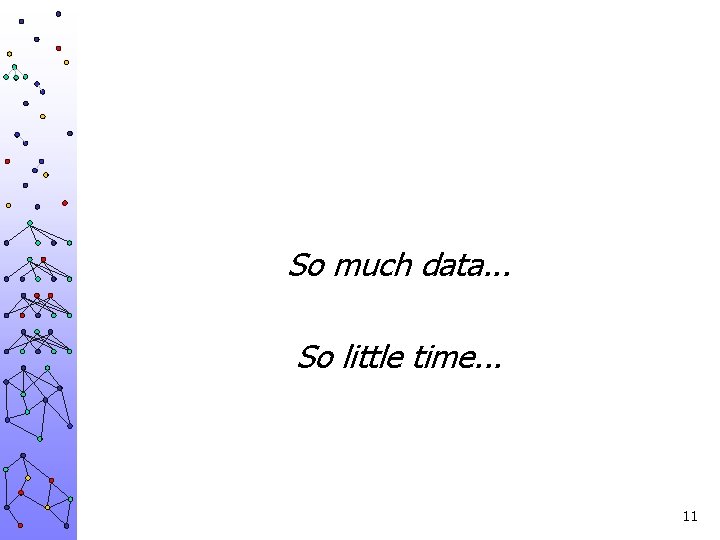
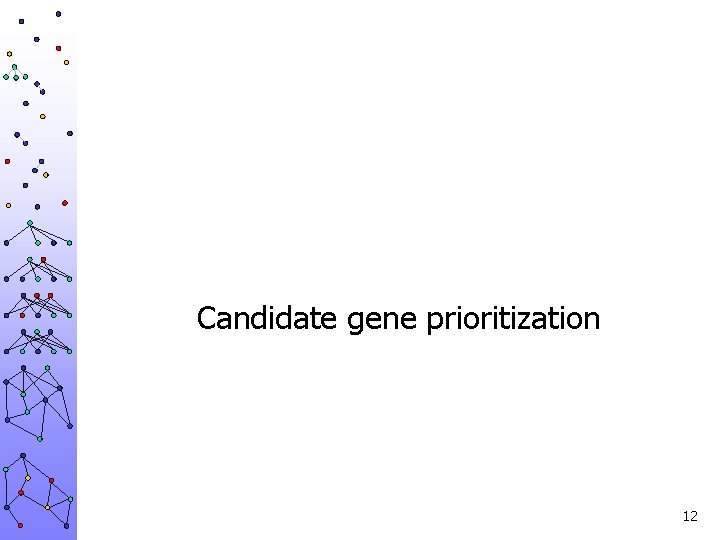
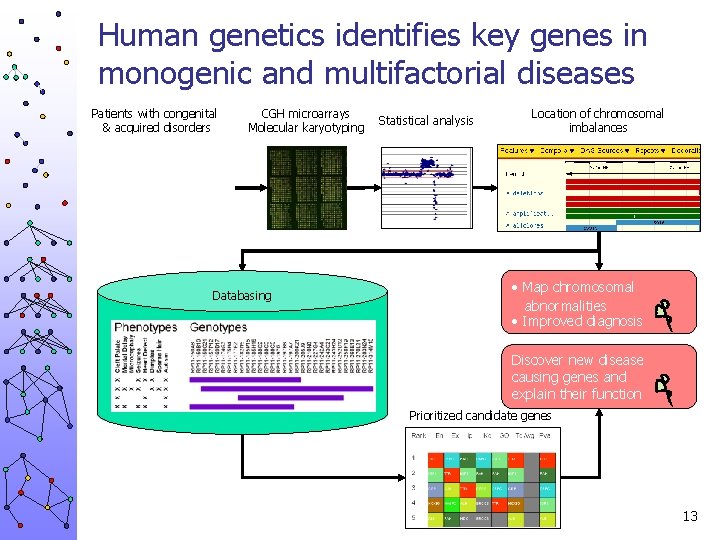
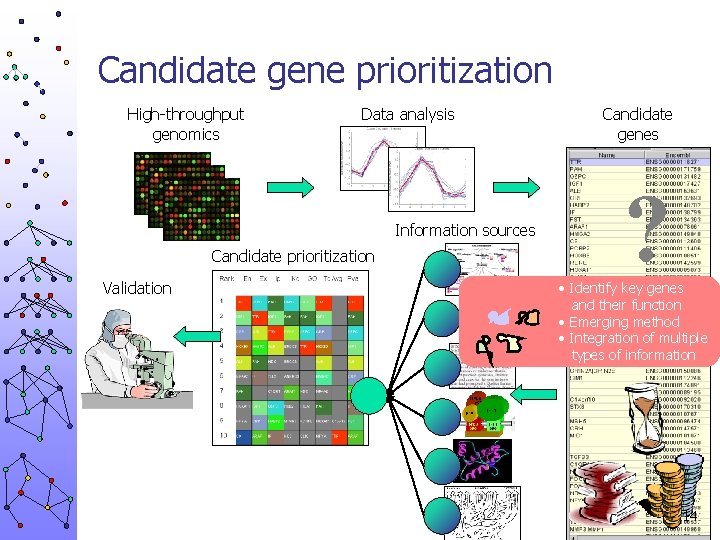
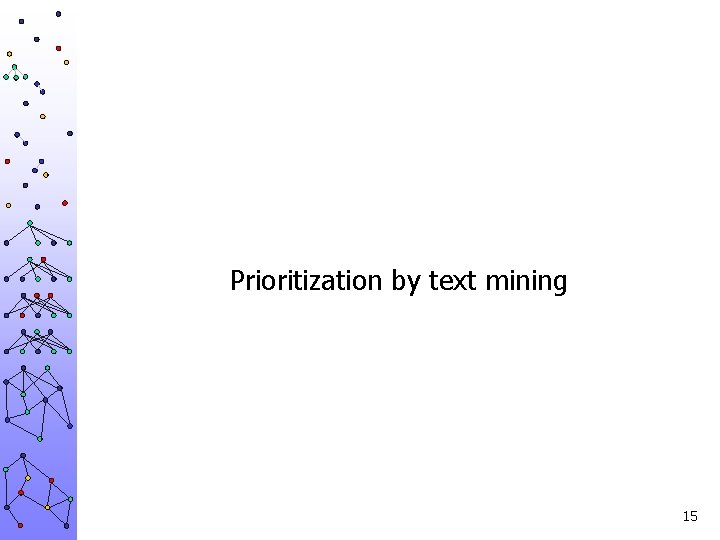
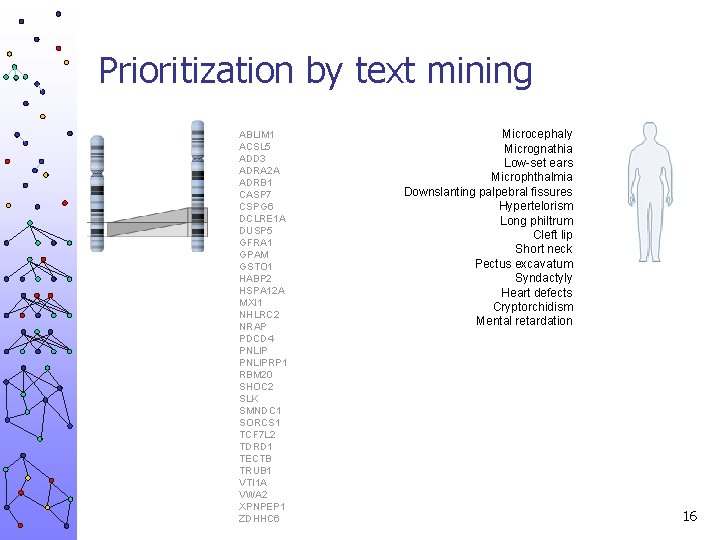
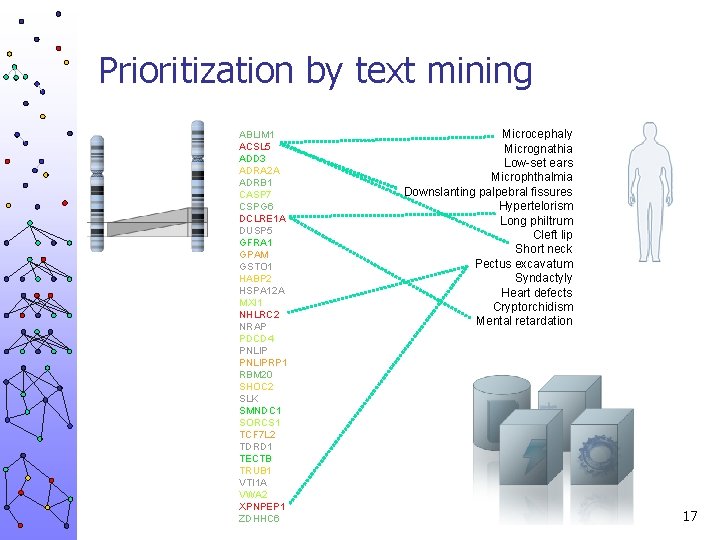
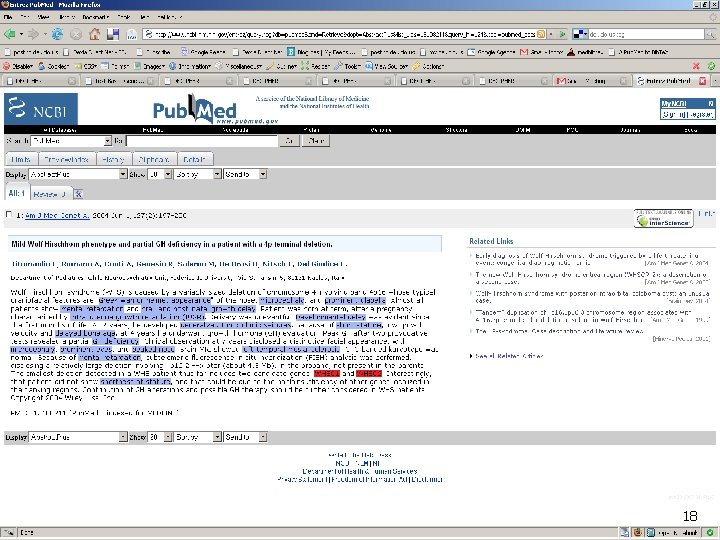
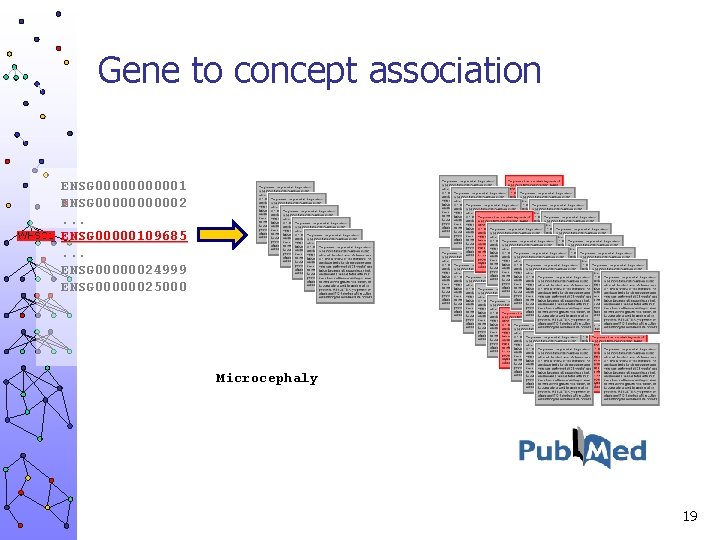
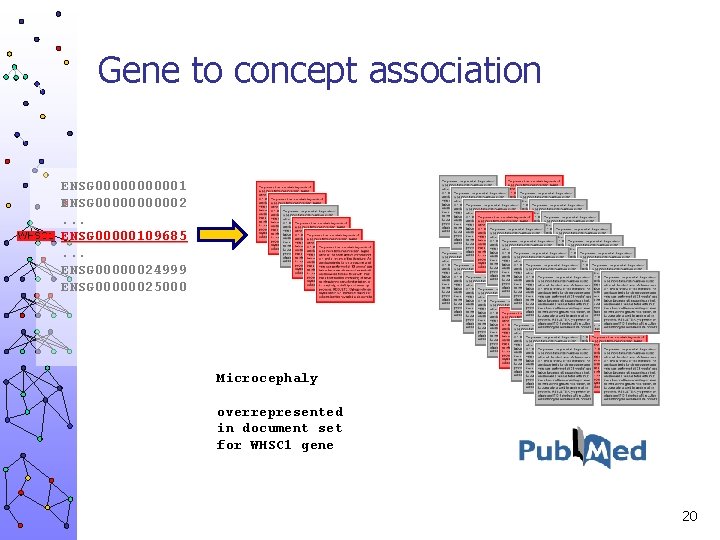
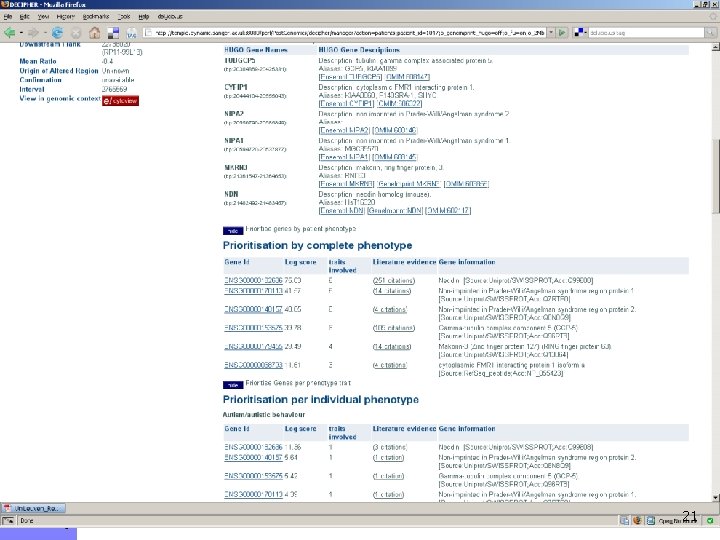
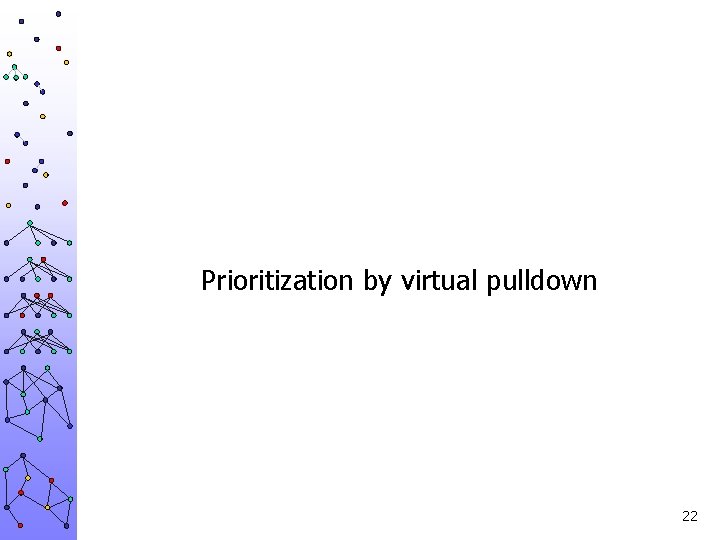
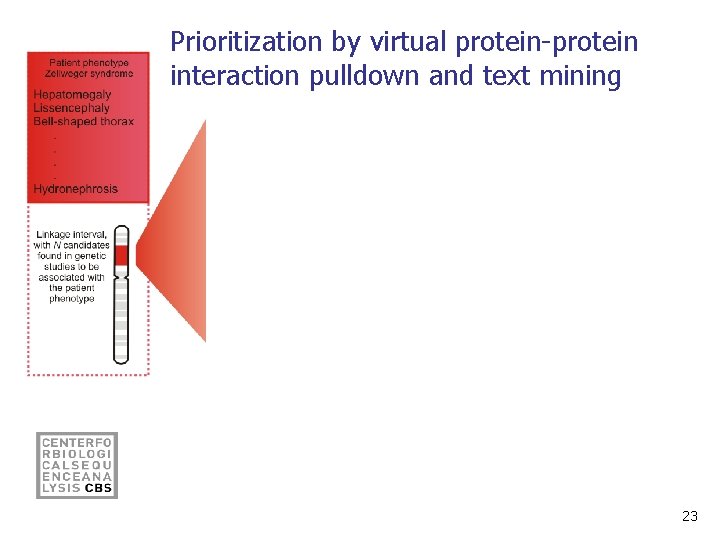
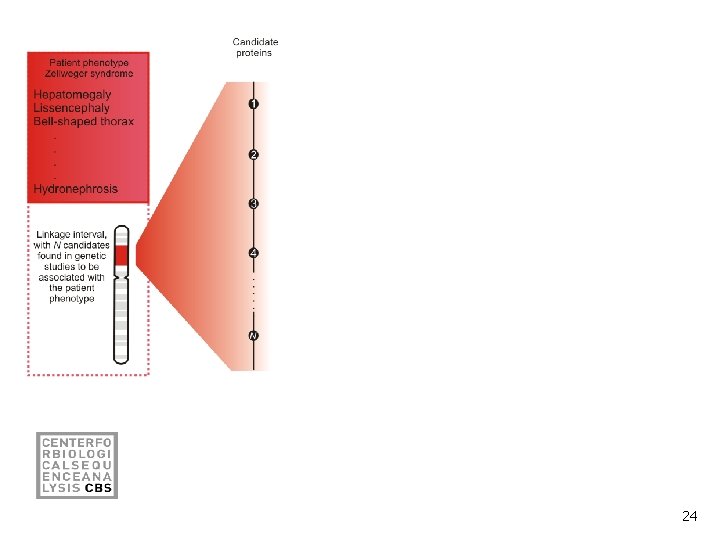
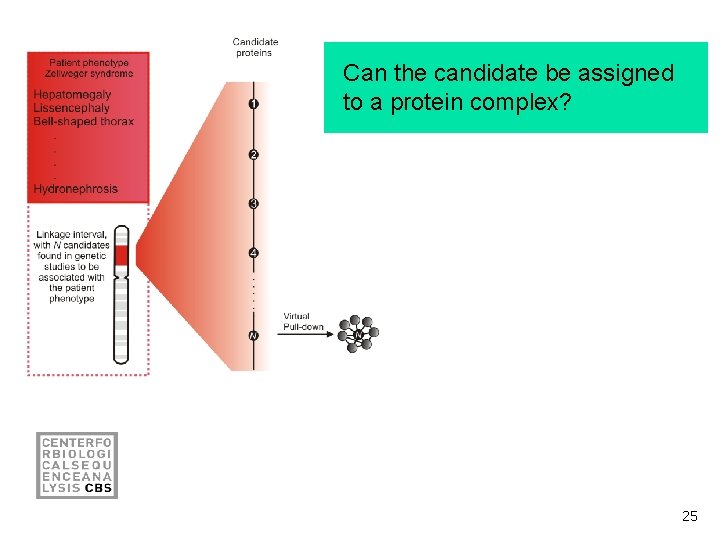
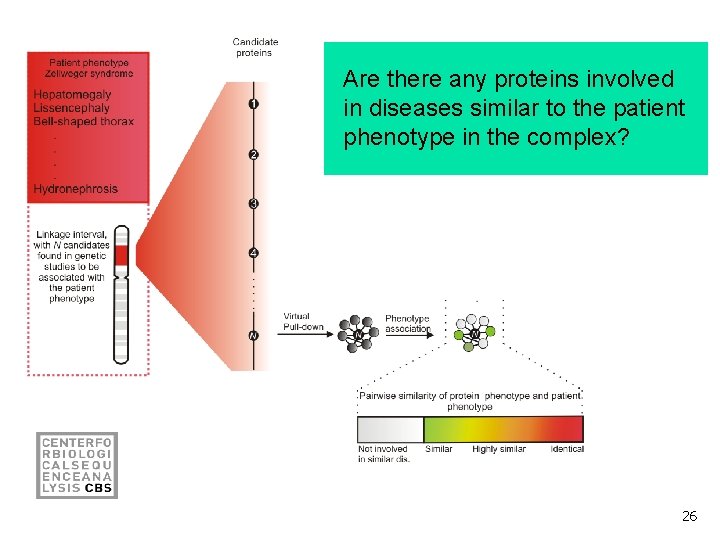
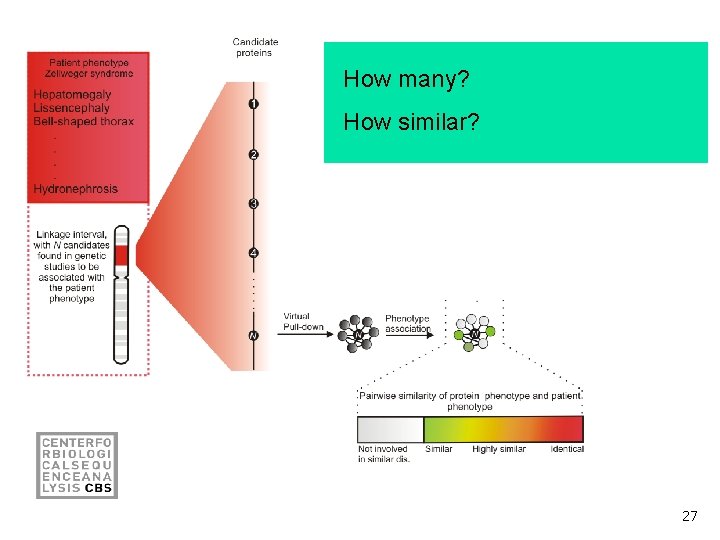
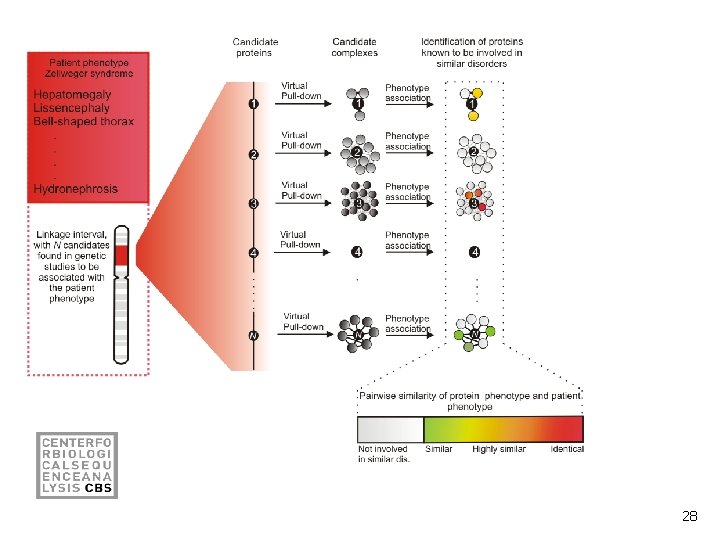
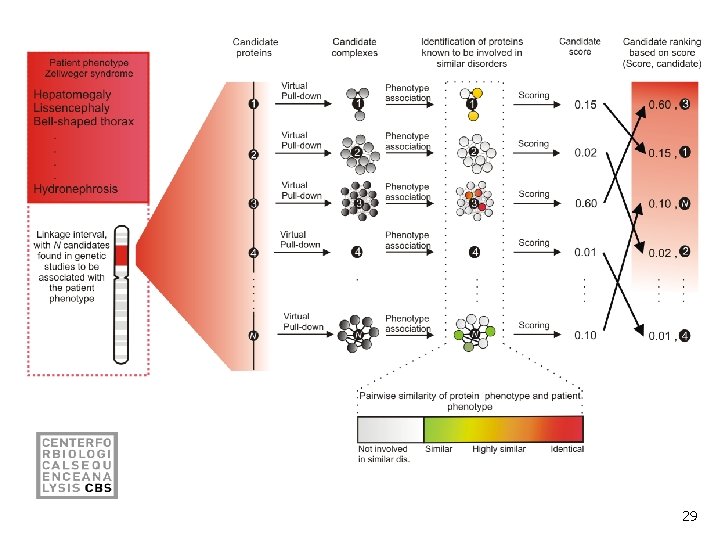
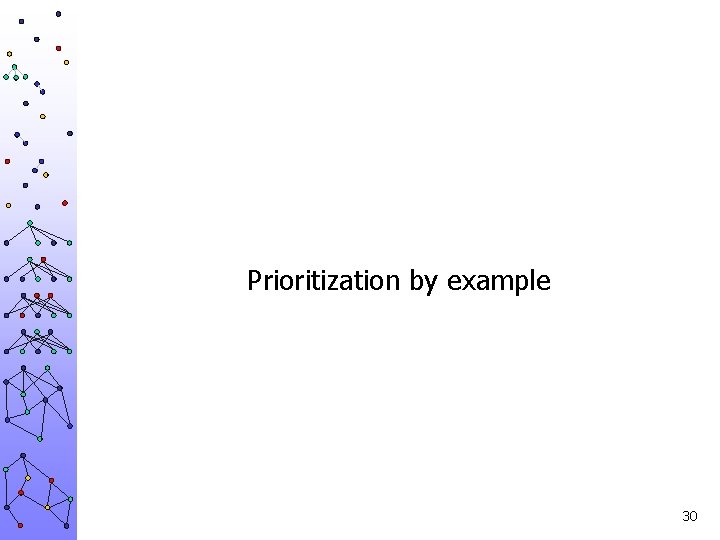
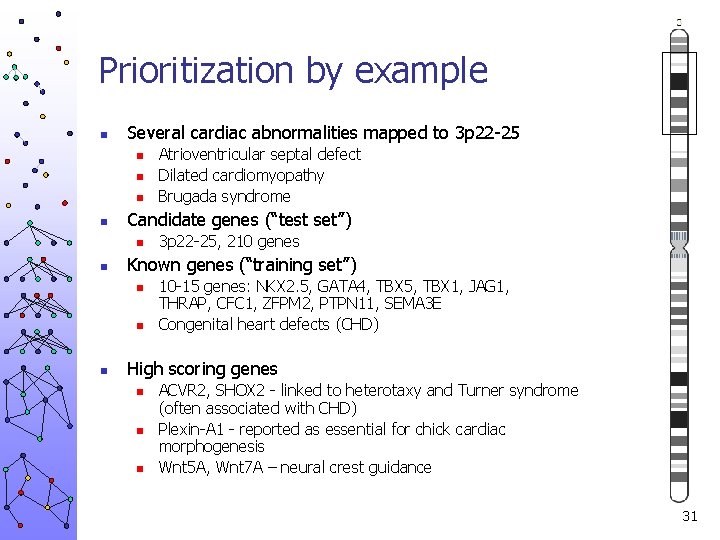
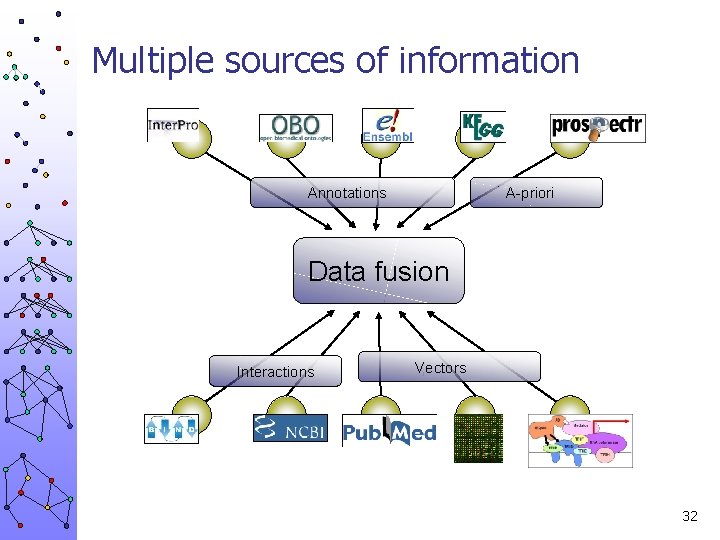
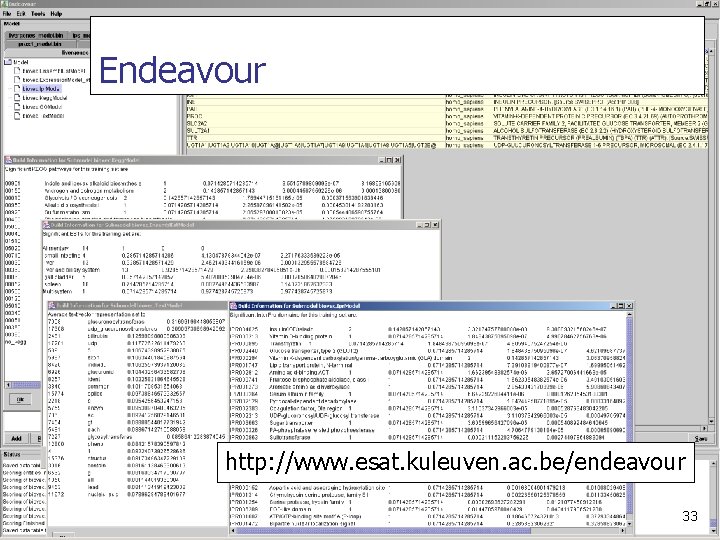
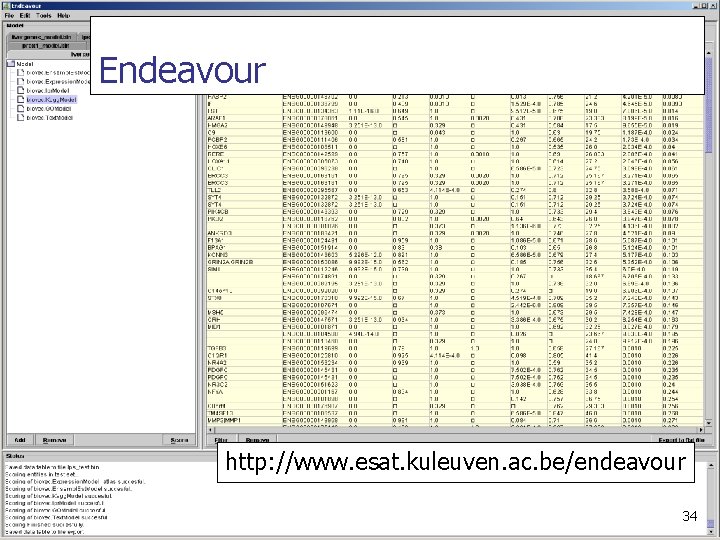
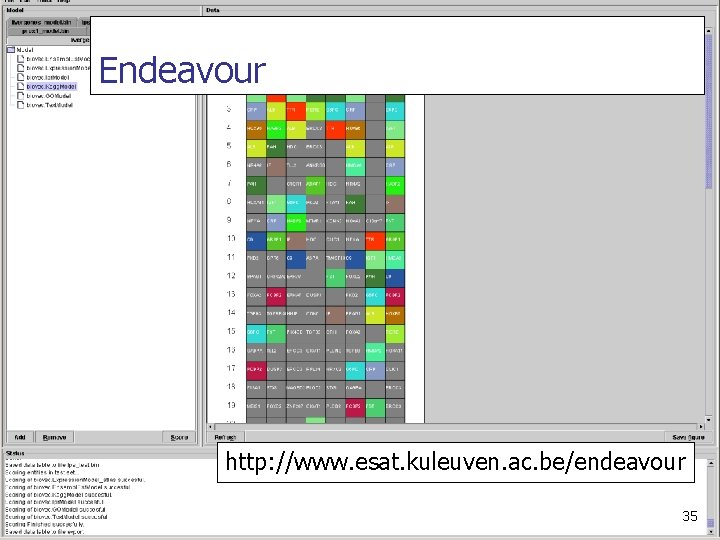
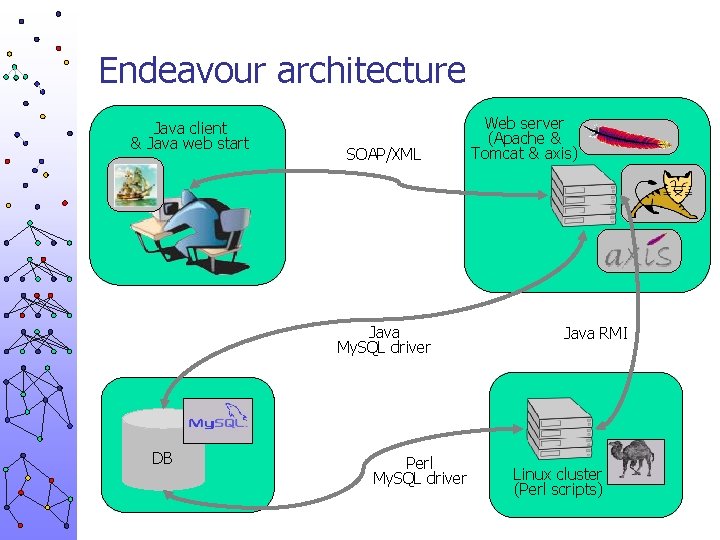
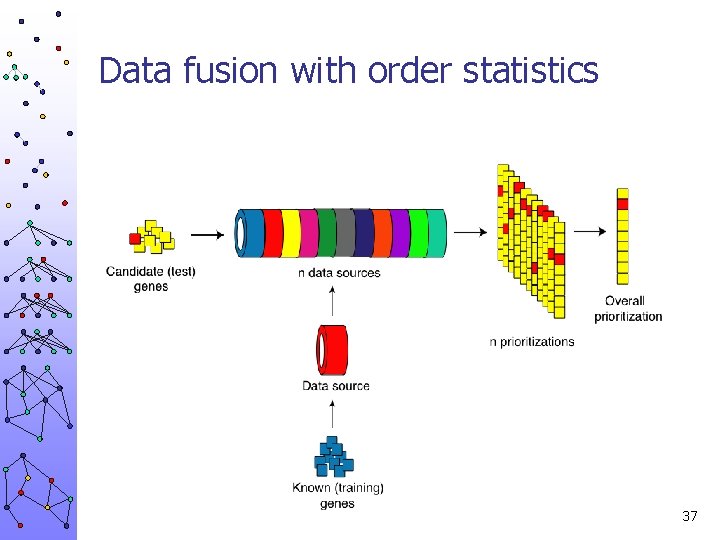
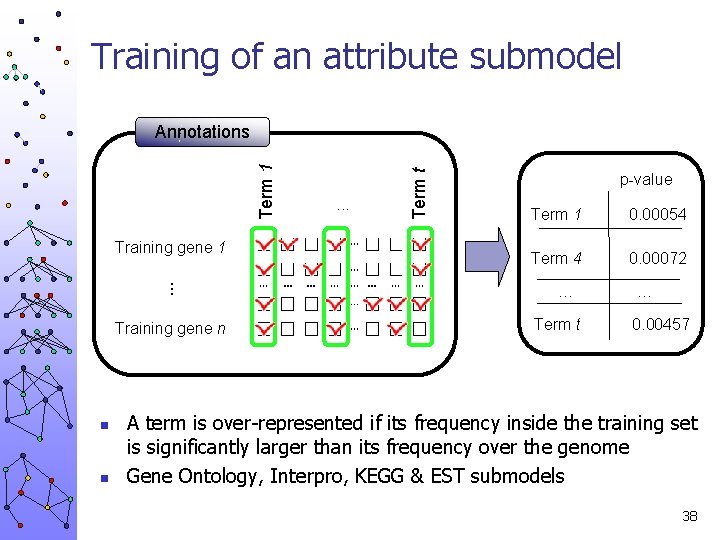
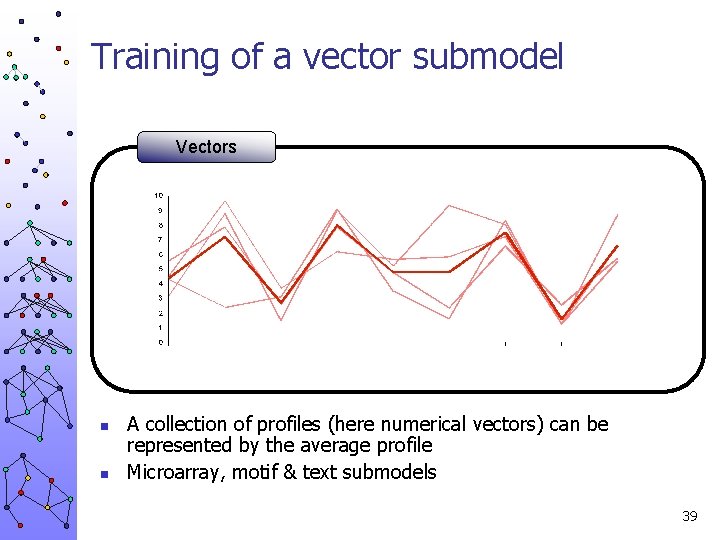
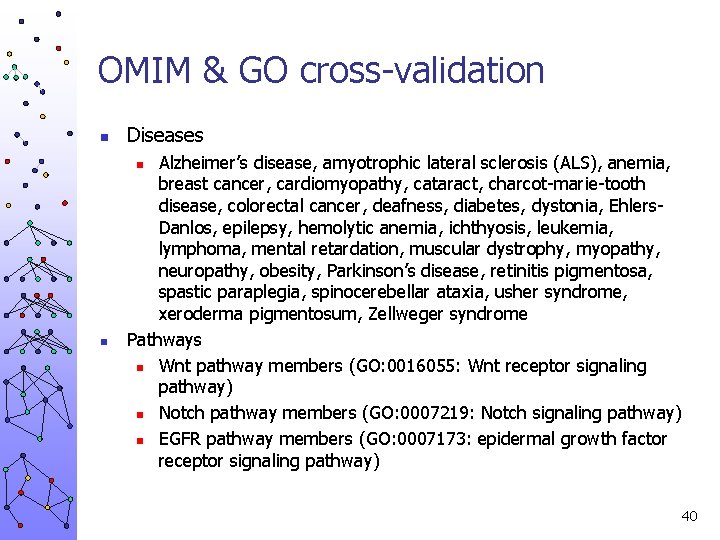
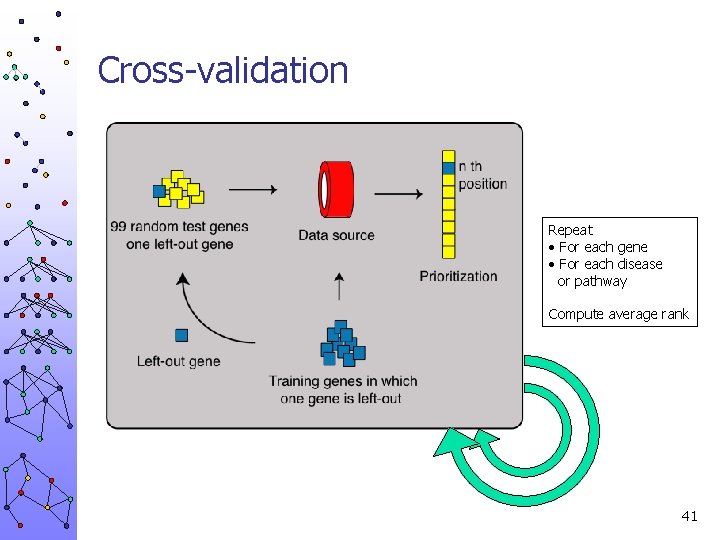
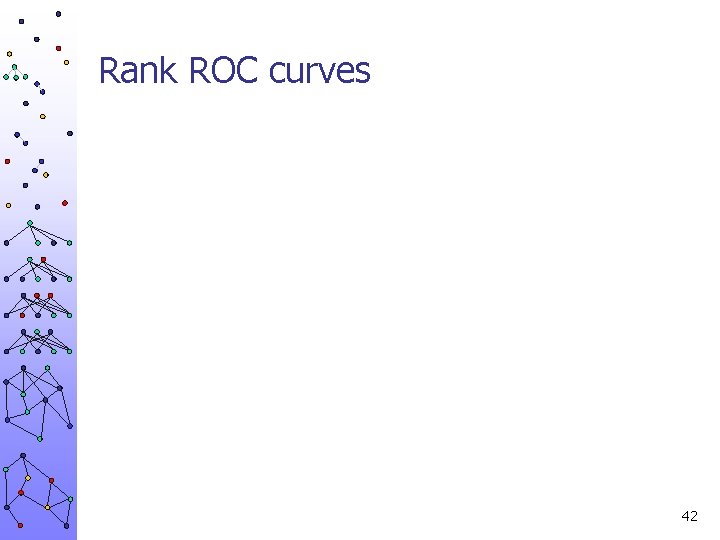
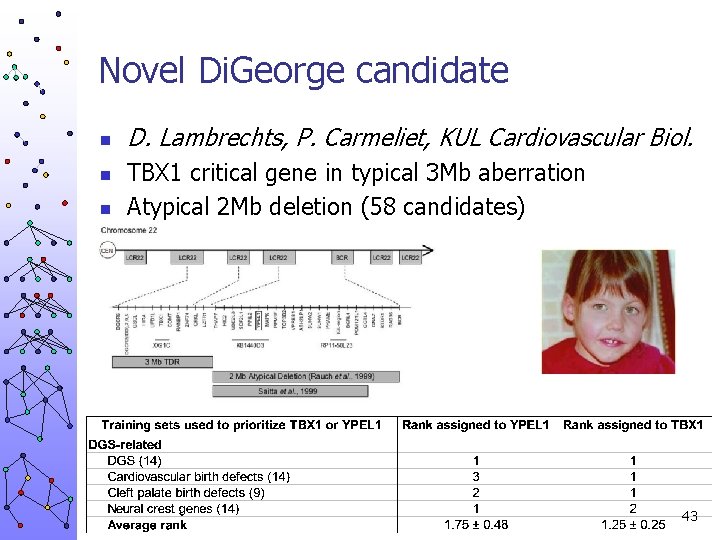
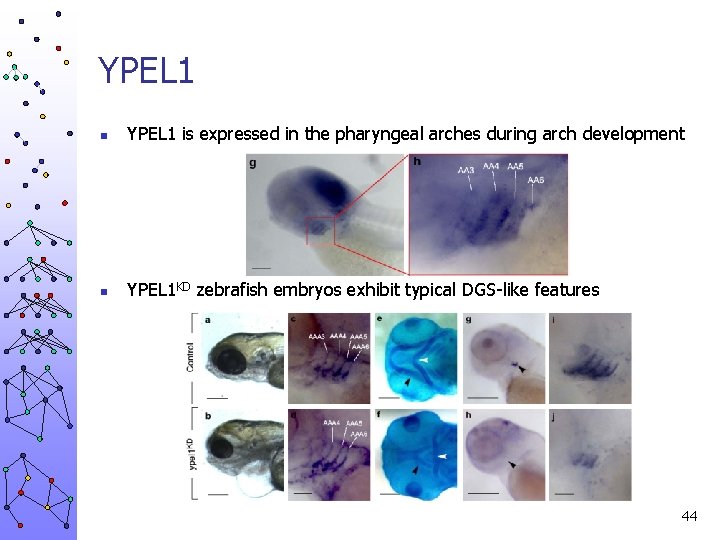
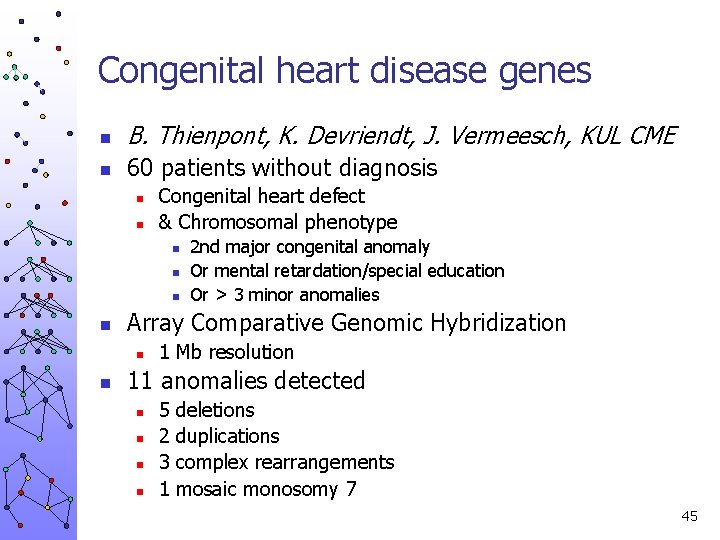
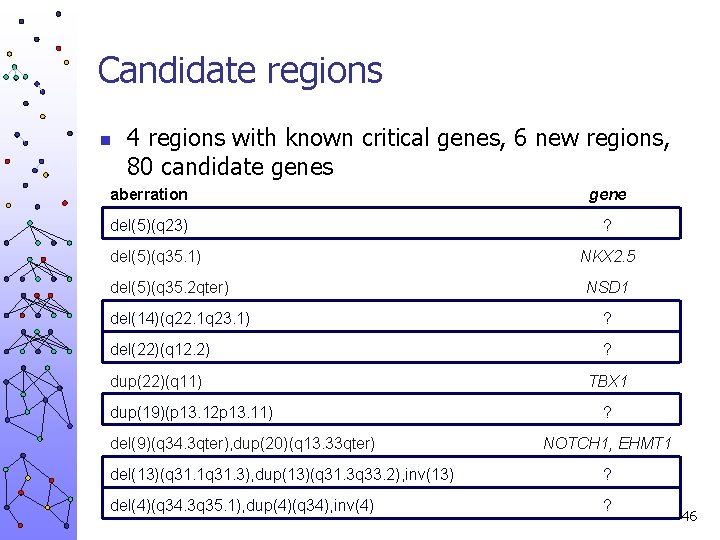
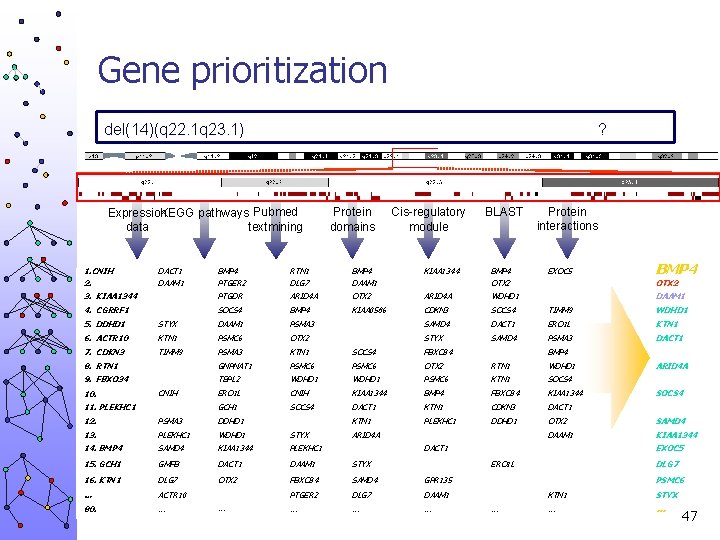
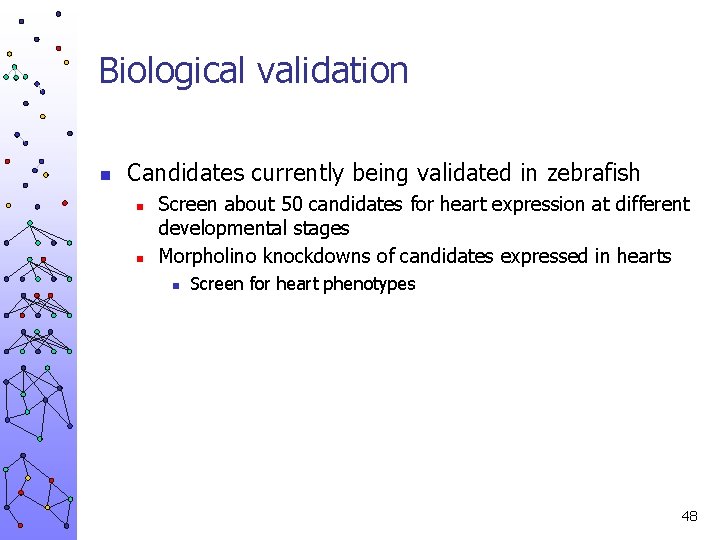
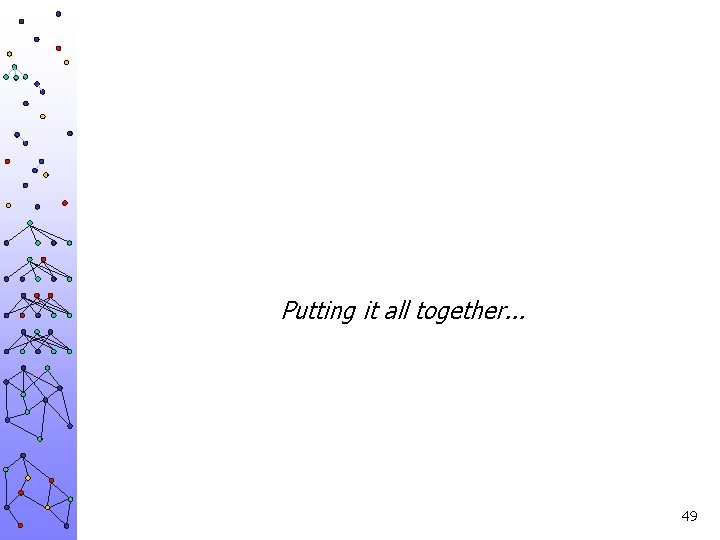
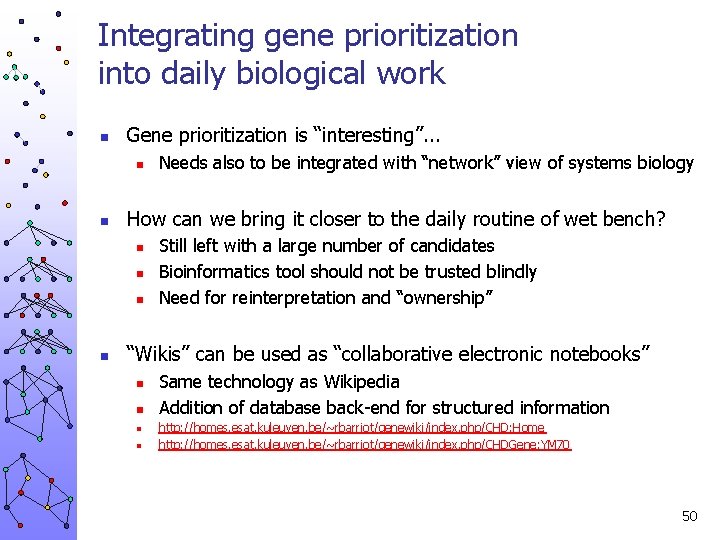
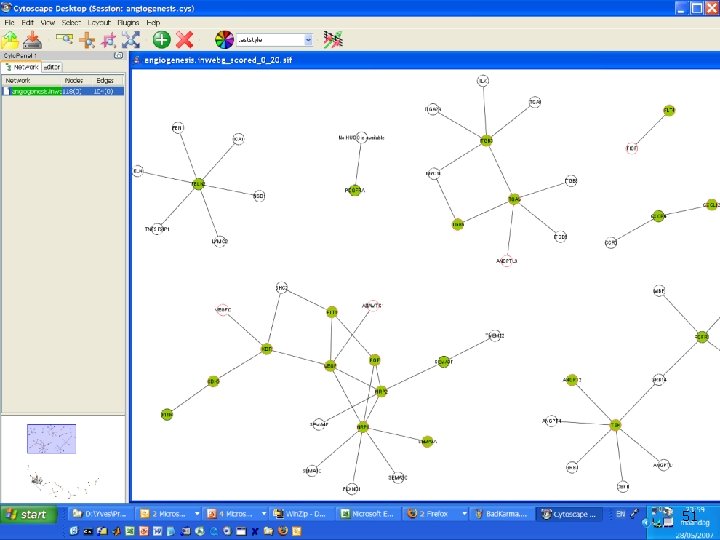
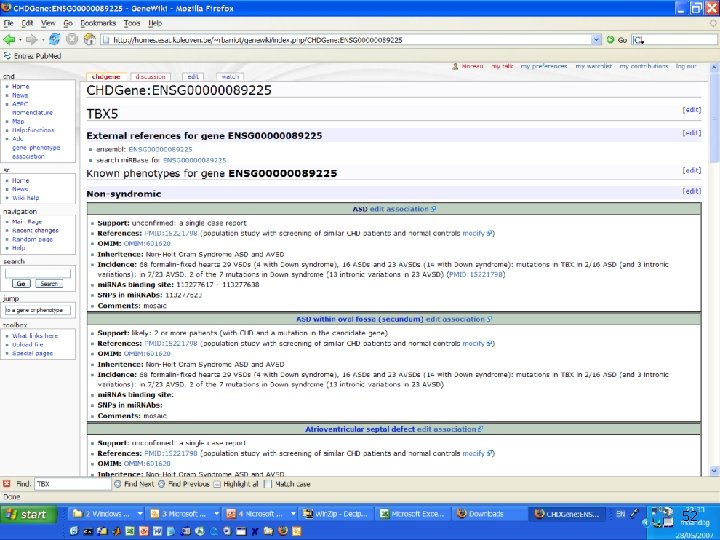
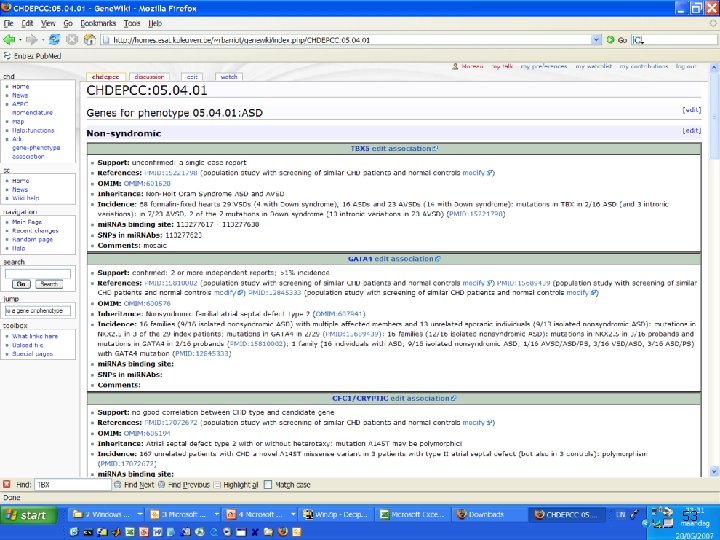
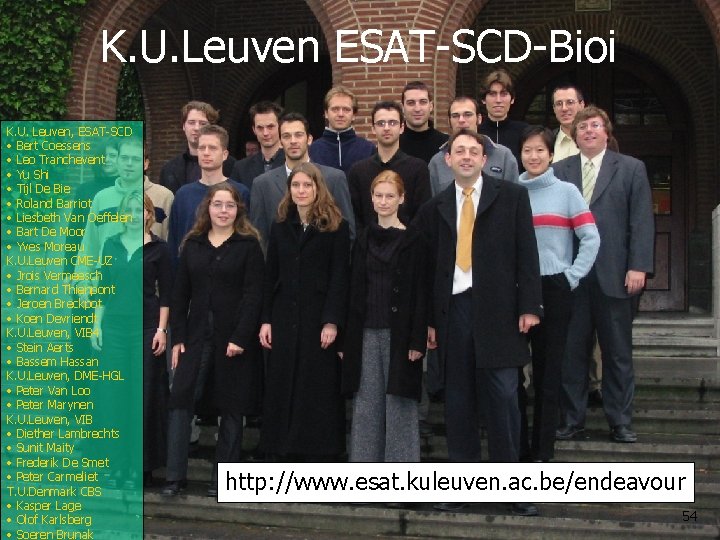
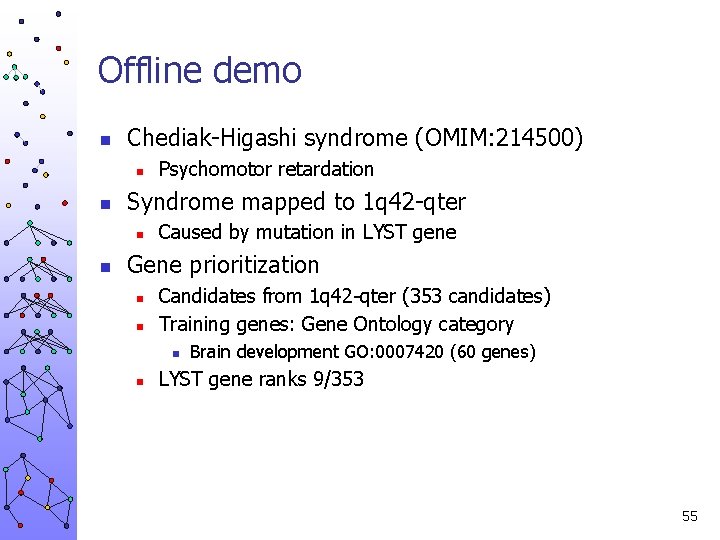
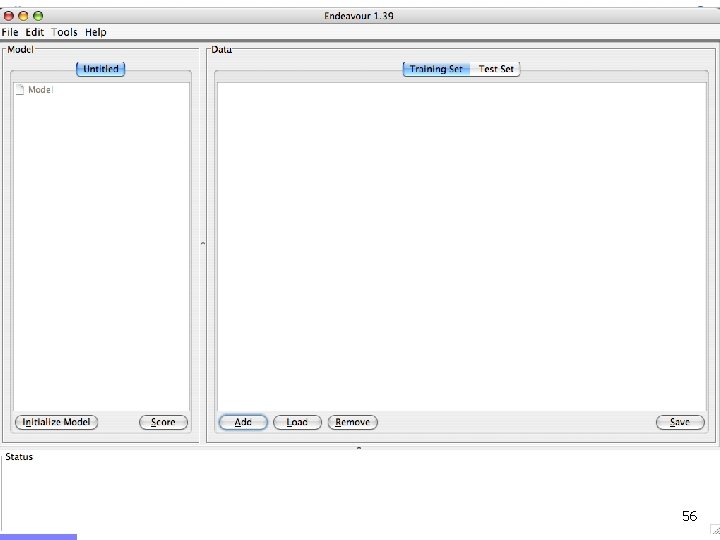
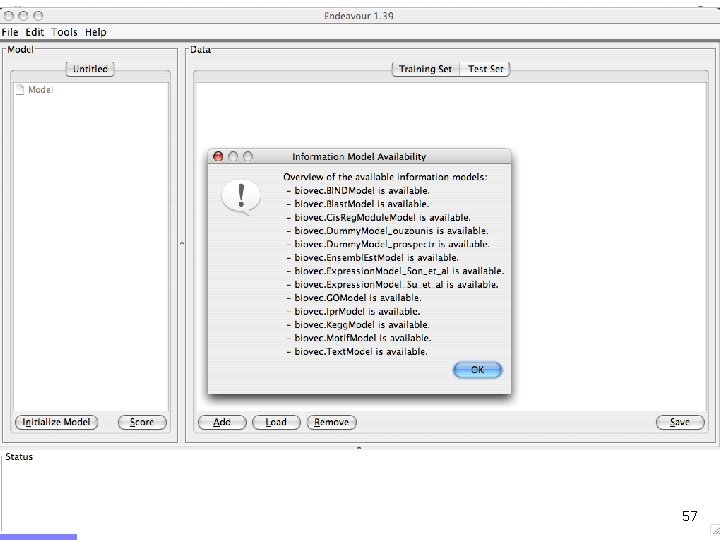
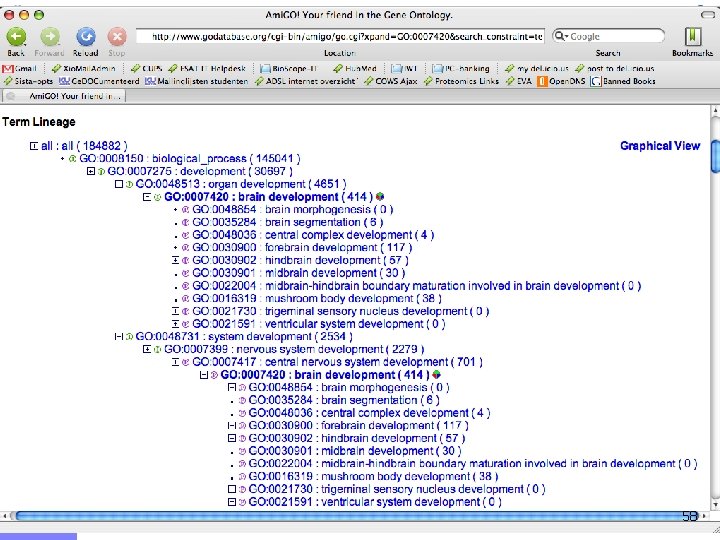
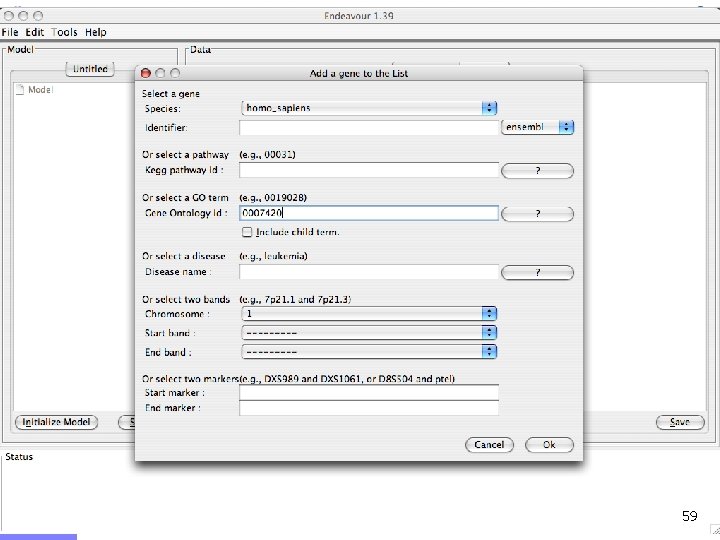
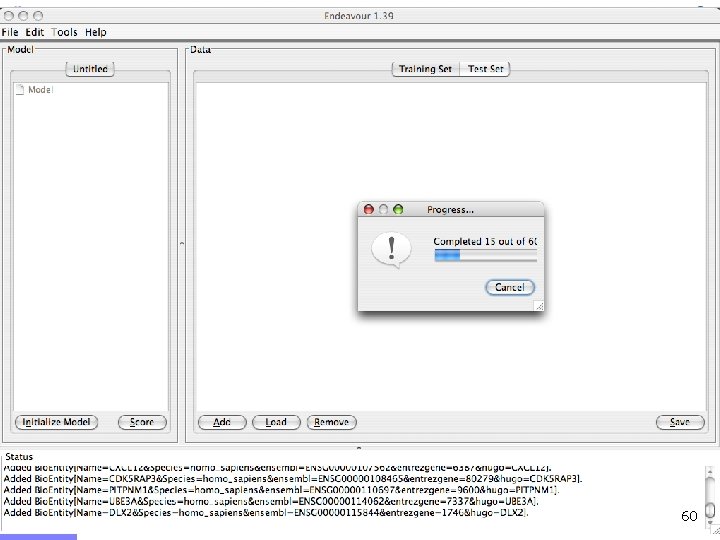
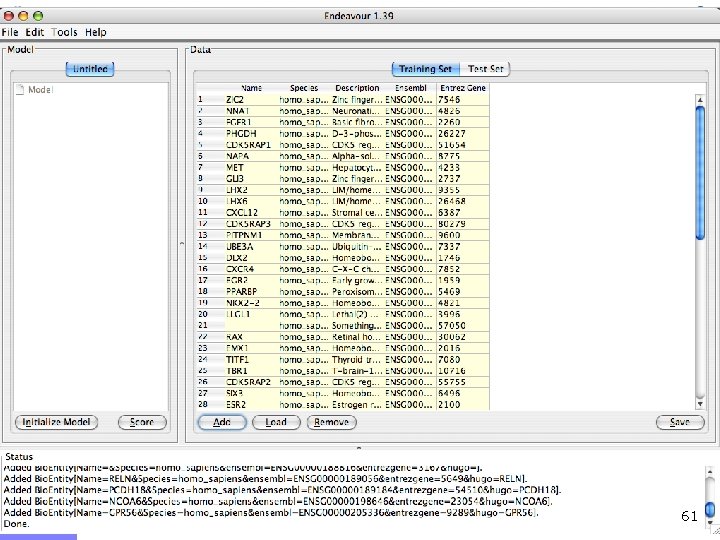
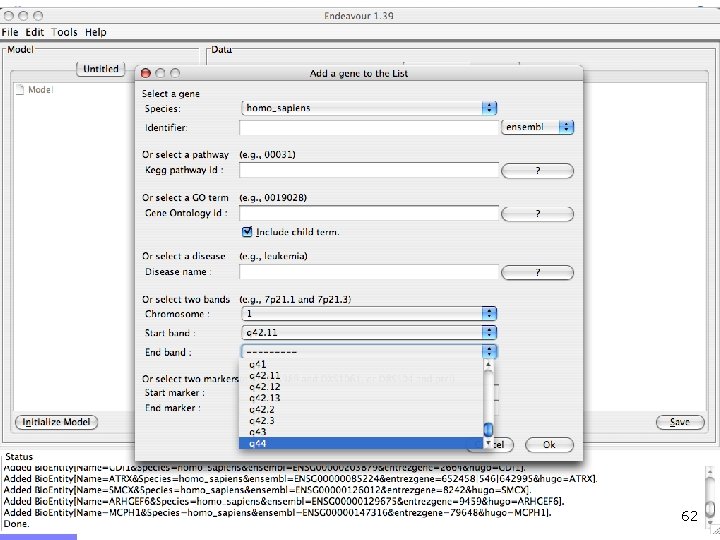
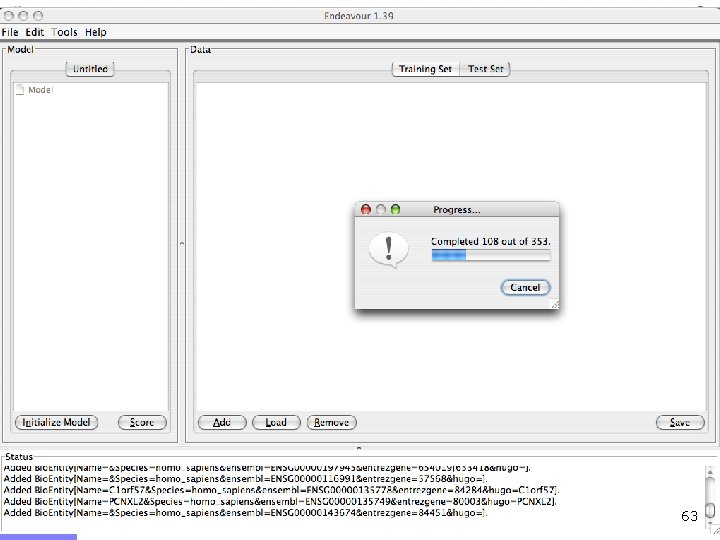
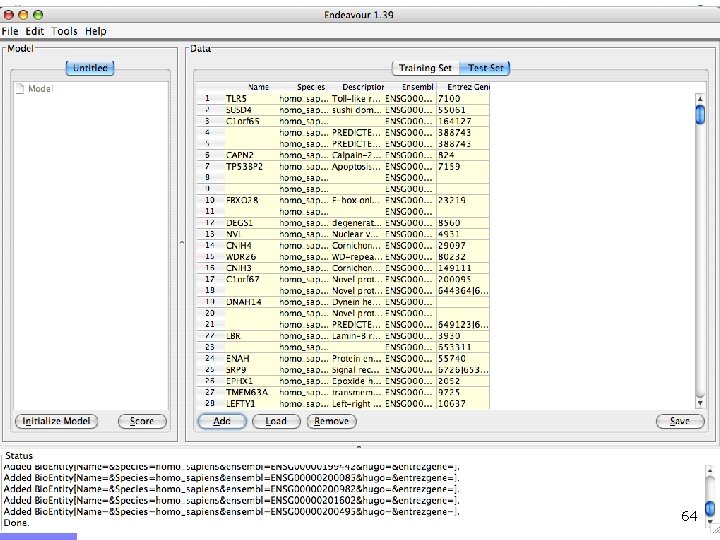
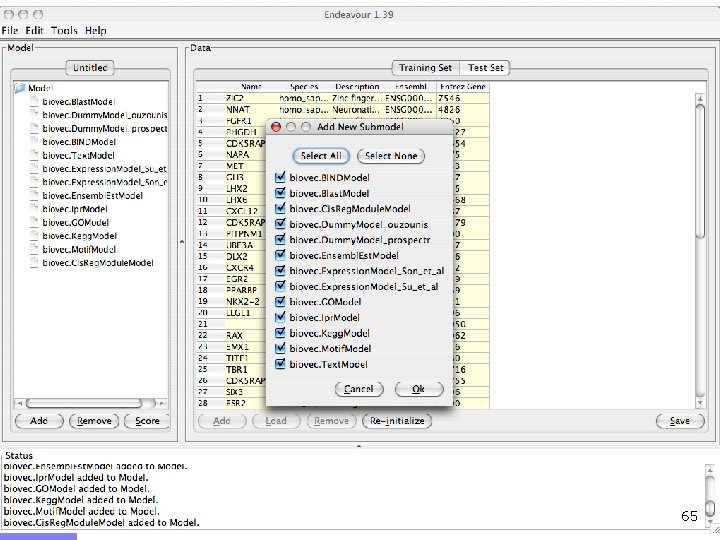
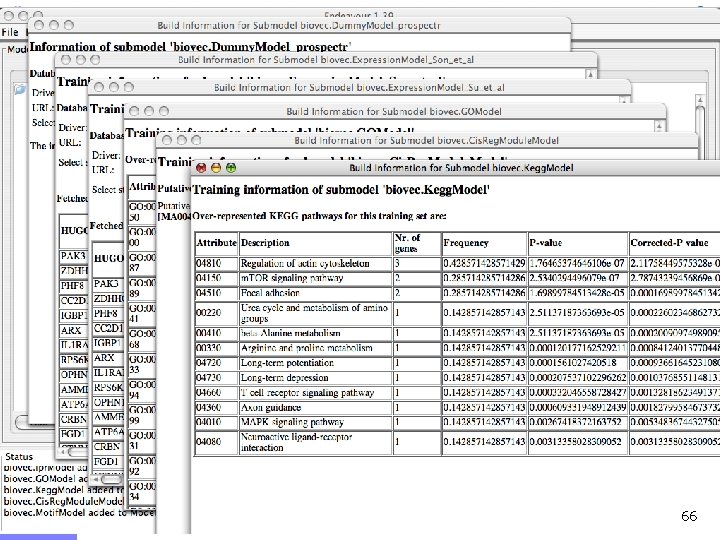
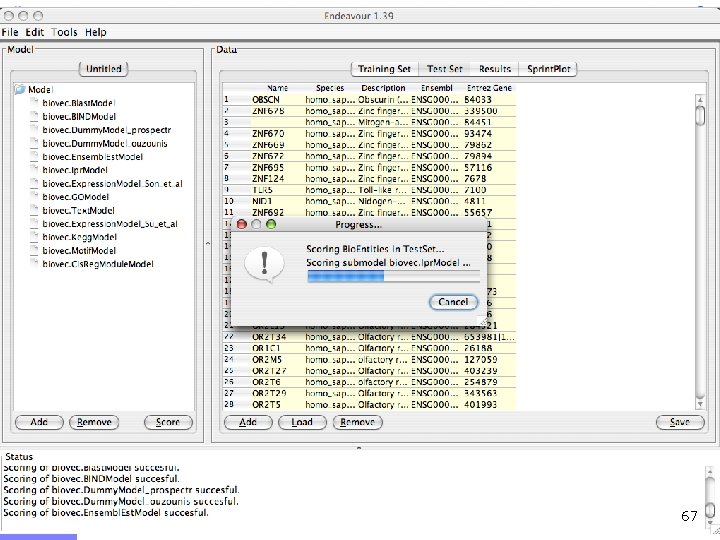
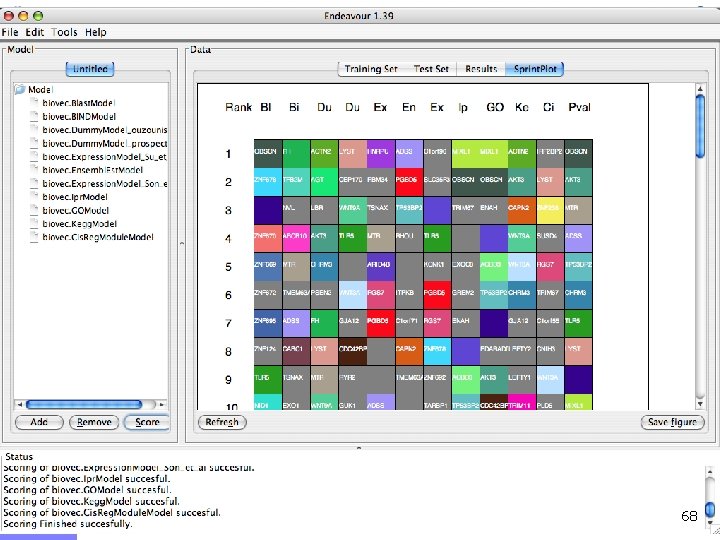
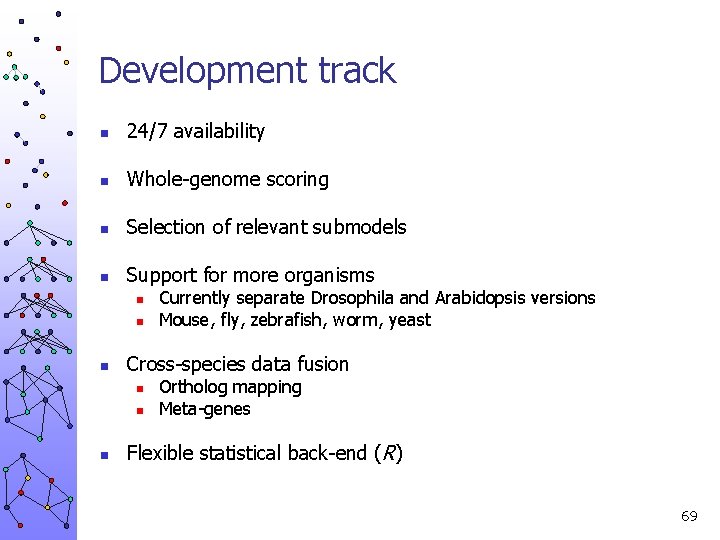
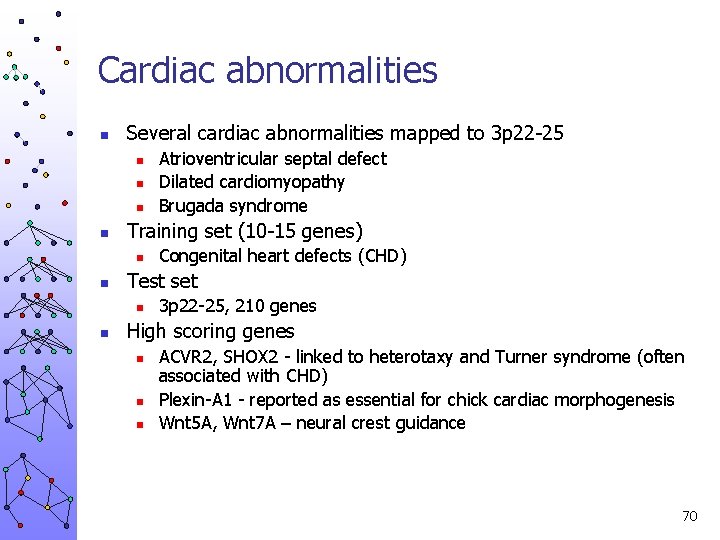
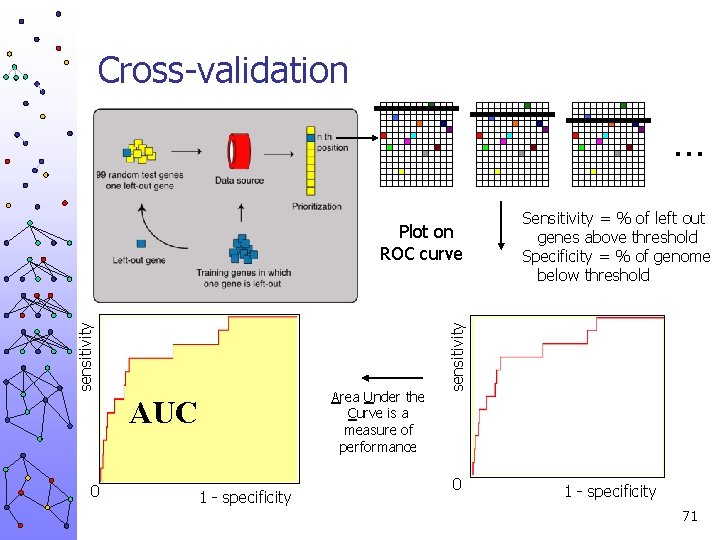
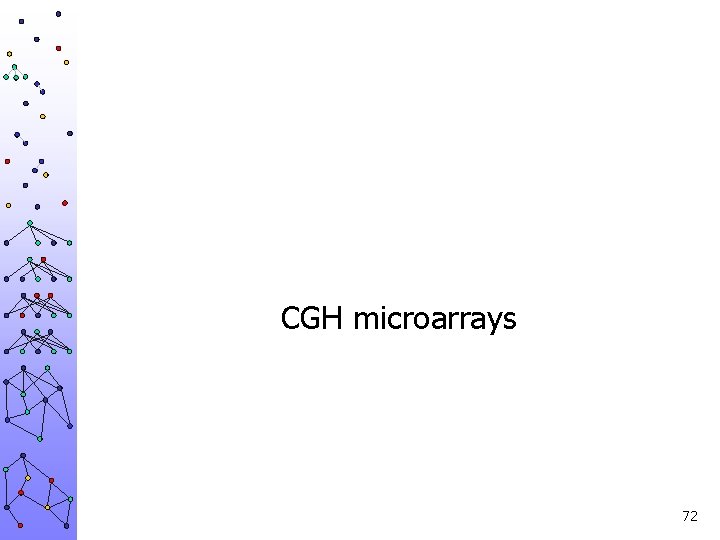
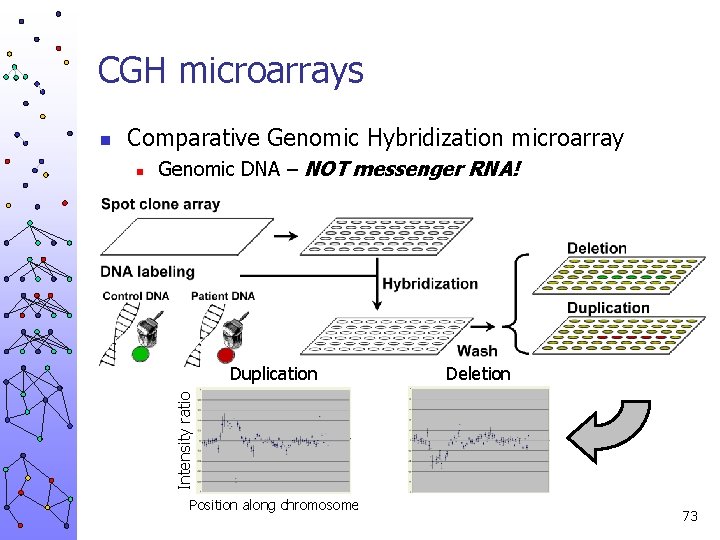
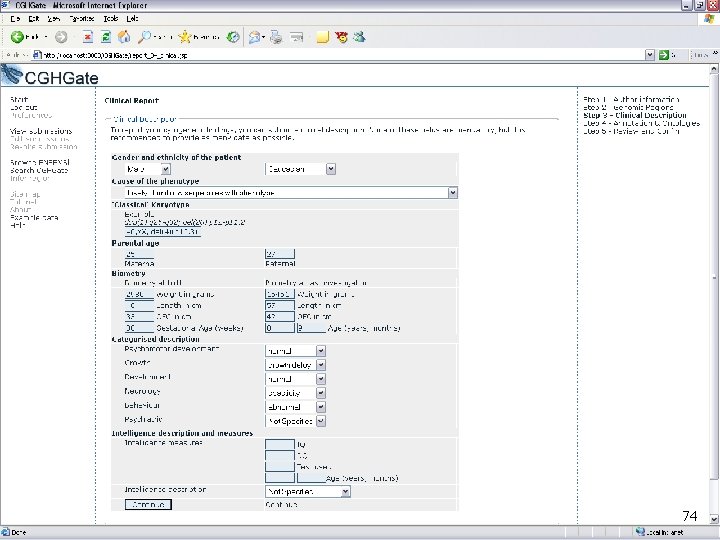
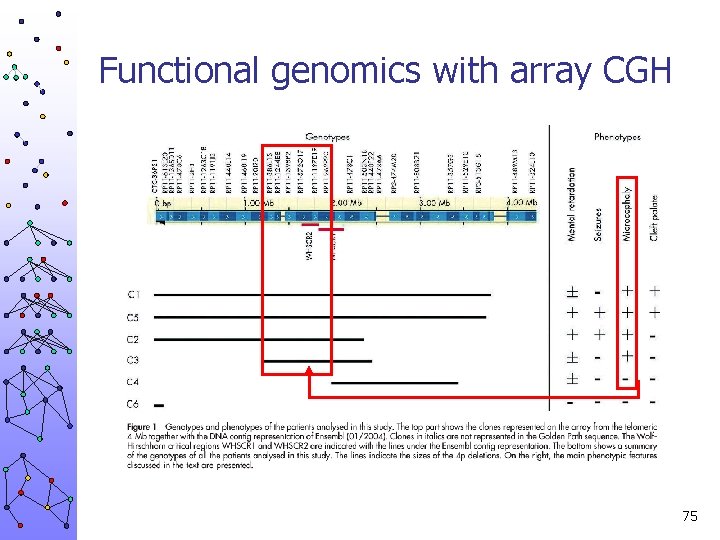
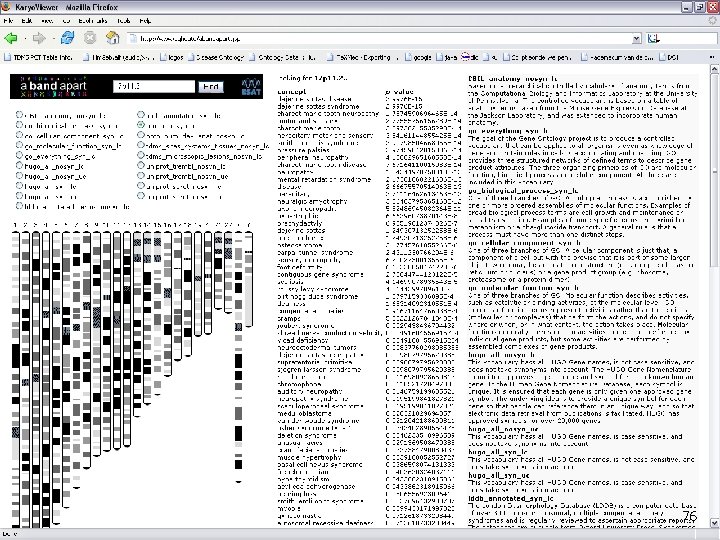
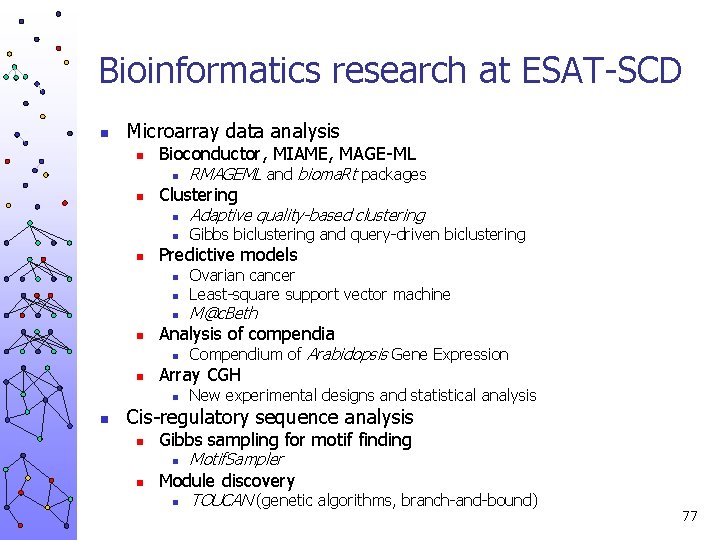
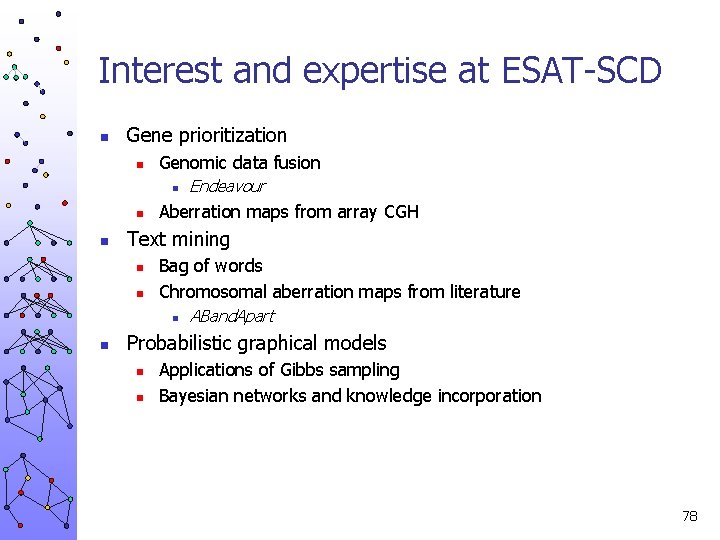
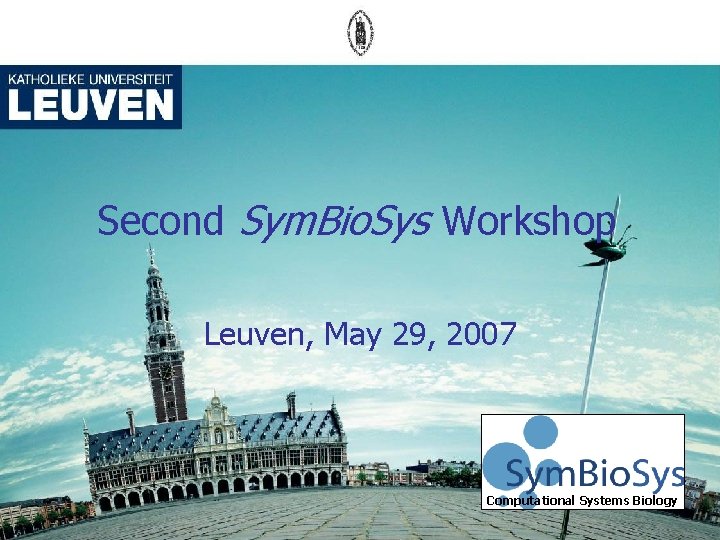
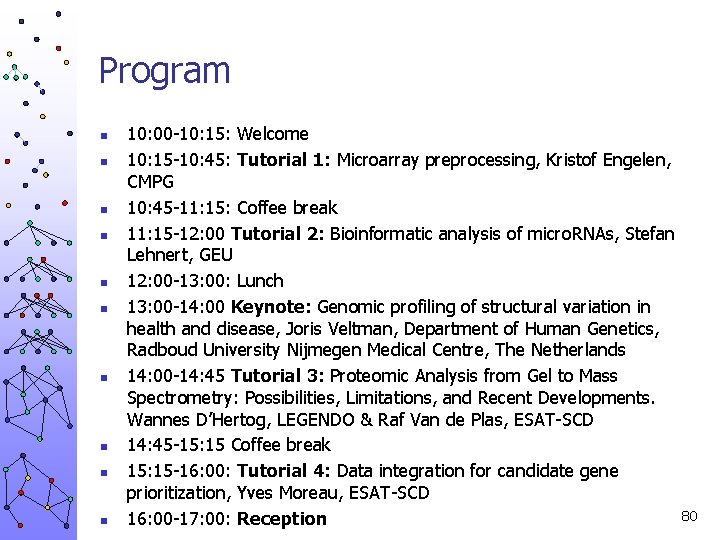
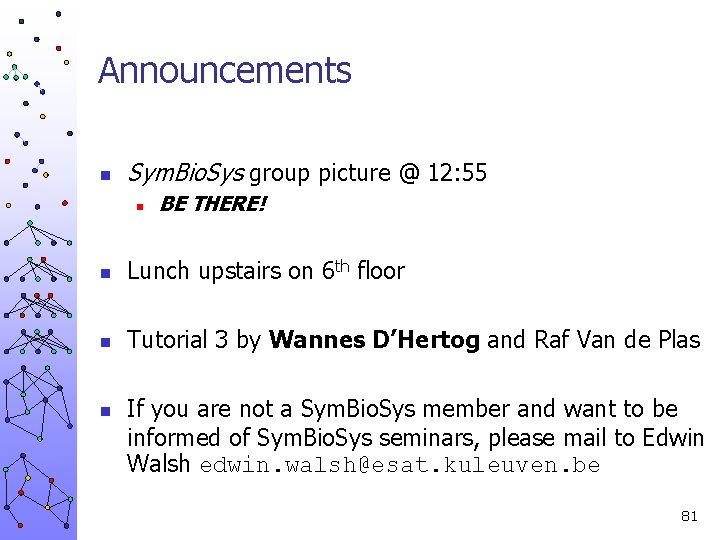
- Slides: 81
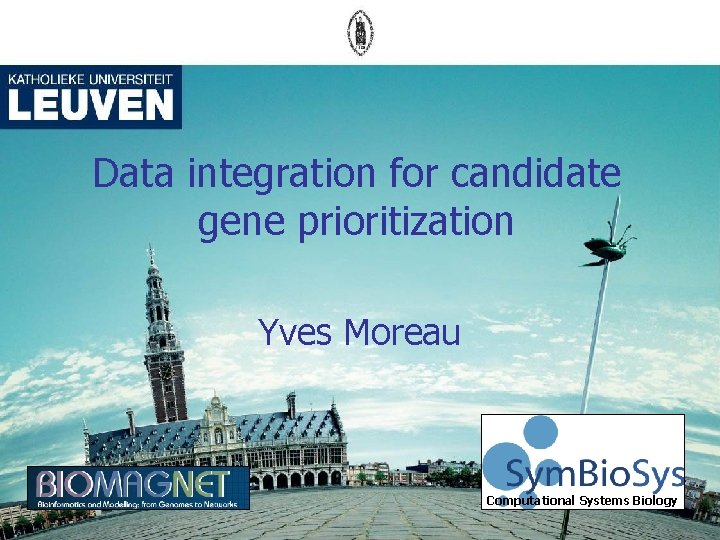
Data integration for candidate gene prioritization Yves Moreau Computational Systems Biology
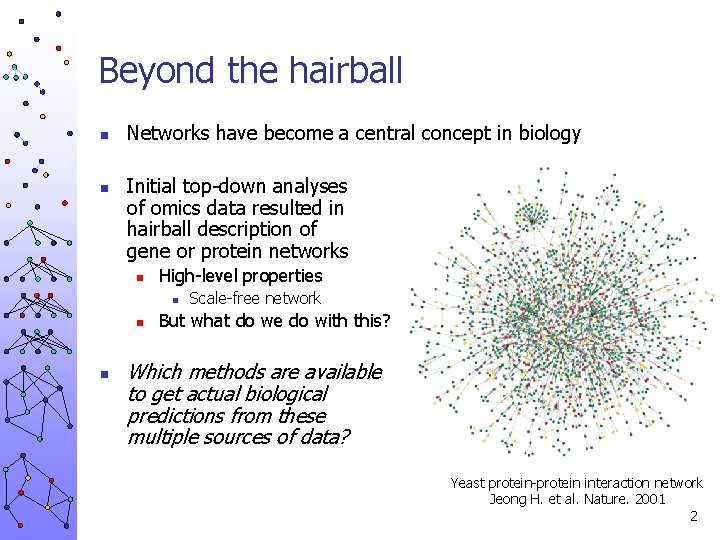
Beyond the hairball n n Networks have become a central concept in biology Initial top-down analyses of omics data resulted in hairball description of gene or protein networks n High-level properties n n n Scale-free network But what do we do with this? Which methods are available to get actual biological predictions from these multiple sources of data? Yeast protein-protein interaction network Jeong H. et al. Nature. 2001 2
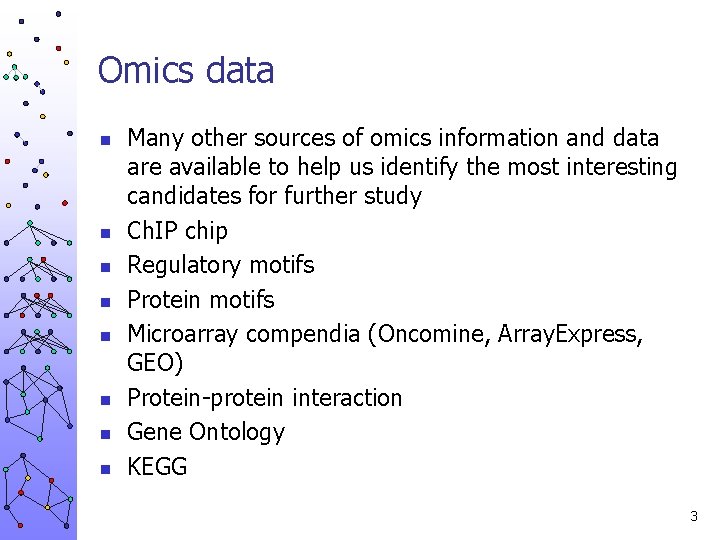
Omics data n n n n Many other sources of omics information and data are available to help us identify the most interesting candidates for further study Ch. IP chip Regulatory motifs Protein motifs Microarray compendia (Oncomine, Array. Express, GEO) Protein-protein interaction Gene Ontology KEGG 3
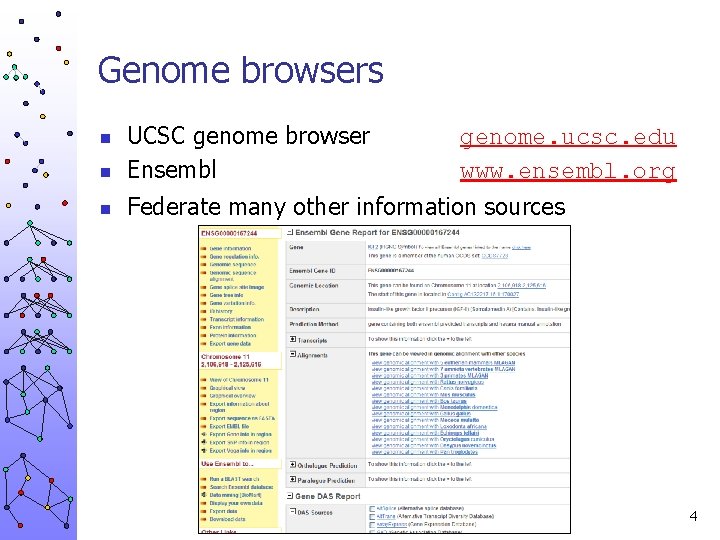
Genome browsers n UCSC genome browser Ensembl n Federate many other information sources n genome. ucsc. edu www. ensembl. org 4
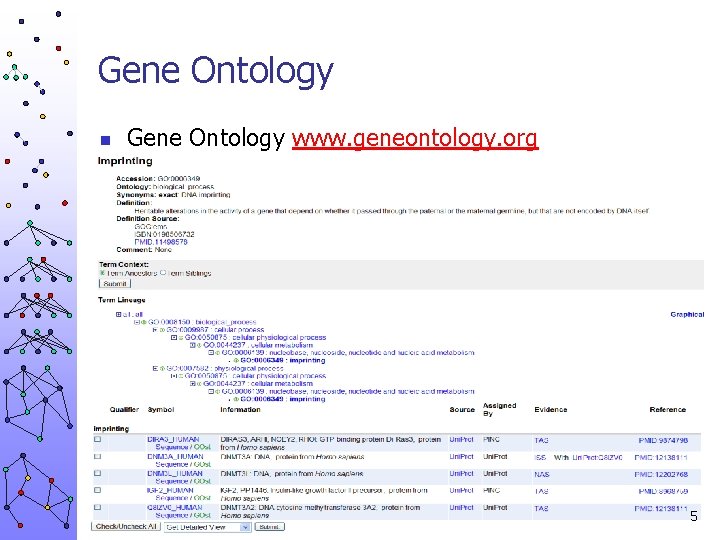
Gene Ontology n Gene Ontology www. geneontology. org 5
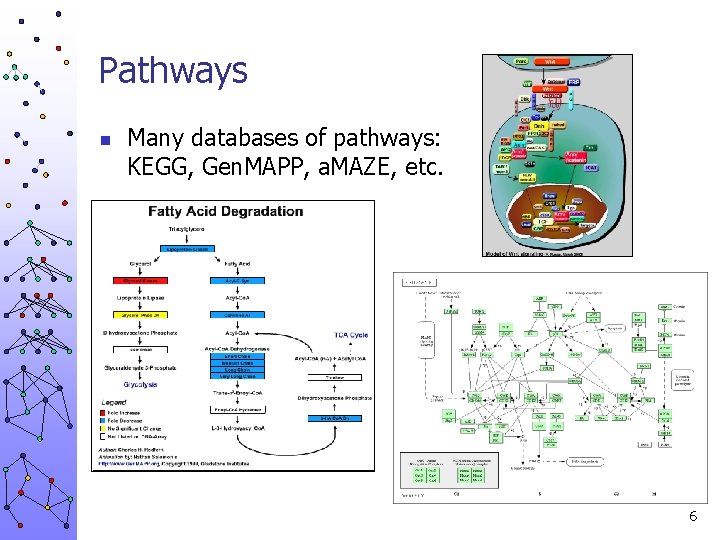
Pathways n Many databases of pathways: KEGG, Gen. MAPP, a. MAZE, etc. 6
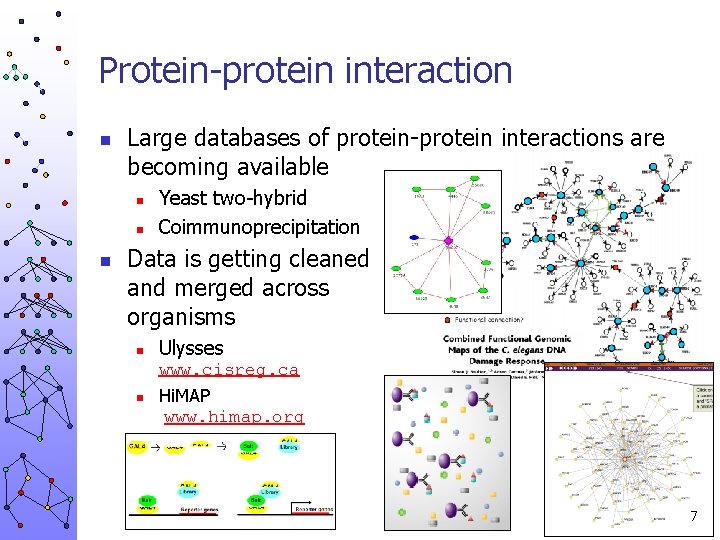
Protein-protein interaction n Large databases of protein-protein interactions are becoming available n n n Yeast two-hybrid Coimmunoprecipitation Data is getting cleaned and merged across organisms n Ulysses www. cisreg. ca n Hi. MAP www. himap. org 7
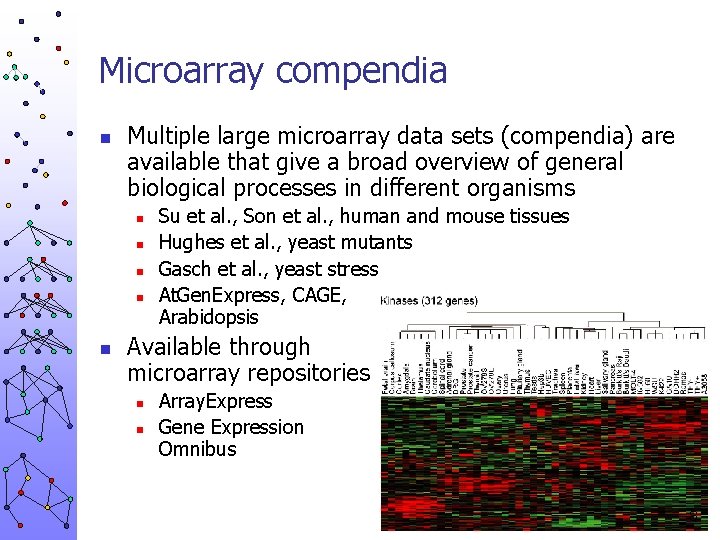
Microarray compendia n Multiple large microarray data sets (compendia) are available that give a broad overview of general biological processes in different organisms n n n Su et al. , Son et al. , human and mouse tissues Hughes et al. , yeast mutants Gasch et al. , yeast stress At. Gen. Express, CAGE, Arabidopsis Available through microarray repositories n n Array. Express Gene Expression Omnibus 8
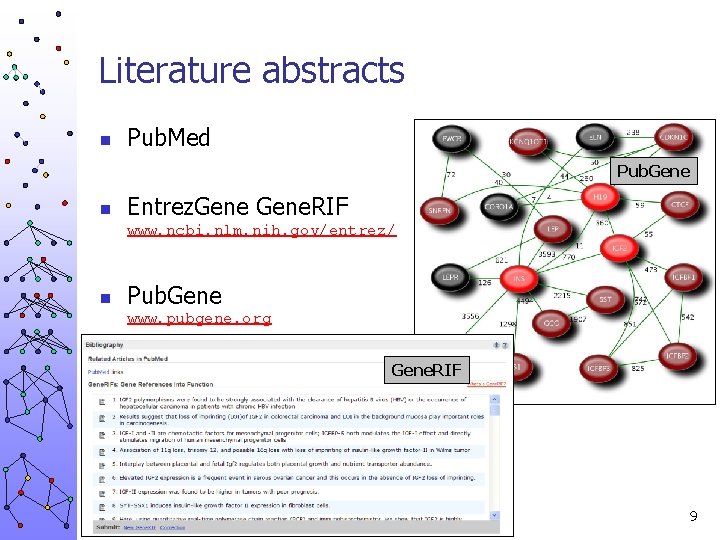
Literature abstracts n Pub. Med Pub. Gene n Entrez. Gene. RIF www. ncbi. nlm. nih. gov/entrez/ n Pub. Gene www. pubgene. org Gene. RIF 9
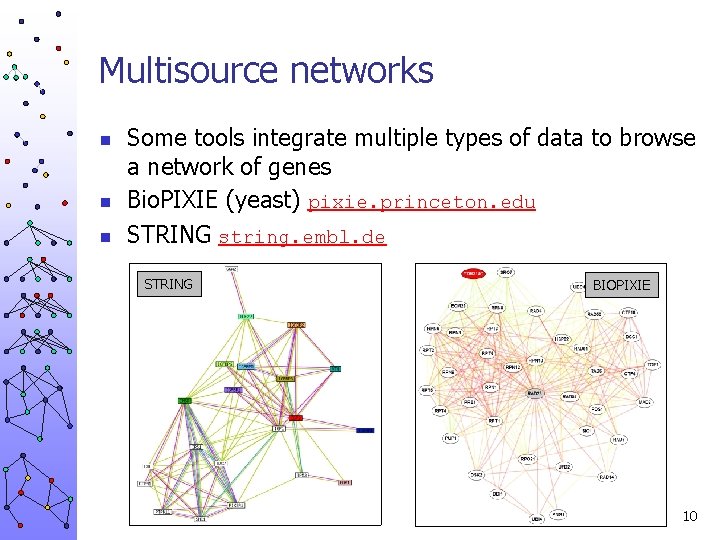
Multisource networks n n n Some tools integrate multiple types of data to browse a network of genes Bio. PIXIE (yeast) pixie. princeton. edu STRING string. embl. de STRING BIOPIXIE 10
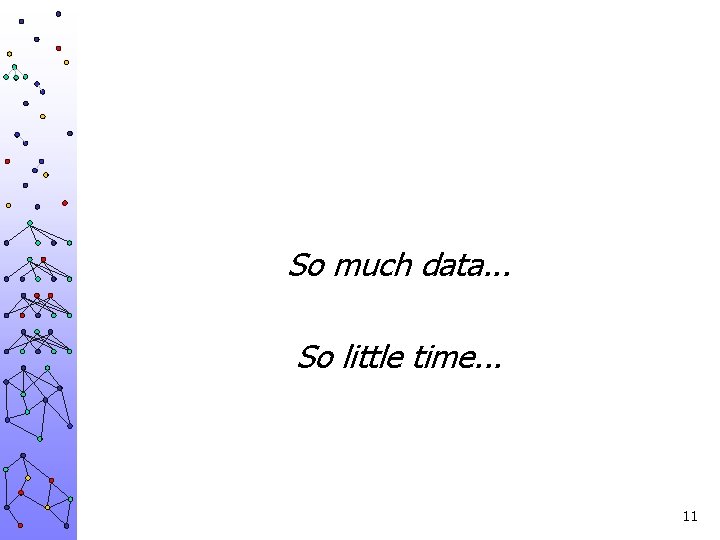
So much data. . . So little time. . . 11
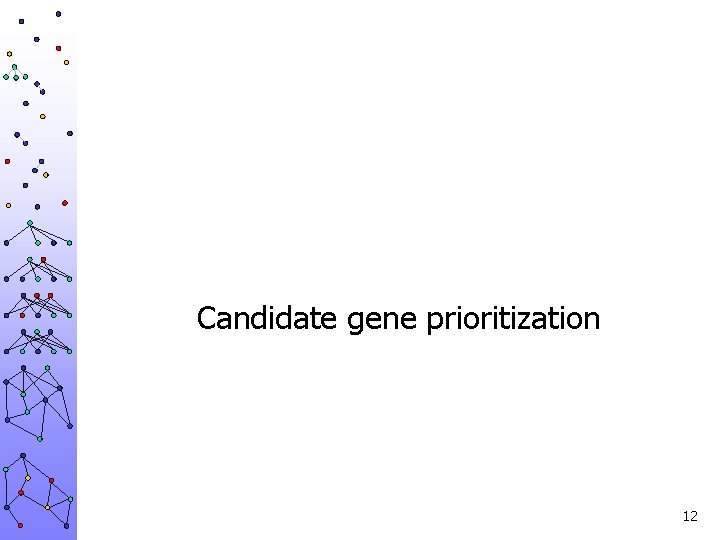
Candidate gene prioritization 12
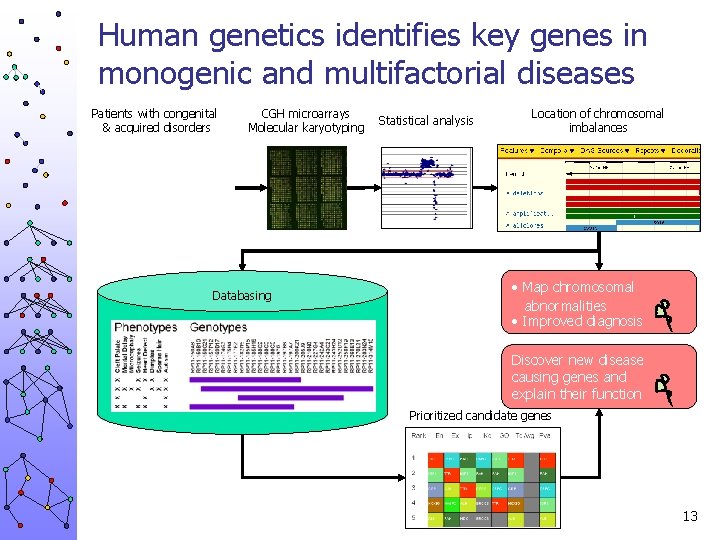
Human genetics identifies key genes in monogenic and multifactorial diseases Patients with congenital & acquired disorders CGH microarrays Molecular karyotyping Databasing Statistical analysis Location of chromosomal imbalances • Map chromosomal abnormalities • Improved diagnosis Discover new disease causing genes and explain their function Prioritized candidate genes 13
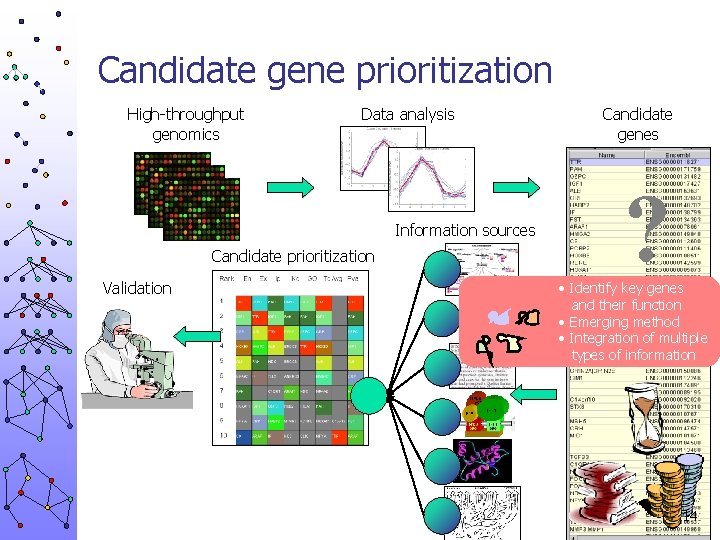
Candidate gene prioritization High-throughput genomics Data analysis Information sources Candidate prioritization Validation Candidate genes ? • Identify key genes and their function • Emerging method • Integration of multiple types of information 14
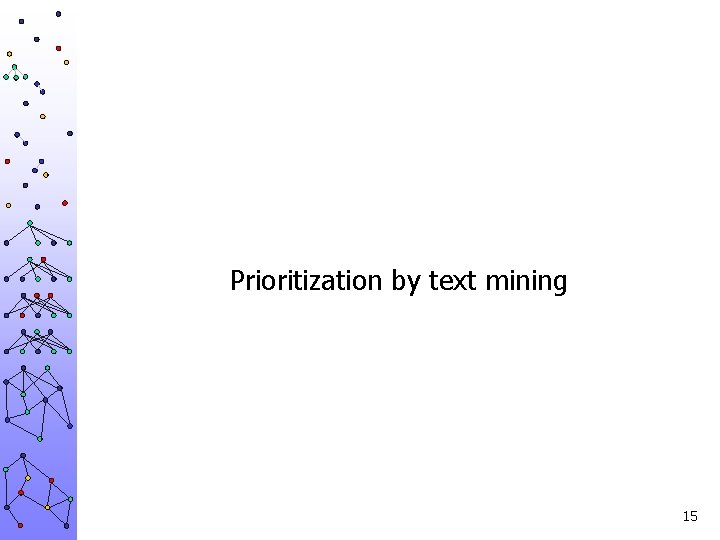
Prioritization by text mining 15
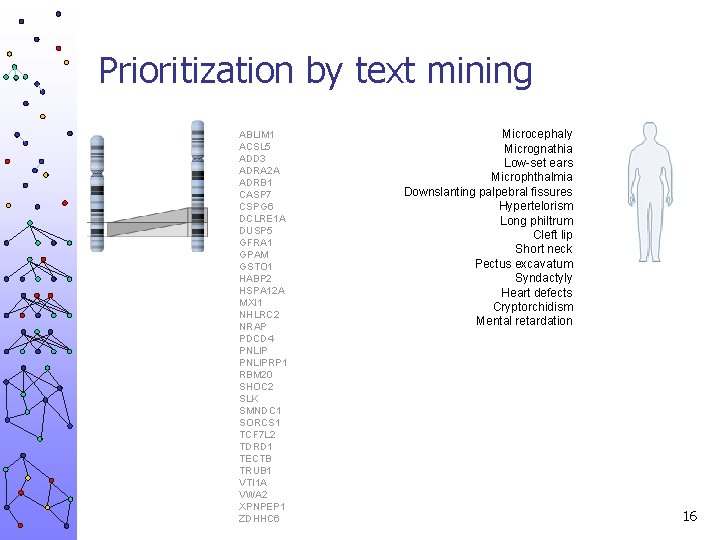
Prioritization by text mining ABLIM 1 ACSL 5 ADD 3 ADRA 2 A ADRB 1 CASP 7 CSPG 6 DCLRE 1 A DUSP 5 GFRA 1 GPAM GSTO 1 HABP 2 HSPA 12 A MXI 1 NHLRC 2 NRAP PDCD 4 PNLIPRP 1 RBM 20 SHOC 2 SLK SMNDC 1 SORCS 1 TCF 7 L 2 TDRD 1 TECTB TRUB 1 VTI 1 A VWA 2 XPNPEP 1 ZDHHC 6 Microcephaly Micrognathia Low-set ears Microphthalmia Downslanting palpebral fissures Hypertelorism Long philtrum Cleft lip Short neck Pectus excavatum Syndactyly Heart defects Cryptorchidism Mental retardation 16
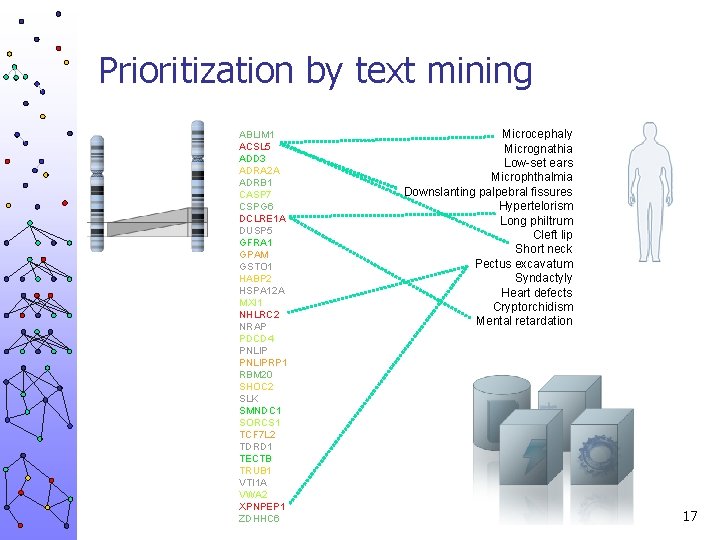
Prioritization by text mining ABLIM 1 ACSL 5 ADD 3 ADRA 2 A ADRB 1 CASP 7 CSPG 6 DCLRE 1 A DUSP 5 GFRA 1 GPAM GSTO 1 HABP 2 HSPA 12 A MXI 1 NHLRC 2 NRAP PDCD 4 PNLIPRP 1 RBM 20 SHOC 2 SLK SMNDC 1 SORCS 1 TCF 7 L 2 TDRD 1 TECTB TRUB 1 VTI 1 A VWA 2 XPNPEP 1 ZDHHC 6 Microcephaly Micrognathia Low-set ears Microphthalmia Downslanting palpebral fissures Hypertelorism Long philtrum Cleft lip Short neck Pectus excavatum Syndactyly Heart defects Cryptorchidism Mental retardation 17
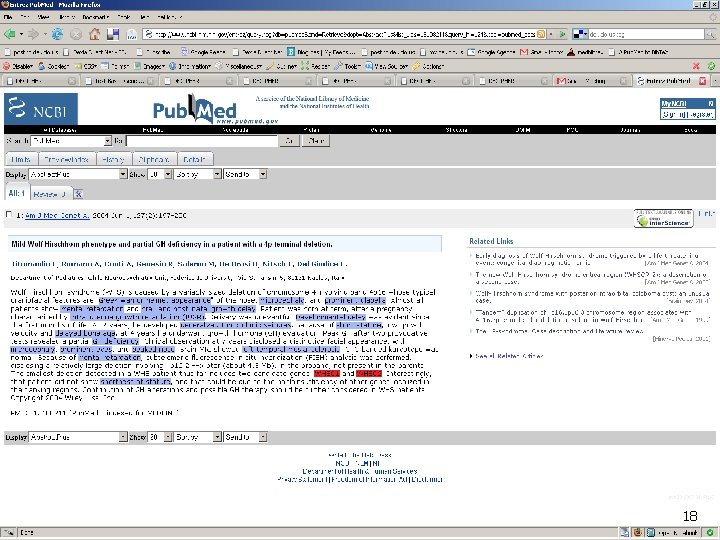
18
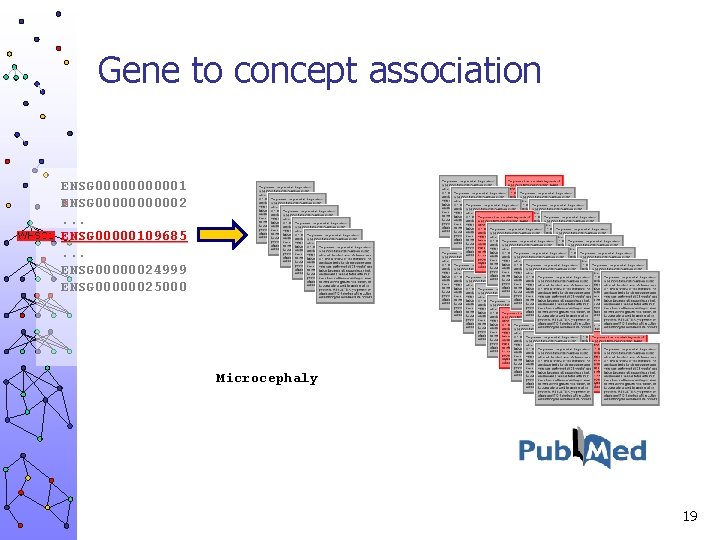
Gene to concept association ENSG 000001 ENSG 000002. . . ENSG 00000109685. . . ENSG 00000024999 ENSG 00000025000 Microcephaly 19
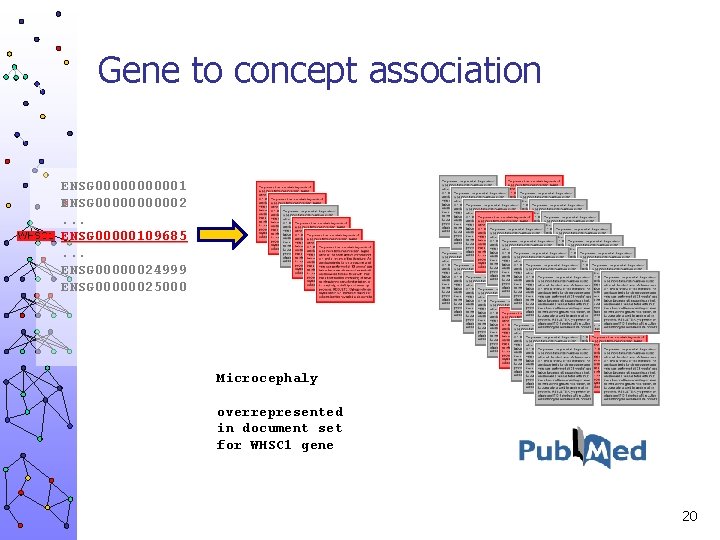
Gene to concept association ENSG 000001 ENSG 000002. . . ENSG 00000109685. . . ENSG 00000024999 ENSG 00000025000 Microcephaly overrepresented in document set for WHSC 1 gene 20
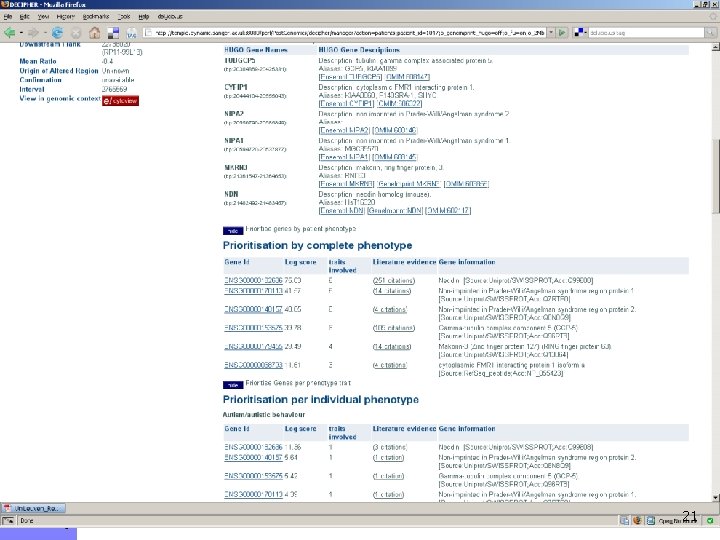
21
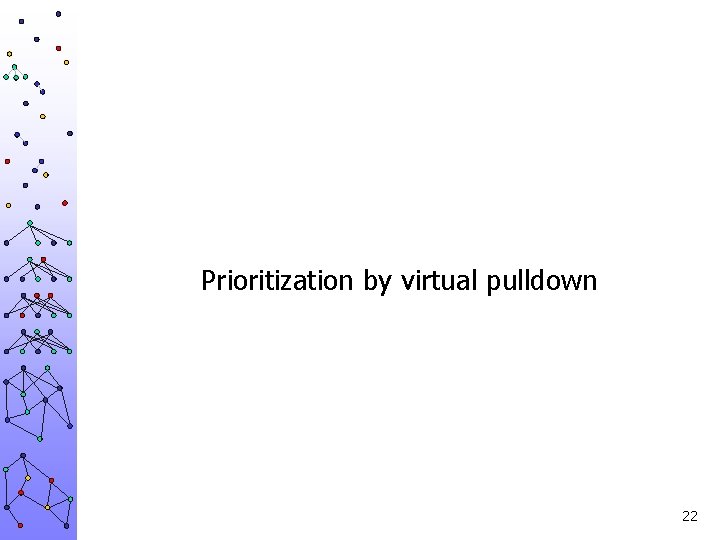
Prioritization by virtual pulldown 22
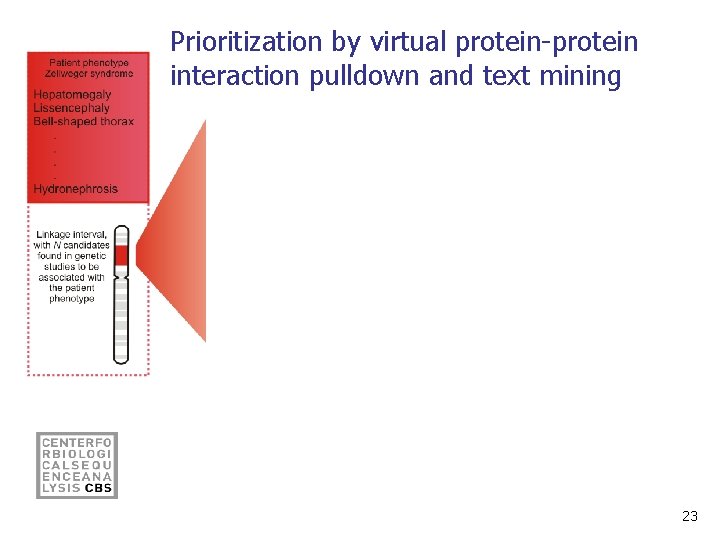
Prioritization by virtual protein-protein interaction pulldown and text mining 23
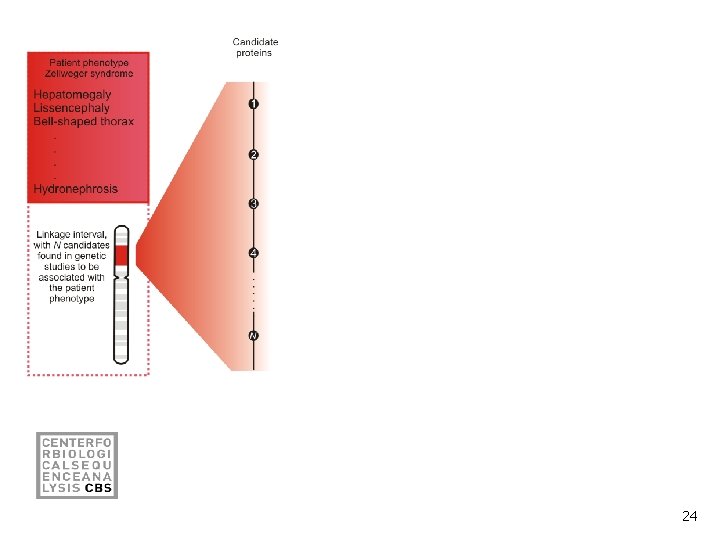
24
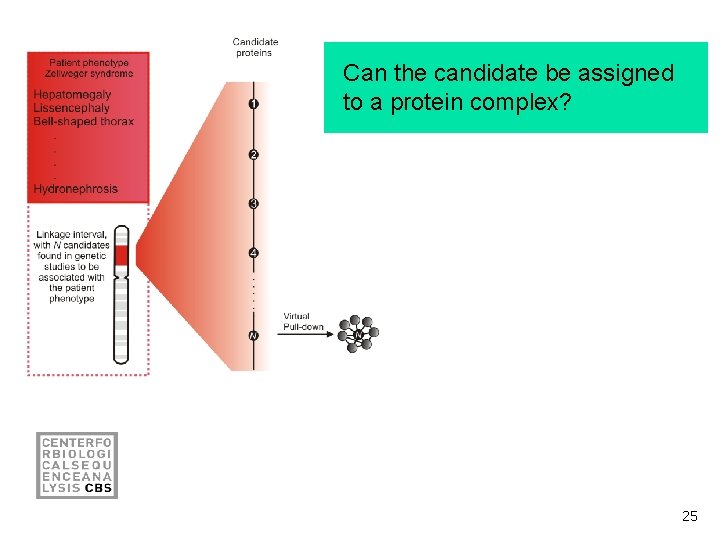
Can the candidate be assigned to a protein complex? 25
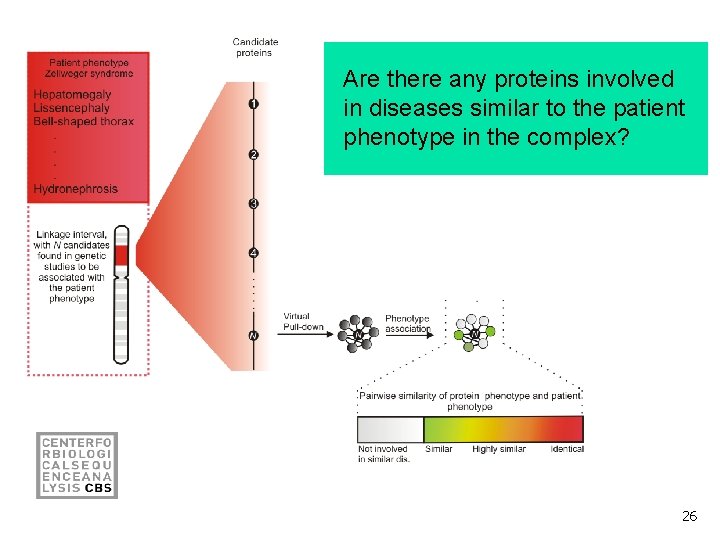
Are there any proteins involved in diseases similar to the patient phenotype in the complex? 26
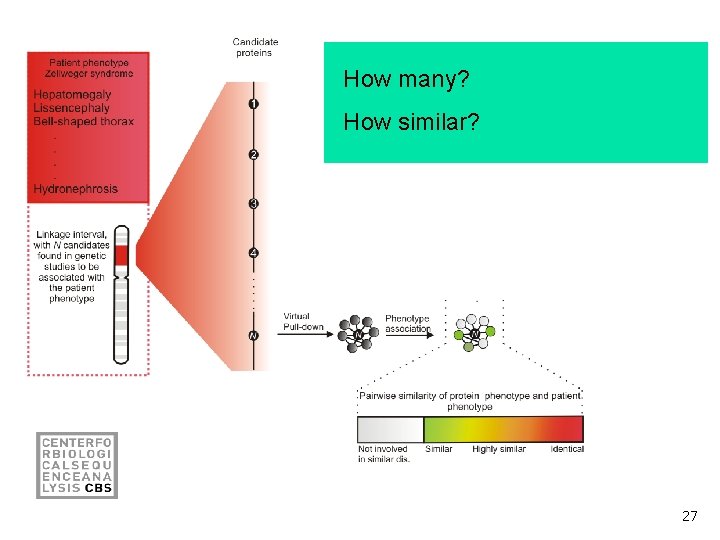
How many? How similar? 27
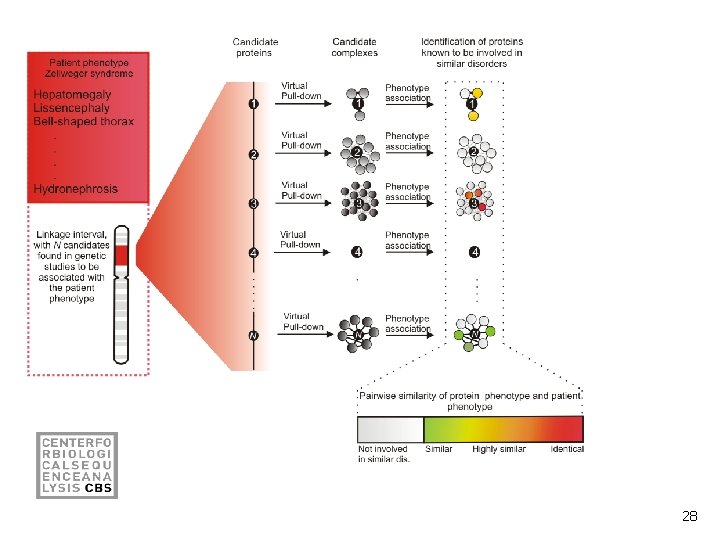
28
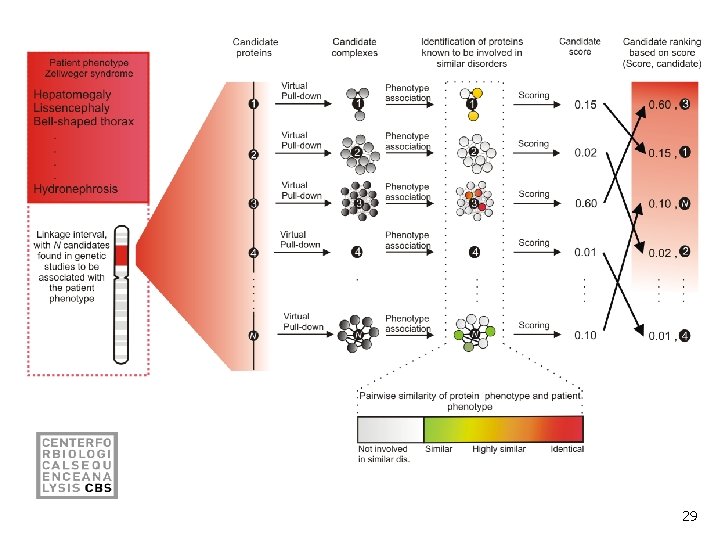
29
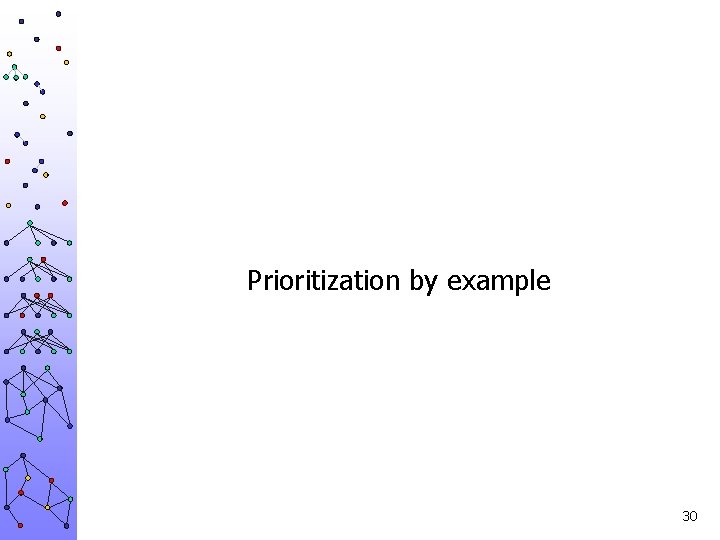
Prioritization by example 30
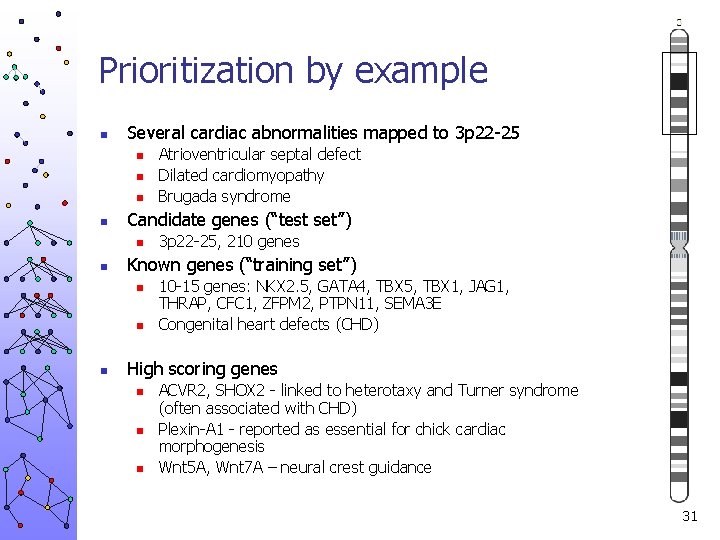
Prioritization by example n Several cardiac abnormalities mapped to 3 p 22 -25 n n Candidate genes (“test set”) n n 3 p 22 -25, 210 genes Known genes (“training set”) n n n Atrioventricular septal defect Dilated cardiomyopathy Brugada syndrome 10 -15 genes: NKX 2. 5, GATA 4, TBX 5, TBX 1, JAG 1, THRAP, CFC 1, ZFPM 2, PTPN 11, SEMA 3 E Congenital heart defects (CHD) High scoring genes n n n ACVR 2, SHOX 2 - linked to heterotaxy and Turner syndrome (often associated with CHD) Plexin-A 1 - reported as essential for chick cardiac morphogenesis Wnt 5 A, Wnt 7 A – neural crest guidance 31
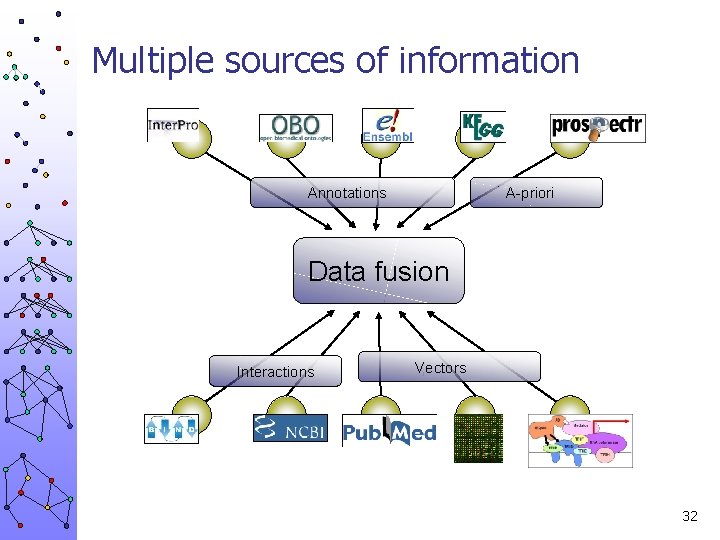
Multiple sources of information Annotations A-priori Data fusion Interactions Vectors 32
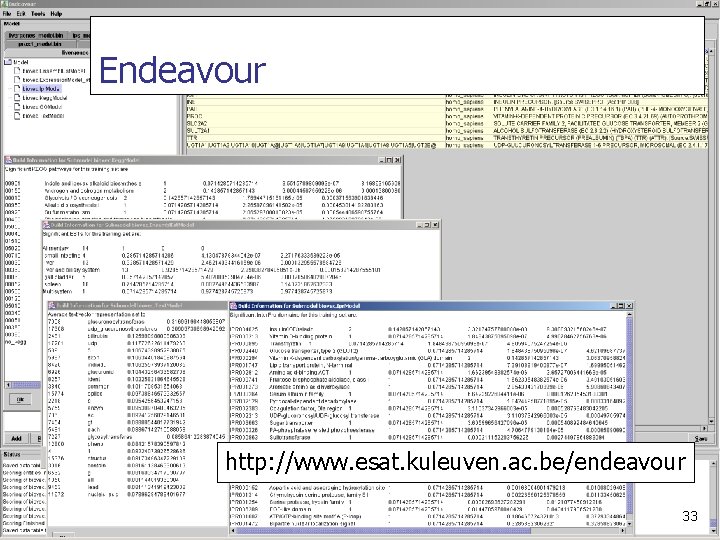
Endeavour http: //www. esat. kuleuven. ac. be/endeavour 33
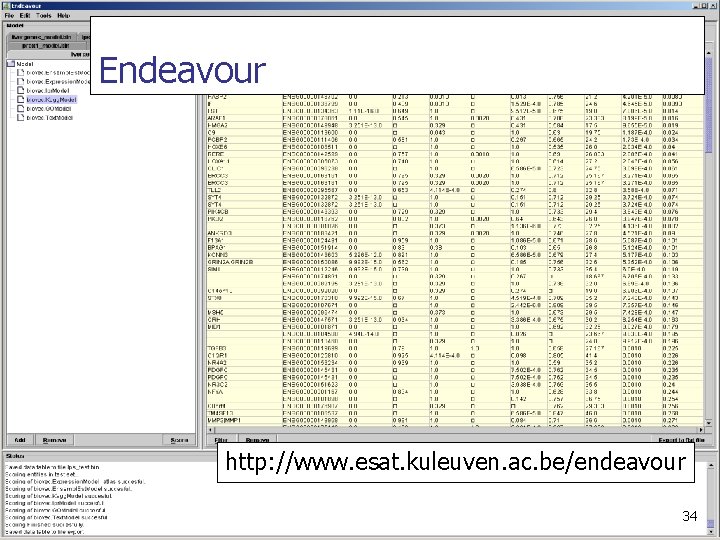
Endeavour http: //www. esat. kuleuven. ac. be/endeavour 34
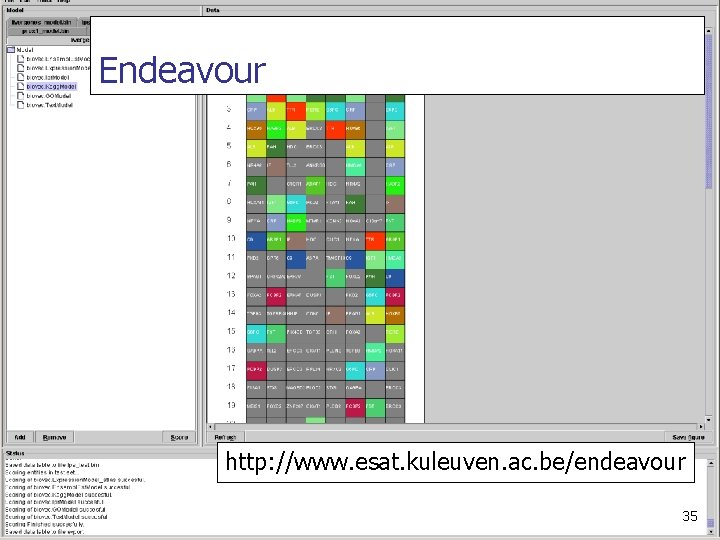
Endeavour http: //www. esat. kuleuven. ac. be/endeavour 35
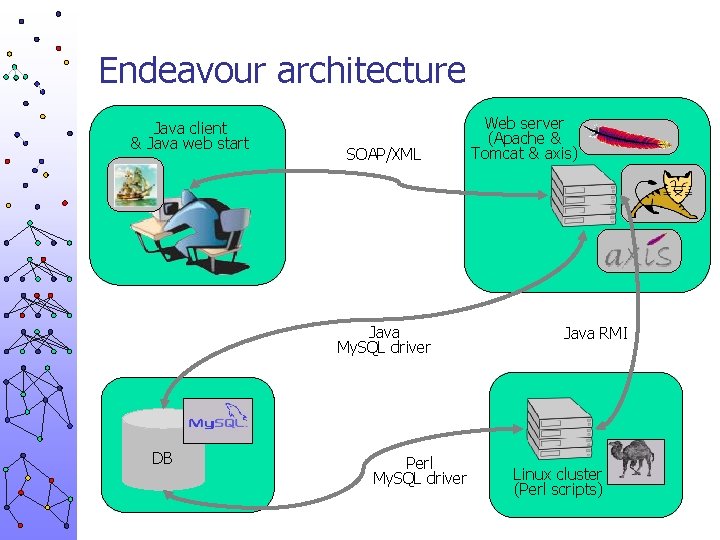
Endeavour architecture Java client & Java web start SOAP/XML Java My. SQL driver DB Perl My. SQL driver Web server (Apache & Tomcat & axis) Java RMI Linux cluster (Perl scripts)
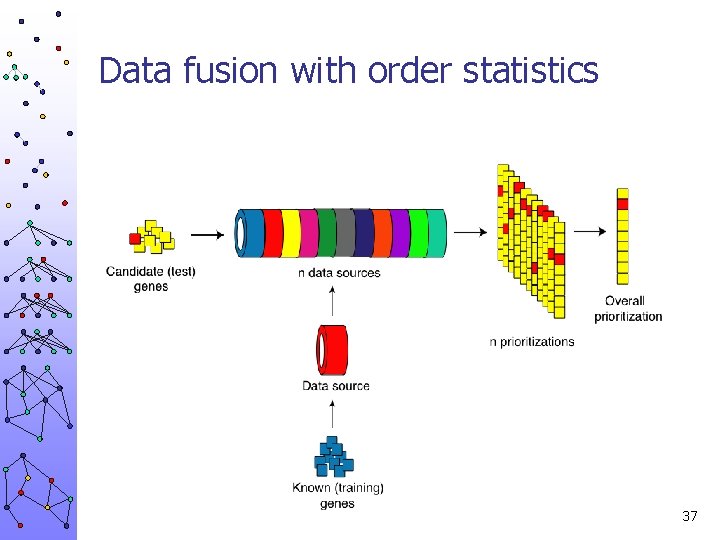
Data fusion with order statistics 37
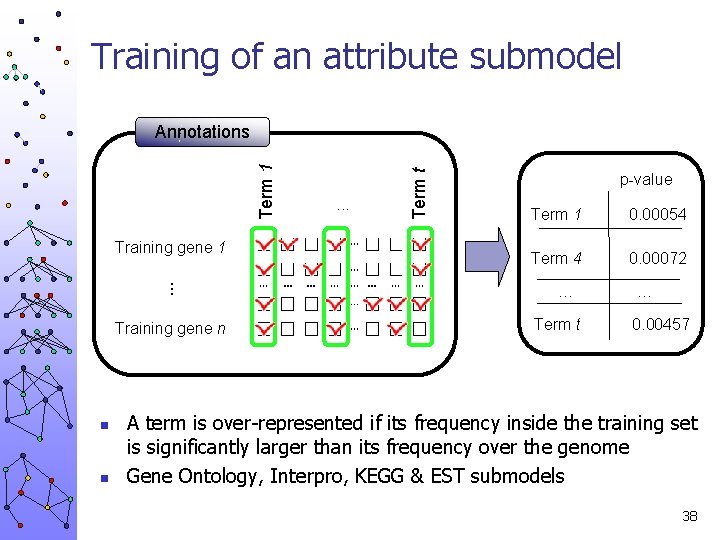
Training of an attribute submodel . . . Training gene 1 Training gene n n n . . . Term t Term 1 Annotations p-value Term 1 0. 00054 Term 4 0. 00072 … Term t … 0. 00457 A term is over-represented if its frequency inside the training set is significantly larger than its frequency over the genome Gene Ontology, Interpro, KEGG & EST submodels 38
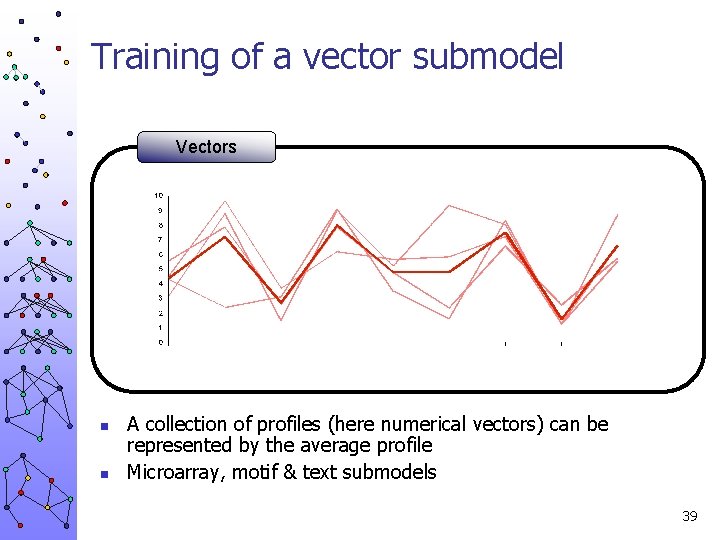
Training of a vector submodel Vectors n n A collection of profiles (here numerical vectors) can be represented by the average profile Microarray, motif & text submodels 39
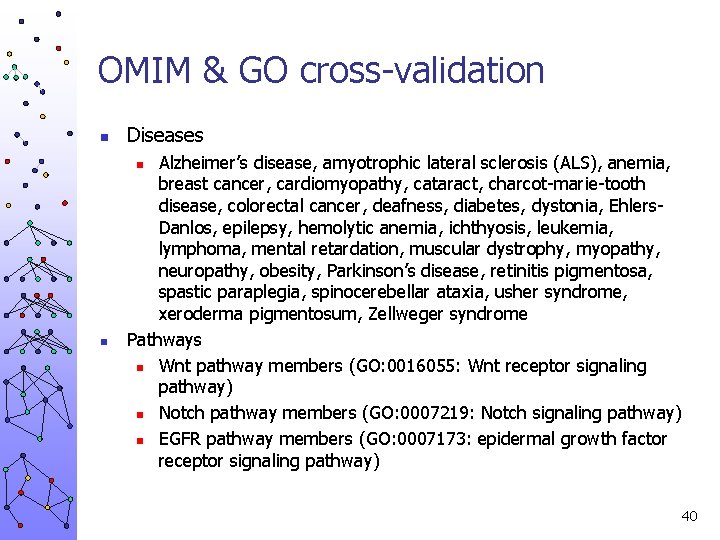
OMIM & GO cross-validation n Diseases Alzheimer’s disease, amyotrophic lateral sclerosis (ALS), anemia, breast cancer, cardiomyopathy, cataract, charcot-marie-tooth disease, colorectal cancer, deafness, diabetes, dystonia, Ehlers. Danlos, epilepsy, hemolytic anemia, ichthyosis, leukemia, lymphoma, mental retardation, muscular dystrophy, myopathy, neuropathy, obesity, Parkinson’s disease, retinitis pigmentosa, spastic paraplegia, spinocerebellar ataxia, usher syndrome, xeroderma pigmentosum, Zellweger syndrome Pathways n Wnt pathway members (GO: 0016055: Wnt receptor signaling pathway) n Notch pathway members (GO: 0007219: Notch signaling pathway) n EGFR pathway members (GO: 0007173: epidermal growth factor receptor signaling pathway) n n 40
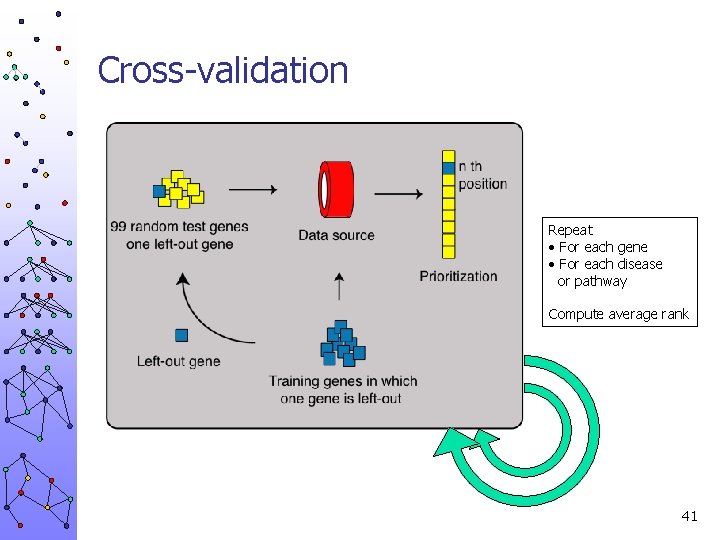
Cross-validation Repeat • For each gene • For each disease or pathway Compute average rank 41
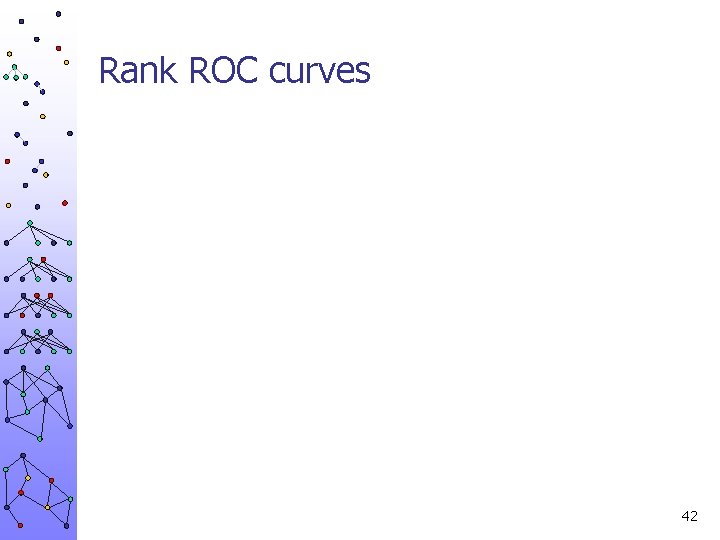
Rank ROC curves 42
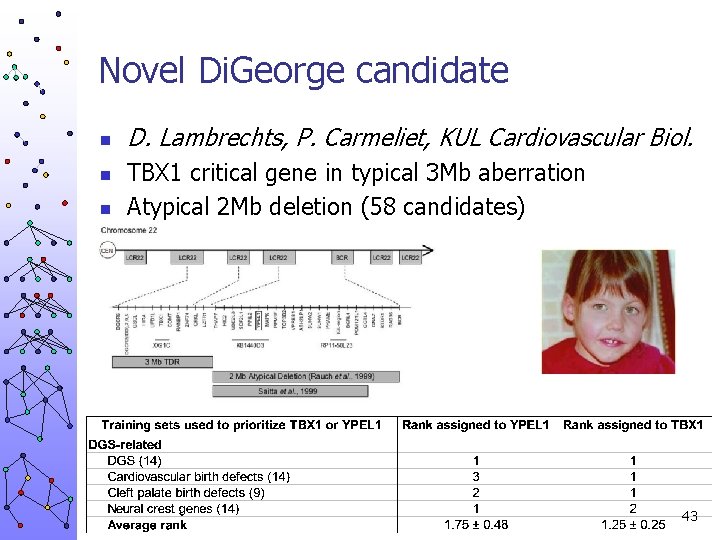
Novel Di. George candidate n n n D. Lambrechts, P. Carmeliet, KUL Cardiovascular Biol. TBX 1 critical gene in typical 3 Mb aberration Atypical 2 Mb deletion (58 candidates) 43
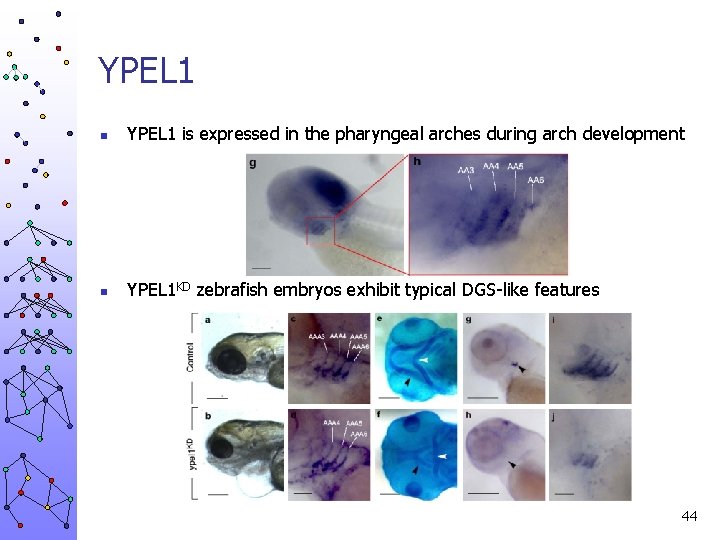
YPEL 1 n YPEL 1 is expressed in the pharyngeal arches during arch development n YPEL 1 KD zebrafish embryos exhibit typical DGS-like features 44
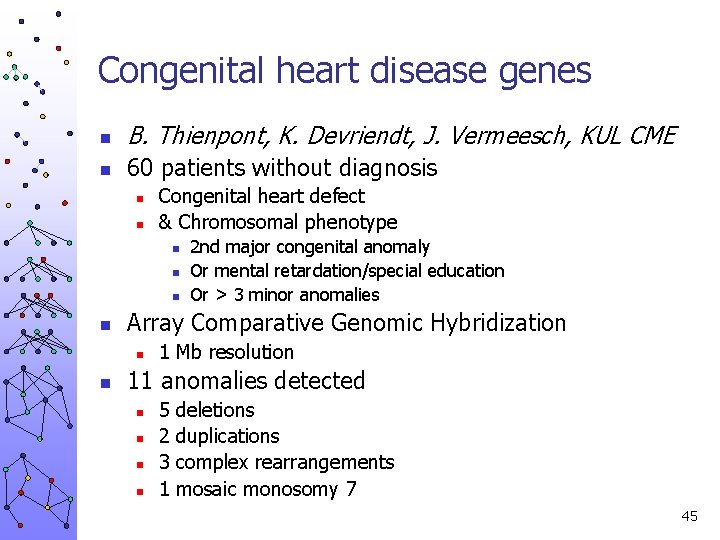
Congenital heart disease genes n B. Thienpont, K. Devriendt, J. Vermeesch, KUL CME n 60 patients without diagnosis n n Congenital heart defect & Chromosomal phenotype n n Array Comparative Genomic Hybridization n n 2 nd major congenital anomaly Or mental retardation/special education Or > 3 minor anomalies 1 Mb resolution 11 anomalies detected n n 5 2 3 1 deletions duplications complex rearrangements mosaic monosomy 7 45
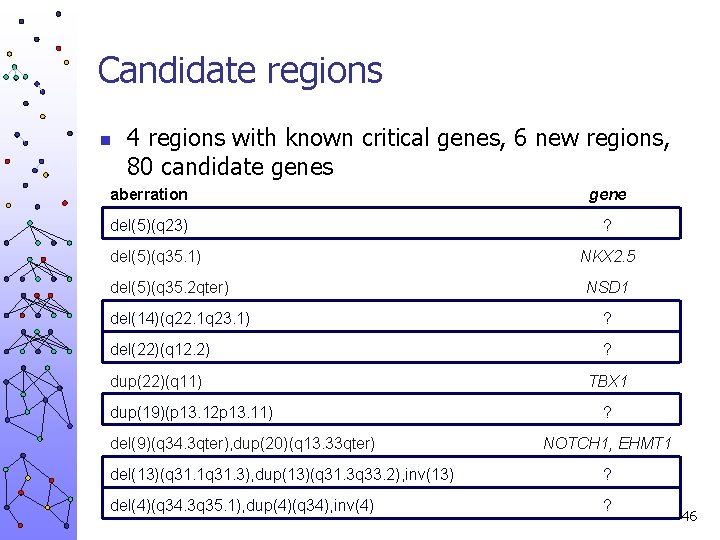
Candidate regions n 4 regions with known critical genes, 6 new regions, 80 candidate genes aberration gene del(5)(q 23) ? del(5)(q 35. 1) del(5)(q 35. 2 qter) NKX 2. 5 NSD 1 del(14)(q 22. 1 q 23. 1) ? del(22)(q 12. 2) ? dup(22)(q 11) TBX 1 dup(19)(p 13. 12 p 13. 11) del(9)(q 34. 3 qter), dup(20)(q 13. 33 qter) ? NOTCH 1, EHMT 1 del(13)(q 31. 1 q 31. 3), dup(13)(q 31. 3 q 33. 2), inv(13) ? del(4)(q 34. 3 q 35. 1), dup(4)(q 34), inv(4) ? 46
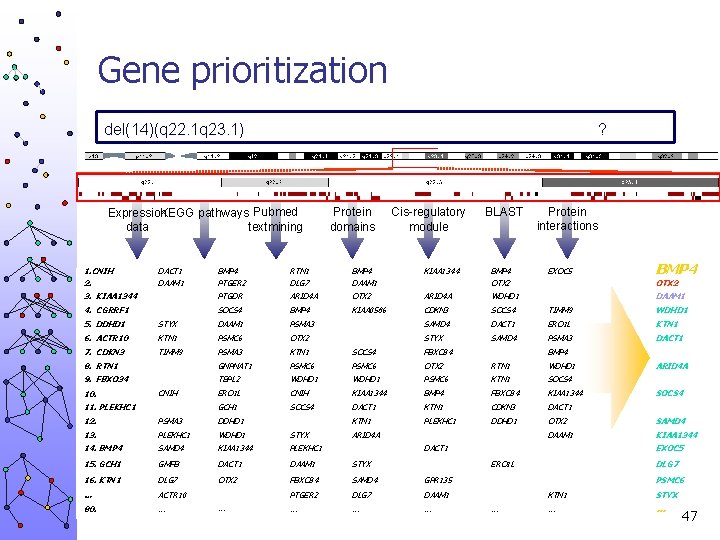
Gene prioritization del(14)(q 22. 1 q 23. 1) ? Expression KEGG pathways Pubmed textmining data 1. CNIH 2. DACT 1 DAAM 1 3. KIAA 1344 4. CGRRF 1 Protein domains Cis-regulatory module BLAST Protein interactions EXOC 5 BMP 4 PTGER 2 RTN 1 DLG 7 BMP 4 DAAM 1 KIAA 1344 BMP 4 OTX 2 PTGDR ARID 4 A OTX 2 ARID 4 A WDHD 1 KIAA 0586 CDKN 3 SOCS 4 TIMM 9 WDHD 1 SAMD 4 DACT 1 ERO 1 L KTN 1 STYX SAMD 4 PSMA 3 DACT 1 OTX 2 DAAM 1 SOCS 4 BMP 4 5. DDHD 1 STYX DAAM 1 PSMA 3 6. ACTR 10 KTN 1 PSMC 6 OTX 2 7. CDKN 3 TIMM 9 PSMA 3 KTN 1 SOCS 4 FBXO 34 8. RTN 1 GNPNAT 1 PSMC 6 OTX 2 RTN 1 WDHD 1 9. FBXO 34 TBPL 2 WDHD 1 PSMC 6 KTN 1 SOCS 4 ERO 1 L CNIH KIAA 1344 BMP 4 FBXO 34 KIAA 1344 GCH 1 SOCS 4 DACT 1 KTN 1 CDKN 3 DACT 1 KTN 1 PLEKHC 1 DDHD 1 OTX 2 SAMD 4 DAAM 1 KIAA 1344 10. CNIH 11. PLEKHC 1 12. PSMA 3 DDHD 1 13. PLEKHC 1 WDHD 1 STYX 14. BMP 4 SAMD 4 KIAA 1344 PLEKHC 1 15. GCH 1 GMFB DACT 1 DAAM 1 STYX 16. KTN 1 DLG 7 OTX 2 FBXO 34 SAMD 4 GPR 135 … ACTR 10 PTGER 2 DLG 7 DAAM 1 80. … … … BMP 4 ARID 4 A DACT 1 ARID 4 A SOCS 4 EXOC 5 ERO 1 L DLG 7 PSMC 6 … KTN 1 STYX … … 47
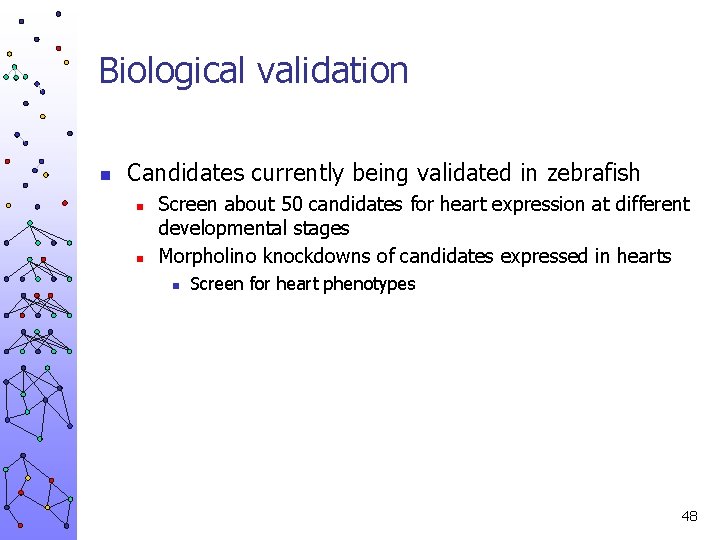
Biological validation n Candidates currently being validated in zebrafish n n Screen about 50 candidates for heart expression at different developmental stages Morpholino knockdowns of candidates expressed in hearts n Screen for heart phenotypes 48
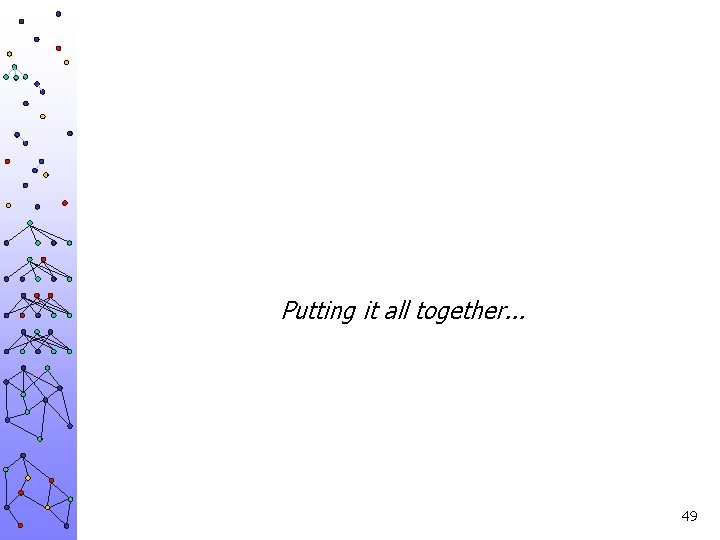
Putting it all together. . . 49
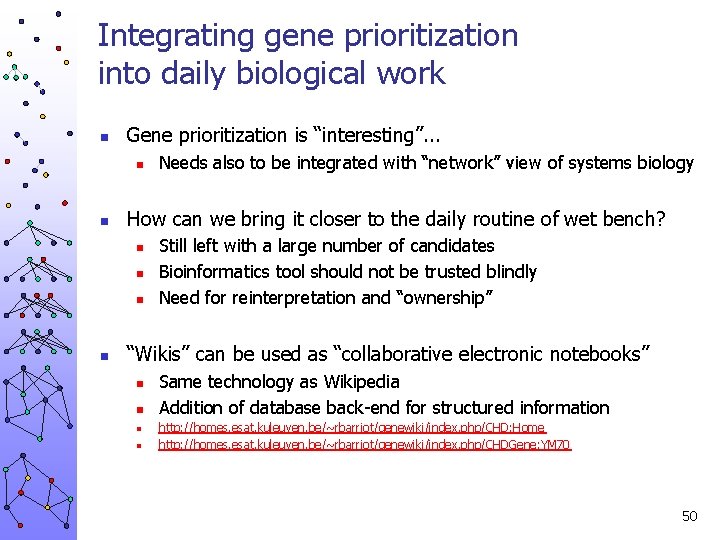
Integrating gene prioritization into daily biological work n Gene prioritization is “interesting”. . . n n How can we bring it closer to the daily routine of wet bench? n n Needs also to be integrated with “network” view of systems biology Still left with a large number of candidates Bioinformatics tool should not be trusted blindly Need for reinterpretation and “ownership” “Wikis” can be used as “collaborative electronic notebooks” n n Same technology as Wikipedia Addition of database back-end for structured information http: //homes. esat. kuleuven. be/~rbarriot/genewiki/index. php/CHD: Home http: //homes. esat. kuleuven. be/~rbarriot/genewiki/index. php/CHDGene: YM 70 50
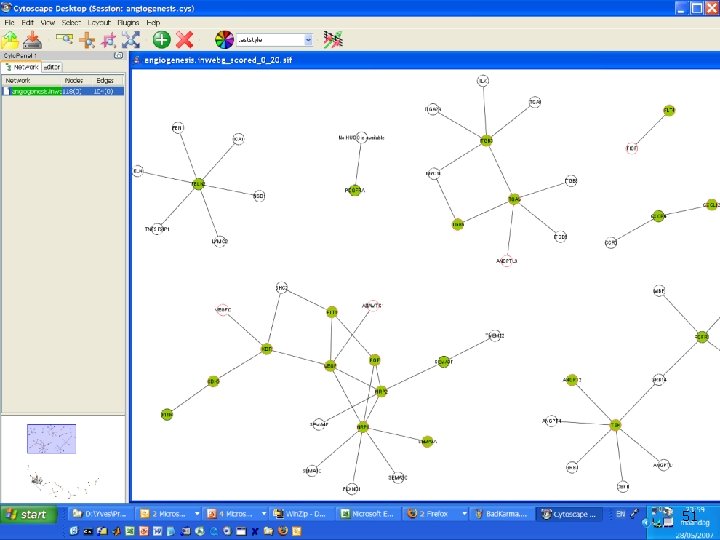
51
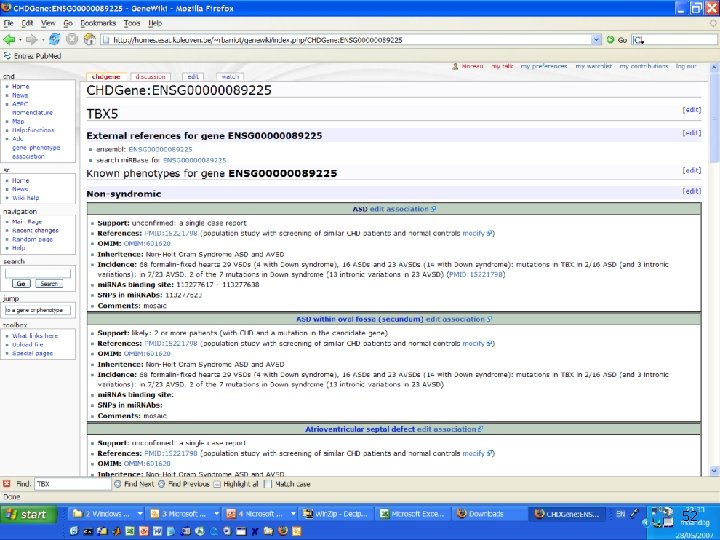
52
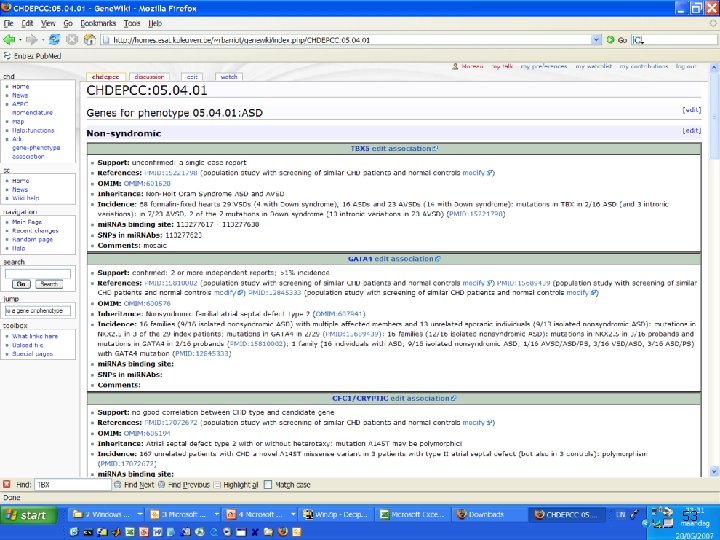
53
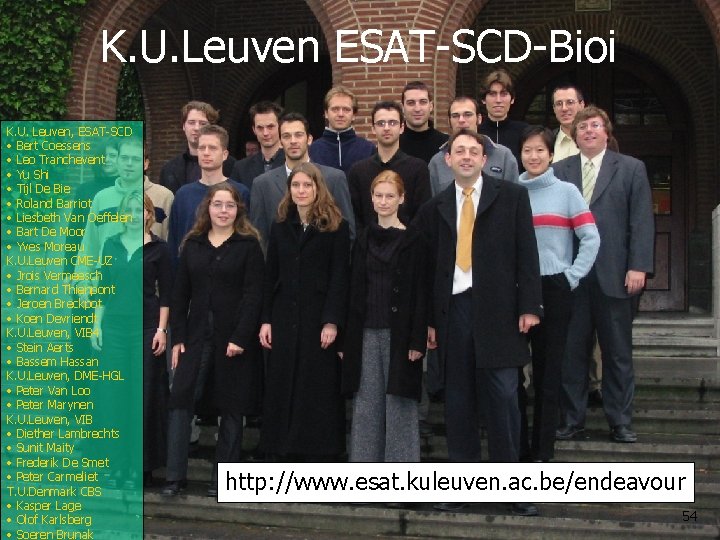
K. U. Leuven ESAT-SCD-Bioi K. U. Leuven, ESAT-SCD • Bert Coessens • Leo Tranchevent • Yu Shi • Tijl De Bie • Roland Barriot • Liesbeth Van Oeffelen • Bart De Moor • Yves Moreau K. U. Leuven CME-UZ • Jrois Vermeesch • Bernard Thienpont • Jeroen Breckpot • Koen Devriendt K. U. Leuven, VIB 4 • Stein Aerts • Bassem Hassan K. U. Leuven, DME-HGL • Peter Van Loo • Peter Marynen K. U. Leuven, VIB • Diether Lambrechts • Sunit Maity • Frederik De Smet • Peter Carmeliet T. U. Denmark CBS • Kasper Lage • Olof Karlsberg • Soeren Brunak http: //www. esat. kuleuven. ac. be/endeavour 54
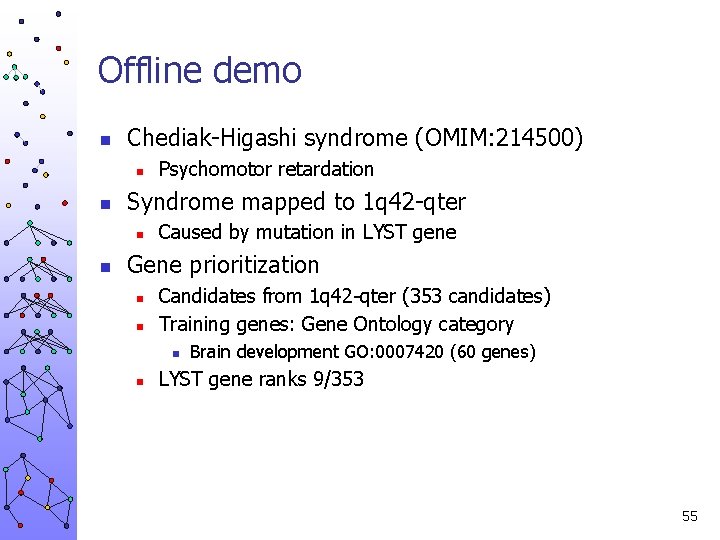
Offline demo n Chediak-Higashi syndrome (OMIM: 214500) n n Syndrome mapped to 1 q 42 -qter n n Psychomotor retardation Caused by mutation in LYST gene Gene prioritization n n Candidates from 1 q 42 -qter (353 candidates) Training genes: Gene Ontology category n n Brain development GO: 0007420 (60 genes) LYST gene ranks 9/353 55
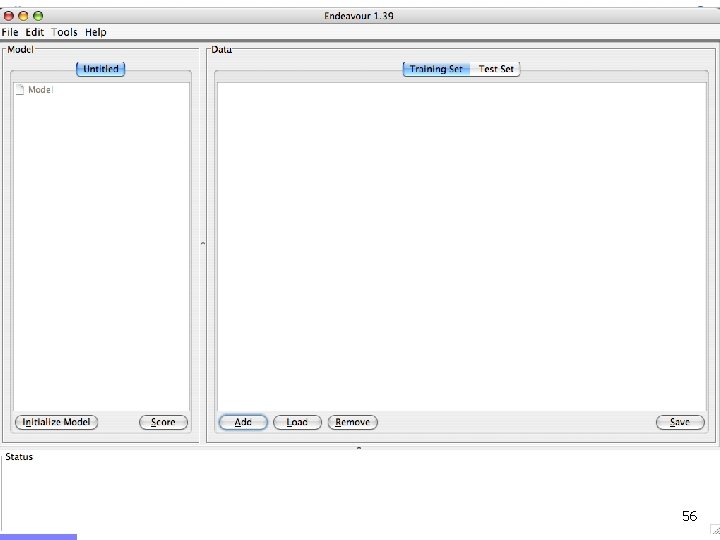
56
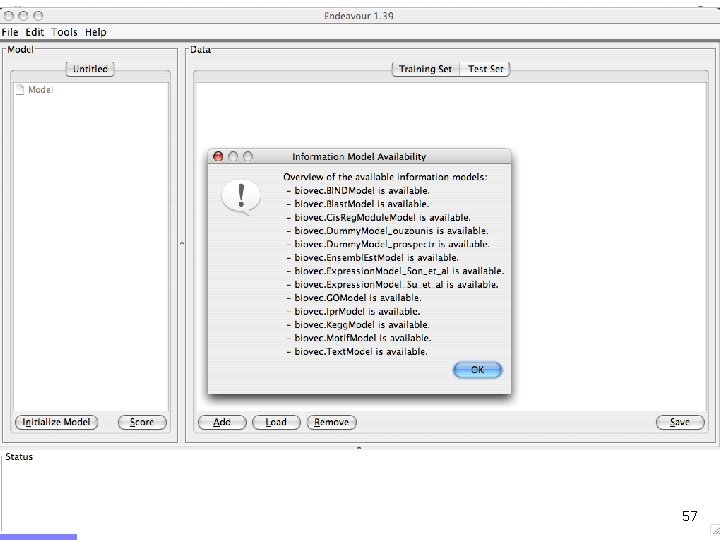
57
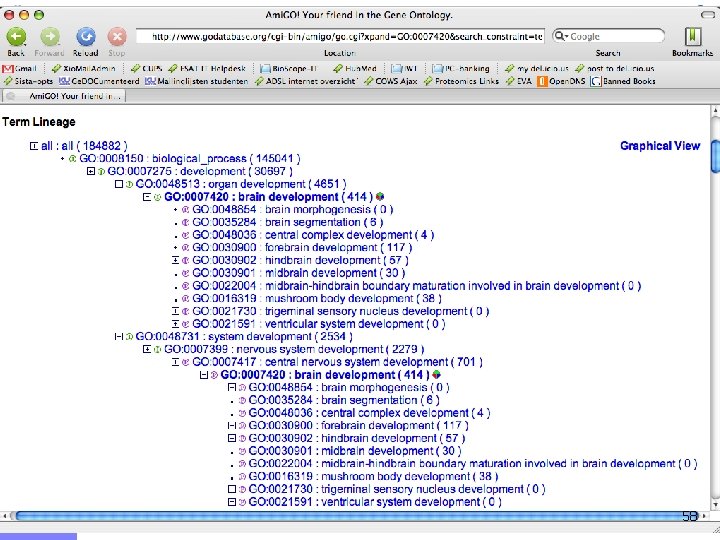
58
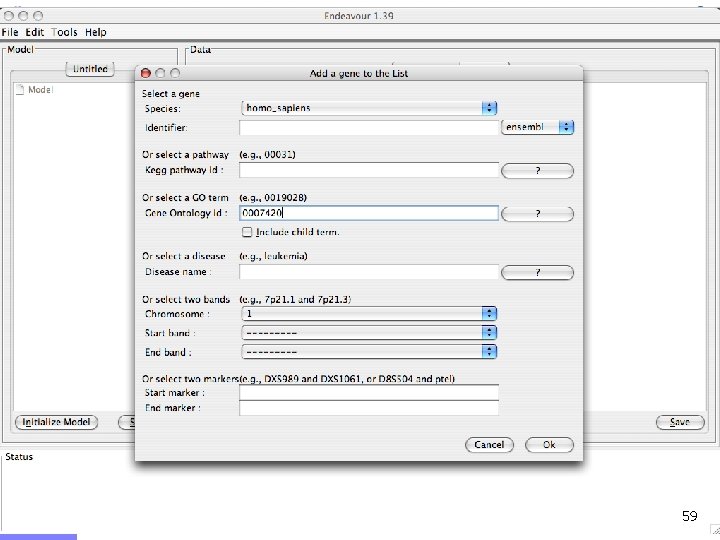
59
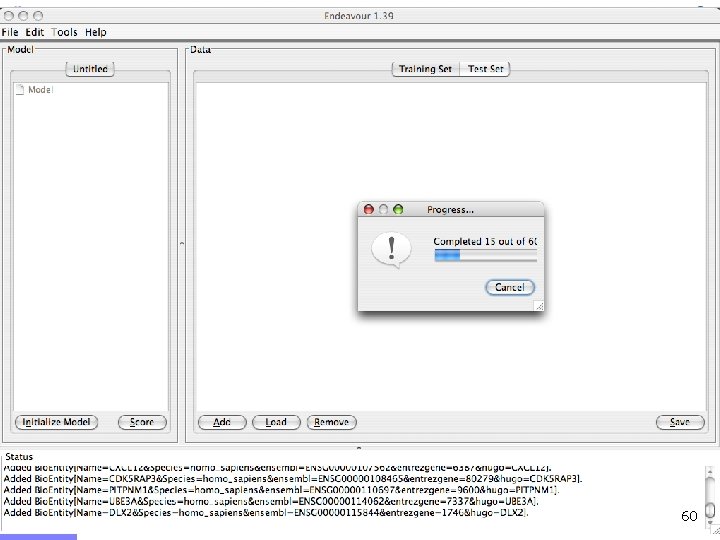
60
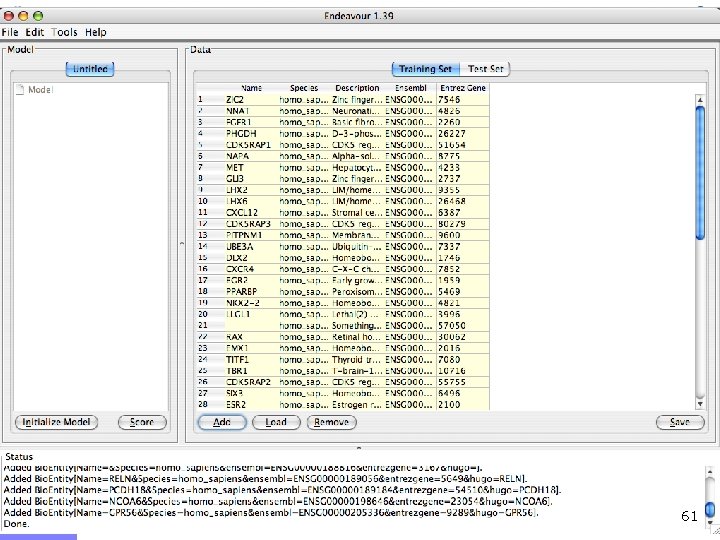
61
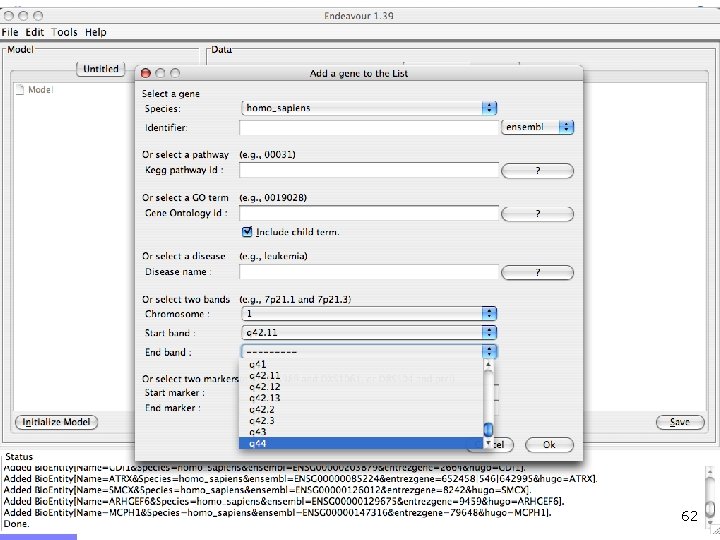
62
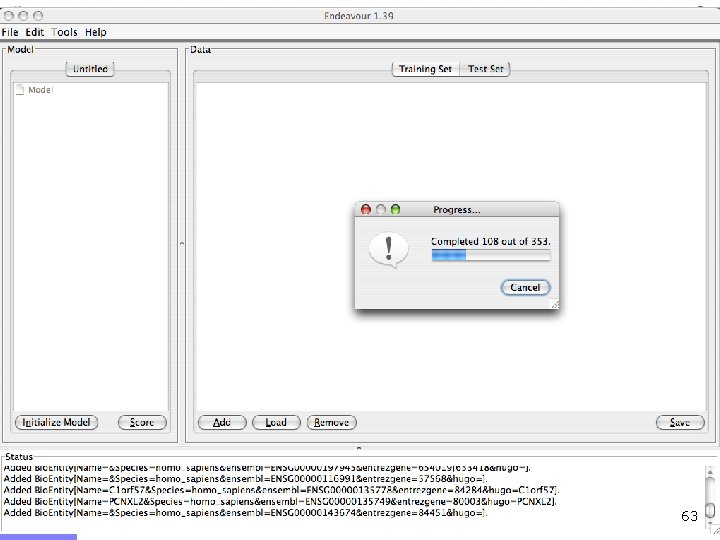
63
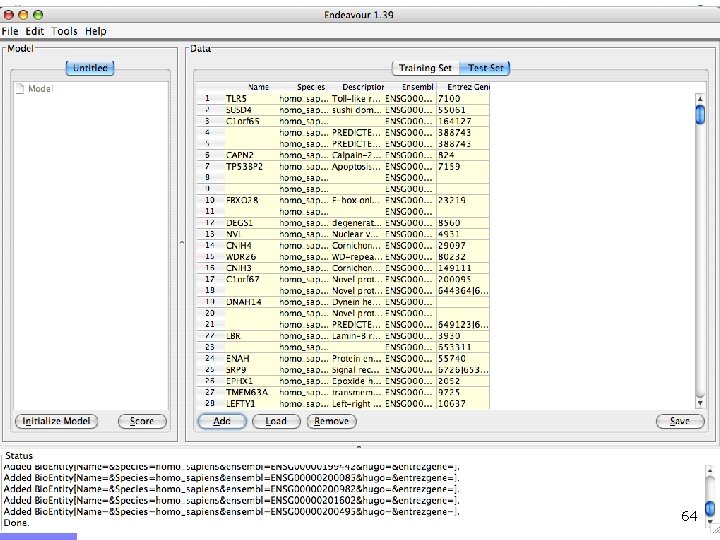
64
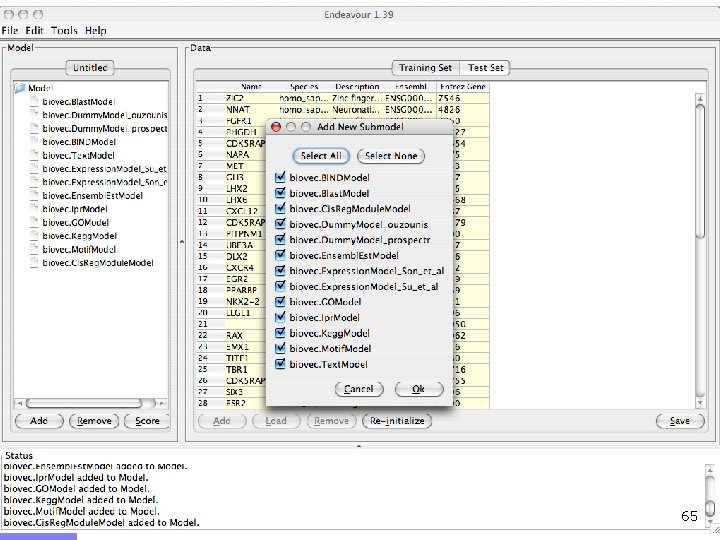
65
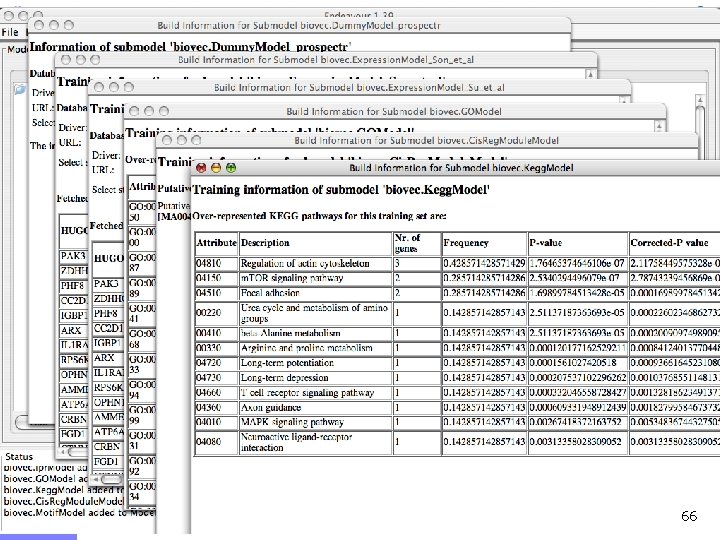
66
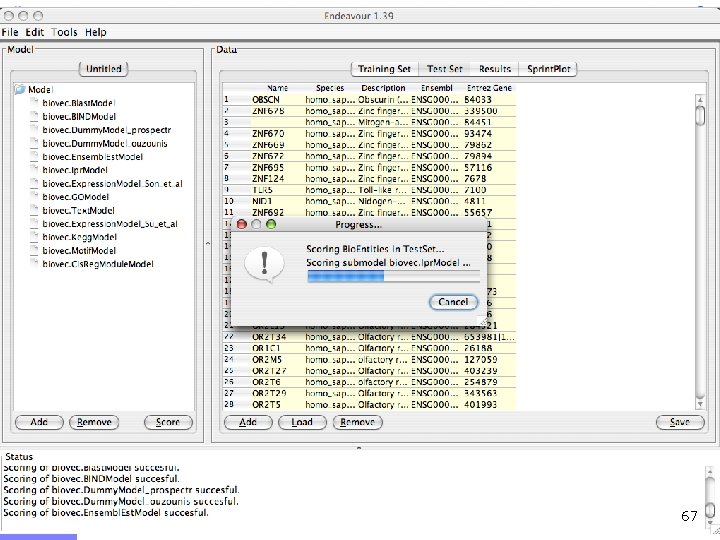
67
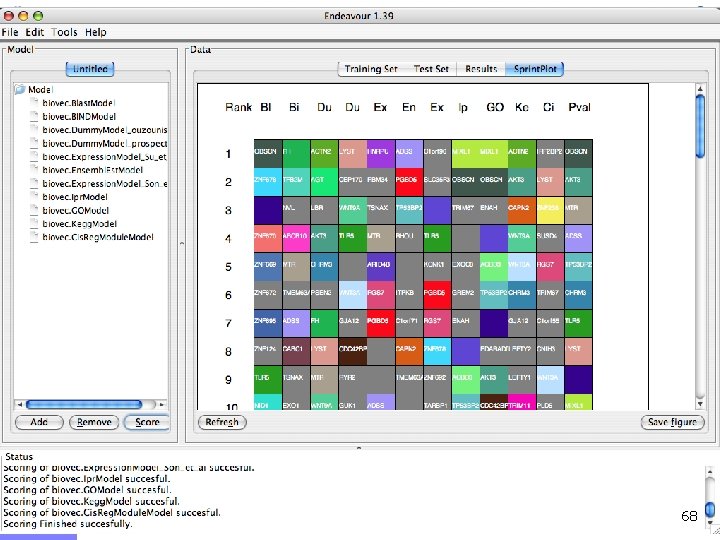
68
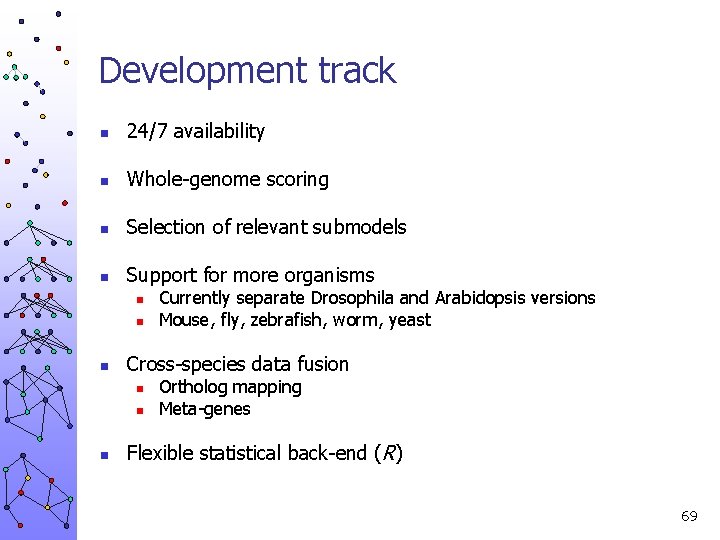
Development track n 24/7 availability n Whole-genome scoring n Selection of relevant submodels n Support for more organisms n n n Cross-species data fusion n Currently separate Drosophila and Arabidopsis versions Mouse, fly, zebrafish, worm, yeast Ortholog mapping Meta-genes Flexible statistical back-end (R) 69
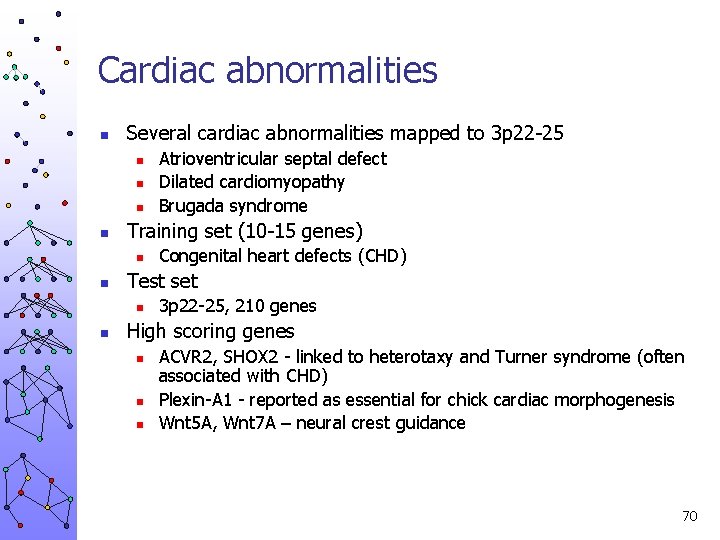
Cardiac abnormalities n Several cardiac abnormalities mapped to 3 p 22 -25 n n Training set (10 -15 genes) n n Congenital heart defects (CHD) Test set n n Atrioventricular septal defect Dilated cardiomyopathy Brugada syndrome 3 p 22 -25, 210 genes High scoring genes n n n ACVR 2, SHOX 2 - linked to heterotaxy and Turner syndrome (often associated with CHD) Plexin-A 1 - reported as essential for chick cardiac morphogenesis Wnt 5 A, Wnt 7 A – neural crest guidance 70
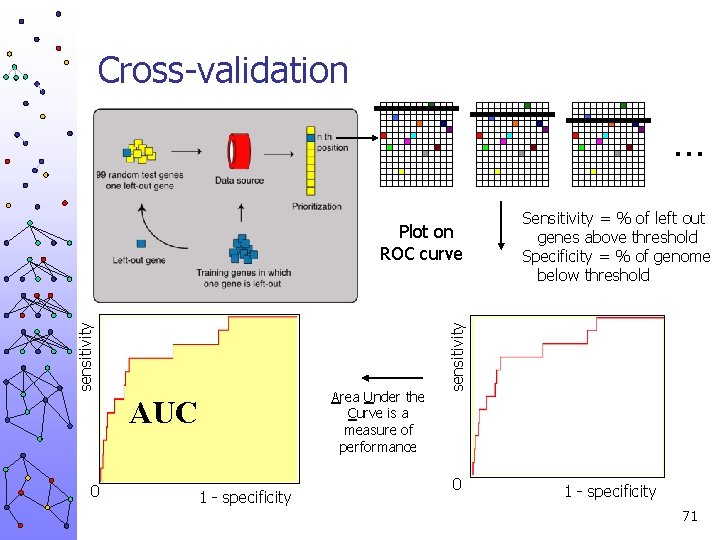
Cross-validation … Area Under the Curve is a measure of performance AUC 0 1 - specificity sensitivity Plot on ROC curve Sensitivity = % of left out genes above threshold Specificity = % of genome below threshold 0 1 - specificity 71
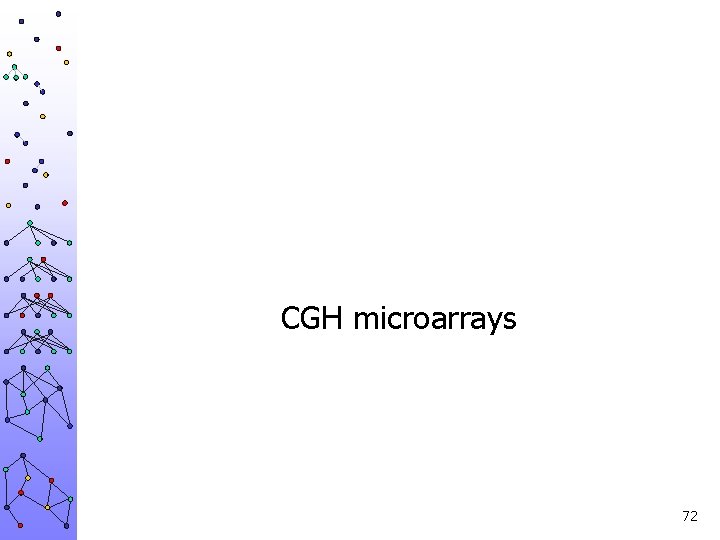
CGH microarrays 72
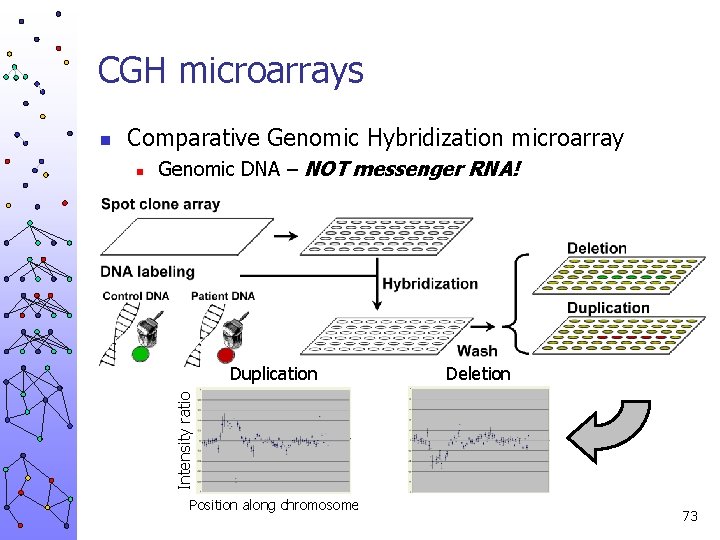
CGH microarrays Comparative Genomic Hybridization microarray n Genomic DNA – NOT messenger RNA! Duplication Deletion Intensity ratio n Position along chromosome 73
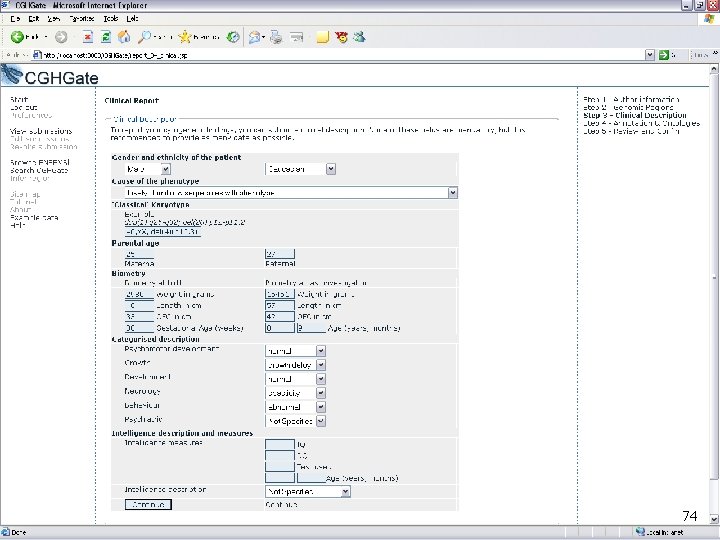
CGHGate Demo 74
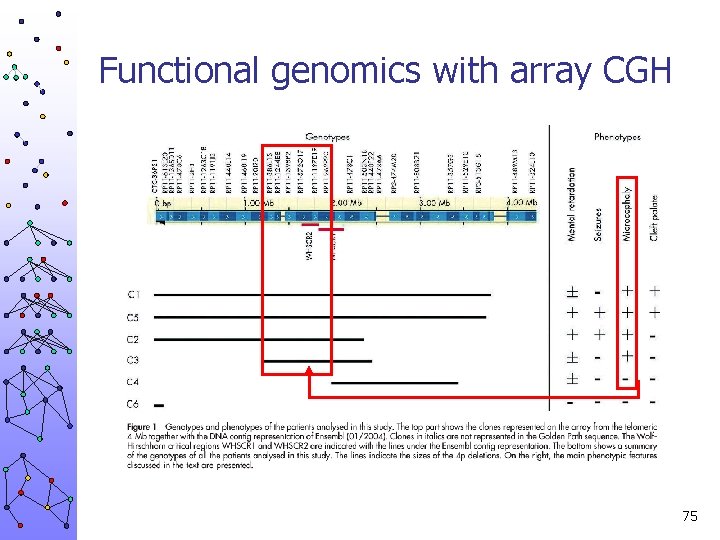
Functional genomics with array CGH 75
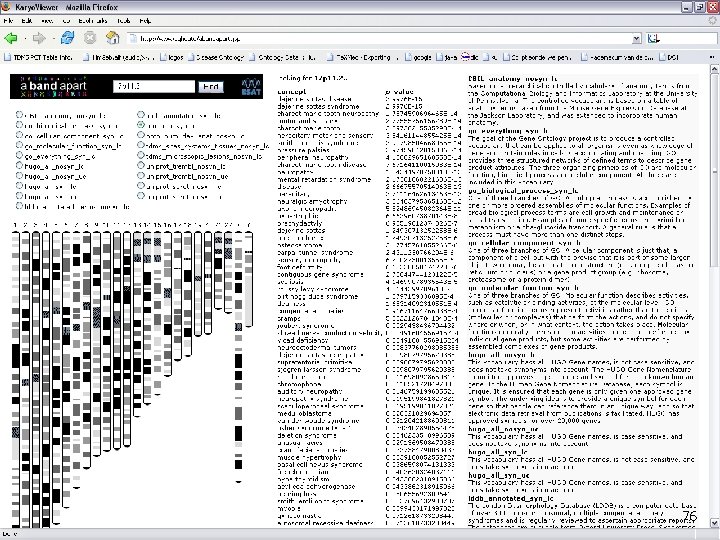
76
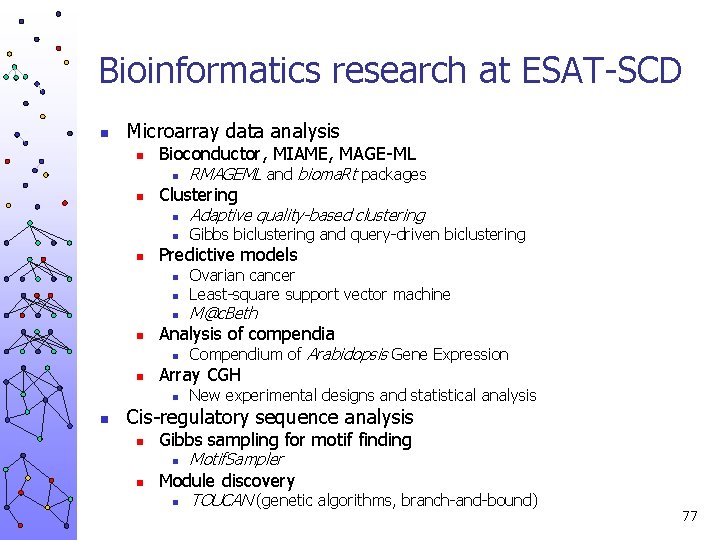
Bioinformatics research at ESAT-SCD n Microarray data analysis n Bioconductor, MIAME, MAGE-ML n n Clustering n n n Ovarian cancer Least-square support vector machine M@c. Beth Compendium of Arabidopsis Gene Expression Array CGH n n Gibbs biclustering and query-driven biclustering Analysis of compendia n n Adaptive quality-based clustering Predictive models n n RMAGEML and bioma. Rt packages New experimental designs and statistical analysis Cis-regulatory sequence analysis n Gibbs sampling for motif finding n n Motif. Sampler Module discovery n TOUCAN (genetic algorithms, branch-and-bound) 77
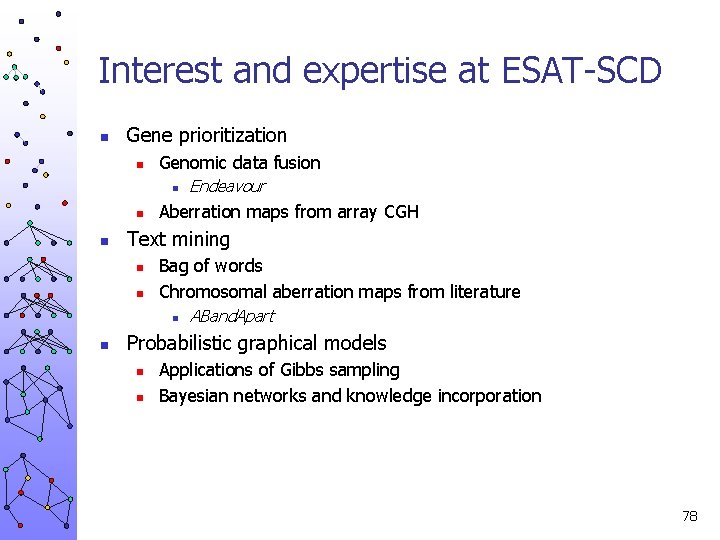
Interest and expertise at ESAT-SCD n Gene prioritization n Genomic data fusion n Aberration maps from array CGH Text mining n n Bag of words Chromosomal aberration maps from literature n n Endeavour ABand. Apart Probabilistic graphical models n n Applications of Gibbs sampling Bayesian networks and knowledge incorporation 78
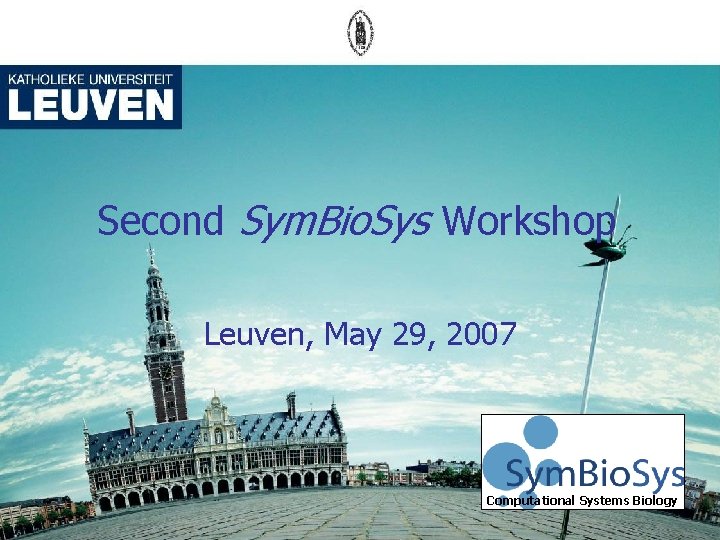
Second Sym. Bio. Sys Workshop Leuven, May 29, 2007 Computational Systems Biology
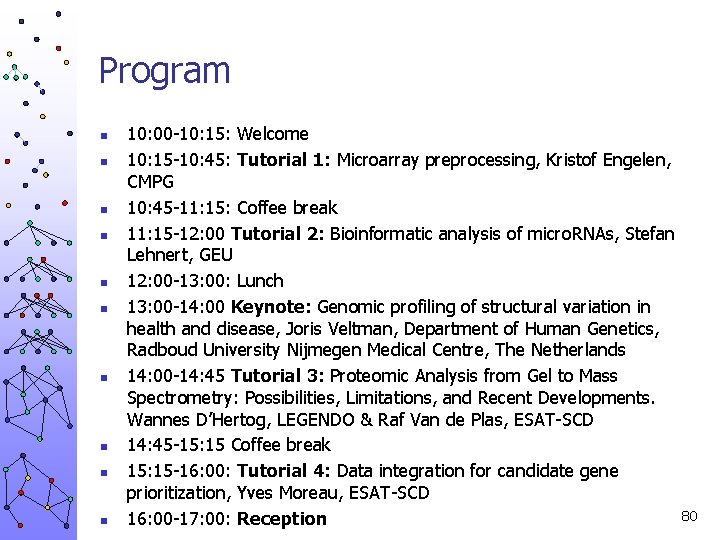
Program n n n n n 10: 00 -10: 15: Welcome 10: 15 -10: 45: Tutorial 1: Microarray preprocessing, Kristof Engelen, CMPG 10: 45 -11: 15: Coffee break 11: 15 -12: 00 Tutorial 2: Bioinformatic analysis of micro. RNAs, Stefan Lehnert, GEU 12: 00 -13: 00: Lunch 13: 00 -14: 00 Keynote: Genomic profiling of structural variation in health and disease, Joris Veltman, Department of Human Genetics, Radboud University Nijmegen Medical Centre, The Netherlands 14: 00 -14: 45 Tutorial 3: Proteomic Analysis from Gel to Mass Spectrometry: Possibilities, Limitations, and Recent Developments. Wannes D’Hertog, LEGENDO & Raf Van de Plas, ESAT-SCD 14: 45 -15: 15 Coffee break 15: 15 -16: 00: Tutorial 4: Data integration for candidate gene prioritization, Yves Moreau, ESAT-SCD 16: 00 -17: 00: Reception 80
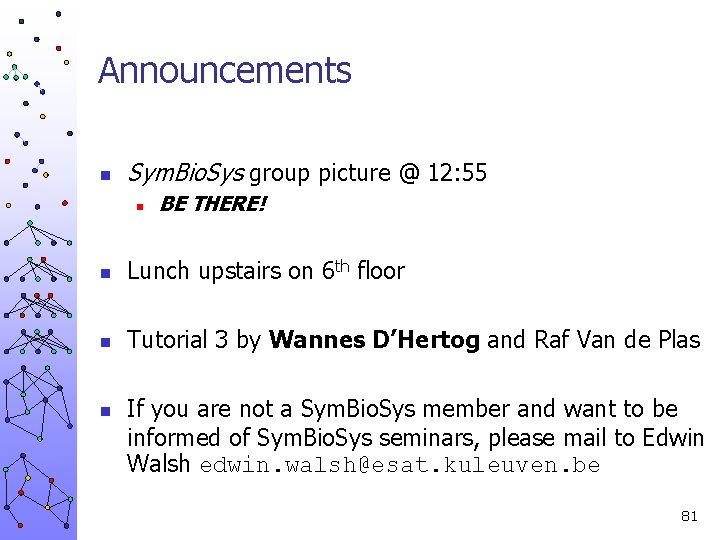
Announcements n Sym. Bio. Sys group picture @ 12: 55 n BE THERE! n Lunch upstairs on 6 th floor n Tutorial 3 by Wannes D’Hertog and Raf Van de Plas n If you are not a Sym. Bio. Sys member and want to be informed of Sym. Bio. Sys seminars, please mail to Edwin Walsh edwin. walsh@esat. kuleuven. be 81
Lllllll
Illustration poésie en vair et contre tous
Gene by gene test results
Chapter 17 gene expression from gene to protein
Project intake and prioritization
Rpa prioritization matrix
Predictive priortization
Prioritization
Wsjf example
Delegation model
Risk prioritization in software engineering
Abcd prioritization nursing
Infrastructure project prioritisation matrix
Prioritization
Ticket prioritization
Program prioritization process
1 improvement per day
Skupina nabis
Thierry moreau ucl
Gustave moreau salome
Myriam charles moreau
Gustave moreau zjevení
Moreau
Emilie moreau psychologue paris
Chemical properties of cardboard
Marjorie moreau
Gustave moreau zjevení
Joris moreau
Jessie moreau
Technine group
Moreau zjevení
Ian moreau
Data integration in data preprocessing
Mashups meaning
Google earth
Data preprocessing
Forward integration and backward integration
Forward backward integration
Simultaneous integration examples
Candidate title
Calling script for it recruiter
Candidate system matrix
A level computer science exemplar candidate work
Candidate ethical principles
Database normalization
Candidate self service portal
Candidate generation in apriori algorithm
Gate.aon.com/candidate/apply
Peoplecert online proctoring
Ice interactive connectivity establishment
Candidate entry information checklist dse
Websams ghs
Which of the following replaces the question mark
Afsp flight training
Ibm candidate zone
Usna candidate information system
National board candidate center
Dbminer
The manchurian candidate
Feasibility analysis matrix example
Candidate experience definition
Nuclear propulsion officer candidate
The candidate elimination algorithm represents the
Mining frequent patterns without candidate generation
Problem definition in system analysis and design
Candidate architecture
Dbms
Inductive biased hypothesis space and unbiased learner
Kontinuitetshantering
Typiska drag för en novell
Nationell inriktning för artificiell intelligens
Returpilarna
Varför kallas perioden 1918-1939 för mellankrigstiden
En lathund för arbete med kontinuitetshantering
Personalliggare bygg undantag
Personlig tidbok fylla i
Anatomi organ reproduksi
Förklara densitet för barn
Datorkunskap för nybörjare
Tack för att ni lyssnade bild
Att skriva en debattartikel
Autokratiskt ledarskap