The Squared Correlation 2 r What Does It
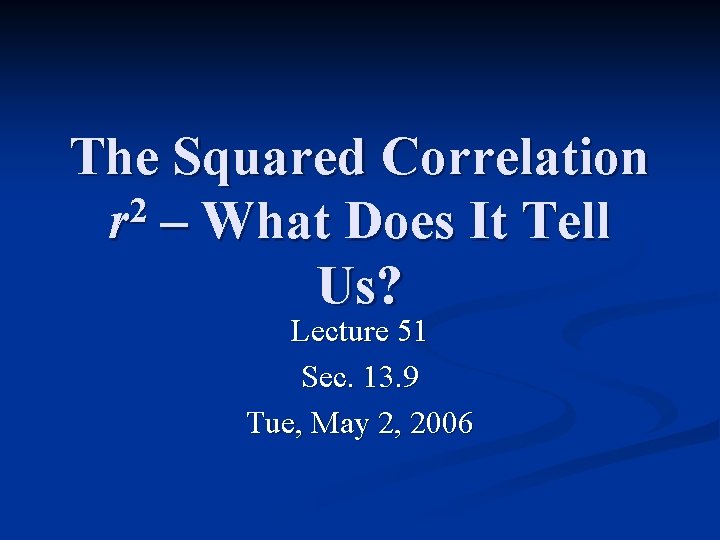
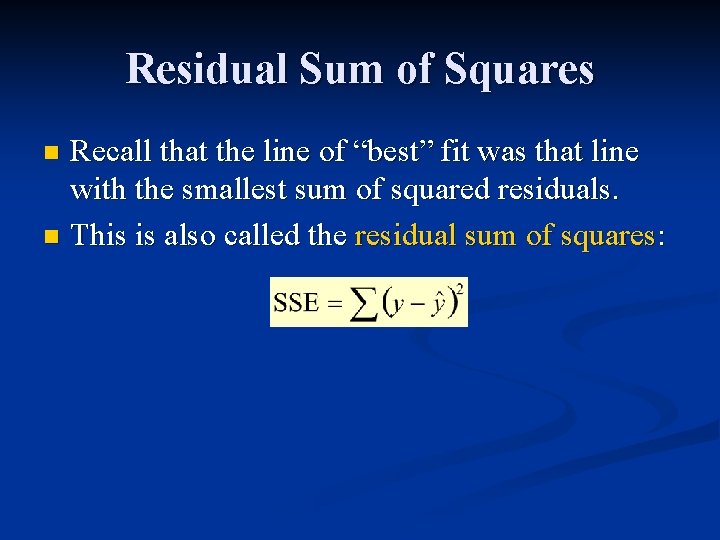
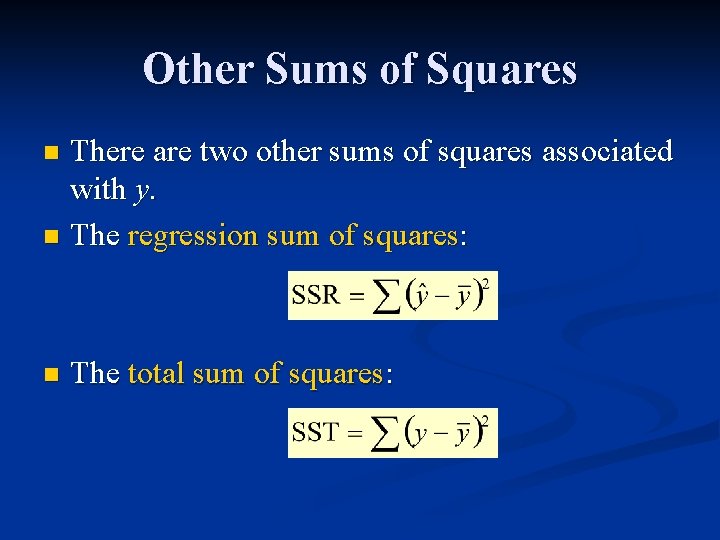
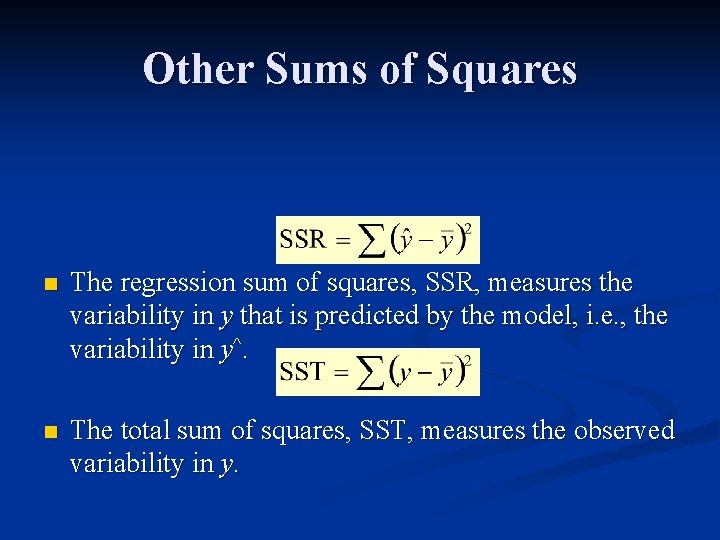
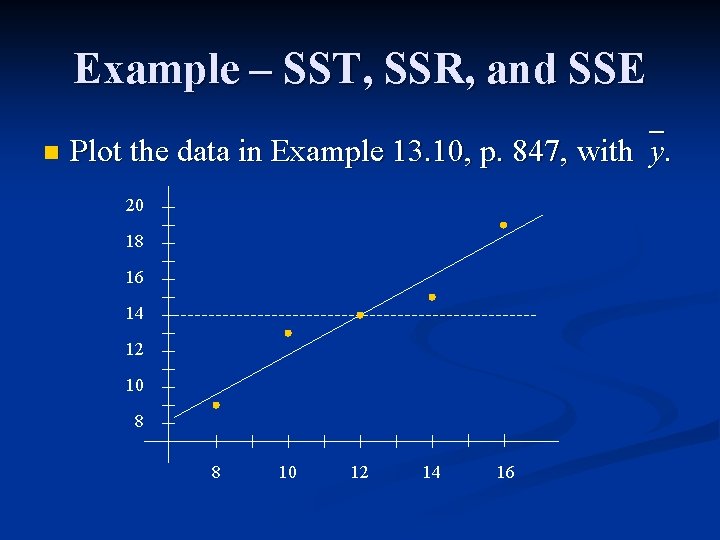
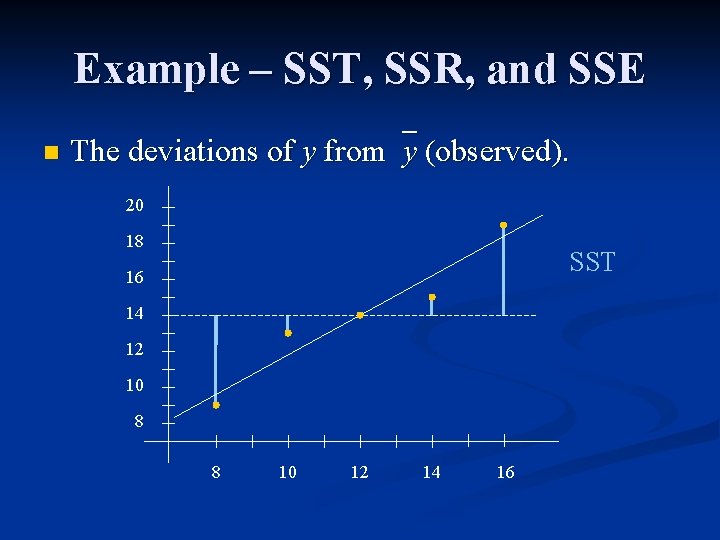
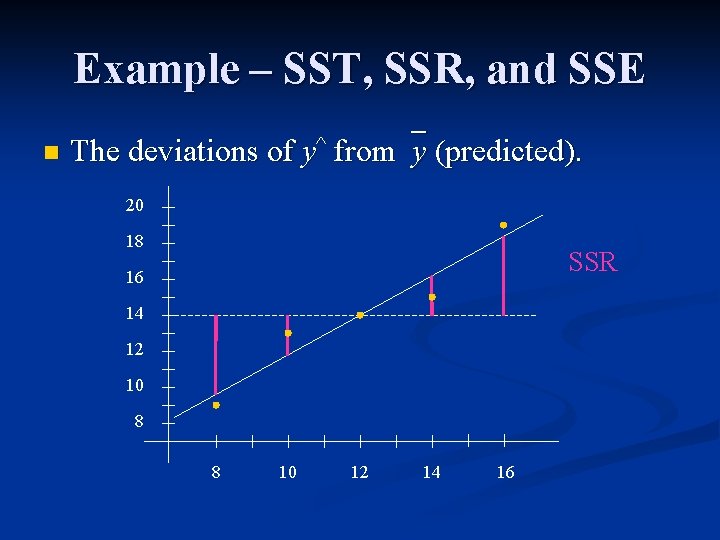
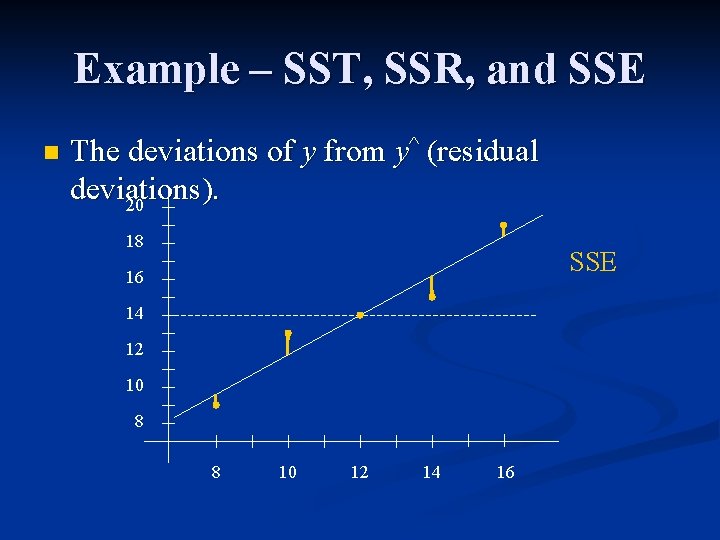
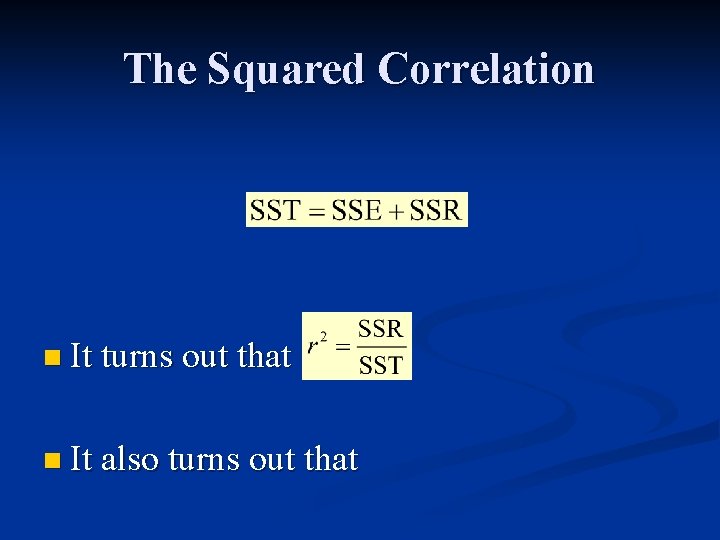
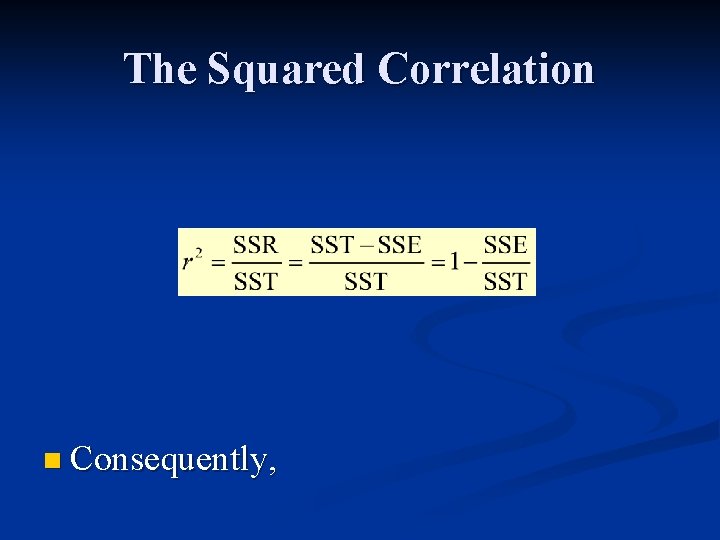
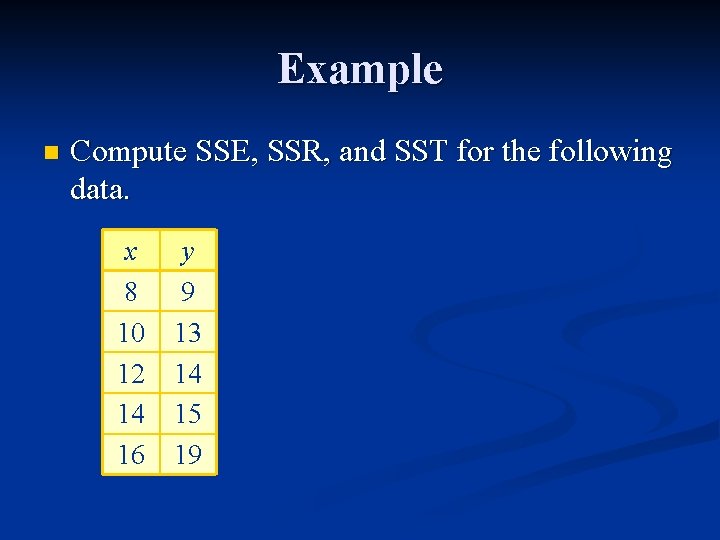
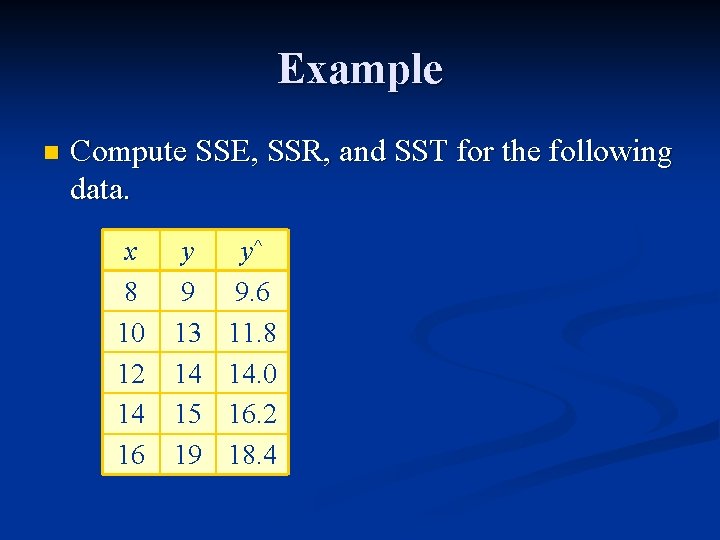
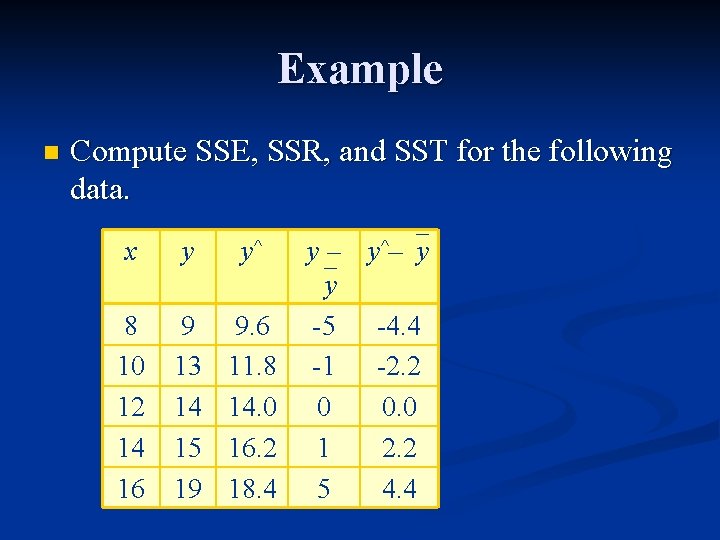
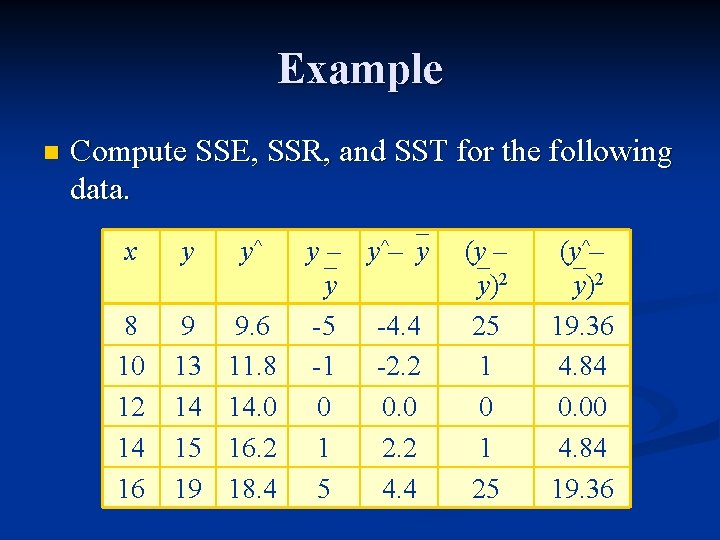
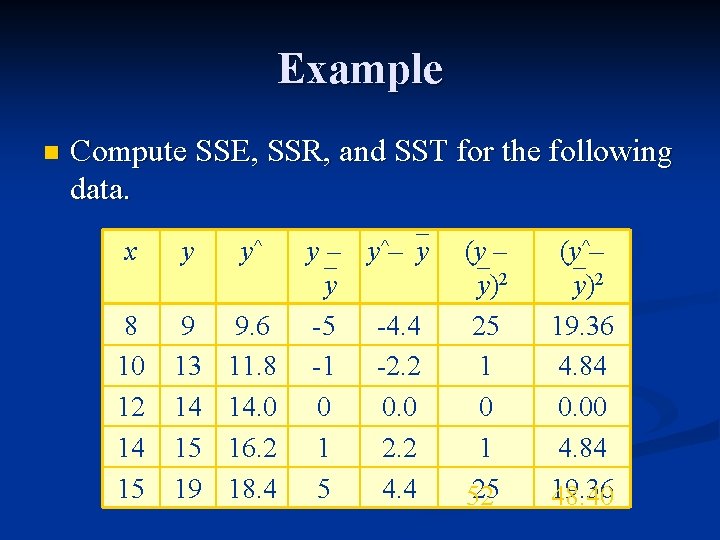
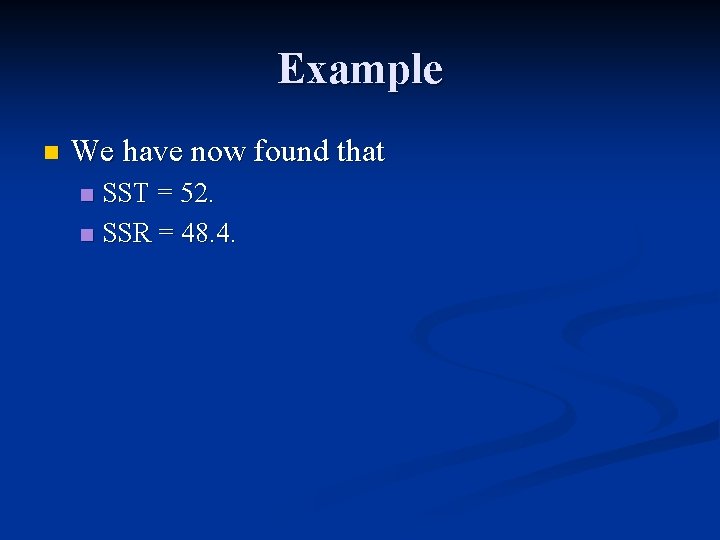
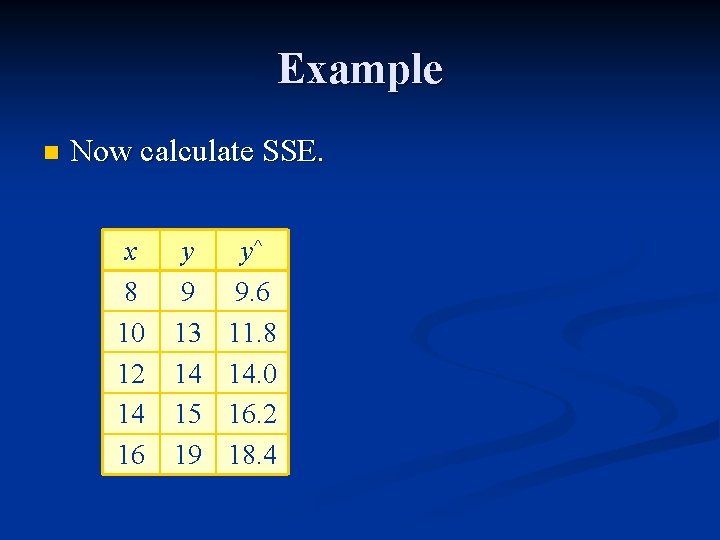
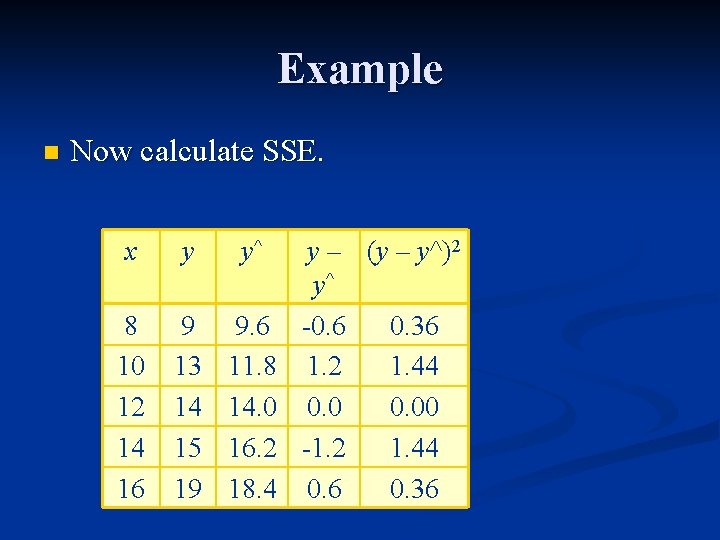
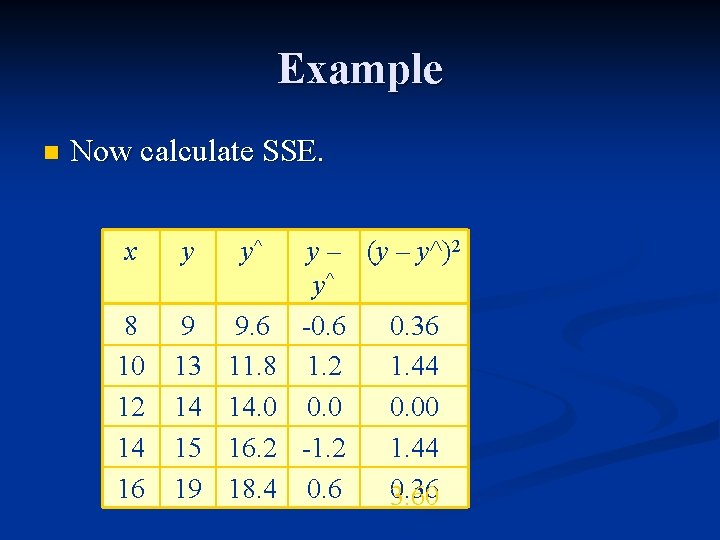
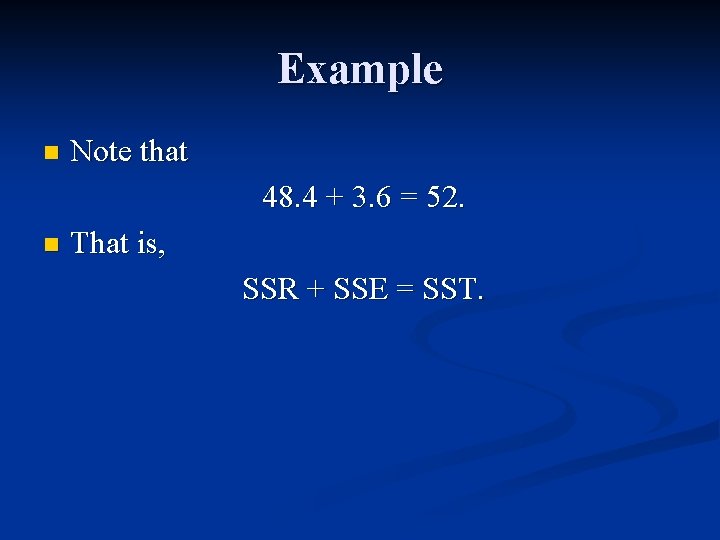
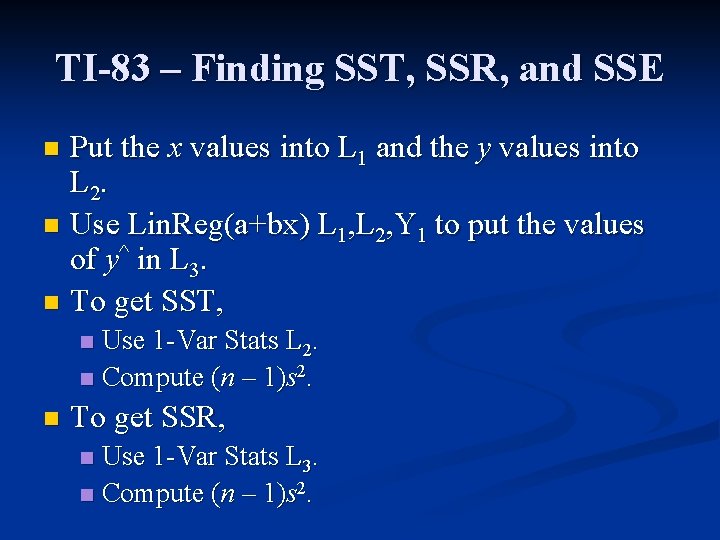
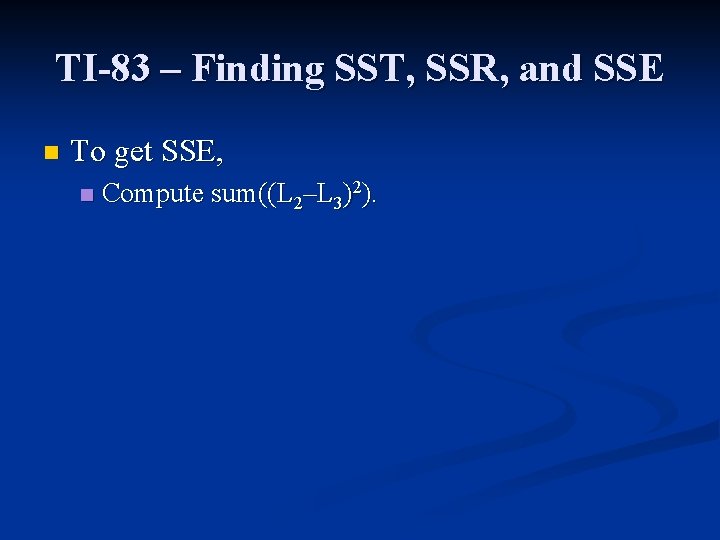
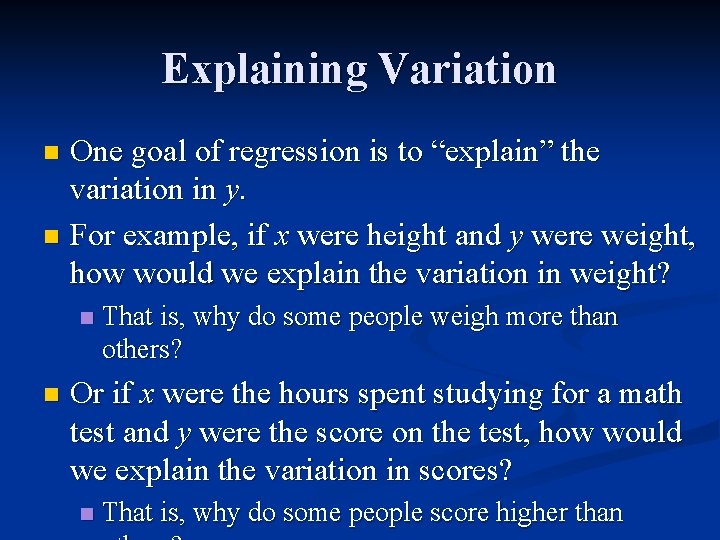
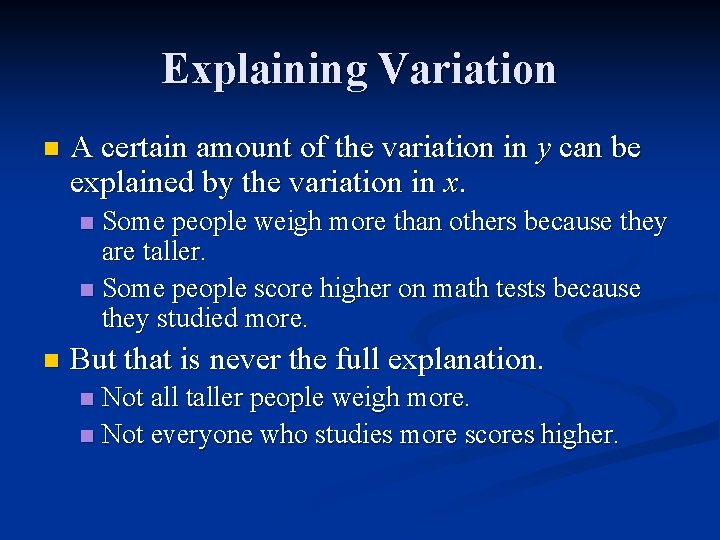
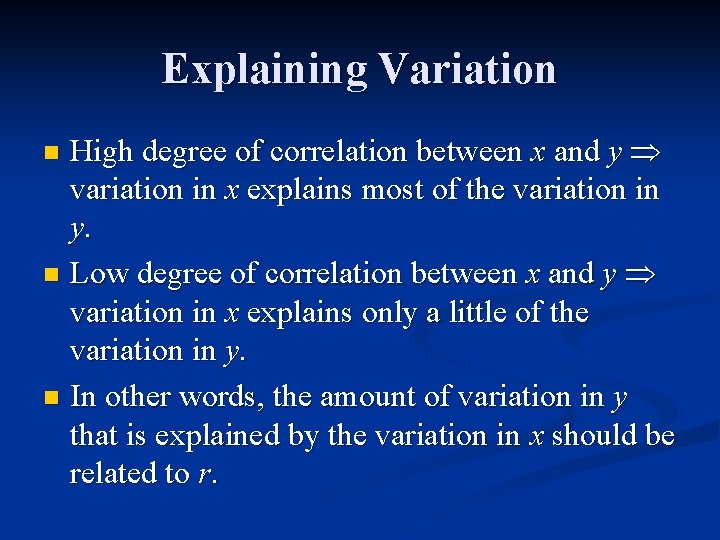
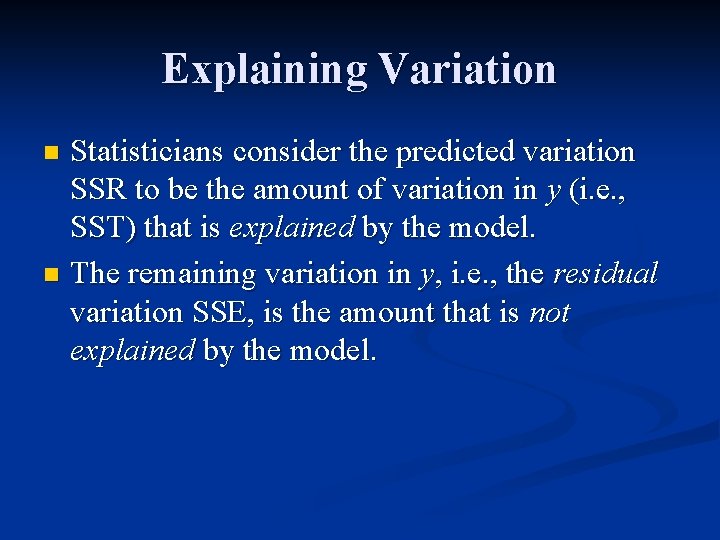
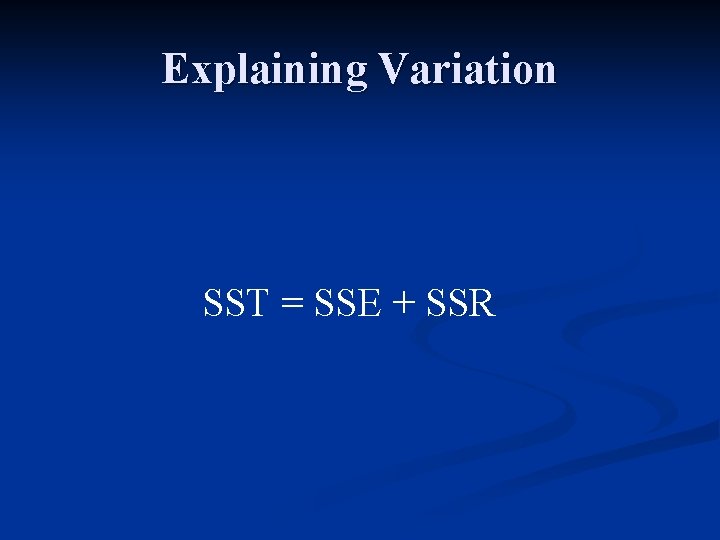
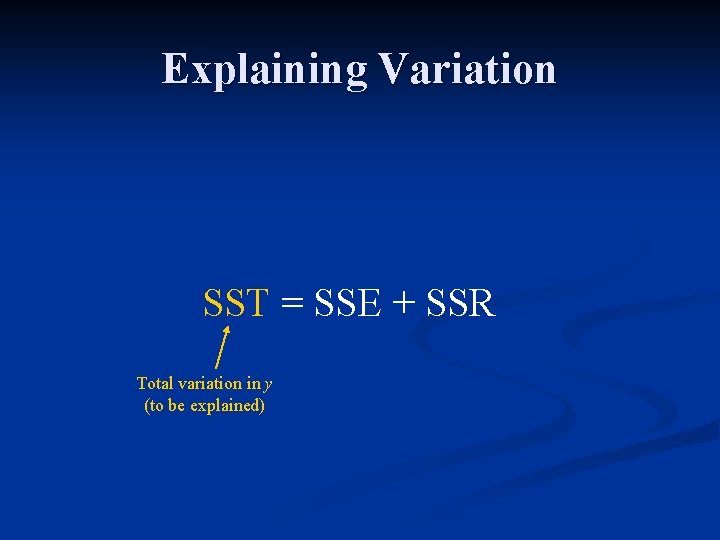
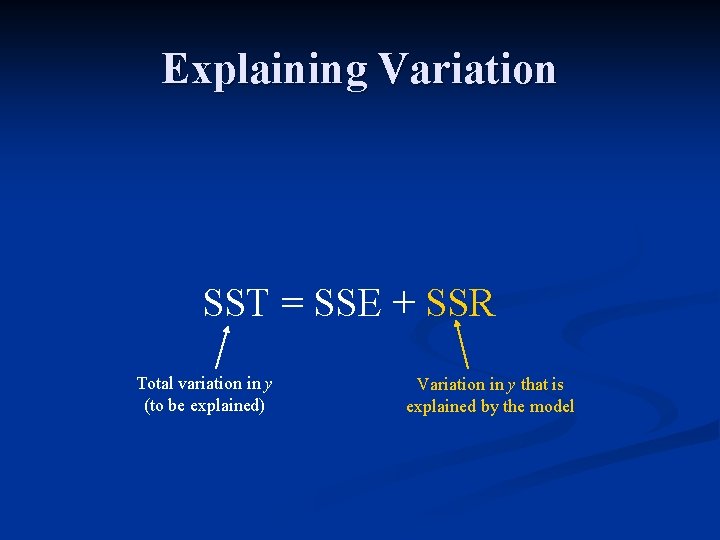
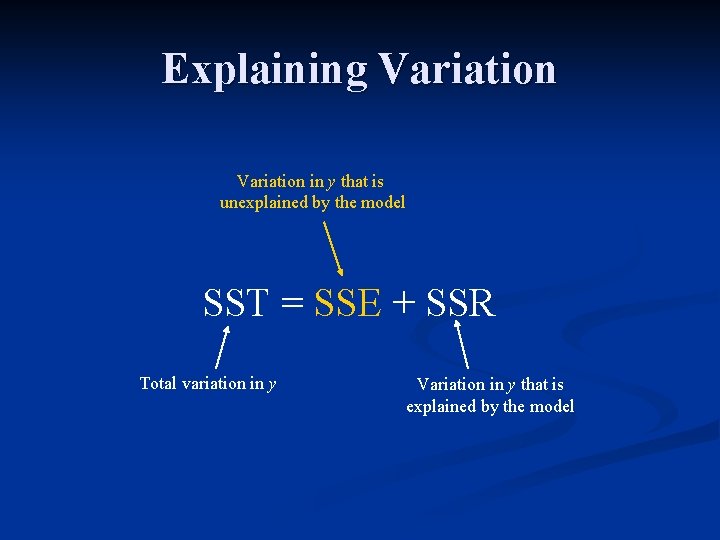
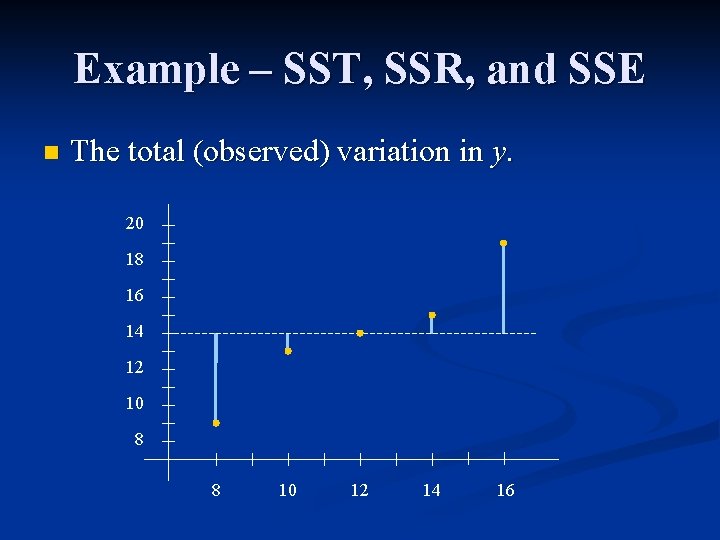
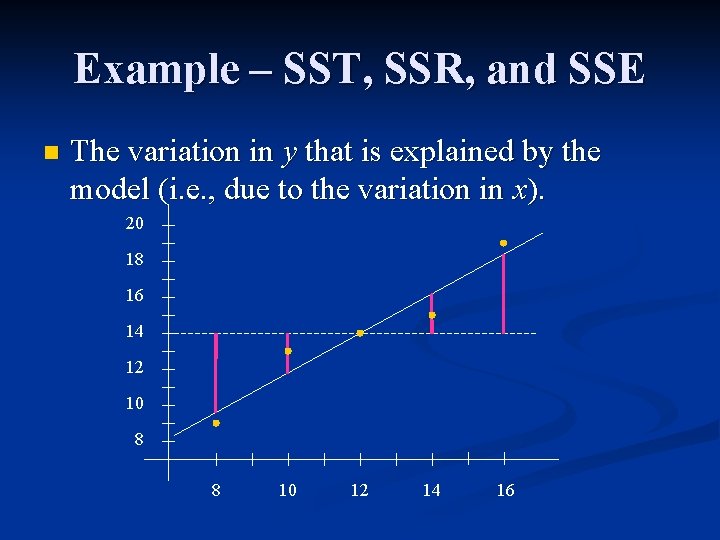
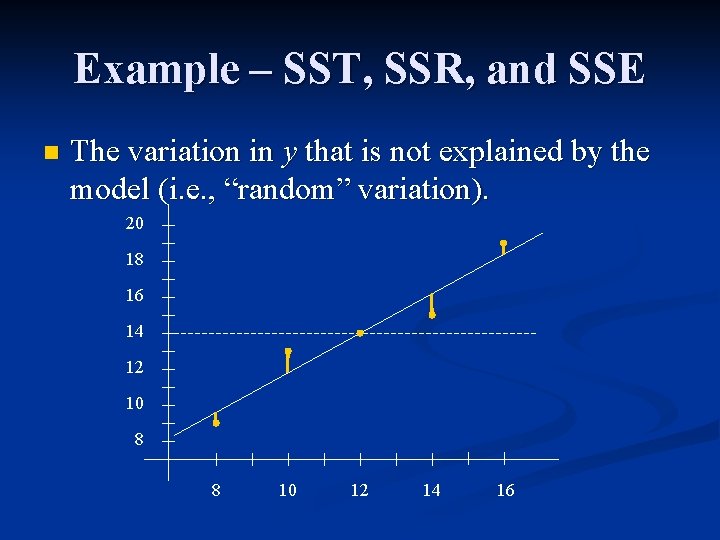
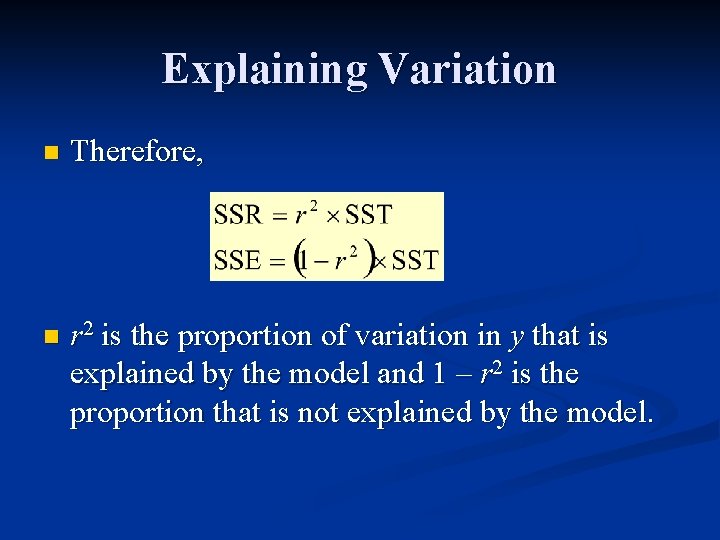
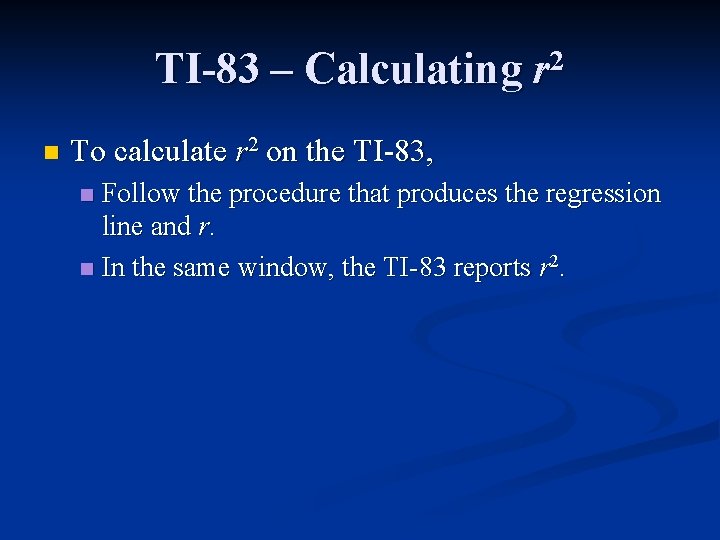
- Slides: 35
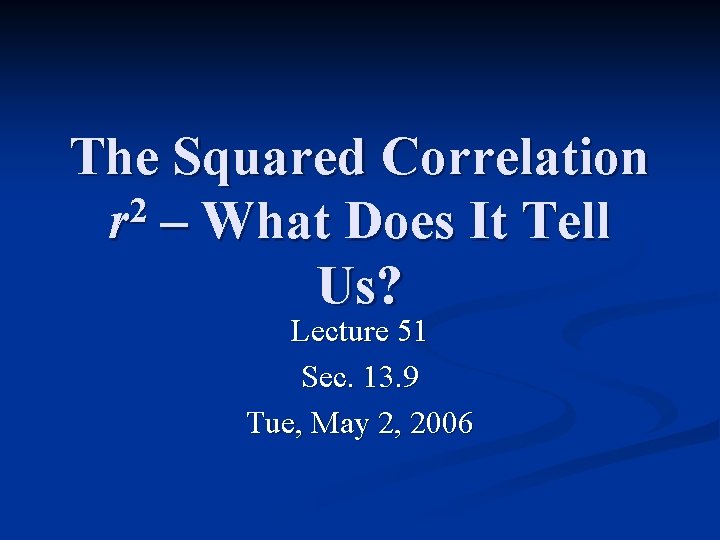
The Squared Correlation 2 r – What Does It Tell Us? Lecture 51 Sec. 13. 9 Tue, May 2, 2006
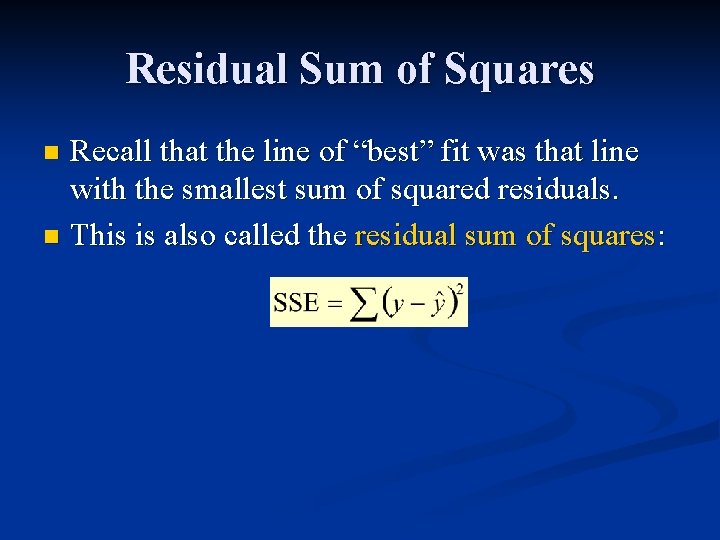
Residual Sum of Squares Recall that the line of “best” fit was that line with the smallest sum of squared residuals. n This is also called the residual sum of squares: n
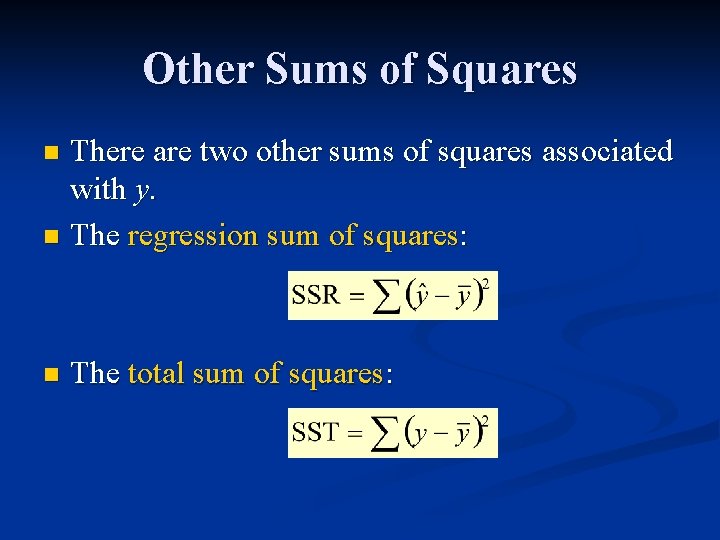
Other Sums of Squares There are two other sums of squares associated with y. n The regression sum of squares: n n The total sum of squares:
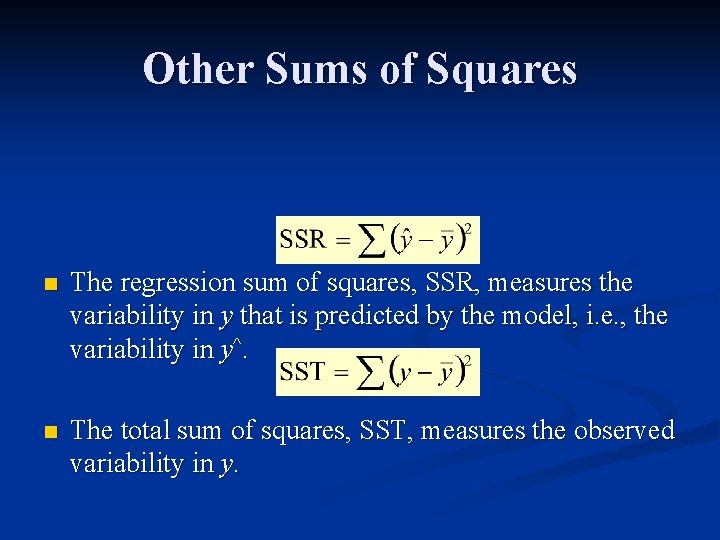
Other Sums of Squares n The regression sum of squares, SSR, measures the variability in y that is predicted by the model, i. e. , the variability in y^. n The total sum of squares, SST, measures the observed variability in y.
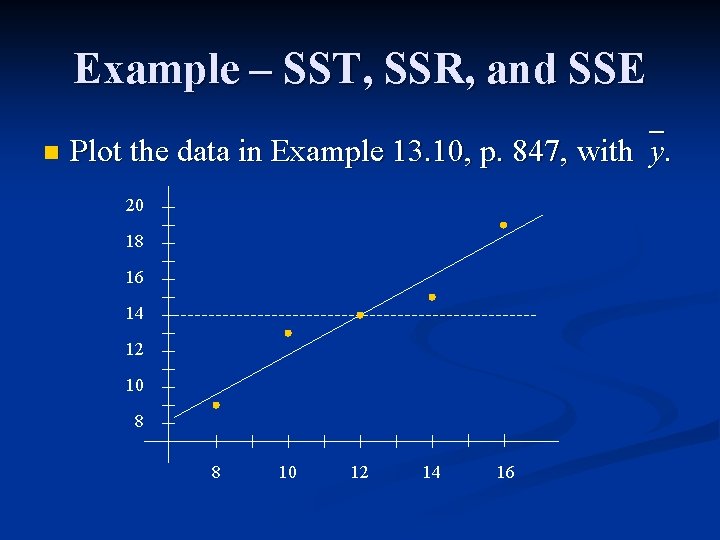
Example – SST, SSR, and SSE n Plot the data in Example 13. 10, p. 847, with y. 20 18 16 14 12 10 8 8 10 12 14 16
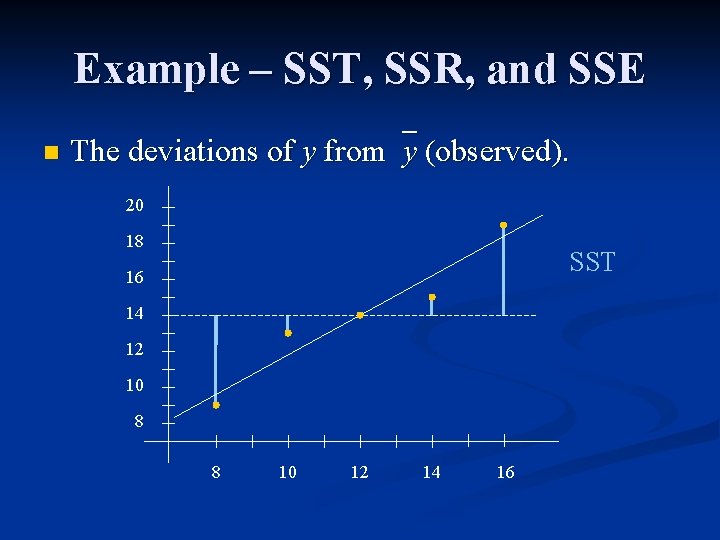
Example – SST, SSR, and SSE n The deviations of y from y (observed). 20 18 SST 16 14 12 10 8 8 10 12 14 16
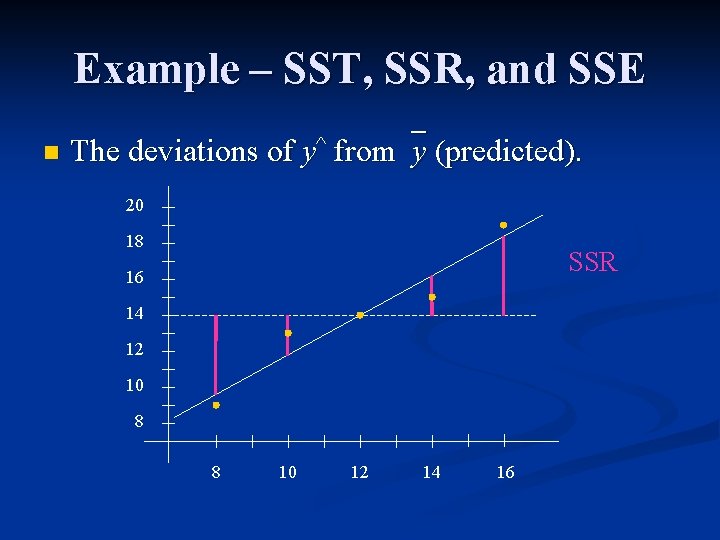
Example – SST, SSR, and SSE n The deviations of y^ from y (predicted). 20 18 SSR 16 14 12 10 8 8 10 12 14 16
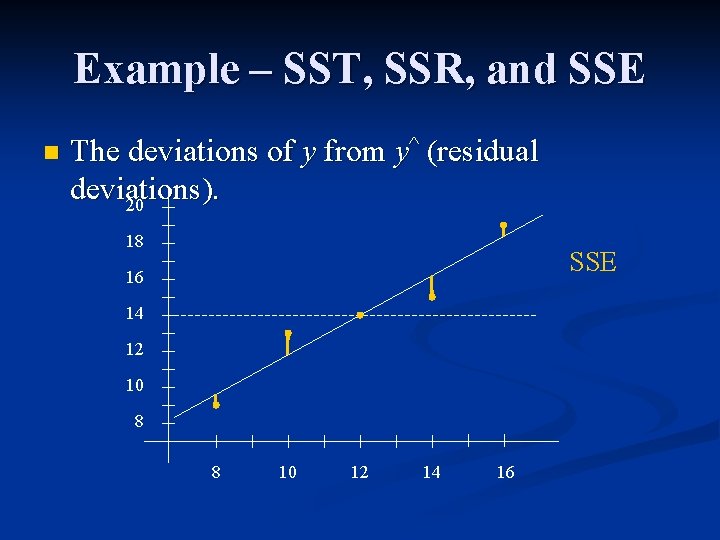
Example – SST, SSR, and SSE n The deviations of y from y^ (residual deviations). 20 18 SSE 16 14 12 10 8 8 10 12 14 16
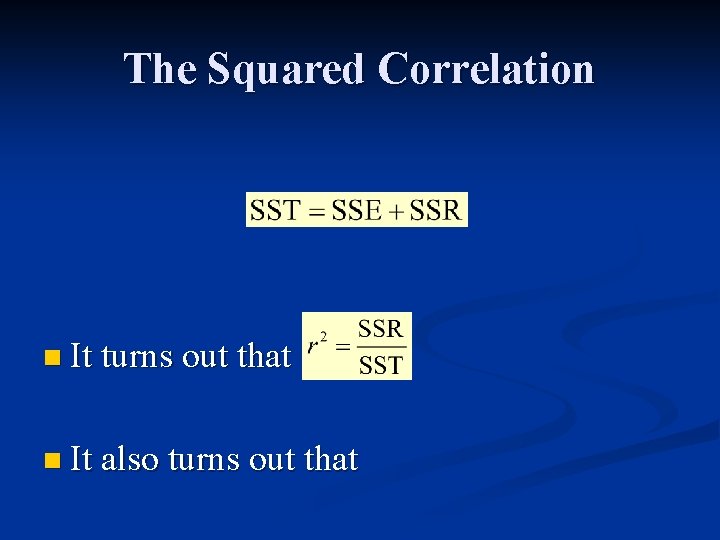
The Squared Correlation n It turns out that n It also turns out that
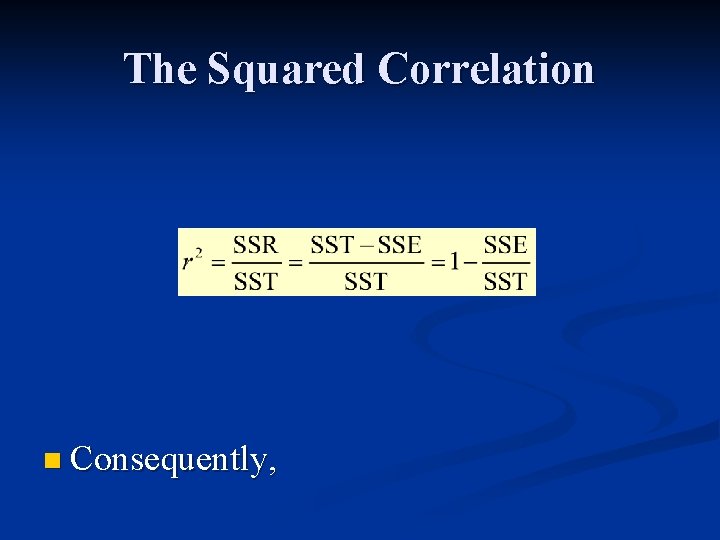
The Squared Correlation n Consequently,
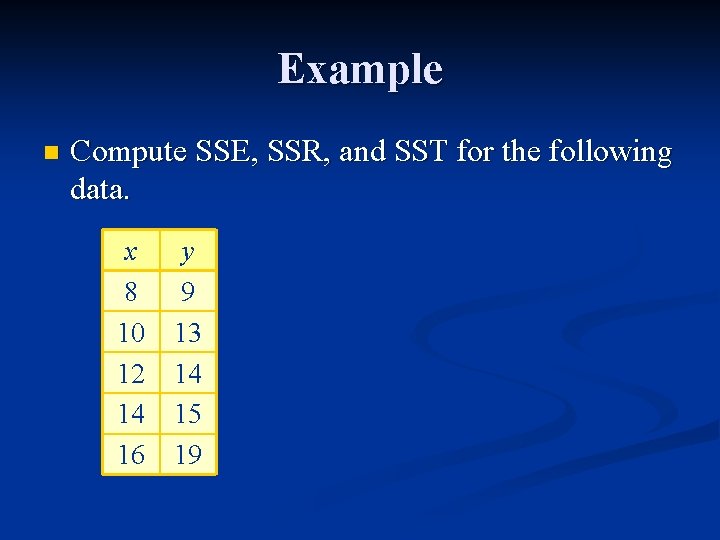
Example n Compute SSE, SSR, and SST for the following data. x 8 10 12 14 16 y 9 13 14 15 19
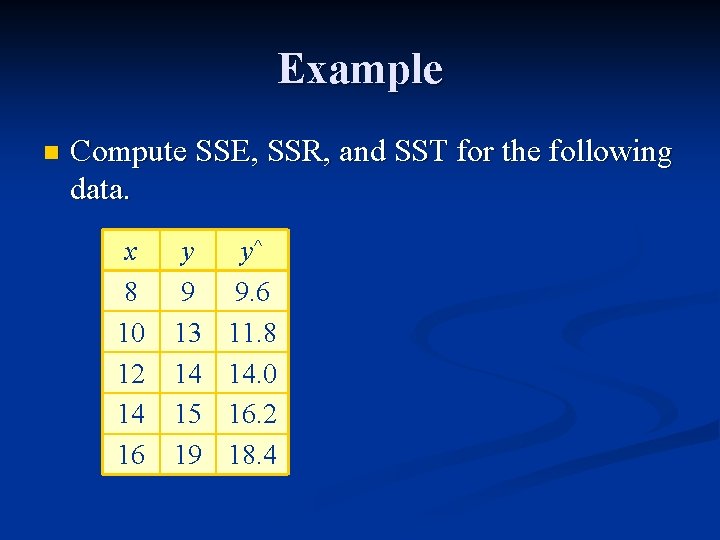
Example n Compute SSE, SSR, and SST for the following data. x 8 10 12 14 16 y 9 13 14 15 19 y^ 9. 6 11. 8 14. 0 16. 2 18. 4
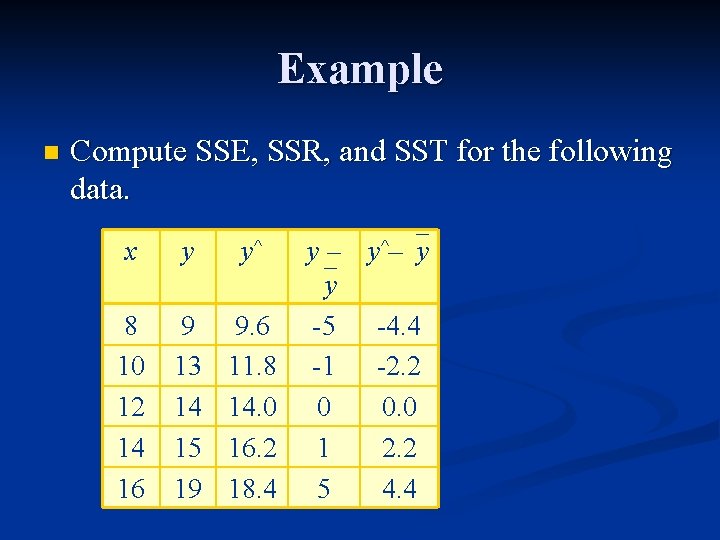
Example n Compute SSE, SSR, and SST for the following data. x y y^ 8 10 12 14 16 9 13 14 15 19 9. 6 11. 8 14. 0 16. 2 18. 4 y – y^– y y -5 -4. 4 -1 -2. 2 0 0. 0 1 2. 2 5 4. 4
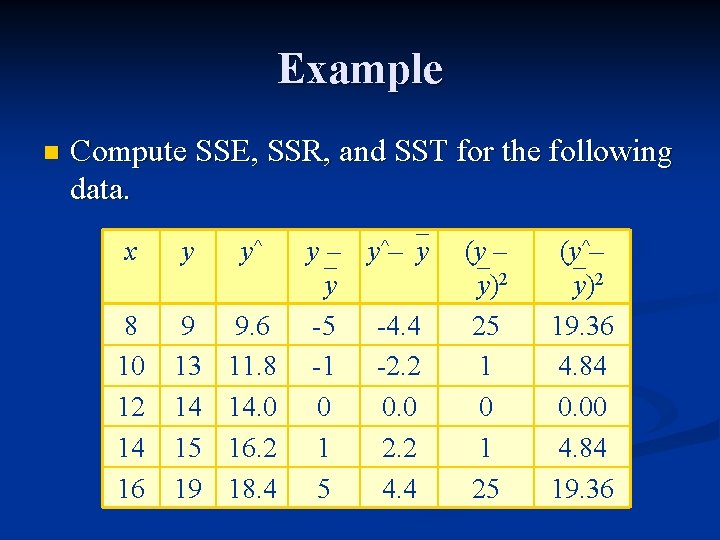
Example n Compute SSE, SSR, and SST for the following data. x y y^ 8 10 12 14 16 9 13 14 15 19 9. 6 11. 8 14. 0 16. 2 18. 4 y – y^– y y -5 -4. 4 -1 -2. 2 0 0. 0 1 2. 2 5 4. 4 (y – y)2 25 1 0 1 25 (y^– y)2 19. 36 4. 84 0. 00 4. 84 19. 36
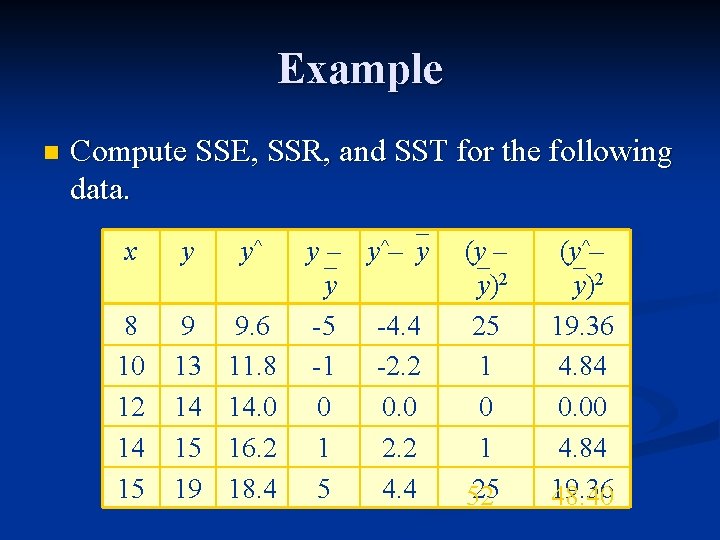
Example n Compute SSE, SSR, and SST for the following data. x y y^ 8 10 12 14 15 9 13 14 15 19 9. 6 11. 8 14. 0 16. 2 18. 4 y – y^– y y -5 -4. 4 -1 -2. 2 0 0. 0 1 2. 2 5 4. 4 (y – y)2 25 1 0 1 25 52 (y^– y)2 19. 36 4. 84 0. 00 4. 84 19. 36 48. 40
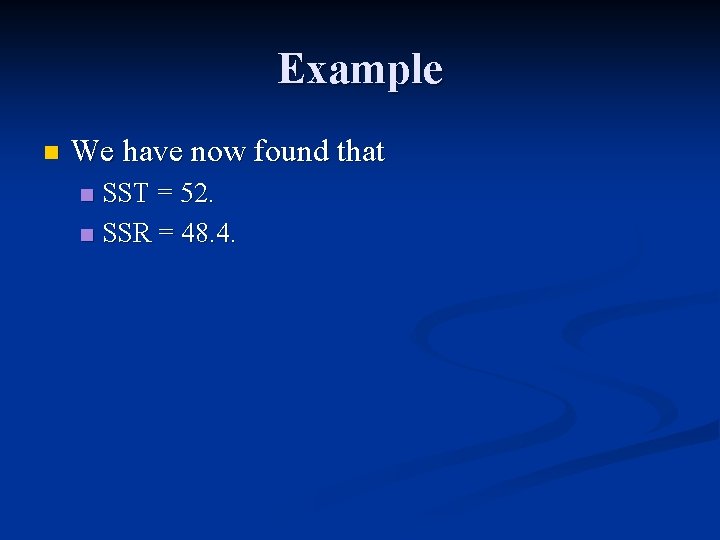
Example n We have now found that SST = 52. n SSR = 48. 4. n
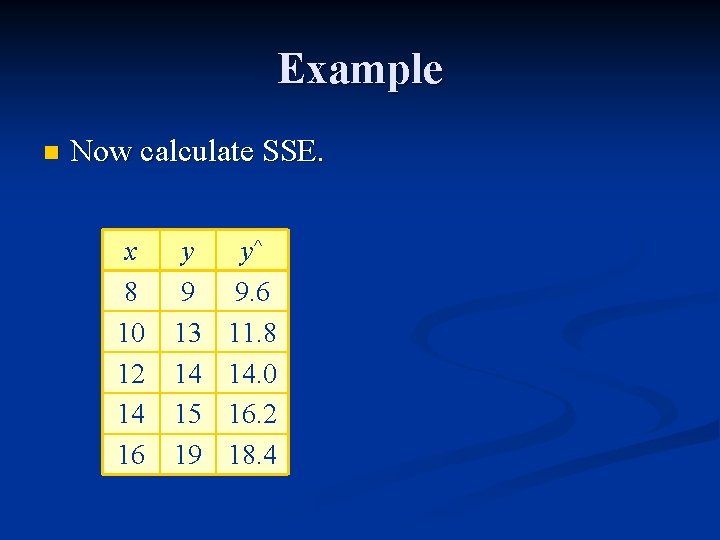
Example n Now calculate SSE. x 8 10 12 14 16 y 9 13 14 15 19 y^ 9. 6 11. 8 14. 0 16. 2 18. 4
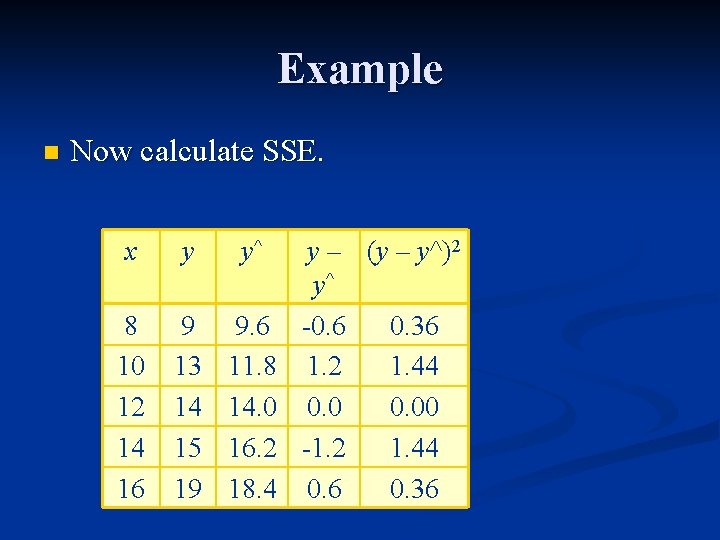
Example n Now calculate SSE. x y y^ 8 10 12 14 16 9 13 14 15 19 9. 6 11. 8 14. 0 16. 2 18. 4 y – (y – y^)2 y^ -0. 6 0. 36 1. 2 1. 44 0. 00 -1. 2 1. 44 0. 6 0. 36
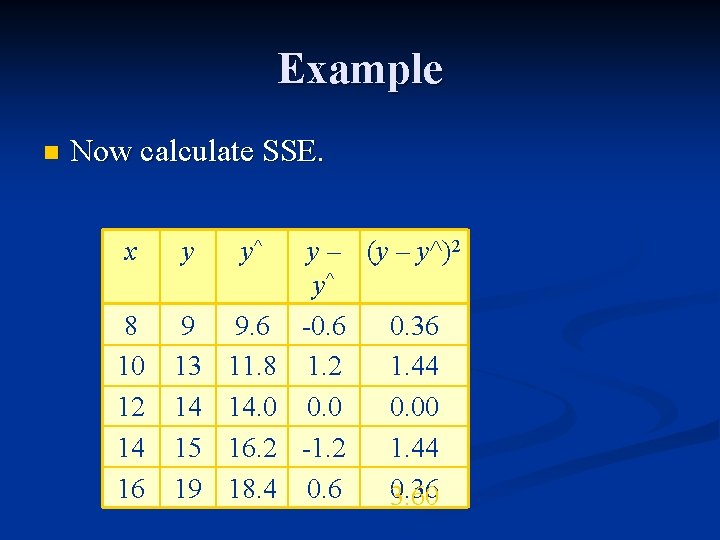
Example n Now calculate SSE. x y y^ 8 10 12 14 16 9 13 14 15 19 9. 6 11. 8 14. 0 16. 2 18. 4 y – (y – y^)2 y^ -0. 6 0. 36 1. 2 1. 44 0. 00 -1. 2 1. 44 0. 6 0. 36 3. 60
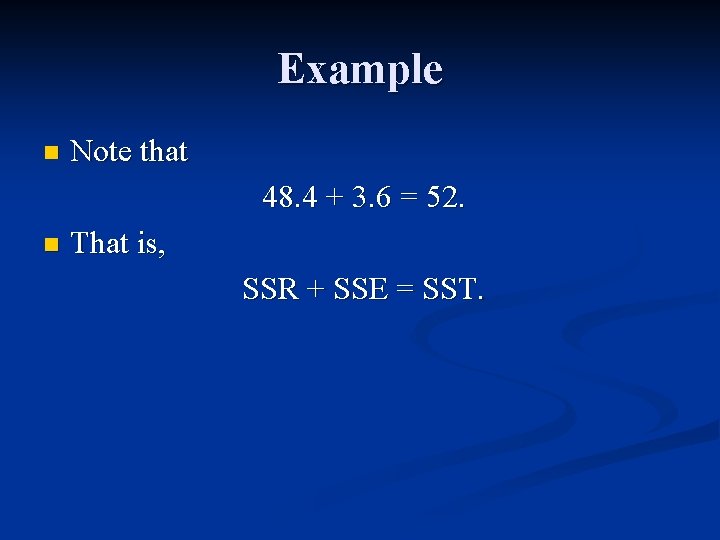
Example n Note that 48. 4 + 3. 6 = 52. n That is, SSR + SSE = SST.
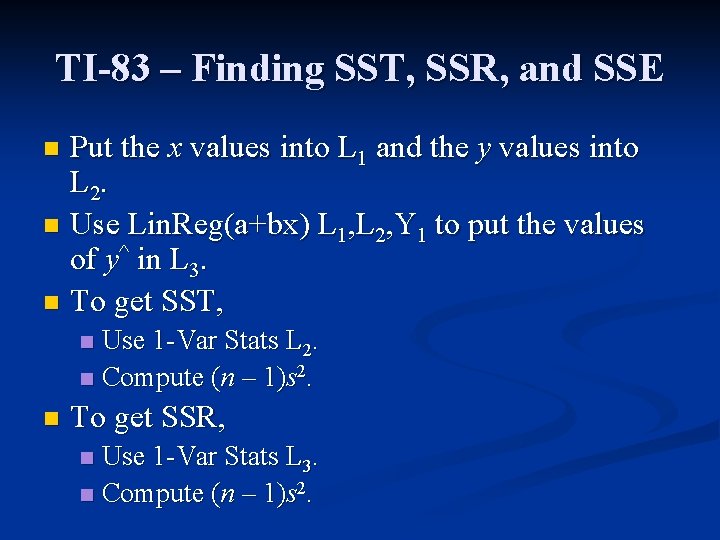
TI-83 – Finding SST, SSR, and SSE Put the x values into L 1 and the y values into L 2. n Use Lin. Reg(a+bx) L 1, L 2, Y 1 to put the values of y^ in L 3. n To get SST, n Use 1 -Var Stats L 2. n Compute (n – 1)s 2. n n To get SSR, Use 1 -Var Stats L 3. n Compute (n – 1)s 2. n
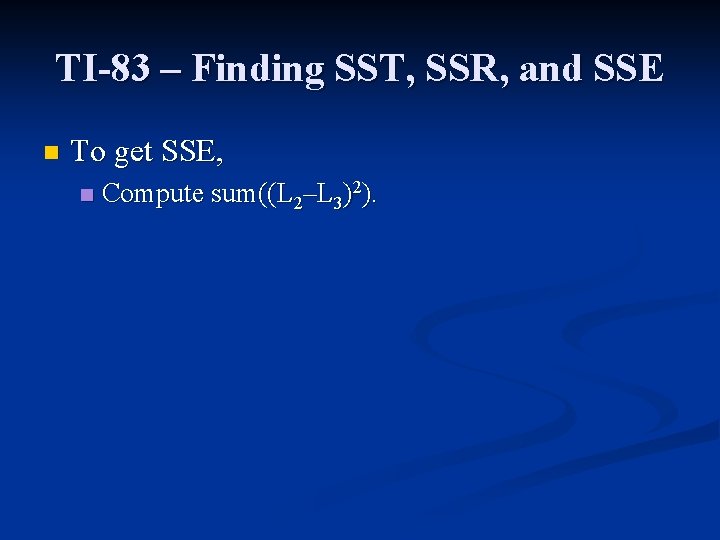
TI-83 – Finding SST, SSR, and SSE n To get SSE, n Compute sum((L 2–L 3)2).
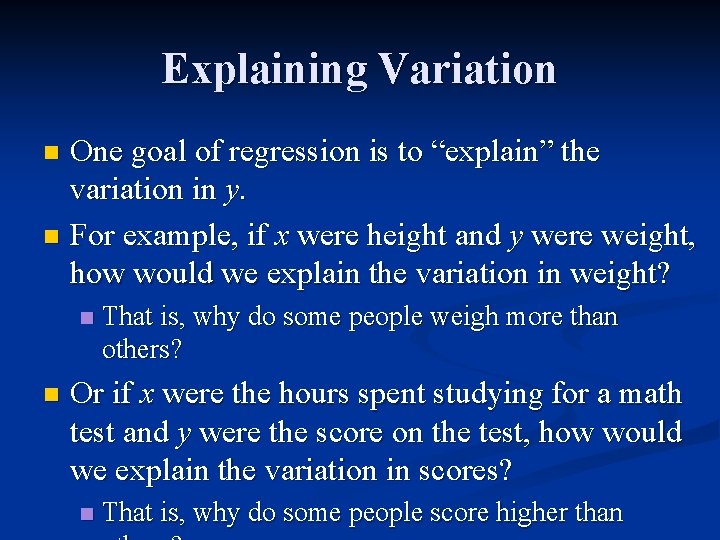
Explaining Variation One goal of regression is to “explain” the variation in y. n For example, if x were height and y were weight, how would we explain the variation in weight? n n n That is, why do some people weigh more than others? Or if x were the hours spent studying for a math test and y were the score on the test, how would we explain the variation in scores? n That is, why do some people score higher than
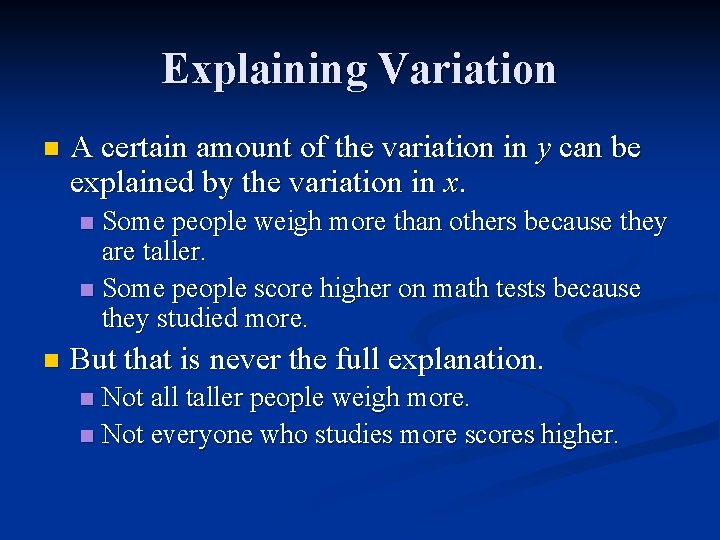
Explaining Variation n A certain amount of the variation in y can be explained by the variation in x. Some people weigh more than others because they are taller. n Some people score higher on math tests because they studied more. n n But that is never the full explanation. Not all taller people weigh more. n Not everyone who studies more scores higher. n
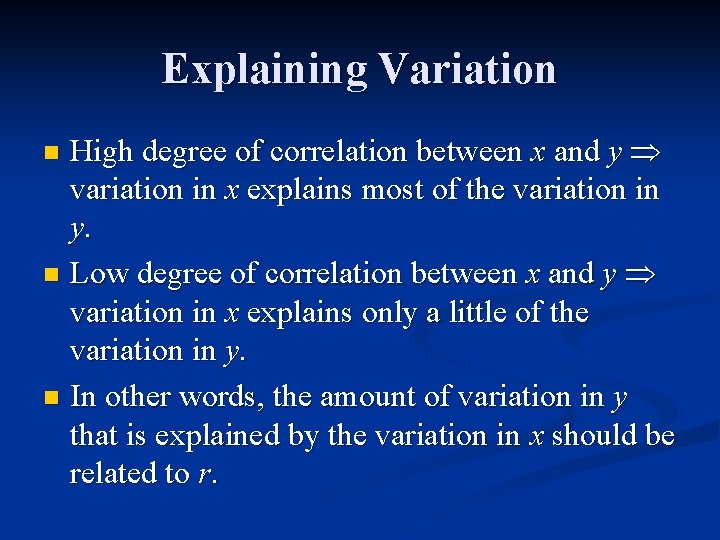
Explaining Variation High degree of correlation between x and y variation in x explains most of the variation in y. n Low degree of correlation between x and y variation in x explains only a little of the variation in y. n In other words, the amount of variation in y that is explained by the variation in x should be related to r. n
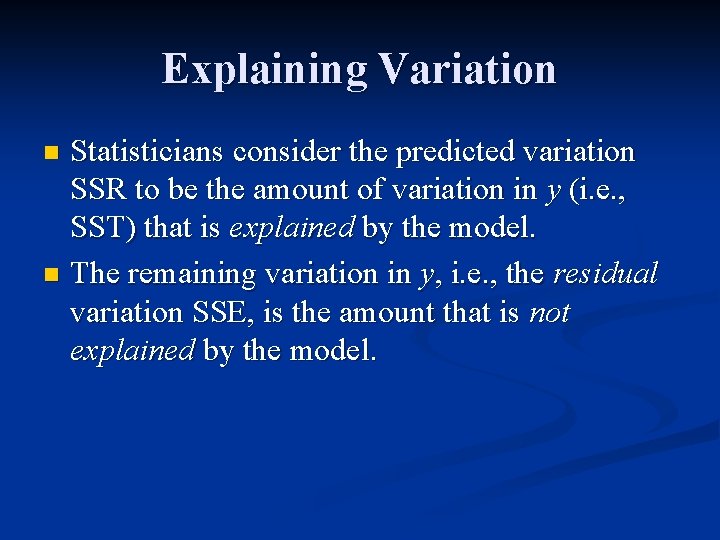
Explaining Variation Statisticians consider the predicted variation SSR to be the amount of variation in y (i. e. , SST) that is explained by the model. n The remaining variation in y, i. e. , the residual variation SSE, is the amount that is not explained by the model. n
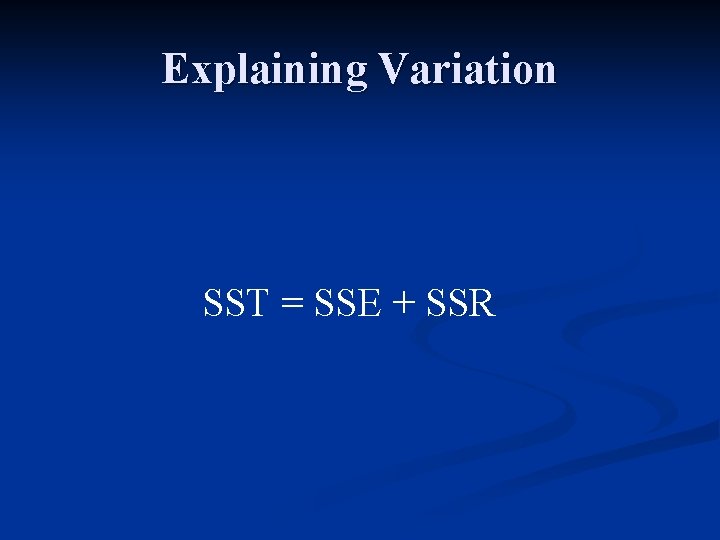
Explaining Variation SST = SSE + SSR
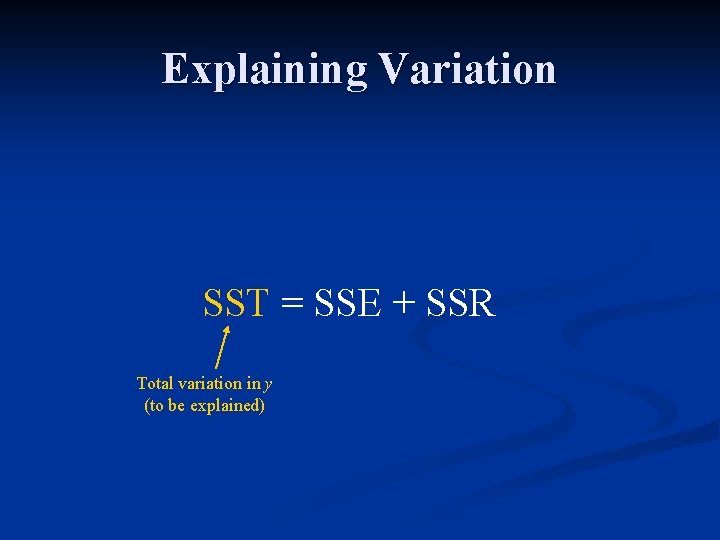
Explaining Variation SST = SSE + SSR Total variation in y (to be explained)
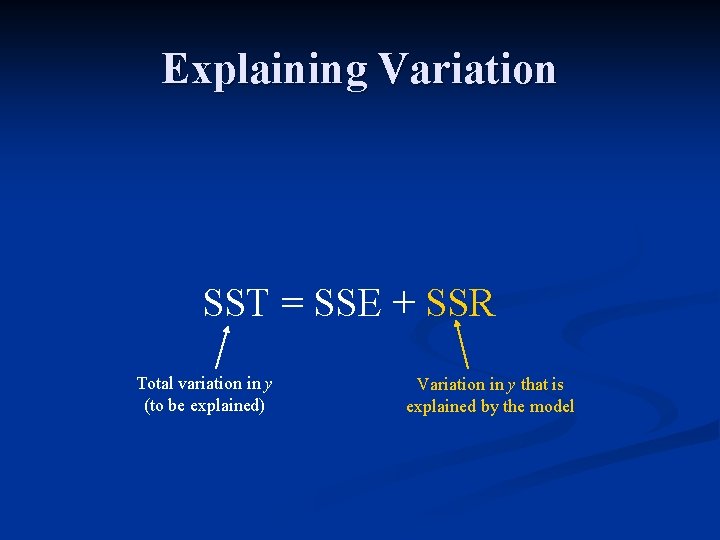
Explaining Variation SST = SSE + SSR Total variation in y (to be explained) Variation in y that is explained by the model
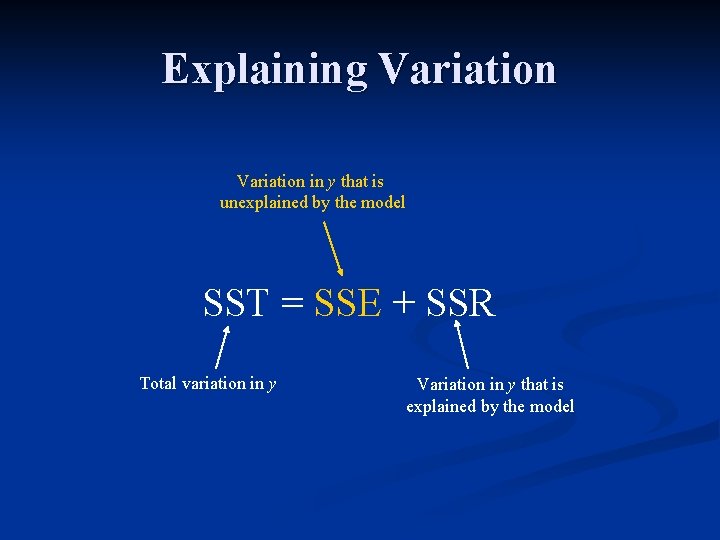
Explaining Variation in y that is unexplained by the model SST = SSE + SSR Total variation in y Variation in y that is explained by the model
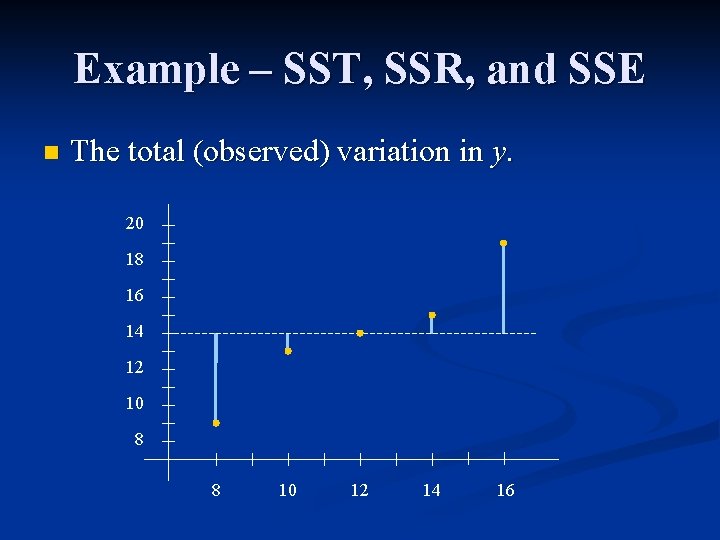
Example – SST, SSR, and SSE n The total (observed) variation in y. 20 18 16 14 12 10 8 8 10 12 14 16
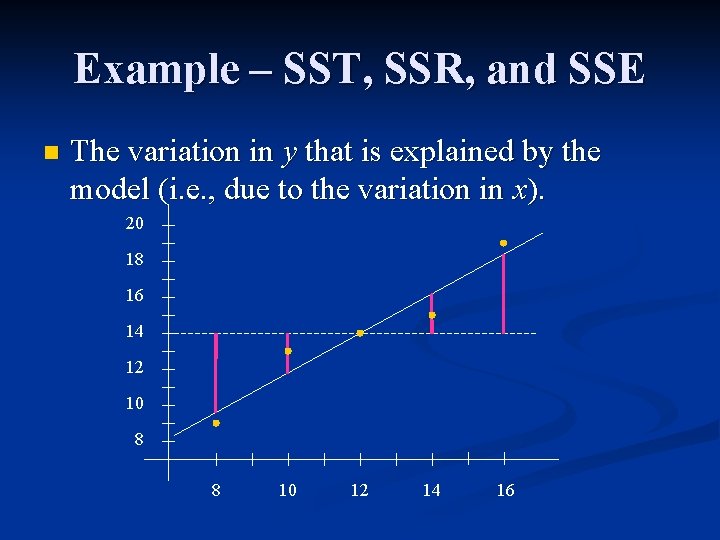
Example – SST, SSR, and SSE n The variation in y that is explained by the model (i. e. , due to the variation in x). 20 18 16 14 12 10 8 8 10 12 14 16
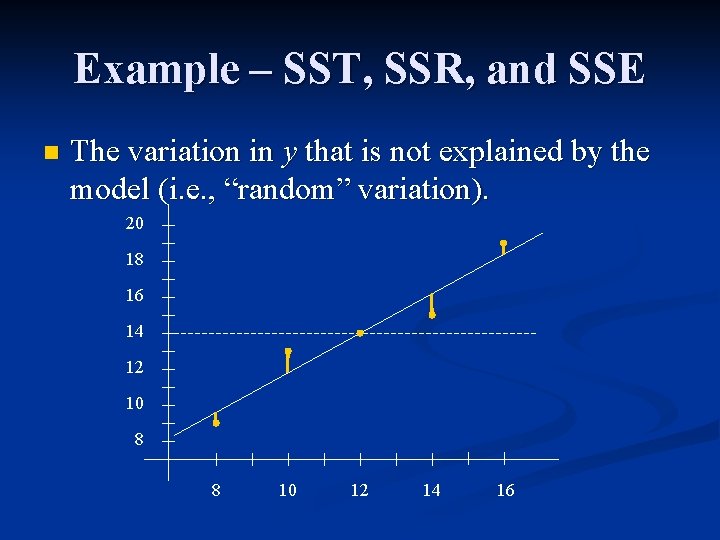
Example – SST, SSR, and SSE n The variation in y that is not explained by the model (i. e. , “random” variation). 20 18 16 14 12 10 8 8 10 12 14 16
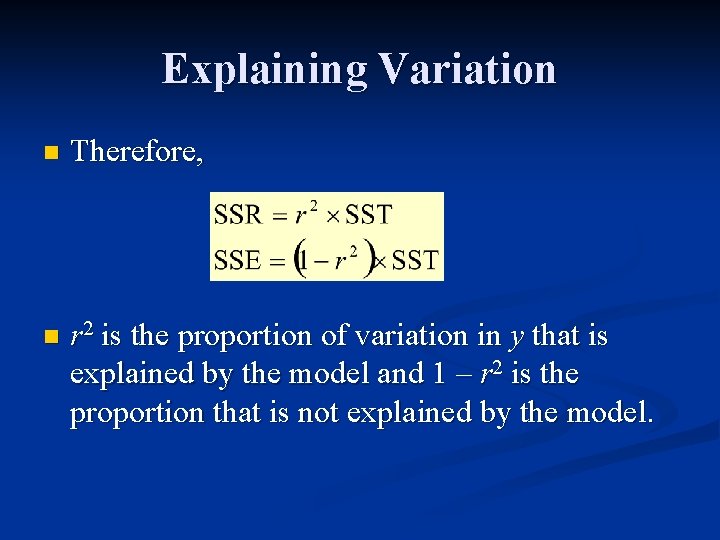
Explaining Variation n Therefore, n r 2 is the proportion of variation in y that is explained by the model and 1 – r 2 is the proportion that is not explained by the model.
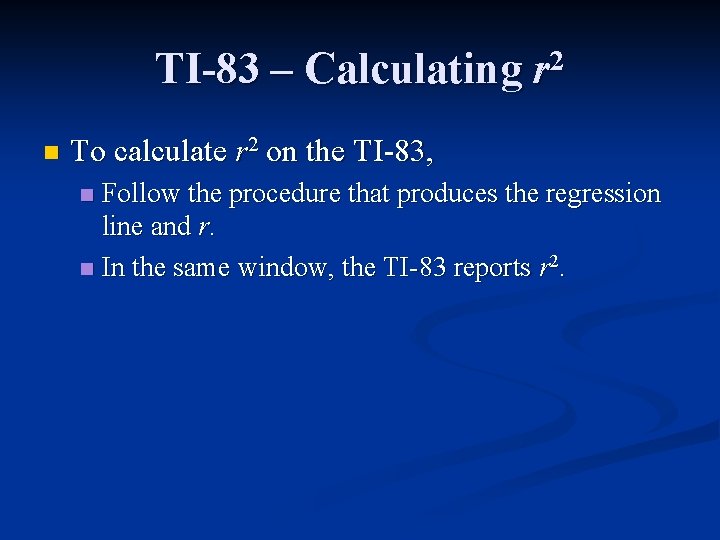
TI-83 – Calculating r 2 n To calculate r 2 on the TI-83, Follow the procedure that produces the regression line and r. n In the same window, the TI-83 reports r 2. n
B squared plus c squared
Correlation and regression
R squared vs correlation coefficient
Positive correlation versus negative correlation
Negative positive no correlation
Tan sec csc
Derivative of sec 2
R squared interpretation
How to calculate r squared
R squared adj
R-squared interpretation example
Knowledge shared is knowledge squared meaning
Cohen manion morrison
Cos 3x formula
Omega squared
Reciprocal squared
Chi square chart ap bio
Critical value chi square
Fundamental identities trig
Is volume squared or cubed
Sampling cohen sample size table
Least squares regression line
Square binomial
What does x cubed look like
Chi square test conclusion
Loaders nfl team
Dmca square root
Cleverness squared puzzle
Test2 chi
Bite with crackling noise candy bar
Square wave fourier
How to know if an effect size is small medium or large
Skipton girls high school term dates
Y=kx squared
Squared paper
How to simplify square root fractions