e Commerce Technology 20 751 Lecture 10 Mass
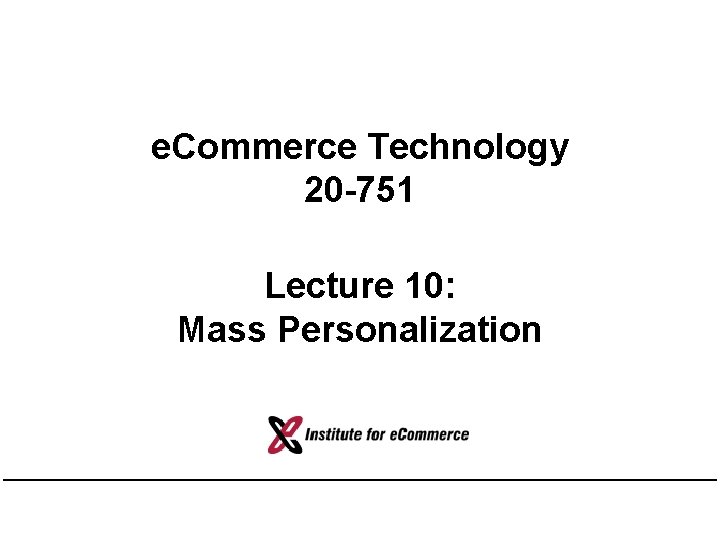
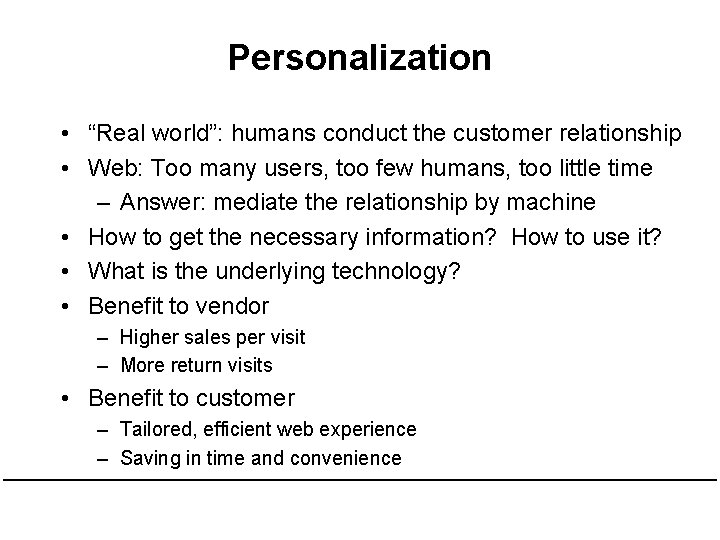
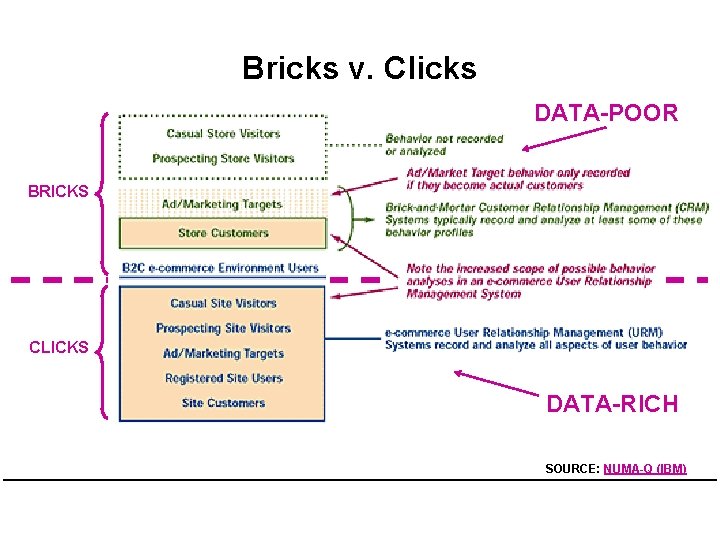
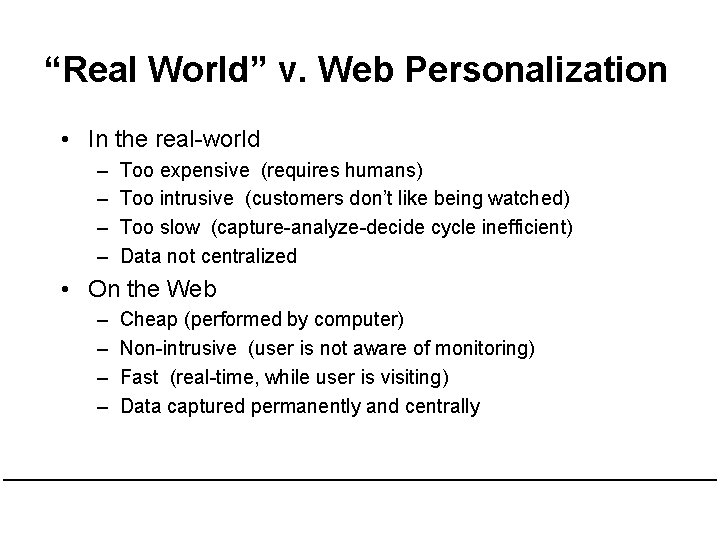
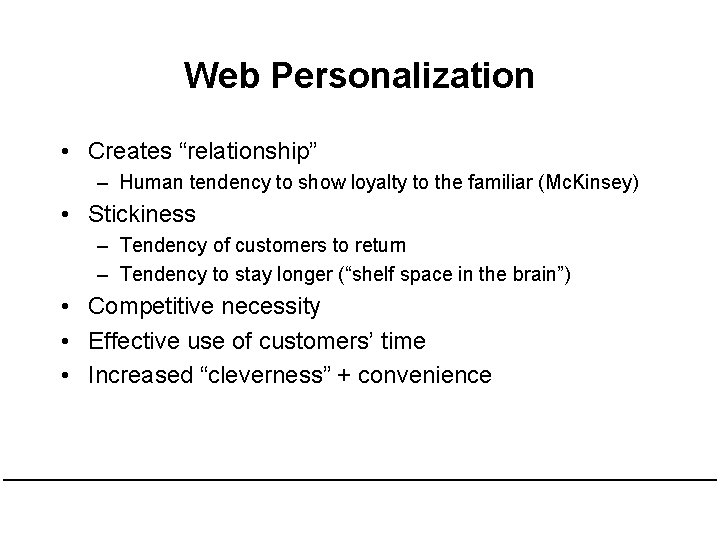
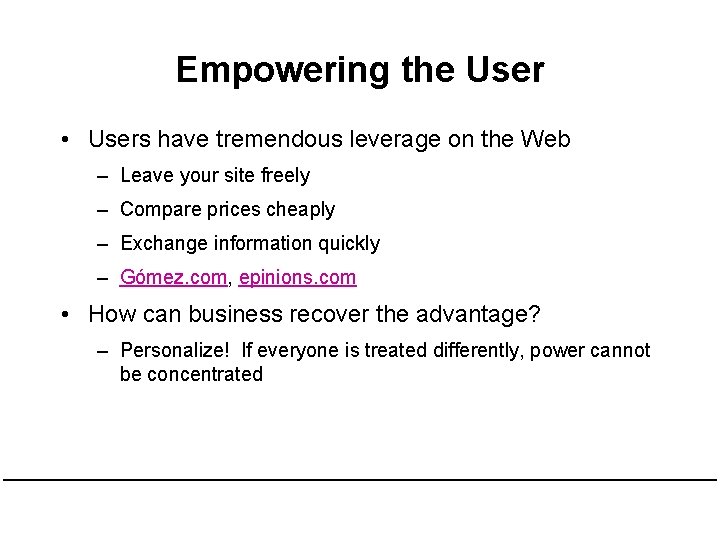
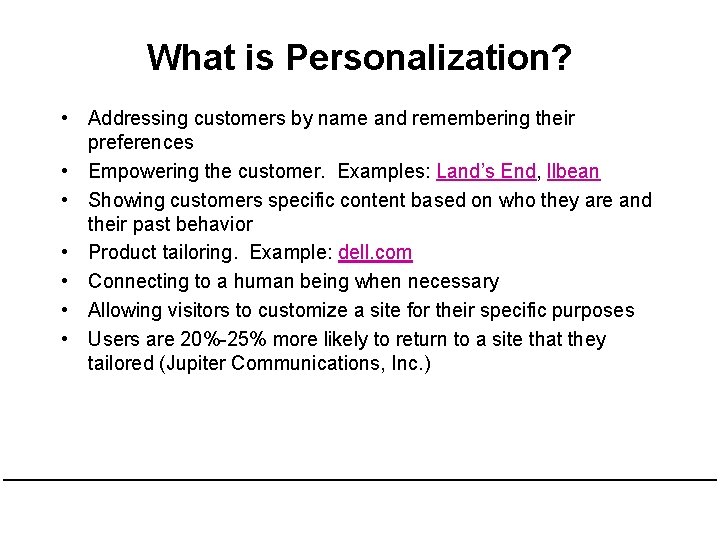
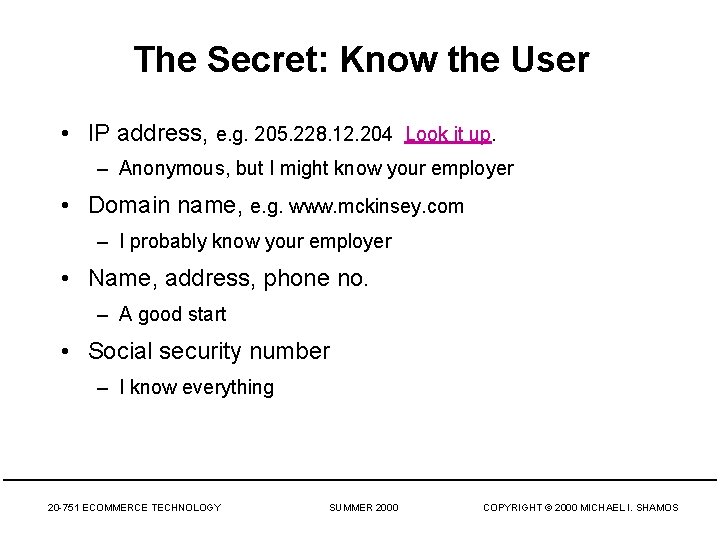
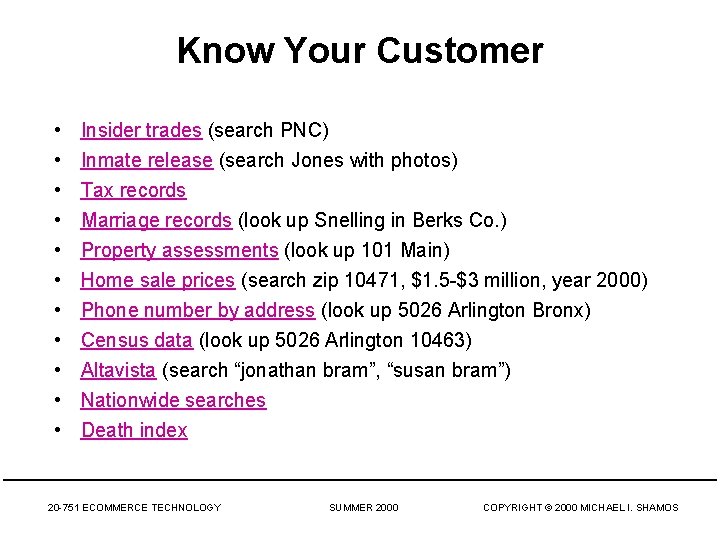
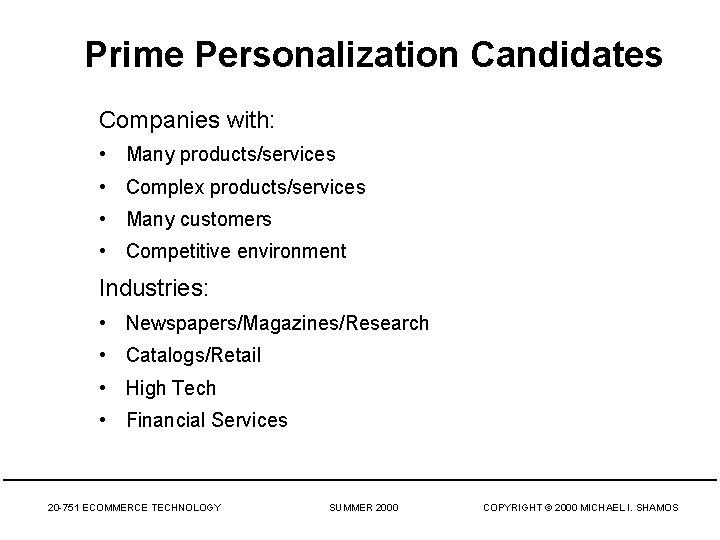
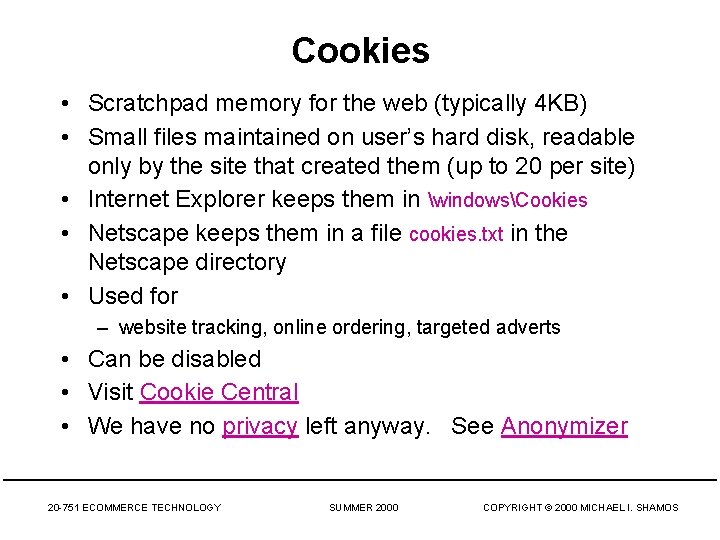
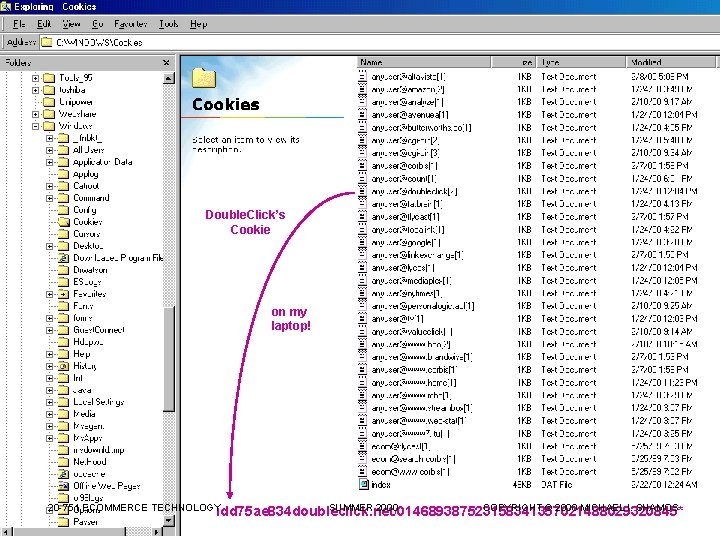
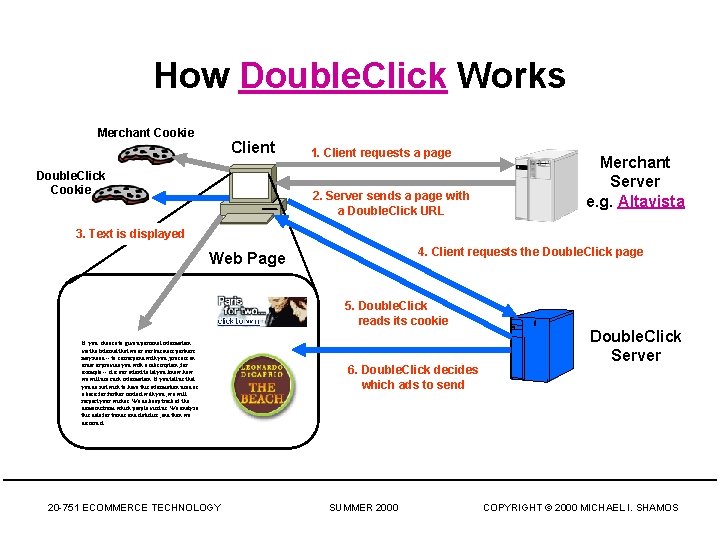
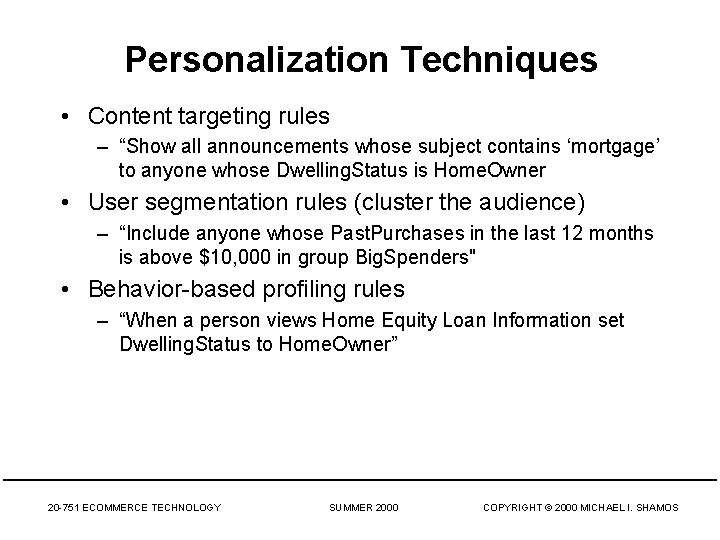
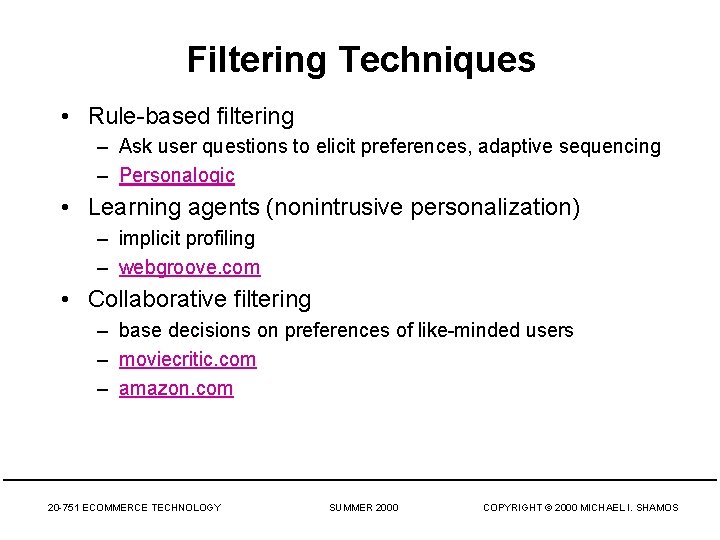
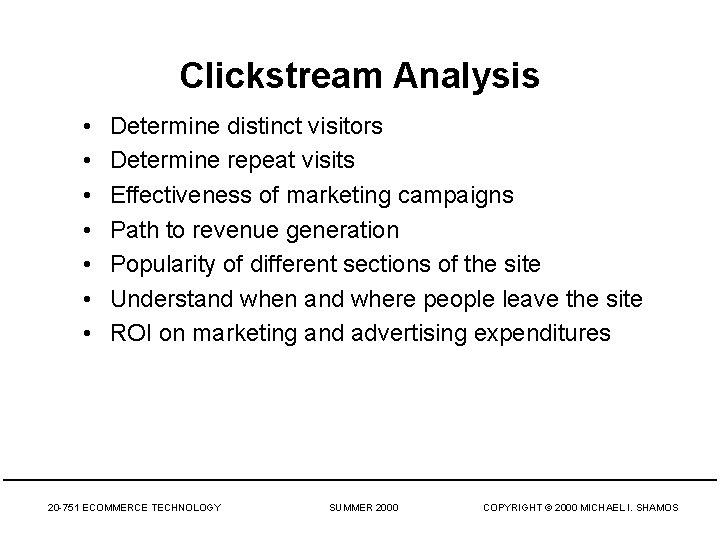
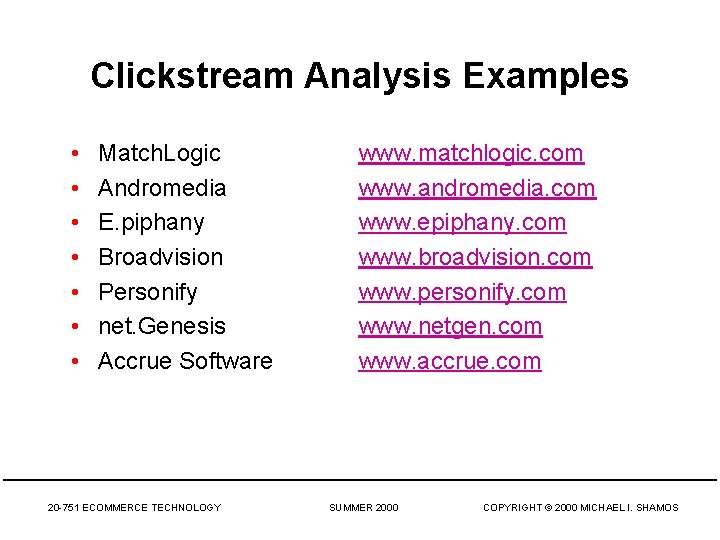
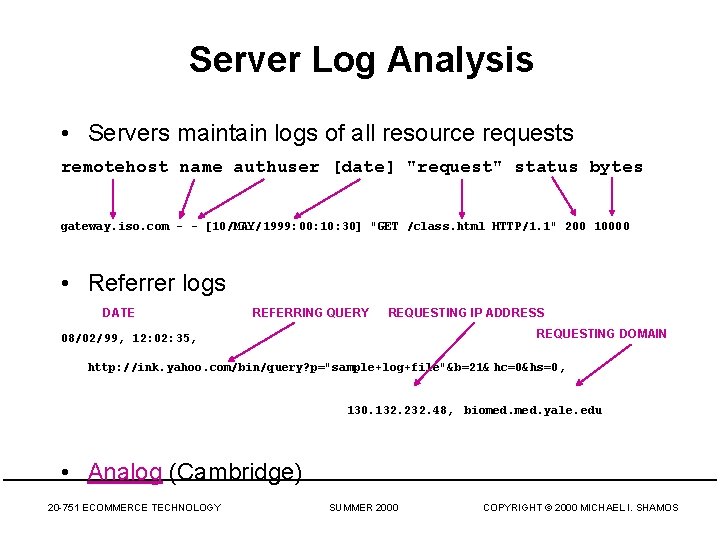
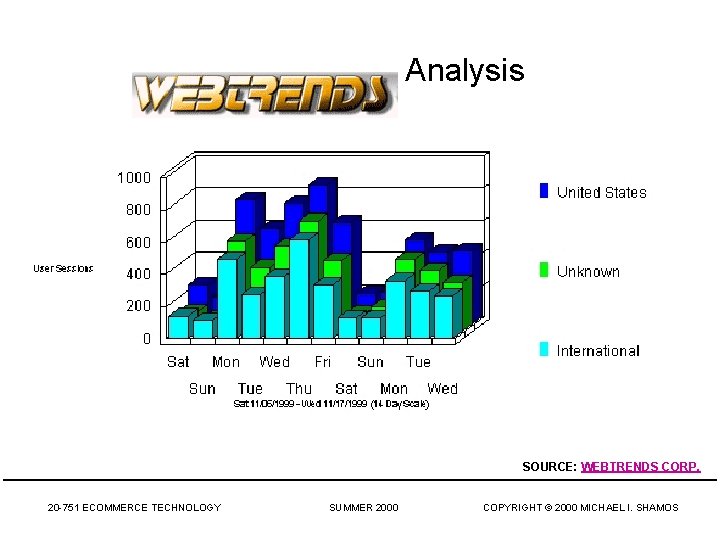
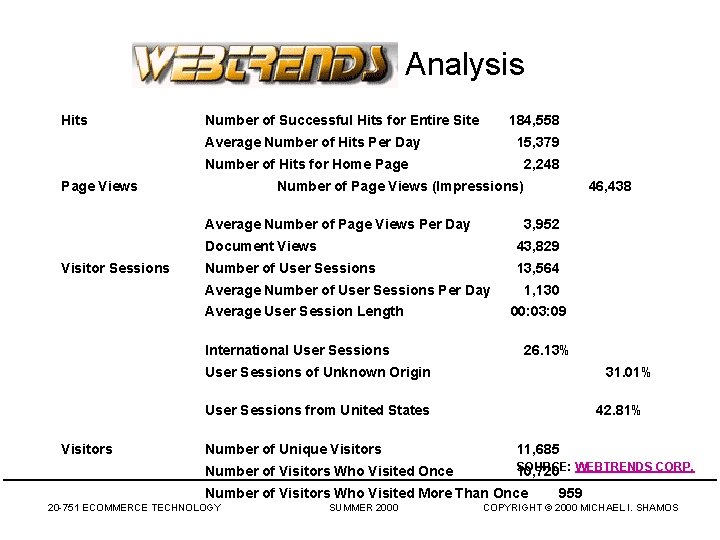
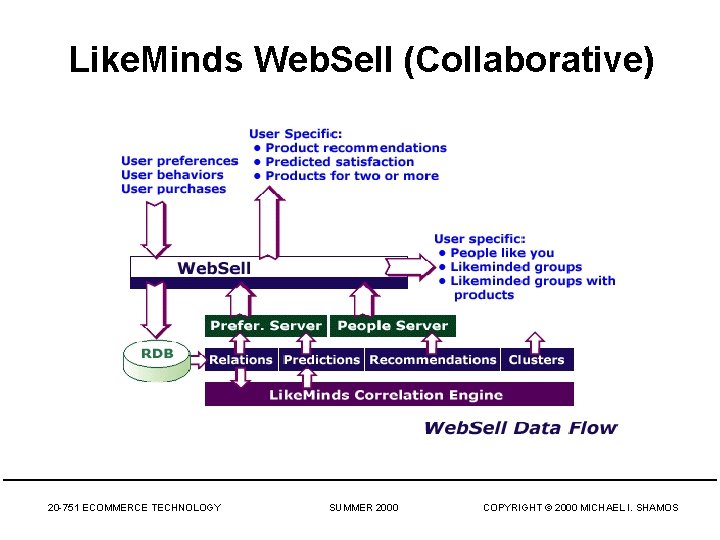
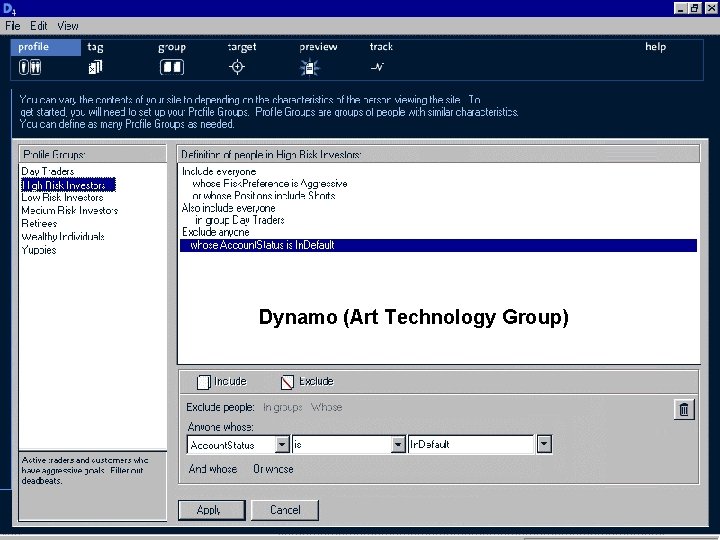
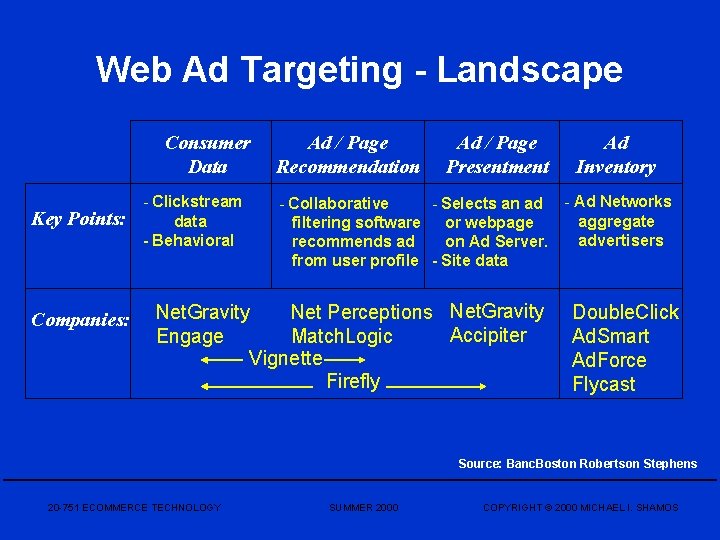
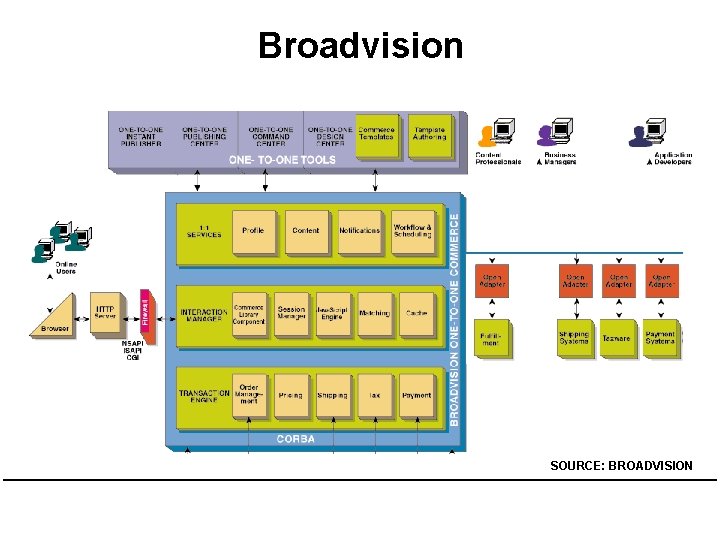
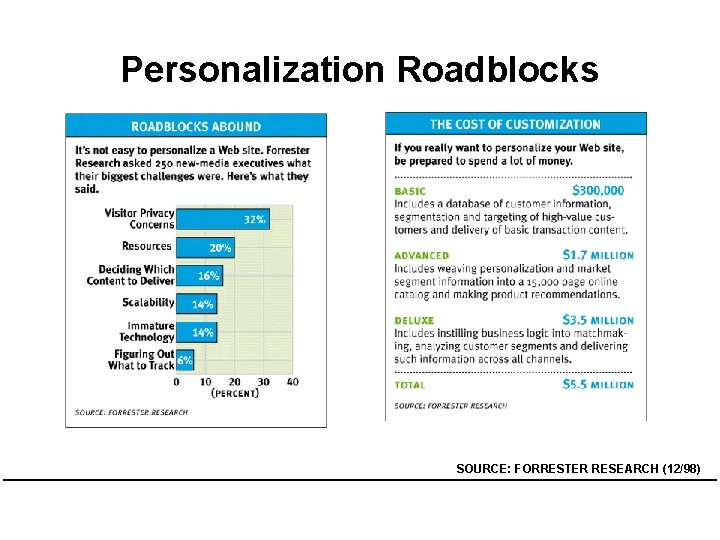
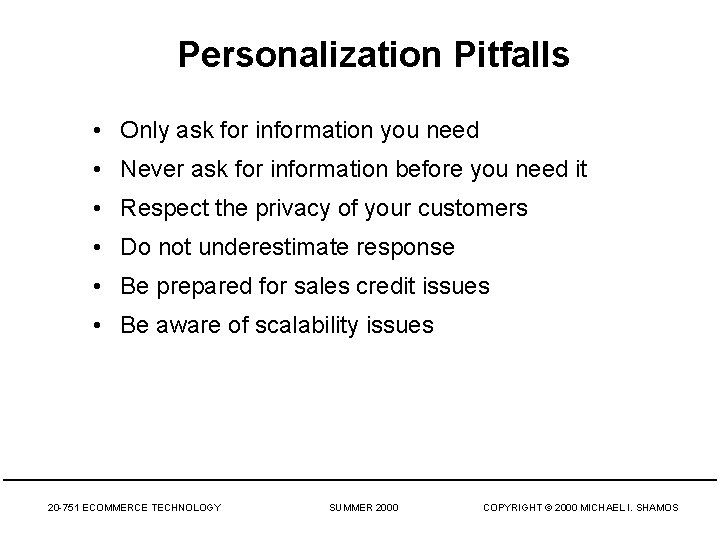
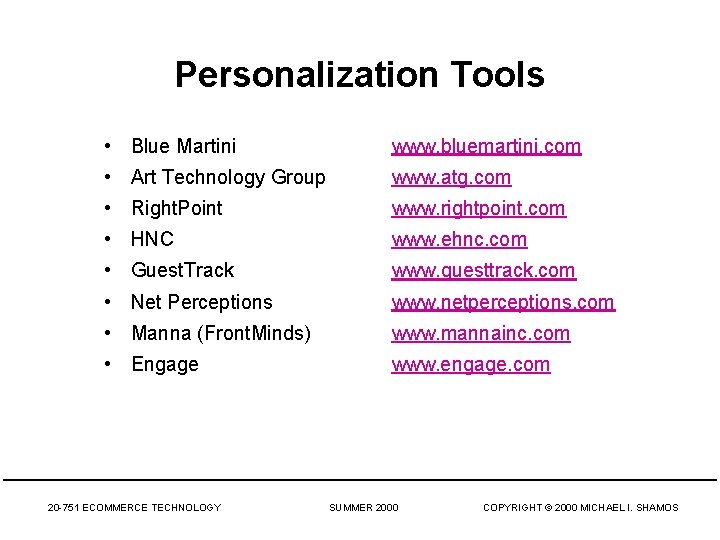
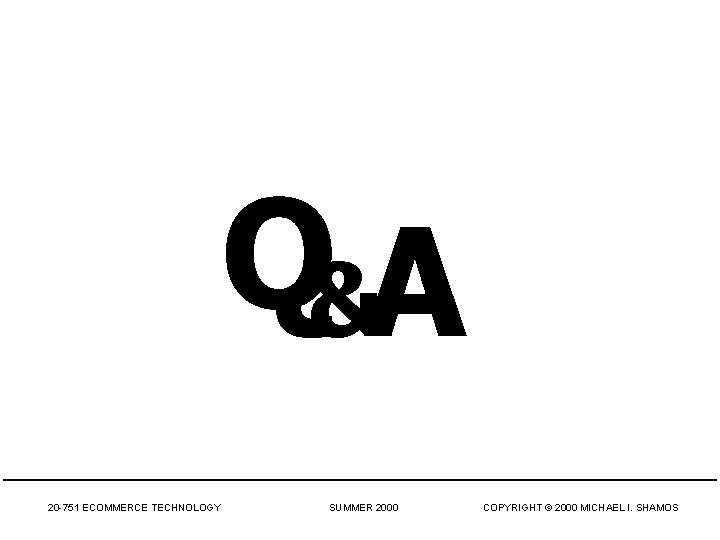
- Slides: 28
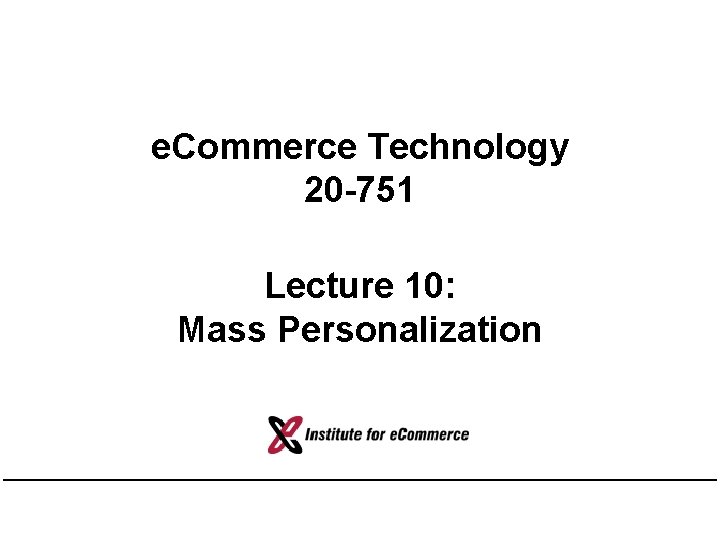
e. Commerce Technology 20 -751 Lecture 10: Mass Personalization
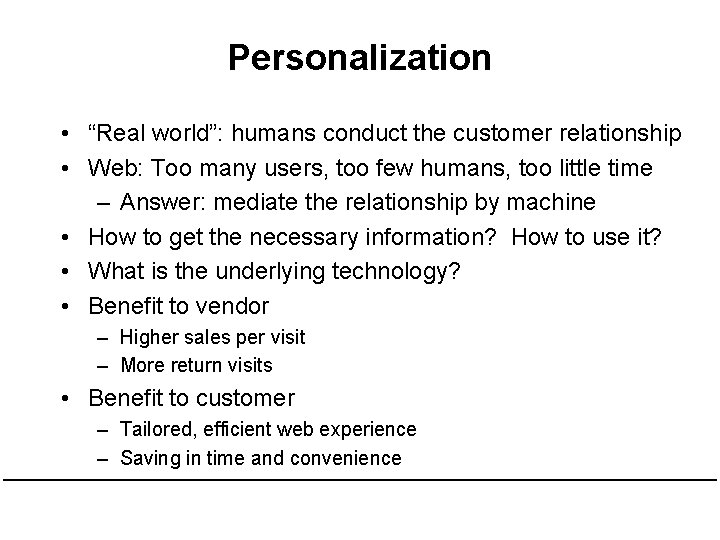
Personalization • “Real world”: humans conduct the customer relationship • Web: Too many users, too few humans, too little time – Answer: mediate the relationship by machine • How to get the necessary information? How to use it? • What is the underlying technology? • Benefit to vendor – Higher sales per visit – More return visits • Benefit to customer – Tailored, efficient web experience – Saving in time and convenience
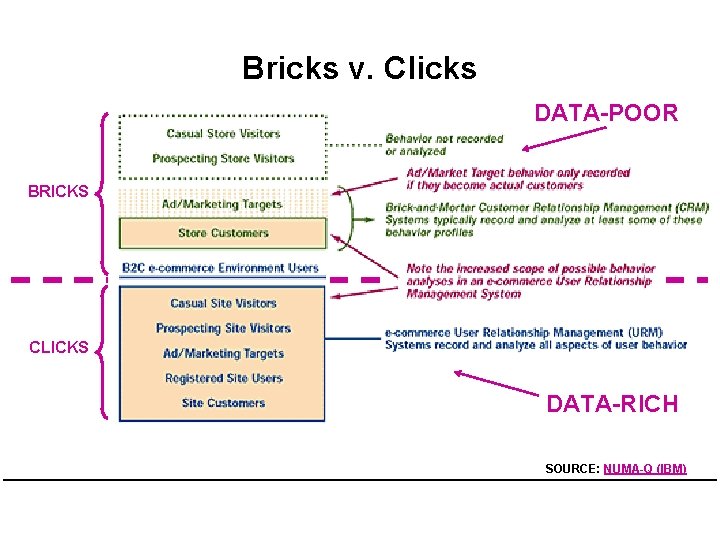
Bricks v. Clicks DATA-POOR BRICKS CLICKS DATA-RICH SOURCE: NUMA-Q (IBM)
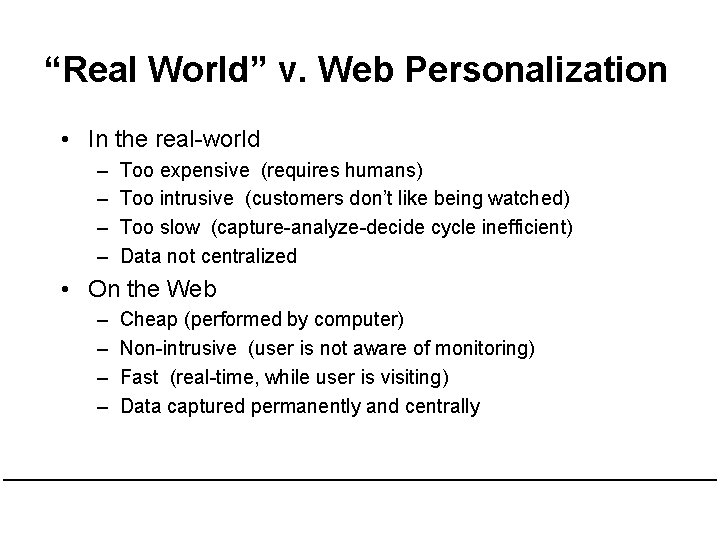
“Real World” v. Web Personalization • In the real-world – – Too expensive (requires humans) Too intrusive (customers don’t like being watched) Too slow (capture-analyze-decide cycle inefficient) Data not centralized • On the Web – – Cheap (performed by computer) Non-intrusive (user is not aware of monitoring) Fast (real-time, while user is visiting) Data captured permanently and centrally
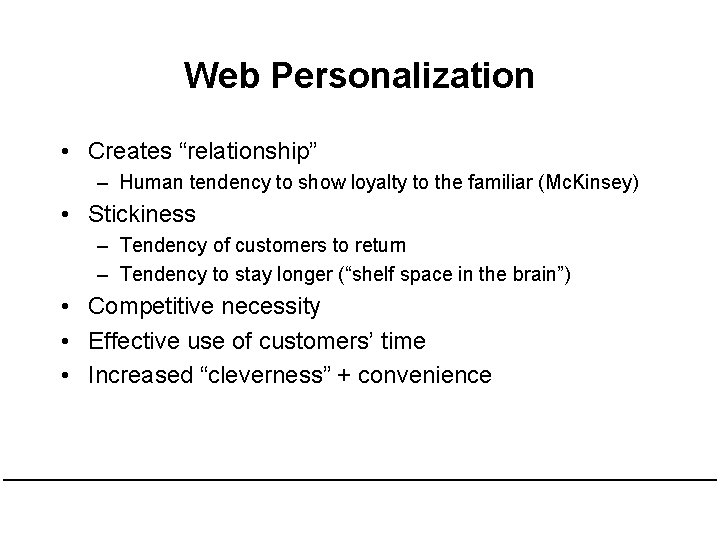
Web Personalization • Creates “relationship” – Human tendency to show loyalty to the familiar (Mc. Kinsey) • Stickiness – Tendency of customers to return – Tendency to stay longer (“shelf space in the brain”) • Competitive necessity • Effective use of customers’ time • Increased “cleverness” + convenience
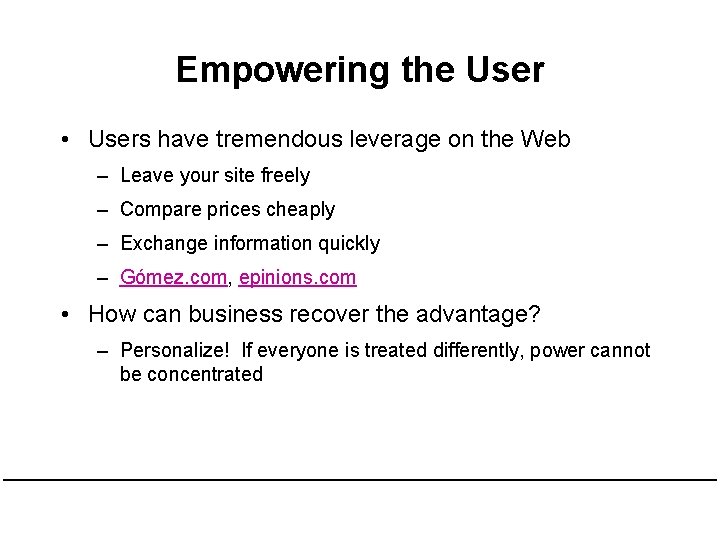
Empowering the User • Users have tremendous leverage on the Web – Leave your site freely – Compare prices cheaply – Exchange information quickly – Gómez. com, epinions. com • How can business recover the advantage? – Personalize! If everyone is treated differently, power cannot be concentrated
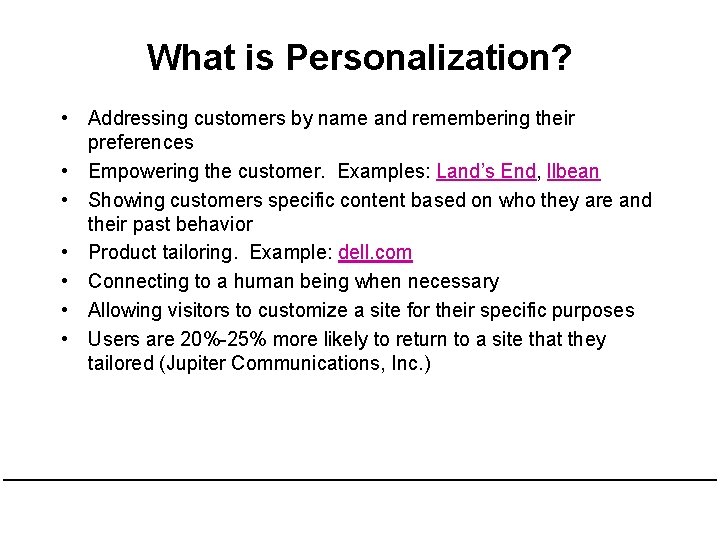
What is Personalization? • Addressing customers by name and remembering their preferences • Empowering the customer. Examples: Land’s End, llbean • Showing customers specific content based on who they are and their past behavior • Product tailoring. Example: dell. com • Connecting to a human being when necessary • Allowing visitors to customize a site for their specific purposes • Users are 20%-25% more likely to return to a site that they tailored (Jupiter Communications, Inc. )
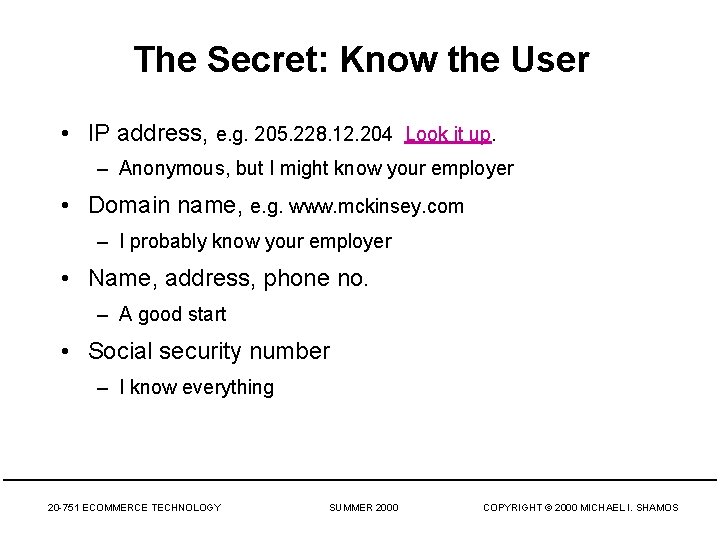
The Secret: Know the User • IP address, e. g. 205. 228. 12. 204 Look it up. – Anonymous, but I might know your employer • Domain name, e. g. www. mckinsey. com – I probably know your employer • Name, address, phone no. – A good start • Social security number – I know everything 20 -751 ECOMMERCE TECHNOLOGY SUMMER 2000 COPYRIGHT © 2000 MICHAEL I. SHAMOS
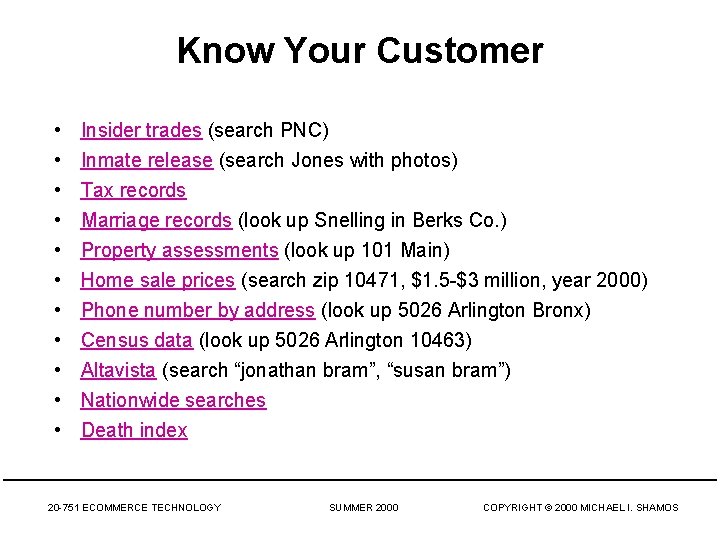
Know Your Customer • • • Insider trades (search PNC) Inmate release (search Jones with photos) Tax records Marriage records (look up Snelling in Berks Co. ) Property assessments (look up 101 Main) Home sale prices (search zip 10471, $1. 5 -$3 million, year 2000) Phone number by address (look up 5026 Arlington Bronx) Census data (look up 5026 Arlington 10463) Altavista (search “jonathan bram”, “susan bram”) Nationwide searches Death index 20 -751 ECOMMERCE TECHNOLOGY SUMMER 2000 COPYRIGHT © 2000 MICHAEL I. SHAMOS
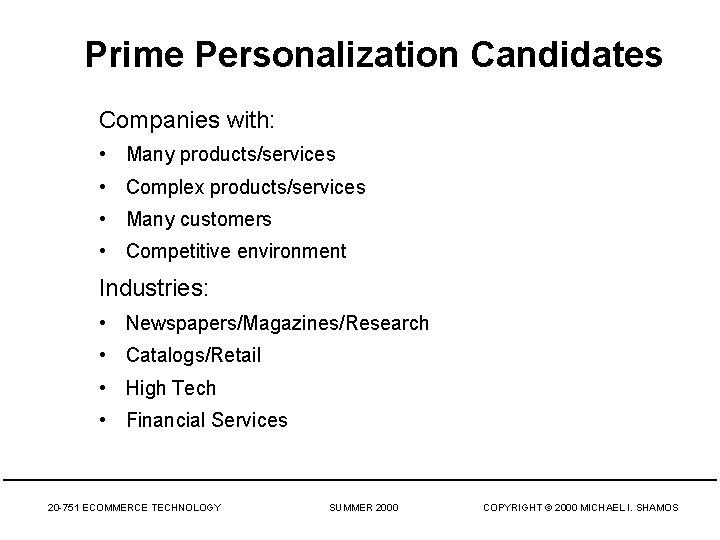
Prime Personalization Candidates Companies with: • Many products/services • Complex products/services • Many customers • Competitive environment Industries: • Newspapers/Magazines/Research • Catalogs/Retail • High Tech • Financial Services 20 -751 ECOMMERCE TECHNOLOGY SUMMER 2000 COPYRIGHT © 2000 MICHAEL I. SHAMOS
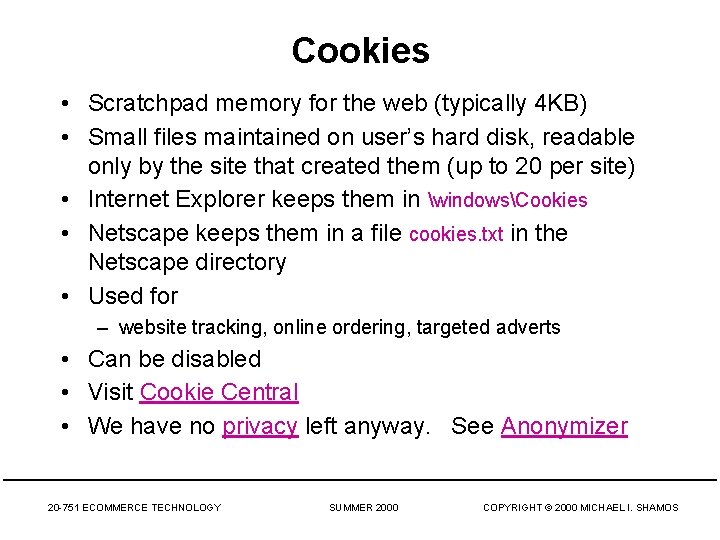
Cookies • Scratchpad memory for the web (typically 4 KB) • Small files maintained on user’s hard disk, readable only by the site that created them (up to 20 per site) • Internet Explorer keeps them in windowsCookies • Netscape keeps them in a file cookies. txt in the Netscape directory • Used for – website tracking, online ordering, targeted adverts • Can be disabled • Visit Cookie Central • We have no privacy left anyway. See Anonymizer 20 -751 ECOMMERCE TECHNOLOGY SUMMER 2000 COPYRIGHT © 2000 MICHAEL I. SHAMOS
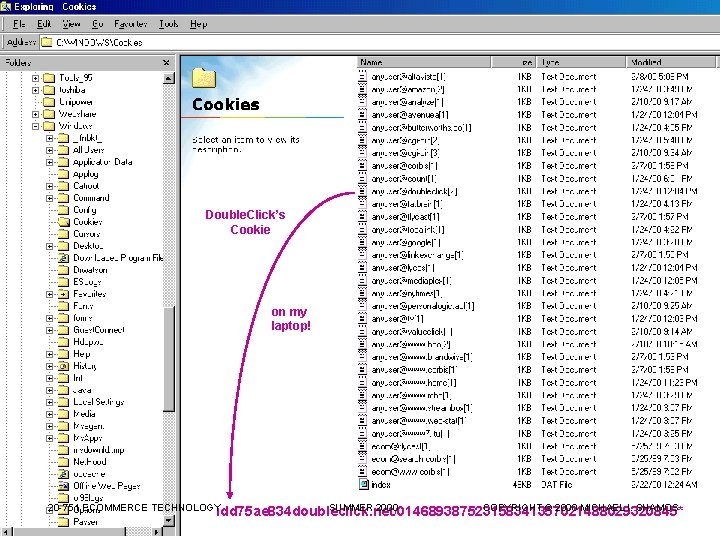
Double. Click’s Cookie on my laptop! 20 -751 ECOMMERCE TECHNOLOGYidd 75 ae 834 doubleclick. net/014689387523158341357021488029320845* SUMMER 2000 COPYRIGHT © 2000 MICHAEL I. SHAMOS
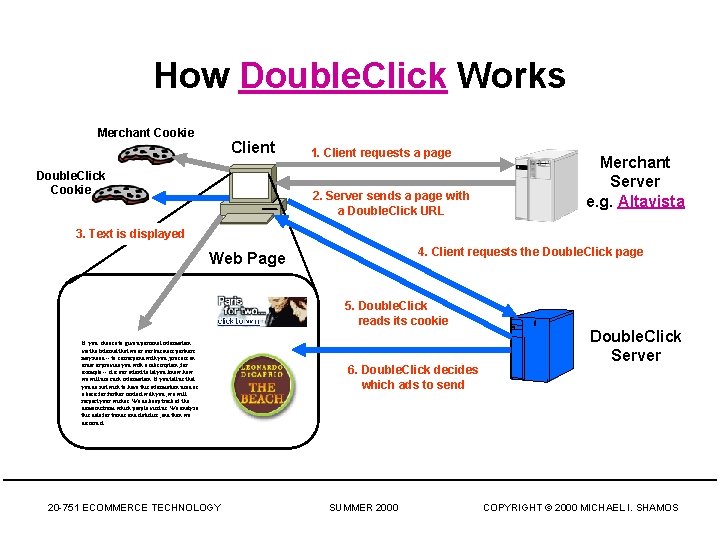
How Double. Click Works Merchant Cookie Client Double. Click Cookie 1. Client requests a page 2. Server sends a page with a Double. Click URL Merchant Server e. g. Altavista 3. Text is displayed 4. Client requests the Double. Click page Web Page 5. Double. Click reads its cookie If you choose to give u personal information via the Internet that we or our business partners may need -- to correspond with you, process an order or provide you with a subscription, for example -- it is our intent to let you know how we will use such information. If you tell us that you do not wish to have this information used as a basis for further contact with you, we will respect your wishes. We do keep track of the domains from which people visit us. We analyze this data for trends and statistics, and then we discard it. 20 -751 ECOMMERCE TECHNOLOGY 6. Double. Click decides which ads to send SUMMER 2000 Double. Click Server COPYRIGHT © 2000 MICHAEL I. SHAMOS
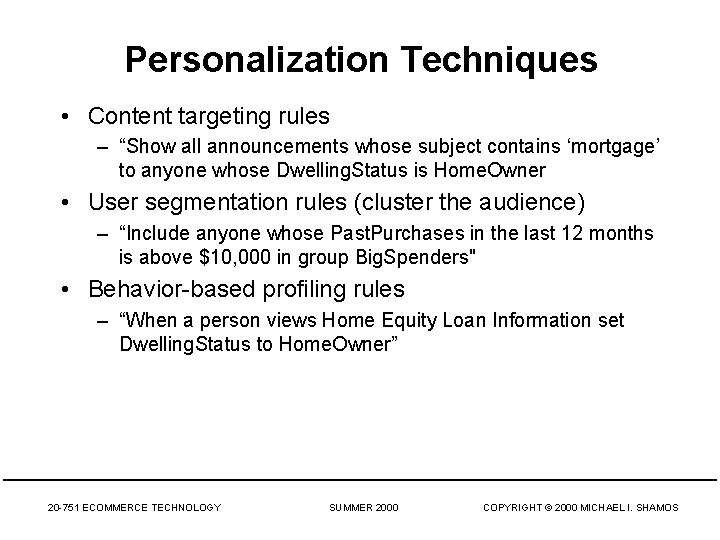
Personalization Techniques • Content targeting rules – “Show all announcements whose subject contains ‘mortgage’ to anyone whose Dwelling. Status is Home. Owner • User segmentation rules (cluster the audience) – “Include anyone whose Past. Purchases in the last 12 months is above $10, 000 in group Big. Spenders" • Behavior-based profiling rules – “When a person views Home Equity Loan Information set Dwelling. Status to Home. Owner” 20 -751 ECOMMERCE TECHNOLOGY SUMMER 2000 COPYRIGHT © 2000 MICHAEL I. SHAMOS
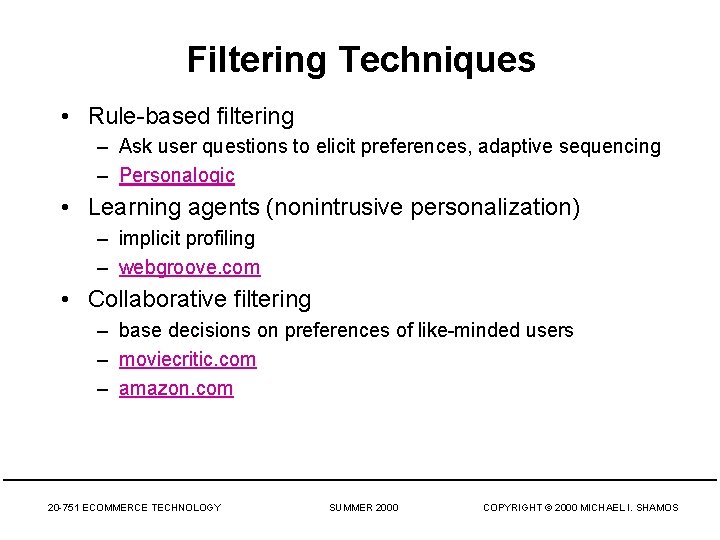
Filtering Techniques • Rule-based filtering – Ask user questions to elicit preferences, adaptive sequencing – Personalogic • Learning agents (nonintrusive personalization) – implicit profiling – webgroove. com • Collaborative filtering – base decisions on preferences of like-minded users – moviecritic. com – amazon. com 20 -751 ECOMMERCE TECHNOLOGY SUMMER 2000 COPYRIGHT © 2000 MICHAEL I. SHAMOS
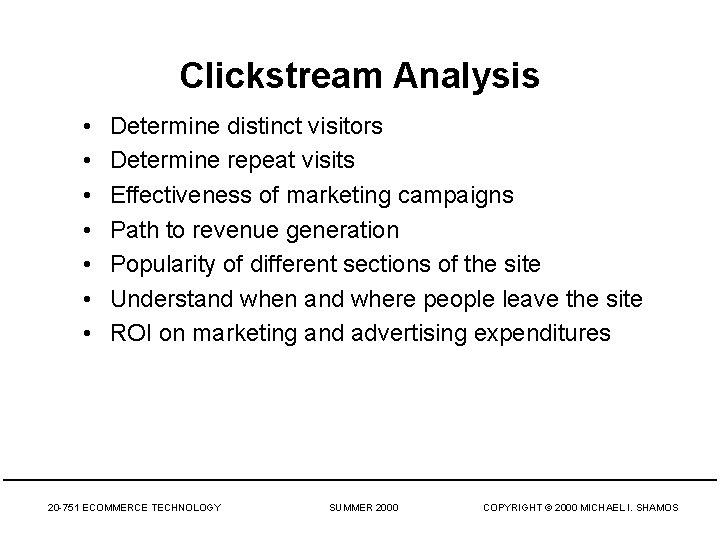
Clickstream Analysis • • Determine distinct visitors Determine repeat visits Effectiveness of marketing campaigns Path to revenue generation Popularity of different sections of the site Understand when and where people leave the site ROI on marketing and advertising expenditures 20 -751 ECOMMERCE TECHNOLOGY SUMMER 2000 COPYRIGHT © 2000 MICHAEL I. SHAMOS
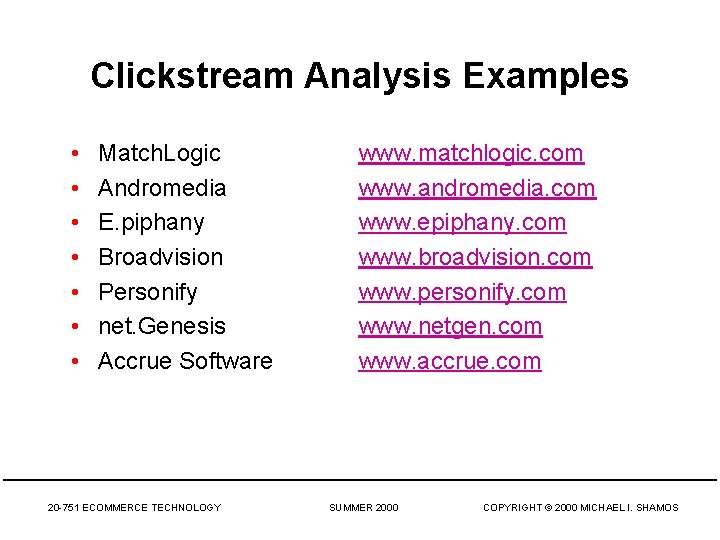
Clickstream Analysis Examples • • Match. Logic Andromedia E. piphany Broadvision Personify net. Genesis Accrue Software 20 -751 ECOMMERCE TECHNOLOGY www. matchlogic. com www. andromedia. com www. epiphany. com www. broadvision. com www. personify. com www. netgen. com www. accrue. com SUMMER 2000 COPYRIGHT © 2000 MICHAEL I. SHAMOS
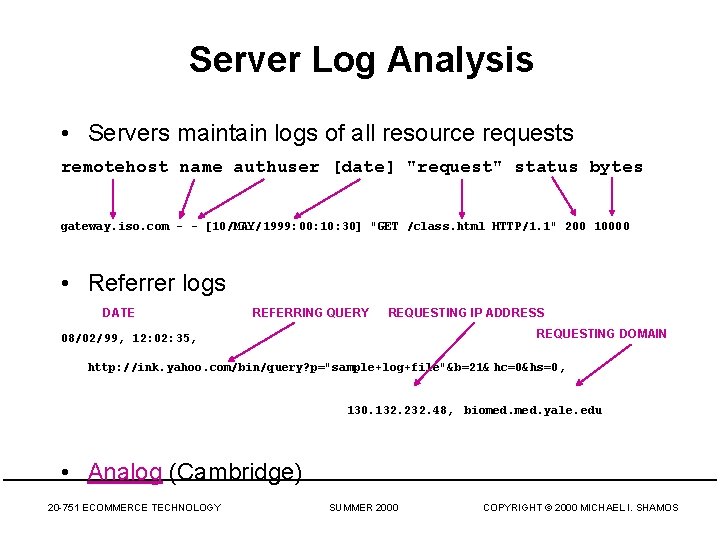
Server Log Analysis • Servers maintain logs of all resource requests remotehost name authuser [date] "request" status bytes gateway. iso. com - - [10/MAY/1999: 00: 10: 30] "GET /class. html HTTP/1. 1" 200 10000 • Referrer logs DATE REFERRING QUERY REQUESTING IP ADDRESS REQUESTING DOMAIN 08/02/99, 12: 02: 35, http: //ink. yahoo. com/bin/query? p="sample+log+file"&b=21& hc=0&hs=0, 130. 132. 232. 48, biomed. yale. edu • Analog (Cambridge) 20 -751 ECOMMERCE TECHNOLOGY SUMMER 2000 COPYRIGHT © 2000 MICHAEL I. SHAMOS
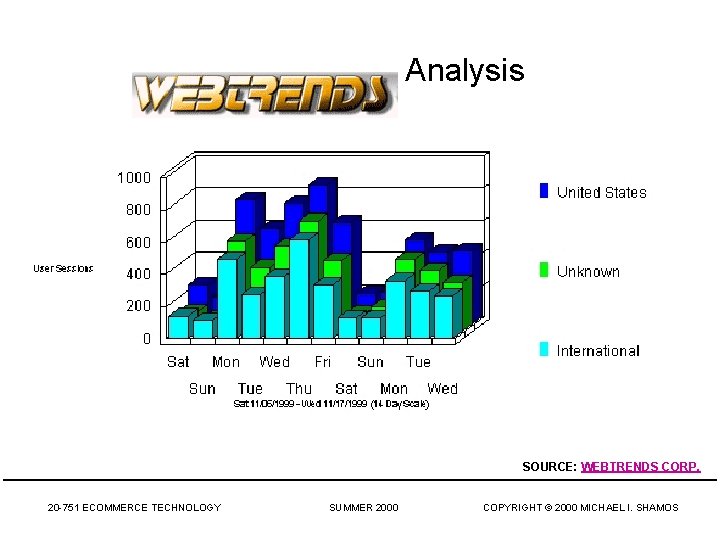
Analysis SOURCE: WEBTRENDS CORP. 20 -751 ECOMMERCE TECHNOLOGY SUMMER 2000 COPYRIGHT © 2000 MICHAEL I. SHAMOS
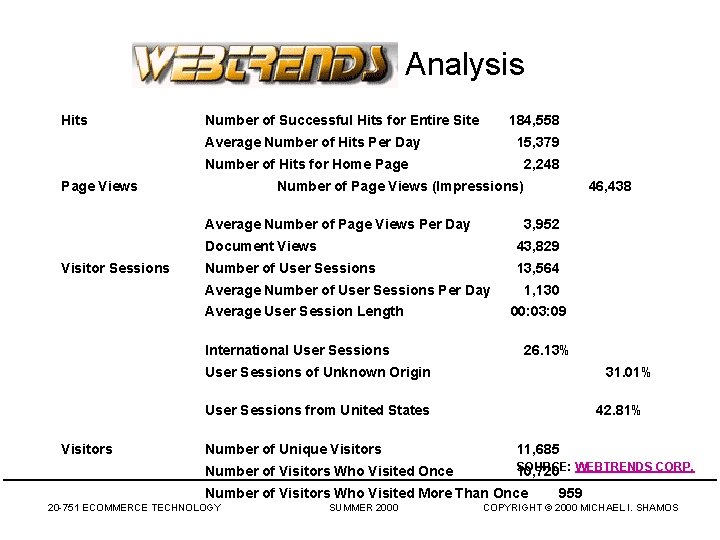
Analysis Hits Number of Successful Hits for Entire Site 184, 558 Average Number of Hits Per Day 15, 379 Number of Hits for Home Page Views 2, 248 Number of Page Views (Impressions) Average Number of Page Views Per Day Visitor Sessions 46, 438 3, 952 Document Views 43, 829 Number of User Sessions 13, 564 Average Number of User Sessions Per Day Average User Session Length International User Sessions 1, 130 00: 03: 09 26. 13% User Sessions of Unknown Origin 31. 01% User Sessions from United States Visitors 42. 81% Number of Unique Visitors 11, 685 Number of Visitors Who Visited Once SOURCE: WEBTRENDS CORP. 10, 720 Number of Visitors Who Visited More Than Once 20 -751 ECOMMERCE TECHNOLOGY SUMMER 2000 959 COPYRIGHT © 2000 MICHAEL I. SHAMOS
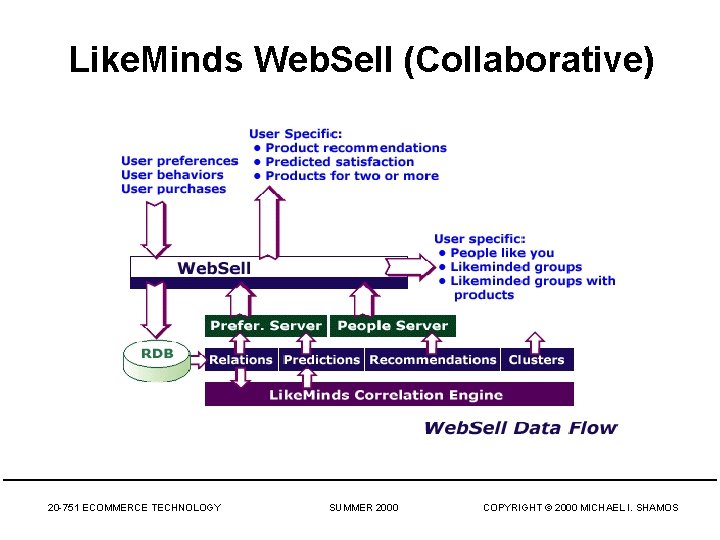
Like. Minds Web. Sell (Collaborative) 20 -751 ECOMMERCE TECHNOLOGY SUMMER 2000 COPYRIGHT © 2000 MICHAEL I. SHAMOS
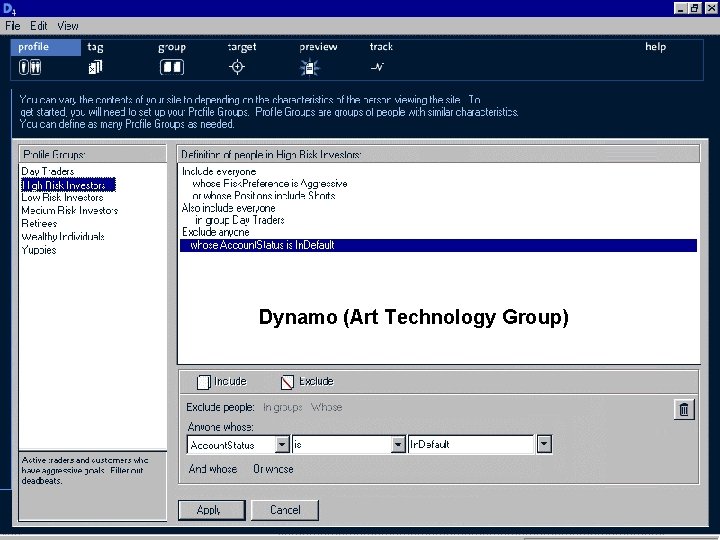
Dynamo (Art Technology Group)
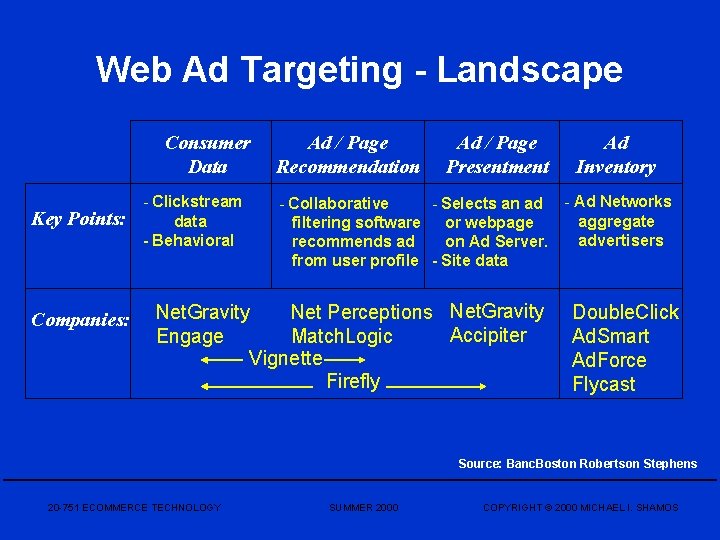
Web Ad Targeting - Landscape Consumer Data Key Points: Companies: - Clickstream data - Behavioral Ad / Page Recommendation Ad / Page Presentment - Collaborative - Selects an ad filtering software or webpage recommends ad on Ad Server. from user profile - Site data Net Perceptions Net. Gravity Accipiter Match. Logic Engage Vignette Firefly Ad Inventory - Ad Networks aggregate advertisers Double. Click Ad. Smart Ad. Force Flycast Source: Banc. Boston Robertson Stephens 20 -751 ECOMMERCE TECHNOLOGY SUMMER 2000 COPYRIGHT © 2000 MICHAEL I. SHAMOS
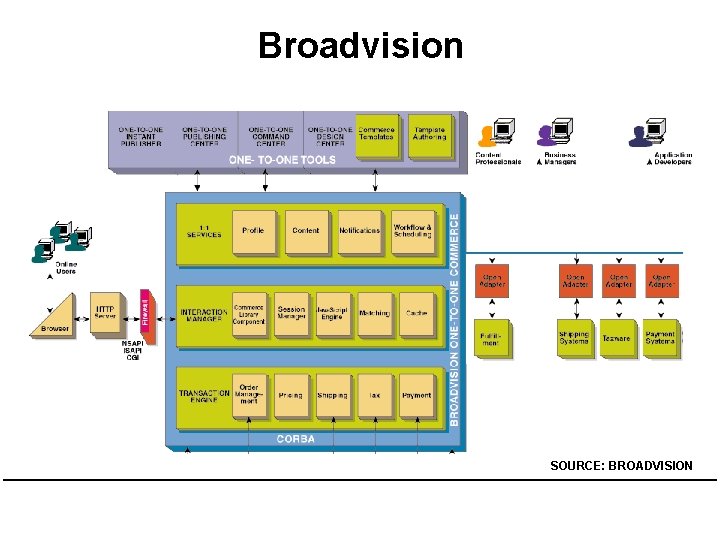
Broadvision SOURCE: BROADVISION
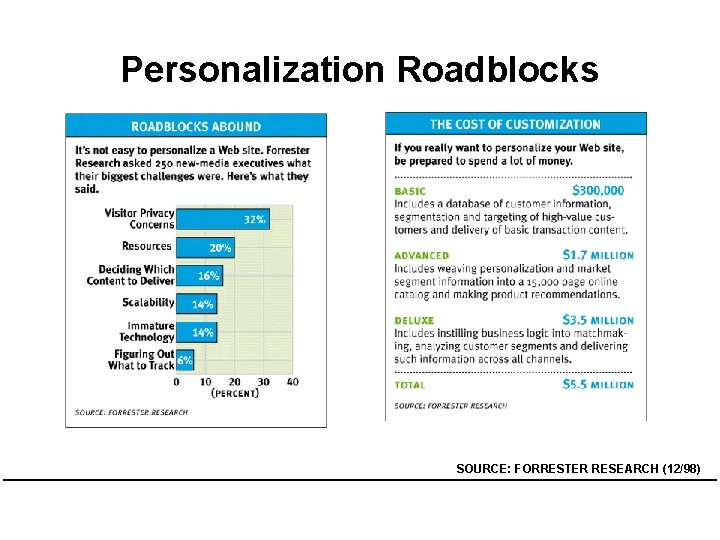
Personalization Roadblocks SOURCE: FORRESTER RESEARCH (12/98)
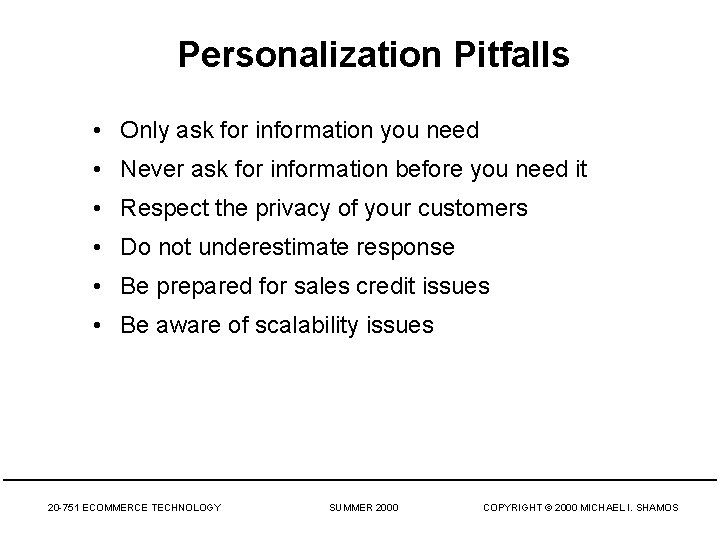
Personalization Pitfalls • Only ask for information you need • Never ask for information before you need it • Respect the privacy of your customers • Do not underestimate response • Be prepared for sales credit issues • Be aware of scalability issues 20 -751 ECOMMERCE TECHNOLOGY SUMMER 2000 COPYRIGHT © 2000 MICHAEL I. SHAMOS
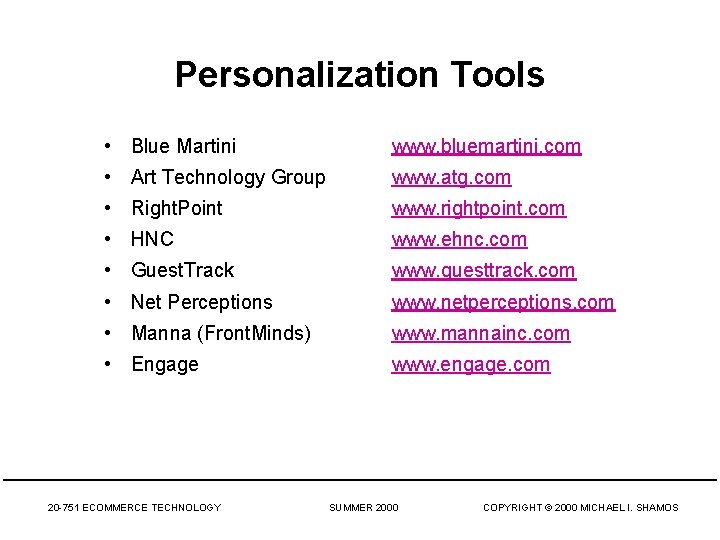
Personalization Tools • Blue Martini www. bluemartini. com • Art Technology Group www. atg. com • Right. Point www. rightpoint. com • HNC www. ehnc. com • Guest. Track www. guesttrack. com • Net Perceptions www. netperceptions. com • Manna (Front. Minds) www. mannainc. com • Engage www. engage. com 20 -751 ECOMMERCE TECHNOLOGY SUMMER 2000 COPYRIGHT © 2000 MICHAEL I. SHAMOS
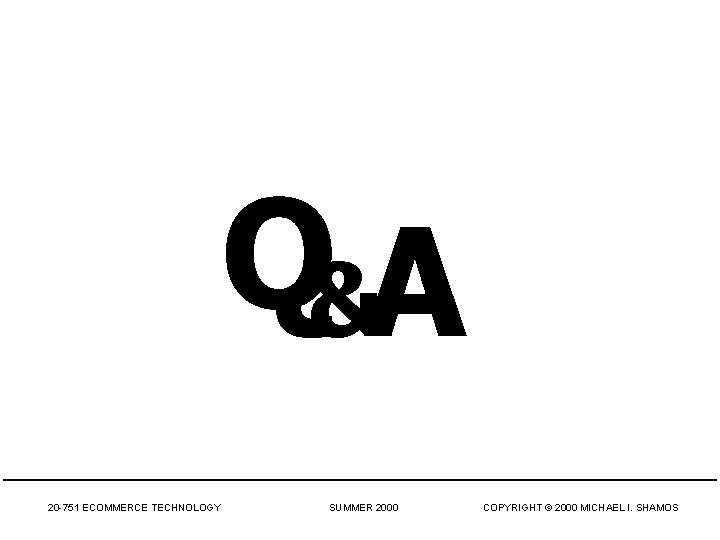
Q&A 20 -751 ECOMMERCE TECHNOLOGY SUMMER 2000 COPYRIGHT © 2000 MICHAEL I. SHAMOS
Ece 751
Cmu 15751
751 talas savaşı
تراکتور 751
Location-based commerce (l-commerce)
01:640:244 lecture notes - lecture 15: plat, idah, farad
Mass spectrometry lecture
E commerce 2017 business technology society
E commerce business technology society
E commerce architecture and technologies in web technology
E-commerce 2019: business, technology and society
Stoichiometry ratio
Atomic mass and mass number
Relative formula mass of hcl
Calculating percentage composition by mass
Inertial mass vs gravitational mass
Grams to moles conversion
Unit of molar mass
Molar mass unit
Moles to particles
Read pp
Mass/mass problems
Atomic
Gravitational mass vs inertial mass
Formula mass
Molecular mass
Formula mass vs molecular mass
Mass number formula
Concentration of solution