2010 Cengage Learning Characteristics of Effective Selection Techniques
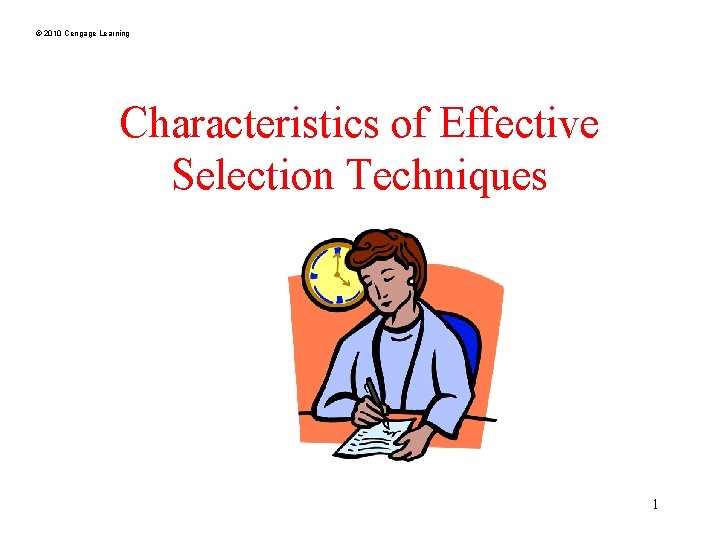
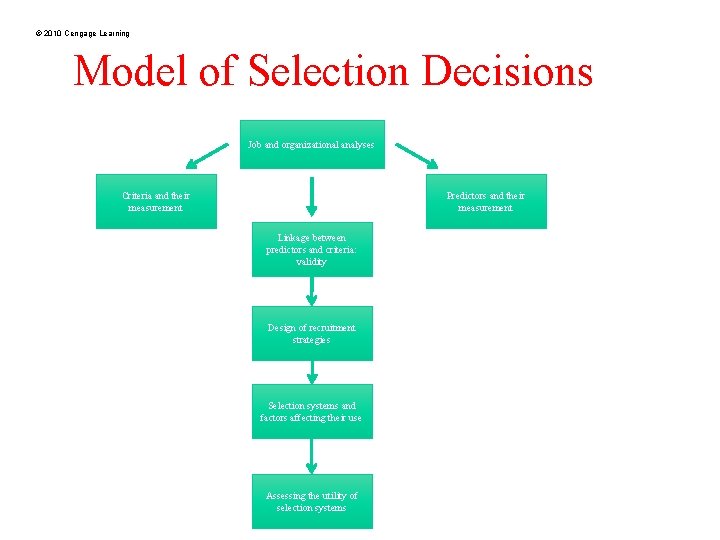
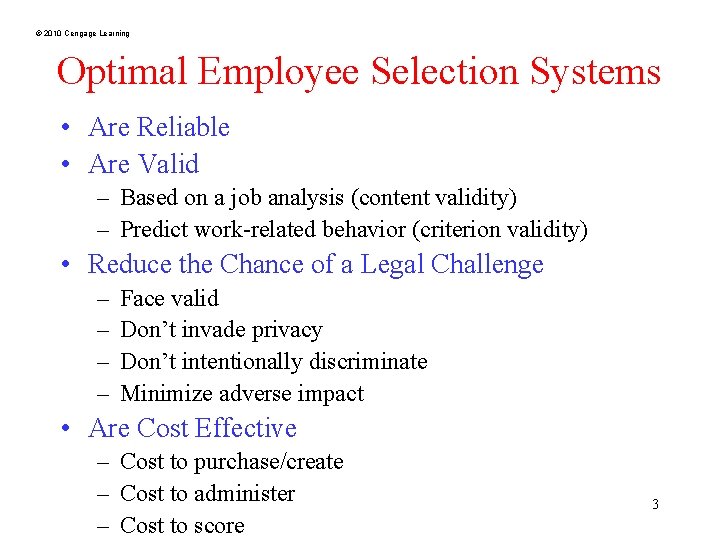
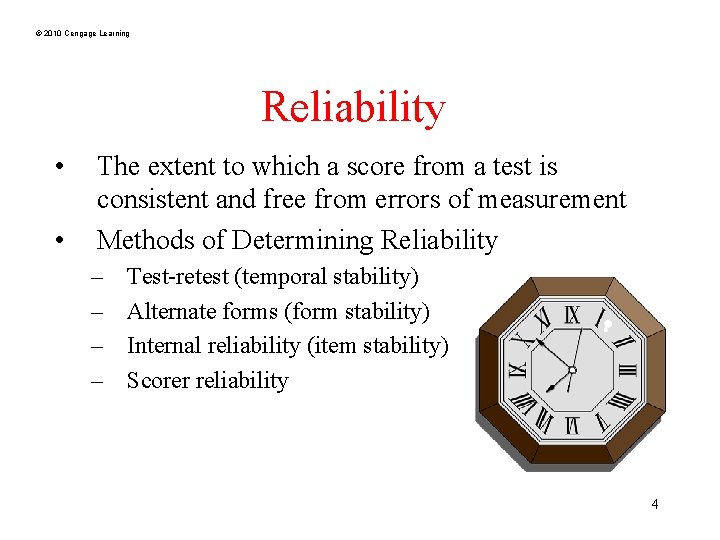
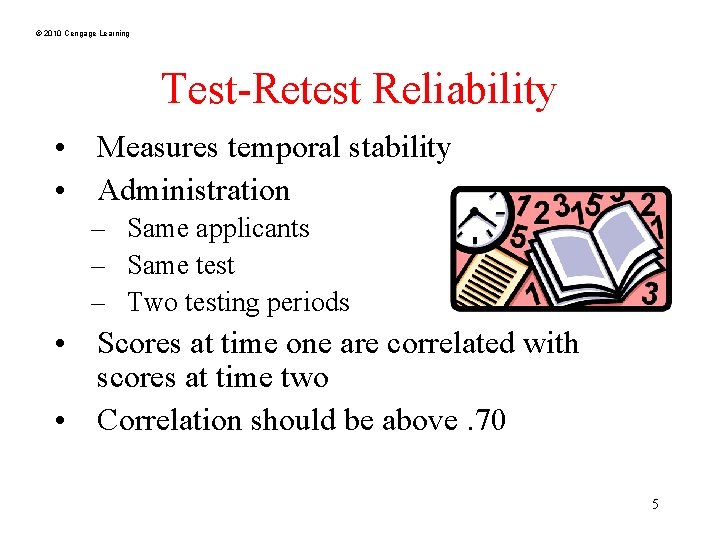
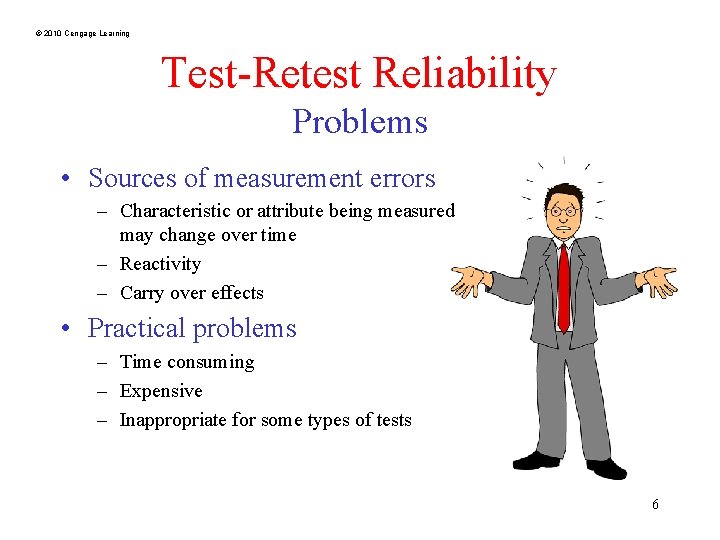
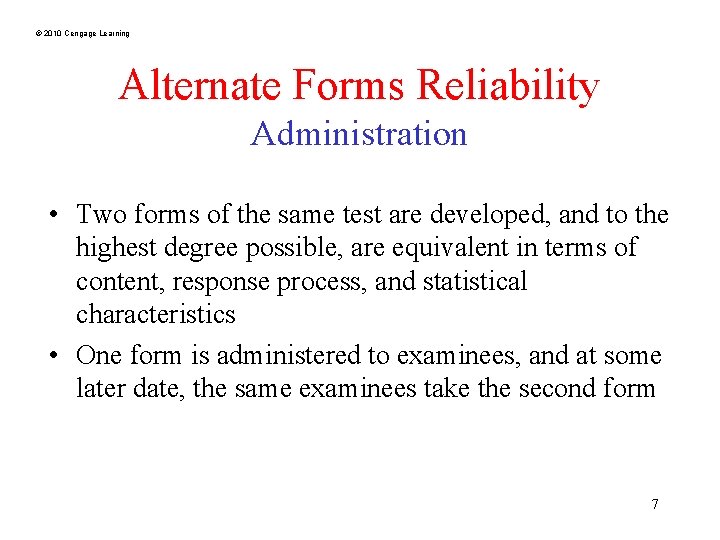
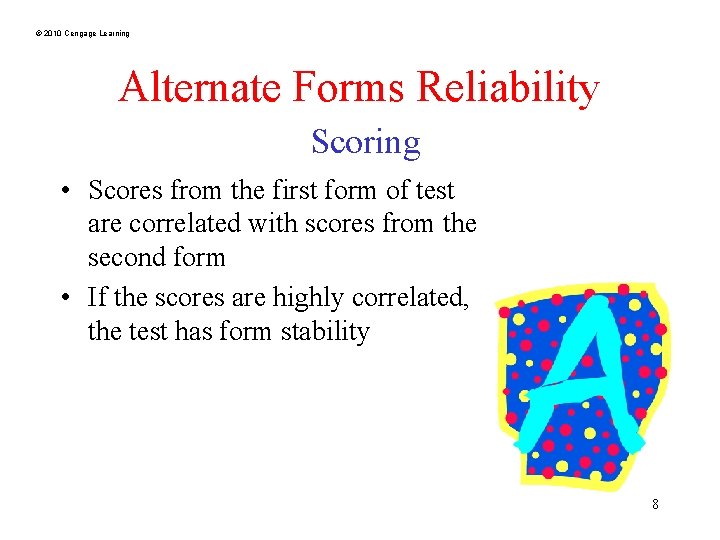
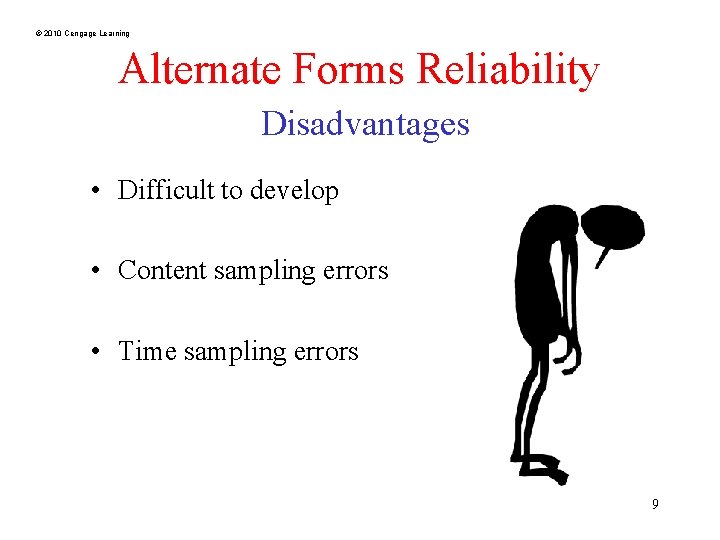
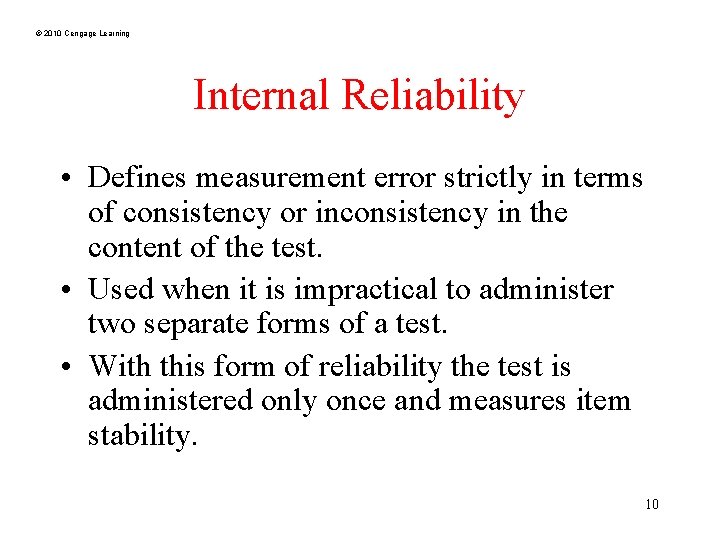
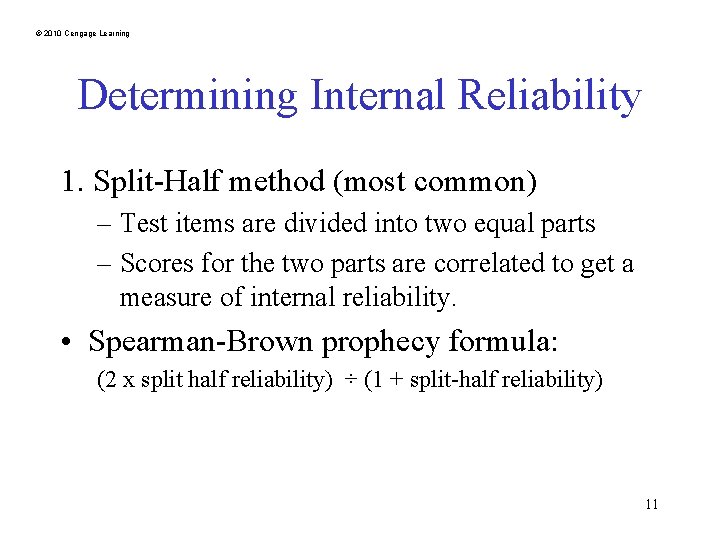
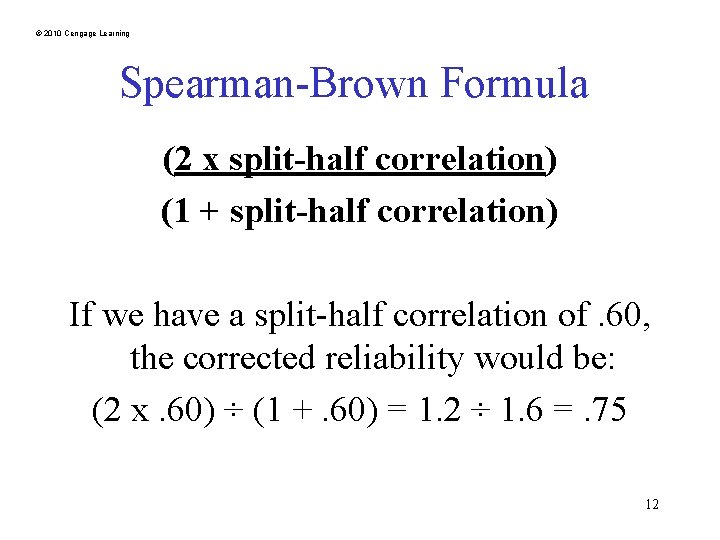
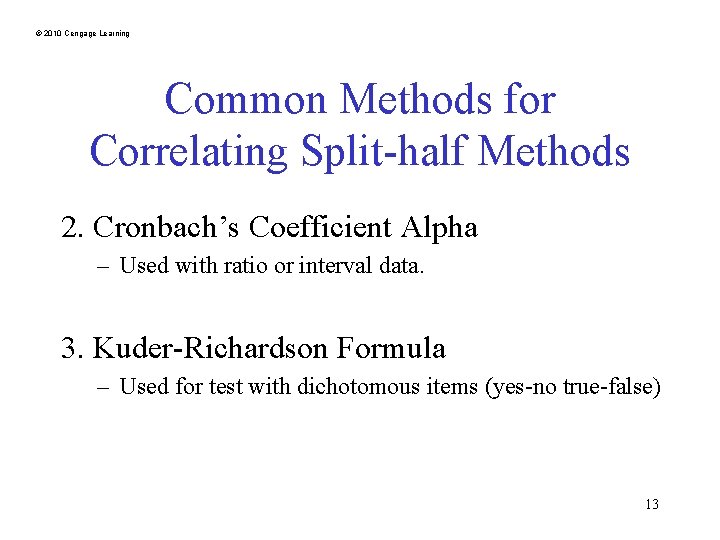
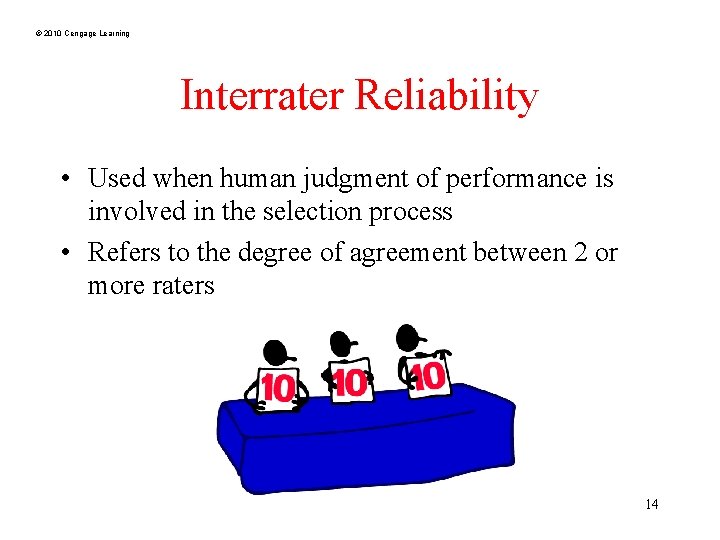
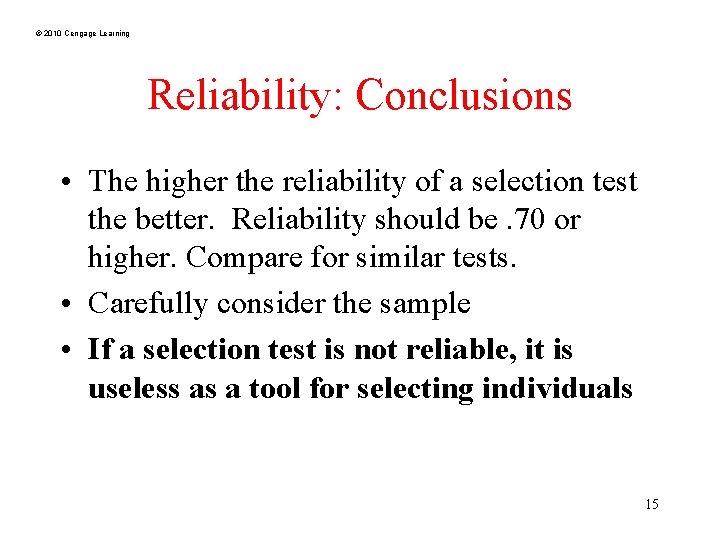
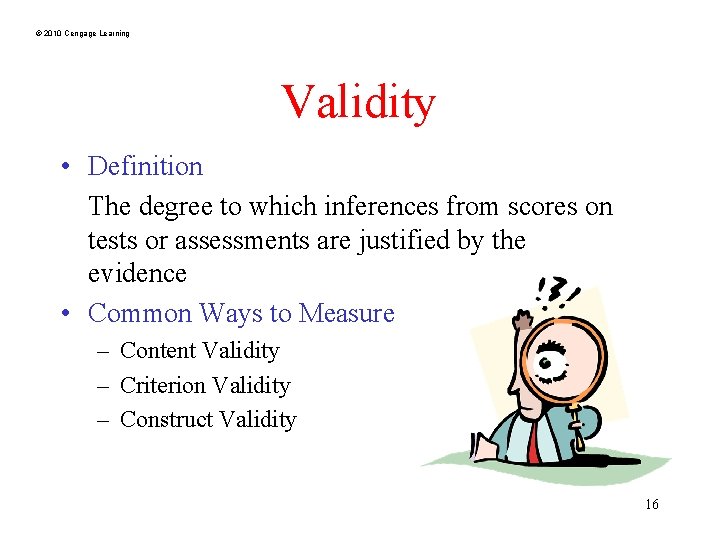
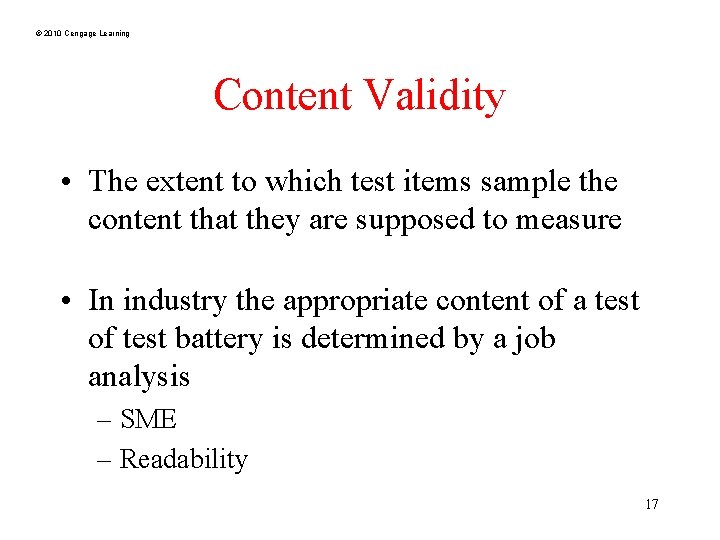
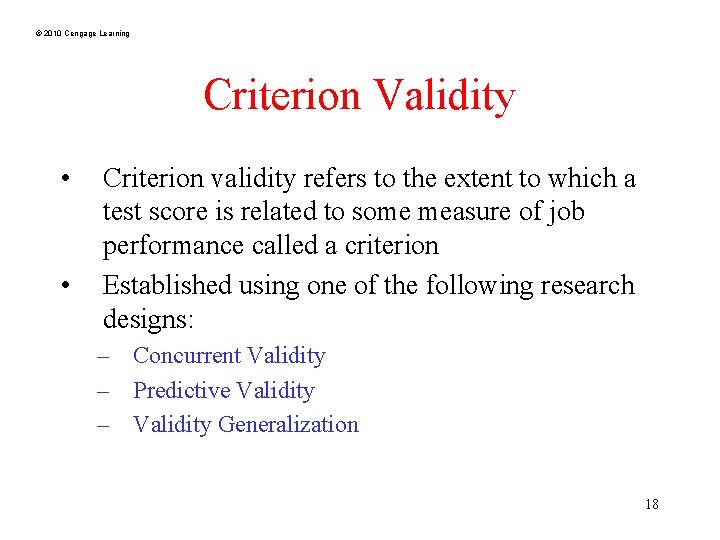
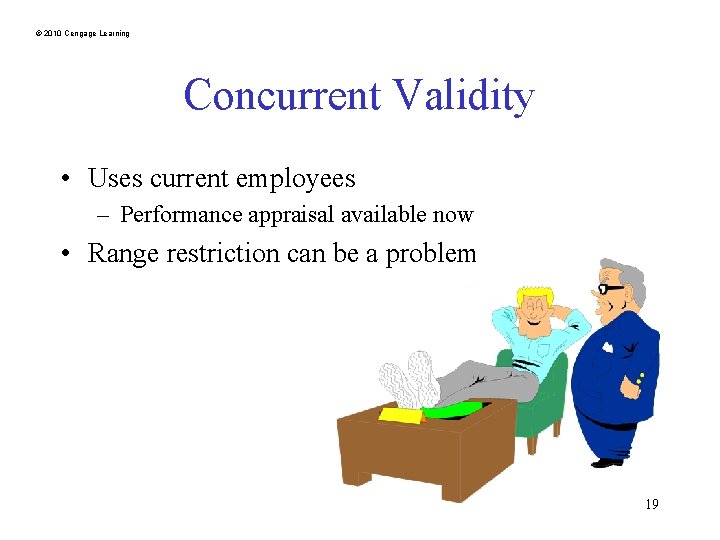
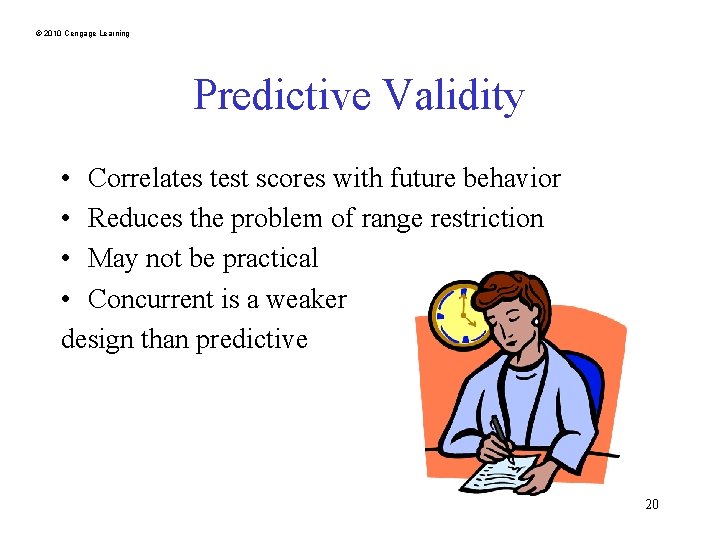
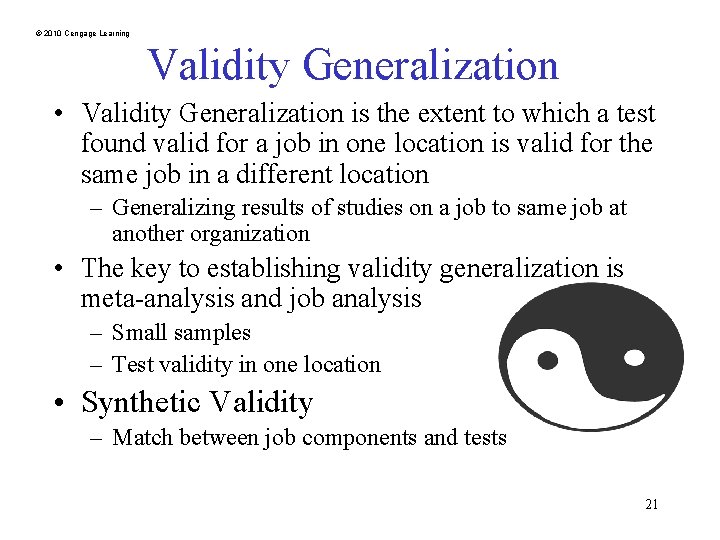
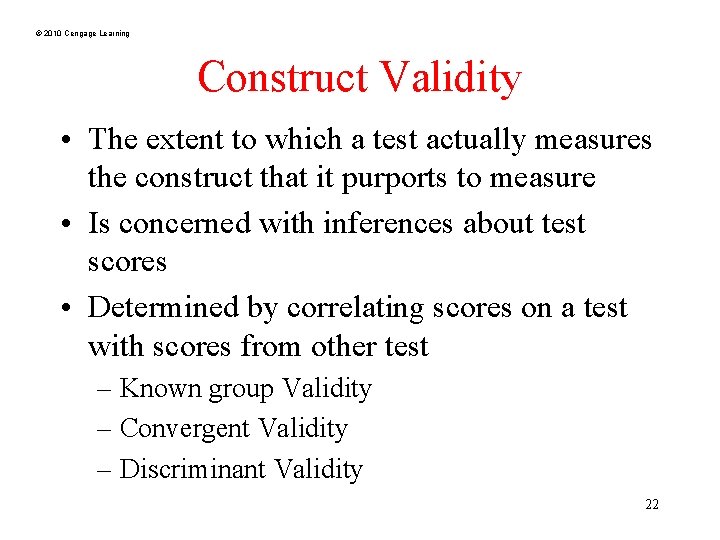
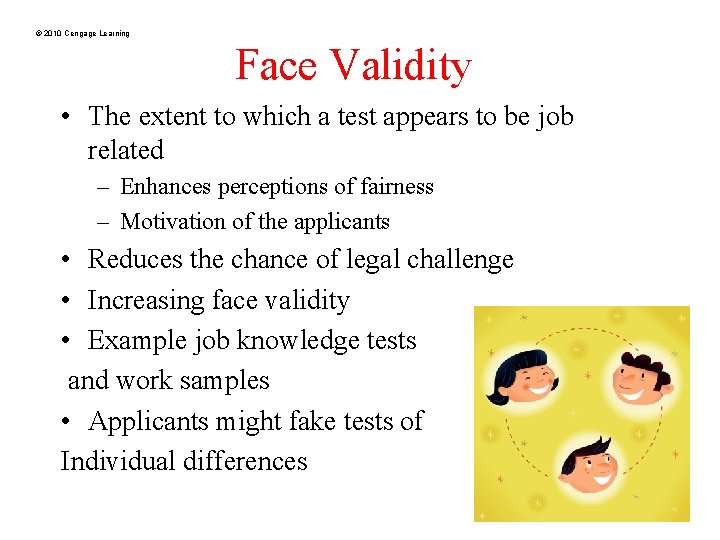
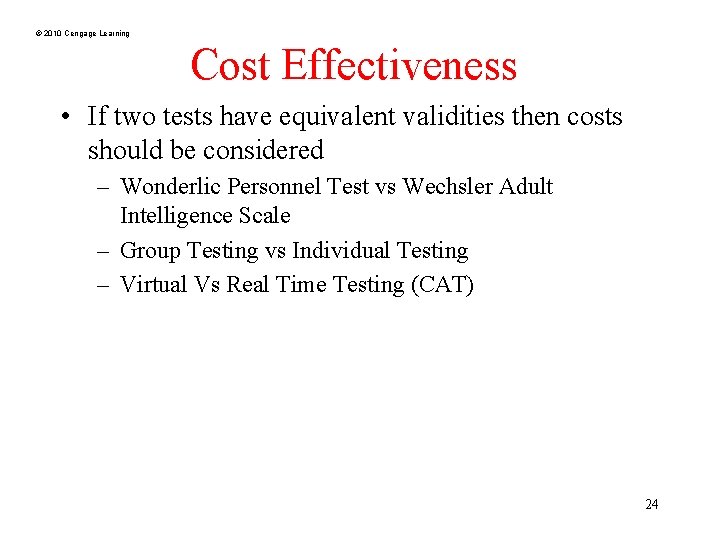
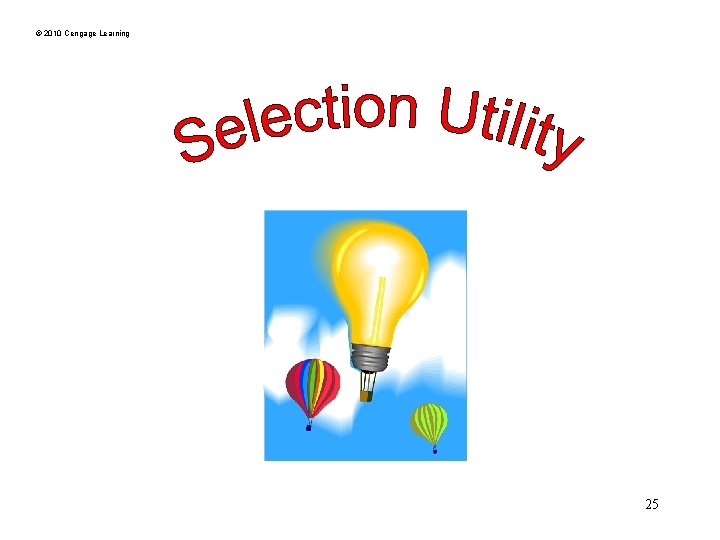
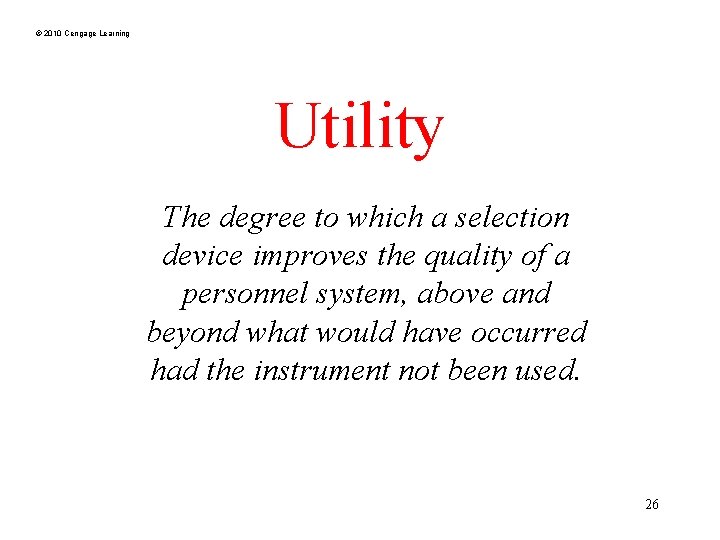
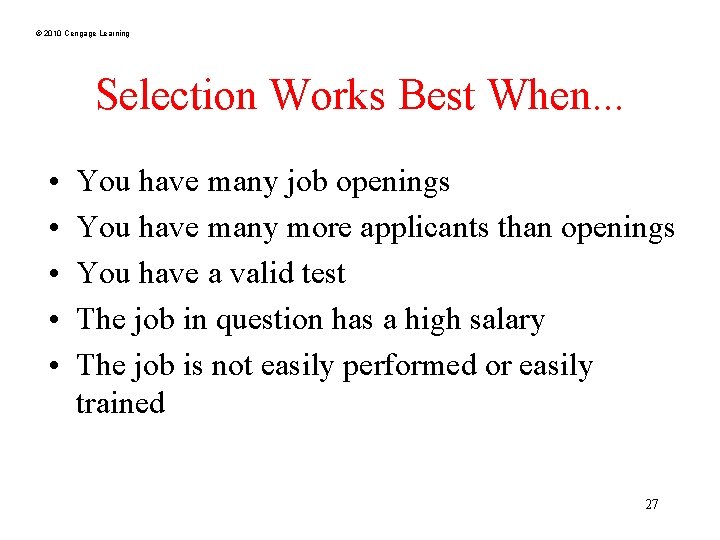
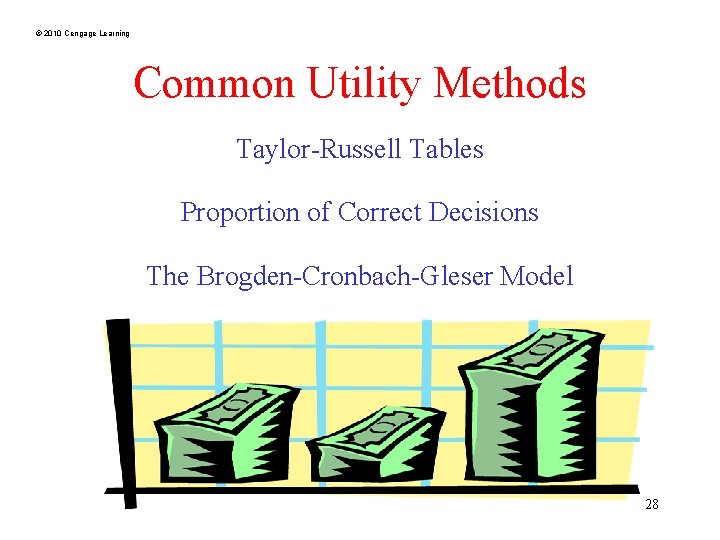
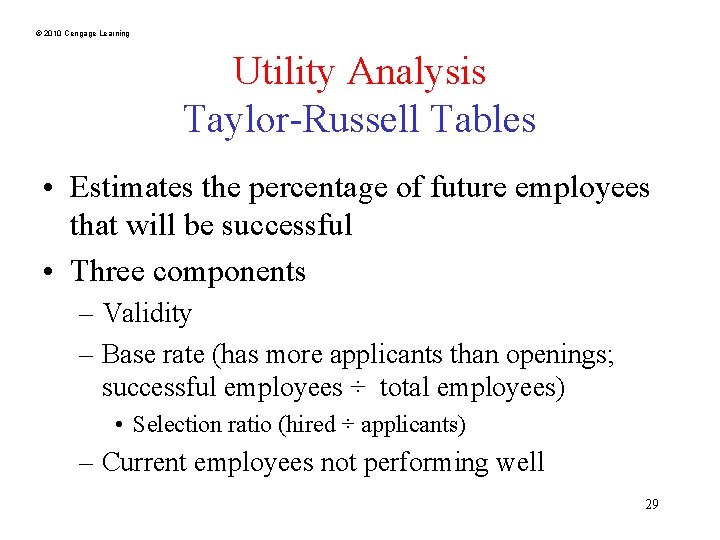
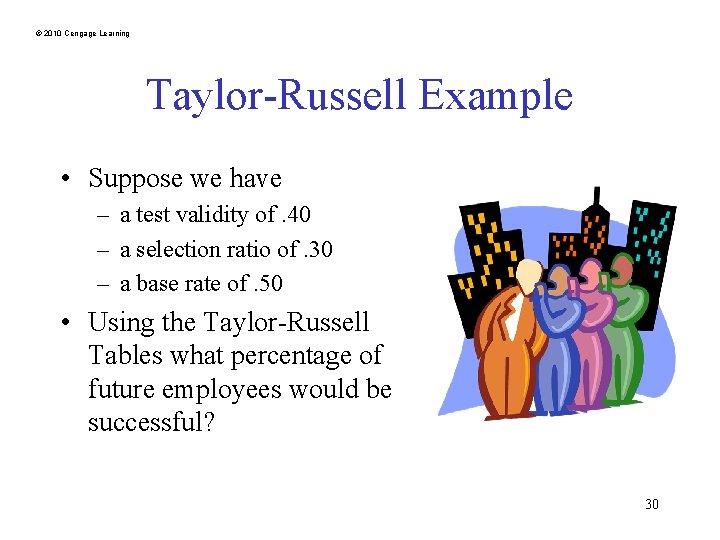
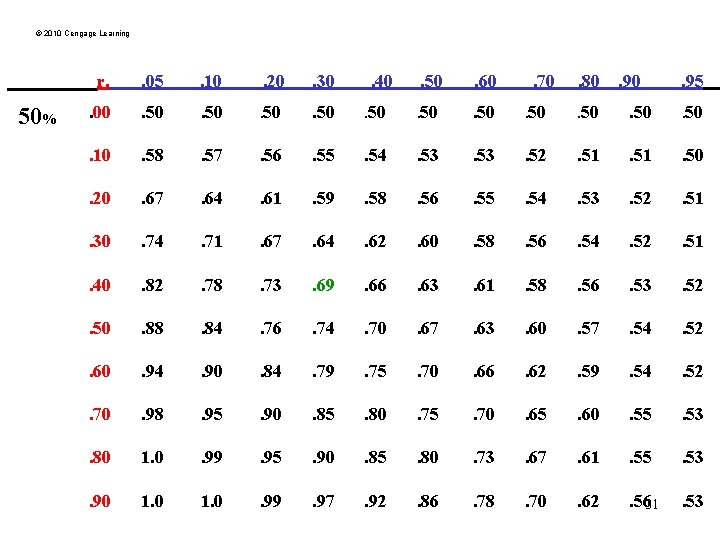
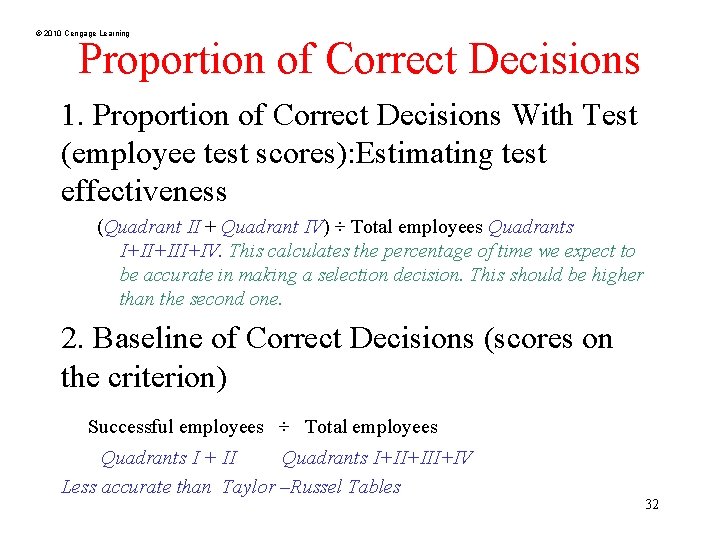
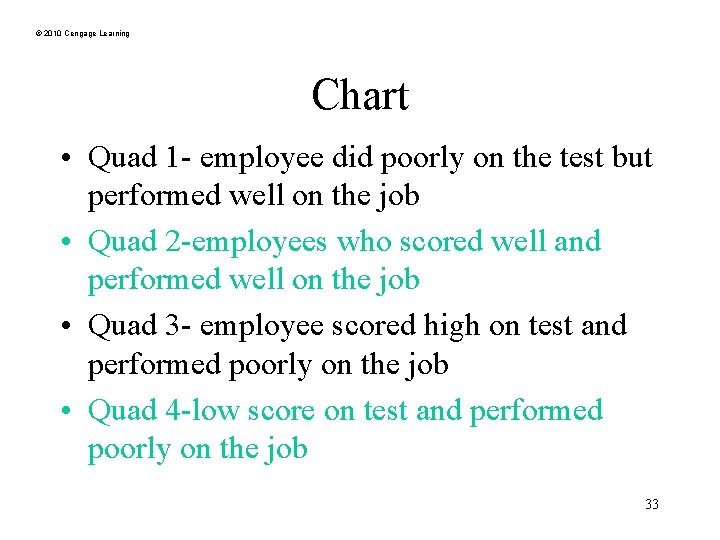
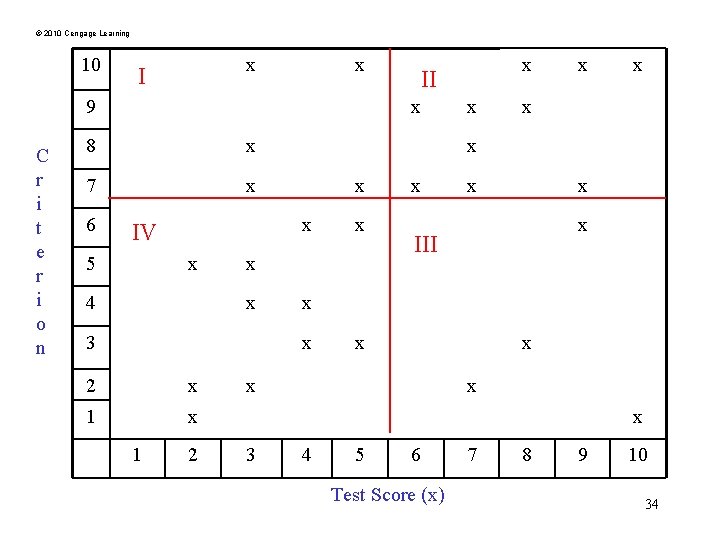
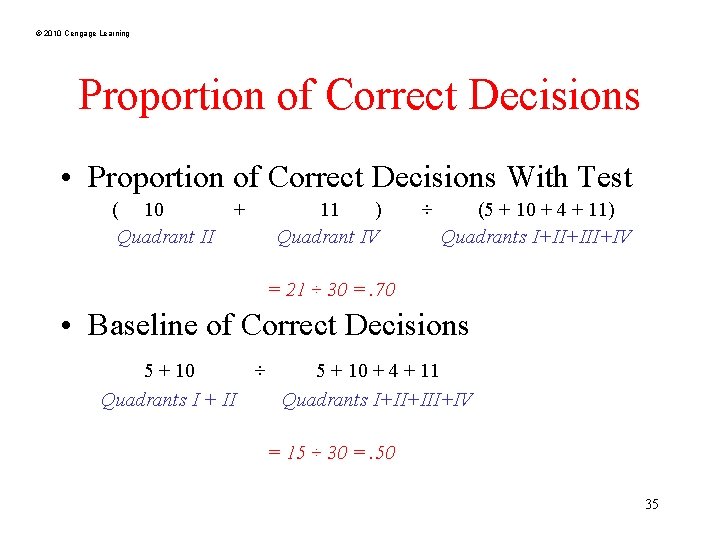
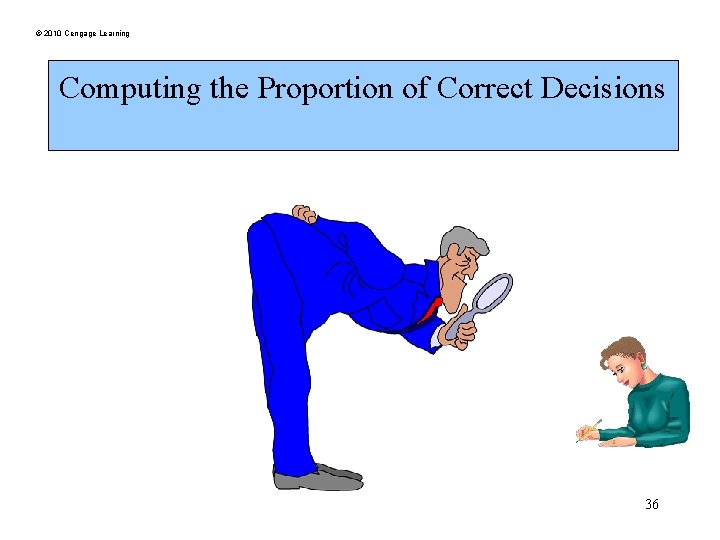
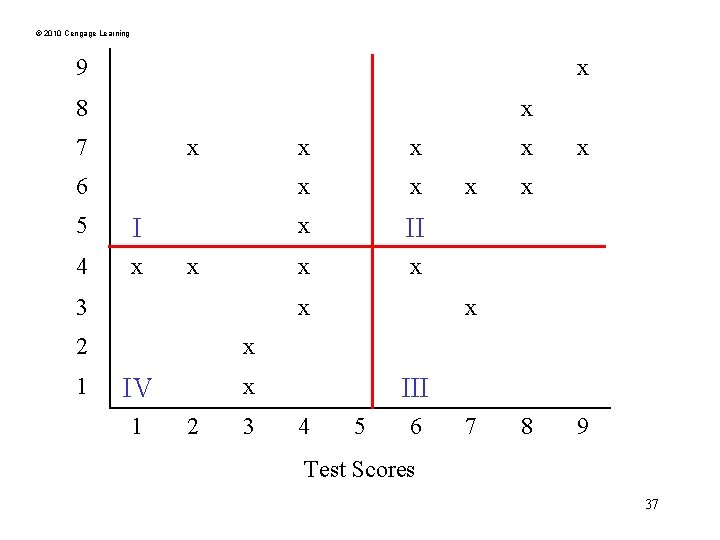
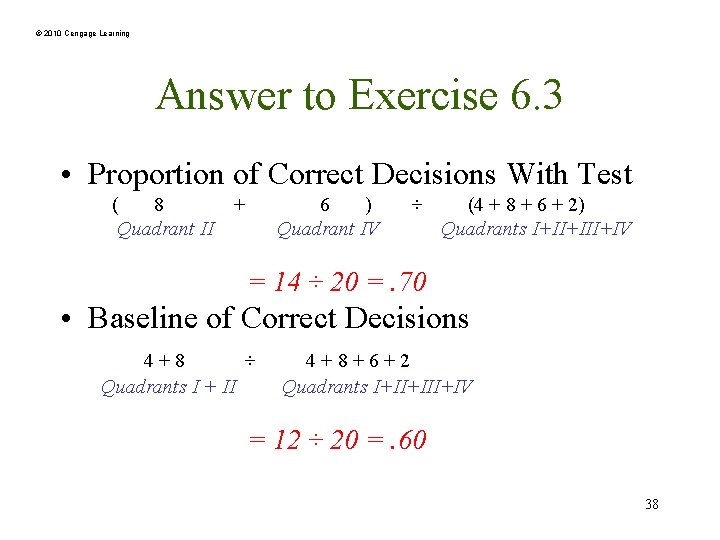
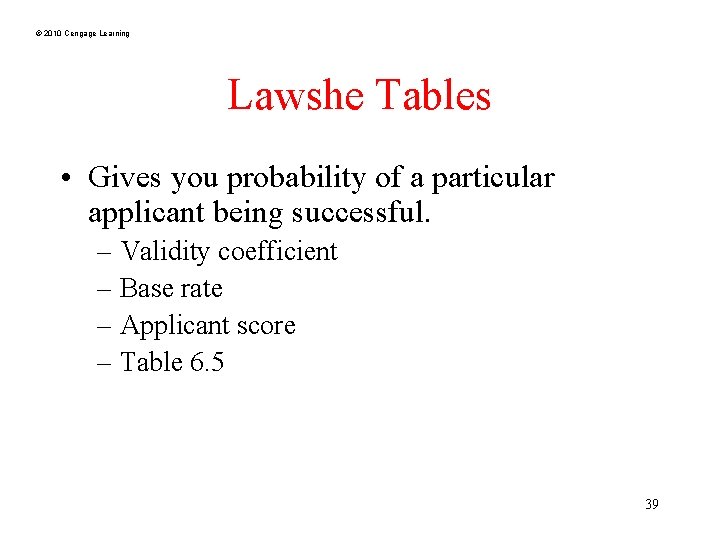
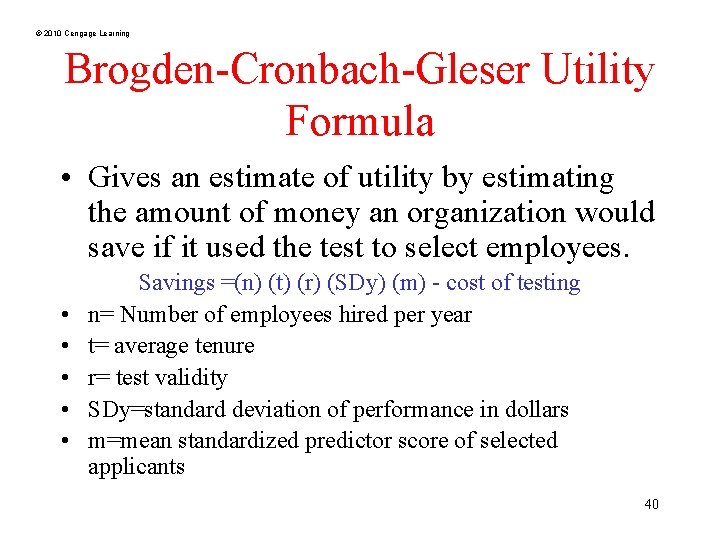
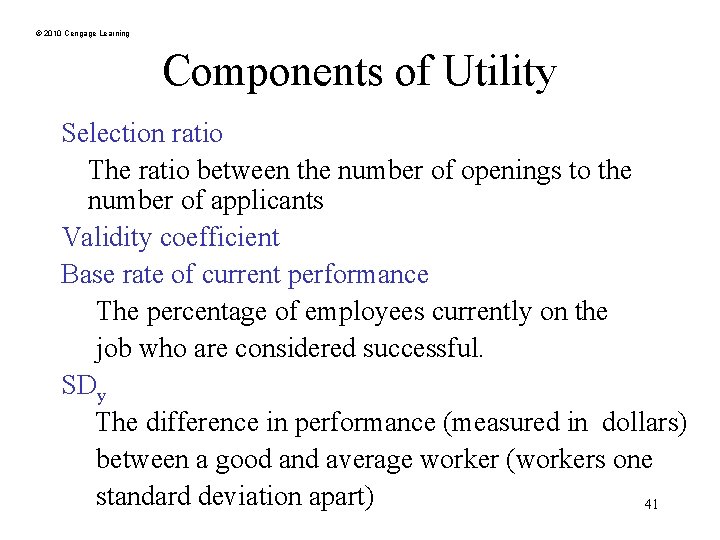
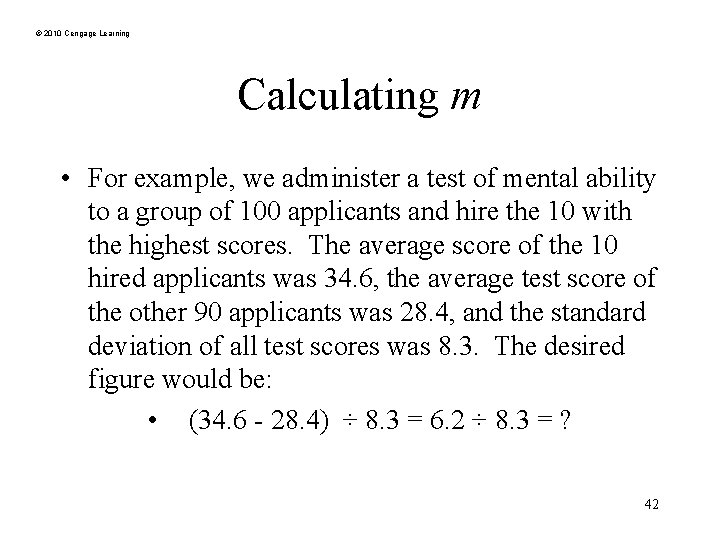
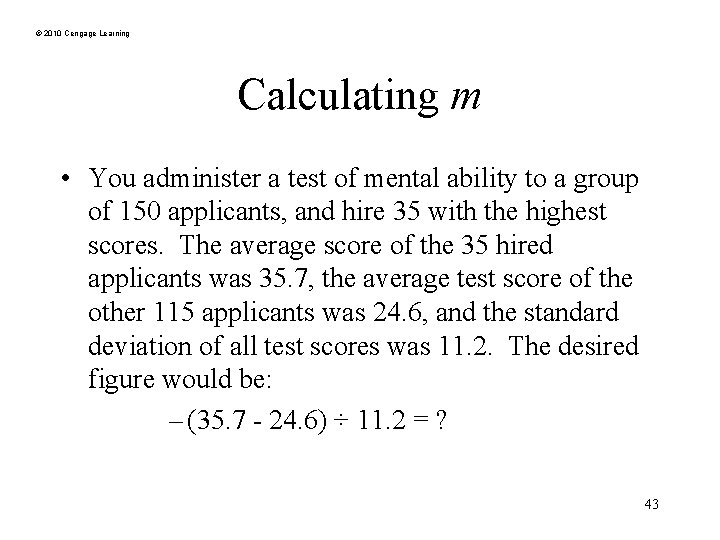
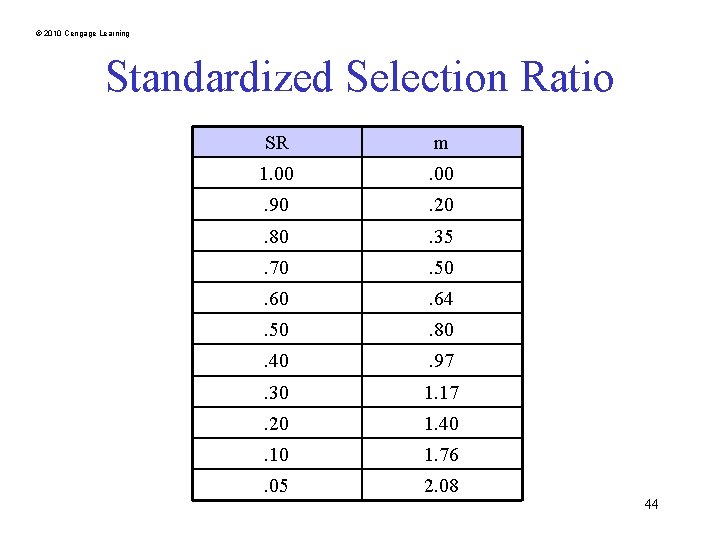
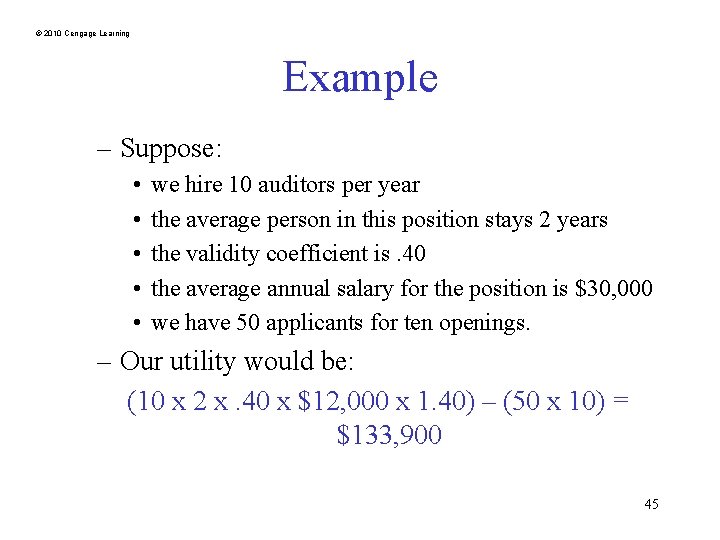
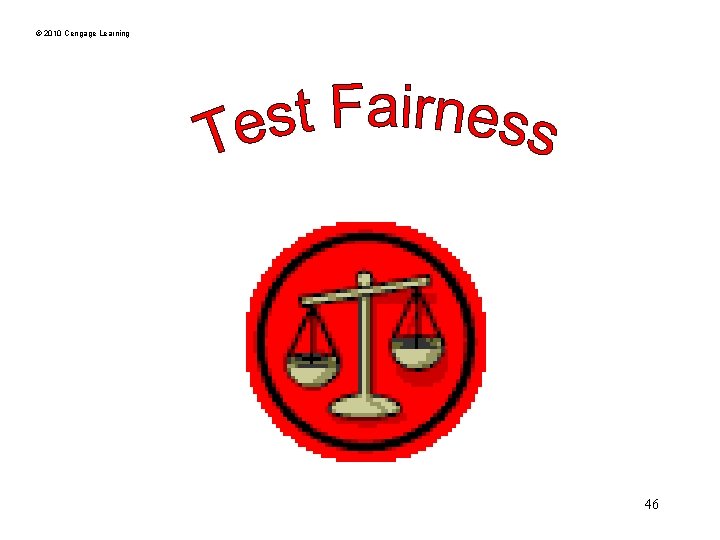
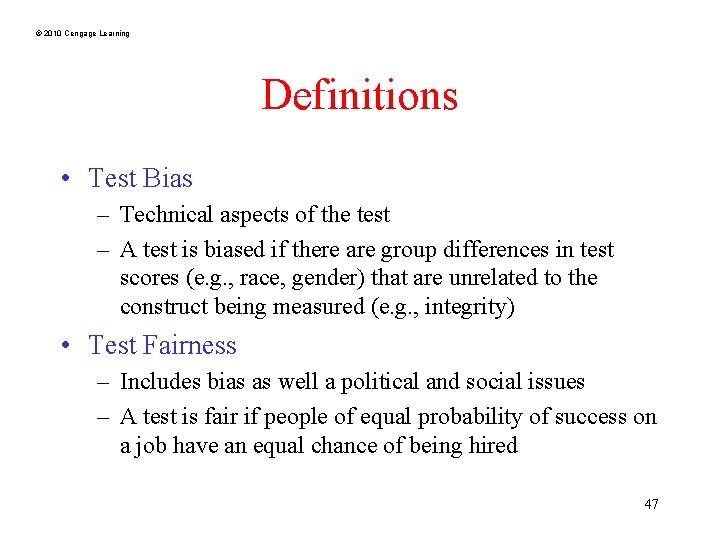
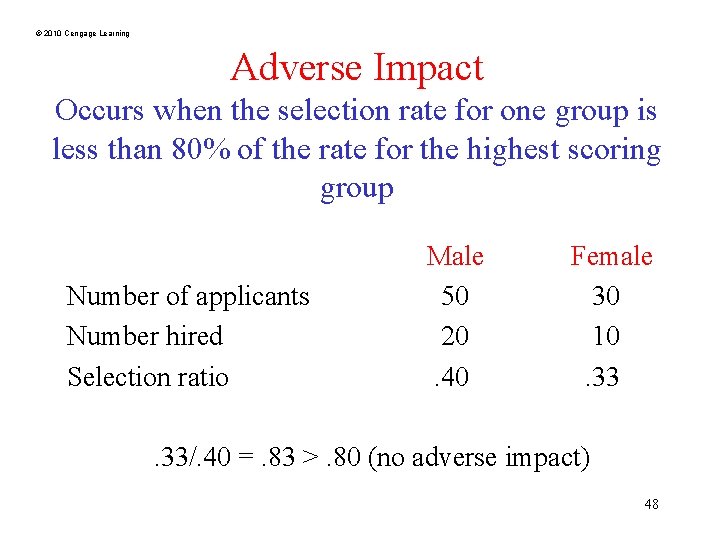
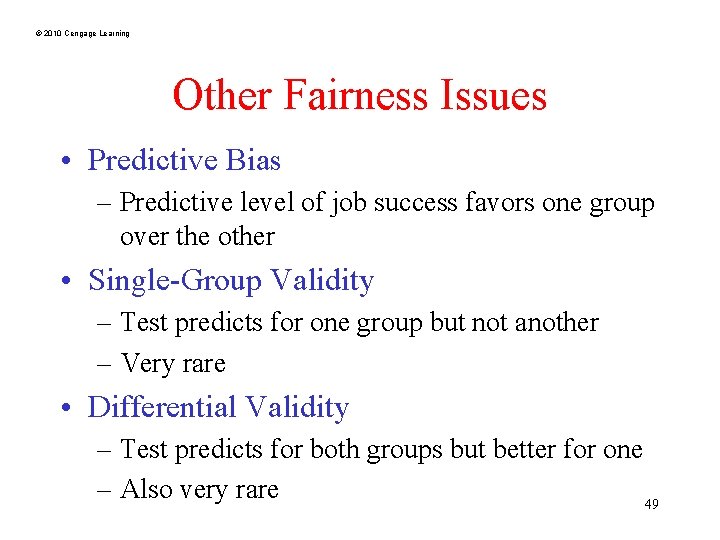
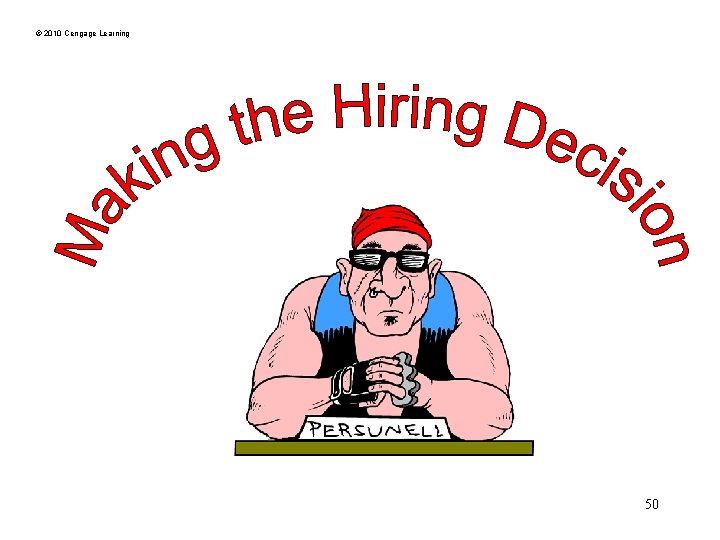
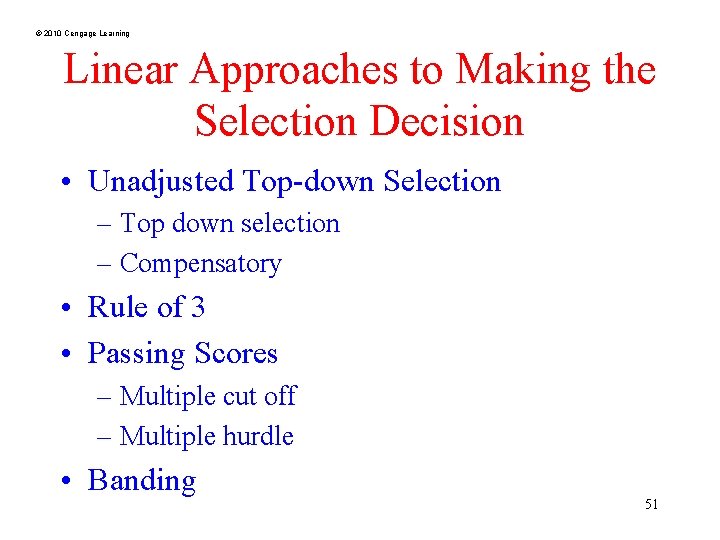
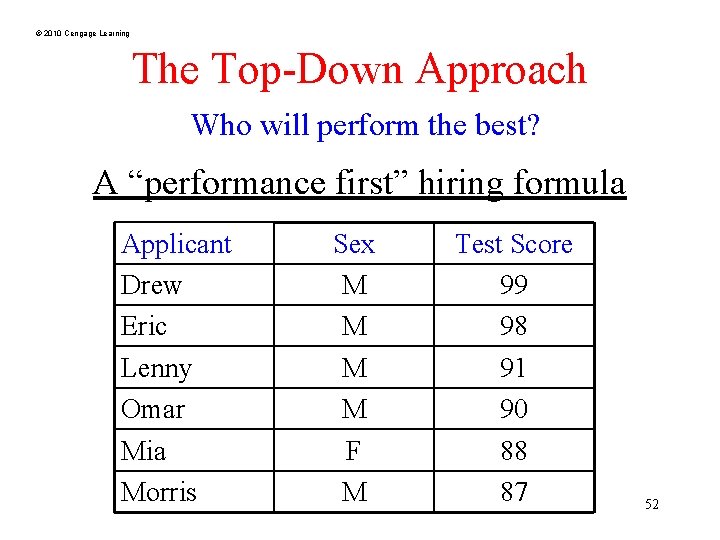
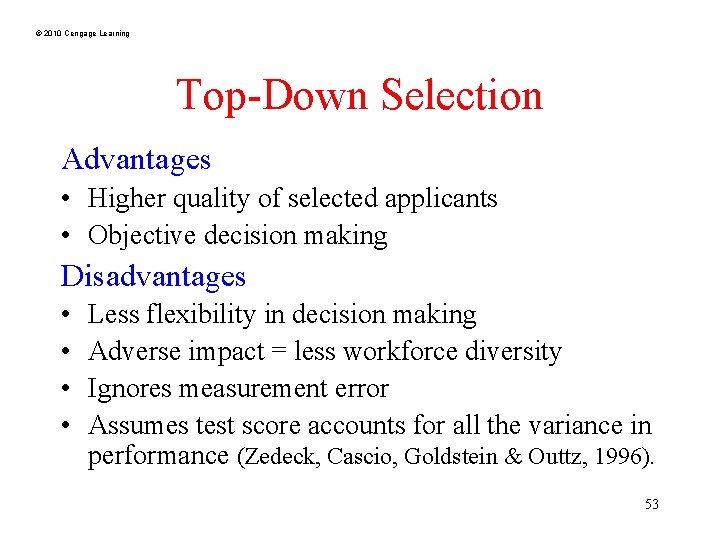
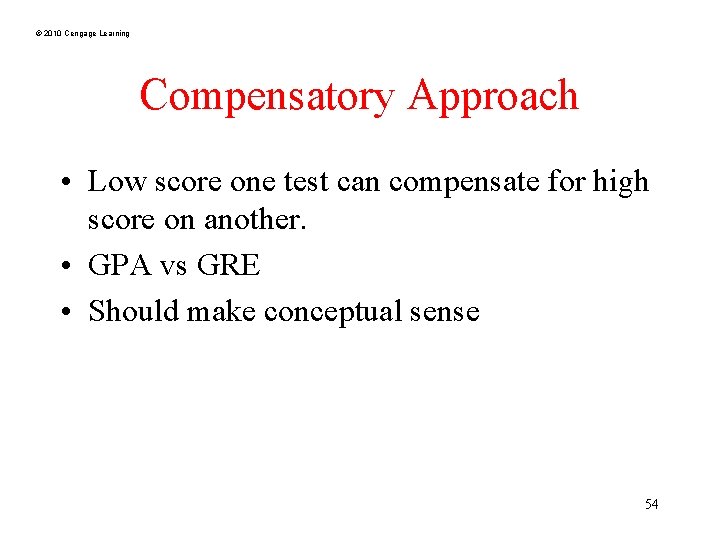
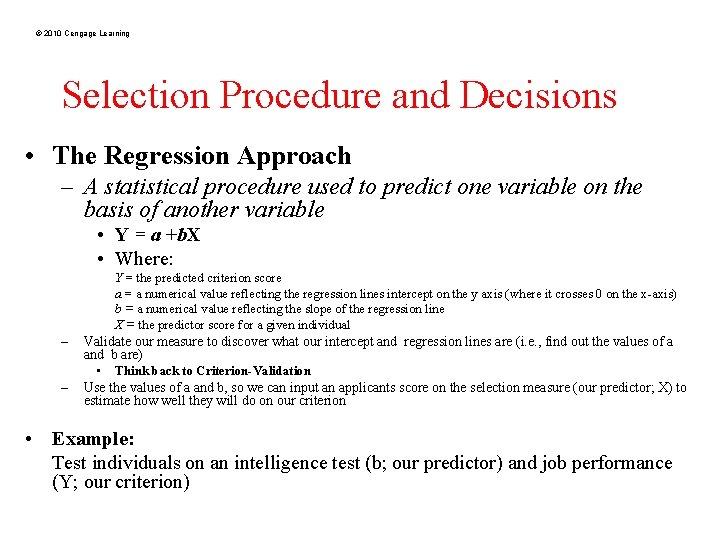
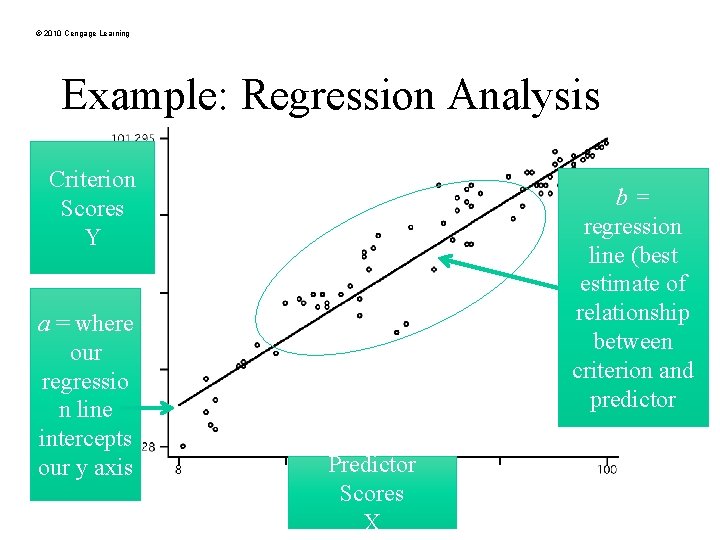
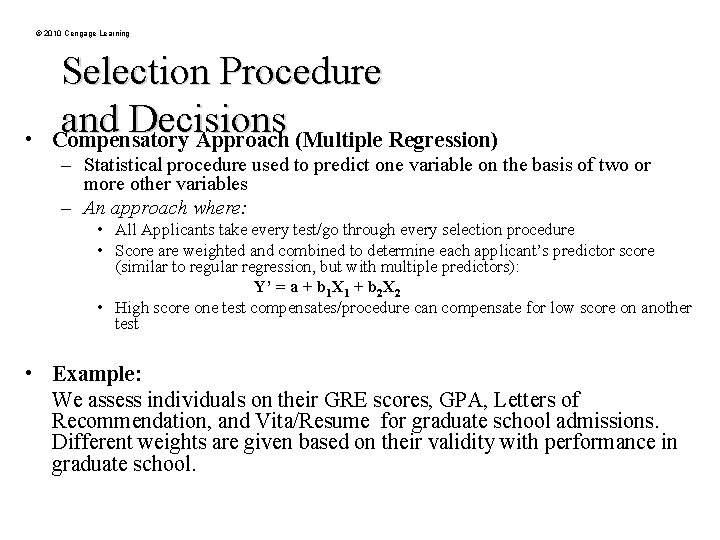
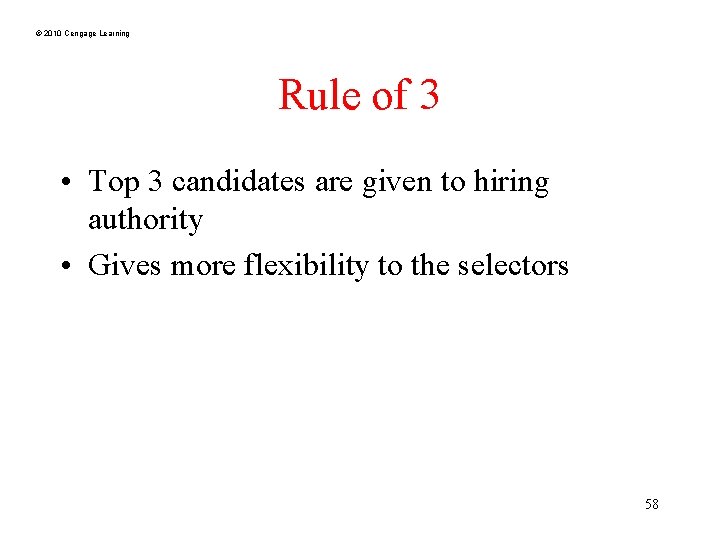
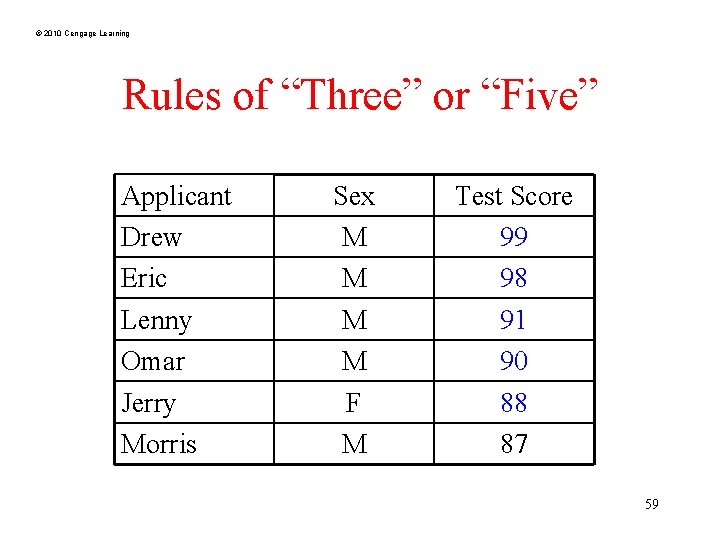
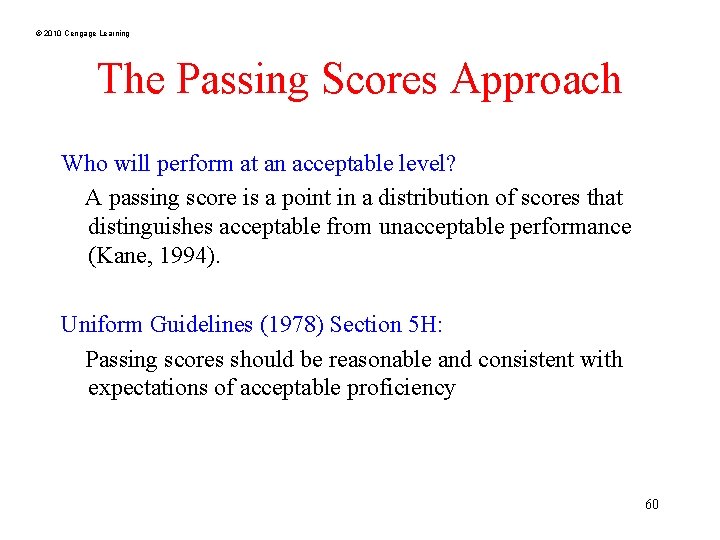
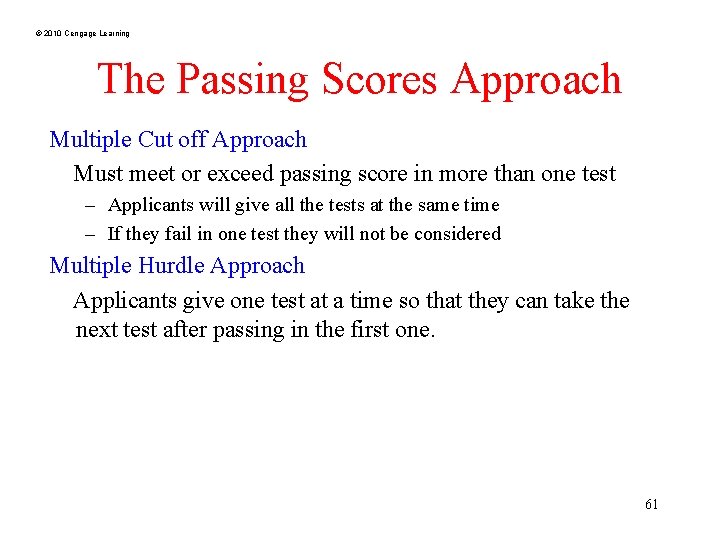
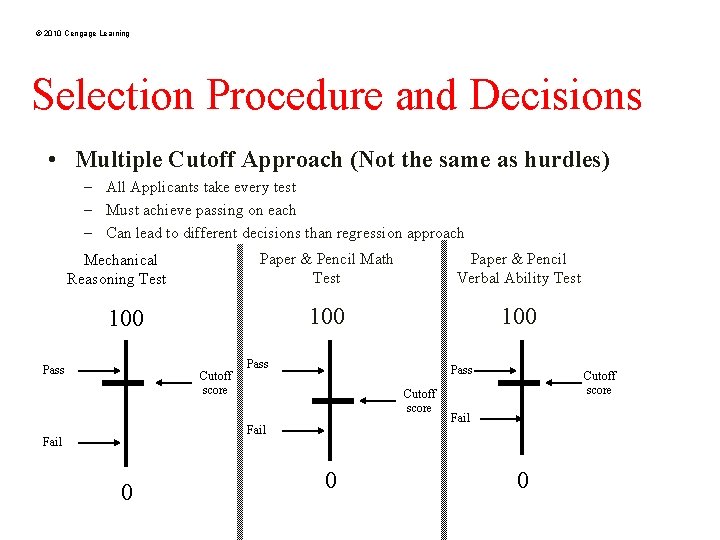
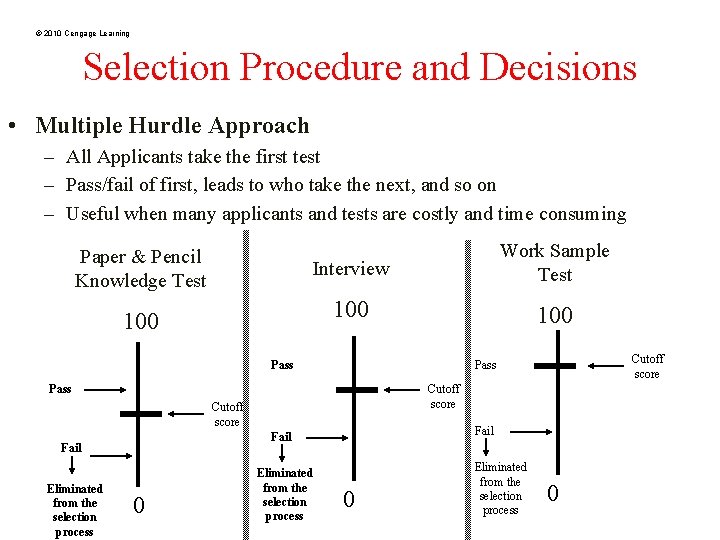
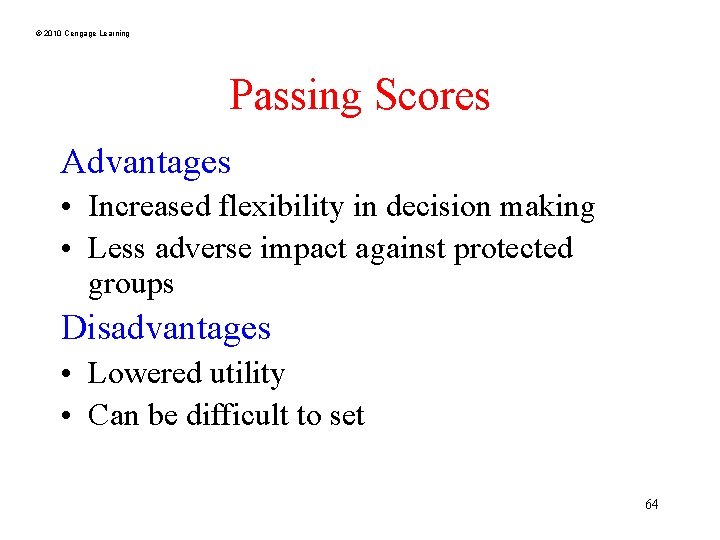
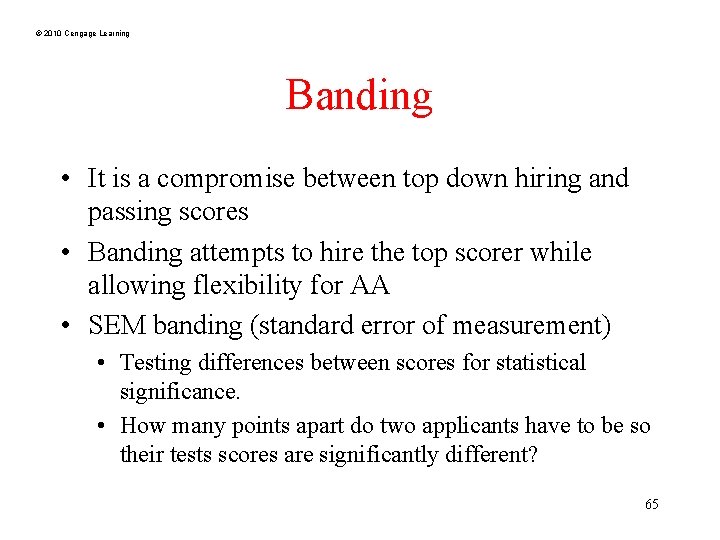
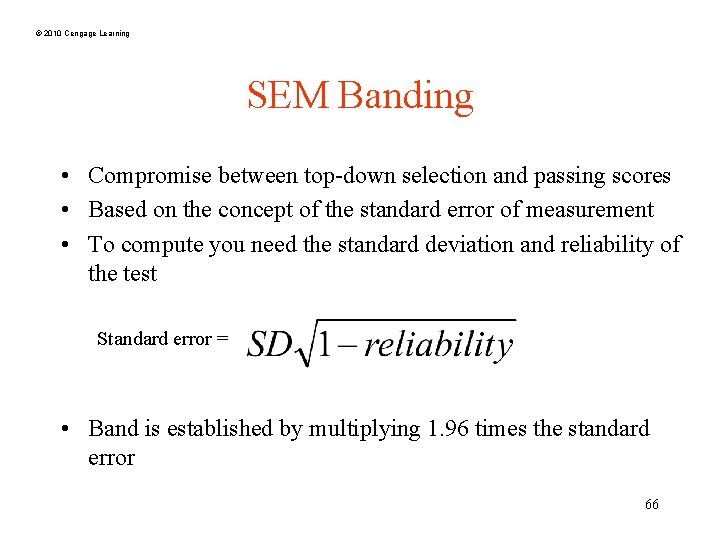
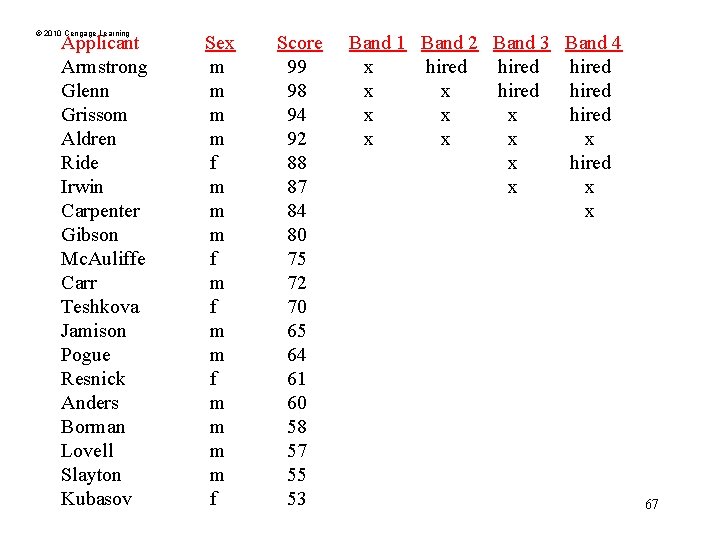
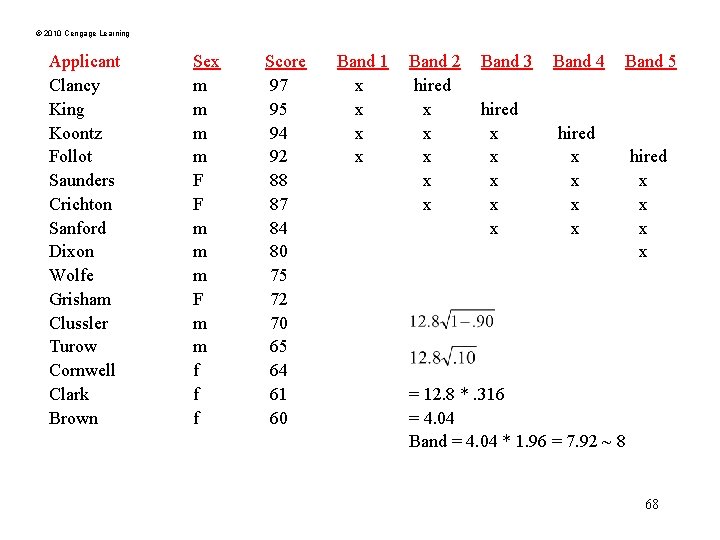
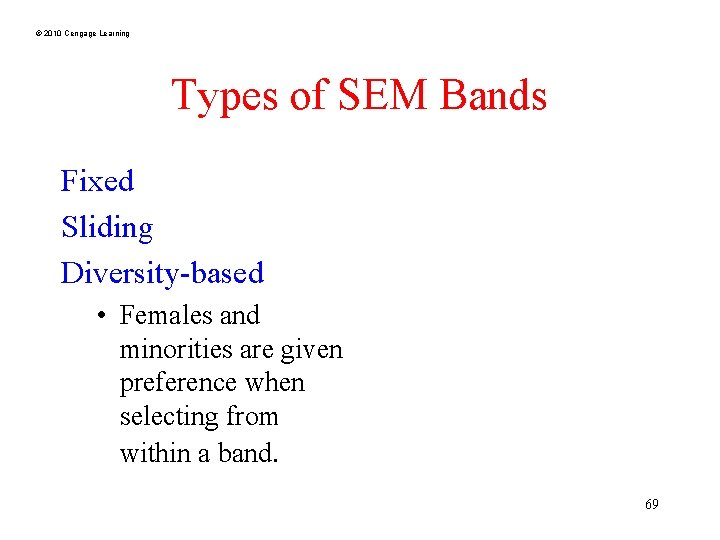
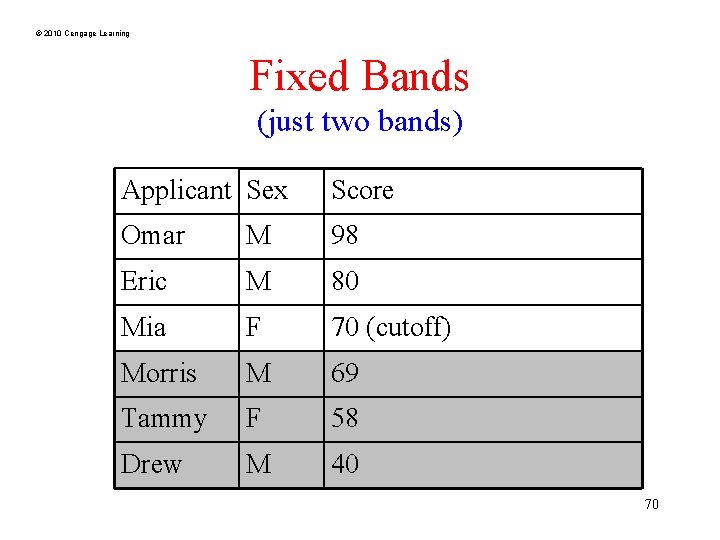
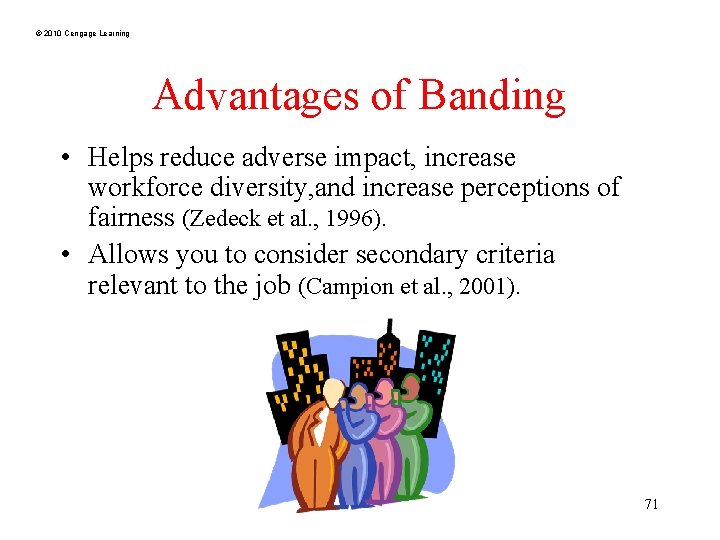
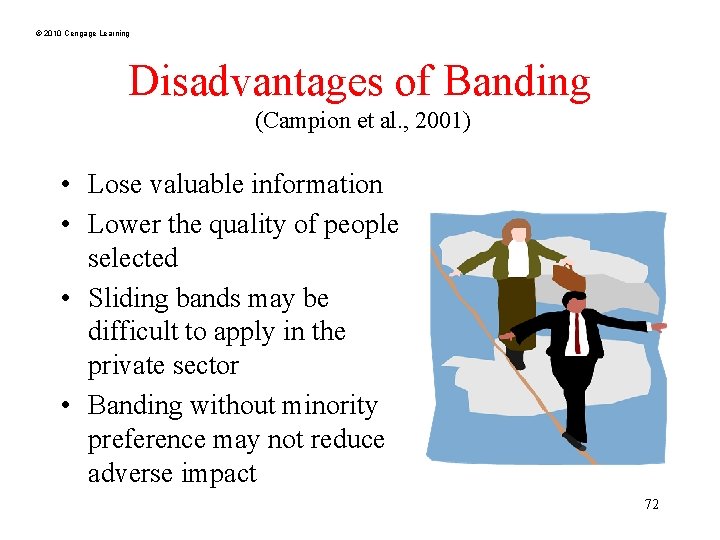
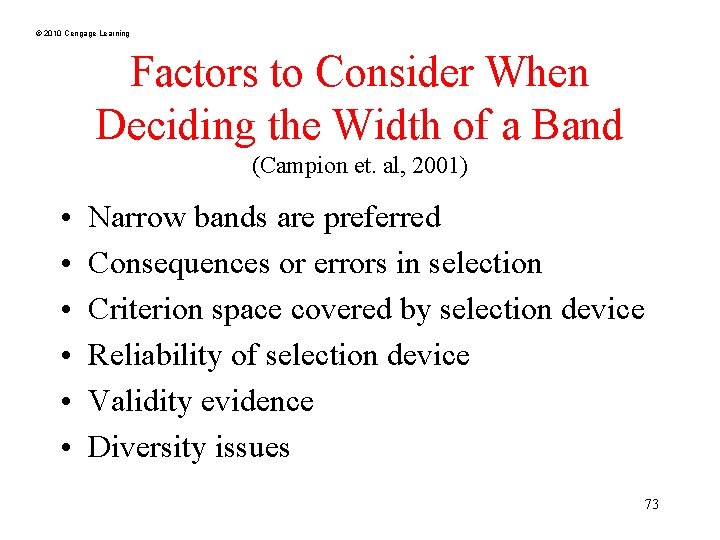
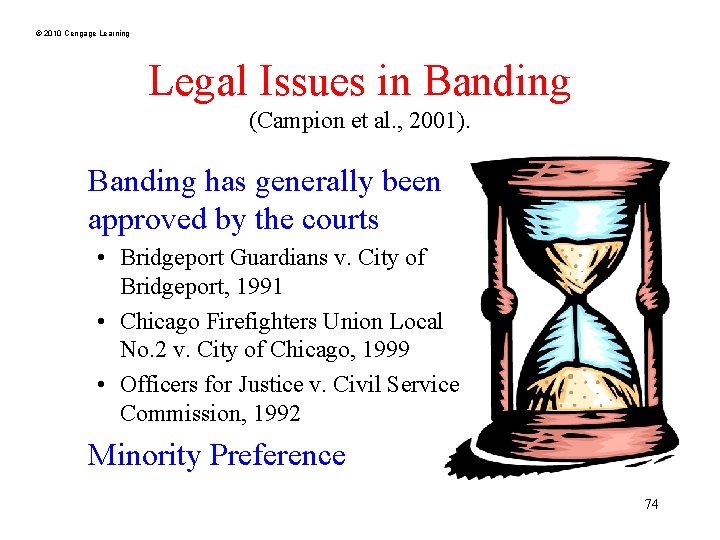
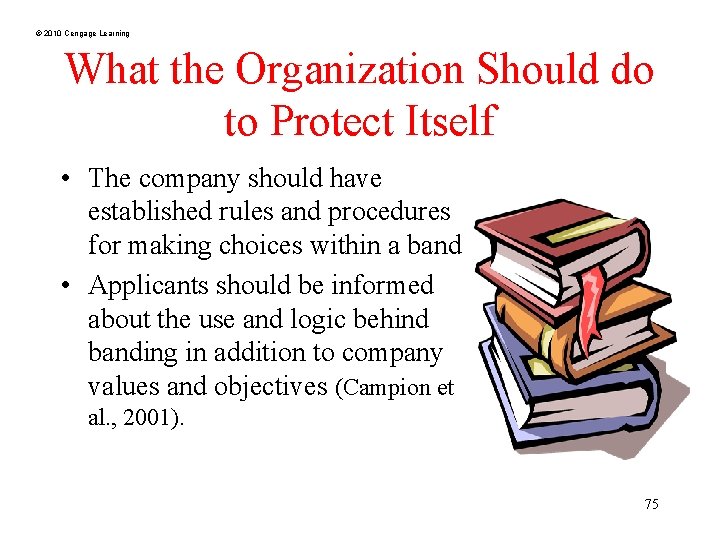
- Slides: 75
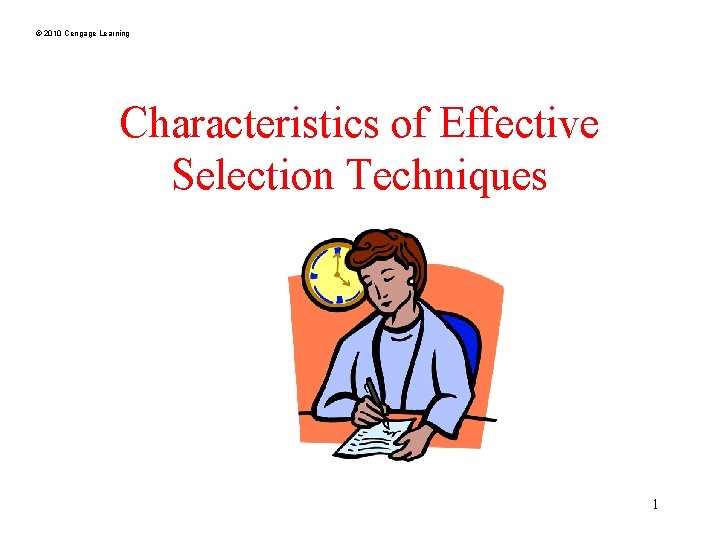
© 2010 Cengage Learning Characteristics of Effective Selection Techniques 1
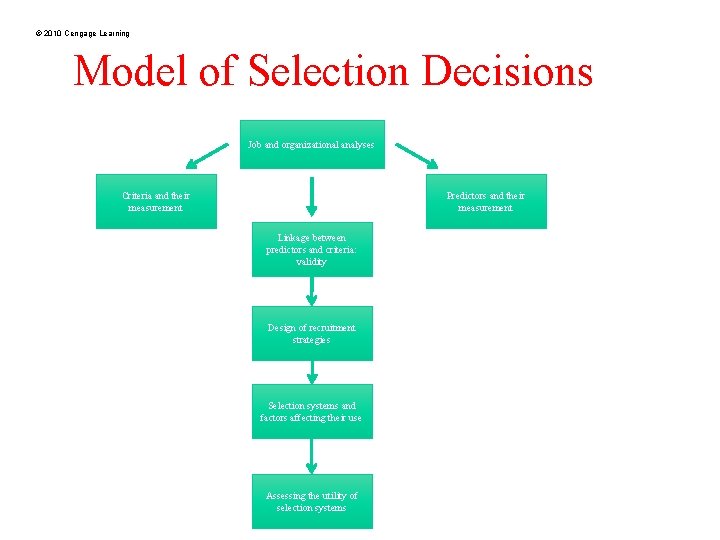
© 2010 Cengage Learning Model of Selection Decisions Job and organizational analyses Criteria and their measurement Predictors and their measurement Linkage between predictors and criteria: validity Design of recruitment strategies Selection systems and factors affecting their use Assessing the utility of selection systems
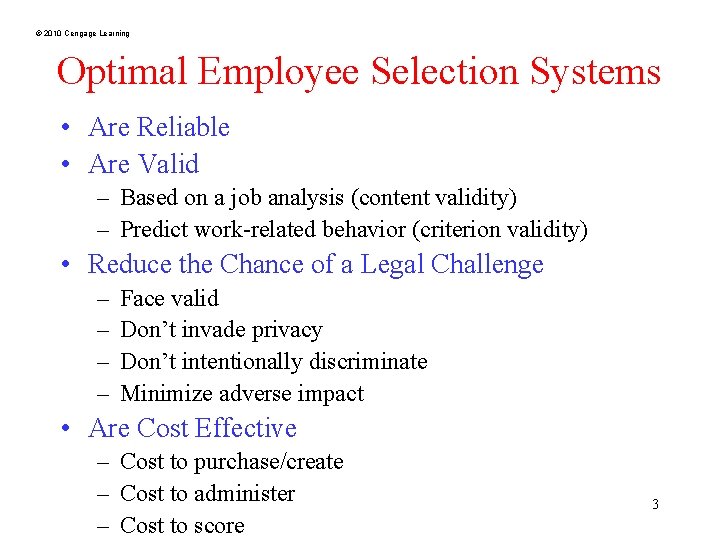
© 2010 Cengage Learning Optimal Employee Selection Systems • Are Reliable • Are Valid – Based on a job analysis (content validity) – Predict work-related behavior (criterion validity) • Reduce the Chance of a Legal Challenge – – Face valid Don’t invade privacy Don’t intentionally discriminate Minimize adverse impact • Are Cost Effective – Cost to purchase/create – Cost to administer – Cost to score 3
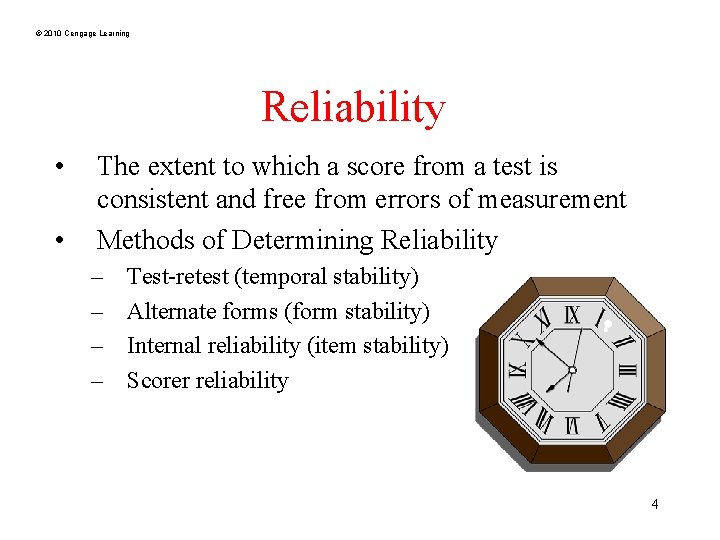
© 2010 Cengage Learning Reliability • • The extent to which a score from a test is consistent and free from errors of measurement Methods of Determining Reliability – – Test-retest (temporal stability) Alternate forms (form stability) Internal reliability (item stability) Scorer reliability 4
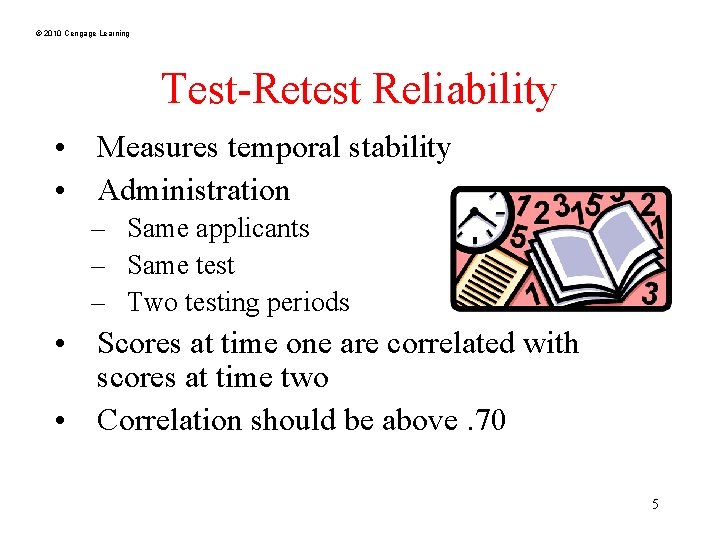
© 2010 Cengage Learning Test-Retest Reliability • Measures temporal stability • Administration – Same applicants – Same test – Two testing periods • Scores at time one are correlated with scores at time two • Correlation should be above. 70 5
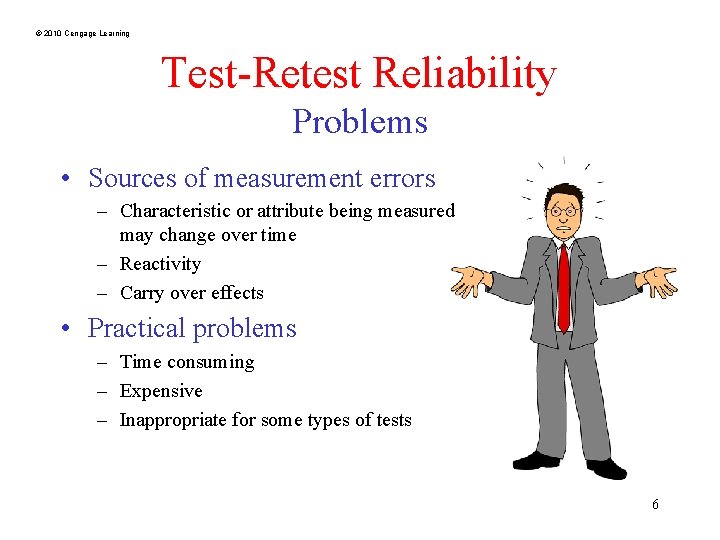
© 2010 Cengage Learning Test-Retest Reliability Problems • Sources of measurement errors – Characteristic or attribute being measured may change over time – Reactivity – Carry over effects • Practical problems – Time consuming – Expensive – Inappropriate for some types of tests 6
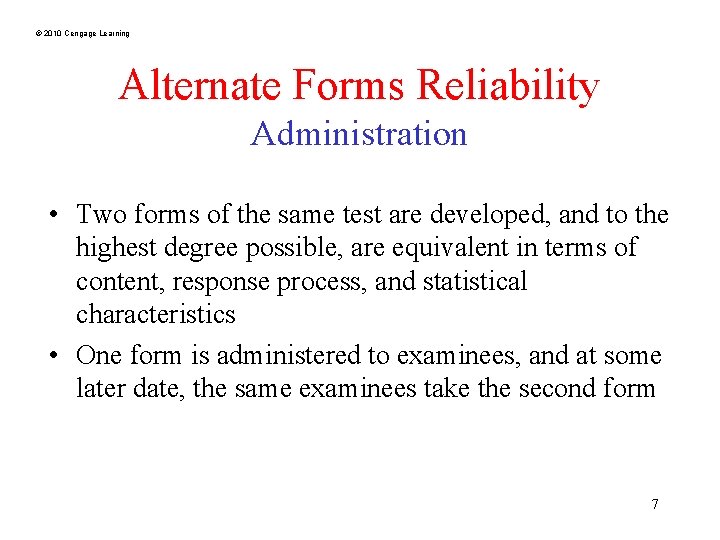
© 2010 Cengage Learning Alternate Forms Reliability Administration • Two forms of the same test are developed, and to the highest degree possible, are equivalent in terms of content, response process, and statistical characteristics • One form is administered to examinees, and at some later date, the same examinees take the second form 7
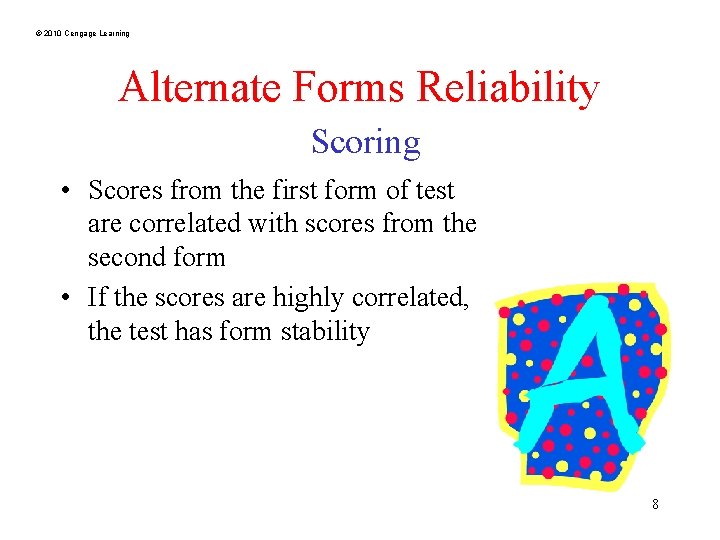
© 2010 Cengage Learning Alternate Forms Reliability Scoring • Scores from the first form of test are correlated with scores from the second form • If the scores are highly correlated, the test has form stability 8
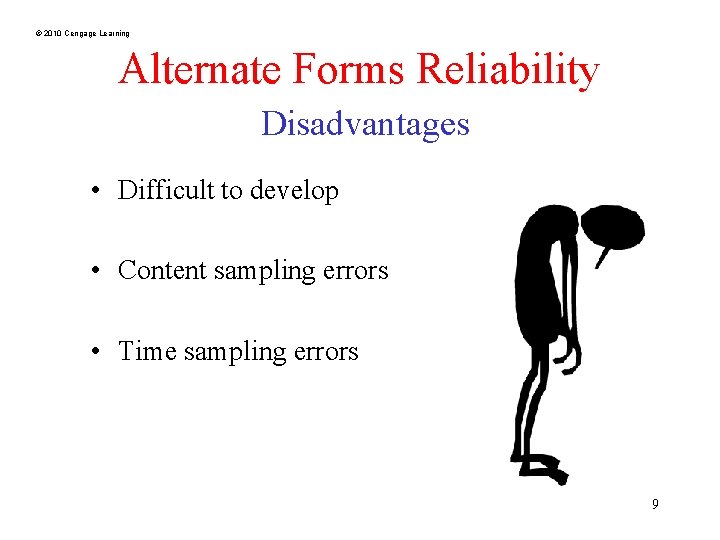
© 2010 Cengage Learning Alternate Forms Reliability Disadvantages • Difficult to develop • Content sampling errors • Time sampling errors 9
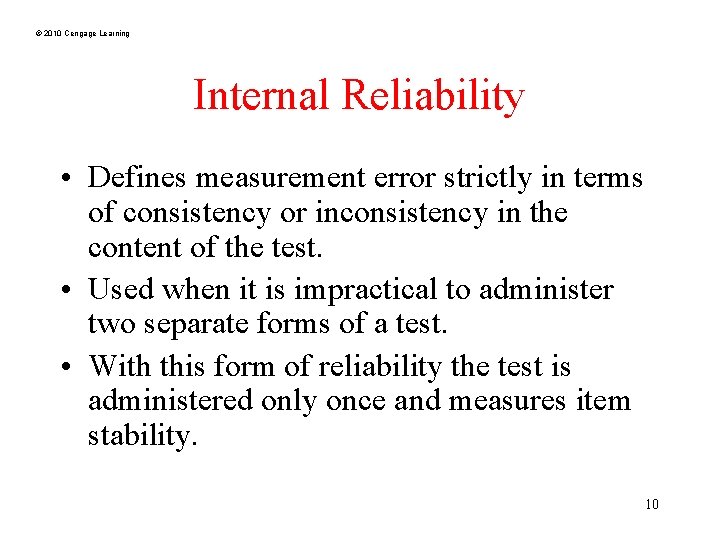
© 2010 Cengage Learning Internal Reliability • Defines measurement error strictly in terms of consistency or inconsistency in the content of the test. • Used when it is impractical to administer two separate forms of a test. • With this form of reliability the test is administered only once and measures item stability. 10
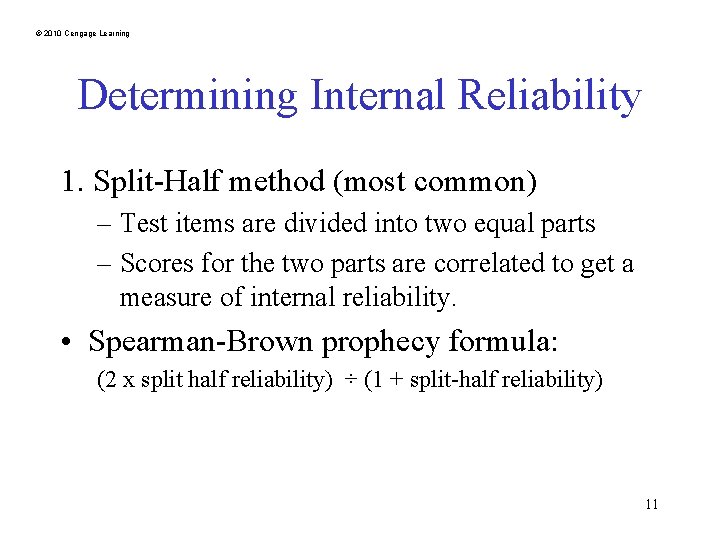
© 2010 Cengage Learning Determining Internal Reliability 1. Split-Half method (most common) – Test items are divided into two equal parts – Scores for the two parts are correlated to get a measure of internal reliability. • Spearman-Brown prophecy formula: (2 x split half reliability) ÷ (1 + split-half reliability) 11
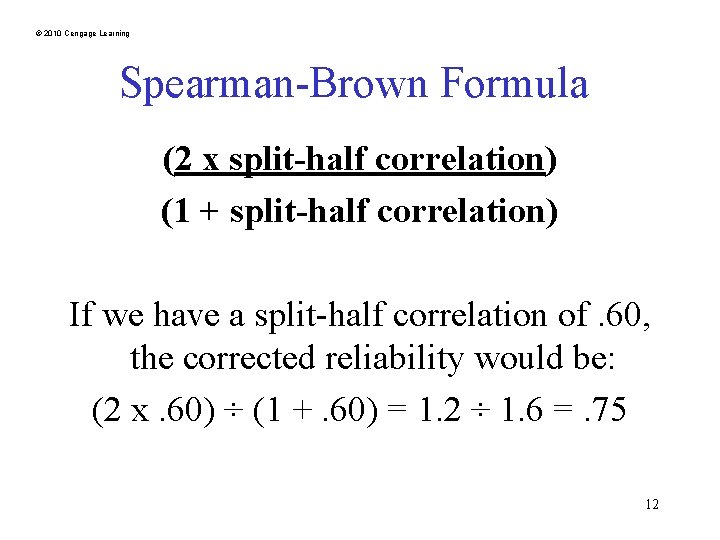
© 2010 Cengage Learning Spearman-Brown Formula (2 x split-half correlation) (1 + split-half correlation) If we have a split-half correlation of. 60, the corrected reliability would be: (2 x. 60) ÷ (1 +. 60) = 1. 2 ÷ 1. 6 =. 75 12
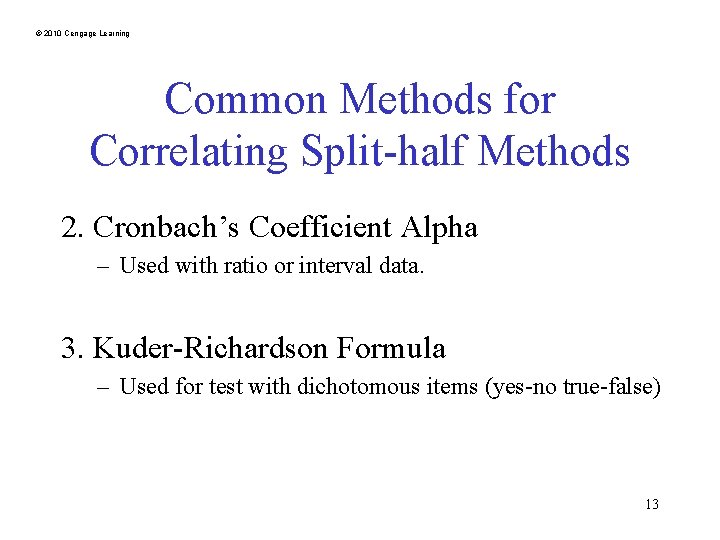
© 2010 Cengage Learning Common Methods for Correlating Split-half Methods 2. Cronbach’s Coefficient Alpha – Used with ratio or interval data. 3. Kuder-Richardson Formula – Used for test with dichotomous items (yes-no true-false) 13
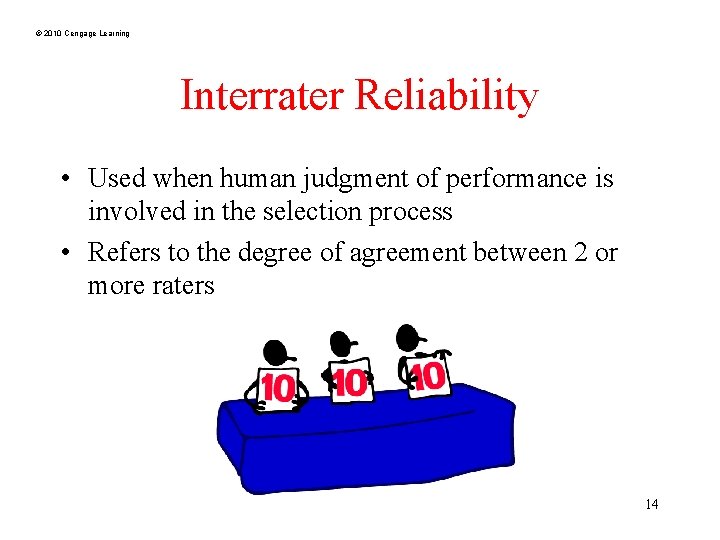
© 2010 Cengage Learning Interrater Reliability • Used when human judgment of performance is involved in the selection process • Refers to the degree of agreement between 2 or more raters 14
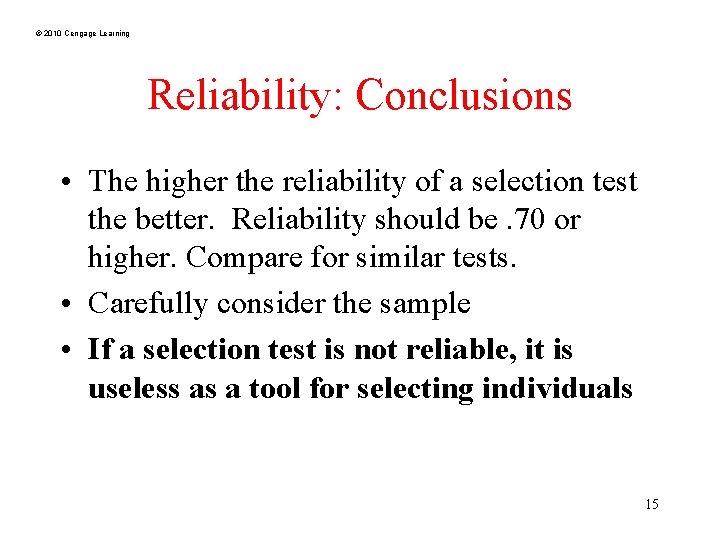
© 2010 Cengage Learning Reliability: Conclusions • The higher the reliability of a selection test the better. Reliability should be. 70 or higher. Compare for similar tests. • Carefully consider the sample • If a selection test is not reliable, it is useless as a tool for selecting individuals 15
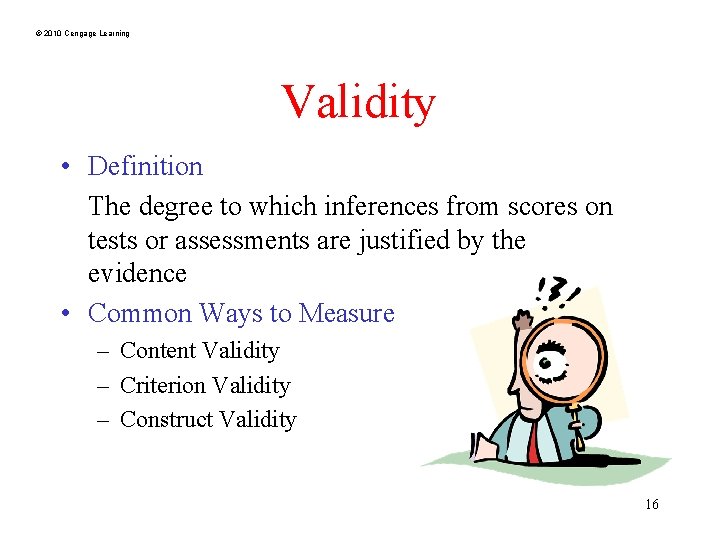
© 2010 Cengage Learning Validity • Definition The degree to which inferences from scores on tests or assessments are justified by the evidence • Common Ways to Measure – Content Validity – Criterion Validity – Construct Validity 16
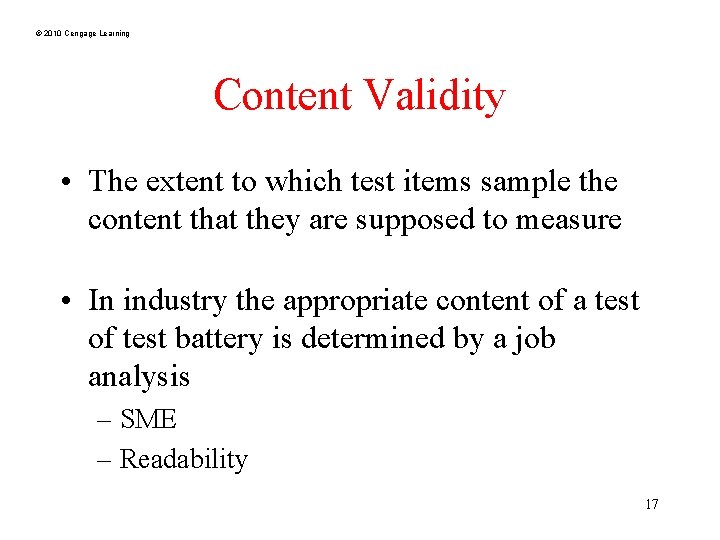
© 2010 Cengage Learning Content Validity • The extent to which test items sample the content that they are supposed to measure • In industry the appropriate content of a test of test battery is determined by a job analysis – SME – Readability 17
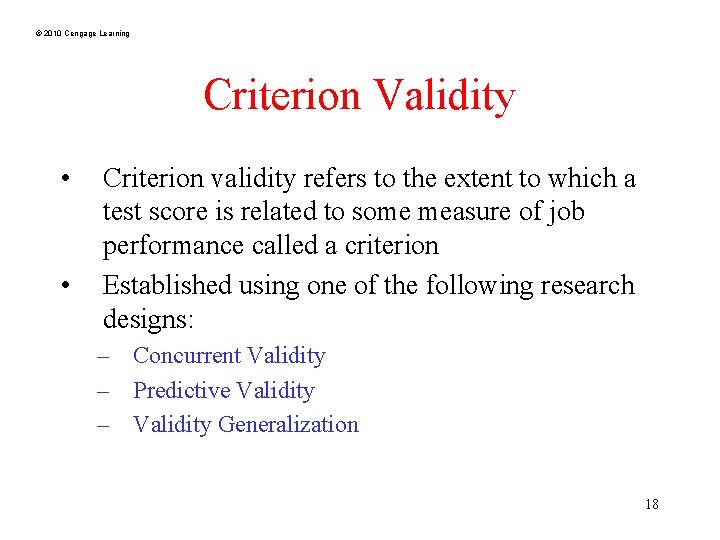
© 2010 Cengage Learning Criterion Validity • • Criterion validity refers to the extent to which a test score is related to some measure of job performance called a criterion Established using one of the following research designs: – Concurrent Validity – Predictive Validity – Validity Generalization 18
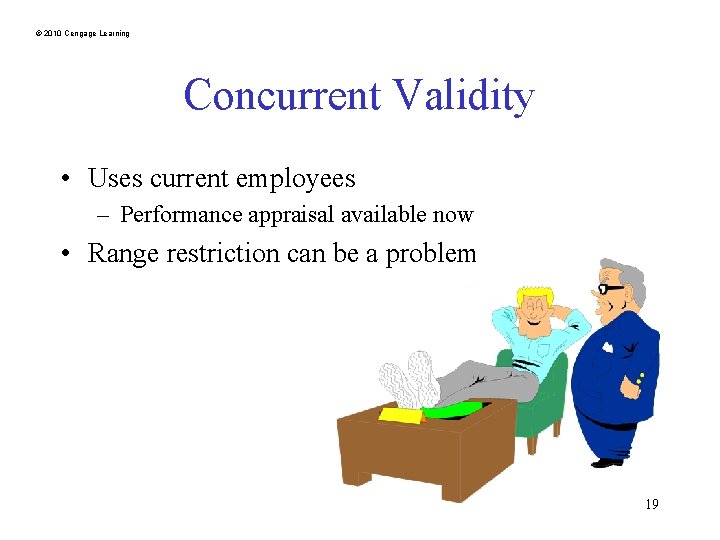
© 2010 Cengage Learning Concurrent Validity • Uses current employees – Performance appraisal available now • Range restriction can be a problem 19
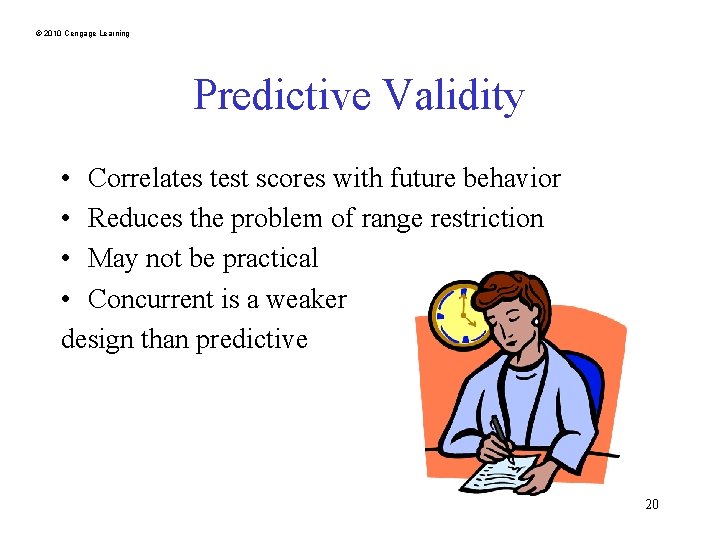
© 2010 Cengage Learning Predictive Validity • Correlates test scores with future behavior • Reduces the problem of range restriction • May not be practical • Concurrent is a weaker design than predictive 20
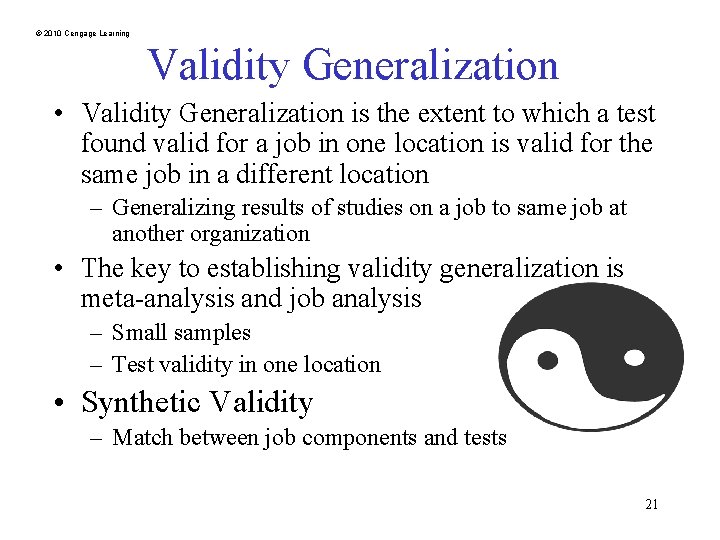
© 2010 Cengage Learning Validity Generalization • Validity Generalization is the extent to which a test found valid for a job in one location is valid for the same job in a different location – Generalizing results of studies on a job to same job at another organization • The key to establishing validity generalization is meta-analysis and job analysis – Small samples – Test validity in one location • Synthetic Validity – Match between job components and tests 21
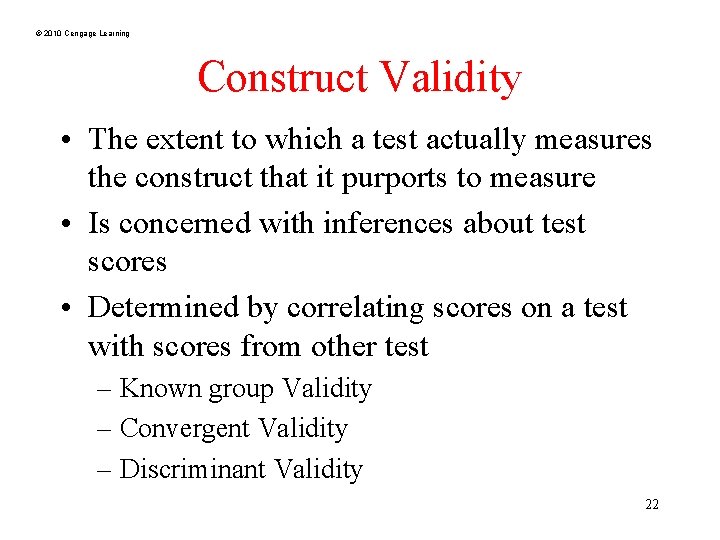
© 2010 Cengage Learning Construct Validity • The extent to which a test actually measures the construct that it purports to measure • Is concerned with inferences about test scores • Determined by correlating scores on a test with scores from other test – Known group Validity – Convergent Validity – Discriminant Validity 22
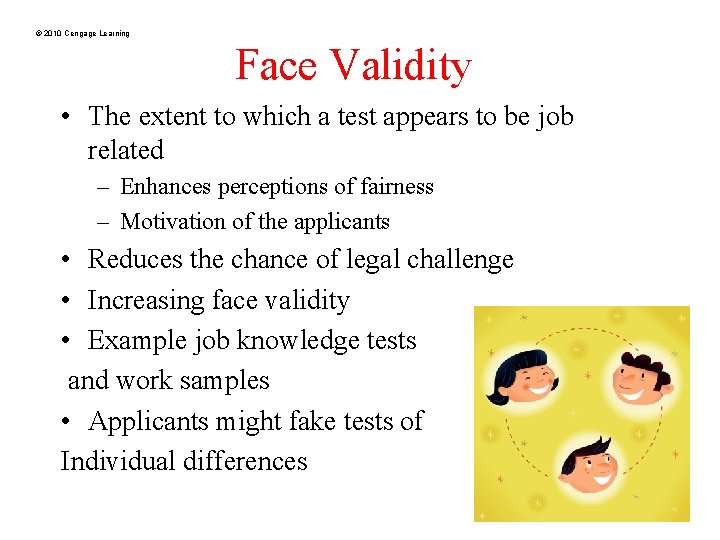
© 2010 Cengage Learning Face Validity • The extent to which a test appears to be job related – Enhances perceptions of fairness – Motivation of the applicants • Reduces the chance of legal challenge • Increasing face validity • Example job knowledge tests and work samples • Applicants might fake tests of Individual differences 23
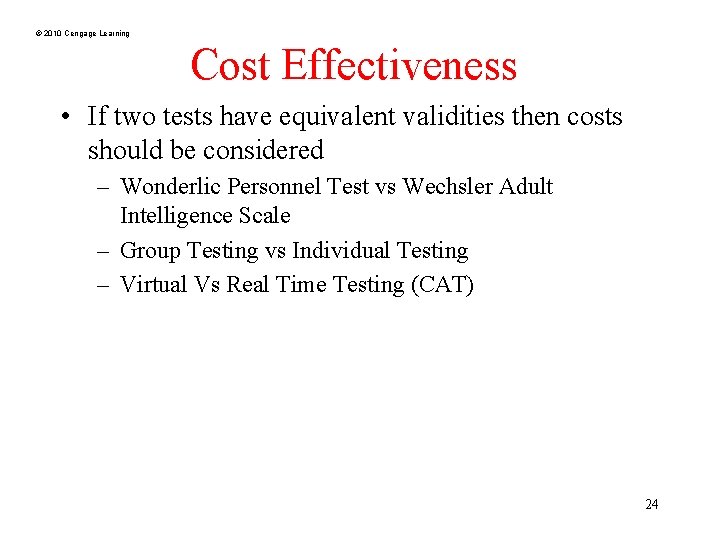
© 2010 Cengage Learning Cost Effectiveness • If two tests have equivalent validities then costs should be considered – Wonderlic Personnel Test vs Wechsler Adult Intelligence Scale – Group Testing vs Individual Testing – Virtual Vs Real Time Testing (CAT) 24
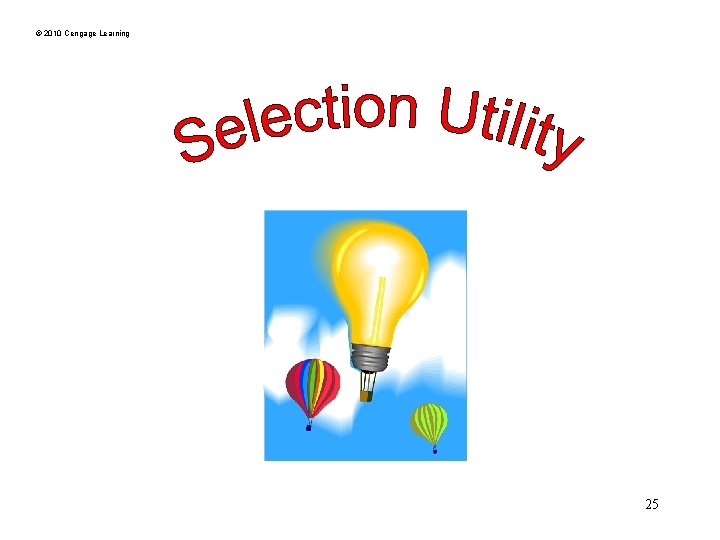
© 2010 Cengage Learning 25
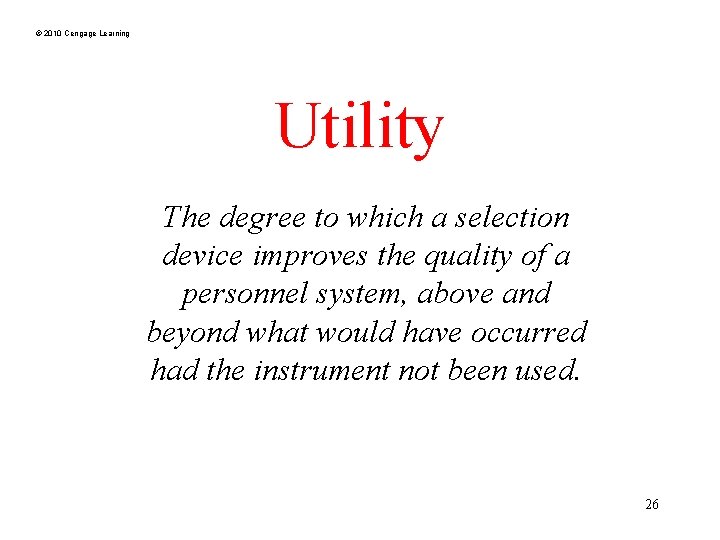
© 2010 Cengage Learning Utility The degree to which a selection device improves the quality of a personnel system, above and beyond what would have occurred had the instrument not been used. 26
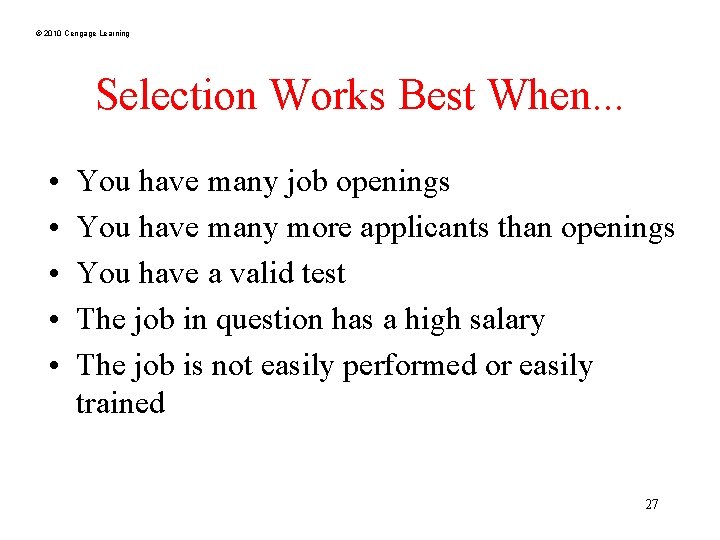
© 2010 Cengage Learning Selection Works Best When. . . • • • You have many job openings You have many more applicants than openings You have a valid test The job in question has a high salary The job is not easily performed or easily trained 27
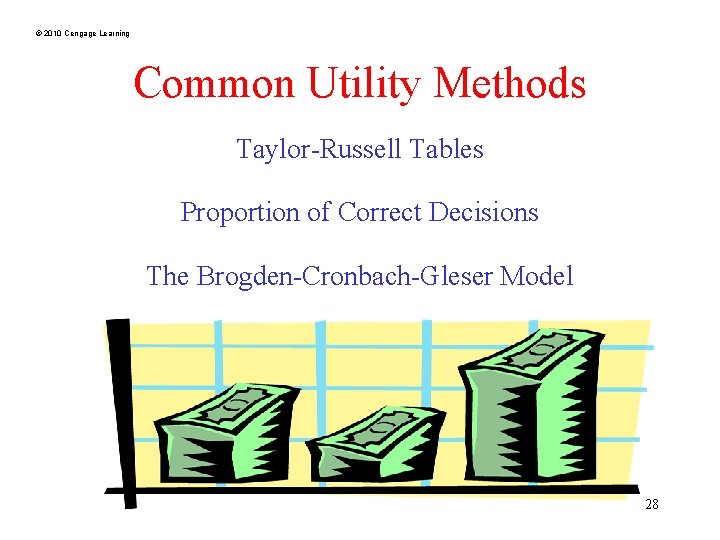
© 2010 Cengage Learning Common Utility Methods Taylor-Russell Tables Proportion of Correct Decisions The Brogden-Cronbach-Gleser Model 28
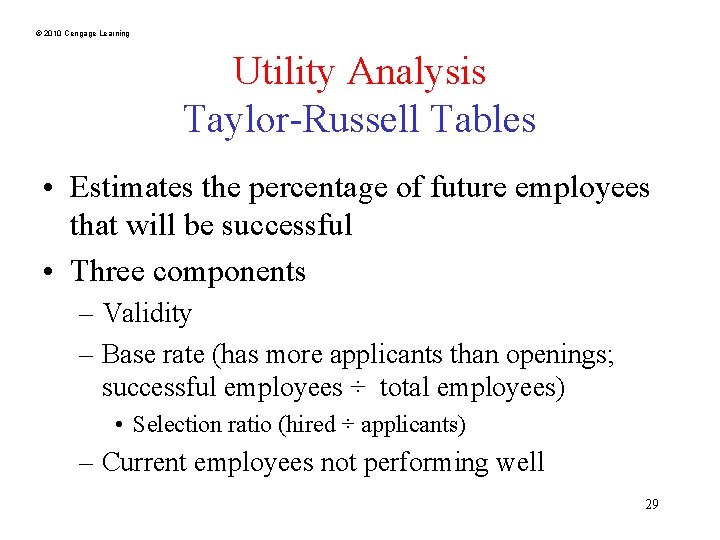
© 2010 Cengage Learning Utility Analysis Taylor-Russell Tables • Estimates the percentage of future employees that will be successful • Three components – Validity – Base rate (has more applicants than openings; successful employees ÷ total employees) • Selection ratio (hired ÷ applicants) – Current employees not performing well 29
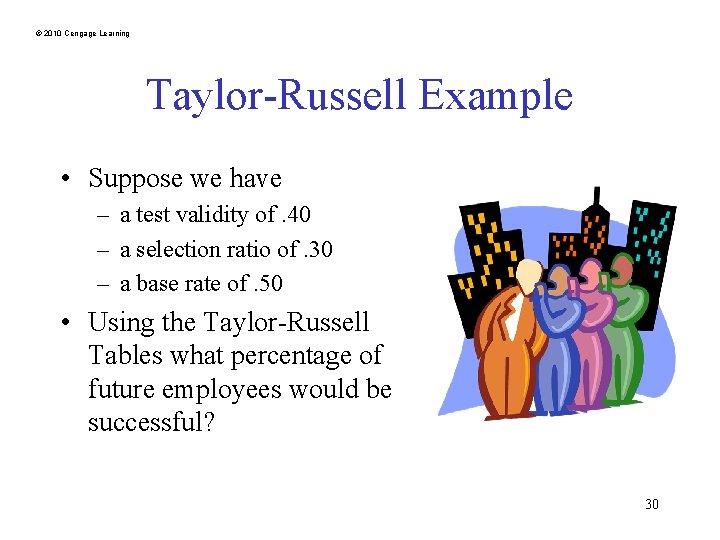
© 2010 Cengage Learning Taylor-Russell Example • Suppose we have – a test validity of. 40 – a selection ratio of. 30 – a base rate of. 50 • Using the Taylor-Russell Tables what percentage of future employees would be successful? 30
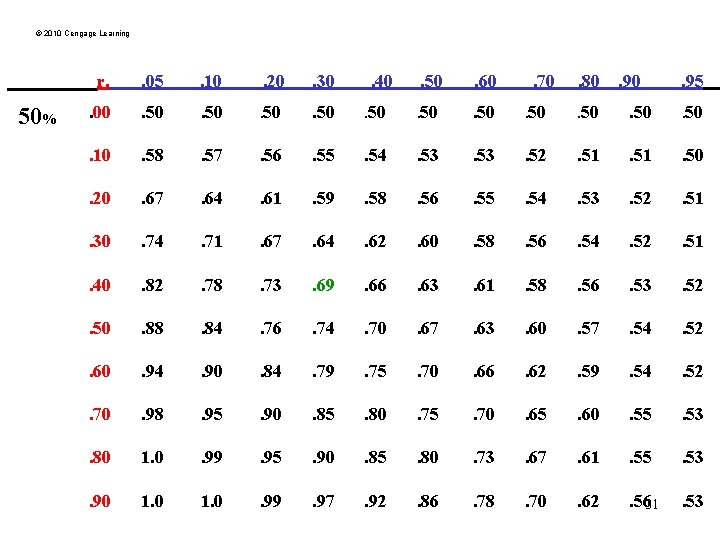
© 2010 Cengage Learning r. . 05 . 10 . 20 . 30 . 40 . 50 . 60 . 70 . 80 . 95 50% . 00 . 50 . 10 . 58 . 57 . 56 . 55 . 54 . 53 . 52 . 51 . 50 . 20 . 67 . 64 . 61 . 59 . 58 . 56 . 55 . 54 . 53 . 52 . 51 . 30 . 74 . 71 . 67 . 64 . 62 . 60 . 58 . 56 . 54 . 52 . 51 . 40 . 82 . 78 . 73 . 69 . 66 . 63 . 61 . 58 . 56 . 53 . 52 . 50 . 88 . 84 . 76 . 74 . 70 . 67 . 63 . 60 . 57 . 54 . 52 . 60 . 94 . 90 . 84 . 79 . 75 . 70 . 66 . 62 . 59 . 54 . 52 . 70 . 98 . 95 . 90 . 85 . 80 . 75 . 70 . 65 . 60 . 55 . 53 . 80 1. 0 . 99 . 95 . 90 . 85 . 80 . 73 . 67 . 61 . 55 . 53 . 90 1. 0 . 99 . 97 . 92 . 86 . 78 . 70 . 62 . 5631 . 53
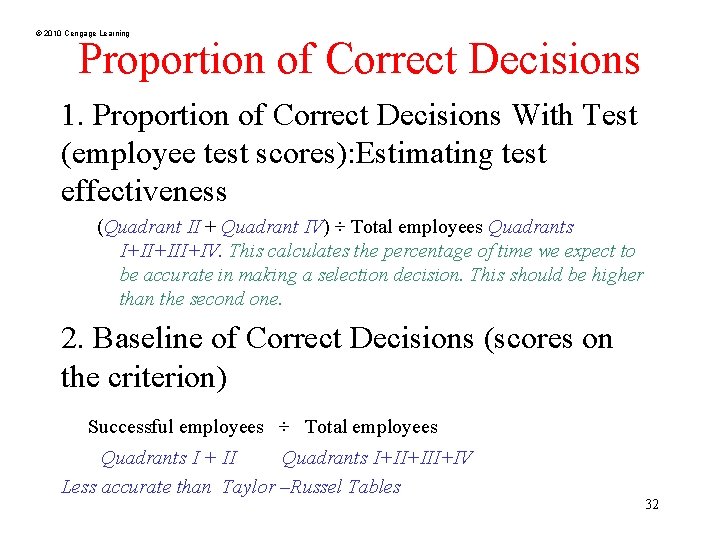
© 2010 Cengage Learning Proportion of Correct Decisions 1. Proportion of Correct Decisions With Test (employee test scores): Estimating test effectiveness (Quadrant II + Quadrant IV) ÷ Total employees Quadrants I+II+IV. This calculates the percentage of time we expect to be accurate in making a selection decision. This should be higher than the second one. 2. Baseline of Correct Decisions (scores on the criterion) Successful employees ÷ Total employees Quadrants I + II Quadrants I+II+IV Less accurate than Taylor –Russel Tables 32
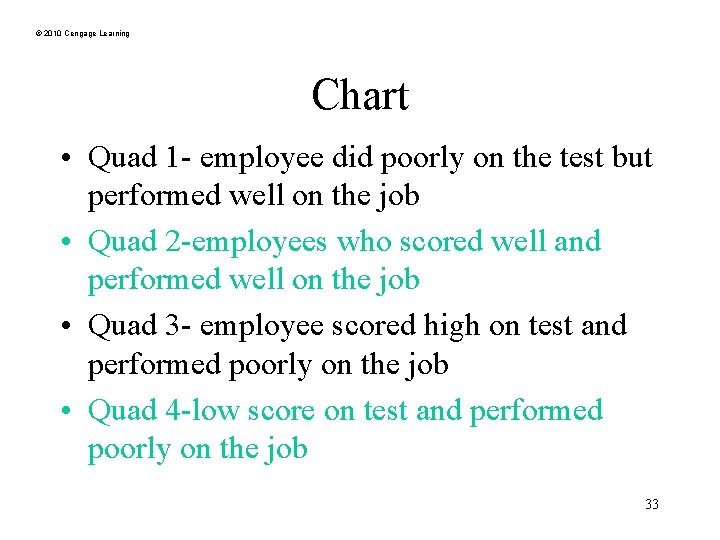
© 2010 Cengage Learning Chart • Quad 1 - employee did poorly on the test but performed well on the job • Quad 2 -employees who scored well and performed well on the job • Quad 3 - employee scored high on test and performed poorly on the job • Quad 4 -low score on test and performed poorly on the job 33
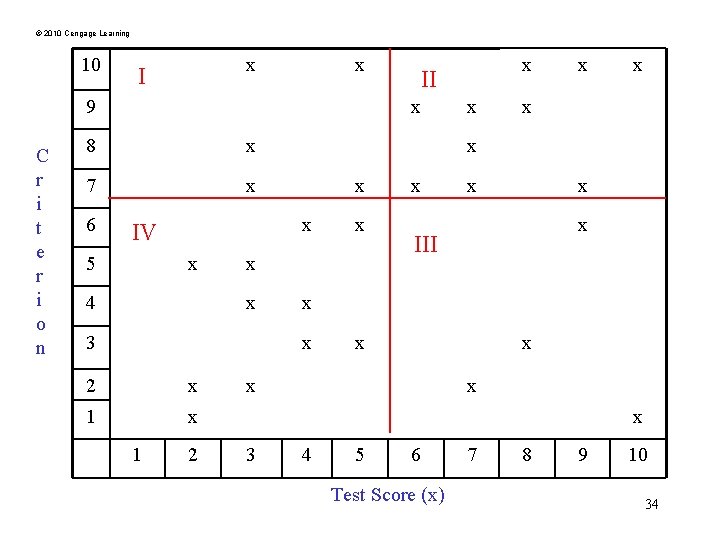
© 2010 Cengage Learning 10 x I x 9 C r i t e r i o n x 7 x 6 II x 8 5 x 4 x 3 x 1 2 x x x III x x 2 x x x x IV x x x 3 4 5 6 Test Score (x) 7 8 9 10 34
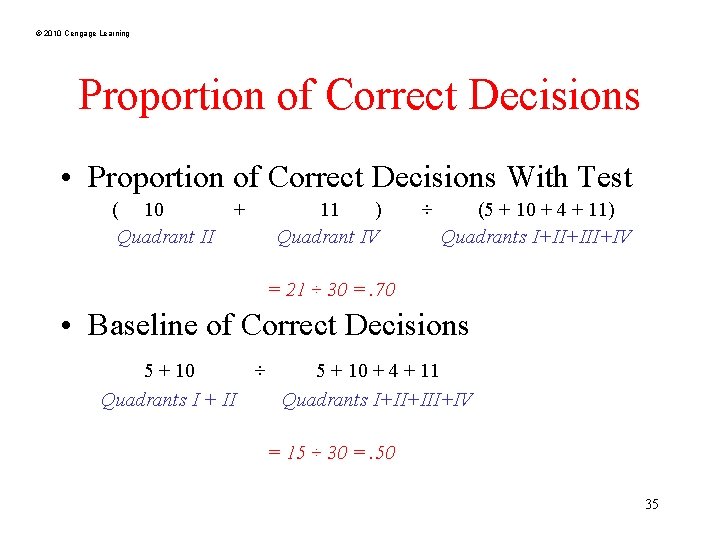
© 2010 Cengage Learning Proportion of Correct Decisions • Proportion of Correct Decisions With Test ( 10 Quadrant II + 11 ) Quadrant IV ÷ (5 + 10 + 4 + 11) Quadrants I+II+IV = 21 ÷ 30 =. 70 • Baseline of Correct Decisions 5 + 10 Quadrants I + II ÷ 5 + 10 + 4 + 11 Quadrants I+II+IV = 15 ÷ 30 =. 50 35
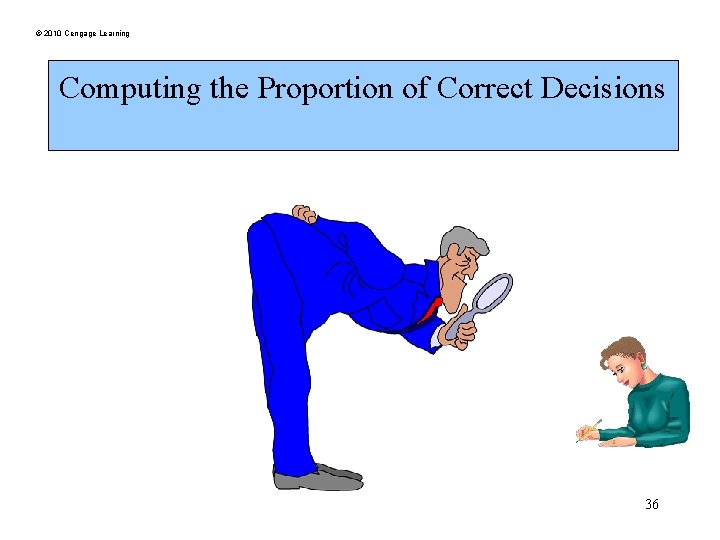
© 2010 Cengage Learning Computing the Proportion of Correct Decisions 36
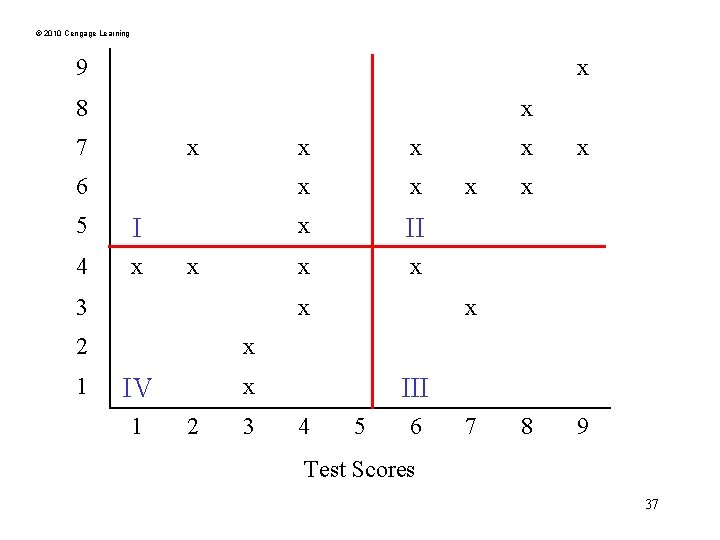
© 2010 Cengage Learning 9 x 8 x 7 x 6 5 I 4 x x 3 x x II x x x 2 1 x x x x IV 1 2 3 III 4 5 6 7 8 9 Test Scores 37
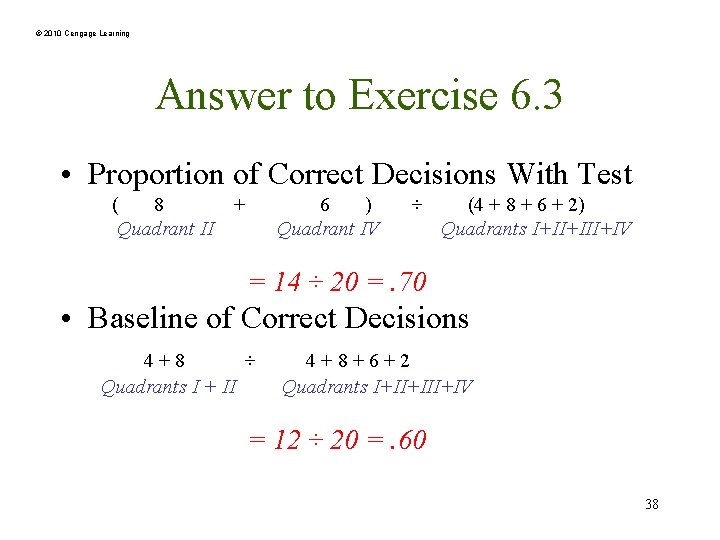
© 2010 Cengage Learning Answer to Exercise 6. 3 • Proportion of Correct Decisions With Test ( 8 Quadrant II + 6 ) Quadrant IV ÷ (4 + 8 + 6 + 2) Quadrants I+II+IV = 14 ÷ 20 =. 70 • Baseline of Correct Decisions 4+8 ÷ Quadrants I + II 4+8+6+2 Quadrants I+II+IV = 12 ÷ 20 =. 60 38
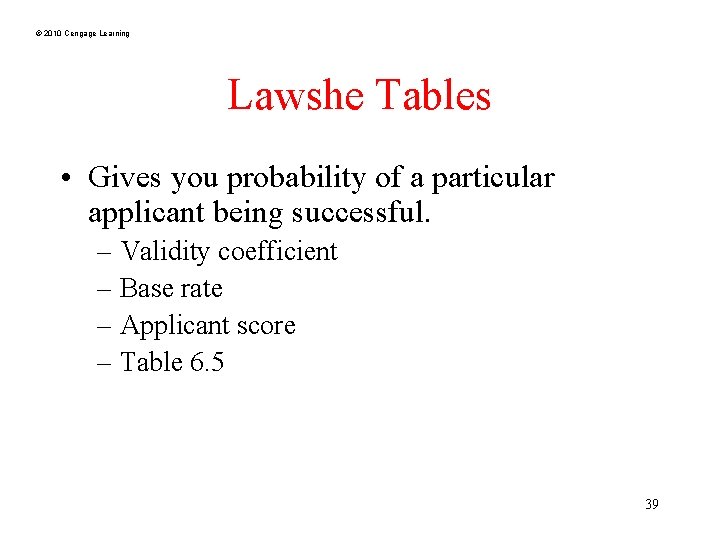
© 2010 Cengage Learning Lawshe Tables • Gives you probability of a particular applicant being successful. – Validity coefficient – Base rate – Applicant score – Table 6. 5 39
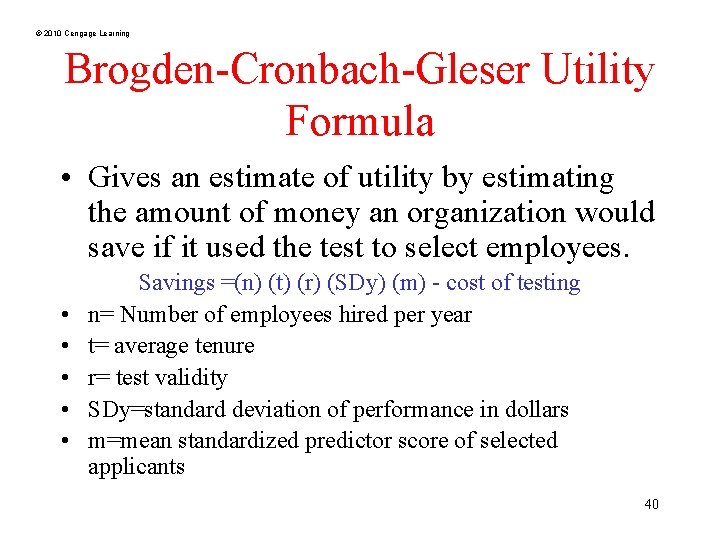
© 2010 Cengage Learning Brogden-Cronbach-Gleser Utility Formula • Gives an estimate of utility by estimating the amount of money an organization would save if it used the test to select employees. • • • Savings =(n) (t) (r) (SDy) (m) - cost of testing n= Number of employees hired per year t= average tenure r= test validity SDy=standard deviation of performance in dollars m=mean standardized predictor score of selected applicants 40
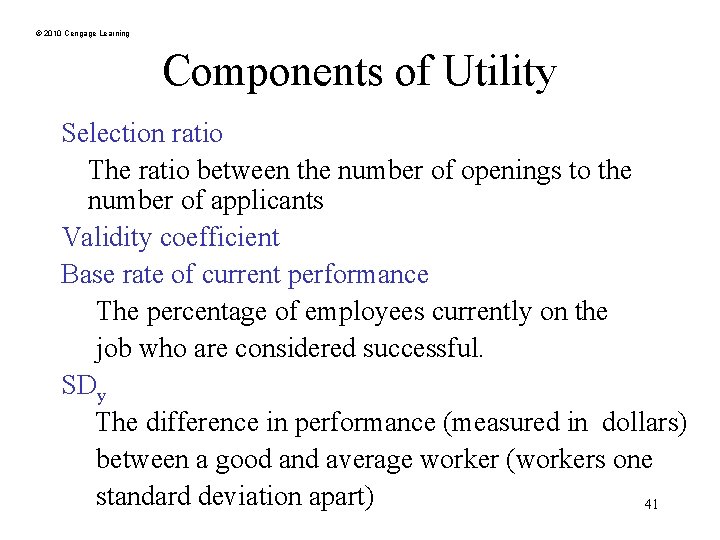
© 2010 Cengage Learning Components of Utility Selection ratio The ratio between the number of openings to the number of applicants Validity coefficient Base rate of current performance The percentage of employees currently on the job who are considered successful. SDy The difference in performance (measured in dollars) between a good and average worker (workers one standard deviation apart) 41
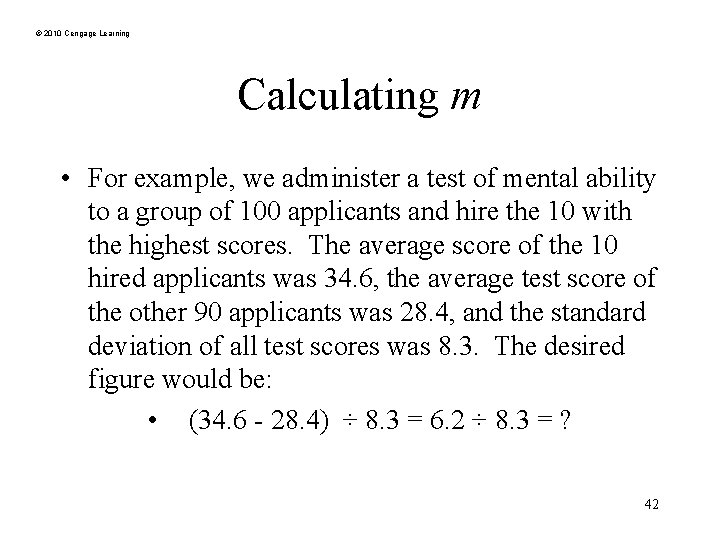
© 2010 Cengage Learning Calculating m • For example, we administer a test of mental ability to a group of 100 applicants and hire the 10 with the highest scores. The average score of the 10 hired applicants was 34. 6, the average test score of the other 90 applicants was 28. 4, and the standard deviation of all test scores was 8. 3. The desired figure would be: • (34. 6 - 28. 4) ÷ 8. 3 = 6. 2 ÷ 8. 3 = ? 42
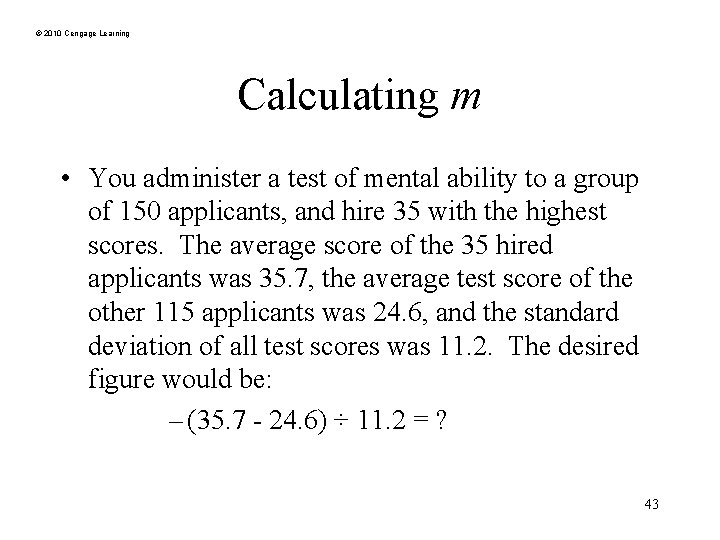
© 2010 Cengage Learning Calculating m • You administer a test of mental ability to a group of 150 applicants, and hire 35 with the highest scores. The average score of the 35 hired applicants was 35. 7, the average test score of the other 115 applicants was 24. 6, and the standard deviation of all test scores was 11. 2. The desired figure would be: – (35. 7 - 24. 6) ÷ 11. 2 = ? 43
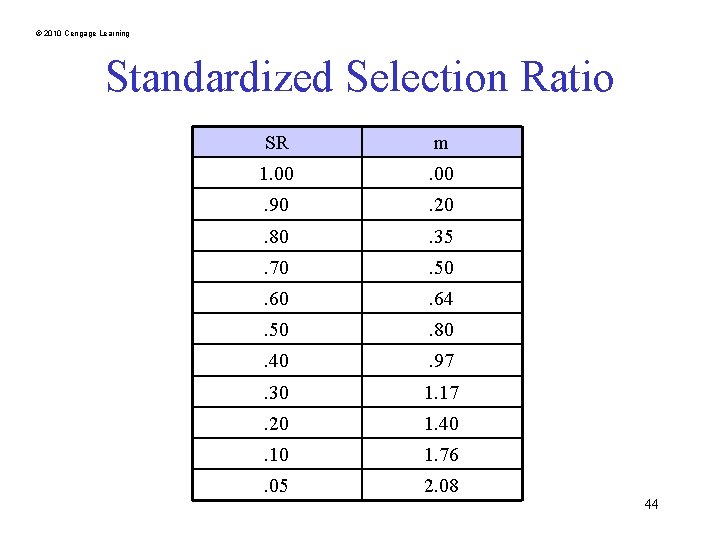
© 2010 Cengage Learning Standardized Selection Ratio SR m 1. 00 . 90 . 20 . 80 . 35 . 70 . 50 . 64 . 50 . 80 . 40 . 97 . 30 1. 17 . 20 1. 40 . 10 1. 76 . 05 2. 08 44
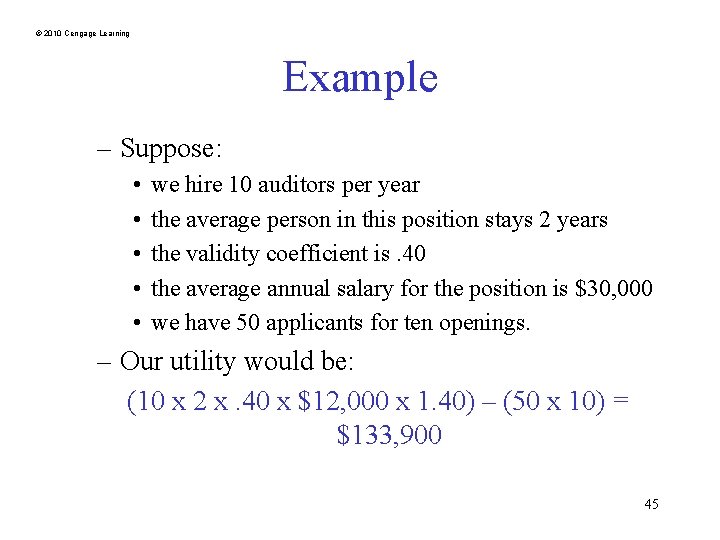
© 2010 Cengage Learning Example – Suppose: • • • we hire 10 auditors per year the average person in this position stays 2 years the validity coefficient is. 40 the average annual salary for the position is $30, 000 we have 50 applicants for ten openings. – Our utility would be: (10 x 2 x. 40 x $12, 000 x 1. 40) – (50 x 10) = $133, 900 45
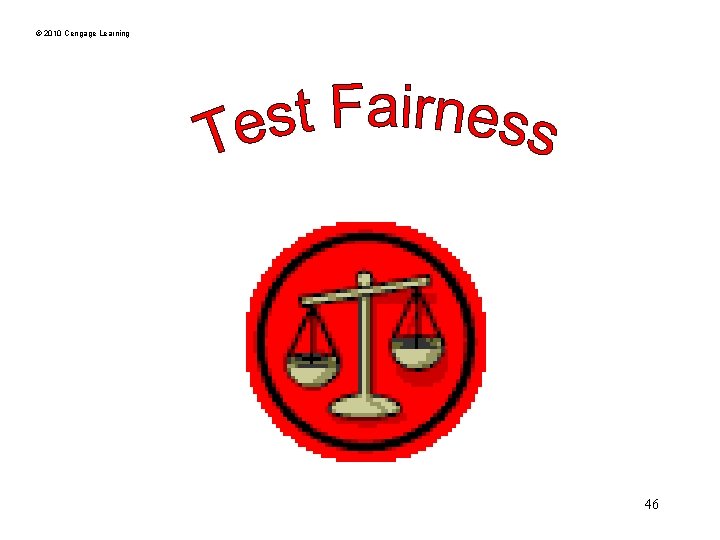
© 2010 Cengage Learning 46
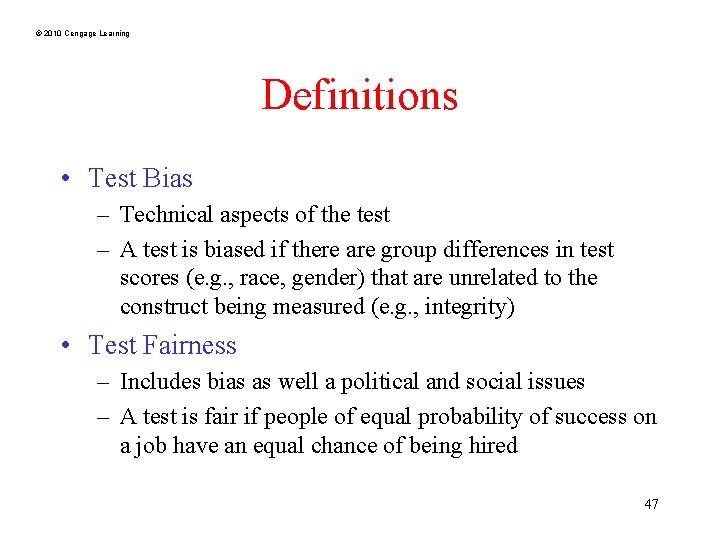
© 2010 Cengage Learning Definitions • Test Bias – Technical aspects of the test – A test is biased if there are group differences in test scores (e. g. , race, gender) that are unrelated to the construct being measured (e. g. , integrity) • Test Fairness – Includes bias as well a political and social issues – A test is fair if people of equal probability of success on a job have an equal chance of being hired 47
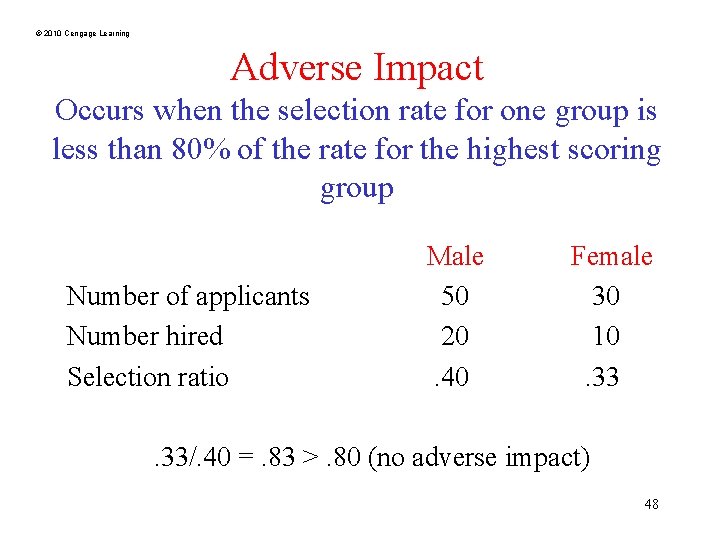
© 2010 Cengage Learning Adverse Impact Occurs when the selection rate for one group is less than 80% of the rate for the highest scoring group Number of applicants Number hired Selection ratio Male 50 20. 40 Female 30 10. 33/. 40 =. 83 >. 80 (no adverse impact) 48
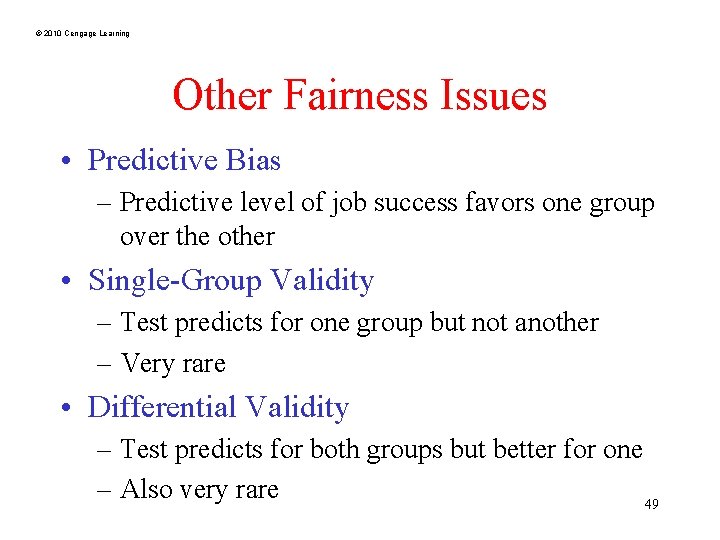
© 2010 Cengage Learning Other Fairness Issues • Predictive Bias – Predictive level of job success favors one group over the other • Single-Group Validity – Test predicts for one group but not another – Very rare • Differential Validity – Test predicts for both groups but better for one – Also very rare 49
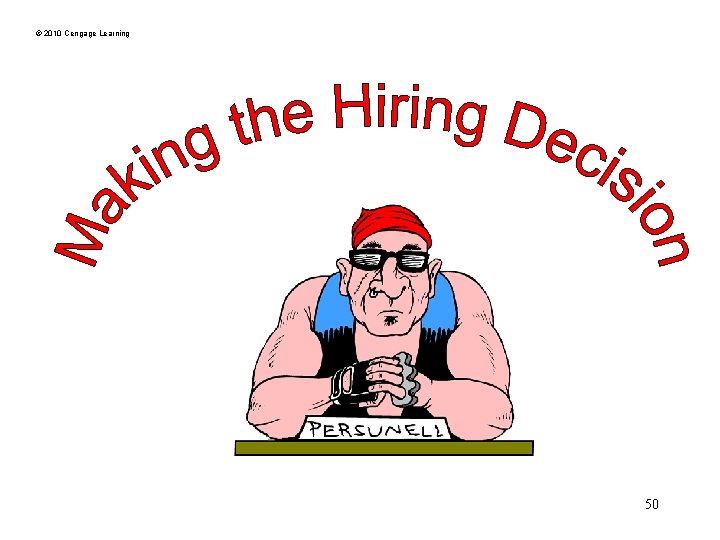
© 2010 Cengage Learning 50
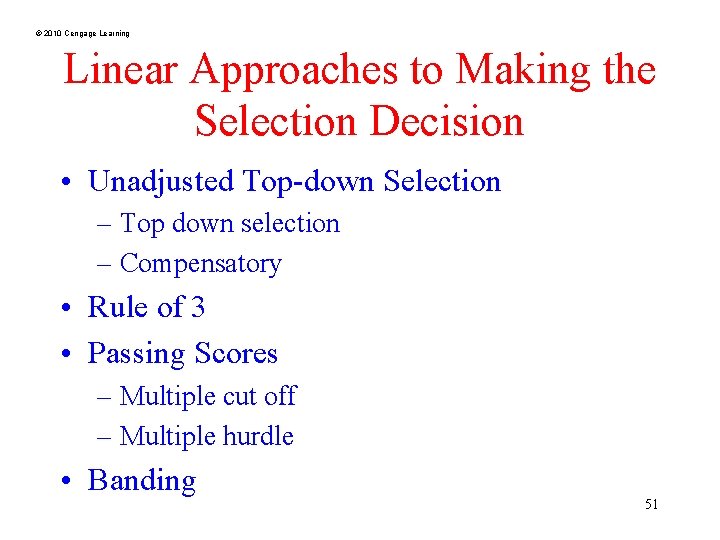
© 2010 Cengage Learning Linear Approaches to Making the Selection Decision • Unadjusted Top-down Selection – Top down selection – Compensatory • Rule of 3 • Passing Scores – Multiple cut off – Multiple hurdle • Banding 51
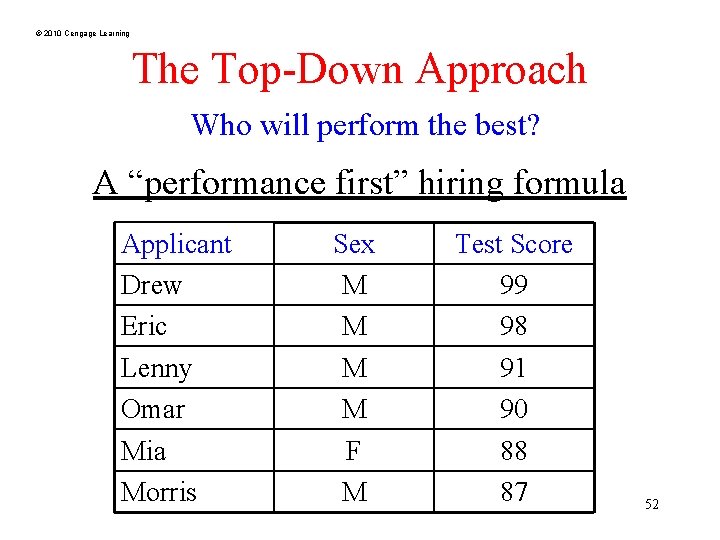
© 2010 Cengage Learning The Top-Down Approach Who will perform the best? A “performance first” hiring formula Applicant Drew Eric Lenny Omar Mia Morris Sex M M F M Test Score 99 98 91 90 88 87 52
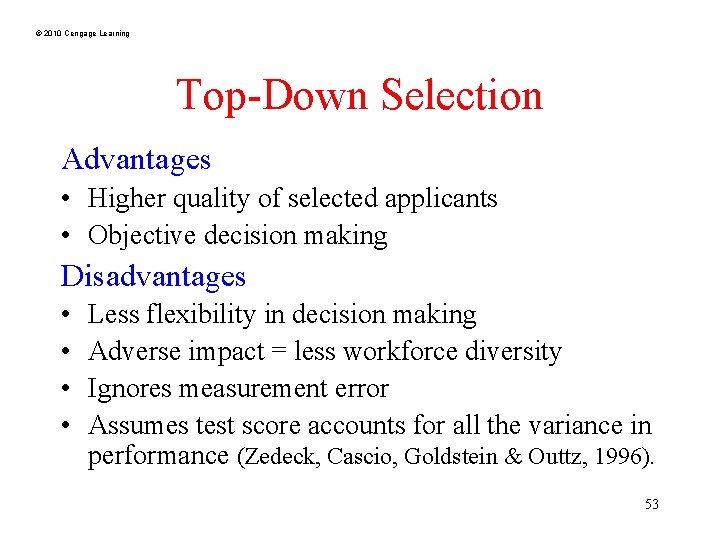
© 2010 Cengage Learning Top-Down Selection Advantages • Higher quality of selected applicants • Objective decision making Disadvantages • • Less flexibility in decision making Adverse impact = less workforce diversity Ignores measurement error Assumes test score accounts for all the variance in performance (Zedeck, Cascio, Goldstein & Outtz, 1996). 53
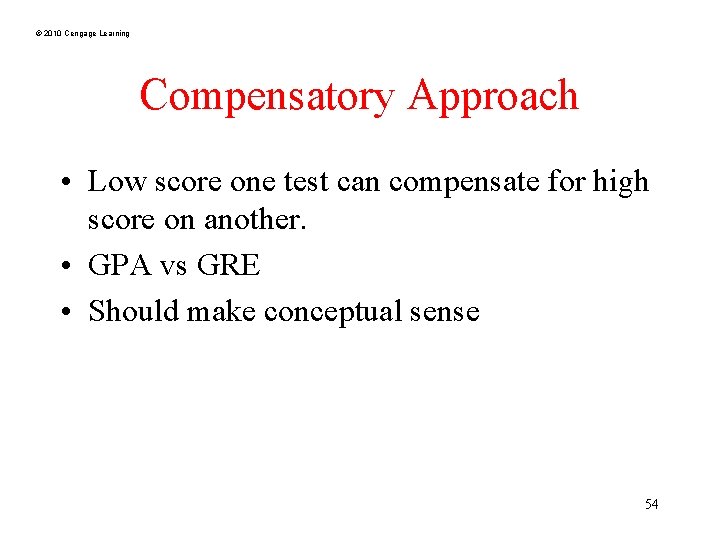
© 2010 Cengage Learning Compensatory Approach • Low score one test can compensate for high score on another. • GPA vs GRE • Should make conceptual sense 54
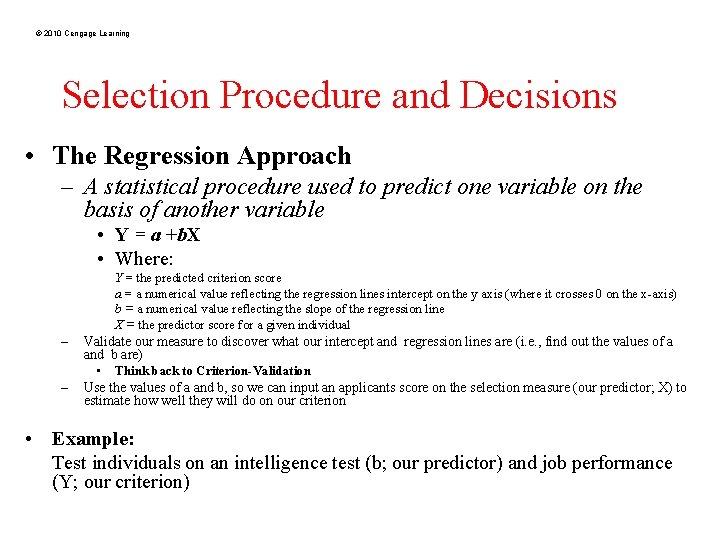
© 2010 Cengage Learning Selection Procedure and Decisions • The Regression Approach – A statistical procedure used to predict one variable on the basis of another variable • Y = a +b. X • Where: Y = the predicted criterion score a = a numerical value reflecting the regression lines intercept on the y axis (where it crosses 0 on the x-axis) b = a numerical value reflecting the slope of the regression line X = the predictor score for a given individual – – Validate our measure to discover what our intercept and regression lines are (i. e. , find out the values of a and b are) • Think back to Criterion-Validation Use the values of a and b, so we can input an applicants score on the selection measure (our predictor; X) to estimate how well they will do on our criterion • Example: Test individuals on an intelligence test (b; our predictor) and job performance (Y; our criterion)
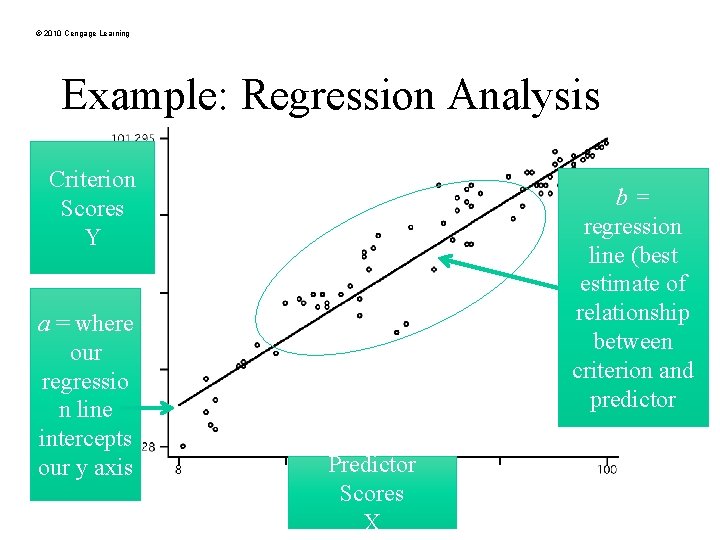
© 2010 Cengage Learning Example: Regression Analysis Criterion Scores Y a = where our regressio n line intercepts our y axis b= regression line (best estimate of relationship between criterion and predictor Predictor Scores X
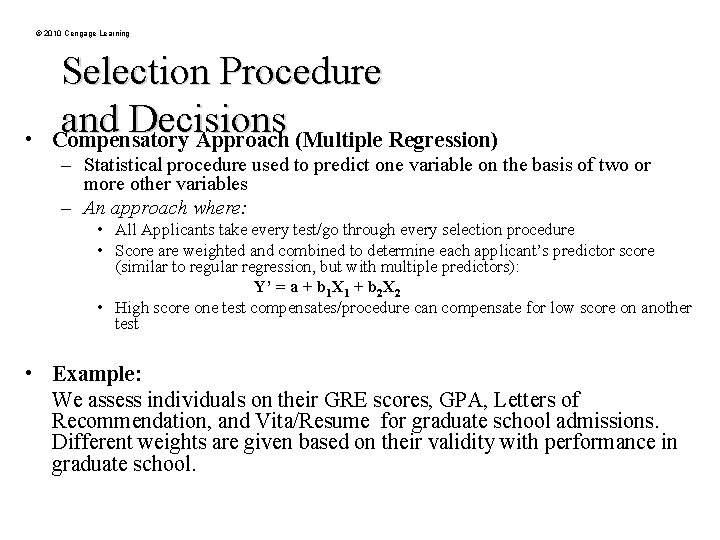
© 2010 Cengage Learning • Selection Procedure and Decisions Compensatory Approach (Multiple Regression) – Statistical procedure used to predict one variable on the basis of two or more other variables – An approach where: • All Applicants take every test/go through every selection procedure • Score are weighted and combined to determine each applicant’s predictor score (similar to regular regression, but with multiple predictors): Y’ = a + b 1 X 1 + b 2 X 2 • High score one test compensates/procedure can compensate for low score on another test • Example: We assess individuals on their GRE scores, GPA, Letters of Recommendation, and Vita/Resume for graduate school admissions. Different weights are given based on their validity with performance in graduate school.
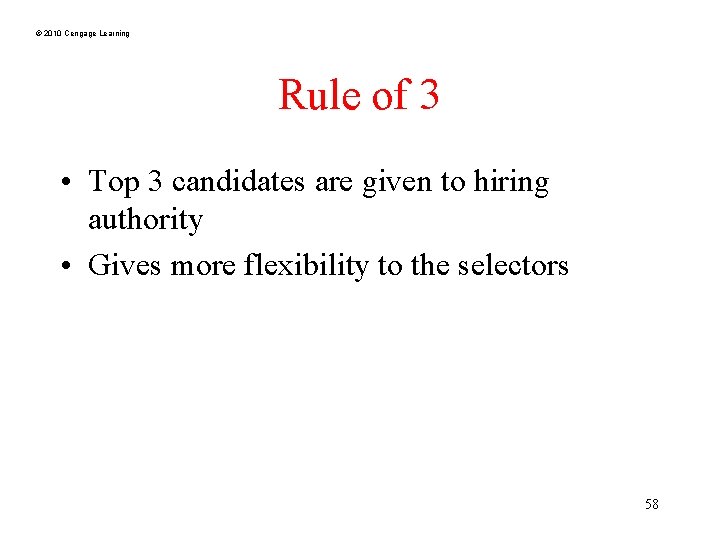
© 2010 Cengage Learning Rule of 3 • Top 3 candidates are given to hiring authority • Gives more flexibility to the selectors 58
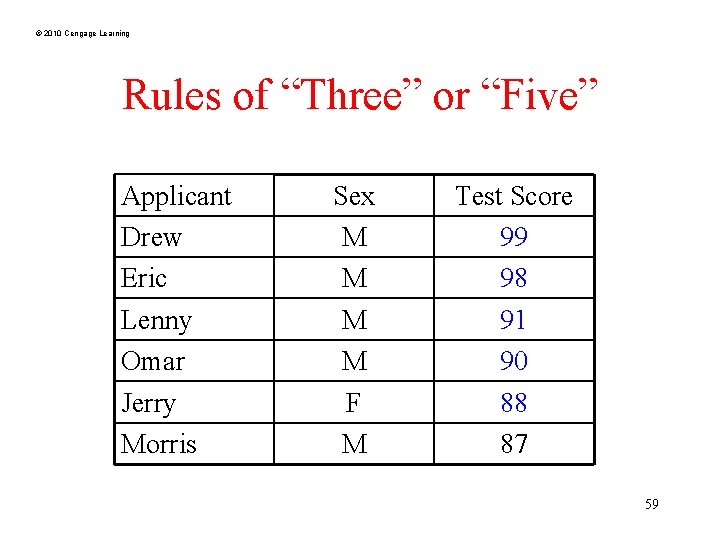
© 2010 Cengage Learning Rules of “Three” or “Five” Applicant Drew Eric Lenny Omar Jerry Morris Sex M M F M Test Score 99 98 91 90 88 87 59
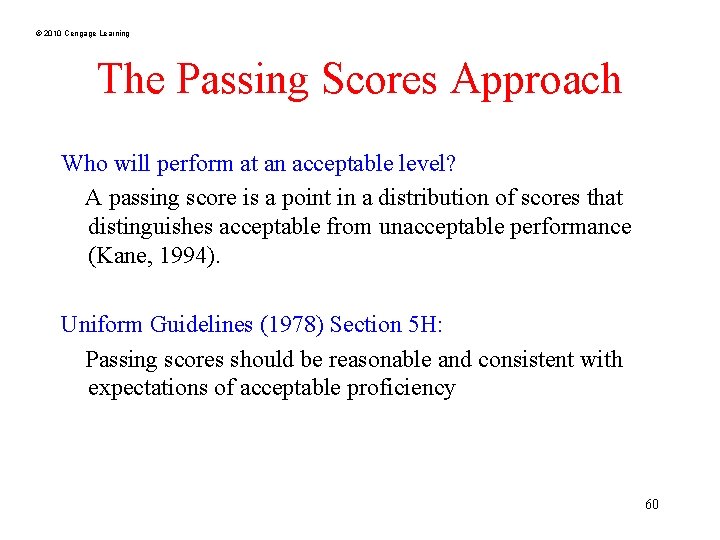
© 2010 Cengage Learning The Passing Scores Approach Who will perform at an acceptable level? A passing score is a point in a distribution of scores that distinguishes acceptable from unacceptable performance (Kane, 1994). Uniform Guidelines (1978) Section 5 H: Passing scores should be reasonable and consistent with expectations of acceptable proficiency 60
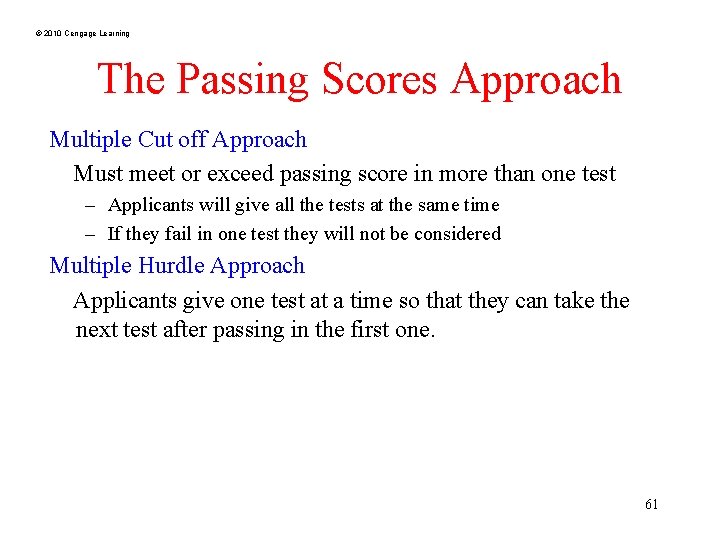
© 2010 Cengage Learning The Passing Scores Approach Multiple Cut off Approach Must meet or exceed passing score in more than one test – Applicants will give all the tests at the same time – If they fail in one test they will not be considered Multiple Hurdle Approach Applicants give one test at a time so that they can take the next test after passing in the first one. 61
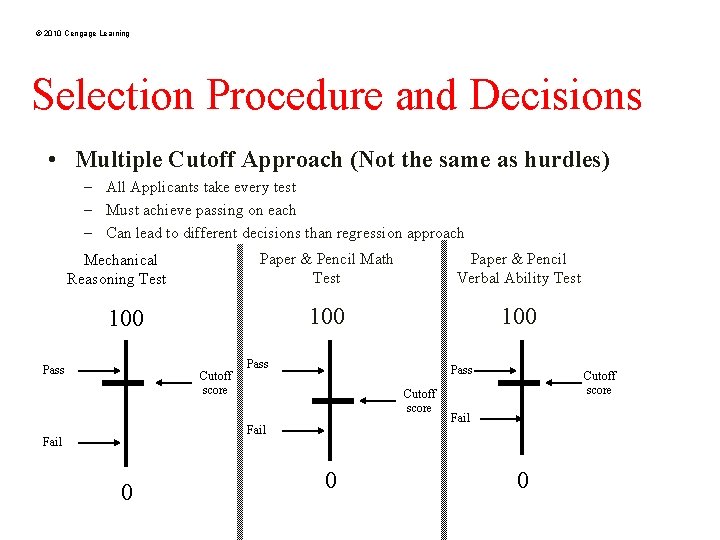
© 2010 Cengage Learning Selection Procedure and Decisions • Multiple Cutoff Approach (Not the same as hurdles) – All Applicants take every test – Must achieve passing on each – Can lead to different decisions than regression approach Mechanical Reasoning Test Paper & Pencil Math Test Paper & Pencil Verbal Ability Test 100 100 Pass Cutoff score Fail 0 0 Cutoff score Fail 0
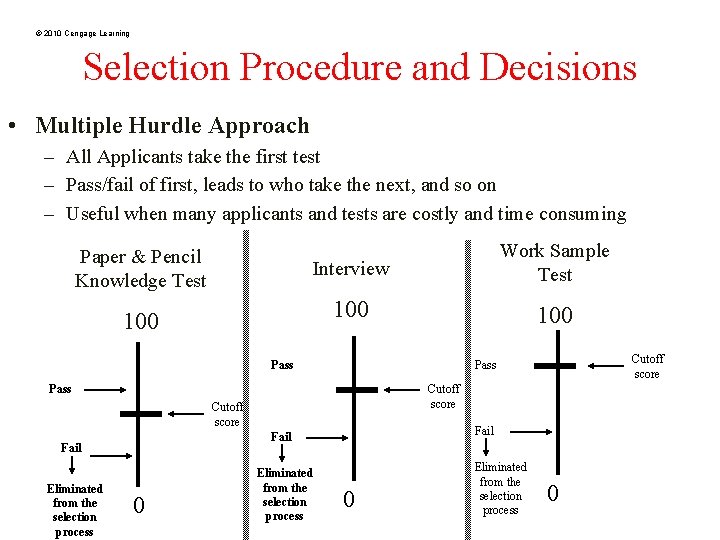
© 2010 Cengage Learning Selection Procedure and Decisions • Multiple Hurdle Approach – All Applicants take the first test – Pass/fail of first, leads to who take the next, and so on – Useful when many applicants and tests are costly and time consuming Paper & Pencil Knowledge Test Interview Work Sample Test 100 100 Pass Cutoff score Eliminated from the selection process Fail 0 Cutoff score Pass Eliminated from the selection process 0
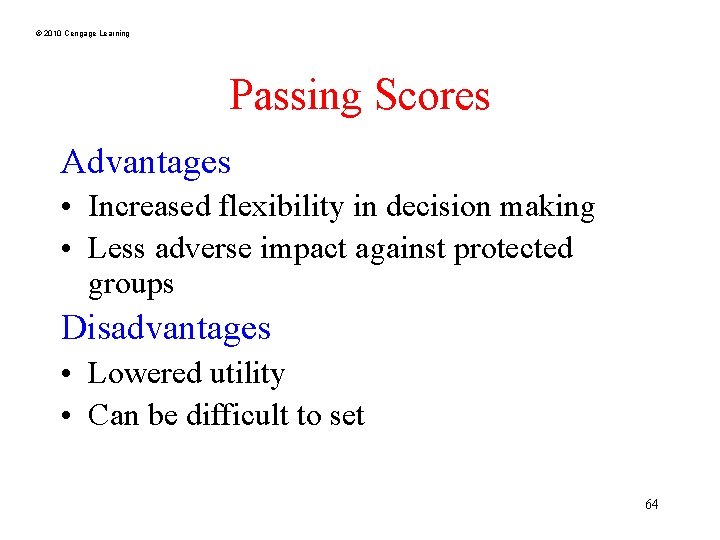
© 2010 Cengage Learning Passing Scores Advantages • Increased flexibility in decision making • Less adverse impact against protected groups Disadvantages • Lowered utility • Can be difficult to set 64
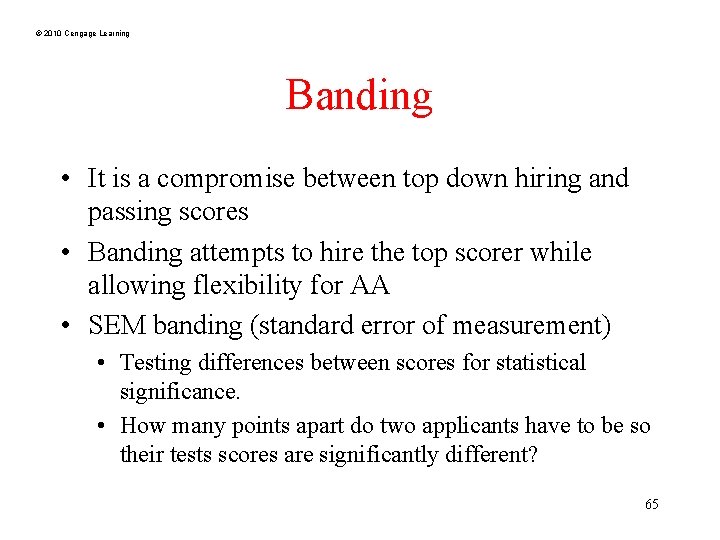
© 2010 Cengage Learning Banding • It is a compromise between top down hiring and passing scores • Banding attempts to hire the top scorer while allowing flexibility for AA • SEM banding (standard error of measurement) • Testing differences between scores for statistical significance. • How many points apart do two applicants have to be so their tests scores are significantly different? 65
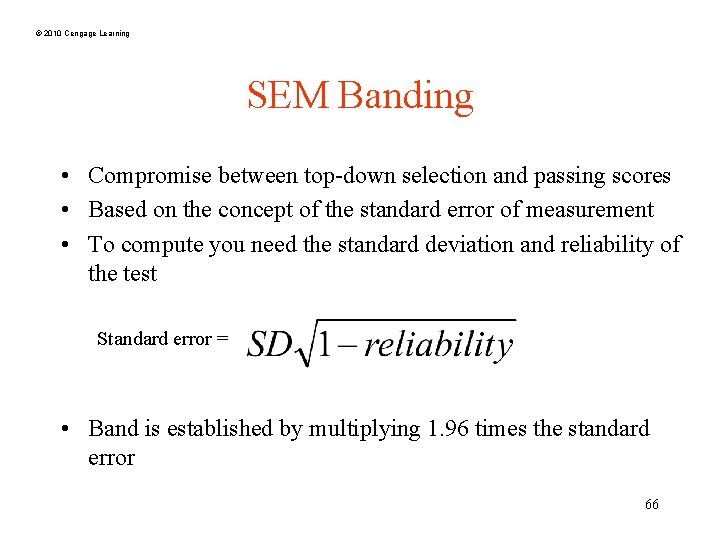
© 2010 Cengage Learning SEM Banding • Compromise between top-down selection and passing scores • Based on the concept of the standard error of measurement • To compute you need the standard deviation and reliability of the test Standard error = • Band is established by multiplying 1. 96 times the standard error 66
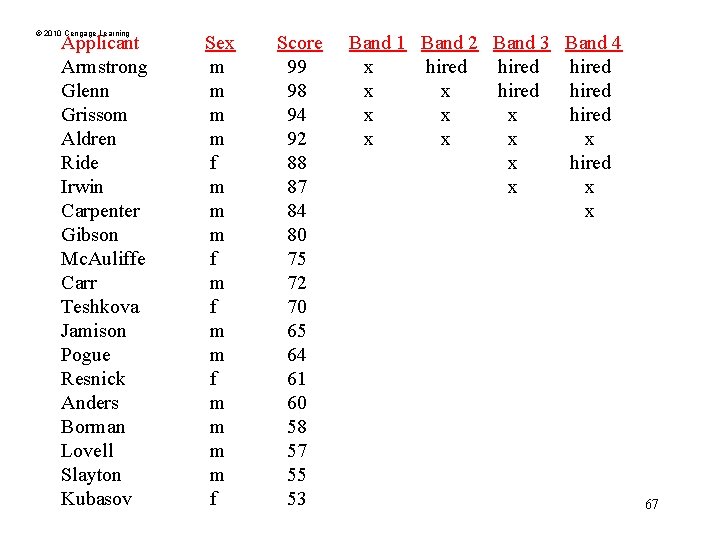
© 2010 Cengage Learning Applicant Armstrong Glenn Grissom Aldren Ride Irwin Carpenter Gibson Mc. Auliffe Carr Teshkova Jamison Pogue Resnick Anders Borman Lovell Slayton Kubasov Sex m m f m m f m m f Score 99 98 94 92 88 87 84 80 75 72 70 65 64 61 60 58 57 55 53 Band 1 Band 2 Band 3 x hired x x x x Band 4 hired x x 67
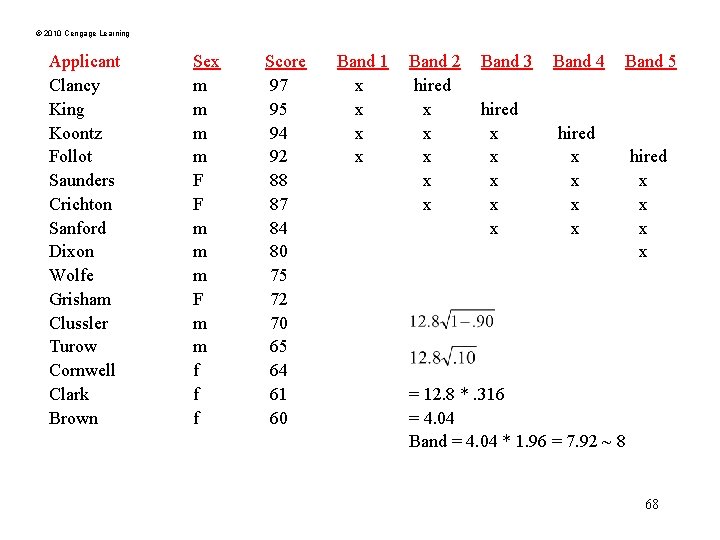
© 2010 Cengage Learning Applicant Clancy King Koontz Follot Saunders Crichton Sanford Dixon Wolfe Grisham Clussler Turow Cornwell Clark Brown Sex m m F F m m m F m m f f f Score 97 95 94 92 88 87 84 80 75 72 70 65 64 61 60 Band 1 x x Band 2 hired x x x Band 3 Band 4 hired x x x hired x x Band 5 hired x x = 12. 8 *. 316 = 4. 04 Band = 4. 04 * 1. 96 = 7. 92 ~ 8 68
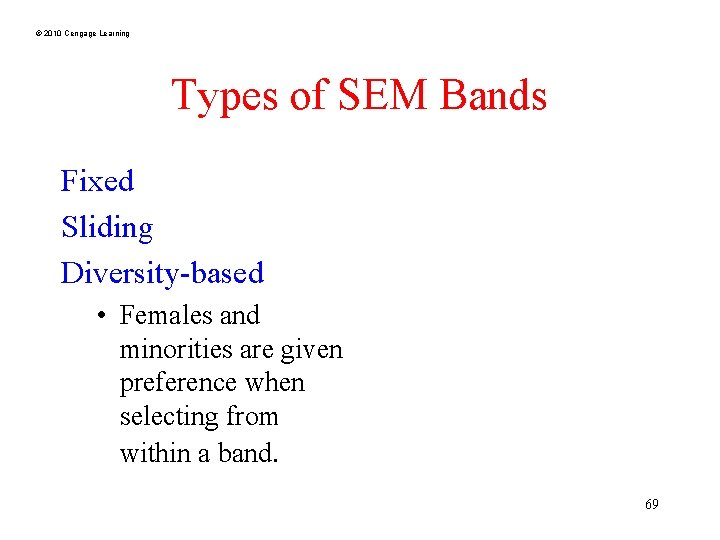
© 2010 Cengage Learning Types of SEM Bands Fixed Sliding Diversity-based • Females and minorities are given preference when selecting from within a band. 69
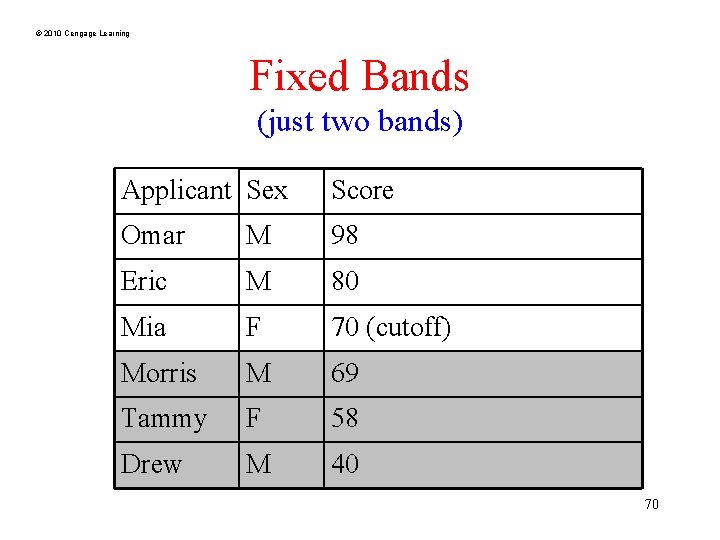
© 2010 Cengage Learning Fixed Bands (just two bands) Applicant Sex Score Omar M 98 Eric M 80 Mia F 70 (cutoff) Morris M 69 Tammy F 58 Drew M 40 70
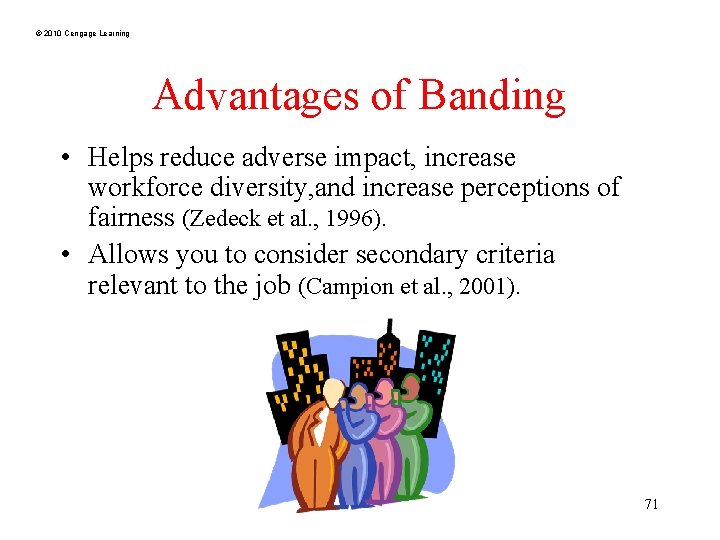
© 2010 Cengage Learning Advantages of Banding • Helps reduce adverse impact, increase workforce diversity, and increase perceptions of fairness (Zedeck et al. , 1996). • Allows you to consider secondary criteria relevant to the job (Campion et al. , 2001). 71
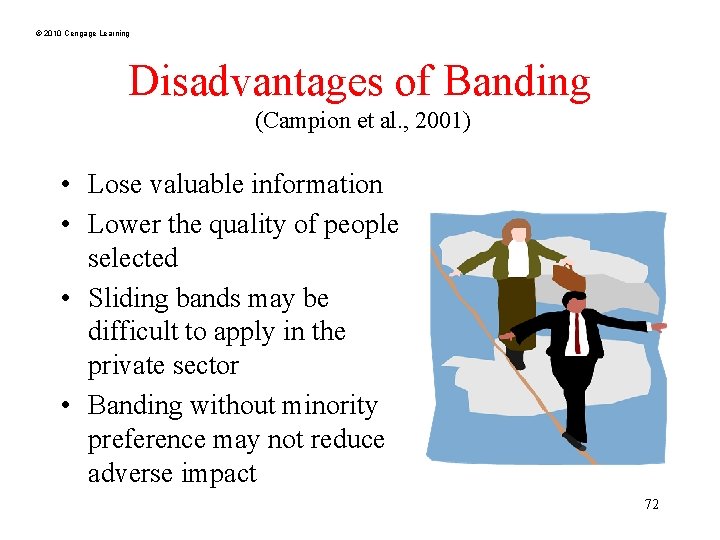
© 2010 Cengage Learning Disadvantages of Banding (Campion et al. , 2001) • Lose valuable information • Lower the quality of people selected • Sliding bands may be difficult to apply in the private sector • Banding without minority preference may not reduce adverse impact 72
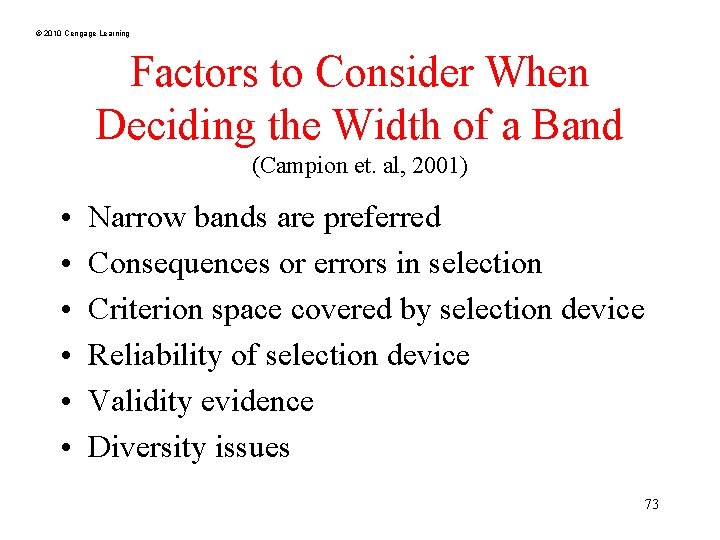
© 2010 Cengage Learning Factors to Consider When Deciding the Width of a Band (Campion et. al, 2001) • • • Narrow bands are preferred Consequences or errors in selection Criterion space covered by selection device Reliability of selection device Validity evidence Diversity issues 73
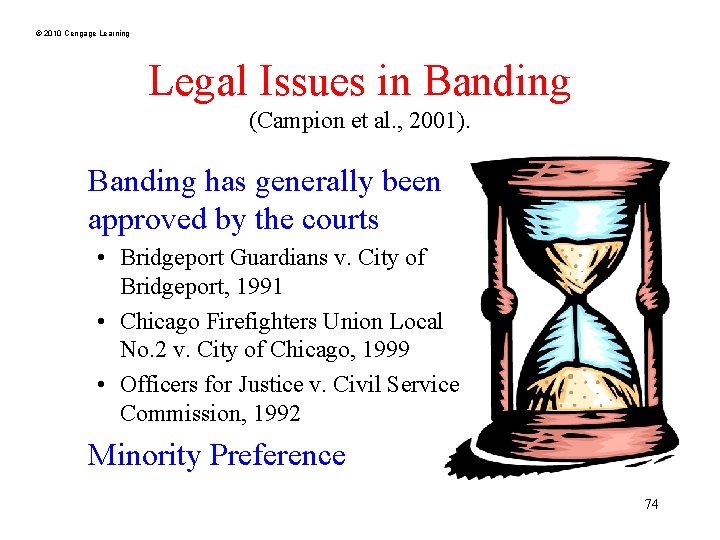
© 2010 Cengage Learning Legal Issues in Banding (Campion et al. , 2001). Banding has generally been approved by the courts • Bridgeport Guardians v. City of Bridgeport, 1991 • Chicago Firefighters Union Local No. 2 v. City of Chicago, 1999 • Officers for Justice v. Civil Service Commission, 1992 Minority Preference 74
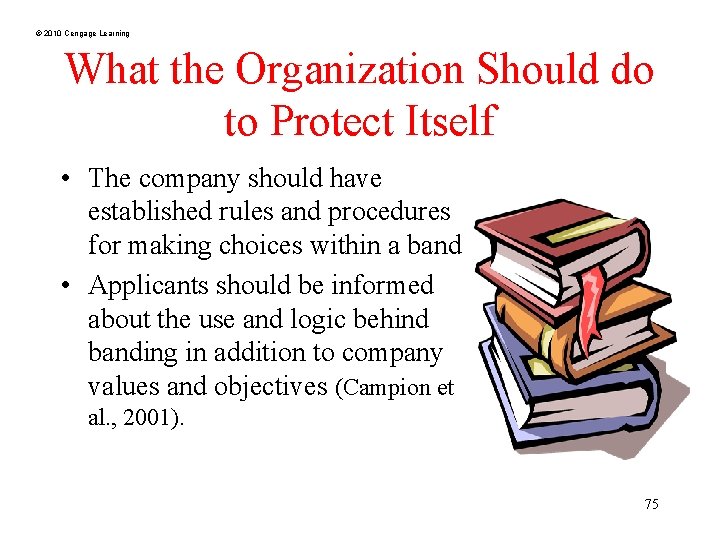
© 2010 Cengage Learning What the Organization Should do to Protect Itself • The company should have established rules and procedures for making choices within a band • Applicants should be informed about the use and logic behind banding in addition to company values and objectives (Campion et al. , 2001). 75
Characteristics of effective selection techniques
Unadjusted top down selection
2010 cengage learning
Bristol early years
Delmar cengage learning medical terminology
Cengage learning heart diagram
Cengage learning heart diagram
South-western cengage learning
Chapter 13 medical math
2009 delmar cengage learning
Cengage learning heart diagram
Delmar learning medical abbreviations
Cengage learning australia
2009 delmar cengage learning
Cengage learning
Cengage learning
Wadsworth cengage learning
Cengage learning
Cengage learning plant cell
Cengage learning
Cengage learning
Brooks cole cengage learning
Cengage learning chapter 7 answers
2014 cengage learning accounting answers
Cengage chapter 7
Cengage learning
Cengage learning
2009 delmar cengage learning
Cengage learning
Chapter 10 cultural diversity
Marketing implications
2012 cengage learning
Training evaluation
Cengage learning psychology
Course technology cengage learning
Course technology cengage learning
Course technology cengage learning
Course technology cengage learning
2016 cengage learning
Delmar cengage learning instructor resources
2014 cengage learning
Cuadro comparativo entre e-learning b-learning y m-learning
Balancing selection vs stabilizing selection
Similarities
K selected
Natural selection vs artificial selection
Artificial selection vs natural selection
Directional selection example
Logistic model of population growth
Natural selection vs artificial selection
Two way selection and multiway selection
Multiway selection
Mass selection and pure line selection
Effective memorization techniques
Effective oral presentation techniques
Effective management techniques
Evangelist morgan dead
Entertainment speech
Effective oral presentation techniques
Effective oral presentation techniques
Effective oral presentation techniques
Evaluating selection techniques and decisions
Classic trio' of selection techniques
Fonctions techniques et solutions techniques
Ros smith
Summarizing is?
What is effective citizenship
Characteristics of effective helpers
Characteristics of a good interviewer
Features of an effective law
Features of effective law
Characteristics of effective informative speaking
Characteristics of effective classroom rules
Characteristics of effective feedback
Characteristics of effective teams
Characteristics of effective feedback