Scheduling Grids and Uncertainty Theory and Practice Grzegorz
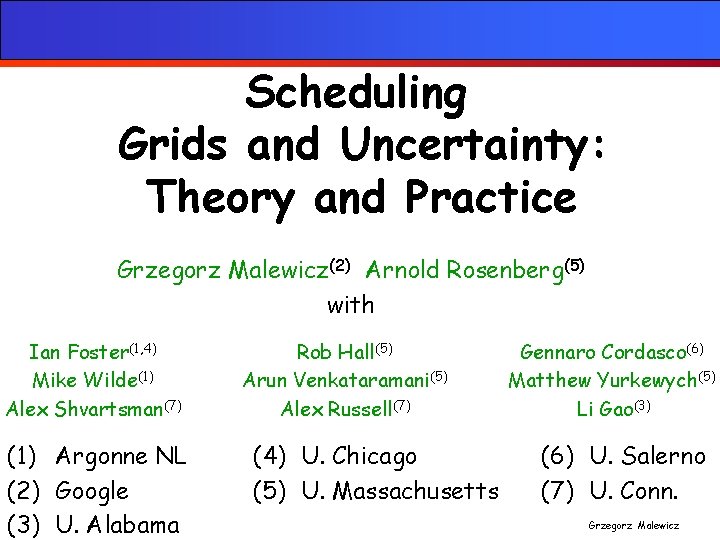
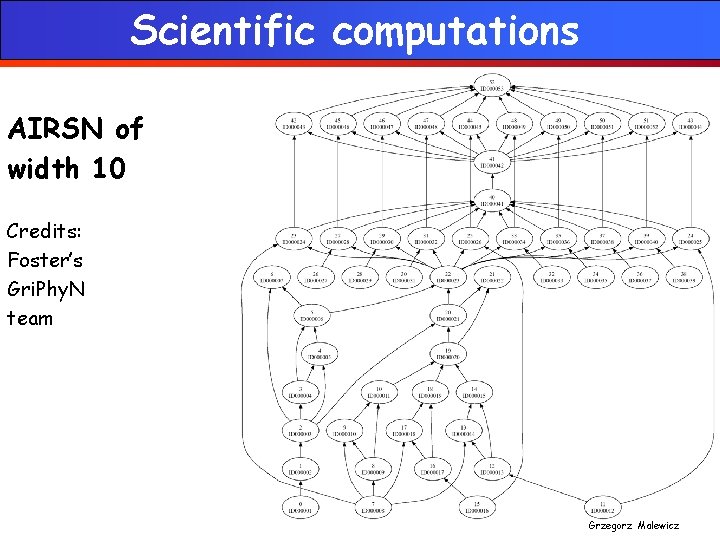
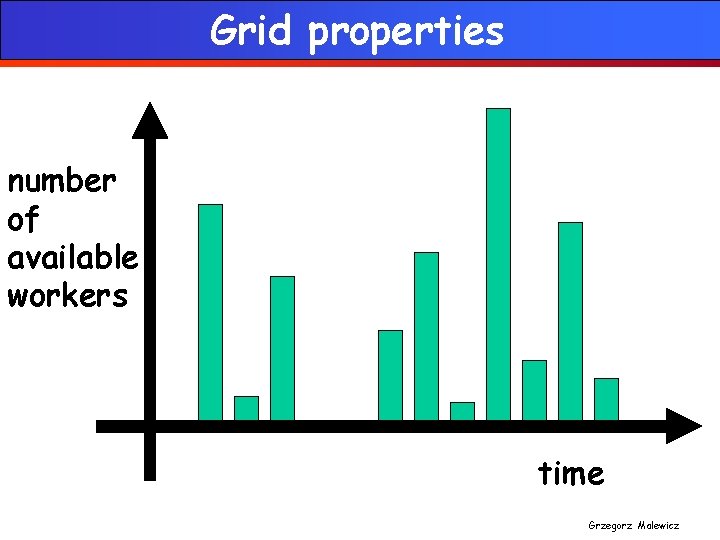
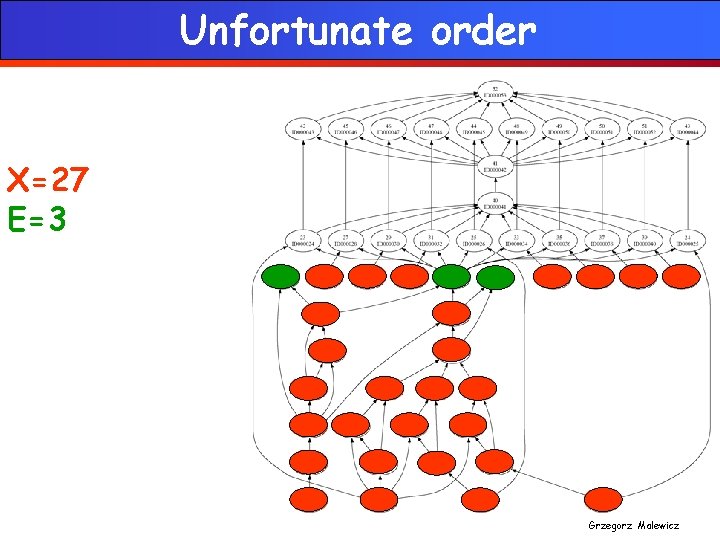
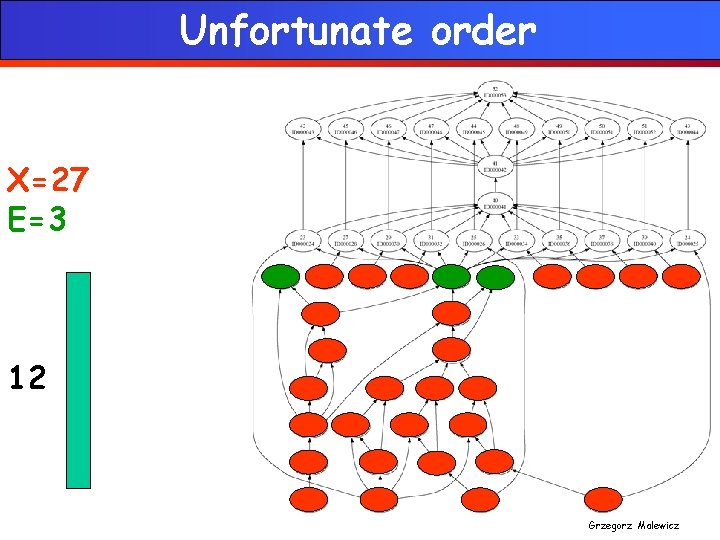
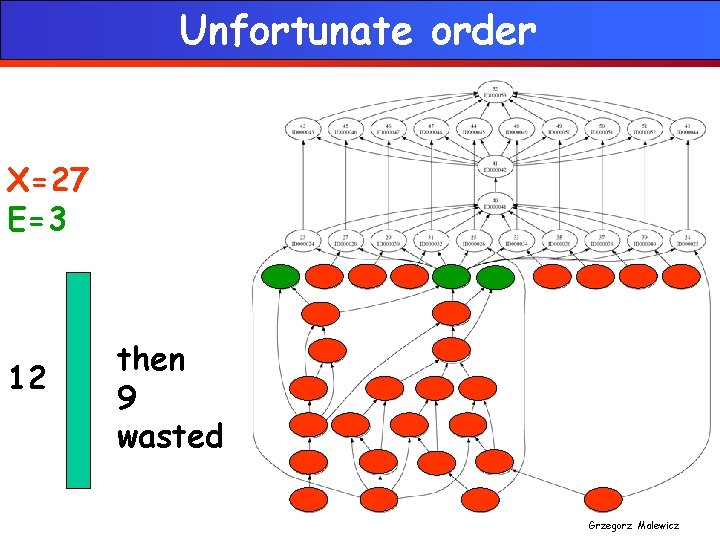
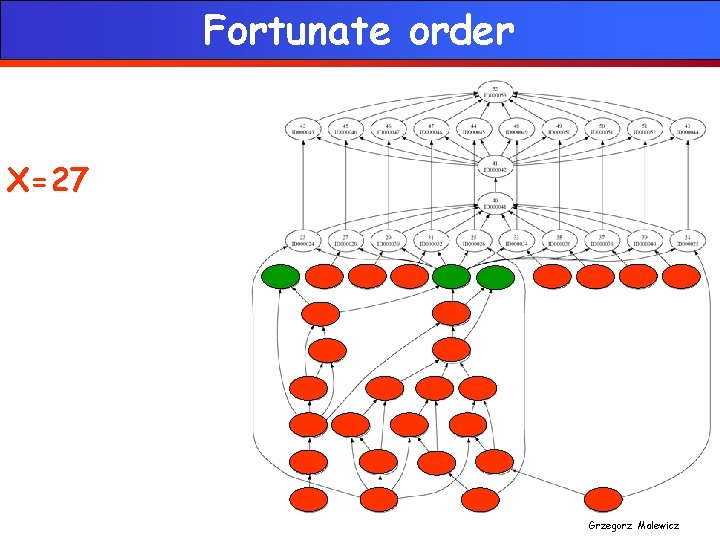
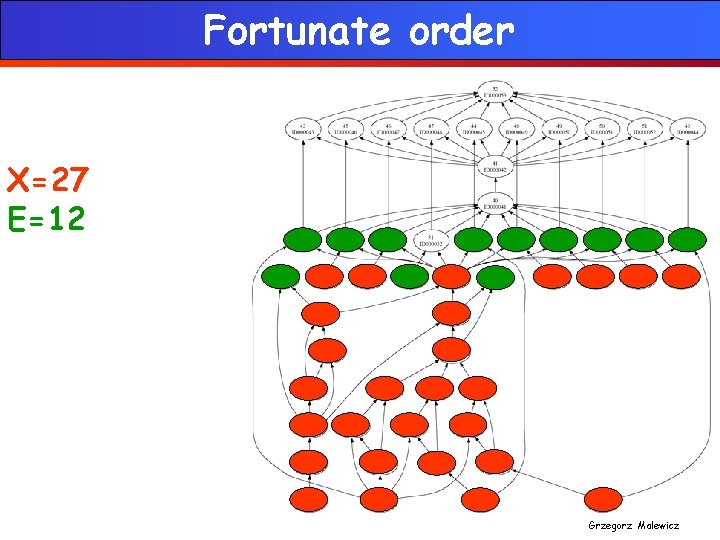
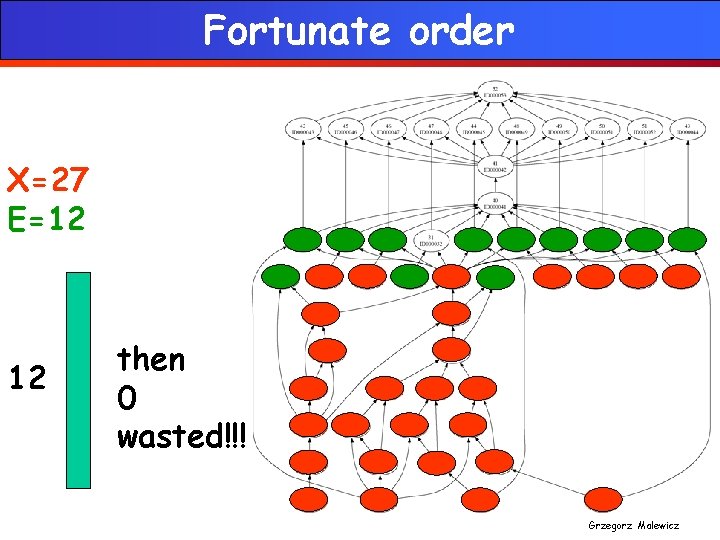
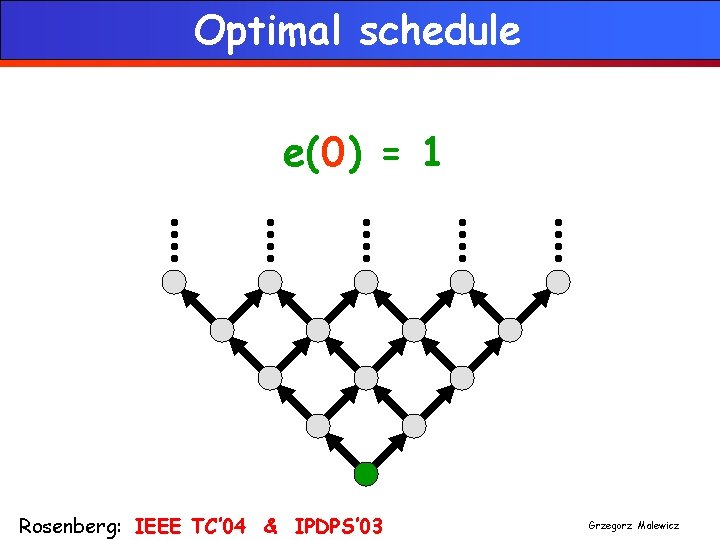
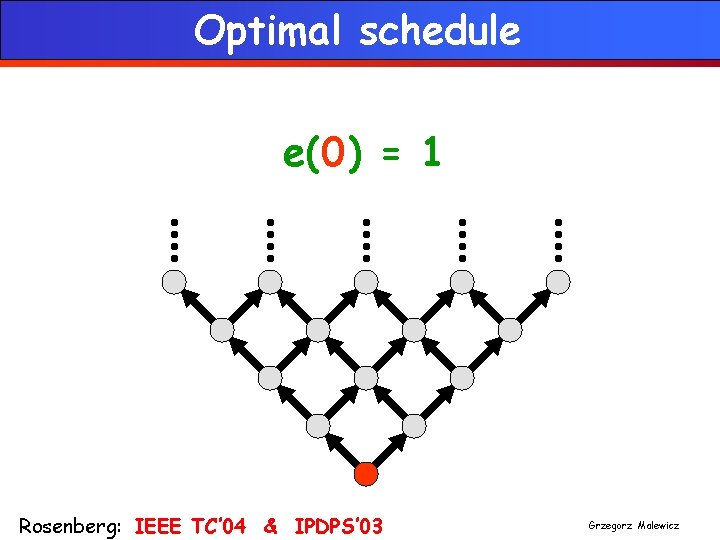
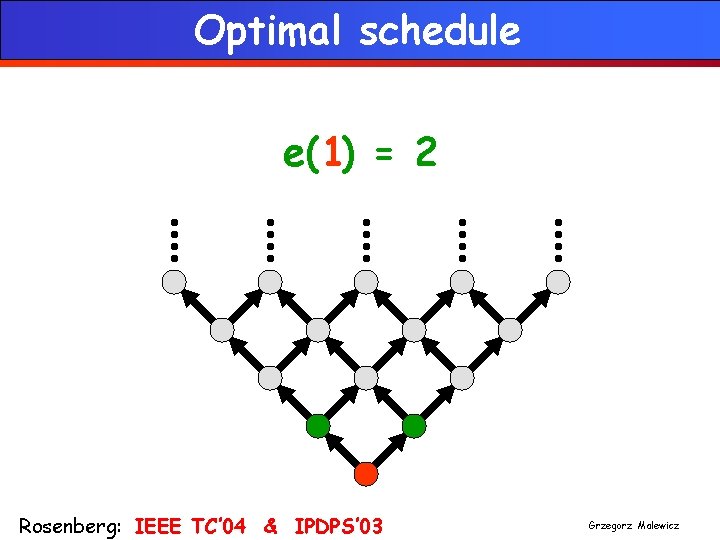
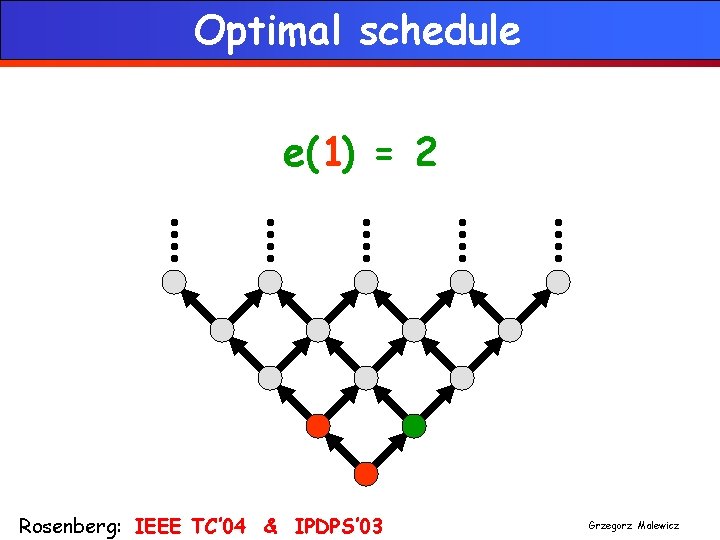
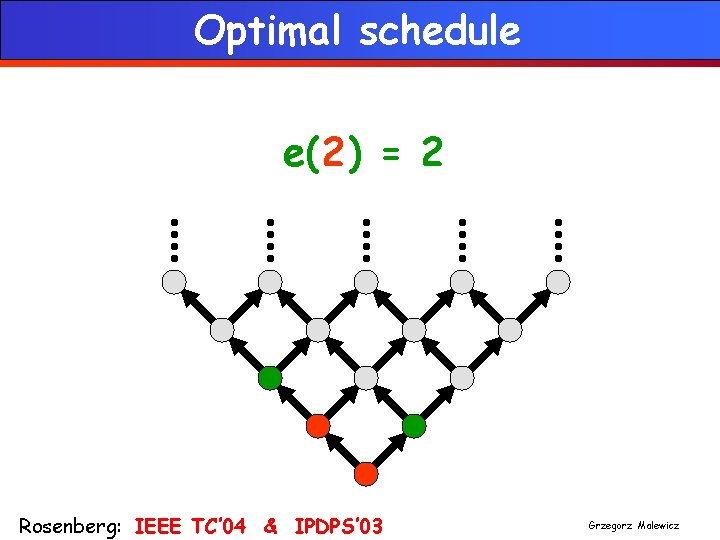
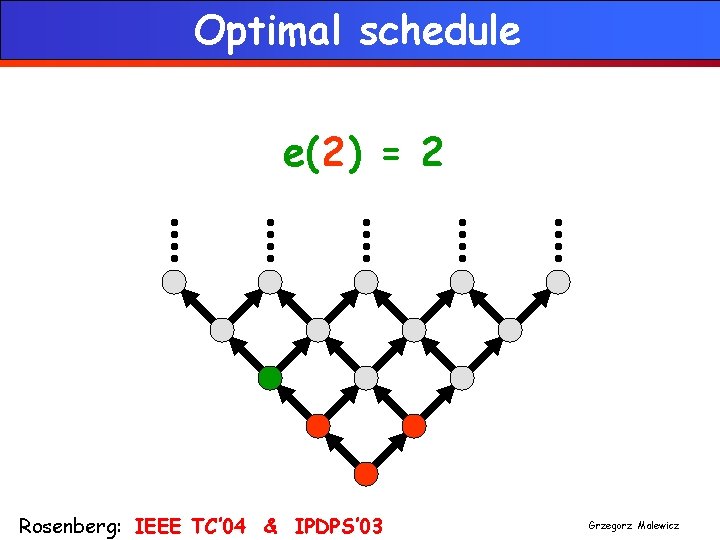
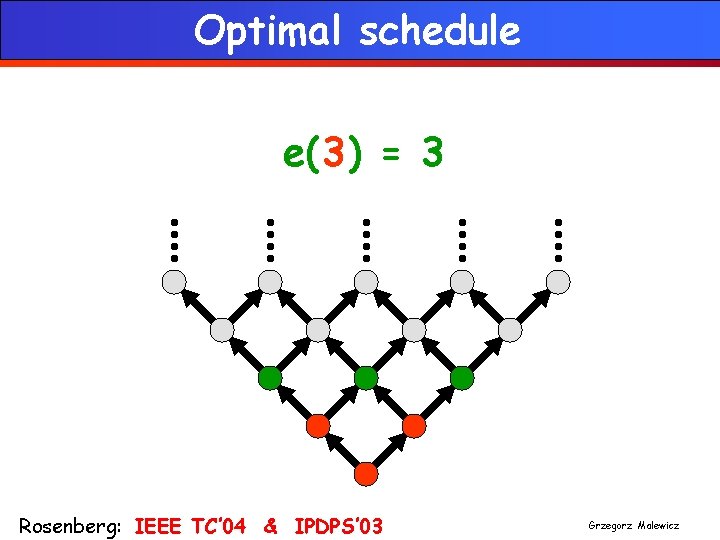
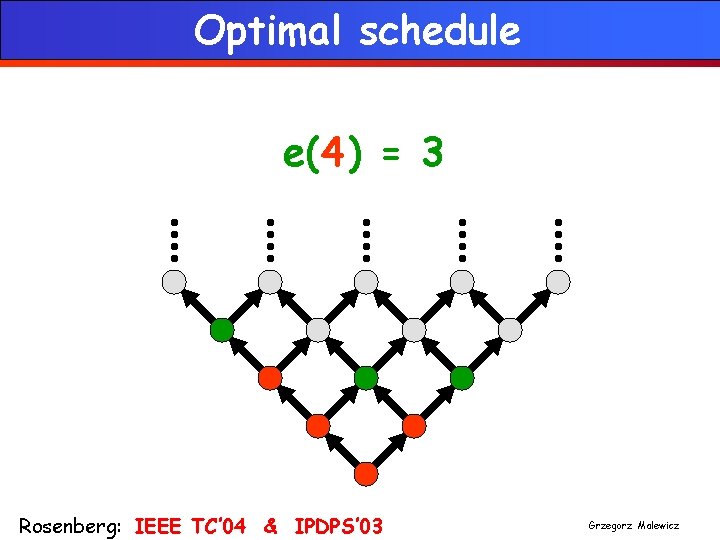
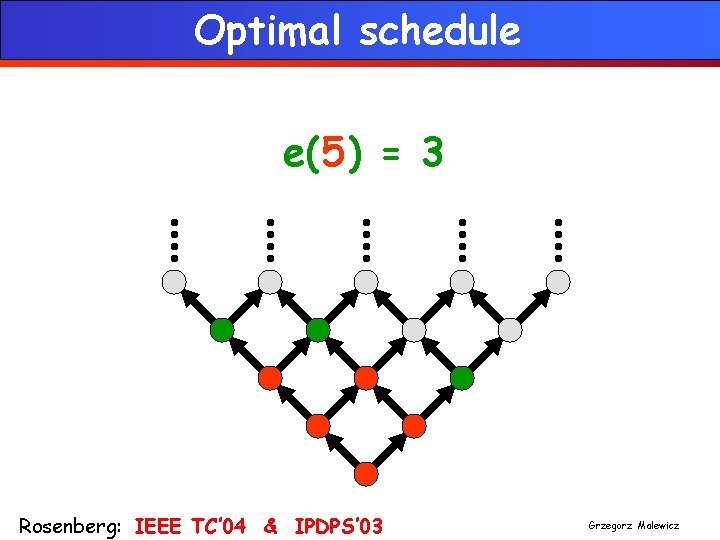
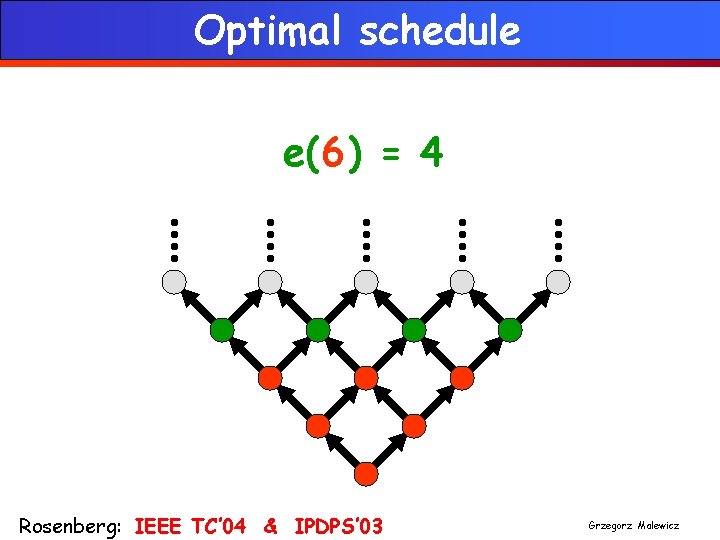
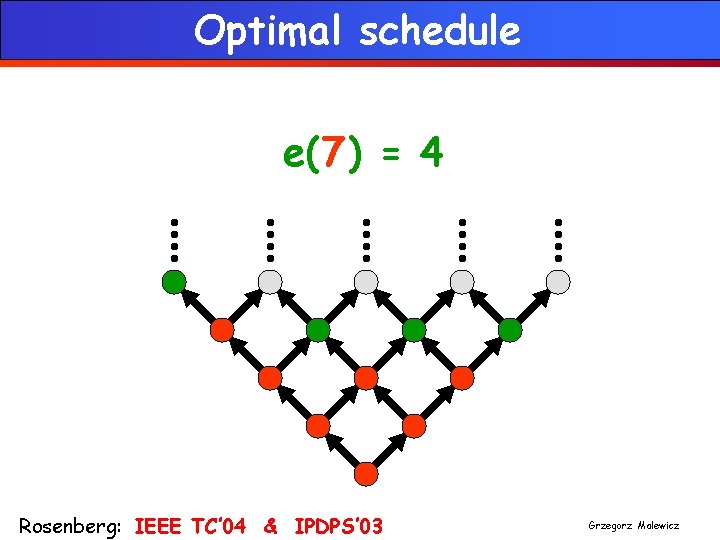
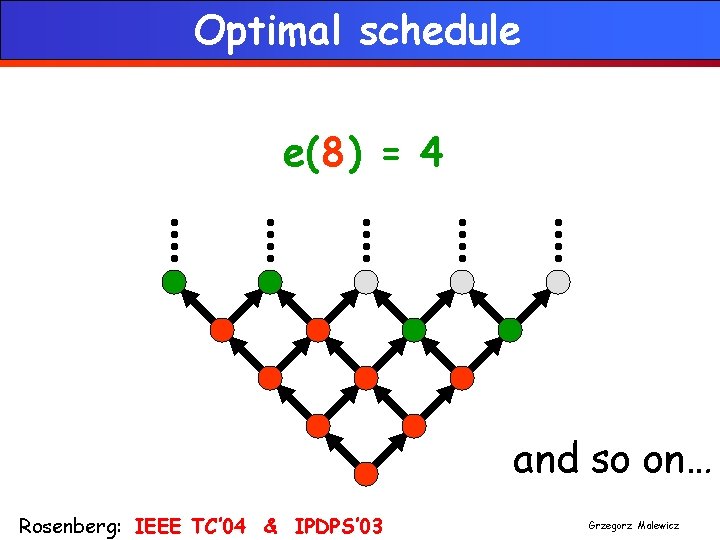
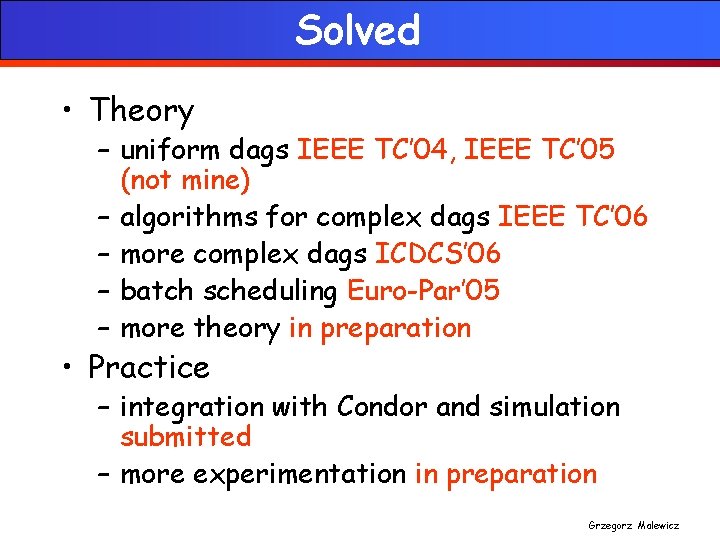
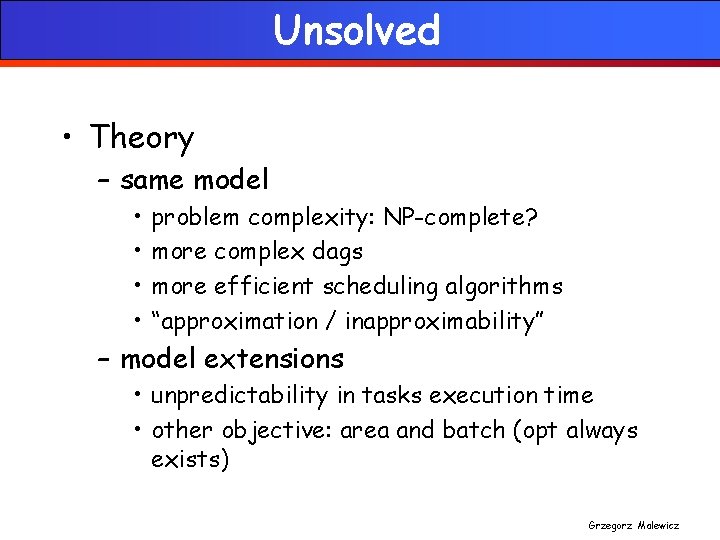
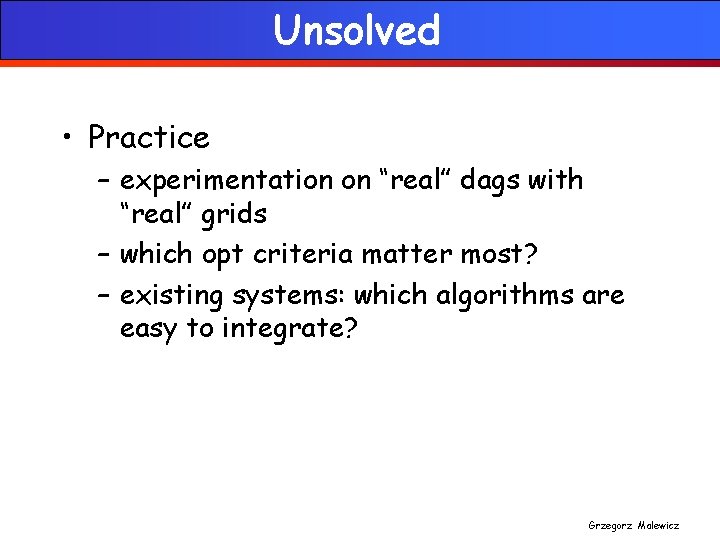
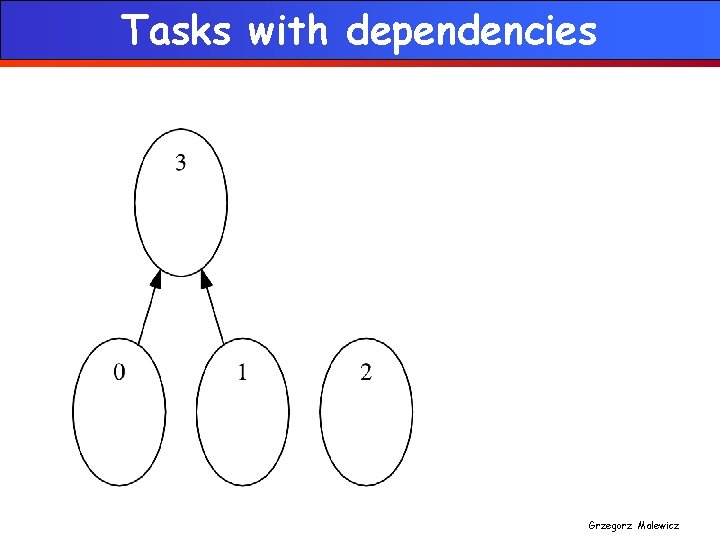
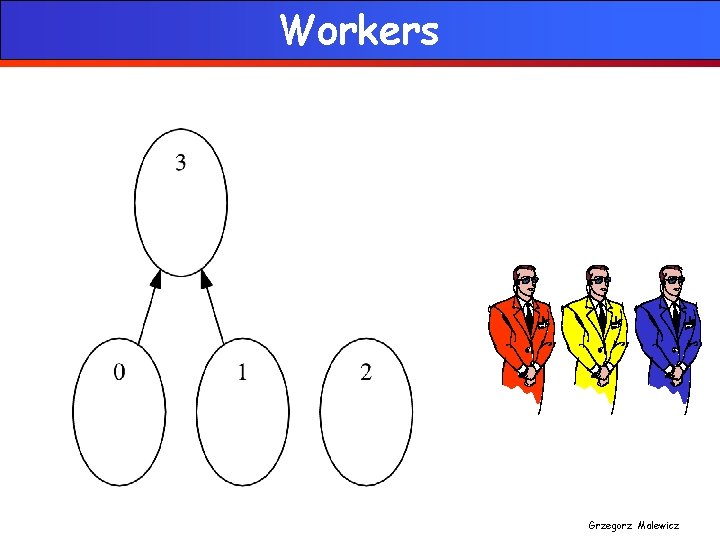
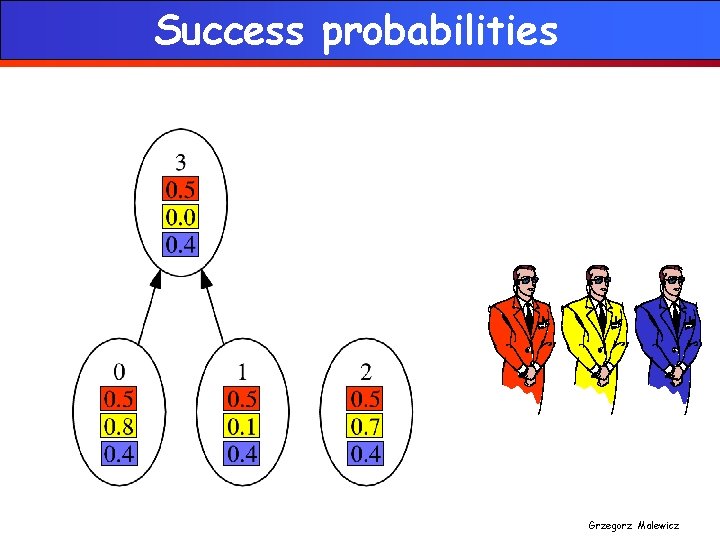
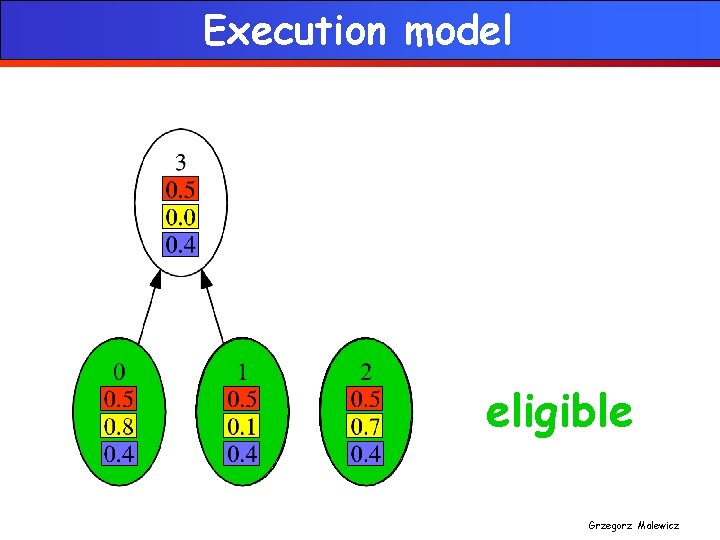
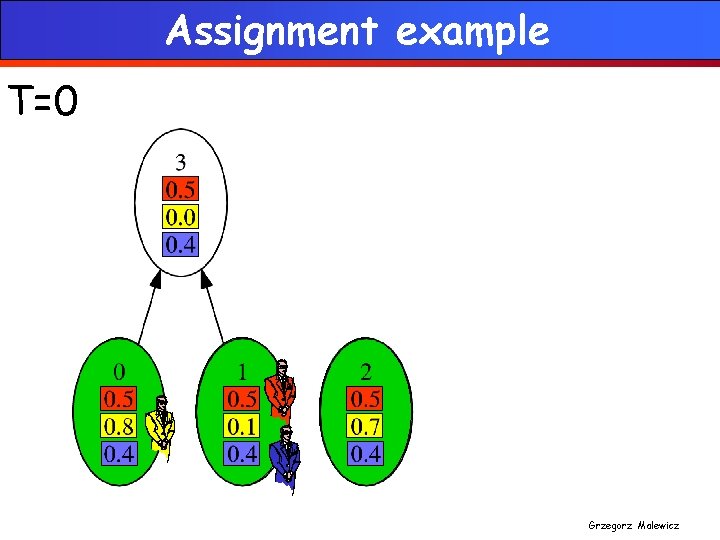
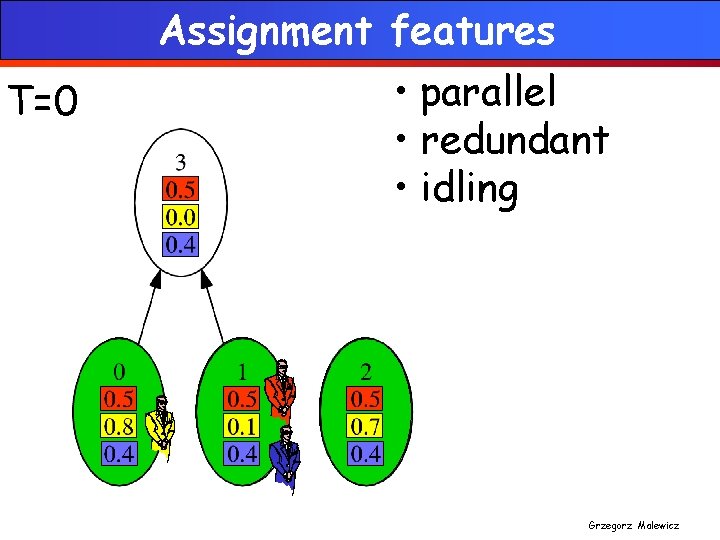
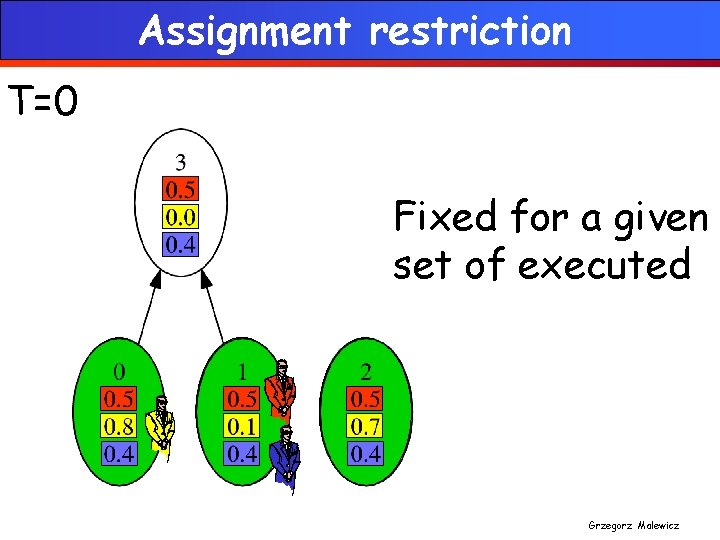
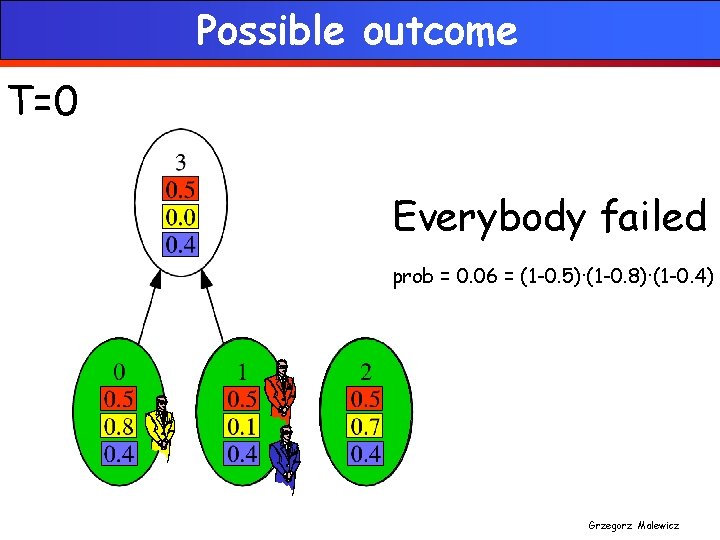
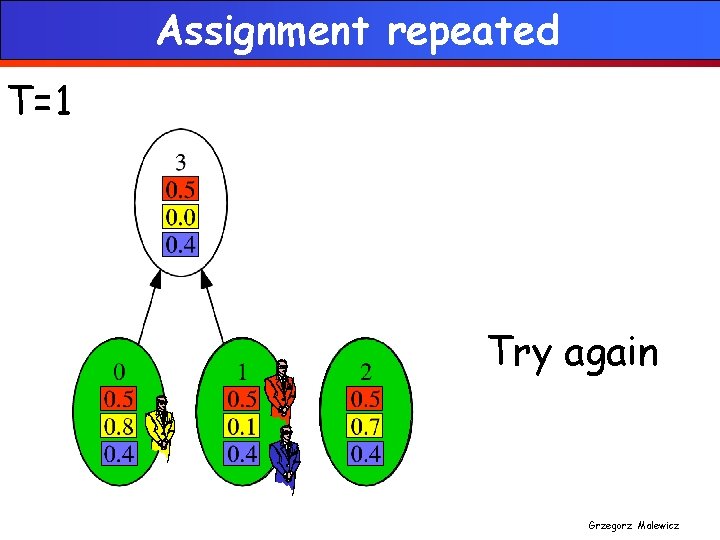
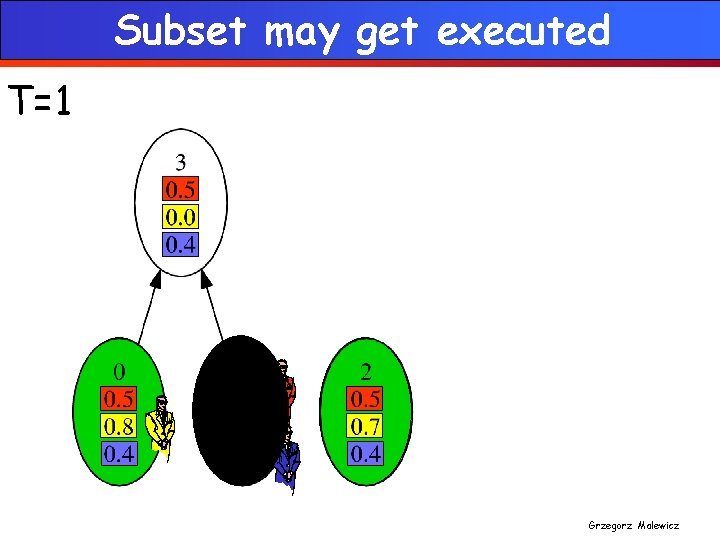
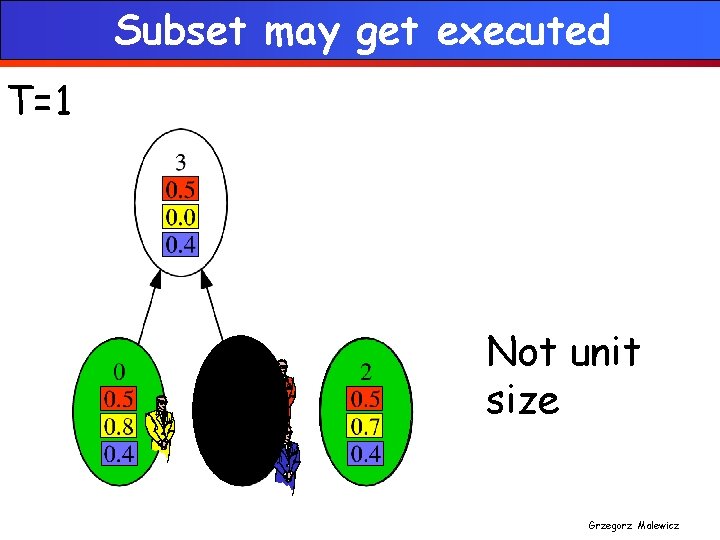
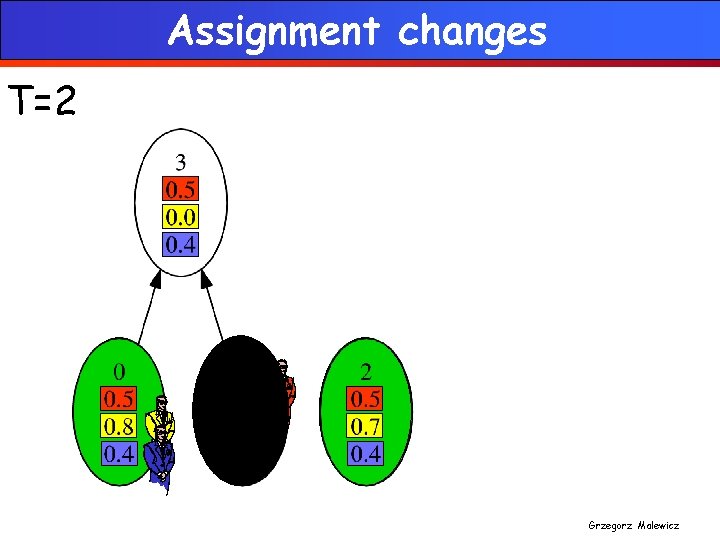
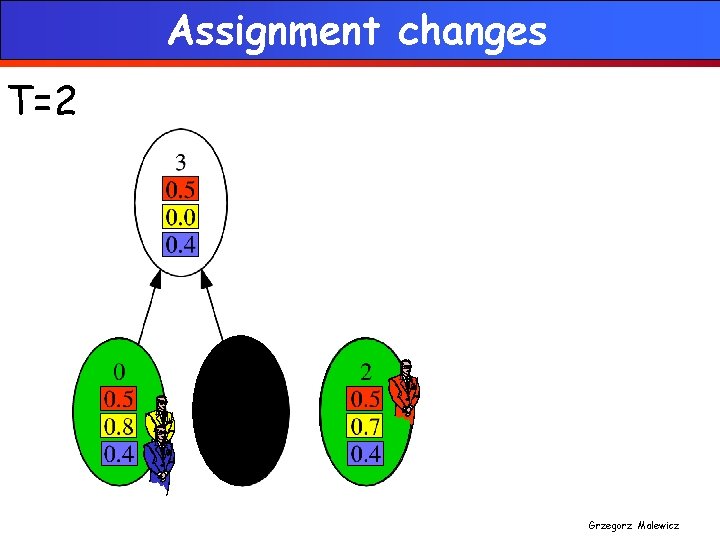
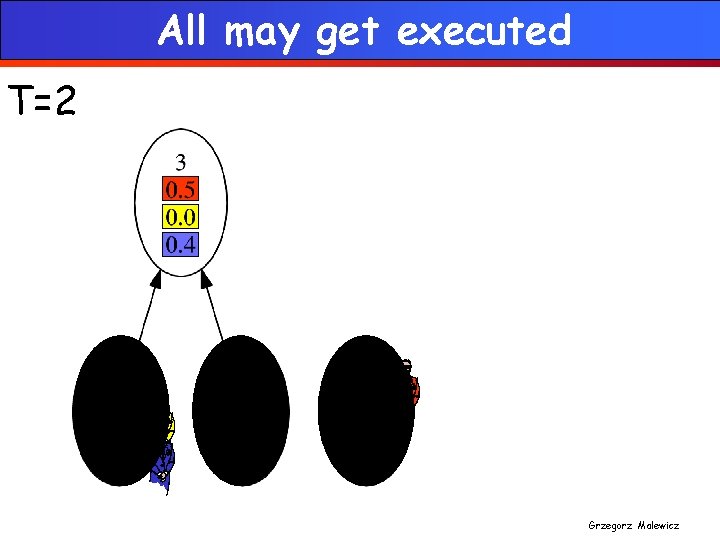
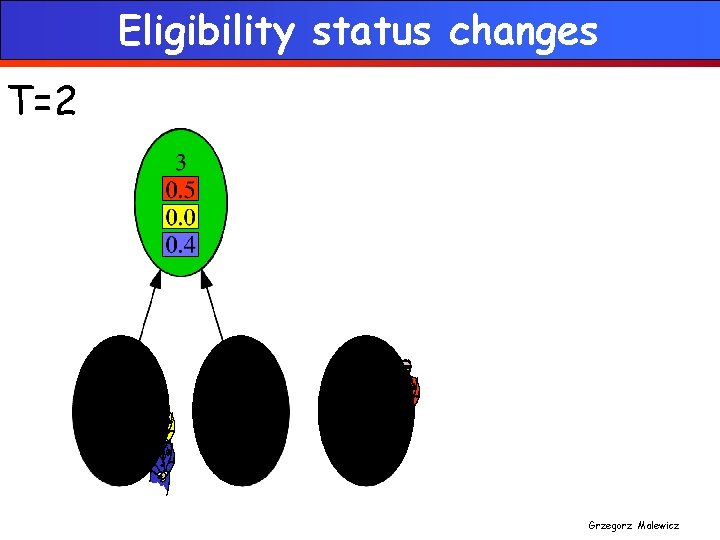
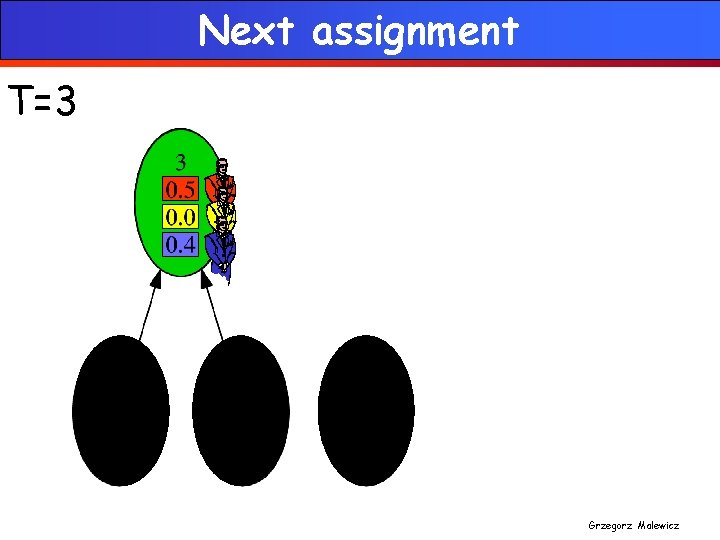
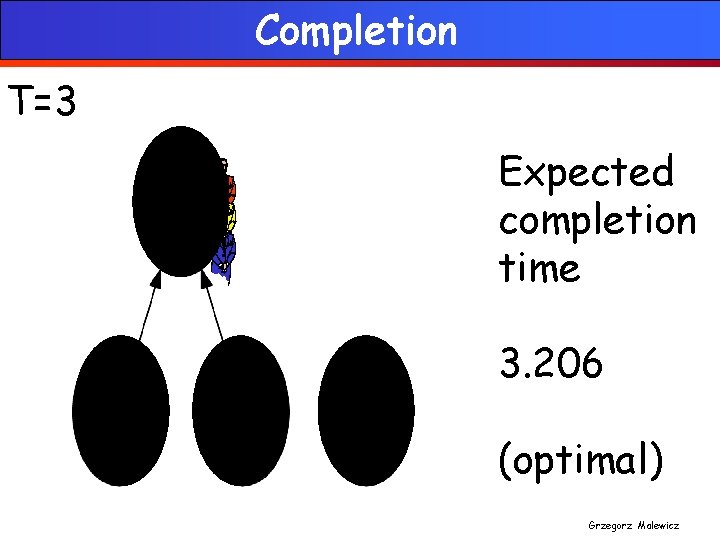
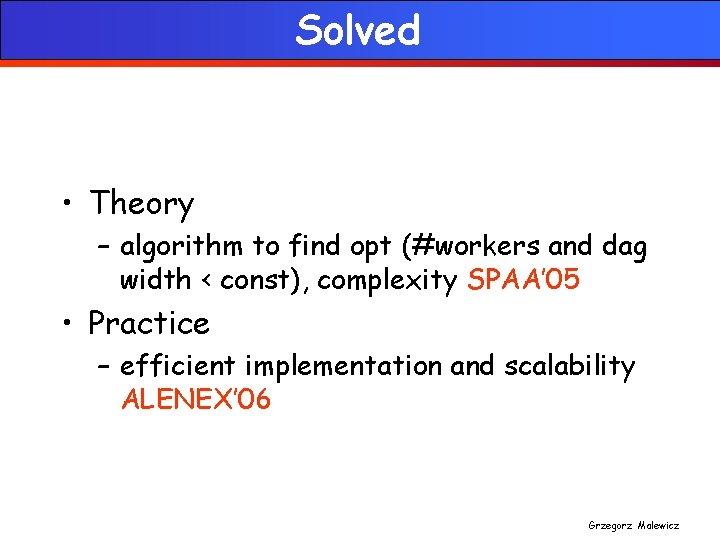
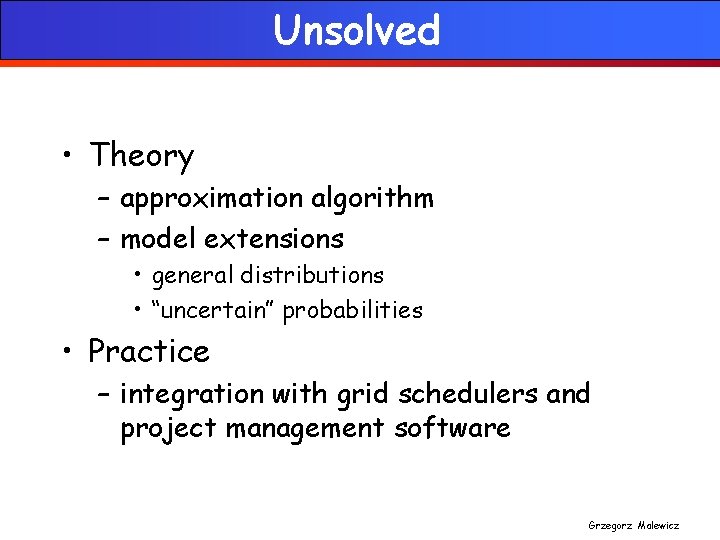
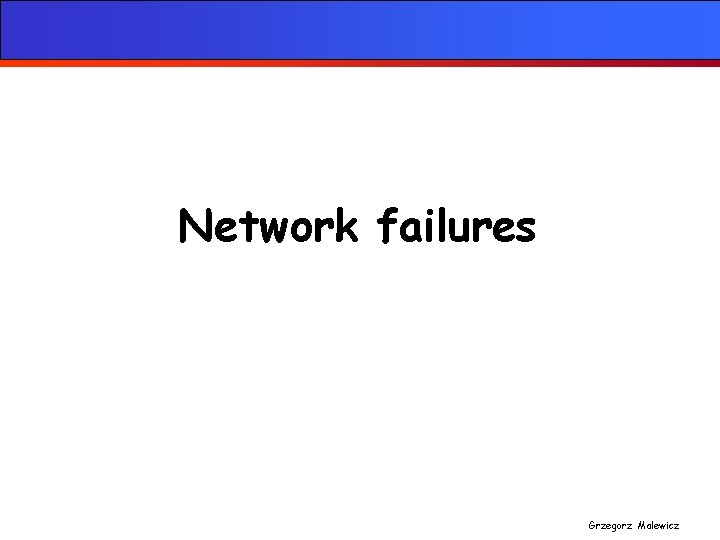
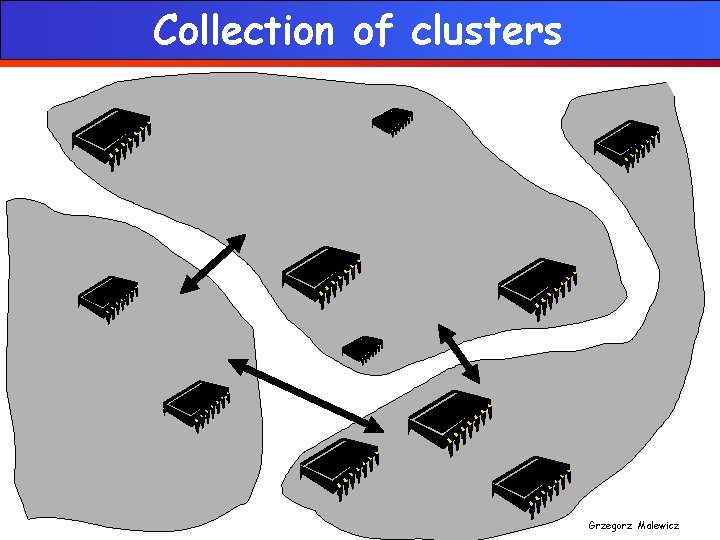
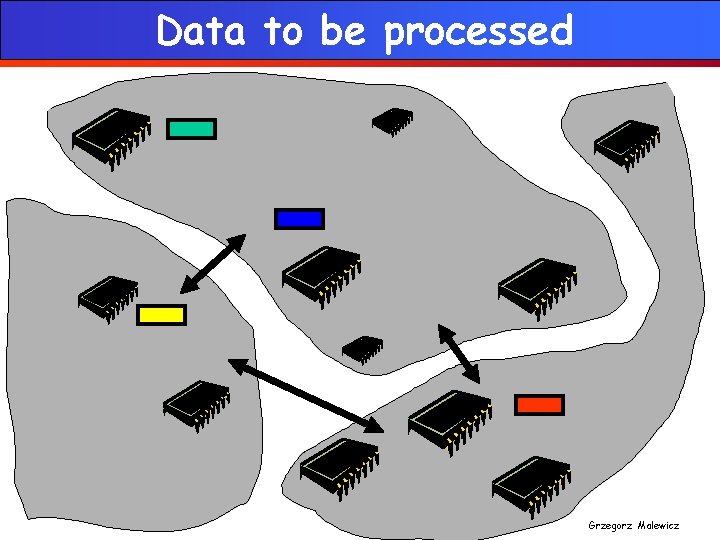
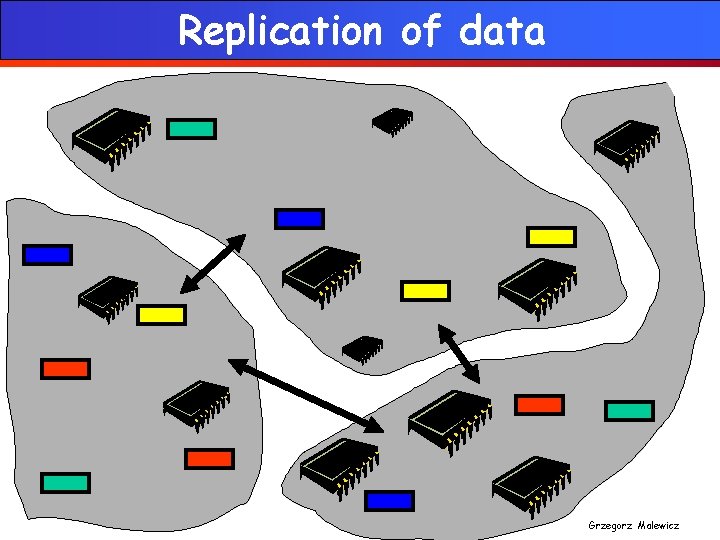
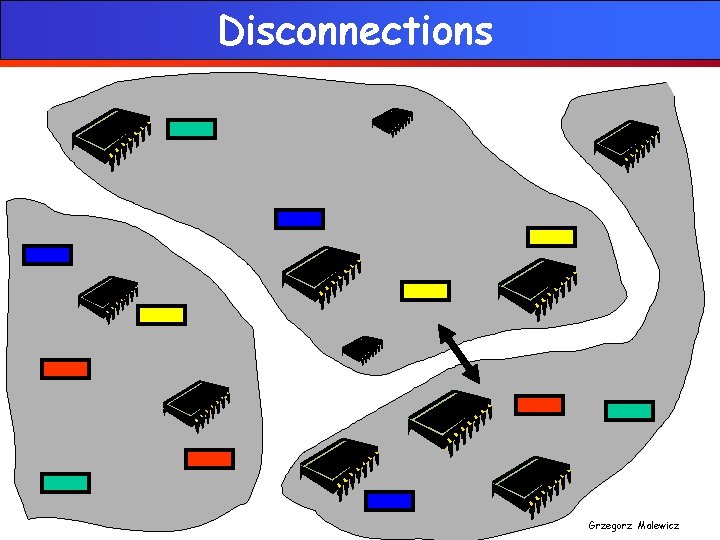
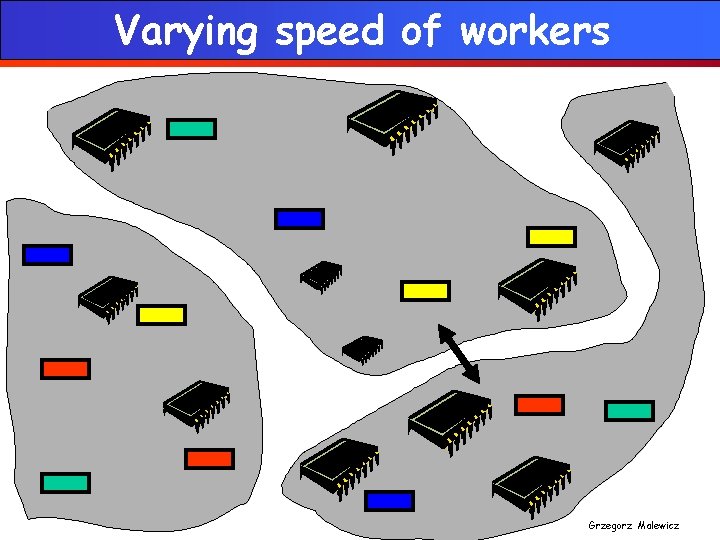
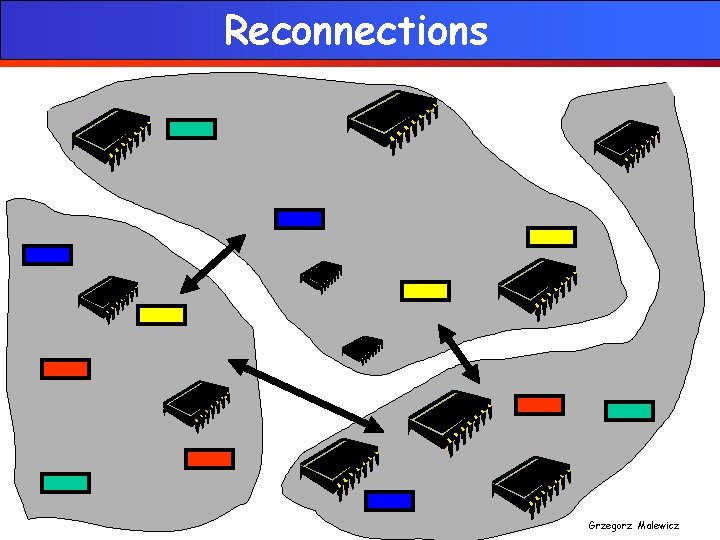
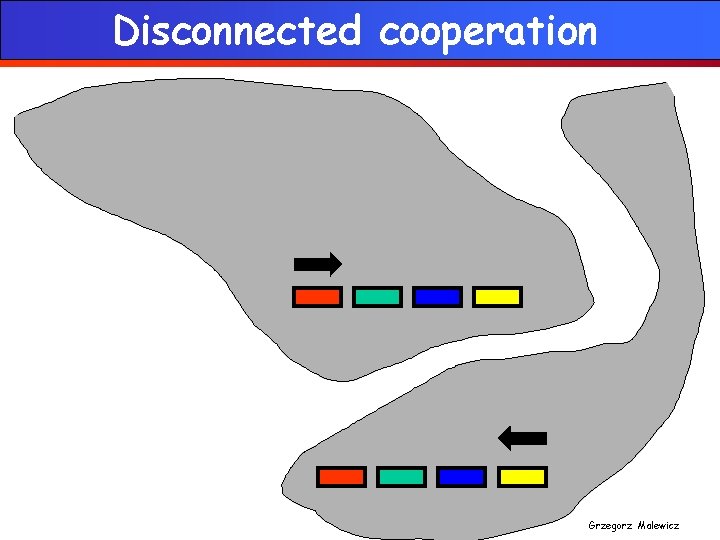
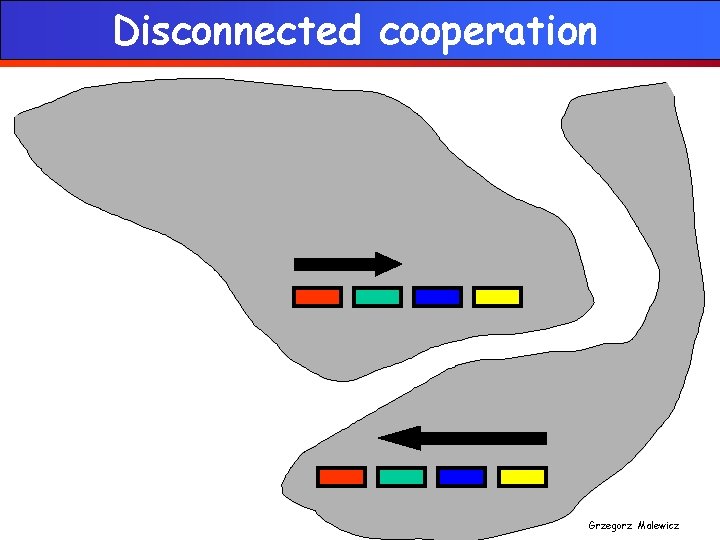
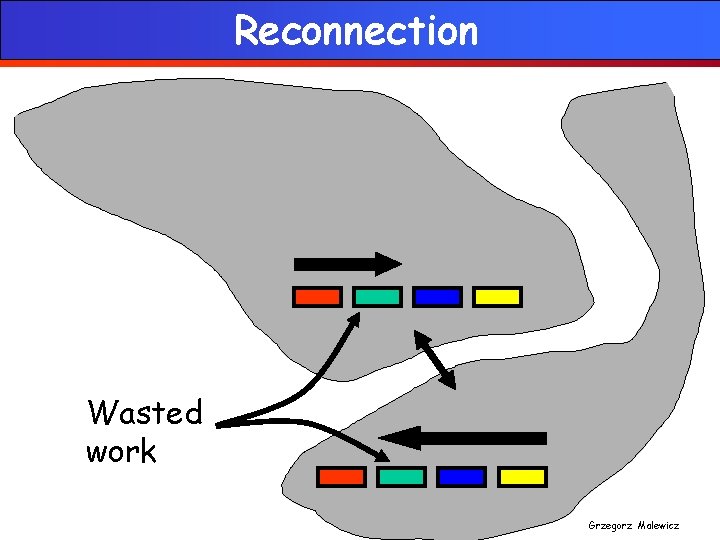
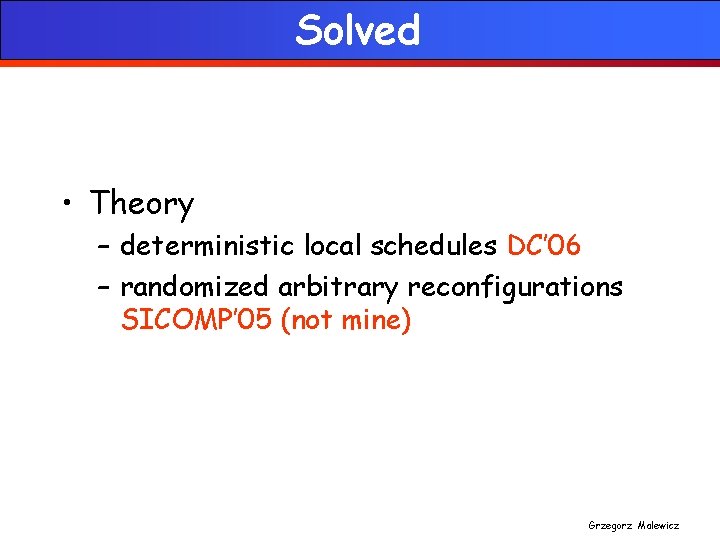
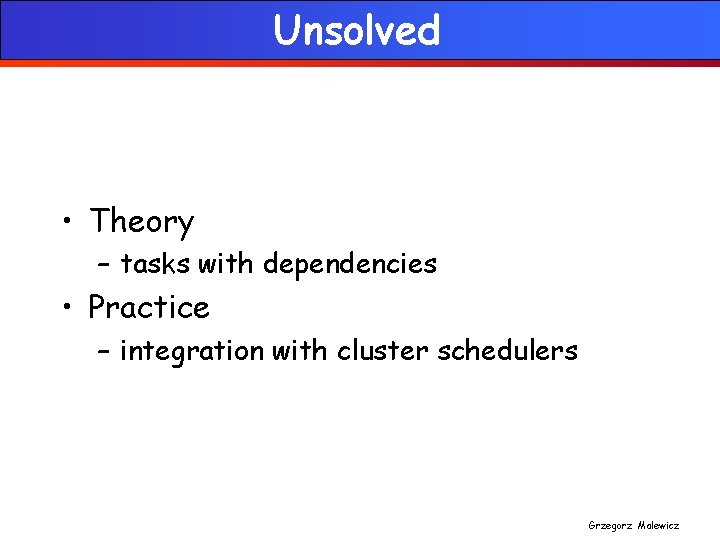
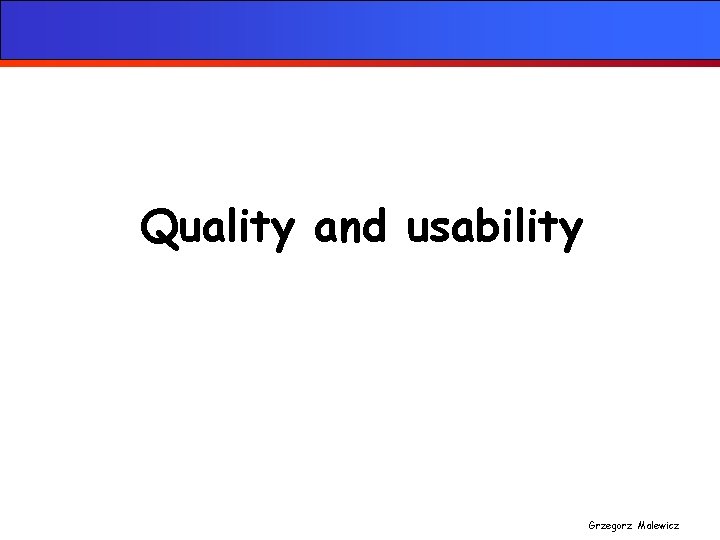
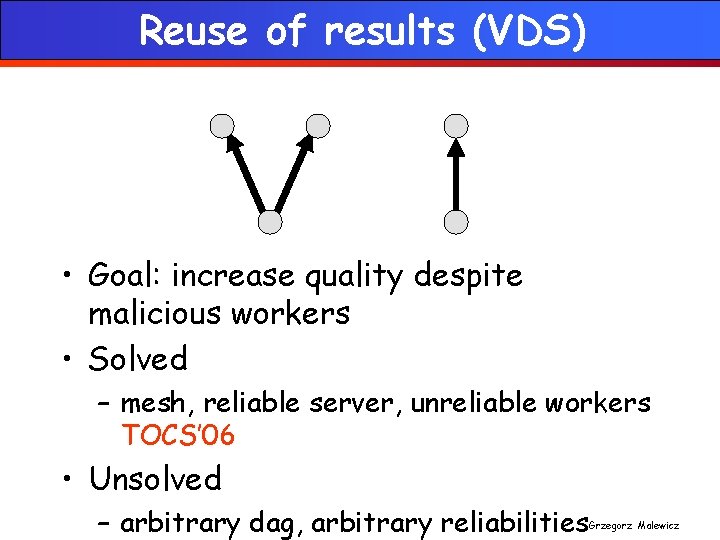
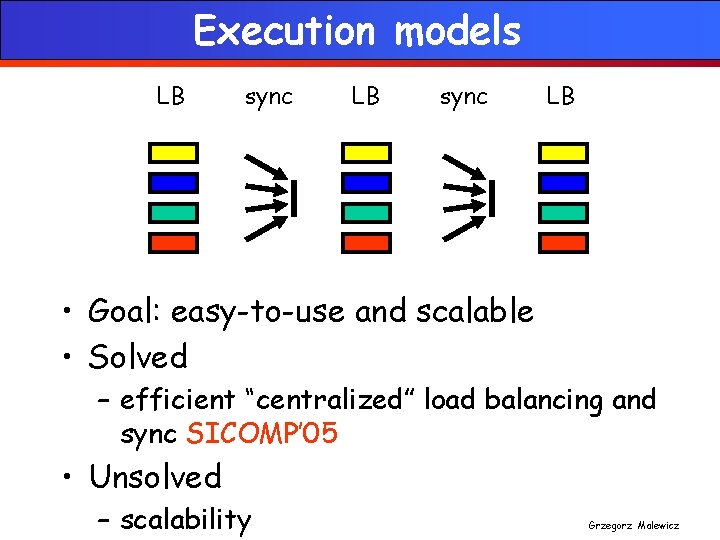
- Slides: 58
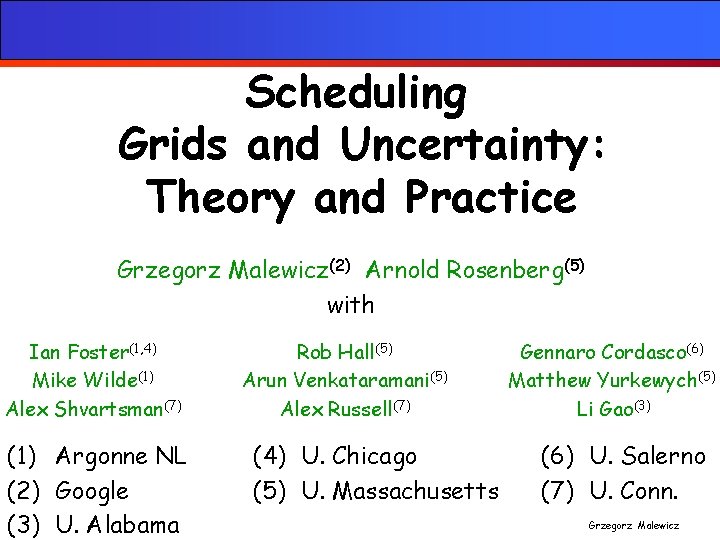
Scheduling Grids and Uncertainty: Theory and Practice Grzegorz Malewicz(2) Arnold Rosenberg(5) with Ian Foster(1, 4) Mike Wilde(1) Alex Shvartsman(7) (1) Argonne NL (2) Google (3) U. Alabama Rob Hall(5) Arun Venkataramani(5) Alex Russell(7) (4) U. Chicago (5) U. Massachusetts Gennaro Cordasco(6) Matthew Yurkewych(5) Li Gao(3) (6) U. Salerno (7) U. Conn. Grzegorz Malewicz
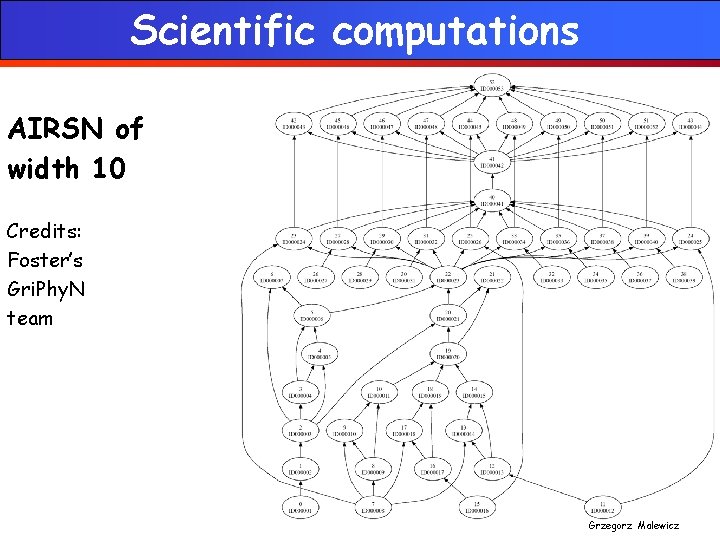
Scientific computations AIRSN of width 10 Credits: Foster’s Gri. Phy. N team Grzegorz Malewicz
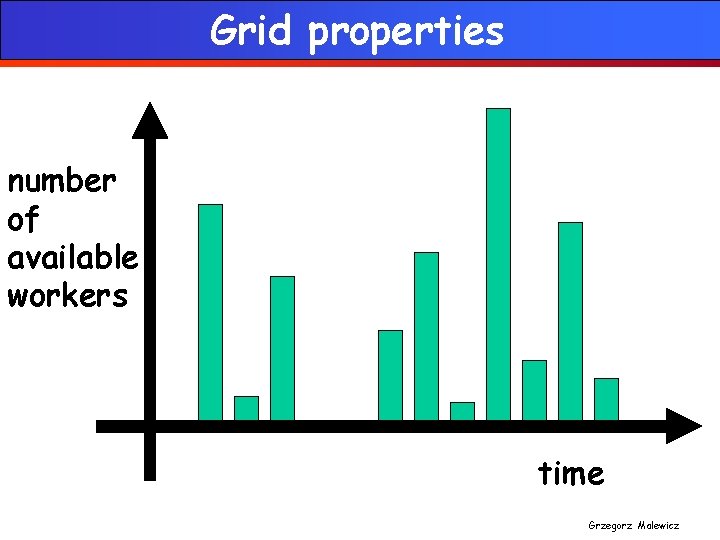
Grid properties number of available workers time Grzegorz Malewicz
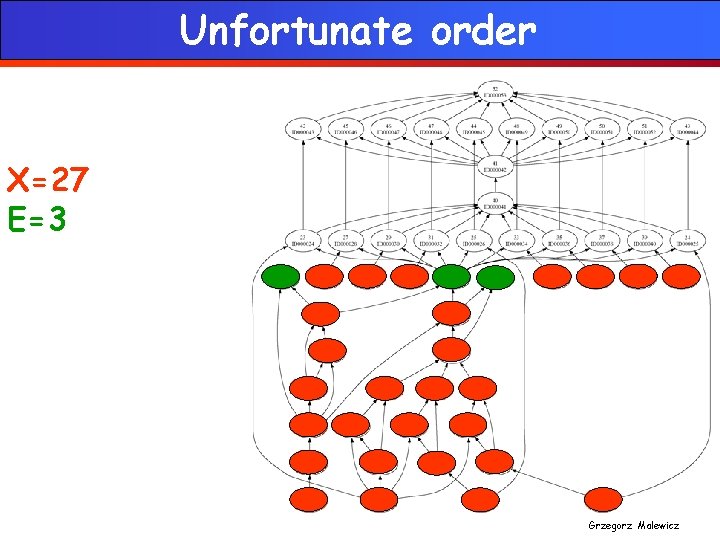
Unfortunate order X=27 E=3 Grzegorz Malewicz
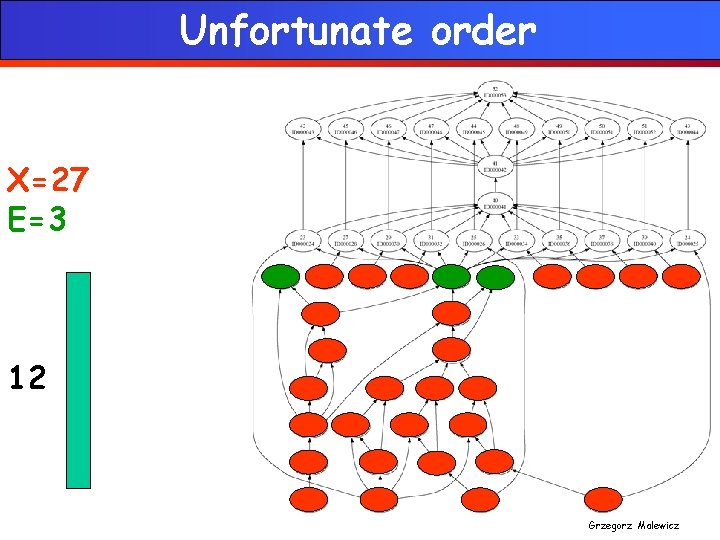
Unfortunate order X=27 E=3 12 Grzegorz Malewicz
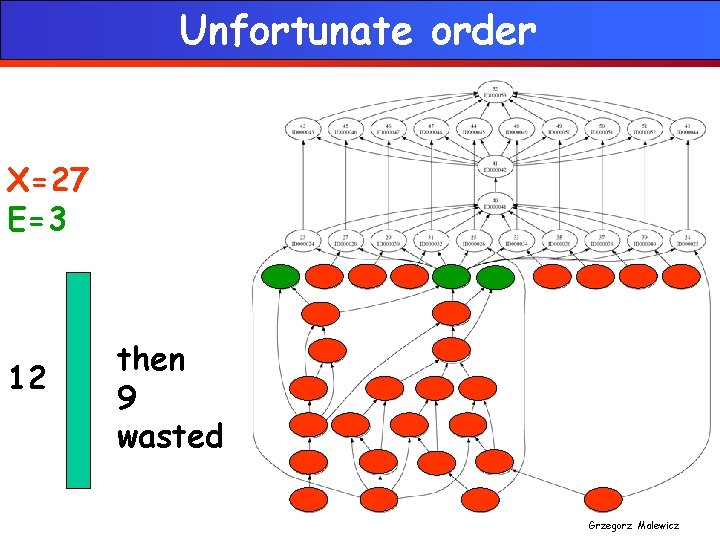
Unfortunate order X=27 E=3 12 then 9 wasted Grzegorz Malewicz
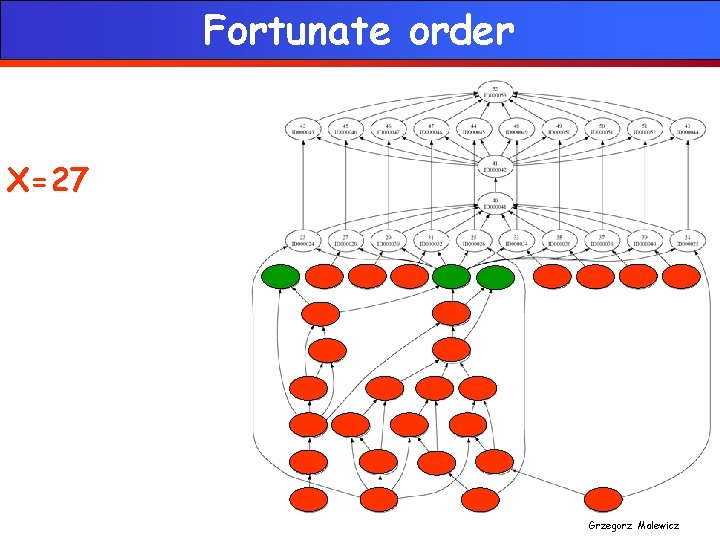
Fortunate order X=27 Grzegorz Malewicz
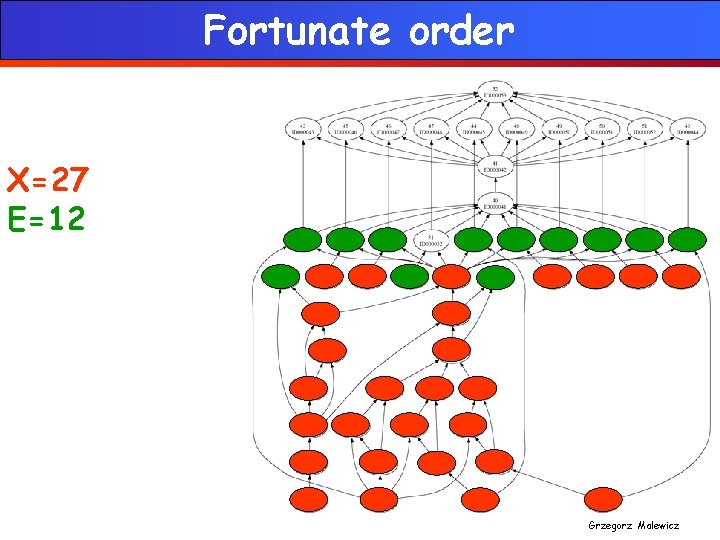
Fortunate order X=27 E=12 Grzegorz Malewicz
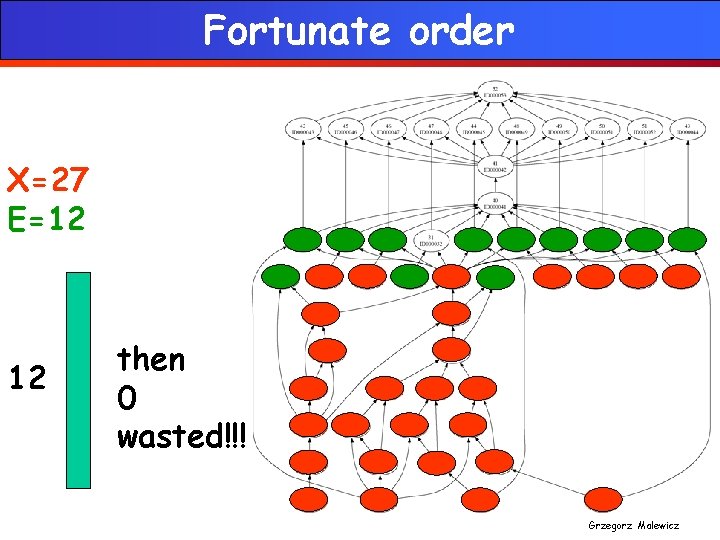
Fortunate order X=27 E=12 12 then 0 wasted!!! Grzegorz Malewicz
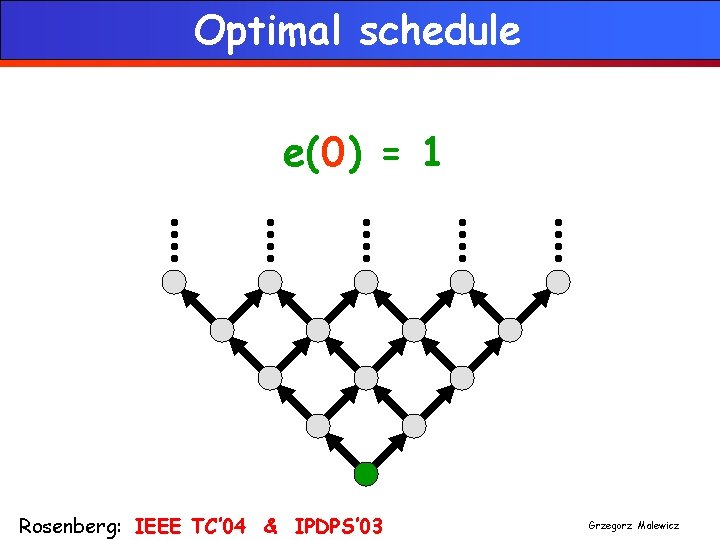
Optimal schedule e(0) = 1 Rosenberg: IEEE TC’ 04 & IPDPS’ 03 Grzegorz Malewicz
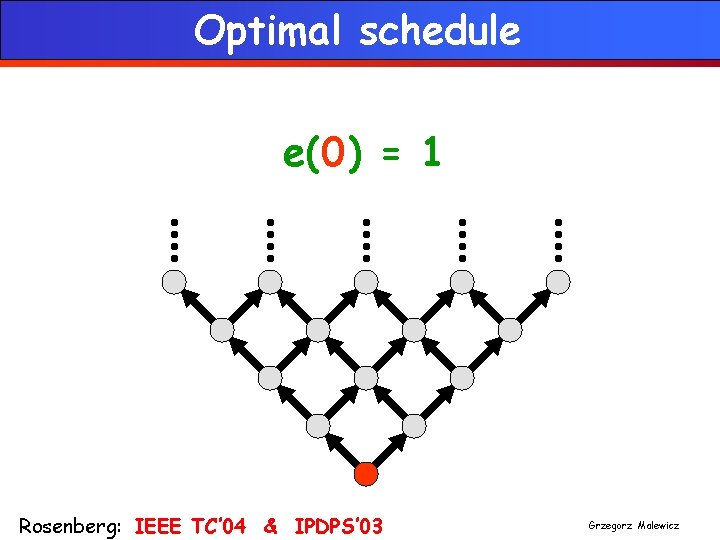
Optimal schedule e(0) = 1 Rosenberg: IEEE TC’ 04 & IPDPS’ 03 Grzegorz Malewicz
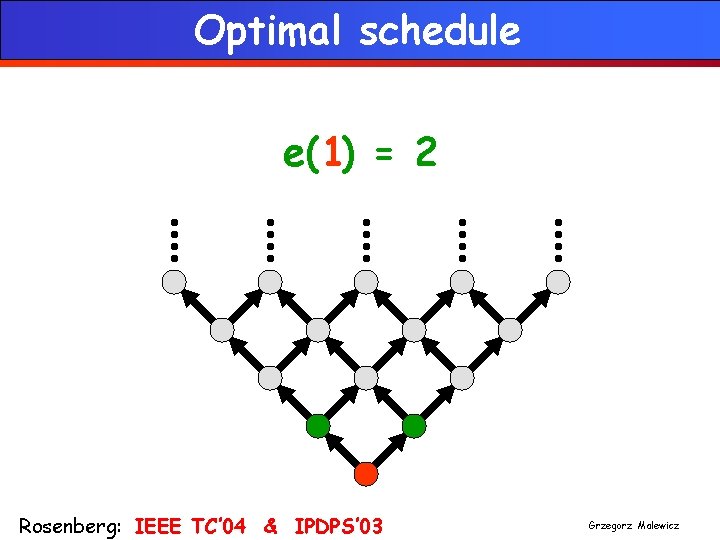
Optimal schedule e(1) = 2 Rosenberg: IEEE TC’ 04 & IPDPS’ 03 Grzegorz Malewicz
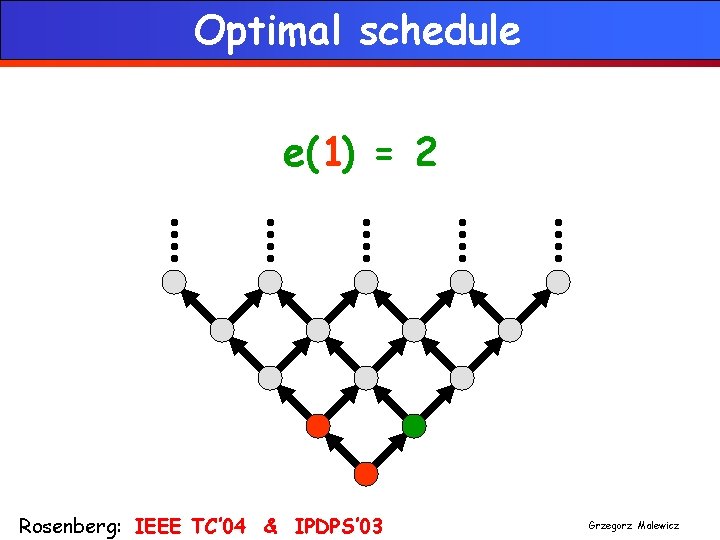
Optimal schedule e(1) = 2 Rosenberg: IEEE TC’ 04 & IPDPS’ 03 Grzegorz Malewicz
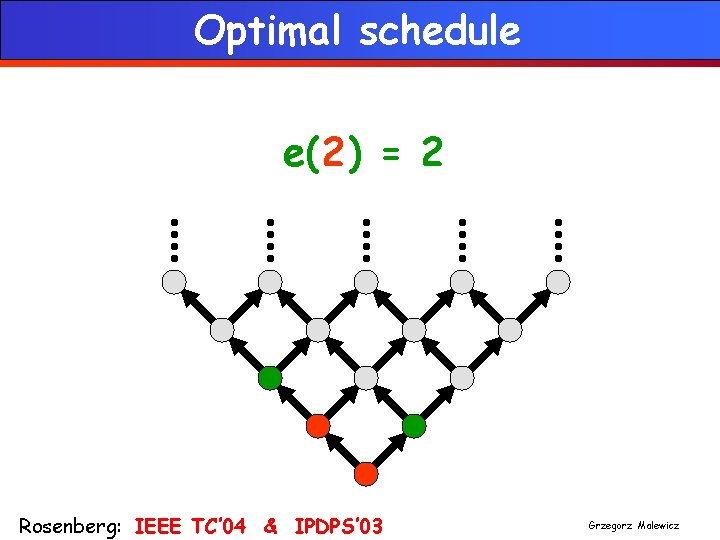
Optimal schedule e(2) = 2 Rosenberg: IEEE TC’ 04 & IPDPS’ 03 Grzegorz Malewicz
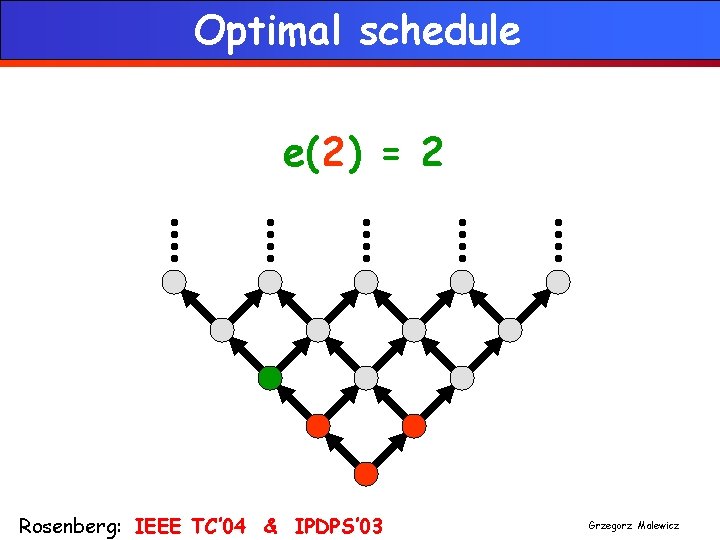
Optimal schedule e(2) = 2 Rosenberg: IEEE TC’ 04 & IPDPS’ 03 Grzegorz Malewicz
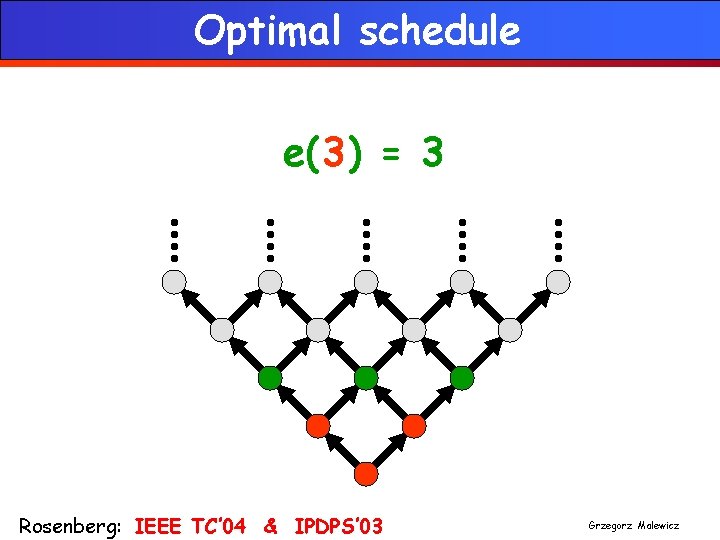
Optimal schedule e(3) = 3 Rosenberg: IEEE TC’ 04 & IPDPS’ 03 Grzegorz Malewicz
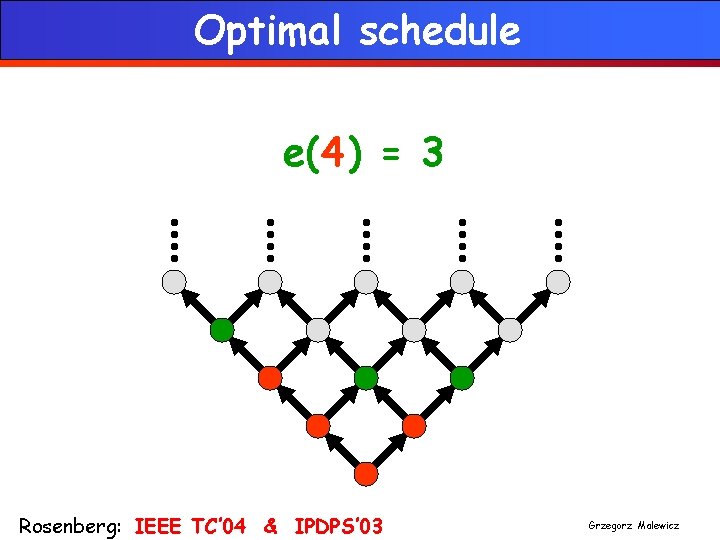
Optimal schedule e(4) = 3 Rosenberg: IEEE TC’ 04 & IPDPS’ 03 Grzegorz Malewicz
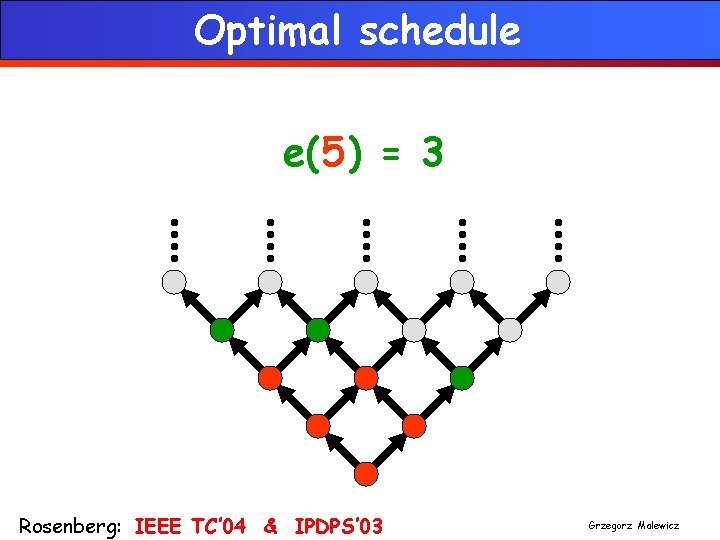
Optimal schedule e(5) = 3 Rosenberg: IEEE TC’ 04 & IPDPS’ 03 Grzegorz Malewicz
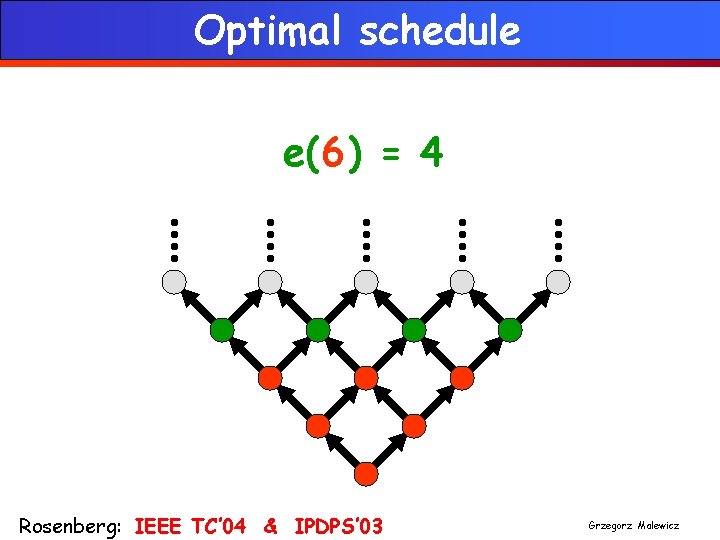
Optimal schedule e(6) = 4 Rosenberg: IEEE TC’ 04 & IPDPS’ 03 Grzegorz Malewicz
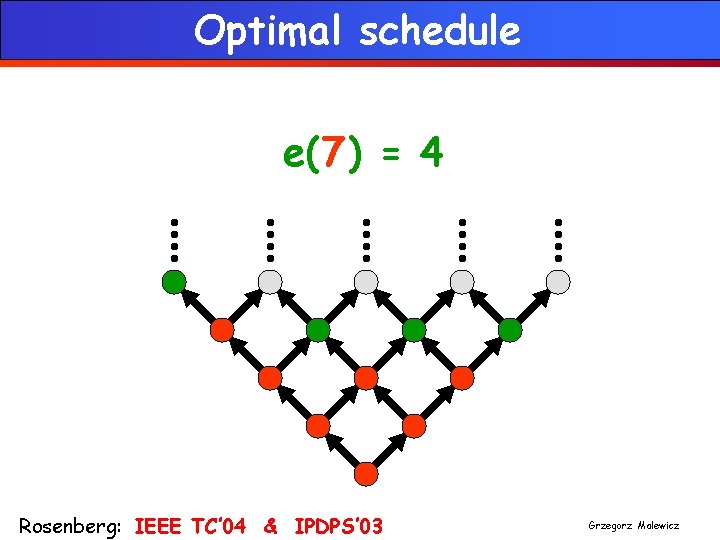
Optimal schedule e(7) = 4 Rosenberg: IEEE TC’ 04 & IPDPS’ 03 Grzegorz Malewicz
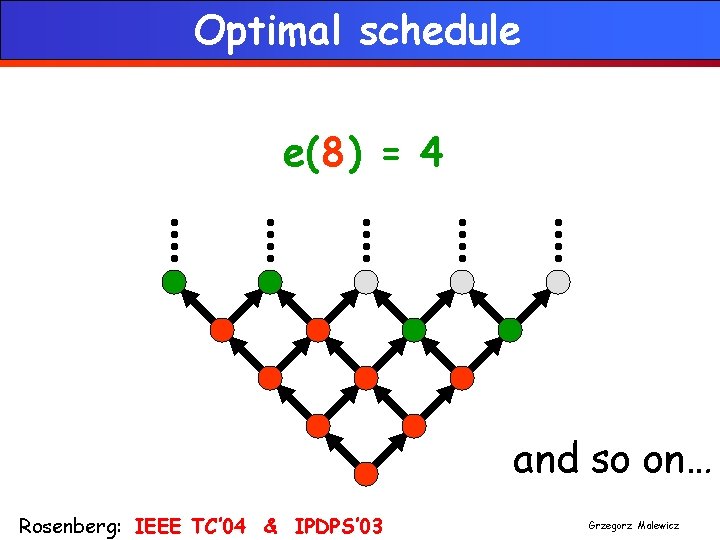
Optimal schedule e(8) = 4 and so on… Rosenberg: IEEE TC’ 04 & IPDPS’ 03 Grzegorz Malewicz
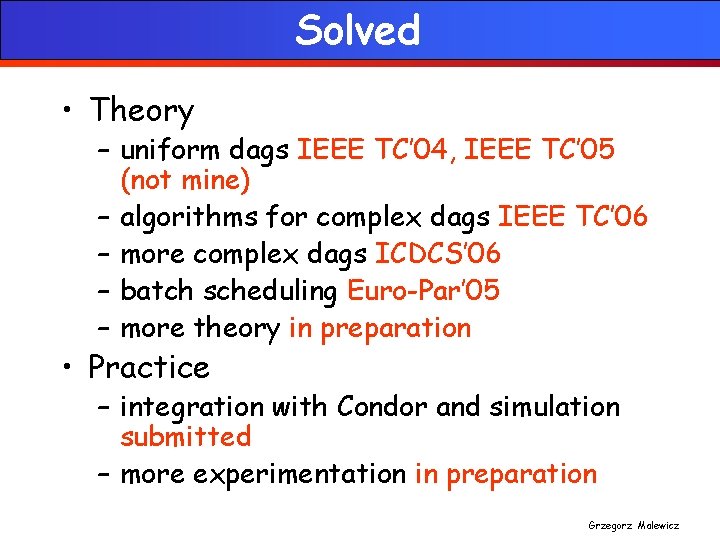
Solved • Theory – uniform dags IEEE TC’ 04, IEEE TC’ 05 (not mine) – algorithms for complex dags IEEE TC’ 06 – more complex dags ICDCS’ 06 – batch scheduling Euro-Par’ 05 – more theory in preparation • Practice – integration with Condor and simulation submitted – more experimentation in preparation Grzegorz Malewicz
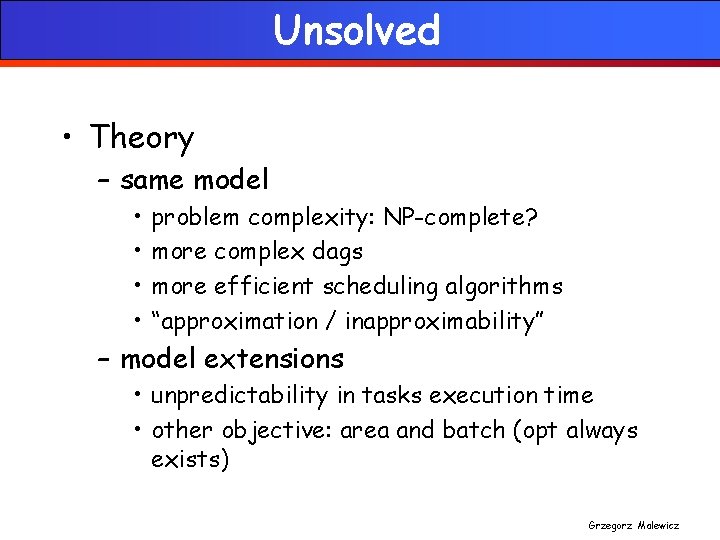
Unsolved • Theory – same model • • problem complexity: NP-complete? more complex dags more efficient scheduling algorithms “approximation / inapproximability” – model extensions • unpredictability in tasks execution time • other objective: area and batch (opt always exists) Grzegorz Malewicz
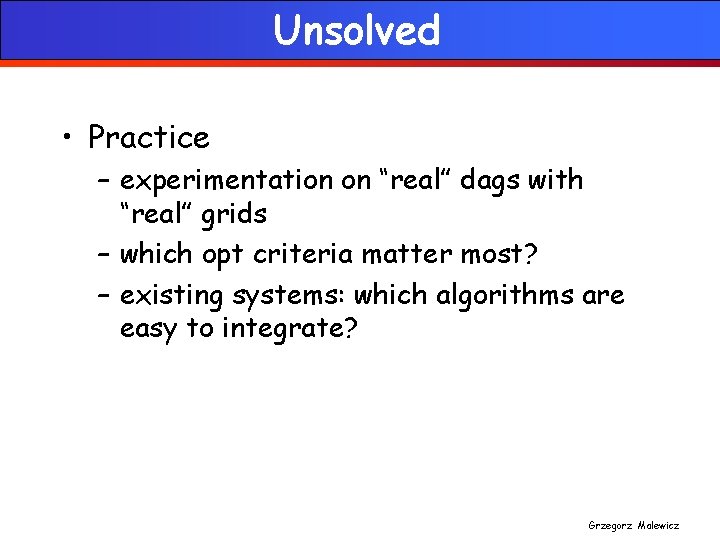
Unsolved • Practice – experimentation on “real” dags with “real” grids – which opt criteria matter most? – existing systems: which algorithms are easy to integrate? Grzegorz Malewicz
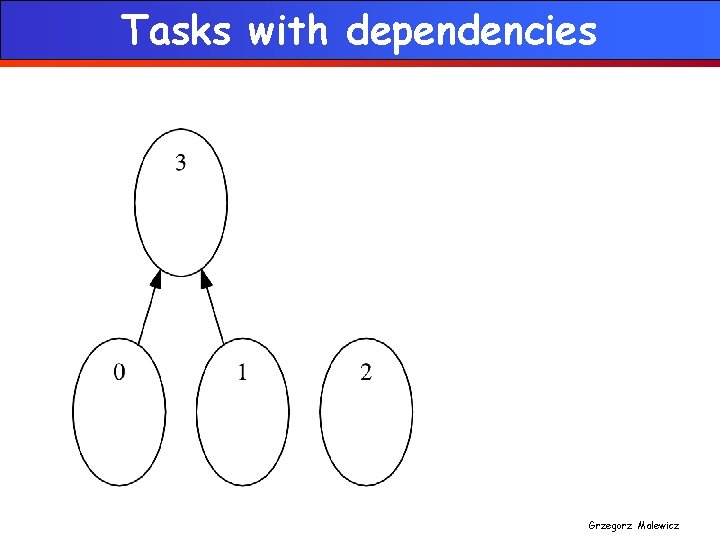
Tasks with dependencies Grzegorz Malewicz
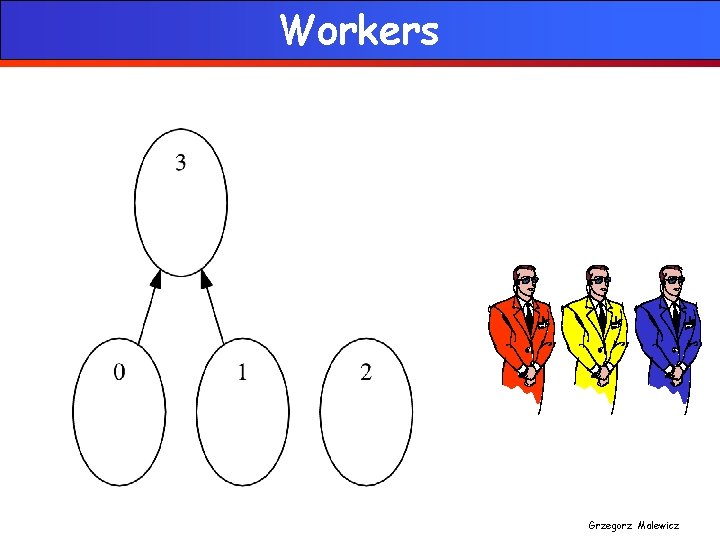
Workers Grzegorz Malewicz
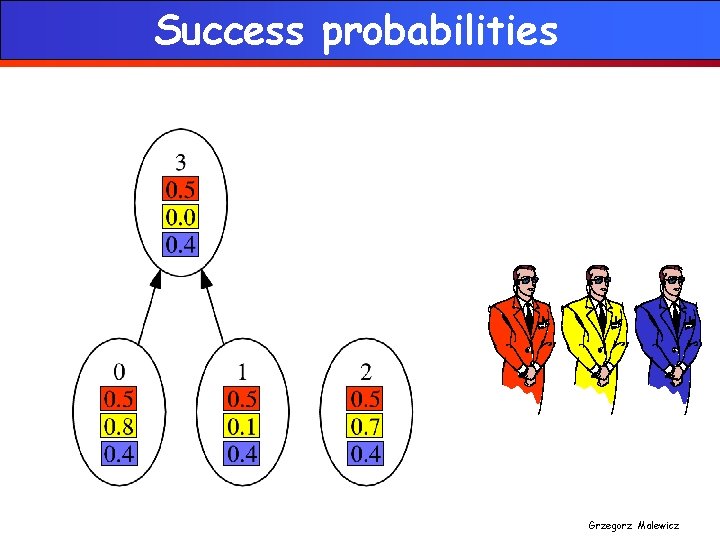
Success probabilities Grzegorz Malewicz
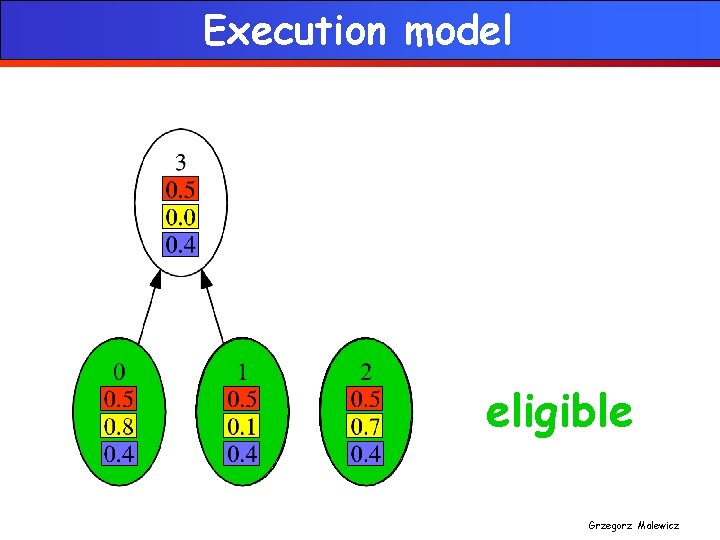
Execution model eligible Grzegorz Malewicz
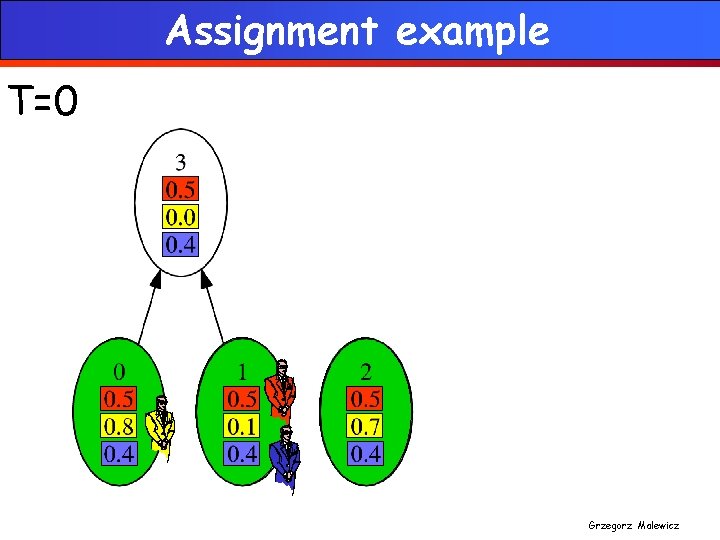
Assignment example T=0 Grzegorz Malewicz
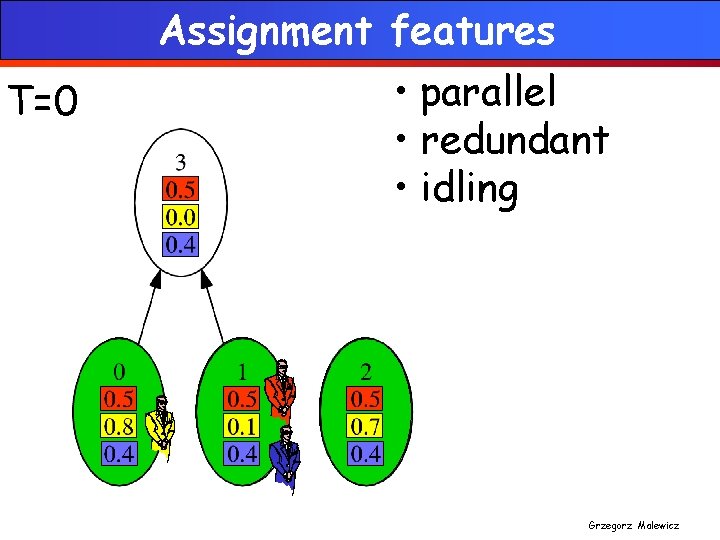
Assignment features T=0 • parallel • redundant • idling Grzegorz Malewicz
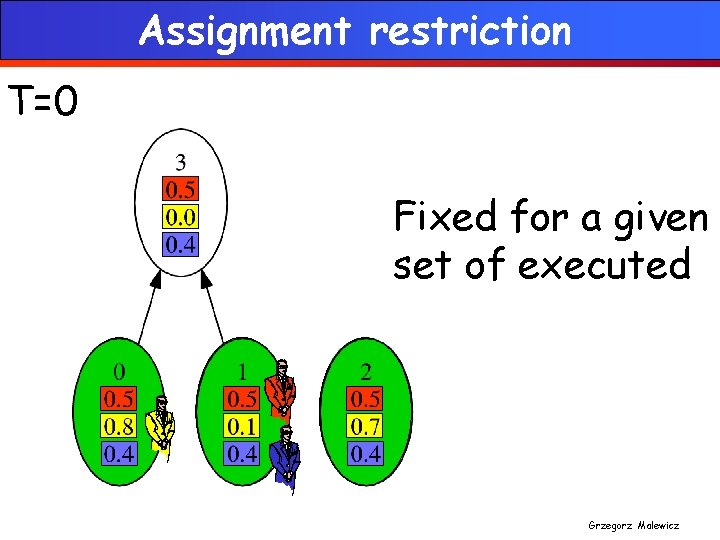
Assignment restriction T=0 Fixed for a given set of executed Grzegorz Malewicz
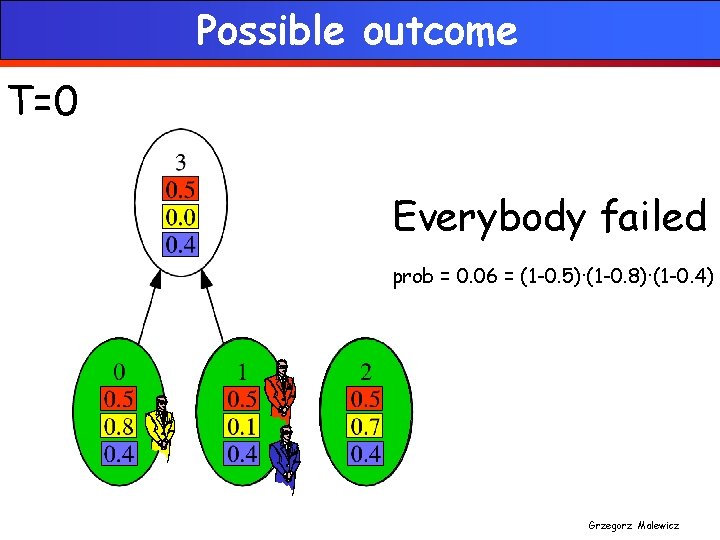
Possible outcome T=0 Everybody failed prob = 0. 06 = (1 -0. 5)∙(1 -0. 8)∙(1 -0. 4) Grzegorz Malewicz
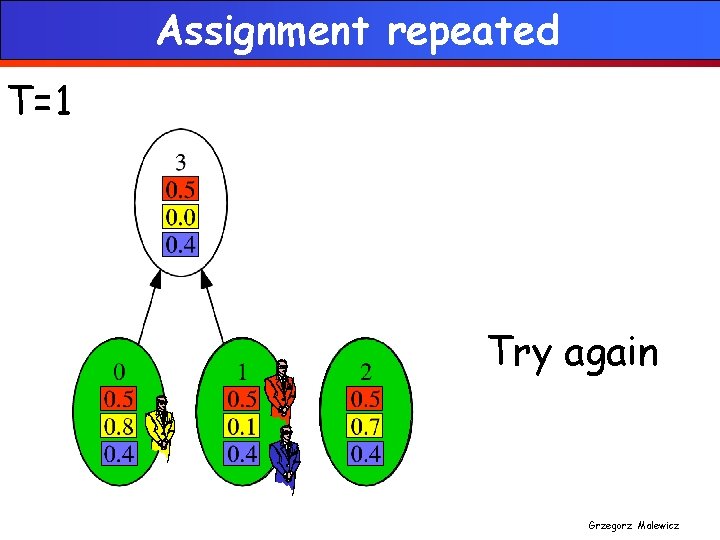
Assignment repeated T=1 Try again Grzegorz Malewicz
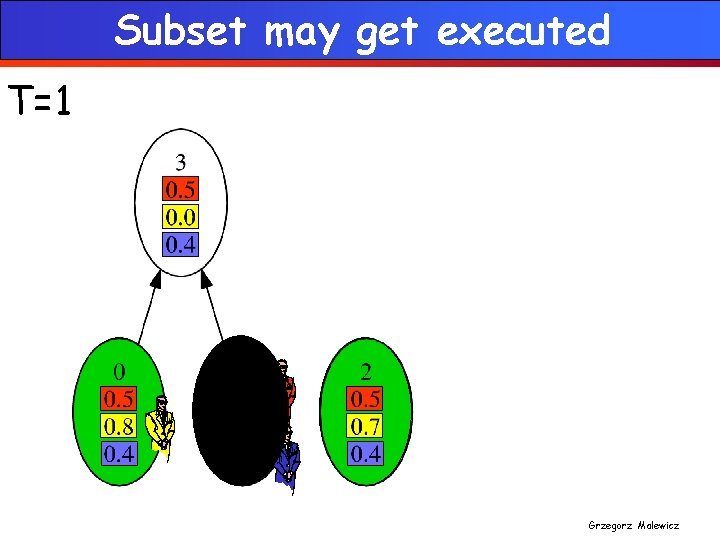
Subset may get executed T=1 Grzegorz Malewicz
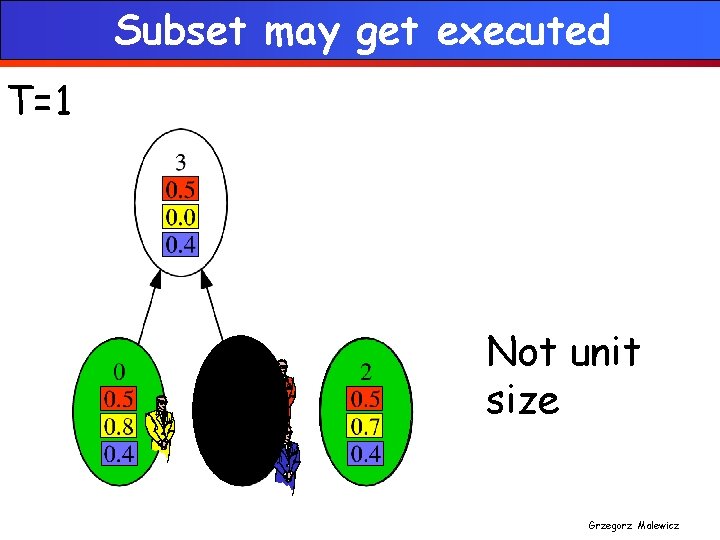
Subset may get executed T=1 Not unit size Grzegorz Malewicz
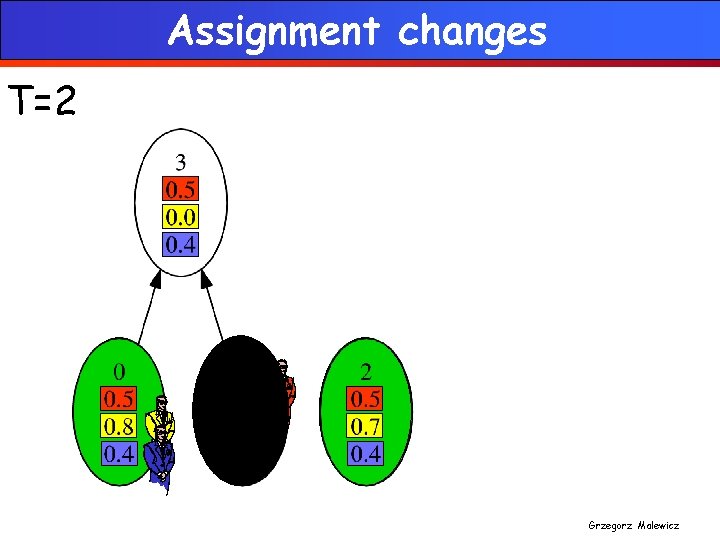
Assignment changes T=2 Grzegorz Malewicz
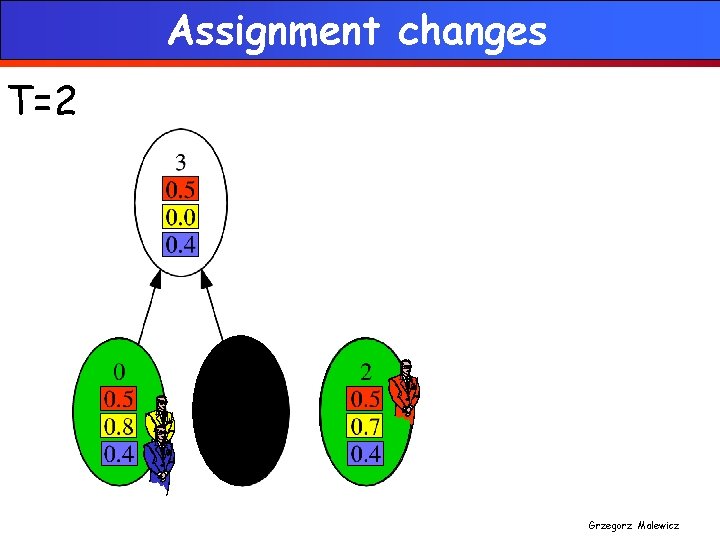
Assignment changes T=2 Grzegorz Malewicz
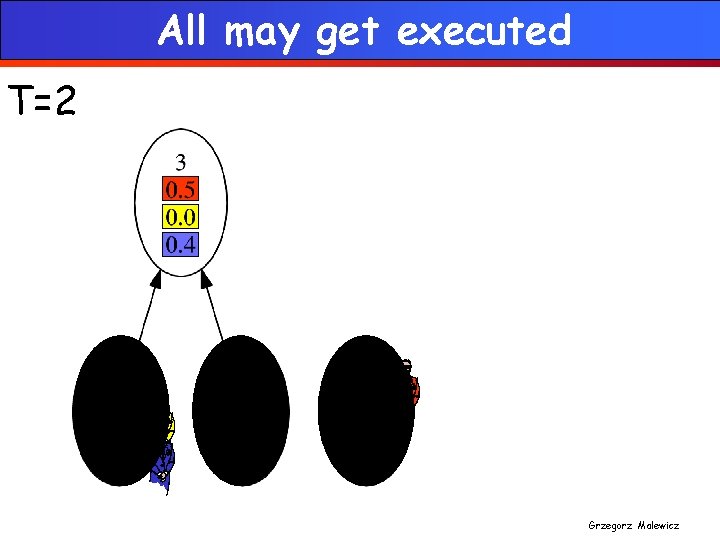
All may get executed T=2 Grzegorz Malewicz
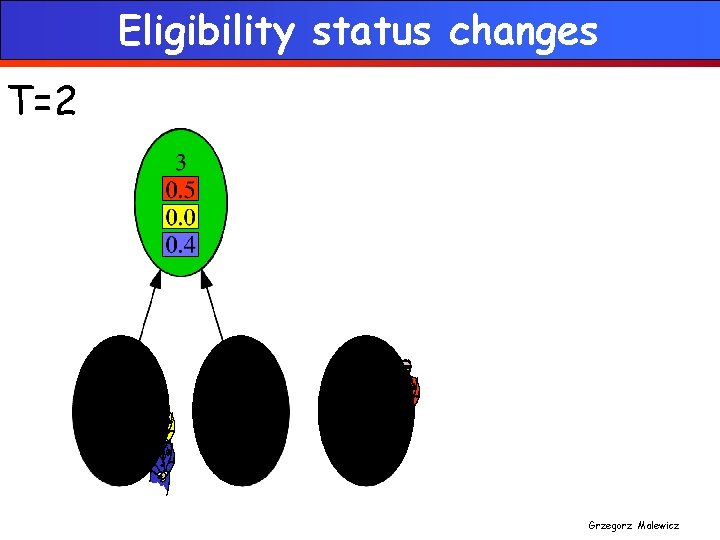
Eligibility status changes T=2 Grzegorz Malewicz
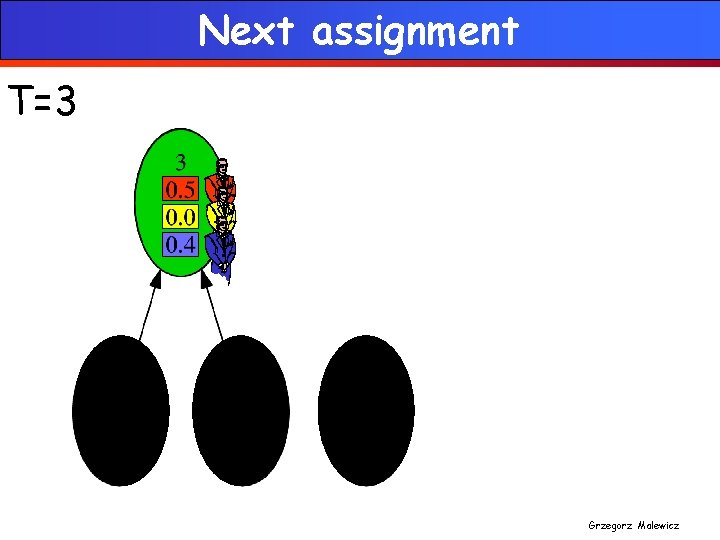
Next assignment T=3 Grzegorz Malewicz
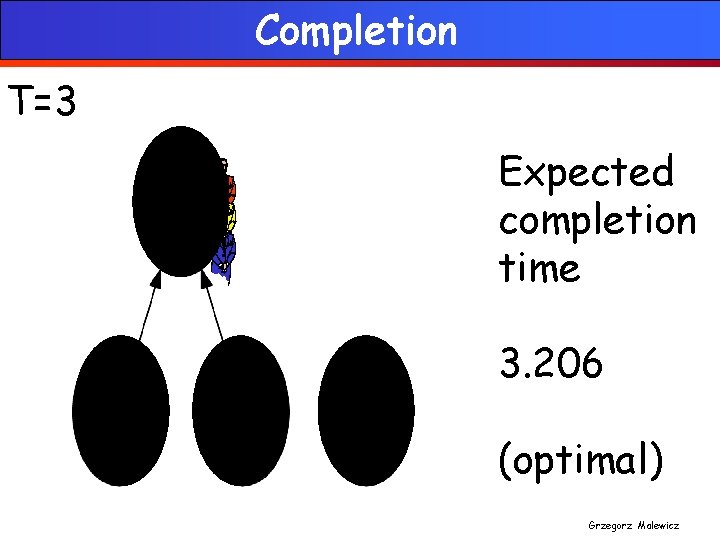
Completion T=3 Expected completion time 3. 206 (optimal) Grzegorz Malewicz
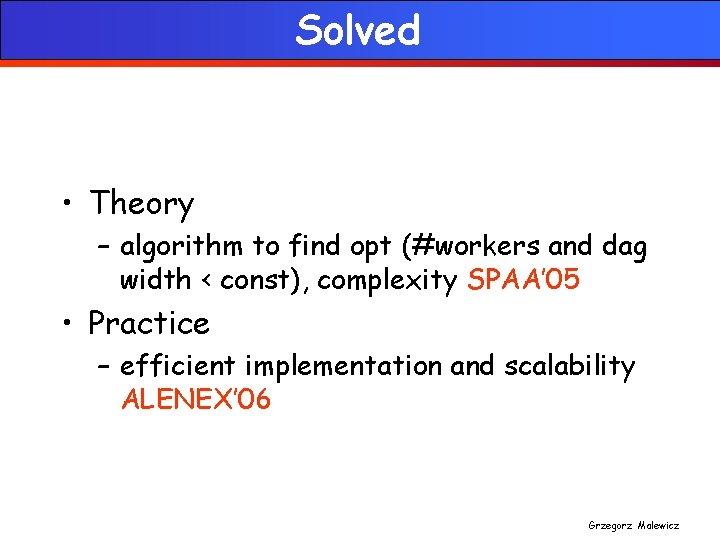
Solved • Theory – algorithm to find opt (#workers and dag width < const), complexity SPAA’ 05 • Practice – efficient implementation and scalability ALENEX’ 06 Grzegorz Malewicz
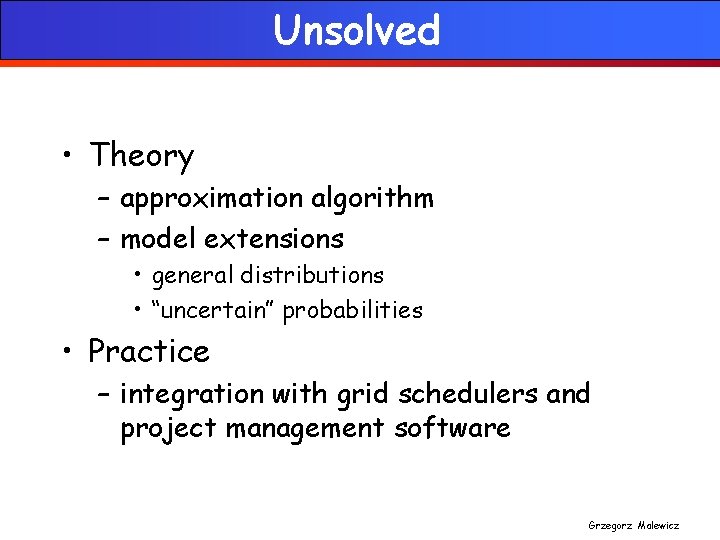
Unsolved • Theory – approximation algorithm – model extensions • general distributions • “uncertain” probabilities • Practice – integration with grid schedulers and project management software Grzegorz Malewicz
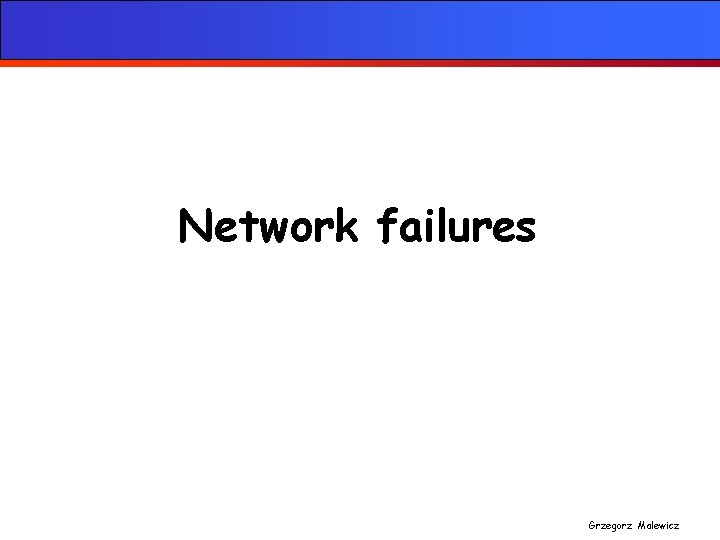
Network failures Grzegorz Malewicz
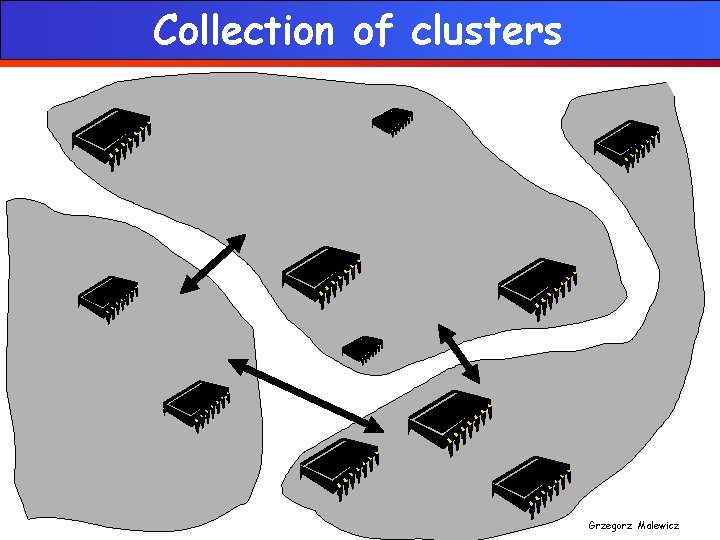
Collection of clusters Grzegorz Malewicz
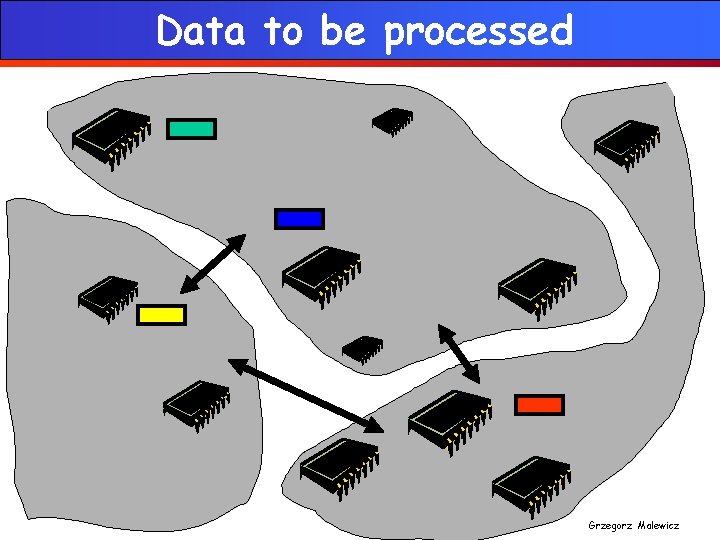
Data to be processed Grzegorz Malewicz
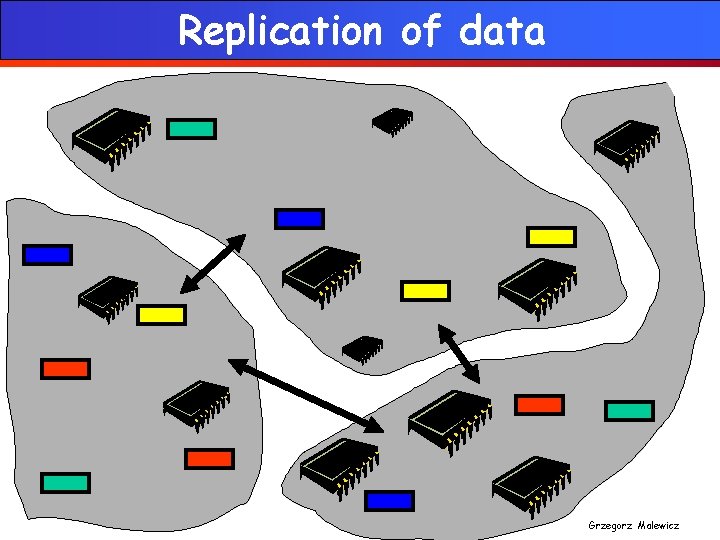
Replication of data Grzegorz Malewicz
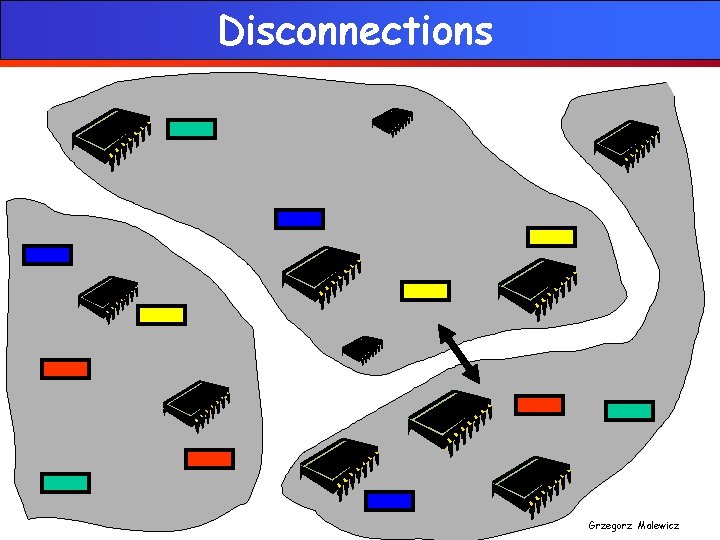
Disconnections Grzegorz Malewicz
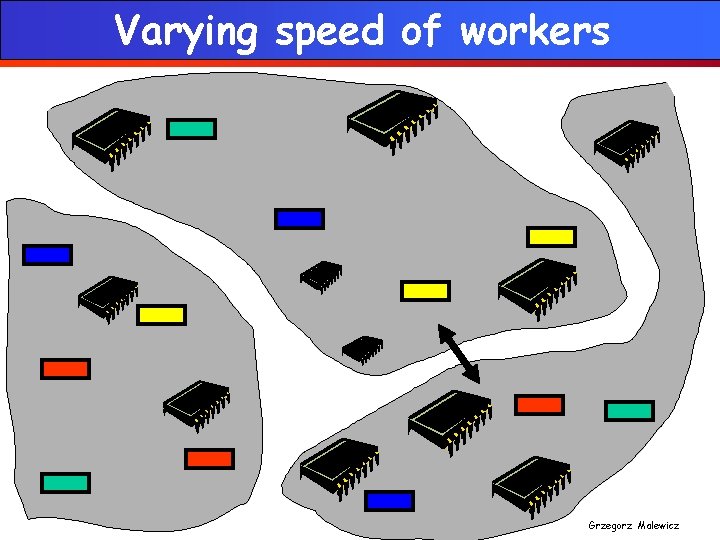
Varying speed of workers Grzegorz Malewicz
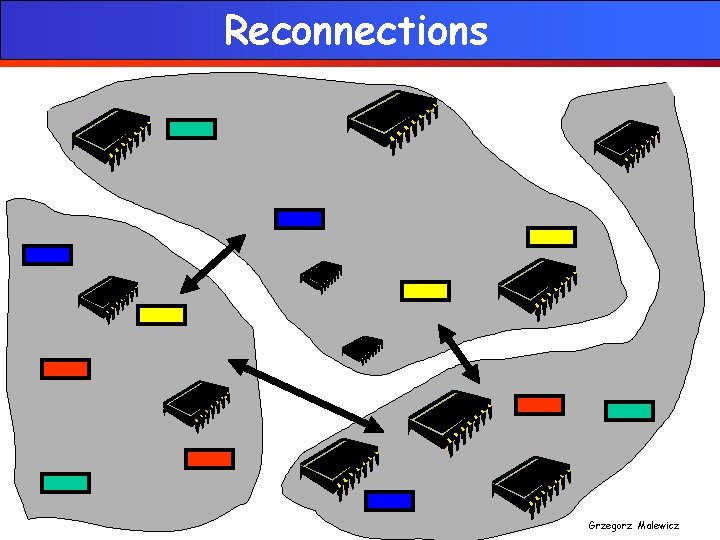
Reconnections Grzegorz Malewicz
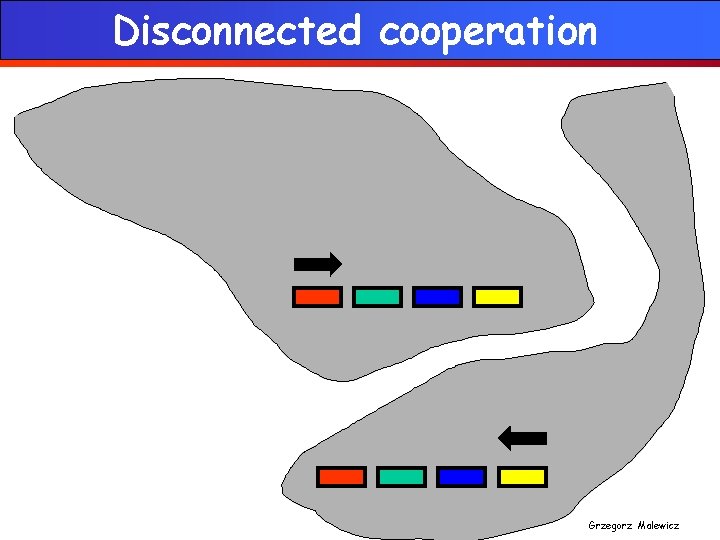
Disconnected cooperation Grzegorz Malewicz
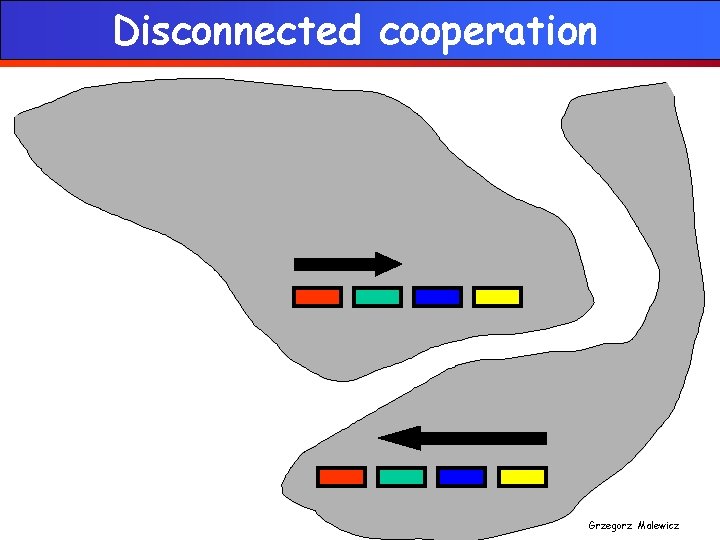
Disconnected cooperation Grzegorz Malewicz
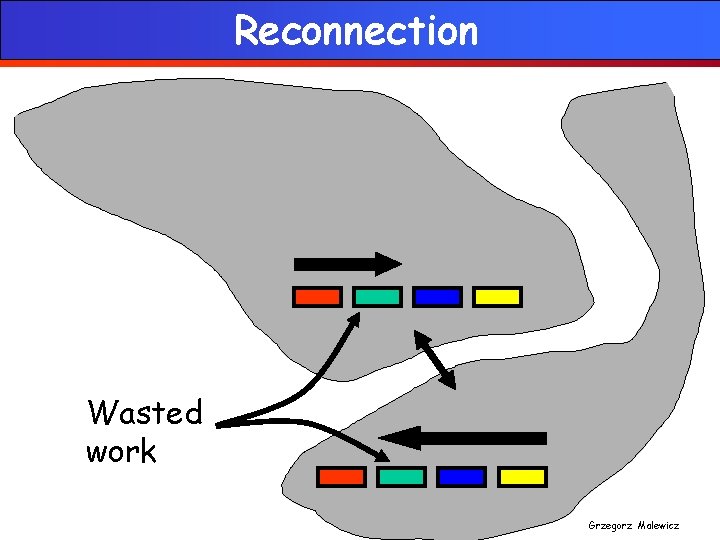
Reconnection Wasted work Grzegorz Malewicz
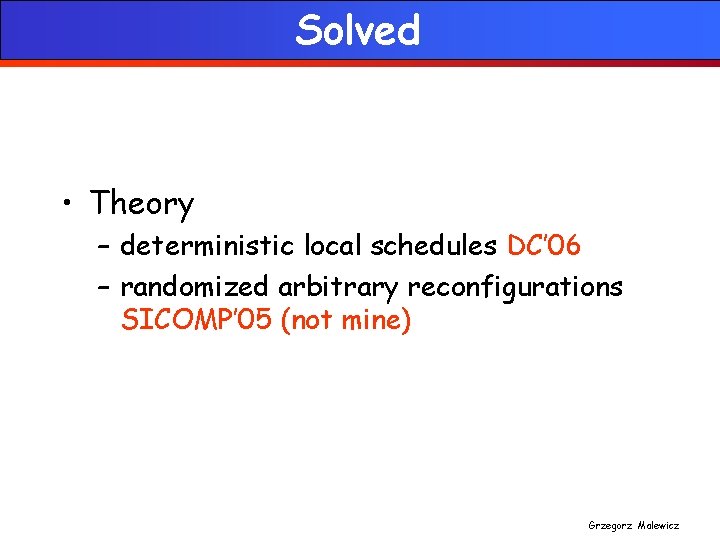
Solved • Theory – deterministic local schedules DC’ 06 – randomized arbitrary reconfigurations SICOMP’ 05 (not mine) Grzegorz Malewicz
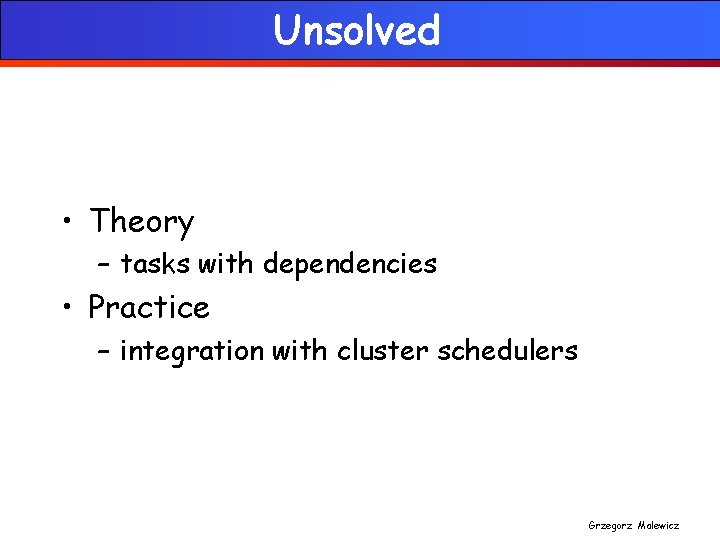
Unsolved • Theory – tasks with dependencies • Practice – integration with cluster schedulers Grzegorz Malewicz
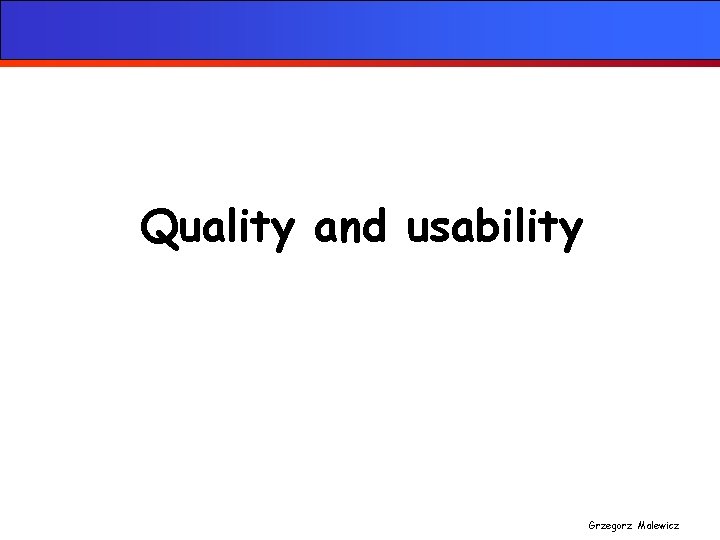
Quality and usability Grzegorz Malewicz
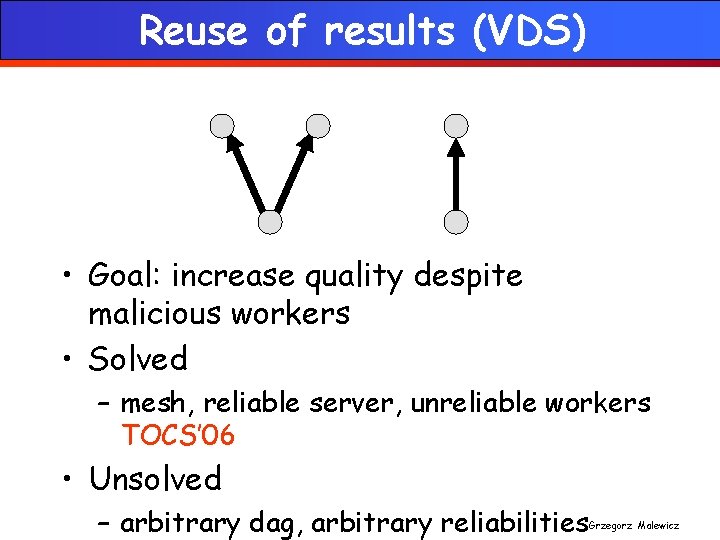
Reuse of results (VDS) • Goal: increase quality despite malicious workers • Solved – mesh, reliable server, unreliable workers TOCS’ 06 • Unsolved – arbitrary dag, arbitrary reliabilities Grzegorz Malewicz
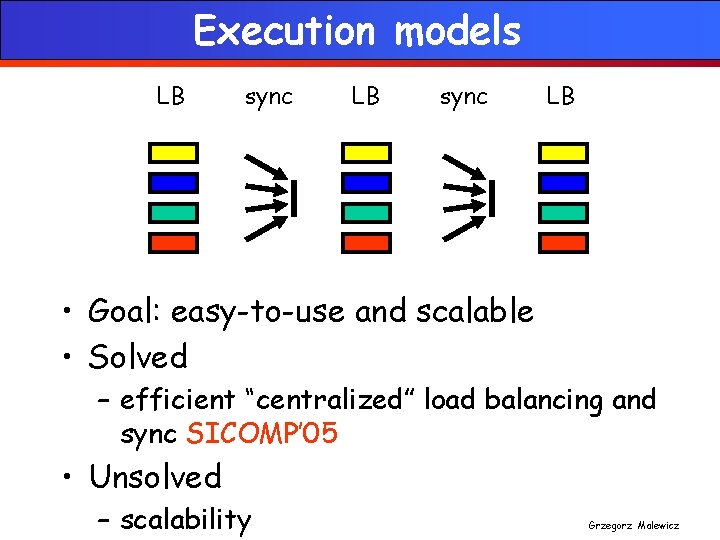
Execution models LB sync LB • Goal: easy-to-use and scalable • Solved – efficient “centralized” load balancing and sync SICOMP’ 05 • Unsolved – scalability Grzegorz Malewicz
Layered architecture for web services and grids
Job scheduling vs process scheduling
Grid ratio formula
Glow discharge tem
Decimal using grids
Parallel research kernels
Demand response in smart grids
Salary grid
Bootstrap 4 osztály
Differentiation grids
Grzegorz jacewicz
Grzegorz malewicz
Mzyk pwr
Grzegorz jokiel
Grzegorz zuzel
Grzegorz brychczyński
Grzegorz goryl
Endometrioza stopnie
Grzegorz musiał uam
Grzegorz walczyk
Grzegorz zuzel
Grzegorz osyra
Stegonography
Grzegorz gogolewski
Grzegorz markocki nauczyciel
Grzegorz jokiel
Anna głodek
Mikrologistyka
Grzegorz figiel
Grzegorz zubowicz
Rudolf leim
Mendel grzegorz
Grzegorz pytel
Grzegorz koczyk
Uncertainty reduction theory
Practice assessor and practice supervisor
Quality assurance theory
Health and fitness: theory and practice
Quality revolution in software testing
Software testing and quality assurance theory and practice
Software testing and quality assurance theory and practice
Software testing and quality assurance theory and practice
Significant figures cartoon
What is risk continuum
Position paper importance
Power distance map
Difference between risk and uncertainty
Low and high uncertainty avoidance
Multiplying and dividing uncertainties
Experimental errors and uncertainty
Mass society and democracy lesson 1
Measurements and their uncertainty
Natural broadening
Capital budgeting under risk and uncertainty
Risk and uncertainty in farm management
Adjusted discount rate formula
Heisenberg uncertainty principle statement
Theory and practice of histotechnology
Oligarchical collectivism