Infineon GOR AG Optimization under Uncertainty Physikalisches Institut
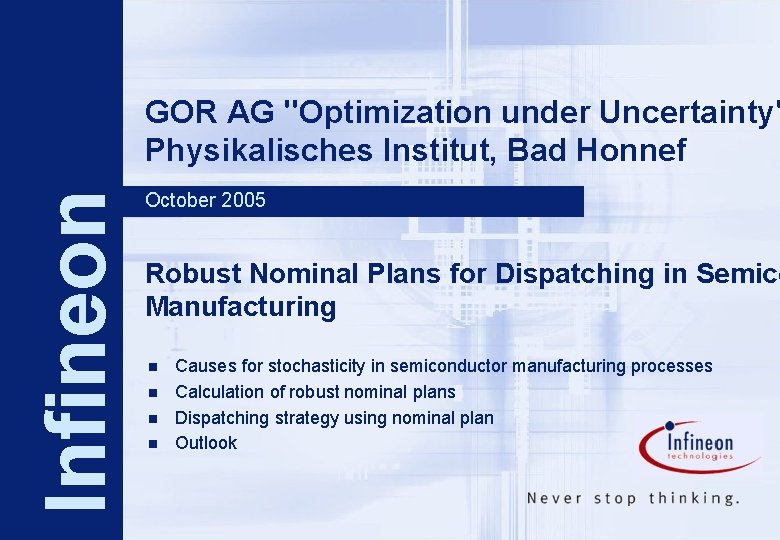
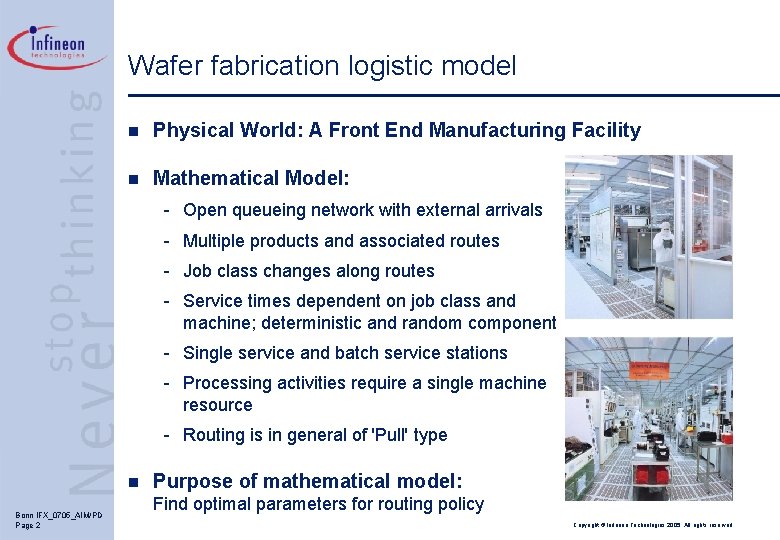
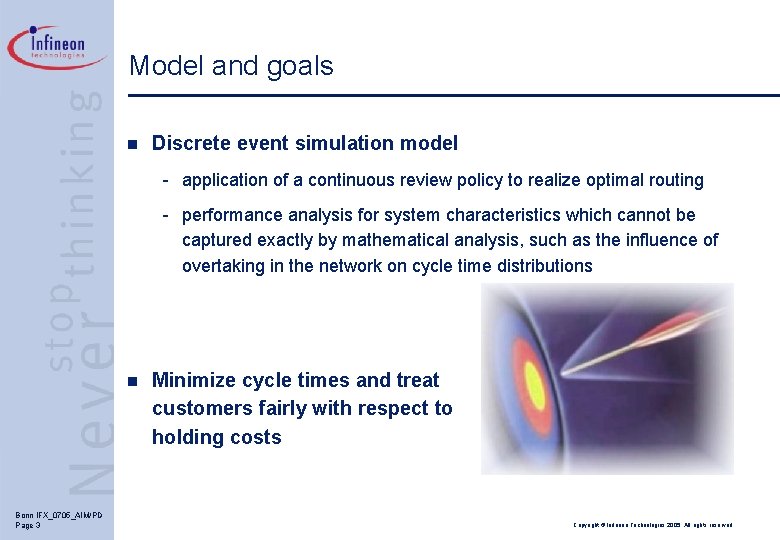
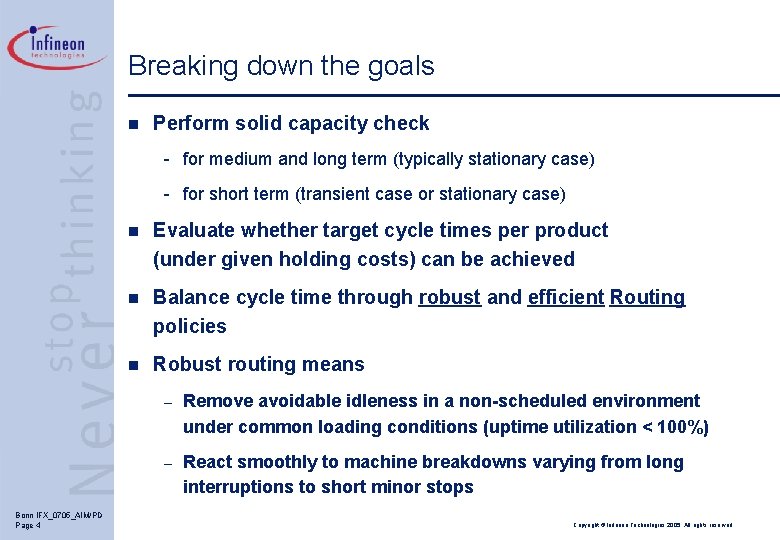
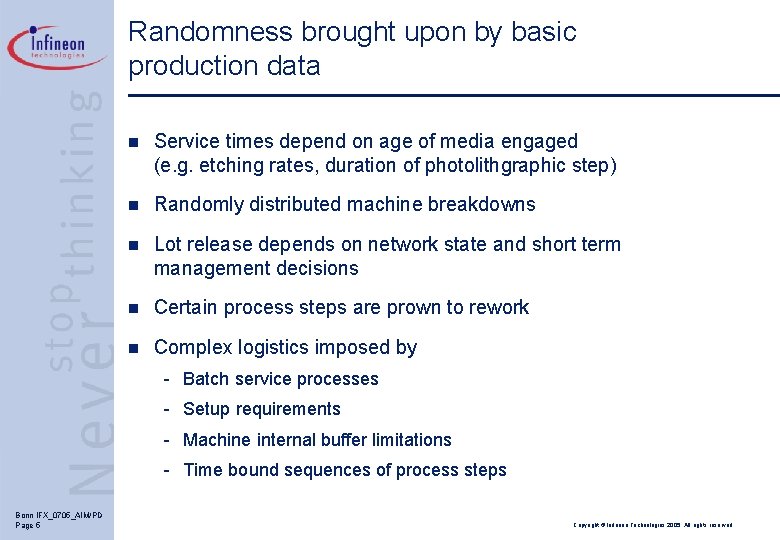
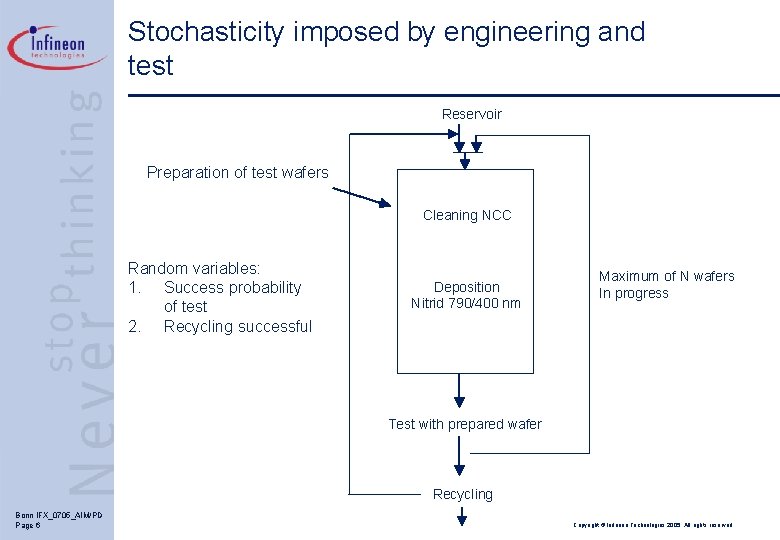
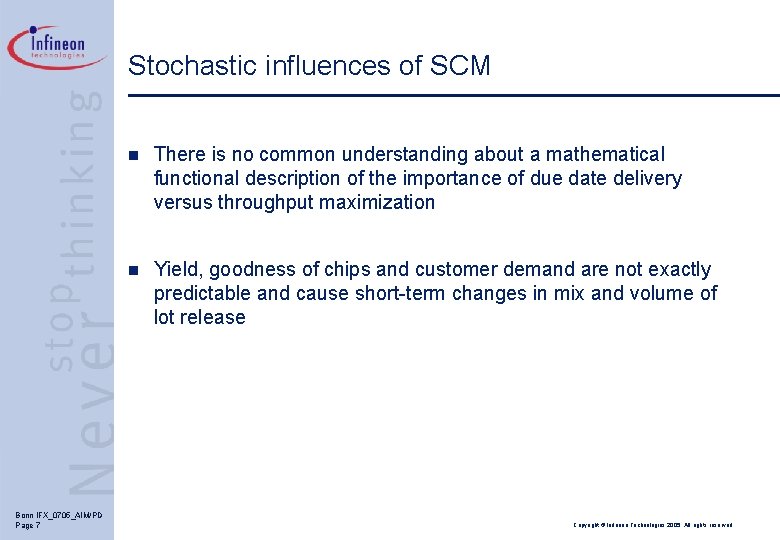
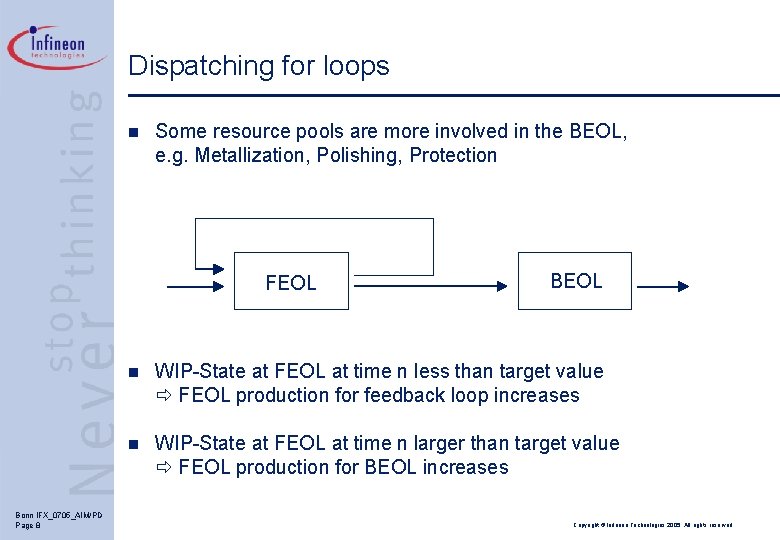
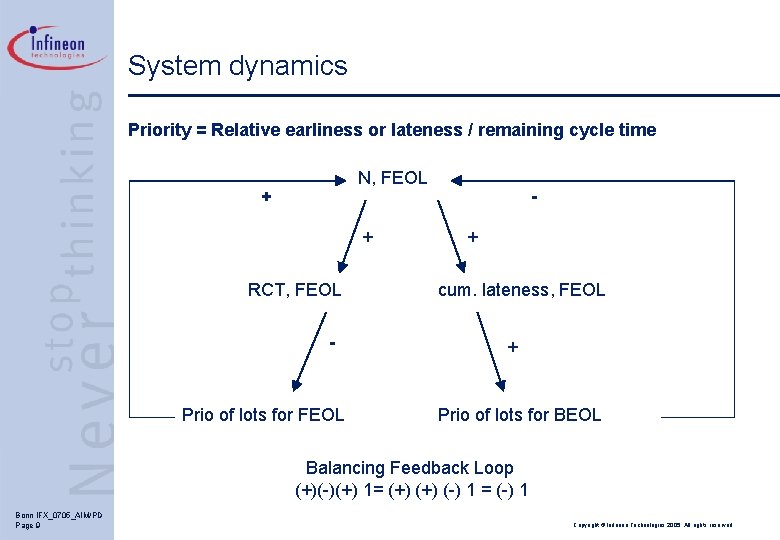
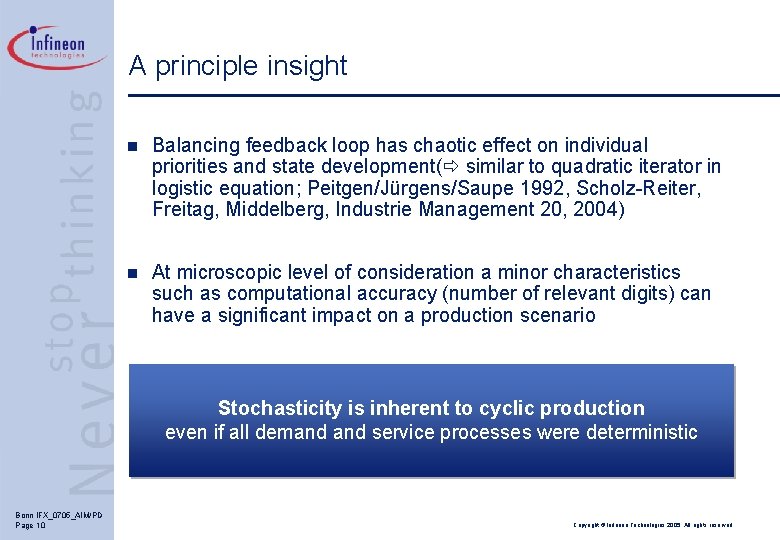
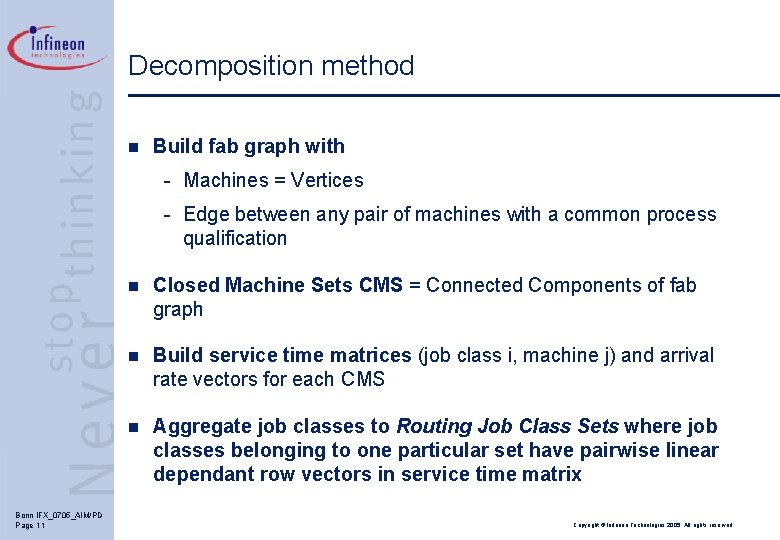
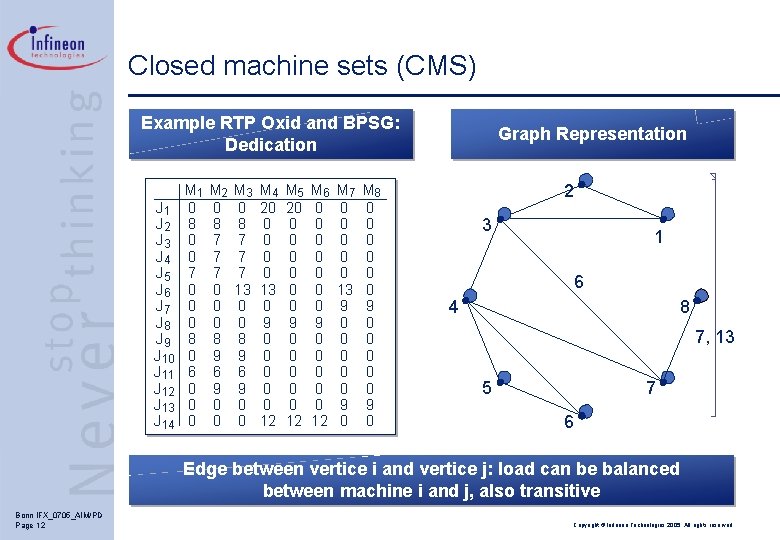
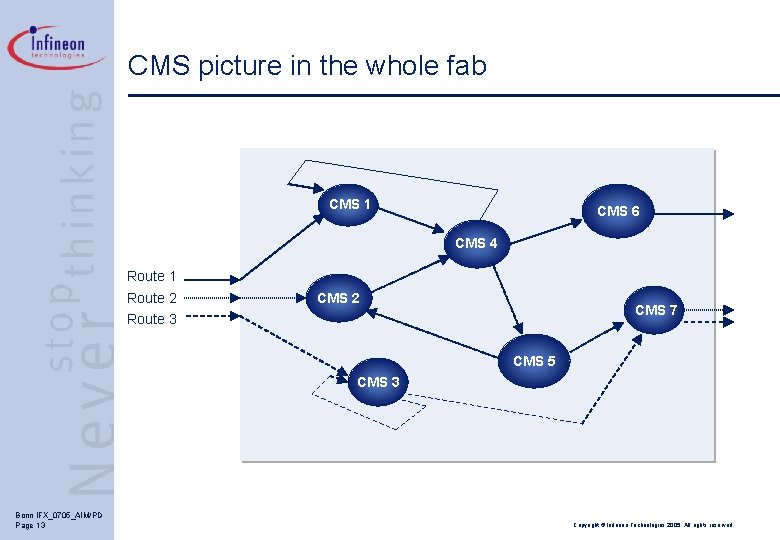
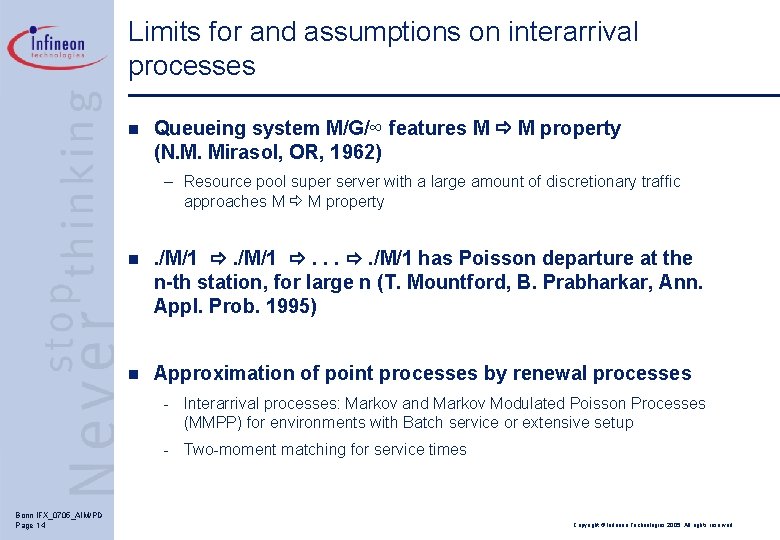
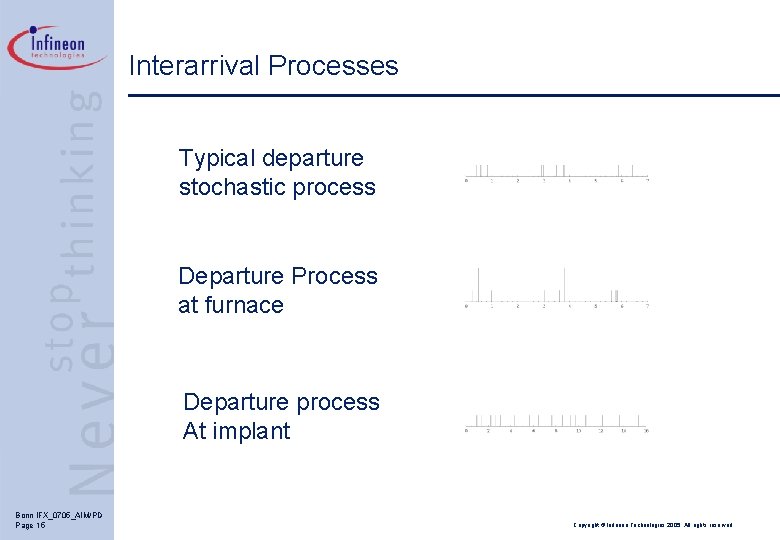
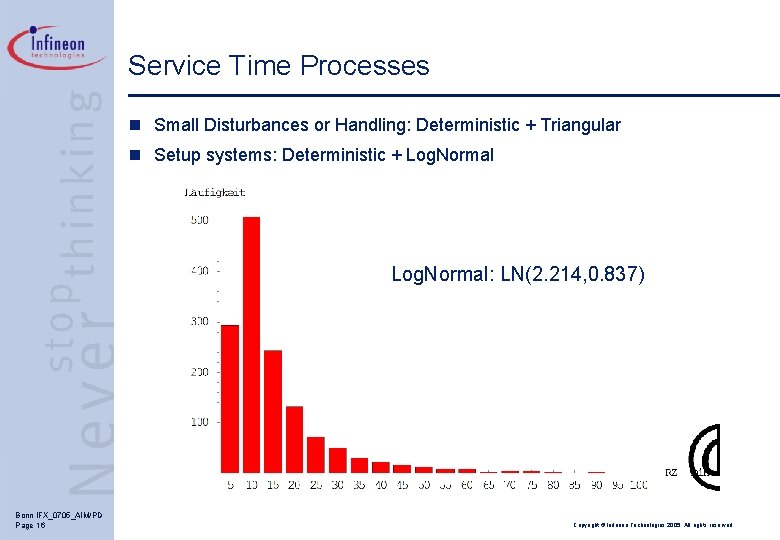
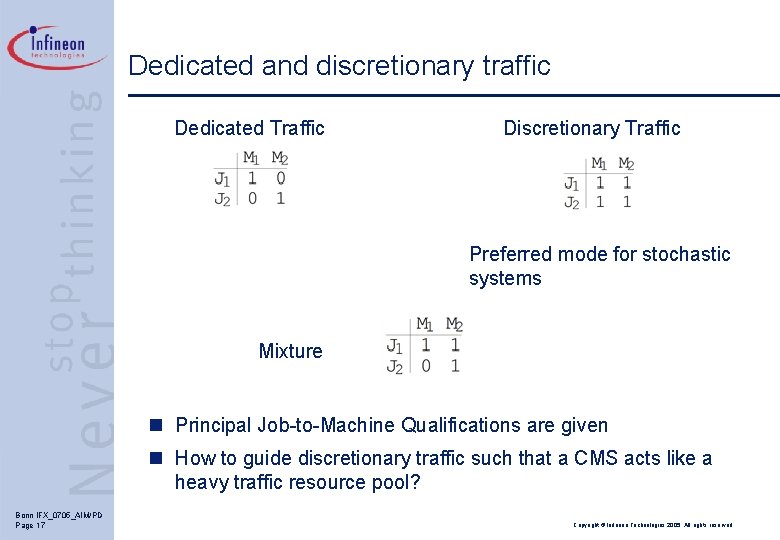
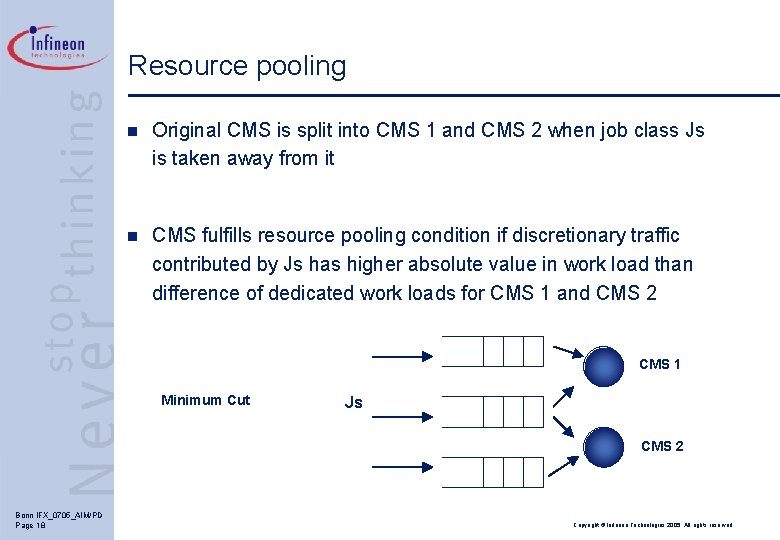
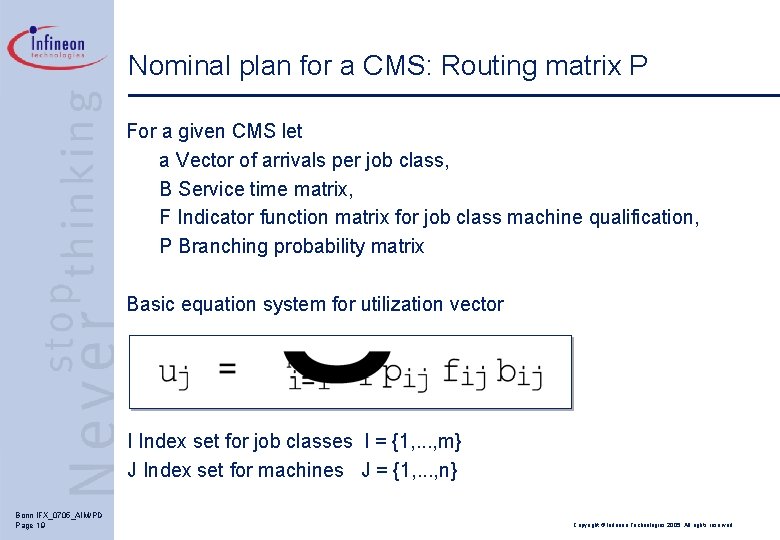
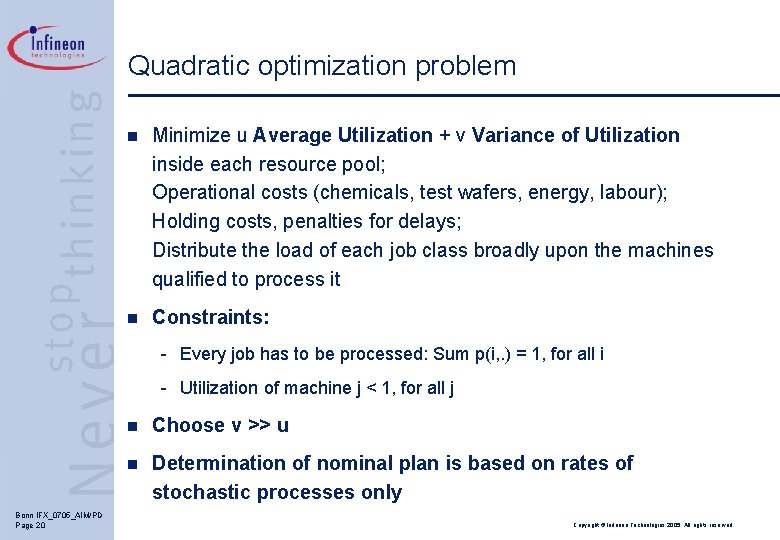
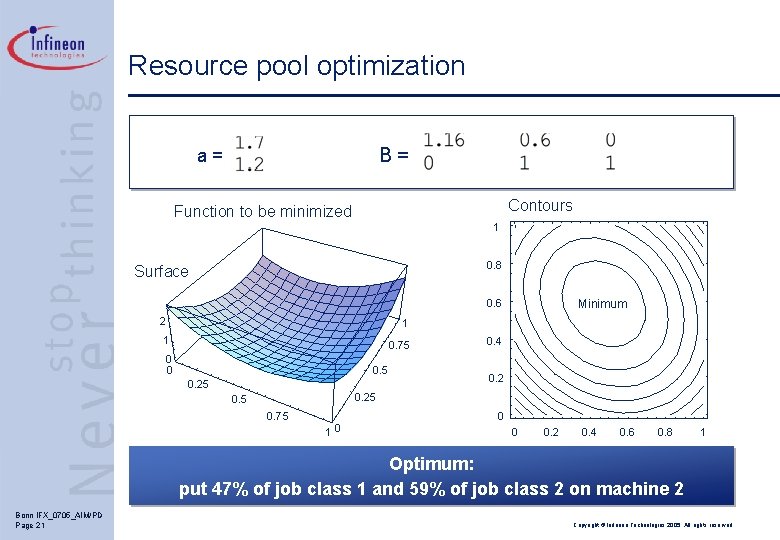
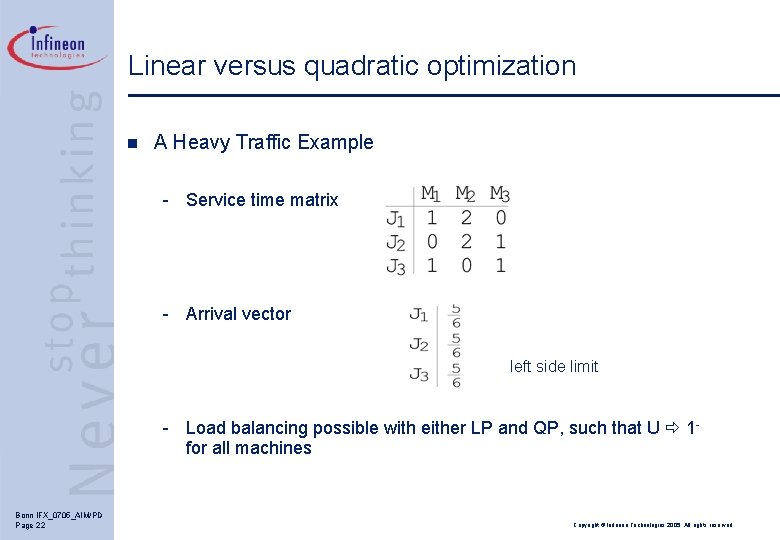
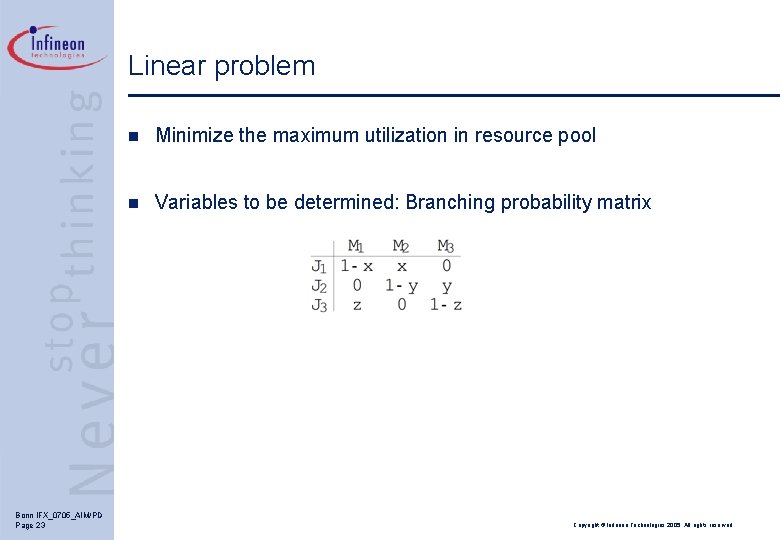
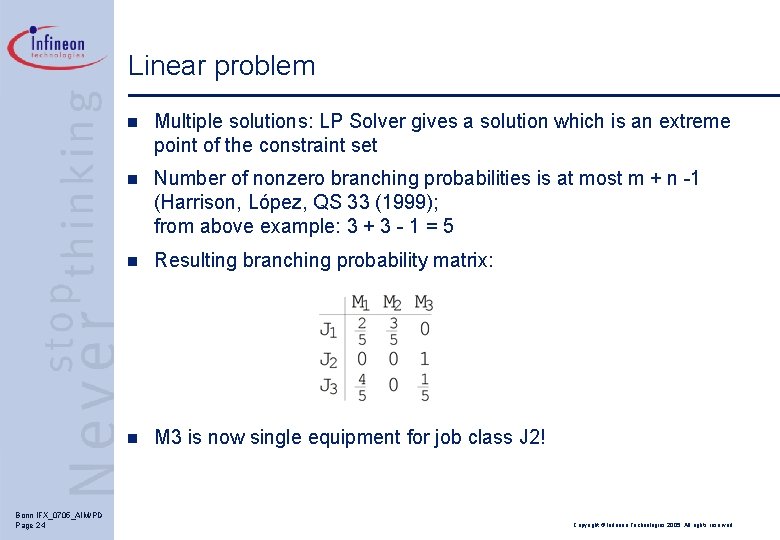
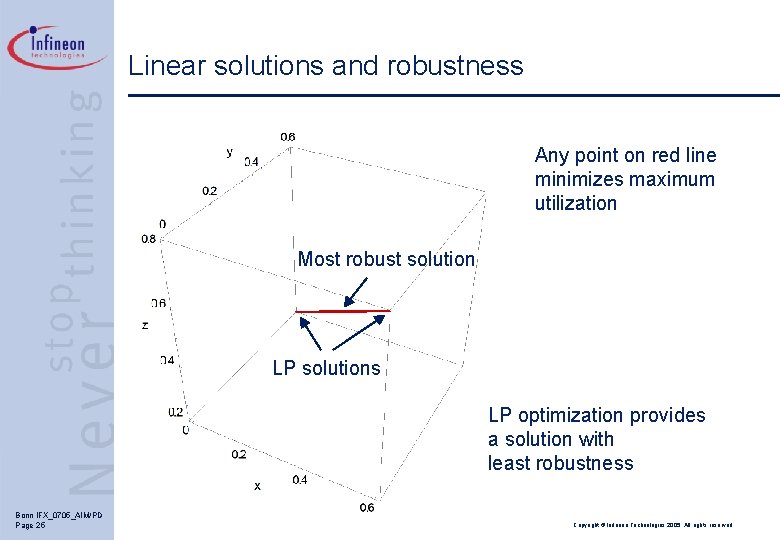
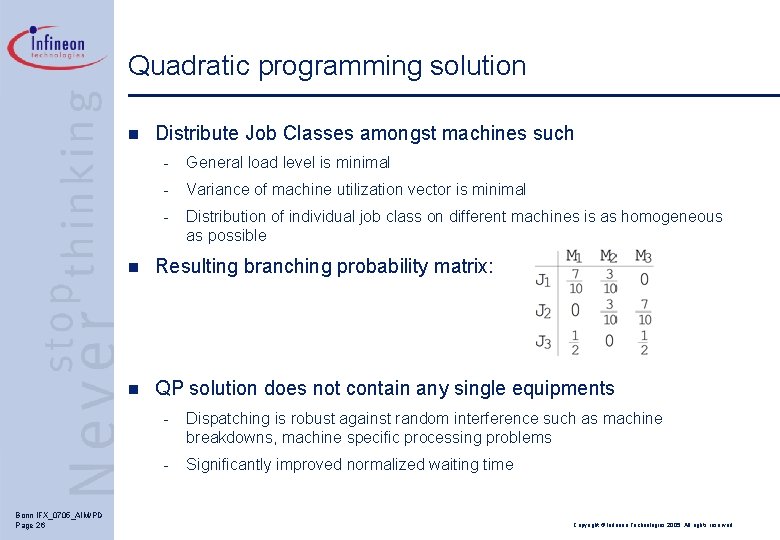
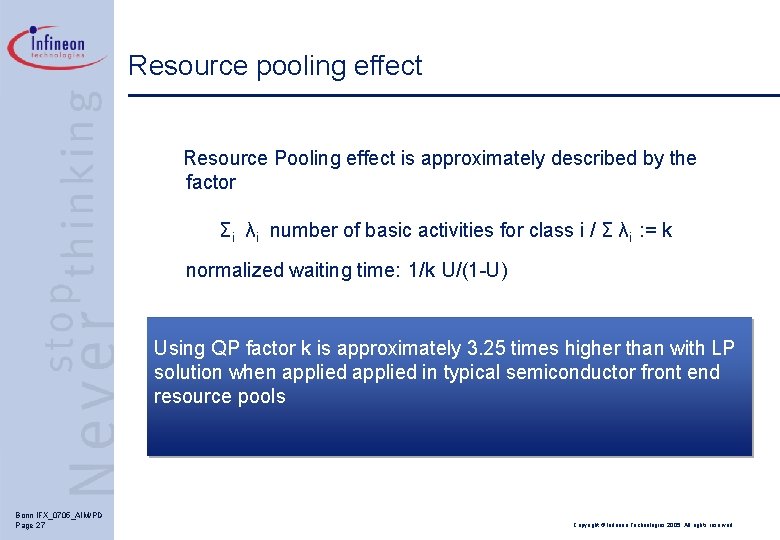
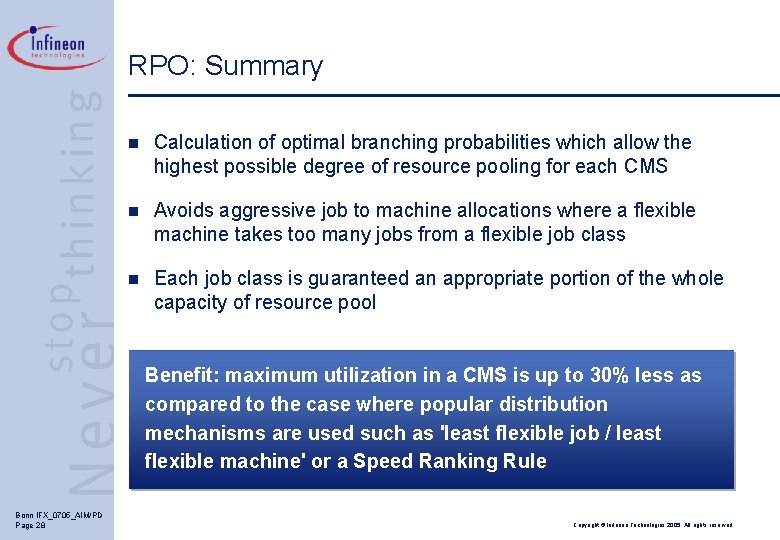
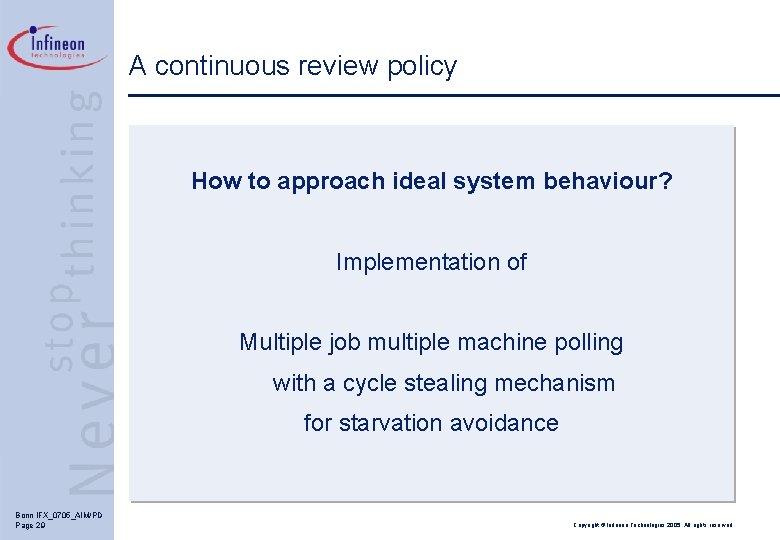
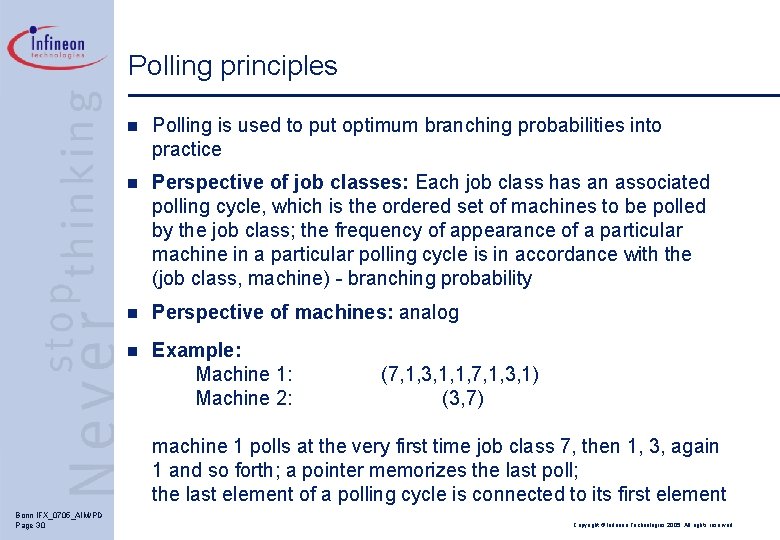
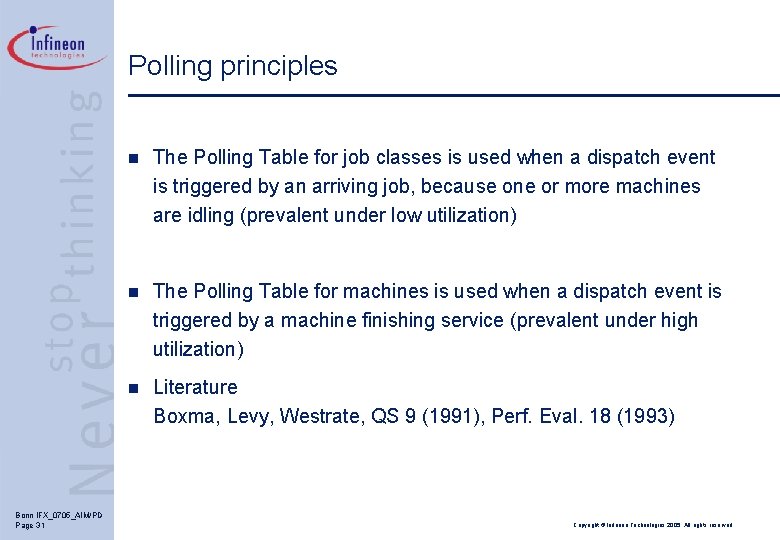
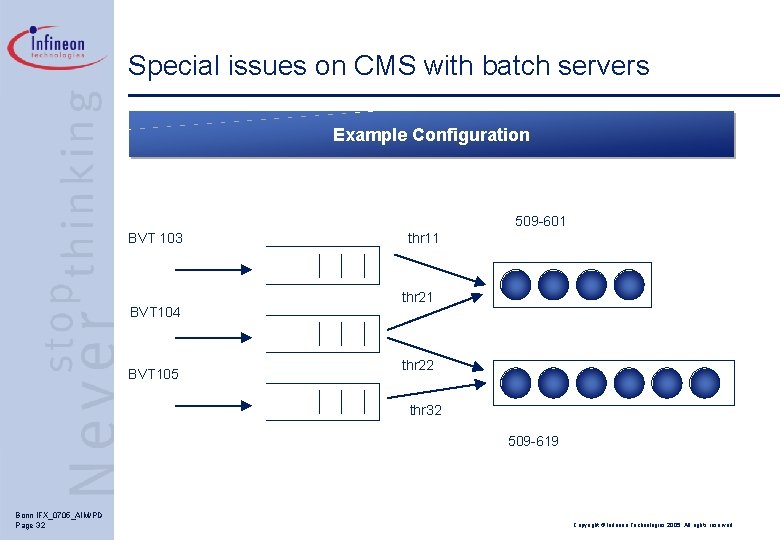
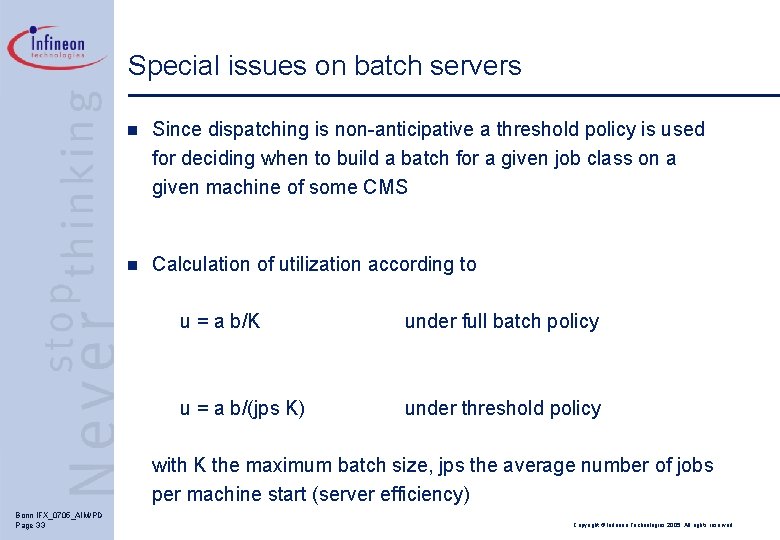
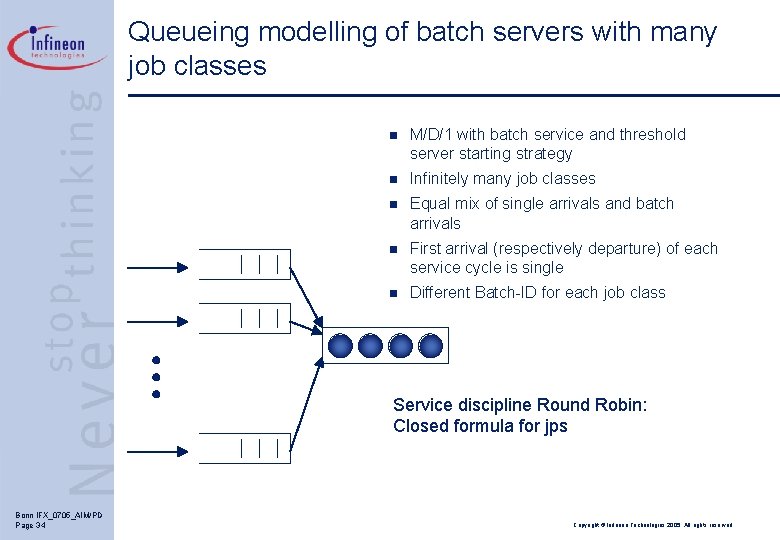
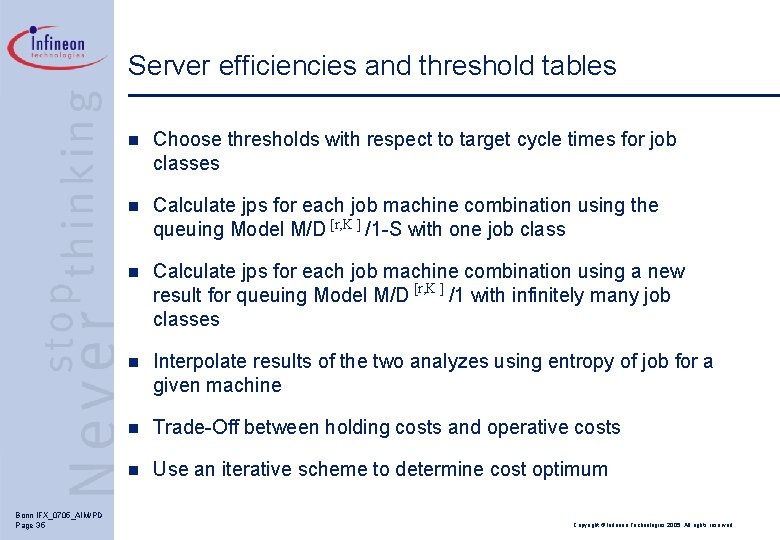
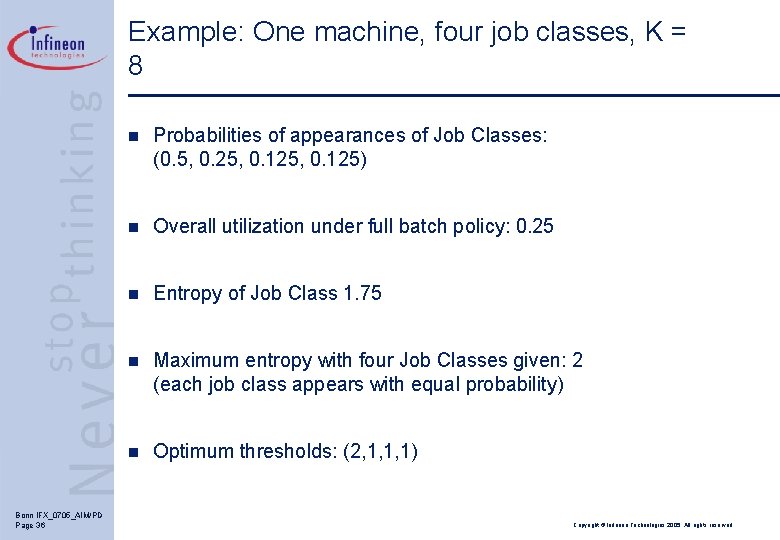
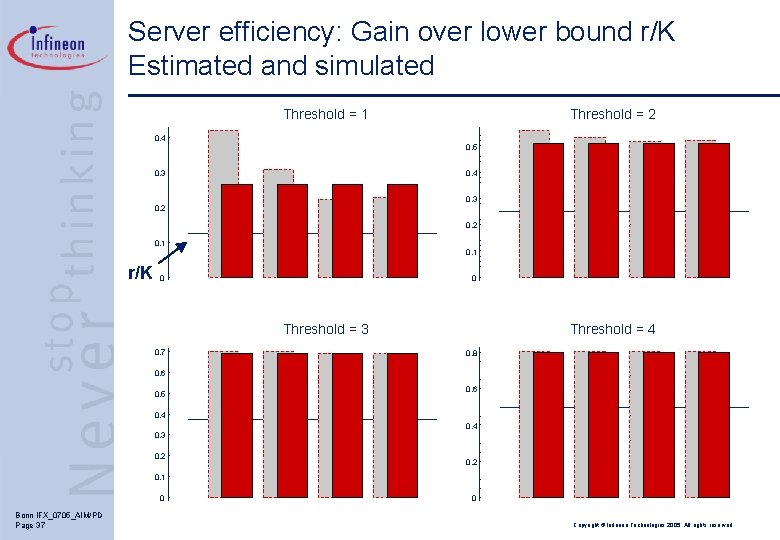
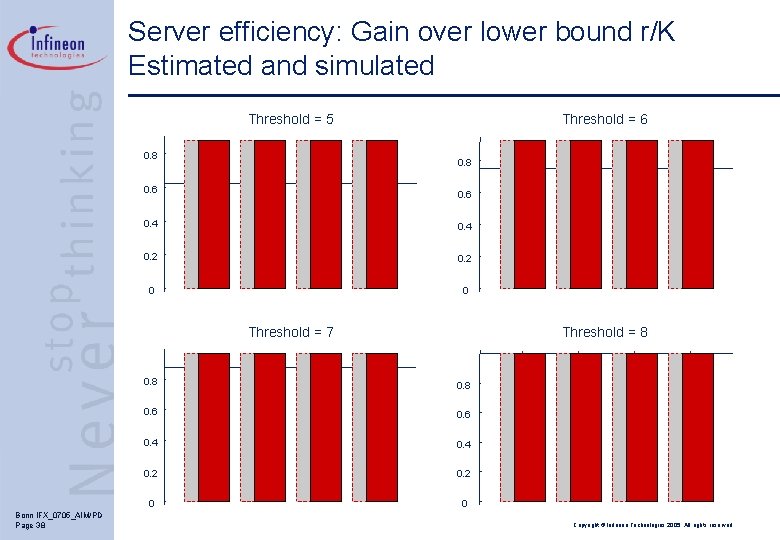
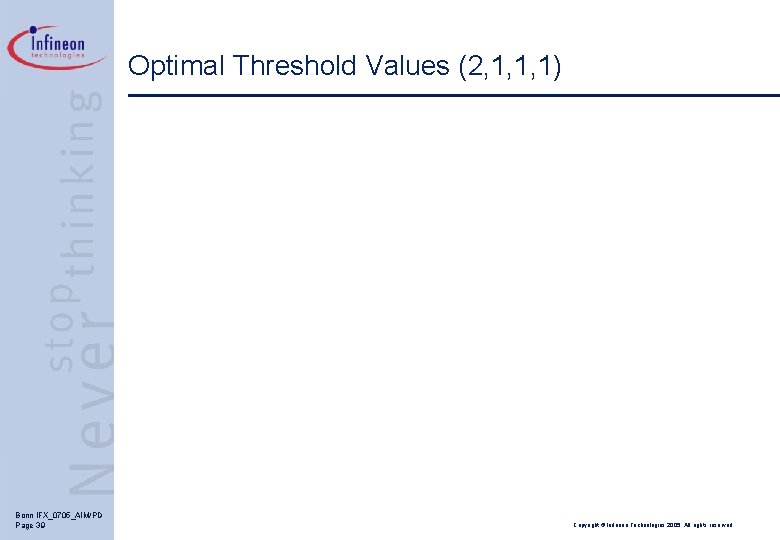
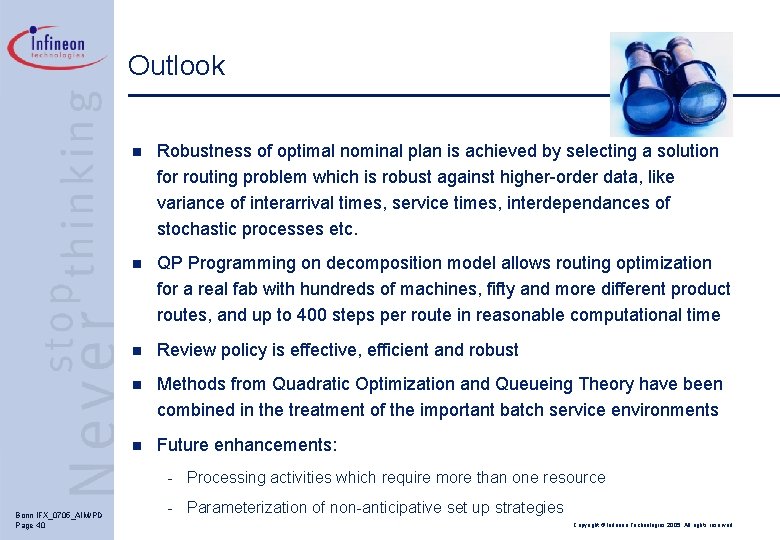
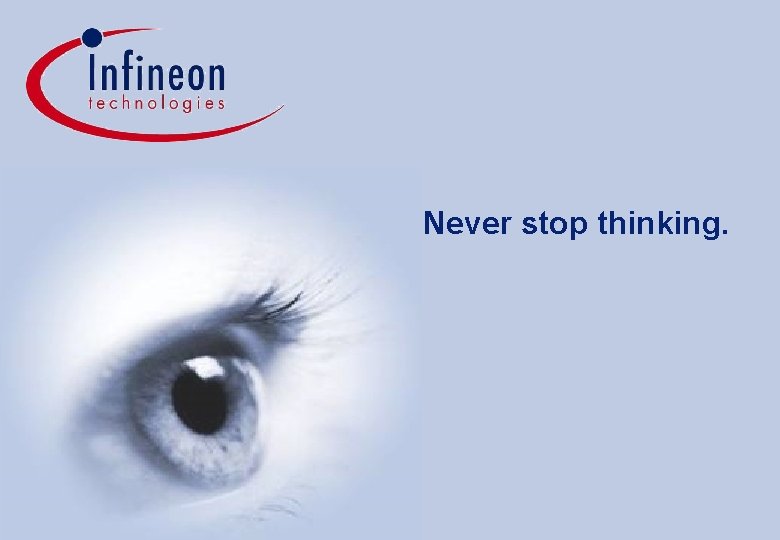
- Slides: 41
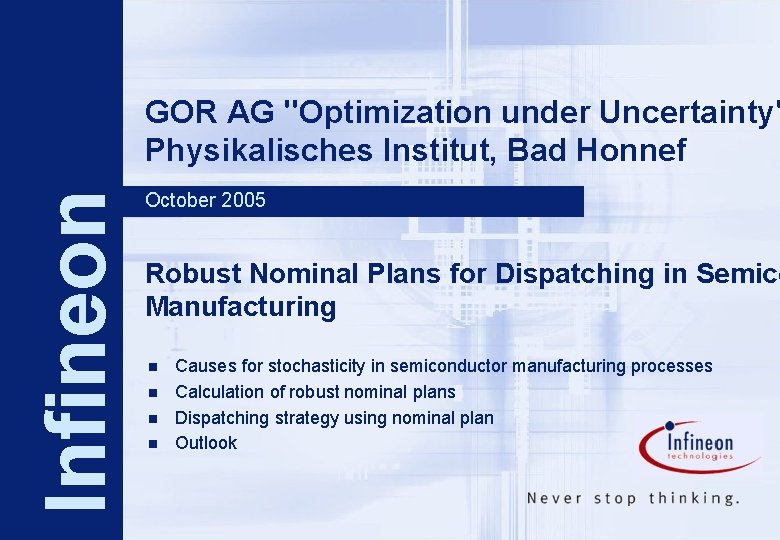
Infineon GOR AG "Optimization under Uncertainty" Physikalisches Institut, Bad Honnef Bonn IFX_0705_AIM/PD Page 1 October 2005 Robust Nominal Plans for Dispatching in Semico Manufacturing n n Causes for stochasticity in semiconductor manufacturing processes Calculation of robust nominal plans Dispatching strategy using nominal plan Outlook Copyright © Infineon Technologies 2005. All rights reserved.
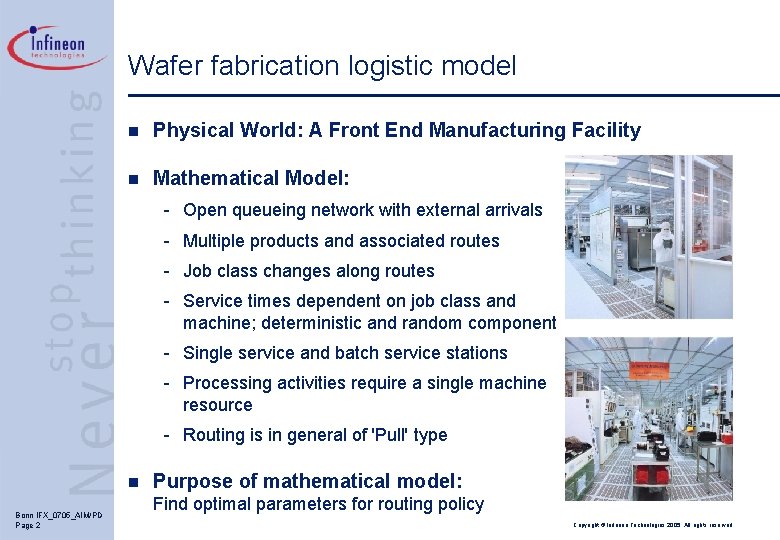
Wafer fabrication logistic model n Physical World: A Front End Manufacturing Facility n Mathematical Model: - Open queueing network with external arrivals - Multiple products and associated routes - Job class changes along routes - Service times dependent on job class and machine; deterministic and random component - Single service and batch service stations - Processing activities require a single machine resource - Routing is in general of 'Pull' type n Bonn IFX_0705_AIM/PD Page 2 Purpose of mathematical model: Find optimal parameters for routing policy Copyright © Infineon Technologies 2005. All rights reserved.
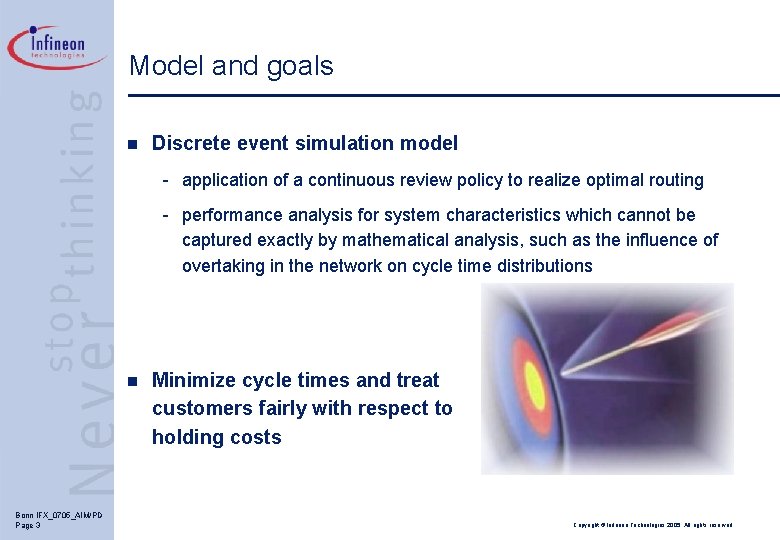
Model and goals n Discrete event simulation model - application of a continuous review policy to realize optimal routing - performance analysis for system characteristics which cannot be captured exactly by mathematical analysis, such as the influence of overtaking in the network on cycle time distributions n Bonn IFX_0705_AIM/PD Page 3 Minimize cycle times and treat customers fairly with respect to holding costs Copyright © Infineon Technologies 2005. All rights reserved.
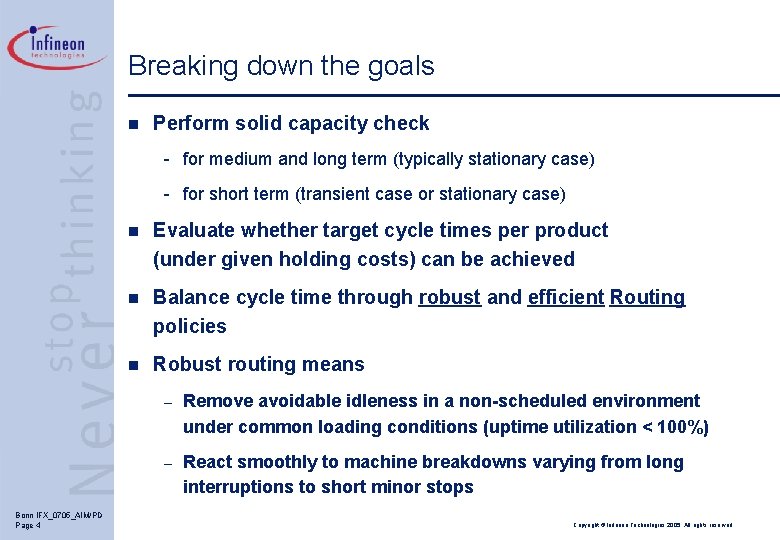
Breaking down the goals n Perform solid capacity check - for medium and long term (typically stationary case) - for short term (transient case or stationary case) Bonn IFX_0705_AIM/PD Page 4 n Evaluate whether target cycle times per product (under given holding costs) can be achieved n Balance cycle time through robust and efficient Routing policies n Robust routing means – Remove avoidable idleness in a non-scheduled environment under common loading conditions (uptime utilization < 100%) – React smoothly to machine breakdowns varying from long interruptions to short minor stops Copyright © Infineon Technologies 2005. All rights reserved.
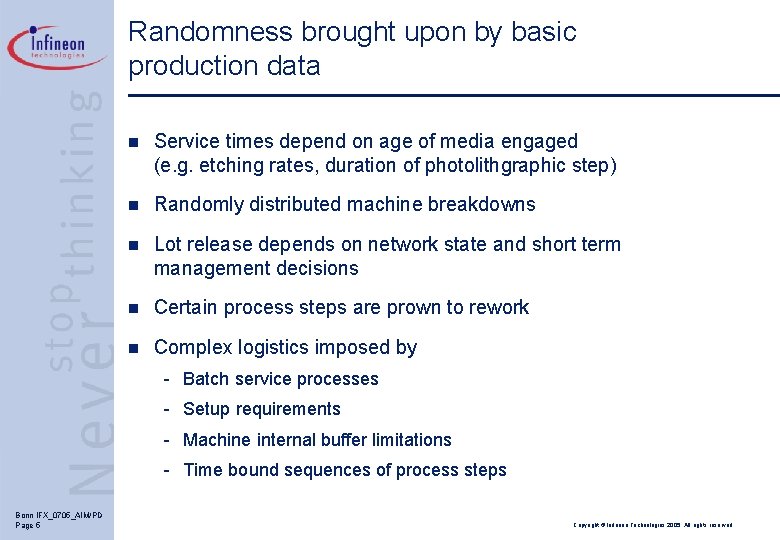
Randomness brought upon by basic production data n Service times depend on age of media engaged (e. g. etching rates, duration of photolithgraphic step) n Randomly distributed machine breakdowns n Lot release depends on network state and short term management decisions n Certain process steps are prown to rework n Complex logistics imposed by - Batch service processes - Setup requirements - Machine internal buffer limitations - Time bound sequences of process steps Bonn IFX_0705_AIM/PD Page 5 Copyright © Infineon Technologies 2005. All rights reserved.
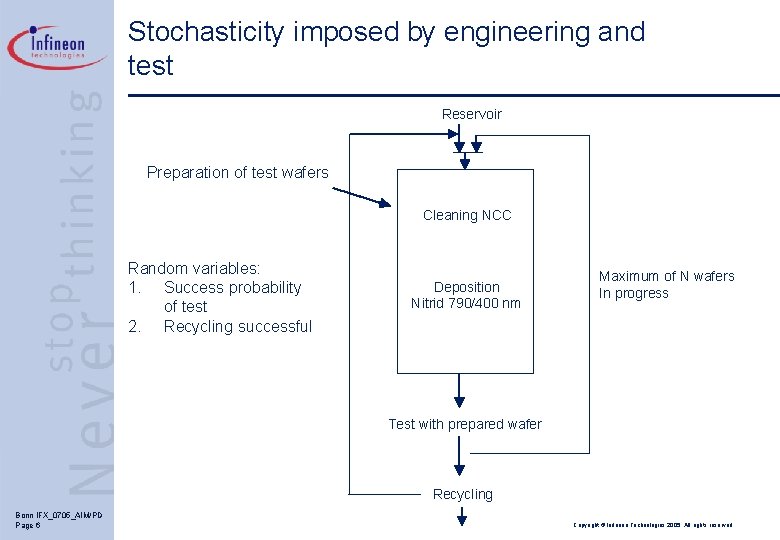
Stochasticity imposed by engineering and test Reservoir Preparation of test wafers Cleaning NCC Random variables: 1. Success probability of test 2. Recycling successful Deposition Nitrid 790/400 nm Maximum of N wafers In progress Test with prepared wafer Recycling Bonn IFX_0705_AIM/PD Page 6 Copyright © Infineon Technologies 2005. All rights reserved.
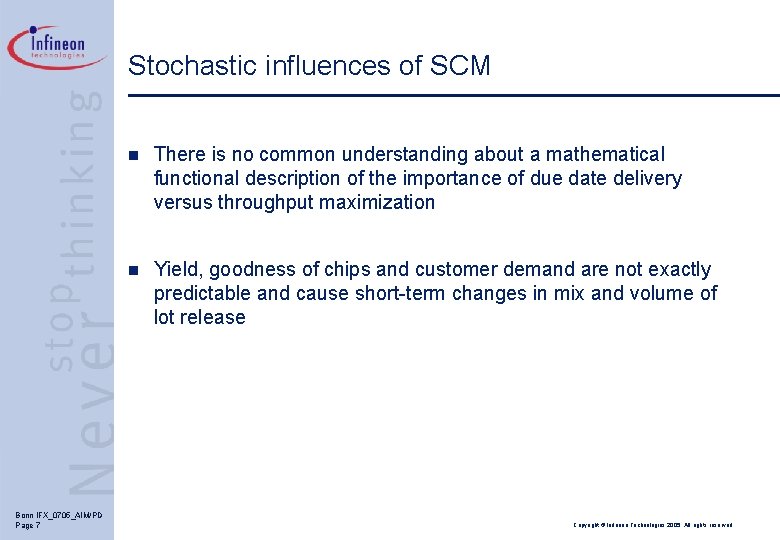
Stochastic influences of SCM Bonn IFX_0705_AIM/PD Page 7 n There is no common understanding about a mathematical functional description of the importance of due date delivery versus throughput maximization n Yield, goodness of chips and customer demand are not exactly predictable and cause short-term changes in mix and volume of lot release Copyright © Infineon Technologies 2005. All rights reserved.
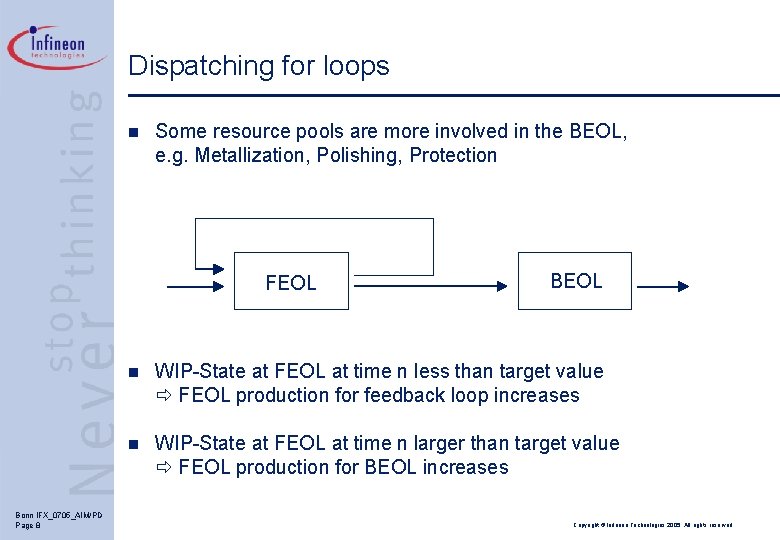
Dispatching for loops n Some resource pools are more involved in the BEOL, e. g. Metallization, Polishing, Protection FEOL Bonn IFX_0705_AIM/PD Page 8 BEOL n WIP-State at FEOL at time n less than target value FEOL production for feedback loop increases n WIP-State at FEOL at time n larger than target value FEOL production for BEOL increases Copyright © Infineon Technologies 2005. All rights reserved.
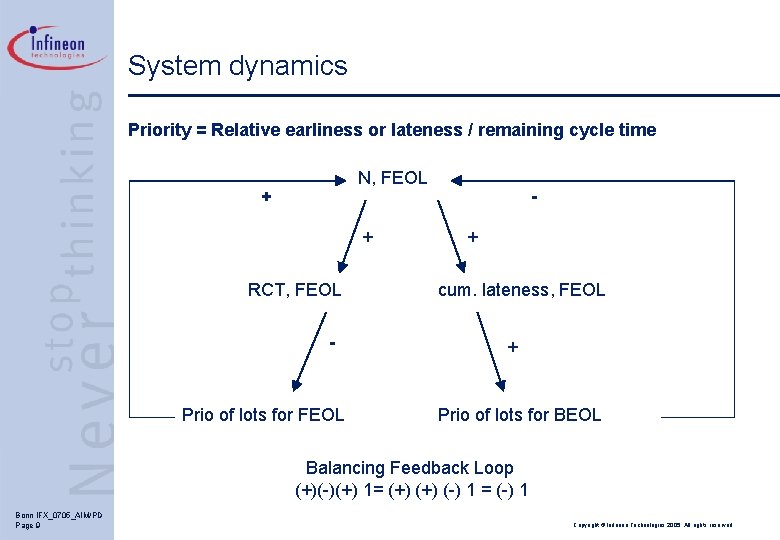
System dynamics Priority = Relative earliness or lateness / remaining cycle time N, FEOL + + RCT, FEOL - Prio of lots for FEOL + cum. lateness, FEOL + Prio of lots for BEOL Balancing Feedback Loop (+)(-)(+) 1= (+) (-) 1 = (-) 1 Bonn IFX_0705_AIM/PD Page 9 Copyright © Infineon Technologies 2005. All rights reserved.
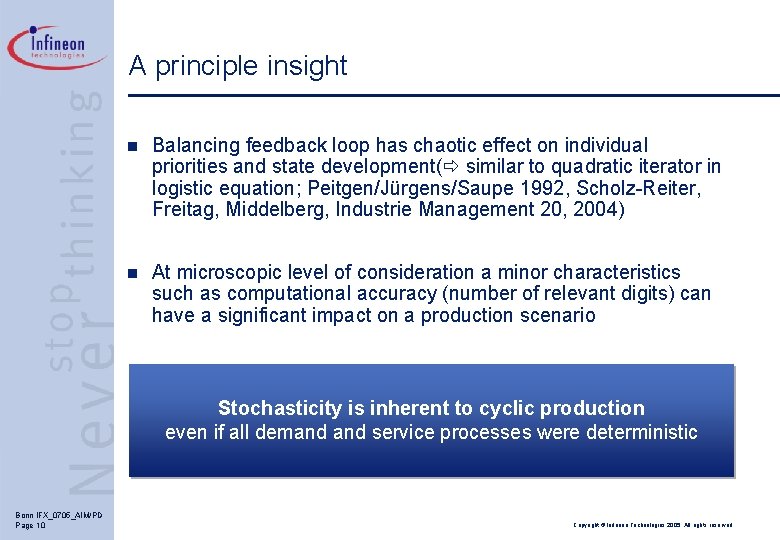
A principle insight n Balancing feedback loop has chaotic effect on individual priorities and state development( similar to quadratic iterator in logistic equation; Peitgen/Jürgens/Saupe 1992, Scholz-Reiter, Freitag, Middelberg, Industrie Management 20, 2004) n At microscopic level of consideration a minor characteristics such as computational accuracy (number of relevant digits) can have a significant impact on a production scenario Stochasticity is inherent to cyclic production even if all demand service processes were deterministic Bonn IFX_0705_AIM/PD Page 10 Copyright © Infineon Technologies 2005. All rights reserved.
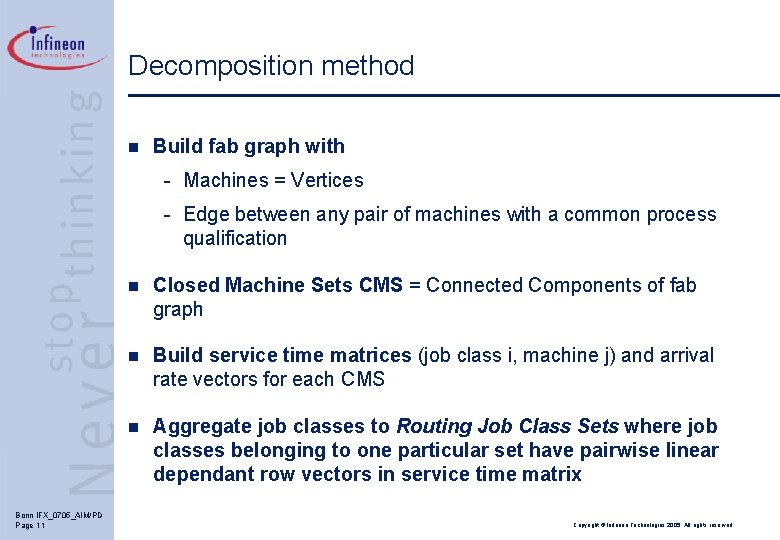
Decomposition method n Build fab graph with - Machines = Vertices - Edge between any pair of machines with a common process qualification Bonn IFX_0705_AIM/PD Page 11 n Closed Machine Sets CMS = Connected Components of fab graph n Build service time matrices (job class i, machine j) and arrival rate vectors for each CMS n Aggregate job classes to Routing Job Class Sets where job classes belonging to one particular set have pairwise linear dependant row vectors in service time matrix Copyright © Infineon Technologies 2005. All rights reserved.
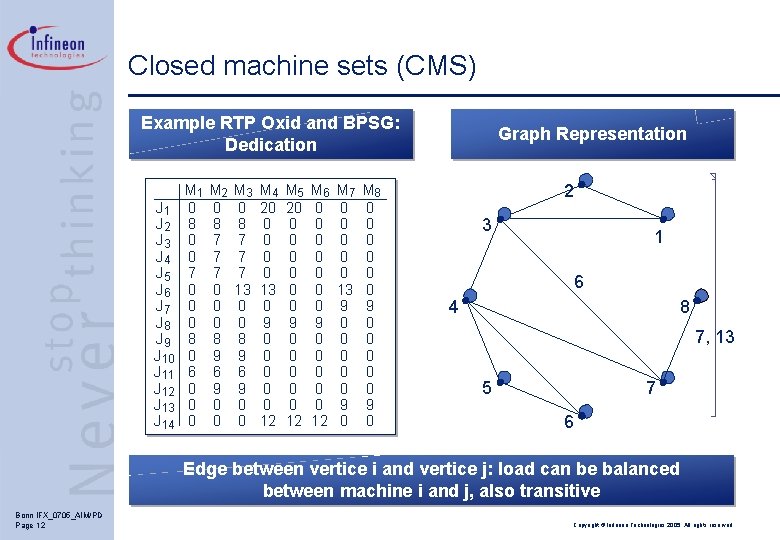
Closed machine sets (CMS) Example RTP Oxid and BPSG: Dedication J 1 J 2 J 3 J 4 J 5 J 6 J 7 J 8 J 9 J 10 J 11 J 12 J 13 J 14 M 1 0 8 0 0 7 0 0 0 8 0 6 0 0 0 M 2 0 8 7 7 7 0 0 0 8 9 6 9 0 0 M 3 0 8 7 7 7 13 0 0 8 9 6 9 0 0 M 4 20 0 0 13 0 9 0 0 0 12 M 5 20 0 0 0 9 0 0 0 12 M 6 0 0 0 0 9 0 0 0 12 M 7 0 0 0 13 9 0 0 0 9 0 M 8 0 0 0 9 0 Graph Representation 2 3 1 6 4 8 7, 13 5 7 6 Edge between vertice i and vertice j: load can be balanced between machine i and j, also transitive Bonn IFX_0705_AIM/PD Page 12 Copyright © Infineon Technologies 2005. All rights reserved.
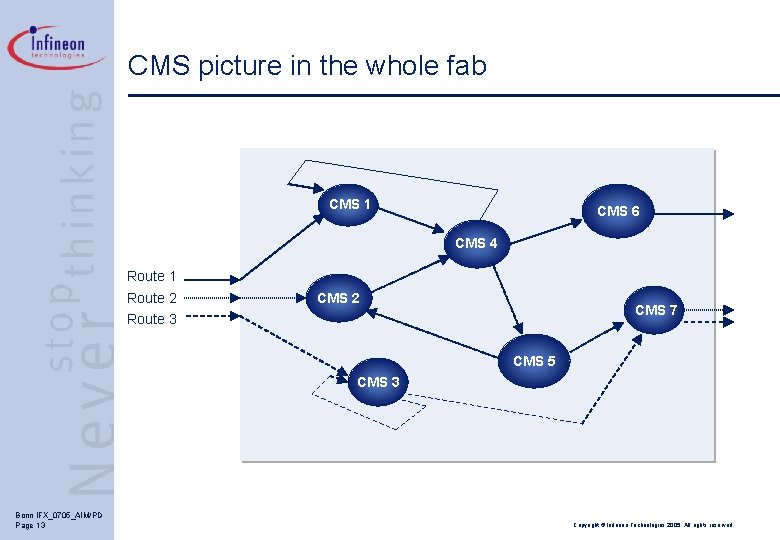
CMS picture in the whole fab CMS 1 CMS 6 CMS 4 Route 1 Route 2 CMS 7 Route 3 CMS 5 CMS 3 Bonn IFX_0705_AIM/PD Page 13 Copyright © Infineon Technologies 2005. All rights reserved.
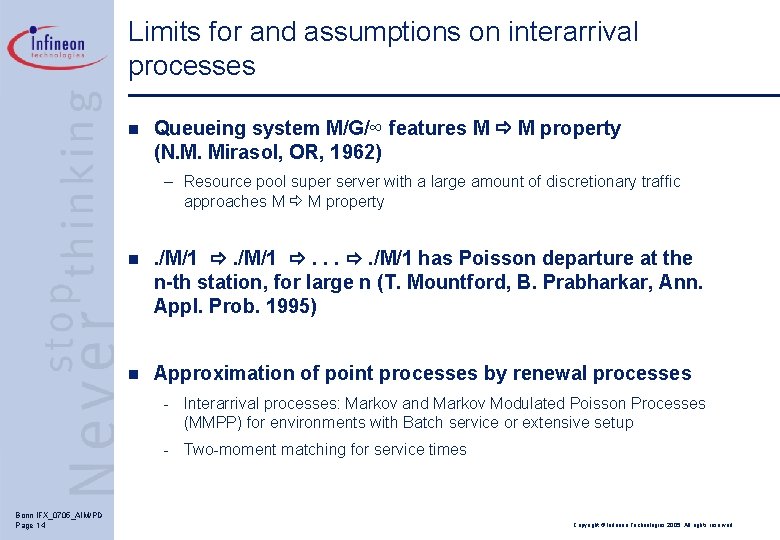
Limits for and assumptions on interarrival processes n Queueing system M/G/∞ features M M property (N. M. Mirasol, OR, 1962) – Resource pool super server with a large amount of discretionary traffic approaches M M property n . /M/1 . . . . /M/1 has Poisson departure at the n-th station, for large n (T. Mountford, B. Prabharkar, Ann. Appl. Prob. 1995) n Approximation of point processes by renewal processes - Interarrival processes: Markov and Markov Modulated Poisson Processes (MMPP) for environments with Batch service or extensive setup - Two-moment matching for service times Bonn IFX_0705_AIM/PD Page 14 Copyright © Infineon Technologies 2005. All rights reserved.
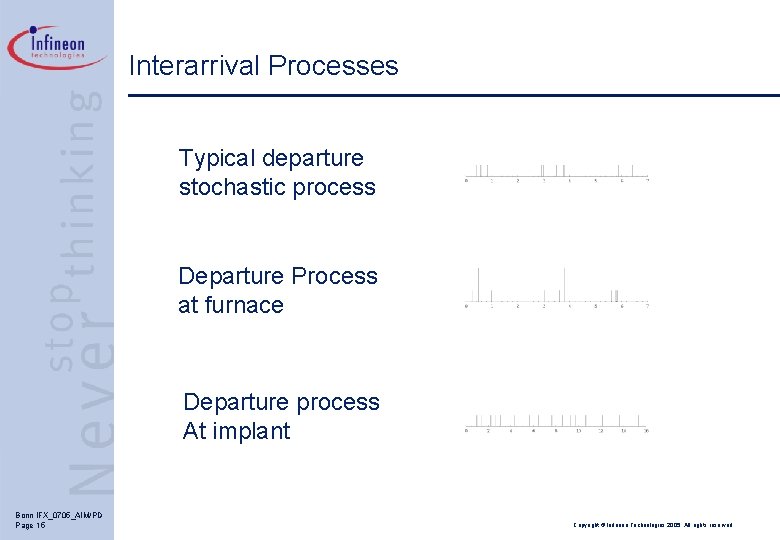
Interarrival Processes Typical departure stochastic process Departure Process at furnace Departure process At implant Bonn IFX_0705_AIM/PD Page 15 Copyright © Infineon Technologies 2005. All rights reserved.
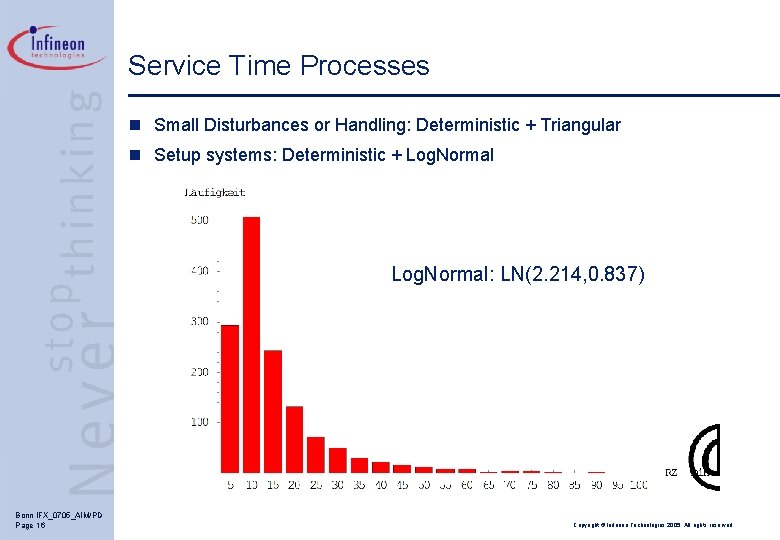
Service Time Processes n Small Disturbances or Handling: Deterministic + Triangular n Setup systems: Deterministic + Log. Normal: LN(2. 214, 0. 837) Bonn IFX_0705_AIM/PD Page 16 Copyright © Infineon Technologies 2005. All rights reserved.
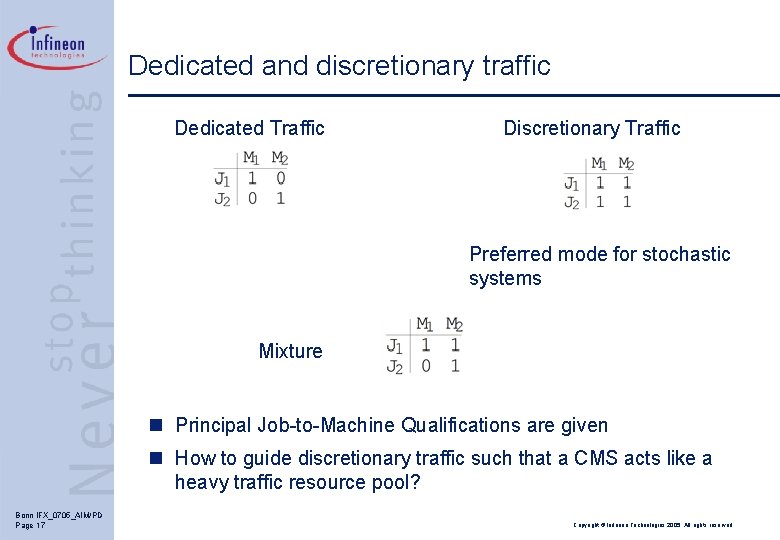
Dedicated and discretionary traffic Dedicated Traffic Discretionary Traffic Preferred mode for stochastic systems Mixture n Principal Job-to-Machine Qualifications are given n How to guide discretionary traffic such that a CMS acts like a heavy traffic resource pool? Bonn IFX_0705_AIM/PD Page 17 Copyright © Infineon Technologies 2005. All rights reserved.
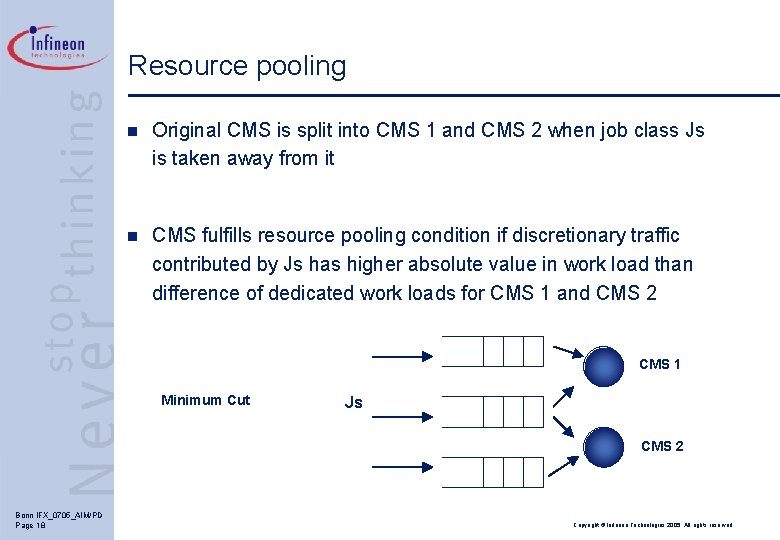
Resource pooling n Original CMS is split into CMS 1 and CMS 2 when job class Js is taken away from it n CMS fulfills resource pooling condition if discretionary traffic contributed by Js has higher absolute value in work load than difference of dedicated work loads for CMS 1 and CMS 2 CMS 1 Minimum Cut Js CMS 2 Bonn IFX_0705_AIM/PD Page 18 Copyright © Infineon Technologies 2005. All rights reserved.
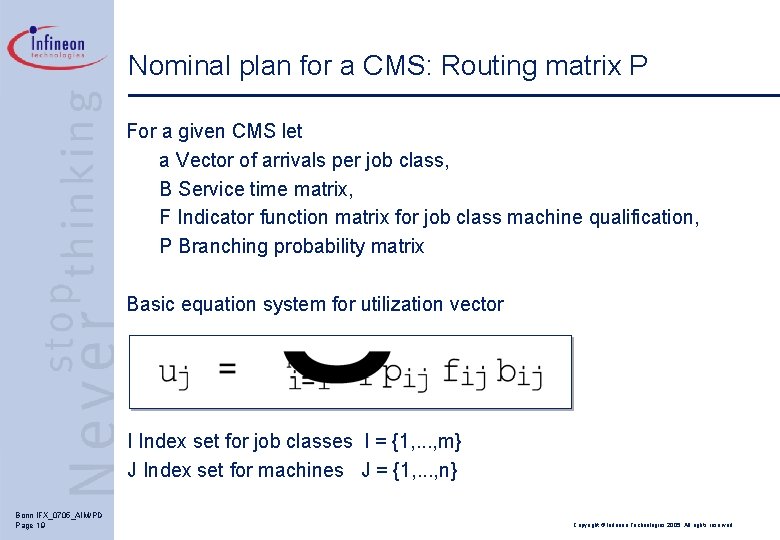
Nominal plan for a CMS: Routing matrix P For a given CMS let a Vector of arrivals per job class, B Service time matrix, F Indicator function matrix for job class machine qualification, P Branching probability matrix Basic equation system for utilization vector I Index set for job classes I = {1, . . . , m} J Index set for machines J = {1, . . . , n} Bonn IFX_0705_AIM/PD Page 19 Copyright © Infineon Technologies 2005. All rights reserved.
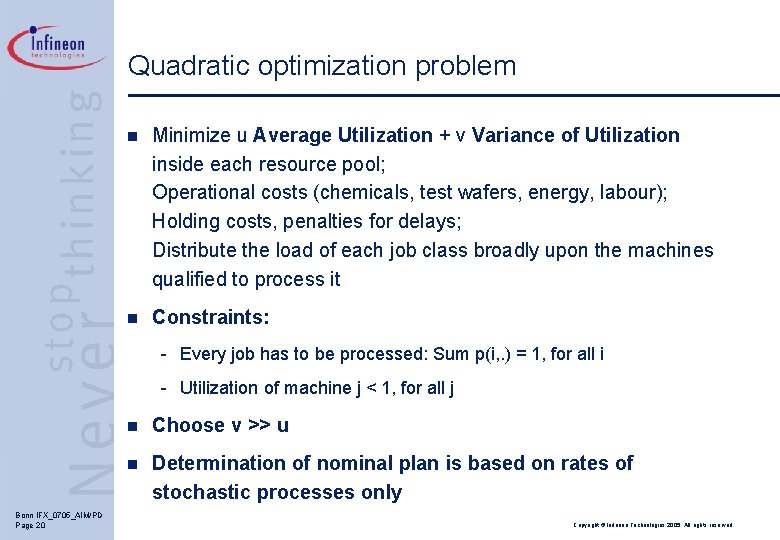
Quadratic optimization problem n Minimize u Average Utilization + v Variance of Utilization inside each resource pool; Operational costs (chemicals, test wafers, energy, labour); Holding costs, penalties for delays; Distribute the load of each job class broadly upon the machines qualified to process it n Constraints: - Every job has to be processed: Sum p(i, . ) = 1, for all i - Utilization of machine j < 1, for all j Bonn IFX_0705_AIM/PD Page 20 n Choose v >> u n Determination of nominal plan is based on rates of stochastic processes only Copyright © Infineon Technologies 2005. All rights reserved.
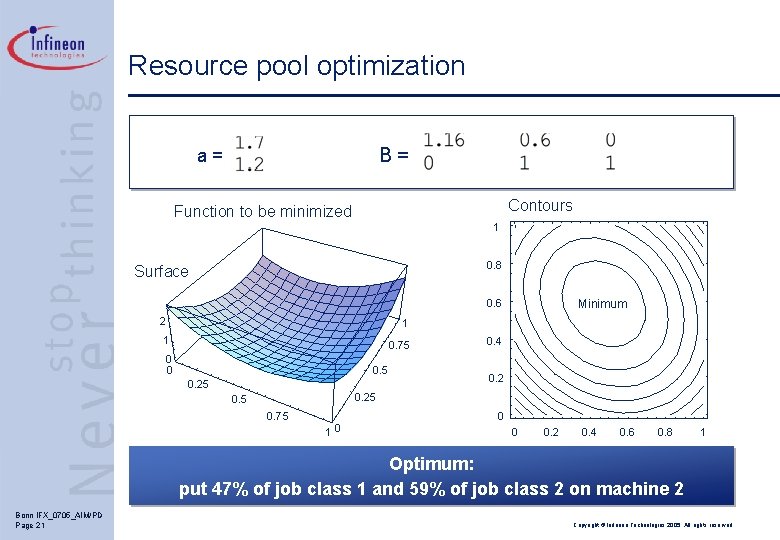
Resource pool optimization B= a= Contours Function to be minimized 1 0. 8 Surface 0. 6 2 Minimum 1 1 0. 75 0 0 0. 5 0. 25 0. 4 0. 25 0. 75 10 0 0 0. 2 0. 4 0. 6 0. 8 1 Optimum: put 47% of job class 1 and 59% of job class 2 on machine 2 Bonn IFX_0705_AIM/PD Page 21 Copyright © Infineon Technologies 2005. All rights reserved.
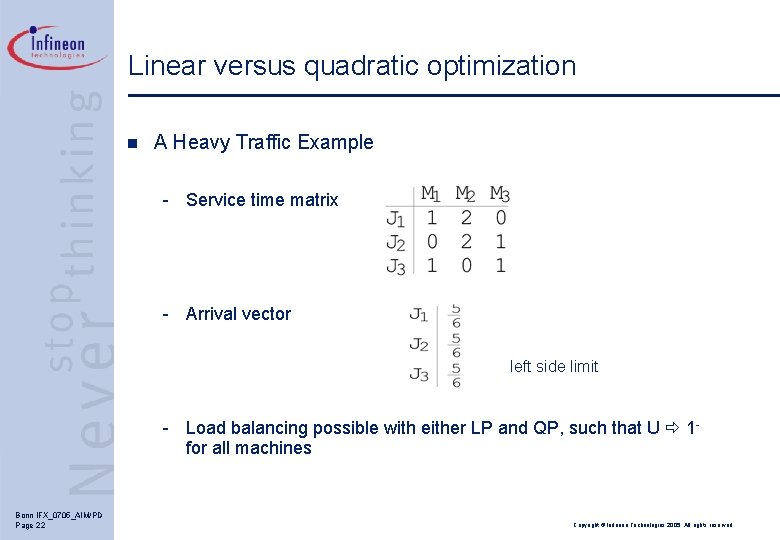
Linear versus quadratic optimization n A Heavy Traffic Example - Service time matrix - Arrival vector left side limit - Load balancing possible with either LP and QP, such that U 1 for all machines Bonn IFX_0705_AIM/PD Page 22 Copyright © Infineon Technologies 2005. All rights reserved.
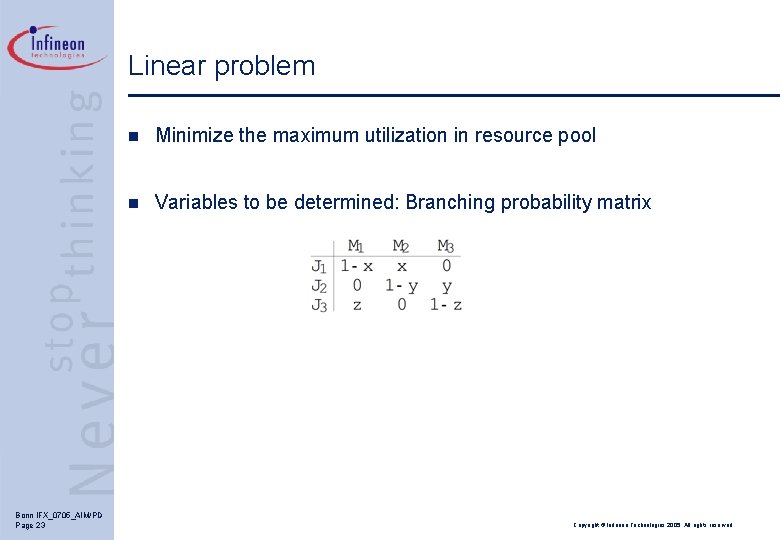
Linear problem Bonn IFX_0705_AIM/PD Page 23 n Minimize the maximum utilization in resource pool n Variables to be determined: Branching probability matrix Copyright © Infineon Technologies 2005. All rights reserved.
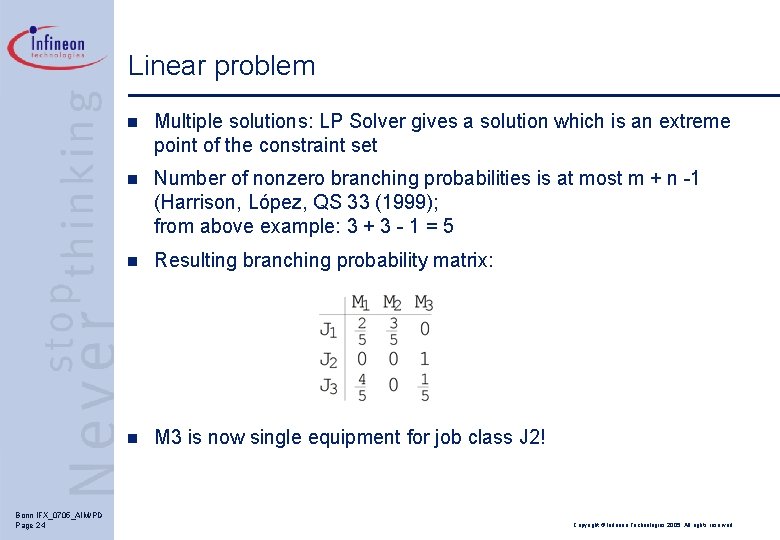
Linear problem Bonn IFX_0705_AIM/PD Page 24 n Multiple solutions: LP Solver gives a solution which is an extreme point of the constraint set n Number of nonzero branching probabilities is at most m + n -1 (Harrison, López, QS 33 (1999); from above example: 3 + 3 - 1 = 5 n Resulting branching probability matrix: n M 3 is now single equipment for job class J 2! Copyright © Infineon Technologies 2005. All rights reserved.
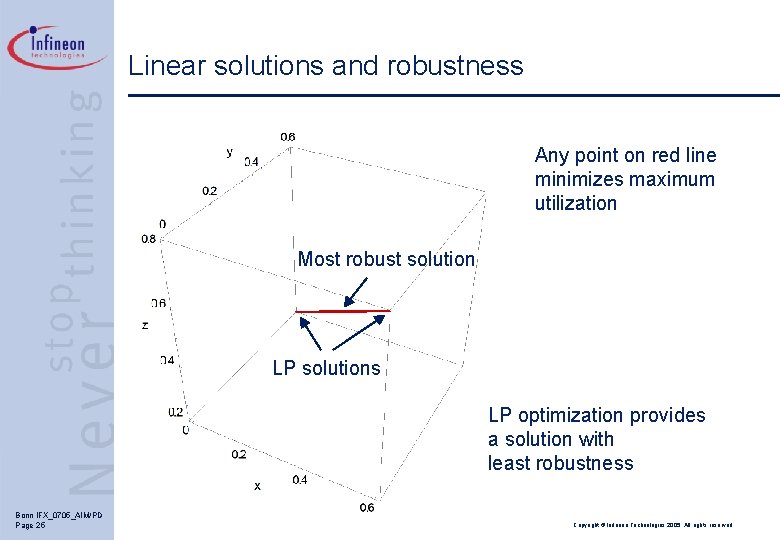
Linear solutions and robustness Any point on red line minimizes maximum utilization Most robust solution Robust solution LP solutions LP optimization provides a solution with least robustness Bonn IFX_0705_AIM/PD Page 25 Copyright © Infineon Technologies 2005. All rights reserved.
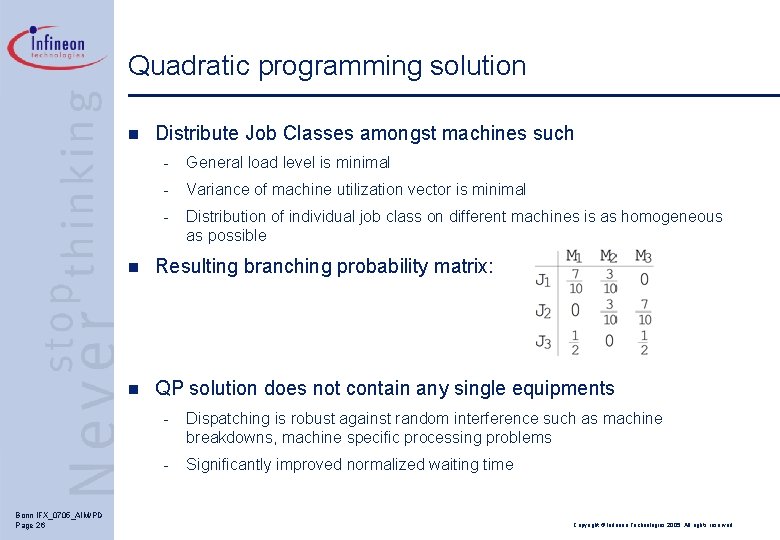
Quadratic programming solution n Bonn IFX_0705_AIM/PD Page 26 Distribute Job Classes amongst machines such - General load level is minimal - Variance of machine utilization vector is minimal - Distribution of individual job class on different machines is as homogeneous as possible n Resulting branching probability matrix: n QP solution does not contain any single equipments - Dispatching is robust against random interference such as machine breakdowns, machine specific processing problems - Significantly improved normalized waiting time Copyright © Infineon Technologies 2005. All rights reserved.
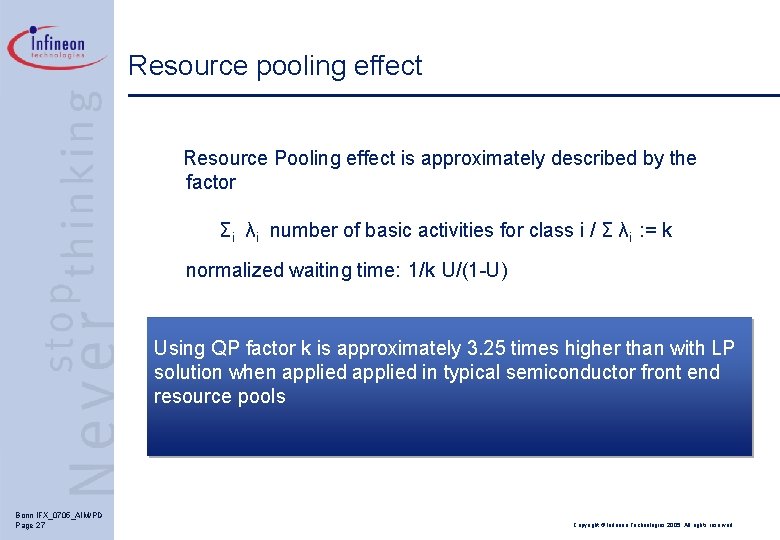
Resource pooling effect Resource Pooling effect is approximately described by the factor Σi λi number of basic activities for class i / Σ λi : = k normalized waiting time: 1/k U/(1 -U) Using QP factor k is approximately 3. 25 times higher than with LP solution when applied in typical semiconductor front end resource pools Bonn IFX_0705_AIM/PD Page 27 Copyright © Infineon Technologies 2005. All rights reserved.
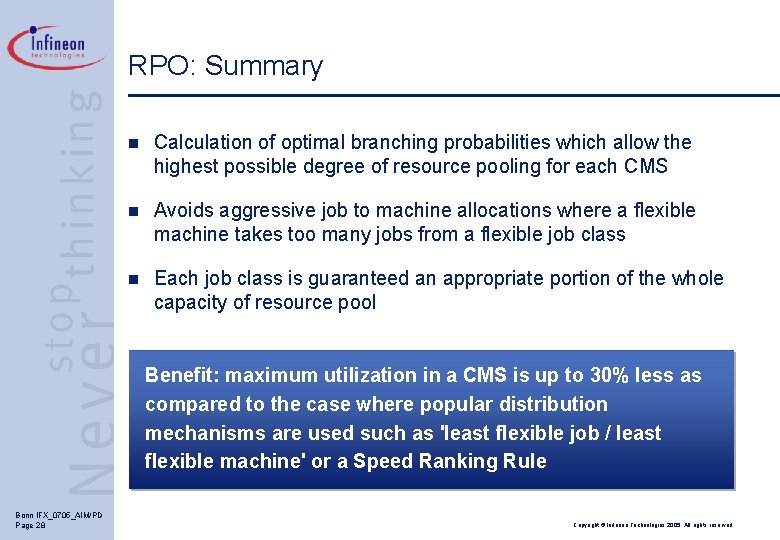
RPO: Summary n Calculation of optimal branching probabilities which allow the highest possible degree of resource pooling for each CMS n Avoids aggressive job to machine allocations where a flexible machine takes too many jobs from a flexible job class n Each job class is guaranteed an appropriate portion of the whole capacity of resource pool Benefit: maximum utilization in a CMS is up to 30% less as compared to the case where popular distribution mechanisms are used such as 'least flexible job / least flexible machine' or a Speed Ranking Rule Bonn IFX_0705_AIM/PD Page 28 Copyright © Infineon Technologies 2005. All rights reserved.
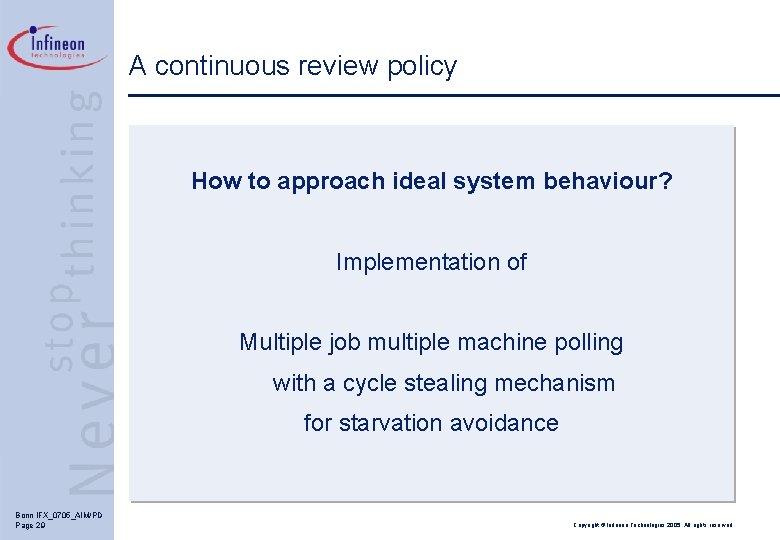
A continuous review policy How to approach ideal system behaviour? Implementation of Multiple job multiple machine polling with a cycle stealing mechanism for starvation avoidance Bonn IFX_0705_AIM/PD Page 29 Copyright © Infineon Technologies 2005. All rights reserved.
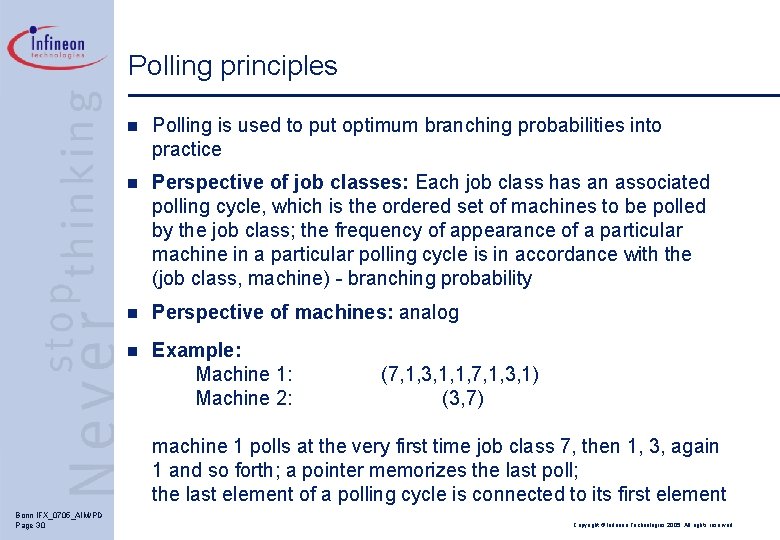
Polling principles n Polling is used to put optimum branching probabilities into practice n Perspective of job classes: Each job class has an associated polling cycle, which is the ordered set of machines to be polled by the job class; the frequency of appearance of a particular machine in a particular polling cycle is in accordance with the (job class, machine) - branching probability n Perspective of machines: analog n Example: Machine 1: Machine 2: (7, 1, 3, 1, 1, 7, 1, 3, 1) (3, 7) machine 1 polls at the very first time job class 7, then 1, 3, again 1 and so forth; a pointer memorizes the last poll; the last element of a polling cycle is connected to its first element Bonn IFX_0705_AIM/PD Page 30 Copyright © Infineon Technologies 2005. All rights reserved.
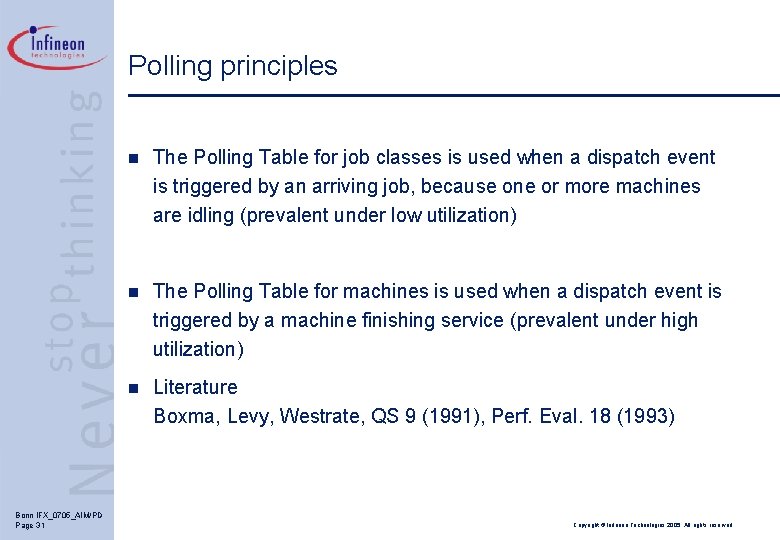
Polling principles Bonn IFX_0705_AIM/PD Page 31 n The Polling Table for job classes is used when a dispatch event is triggered by an arriving job, because one or more machines are idling (prevalent under low utilization) n The Polling Table for machines is used when a dispatch event is triggered by a machine finishing service (prevalent under high utilization) n Literature Boxma, Levy, Westrate, QS 9 (1991), Perf. Eval. 18 (1993) Copyright © Infineon Technologies 2005. All rights reserved.
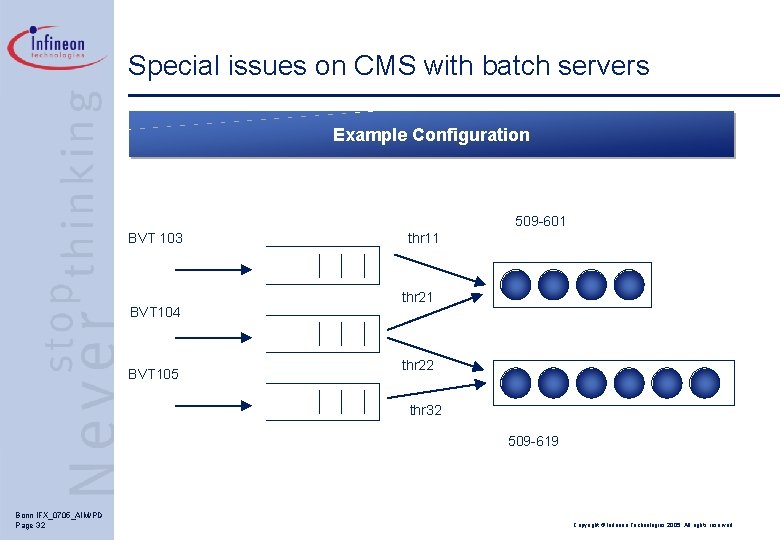
Special issues on CMS with batch servers Example Configuration 509 -601 BVT 103 BVT 104 BVT 105 thr 11 thr 22 thr 32 509 -619 Bonn IFX_0705_AIM/PD Page 32 Copyright © Infineon Technologies 2005. All rights reserved.
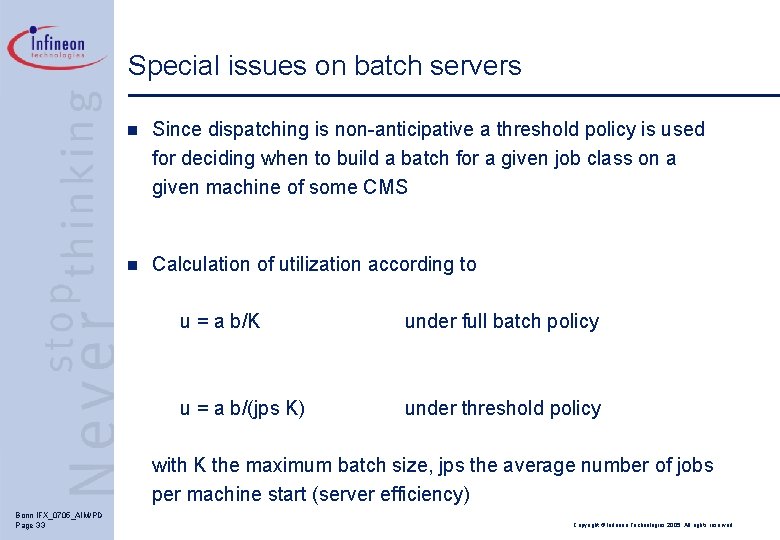
Special issues on batch servers n Since dispatching is non-anticipative a threshold policy is used for deciding when to build a batch for a given job class on a given machine of some CMS n Calculation of utilization according to u = a b/K under full batch policy u = a b/(jps K) under threshold policy with K the maximum batch size, jps the average number of jobs per machine start (server efficiency) Bonn IFX_0705_AIM/PD Page 33 Copyright © Infineon Technologies 2005. All rights reserved.
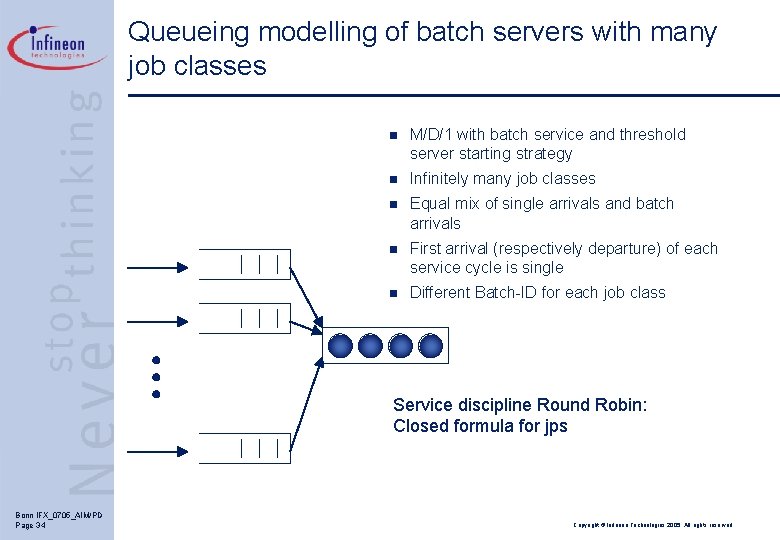
Queueing modelling of batch servers with many job classes n M/D/1 with batch service and threshold server starting strategy n Infinitely many job classes n Equal mix of single arrivals and batch arrivals n First arrival (respectively departure) of each service cycle is single n Different Batch-ID for each job class Service discipline Round Robin: Closed formula for jps Bonn IFX_0705_AIM/PD Page 34 Copyright © Infineon Technologies 2005. All rights reserved.
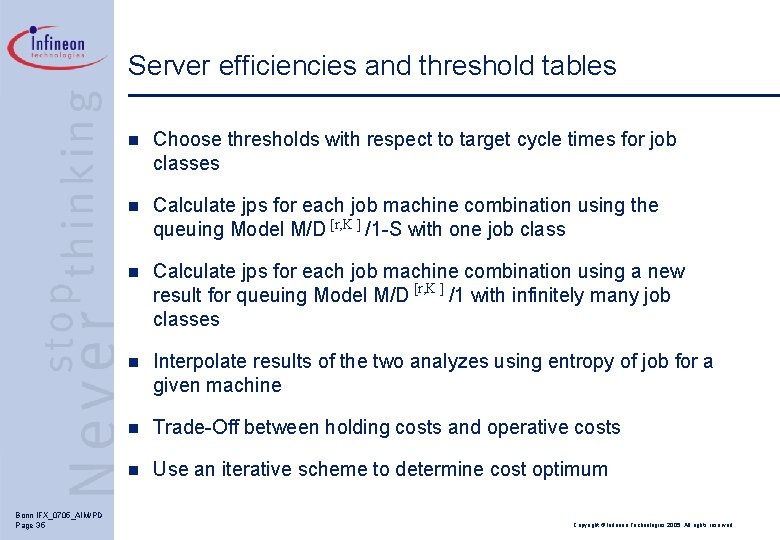
Server efficiencies and threshold tables Bonn IFX_0705_AIM/PD Page 35 n Choose thresholds with respect to target cycle times for job classes n Calculate jps for each job machine combination using the queuing Model M/D [r, K ] /1 -S with one job class n Calculate jps for each job machine combination using a new result for queuing Model M/D [r, K ] /1 with infinitely many job classes n Interpolate results of the two analyzes using entropy of job for a given machine n Trade-Off between holding costs and operative costs n Use an iterative scheme to determine cost optimum Copyright © Infineon Technologies 2005. All rights reserved.
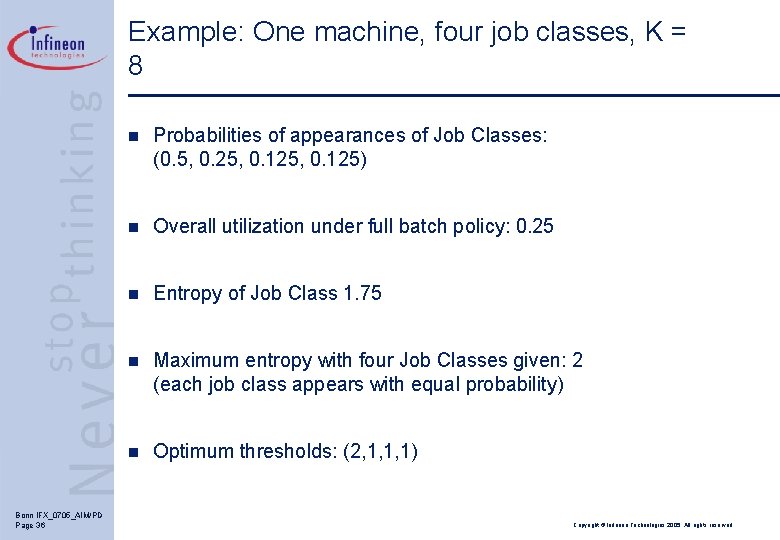
Example: One machine, four job classes, K = 8 Bonn IFX_0705_AIM/PD Page 36 n Probabilities of appearances of Job Classes: (0. 5, 0. 25, 0. 125) n Overall utilization under full batch policy: 0. 25 n Entropy of Job Class 1. 75 n Maximum entropy with four Job Classes given: 2 (each job class appears with equal probability) n Optimum thresholds: (2, 1, 1, 1) Copyright © Infineon Technologies 2005. All rights reserved.
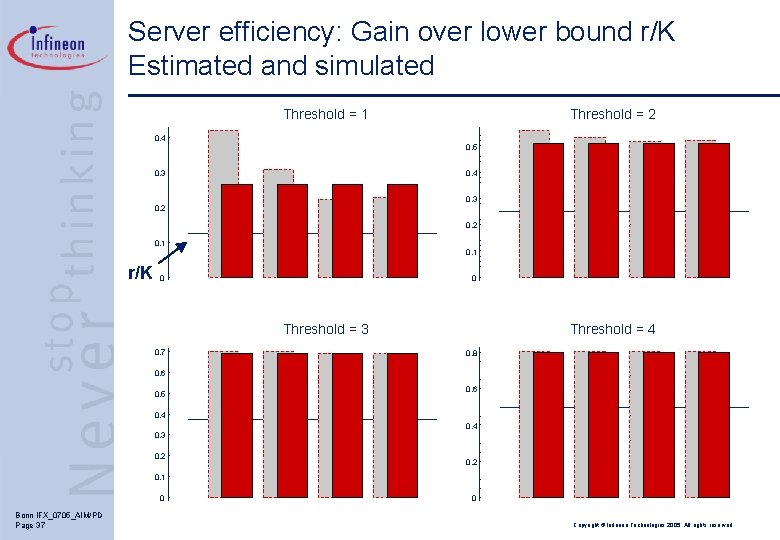
Server efficiency: Gain over lower bound r/K Estimated and simulated Threshold = 1 Threshold = 2 0. 4 0. 5 0. 3 0. 4 0. 3 0. 2 0. 1 r/K Class 1 Class 2 Class 3 Class 4 0. 1 0 0 Threshold = 3 Threshold = 4 0. 7 0. 8 0. 6 0. 5 Class 1 0. 4 0. 3 0. 2 Class 1 Class 2 Class 3 Class 4 0. 2 0. 1 0 Bonn IFX_0705_AIM/PD Page 37 0 Copyright © Infineon Technologies 2005. All rights reserved.
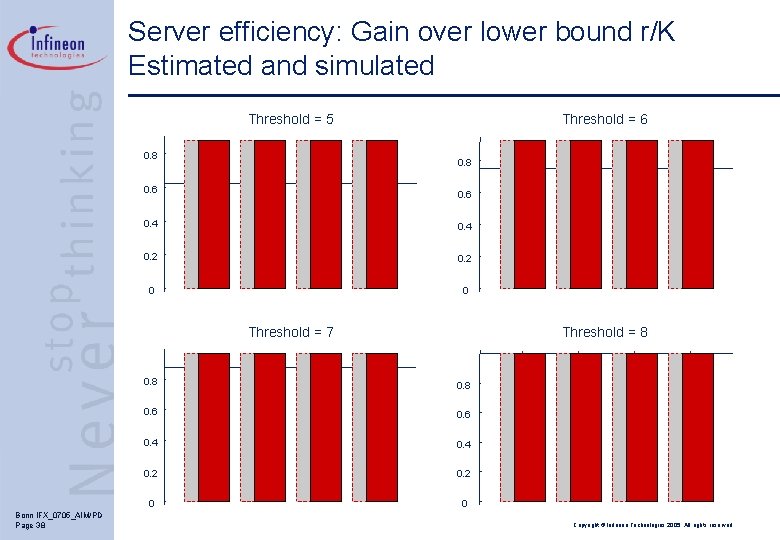
Server efficiency: Gain over lower bound r/K Estimated and simulated Threshold = 5 Threshold = 6 0. 8 Class 1 0. 6 Class 1 Class 2 Class 3 Class 4 0. 2 0 0 Threshold = 7 0. 8 Bonn IFX_0705_AIM/PD Page 38 Class 3 Class 4 Threshold = 8 Class 1 Class 2 Class 3 0. 6 0. 4 Class 1 Class 2 Class 4 Class 2 Class 3 Class 4 0. 8 0. 6 0. 4 0. 2 0 0 Copyright © Infineon Technologies 2005. All rights reserved.
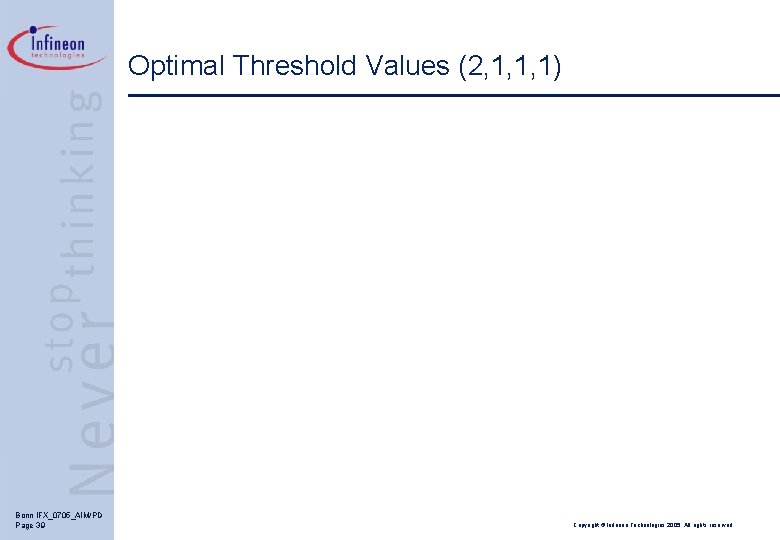
Optimal Threshold Values (2, 1, 1, 1) Bonn IFX_0705_AIM/PD Page 39 Copyright © Infineon Technologies 2005. All rights reserved.
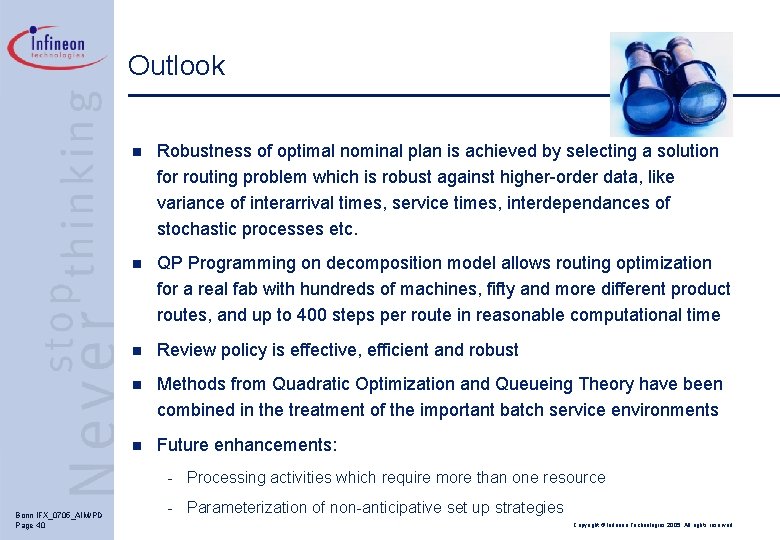
Outlook n Robustness of optimal nominal plan is achieved by selecting a solution for routing problem which is robust against higher-order data, like variance of interarrival times, service times, interdependances of stochastic processes etc. n QP Programming on decomposition model allows routing optimization for a real fab with hundreds of machines, fifty and more different product routes, and up to 400 steps per route in reasonable computational time n Review policy is effective, efficient and robust n Methods from Quadratic Optimization and Queueing Theory have been combined in the treatment of the important batch service environments n Future enhancements: - Processing activities which require more than one resource Bonn IFX_0705_AIM/PD Page 40 - Parameterization of non-anticipative set up strategies Copyright © Infineon Technologies 2005. All rights reserved.
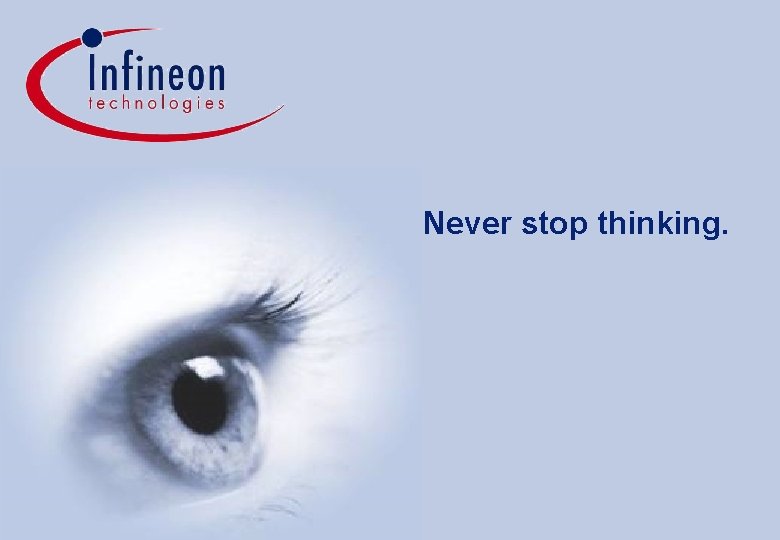
Never stop thinking. Bonn IFX_0705_AIM/PD Page 41 Copyright © Infineon Technologies 2005. All rights reserved.
Kolloquium heidelberg
Roca rsa
Infineon pronunciation
Infineon technologies romania
Holding current and latching current
Infineon technologies dresden
Infineon training
Decision-making under uncertainty
Capital budgeting under risk and uncertainty
Symbolic reasoning under uncertainty
Expected profit under uncertainty
Decision-making under uncertainty
Trepunktsperspektiv
Karolina watkowska nago
Vertrebral
Vad gör unesco
Hoppe hare text
Gor-ag
Informerande tal exempel ämne
Regeringsformen sammanfattning
Dextrosol kalorier
Bildanalys frågor
Kvantitativ intervju
Logo segoro amarto
Vad gör en fogare
Milosc twa glebsza niz ocean bez dna
Kirchhoff institut für physik
Ins pere borrell
Institut za reumatologiju
Institut za javno zdravlje beograd
Institut for uddannelse og pædagogik
Hessisches kultusministerium
Michael hauke
Gestin institut roquetes
Institut za javno zdravlje kragujevac
Gestin institut roquetes
Institut la guineueta
Kategori aset alih
Barbora vincurova
Psychologisches institut mainz
Institut igh d.d.
Ifak institut gmbh & co. kg