Chapter 16 Quantitative Data Analysis Copyright 2018 Elsevier
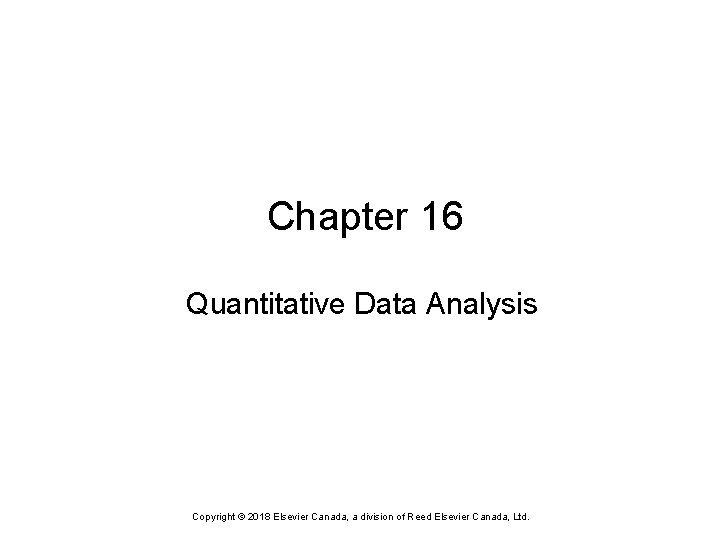
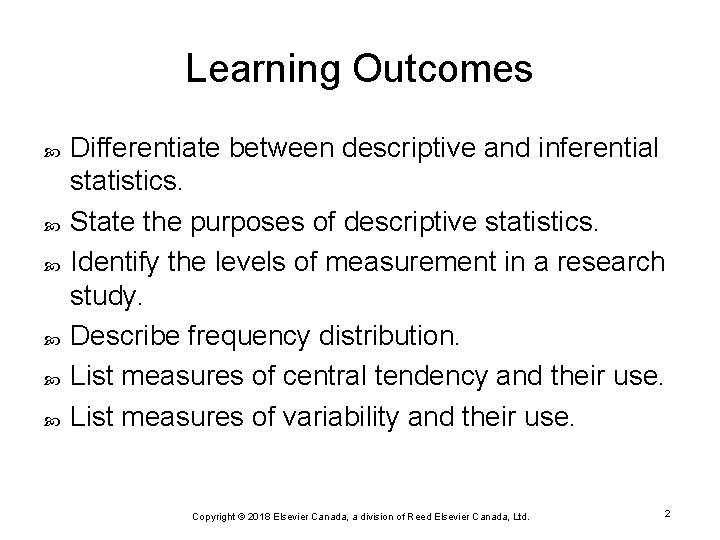
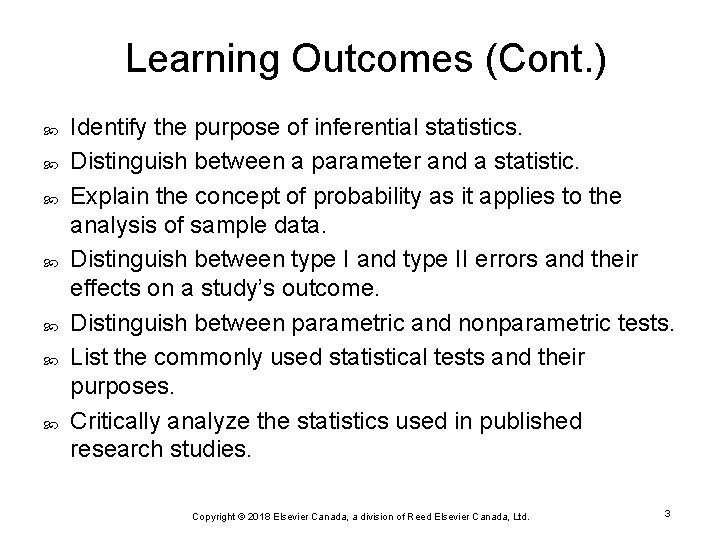
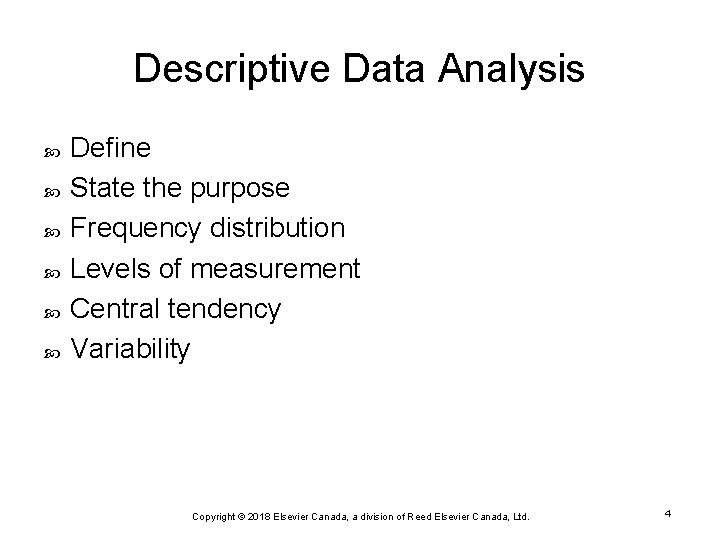
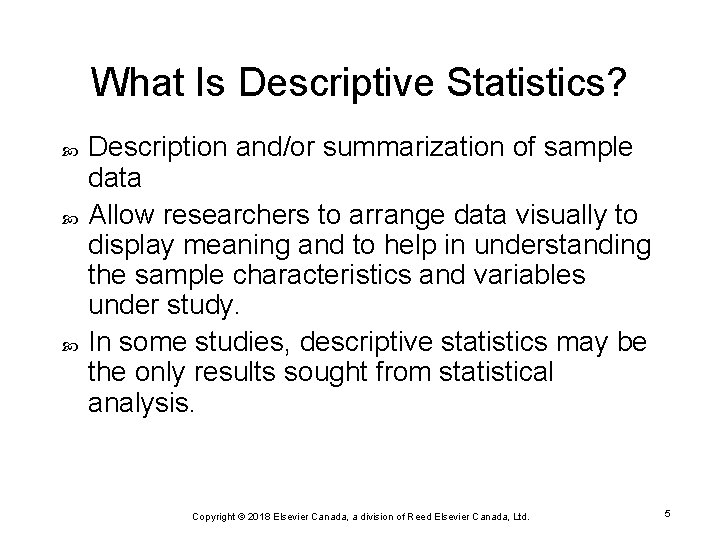
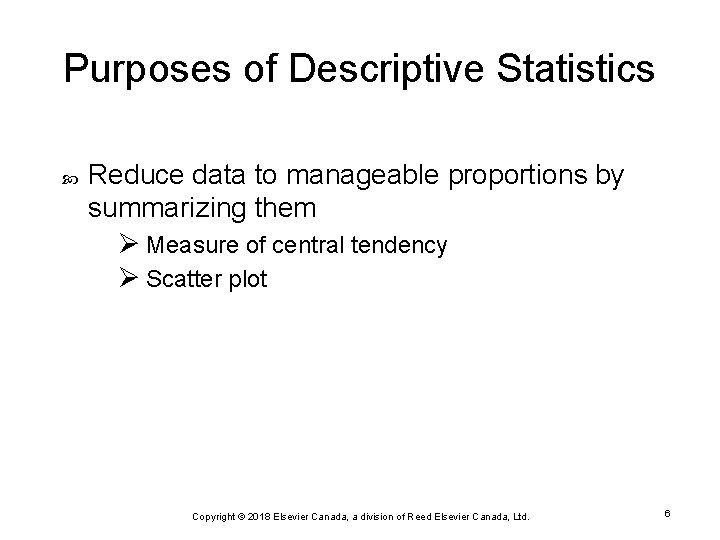
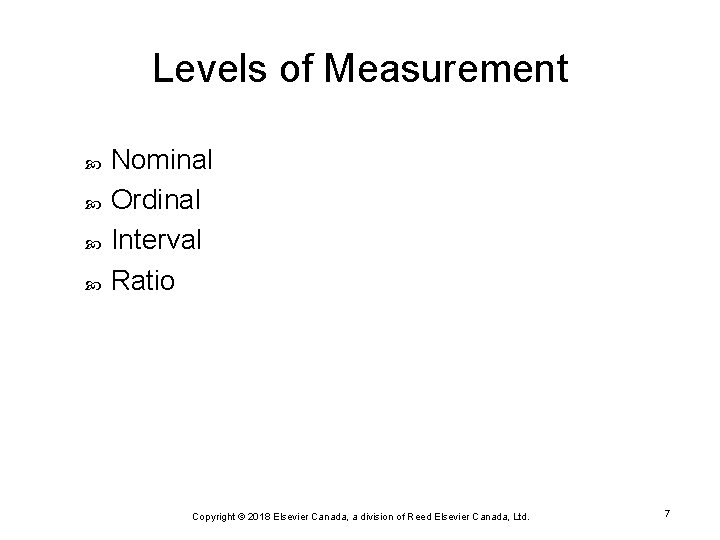
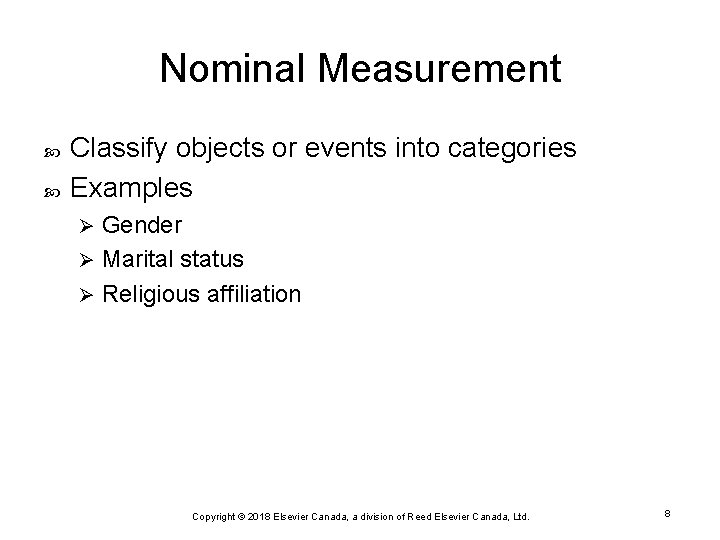
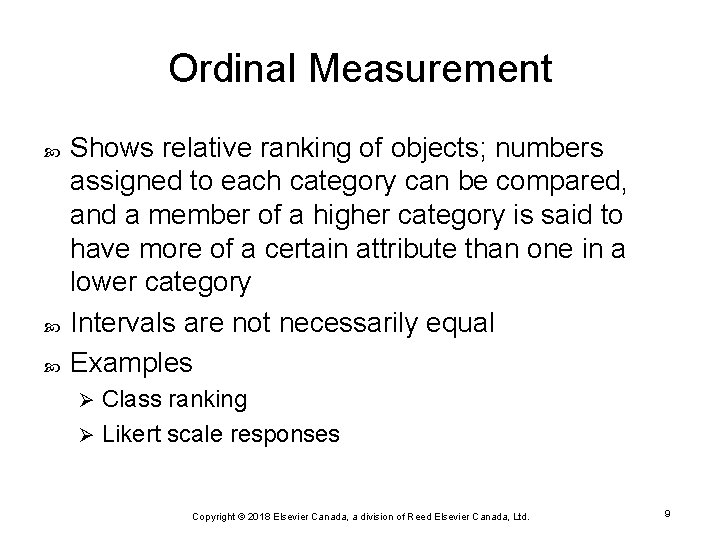
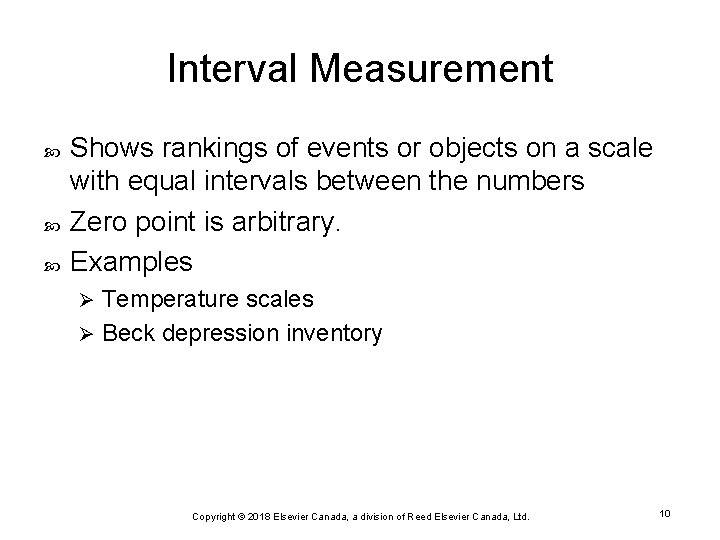
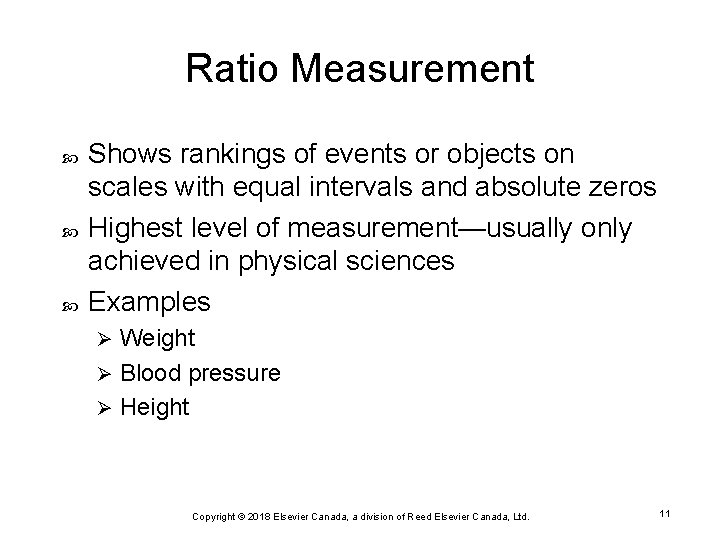
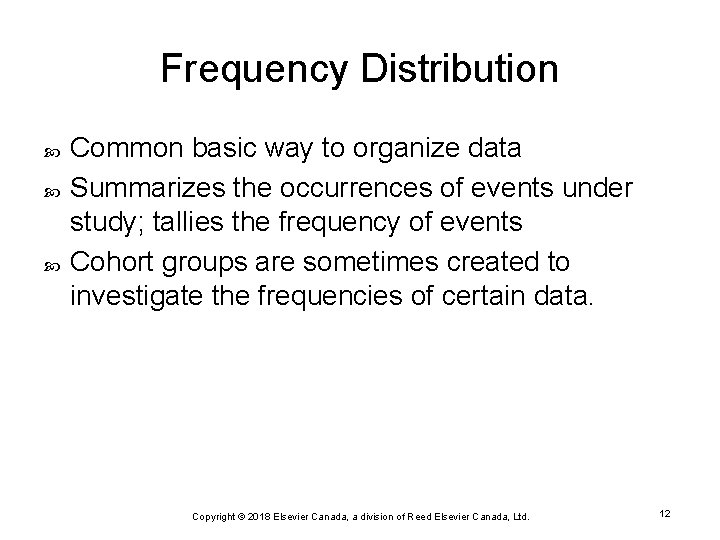
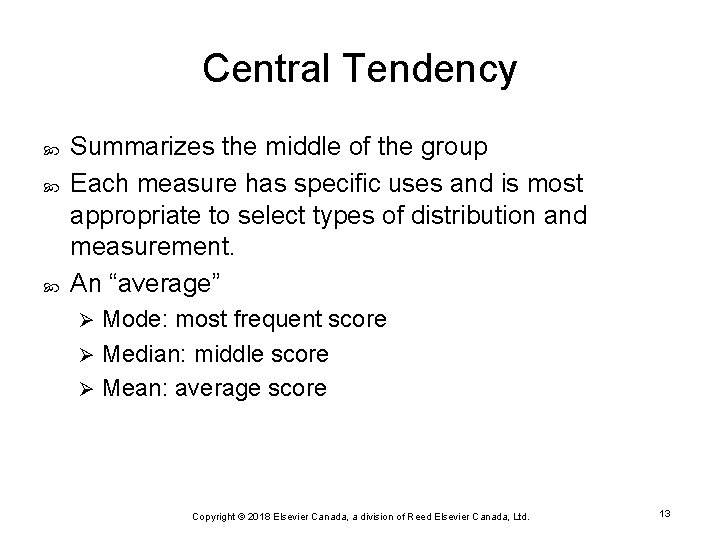
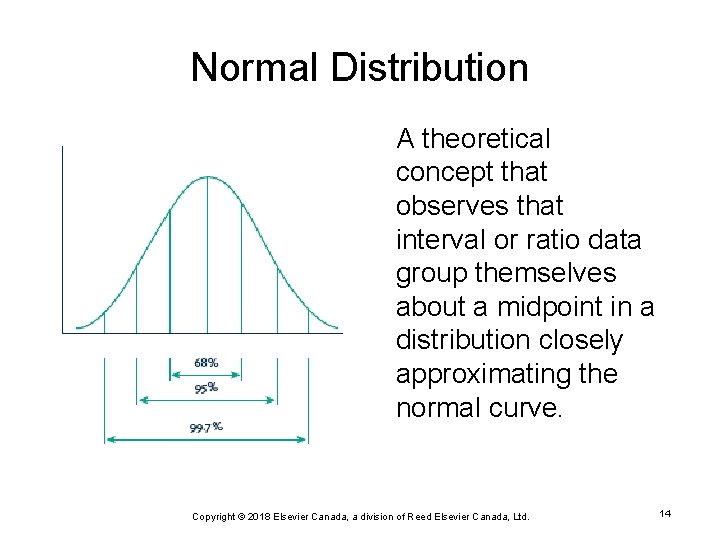
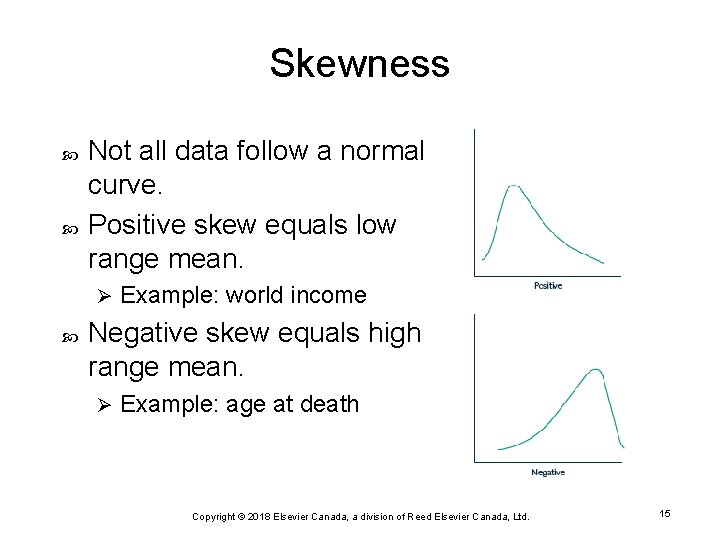
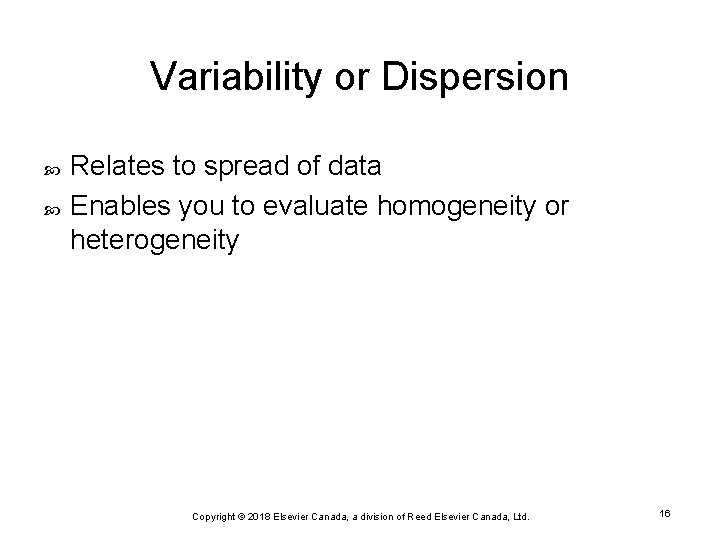
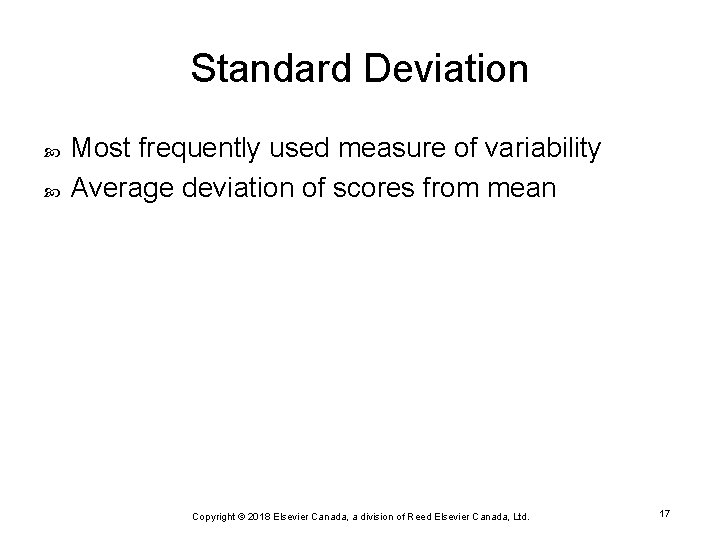
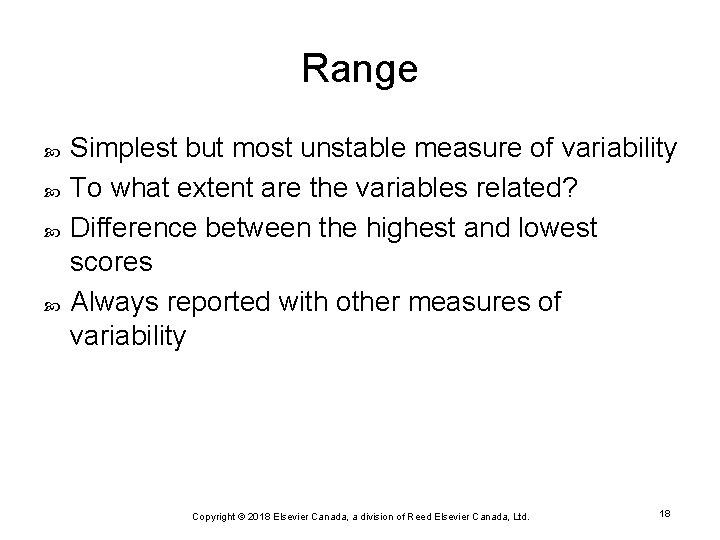
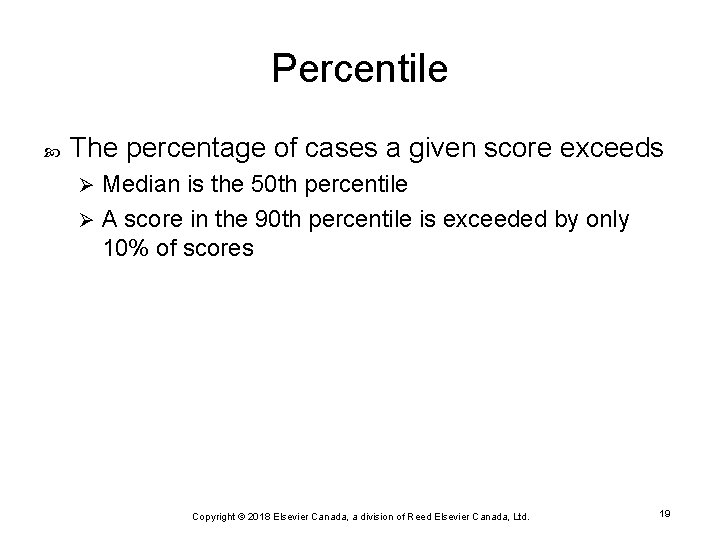
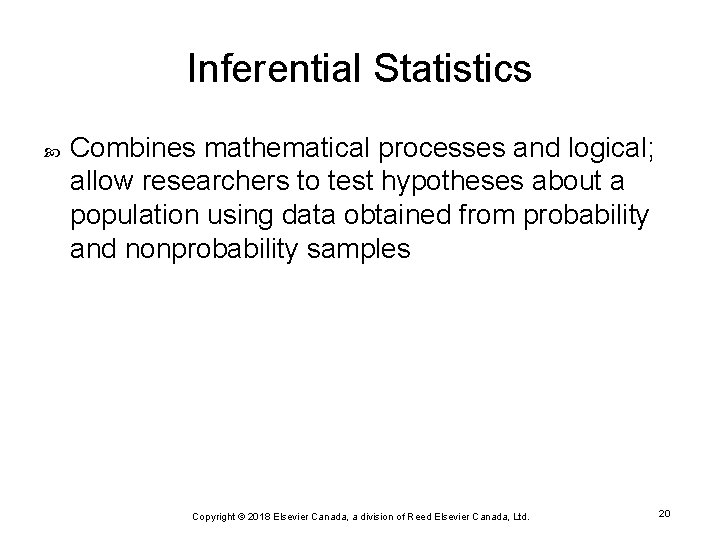
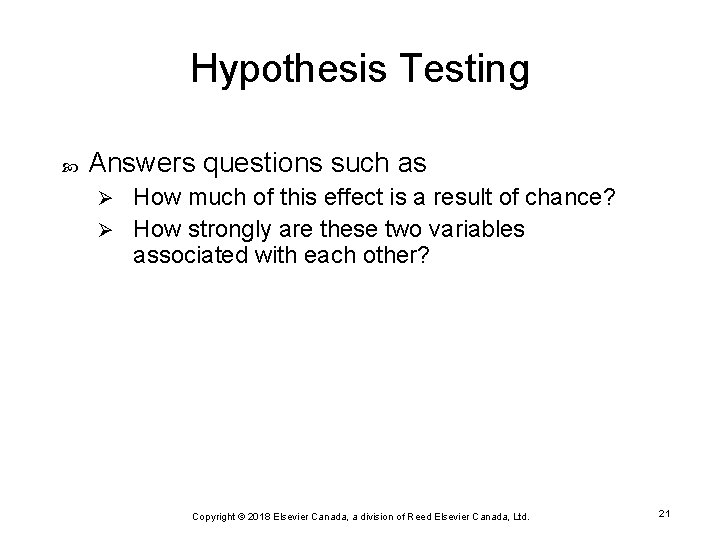
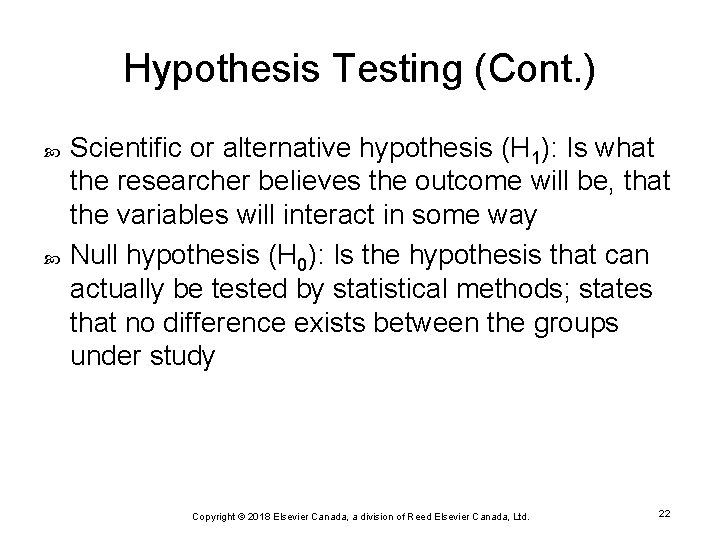
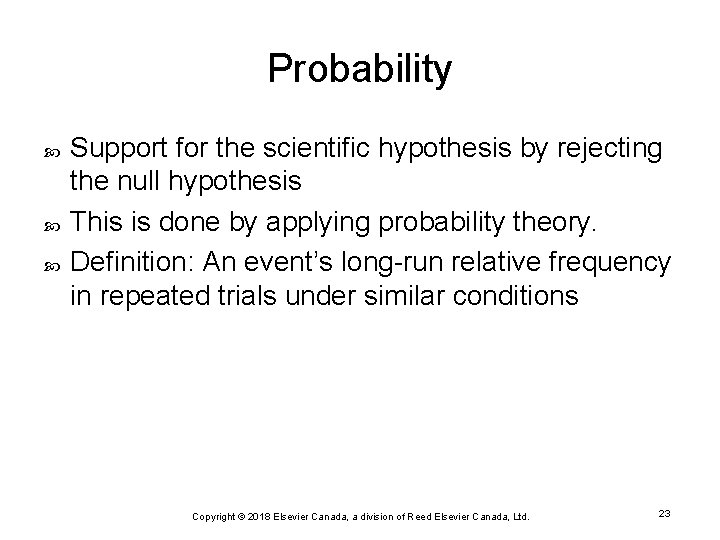
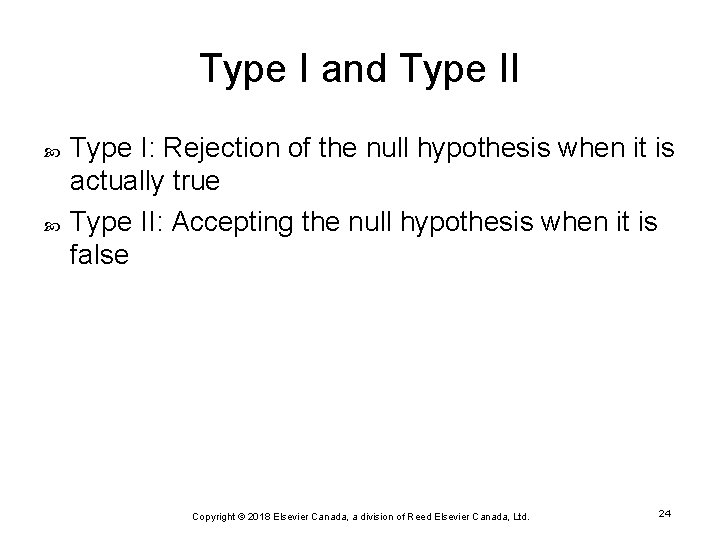
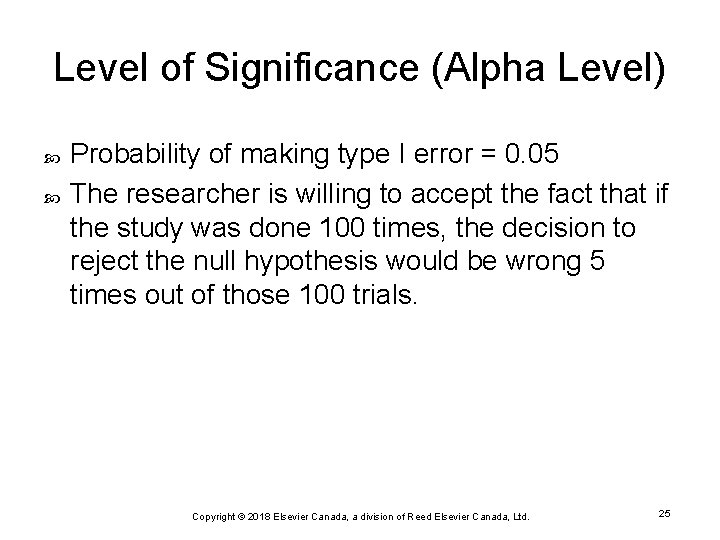
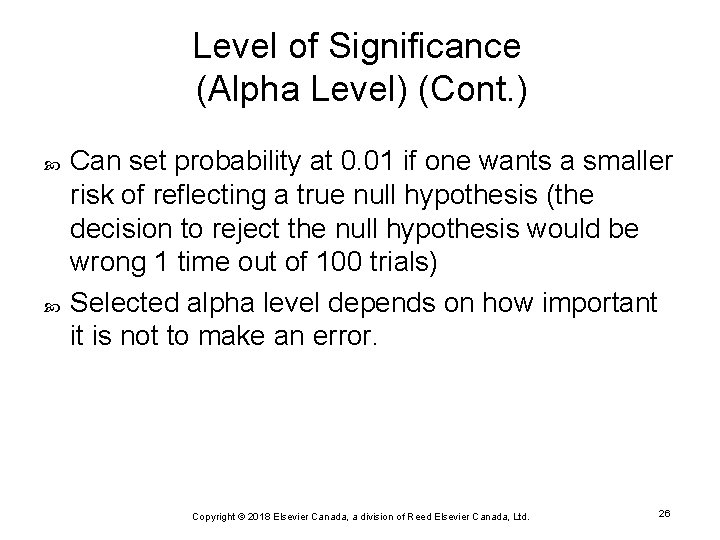
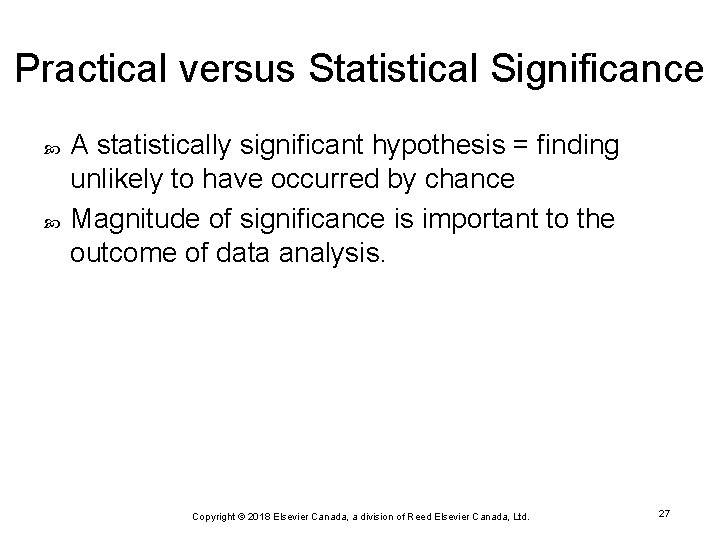
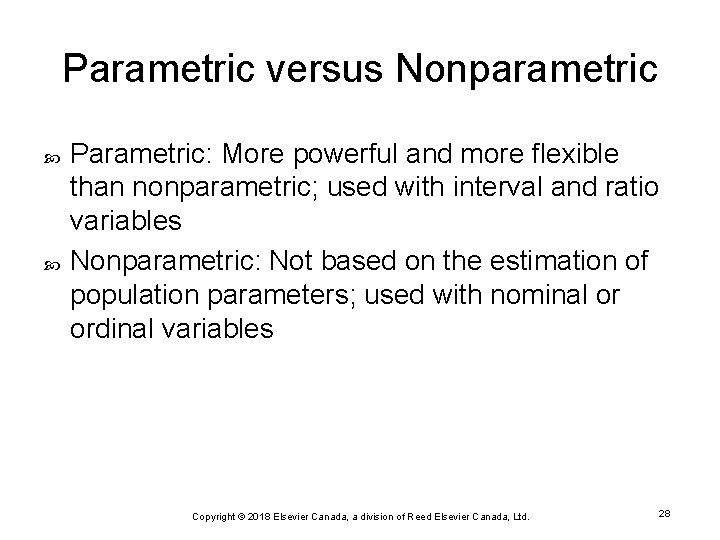
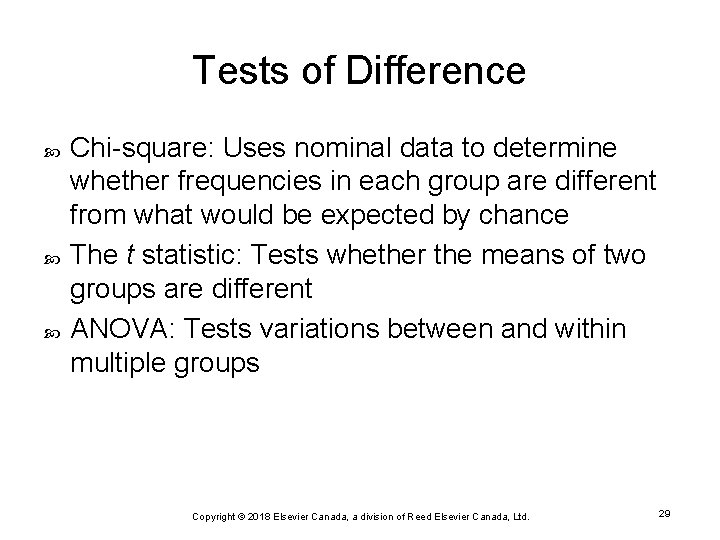
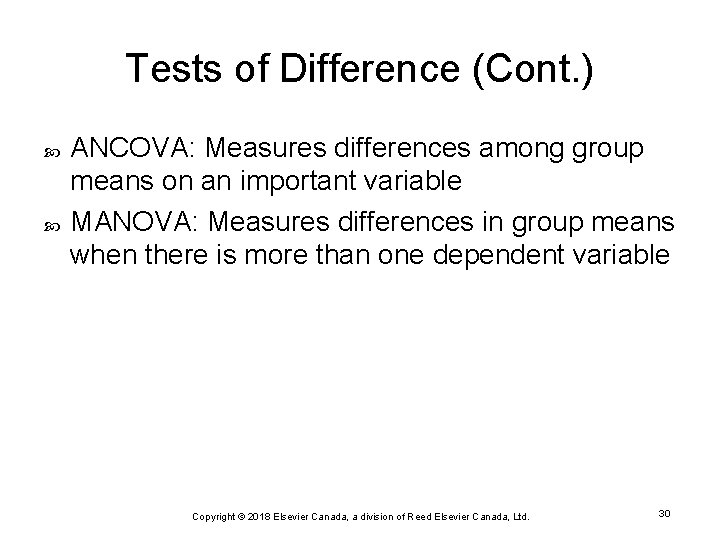
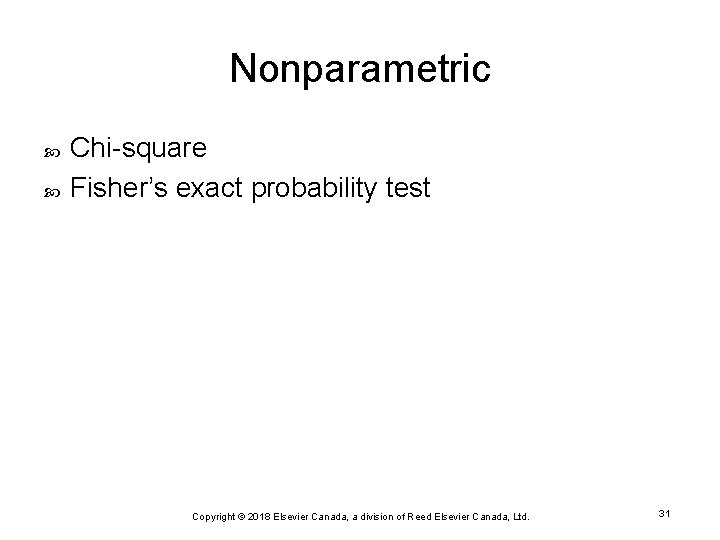
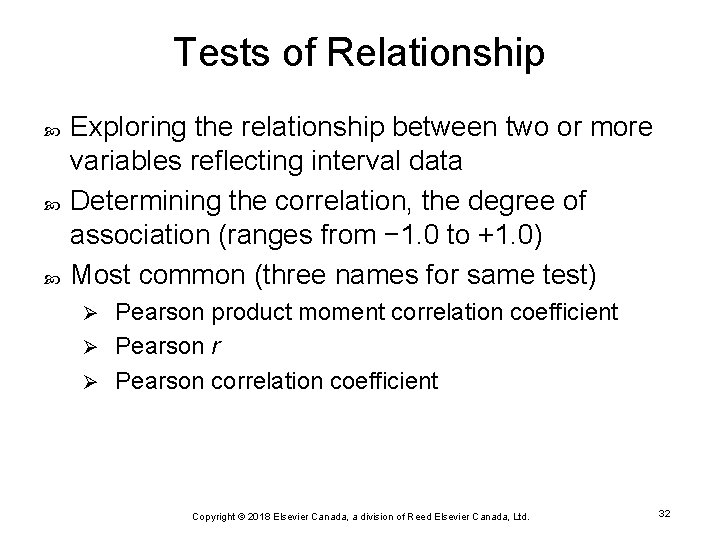
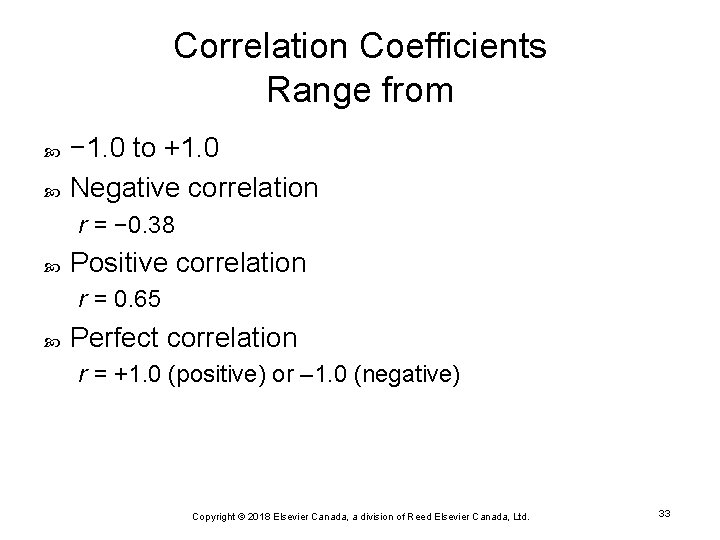
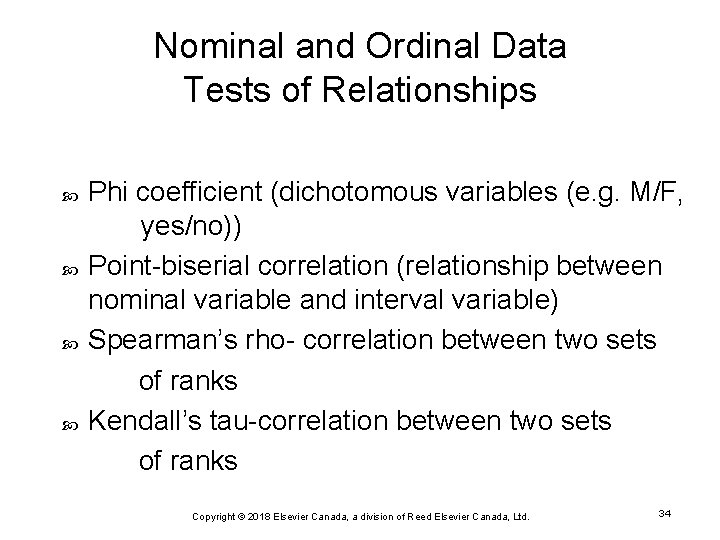
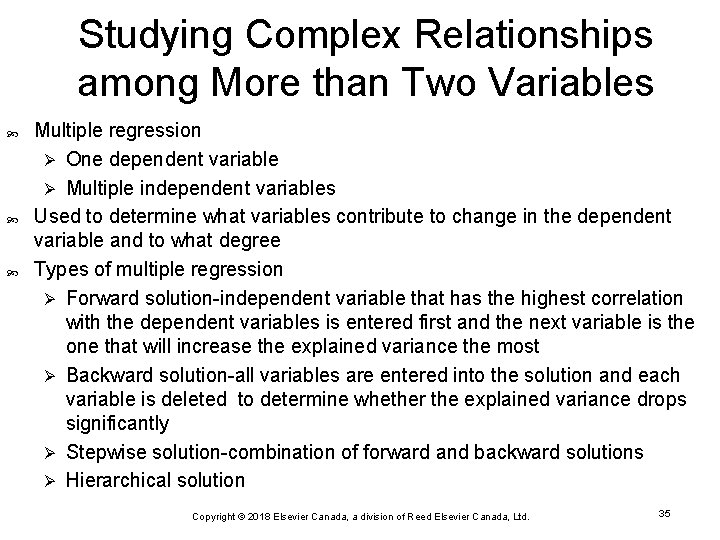
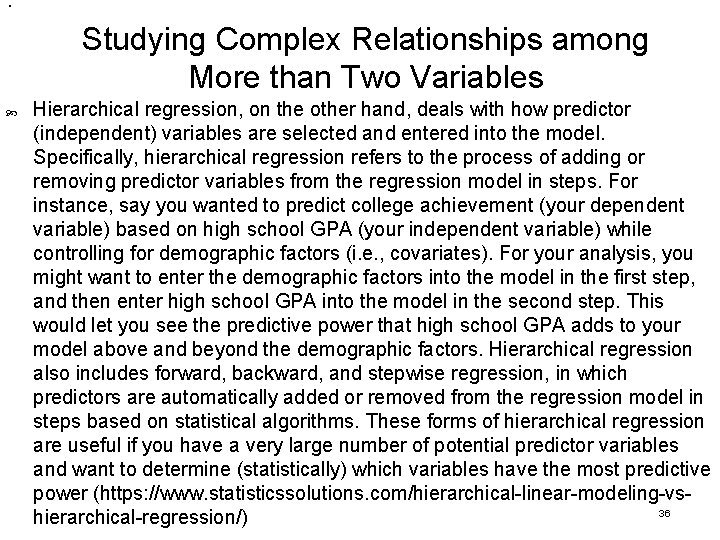
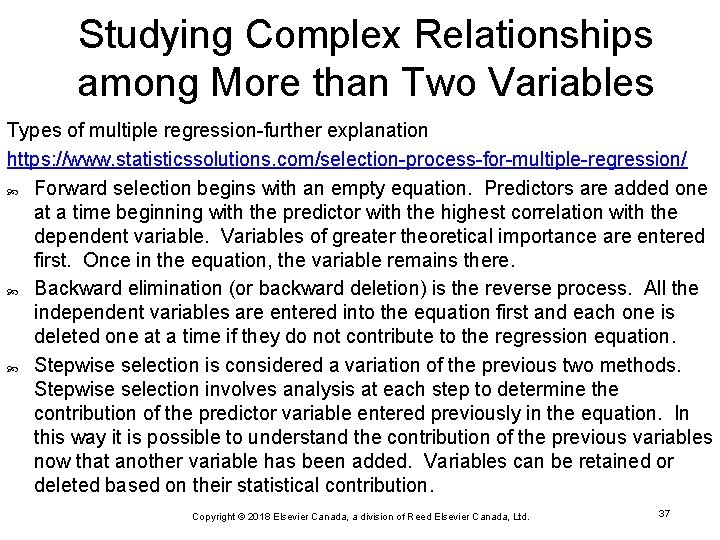
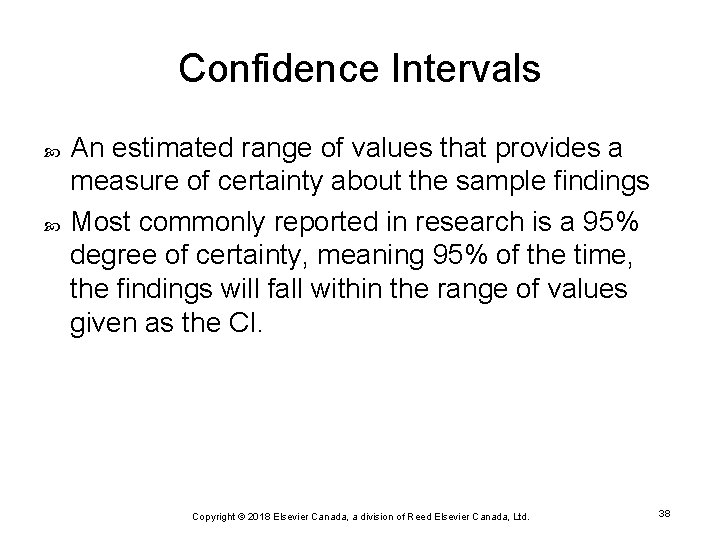
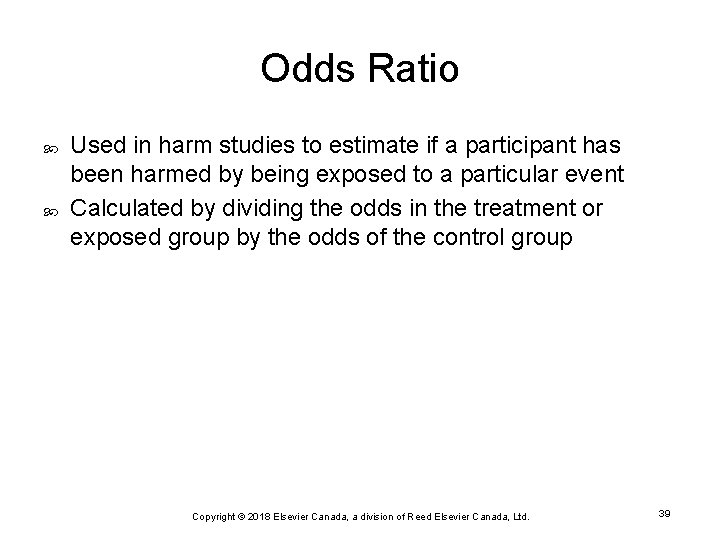
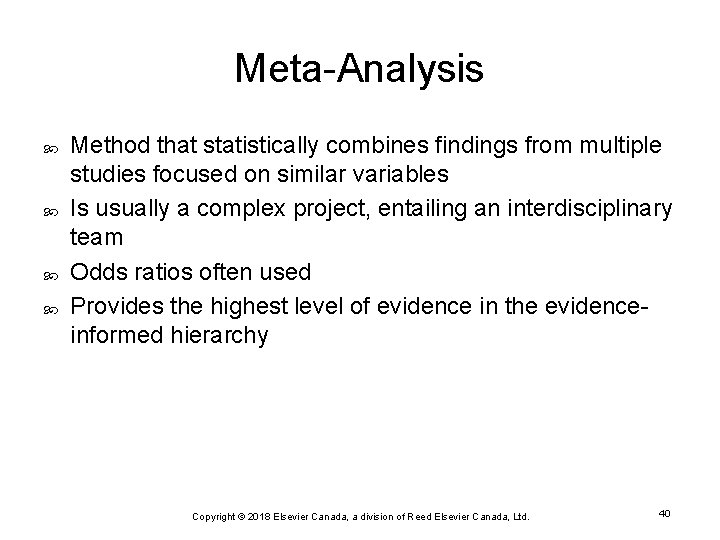
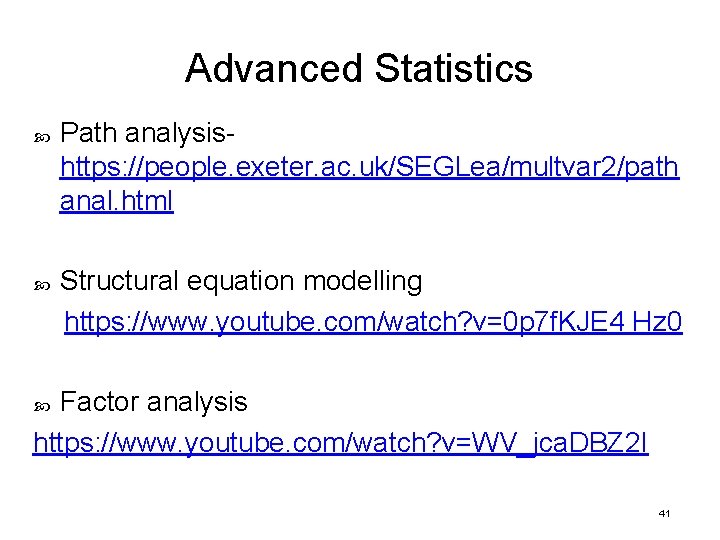
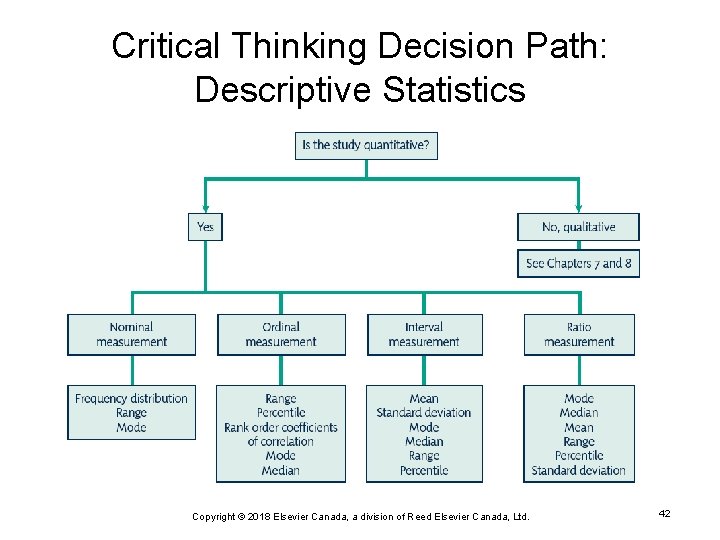
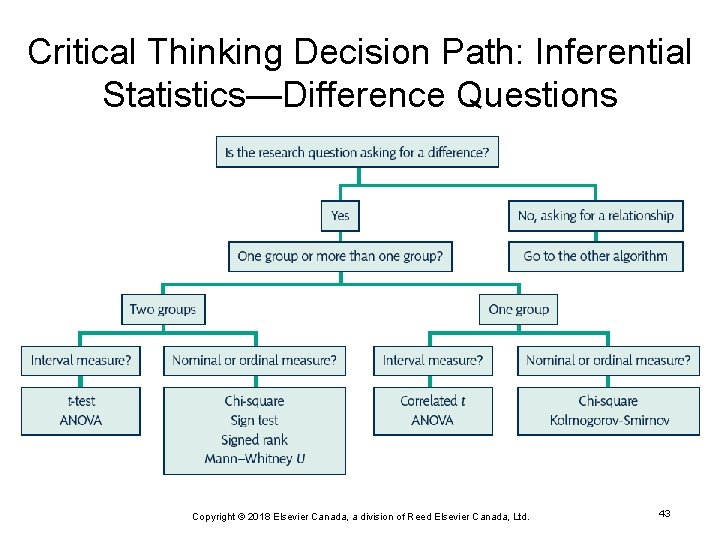
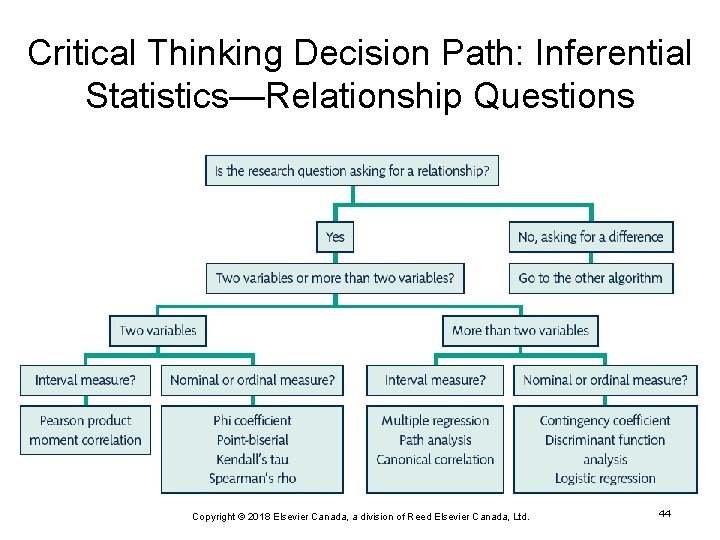
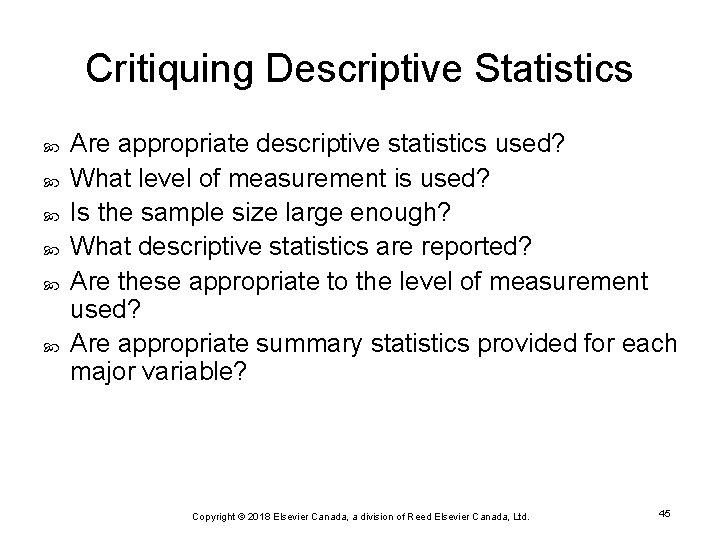
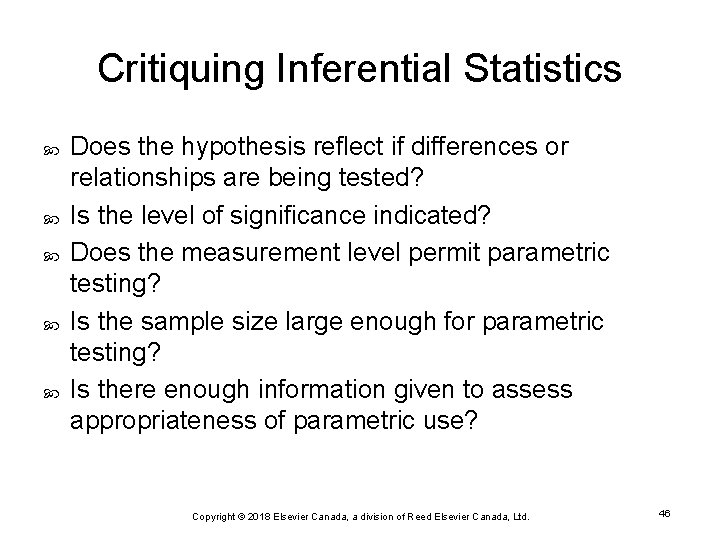
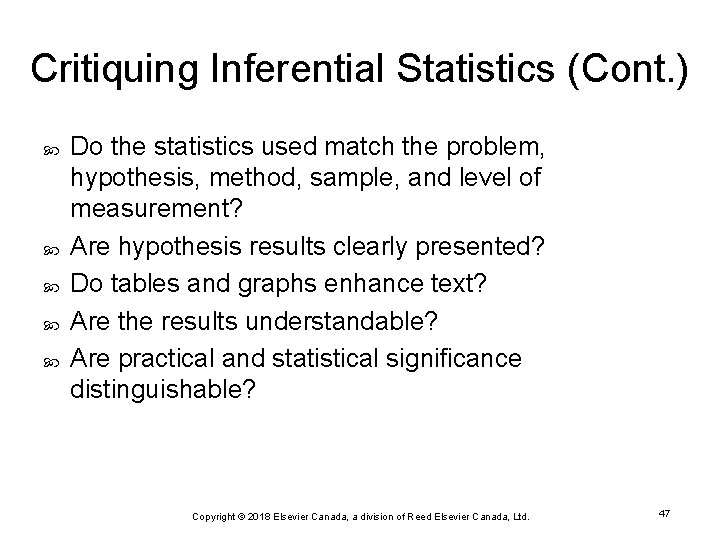
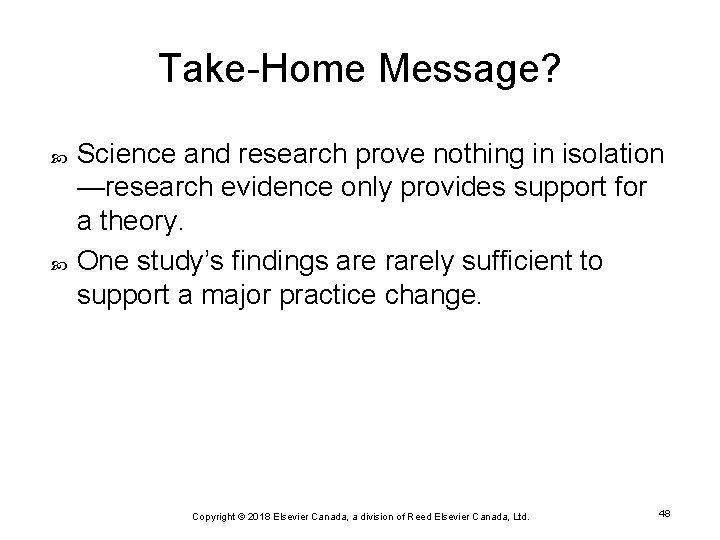
- Slides: 48
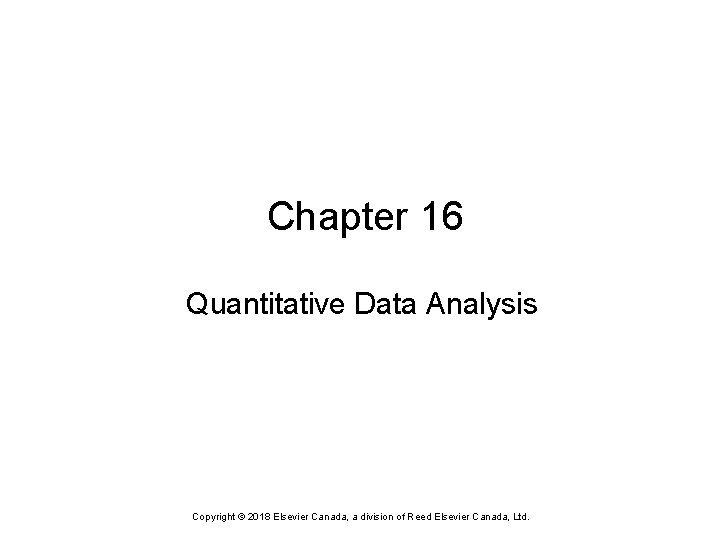
Chapter 16 Quantitative Data Analysis Copyright © 2018 Elsevier Canada, a division of Reed Elsevier Canada, Ltd.
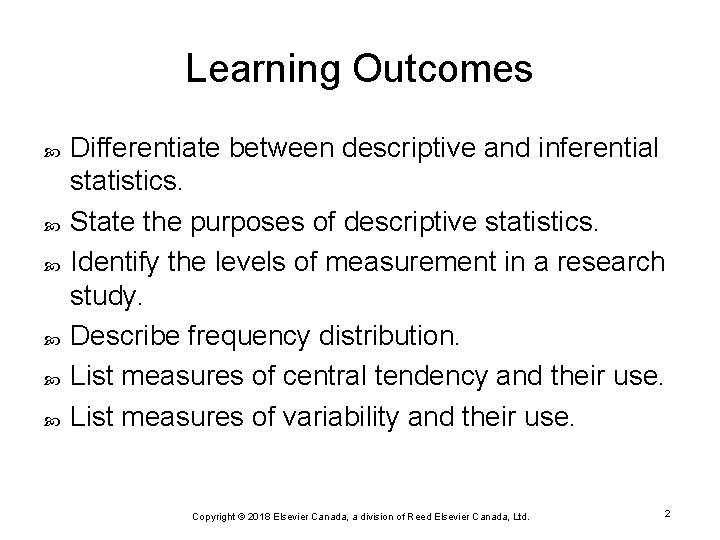
Learning Outcomes Differentiate between descriptive and inferential statistics. State the purposes of descriptive statistics. Identify the levels of measurement in a research study. Describe frequency distribution. List measures of central tendency and their use. List measures of variability and their use. Copyright © 2018 Elsevier Canada, a division of Reed Elsevier Canada, Ltd. 2
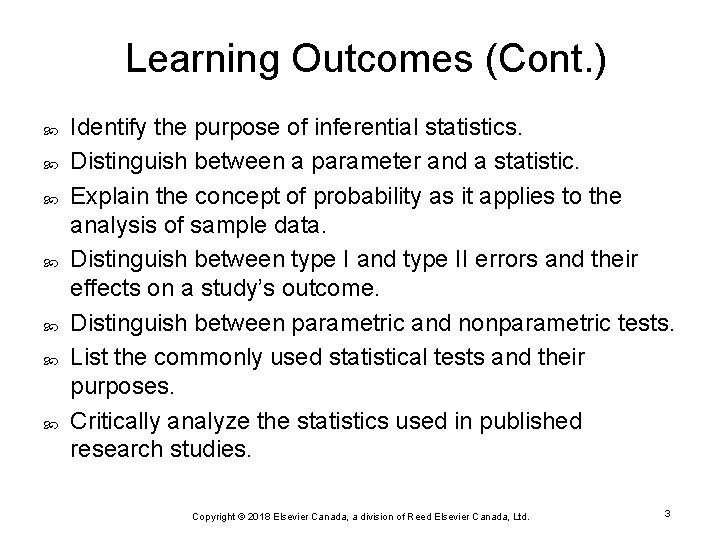
Learning Outcomes (Cont. ) Identify the purpose of inferential statistics. Distinguish between a parameter and a statistic. Explain the concept of probability as it applies to the analysis of sample data. Distinguish between type I and type II errors and their effects on a study’s outcome. Distinguish between parametric and nonparametric tests. List the commonly used statistical tests and their purposes. Critically analyze the statistics used in published research studies. Copyright © 2018 Elsevier Canada, a division of Reed Elsevier Canada, Ltd. 3
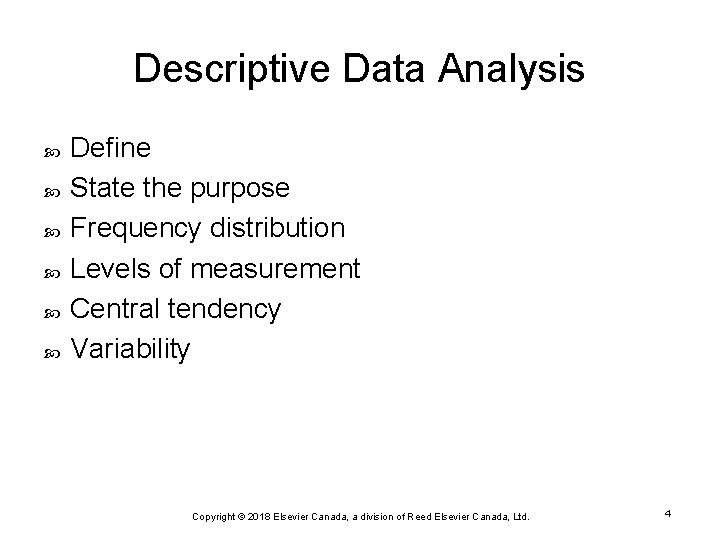
Descriptive Data Analysis Define State the purpose Frequency distribution Levels of measurement Central tendency Variability Copyright © 2018 Elsevier Canada, a division of Reed Elsevier Canada, Ltd. 4
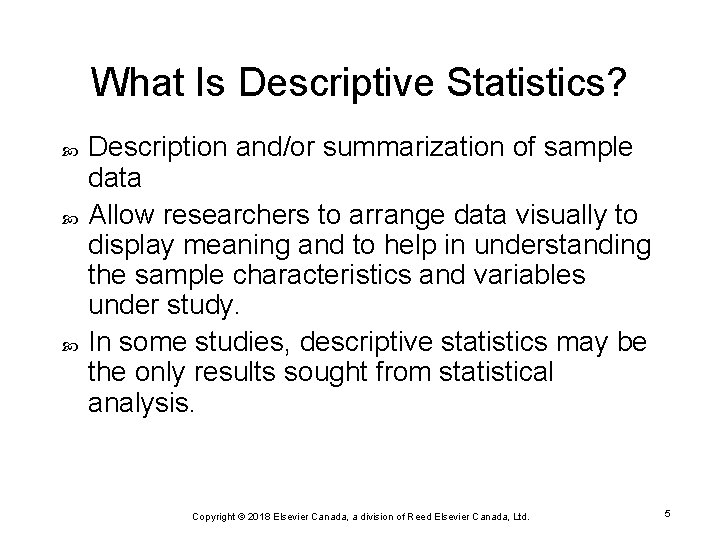
What Is Descriptive Statistics? Description and/or summarization of sample data Allow researchers to arrange data visually to display meaning and to help in understanding the sample characteristics and variables under study. In some studies, descriptive statistics may be the only results sought from statistical analysis. Copyright © 2018 Elsevier Canada, a division of Reed Elsevier Canada, Ltd. 5
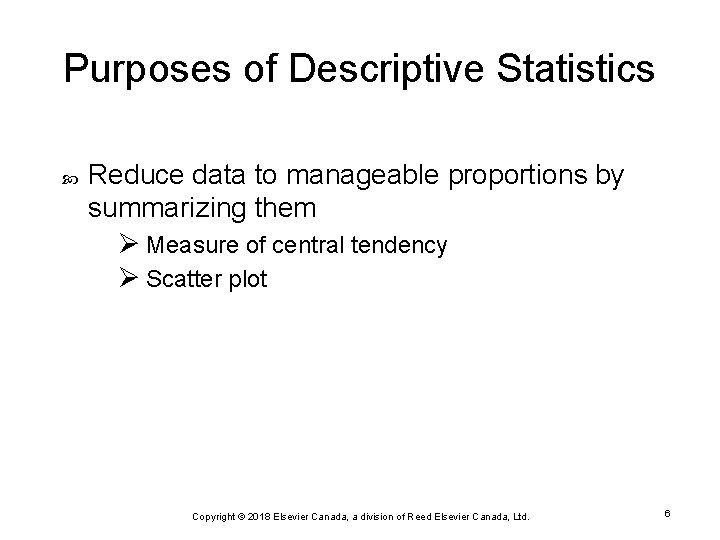
Purposes of Descriptive Statistics Reduce data to manageable proportions by summarizing them Ø Measure of central tendency Ø Scatter plot Copyright © 2018 Elsevier Canada, a division of Reed Elsevier Canada, Ltd. 6
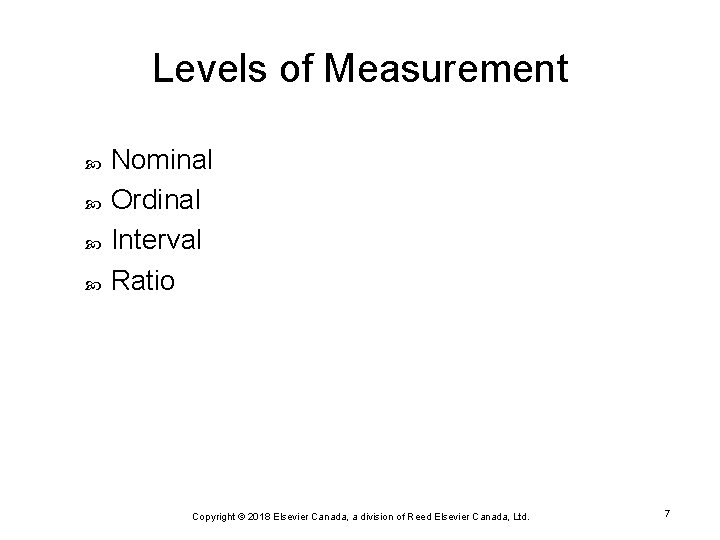
Levels of Measurement Nominal Ordinal Interval Ratio Copyright © 2018 Elsevier Canada, a division of Reed Elsevier Canada, Ltd. 7
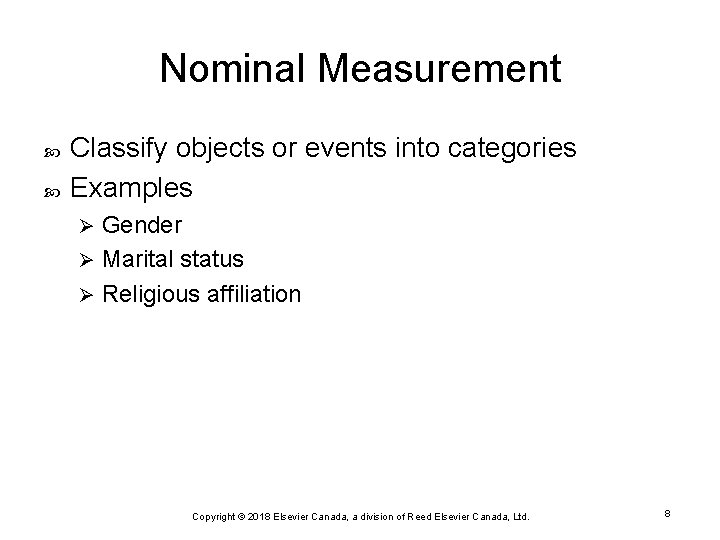
Nominal Measurement Classify objects or events into categories Examples Gender Ø Marital status Ø Religious affiliation Ø Copyright © 2018 Elsevier Canada, a division of Reed Elsevier Canada, Ltd. 8
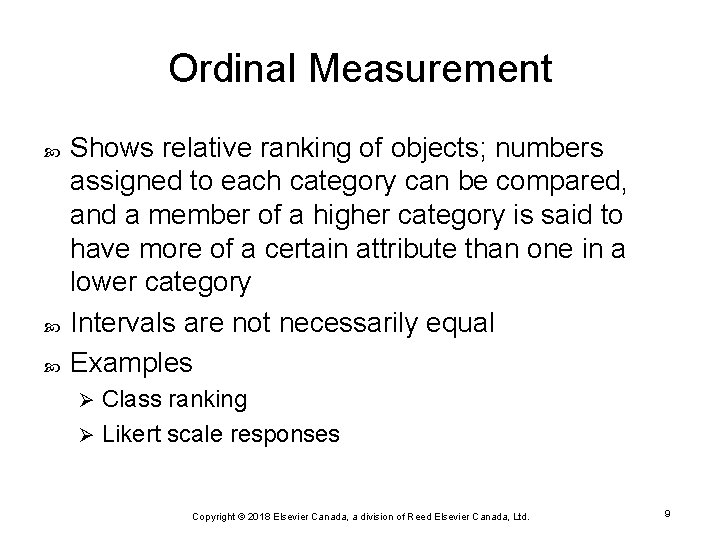
Ordinal Measurement Shows relative ranking of objects; numbers assigned to each category can be compared, and a member of a higher category is said to have more of a certain attribute than one in a lower category Intervals are not necessarily equal Examples Class ranking Ø Likert scale responses Ø Copyright © 2018 Elsevier Canada, a division of Reed Elsevier Canada, Ltd. 9
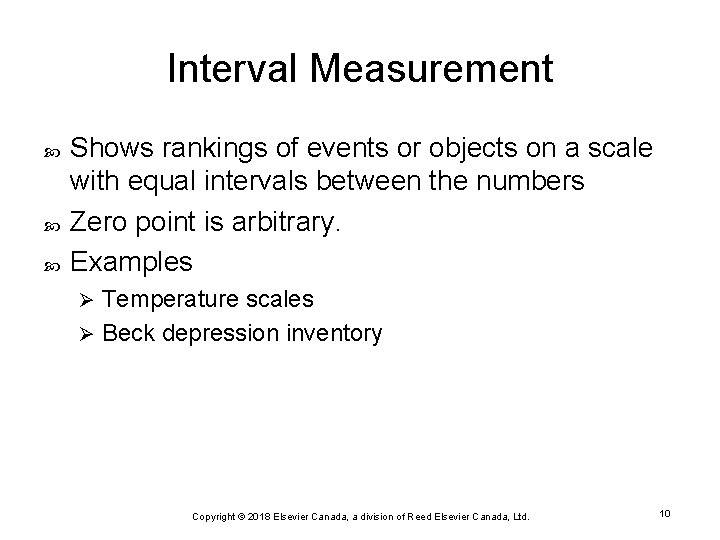
Interval Measurement Shows rankings of events or objects on a scale with equal intervals between the numbers Zero point is arbitrary. Examples Temperature scales Ø Beck depression inventory Ø Copyright © 2018 Elsevier Canada, a division of Reed Elsevier Canada, Ltd. 10
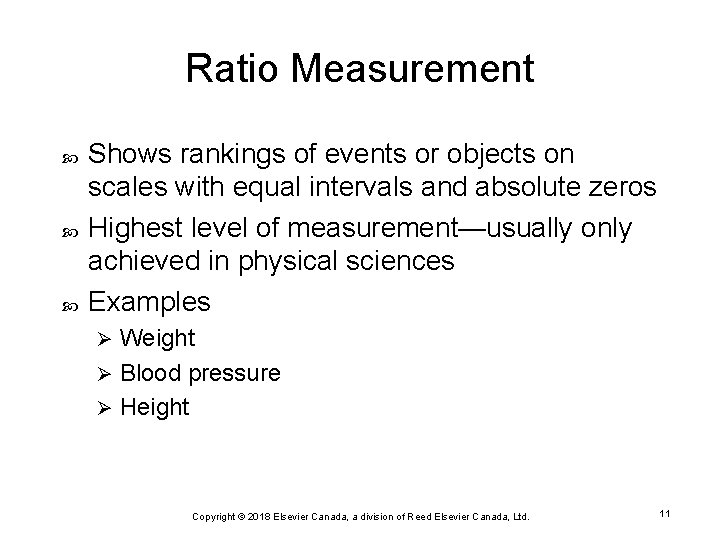
Ratio Measurement Shows rankings of events or objects on scales with equal intervals and absolute zeros Highest level of measurement—usually only achieved in physical sciences Examples Weight Ø Blood pressure Ø Height Ø Copyright © 2018 Elsevier Canada, a division of Reed Elsevier Canada, Ltd. 11
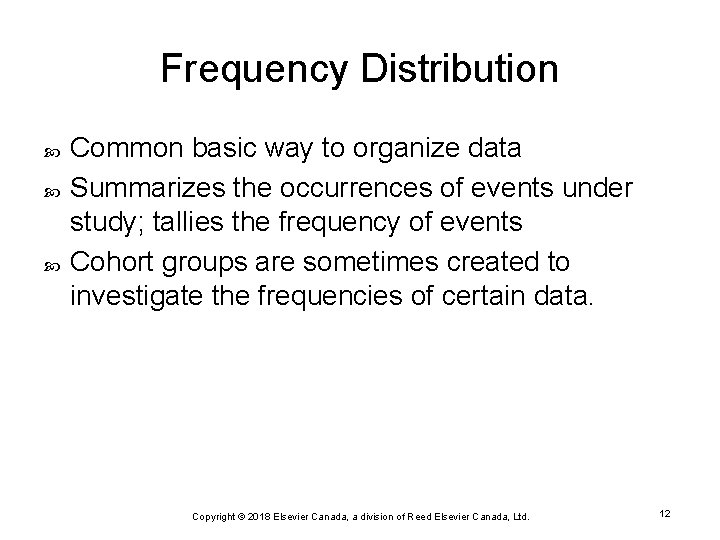
Frequency Distribution Common basic way to organize data Summarizes the occurrences of events under study; tallies the frequency of events Cohort groups are sometimes created to investigate the frequencies of certain data. Copyright © 2018 Elsevier Canada, a division of Reed Elsevier Canada, Ltd. 12
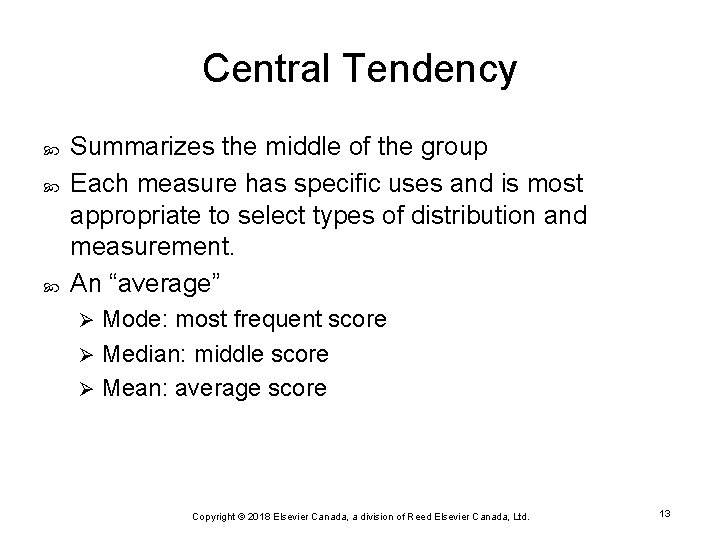
Central Tendency Summarizes the middle of the group Each measure has specific uses and is most appropriate to select types of distribution and measurement. An “average” Mode: most frequent score Ø Median: middle score Ø Mean: average score Ø Copyright © 2018 Elsevier Canada, a division of Reed Elsevier Canada, Ltd. 13
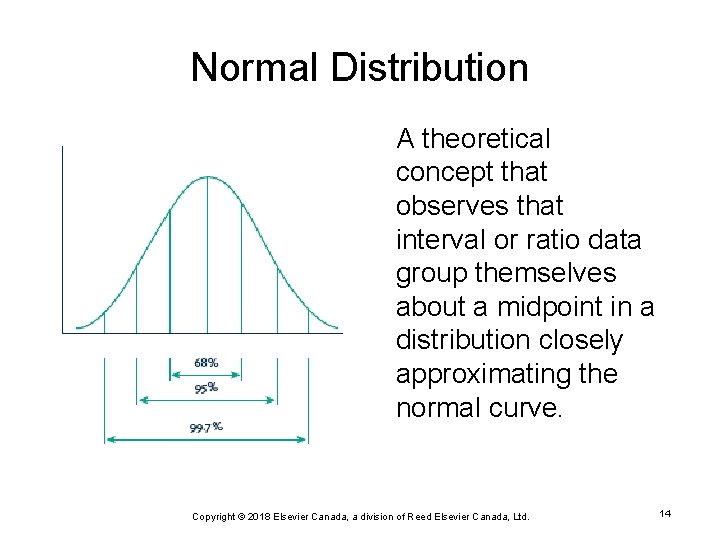
Normal Distribution A theoretical concept that observes that interval or ratio data group themselves about a midpoint in a distribution closely approximating the normal curve. Copyright © 2018 Elsevier Canada, a division of Reed Elsevier Canada, Ltd. 14
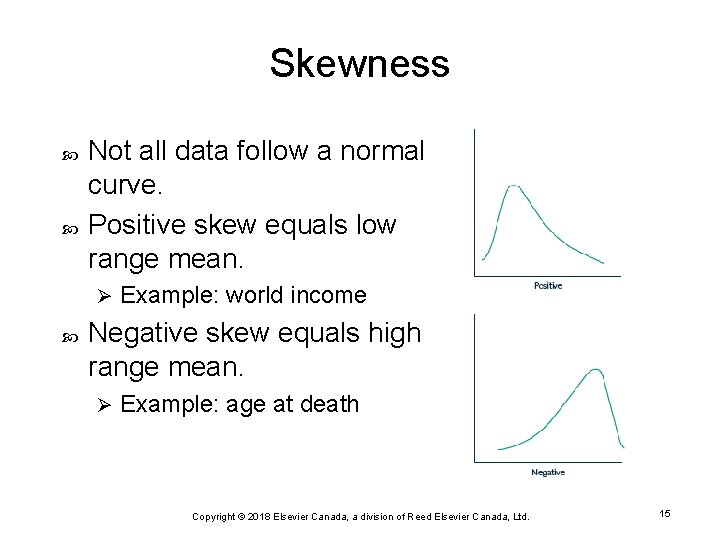
Skewness Not all data follow a normal curve. Positive skew equals low range mean. Ø Example: world income Negative skew equals high range mean. Ø Example: age at death Copyright © 2018 Elsevier Canada, a division of Reed Elsevier Canada, Ltd. 15
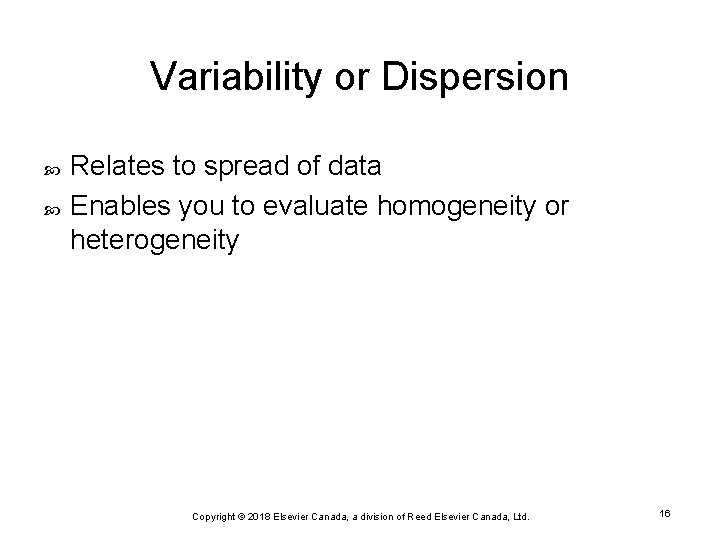
Variability or Dispersion Relates to spread of data Enables you to evaluate homogeneity or heterogeneity Copyright © 2018 Elsevier Canada, a division of Reed Elsevier Canada, Ltd. 16
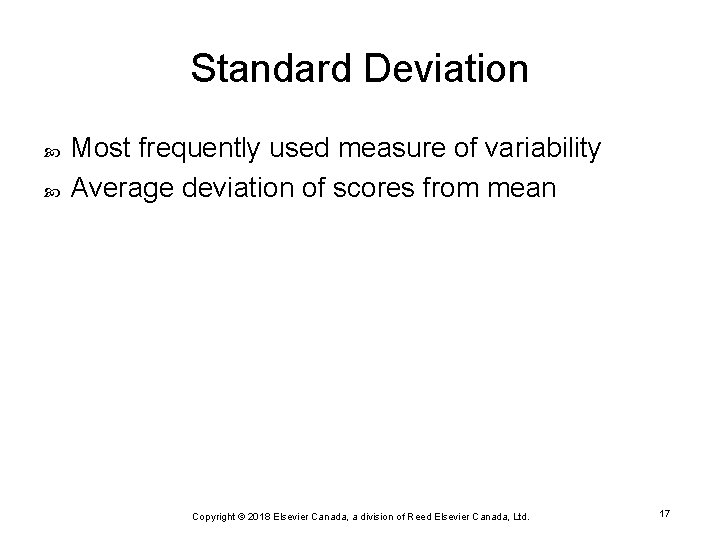
Standard Deviation Most frequently used measure of variability Average deviation of scores from mean Copyright © 2018 Elsevier Canada, a division of Reed Elsevier Canada, Ltd. 17
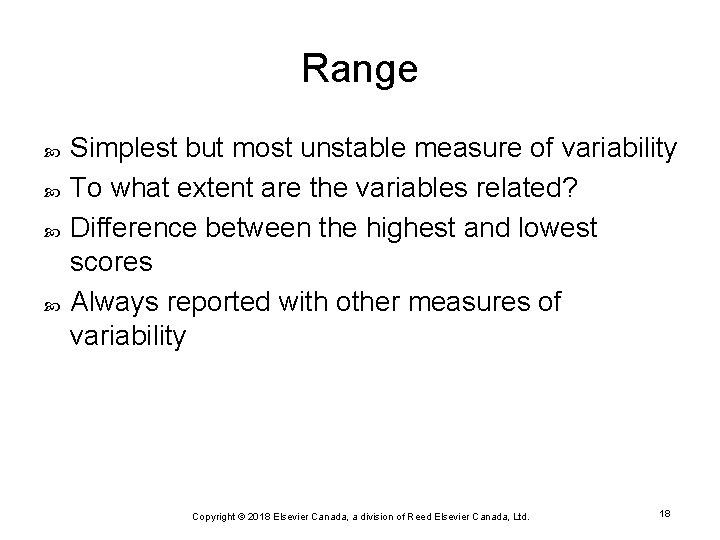
Range Simplest but most unstable measure of variability To what extent are the variables related? Difference between the highest and lowest scores Always reported with other measures of variability Copyright © 2018 Elsevier Canada, a division of Reed Elsevier Canada, Ltd. 18
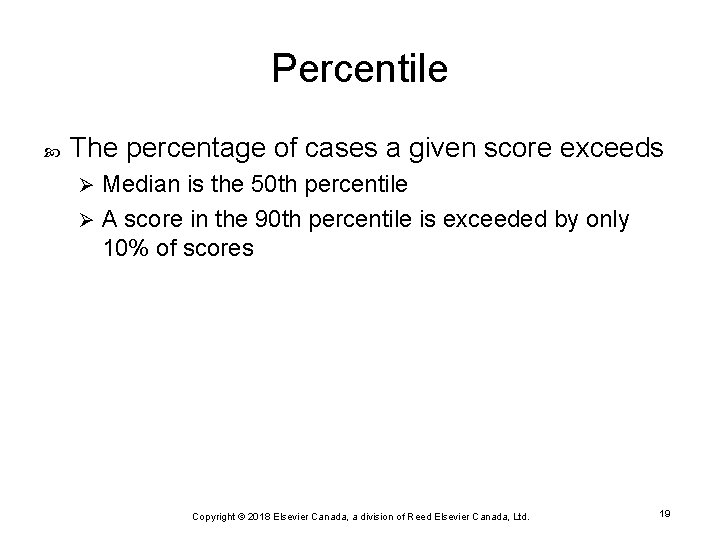
Percentile The percentage of cases a given score exceeds Median is the 50 th percentile Ø A score in the 90 th percentile is exceeded by only 10% of scores Ø Copyright © 2018 Elsevier Canada, a division of Reed Elsevier Canada, Ltd. 19
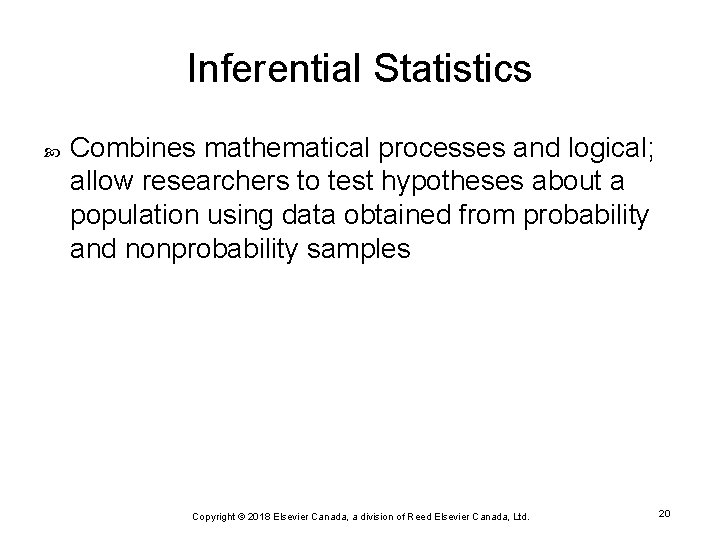
Inferential Statistics Combines mathematical processes and logical; allow researchers to test hypotheses about a population using data obtained from probability and nonprobability samples Copyright © 2018 Elsevier Canada, a division of Reed Elsevier Canada, Ltd. 20
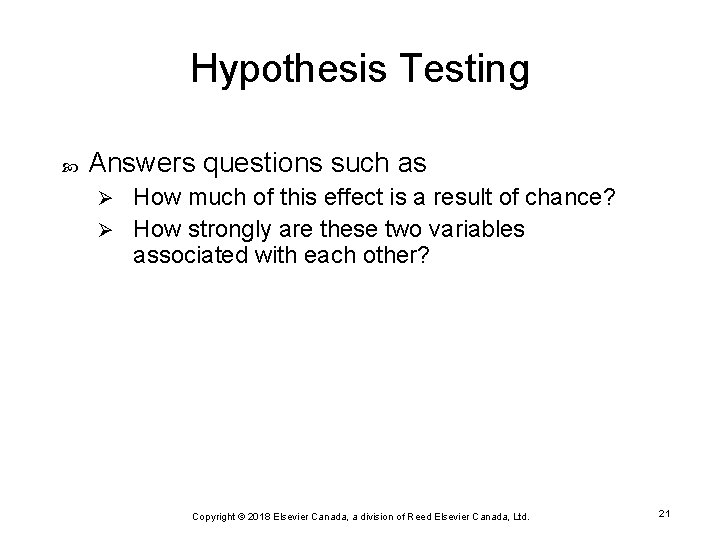
Hypothesis Testing Answers questions such as How much of this effect is a result of chance? Ø How strongly are these two variables associated with each other? Ø Copyright © 2018 Elsevier Canada, a division of Reed Elsevier Canada, Ltd. 21
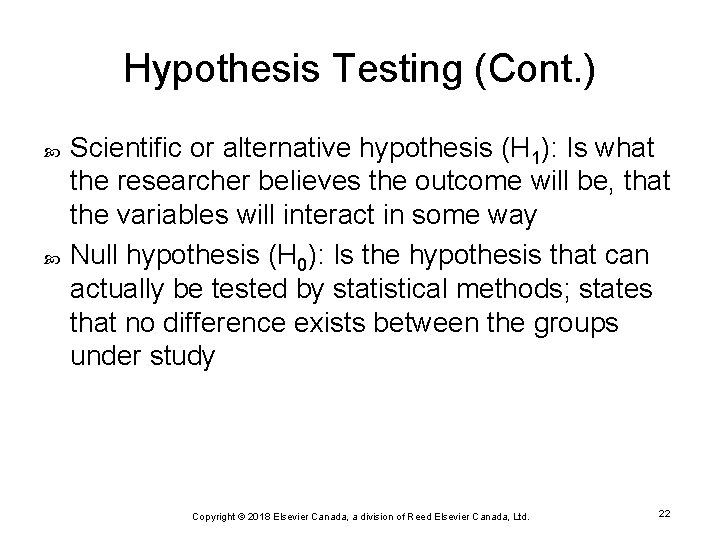
Hypothesis Testing (Cont. ) Scientific or alternative hypothesis (H 1): Is what the researcher believes the outcome will be, that the variables will interact in some way Null hypothesis (H 0): Is the hypothesis that can actually be tested by statistical methods; states that no difference exists between the groups under study Copyright © 2018 Elsevier Canada, a division of Reed Elsevier Canada, Ltd. 22
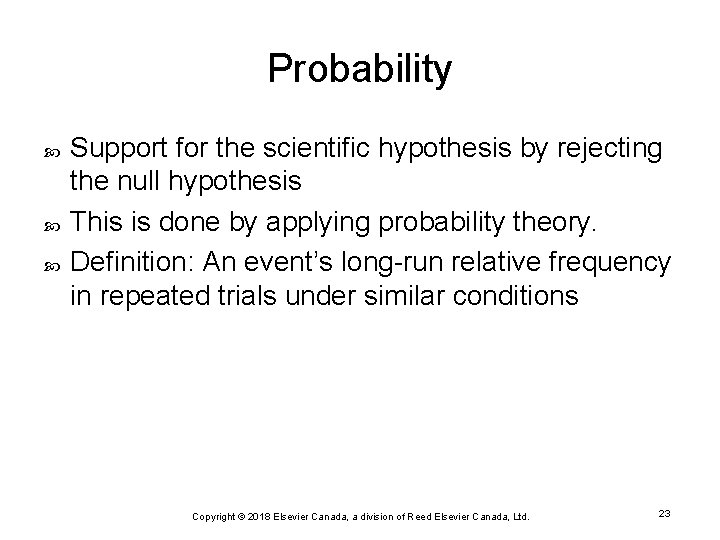
Probability Support for the scientific hypothesis by rejecting the null hypothesis This is done by applying probability theory. Definition: An event’s long-run relative frequency in repeated trials under similar conditions Copyright © 2018 Elsevier Canada, a division of Reed Elsevier Canada, Ltd. 23
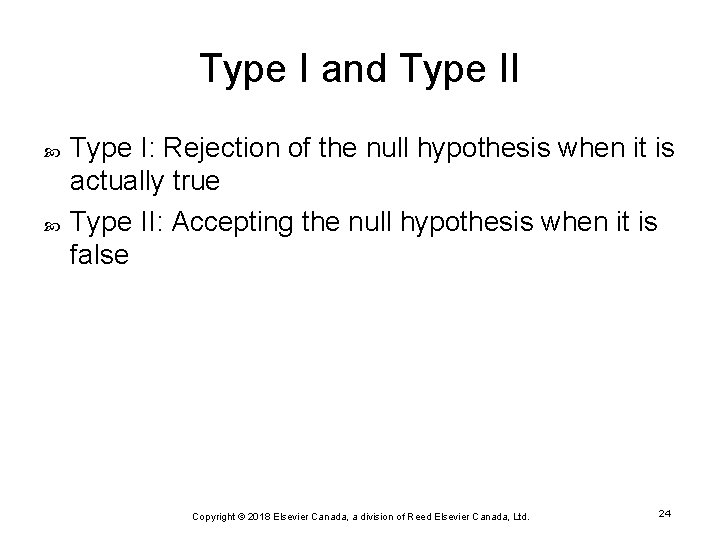
Type I and Type II Type I: Rejection of the null hypothesis when it is actually true Type II: Accepting the null hypothesis when it is false Copyright © 2018 Elsevier Canada, a division of Reed Elsevier Canada, Ltd. 24
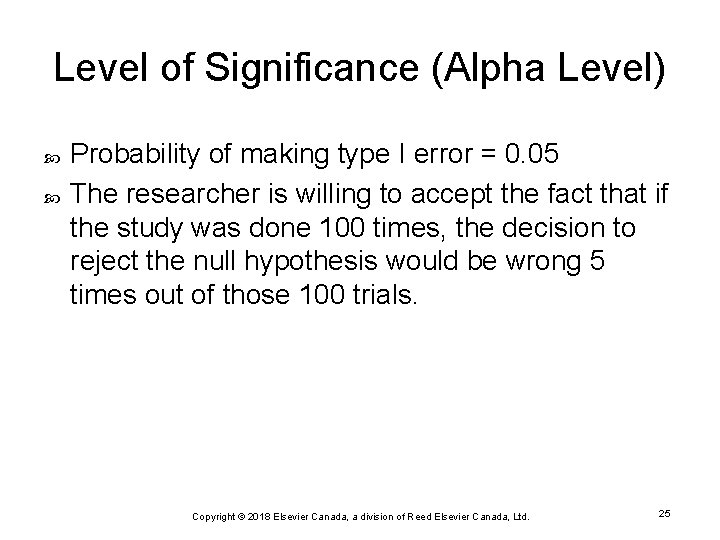
Level of Significance (Alpha Level) Probability of making type I error = 0. 05 The researcher is willing to accept the fact that if the study was done 100 times, the decision to reject the null hypothesis would be wrong 5 times out of those 100 trials. Copyright © 2018 Elsevier Canada, a division of Reed Elsevier Canada, Ltd. 25
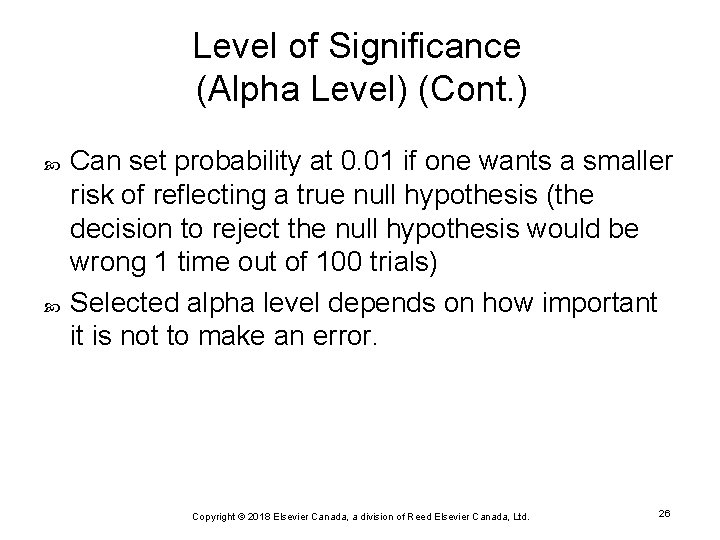
Level of Significance (Alpha Level) (Cont. ) Can set probability at 0. 01 if one wants a smaller risk of reflecting a true null hypothesis (the decision to reject the null hypothesis would be wrong 1 time out of 100 trials) Selected alpha level depends on how important it is not to make an error. Copyright © 2018 Elsevier Canada, a division of Reed Elsevier Canada, Ltd. 26
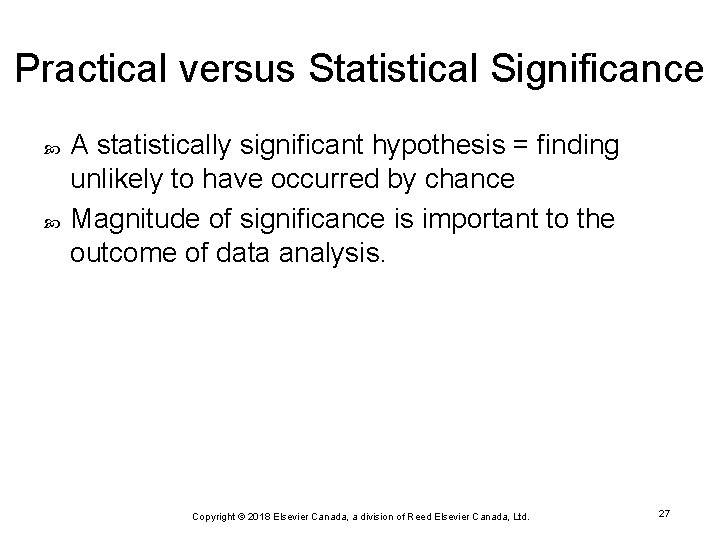
Practical versus Statistical Significance A statistically significant hypothesis = finding unlikely to have occurred by chance Magnitude of significance is important to the outcome of data analysis. Copyright © 2018 Elsevier Canada, a division of Reed Elsevier Canada, Ltd. 27
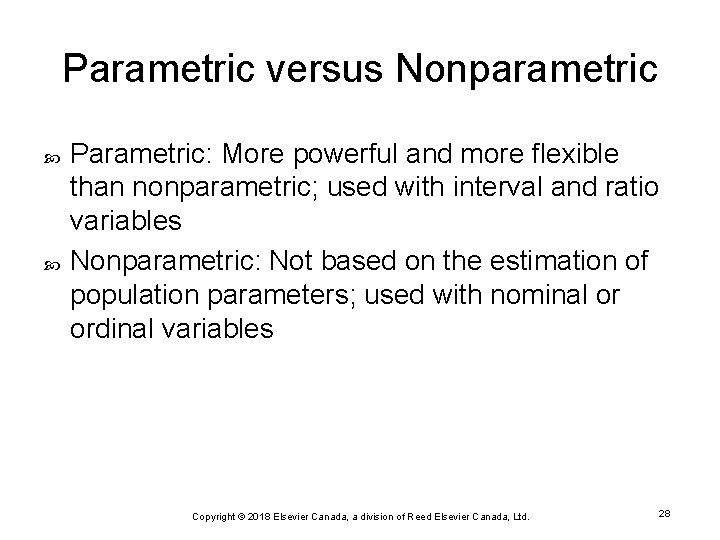
Parametric versus Nonparametric Parametric: More powerful and more flexible than nonparametric; used with interval and ratio variables Nonparametric: Not based on the estimation of population parameters; used with nominal or ordinal variables Copyright © 2018 Elsevier Canada, a division of Reed Elsevier Canada, Ltd. 28
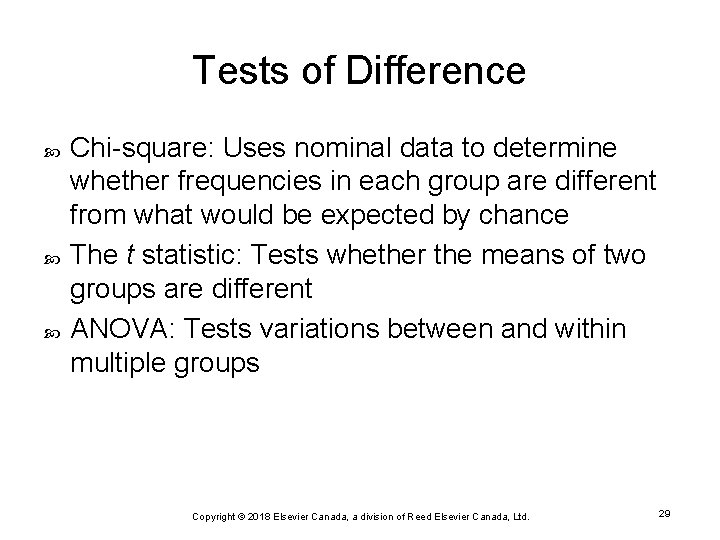
Tests of Difference Chi-square: Uses nominal data to determine whether frequencies in each group are different from what would be expected by chance The t statistic: Tests whether the means of two groups are different ANOVA: Tests variations between and within multiple groups Copyright © 2018 Elsevier Canada, a division of Reed Elsevier Canada, Ltd. 29
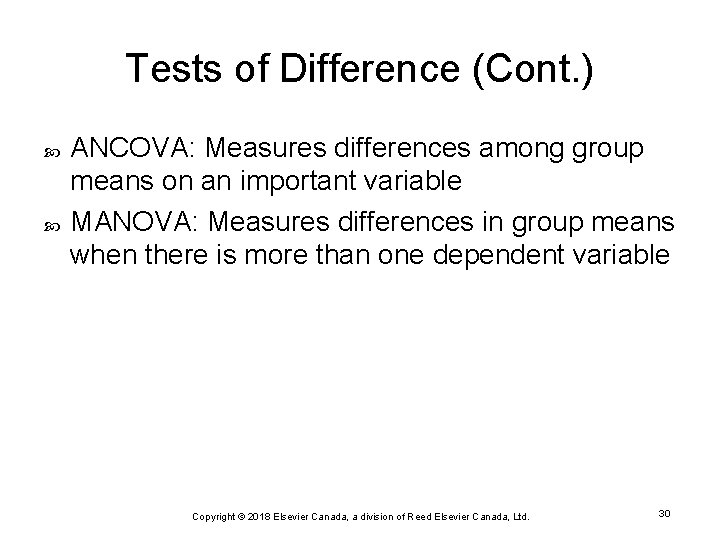
Tests of Difference (Cont. ) ANCOVA: Measures differences among group means on an important variable MANOVA: Measures differences in group means when there is more than one dependent variable Copyright © 2018 Elsevier Canada, a division of Reed Elsevier Canada, Ltd. 30
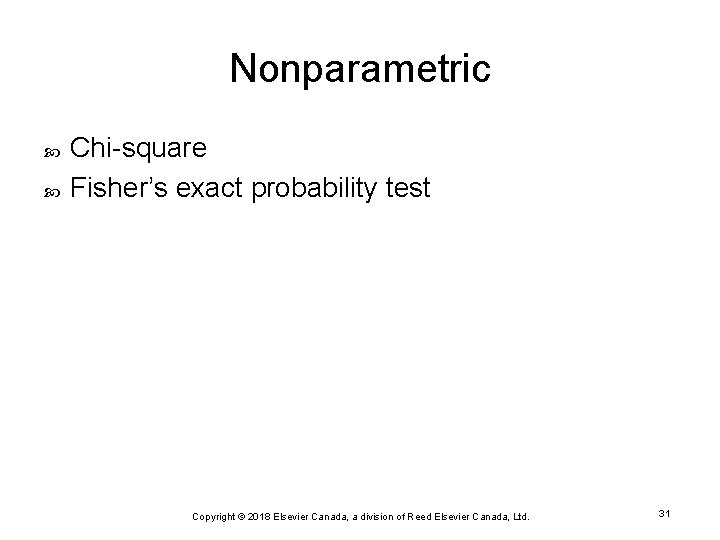
Nonparametric Chi-square Fisher’s exact probability test Copyright © 2018 Elsevier Canada, a division of Reed Elsevier Canada, Ltd. 31
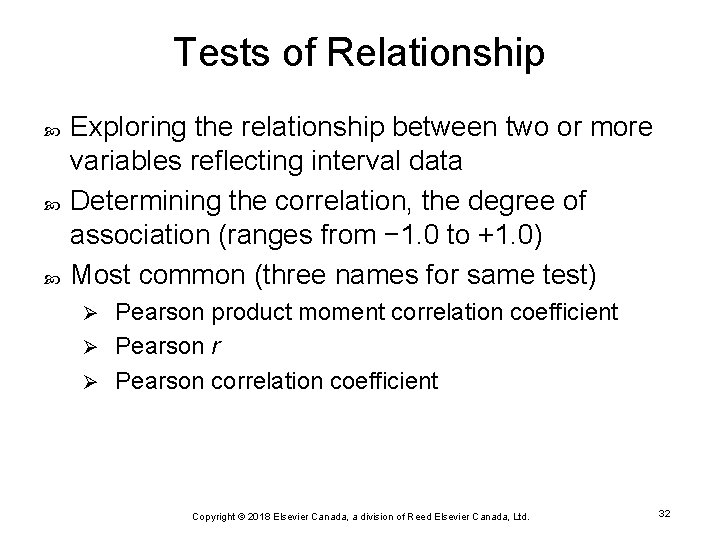
Tests of Relationship Exploring the relationship between two or more variables reflecting interval data Determining the correlation, the degree of association (ranges from − 1. 0 to +1. 0) Most common (three names for same test) Pearson product moment correlation coefficient Ø Pearson r Ø Pearson correlation coefficient Ø Copyright © 2018 Elsevier Canada, a division of Reed Elsevier Canada, Ltd. 32
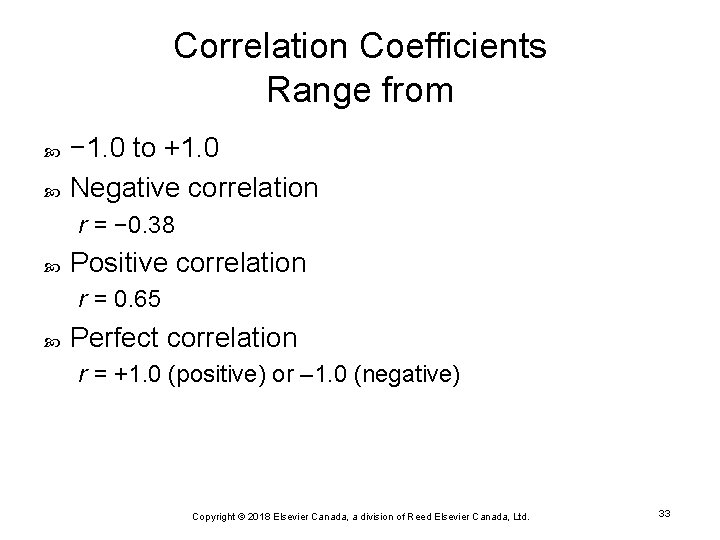
Correlation Coefficients Range from − 1. 0 to +1. 0 Negative correlation r = − 0. 38 Positive correlation r = 0. 65 Perfect correlation r = +1. 0 (positive) or – 1. 0 (negative) Copyright © 2018 Elsevier Canada, a division of Reed Elsevier Canada, Ltd. 33
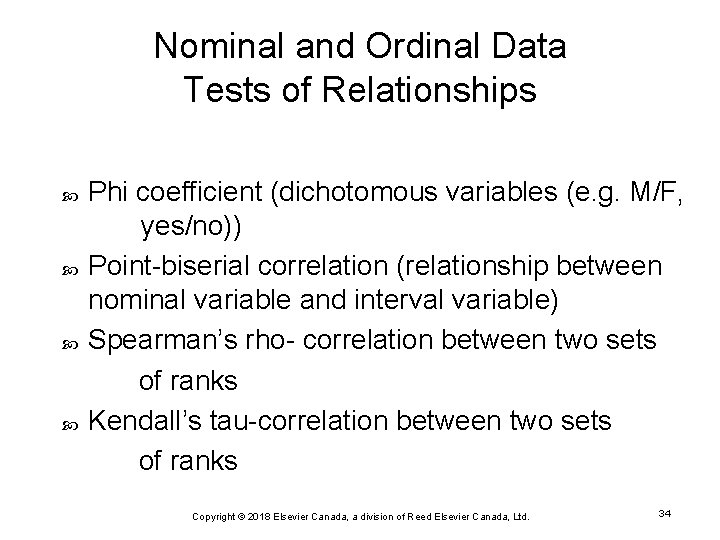
Nominal and Ordinal Data Tests of Relationships Phi coefficient (dichotomous variables (e. g. M/F, yes/no)) Point-biserial correlation (relationship between nominal variable and interval variable) Spearman’s rho- correlation between two sets of ranks Kendall’s tau-correlation between two sets of ranks Copyright © 2018 Elsevier Canada, a division of Reed Elsevier Canada, Ltd. 34
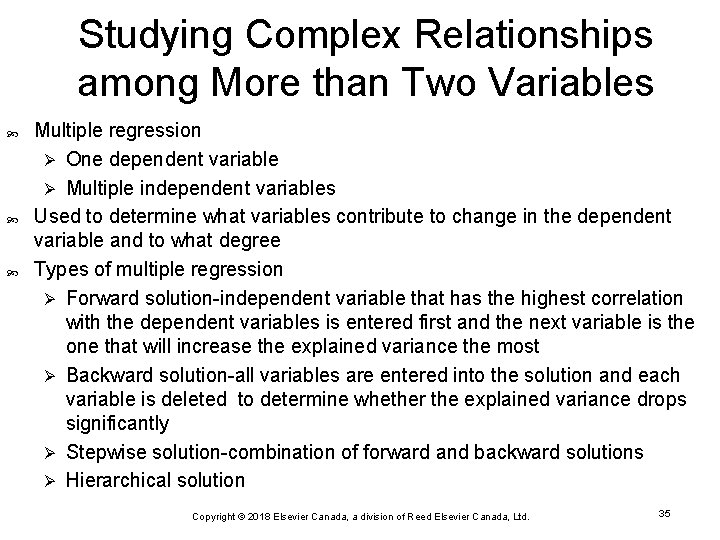
Studying Complex Relationships among More than Two Variables Multiple regression Ø One dependent variable Ø Multiple independent variables Used to determine what variables contribute to change in the dependent variable and to what degree Types of multiple regression Ø Forward solution-independent variable that has the highest correlation with the dependent variables is entered first and the next variable is the one that will increase the explained variance the most Ø Backward solution-all variables are entered into the solution and each variable is deleted to determine whether the explained variance drops significantly Ø Stepwise solution-combination of forward and backward solutions Ø Hierarchical solution Copyright © 2018 Elsevier Canada, a division of Reed Elsevier Canada, Ltd. 35
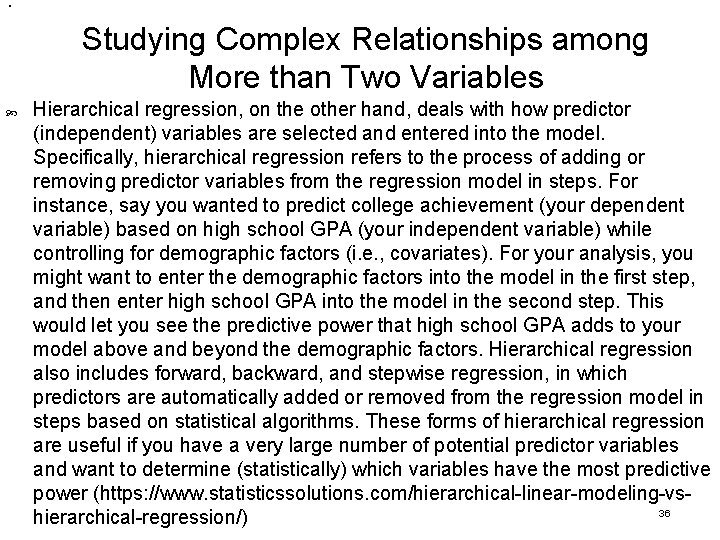
. Studying Complex Relationships among More than Two Variables Hierarchical regression, on the other hand, deals with how predictor (independent) variables are selected and entered into the model. Specifically, hierarchical regression refers to the process of adding or removing predictor variables from the regression model in steps. For instance, say you wanted to predict college achievement (your dependent variable) based on high school GPA (your independent variable) while controlling for demographic factors (i. e. , covariates). For your analysis, you might want to enter the demographic factors into the model in the first step, and then enter high school GPA into the model in the second step. This would let you see the predictive power that high school GPA adds to your model above and beyond the demographic factors. Hierarchical regression also includes forward, backward, and stepwise regression, in which predictors are automatically added or removed from the regression model in steps based on statistical algorithms. These forms of hierarchical regression are useful if you have a very large number of potential predictor variables and want to determine (statistically) which variables have the most predictive power (https: //www. statisticssolutions. com/hierarchical-linear-modeling-vs 36 hierarchical-regression/)
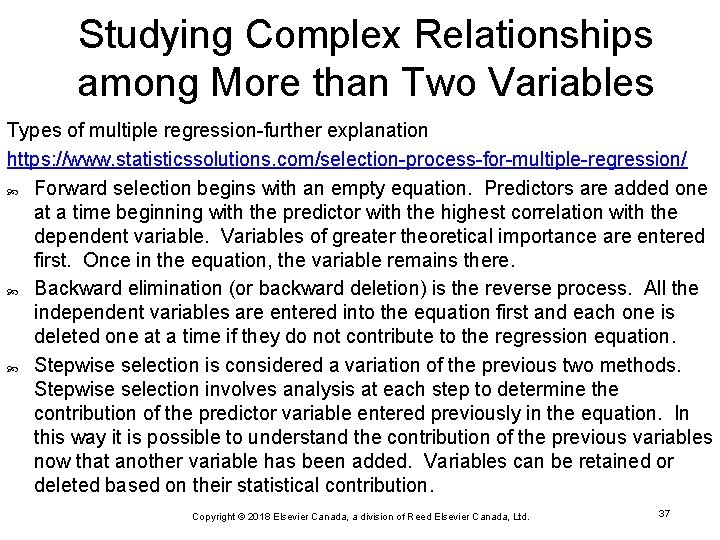
Studying Complex Relationships among More than Two Variables Types of multiple regression-further explanation https: //www. statisticssolutions. com/selection-process-for-multiple-regression/ Forward selection begins with an empty equation. Predictors are added one at a time beginning with the predictor with the highest correlation with the dependent variable. Variables of greater theoretical importance are entered first. Once in the equation, the variable remains there. Backward elimination (or backward deletion) is the reverse process. All the independent variables are entered into the equation first and each one is deleted one at a time if they do not contribute to the regression equation. Stepwise selection is considered a variation of the previous two methods. Stepwise selection involves analysis at each step to determine the contribution of the predictor variable entered previously in the equation. In this way it is possible to understand the contribution of the previous variables now that another variable has been added. Variables can be retained or deleted based on their statistical contribution. Copyright © 2018 Elsevier Canada, a division of Reed Elsevier Canada, Ltd. 37
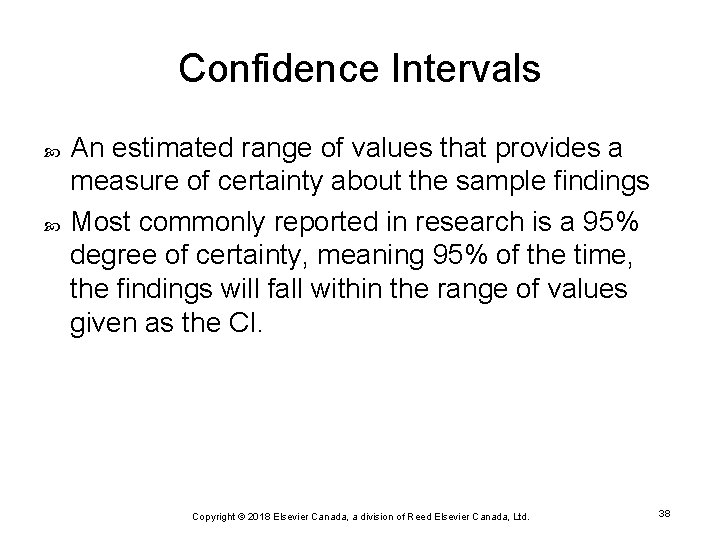
Confidence Intervals An estimated range of values that provides a measure of certainty about the sample findings Most commonly reported in research is a 95% degree of certainty, meaning 95% of the time, the findings will fall within the range of values given as the CI. Copyright © 2018 Elsevier Canada, a division of Reed Elsevier Canada, Ltd. 38
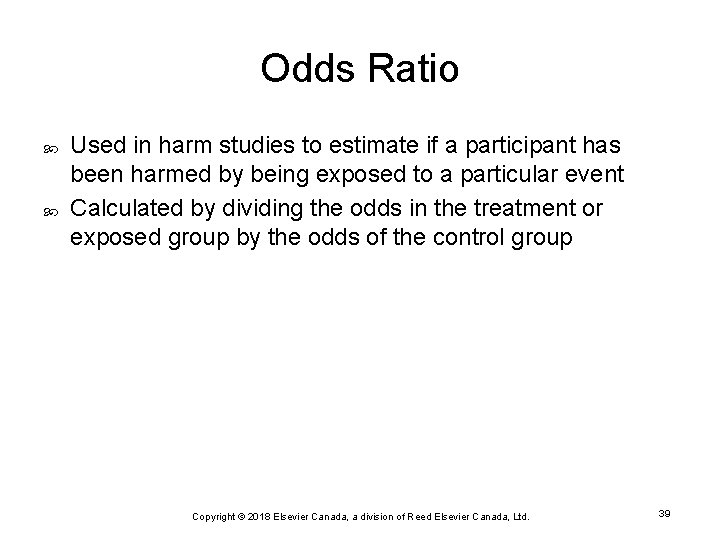
Odds Ratio Used in harm studies to estimate if a participant has been harmed by being exposed to a particular event Calculated by dividing the odds in the treatment or exposed group by the odds of the control group Copyright © 2018 Elsevier Canada, a division of Reed Elsevier Canada, Ltd. 39
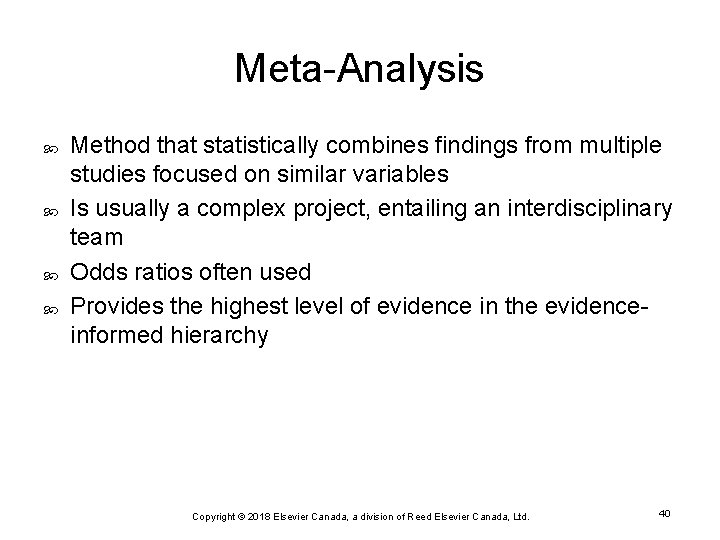
Meta-Analysis Method that statistically combines findings from multiple studies focused on similar variables Is usually a complex project, entailing an interdisciplinary team Odds ratios often used Provides the highest level of evidence in the evidenceinformed hierarchy Copyright © 2018 Elsevier Canada, a division of Reed Elsevier Canada, Ltd. 40
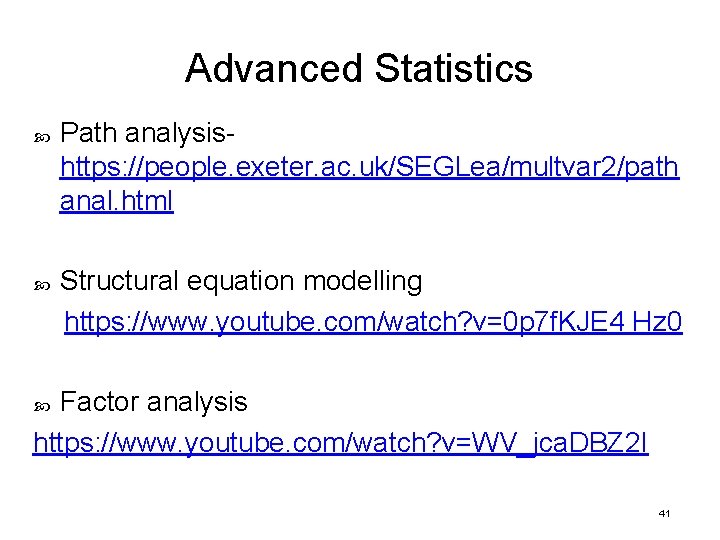
Advanced Statistics Path analysis- https: //people. exeter. ac. uk/SEGLea/multvar 2/path anal. html Structural equation modelling https: //www. youtube. com/watch? v=0 p 7 f. KJE 4 Hz 0 Factor analysis https: //www. youtube. com/watch? v=WV_jca. DBZ 2 I 41
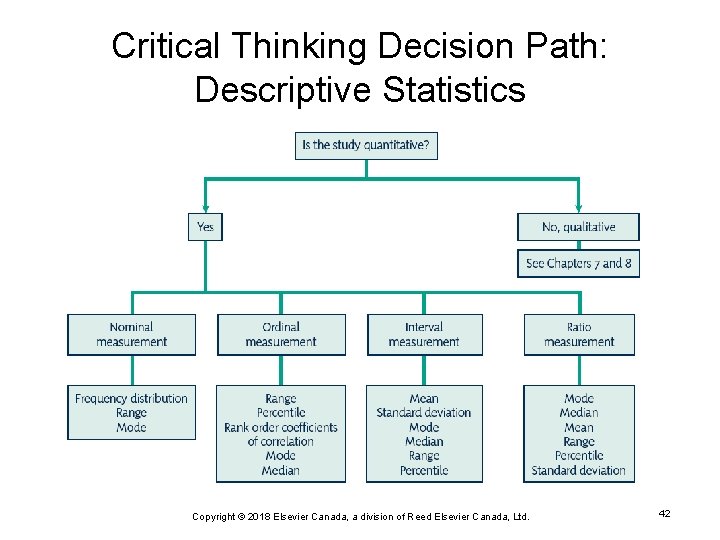
Critical Thinking Decision Path: Descriptive Statistics Copyright © 2018 Elsevier Canada, a division of Reed Elsevier Canada, Ltd. 42
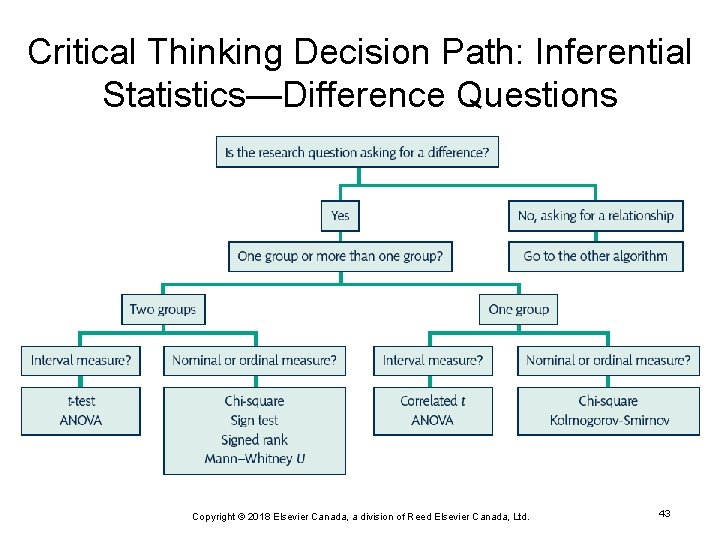
Critical Thinking Decision Path: Inferential Statistics—Difference Questions Copyright © 2018 Elsevier Canada, a division of Reed Elsevier Canada, Ltd. 43
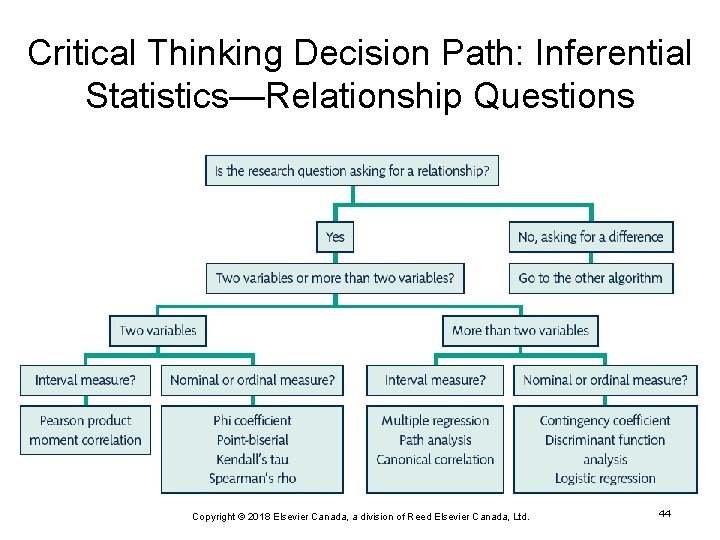
Critical Thinking Decision Path: Inferential Statistics—Relationship Questions Copyright © 2018 Elsevier Canada, a division of Reed Elsevier Canada, Ltd. 44
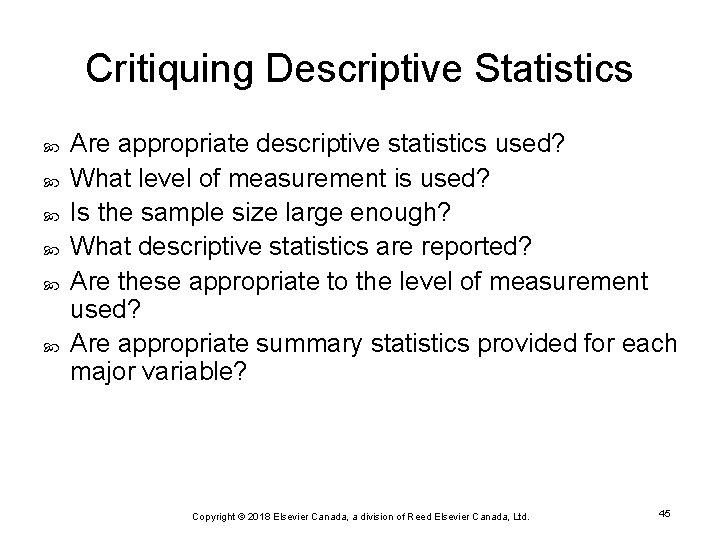
Critiquing Descriptive Statistics Are appropriate descriptive statistics used? What level of measurement is used? Is the sample size large enough? What descriptive statistics are reported? Are these appropriate to the level of measurement used? Are appropriate summary statistics provided for each major variable? Copyright © 2018 Elsevier Canada, a division of Reed Elsevier Canada, Ltd. 45
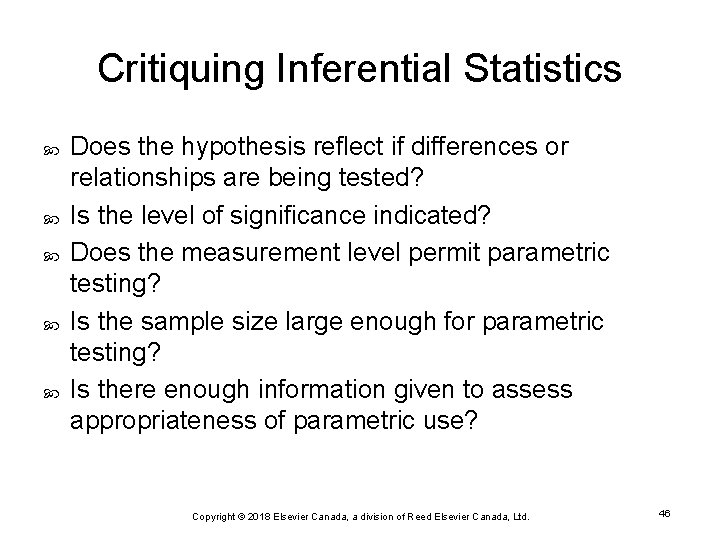
Critiquing Inferential Statistics Does the hypothesis reflect if differences or relationships are being tested? Is the level of significance indicated? Does the measurement level permit parametric testing? Is the sample size large enough for parametric testing? Is there enough information given to assess appropriateness of parametric use? Copyright © 2018 Elsevier Canada, a division of Reed Elsevier Canada, Ltd. 46
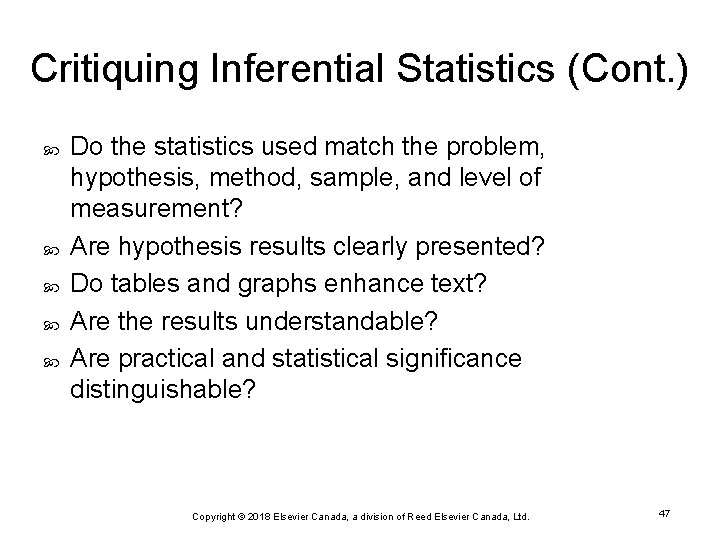
Critiquing Inferential Statistics (Cont. ) Do the statistics used match the problem, hypothesis, method, sample, and level of measurement? Are hypothesis results clearly presented? Do tables and graphs enhance text? Are the results understandable? Are practical and statistical significance distinguishable? Copyright © 2018 Elsevier Canada, a division of Reed Elsevier Canada, Ltd. 47
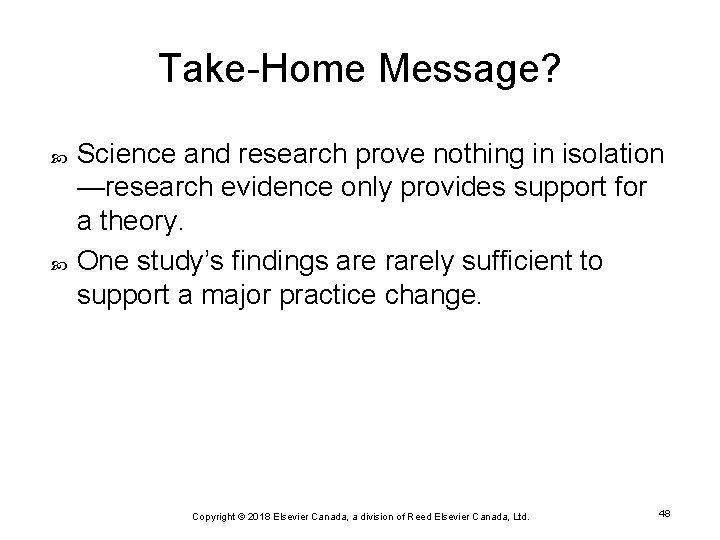
Take-Home Message? Science and research prove nothing in isolation —research evidence only provides support for a theory. One study’s findings are rarely sufficient to support a major practice change. Copyright © 2018 Elsevier Canada, a division of Reed Elsevier Canada, Ltd. 48
Copyright © 2018
Copyright © 2018 all rights reserved
Que letra continua m v t m j
Data gathering for quantitative research
Who is pictured above?
Quantitative vs qualitative data collection
T test quantitative research
Bad research questions
Qualitative data quantitative data
Quantitative analysis for management chapter 3 answers
Summarizing quantitative data
Elsevier statdx
Ees elsevier
Elsevier vs ieee
Elsevier clinical key
Elsevier 2005
Pancreas divisum surgery
Descending limb of nephron loop
General sense
Elsevier
Elsevier
Ees elsevier
Elsevier
Elsevier
Elsevier
Elsevier
Elsevier
Elsevier
Raexys
Elsevier
Elsevier
Elsevier
Elsevier
Ees elsevier
Springer, elsevier, taylor & francis etc. are ______. *
Reaxys elsevier
Chapter 8 skin disorders and diseases
Chapter 52 removable prosthodontics short answers
Elsevier
Elsevier
Elsevier
Elsevier
Elsevier
What is the function of anus
Ovario
7:4 skeletal system
Hivebench elsevier
Elsevier
General