Using the maximum entropy production principle to understand
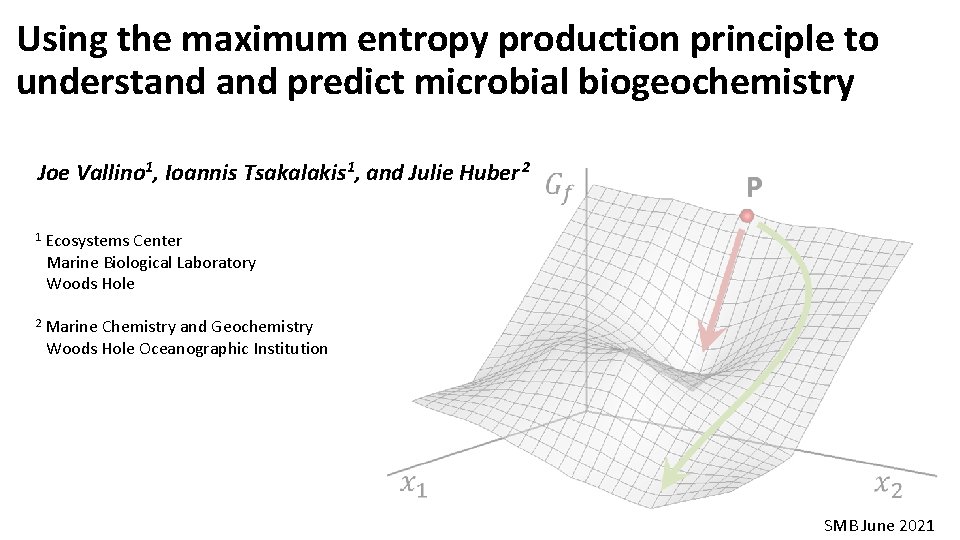
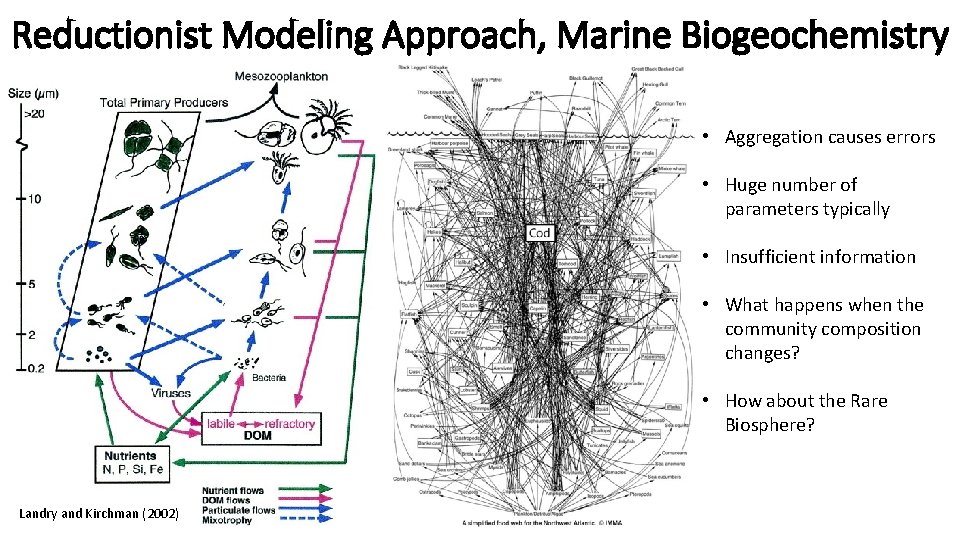
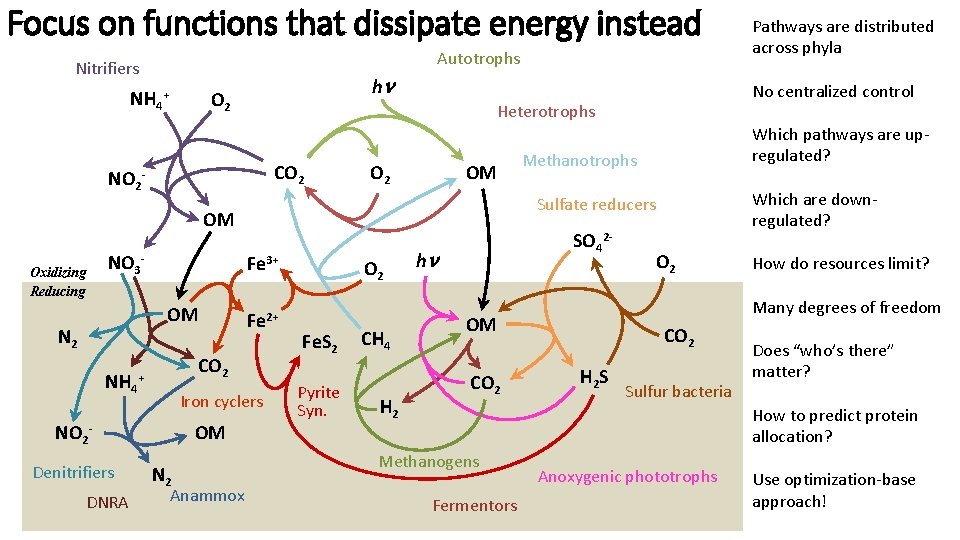
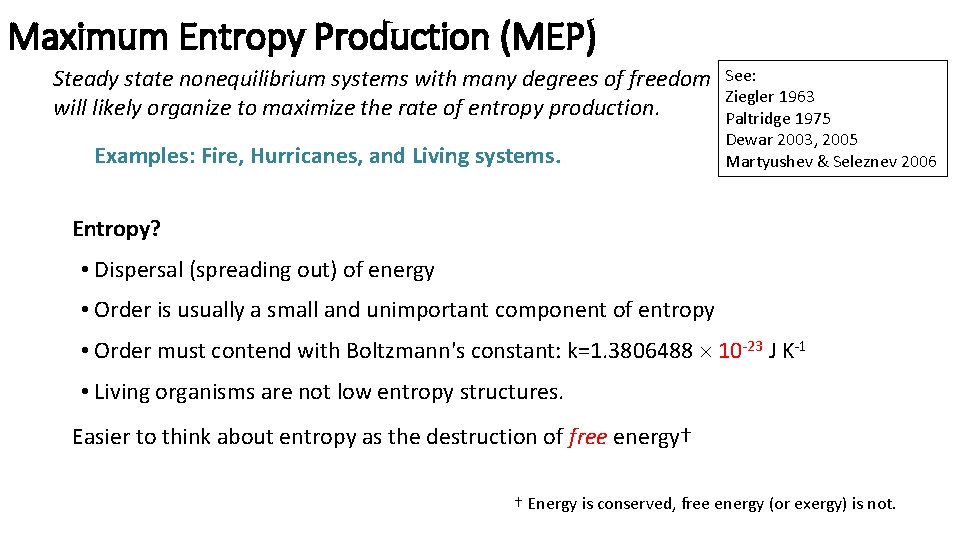
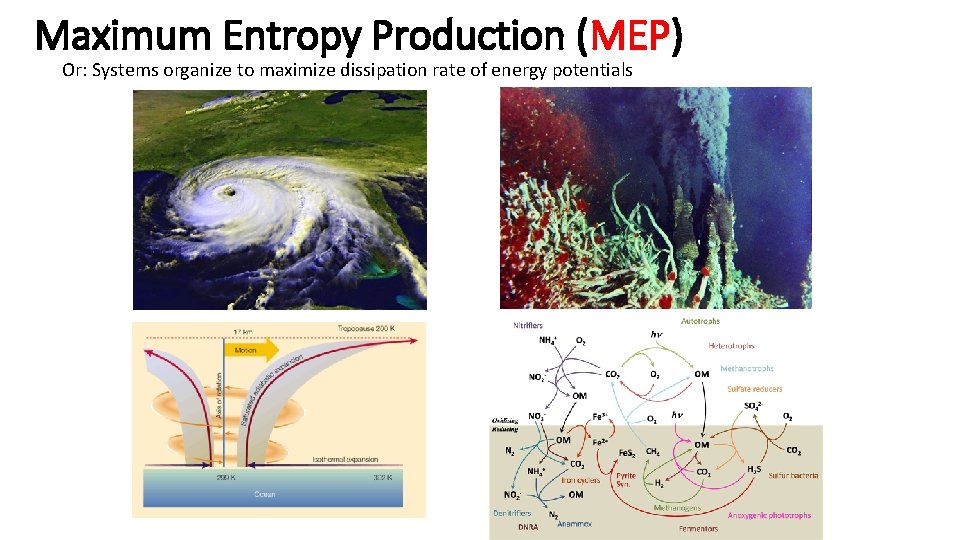
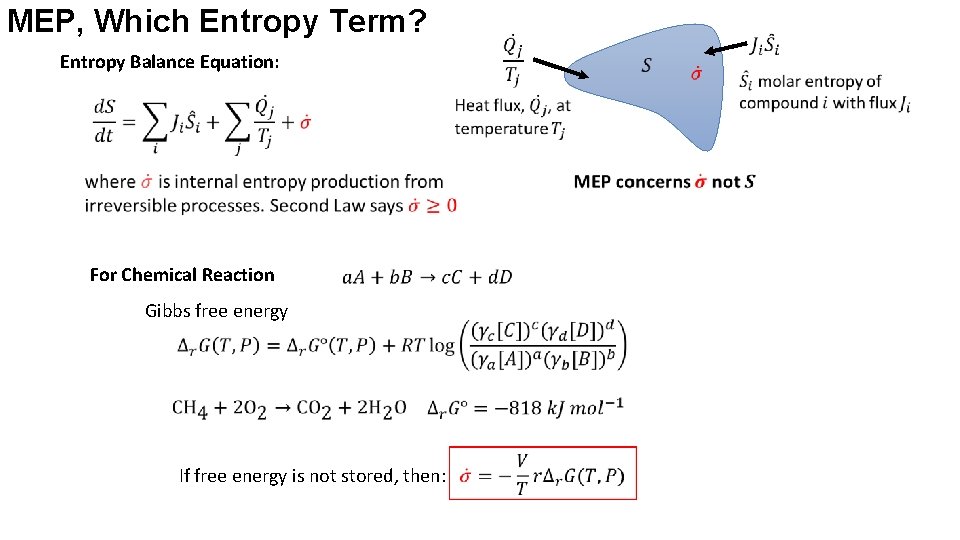
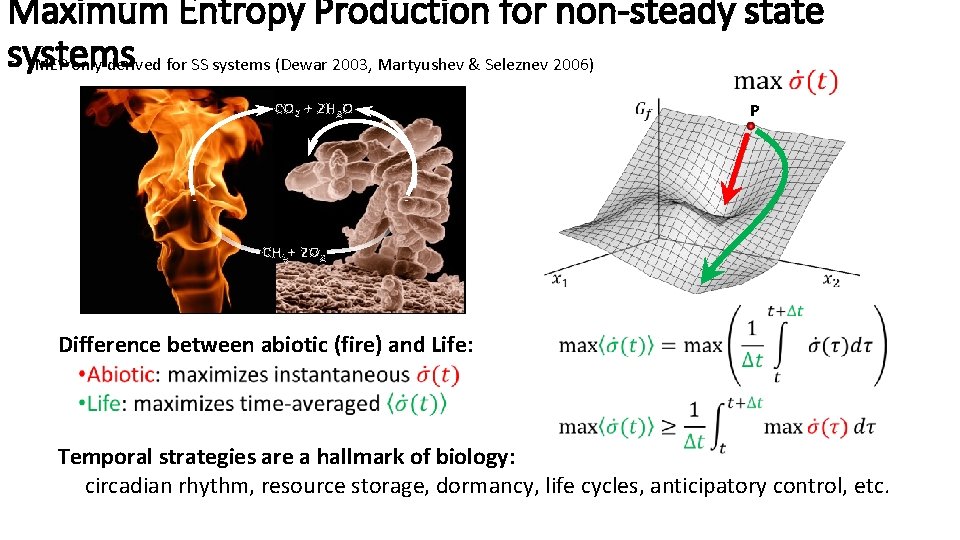
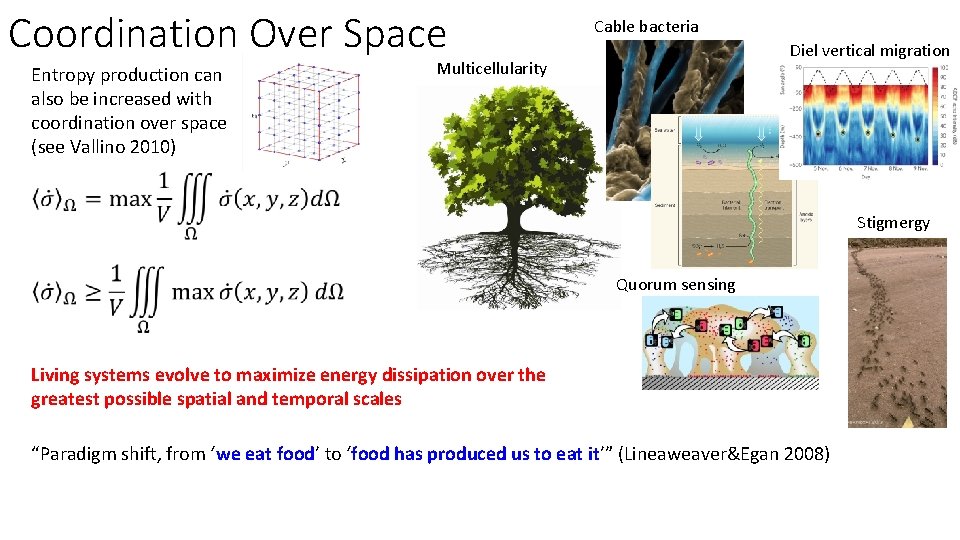
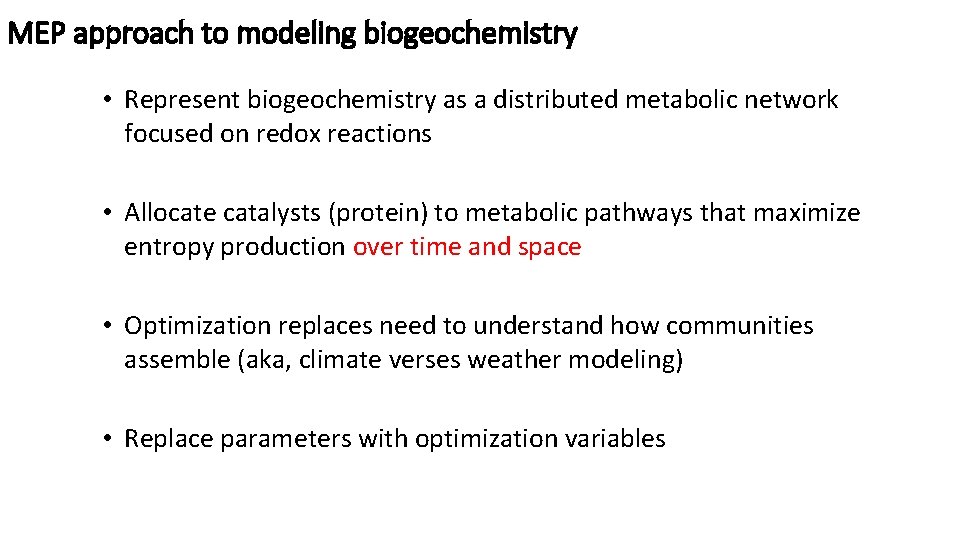
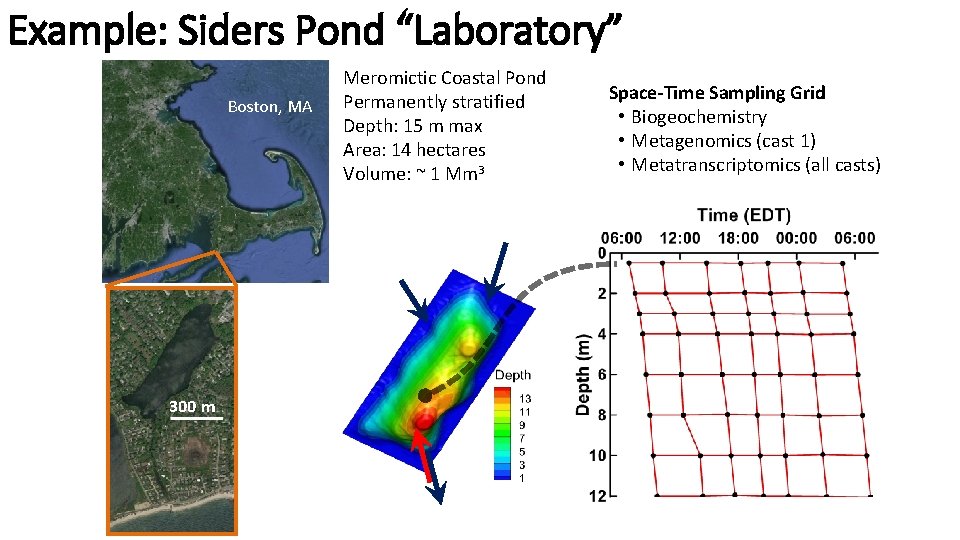
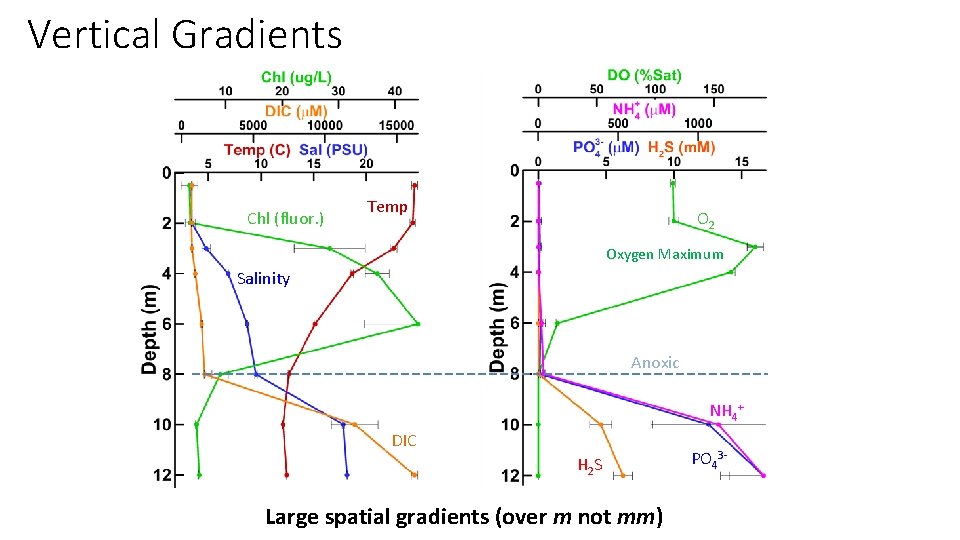
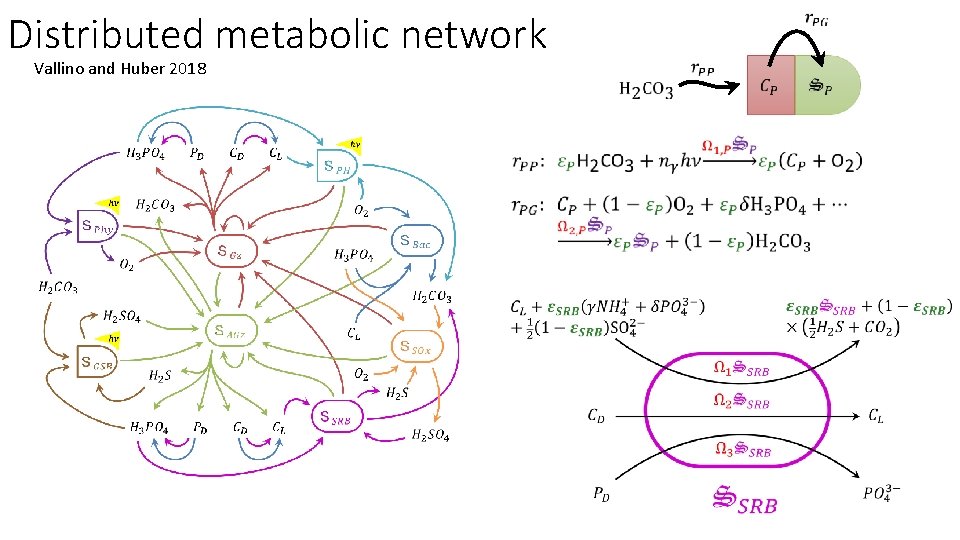
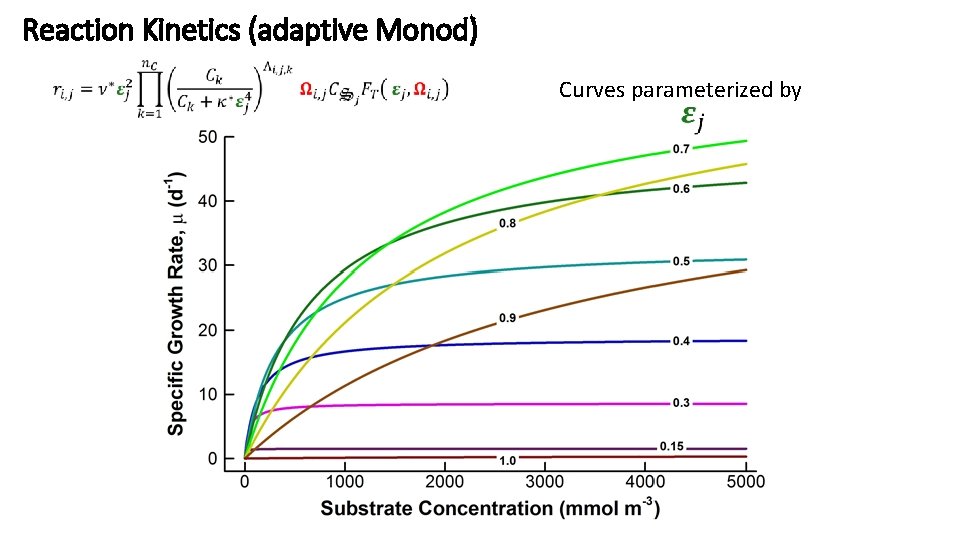
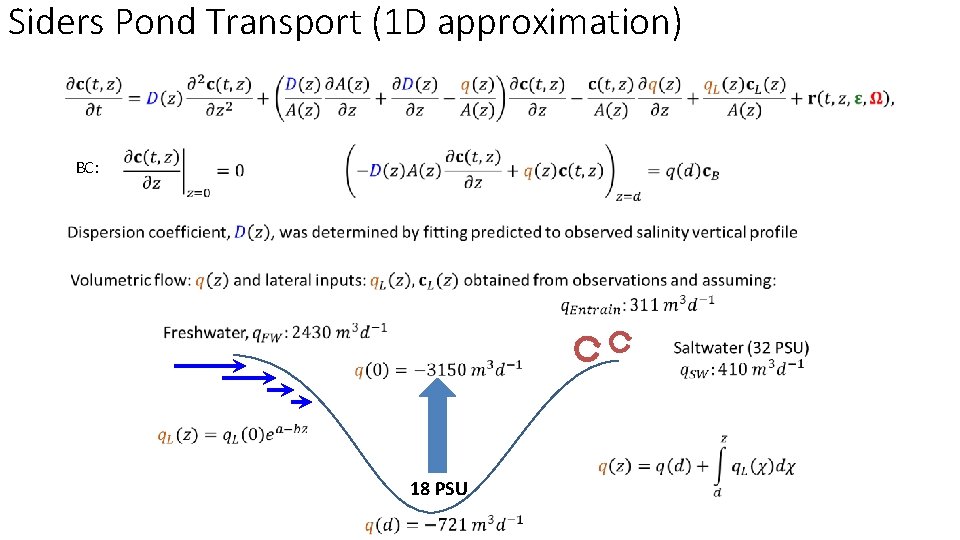
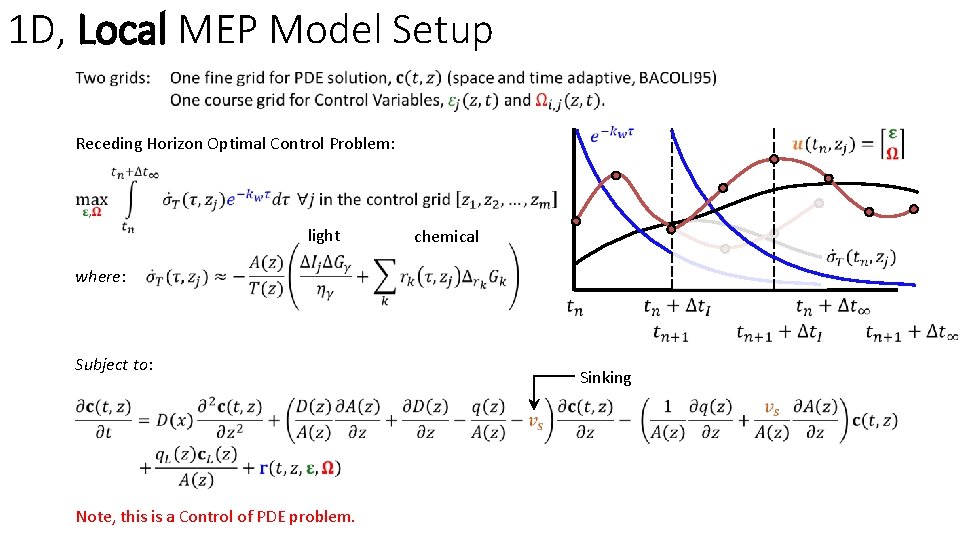
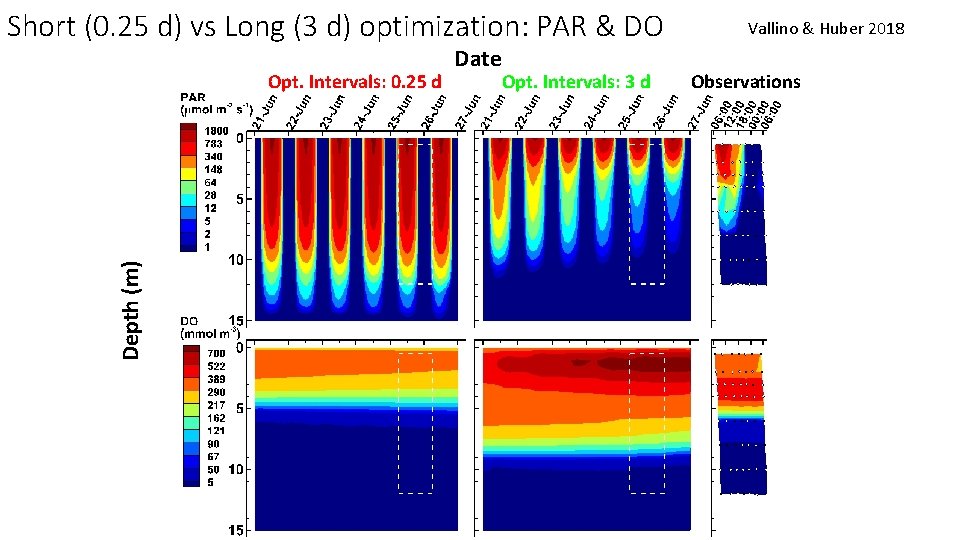
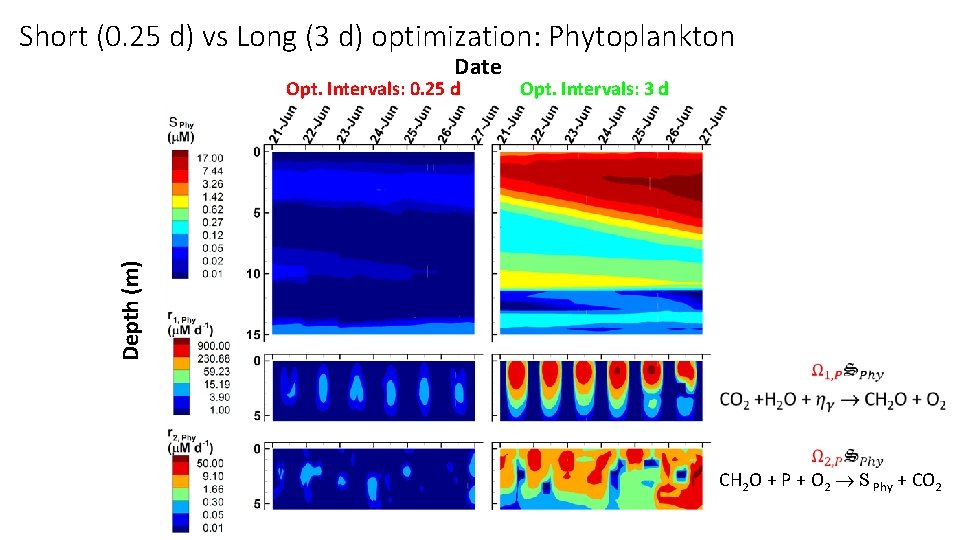
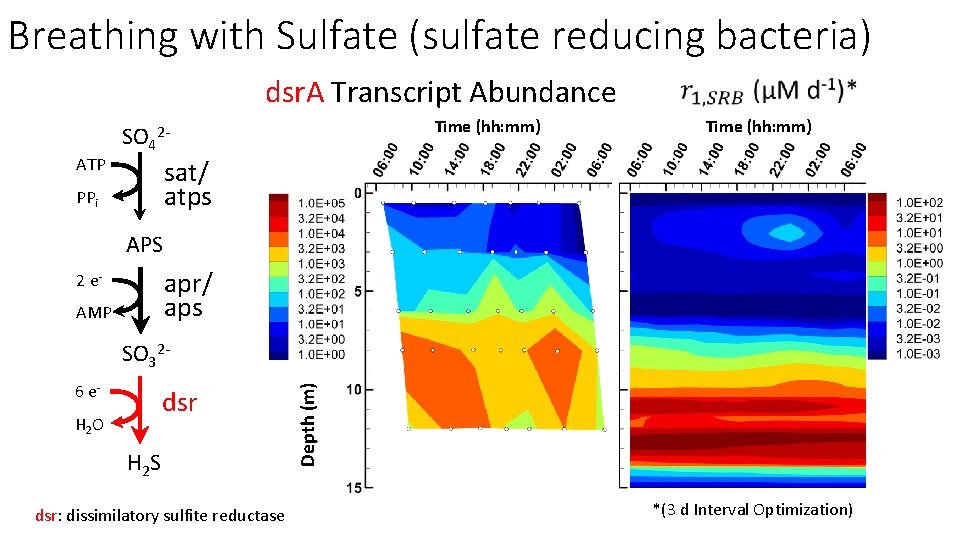
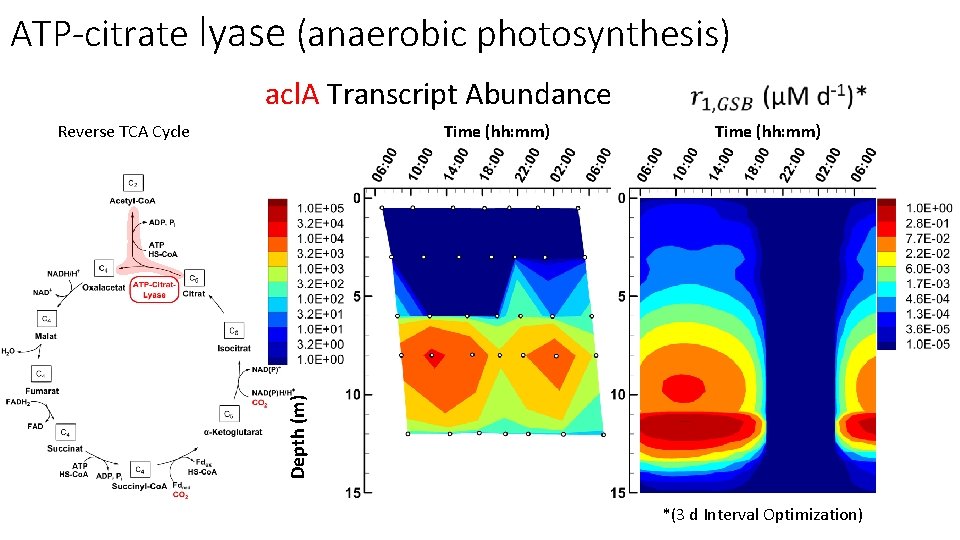
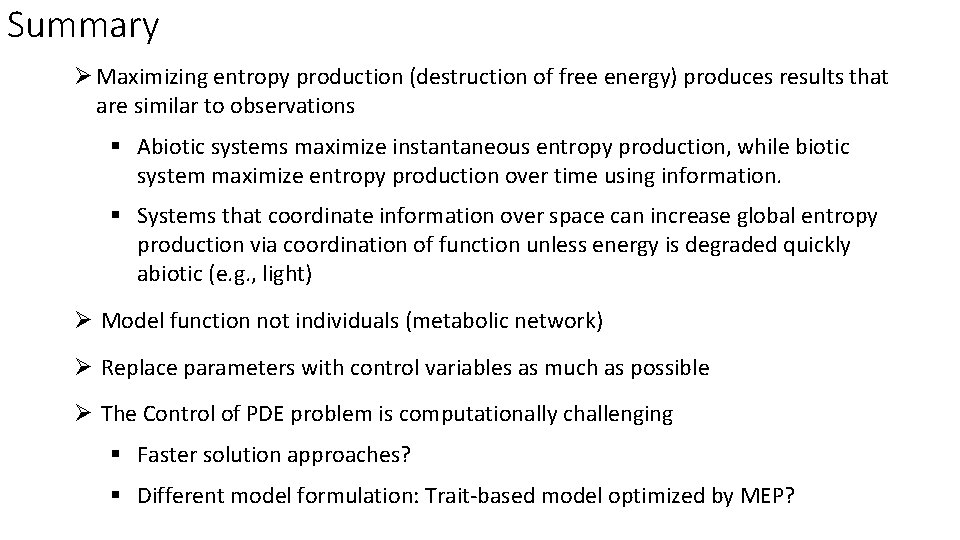
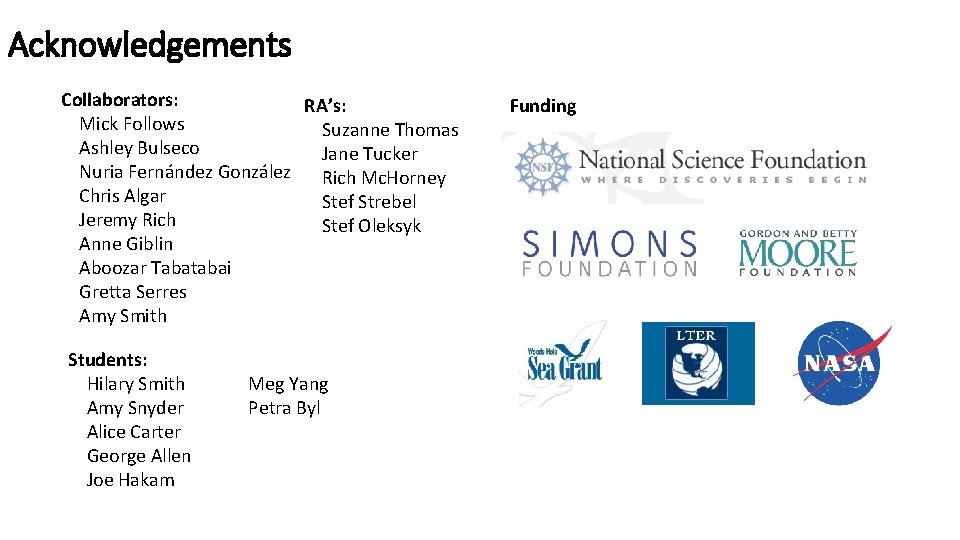
- Slides: 21
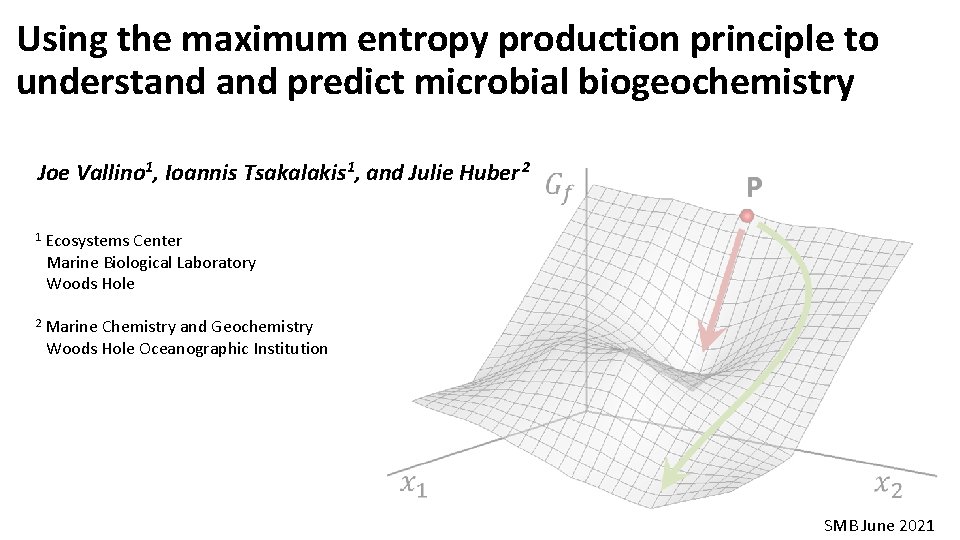
Using the maximum entropy production principle to understand predict microbial biogeochemistry Joe Vallino 1, Ioannis Tsakalakis 1, and Julie Huber 2 1 Ecosystems Center Marine Biological Laboratory Woods Hole 2 Marine Chemistry and Geochemistry Woods Hole Oceanographic Institution SMB June 2021
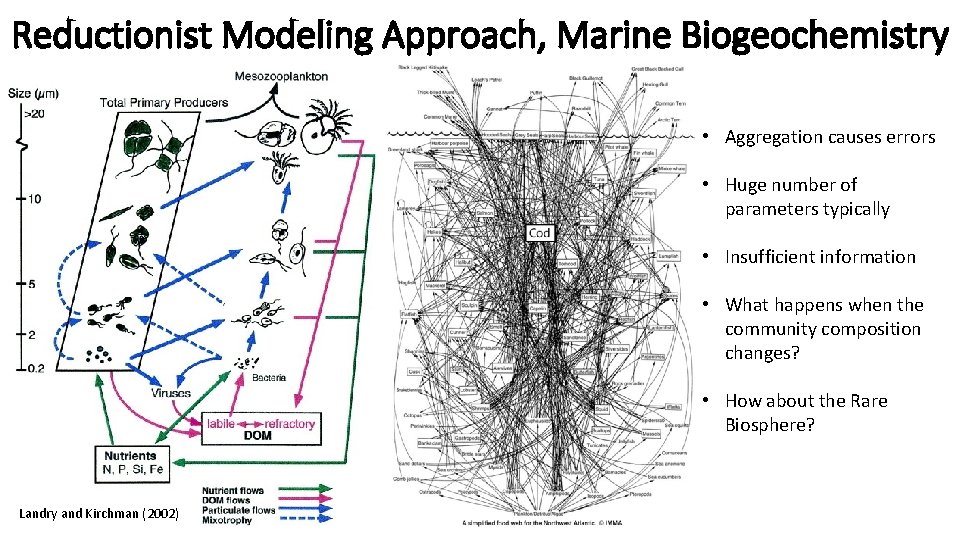
Reductionist Modeling Approach, Marine Biogeochemistry • Aggregation causes errors • Huge number of parameters typically • Insufficient information • What happens when the community composition changes? • How about the Rare Biosphere? Landry and Kirchman (2002)
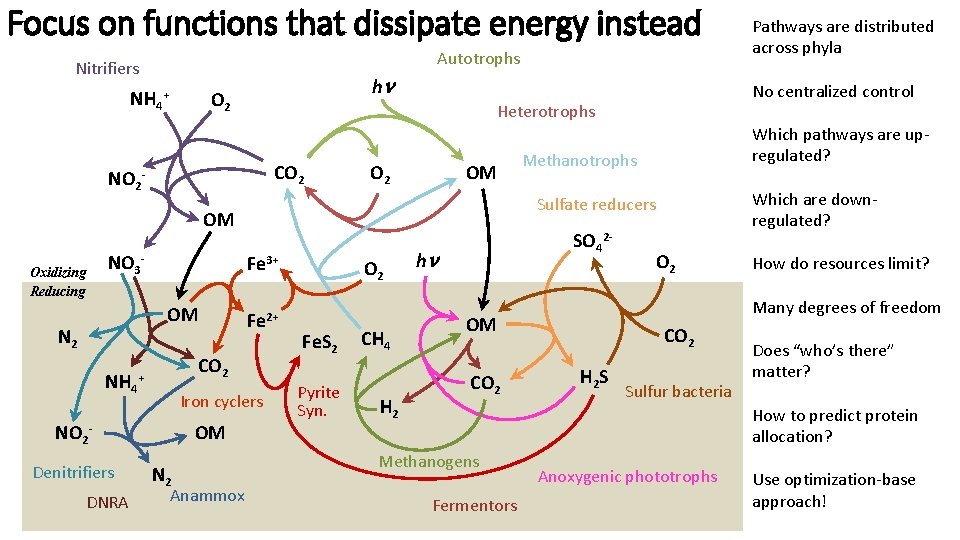
Focus on functions that dissipate energy instead Autotrophs Nitrifiers NH 4+ NO 2 h O 2 CO 2 OM Oxidizing Reducing Fe 3+ OM N 2 NH 4 NO 2 Iron cyclers OM - Denitrifiers DNRA Fe 2+ CO 2 + N 2 Anammox Which pathways are upregulated? Methanotrophs Which are downregulated? Sulfate reducers OM NO 3 - No centralized control Heterotrophs - O 2 Fe. S 2 Pyrite Syn. CH 4 H 2 SO 42 - h O 2 How do resources limit? Many degrees of freedom OM CO 2 Pathways are distributed across phyla CO 2 H 2 S Sulfur bacteria Does “who’s there” matter? How to predict protein allocation? Methanogens Fermentors Anoxygenic phototrophs Use optimization-base approach!
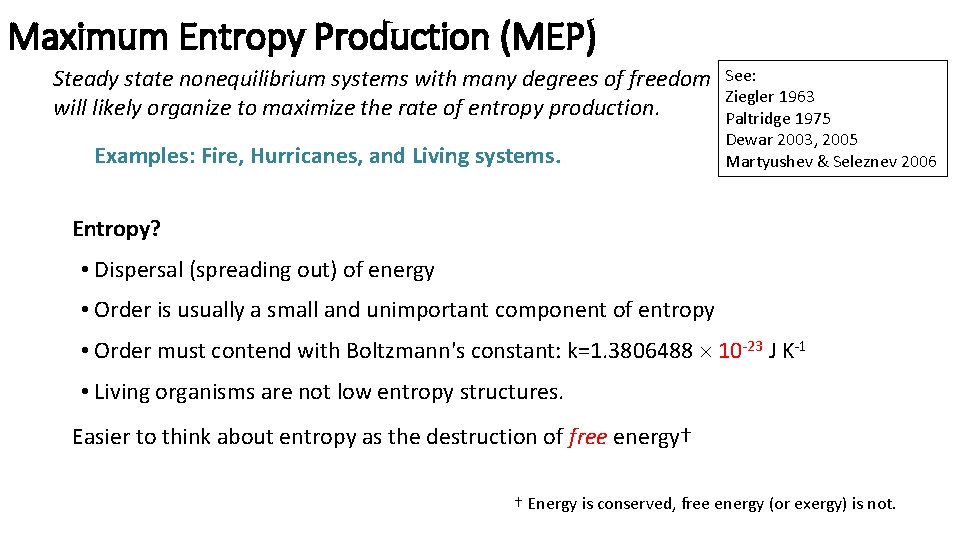
Maximum Entropy Production (MEP) Steady state nonequilibrium systems with many degrees of freedom will likely organize to maximize the rate of entropy production. Examples: Fire, Hurricanes, and Living systems. See: Ziegler 1963 Paltridge 1975 Dewar 2003, 2005 Martyushev & Seleznev 2006 Entropy? • Dispersal (spreading out) of energy • Order is usually a small and unimportant component of entropy • Order must contend with Boltzmann's constant: k=1. 3806488 10 -23 J K-1 • Living organisms are not low entropy structures. Easier to think about entropy as the destruction of free energy† † Energy is conserved, free energy (or exergy) is not.
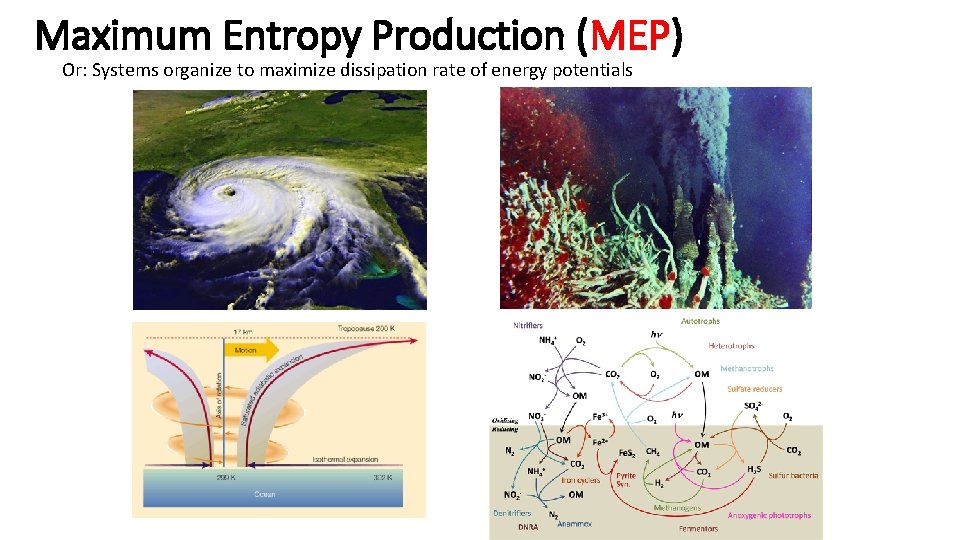
Maximum Entropy Production (MEP) Or: Systems organize to maximize dissipation rate of energy potentials
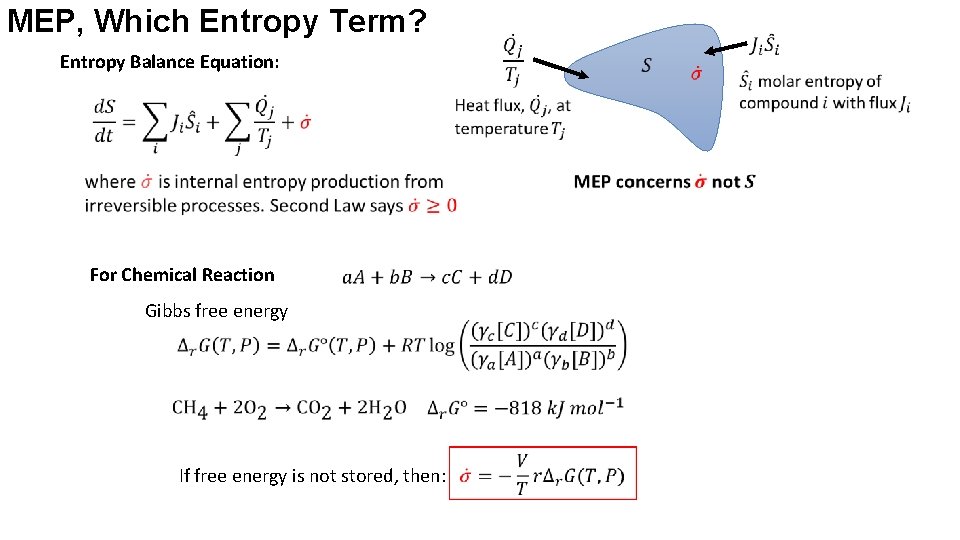
MEP, Which Entropy Term? Entropy Balance Equation: For Chemical Reaction Gibbs free energy If free energy is not stored, then:
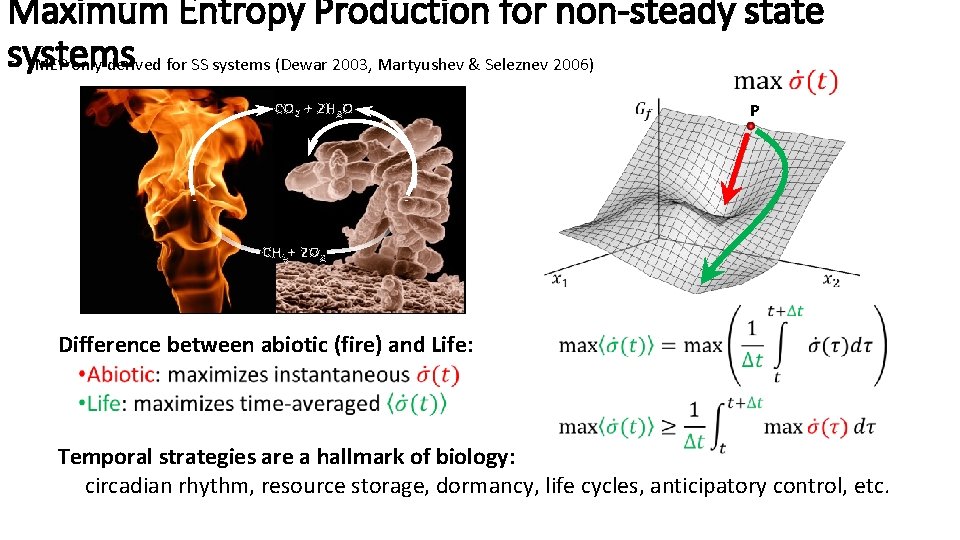
Maximum Entropy Production for non-steady state systems MEP only derived for SS systems (Dewar 2003, Martyushev & Seleznev 2006) CO 2 + 2 H 2 O P CH 4 + 2 O 2 Difference between abiotic (fire) and Life: Temporal strategies are a hallmark of biology: circadian rhythm, resource storage, dormancy, life cycles, anticipatory control, etc.
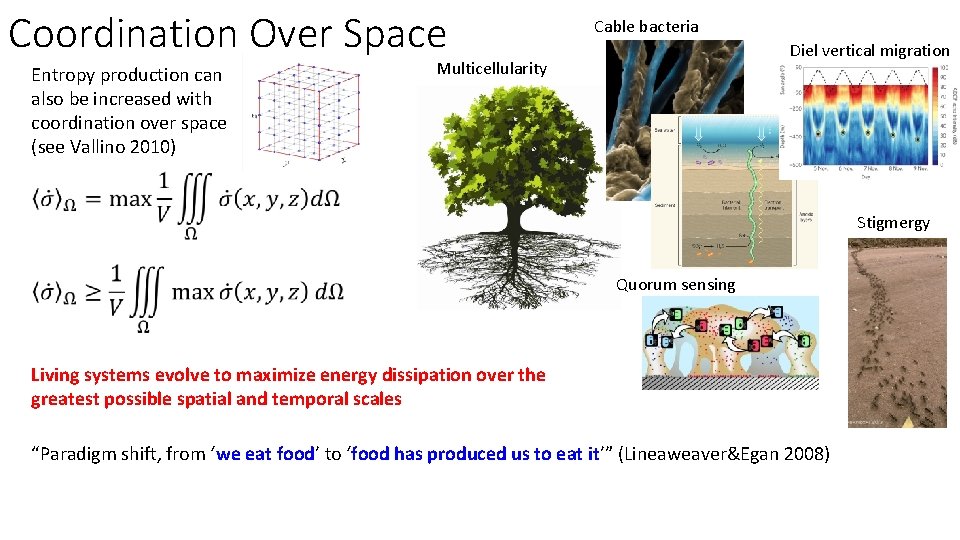
Coordination Over Space Entropy production can also be increased with coordination over space (see Vallino 2010) Cable bacteria Diel vertical migration Multicellularity Stigmergy Quorum sensing Living systems evolve to maximize energy dissipation over the greatest possible spatial and temporal scales “Paradigm shift, from ‘we eat food’ to ‘food has produced us to eat it’” (Lineaweaver&Egan 2008)
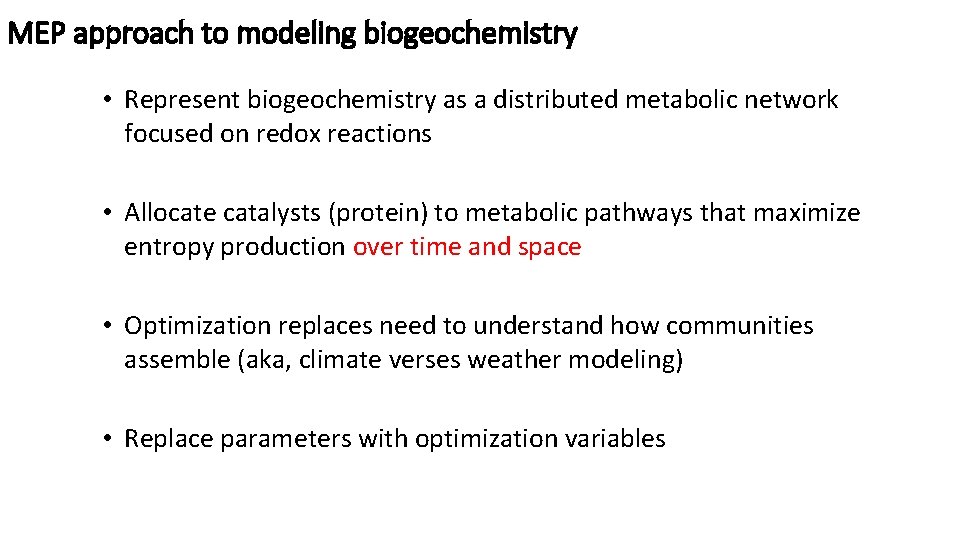
MEP approach to modeling biogeochemistry • Represent biogeochemistry as a distributed metabolic network focused on redox reactions • Allocate catalysts (protein) to metabolic pathways that maximize entropy production over time and space • Optimization replaces need to understand how communities assemble (aka, climate verses weather modeling) • Replace parameters with optimization variables
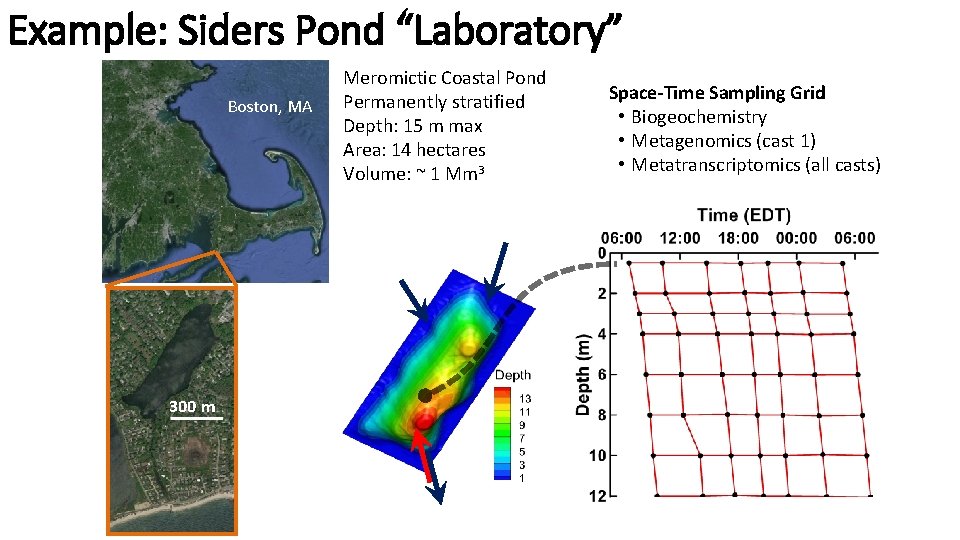
Example: Siders Pond “Laboratory” Boston, MA 300 m Meromictic Coastal Pond Permanently stratified Depth: 15 m max Area: 14 hectares Volume: ~ 1 Mm 3 Space-Time Sampling Grid • Biogeochemistry • Metagenomics (cast 1) • Metatranscriptomics (all casts)
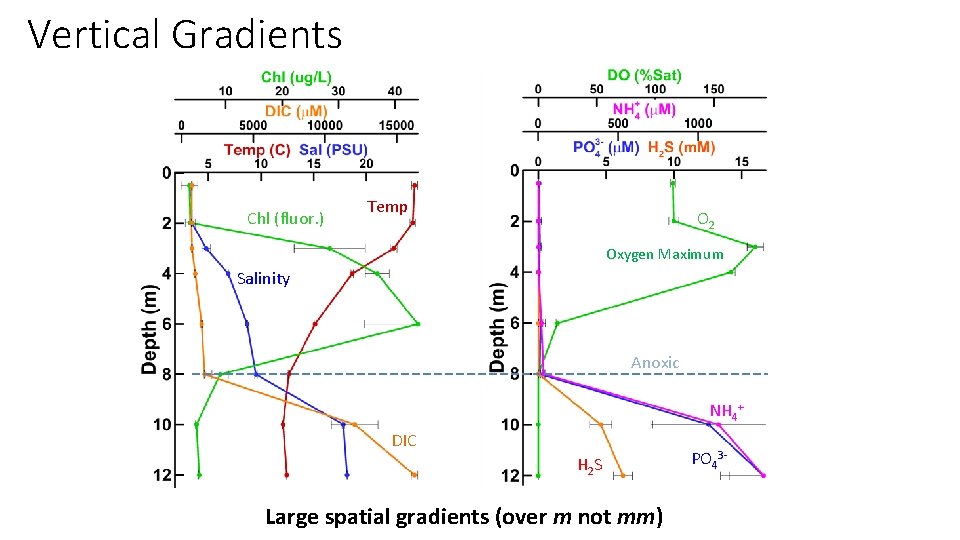
Vertical Gradients Chl (fluor. ) Temp O 2 Oxygen Maximum Salinity Anoxic NH 4+ DIC H 2 S Large spatial gradients (over m not mm) PO 43 -
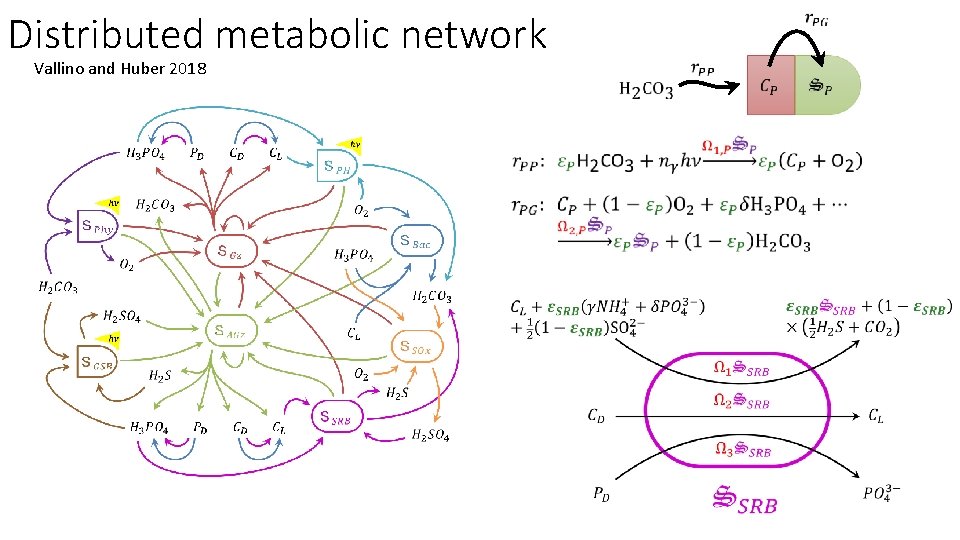
Distributed metabolic network Vallino and Huber 2018
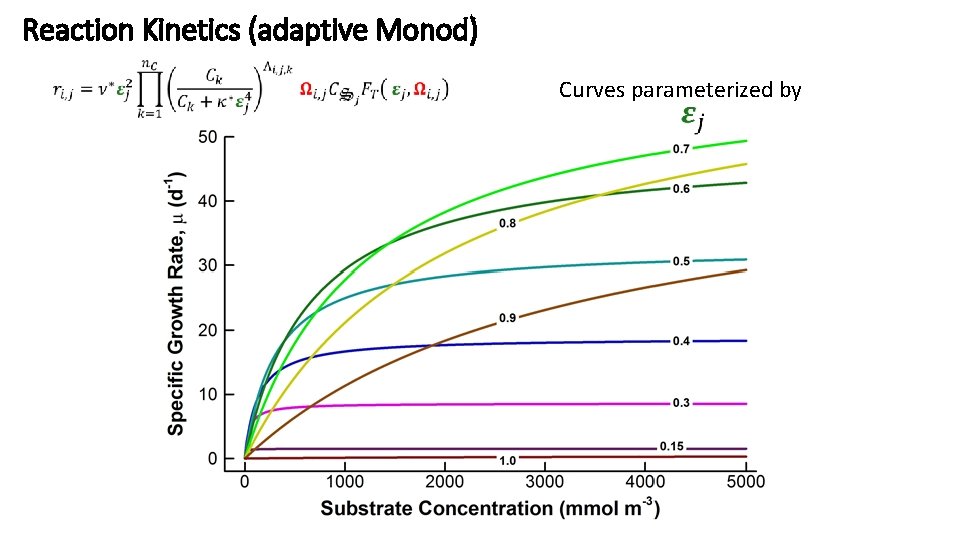
Reaction Kinetics (adaptive Monod) Curves parameterized by
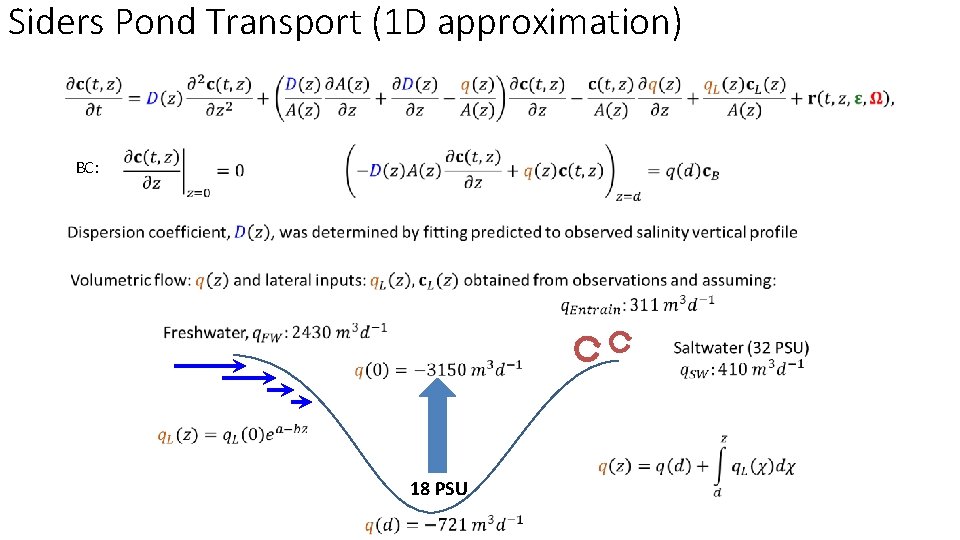
Siders Pond Transport (1 D approximation) BC: 18 PSU
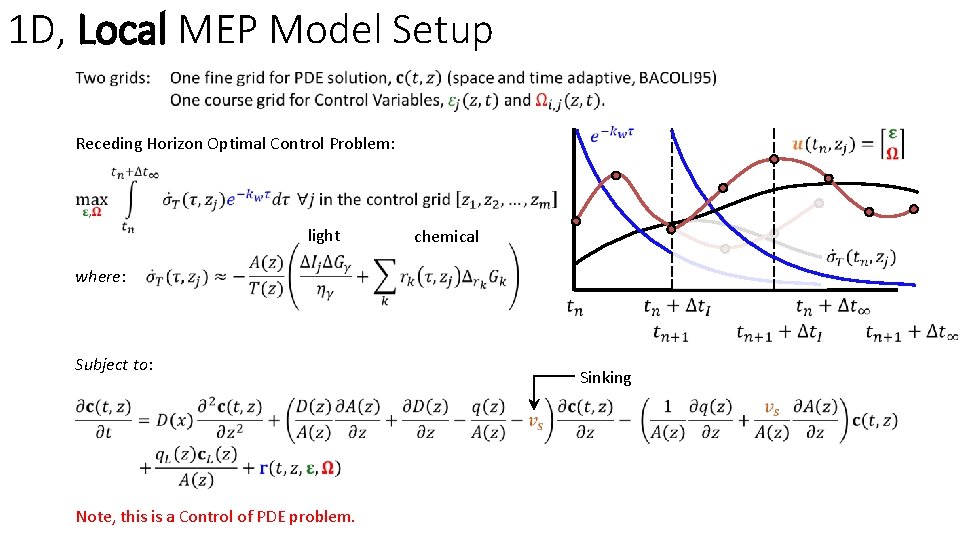
1 D, Local MEP Model Setup Receding Horizon Optimal Control Problem: light chemical where: Subject to: Note, this is a Control of PDE problem. Sinking
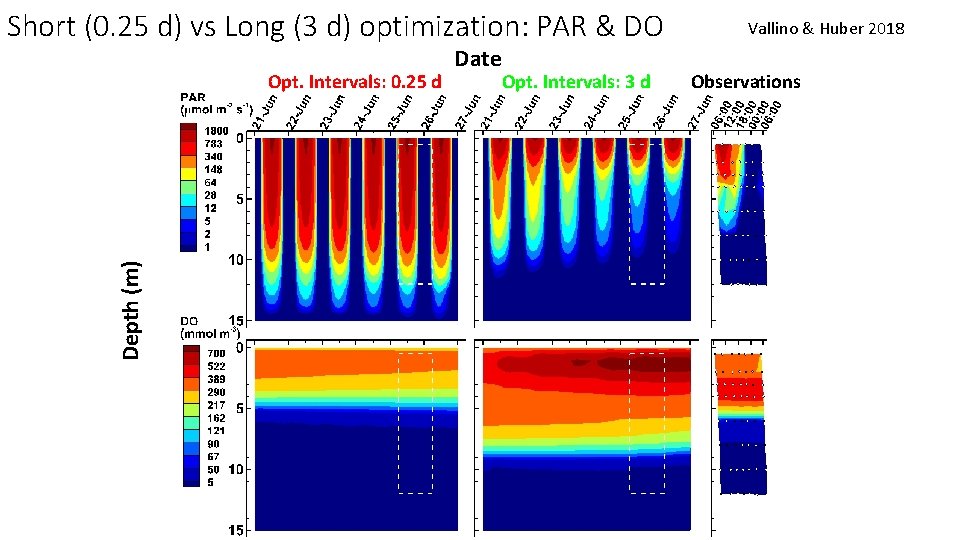
Short (0. 25 d) vs Long (3 d) optimization: PAR & DO Depth (m) Opt. Intervals: 0. 25 d Date Opt. Intervals: 3 d Vallino & Huber 2018 Observations
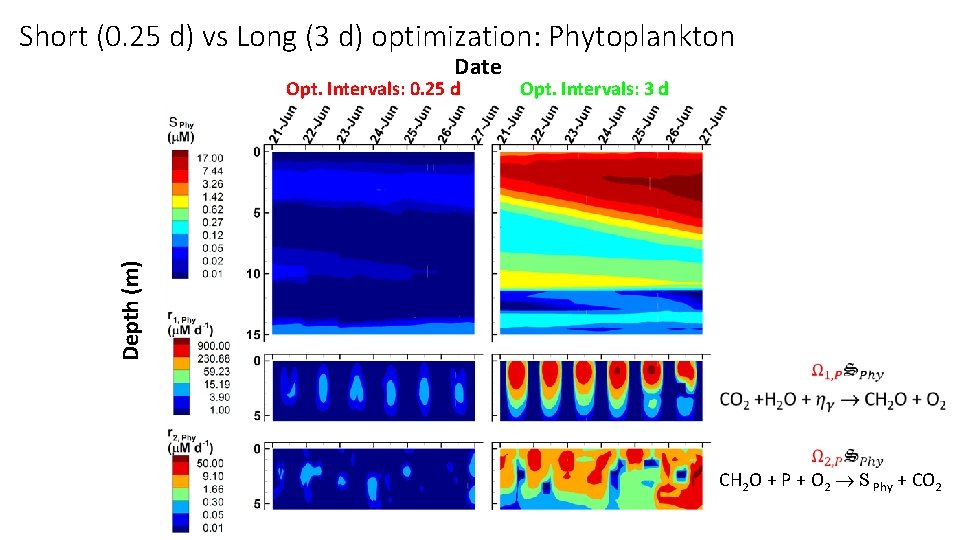
Short (0. 25 d) vs Long (3 d) optimization: Phytoplankton Date Opt. Intervals: 3 d Depth (m) Opt. Intervals: 0. 25 d CH 2 O + P + O 2 S Phy + CO 2
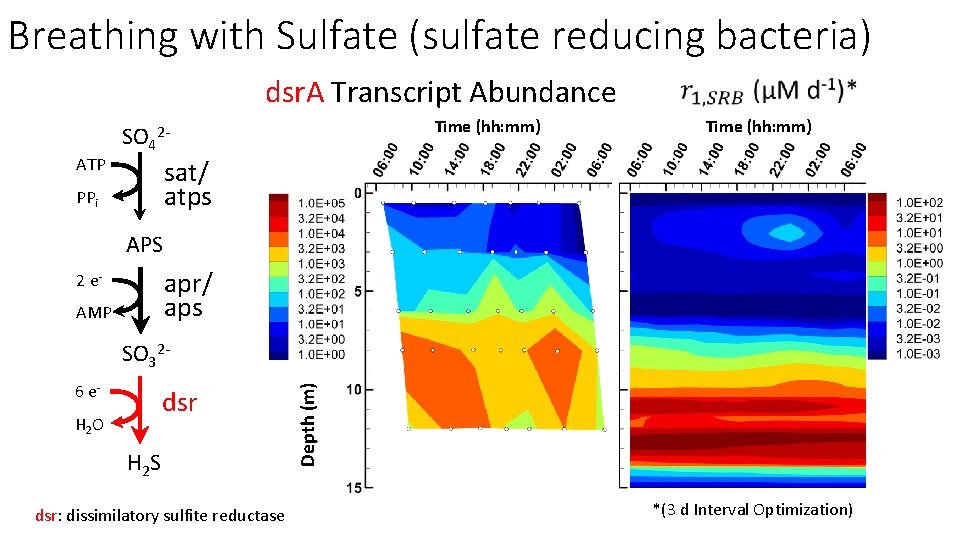
Breathing with Sulfate (sulfate reducing bacteria) dsr. A Transcript Abundance ATP Time (hh: mm) SO 42 - Time (hh: mm) sat/ atps PPi APS apr/ aps 2 e. AMP 6 e- dsr H 2 O H 2 S dsr: dissimilatory sulfite reductase Depth (m) SO 32 - *(3 d Interval Optimization)
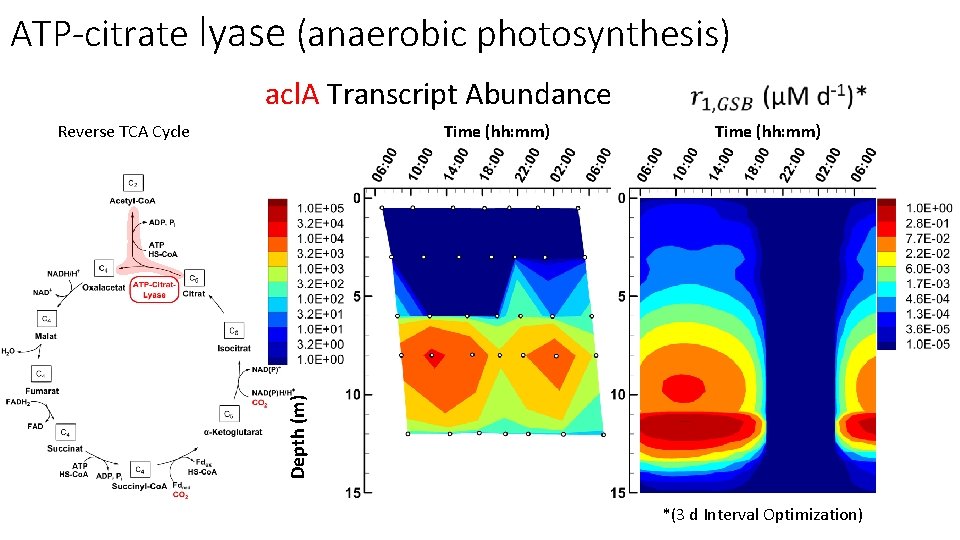
ATP-citrate lyase (anaerobic photosynthesis) acl. A Transcript Abundance Time (hh: mm) Depth (m) Reverse TCA Cycle *(3 d Interval Optimization)
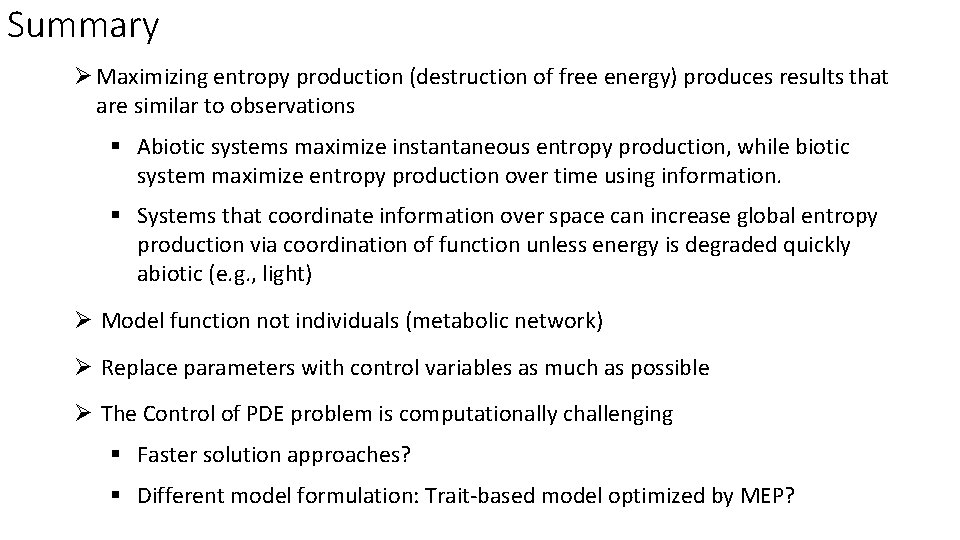
Summary Ø Maximizing entropy production (destruction of free energy) produces results that are similar to observations § Abiotic systems maximize instantaneous entropy production, while biotic system maximize entropy production over time using information. § Systems that coordinate information over space can increase global entropy production via coordination of function unless energy is degraded quickly abiotic (e. g. , light) Ø Model function not individuals (metabolic network) Ø Replace parameters with control variables as much as possible Ø The Control of PDE problem is computationally challenging § Faster solution approaches? § Different model formulation: Trait-based model optimized by MEP?
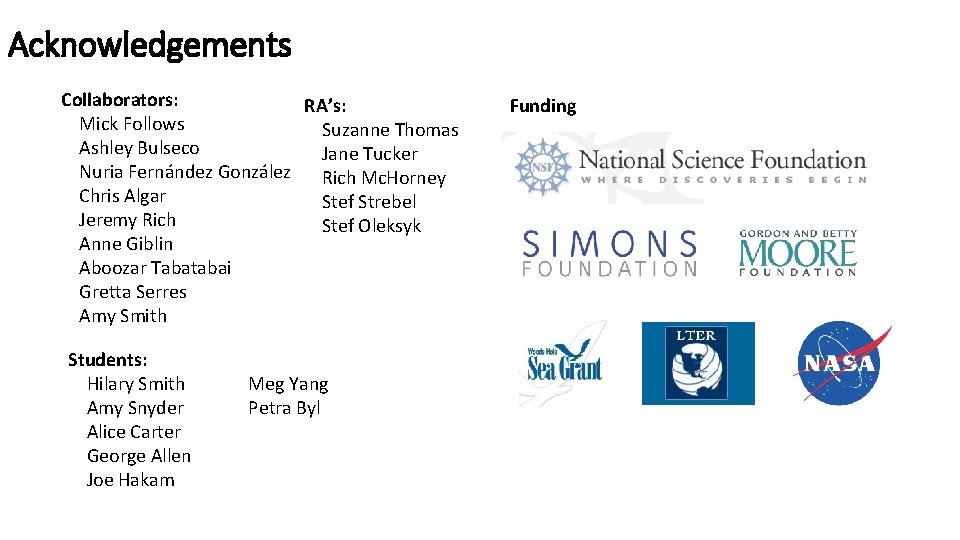
Acknowledgements Collaborators: RA’s: Mick Follows Suzanne Thomas Ashley Bulseco Jane Tucker Nuria Fernández González Rich Mc. Horney Chris Algar Stef Strebel Jeremy Rich Stef Oleksyk Anne Giblin Aboozar Tabatabai Gretta Serres Amy Smith Students: Hilary Smith Amy Snyder Alice Carter George Allen Joe Hakam Meg Yang Petra Byl Funding
Pre-production adalah
Enthalpy
To understand recursion you must understand recursion
Critical numbers
Maximum likelihood vs maximum parsimony
Maximum likelihood vs maximum parsimony
The increase of entropy principle
Illustrations text features
The principle of maximum social advantage is explained by
Hindi transliteration
Principle of maximum social advantage
Hát kết hợp bộ gõ cơ thể
Ng-html
Bổ thể
Tỉ lệ cơ thể trẻ em
Chó sói
Tư thế worms-breton
Alleluia hat len nguoi oi
Các môn thể thao bắt đầu bằng tiếng nhảy
Thế nào là hệ số cao nhất
Các châu lục và đại dương trên thế giới
Công thức tiính động năng