shotgun sequencing 1 st 2 nd 3 rd
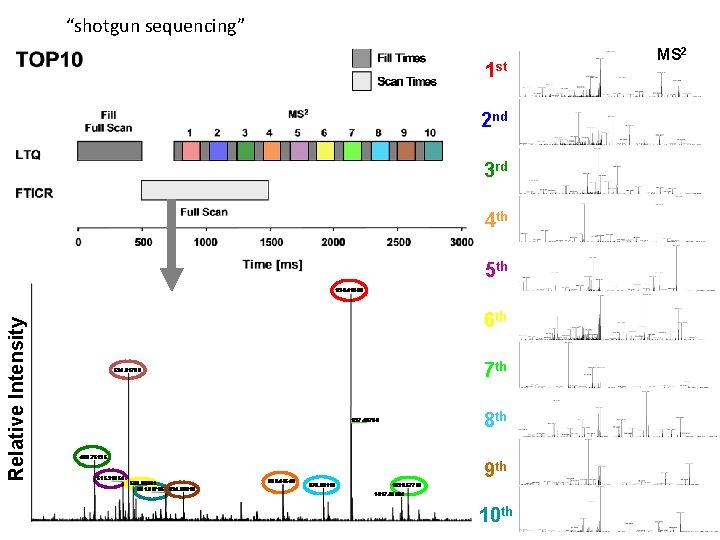
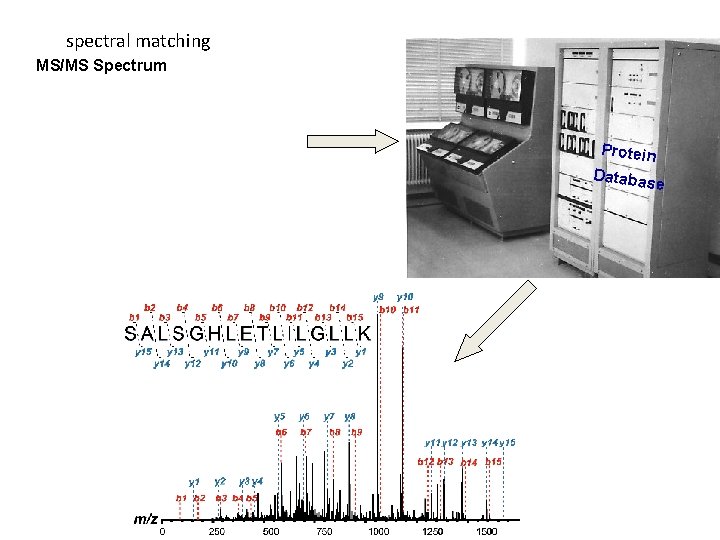
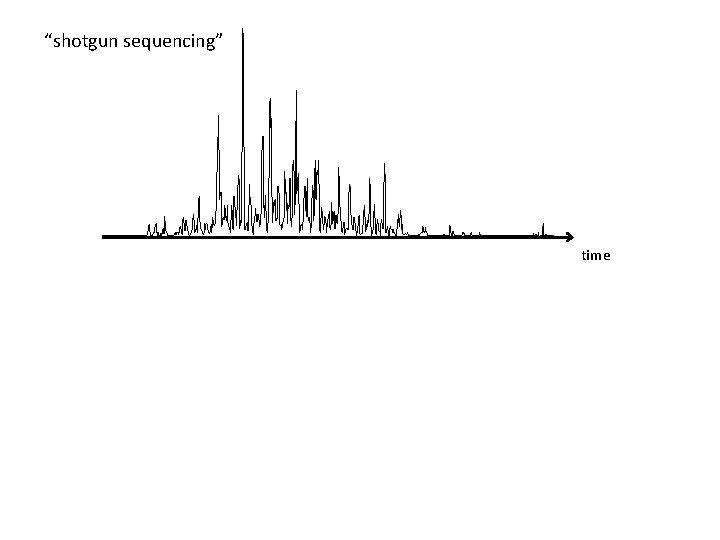
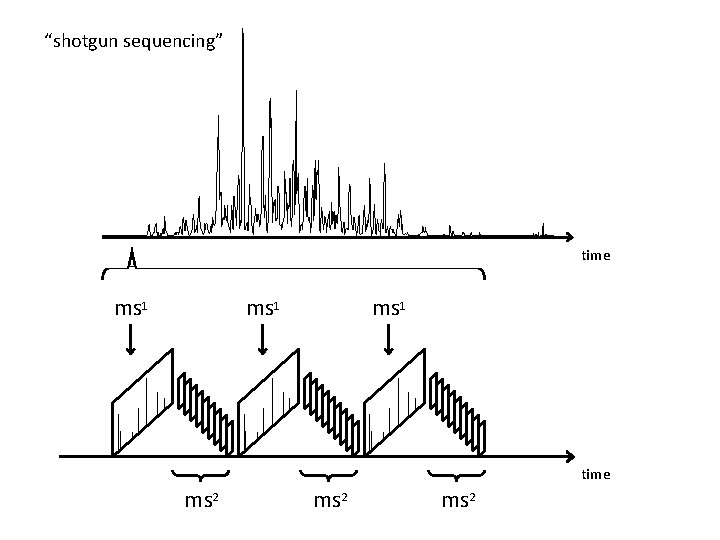
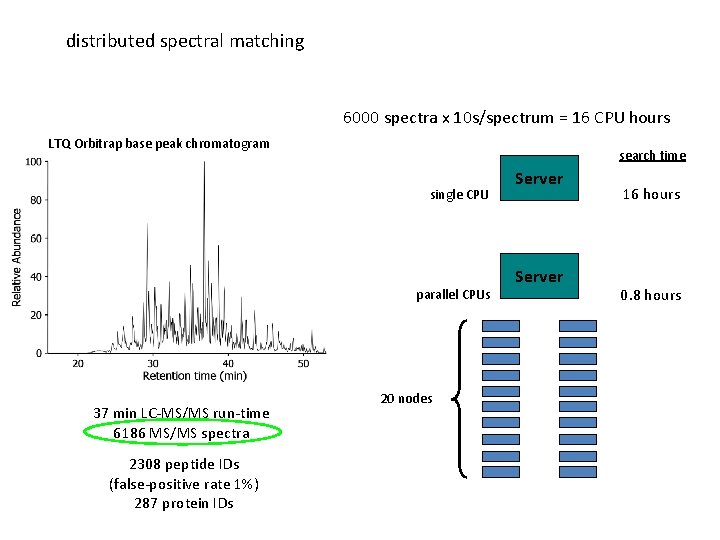
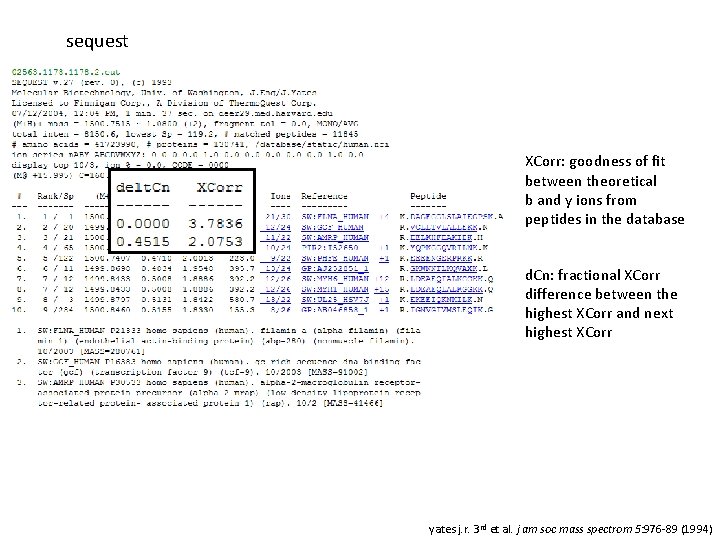
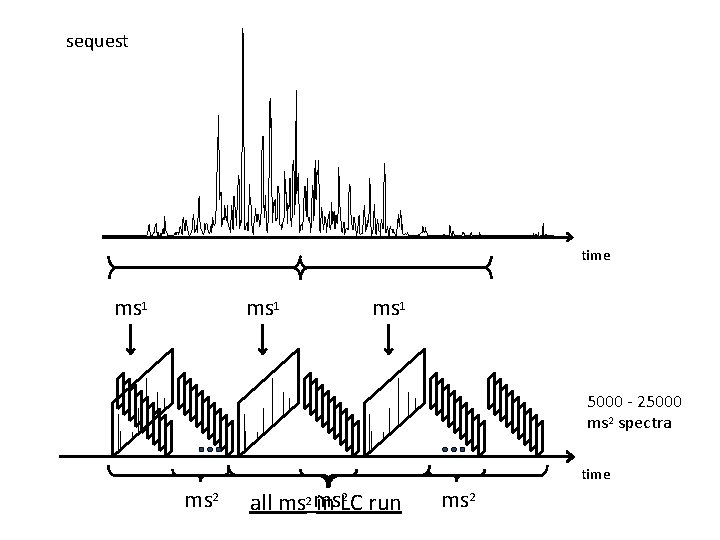
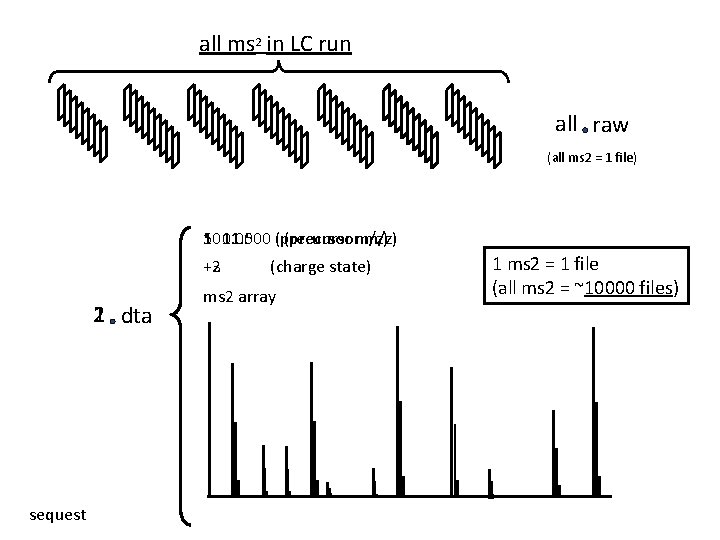
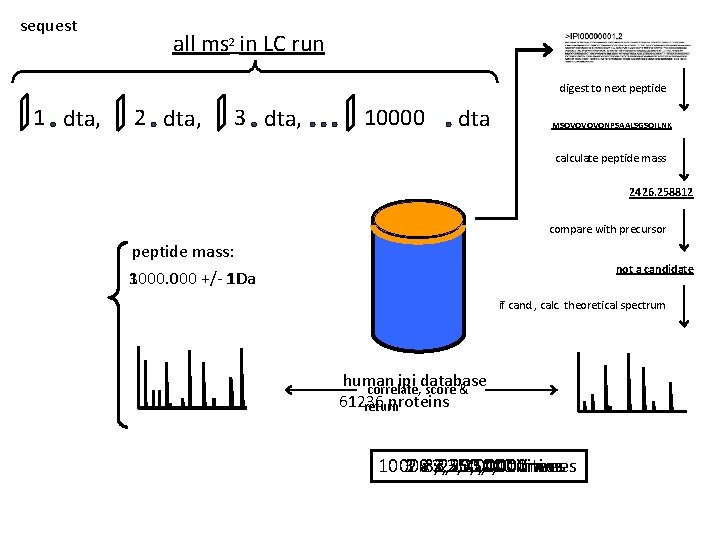
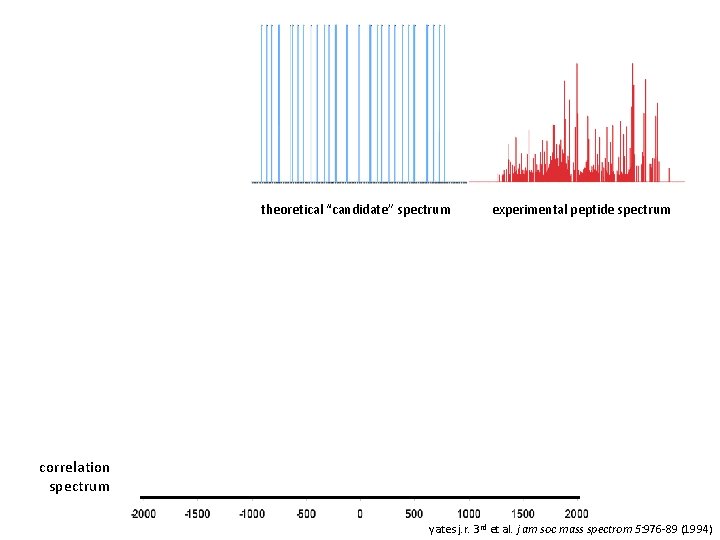
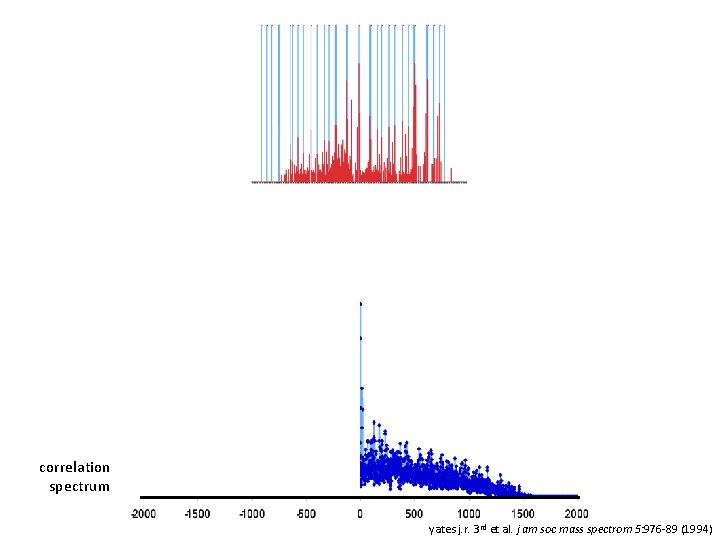
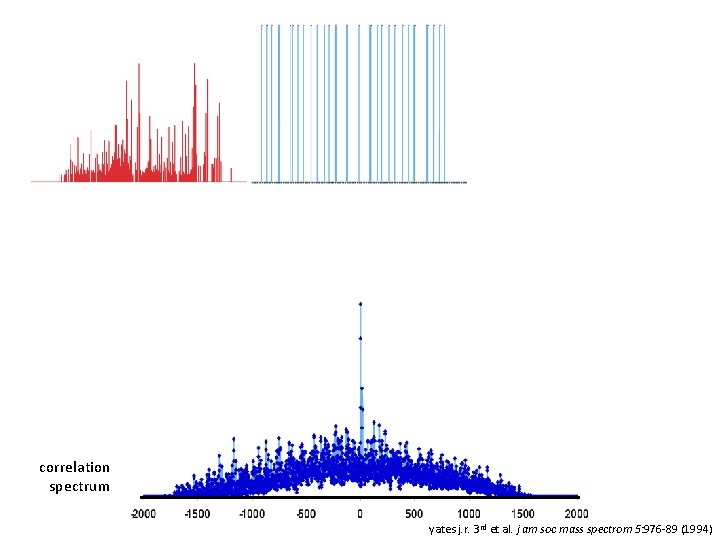
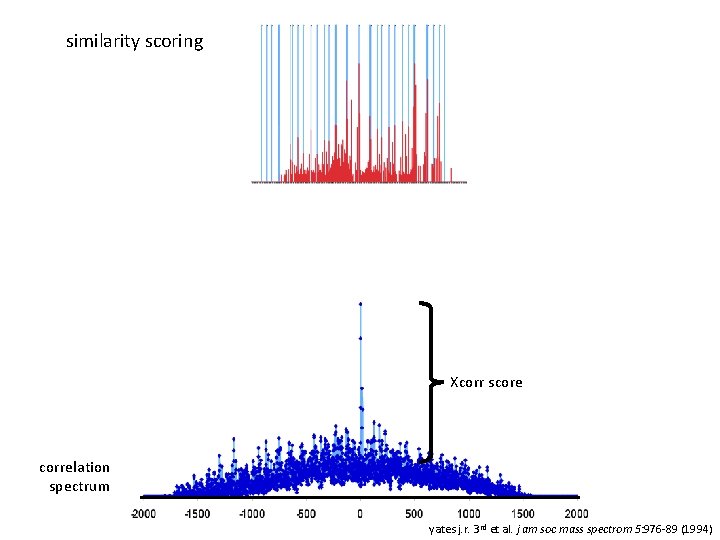
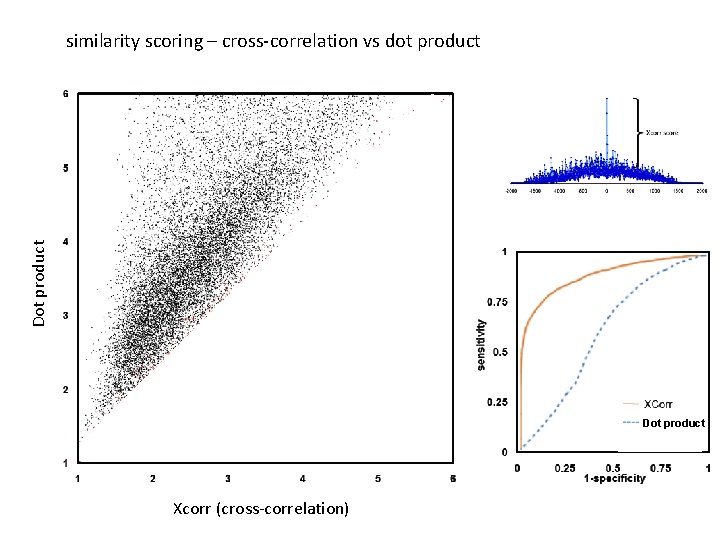
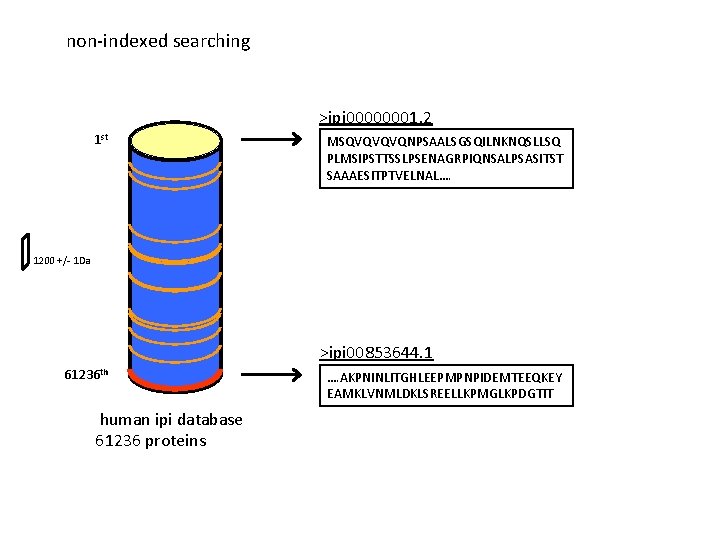
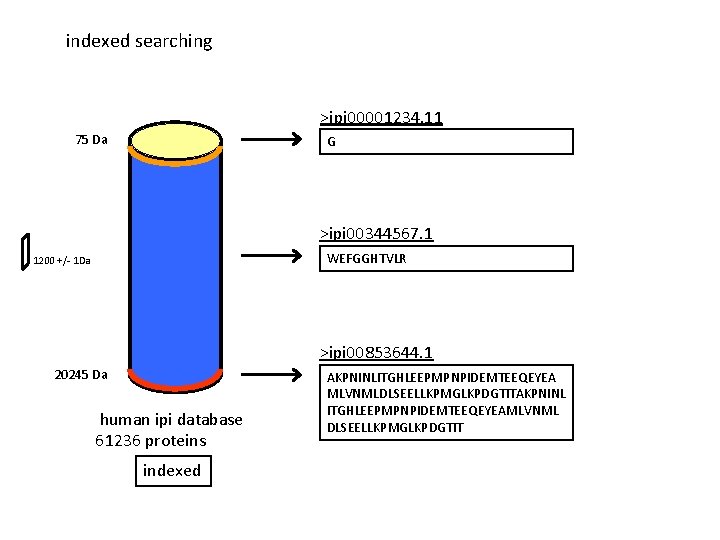
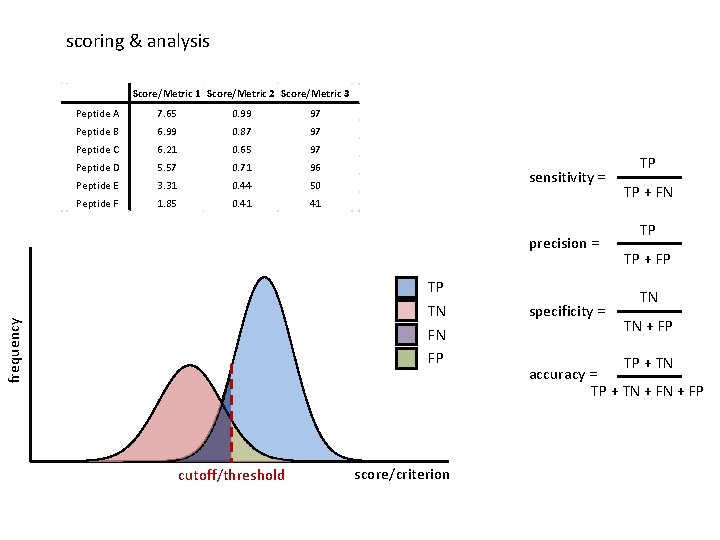
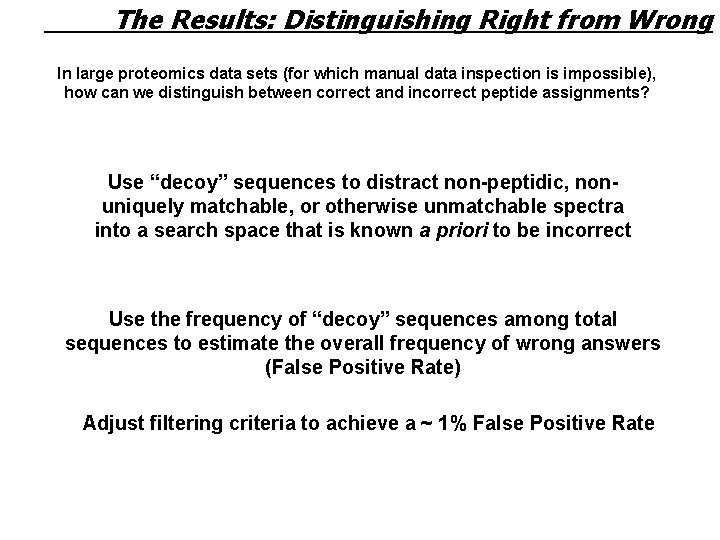
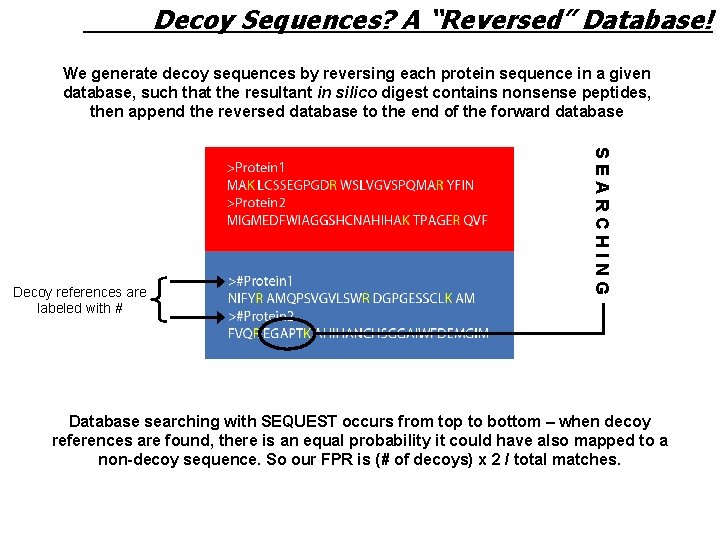
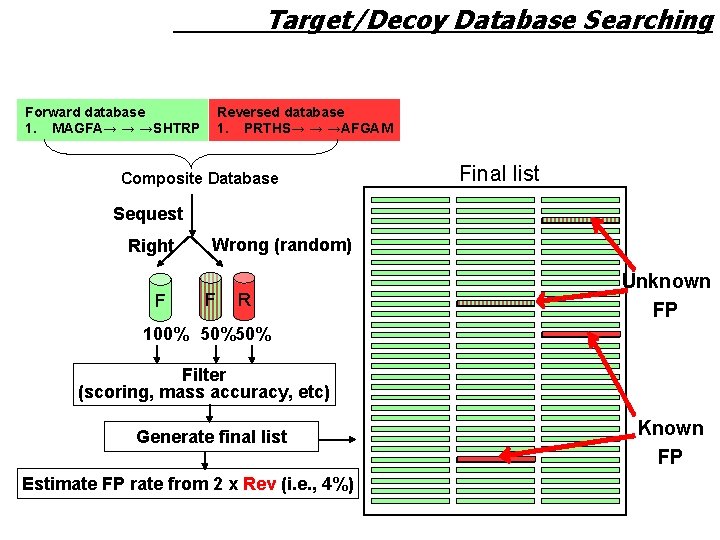
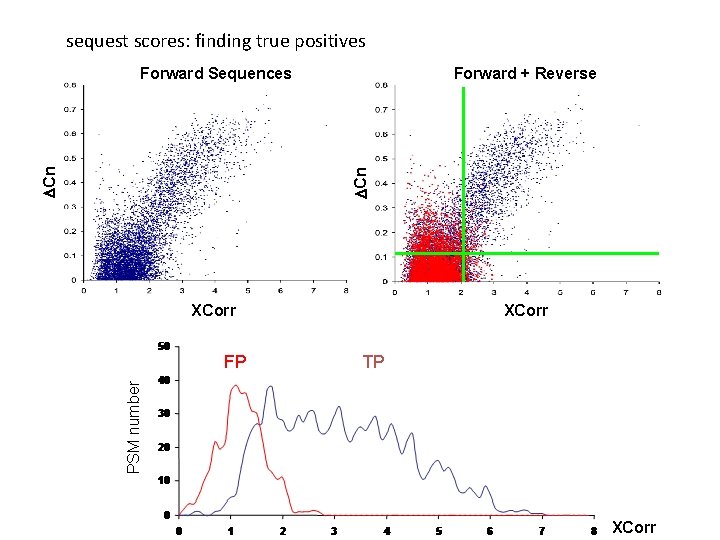
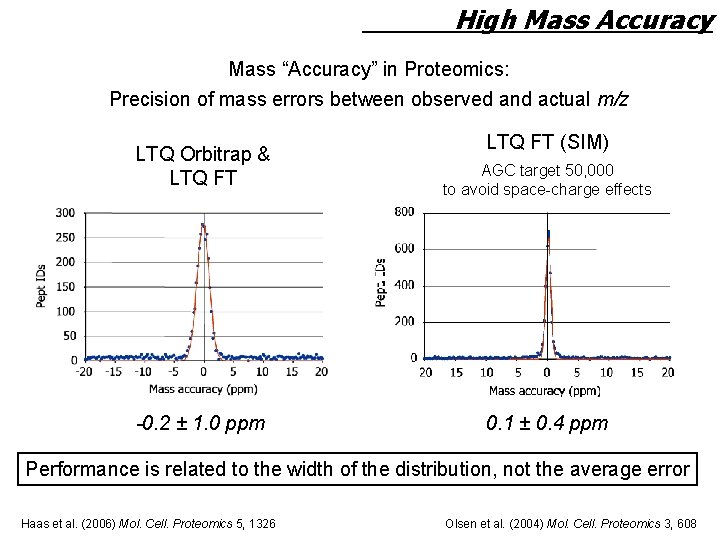
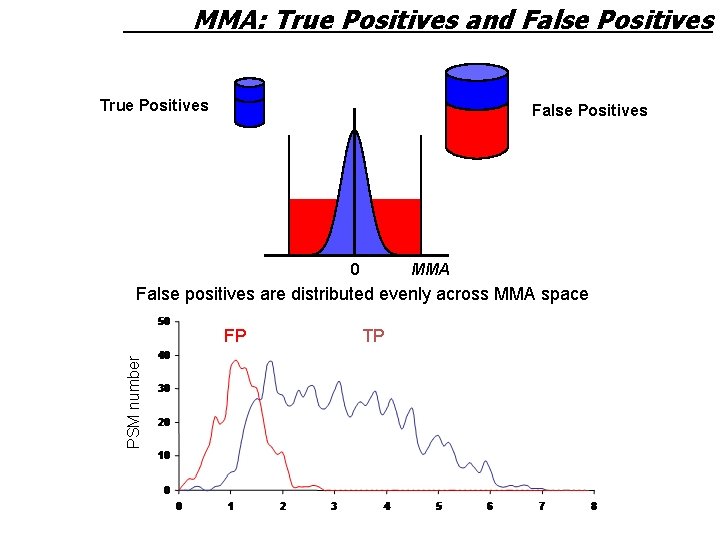
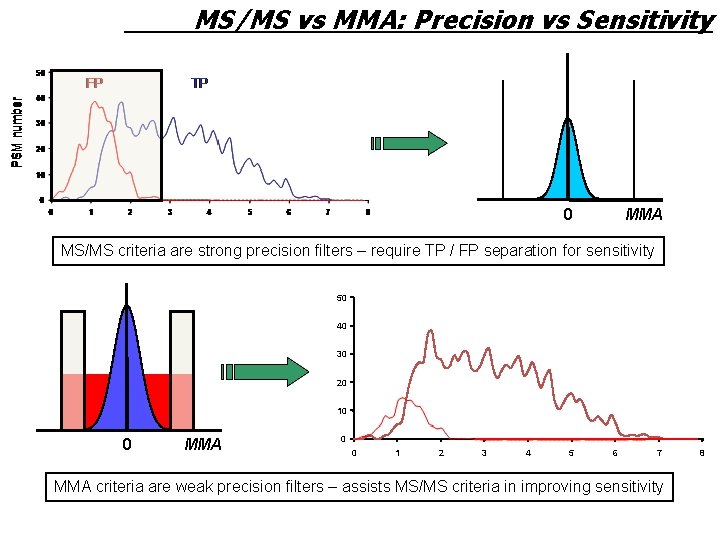
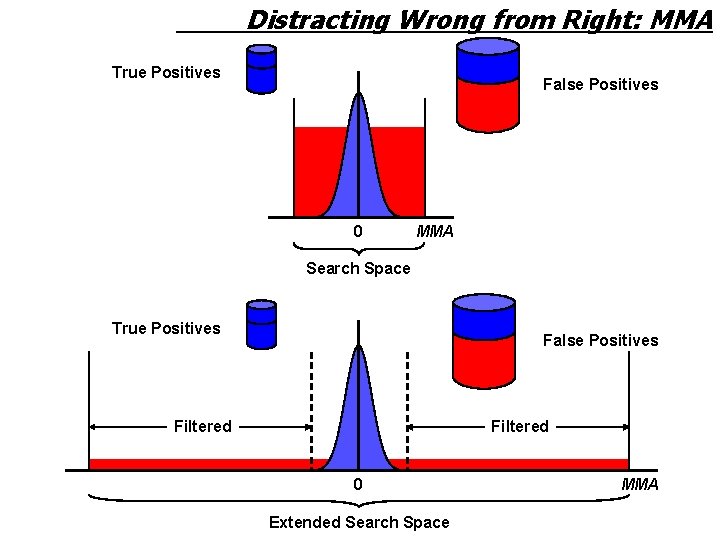
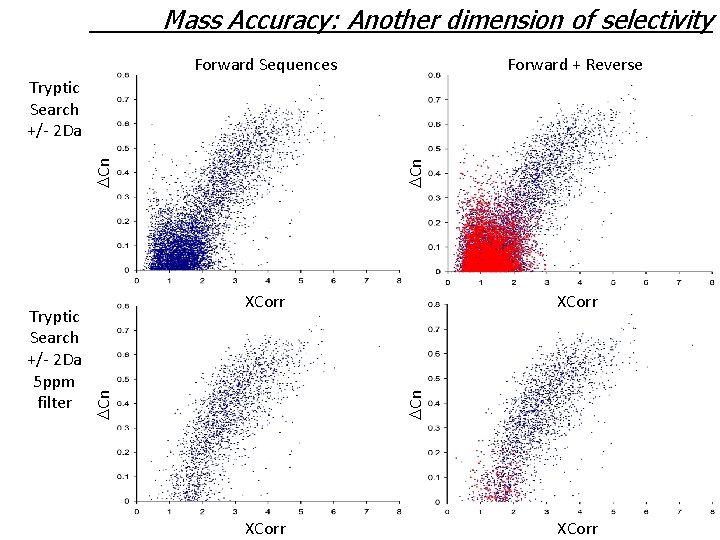
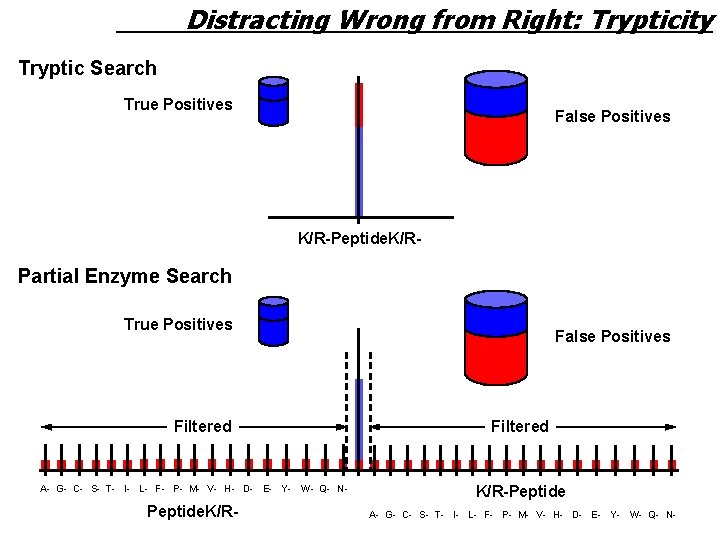
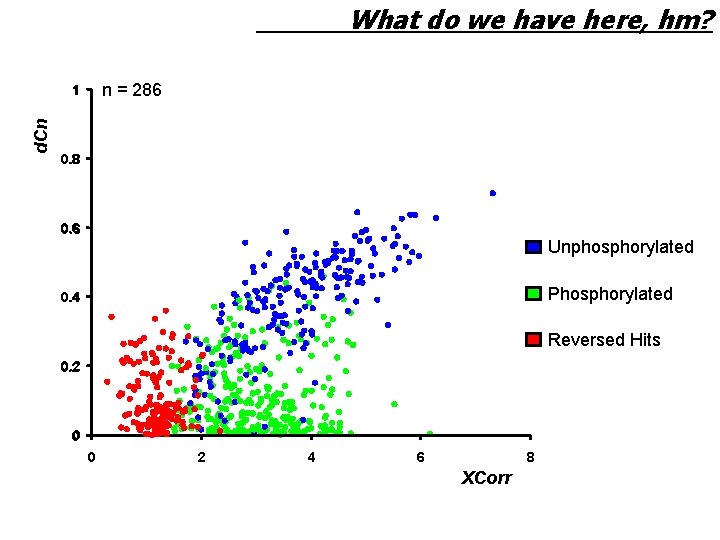
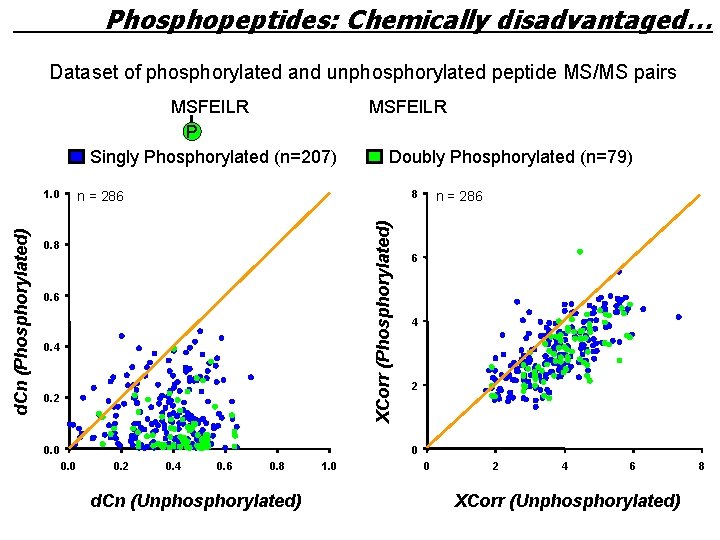
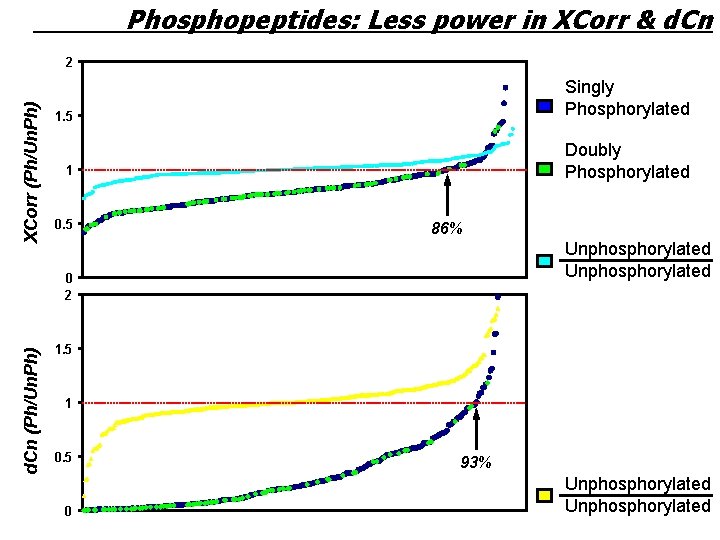
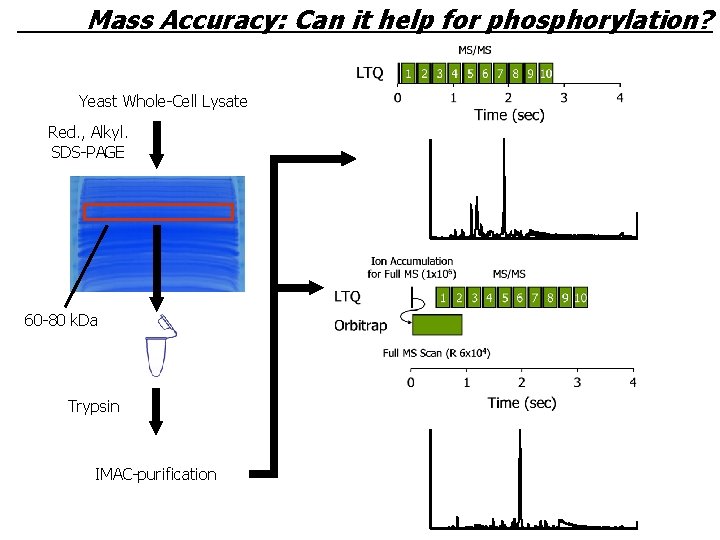
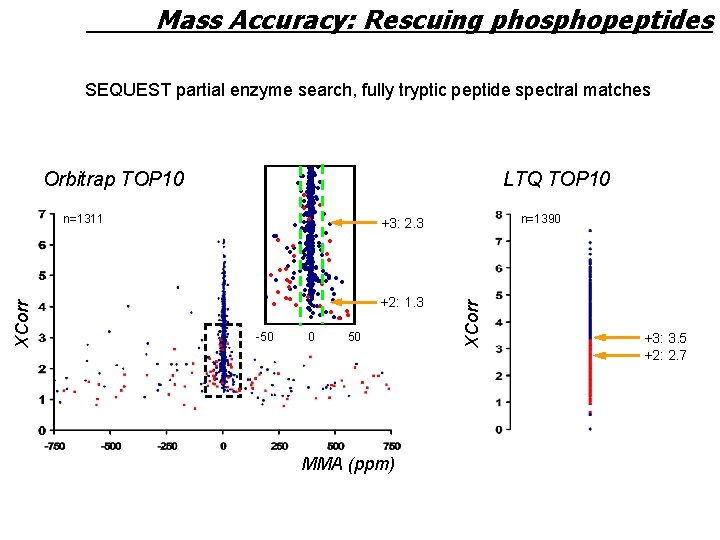
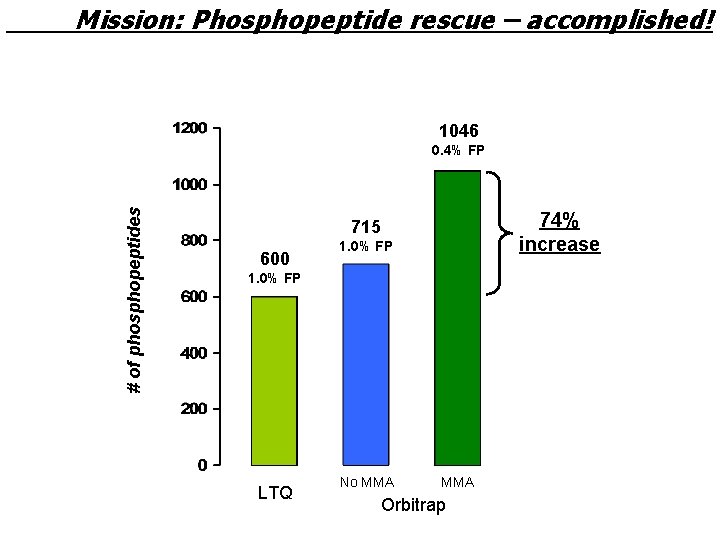
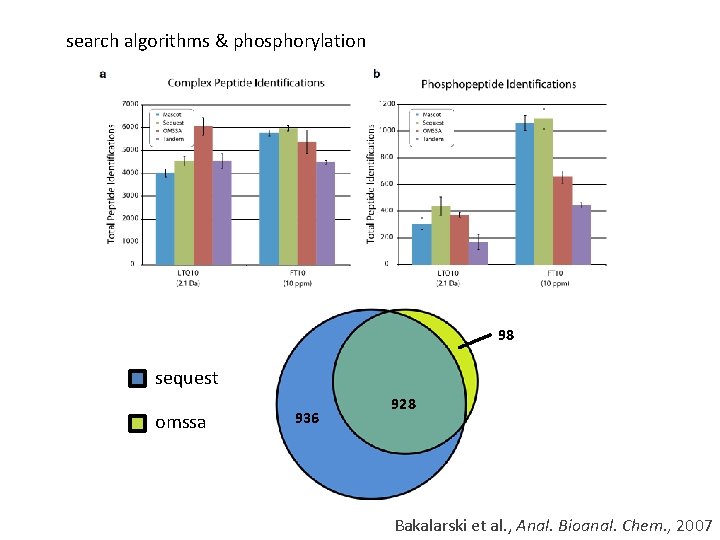
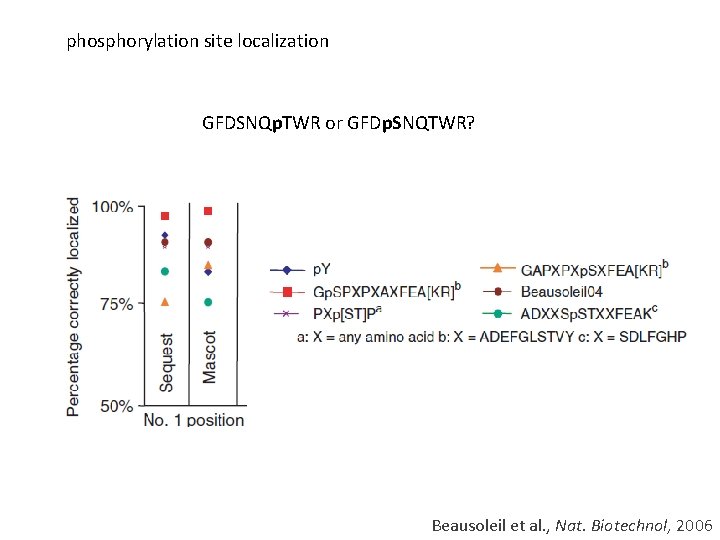
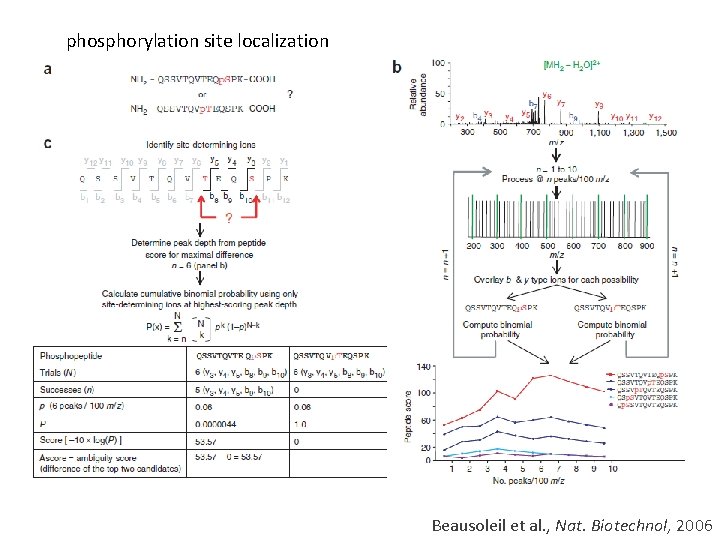
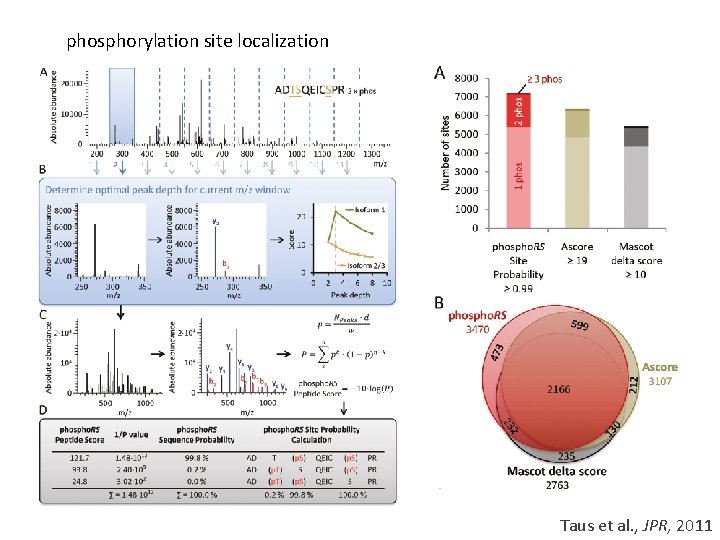
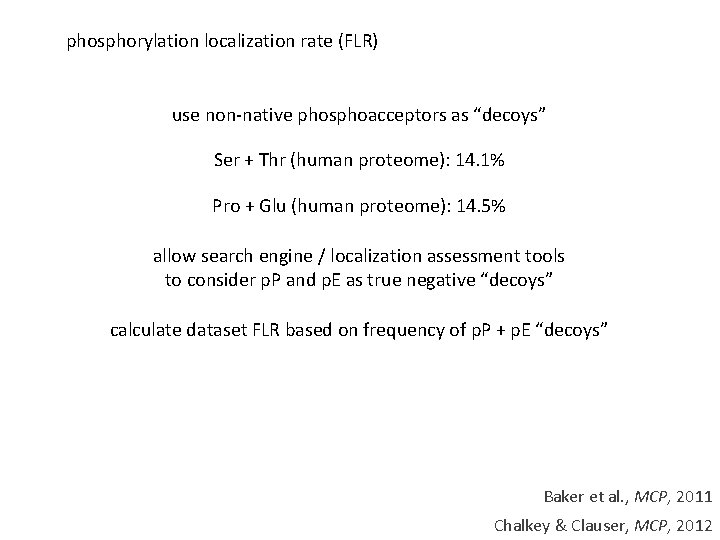
- Slides: 38
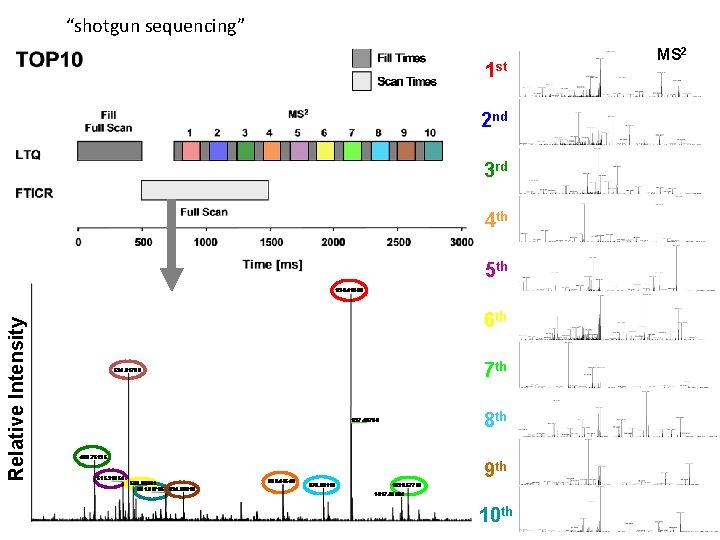
“shotgun sequencing” 1 st 2 nd 3 rd 4 th Relative Intensity 5 th 6 th 7 th 8 th 9 th 10 th MS 2
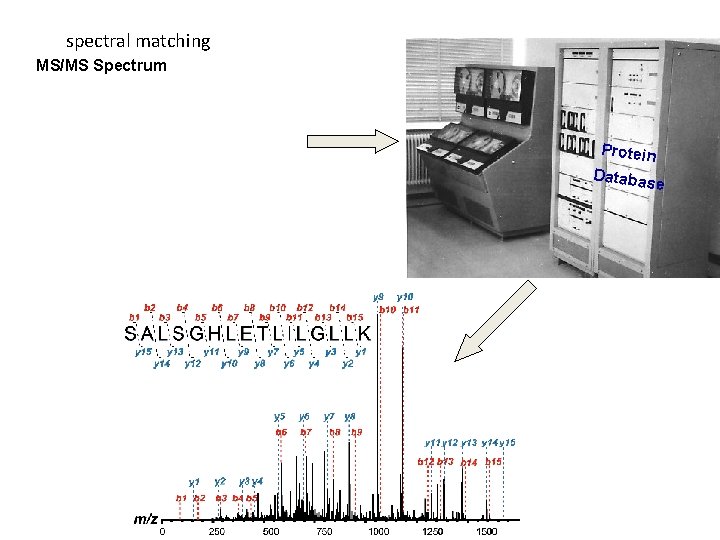
spectral matching MS/MS Spectrum Protein Databas e
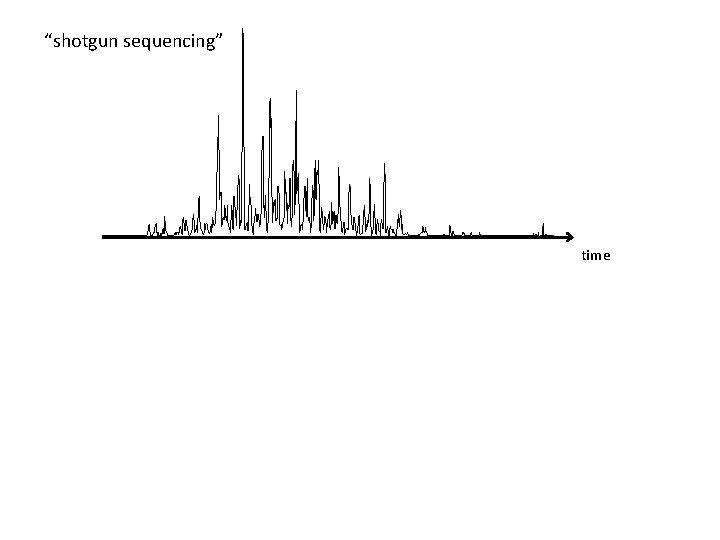
“shotgun sequencing” time
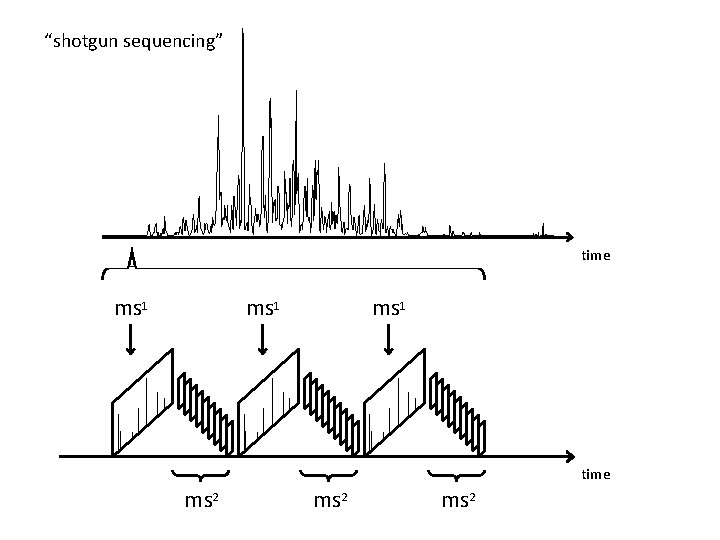
“shotgun sequencing” time ms 1 time ms 2
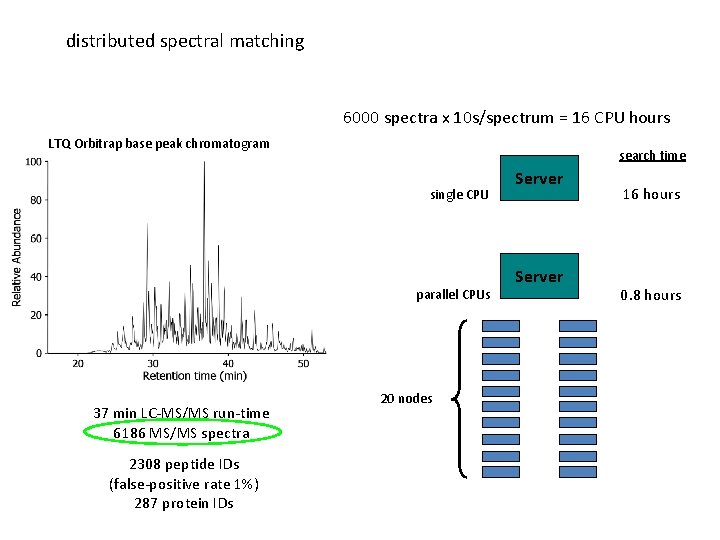
distributed spectral matching 6000 spectra x 10 s/spectrum = 16 CPU hours LTQ Orbitrap base peak chromatogram search time single CPU parallel CPUs 37 min LC-MS/MS run-time 6186 MS/MS spectra 2308 peptide IDs (false-positive rate 1%) 287 protein IDs 20 nodes Server 16 hours 0. 8 hours
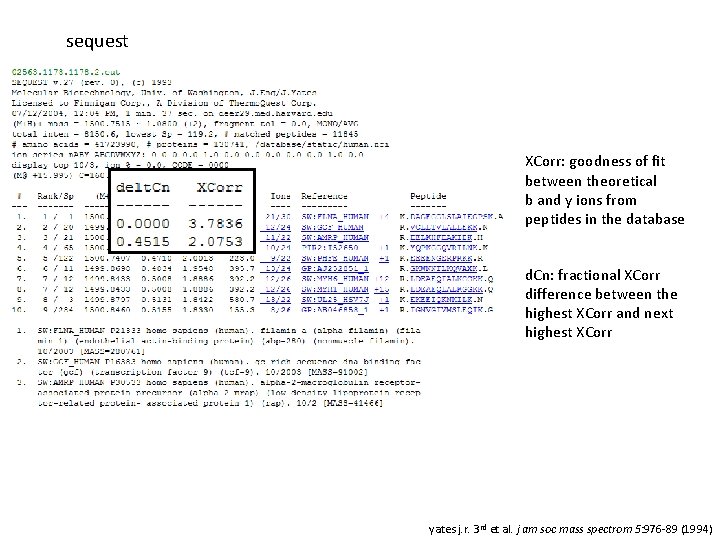
sequest XCorr: goodness of fit between theoretical b and y ions from peptides in the database d. Cn: fractional XCorr difference between the highest XCorr and next highest XCorr yates j. r. 3 rd et al. j am soc mass spectrom 5: 976 -89 (1994)
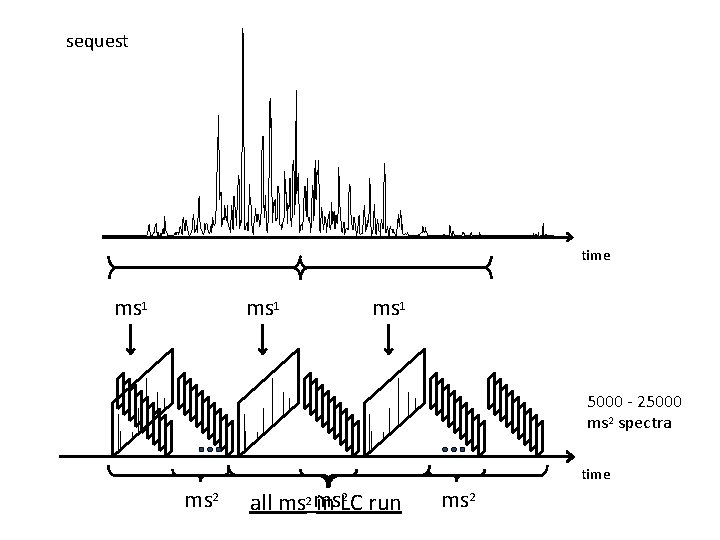
sequest time ms 1 5000 - 25000 ms 2 spectra time ms 2 2 all ms 2 ms in LC run ms 2
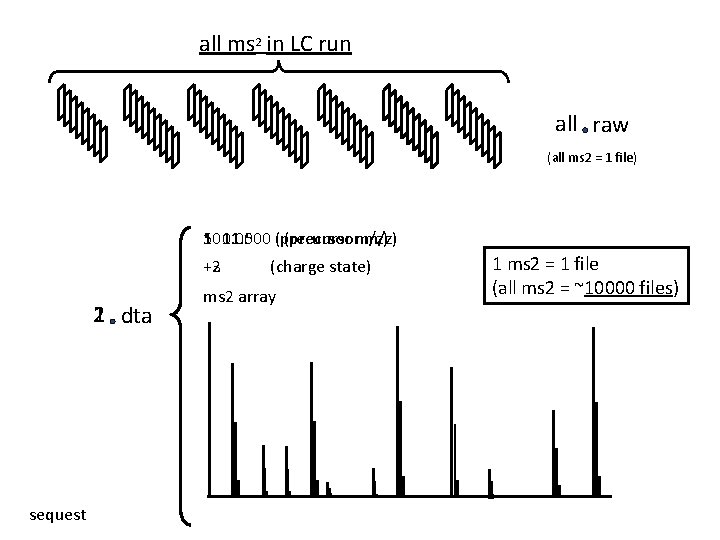
all ms 2 in LC run all raw (all ms 2 = 1 file) 501. 000 (precursor 1001. 500 (precursorm/z) +2 +3 1 dta 2 sequest (charge state) ms 2 array 1 ms 2 = 1 file (all ms 2 = ~10000 files)
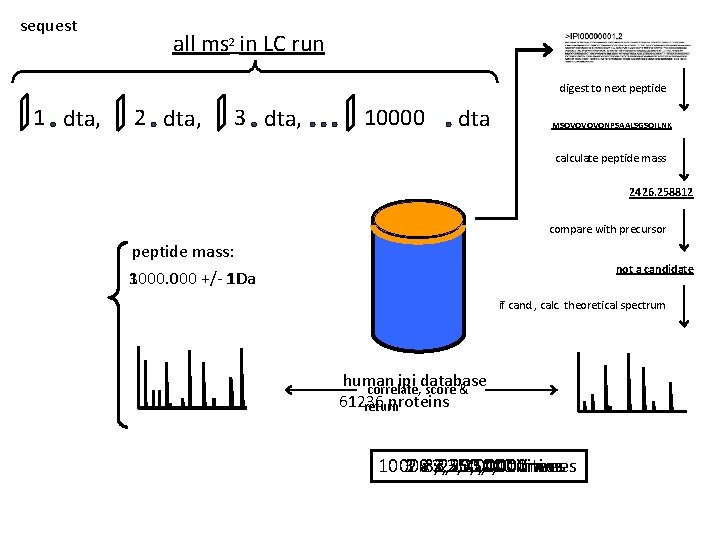
sequest all ms 2 in LC run digest to next peptide 1 dta, 2 dta, 3 dta, 10000 dta MSQVQVQVQNPSAALSGSQILNK calculate peptide mass 2426. 258812 compare with precursor peptide mass: not a candidate 1000. 000 3000. 000 +/- 1 Da if cand. , calc. theoretical spectrum human ipi database correlate, score & 61236 proteins return 10000 32 xx 3, 250, 000 times
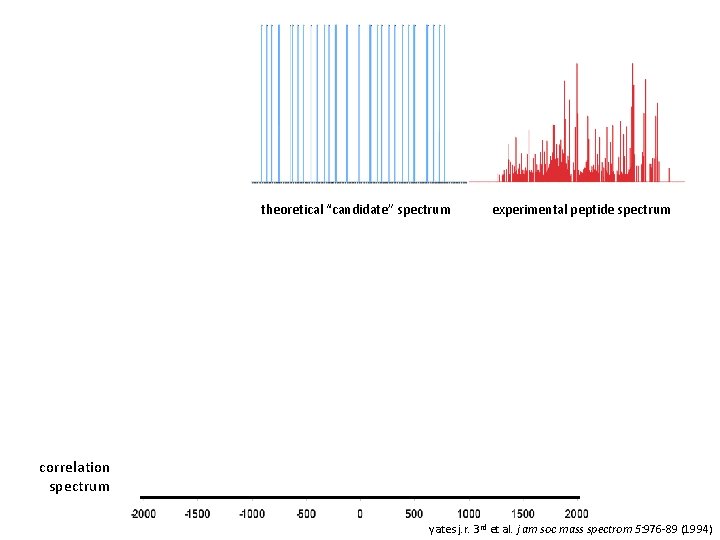
theoretical “candidate” spectrum experimental peptide spectrum correlation spectrum yates j. r. 3 rd et al. j am soc mass spectrom 5: 976 -89 (1994)
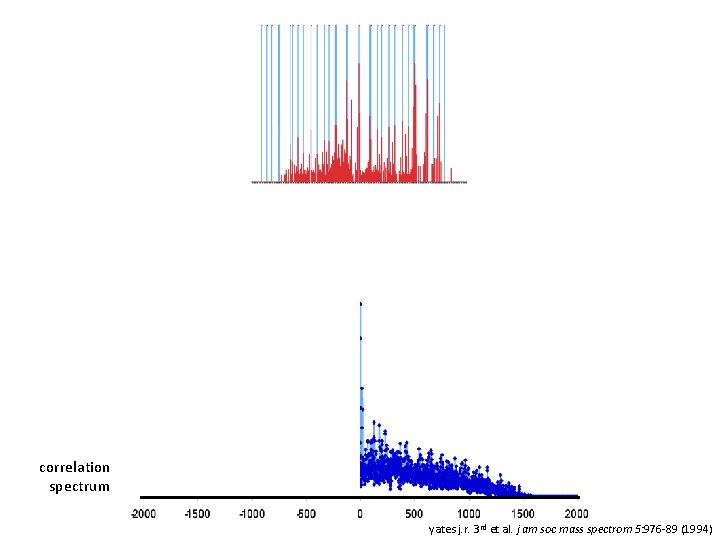
correlation spectrum yates j. r. 3 rd et al. j am soc mass spectrom 5: 976 -89 (1994)
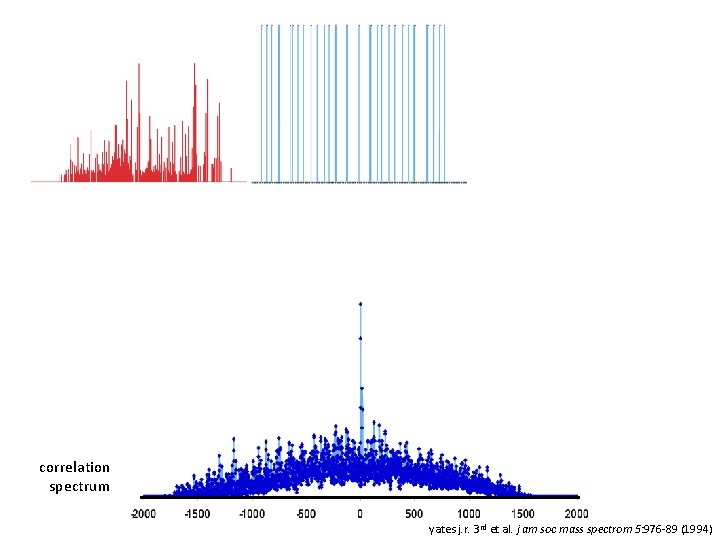
correlation spectrum yates j. r. 3 rd et al. j am soc mass spectrom 5: 976 -89 (1994)
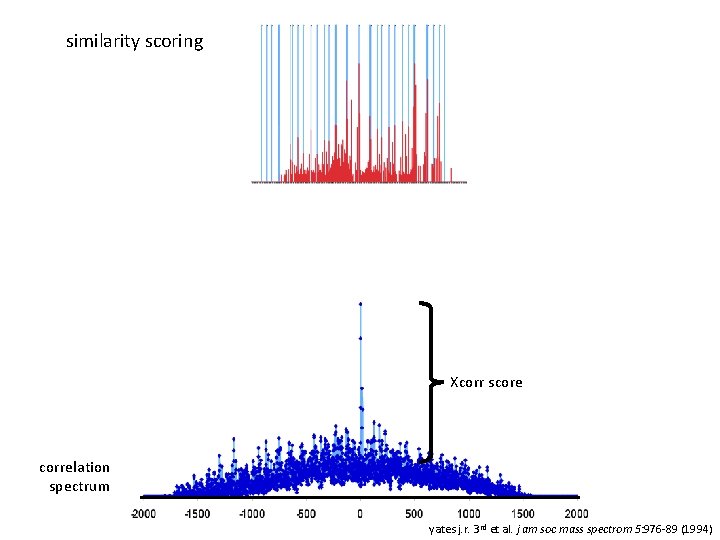
similarity scoring Xcorr score correlation spectrum yates j. r. 3 rd et al. j am soc mass spectrom 5: 976 -89 (1994)
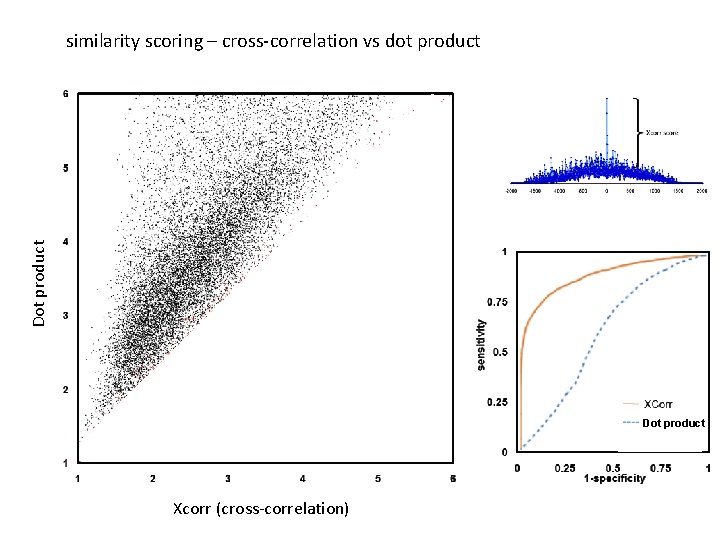
Dot product similarity scoring – cross-correlation vs dot product Dot product Xcorr (cross-correlation)
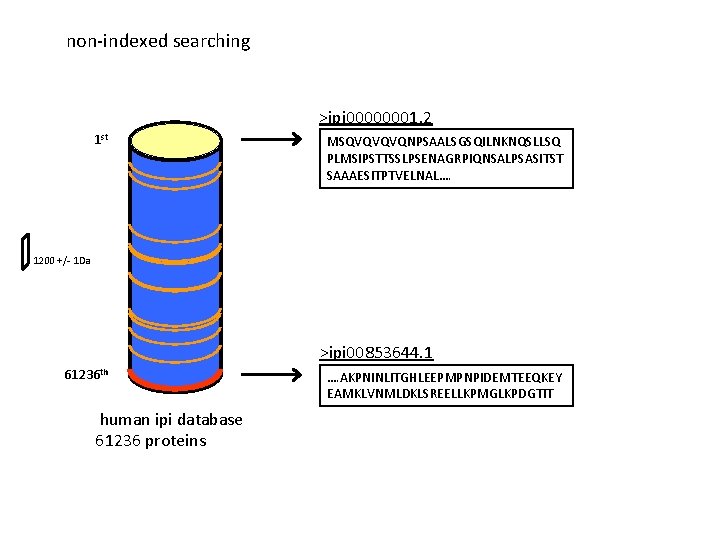
non-indexed searching >ipi 00000001. 2 1 st MSQVQVQVQNPSAALSGSQILNKNQSLLSQ PLMSIPSTTSSLPSENAGRPIQNSALPSASITST SAAAESITPTVELNAL…. 1200 +/- 1 Da >ipi 00853644. 1 61236 th human ipi database 61236 proteins …. AKPNINLITGHLEEPMPNPIDEMTEEQKEY EAMKLVNMLDKLSREELLKPMGLKPDGTIT
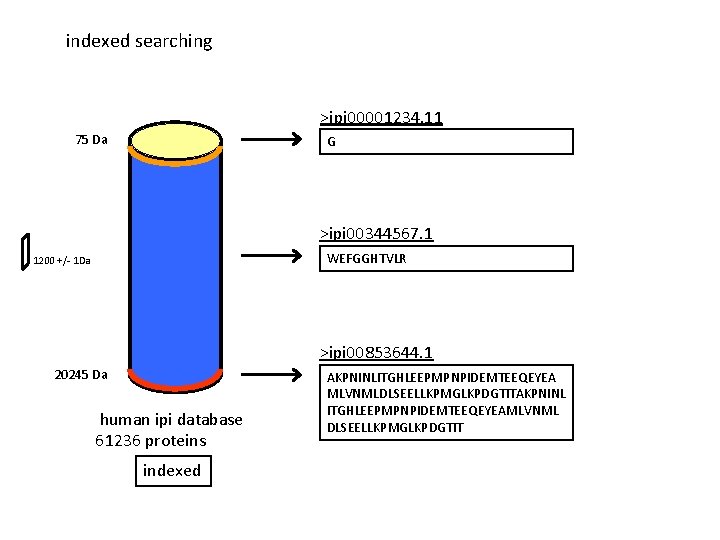
indexed searching >ipi 00001234. 11 75 Da G >ipi 00344567. 1 WEFGGHTVLR 1200 +/- 1 Da >ipi 00853644. 1 20245 Da human ipi database 61236 proteins indexed AKPNINLITGHLEEPMPNPIDEMTEEQEYEA MLVNMLDLSEELLKPMGLKPDGTITAKPNINL ITGHLEEPMPNPIDEMTEEQEYEAMLVNML DLSEELLKPMGLKPDGTIT
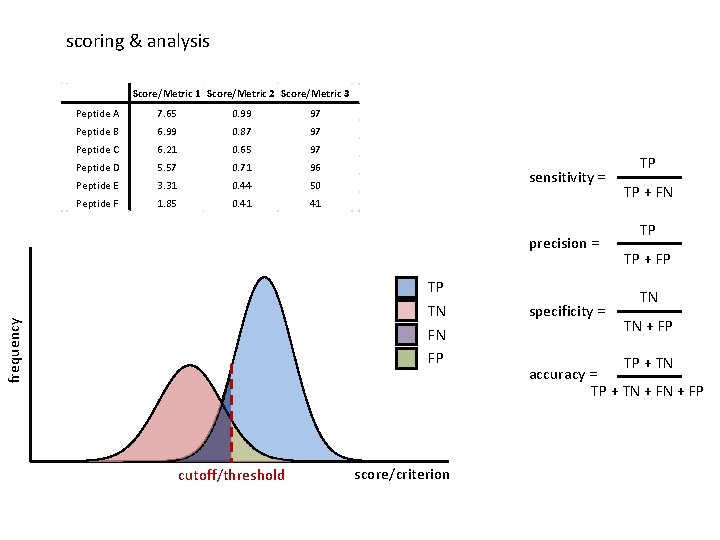
scoring & analysis Score/Metric 1 Score/Metric 2 Score/Metric 3 Peptide A 7. 65 0. 99 97 Peptide B 6. 99 0. 87 97 Peptide C 6. 21 0. 65 97 Peptide D 5. 57 0. 71 96 Peptide E 3. 31 0. 44 50 Peptide F 1. 85 0. 41 41 sensitivity = precision = frequency TP TN FN FP cutoff/threshold score/criterion specificity = TP TP + FN TP TP + FP TN TN + FP TP + TN accuracy = TP + TN + FP
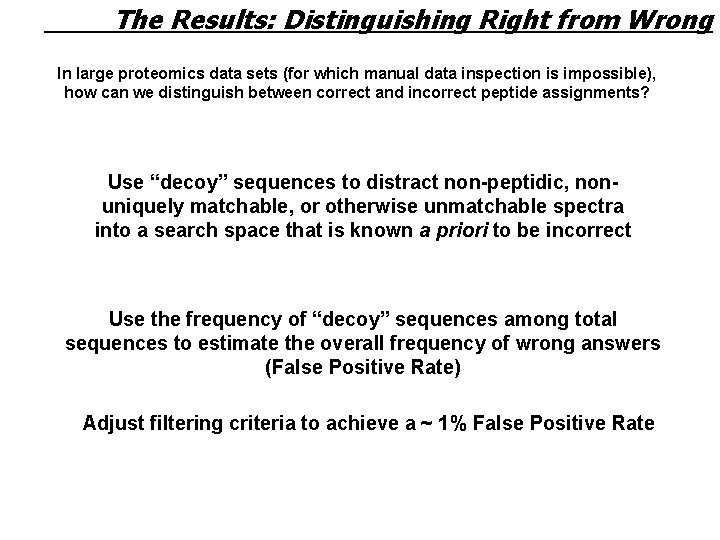
The Results: Distinguishing Right from Wrong In large proteomics data sets (for which manual data inspection is impossible), how can we distinguish between correct and incorrect peptide assignments? Use “decoy” sequences to distract non-peptidic, nonuniquely matchable, or otherwise unmatchable spectra into a search space that is known a priori to be incorrect Use the frequency of “decoy” sequences among total sequences to estimate the overall frequency of wrong answers (False Positive Rate) Adjust filtering criteria to achieve a ~ 1% False Positive Rate
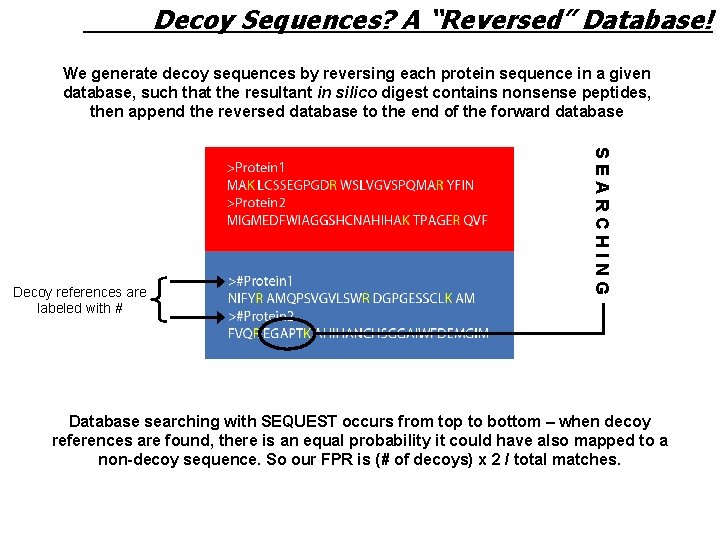
Decoy Sequences? A “Reversed” Database! We generate decoy sequences by reversing each protein sequence in a given database, such that the resultant in silico digest contains nonsense peptides, then append the reversed database to the end of the forward database SEARCHING Decoy references are labeled with # Database searching with SEQUEST occurs from top to bottom – when decoy references are found, there is an equal probability it could have also mapped to a non-decoy sequence. So our FPR is (# of decoys) x 2 / total matches.
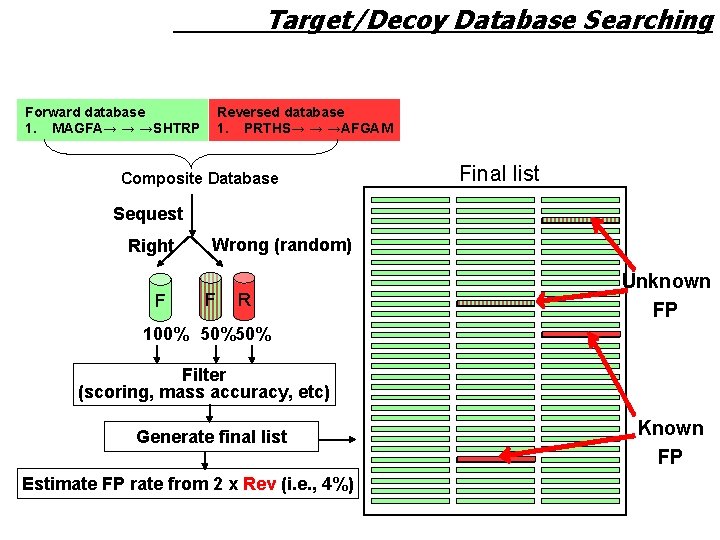
Target/Decoy Database Searching Forward database 1. MAGFA→ → →SHTRP Reversed database 1. PRTHS→ → →AFGAM Composite Database Final list Sequest Right F Wrong (random) F R Unknown FP 100% 50%50% Filter (scoring, mass accuracy, etc) Generate final list Estimate FP rate from 2 x Rev (i. e. , 4%) Known FP
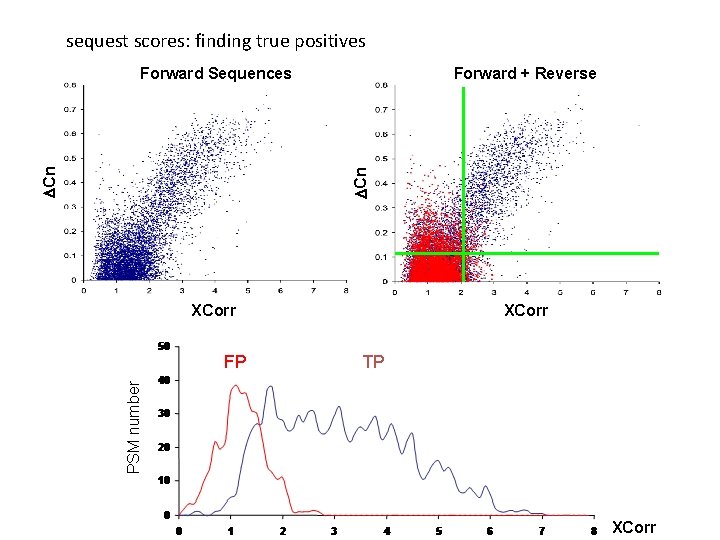
sequest scores: finding true positives Forward + Reverse DCn Forward Sequences XCorr TP PSM number FP XCorr
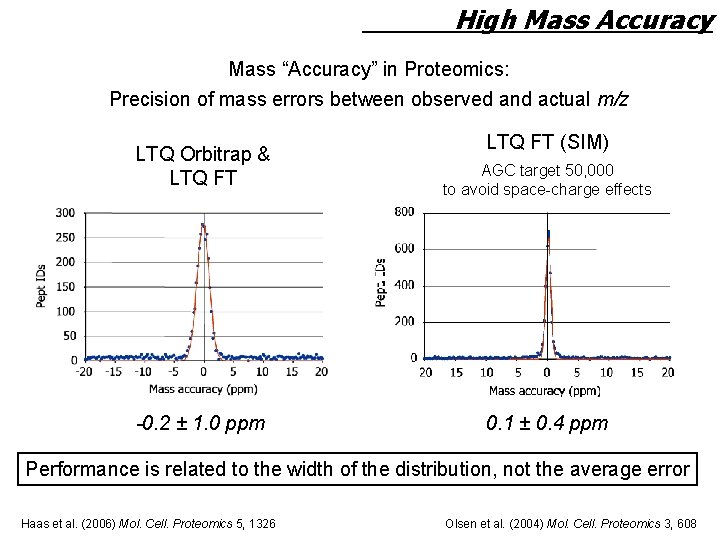
High Mass Accuracy Mass “Accuracy” in Proteomics: Precision of mass errors between observed and actual m/z LTQ Orbitrap & LTQ FT -0. 2 ± 1. 0 ppm LTQ FT (SIM) AGC target 50, 000 to avoid space-charge effects 0. 1 ± 0. 4 ppm Performance is related to the width of the distribution, not the average error Haas et al. (2006) Mol. Cell. Proteomics 5, 1326 Olsen et al. (2004) Mol. Cell. Proteomics 3, 608
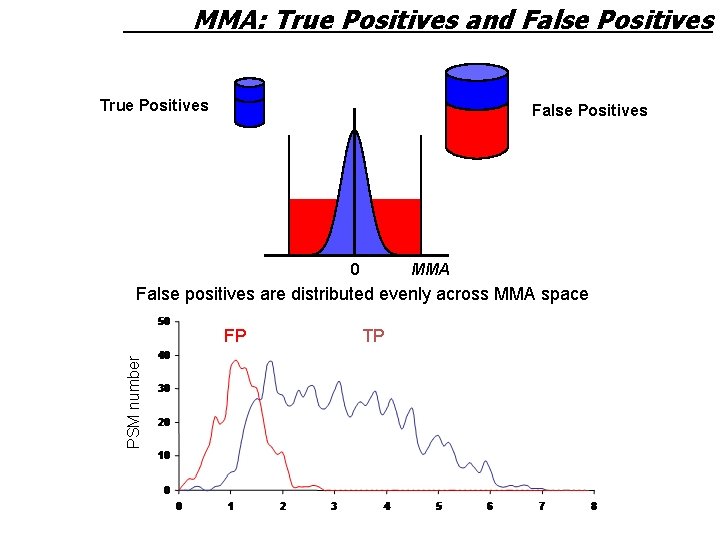
MMA: True Positives and False Positives True Positives False Positives 0 MMA False positives are distributed evenly across MMA space PSM number FP TP
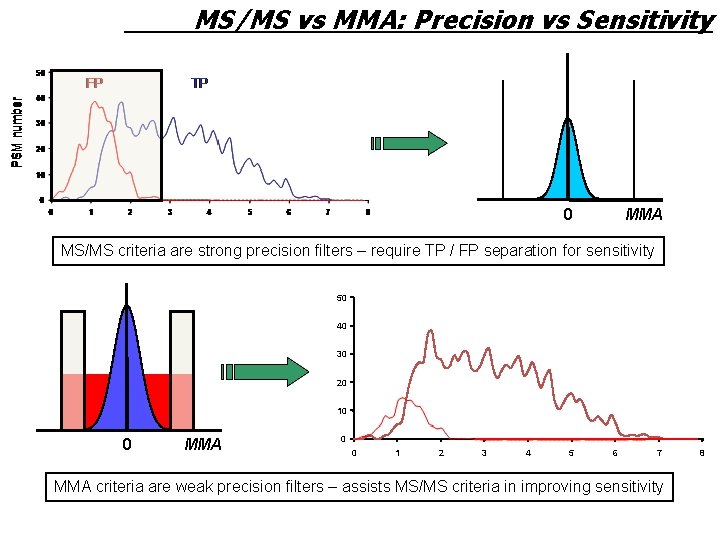
MS/MS vs MMA: Precision vs Sensitivity 0 MMA MS/MS criteria are strong precision filters – require TP / FP separation for sensitivity 50 40 30 20 10 0 MMA 0 0 1 2 3 4 5 6 7 MMA criteria are weak precision filters – assists MS/MS criteria in improving sensitivity 8
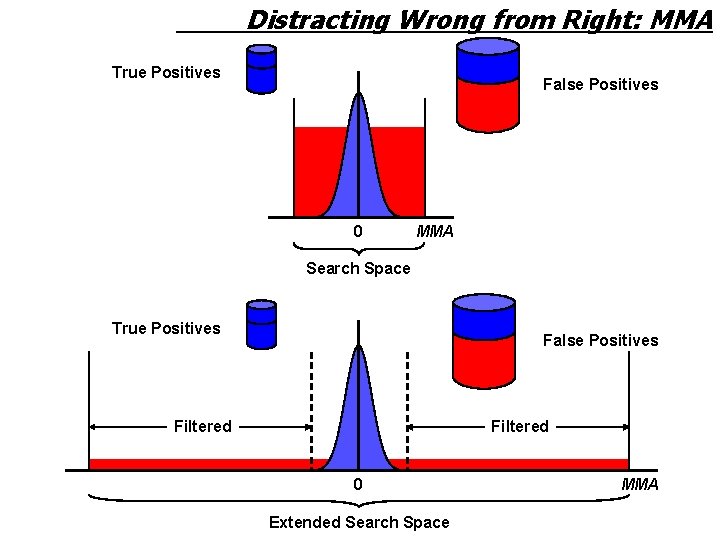
Distracting Wrong from Right: MMA True Positives False Positives 0 MMA Search Space True Positives False Positives Filtered 0 Extended Search Space MMA
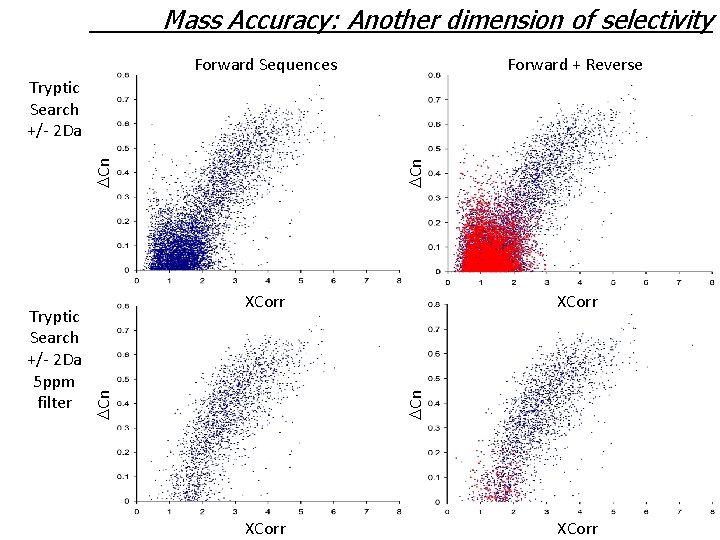
Mass Accuracy: Another dimension of selectivity Forward Sequences Forward + Reverse XCorr DCn Tryptic Search +/- 2 Da 5 ppm filter DCn Tryptic Search +/- 2 Da XCorr
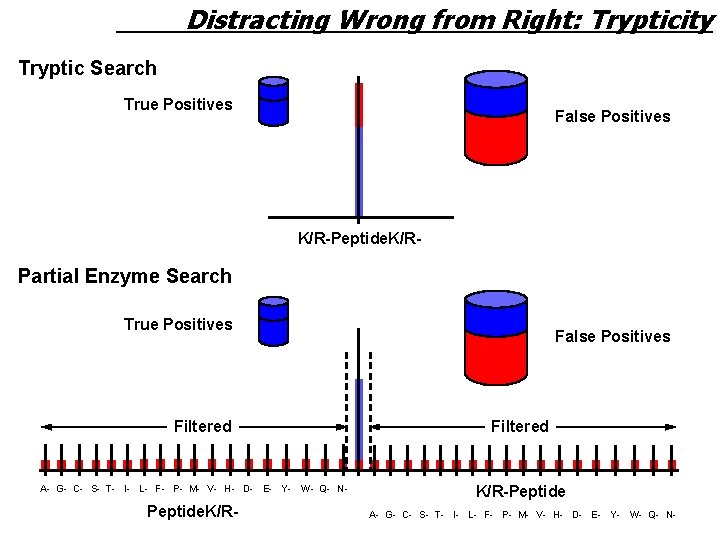
Distracting Wrong from Right: Trypticity Tryptic Search True Positives False Positives K/R-Peptide. K/R- Partial Enzyme Search True Positives Filtered False Positives Filtered A- G- C- S- T- I- L- F- P- M- V- H- D- E- Y- W- Q- N- K/R-Peptide. K/R- A- G- C- S- T- I- L- F- P- M- V- H- D- E- Y- W- Q- N-
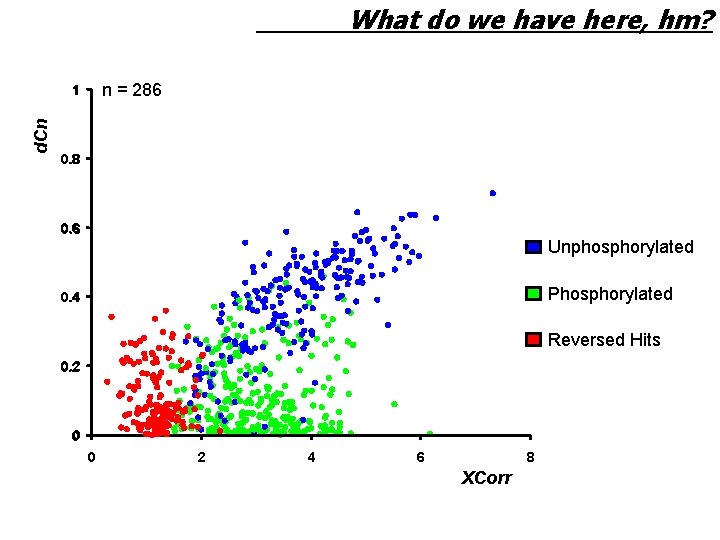
What do we have here, hm? n = 286 d. Cn 1 0. 8 0. 6 Unphosphorylated Phosphorylated 0. 4 Reversed Hits 0. 2 0 0 2 4 6 8 XCorr
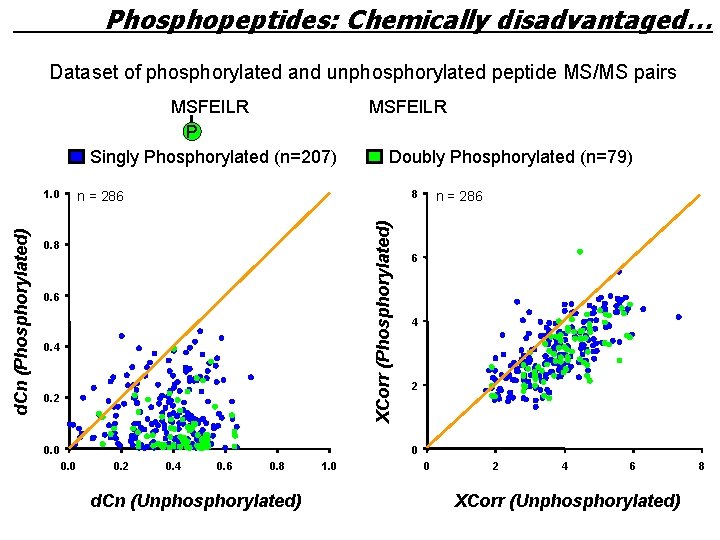
Phosphopeptides: Chemically disadvantaged… Dataset of phosphorylated and unphosphorylated peptide MS/MS pairs MSFEILR P Singly Phosphorylated (n=207) Doubly Phosphorylated (n=79) 8 n = 286 XCorr (Phosphorylated) d. Cn (Phosphorylated) 1. 0 MSFEILR 0. 8 0. 6 0. 4 0. 2 0. 0 n = 286 6 4 2 0 0. 2 0. 4 0. 6 0. 8 d. Cn (Unphosphorylated) 1. 0 0 2 4 6 XCorr (Unphosphorylated) 8
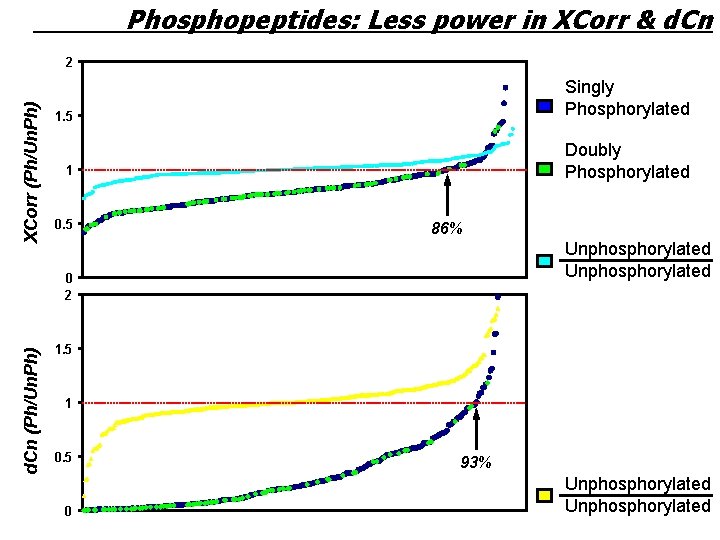
Phosphopeptides: Less power in XCorr & d. Cn XCorr (Ph/Un. Ph) 2 1. 5 Singly Phosphorylated 1 Doubly Phosphorylated 0. 5 86% Unphosphorylated d. Cn (Ph/Un. Ph) 0 2 1. 5 1 0. 5 0 93% Unphosphorylated
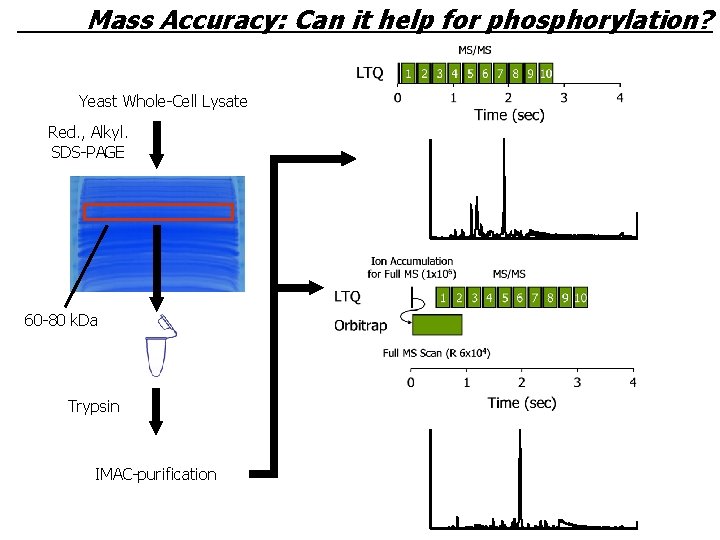
Mass Accuracy: Can it help for phosphorylation? Yeast Whole-Cell Lysate Red. , Alkyl. SDS-PAGE 60 -80 k. Da Trypsin IMAC-purification
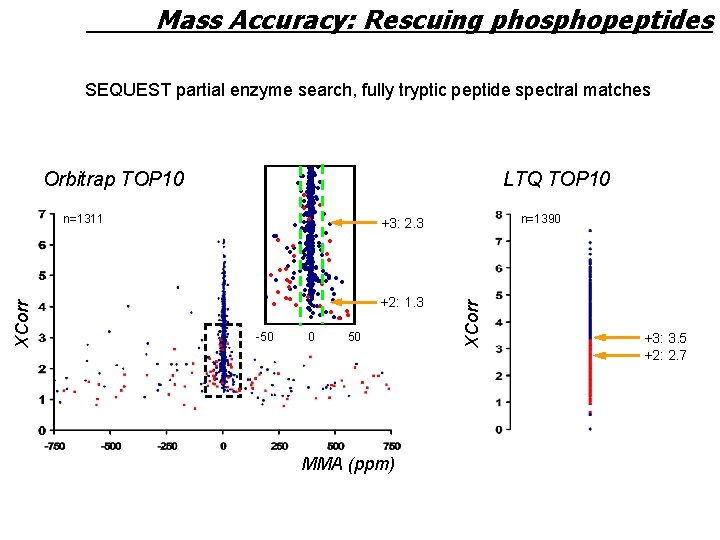
Mass Accuracy: Rescuing phosphopeptides SEQUEST partial enzyme search, fully tryptic peptide spectral matches Orbitrap TOP 10 LTQ TOP 10 n=1390 +3: 2. 3 +2: 1. 3 -50 0 50 MMA (ppm) XCorr n=1311 +3: 3. 5 +2: 2. 7
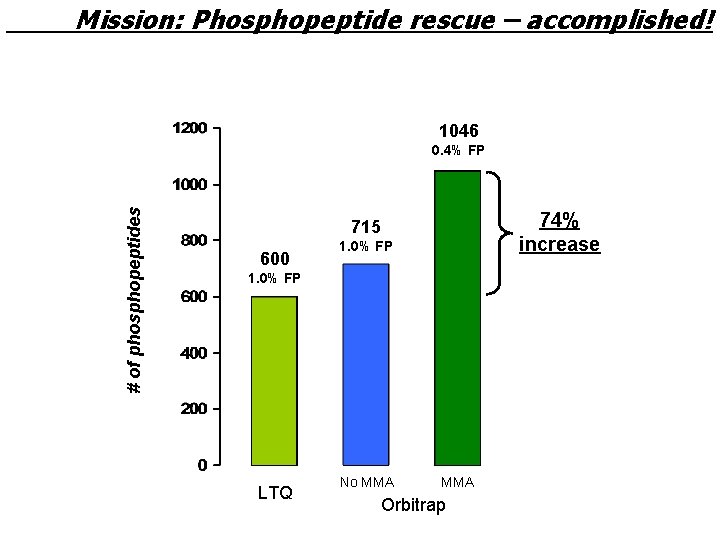
Mission: Phosphopeptide rescue – accomplished! 1046 # of phosphopeptides 0. 4% FP 74% increase 715 600 1. 0% FP LTQ No MMA Orbitrap
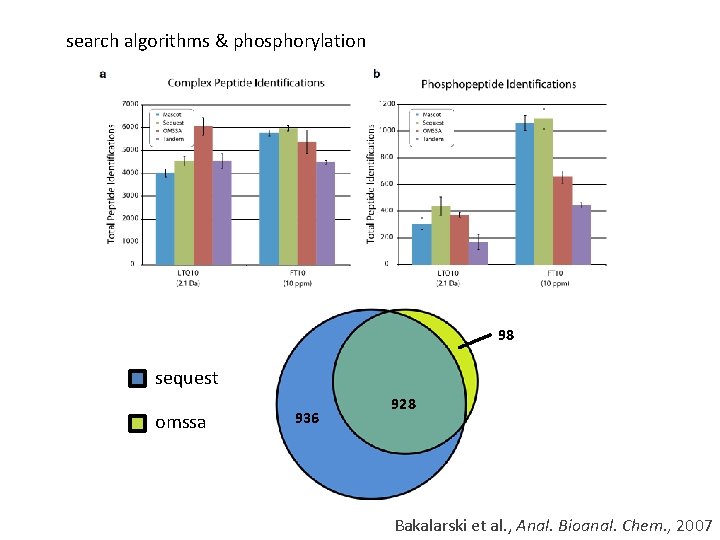
search algorithms & phosphorylation 98 sequest omssa 936 928 Bakalarski et al. , Anal. Bioanal. Chem. , 2007
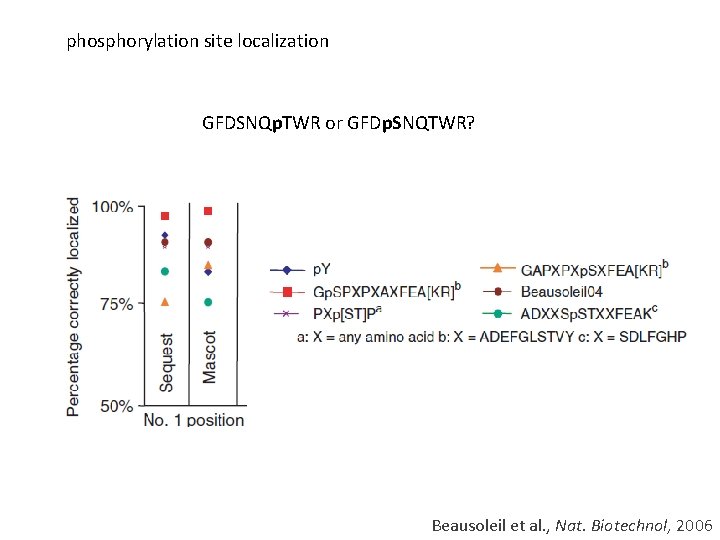
phosphorylation site localization GFDSNQp. TWR or GFDp. SNQTWR? Beausoleil et al. , Nat. Biotechnol, 2006
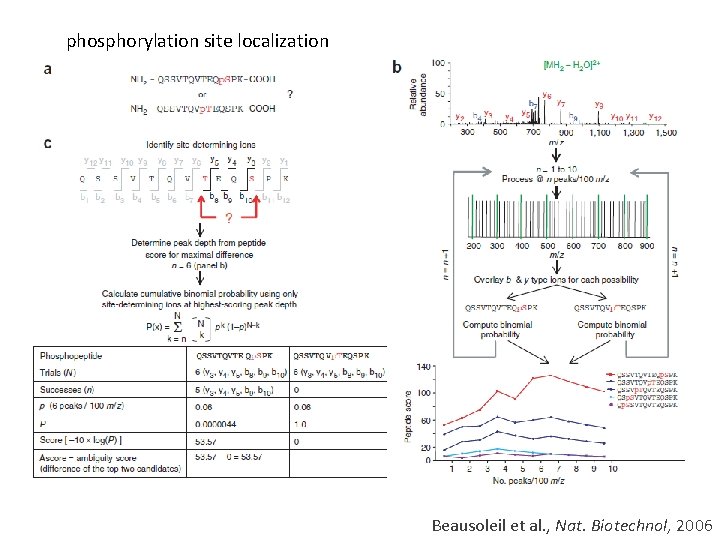
phosphorylation site localization Beausoleil et al. , Nat. Biotechnol, 2006
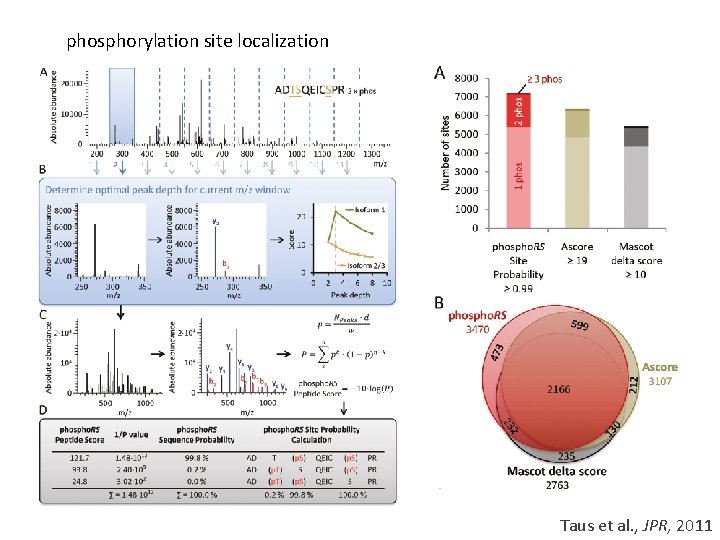
phosphorylation site localization Taus et al. , JPR, 2011
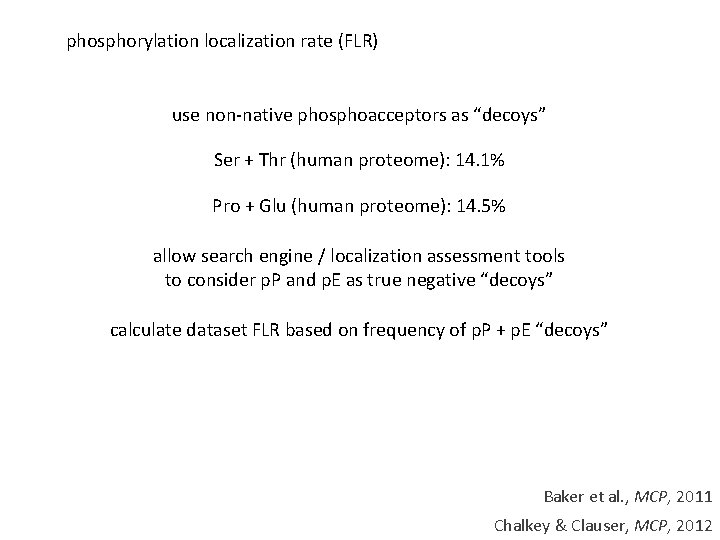
phosphorylation localization rate (FLR) use non-native phosphoacceptors as “decoys” Ser + Thr (human proteome): 14. 1% Pro + Glu (human proteome): 14. 5% allow search engine / localization assessment tools to consider p. P and p. E as true negative “decoys” calculate dataset FLR based on frequency of p. P + p. E “decoys” Baker et al. , MCP, 2011 Chalkey & Clauser, MCP, 2012
What gangs are in oklahoma
Pyrosequencing animation
Hierarchical shotgun sequencing vs whole genome
Hierarchical shotgun sequencing vs whole genome
How to pattern a shotgun for turkey
The power i offense
Railgun egun
870 p
Barrel markings
Blowback phenomenon wound
Sequencing suki
5.9 commonly fingerspelled words
Dna sequencing applications
Sequence selection iteration
Contigs
Clue words for sequencing
Cyclopeptide sequencing problem
Basil khuder
Are you my mother sequencing cards
Sequencing analysis viewer
Dna
Ngs sequencing data analysis
Illumina sequencing video
The very lonely firefly sequencing
Days of the week sequencing
Exome sequencing project
Helioscope sequencing
Loai tawalbeh
Algorithm
Rooster's off to see the world sequencing
Sequencing batch reactor advantages and disadvantages
Microinstruction example
Sanger vs maxam gilbert sequencing
Cloning and sequencing explorer series
Symbolic microprogram
Shortest processing time example
History of human genome project
Sequencing human genome
The cask of amontillado quiz