DNA Sequencing Project DNA sequencing How we obtain
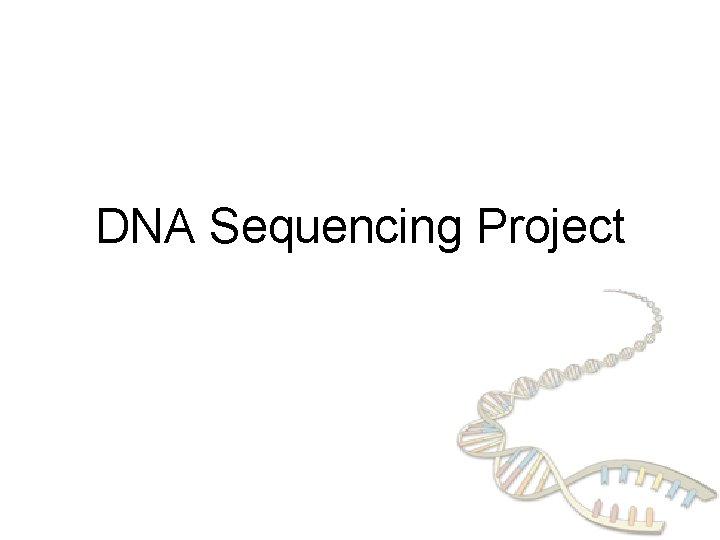
DNA Sequencing Project
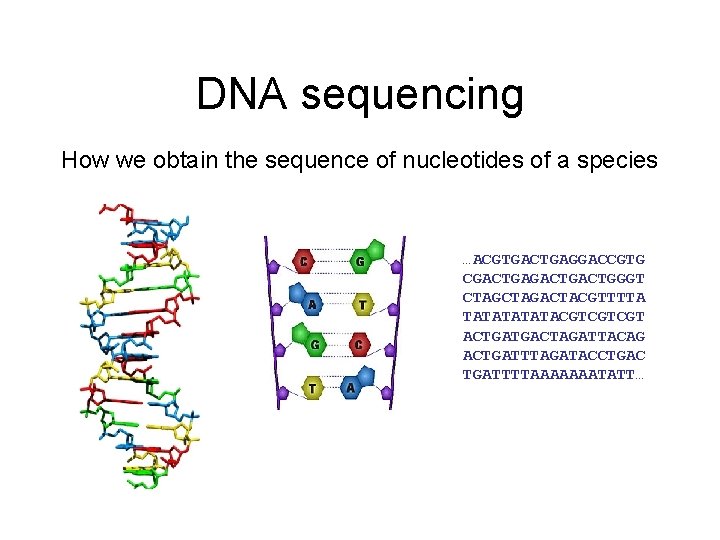
DNA sequencing How we obtain the sequence of nucleotides of a species …ACGTGACTGAGGACCGTG CGACTGACTGGGT CTAGACTACGTTTTA TATATACGTCGTCGT ACTGATGACTAGATTACAG ACTGATTTAGATACCTGAC TGATTTTAAAAAAATATT…
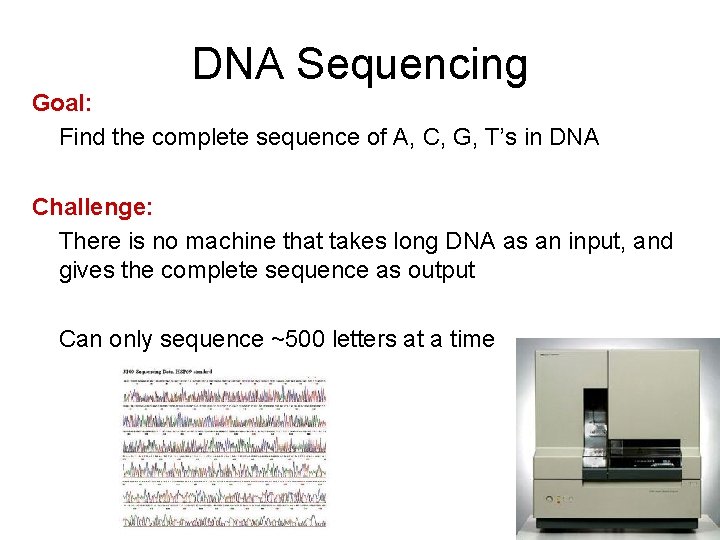
DNA Sequencing Goal: Find the complete sequence of A, C, G, T’s in DNA Challenge: There is no machine that takes long DNA as an input, and gives the complete sequence as output Can only sequence ~500 letters at a time
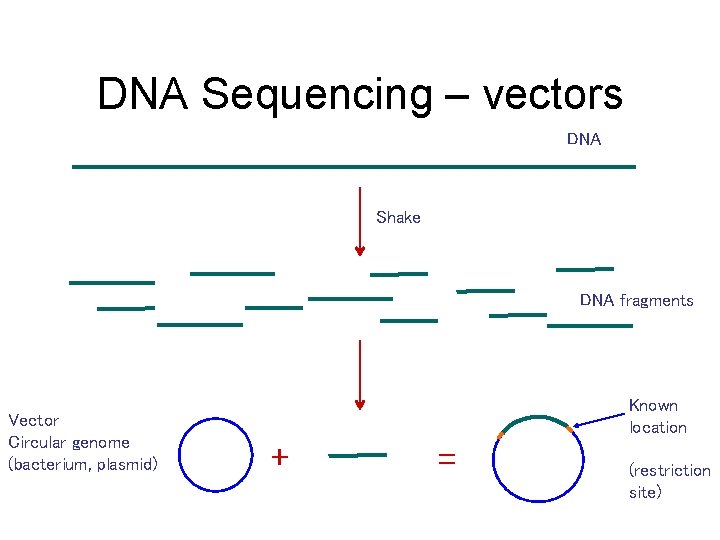
DNA Sequencing – vectors DNA Shake DNA fragments Vector Circular genome (bacterium, plasmid) Known location + = (restriction site)
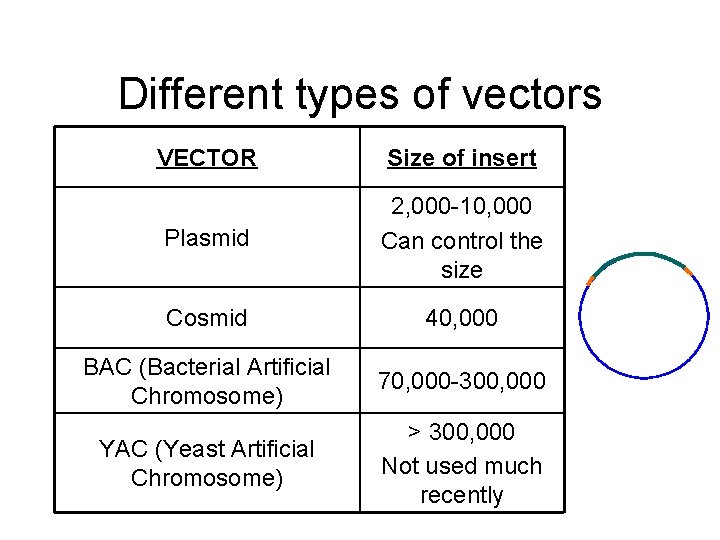
Different types of vectors VECTOR Size of insert Plasmid 2, 000 -10, 000 Can control the size Cosmid 40, 000 BAC (Bacterial Artificial Chromosome) 70, 000 -300, 000 YAC (Yeast Artificial Chromosome) > 300, 000 Not used much recently
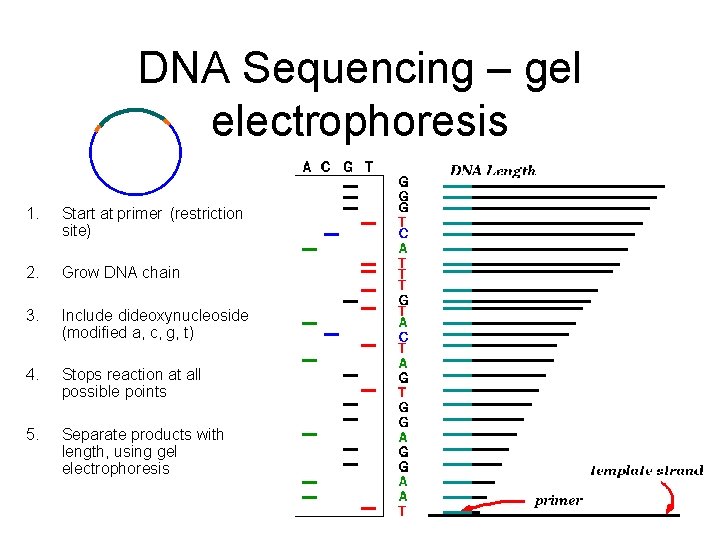
DNA Sequencing – gel electrophoresis 1. Start at primer (restriction site) 2. Grow DNA chain 3. Include dideoxynucleoside (modified a, c, g, t) 4. Stops reaction at all possible points 5. Separate products with length, using gel electrophoresis
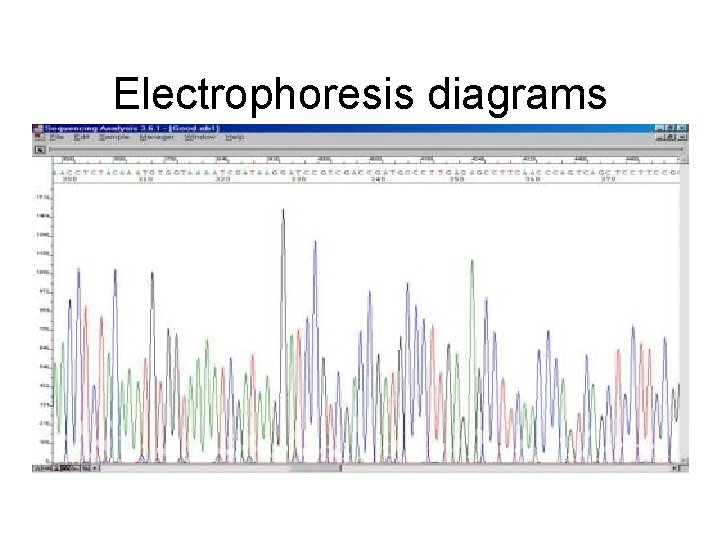
Electrophoresis diagrams
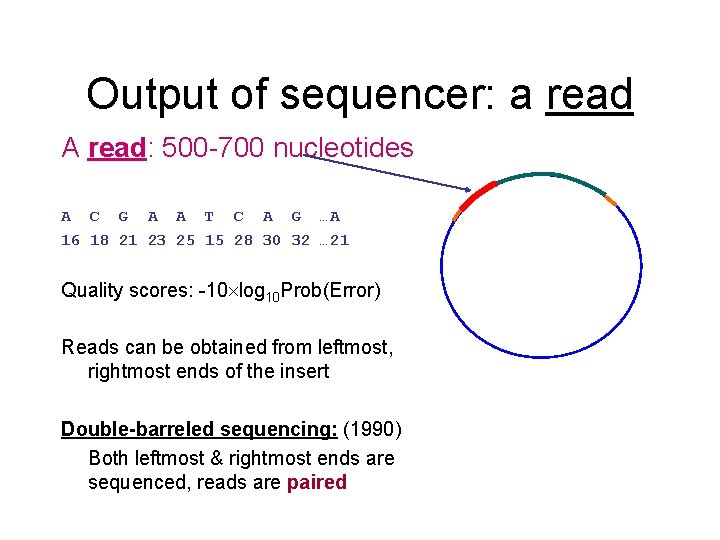
Output of sequencer: a read A read: 500 -700 nucleotides A C G A A T C A G …A 16 18 21 23 25 15 28 30 32 … 21 Quality scores: -10 log 10 Prob(Error) Reads can be obtained from leftmost, rightmost ends of the insert Double-barreled sequencing: (1990) Both leftmost & rightmost ends are sequenced, reads are paired
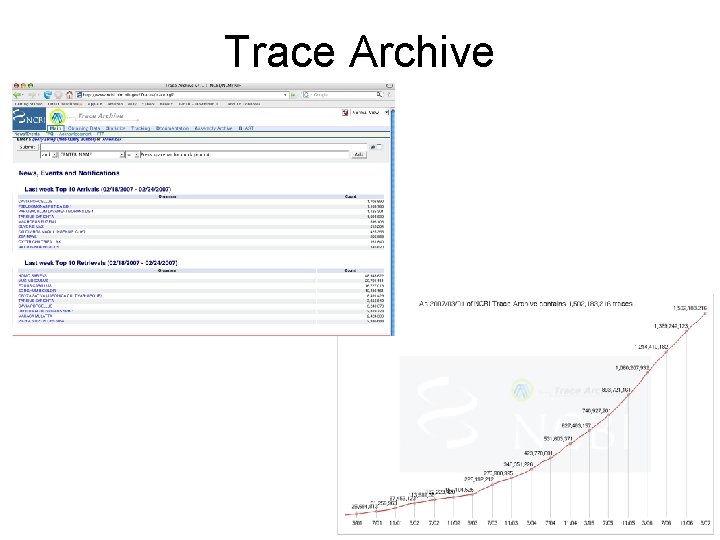
Trace Archive
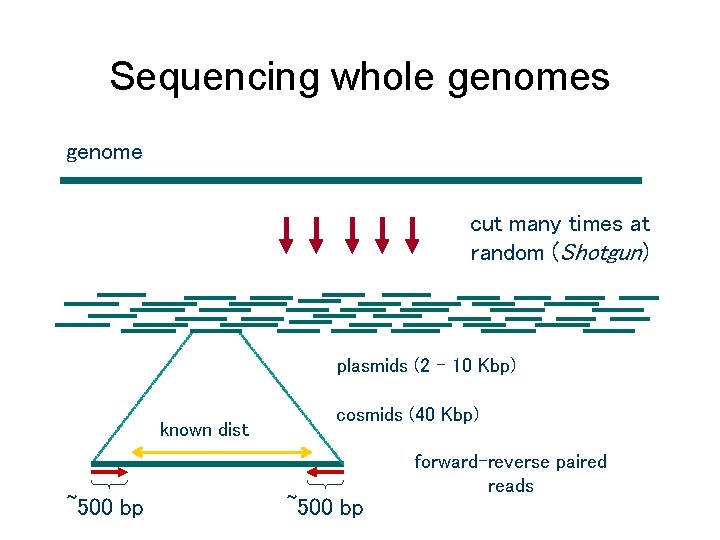
Sequencing whole genomes genome cut many times at random (Shotgun) plasmids (2 – 10 Kbp) known dist ~500 bp cosmids (40 Kbp) ~500 bp forward-reverse paired reads
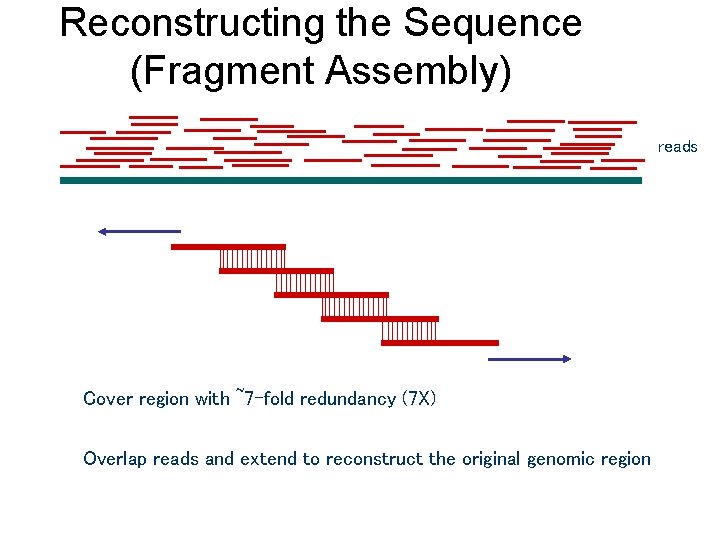
Reconstructing the Sequence (Fragment Assembly) reads Cover region with ~7 -fold redundancy (7 X) Overlap reads and extend to reconstruct the original genomic region
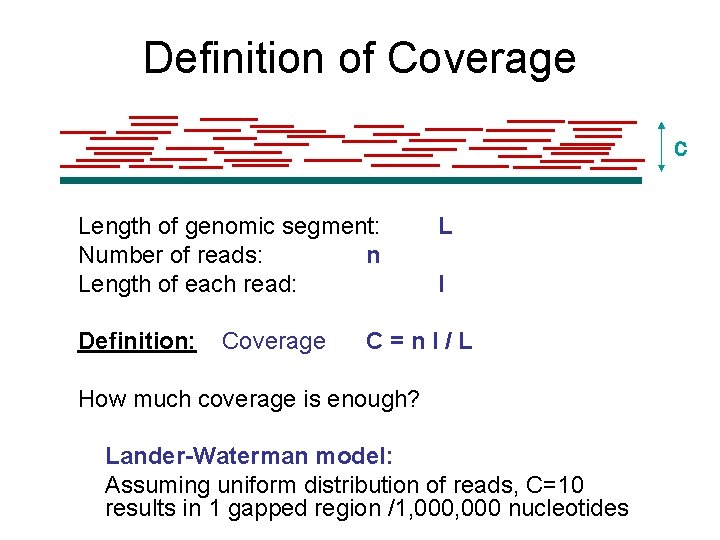
Definition of Coverage C Length of genomic segment: Number of reads: n Length of each read: Definition: Coverage L l C=nl/L How much coverage is enough? Lander-Waterman model: Assuming uniform distribution of reads, C=10 results in 1 gapped region /1, 000 nucleotides
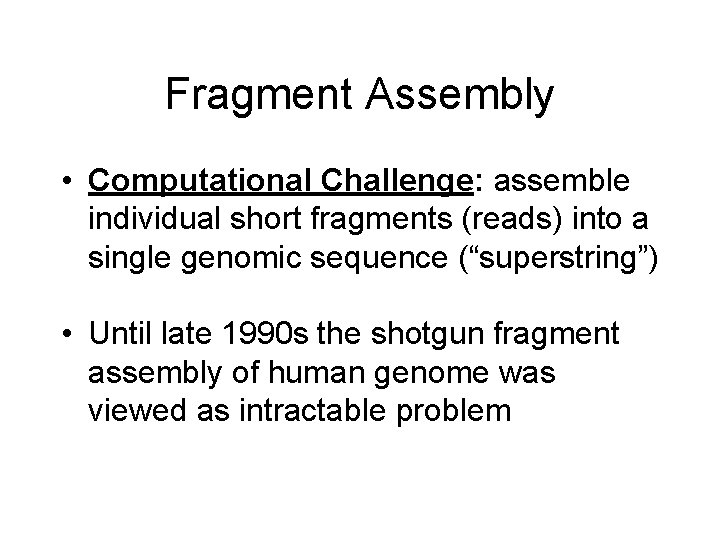
Fragment Assembly • Computational Challenge: assemble individual short fragments (reads) into a single genomic sequence (“superstring”) • Until late 1990 s the shotgun fragment assembly of human genome was viewed as intractable problem
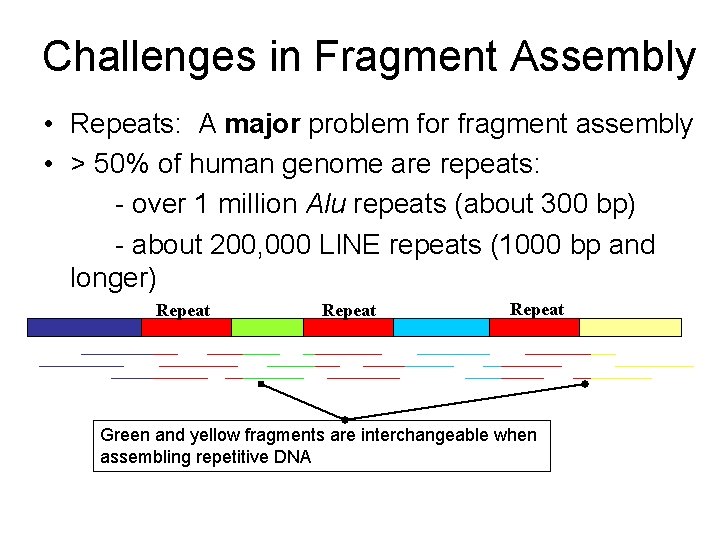
Challenges in Fragment Assembly • Repeats: A major problem for fragment assembly • > 50% of human genome are repeats: - over 1 million Alu repeats (about 300 bp) - about 200, 000 LINE repeats (1000 bp and longer) Repeat Green and yellow fragments are interchangeable when assembling repetitive DNA
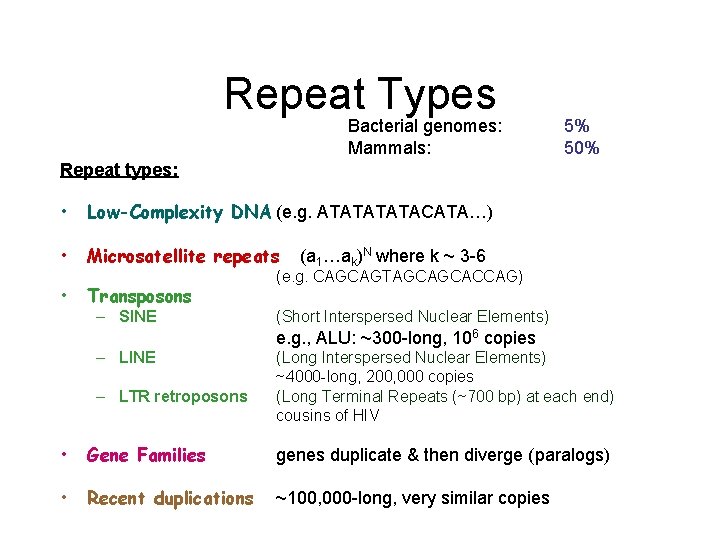
Repeat Types Bacterial genomes: Mammals: 5% 50% Repeat types: • Low-Complexity DNA (e. g. ATATACATA…) • Microsatellite repeats • Transposons – SINE (a 1…ak)N where k ~ 3 -6 (e. g. CAGCAGTAGCAGCACCAG) (Short Interspersed Nuclear Elements) e. g. , ALU: ~300 -long, 106 copies – LINE – LTR retroposons (Long Interspersed Nuclear Elements) ~4000 -long, 200, 000 copies (Long Terminal Repeats (~700 bp) at each end) cousins of HIV • Gene Families genes duplicate & then diverge (paralogs) • Recent duplications ~100, 000 -long, very similar copies
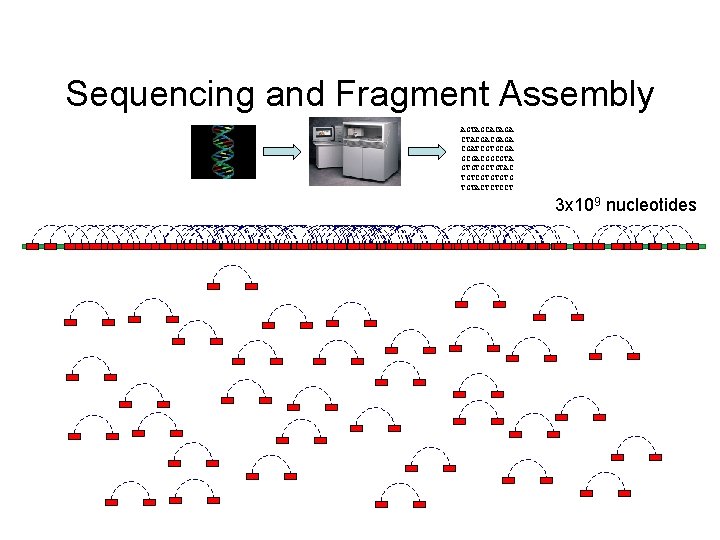
Sequencing and Fragment Assembly AGTAGCACAGA CTACGACGAGA CGATCGTGCGACGGCGTA GTGTGCTGTAC TGTCGTGTGTG TGTACTCTCCT 3 x 109 nucleotides
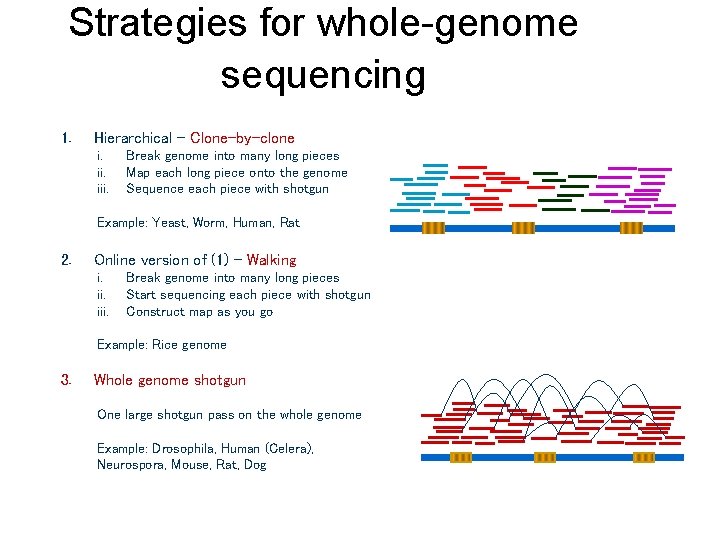
Strategies for whole-genome sequencing 1. Hierarchical – Clone-by-clone i. iii. Break genome into many long pieces Map each long piece onto the genome Sequence each piece with shotgun Example: Yeast, Worm, Human, Rat 2. Online version of (1) – Walking i. iii. Break genome into many long pieces Start sequencing each piece with shotgun Construct map as you go Example: Rice genome 3. Whole genome shotgun One large shotgun pass on the whole genome Example: Drosophila, Human (Celera), Neurospora, Mouse, Rat, Dog
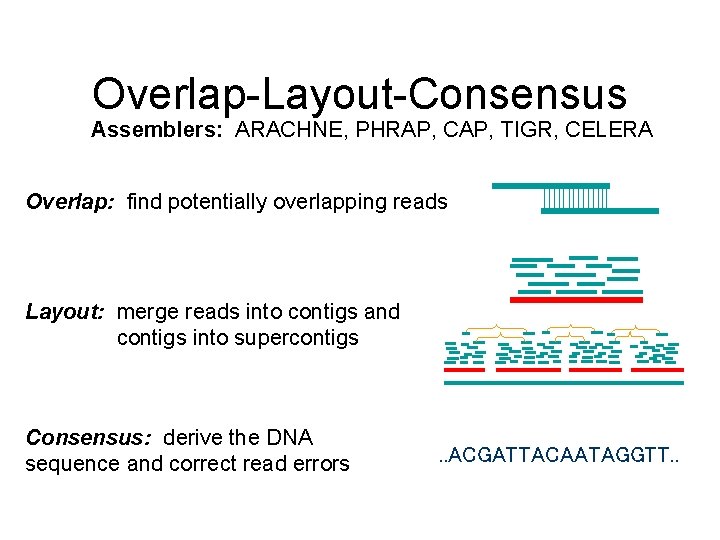
Overlap-Layout-Consensus Assemblers: ARACHNE, PHRAP, CAP, TIGR, CELERA Overlap: find potentially overlapping reads Layout: merge reads into contigs and contigs into supercontigs Consensus: derive the DNA sequence and correct read errors . . ACGATTACAATAGGTT. .
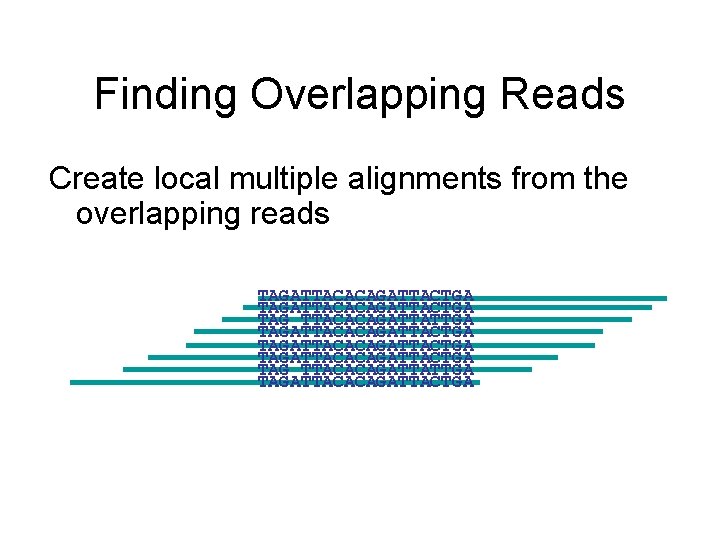
Finding Overlapping Reads Create local multiple alignments from the overlapping reads TAGATTACACAGATTACTGA TAG TTACACAGATTATTGA TAGATTACACAGATTACTGA
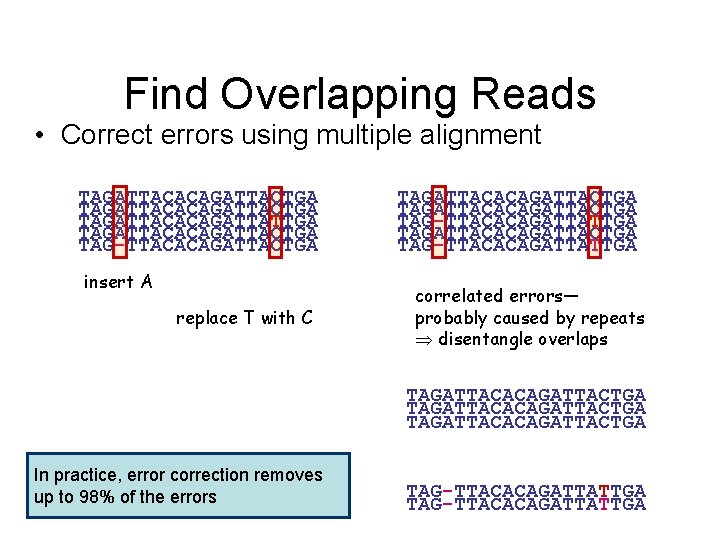
Find Overlapping Reads • Correct errors using multiple alignment TAGATTACACAGATTACTGA TAGATTACACAGATTATTGA TAGATTACACAGATTACTGA TAG-TTACACAGATTACTGA insert A replace T with C TAGATTACACAGATTACTGA TAG-TTACACAGATTATTGA correlated errors— probably caused by repeats disentangle overlaps TAGATTACACAGATTACTGA In practice, error correction removes up to 98% of the errors TAG-TTACACAGATTATTGA
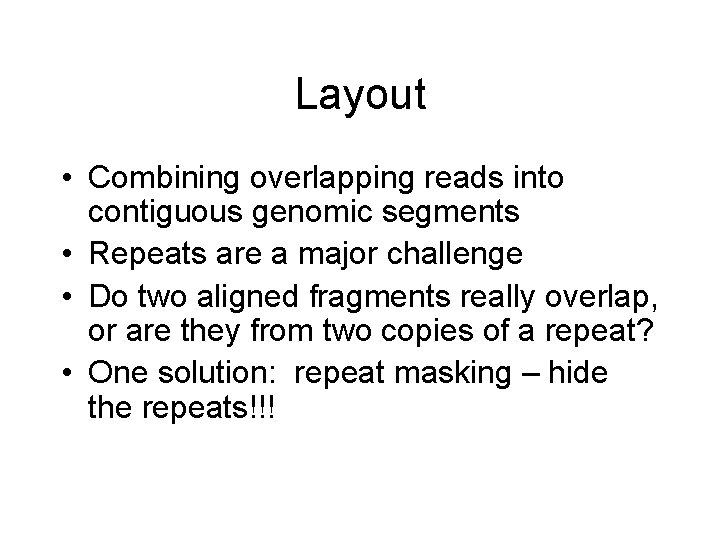
Layout • Combining overlapping reads into contiguous genomic segments • Repeats are a major challenge • Do two aligned fragments really overlap, or are they from two copies of a repeat? • One solution: repeat masking – hide the repeats!!!
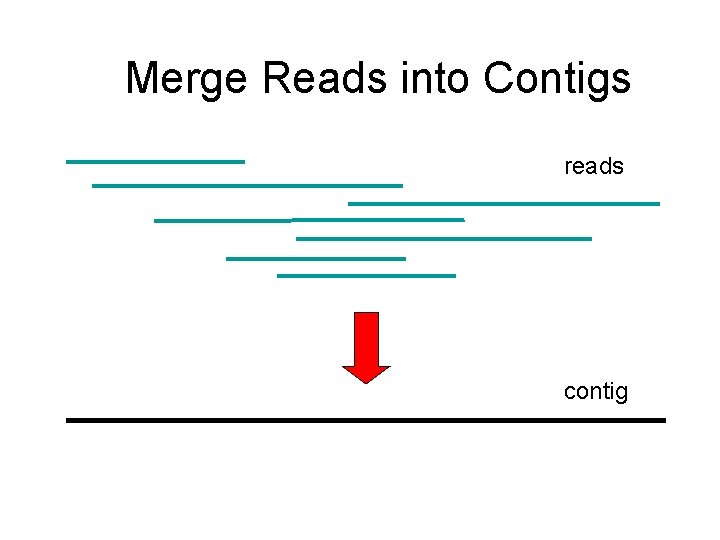
Merge Reads into Contigs reads contig
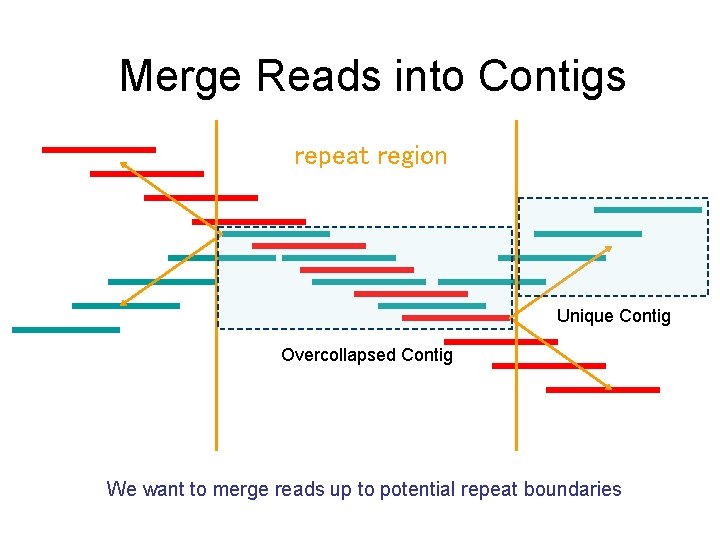
Merge Reads into Contigs repeat region Unique Contig Overcollapsed Contig We want to merge reads up to potential repeat boundaries
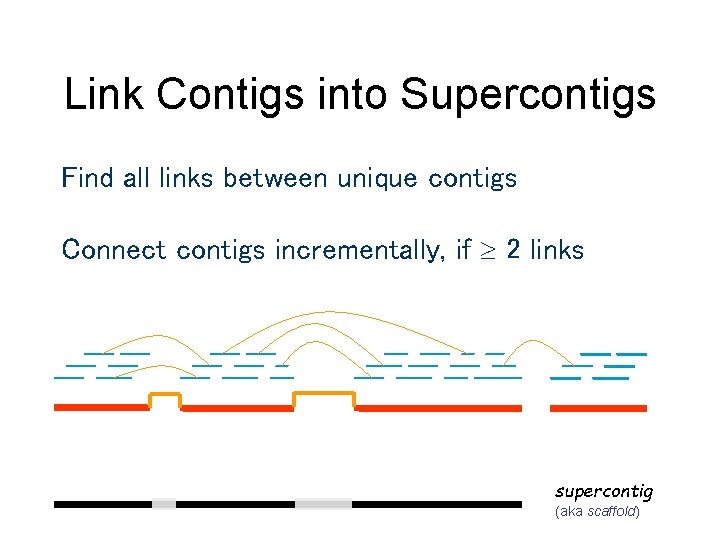
Link Contigs into Supercontigs Find all links between unique contigs Connect contigs incrementally, if 2 links supercontig (aka scaffold)
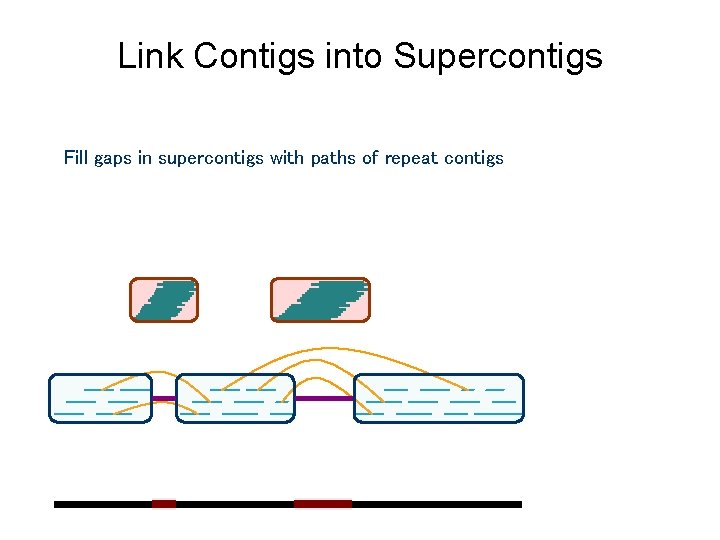
Link Contigs into Supercontigs Fill gaps in supercontigs with paths of repeat contigs
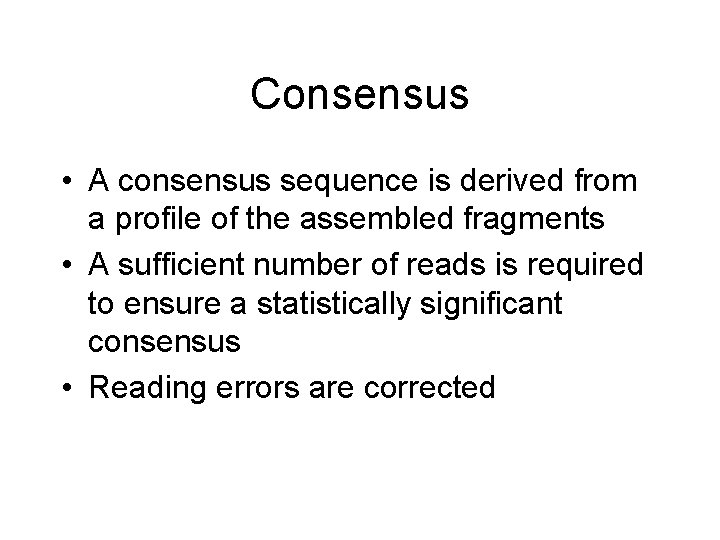
Consensus • A consensus sequence is derived from a profile of the assembled fragments • A sufficient number of reads is required to ensure a statistically significant consensus • Reading errors are corrected
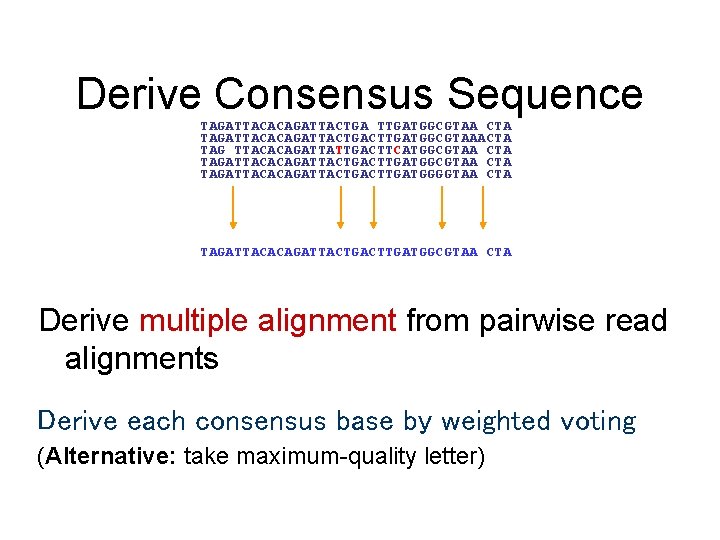
Derive Consensus Sequence TAGATTACACAGATTACTGA TTGATGGCGTAA CTA TAGATTACACAGATTACTGACTTGATGGCGTAAACTA TAG TTACACAGATTATTGACTTCATGGCGTAA CTA TAGATTACACAGATTACTGACTTGATGGGGTAA CTA TAGATTACACAGATTACTGACTTGATGGCGTAA CTA Derive multiple alignment from pairwise read alignments Derive each consensus base by weighted voting (Alternative: take maximum-quality letter)
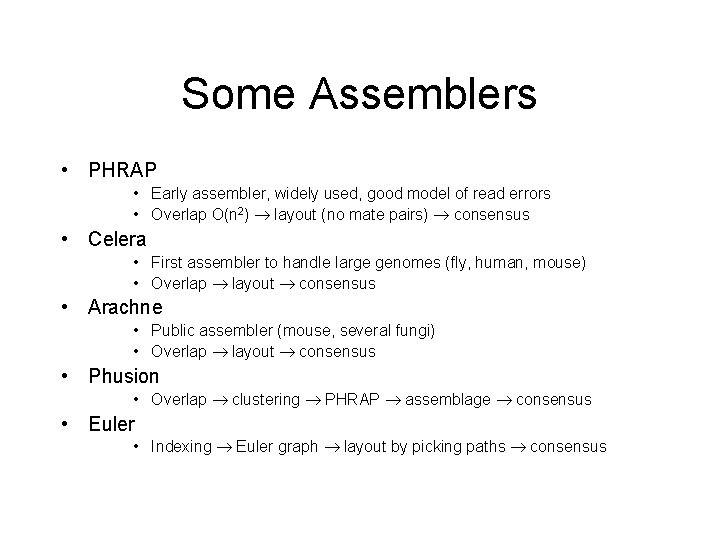
Some Assemblers • PHRAP • Early assembler, widely used, good model of read errors • Overlap O(n 2) layout (no mate pairs) consensus • Celera • First assembler to handle large genomes (fly, human, mouse) • Overlap layout consensus • Arachne • Public assembler (mouse, several fungi) • Overlap layout consensus • Phusion • Overlap clustering PHRAP assemblage consensus • Euler • Indexing Euler graph layout by picking paths consensus
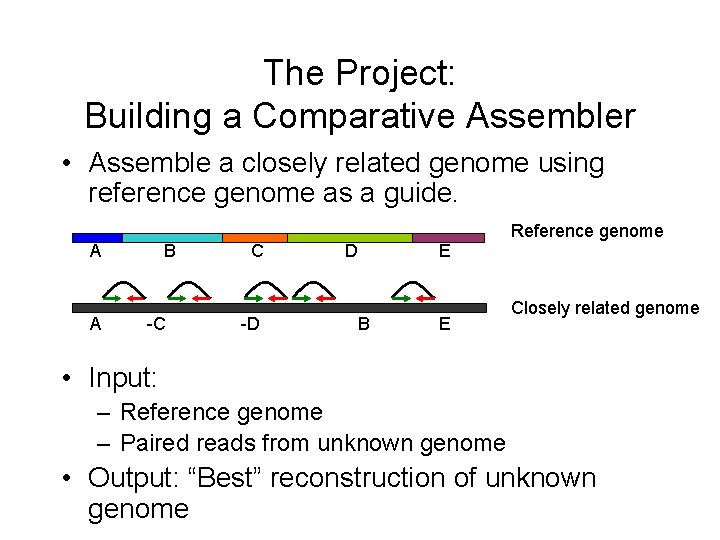
The Project: Building a Comparative Assembler • Assemble a closely related genome using reference genome as a guide. A A B -C C -D D B E E Reference genome Closely related genome • Input: – Reference genome – Paired reads from unknown genome • Output: “Best” reconstruction of unknown genome
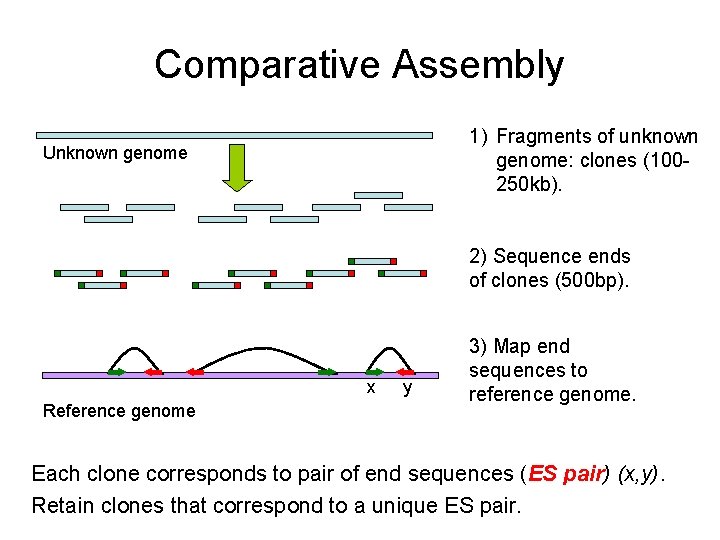
Comparative Assembly 1) Fragments of unknown genome: clones (100250 kb). Unknown genome 2) Sequence ends of clones (500 bp). x Reference genome y 3) Map end sequences to reference genome. Each clone corresponds to pair of end sequences (ES pair) (x, y). Retain clones that correspond to a unique ES pair.
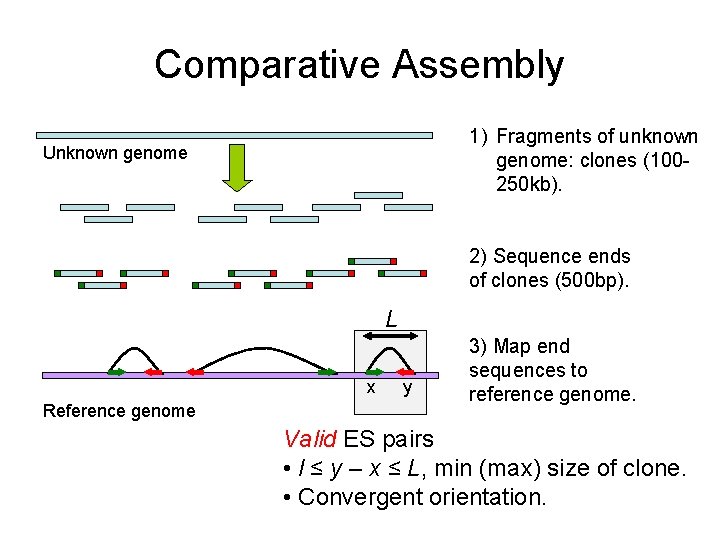
Comparative Assembly 1) Fragments of unknown genome: clones (100250 kb). Unknown genome 2) Sequence ends of clones (500 bp). L x Reference genome y 3) Map end sequences to reference genome. Valid ES pairs • l ≤ y – x ≤ L, min (max) size of clone. • Convergent orientation.
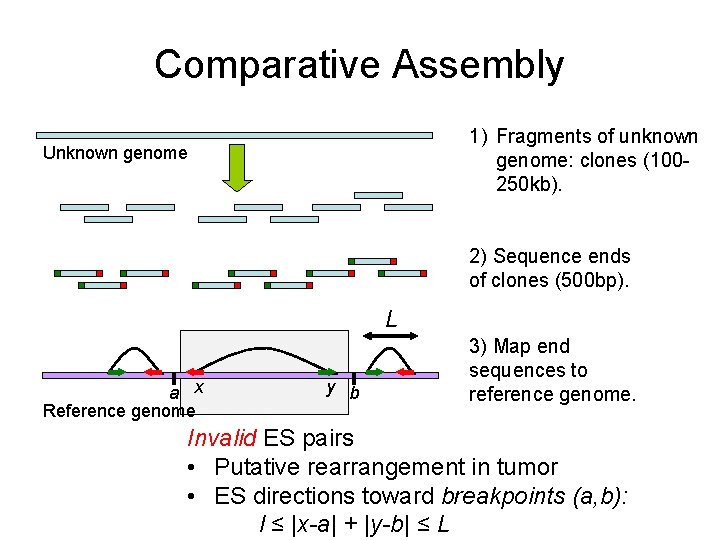
Comparative Assembly 1) Fragments of unknown genome: clones (100250 kb). Unknown genome 2) Sequence ends of clones (500 bp). L a x Reference genome y b 3) Map end sequences to reference genome. Invalid ES pairs • Putative rearrangement in tumor • ES directions toward breakpoints (a, b): l ≤ |x-a| + |y-b| ≤ L
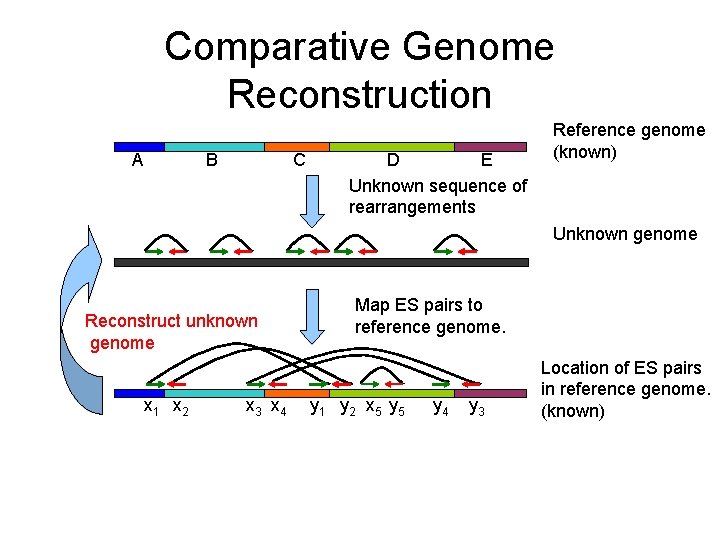
Comparative Genome Reconstruction A C B E D Unknown sequence of rearrangements Reference genome (known) Unknown genome Reconstruct unknown genome x 1 x 2 x 3 x 4 Map ES pairs to reference genome. y 1 y 2 x 5 y 4 y 3 Location of ES pairs in reference genome. (known)
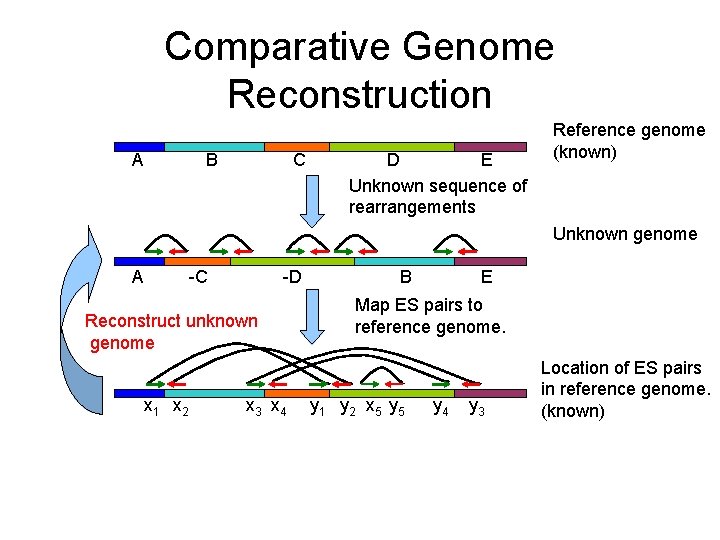
Comparative Genome Reconstruction A C B E D Unknown sequence of rearrangements Reference genome (known) Unknown genome A -C -D Reconstruct unknown genome x 1 x 2 x 3 x 4 E B Map ES pairs to reference genome. y 1 y 2 x 5 y 4 y 3 Location of ES pairs in reference genome. (known)
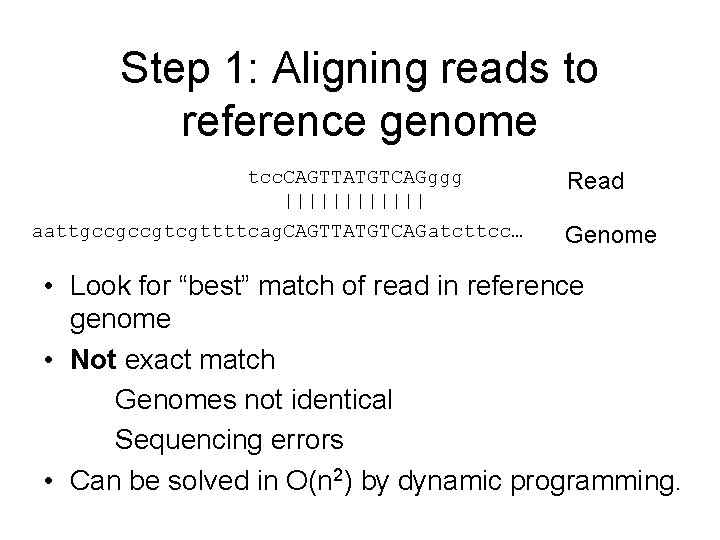
Step 1: Aligning reads to reference genome tcc. CAGTTATGTCAGggg |||||| aattgccgccgtcgttttcag. CAGTTATGTCAGatcttcc… Read Genome • Look for “best” match of read in reference genome • Not exact match Genomes not identical Sequencing errors • Can be solved in O(n 2) by dynamic programming.
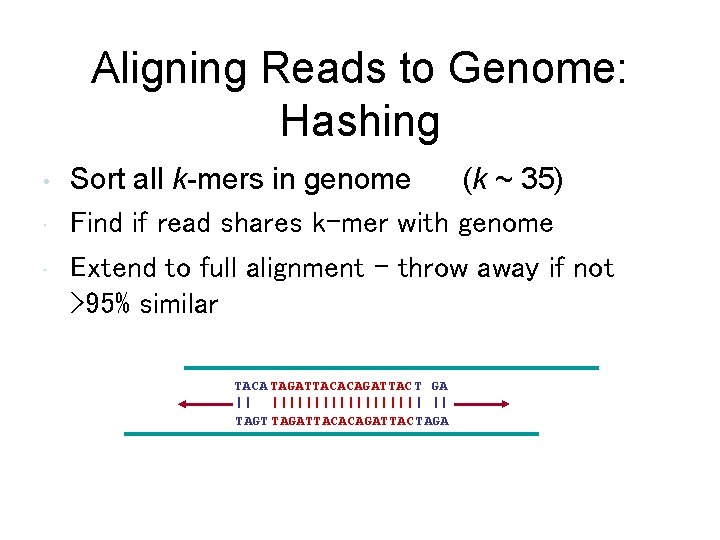
Aligning Reads to Genome: Hashing • • • Sort all k-mers in genome (k ~ 35) Find if read shares k-mer with genome Extend to full alignment – throw away if not >95% similar TACA TAGATTACACAGATTAC T GA || ||||||||| | || TAGT TAGATTACACAGATTAC TAGA
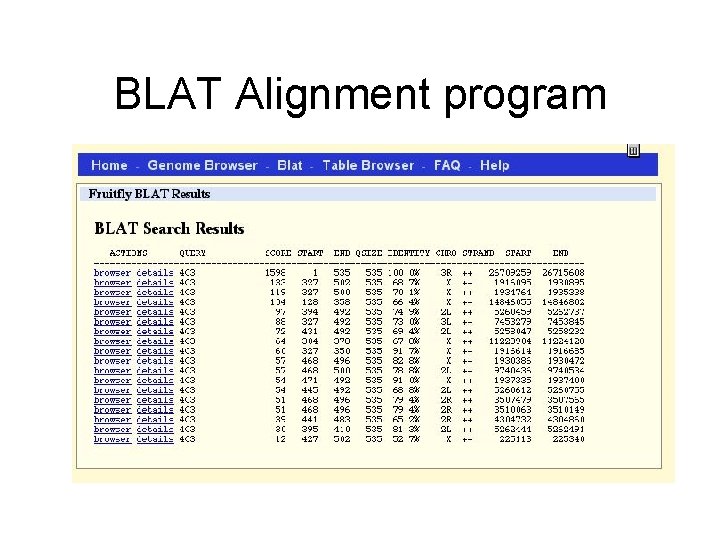
BLAT Alignment program
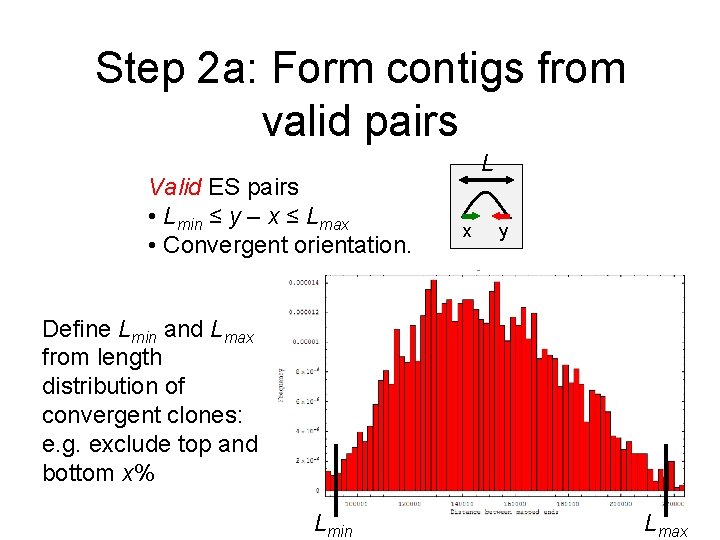
Step 2 a: Form contigs from valid pairs Valid ES pairs • Lmin ≤ y – x ≤ Lmax • Convergent orientation. L x y Define Lmin and Lmax from length distribution of convergent clones: e. g. exclude top and bottom x% Lmin Lmax
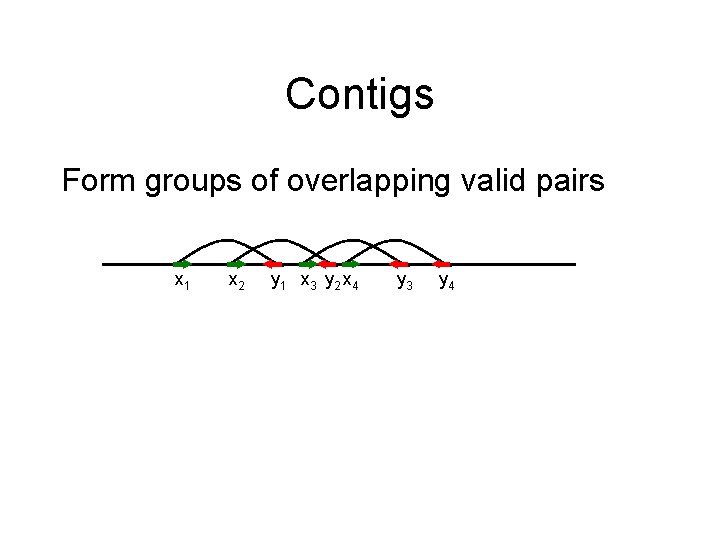
Contigs Form groups of overlapping valid pairs x 1 x 2 y 1 x 3 y 2 x 4 y 3 y 4
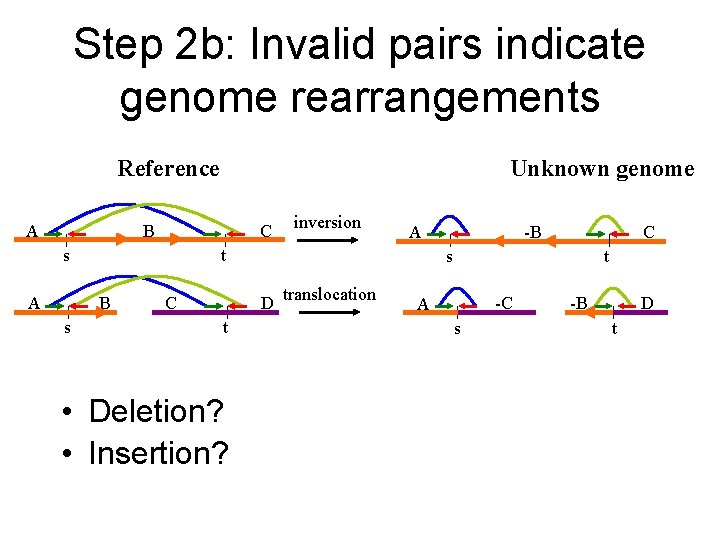
Step 2 b: Invalid pairs indicate genome rearrangements Reference A Unknown genome B C B s A t s A inversion t • Deletion? • Insertion? C t s D translocation C -B -C A s -B D t
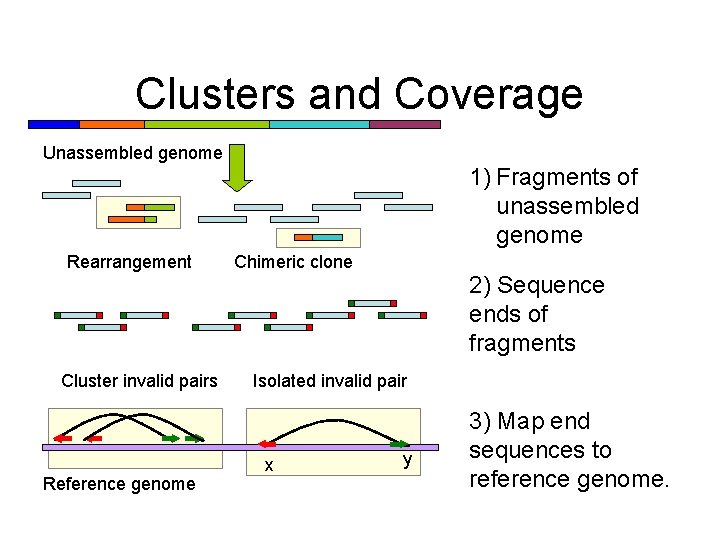
Clusters and Coverage Unassembled genome 1) Fragments of unassembled genome Rearrangement Cluster invalid pairs Reference genome Chimeric clone 2) Sequence ends of fragments Isolated invalid pair x y 3) Map end sequences to reference genome.
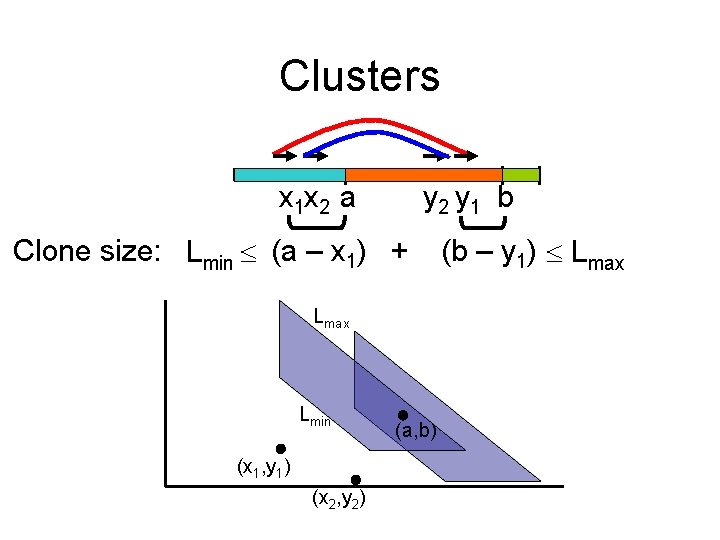
Clusters x 1 x 2 a y 2 y 1 b Clone size: Lmin (a – x 1) + Lmax Lmin (x 1, y 1) (x 2, y 2) (a, b) (b – y 1) Lmax
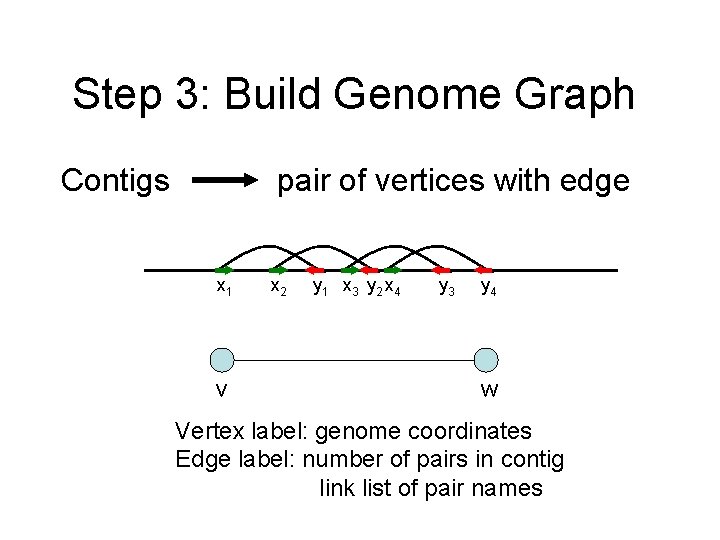
Step 3: Build Genome Graph Contigs pair of vertices with edge x 1 v x 2 y 1 x 3 y 2 x 4 y 3 y 4 w Vertex label: genome coordinates Edge label: number of pairs in contig link list of pair names
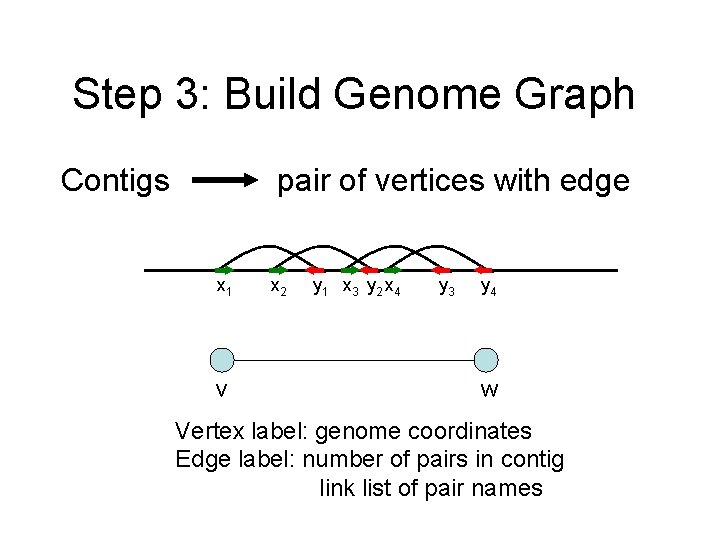
Step 3: Build Genome Graph Contigs pair of vertices with edge x 1 v x 2 y 1 x 3 y 2 x 4 y 3 y 4 w Vertex label: genome coordinates Edge label: number of pairs in contig link list of pair names
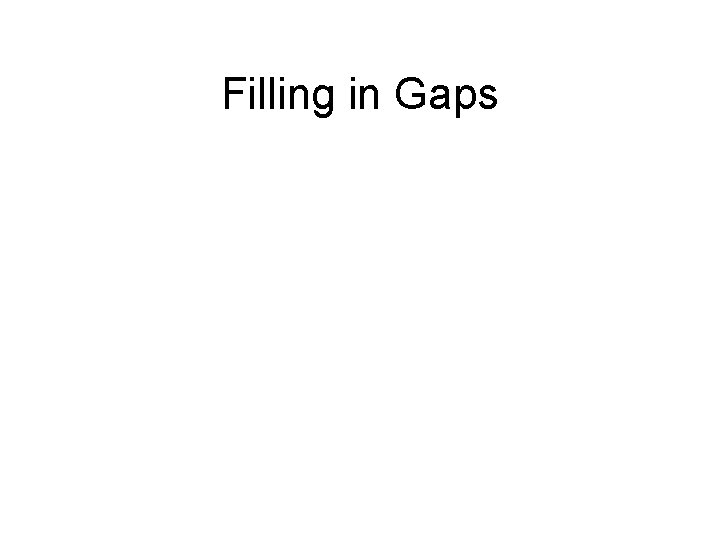
Filling in Gaps
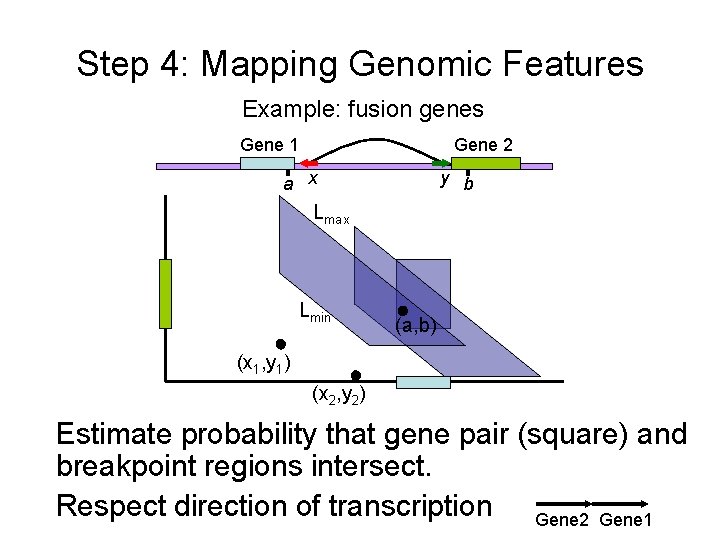
Step 4: Mapping Genomic Features Example: fusion genes Gene 1 Gene 2 a x y b Lmax Lmin (a, b) (x 1, y 1) (x 2, y 2) Estimate probability that gene pair (square) and breakpoint regions intersect. Respect direction of transcription Gene 2 Gene 1
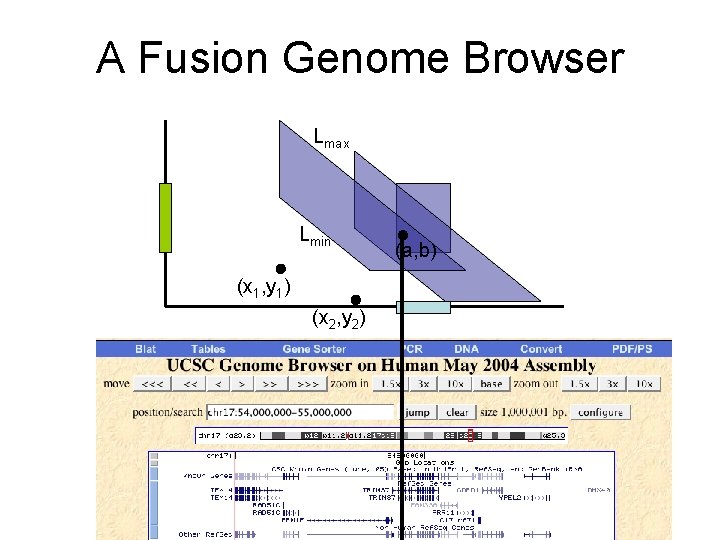
A Fusion Genome Browser Lmax Lmin (x 1, y 1) (x 2, y 2) (a, b)
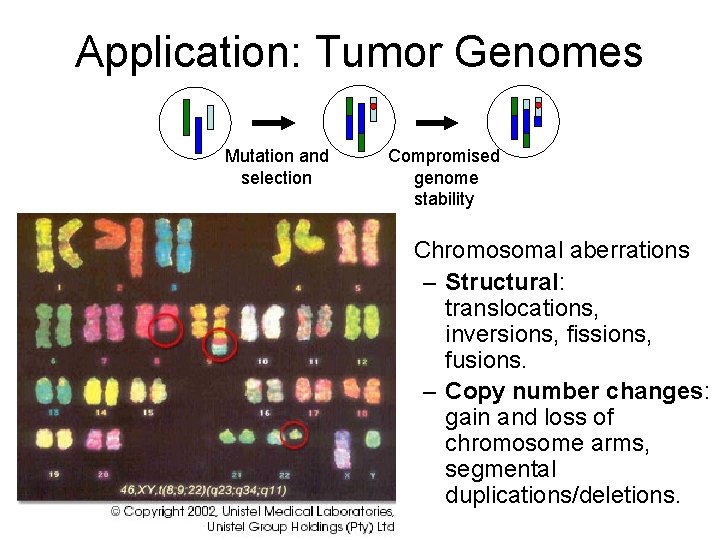
Application: Tumor Genomes Mutation and selection Compromised genome stability • Chromosomal aberrations – Structural: translocations, inversions, fissions, fusions. – Copy number changes: gain and loss of chromosome arms, segmental duplications/deletions.
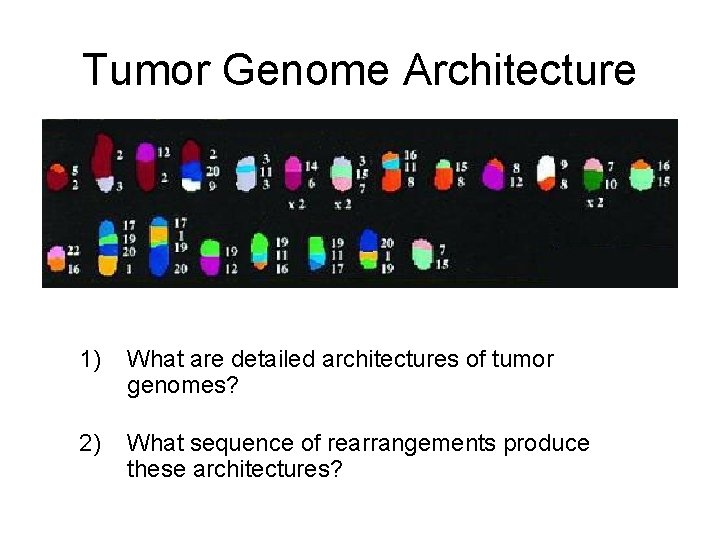
Tumor Genome Architecture 1) What are detailed architectures of tumor genomes? 2) What sequence of rearrangements produce these architectures?
- Slides: 49