Multiple Regression Multiple Regression n Regression Attempts to
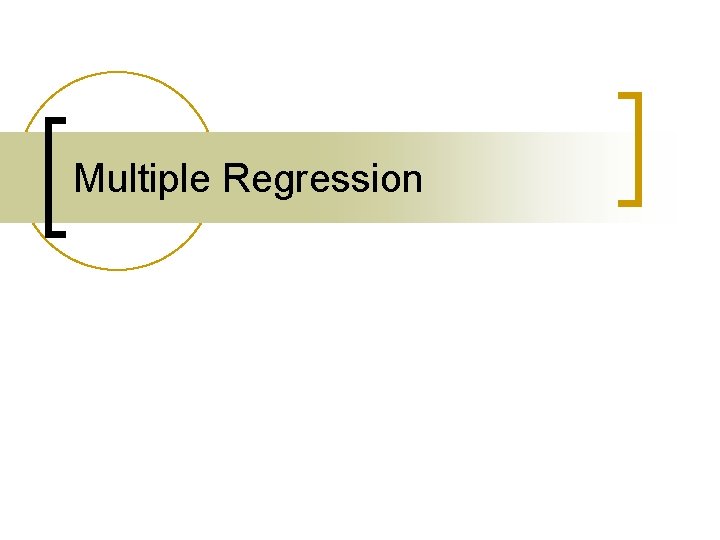
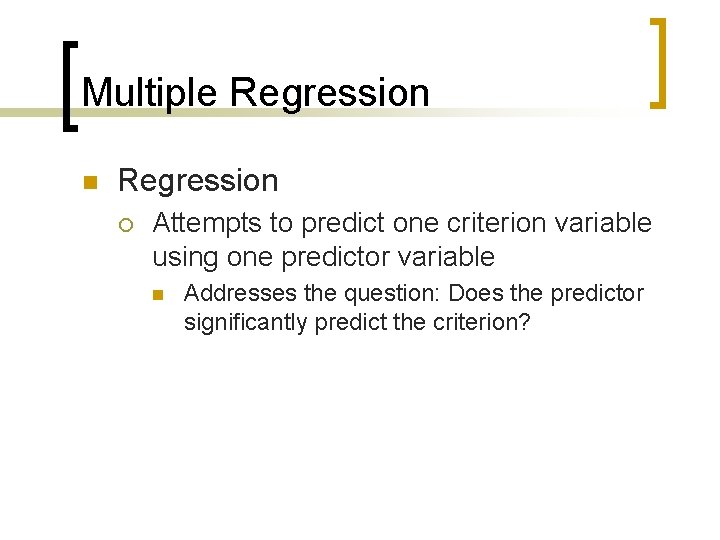
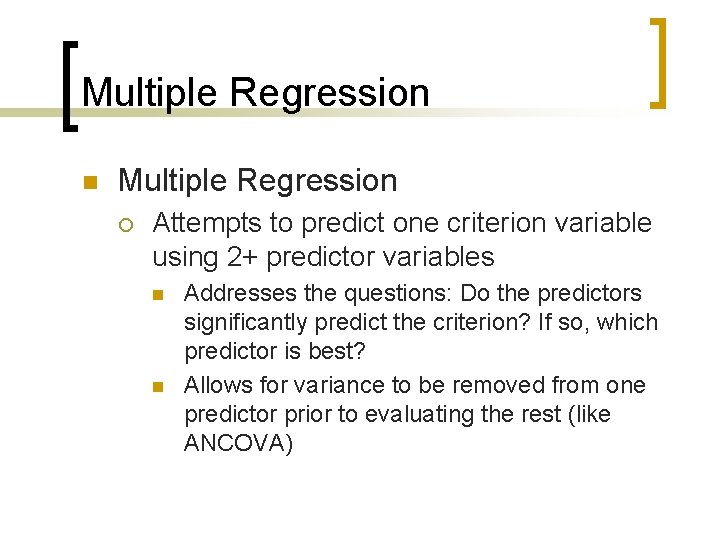
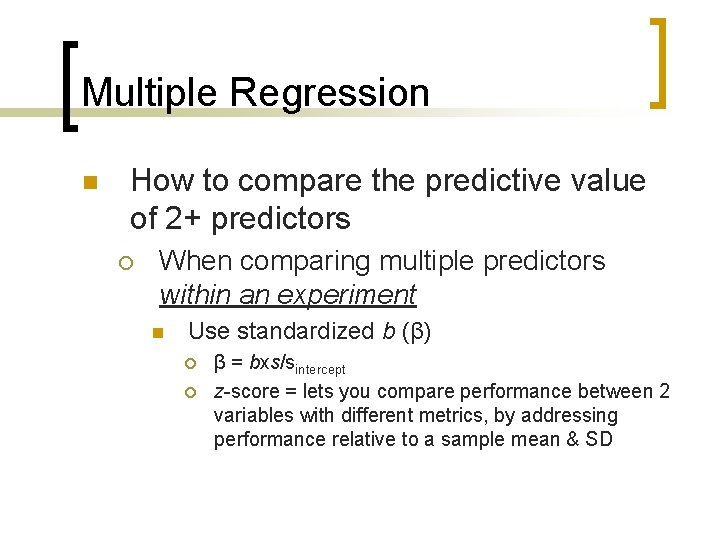
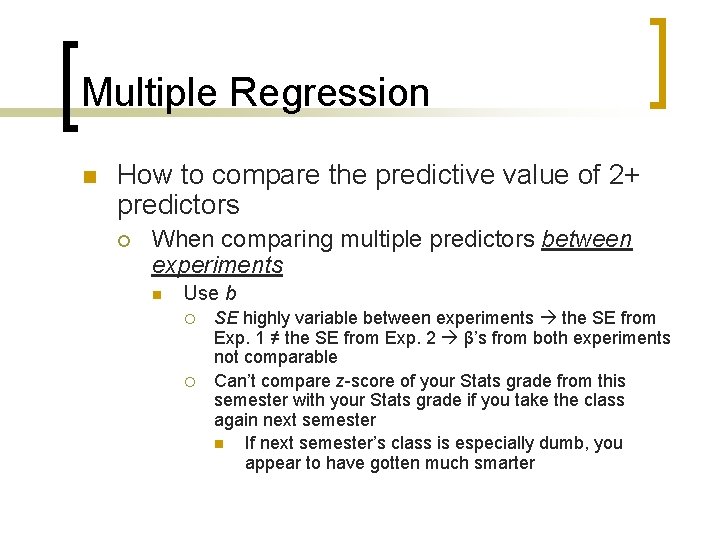
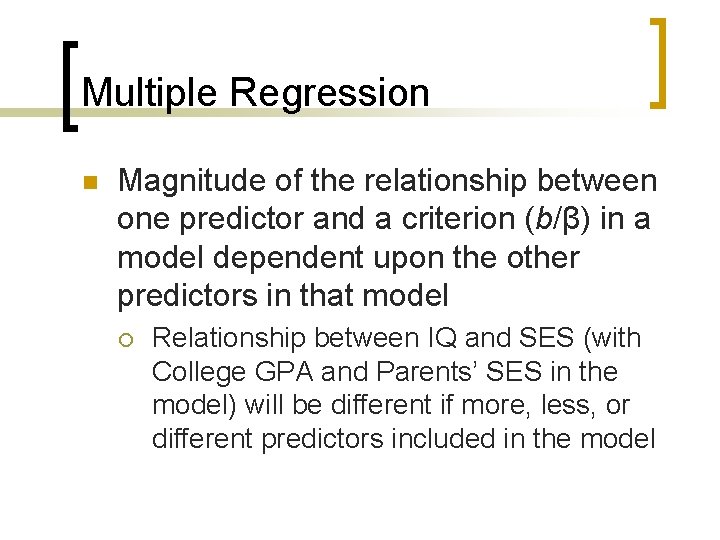
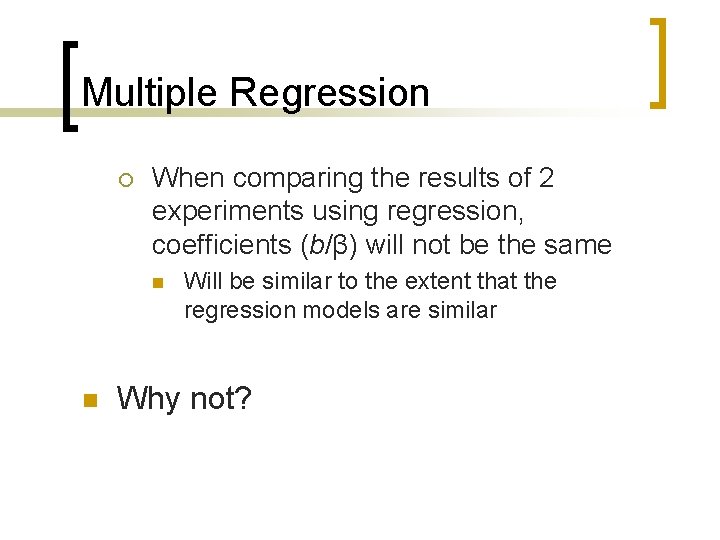
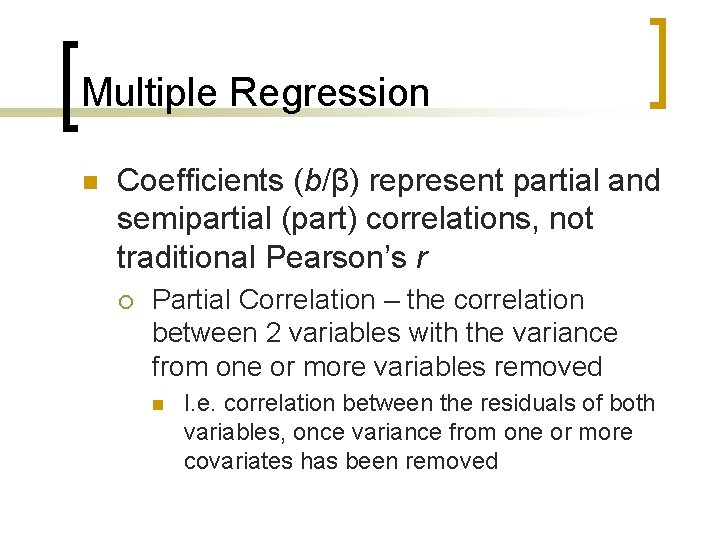
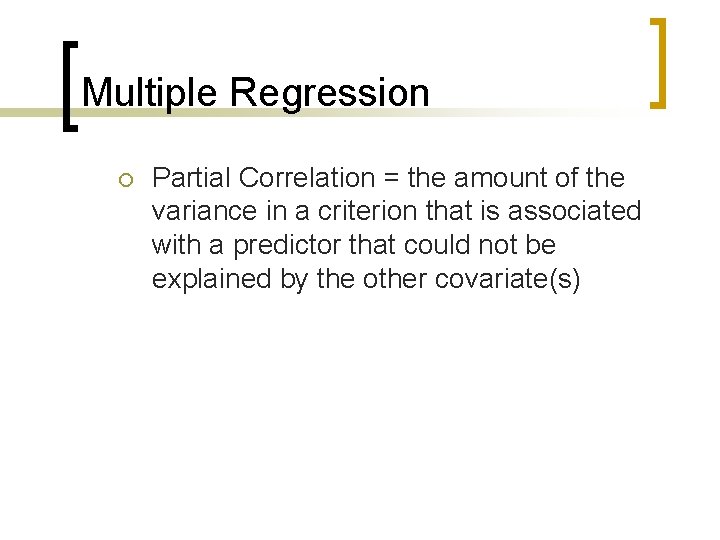
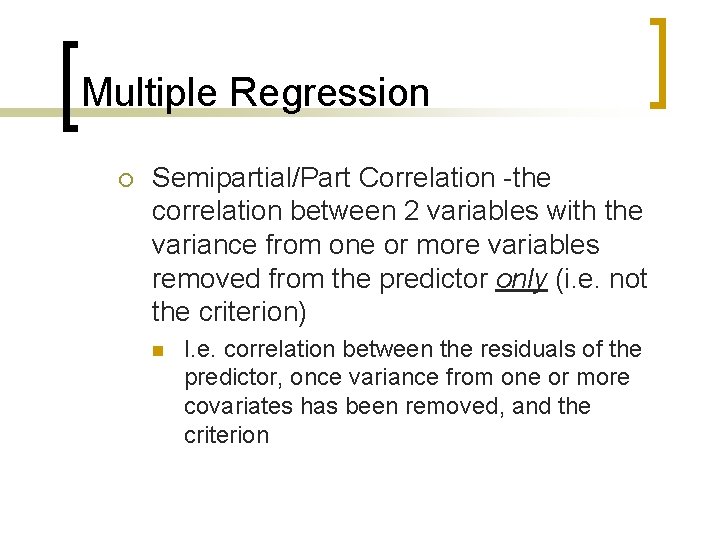
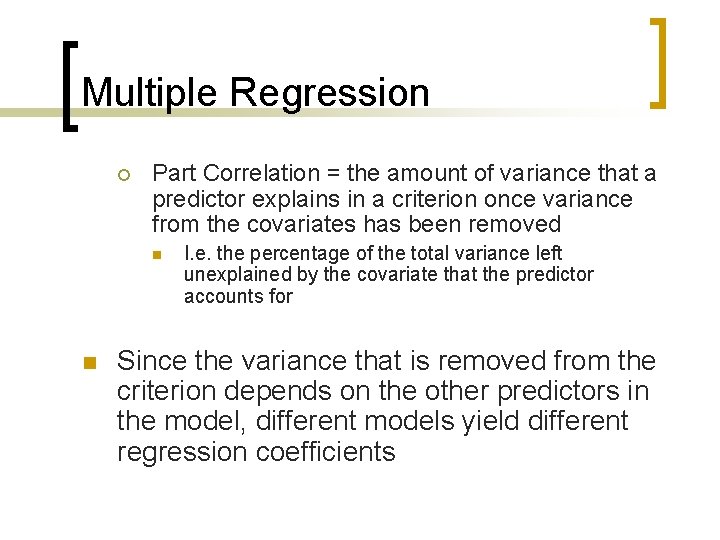
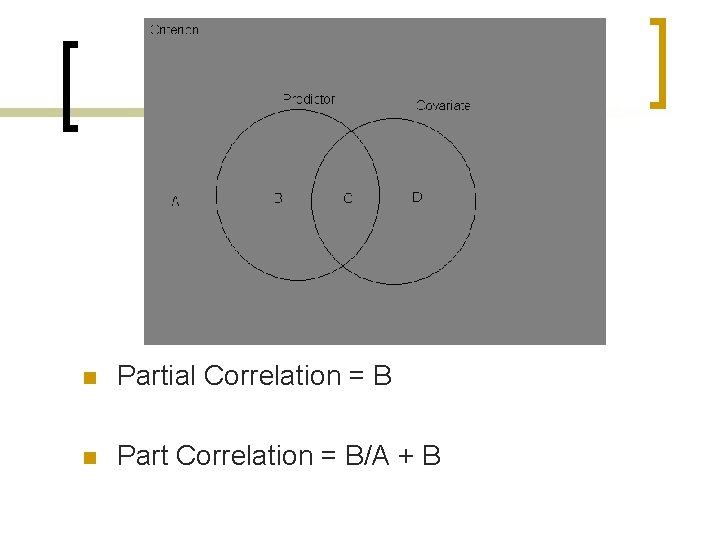
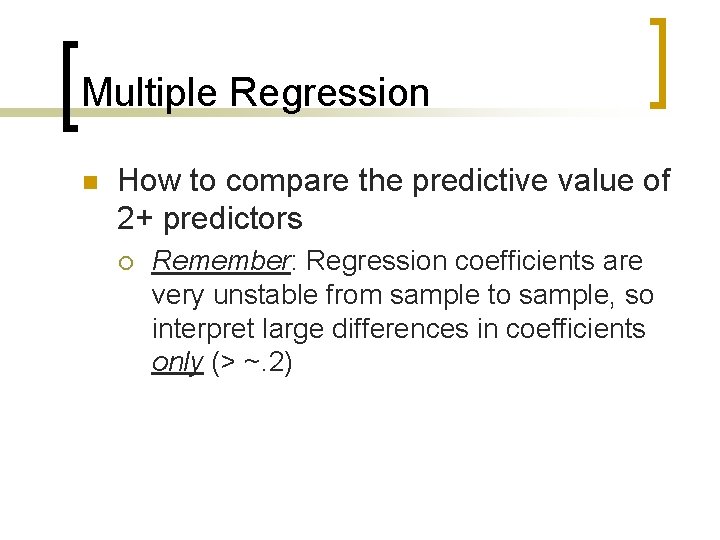
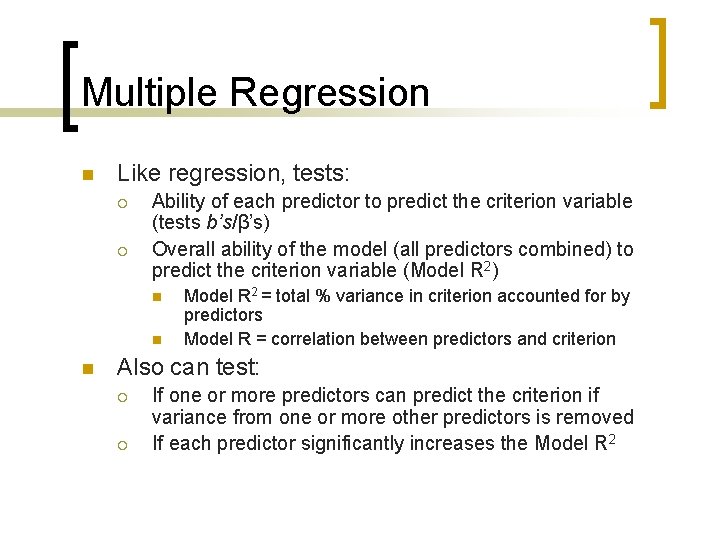
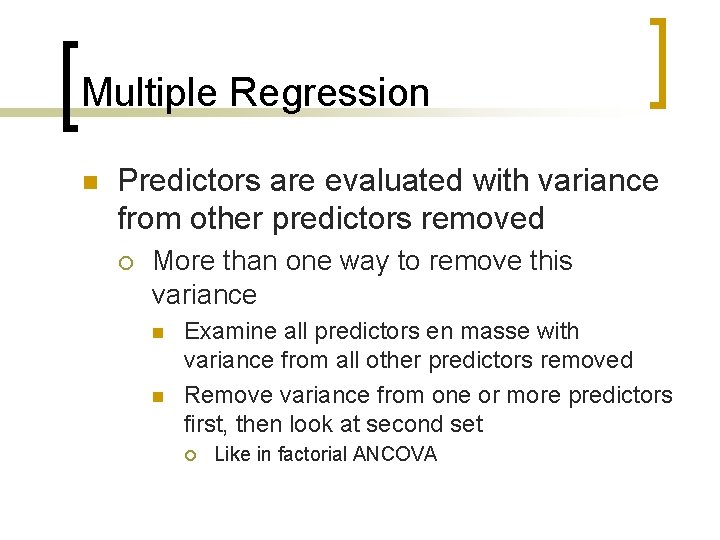
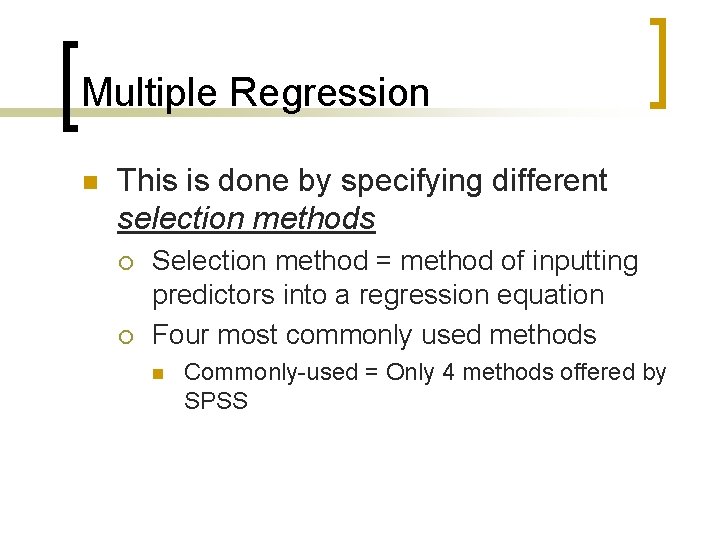
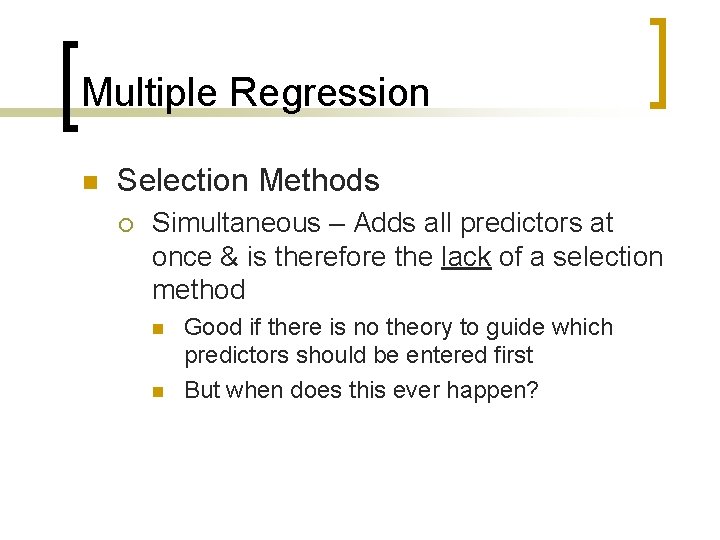
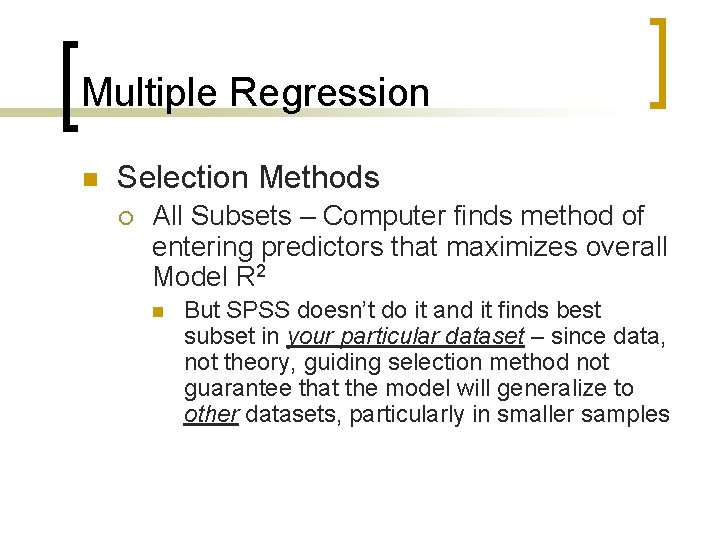
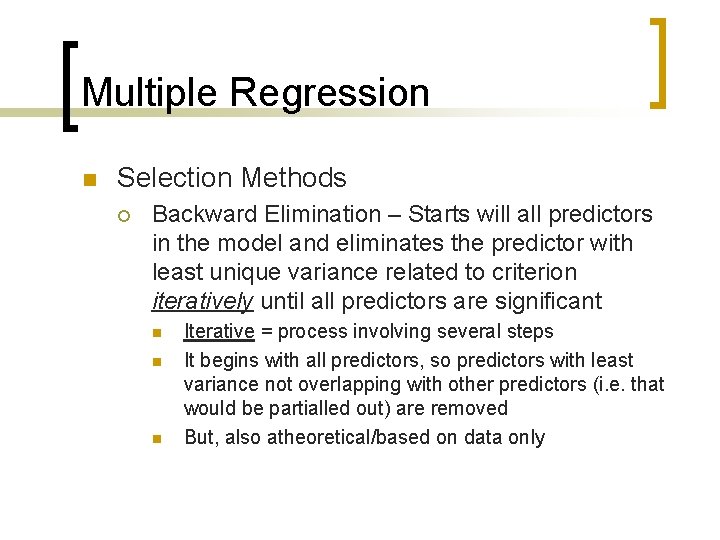
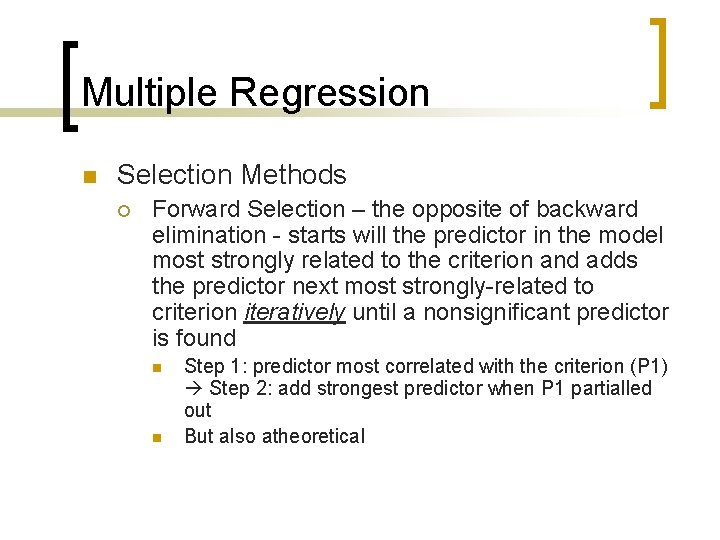
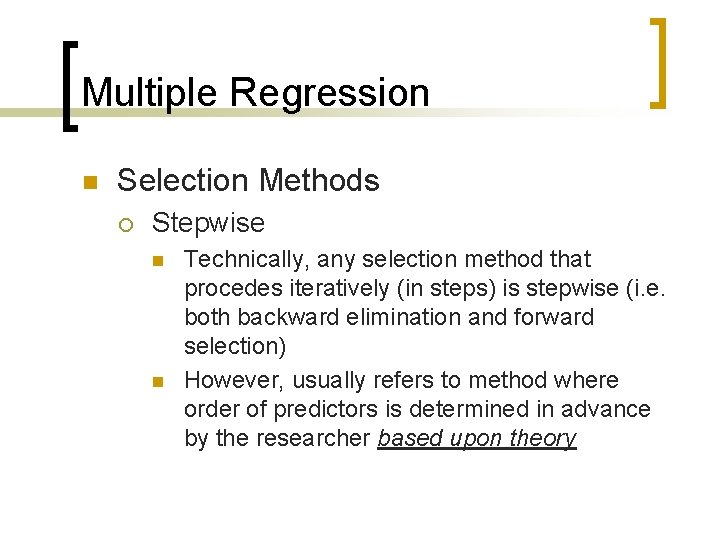
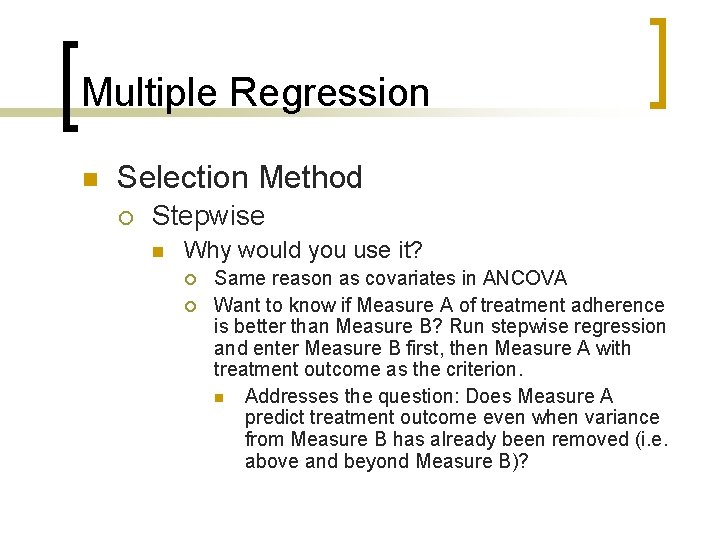
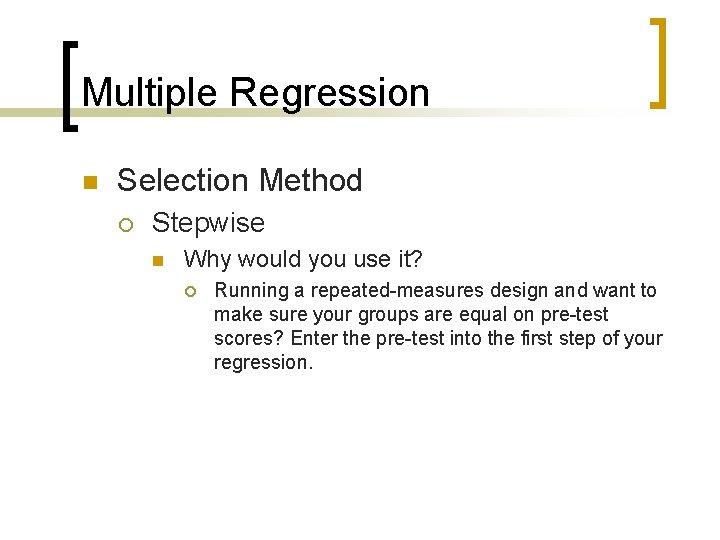
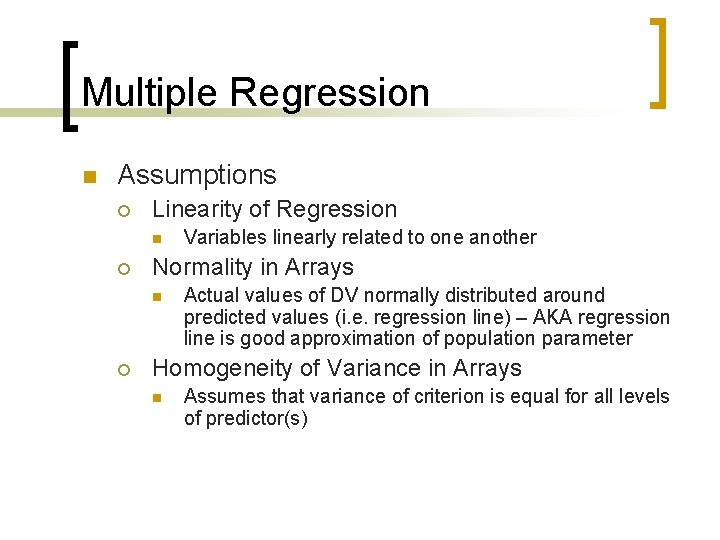
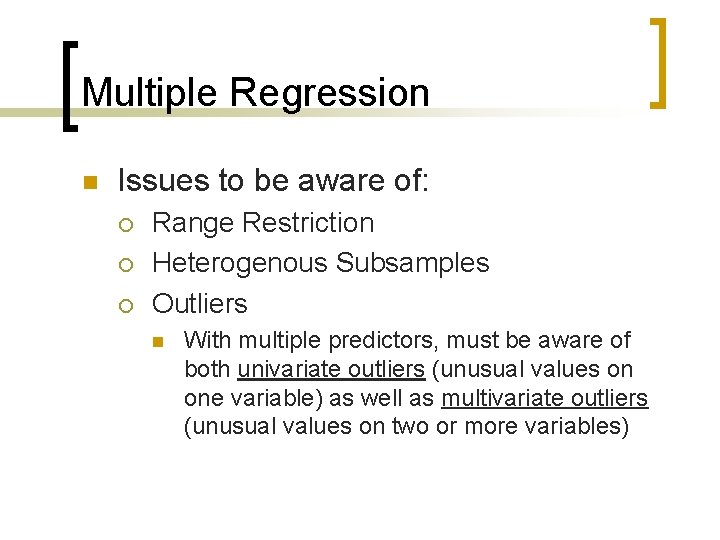
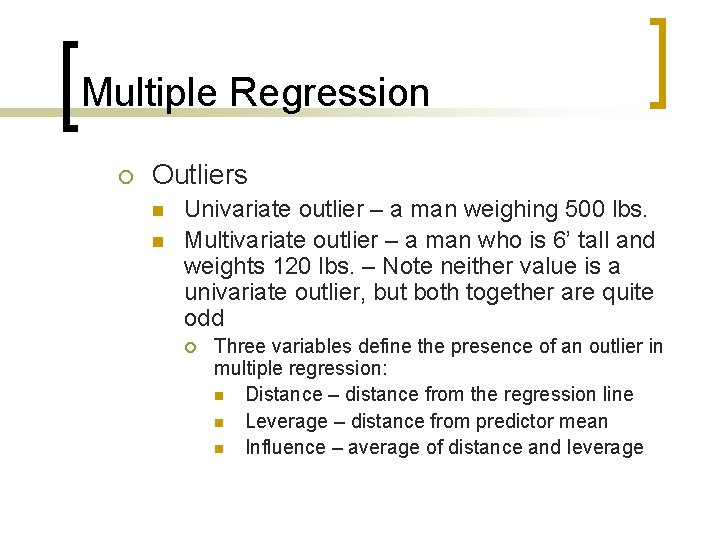
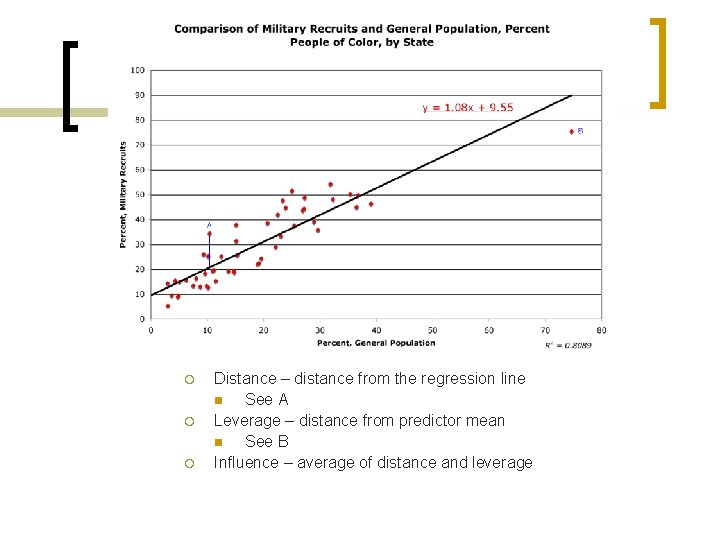
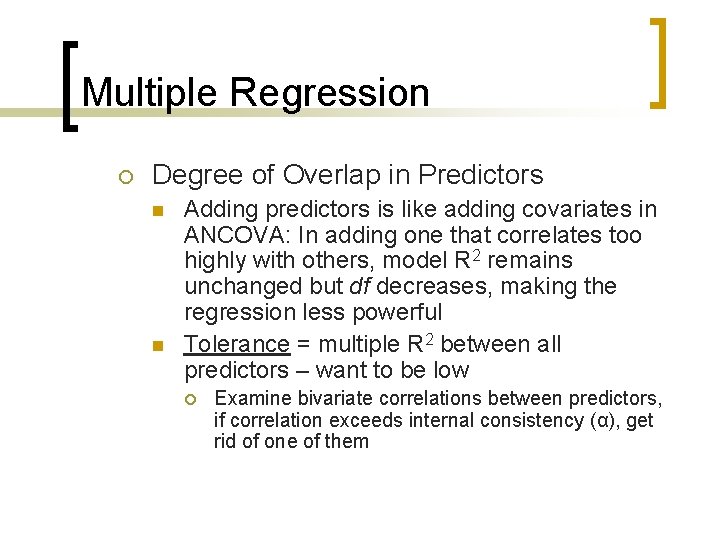
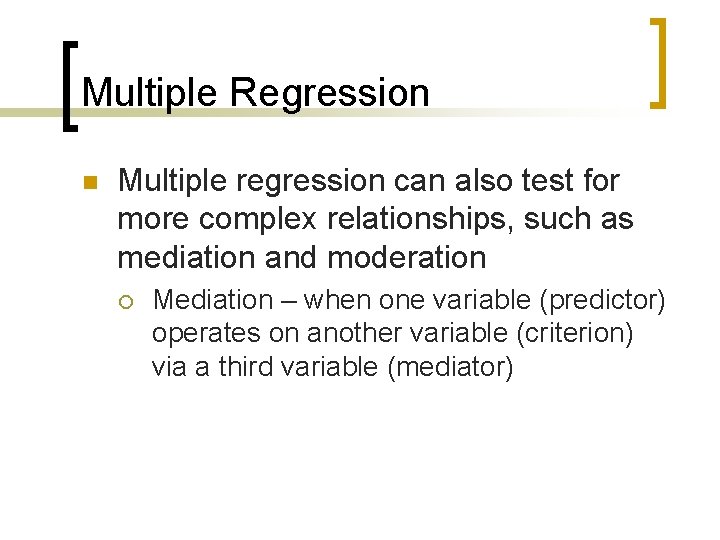
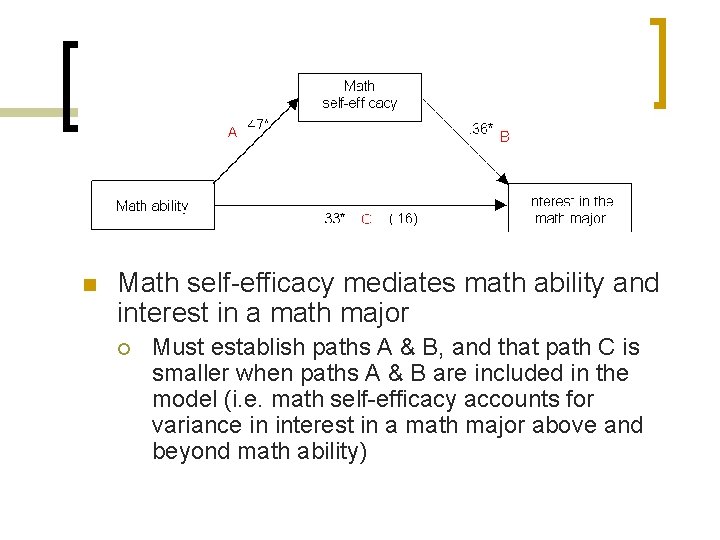
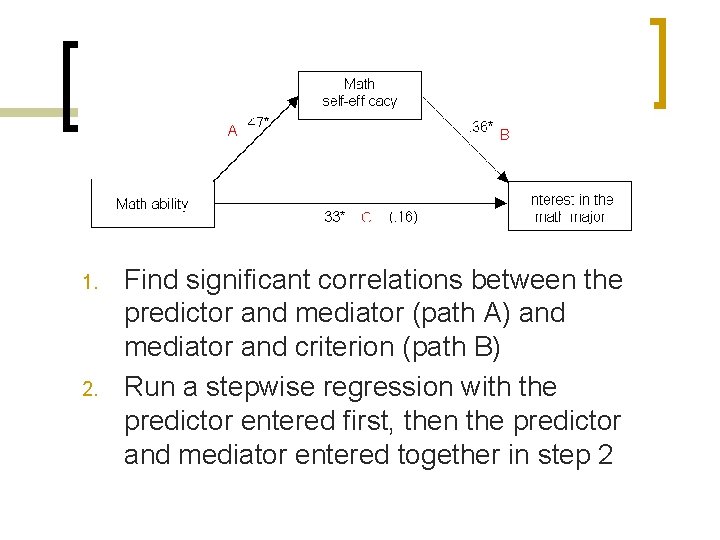
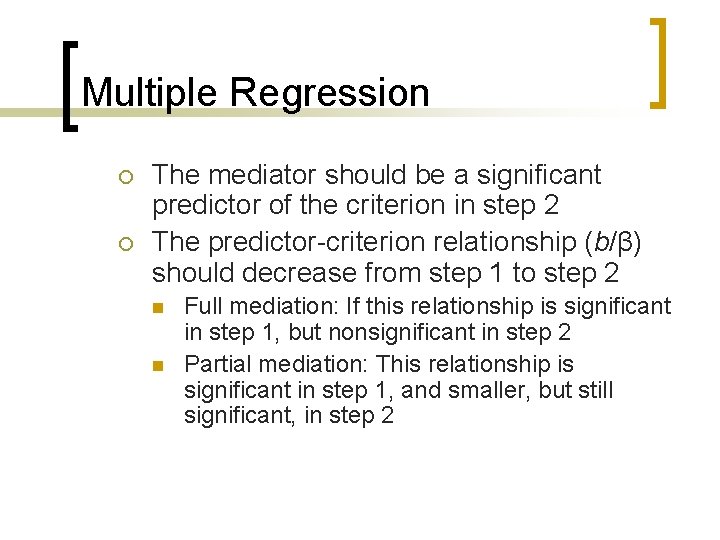
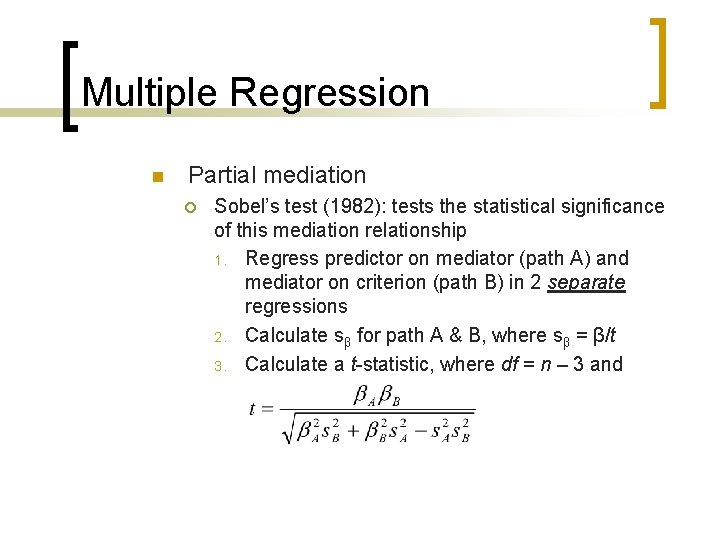
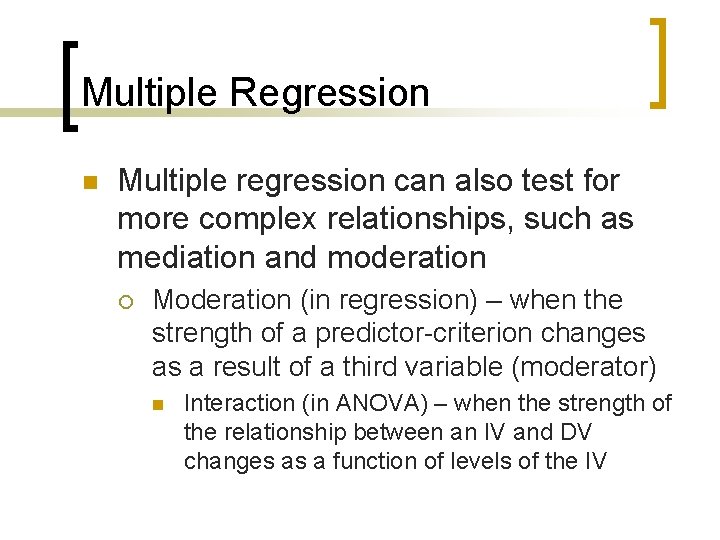
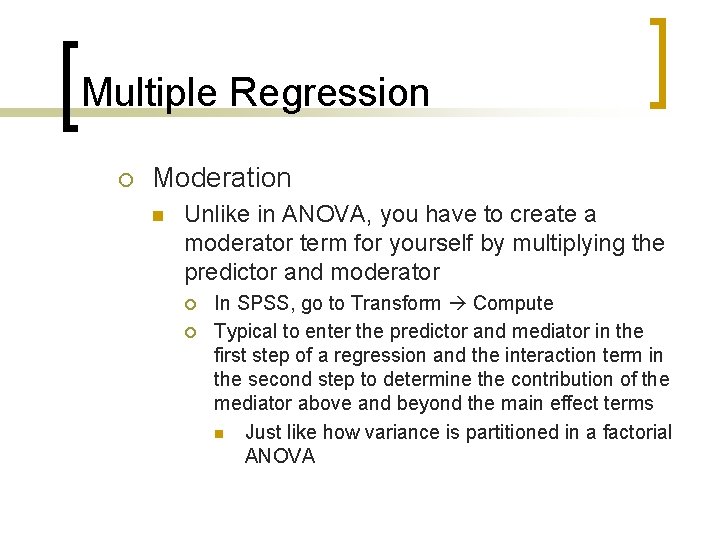
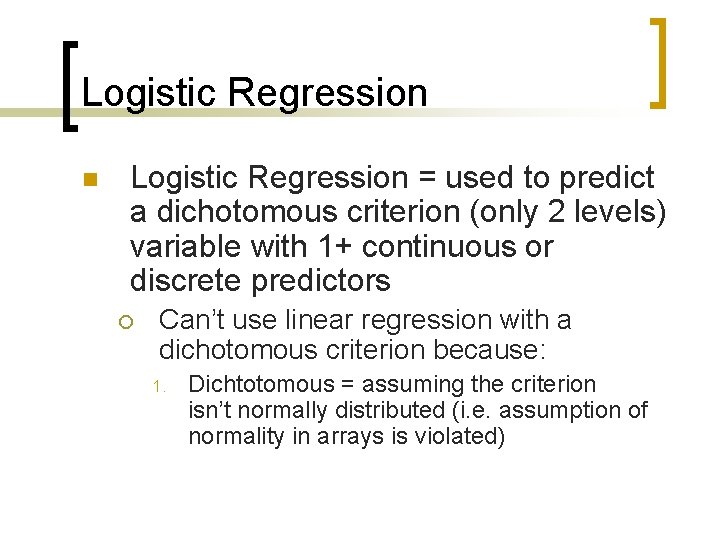
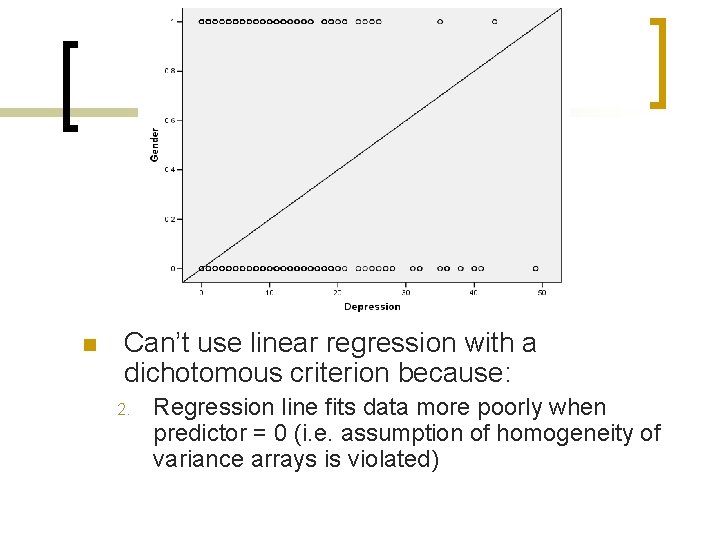
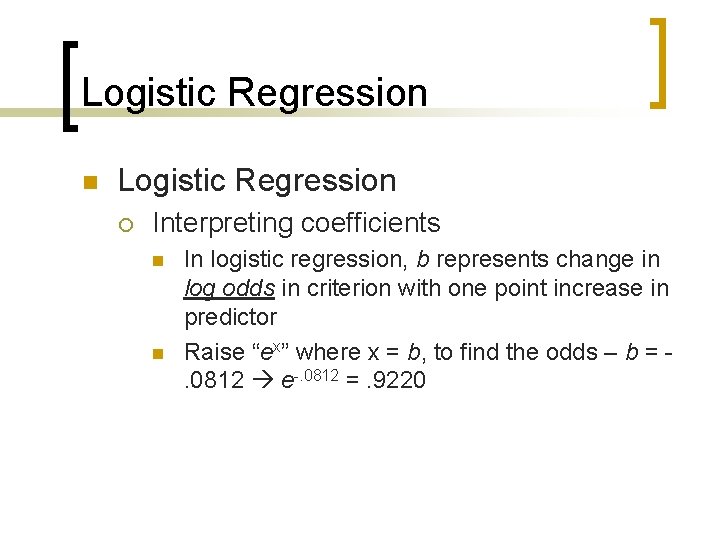
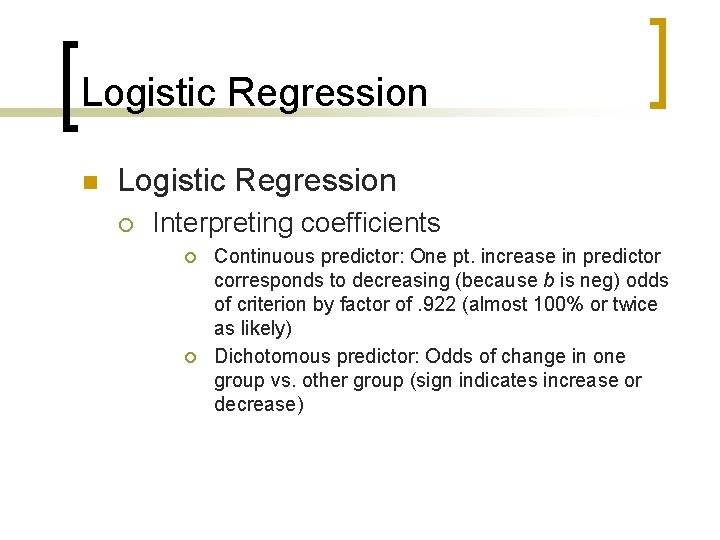
- Slides: 39
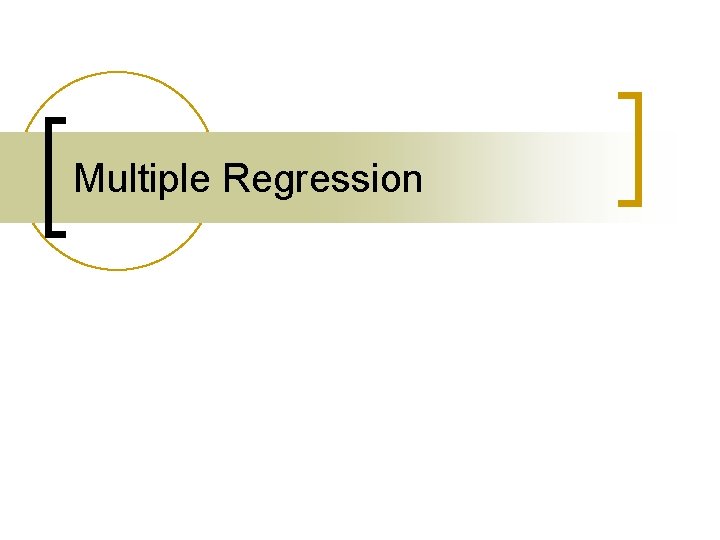
Multiple Regression
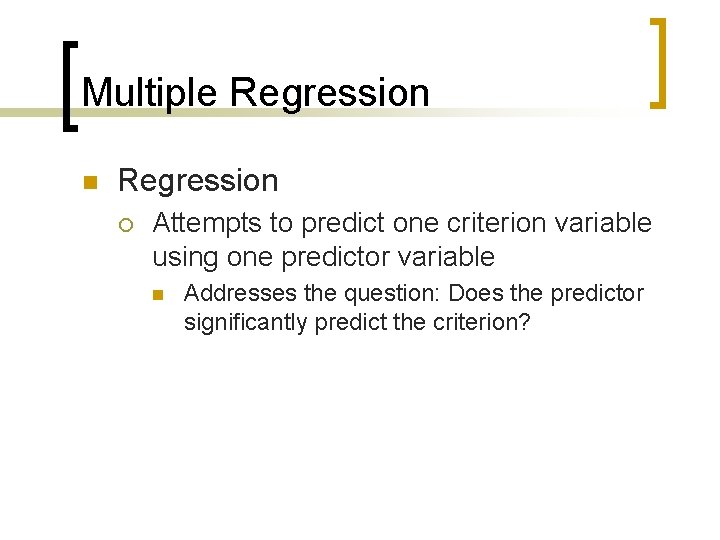
Multiple Regression n Regression ¡ Attempts to predict one criterion variable using one predictor variable n Addresses the question: Does the predictor significantly predict the criterion?
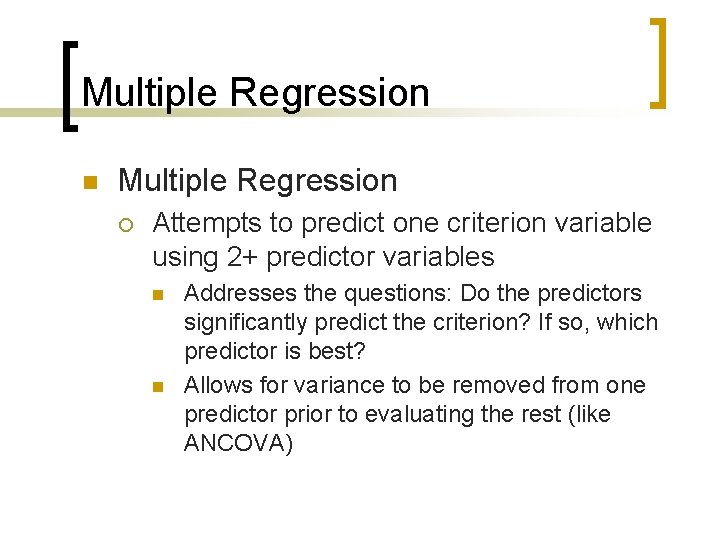
Multiple Regression n Multiple Regression ¡ Attempts to predict one criterion variable using 2+ predictor variables n n Addresses the questions: Do the predictors significantly predict the criterion? If so, which predictor is best? Allows for variance to be removed from one predictor prior to evaluating the rest (like ANCOVA)
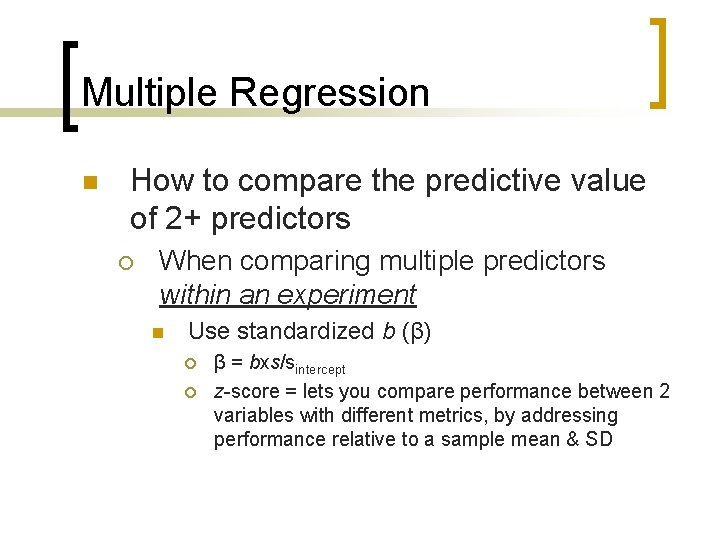
Multiple Regression n How to compare the predictive value of 2+ predictors ¡ When comparing multiple predictors within an experiment n Use standardized b (β) ¡ ¡ β = bxs/sintercept z-score = lets you compare performance between 2 variables with different metrics, by addressing performance relative to a sample mean & SD
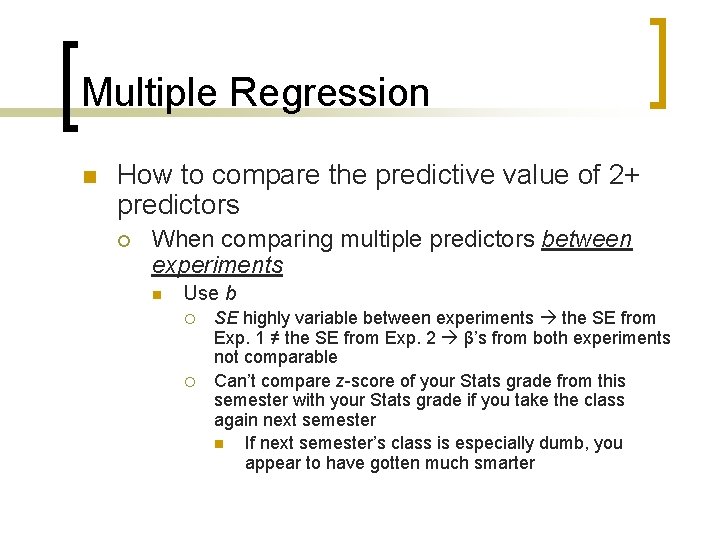
Multiple Regression n How to compare the predictive value of 2+ predictors ¡ When comparing multiple predictors between experiments n Use b ¡ ¡ SE highly variable between experiments the SE from Exp. 1 ≠ the SE from Exp. 2 β’s from both experiments not comparable Can’t compare z-score of your Stats grade from this semester with your Stats grade if you take the class again next semester n If next semester’s class is especially dumb, you appear to have gotten much smarter
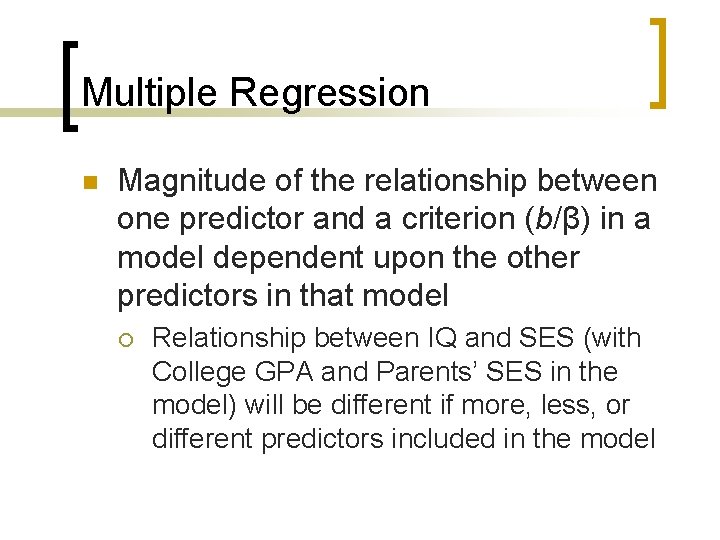
Multiple Regression n Magnitude of the relationship between one predictor and a criterion (b/β) in a model dependent upon the other predictors in that model ¡ Relationship between IQ and SES (with College GPA and Parents’ SES in the model) will be different if more, less, or different predictors included in the model
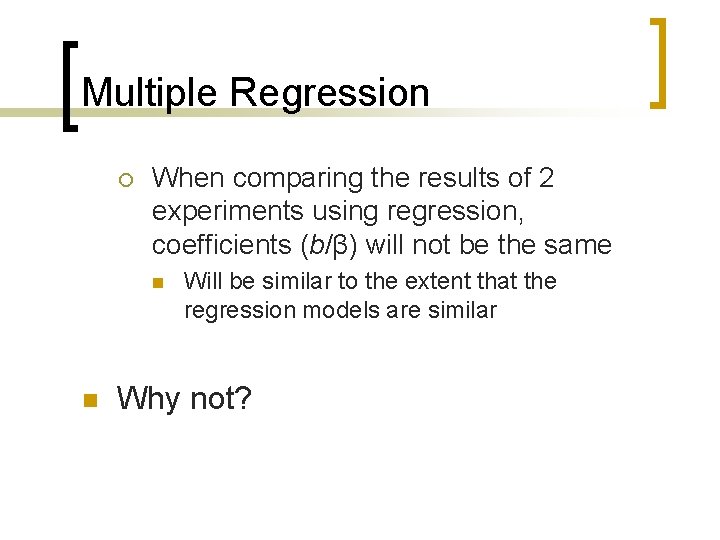
Multiple Regression ¡ When comparing the results of 2 experiments using regression, coefficients (b/β) will not be the same n n Will be similar to the extent that the regression models are similar Why not?
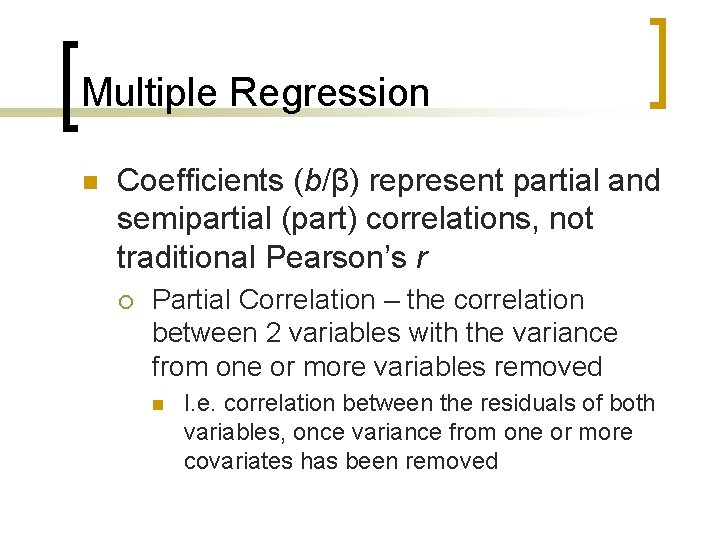
Multiple Regression n Coefficients (b/β) represent partial and semipartial (part) correlations, not traditional Pearson’s r ¡ Partial Correlation – the correlation between 2 variables with the variance from one or more variables removed n I. e. correlation between the residuals of both variables, once variance from one or more covariates has been removed
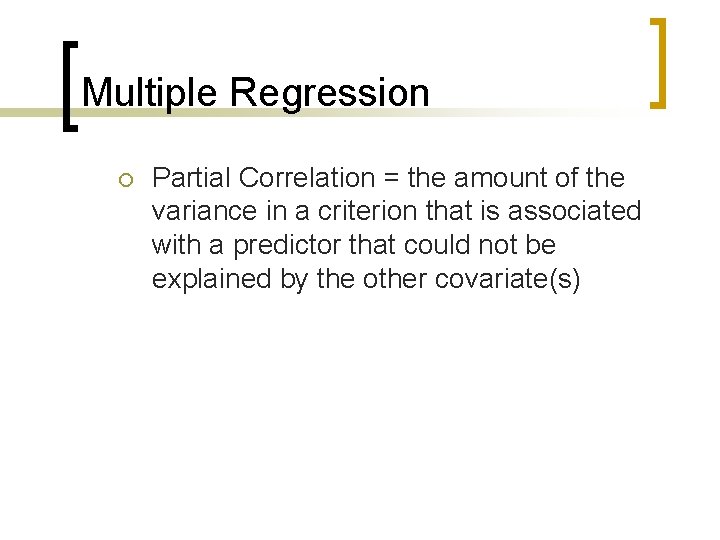
Multiple Regression ¡ Partial Correlation = the amount of the variance in a criterion that is associated with a predictor that could not be explained by the other covariate(s)
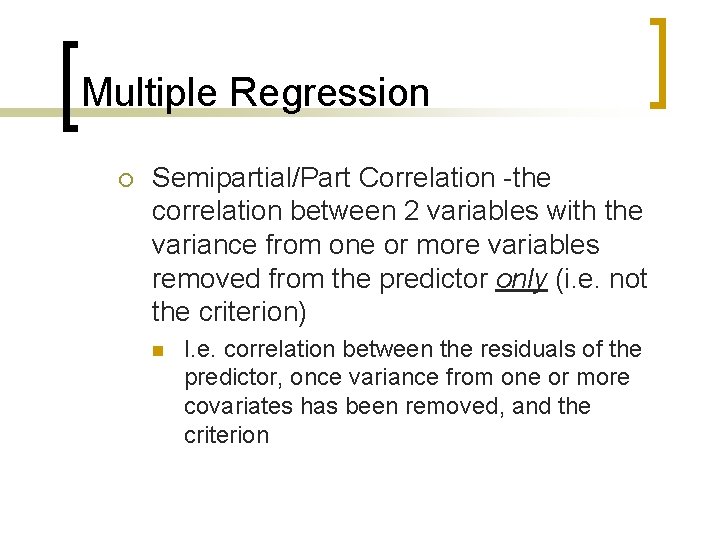
Multiple Regression ¡ Semipartial/Part Correlation -the correlation between 2 variables with the variance from one or more variables removed from the predictor only (i. e. not the criterion) n I. e. correlation between the residuals of the predictor, once variance from one or more covariates has been removed, and the criterion
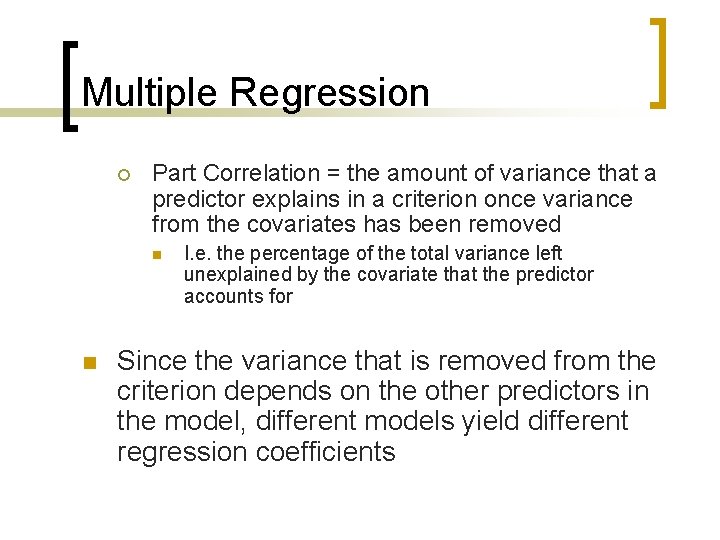
Multiple Regression ¡ Part Correlation = the amount of variance that a predictor explains in a criterion once variance from the covariates has been removed n n I. e. the percentage of the total variance left unexplained by the covariate that the predictor accounts for Since the variance that is removed from the criterion depends on the other predictors in the model, different models yield different regression coefficients
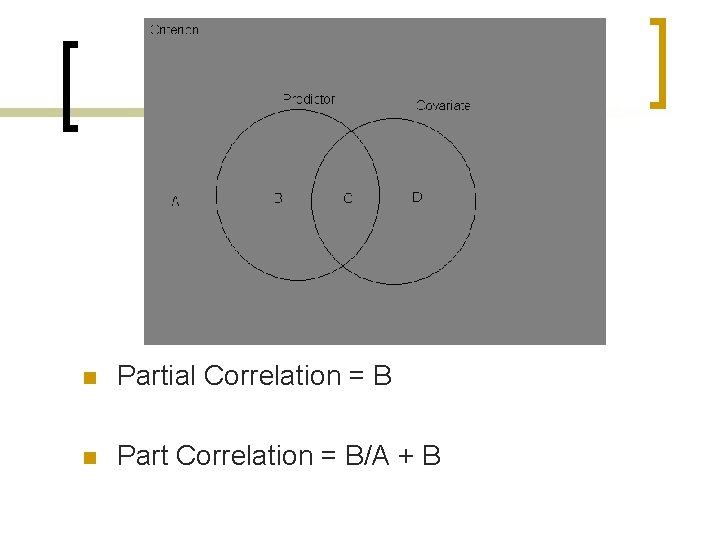
n Partial Correlation = B n Part Correlation = B/A + B
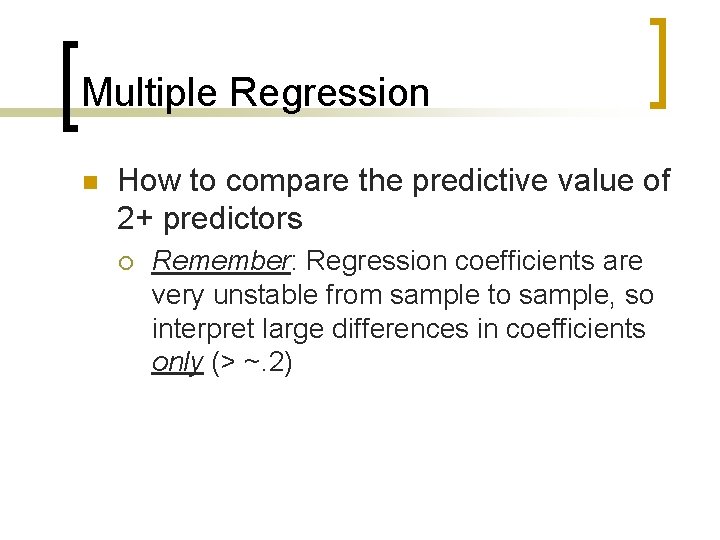
Multiple Regression n How to compare the predictive value of 2+ predictors ¡ Remember: Regression coefficients are very unstable from sample to sample, so interpret large differences in coefficients only (> ~. 2)
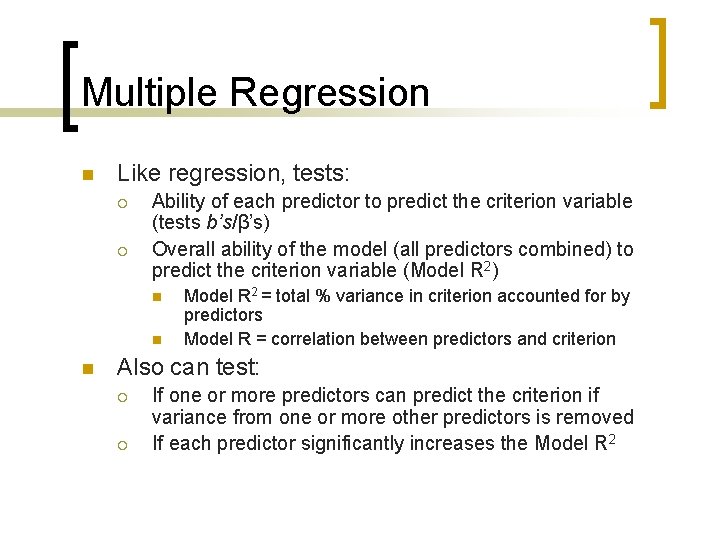
Multiple Regression n Like regression, tests: ¡ ¡ Ability of each predictor to predict the criterion variable (tests b’s/β’s) Overall ability of the model (all predictors combined) to predict the criterion variable (Model R 2) n n n Model R 2 = total % variance in criterion accounted for by predictors Model R = correlation between predictors and criterion Also can test: ¡ ¡ If one or more predictors can predict the criterion if variance from one or more other predictors is removed If each predictor significantly increases the Model R 2
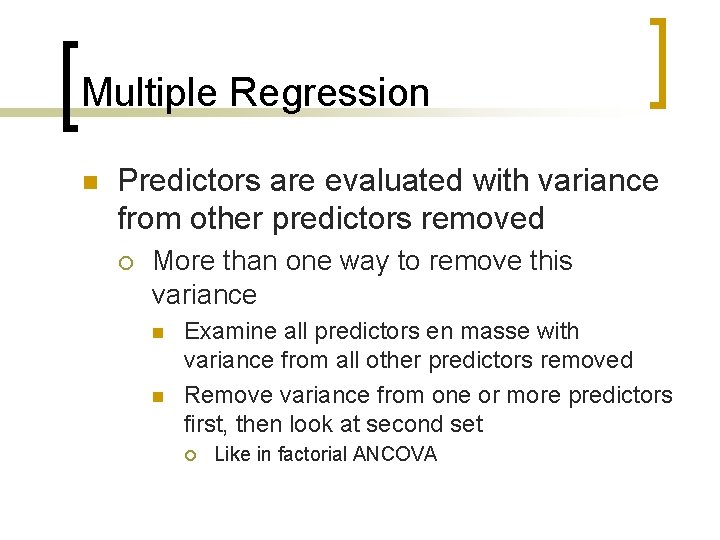
Multiple Regression n Predictors are evaluated with variance from other predictors removed ¡ More than one way to remove this variance n n Examine all predictors en masse with variance from all other predictors removed Remove variance from one or more predictors first, then look at second set ¡ Like in factorial ANCOVA
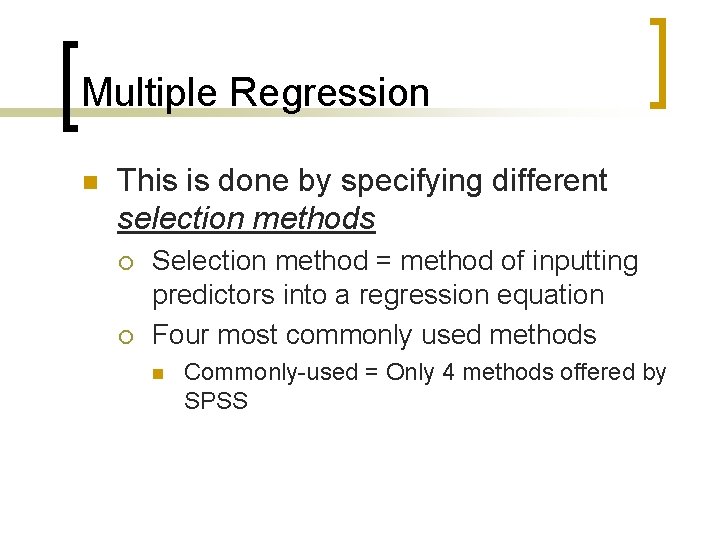
Multiple Regression n This is done by specifying different selection methods ¡ ¡ Selection method = method of inputting predictors into a regression equation Four most commonly used methods n Commonly-used = Only 4 methods offered by SPSS
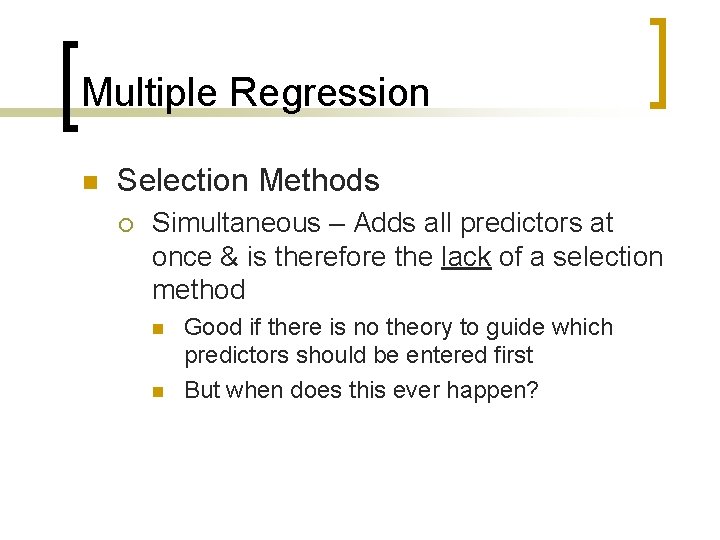
Multiple Regression n Selection Methods ¡ Simultaneous – Adds all predictors at once & is therefore the lack of a selection method n n Good if there is no theory to guide which predictors should be entered first But when does this ever happen?
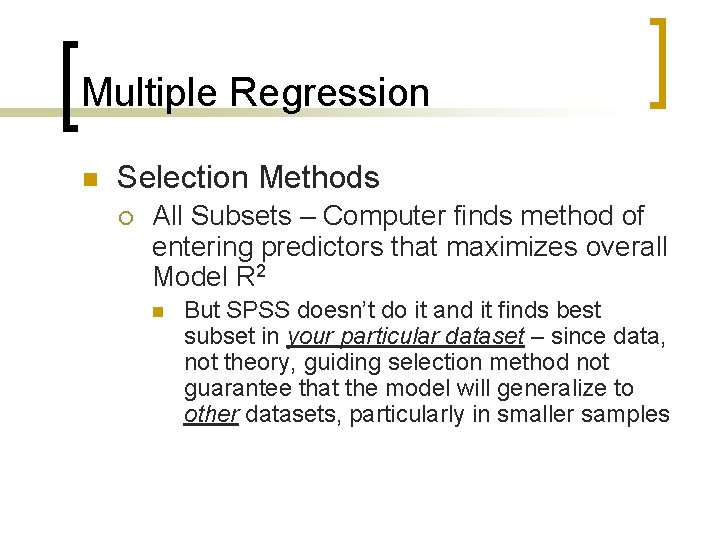
Multiple Regression n Selection Methods ¡ All Subsets – Computer finds method of entering predictors that maximizes overall Model R 2 n But SPSS doesn’t do it and it finds best subset in your particular dataset – since data, not theory, guiding selection method not guarantee that the model will generalize to other datasets, particularly in smaller samples
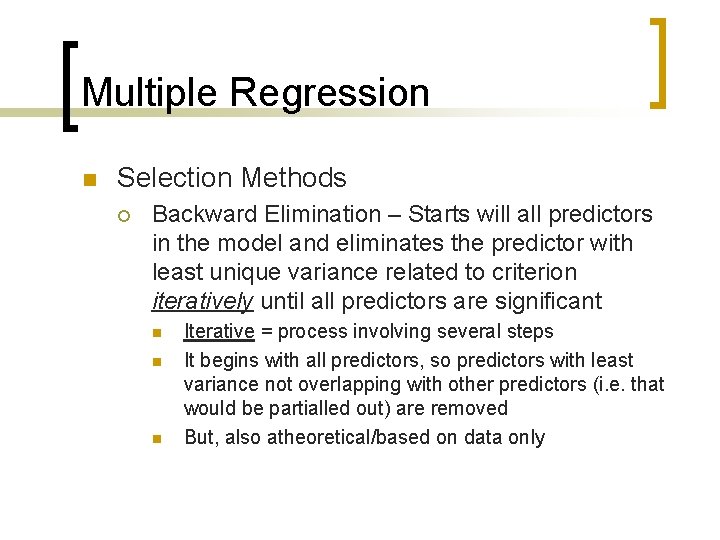
Multiple Regression n Selection Methods ¡ Backward Elimination – Starts will all predictors in the model and eliminates the predictor with least unique variance related to criterion iteratively until all predictors are significant n n n Iterative = process involving several steps It begins with all predictors, so predictors with least variance not overlapping with other predictors (i. e. that would be partialled out) are removed But, also atheoretical/based on data only
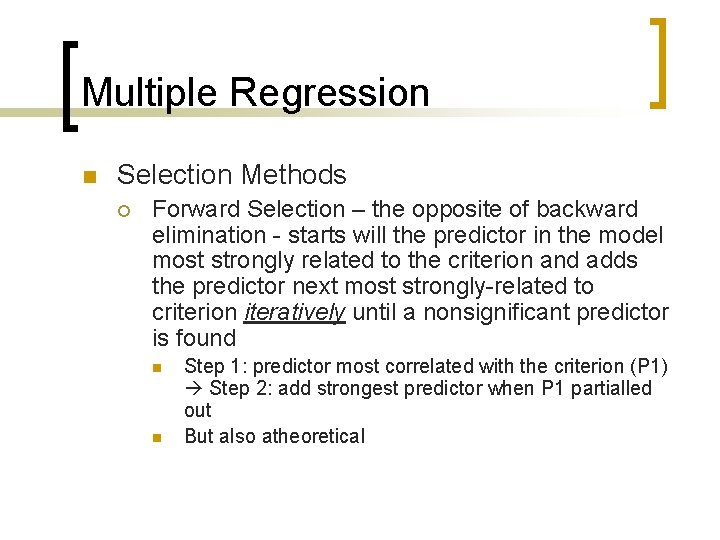
Multiple Regression n Selection Methods ¡ Forward Selection – the opposite of backward elimination - starts will the predictor in the model most strongly related to the criterion and adds the predictor next most strongly-related to criterion iteratively until a nonsignificant predictor is found n n Step 1: predictor most correlated with the criterion (P 1) Step 2: add strongest predictor when P 1 partialled out But also atheoretical
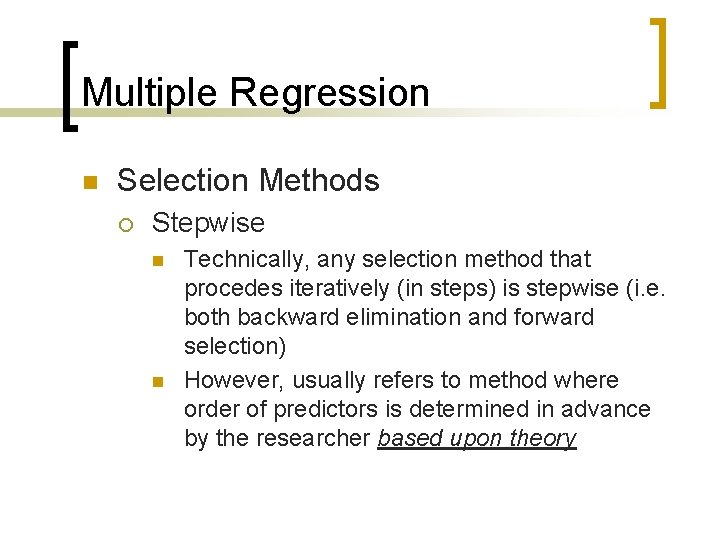
Multiple Regression n Selection Methods ¡ Stepwise n n Technically, any selection method that procedes iteratively (in steps) is stepwise (i. e. both backward elimination and forward selection) However, usually refers to method where order of predictors is determined in advance by the researcher based upon theory
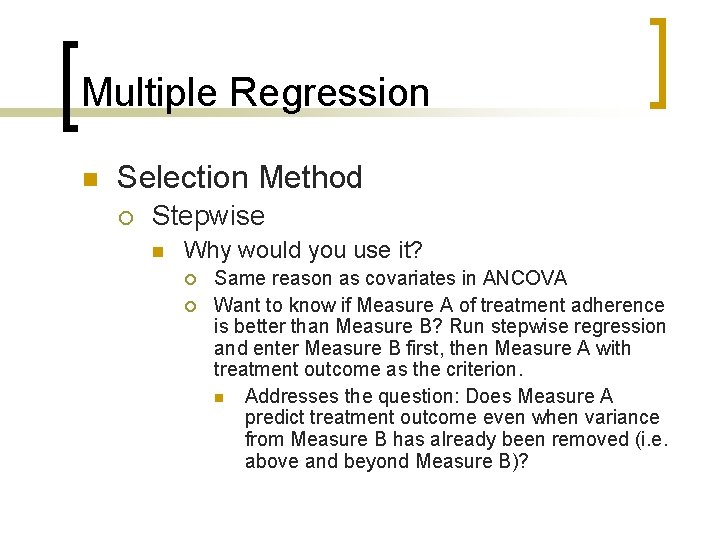
Multiple Regression n Selection Method ¡ Stepwise n Why would you use it? ¡ ¡ Same reason as covariates in ANCOVA Want to know if Measure A of treatment adherence is better than Measure B? Run stepwise regression and enter Measure B first, then Measure A with treatment outcome as the criterion. n Addresses the question: Does Measure A predict treatment outcome even when variance from Measure B has already been removed (i. e. above and beyond Measure B)?
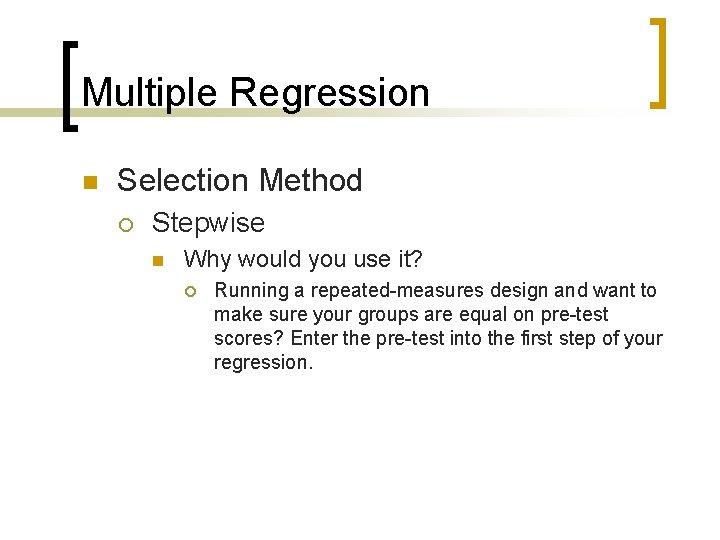
Multiple Regression n Selection Method ¡ Stepwise n Why would you use it? ¡ Running a repeated-measures design and want to make sure your groups are equal on pre-test scores? Enter the pre-test into the first step of your regression.
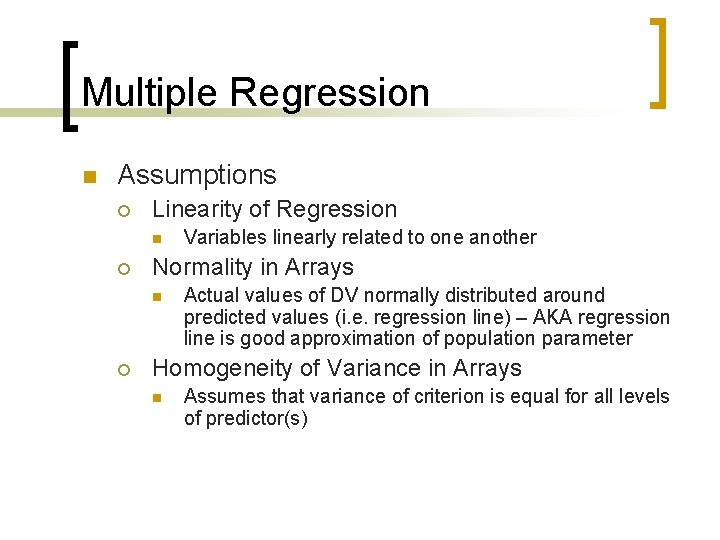
Multiple Regression n Assumptions ¡ Linearity of Regression n ¡ Normality in Arrays n ¡ Variables linearly related to one another Actual values of DV normally distributed around predicted values (i. e. regression line) – AKA regression line is good approximation of population parameter Homogeneity of Variance in Arrays n Assumes that variance of criterion is equal for all levels of predictor(s)
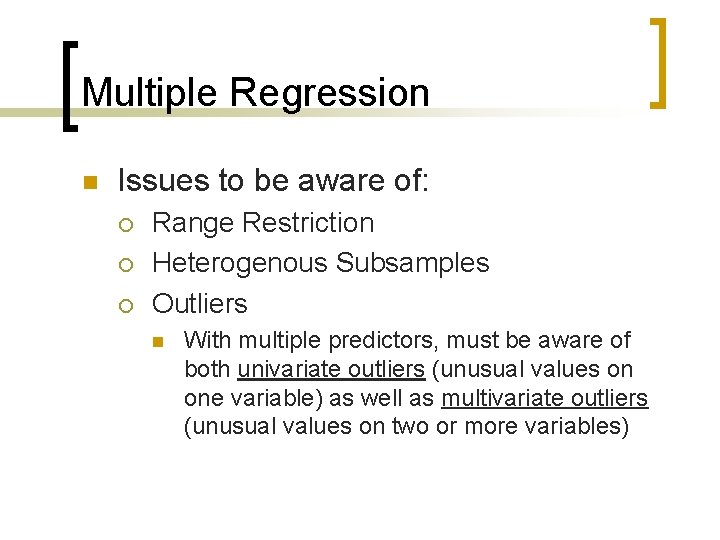
Multiple Regression n Issues to be aware of: ¡ ¡ ¡ Range Restriction Heterogenous Subsamples Outliers n With multiple predictors, must be aware of both univariate outliers (unusual values on one variable) as well as multivariate outliers (unusual values on two or more variables)
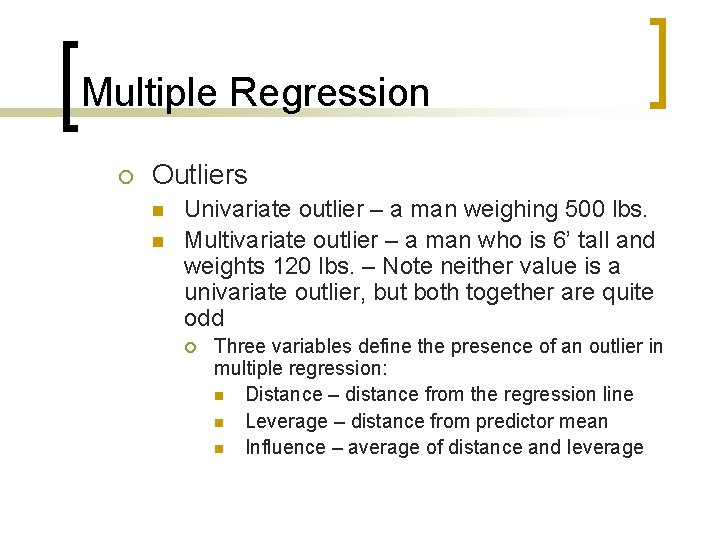
Multiple Regression ¡ Outliers n n Univariate outlier – a man weighing 500 lbs. Multivariate outlier – a man who is 6’ tall and weights 120 lbs. – Note neither value is a univariate outlier, but both together are quite odd ¡ Three variables define the presence of an outlier in multiple regression: n Distance – distance from the regression line n Leverage – distance from predictor mean n Influence – average of distance and leverage
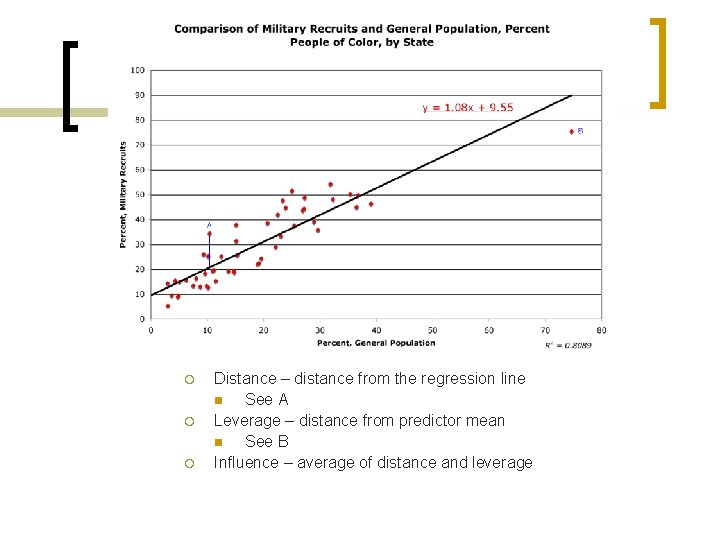
¡ ¡ ¡ Distance – distance from the regression line n See A Leverage – distance from predictor mean n See B Influence – average of distance and leverage
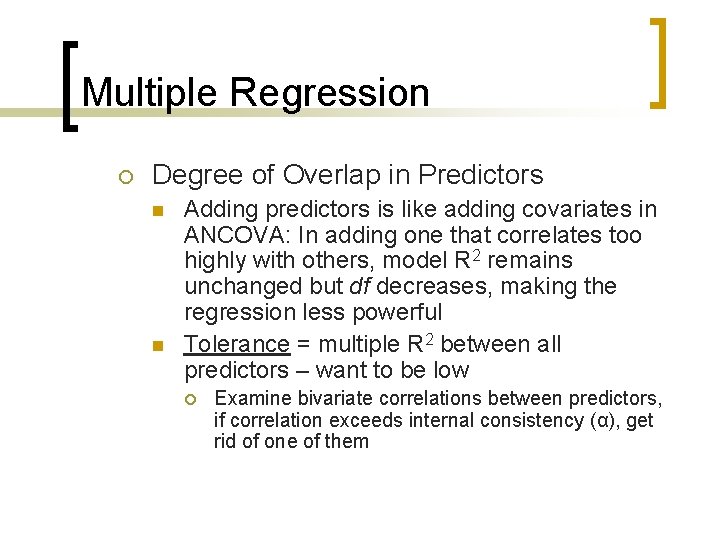
Multiple Regression ¡ Degree of Overlap in Predictors n n Adding predictors is like adding covariates in ANCOVA: In adding one that correlates too highly with others, model R 2 remains unchanged but df decreases, making the regression less powerful Tolerance = multiple R 2 between all predictors – want to be low ¡ Examine bivariate correlations between predictors, if correlation exceeds internal consistency (α), get rid of one of them
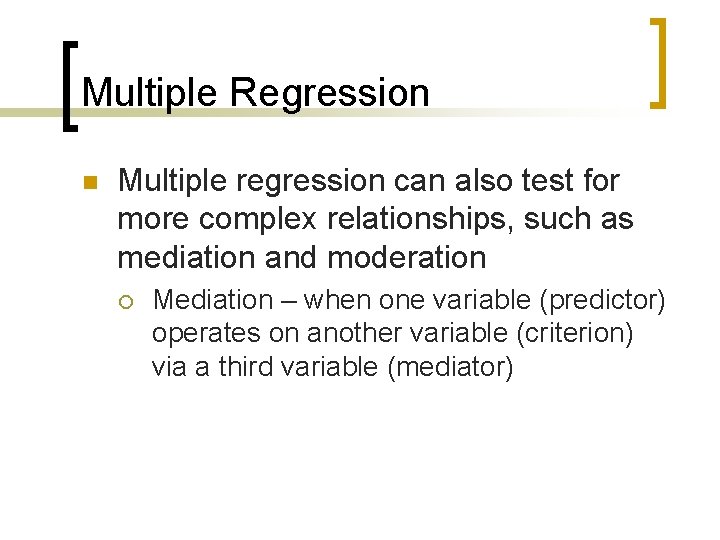
Multiple Regression n Multiple regression can also test for more complex relationships, such as mediation and moderation ¡ Mediation – when one variable (predictor) operates on another variable (criterion) via a third variable (mediator)
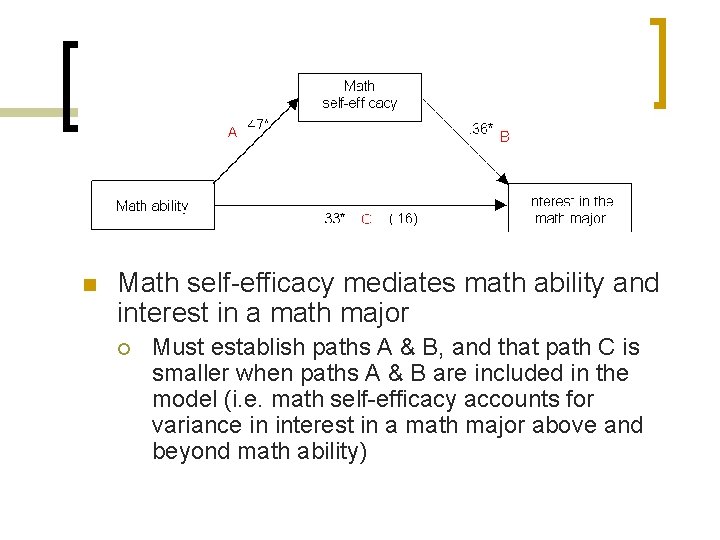
n Math self-efficacy mediates math ability and interest in a math major ¡ Must establish paths A & B, and that path C is smaller when paths A & B are included in the model (i. e. math self-efficacy accounts for variance in interest in a math major above and beyond math ability)
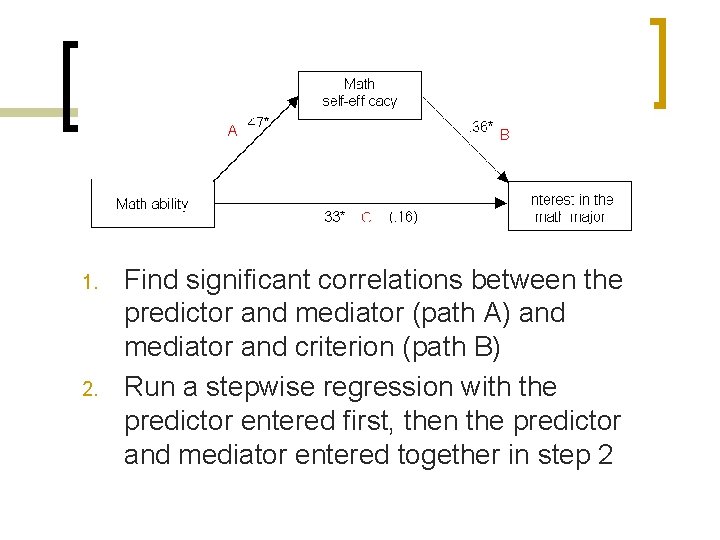
1. 2. Find significant correlations between the predictor and mediator (path A) and mediator and criterion (path B) Run a stepwise regression with the predictor entered first, then the predictor and mediator entered together in step 2
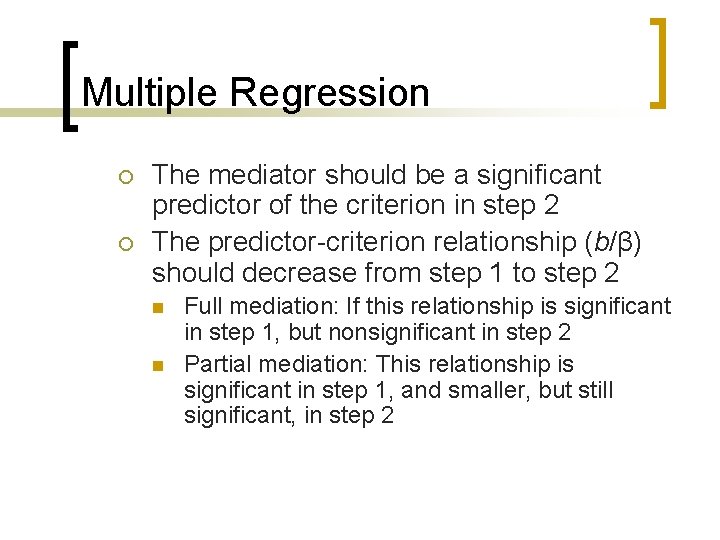
Multiple Regression ¡ ¡ The mediator should be a significant predictor of the criterion in step 2 The predictor-criterion relationship (b/β) should decrease from step 1 to step 2 n n Full mediation: If this relationship is significant in step 1, but nonsignificant in step 2 Partial mediation: This relationship is significant in step 1, and smaller, but still significant, in step 2
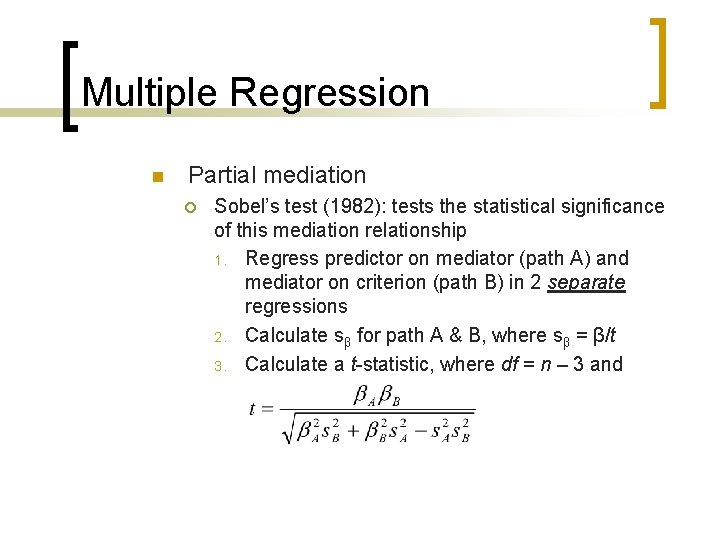
Multiple Regression n Partial mediation ¡ Sobel’s test (1982): tests the statistical significance of this mediation relationship 1. Regress predictor on mediator (path A) and mediator on criterion (path B) in 2 separate regressions 2. Calculate sβ for path A & B, where sβ = β/t 3. Calculate a t-statistic, where df = n – 3 and
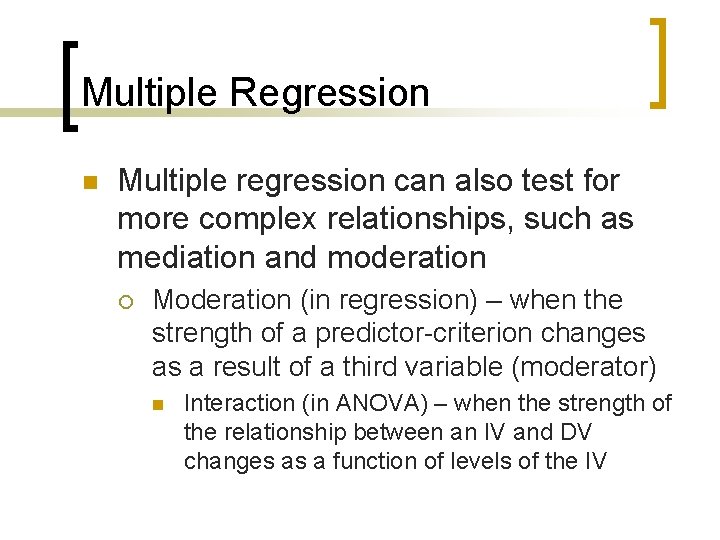
Multiple Regression n Multiple regression can also test for more complex relationships, such as mediation and moderation ¡ Moderation (in regression) – when the strength of a predictor-criterion changes as a result of a third variable (moderator) n Interaction (in ANOVA) – when the strength of the relationship between an IV and DV changes as a function of levels of the IV
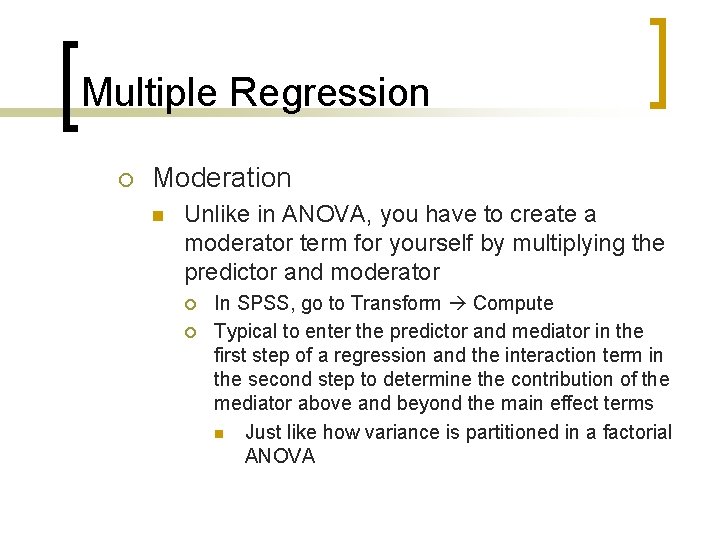
Multiple Regression ¡ Moderation n Unlike in ANOVA, you have to create a moderator term for yourself by multiplying the predictor and moderator ¡ ¡ In SPSS, go to Transform Compute Typical to enter the predictor and mediator in the first step of a regression and the interaction term in the second step to determine the contribution of the mediator above and beyond the main effect terms n Just like how variance is partitioned in a factorial ANOVA
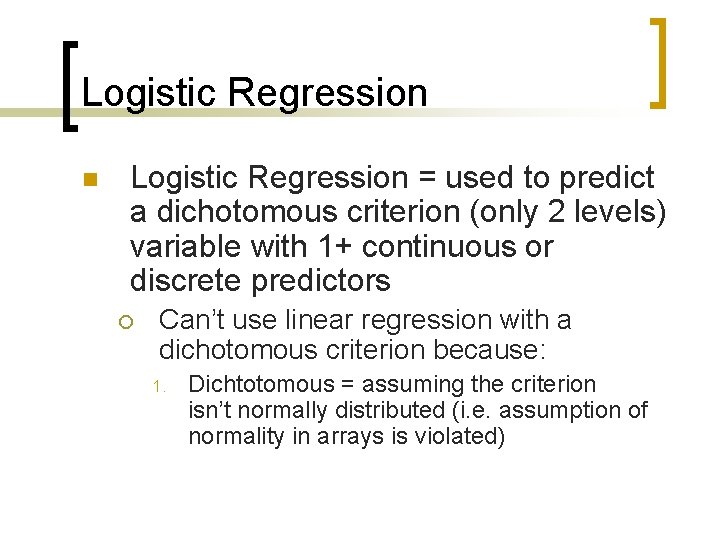
Logistic Regression n Logistic Regression = used to predict a dichotomous criterion (only 2 levels) variable with 1+ continuous or discrete predictors ¡ Can’t use linear regression with a dichotomous criterion because: 1. Dichtotomous = assuming the criterion isn’t normally distributed (i. e. assumption of normality in arrays is violated)
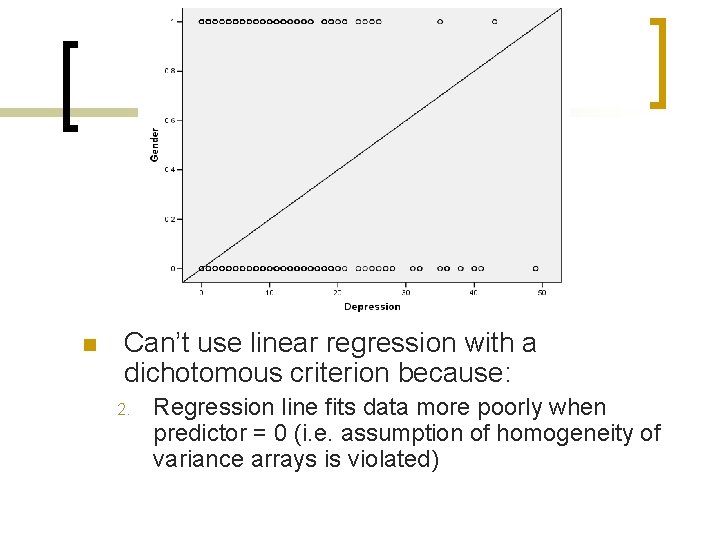
n Can’t use linear regression with a dichotomous criterion because: 2. Regression line fits data more poorly when predictor = 0 (i. e. assumption of homogeneity of variance arrays is violated)
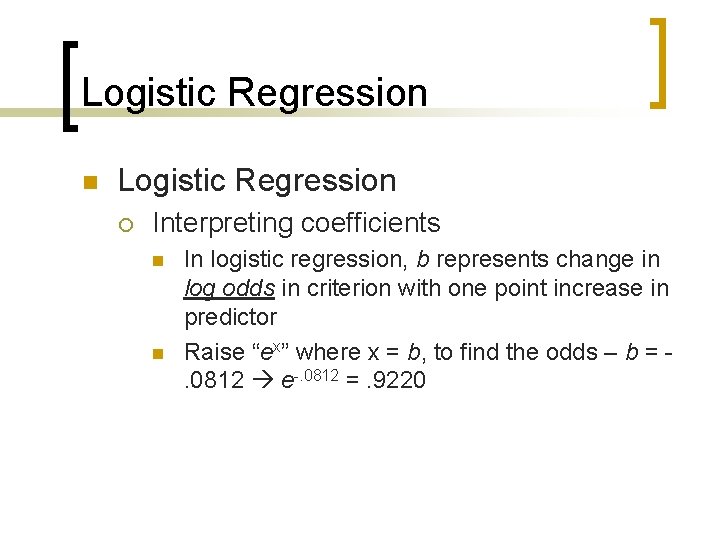
Logistic Regression n Logistic Regression ¡ Interpreting coefficients n n In logistic regression, b represents change in log odds in criterion with one point increase in predictor Raise “ex” where x = b, to find the odds – b =. 0812 e-. 0812 =. 9220
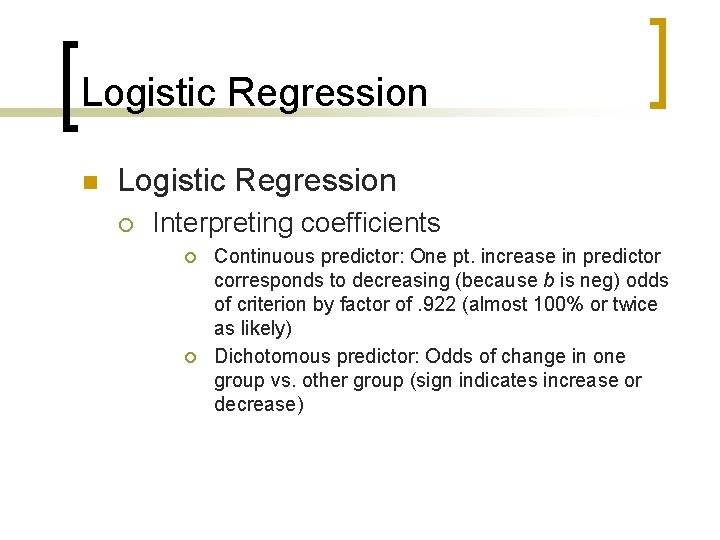
Logistic Regression n Logistic Regression ¡ Interpreting coefficients ¡ ¡ Continuous predictor: One pt. increase in predictor corresponds to decreasing (because b is neg) odds of criterion by factor of. 922 (almost 100% or twice as likely) Dichotomous predictor: Odds of change in one group vs. other group (sign indicates increase or decrease)
Linear regression vs multiple regression
Multiple linear regression
A window washer attempts to lean
Site:slidetodoc.com
Discuss the purpose of the writer to persuade
Under imperialism the stronger nation attempts to
Dylan's mother buys him a sailor's cap
The first english attempts to
Researchers have sneakily dabbed rouge
Self concept and lifestyle
The myth attempts to explain the right handed twin
Risk projection attempts to rate each risk in two ways
Survival analysis vs logistic regression
Logistic regression vs linear regression
Multiple linear regression
Multiple regression analysis inference
Regresi logistik berganda adalah
Dataset for regression analysis
Linear regression assumptions spss
Moderated multiple regression
Multiple regression analysis inference
Sum of squares
Extra sum of squares multiple regression
Linear regression with multiple variables machine learning
Draw a correlation
Mahalanobis distance spss
Multiple linear regression variance
2-5 scatter plots and lines of regression
Multiple regression analysis adalah
Stepwise multiple regression
Logistic regression andy field
Quantitative regression analysis
Multiple nonlinear regression spss
Multiple regression analysis with qualitative information
Multiple regression equation
Hypothesis for multiple regression
Hierarchical multiple regression spss
R-squared interpretation example
Polynomial regression spss
Multiple linear regression interpretation