GEOGG 141 GEOG 3051 Principles Practice of Remote
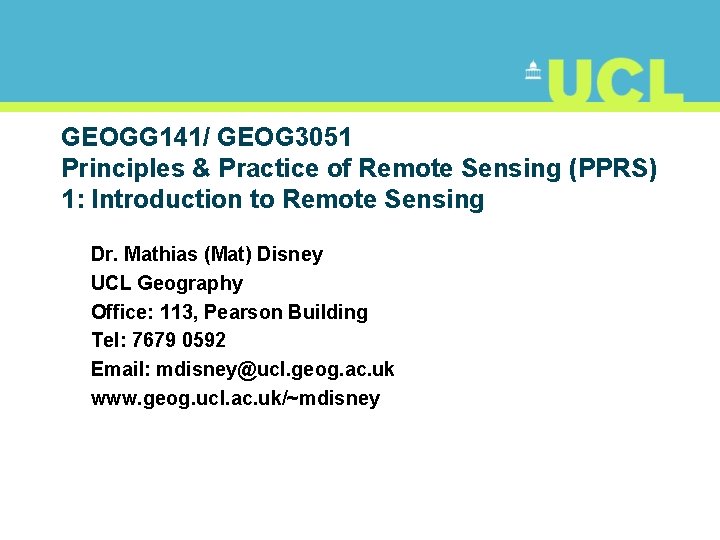
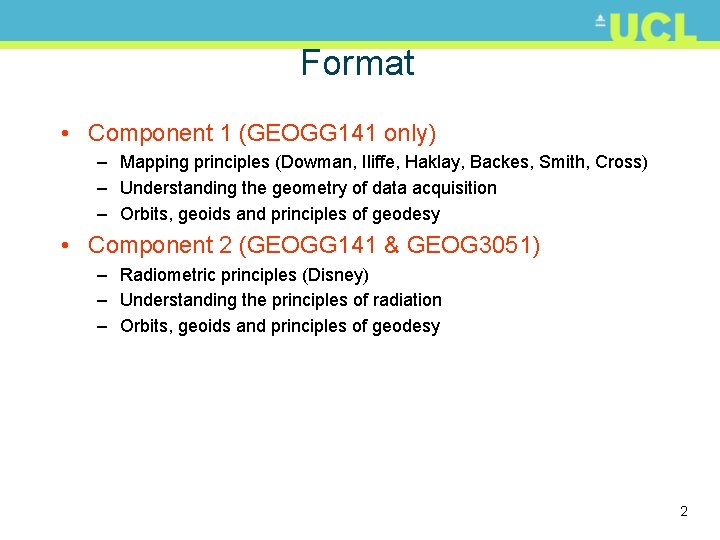
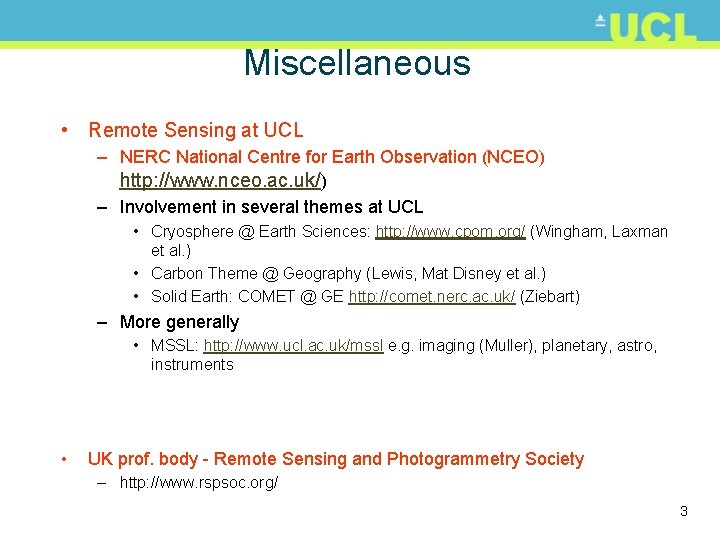
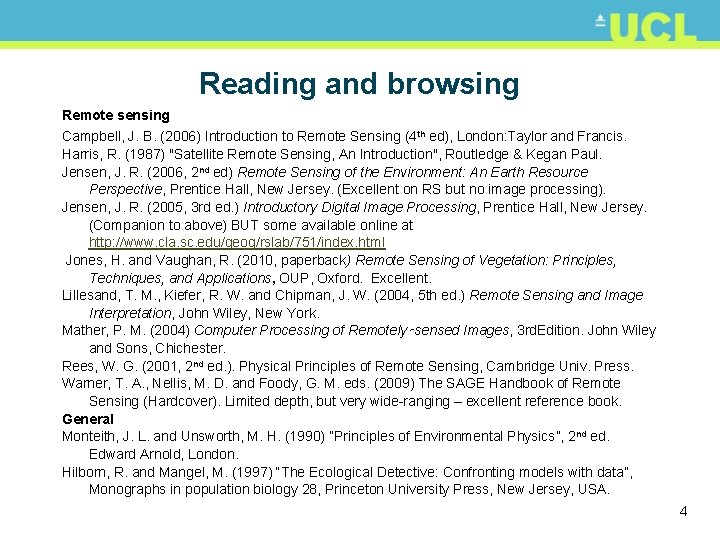
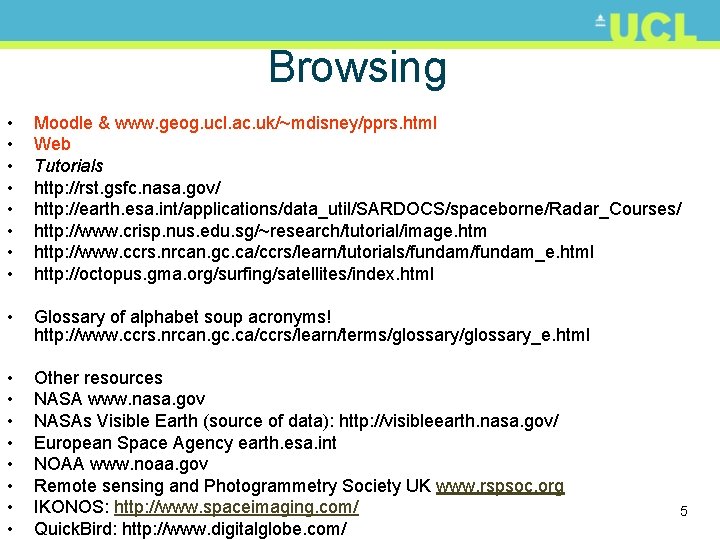
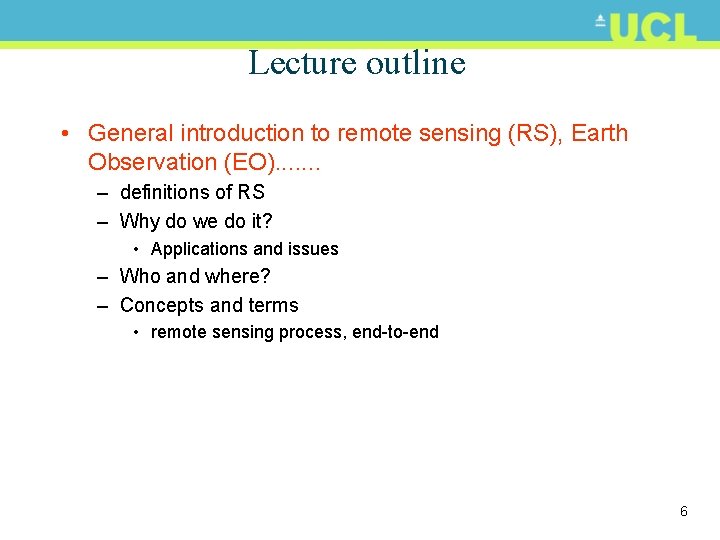
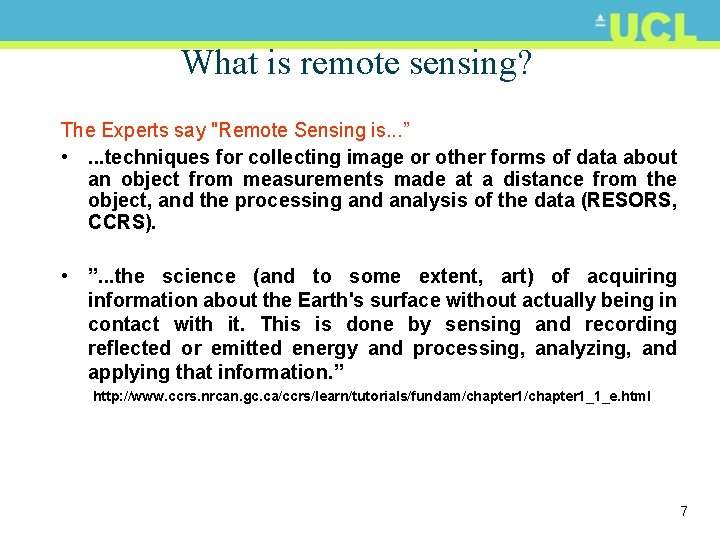
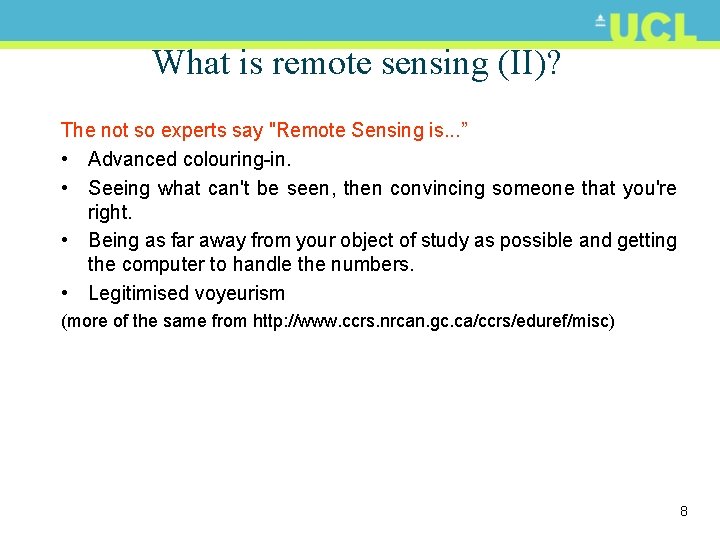
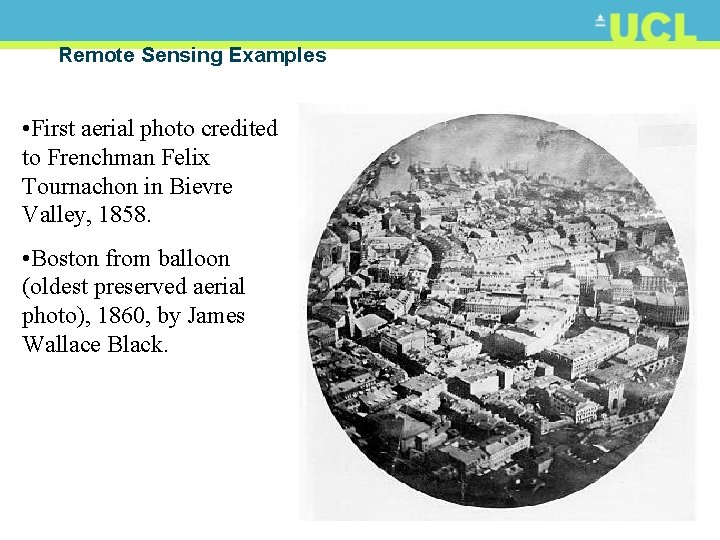
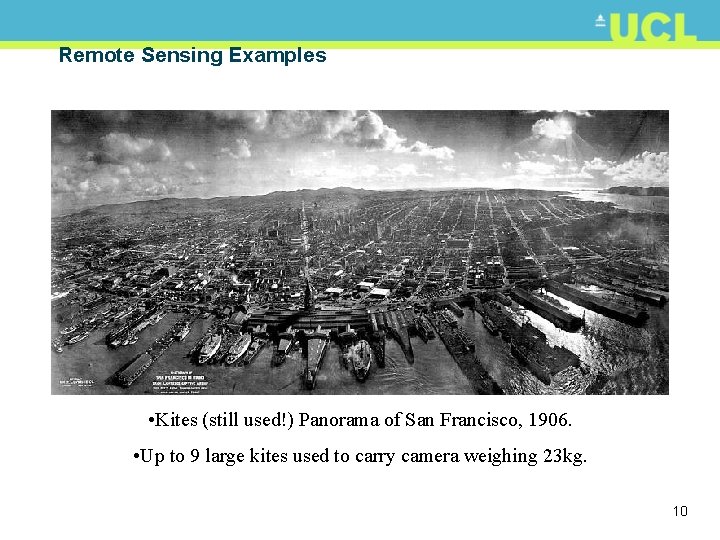
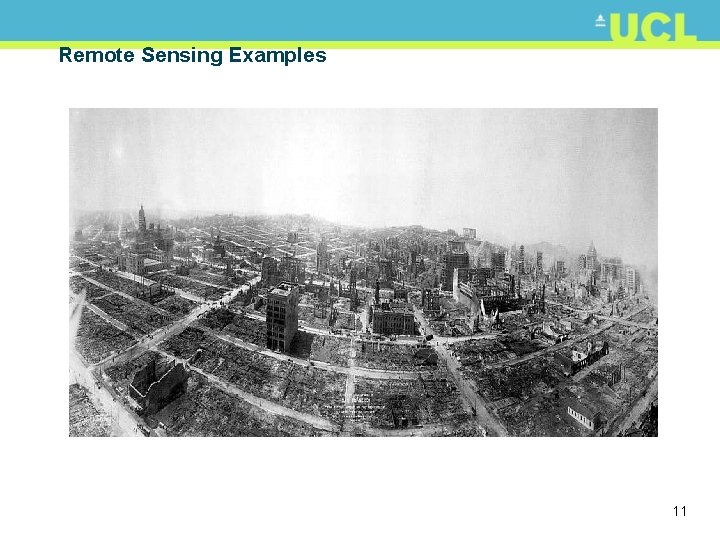
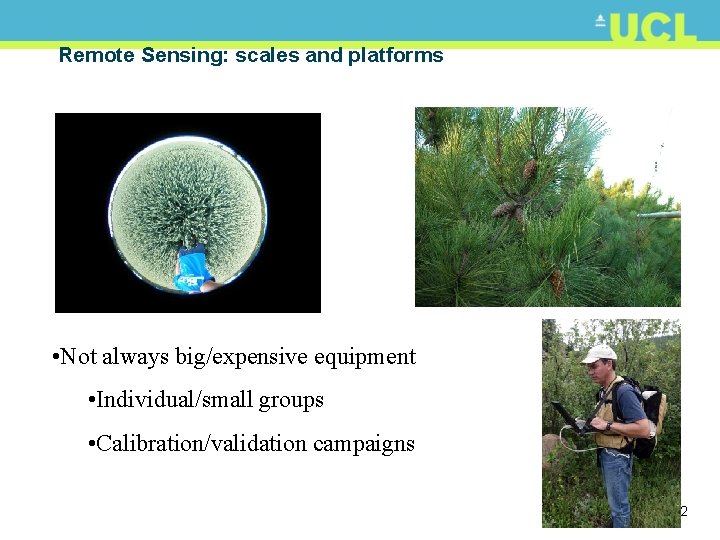
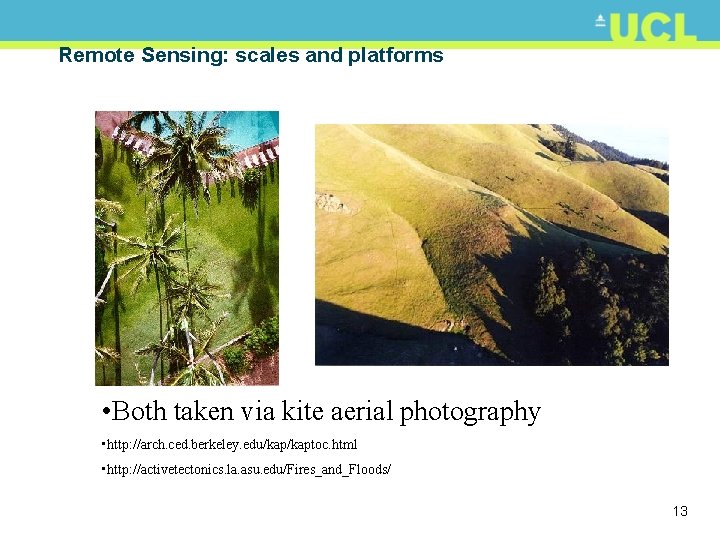
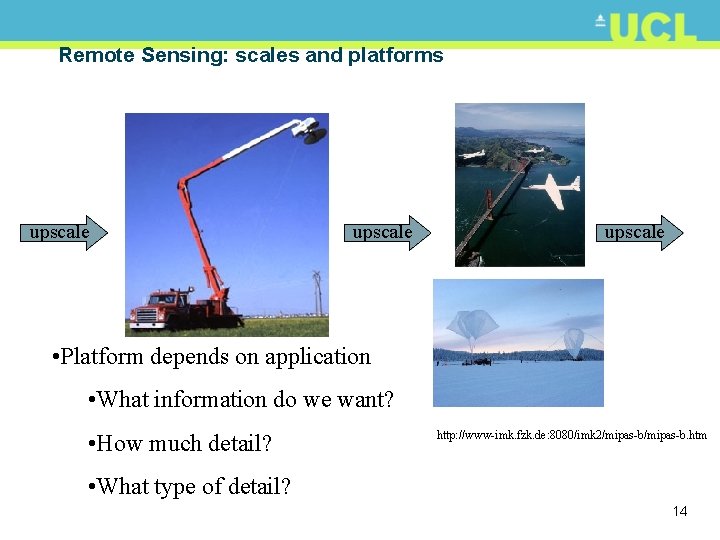
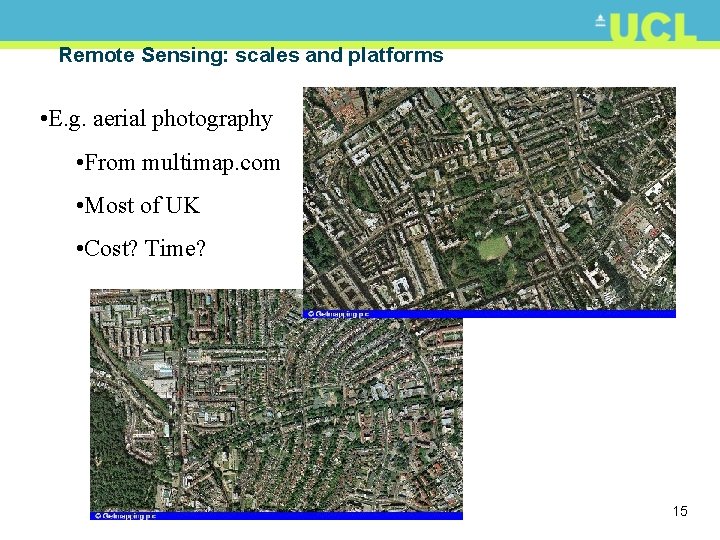
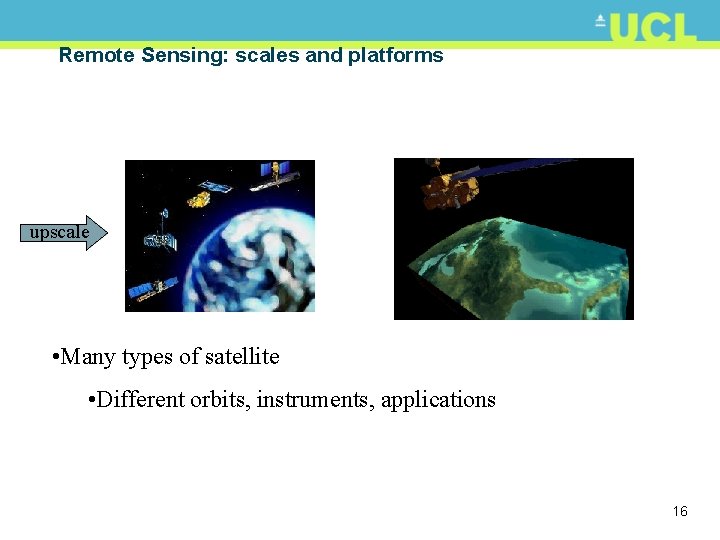
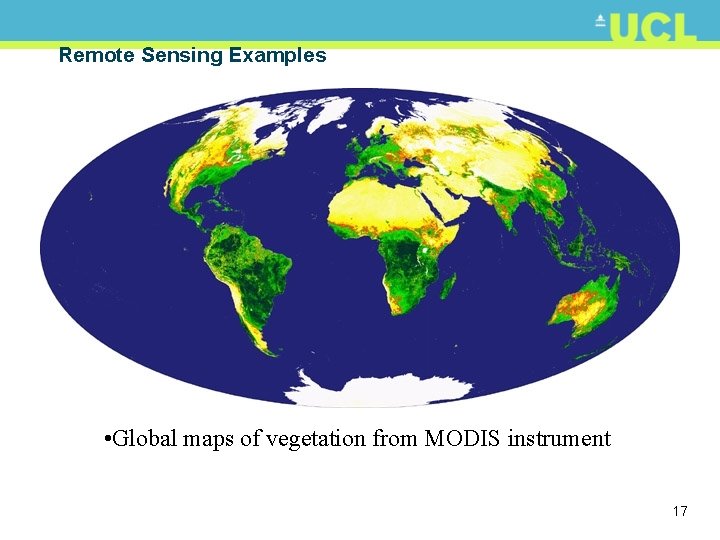
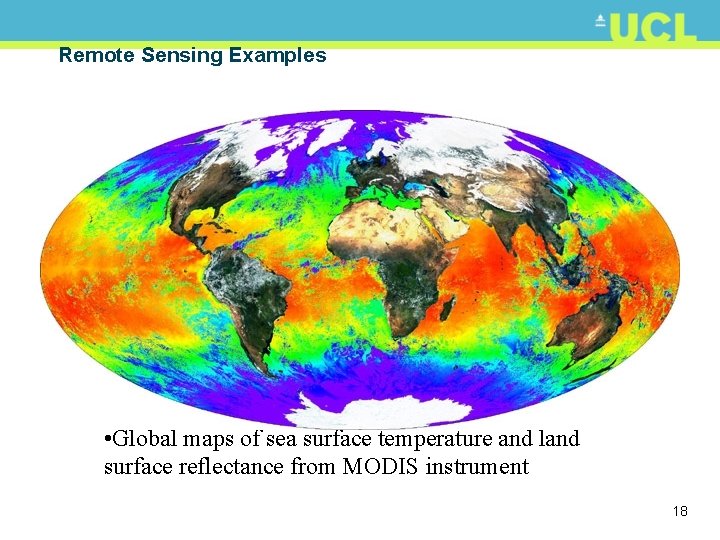
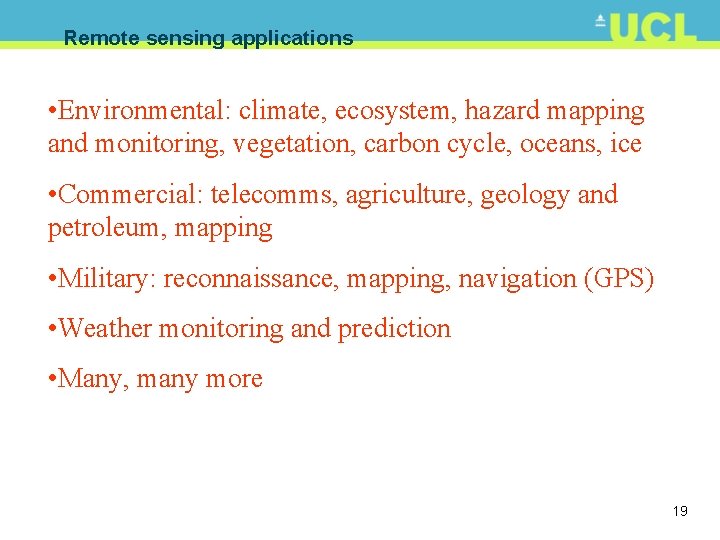
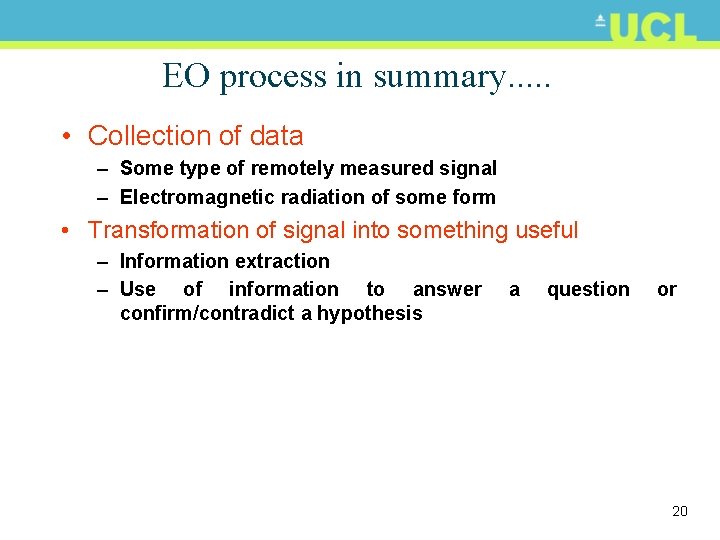
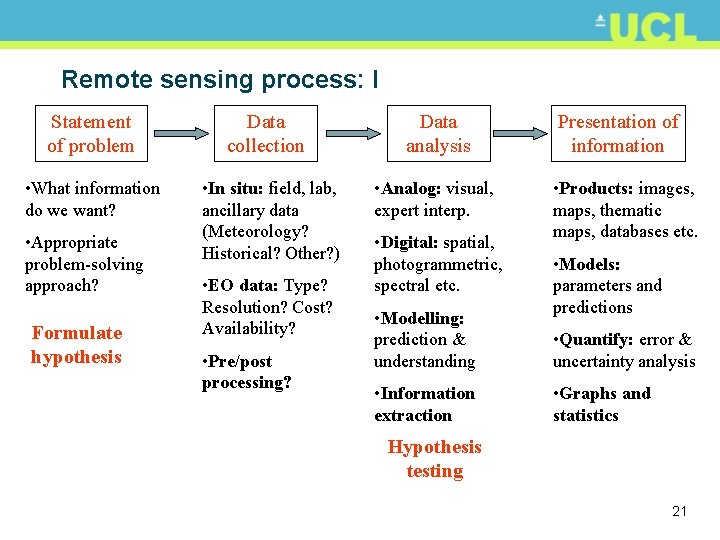
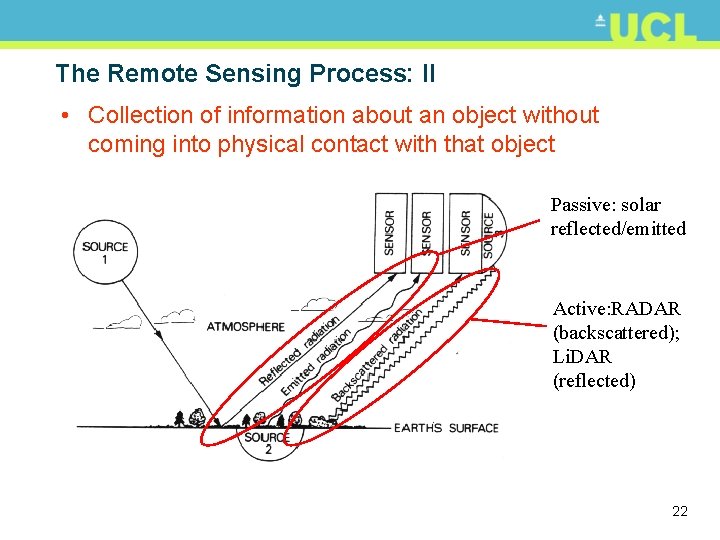
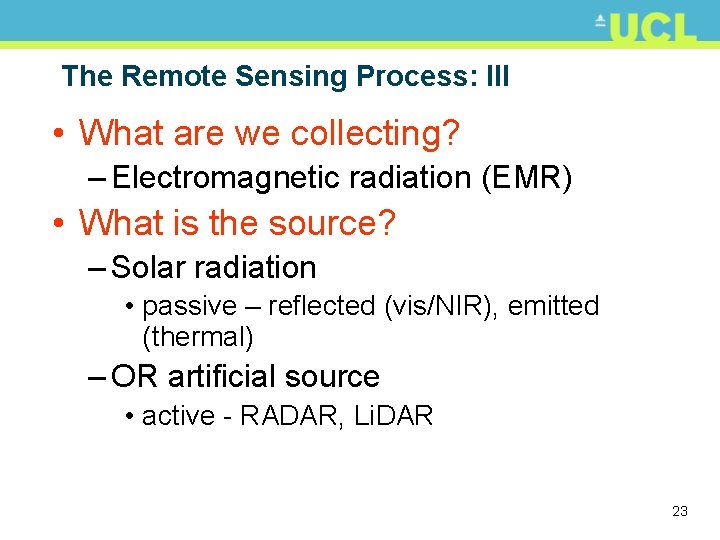
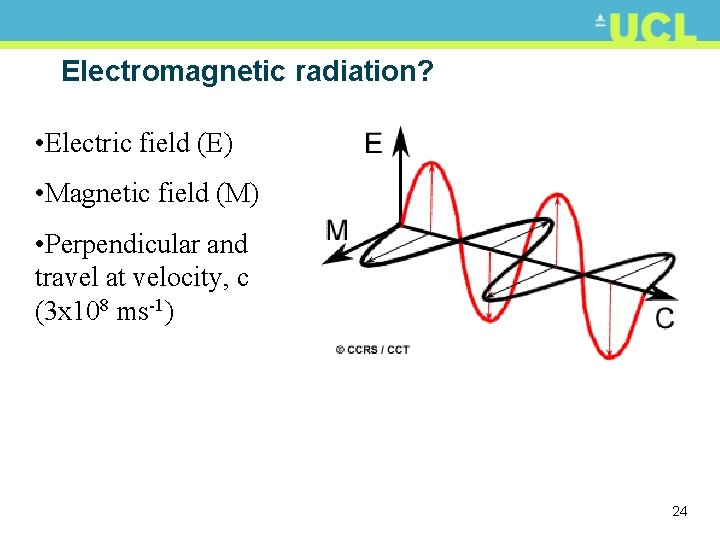
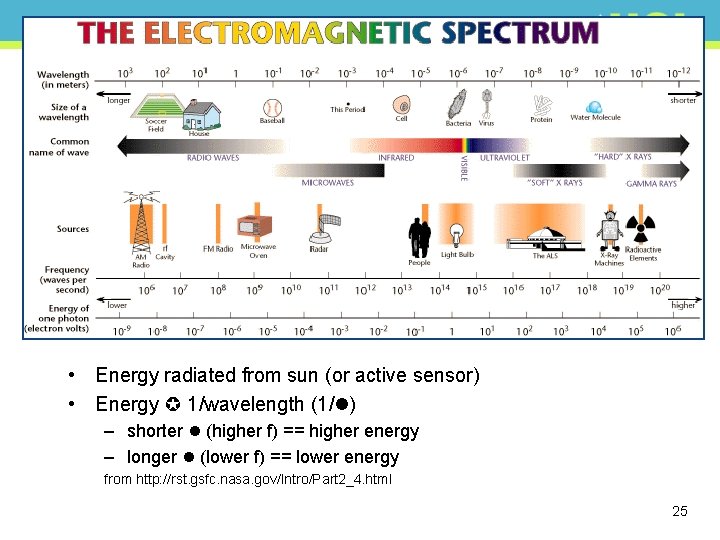
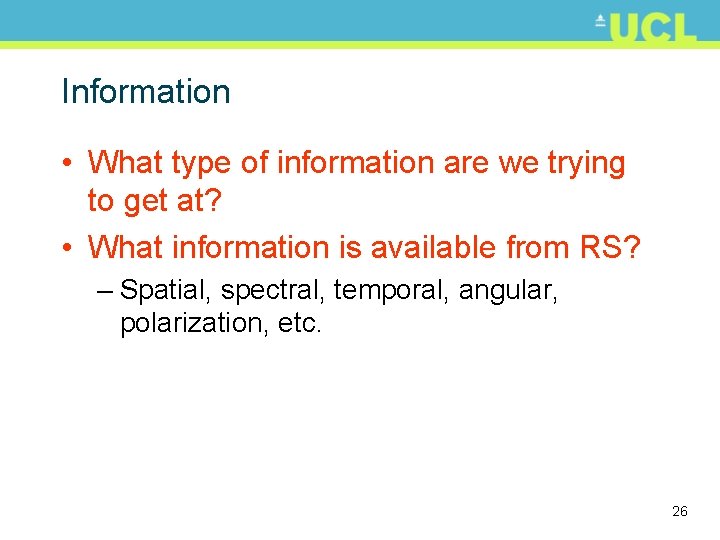
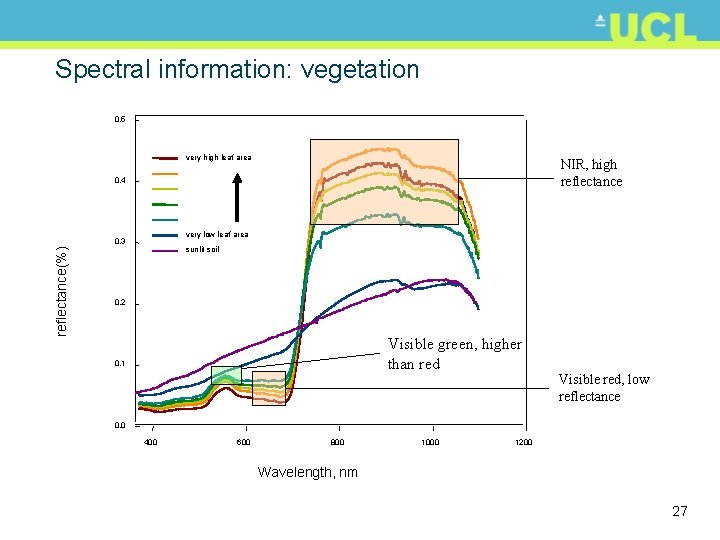
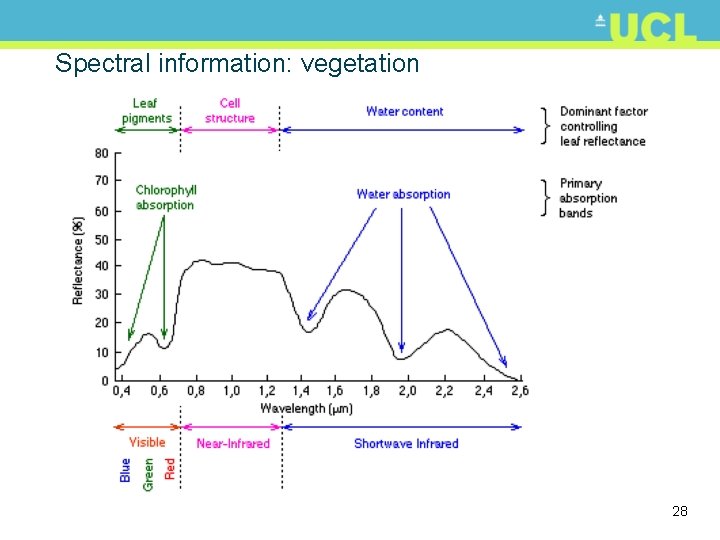
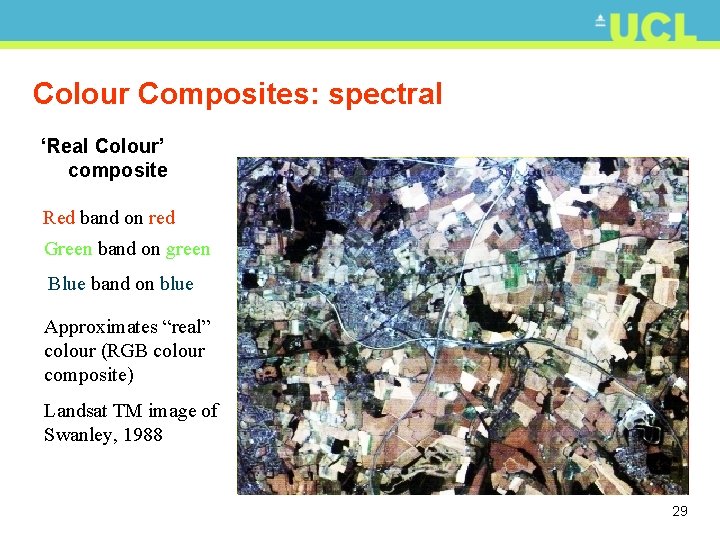
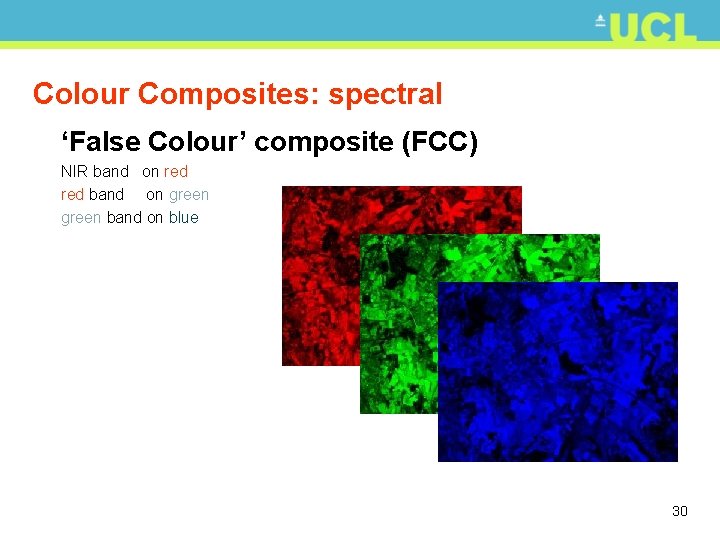
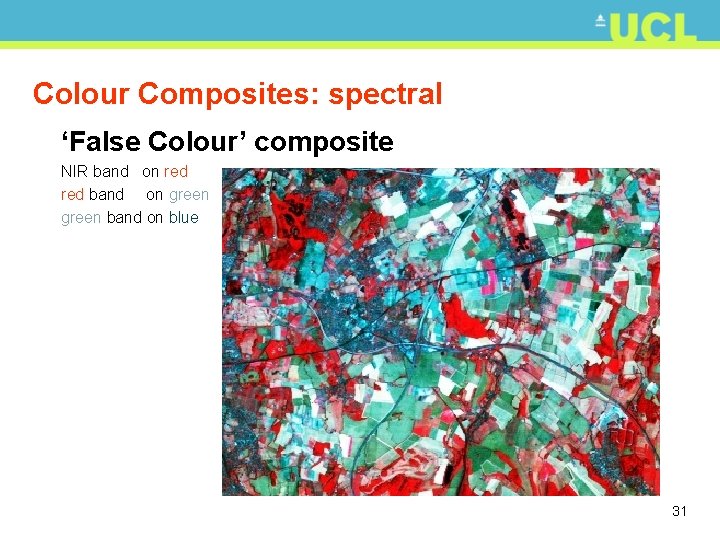
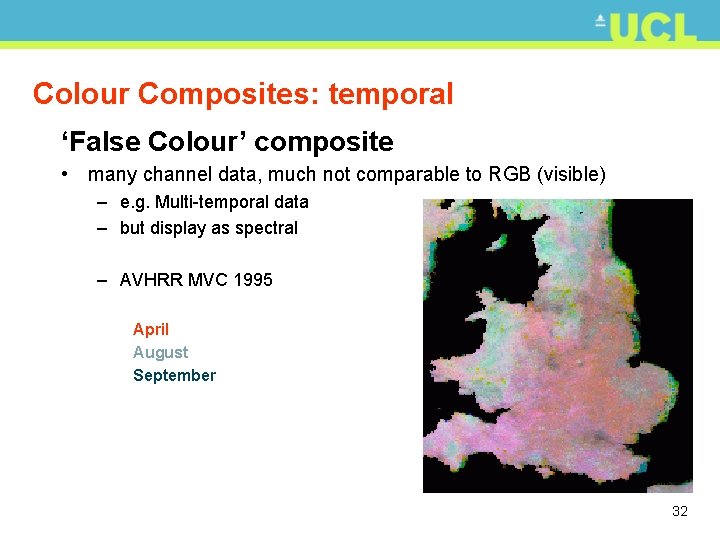
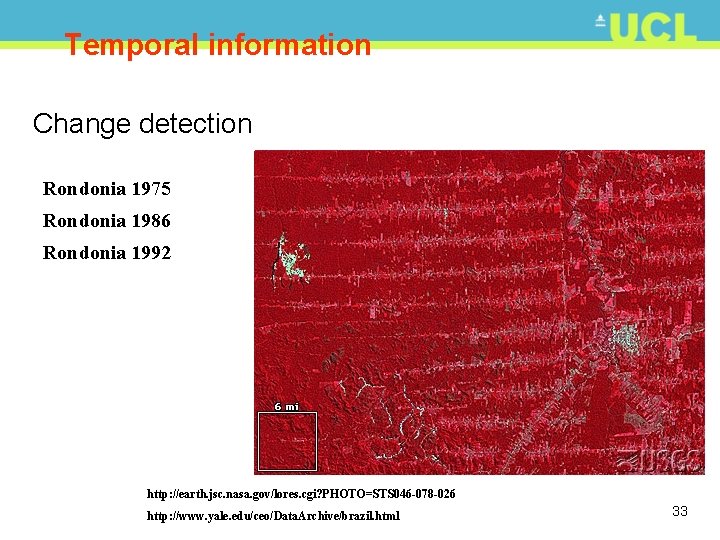
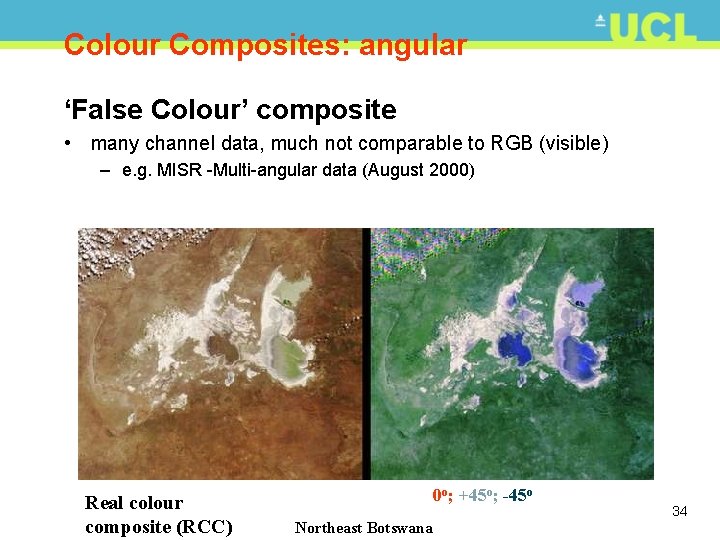
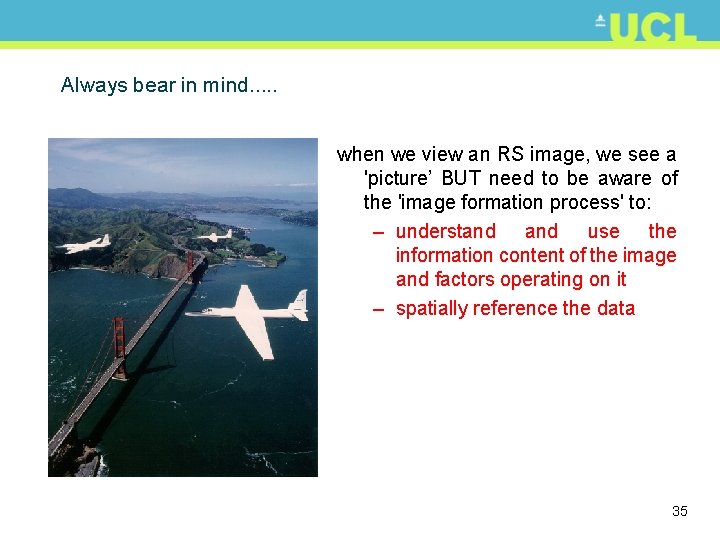
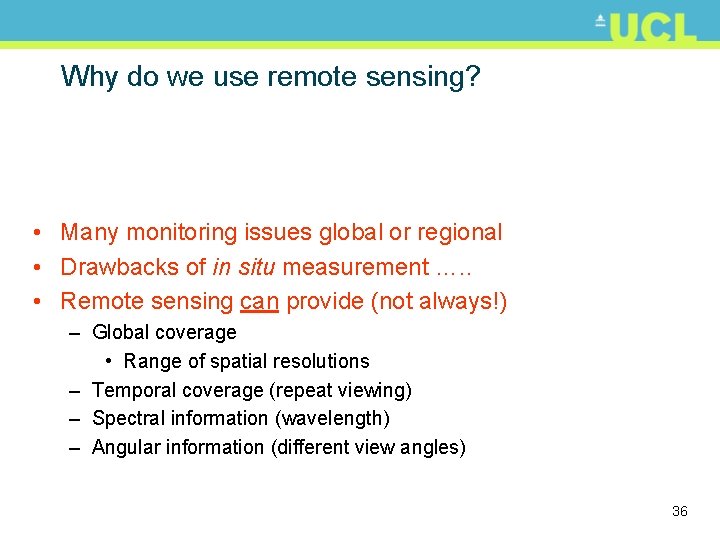
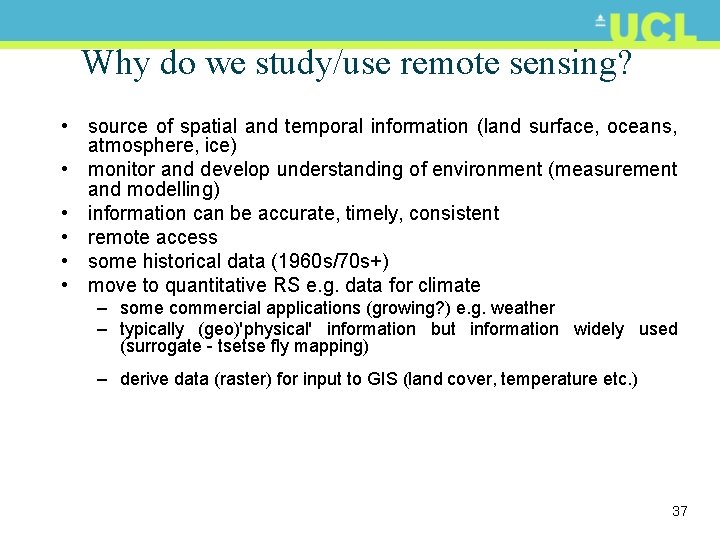
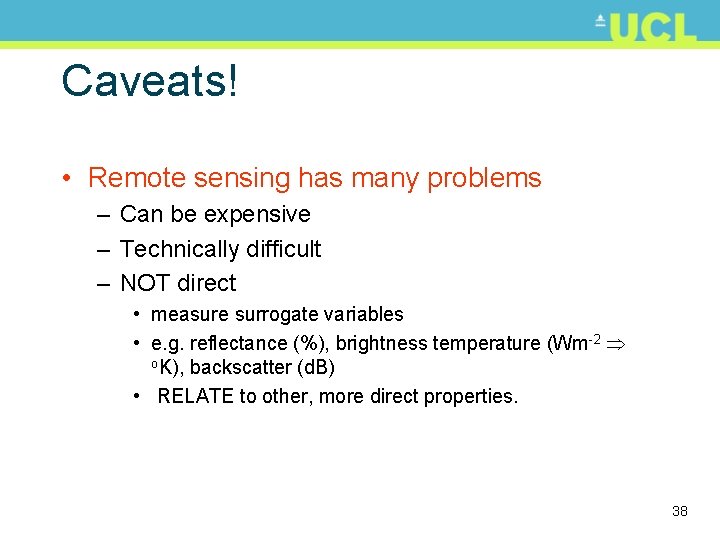
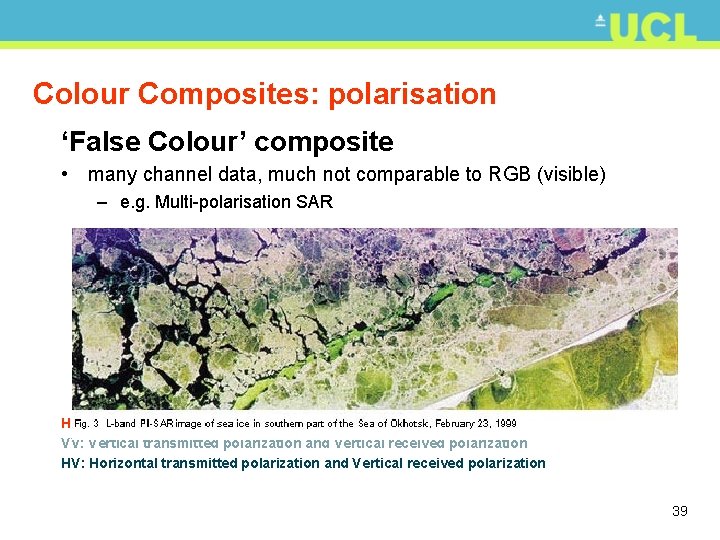
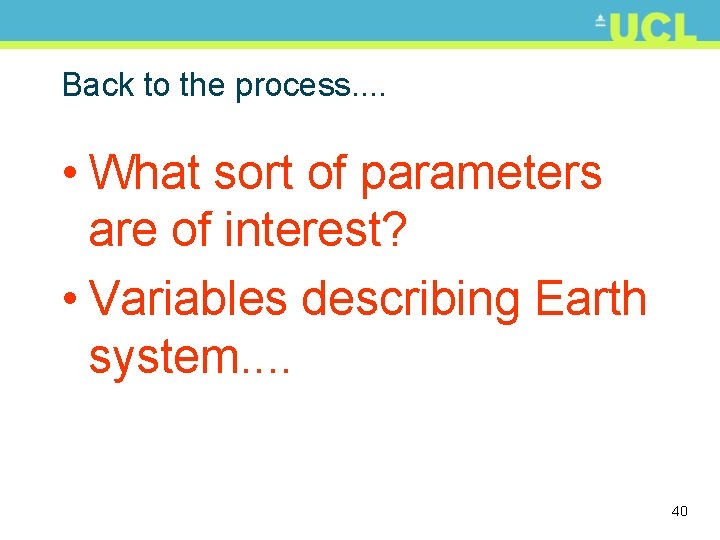
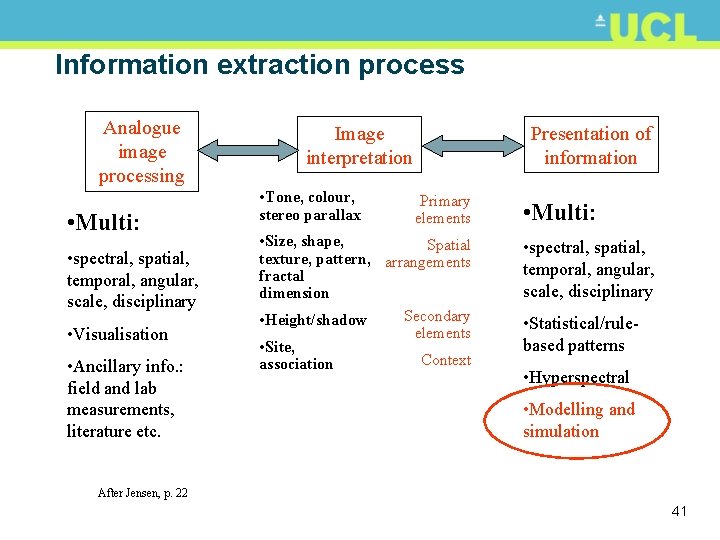
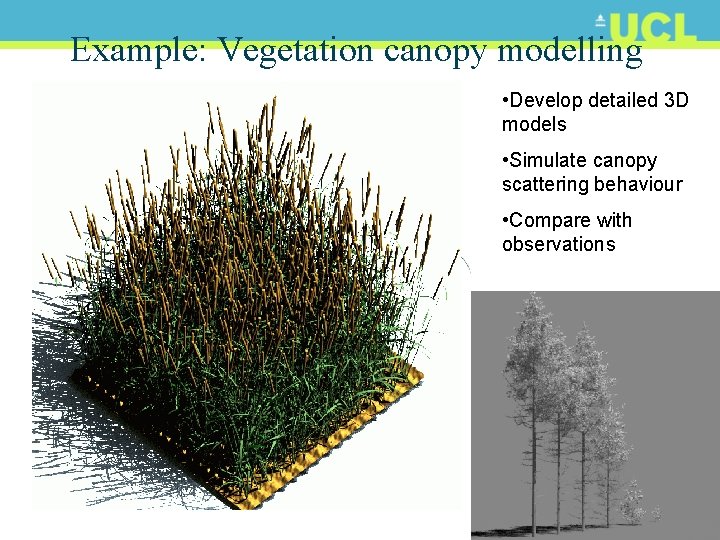
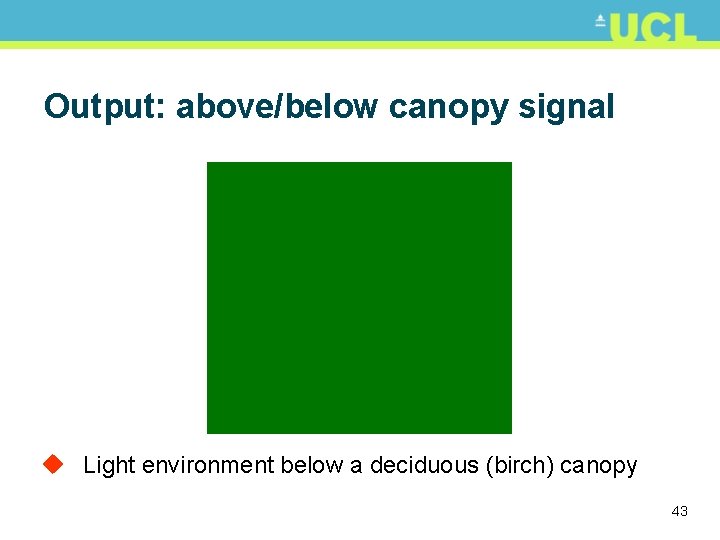
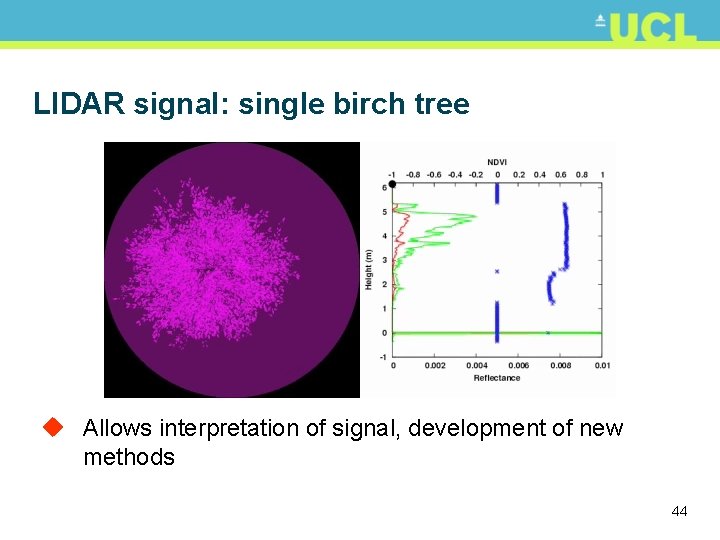
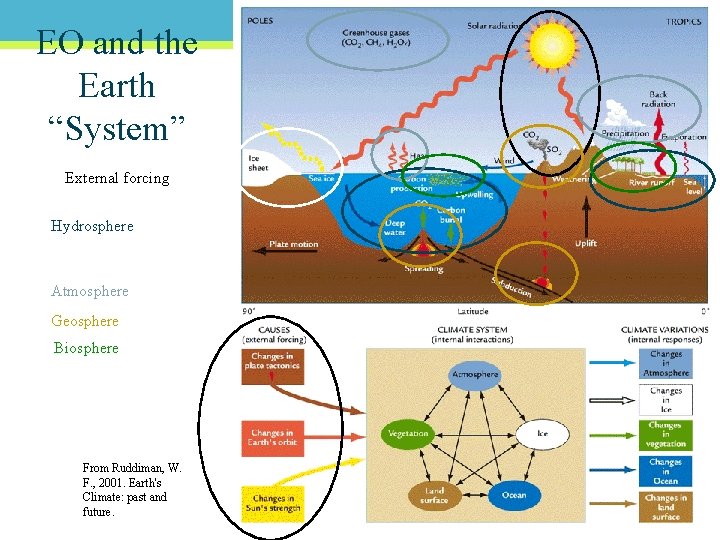
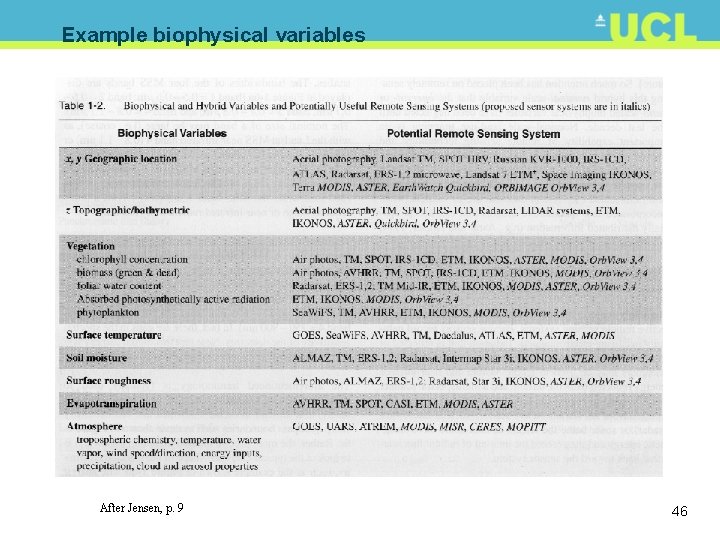
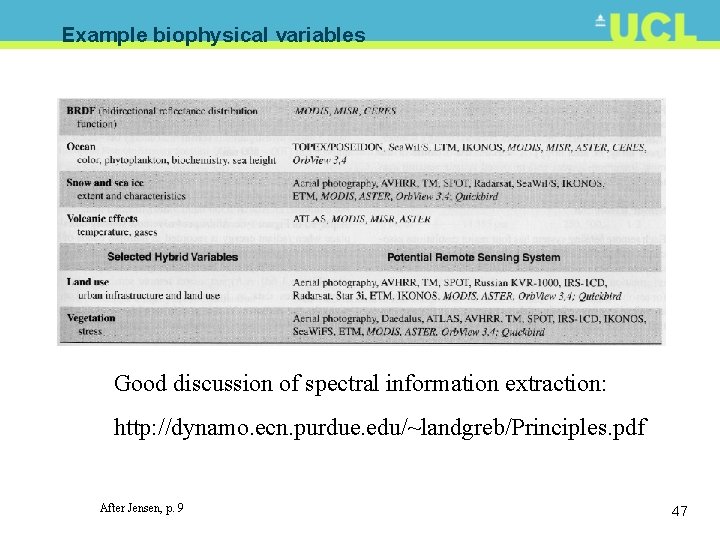
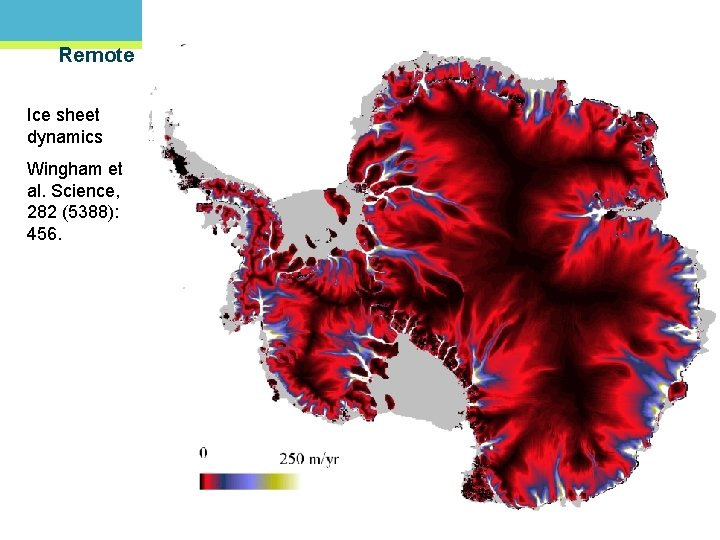
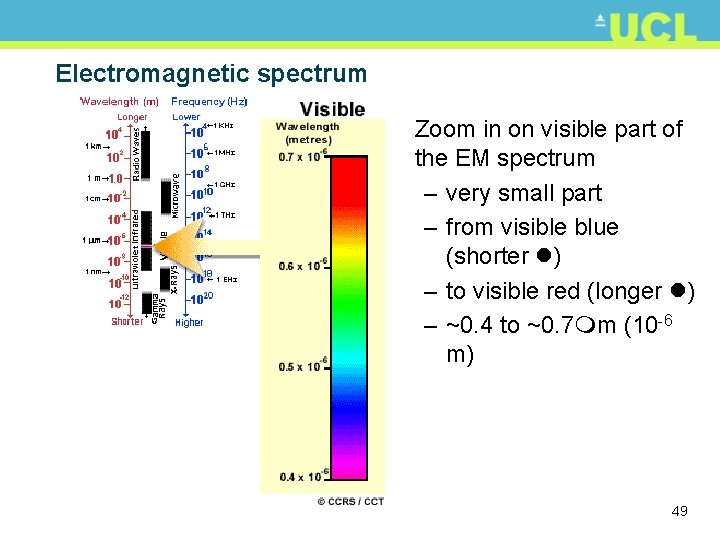
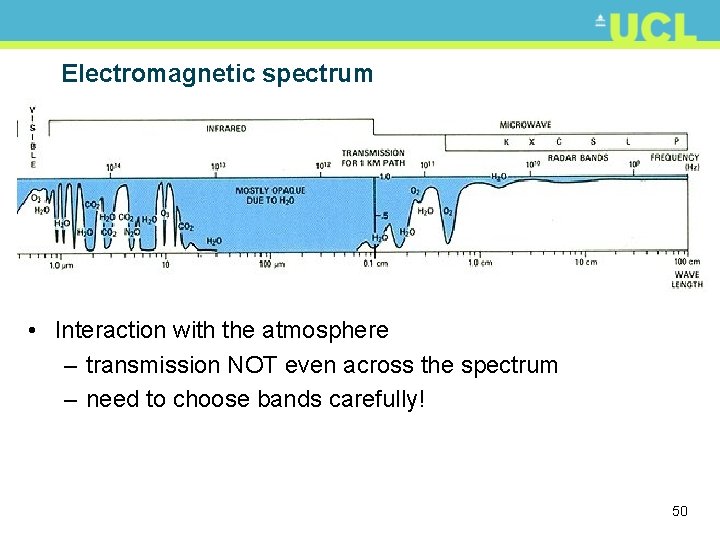
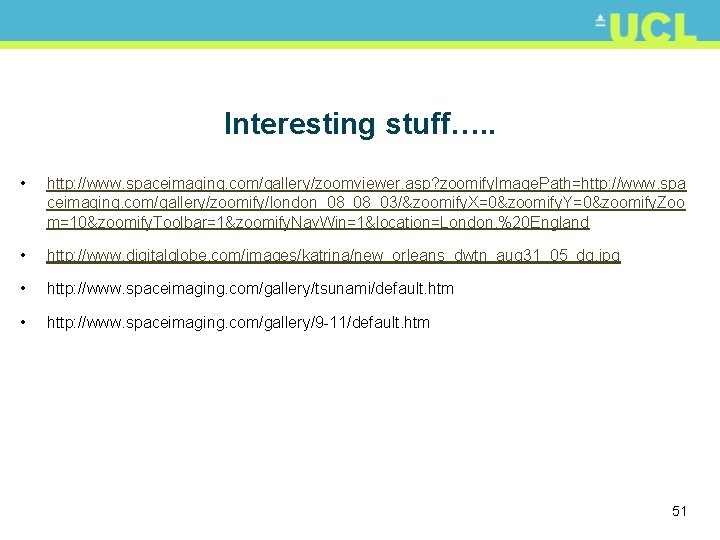
- Slides: 51
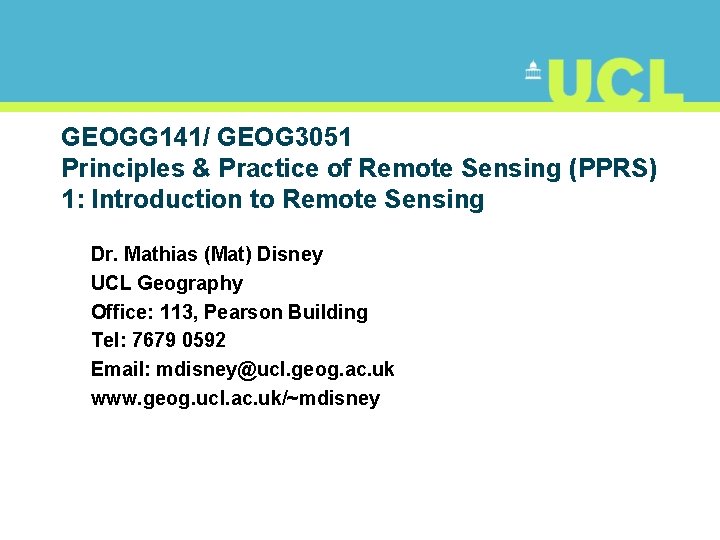
GEOGG 141/ GEOG 3051 Principles & Practice of Remote Sensing (PPRS) 1: Introduction to Remote Sensing Dr. Mathias (Mat) Disney UCL Geography Office: 113, Pearson Building Tel: 7679 0592 Email: mdisney@ucl. geog. ac. uk www. geog. ucl. ac. uk/~mdisney
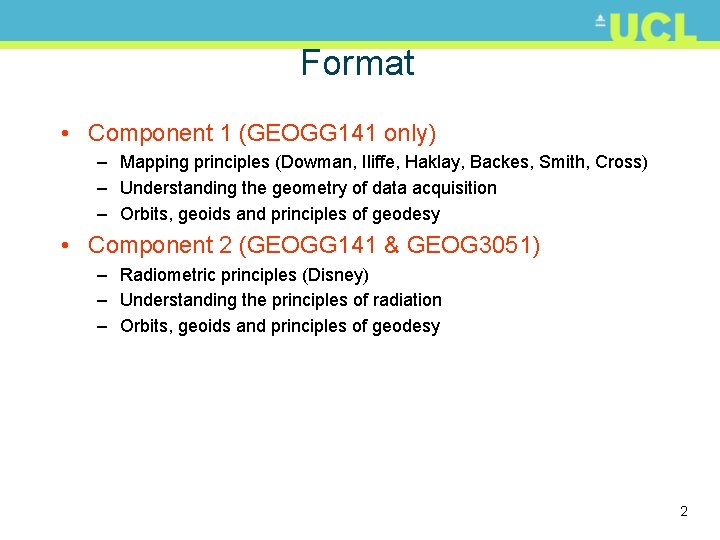
Format • Component 1 (GEOGG 141 only) – Mapping principles (Dowman, Iliffe, Haklay, Backes, Smith, Cross) – Understanding the geometry of data acquisition – Orbits, geoids and principles of geodesy • Component 2 (GEOGG 141 & GEOG 3051) – Radiometric principles (Disney) – Understanding the principles of radiation – Orbits, geoids and principles of geodesy 2
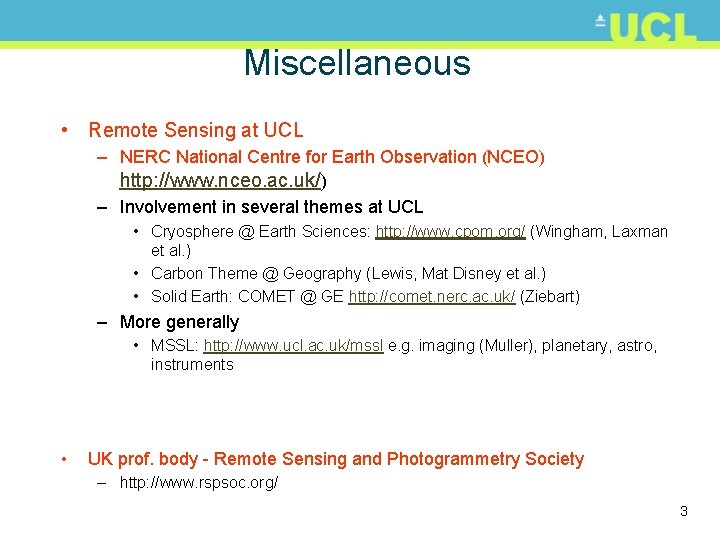
Miscellaneous • Remote Sensing at UCL – NERC National Centre for Earth Observation (NCEO) http: //www. nceo. ac. uk/) – Involvement in several themes at UCL • Cryosphere @ Earth Sciences: http: //www. cpom. org/ (Wingham, Laxman et al. ) • Carbon Theme @ Geography (Lewis, Mat Disney et al. ) • Solid Earth: COMET @ GE http: //comet. nerc. ac. uk/ (Ziebart) – More generally • MSSL: http: //www. ucl. ac. uk/mssl e. g. imaging (Muller), planetary, astro, instruments • UK prof. body - Remote Sensing and Photogrammetry Society – http: //www. rspsoc. org/ 3
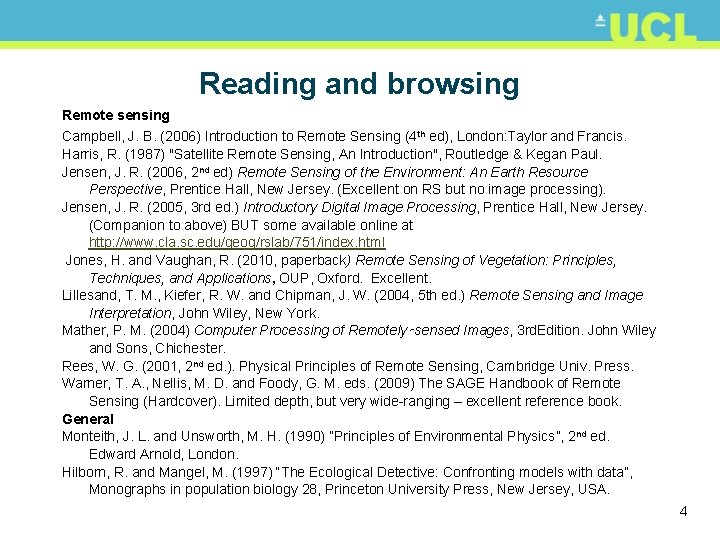
Reading and browsing Remote sensing Campbell, J. B. (2006) Introduction to Remote Sensing (4 th ed), London: Taylor and Francis. Harris, R. (1987) "Satellite Remote Sensing, An Introduction", Routledge & Kegan Paul. Jensen, J. R. (2006, 2 nd ed) Remote Sensing of the Environment: An Earth Resource Perspective, Prentice Hall, New Jersey. (Excellent on RS but no image processing). Jensen, J. R. (2005, 3 rd ed. ) Introductory Digital Image Processing, Prentice Hall, New Jersey. (Companion to above) BUT some available online at http: //www. cla. sc. edu/geog/rslab/751/index. html Jones, H. and Vaughan, R. (2010, paperback) Remote Sensing of Vegetation: Principles, Techniques, and Applications, OUP, Oxford. Excellent. Lillesand, T. M. , Kiefer, R. W. and Chipman, J. W. (2004, 5 th ed. ) Remote Sensing and Image Interpretation, John Wiley, New York. Mather, P. M. (2004) Computer Processing of Remotely‑sensed Images, 3 rd. Edition. John Wiley and Sons, Chichester. Rees, W. G. (2001, 2 nd ed. ). Physical Principles of Remote Sensing, Cambridge Univ. Press. Warner, T. A. , Nellis, M. D. and Foody, G. M. eds. (2009) The SAGE Handbook of Remote Sensing (Hardcover). Limited depth, but very wide-ranging – excellent reference book. General Monteith, J. L. and Unsworth, M. H. (1990) ”Principles of Environmental Physics”, 2 nd ed. Edward Arnold, London. Hilborn, R. and Mangel, M. (1997) “The Ecological Detective: Confronting models with data”, Monographs in population biology 28, Princeton University Press, New Jersey, USA. 4
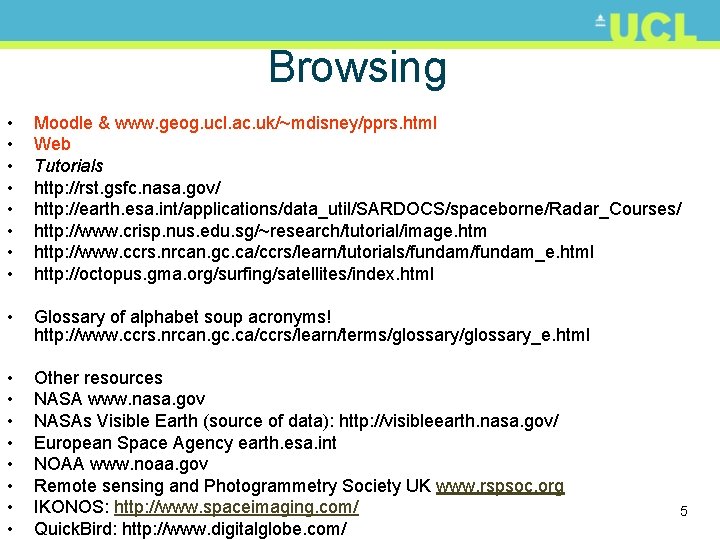
Browsing • • Moodle & www. geog. ucl. ac. uk/~mdisney/pprs. html Web Tutorials http: //rst. gsfc. nasa. gov/ http: //earth. esa. int/applications/data_util/SARDOCS/spaceborne/Radar_Courses/ http: //www. crisp. nus. edu. sg/~research/tutorial/image. htm http: //www. ccrs. nrcan. gc. ca/ccrs/learn/tutorials/fundam_e. html http: //octopus. gma. org/surfing/satellites/index. html • Glossary of alphabet soup acronyms! http: //www. ccrs. nrcan. gc. ca/ccrs/learn/terms/glossary_e. html • • Other resources NASA www. nasa. gov NASAs Visible Earth (source of data): http: //visibleearth. nasa. gov/ European Space Agency earth. esa. int NOAA www. noaa. gov Remote sensing and Photogrammetry Society UK www. rspsoc. org IKONOS: http: //www. spaceimaging. com/ Quick. Bird: http: //www. digitalglobe. com/ 5
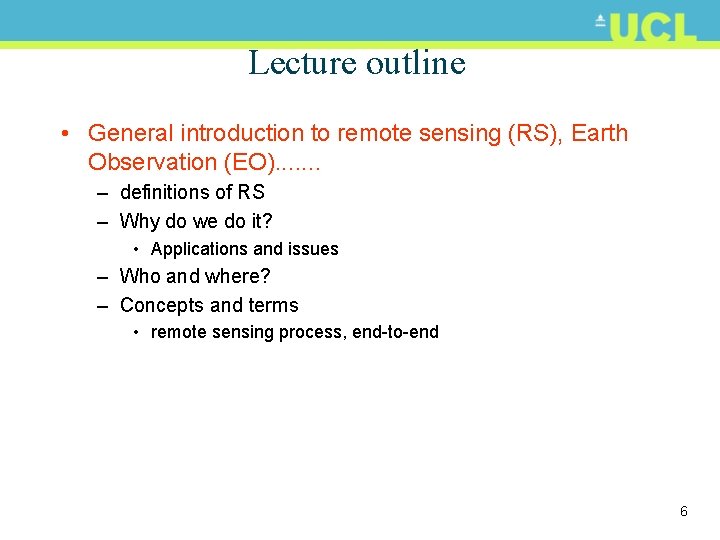
Lecture outline • General introduction to remote sensing (RS), Earth Observation (EO). . . . – definitions of RS – Why do we do it? • Applications and issues – Who and where? – Concepts and terms • remote sensing process, end-to-end 6
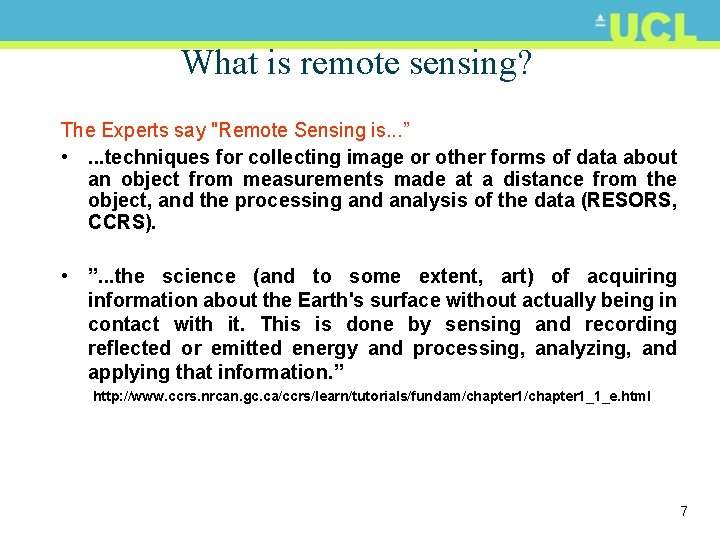
What is remote sensing? The Experts say "Remote Sensing is. . . ” • . . . techniques for collecting image or other forms of data about an object from measurements made at a distance from the object, and the processing and analysis of the data (RESORS, CCRS). • ”. . . the science (and to some extent, art) of acquiring information about the Earth's surface without actually being in contact with it. This is done by sensing and recording reflected or emitted energy and processing, analyzing, and applying that information. ” http: //www. ccrs. nrcan. gc. ca/ccrs/learn/tutorials/fundam/chapter 1_1_e. html 7
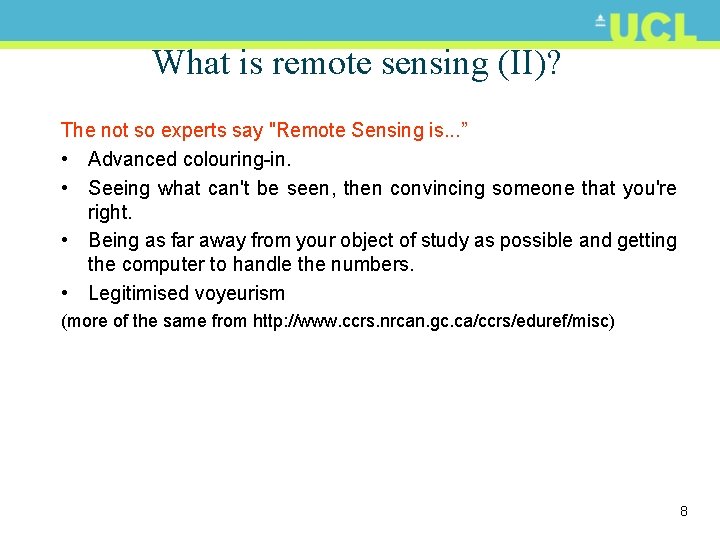
What is remote sensing (II)? The not so experts say "Remote Sensing is. . . ” • Advanced colouring-in. • Seeing what can't be seen, then convincing someone that you're right. • Being as far away from your object of study as possible and getting the computer to handle the numbers. • Legitimised voyeurism (more of the same from http: //www. ccrs. nrcan. gc. ca/ccrs/eduref/misc) 8
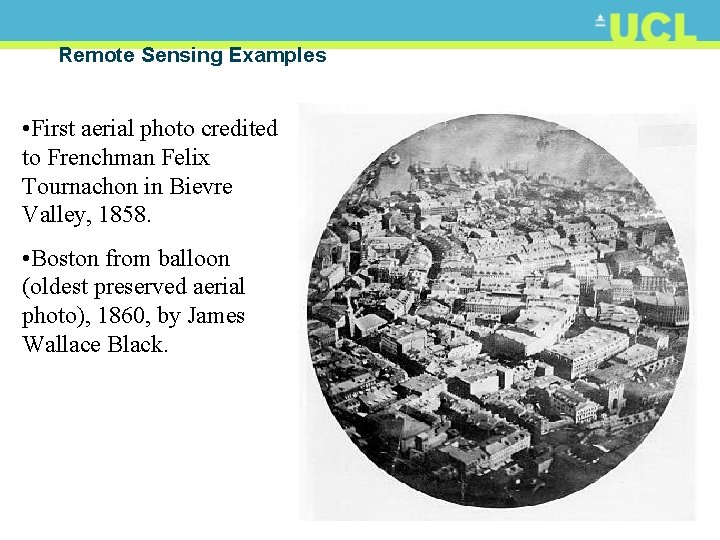
Remote Sensing Examples • First aerial photo credited to Frenchman Felix Tournachon in Bievre Valley, 1858. • Boston from balloon (oldest preserved aerial photo), 1860, by James Wallace Black. 9
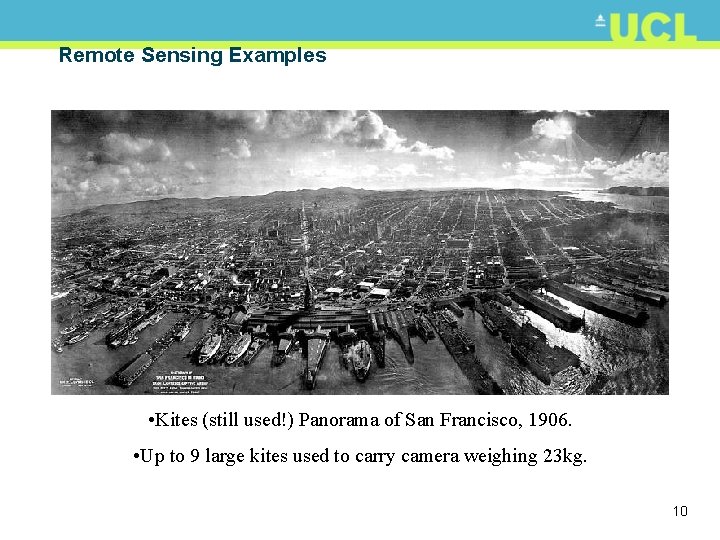
Remote Sensing Examples • Kites (still used!) Panorama of San Francisco, 1906. • Up to 9 large kites used to carry camera weighing 23 kg. 10
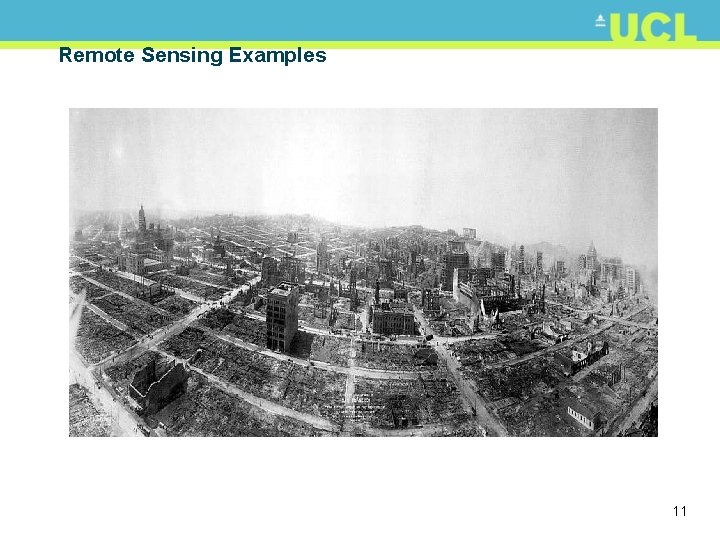
Remote Sensing Examples 11
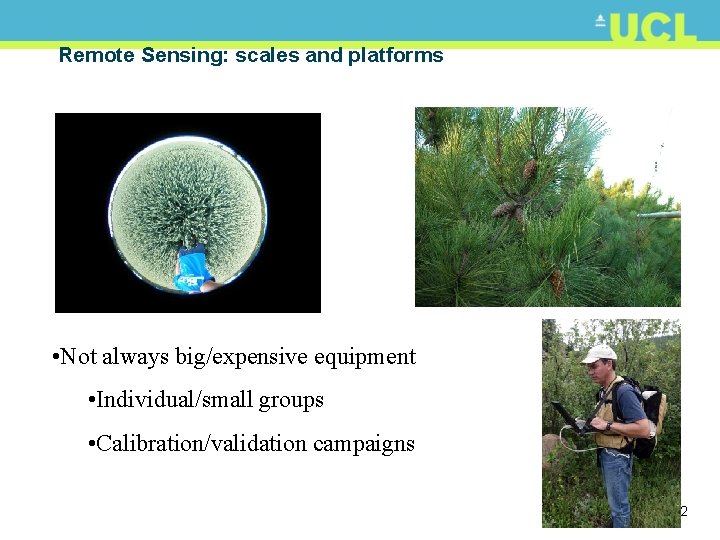
Remote Sensing: scales and platforms • Not always big/expensive equipment • Individual/small groups • Calibration/validation campaigns 12
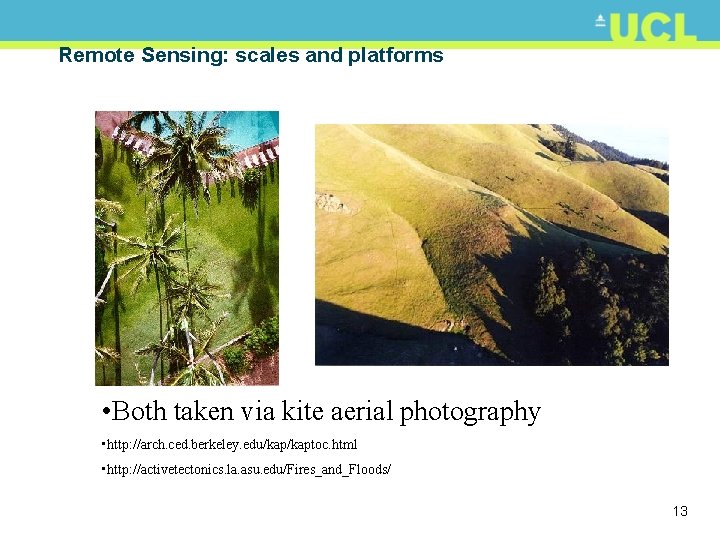
Remote Sensing: scales and platforms • Both taken via kite aerial photography • http: //arch. ced. berkeley. edu/kaptoc. html • http: //activetectonics. la. asu. edu/Fires_and_Floods/ 13
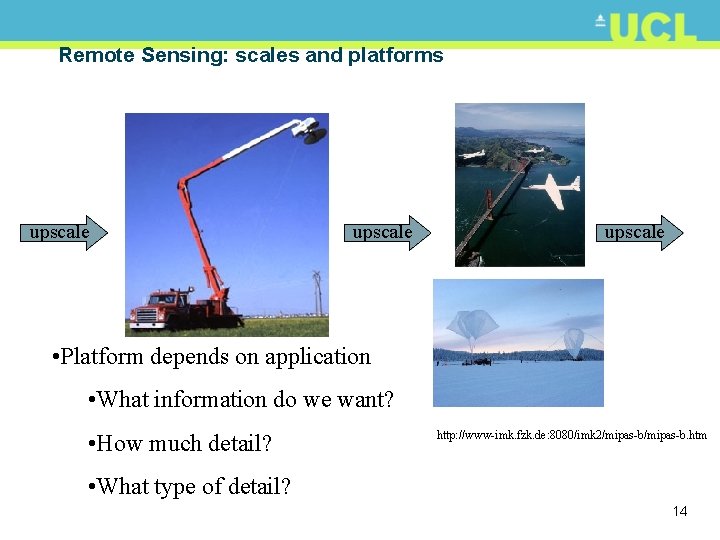
Remote Sensing: scales and platforms upscale • Platform depends on application • What information do we want? • How much detail? http: //www-imk. fzk. de: 8080/imk 2/mipas-b. htm • What type of detail? 14
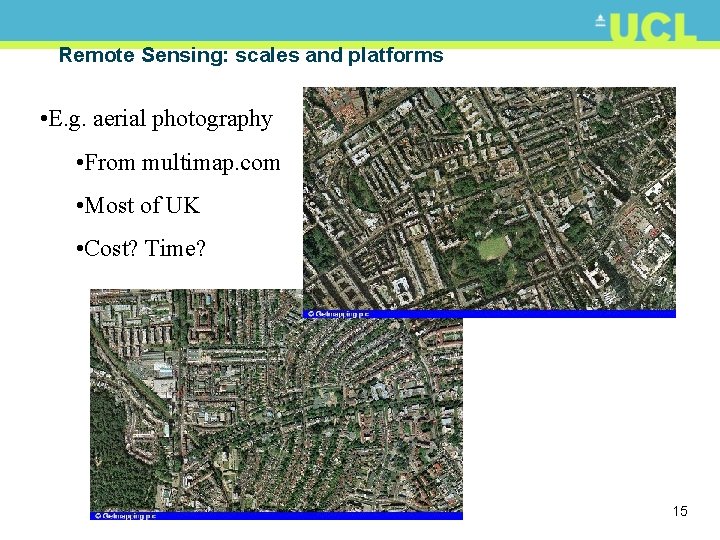
Remote Sensing: scales and platforms • E. g. aerial photography • From multimap. com • Most of UK • Cost? Time? 15
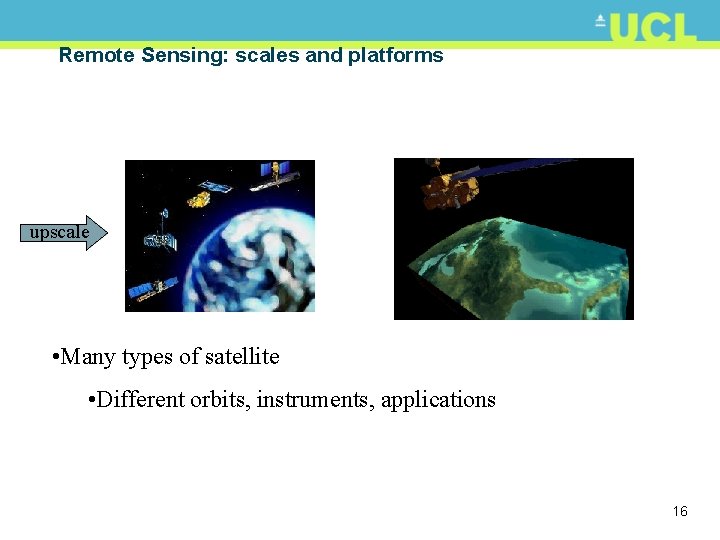
Remote Sensing: scales and platforms upscale • Many types of satellite • Different orbits, instruments, applications 16
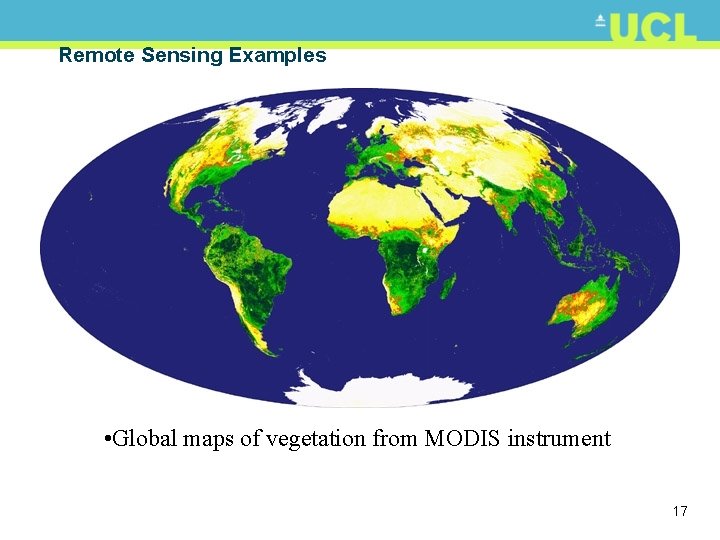
Remote Sensing Examples • Global maps of vegetation from MODIS instrument 17
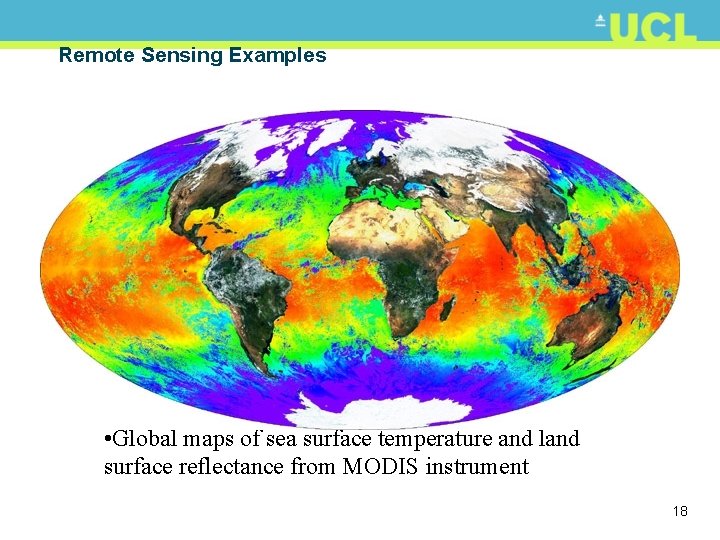
Remote Sensing Examples • Global maps of sea surface temperature and land surface reflectance from MODIS instrument 18
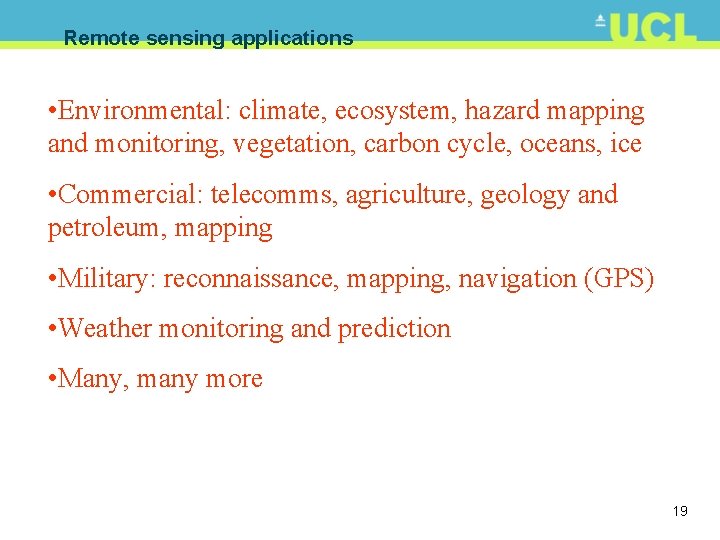
Remote sensing applications • Environmental: climate, ecosystem, hazard mapping and monitoring, vegetation, carbon cycle, oceans, ice • Commercial: telecomms, agriculture, geology and petroleum, mapping • Military: reconnaissance, mapping, navigation (GPS) • Weather monitoring and prediction • Many, many more 19
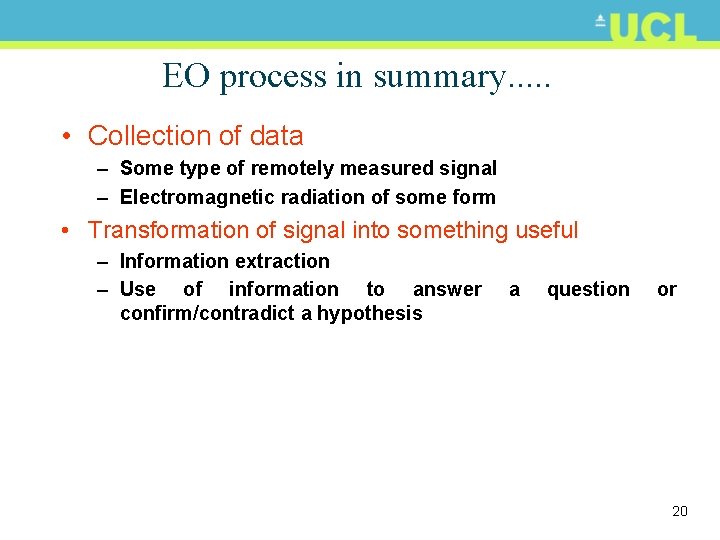
EO process in summary. . . • Collection of data – Some type of remotely measured signal – Electromagnetic radiation of some form • Transformation of signal into something useful – Information extraction – Use of information to answer confirm/contradict a hypothesis a question or 20
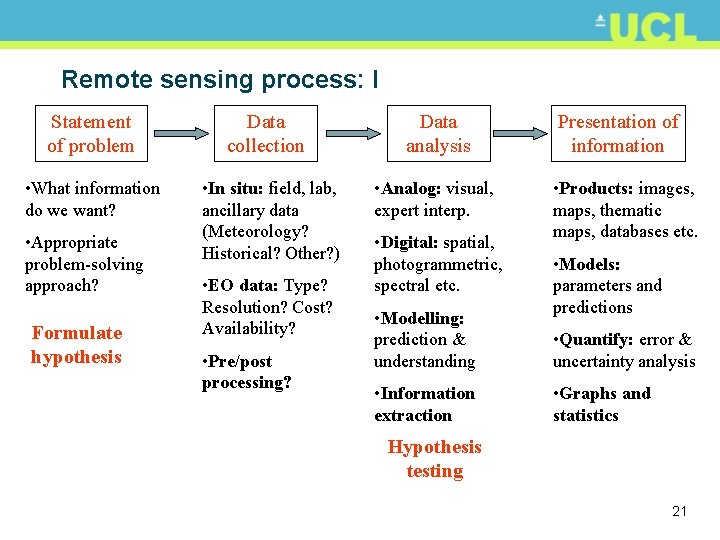
Remote sensing process: I Statement of problem Data collection Data analysis • What information do we want? • In situ: field, lab, ancillary data (Meteorology? Historical? Other? ) • Analog: visual, expert interp. • Appropriate problem-solving approach? Formulate hypothesis • EO data: Type? Resolution? Cost? Availability? • Pre/post processing? • Digital: spatial, photogrammetric, spectral etc. • Modelling: prediction & understanding • Information extraction Presentation of information • Products: images, maps, thematic maps, databases etc. • Models: parameters and predictions • Quantify: error & uncertainty analysis • Graphs and statistics Hypothesis testing 21
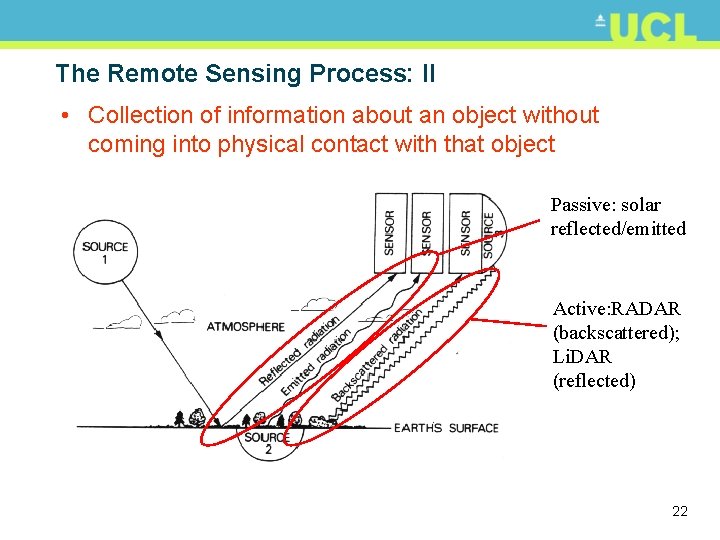
The Remote Sensing Process: II • Collection of information about an object without coming into physical contact with that object Passive: solar reflected/emitted Active: RADAR (backscattered); Li. DAR (reflected) 22
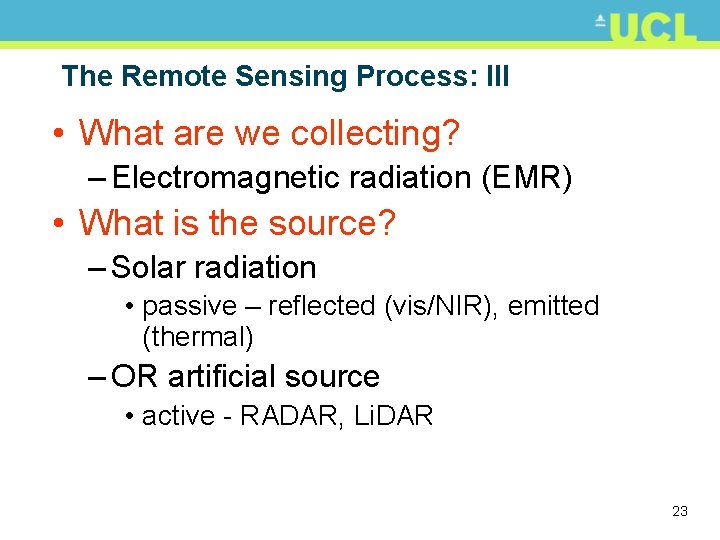
The Remote Sensing Process: III • What are we collecting? – Electromagnetic radiation (EMR) • What is the source? – Solar radiation • passive – reflected (vis/NIR), emitted (thermal) – OR artificial source • active - RADAR, Li. DAR 23
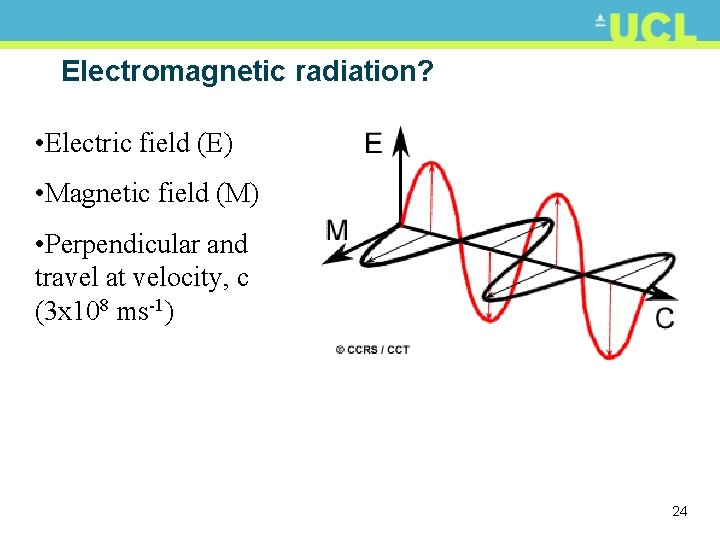
Electromagnetic radiation? • Electric field (E) • Magnetic field (M) • Perpendicular and travel at velocity, c (3 x 108 ms-1) 24
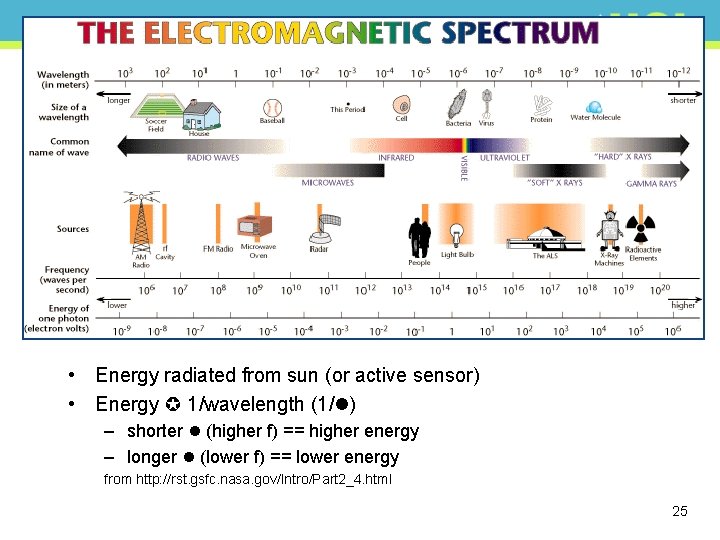
• Energy radiated from sun (or active sensor) • Energy 1/wavelength (1/ ) – shorter (higher f) == higher energy – longer (lower f) == lower energy from http: //rst. gsfc. nasa. gov/Intro/Part 2_4. html 25
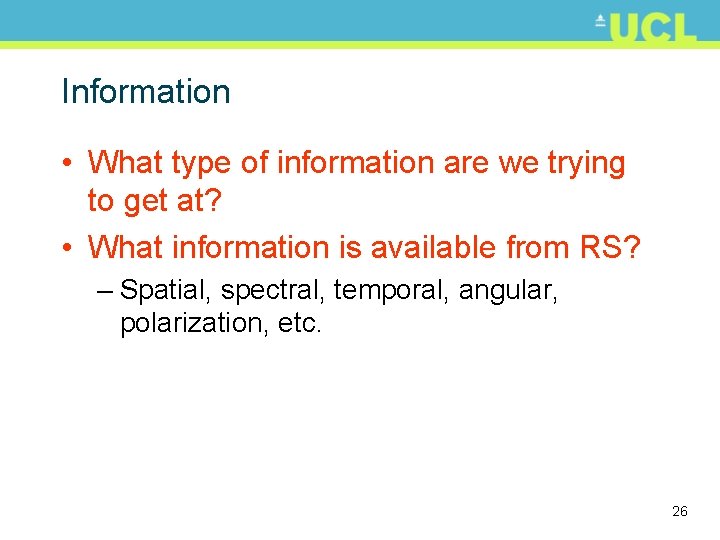
Information • What type of information are we trying to get at? • What information is available from RS? – Spatial, spectral, temporal, angular, polarization, etc. 26
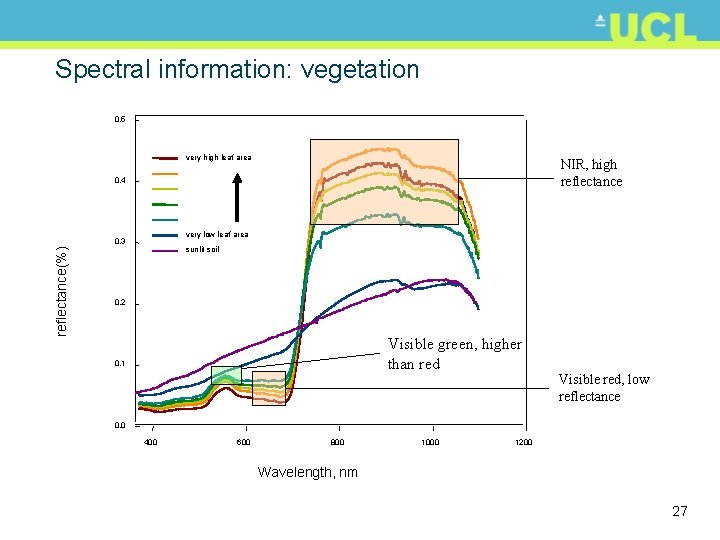
Spectral information: vegetation 0. 5 very high leaf area NIR, high reflectance 0. 4 very low leaf area reflectance(%) 0. 3 sunlit soil 0. 2 Visible green, higher than red 0. 1 Visible red, low reflectance 0. 0 400 600 800 1000 1200 Wavelength, nm 27
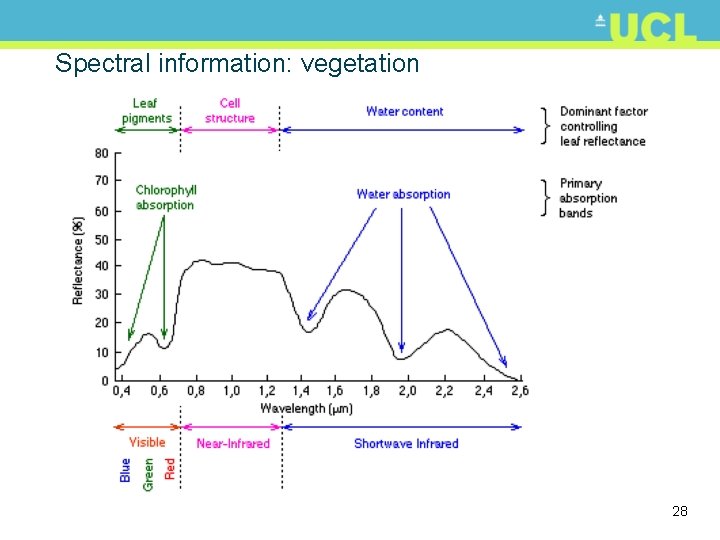
Spectral information: vegetation 28
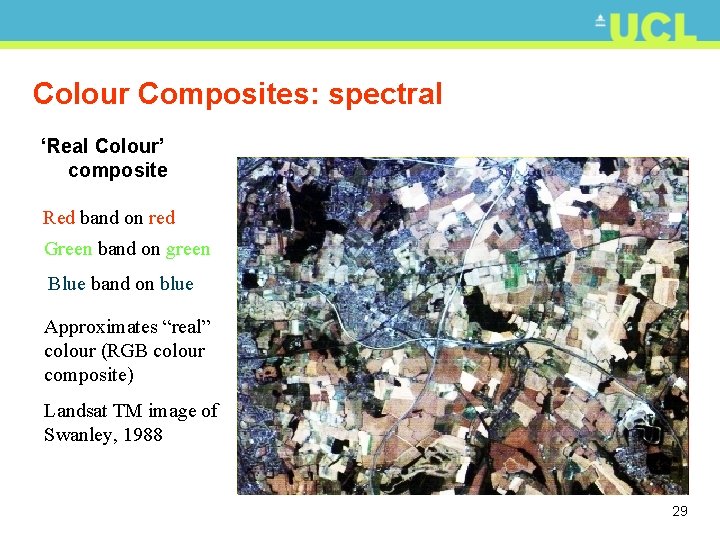
Colour Composites: spectral ‘Real Colour’ composite Red band on red Green band on green Blue band on blue Approximates “real” colour (RGB colour composite) Landsat TM image of Swanley, 1988 29
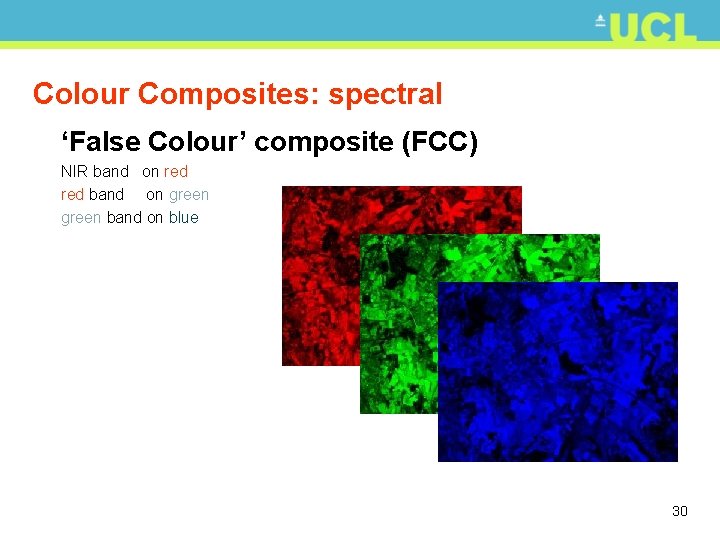
Colour Composites: spectral ‘False Colour’ composite (FCC) NIR band on red band on green band on blue 30
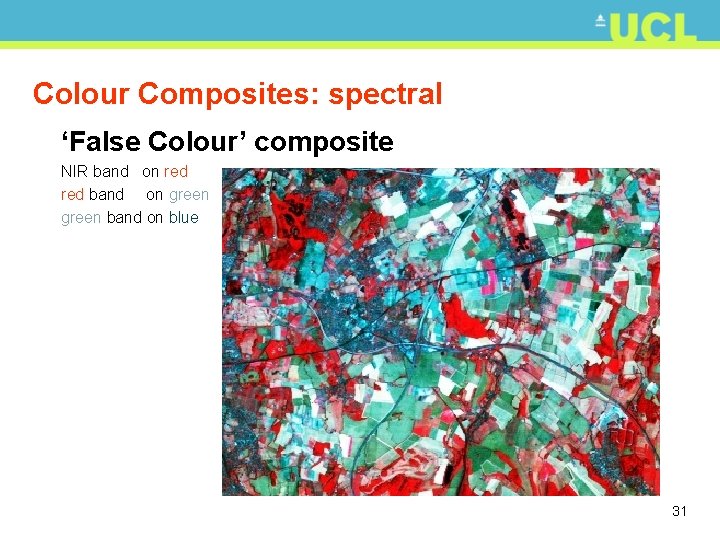
Colour Composites: spectral ‘False Colour’ composite NIR band on red band on green band on blue 31
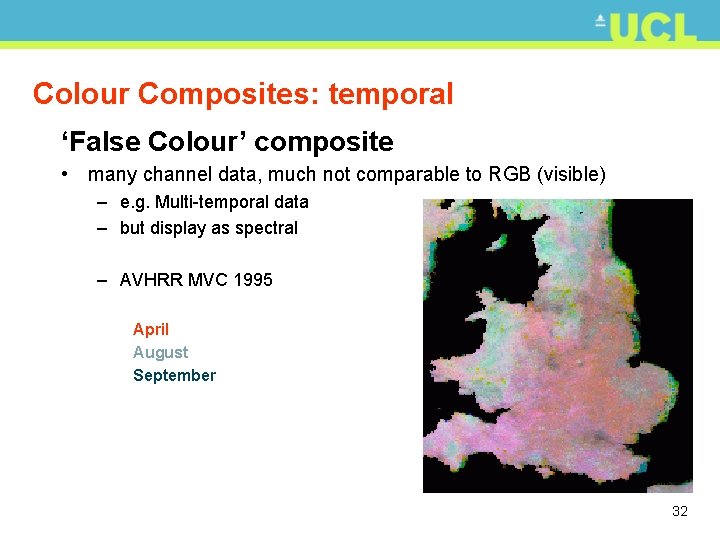
Colour Composites: temporal ‘False Colour’ composite • many channel data, much not comparable to RGB (visible) – e. g. Multi-temporal data – but display as spectral – AVHRR MVC 1995 April August September 32
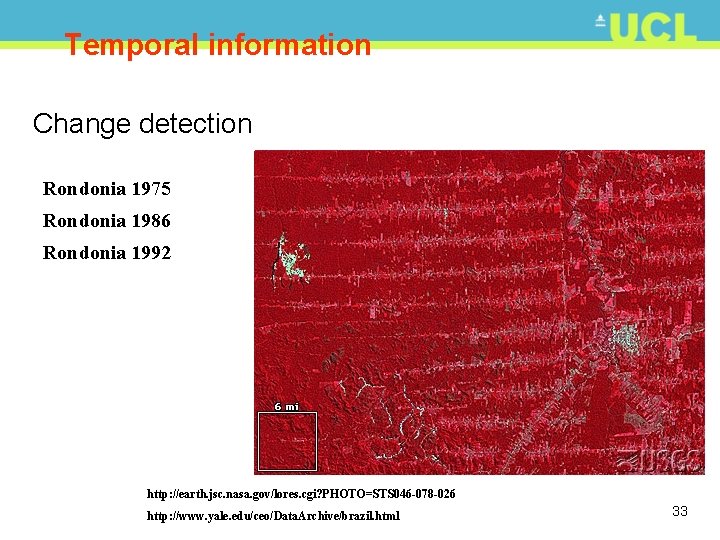
Temporal information Change detection Rondonia 1975 Rondonia 1986 Rondonia 1992 http: //earth. jsc. nasa. gov/lores. cgi? PHOTO=STS 046 -078 -026 http: //www. yale. edu/ceo/Data. Archive/brazil. html 33
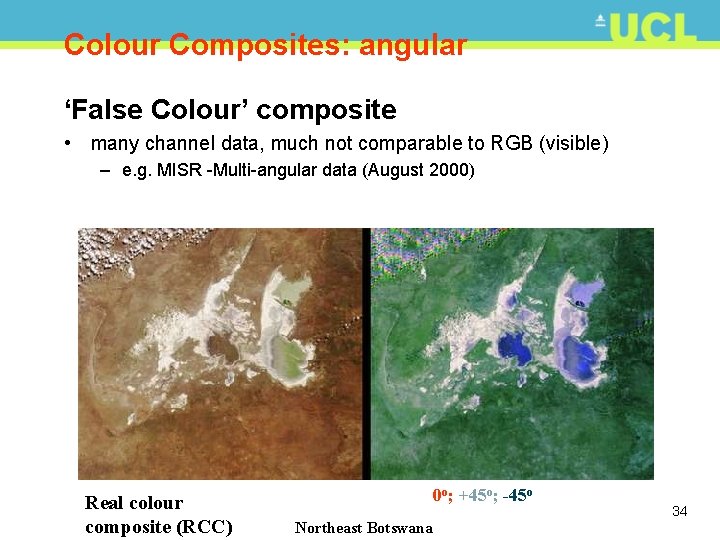
Colour Composites: angular ‘False Colour’ composite • many channel data, much not comparable to RGB (visible) – e. g. MISR -Multi-angular data (August 2000) Real colour composite (RCC) 0 o; +45 o; -45 o Northeast Botswana 34
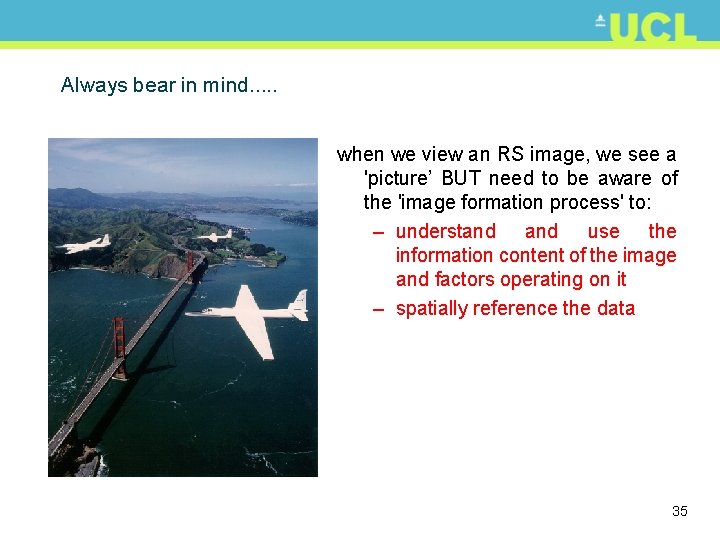
Always bear in mind. . . when we view an RS image, we see a 'picture’ BUT need to be aware of the 'image formation process' to: – understand use the information content of the image and factors operating on it – spatially reference the data 35
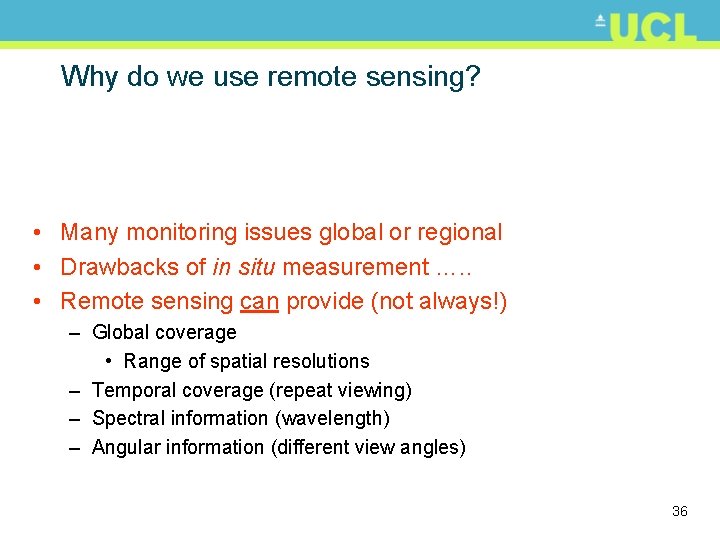
Why do we use remote sensing? • Many monitoring issues global or regional • Drawbacks of in situ measurement …. . • Remote sensing can provide (not always!) – Global coverage • Range of spatial resolutions – Temporal coverage (repeat viewing) – Spectral information (wavelength) – Angular information (different view angles) 36
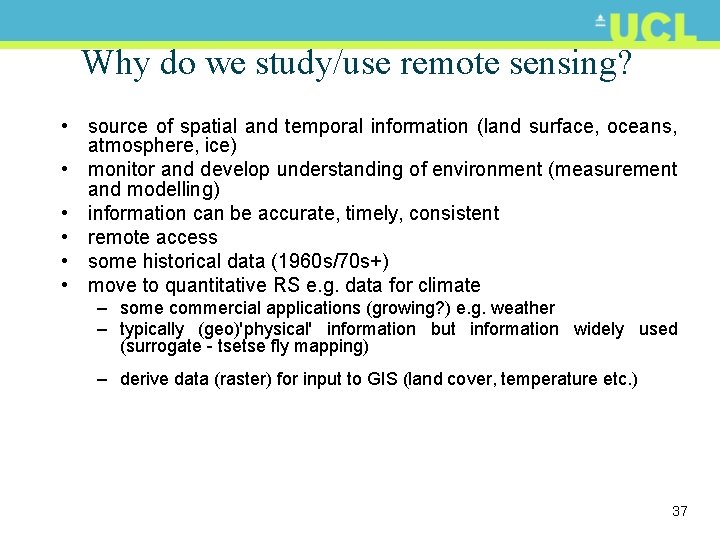
Why do we study/use remote sensing? • source of spatial and temporal information (land surface, oceans, atmosphere, ice) • monitor and develop understanding of environment (measurement and modelling) • information can be accurate, timely, consistent • remote access • some historical data (1960 s/70 s+) • move to quantitative RS e. g. data for climate – some commercial applications (growing? ) e. g. weather – typically (geo)'physical' information but information widely used (surrogate - tsetse fly mapping) – derive data (raster) for input to GIS (land cover, temperature etc. ) 37
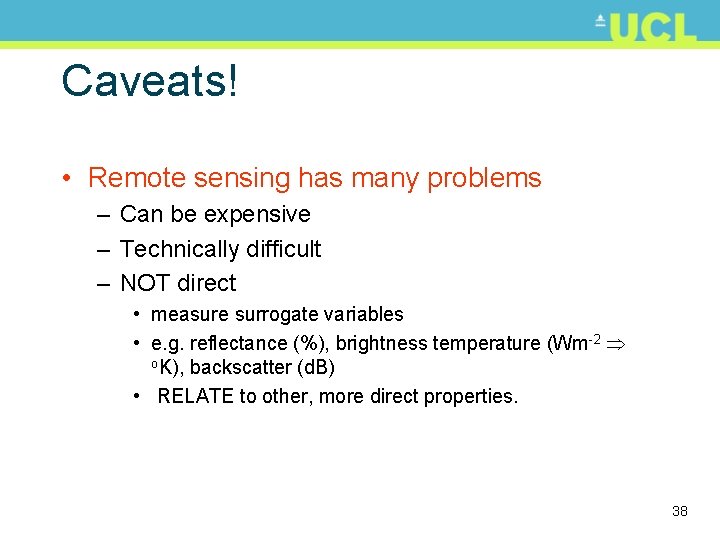
Caveats! • Remote sensing has many problems – Can be expensive – Technically difficult – NOT direct • measure surrogate variables • e. g. reflectance (%), brightness temperature (Wm-2 o. K), backscatter (d. B) • RELATE to other, more direct properties. 38
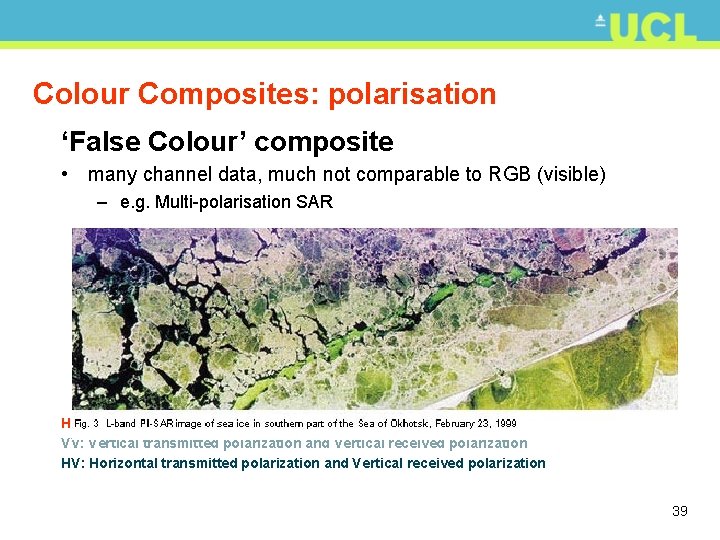
Colour Composites: polarisation ‘False Colour’ composite • many channel data, much not comparable to RGB (visible) – e. g. Multi-polarisation SAR HH: Horizontal transmitted polarization and Horizontal received polarization VV: Vertical transmitted polarization and Vertical received polarization HV: Horizontal transmitted polarization and Vertical received polarization 39
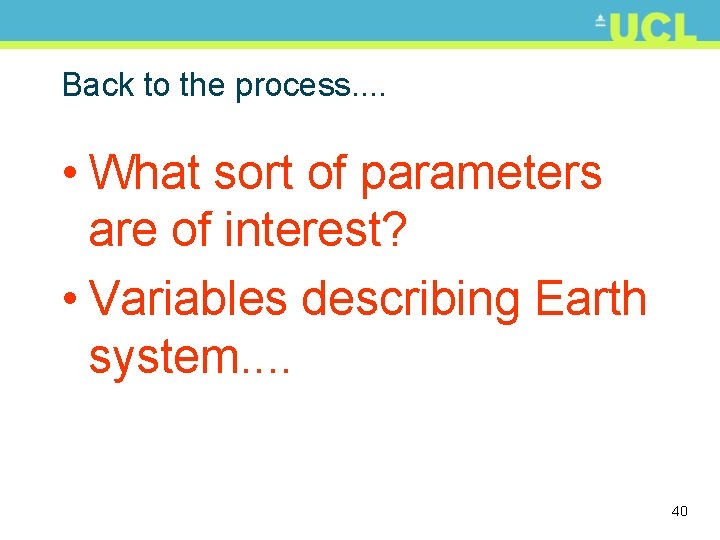
Back to the process. . • What sort of parameters are of interest? • Variables describing Earth system. . 40
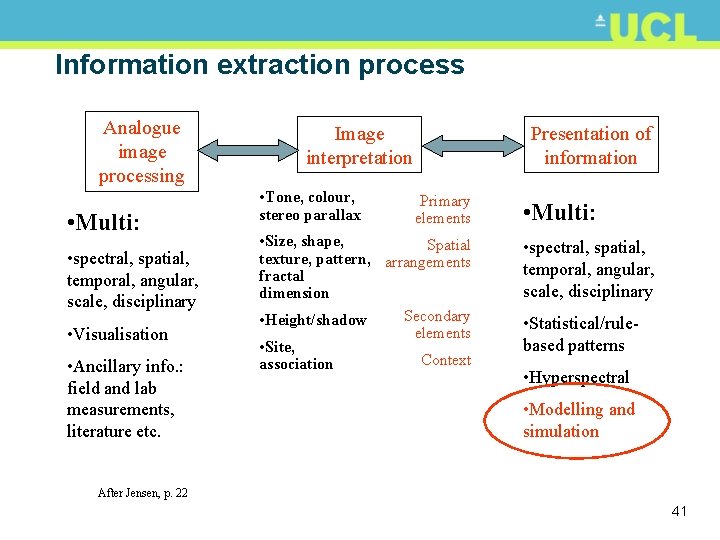
Information extraction process Analogue image processing • Multi: • spectral, spatial, temporal, angular, scale, disciplinary • Visualisation • Ancillary info. : field and lab measurements, literature etc. Image interpretation • Tone, colour, stereo parallax Presentation of information Primary elements • Size, shape, Spatial texture, pattern, arrangements fractal dimension Secondary • Height/shadow elements • Site, Context association • Multi: • spectral, spatial, temporal, angular, scale, disciplinary • Statistical/rulebased patterns • Hyperspectral • Modelling and simulation After Jensen, p. 22 41
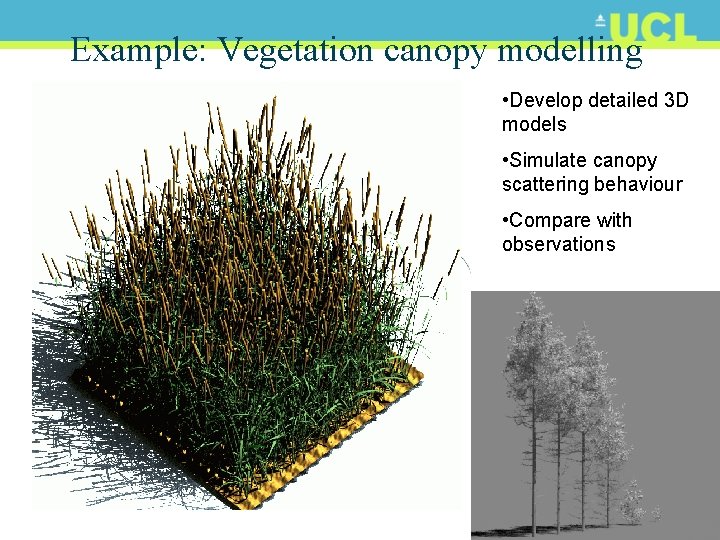
Example: Vegetation canopy modelling • Develop detailed 3 D models • Simulate canopy scattering behaviour • Compare with observations 42
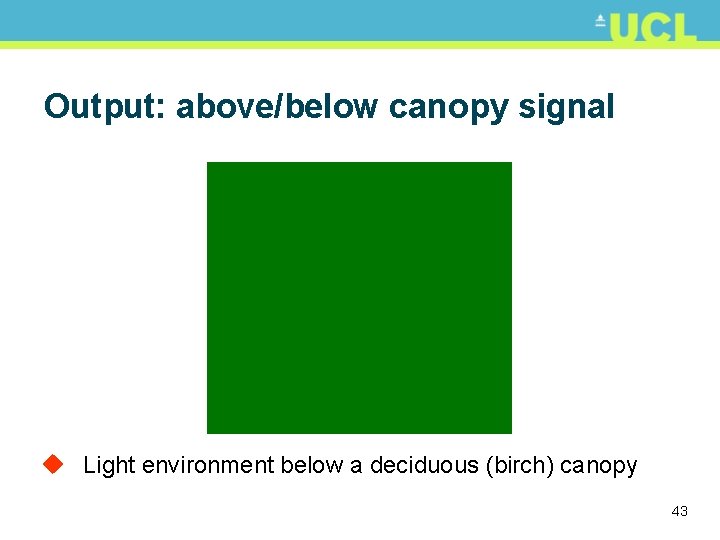
Output: above/below canopy signal u Light environment below a deciduous (birch) canopy 43
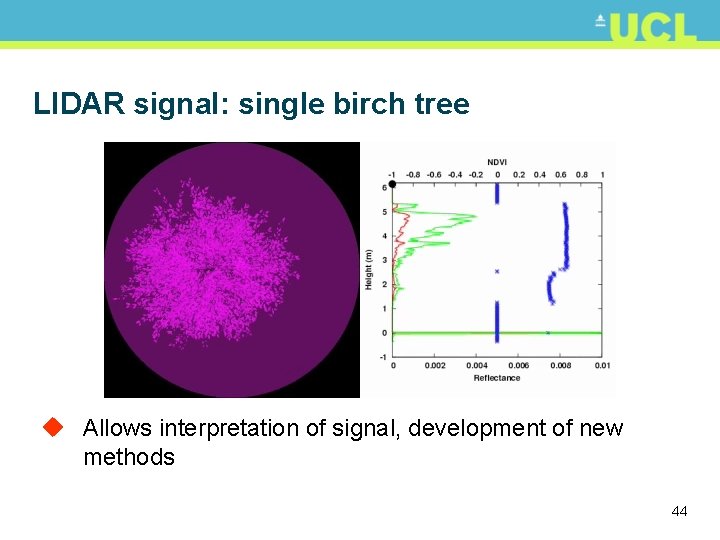
LIDAR signal: single birch tree u Allows interpretation of signal, development of new methods 44
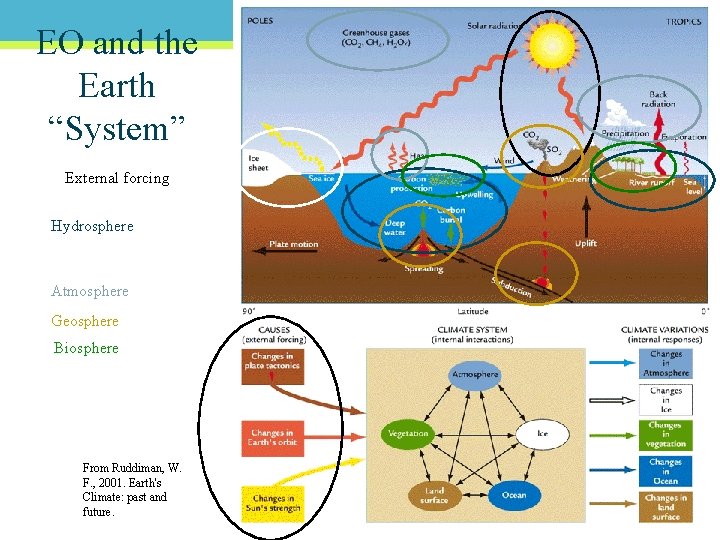
EO and the Earth “System” External forcing Hydrosphere Cryosphere Atmosphere Geosphere Biosphere From Ruddiman, W. F. , 2001. Earth's Climate: past and future. 45
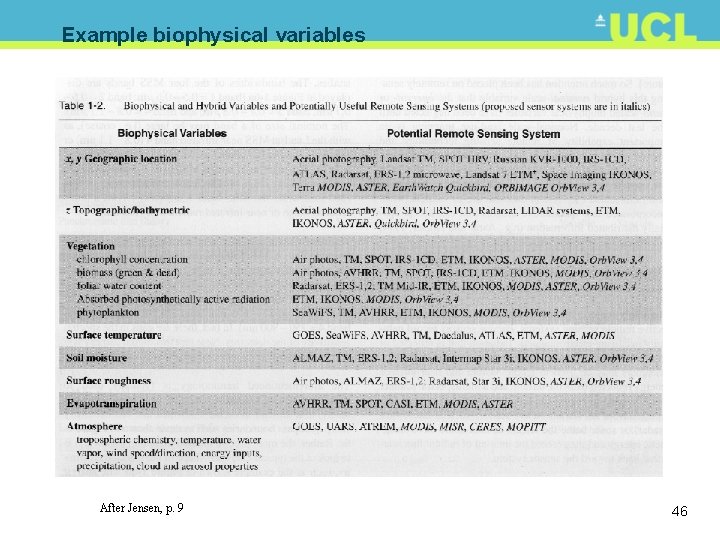
Example biophysical variables After Jensen, p. 9 46
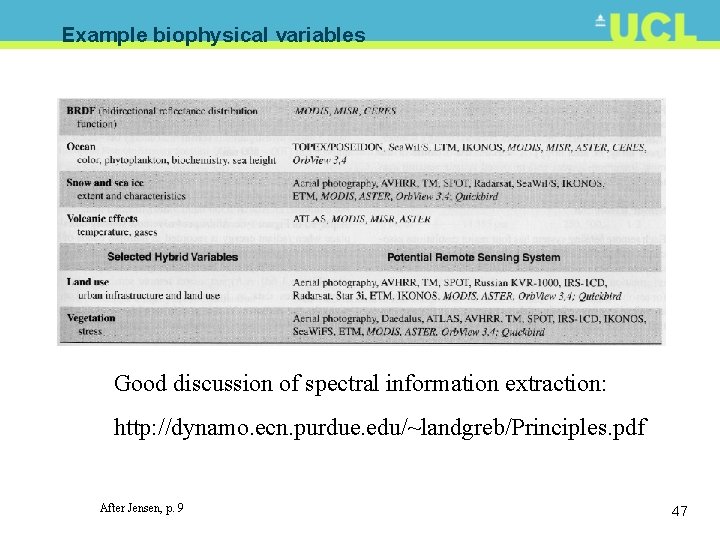
Example biophysical variables Good discussion of spectral information extraction: http: //dynamo. ecn. purdue. edu/~landgreb/Principles. pdf After Jensen, p. 9 47
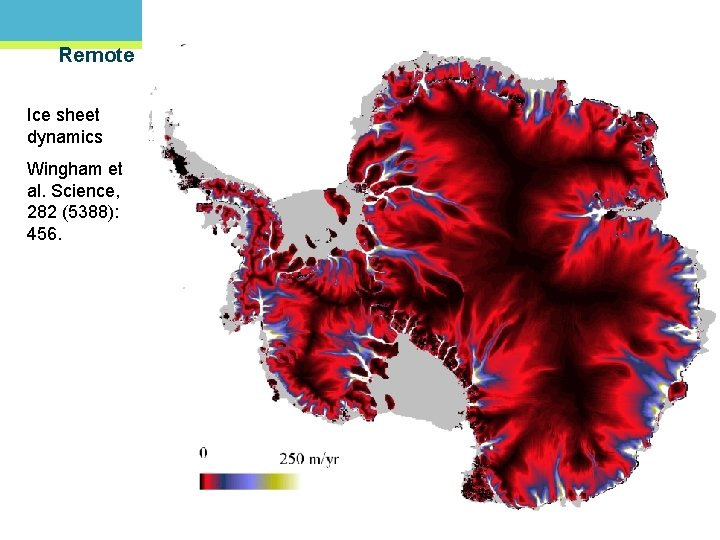
Remote Sensing Examples Ice sheet dynamics Wingham et al. Science, 282 (5388): 456. 48
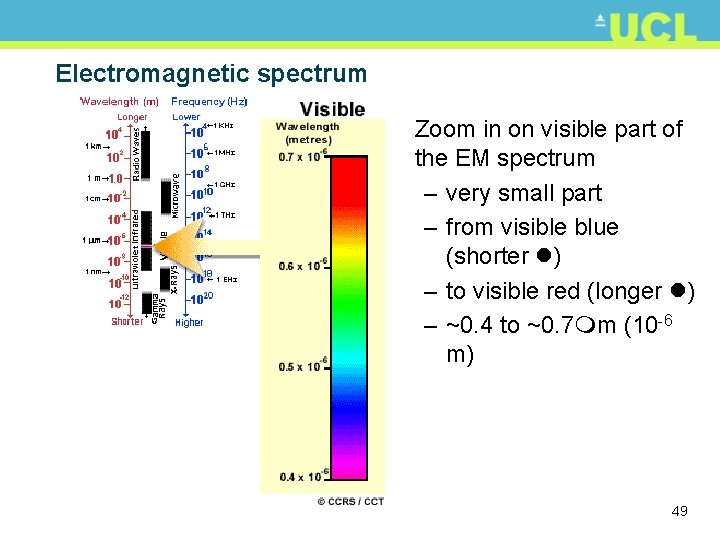
Electromagnetic spectrum • Zoom in on visible part of the EM spectrum – very small part – from visible blue (shorter ) – to visible red (longer ) – ~0. 4 to ~0. 7 m (10 -6 m) 49
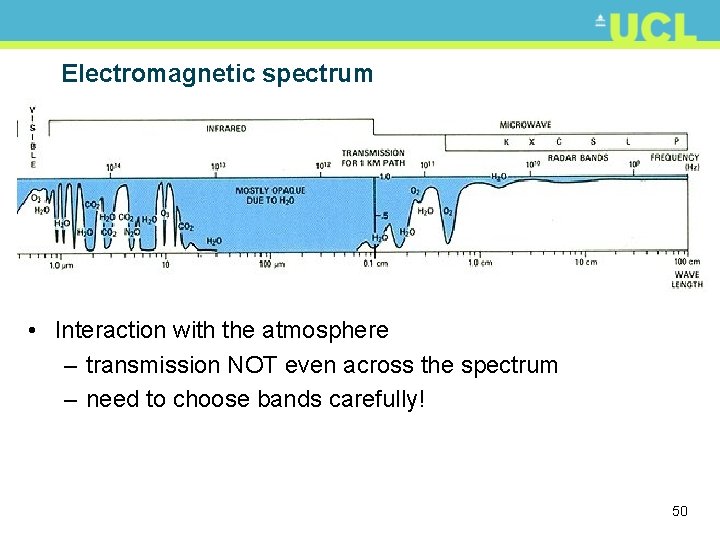
Electromagnetic spectrum • Interaction with the atmosphere – transmission NOT even across the spectrum – need to choose bands carefully! 50
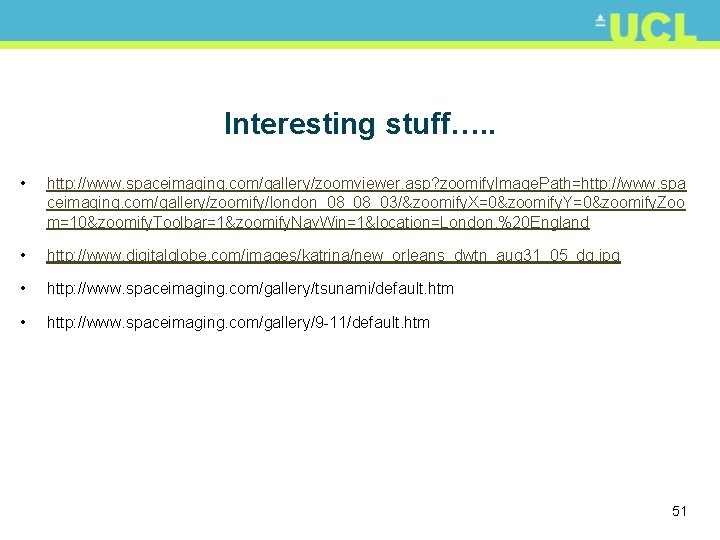
Interesting stuff…. . • http: //www. spaceimaging. com/gallery/zoomviewer. asp? zoomify. Image. Path=http: //www. spa ceimaging. com/gallery/zoomify/london_08_08_03/&zoomify. X=0&zoomify. Y=0&zoomify. Zoo m=10&zoomify. Toolbar=1&zoomify. Nav. Win=1&location=London, %20 England • http: //www. digitalglobe. com/images/katrina/new_orleans_dwtn_aug 31_05_dg. jpg • http: //www. spaceimaging. com/gallery/tsunami/default. htm • http: //www. spaceimaging. com/gallery/9 -11/default. htm 51
Unit 1 geog. of ga/ga’s beginnings
May subd geog
Geog 312 sfu
Dse geog essay sample
Geog 214
Geog
3051cd flow transmitter
Ordin 3051/2016
Emerson ers
Dma-3051
Omen 3051/2016
Mat 3051
Geogg
Geogg
Geogg
Geogg
Kaptoc
Full width at half maximum
Geogg
Geogg
Geogg
Geogg
What is rfc 4949
Rbac 140
Www.141ir
Cse 141
Cse 141
Code checker
Bremelanotide spray
Art 141 cod fiscal
Upn 141
Asc 805 business combinations
How many months is 141 days
Ee 141
D-141
Chemistry 141
Statement of financial accounting standards no 141
Art 141 lgt
Ieee 141
Ee 141
134/141
Cse 141
Ley 141 15
Aflcmc/wny
Ap-141
Practice assessor and practice supervisor
Sustainability principles and practice
Psychology: principles in practice answers
Psychology: principles in practice solutions
Regression psychology
Principles of effective practice
Evidence based practice principles